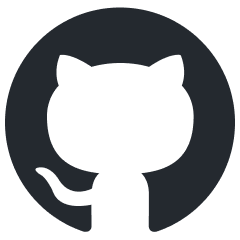
LLMcalc
A tool to determine whether or not your PC can run a given LLM
Stars: 122
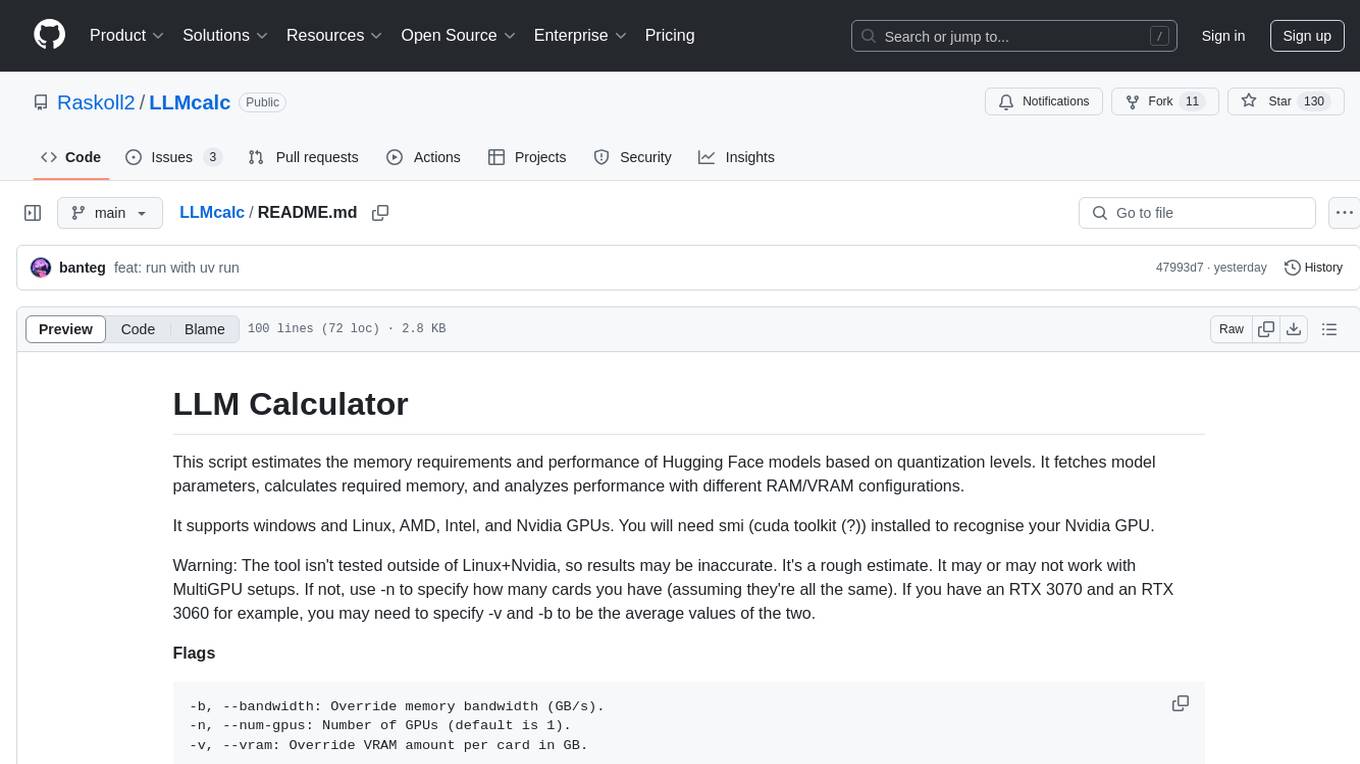
LLM Calculator is a script that estimates the memory requirements and performance of Hugging Face models based on quantization levels. It fetches model parameters, calculates required memory, and analyzes performance with different RAM/VRAM configurations. The tool supports Windows and Linux, AMD, Intel, and Nvidia GPUs. Users can input a Hugging Face model ID to get its parameter count and analyze memory requirements for various quantization schemes. The tool provides estimates for GPU offload percentage and throughput in tk/s. It requires dependencies like python, uv, pciutils for AMD + Linux, and drivers for Nvidia. The tool is designed for rough estimates and may not work with MultiGPU setups.
README:
This script estimates the memory requirements and performance of Hugging Face models based on quantization levels. It fetches model parameters, calculates required memory, and analyzes performance with different RAM/VRAM configurations.
It supports windows and Linux, AMD, Intel, and Nvidia GPUs. You will need smi (cuda toolkit (?)) installed to recognise your Nvidia GPU.
Warning: The tool isn't tested outside of Linux+Nvidia, so results may be inaccurate. It's a rough estimate. It may or may not work with MultiGPU setups. If not, use -n to specify how many cards you have (assuming they're all the same). If you have an RTX 3070 and an RTX 3060 for example, you may need to specify -v and -b to be the average values of the two.
Flags
-b, --bandwidth: Override memory bandwidth (GB/s).
-n, --num-gpus: Number of GPUs (default is 1).
-v, --vram: Override VRAM amount per card in GB.
Example:
python main.py -b 950 -n 2 -v 24
You'll need these to run it in python. 3.12.3 is what I wrote it in, but any modern version should work.
Install uv and run with:
uv run main.py
For AMD + Linux you'll need sudo apt install pciutils
Nvidia will need drivers, as long as nvidia-smi
works this program should.
Intel needs lspci
, dunno if that supports windows.
Enter a Hugging Face model ID (e.g., microsoft/phi-4) to get its parameter count. The script fetches system RAM and VRAM specs. You can override them with flags. It analyzes memory requirements for several quantization schemes and estimates throughput (tk/s).
Hover over a cell to see how many layers you need to offload, and what context you can fit without KV cache quantizing.
Cli Output
Enter Hugging Face model ID (e.g., microsoft/phi-4): microsoft/phi-4
Model Parameters: 14.7B params (14.70B params)
Total RAM: 33.53 GB
VRAM: 8.00 GB, ~448.0GB/s
Estimated RAM Bandwidth: 64.00 GB/s
Analysis for each quantization level:
FP8:
Run Type: Partial offload
Memory Required: 16.43 GB
GPU Offload Percentage: 48.7%
Estimated tk/s: 5.38
Q6_K_S:
Run Type: Partial offload
Memory Required: 13.86 GB
GPU Offload Percentage: 57.7%
Estimated tk/s: 7.39
Q5_K_S:
Run Type: Partial offload
Memory Required: 11.84 GB
GPU Offload Percentage: 67.6%
Estimated tk/s: 10.63
Q4_K_M:
Run Type: Partial offload
Memory Required: 10.55 GB
GPU Offload Percentage: 75.8%
Estimated tk/s: 14.71
IQ4_XS:
Run Type: Partial offload
Memory Required: 9.64 GB
GPU Offload Percentage: 83.0%
Estimated tk/s: 19.92
Q3_K_M:
Run Type: KV cache offload
Memory Required: 8.90 GB
Estimated tk/s: 45.30
IQ3_XS:
Run Type: All in VRAM
Memory Required: 7.80 GB
Estimated tk/s: 57.45
IQ2_XS:
Run Type: All in VRAM
Memory Required: 6.14 GB
Estimated tk/s: 72.90
For Tasks:
Click tags to check more tools for each tasksFor Jobs:
Alternative AI tools for LLMcalc
Similar Open Source Tools
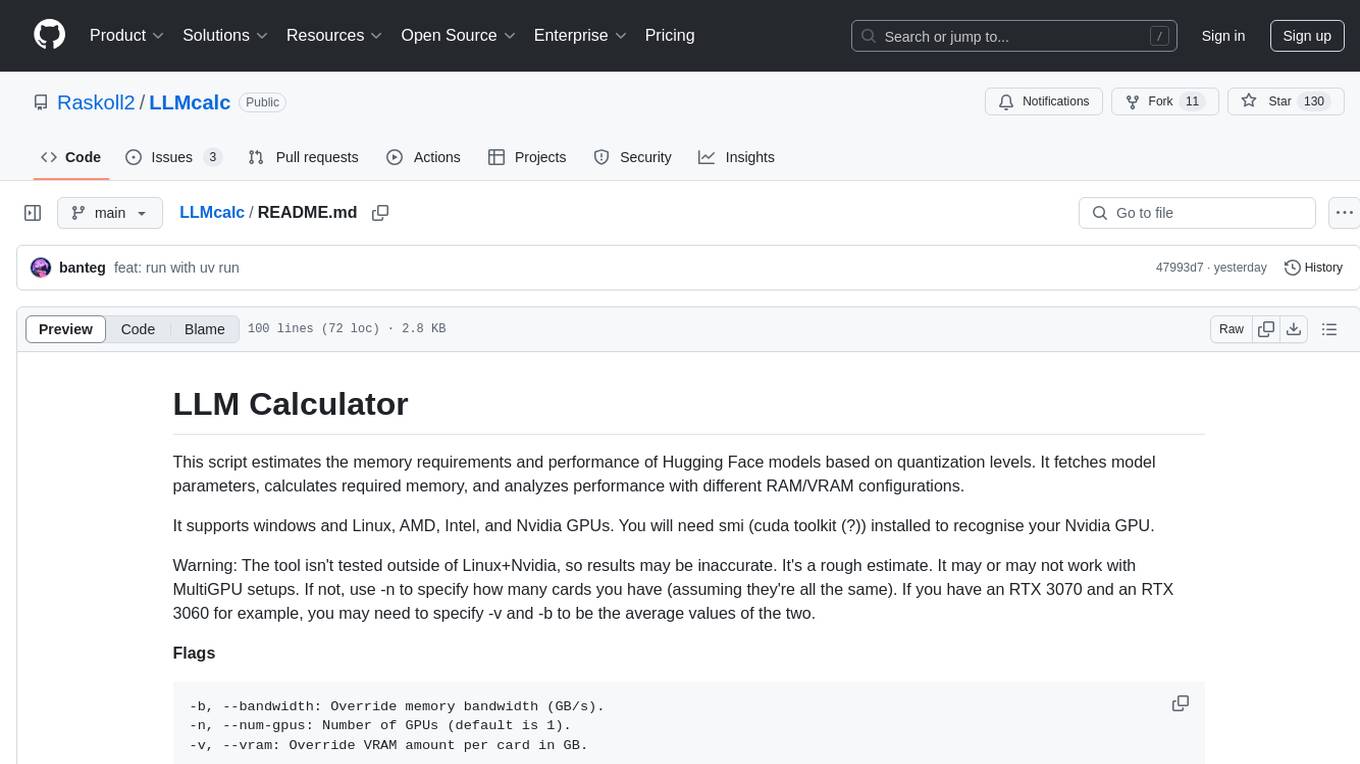
LLMcalc
LLM Calculator is a script that estimates the memory requirements and performance of Hugging Face models based on quantization levels. It fetches model parameters, calculates required memory, and analyzes performance with different RAM/VRAM configurations. The tool supports Windows and Linux, AMD, Intel, and Nvidia GPUs. Users can input a Hugging Face model ID to get its parameter count and analyze memory requirements for various quantization schemes. The tool provides estimates for GPU offload percentage and throughput in tk/s. It requires dependencies like python, uv, pciutils for AMD + Linux, and drivers for Nvidia. The tool is designed for rough estimates and may not work with MultiGPU setups.
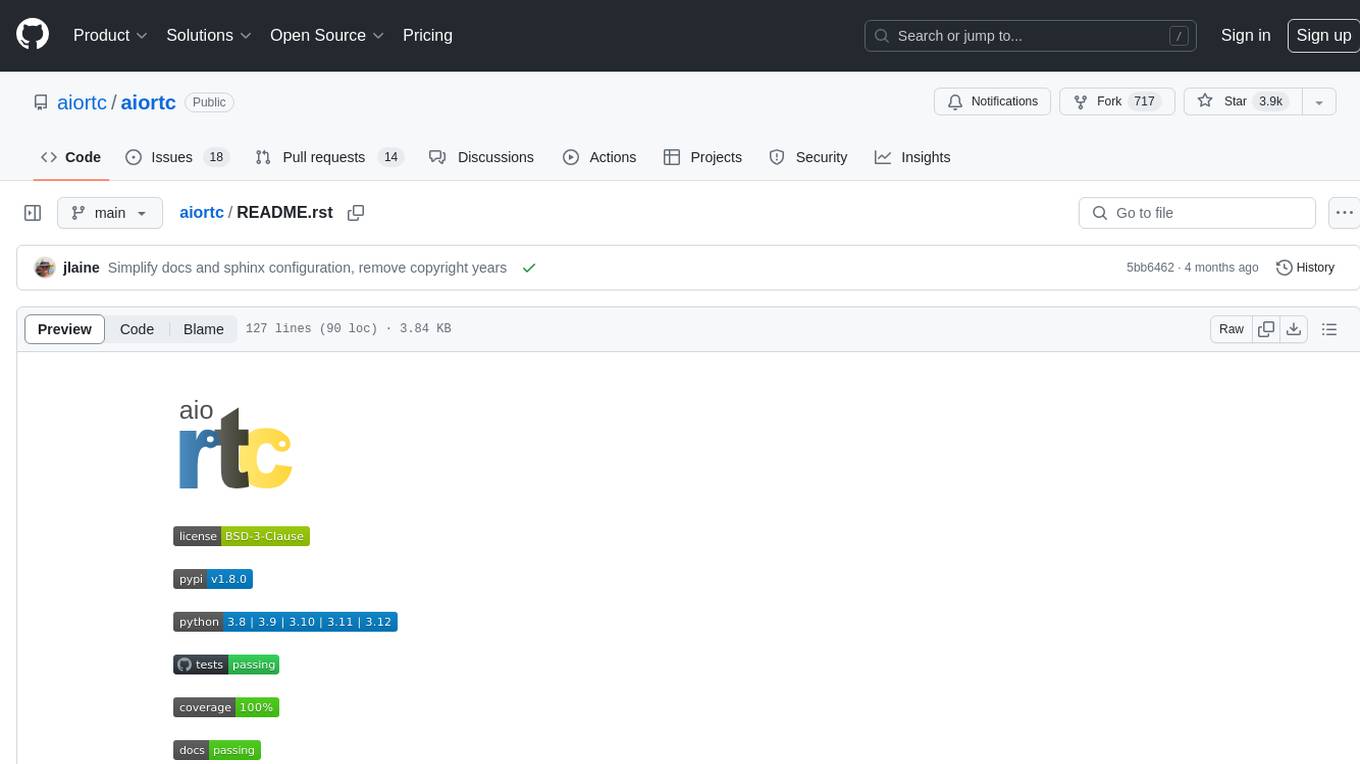
aiortc
aiortc is a Python library for Web Real-Time Communication (WebRTC) and Object Real-Time Communication (ORTC). It provides a simple and readable implementation for programmers to understand and tinker with WebRTC internals. The library allows for exchanging audio, video, and data channels, supports SDP generation/parsing, ICE, DTLS, SRTP, SCTP, and various audio/video codecs. It also enables creating innovative products by leveraging Python ecosystem modules, such as computer vision algorithms with OpenCV. Extensive testing ensures high code quality.
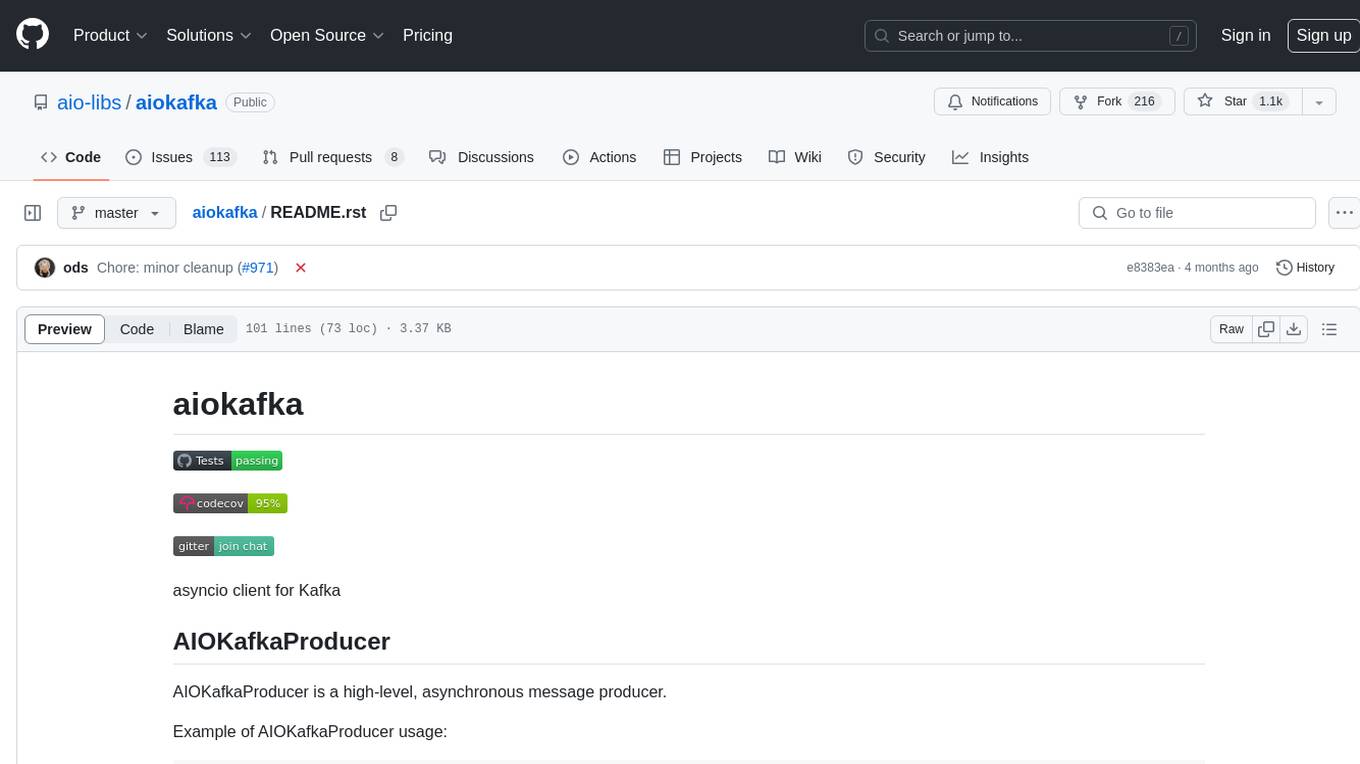
aiokafka
aiokafka is an asyncio client for Kafka that provides high-level, asynchronous message producer and consumer functionalities. It allows users to interact with Kafka for sending and consuming messages in an efficient and scalable manner. The tool supports features like cluster layout retrieval, topic/partition leadership information, group coordination, and message consumption load balancing. Users can easily integrate aiokafka into their Python projects to work with Kafka seamlessly.
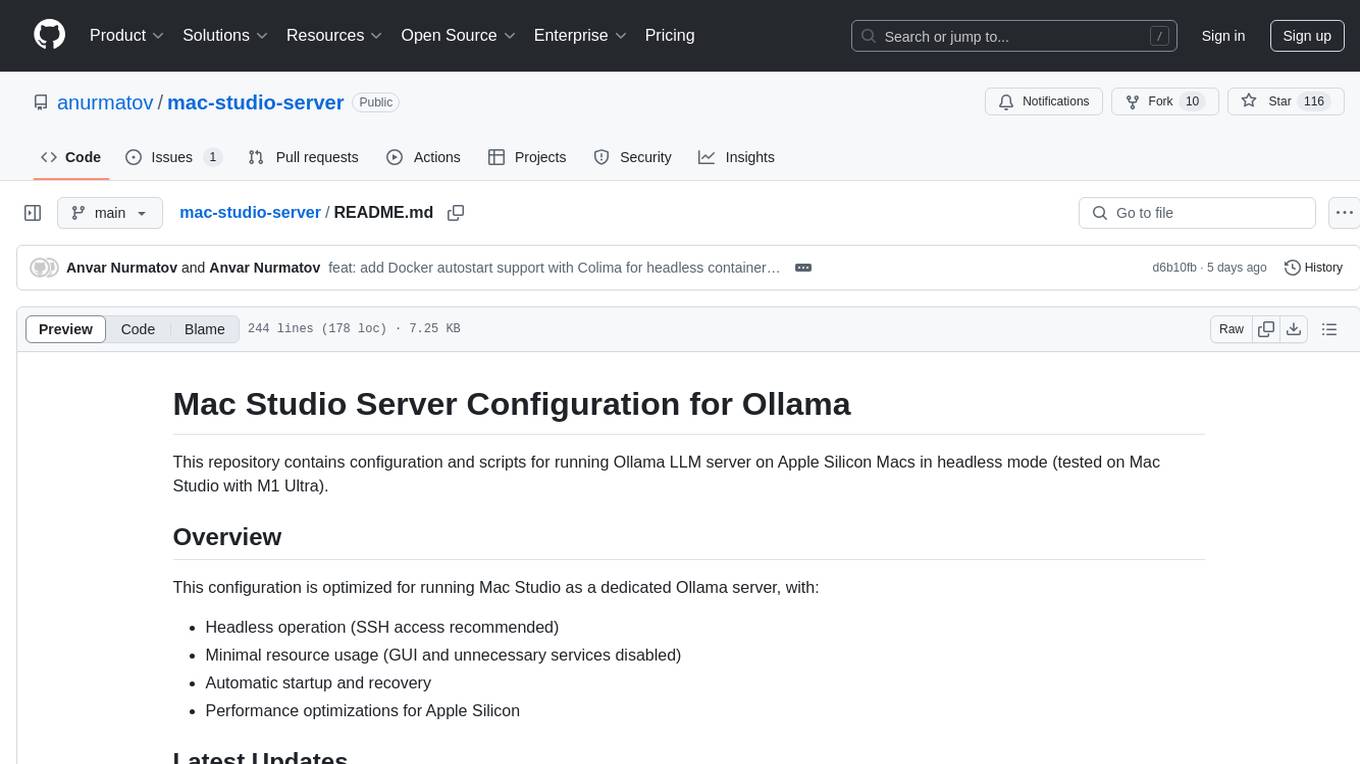
mac-studio-server
This repository provides configuration and scripts for running Ollama LLM server on Apple Silicon Macs in headless mode, optimized for performance and resource usage. It includes features like automatic startup, system resource optimization, external network access, proper logging setup, and SSH-based remote management. Users can customize the Ollama service configuration and enable optional GPU memory optimization and Docker autostart for container applications. The installation process disables unnecessary system services, configures power management, and optimizes for background operation while maintaining Screen Sharing capability for remote management. Performance considerations focus on reducing memory usage, disabling GUI-related services, minimizing background processes, preventing sleep/hibernation, and optimizing for headless operation.
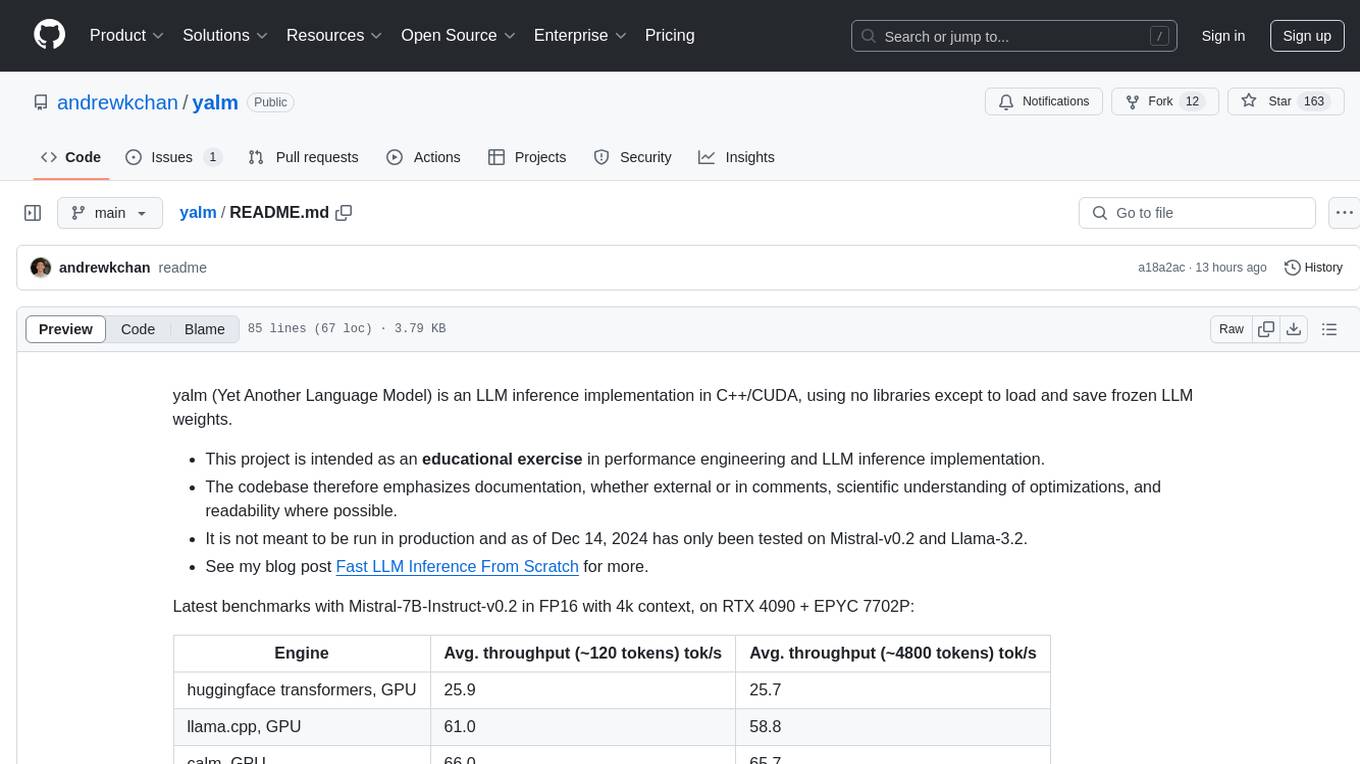
yalm
Yalm (Yet Another Language Model) is an LLM inference implementation in C++/CUDA, emphasizing performance engineering, documentation, scientific optimizations, and readability. It is not for production use and has been tested on Mistral-v0.2 and Llama-3.2. Requires C++20-compatible compiler, CUDA toolkit, and LLM safetensor weights in huggingface format converted to .yalm file.
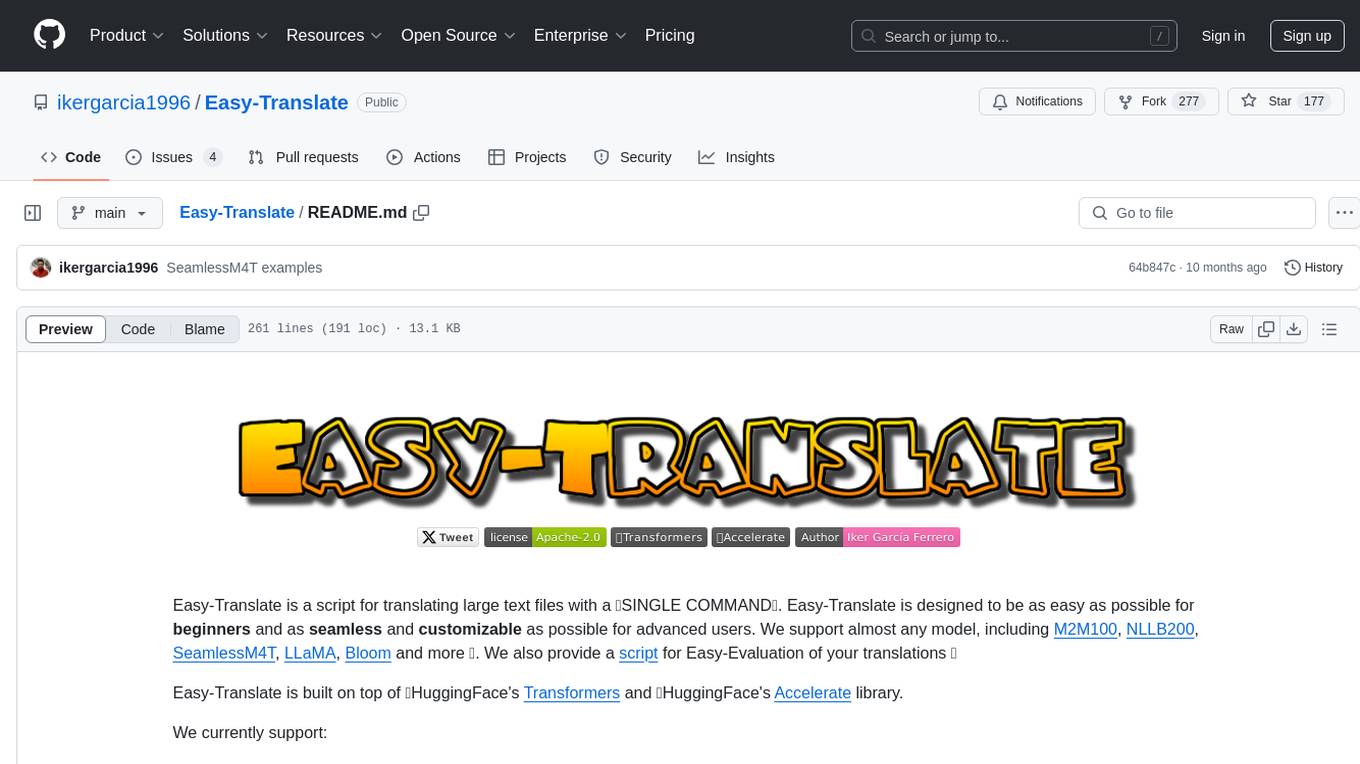
Easy-Translate
Easy-Translate is a script designed for translating large text files with a single command. It supports various models like M2M100, NLLB200, SeamlessM4T, LLaMA, and Bloom. The tool is beginner-friendly and offers seamless and customizable features for advanced users. It allows acceleration on CPU, multi-CPU, GPU, multi-GPU, and TPU, with support for different precisions and decoding strategies. Easy-Translate also provides an evaluation script for translations. Built on HuggingFace's Transformers and Accelerate library, it supports prompt usage and loading huge models efficiently.
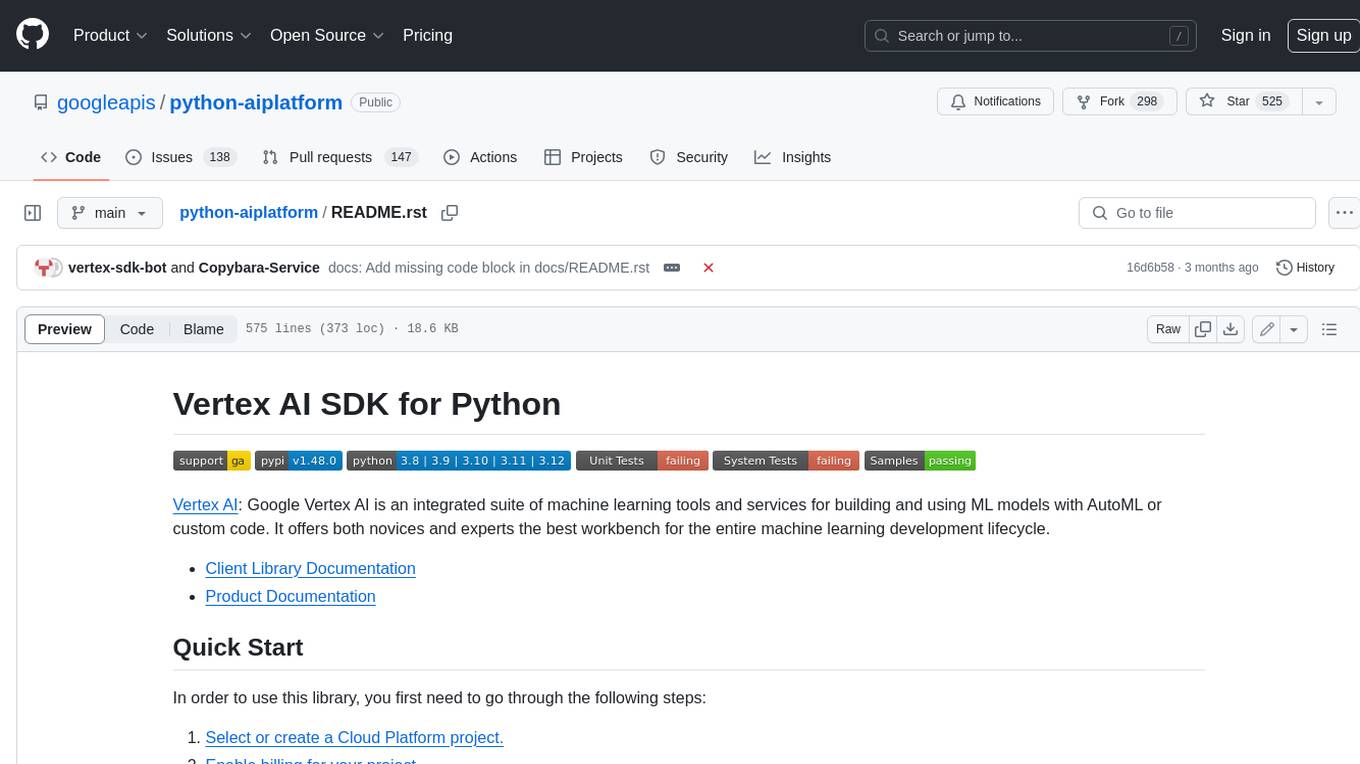
python-aiplatform
The Vertex AI SDK for Python is a library that provides a convenient way to use the Vertex AI API. It offers a high-level interface for creating and managing Vertex AI resources, such as datasets, models, and endpoints. The SDK also provides support for training and deploying custom models, as well as using AutoML models. With the Vertex AI SDK for Python, you can quickly and easily build and deploy machine learning models on Vertex AI.
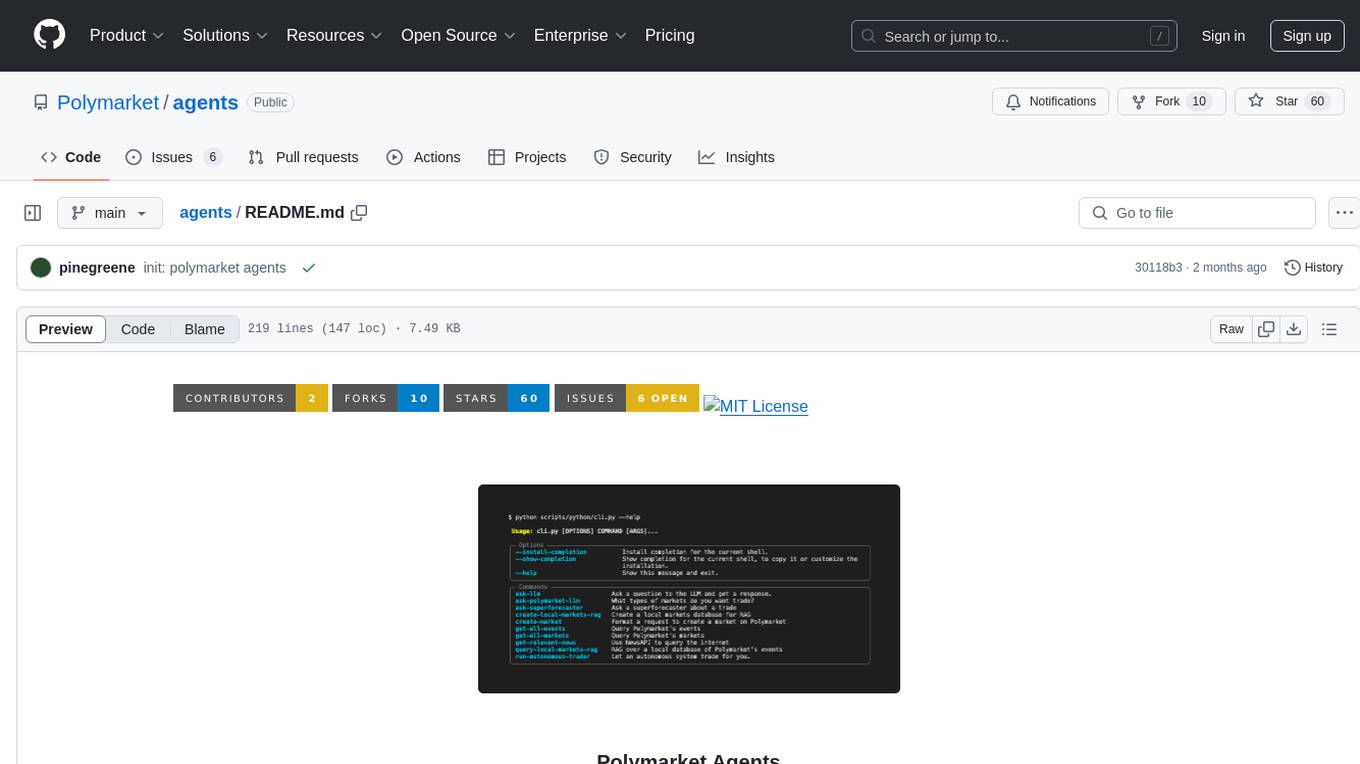
agents
Polymarket Agents is a developer framework and set of utilities for building AI agents to trade autonomously on Polymarket. It integrates with Polymarket API, provides AI agent utilities for prediction markets, supports local and remote RAG, sources data from various services, and offers comprehensive LLM tools for prompt engineering. The architecture features modular components like APIs and scripts for managing local environments, server set-up, and CLI for end-user commands.
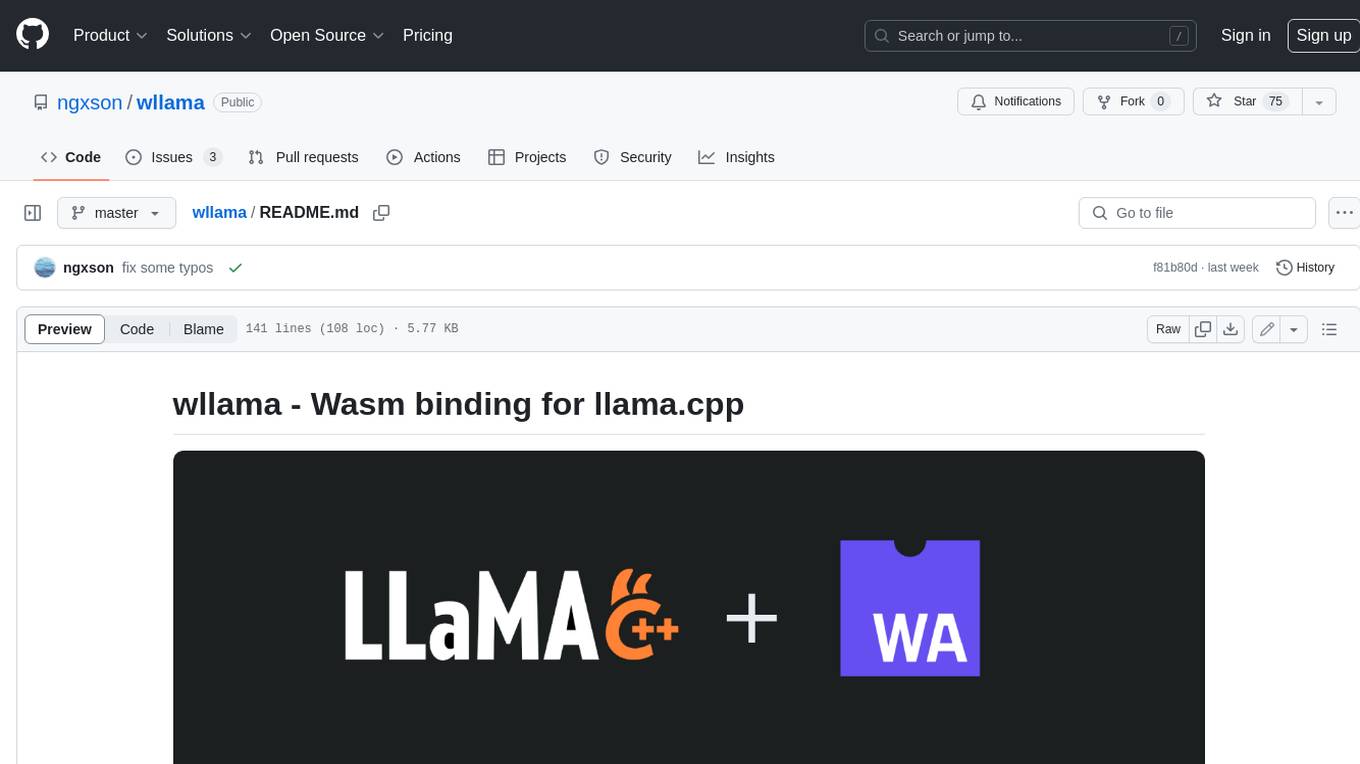
wllama
Wllama is a WebAssembly binding for llama.cpp, a high-performance and lightweight language model library. It enables you to run inference directly on the browser without the need for a backend or GPU. Wllama provides both high-level and low-level APIs, allowing you to perform various tasks such as completions, embeddings, tokenization, and more. It also supports model splitting, enabling you to load large models in parallel for faster download. With its Typescript support and pre-built npm package, Wllama is easy to integrate into your React Typescript projects.
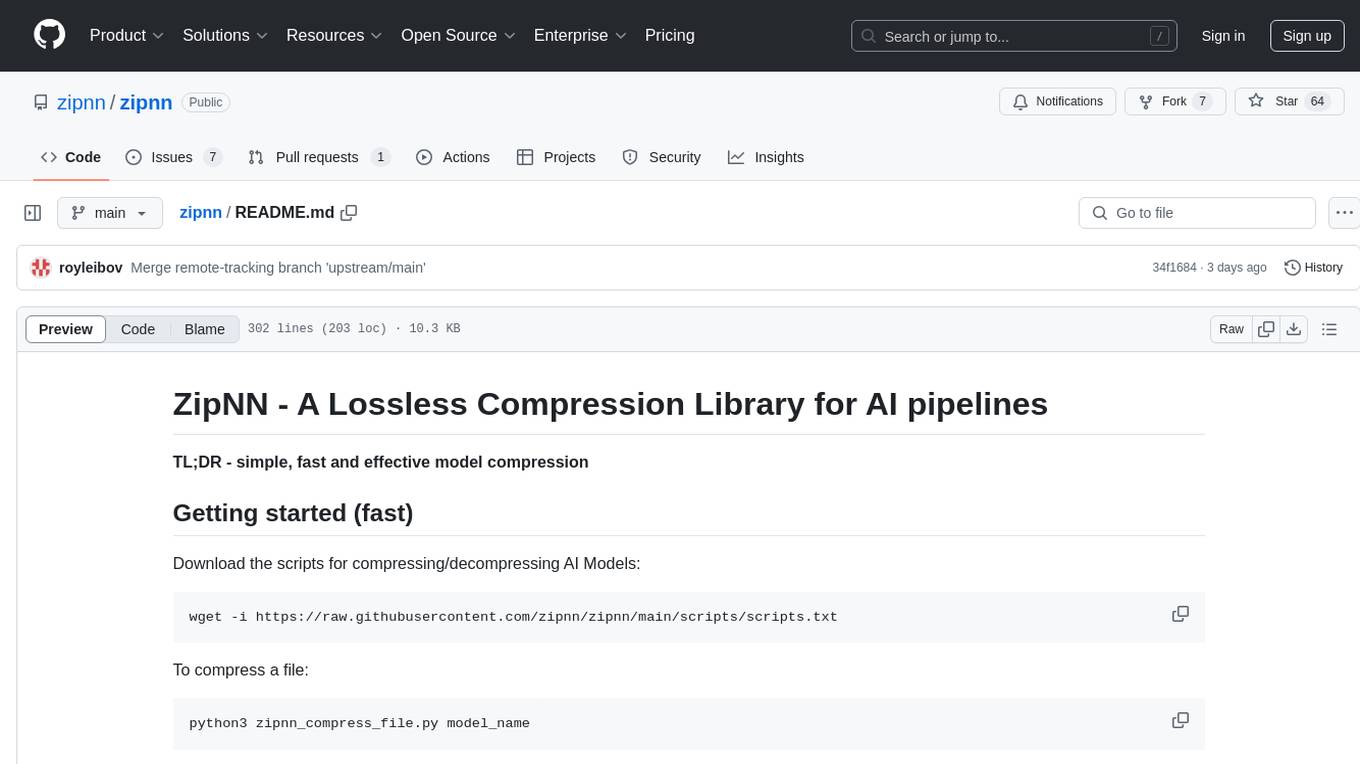
zipnn
ZipNN is a lossless and near-lossless compression library optimized for numbers/tensors in the Foundation Models environment. It automatically prepares data for compression based on its type, allowing users to focus on core tasks without worrying about compression complexities. The library delivers effective compression techniques for different data types and structures, achieving high compression ratios and rates. ZipNN supports various compression methods like ZSTD, lz4, and snappy, and provides ready-made scripts for file compression/decompression. Users can also manually import the package to compress and decompress data. The library offers advanced configuration options for customization and validation tests for different input and compression types.
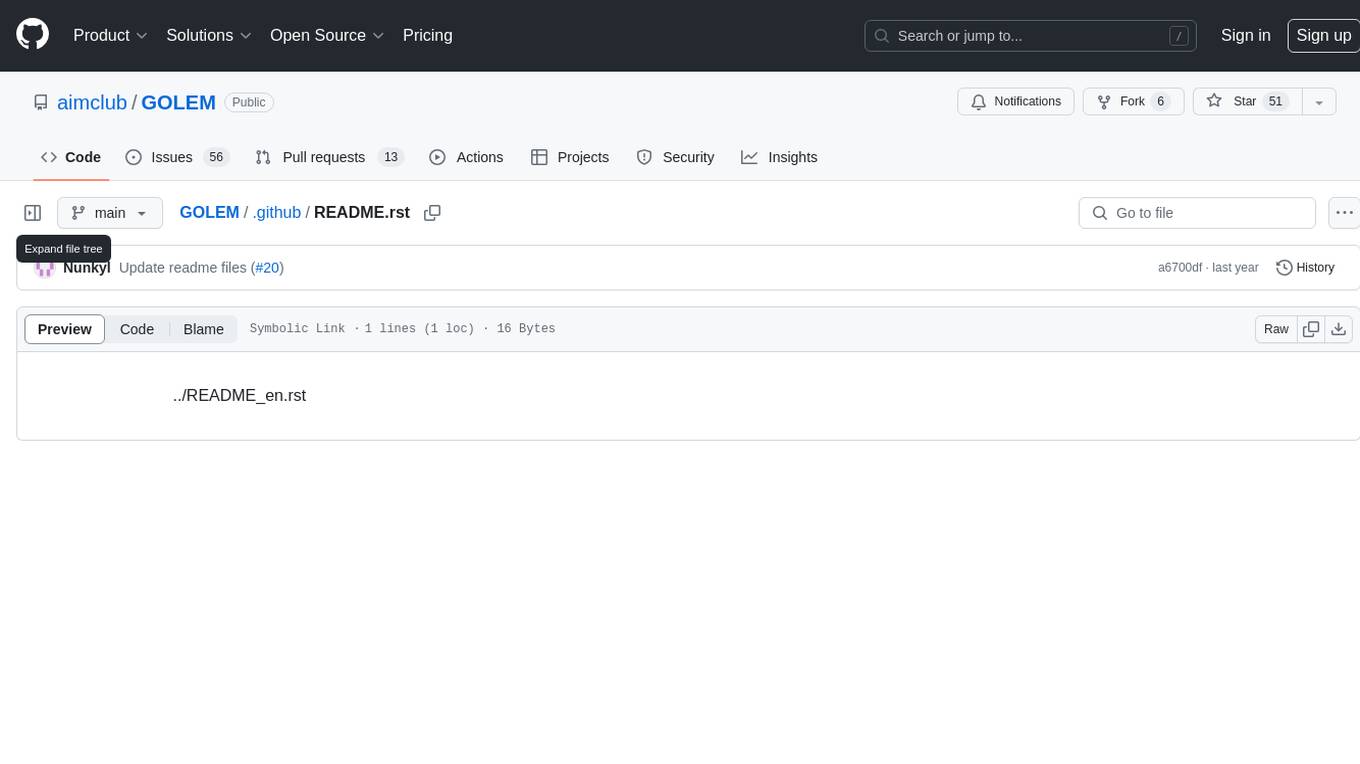
GOLEM
GOLEM is an open-source AI framework focused on optimization and learning of structured graph-based models using meta-heuristic methods. It emphasizes the potential of meta-heuristics in complex problem spaces where gradient-based methods are not suitable, and the importance of structured models in various problem domains. The framework offers features like structured model optimization, metaheuristic methods, multi-objective optimization, constrained optimization, extensibility, interpretability, and reproducibility. It can be applied to optimization problems represented as directed graphs with defined fitness functions. GOLEM has applications in areas like AutoML, Bayesian network structure search, differential equation discovery, geometric design, and neural architecture search. The project structure includes packages for core functionalities, adapters, graph representation, optimizers, genetic algorithms, utilities, serialization, visualization, examples, and testing. Contributions are welcome, and the project is supported by ITMO University's Research Center Strong Artificial Intelligence in Industry.
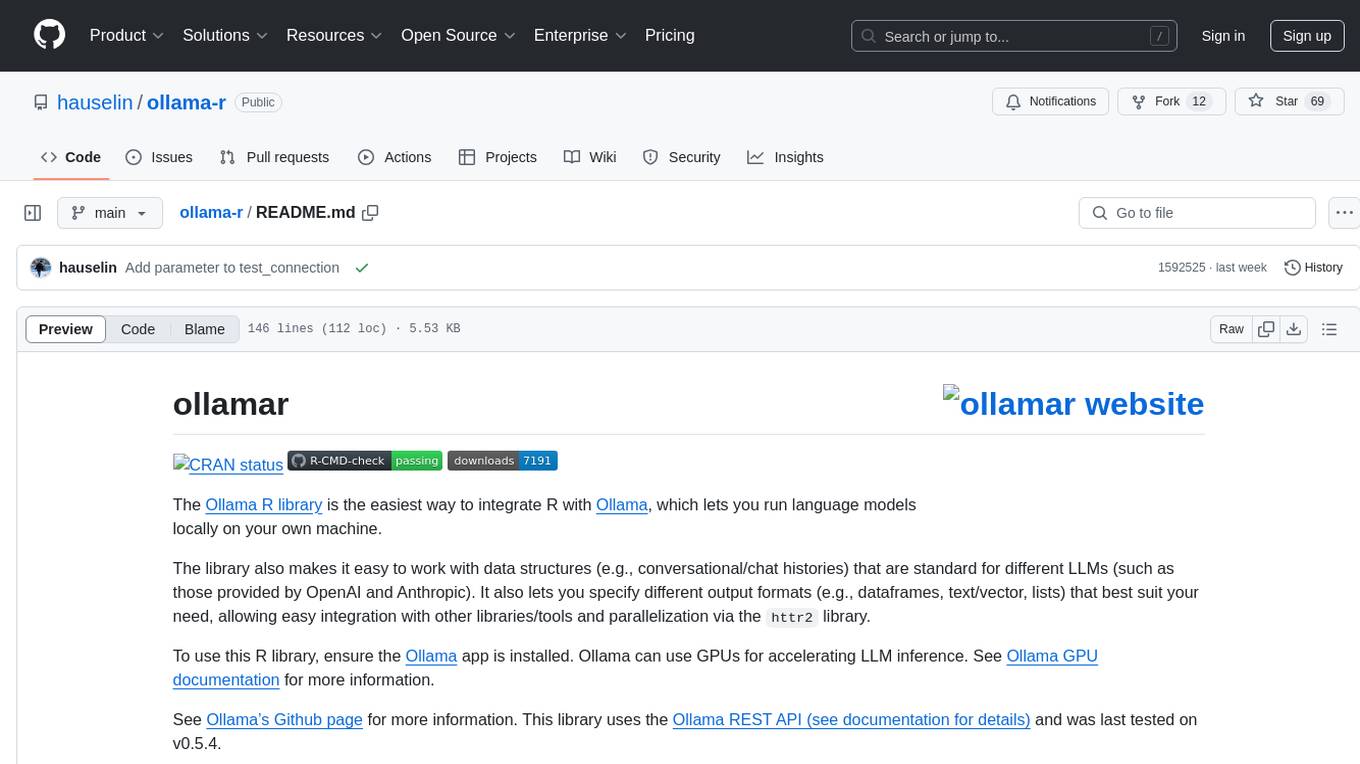
ollama-r
The Ollama R library provides an easy way to integrate R with Ollama for running language models locally on your machine. It supports working with standard data structures for different LLMs, offers various output formats, and enables integration with other libraries/tools. The library uses the Ollama REST API and requires the Ollama app to be installed, with GPU support for accelerating LLM inference. It is inspired by Ollama Python and JavaScript libraries, making it familiar for users of those languages. The installation process involves downloading the Ollama app, installing the 'ollamar' package, and starting the local server. Example usage includes testing connection, downloading models, generating responses, and listing available models.
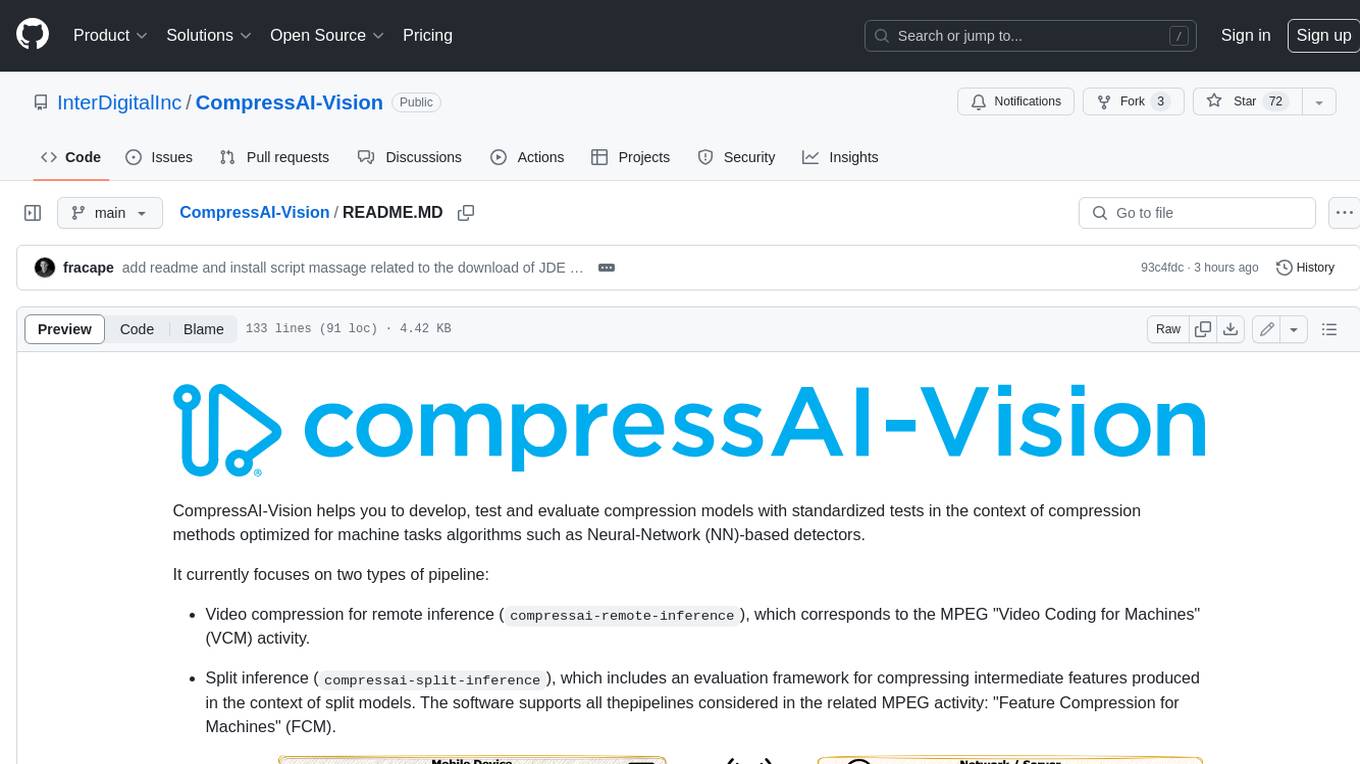
CompressAI-Vision
CompressAI-Vision is a tool that helps you develop, test, and evaluate compression models with standardized tests in the context of compression methods optimized for machine tasks algorithms such as Neural-Network (NN)-based detectors. It currently focuses on two types of pipeline: Video compression for remote inference (`compressai-remote-inference`), which corresponds to the MPEG "Video Coding for Machines" (VCM) activity. Split inference (`compressai-split-inference`), which includes an evaluation framework for compressing intermediate features produced in the context of split models. The software supports all the pipelines considered in the related MPEG activity: "Feature Compression for Machines" (FCM).
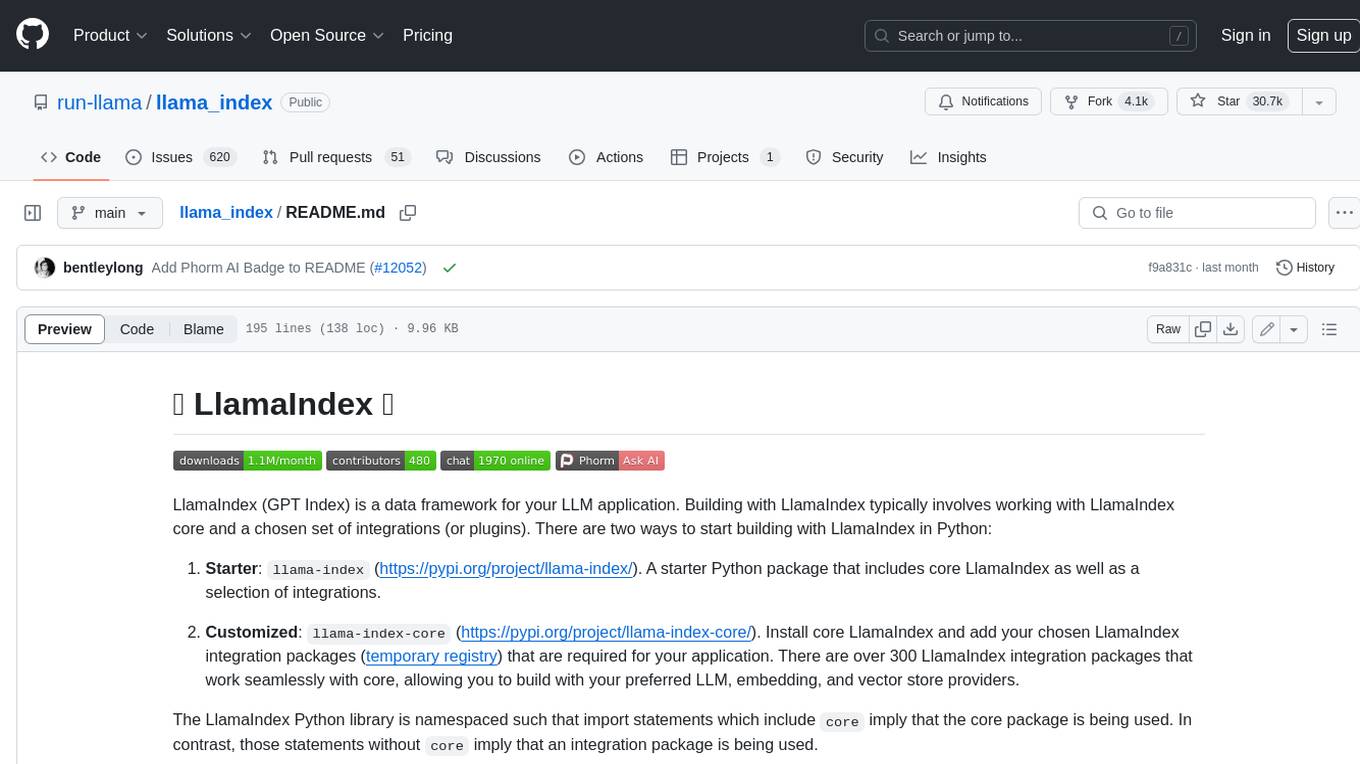
llama_index
LlamaIndex is a data framework for building LLM applications. It provides tools for ingesting, structuring, and querying data, as well as integrating with LLMs and other tools. LlamaIndex is designed to be easy to use for both beginner and advanced users, and it provides a comprehensive set of features for building LLM applications.
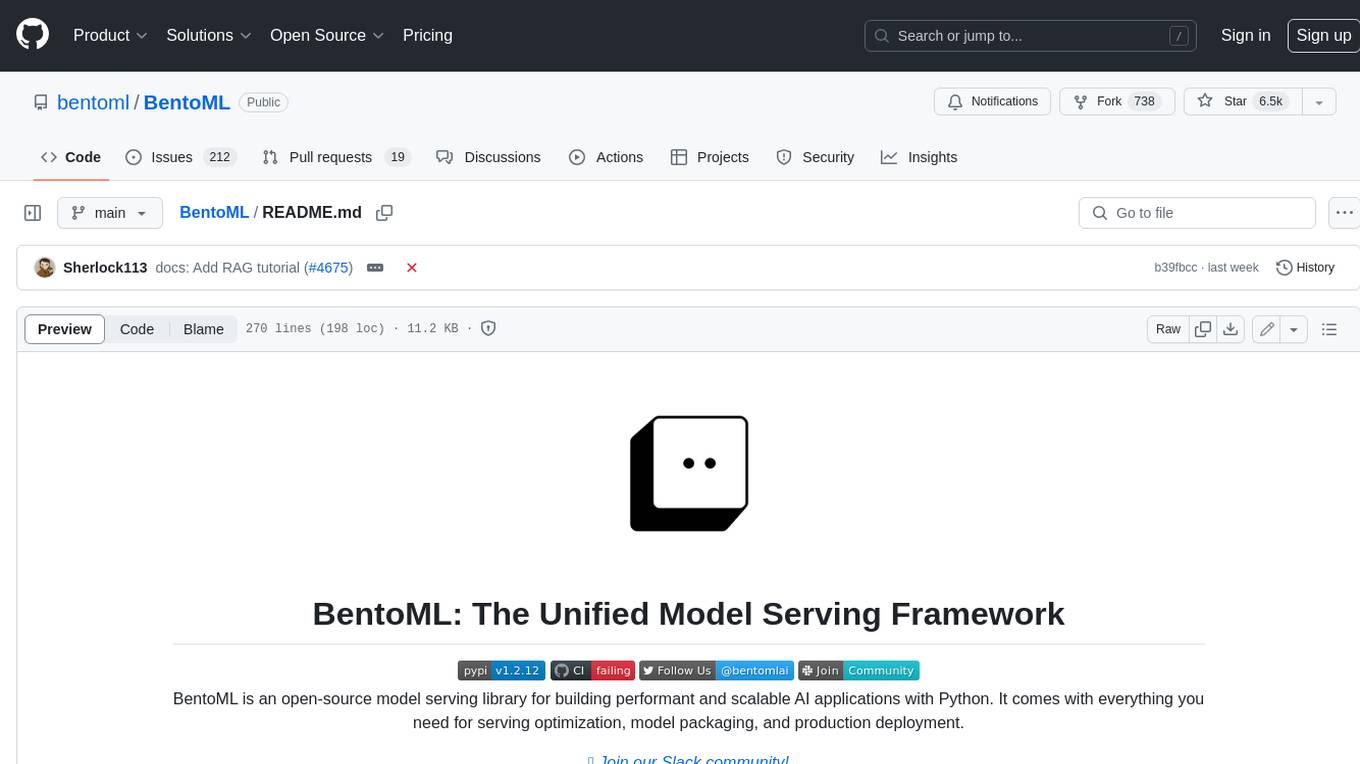
BentoML
BentoML is an open-source model serving library for building performant and scalable AI applications with Python. It comes with everything you need for serving optimization, model packaging, and production deployment.
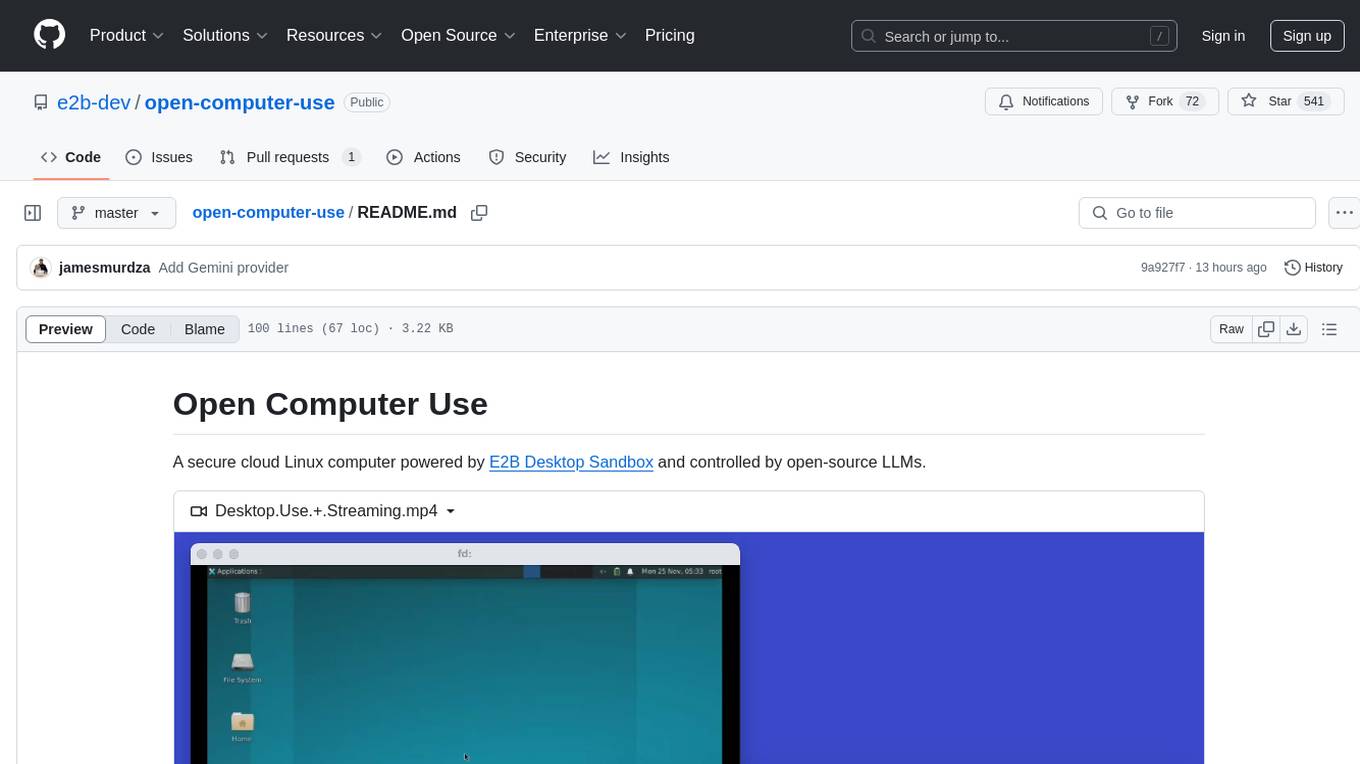
open-computer-use
Open Computer Use is a secure cloud Linux computer powered by E2B Desktop Sandbox and controlled by open-source LLMs. It allows users to operate the computer via keyboard, mouse, and shell commands, live stream the display of the sandbox on the client computer, and pause or prompt the agent at any time. The tool is designed to work with any operating system and supports integration with various LLMs and providers following the OpenAI API specification.
For similar tasks
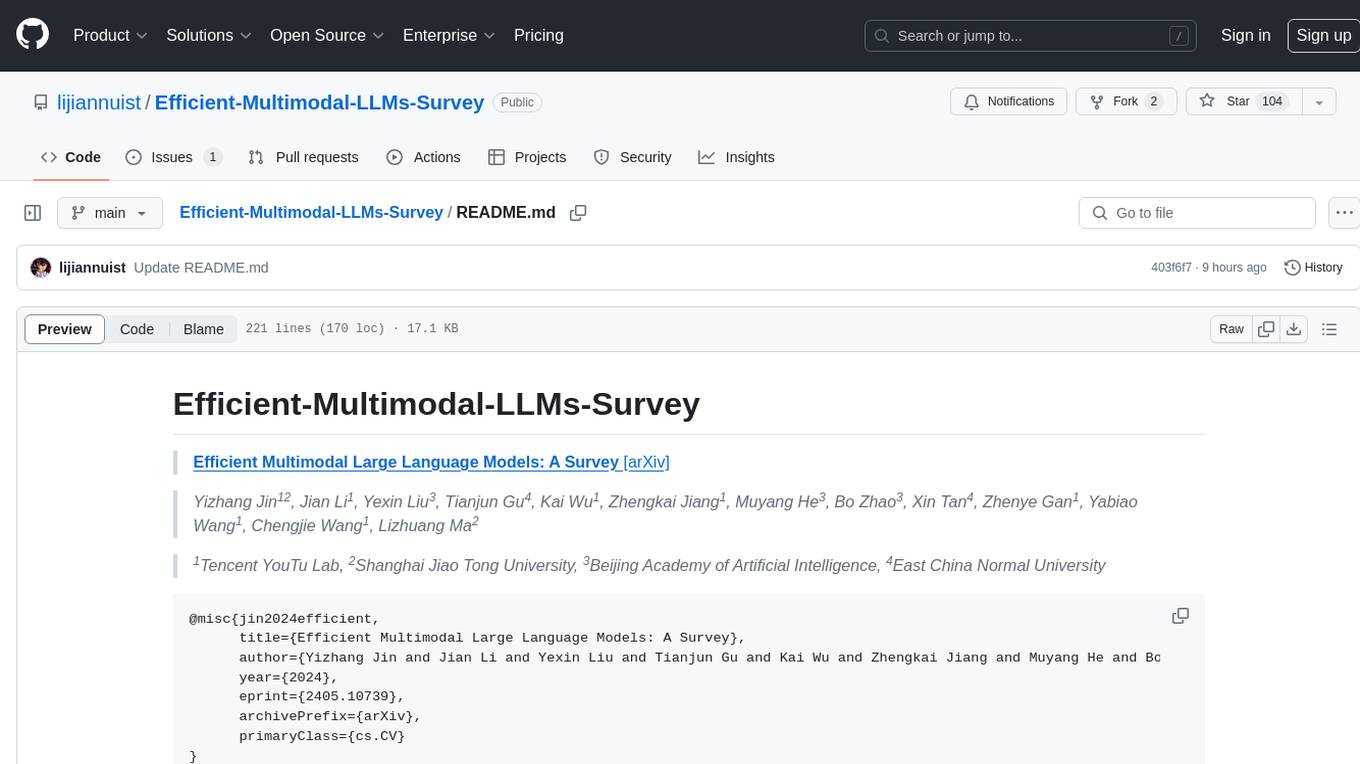
Efficient-Multimodal-LLMs-Survey
Efficient Multimodal Large Language Models: A Survey provides a comprehensive review of efficient and lightweight Multimodal Large Language Models (MLLMs), focusing on model size reduction and cost efficiency for edge computing scenarios. The survey covers the timeline of efficient MLLMs, research on efficient structures and strategies, and applications. It discusses current limitations and future directions in efficient MLLM research.
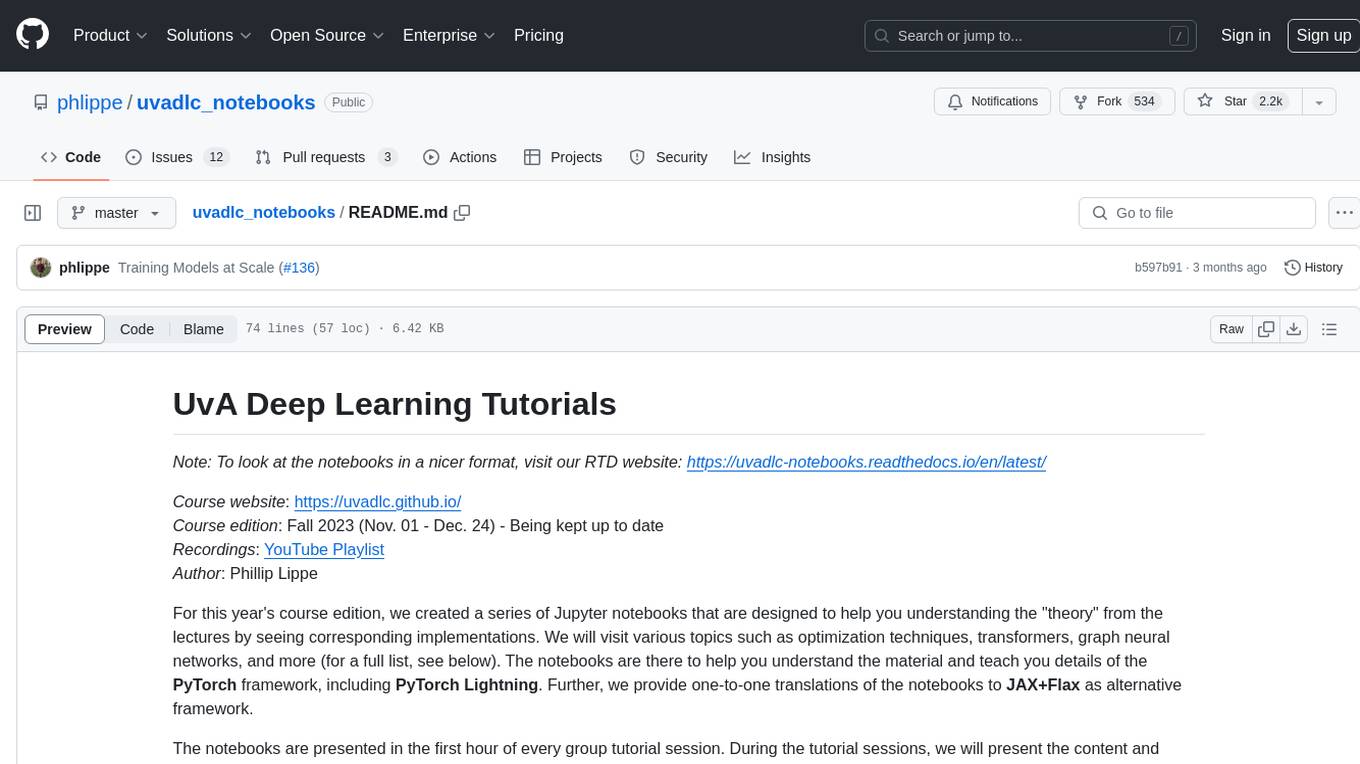
uvadlc_notebooks
The UvA Deep Learning Tutorials repository contains a series of Jupyter notebooks designed to help understand theoretical concepts from lectures by providing corresponding implementations. The notebooks cover topics such as optimization techniques, transformers, graph neural networks, and more. They aim to teach details of the PyTorch framework, including PyTorch Lightning, with alternative translations to JAX+Flax. The tutorials are integrated as official tutorials of PyTorch Lightning and are relevant for graded assignments and exams.
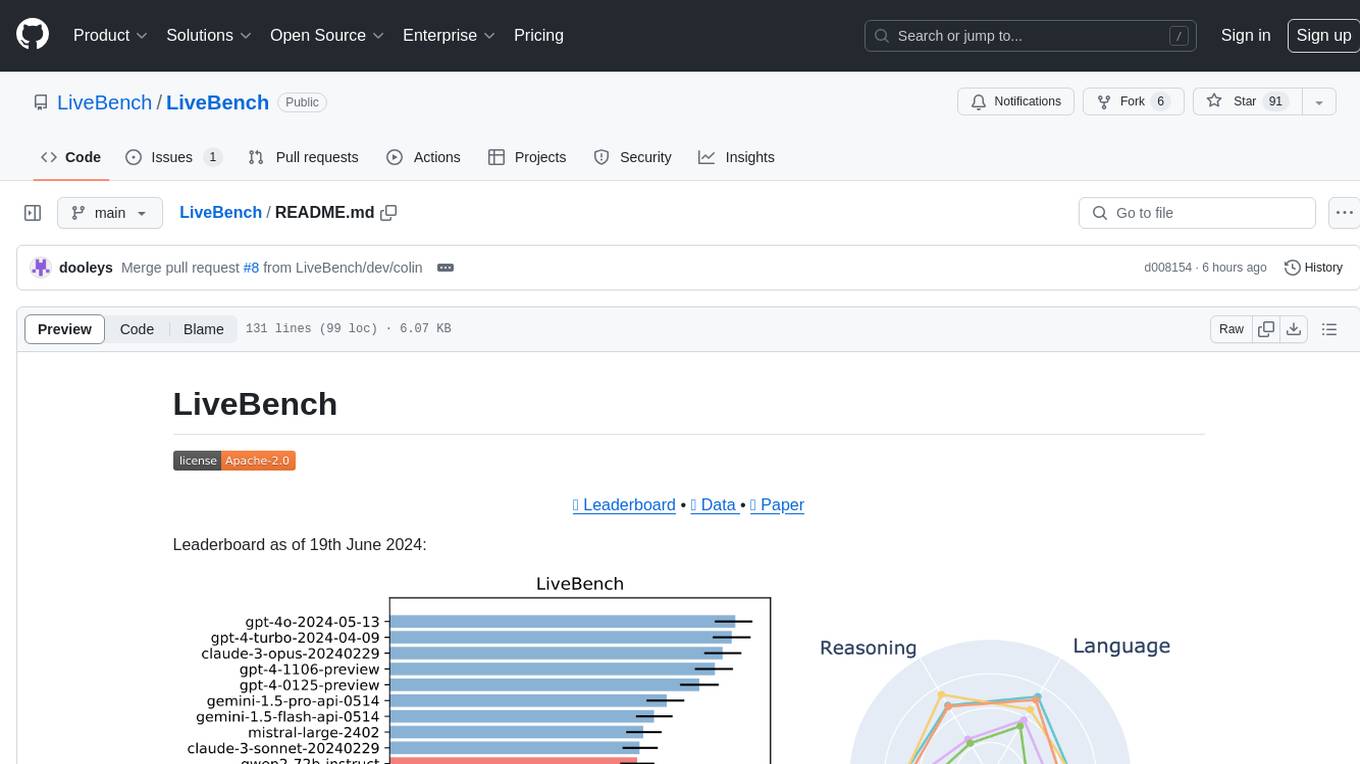
LiveBench
LiveBench is a benchmark tool designed for Language Model Models (LLMs) with a focus on limiting contamination through monthly new questions based on recent datasets, arXiv papers, news articles, and IMDb movie synopses. It provides verifiable, objective ground-truth answers for accurate scoring without an LLM judge. The tool offers 18 diverse tasks across 6 categories and promises to release more challenging tasks over time. LiveBench is built on FastChat's llm_judge module and incorporates code from LiveCodeBench and IFEval.
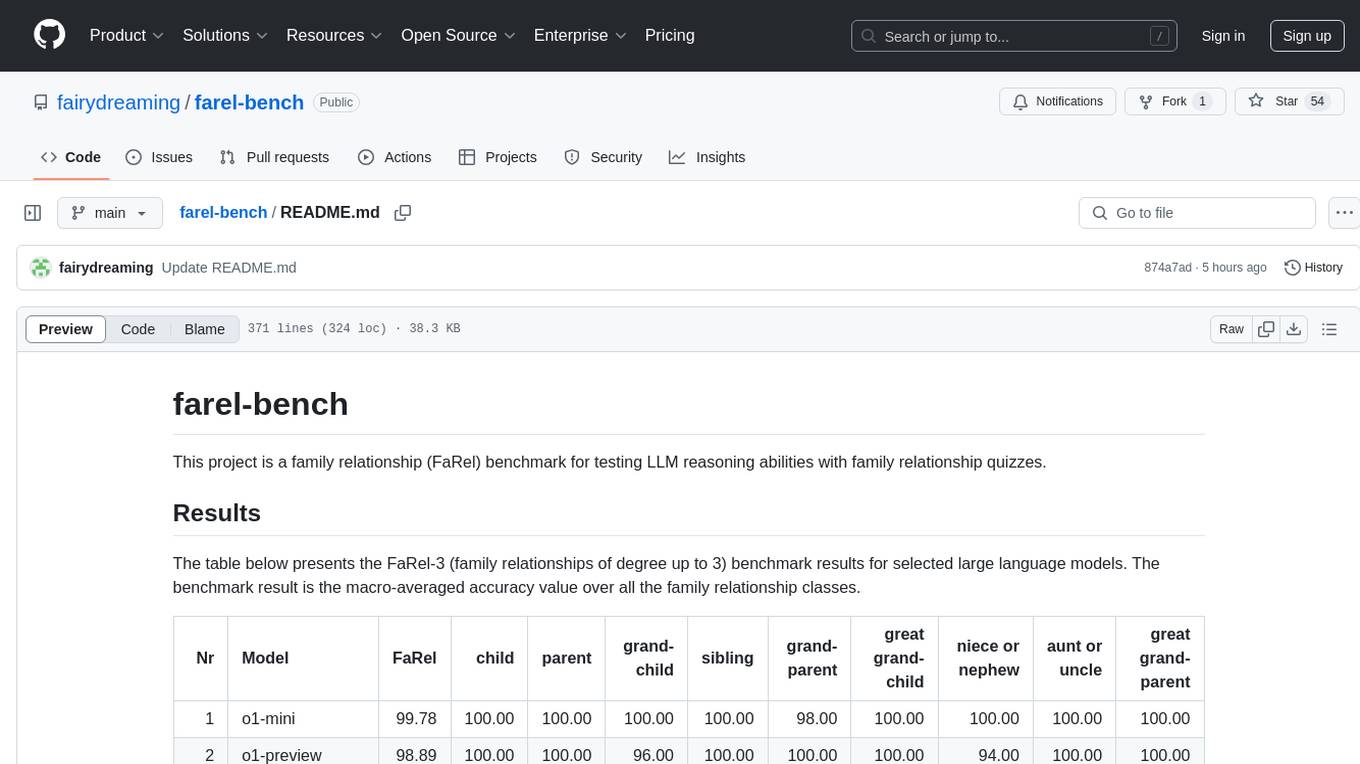
farel-bench
The 'farel-bench' project is a benchmark tool for testing LLM reasoning abilities with family relationship quizzes. It generates quizzes based on family relationships of varying degrees and measures the accuracy of large language models in solving these quizzes. The project provides scripts for generating quizzes, running models locally or via APIs, and calculating benchmark metrics. The quizzes are designed to test logical reasoning skills using family relationship concepts, with the goal of evaluating the performance of language models in this specific domain.
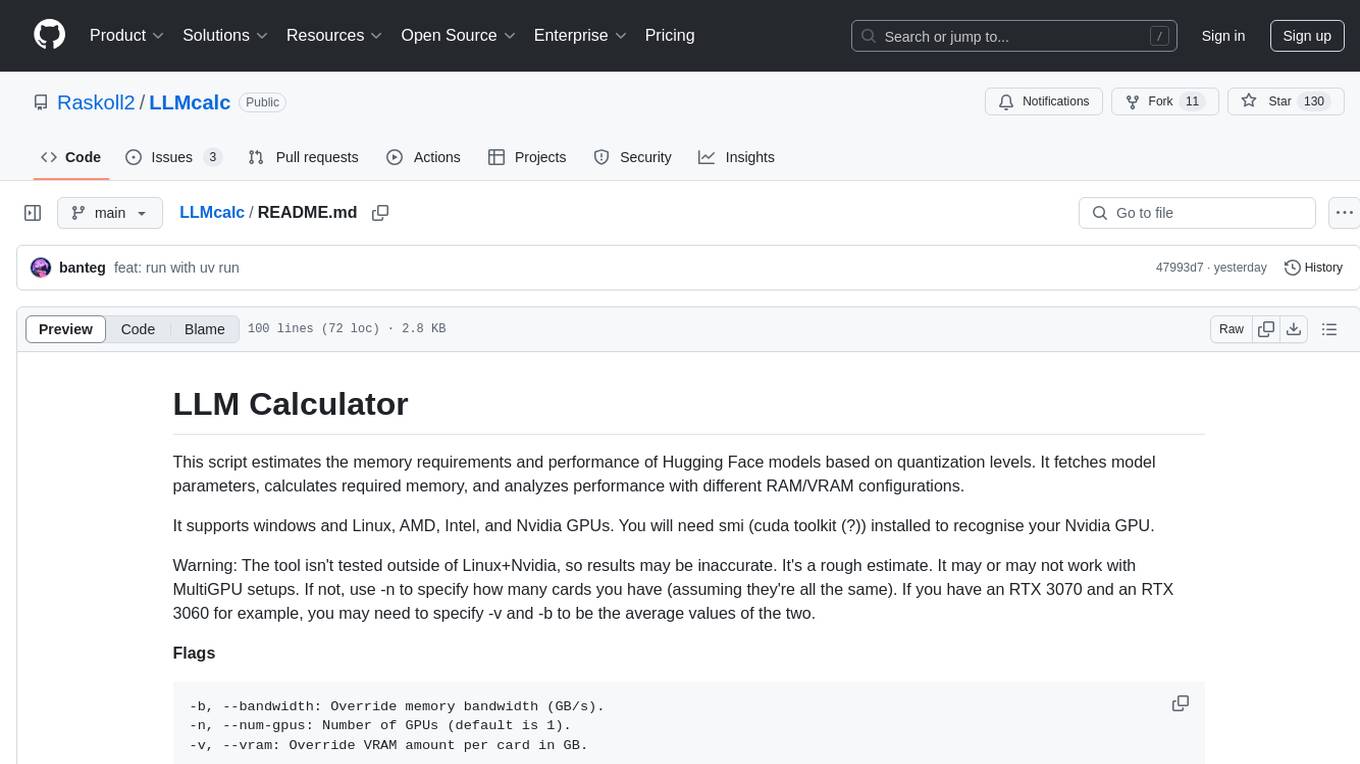
LLMcalc
LLM Calculator is a script that estimates the memory requirements and performance of Hugging Face models based on quantization levels. It fetches model parameters, calculates required memory, and analyzes performance with different RAM/VRAM configurations. The tool supports Windows and Linux, AMD, Intel, and Nvidia GPUs. Users can input a Hugging Face model ID to get its parameter count and analyze memory requirements for various quantization schemes. The tool provides estimates for GPU offload percentage and throughput in tk/s. It requires dependencies like python, uv, pciutils for AMD + Linux, and drivers for Nvidia. The tool is designed for rough estimates and may not work with MultiGPU setups.
For similar jobs
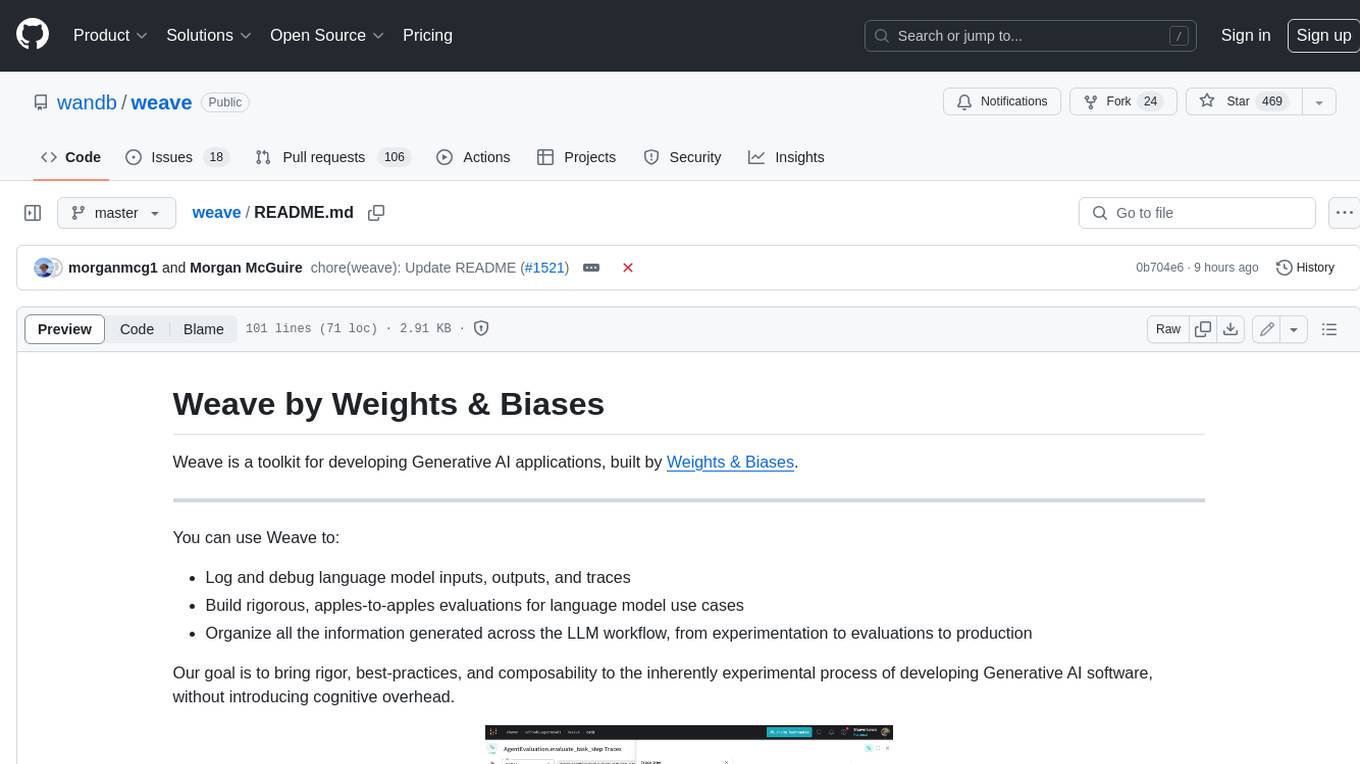
weave
Weave is a toolkit for developing Generative AI applications, built by Weights & Biases. With Weave, you can log and debug language model inputs, outputs, and traces; build rigorous, apples-to-apples evaluations for language model use cases; and organize all the information generated across the LLM workflow, from experimentation to evaluations to production. Weave aims to bring rigor, best-practices, and composability to the inherently experimental process of developing Generative AI software, without introducing cognitive overhead.
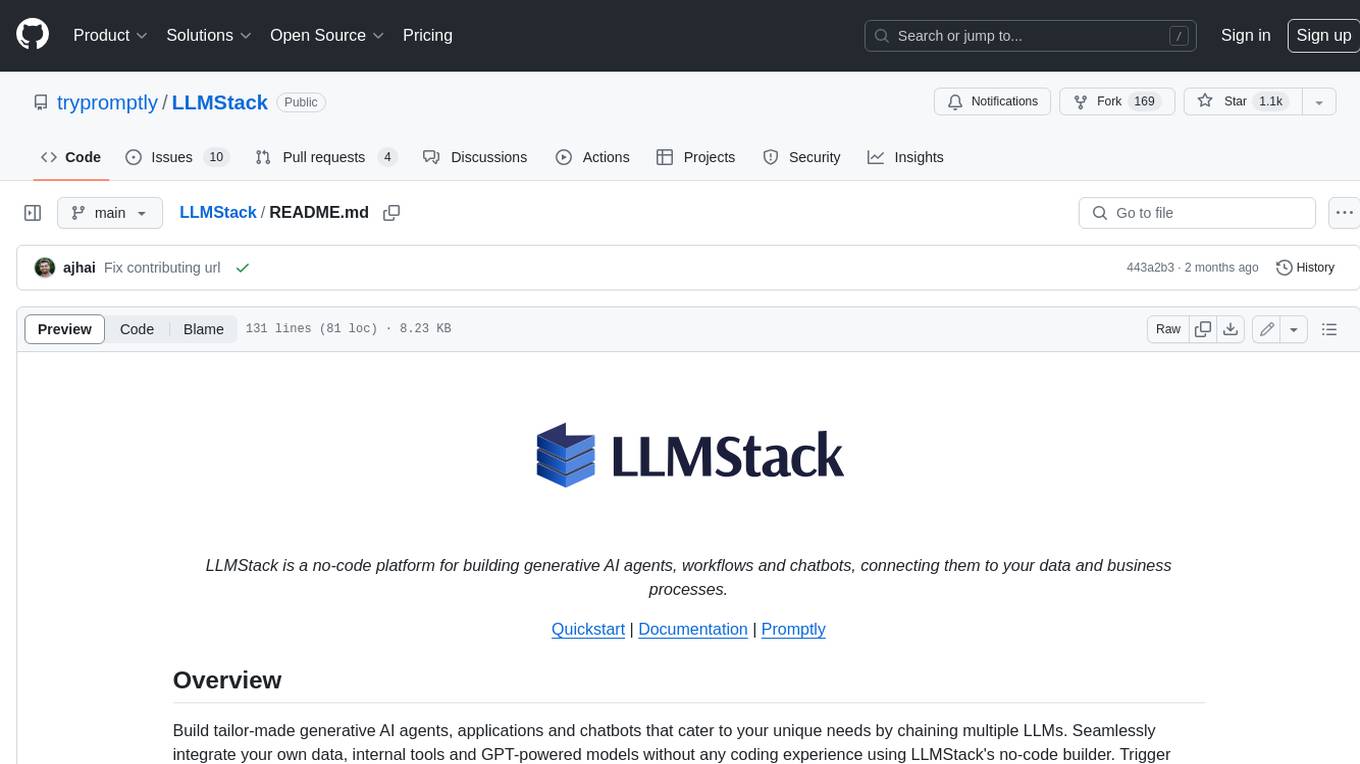
LLMStack
LLMStack is a no-code platform for building generative AI agents, workflows, and chatbots. It allows users to connect their own data, internal tools, and GPT-powered models without any coding experience. LLMStack can be deployed to the cloud or on-premise and can be accessed via HTTP API or triggered from Slack or Discord.
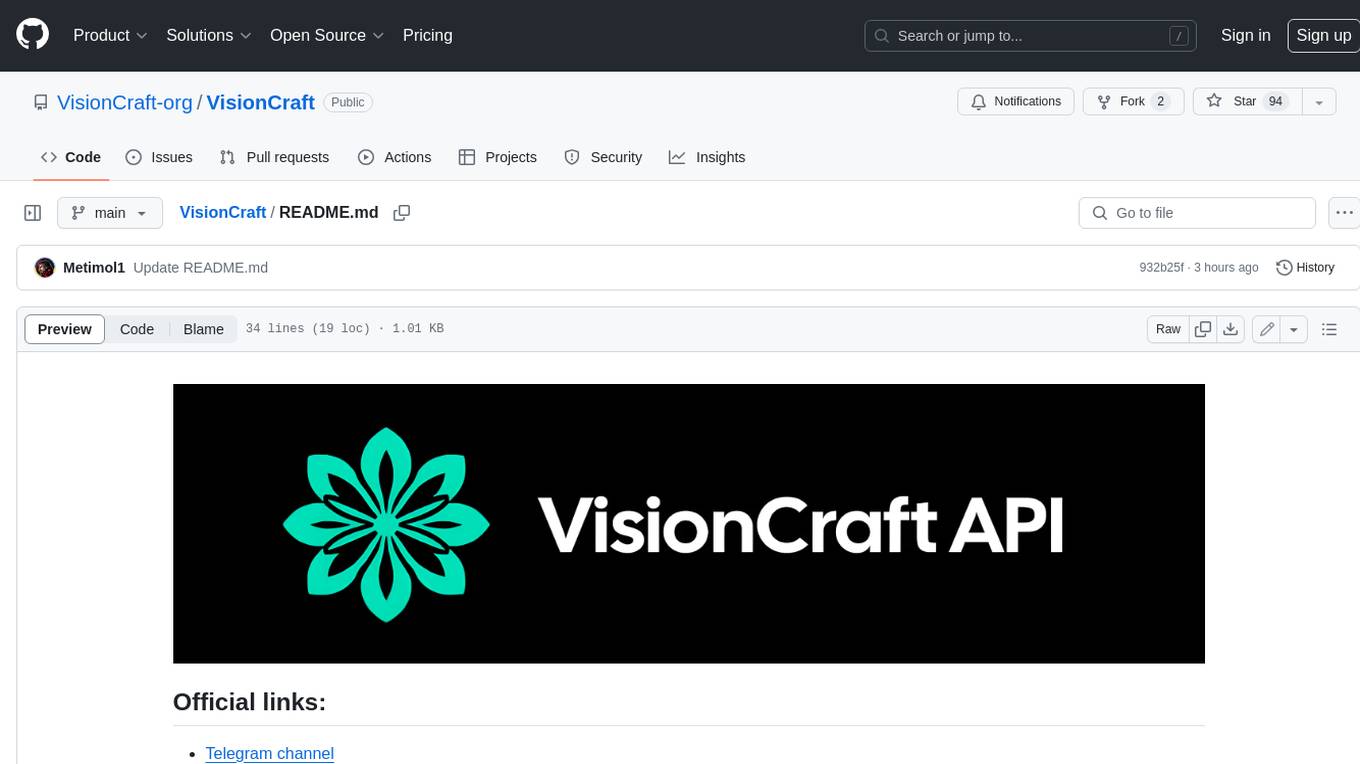
VisionCraft
The VisionCraft API is a free API for using over 100 different AI models. From images to sound.
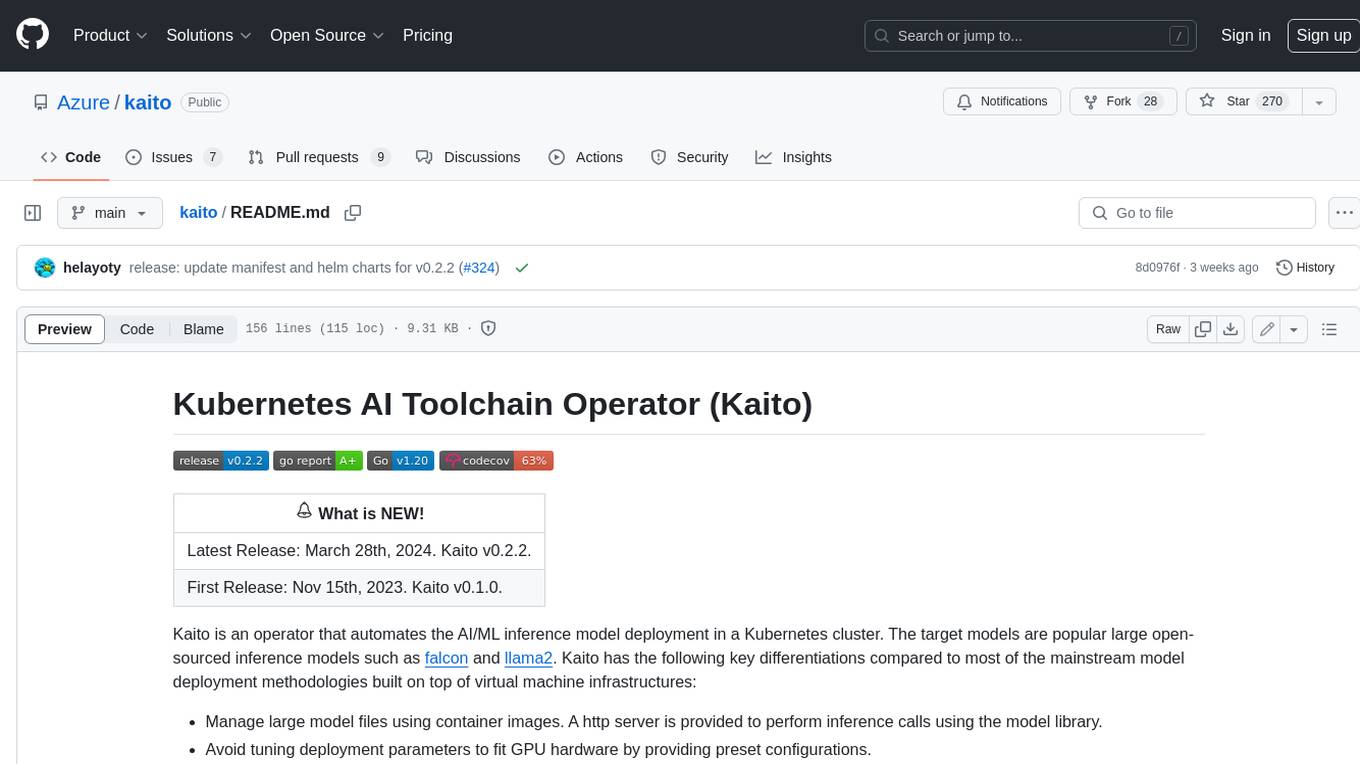
kaito
Kaito is an operator that automates the AI/ML inference model deployment in a Kubernetes cluster. It manages large model files using container images, avoids tuning deployment parameters to fit GPU hardware by providing preset configurations, auto-provisions GPU nodes based on model requirements, and hosts large model images in the public Microsoft Container Registry (MCR) if the license allows. Using Kaito, the workflow of onboarding large AI inference models in Kubernetes is largely simplified.
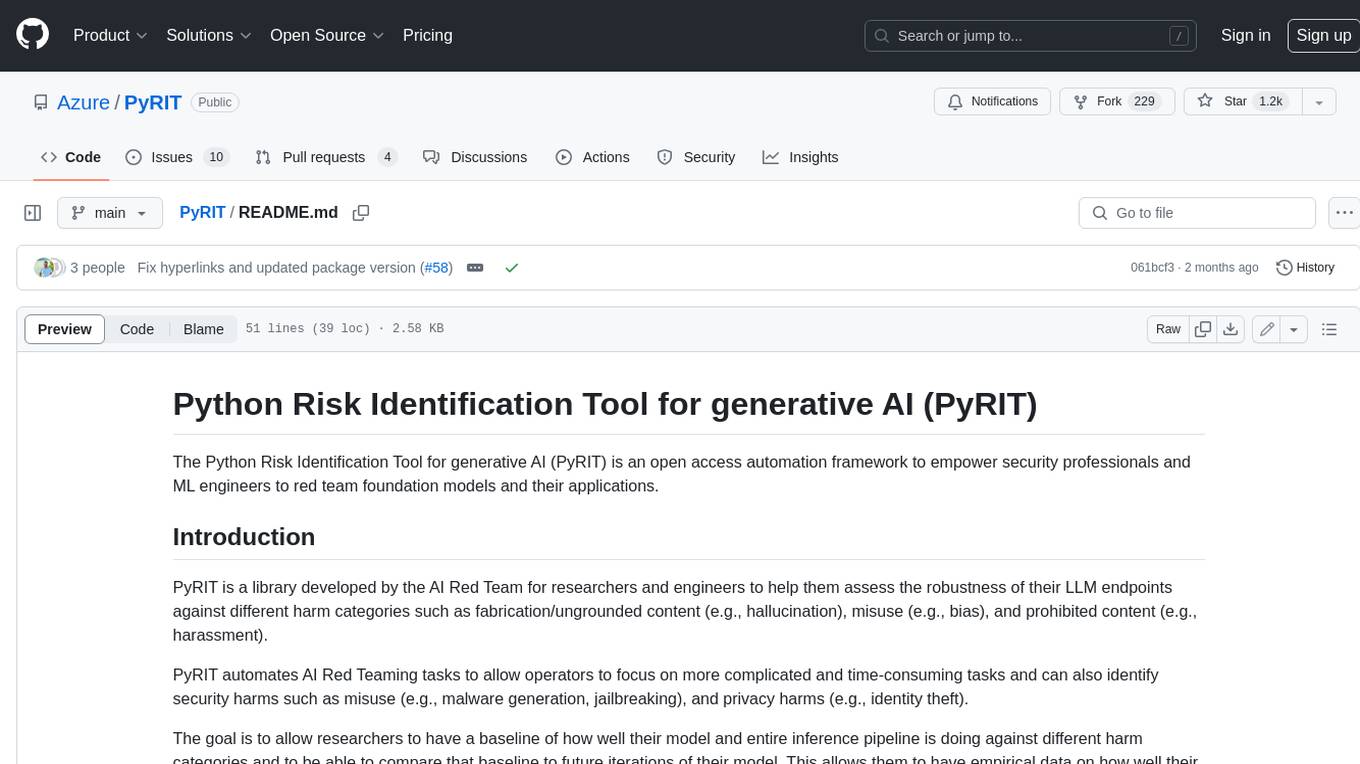
PyRIT
PyRIT is an open access automation framework designed to empower security professionals and ML engineers to red team foundation models and their applications. It automates AI Red Teaming tasks to allow operators to focus on more complicated and time-consuming tasks and can also identify security harms such as misuse (e.g., malware generation, jailbreaking), and privacy harms (e.g., identity theft). The goal is to allow researchers to have a baseline of how well their model and entire inference pipeline is doing against different harm categories and to be able to compare that baseline to future iterations of their model. This allows them to have empirical data on how well their model is doing today, and detect any degradation of performance based on future improvements.
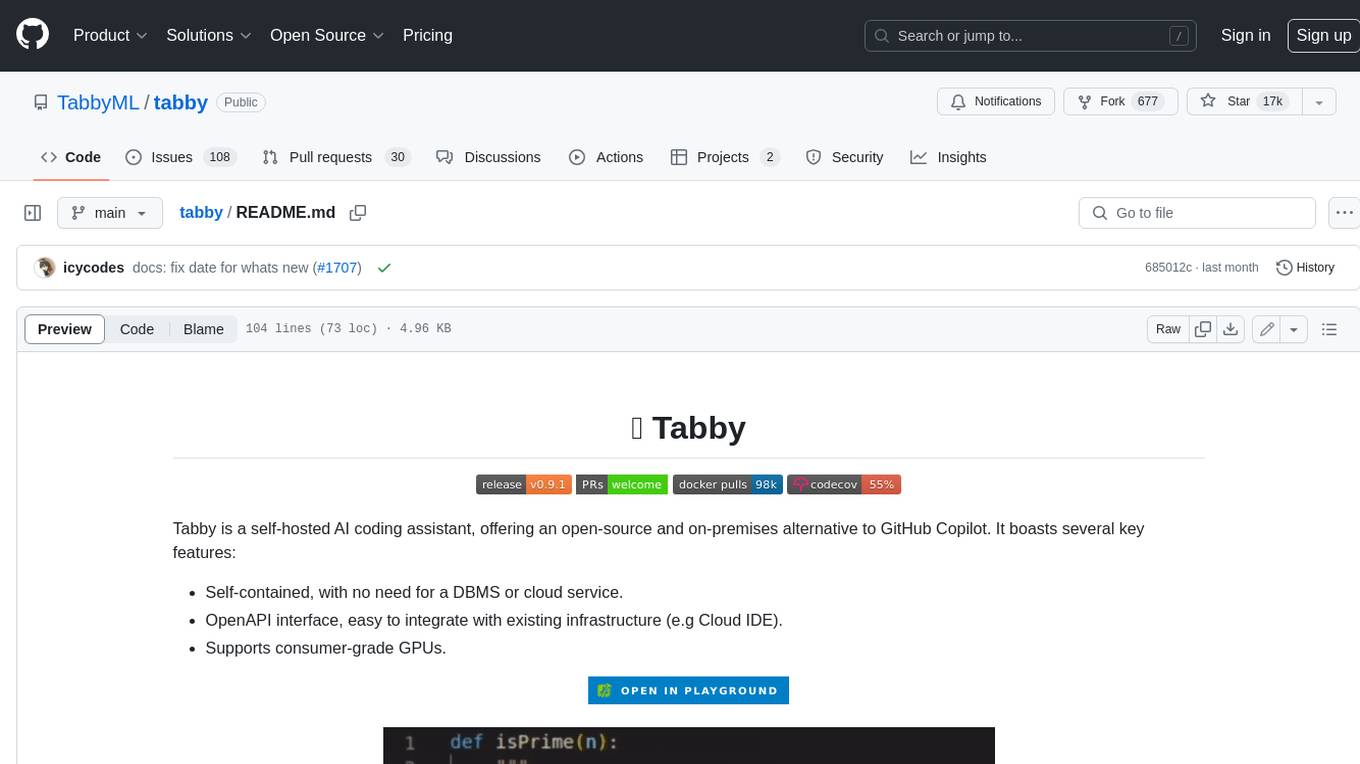
tabby
Tabby is a self-hosted AI coding assistant, offering an open-source and on-premises alternative to GitHub Copilot. It boasts several key features: * Self-contained, with no need for a DBMS or cloud service. * OpenAPI interface, easy to integrate with existing infrastructure (e.g Cloud IDE). * Supports consumer-grade GPUs.
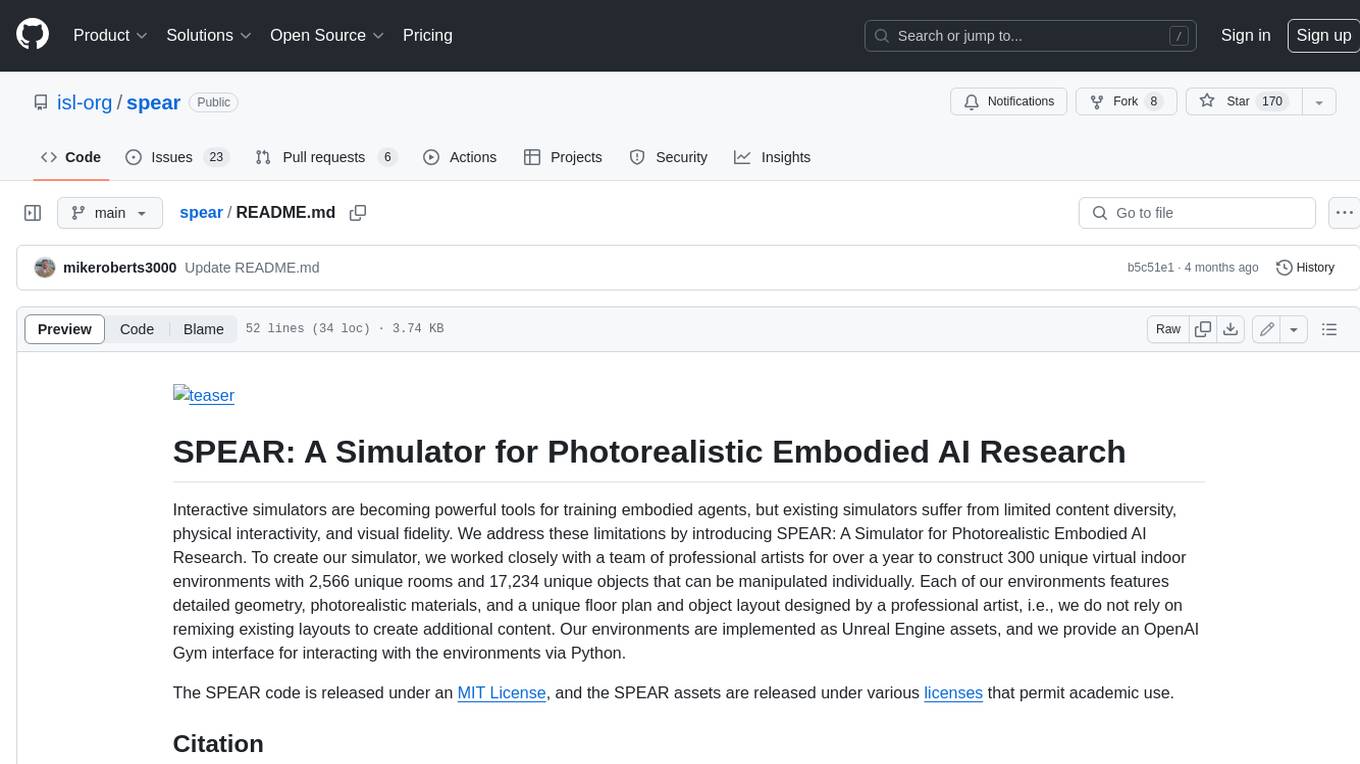
spear
SPEAR (Simulator for Photorealistic Embodied AI Research) is a powerful tool for training embodied agents. It features 300 unique virtual indoor environments with 2,566 unique rooms and 17,234 unique objects that can be manipulated individually. Each environment is designed by a professional artist and features detailed geometry, photorealistic materials, and a unique floor plan and object layout. SPEAR is implemented as Unreal Engine assets and provides an OpenAI Gym interface for interacting with the environments via Python.
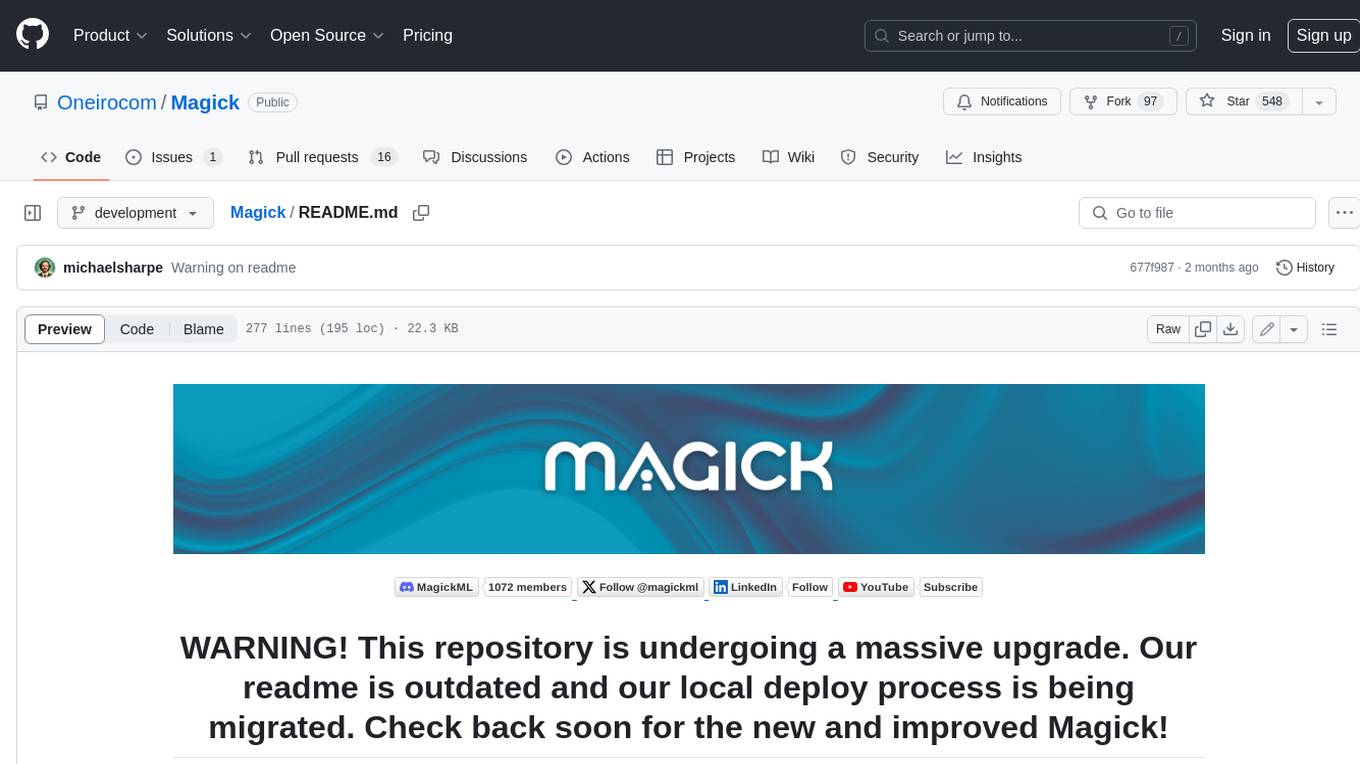
Magick
Magick is a groundbreaking visual AIDE (Artificial Intelligence Development Environment) for no-code data pipelines and multimodal agents. Magick can connect to other services and comes with nodes and templates well-suited for intelligent agents, chatbots, complex reasoning systems and realistic characters.