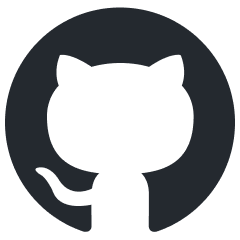
prime
prime (previously called ZeroBand) is a framework for efficient, globally distributed training of AI models over the internet.
Stars: 88
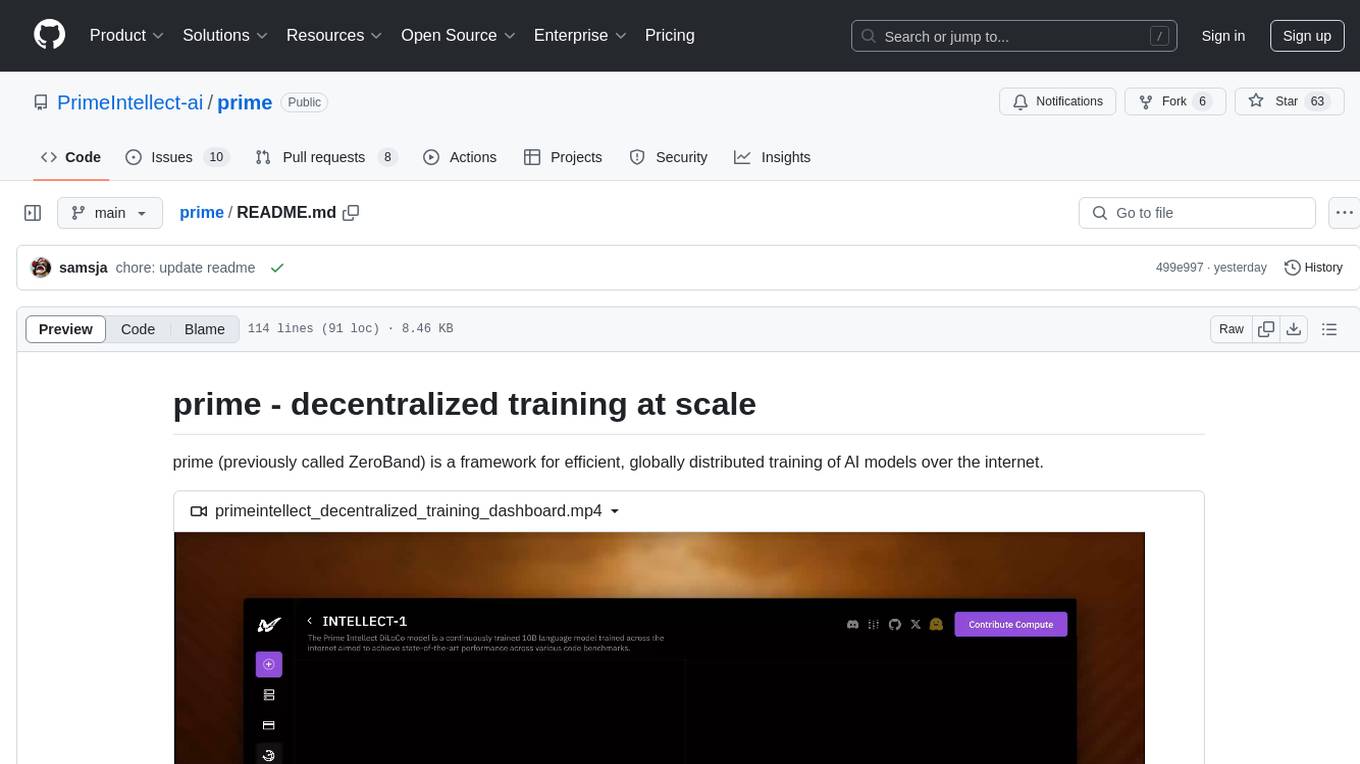
Prime is a framework for efficient, globally distributed training of AI models over the internet. It includes features such as fault-tolerant training with ElasticDeviceMesh, asynchronous distributed checkpointing, live checkpoint recovery, custom Int8 All-Reduce Kernel, maximizing bandwidth utilization, PyTorch FSDP2/DTensor ZeRO-3 implementation, and CPU off-loading. The framework aims to optimize communication, checkpointing, and bandwidth utilization for large-scale AI model training.
README:
prime (previously called ZeroBand) is a framework for efficient, globally distributed training of AI models over the internet.
https://github.com/user-attachments/assets/c034d2a2-400c-4bf8-acd0-c84b6c897d69
-
ElasticDeviceMesh
for Fault Tolerant Training:- In Prime, we’ve added a new distributed abstraction called
ElasticDeviceMesh
which encapsulates dynamic global process groups for fault-tolerant communication across the internet and local process groups for communication within a node or datacenter. - The
ElasticDeviceMesh
manages the resizing of the global process groups when nodes join or leave, unlike the standardDeviceMesh
in torch distributed, which will crash and require a cold restart to resize the process group. - In order to know when to resize the process groups, we use a heartbeat mechanism to discover dead nodes and remove them from the process group. Crashing nodes will attempt a best effort deathrattle to fail their own heartbeat quickly, saving its comrades the timeout.
- In Prime, we’ve added a new distributed abstraction called
-
Asynchronous distributed checkpointing
- Due to the size of the model, checkpointing can be an expensive operation, taking up to 20 minutes on the nodes we tested. This would reduce our compute utilisation if it blocked the main training process.
- In order to minimize the blocking time, we first checkpoint into
/dev/shm
which is a RAM backed filesystem. This operation is much faster and we can unblock the main training process once the checkpoint has been created in/dev/shm
. - We then use two subprocesses to asynchronously copy the checkpoint out of
/dev/shm
into the checkpoint directory on disk as well as upload it to the remote.
-
Live checkpoint recovery
- Nodes that wish to join the run mid-training need to be able to get the most recent state of the model and optimiser before being able to contribute to the training. They must complete this operation in the time window between two outer steps, otherwise, the checkpoint they receive would be stale.
- In order to do this quickly, we have the joining nodes request the checkpoints from its peers which all host a sidecar HTTP server serving the latest checkpoint out of
/dev/shm
. - Once the joining node has downloaded and initialized the model, it skips the inner steps and joins the outer step with zero pseudo-gradients. This is to prevent the joining node from stalling the existing nodes. If the joining node also performed the inner steps, it would be late to the outer step by the time it took to download and load the checkpoint, reducing the clusters compute utilisation.
-
Custom Int8 All-Reduce Kernel
- In our experiments, we found that we are able to perform int8 quantization on the pseudo gradients without any impact on the loss curves. This means that we can reduce the payload size of each outer step all-reduce by 4x if we communicate the pseudo-gradients in int8 instead of fp32.
- However, we need to accumulate the reduce in fp32, dequantizing and re-quantizing intermediate results during the all-reduce. This is not supported by any collective communication libraries.
- We thus implemented our own fully pipelined ring-reduce kernel in C++ which is JIT compiled as a custom operator using the torch library.
- However, with the amount of quantization work we needed to perform, using the torch ops (
quantize_per_tensor
,scatter_add
,index
, etc) was too slow, resulting in underutilisation of our target network bandwidth of 4 Gbps. - We thus implemented our own multithreaded uint8 ops in C++ to perform the quantization and dequantization operations, improving the quantization speed by more than 60x.
-
Maximising bandwidth utilization:
- By sharding our DiLoCo pseudo-gradients in a node, we can maximise network bandwidth utilization by opening multiple connections at the same time when performing the all-reduce. This yielded a transfer speed improvement of 8x on some nodes.
- Relying on the public IP forward resulted in poor or unstable p2p bandwidth on some compute providers. To mitigate this, we employ VPN technology to optimize peer-to-peer connections between nodes, allowing us to better utilize the available internet bandwidth between nodes by modifying the routing of packets through the internet.
- We’ve improved bandwidth utilization between nodes in similar data center settings by up to 40x compared to our OpenDiLoCo release, achieving up to 4Gb/s connections between data centers across the whole United States.
-
PyTorch FSDP2 / DTensor ZeRO-3 implementation
- In order to fit the 10B model training within our given memory resources, we had to do shard the model weights, gradients and optimizer states between intra-node GPUs.
- We achieved this using the
fully_shard
API from PyTorch FSDP2 which wraps the model parameters asDTensor
s and registers hooks to schedule all-gather and reduce-scatter on the tensors when they are used. FSDP2 also optimizes the collectives by bucketing the parameters intoFSDPParamGroup
s. This allows us to execute the collectives on larger tensors, improving protocol-to-payload ratio and improving the overlap from pipelining. We employ the same trick for our pseudo-gradients, bucketing them by layer.
-
CPU Off-Loading
- Our Diloco optimizer does not add any GPU overhead. All the tensors required by the Diloco optimizer are offloaded to CPU memory.
- Since we only perform a global sync every hundreds of steps, the reduced speed of copying and calculating the pseudo-gradient on cpu is negligible relative to the time to execute the inner steps and all-reduce.
A research paper about the framework and our INTELLECT-1 10B experiment is coming soon.
- Install
uv
:
curl -LsSf https://astral.sh/uv/install.sh | sh
source $HOME/.cargo/env
- Set up the environment:
uv venv
source .venv/bin/activate
uv sync --extra all
uv pip install flash-attn --no-build-isolation
git submodule update --init --recursive
Verify your setup:
ZERO_BAND_LOG_LEVEL=DEBUG torchrun --nproc_per_node=2 src/zeroband/train.py @configs/debug/normal.toml
To test DiLoCo locally you can use the helper script scripts/simulatsimulate_multi_nodee_mutl.sh
# Using 4 GPUs
ZERO_BAND_LOG_LEVEL=DEBUG ./scripts/simulate_multi_node_diloco.sh 2 2 src/zeroband/train.py @configs/debug/diloco.toml
# Using 2 GPUs
ZERO_BAND_LOG_LEVEL=DEBUG ./scripts/simulate_multi_node_diloco.sh 2 1 src/zeroband/train.py @configs/debug/diloco.toml
Note: Single GPU setups are currently not supported due to an FSDP implementation bug.
Ensure you have at least two GPU to run the full test suite:
uv run pytest
Environment Variable | Description | Default Value |
---|---|---|
GLOBAL_UNIQUE_ID |
Unique identifier worker in global store. | None |
GLOBAL_ADDR |
IP Address of the global store | None |
GLOBAL_PORT |
Port number of the global store. | None |
GLOBAL_WORLD_SIZE |
The size of the global process group. | 1 |
GLOBAL_RANK |
Rank of the process in the global process group. | 0 |
Environment Variable | Description | Default Value |
---|---|---|
ZERO_BAND_LOG_LEVEL |
Enable debug mode for loge | False |
ZERO_BAND_GLOBAL_STORE_TIMEOUT_SECONDS |
Number of seconds before the global store operations timeout | 300 |
ZERO_BAND_GLOBAL_PG_TIMEOUT_SECONDS |
Number of seconds before the global process group operations timeout | 600 |
ZERO_BAND_GLOBAL_STORE_POLLING_INTERVAL_SECONDS |
Number of seconds between polls to the store when waiting for values | 0.1 |
ZERO_BAND_EDM_HEARTBEAT_INTERVAL_SECONDS |
Interval in seconds between heartbeats | 2 |
ZERO_BAND_EDM_HEARTBEAT_TIMEOUT_SECONDS |
Time in seconds after which a node is considered dead if no heartbeat is received | 10 |
ZERO_BAND_LIVE_RECO_PORT |
Port number for the live recovery server | random |
ZERO_BAND_LIVE_RECO_ADDR |
IP Address for the live recovery server | localhost |
If you encounter any dataset loading errors at the beginning of training, try setting:
export HF_HUB_ETAG_TIMEOUT=500
For Tasks:
Click tags to check more tools for each tasksFor Jobs:
Alternative AI tools for prime
Similar Open Source Tools
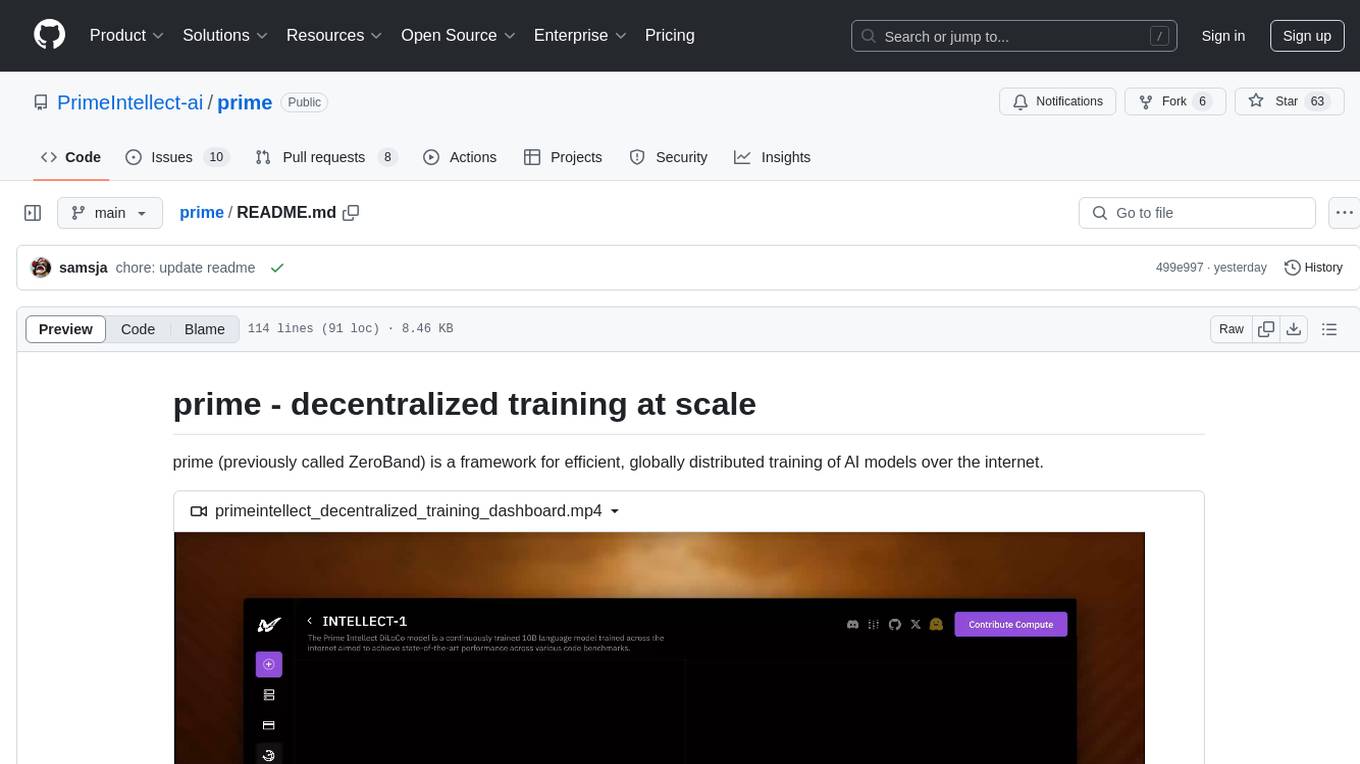
prime
Prime is a framework for efficient, globally distributed training of AI models over the internet. It includes features such as fault-tolerant training with ElasticDeviceMesh, asynchronous distributed checkpointing, live checkpoint recovery, custom Int8 All-Reduce Kernel, maximizing bandwidth utilization, PyTorch FSDP2/DTensor ZeRO-3 implementation, and CPU off-loading. The framework aims to optimize communication, checkpointing, and bandwidth utilization for large-scale AI model training.
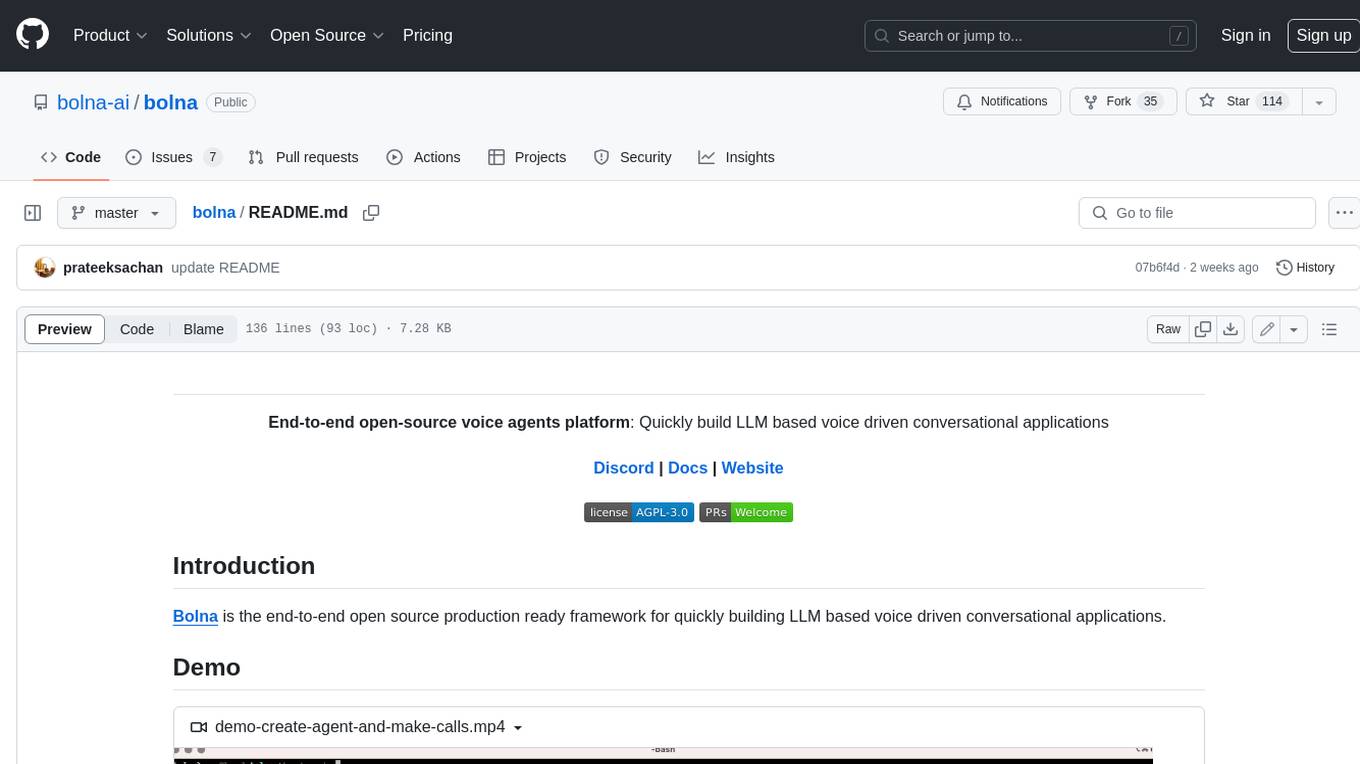
bolna
Bolna is an open-source platform for building voice-driven conversational applications using large language models (LLMs). It provides a comprehensive set of tools and integrations to handle various aspects of voice-based interactions, including telephony, transcription, LLM-based conversation handling, and text-to-speech synthesis. Bolna simplifies the process of creating voice agents that can perform tasks such as initiating phone calls, transcribing conversations, generating LLM-powered responses, and synthesizing speech. It supports multiple providers for each component, allowing users to customize their setup based on their specific needs. Bolna is designed to be easy to use, with a straightforward local setup process and well-documented APIs. It is also extensible, enabling users to integrate with other telephony providers or add custom functionality.
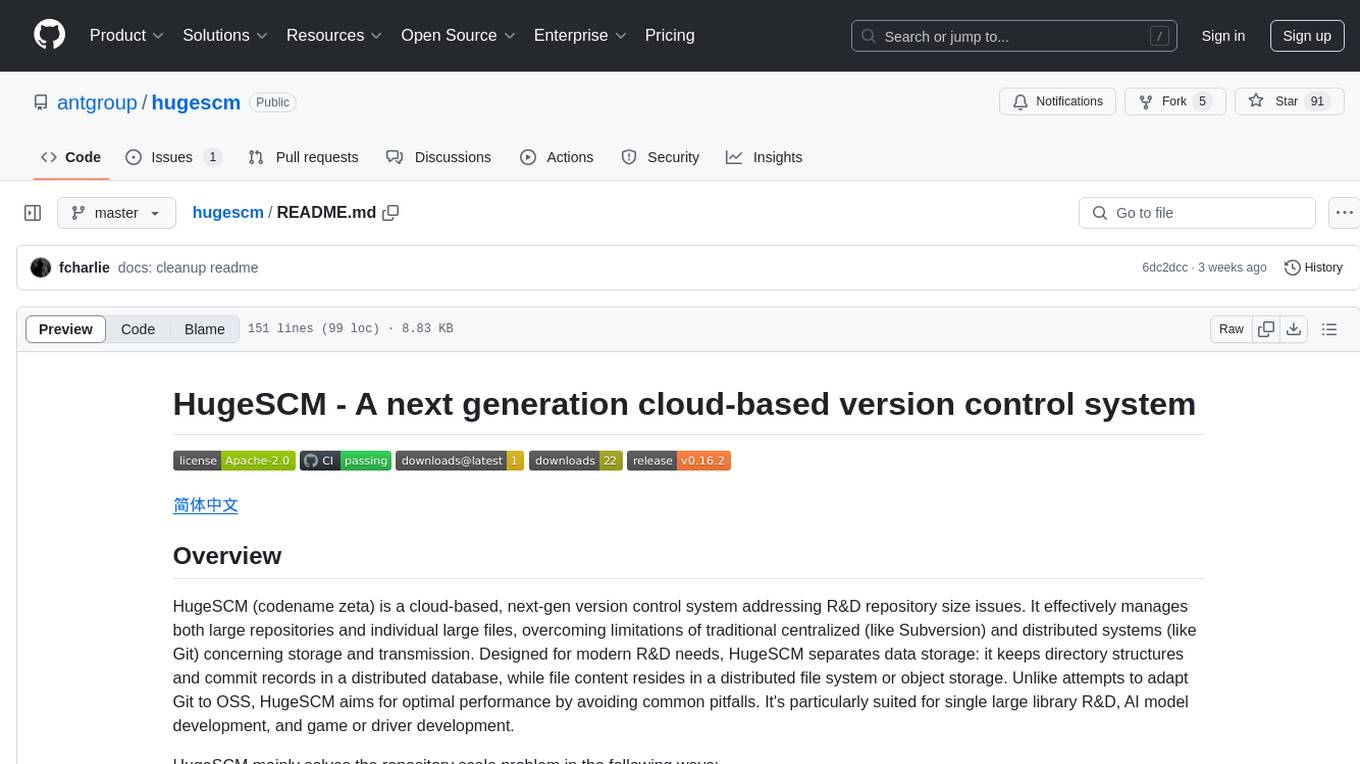
hugescm
HugeSCM is a cloud-based version control system designed to address R&D repository size issues. It effectively manages large repositories and individual large files by separating data storage and utilizing advanced algorithms and data structures. It aims for optimal performance in handling version control operations of large-scale repositories, making it suitable for single large library R&D, AI model development, and game or driver development.
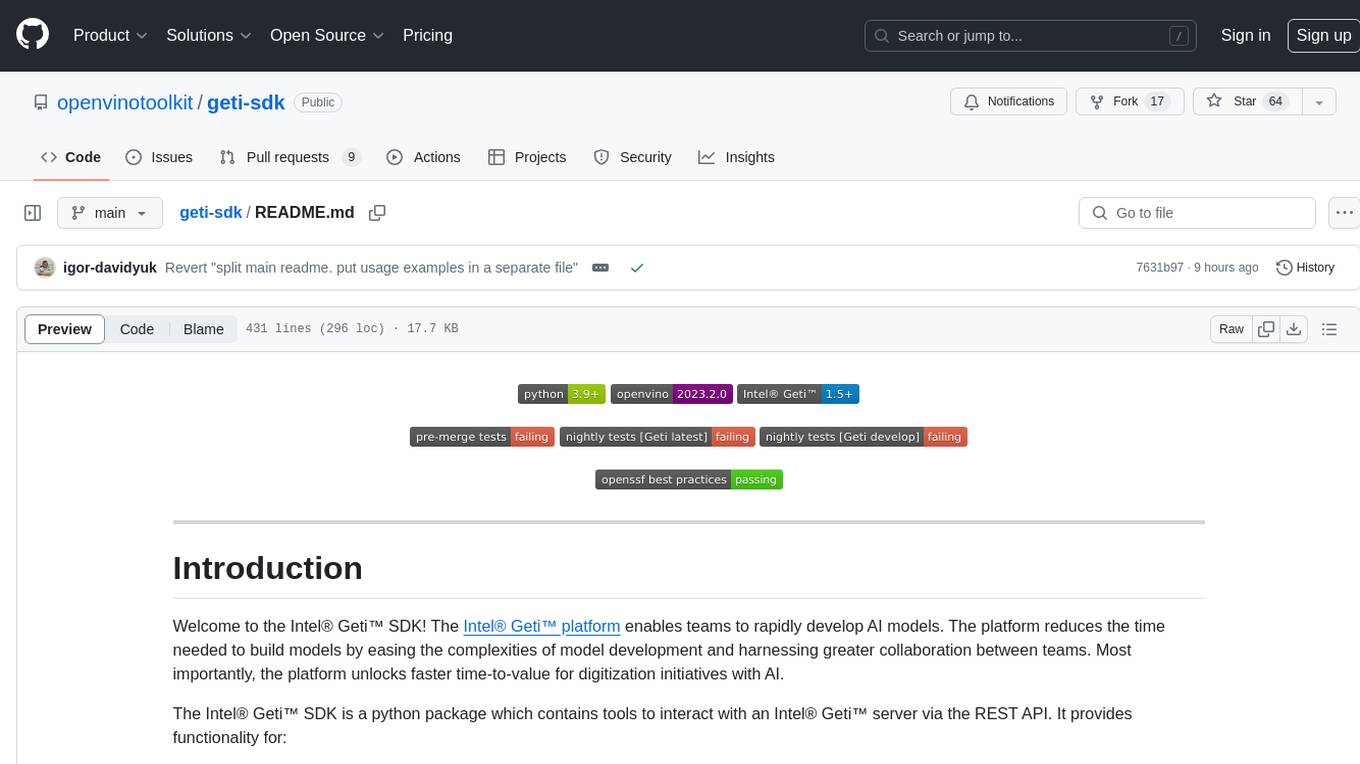
geti-sdk
The Intel® Geti™ SDK is a python package that enables teams to rapidly develop AI models by easing the complexities of model development and enhancing collaboration between teams. It provides tools to interact with an Intel® Geti™ server via the REST API, allowing for project creation, downloading, uploading, deploying for local inference with OpenVINO, setting project and model configuration, launching and monitoring training jobs, and media upload and prediction. The SDK also includes tutorial-style Jupyter notebooks demonstrating its usage.
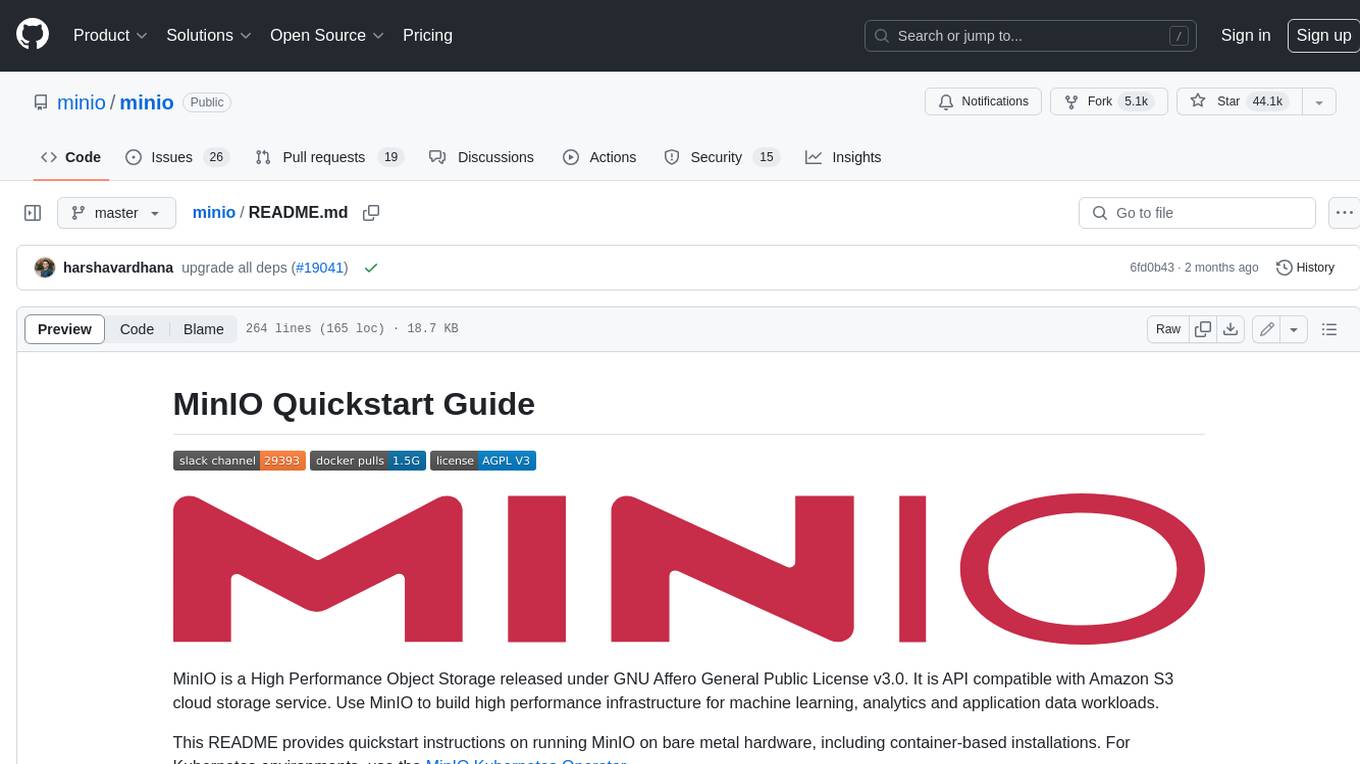
minio
MinIO is a High Performance Object Storage released under GNU Affero General Public License v3.0. It is API compatible with Amazon S3 cloud storage service. Use MinIO to build high performance infrastructure for machine learning, analytics and application data workloads.
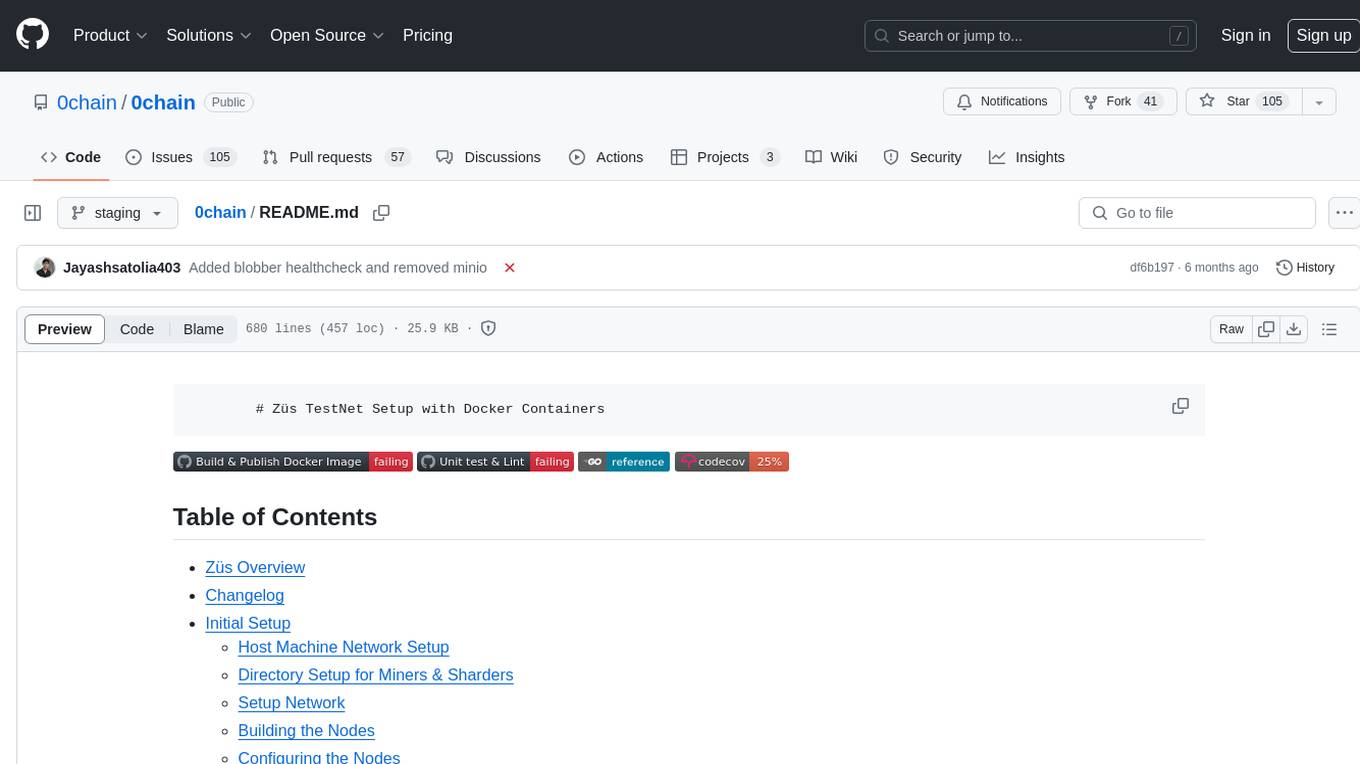
0chain
Züs is a high-performance cloud on a fast blockchain offering privacy and configurable uptime. It uses erasure code to distribute data between data and parity servers, allowing flexibility for IT managers to design for security and uptime. Users can easily share encrypted data with business partners through a proxy key sharing protocol. The ecosystem includes apps like Blimp for cloud migration, Vult for personal cloud storage, and Chalk for NFT artists. Other apps include Bolt for secure wallet and staking, Atlus for blockchain explorer, and Chimney for network participation. The QoS protocol challenges providers based on response time, while the privacy protocol enables secure data sharing. Züs supports hybrid and multi-cloud architectures, allowing users to improve regulatory compliance and security requirements.
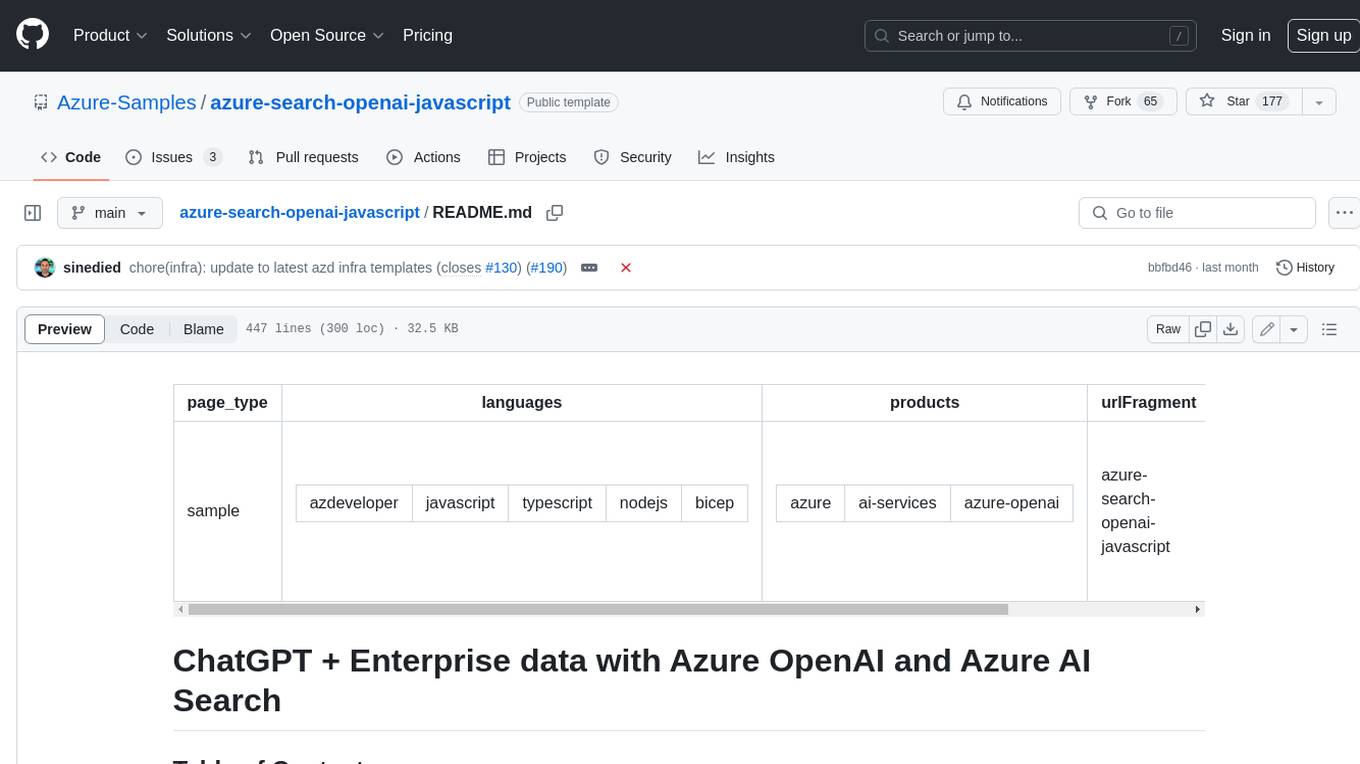
azure-search-openai-javascript
This sample demonstrates a few approaches for creating ChatGPT-like experiences over your own data using the Retrieval Augmented Generation pattern. It uses Azure OpenAI Service to access the ChatGPT model (gpt-35-turbo), and Azure AI Search for data indexing and retrieval.
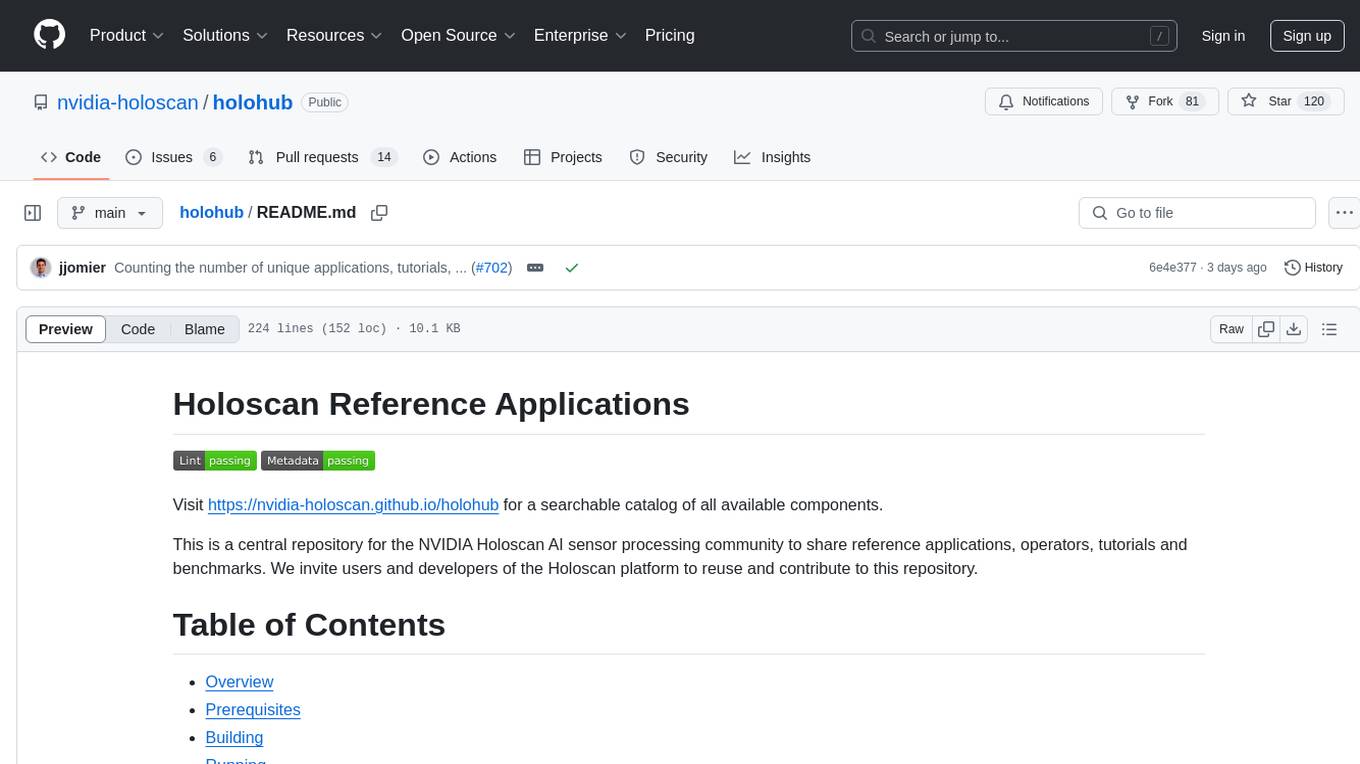
holohub
Holohub is a central repository for the NVIDIA Holoscan AI sensor processing community to share reference applications, operators, tutorials, and benchmarks. It includes example applications, community components, package configurations, and tutorials. Users and developers of the Holoscan platform are invited to reuse and contribute to this repository. The repository provides detailed instructions on prerequisites, building, running applications, contributing, and glossary terms. It also offers a searchable catalog of available components on the Holoscan SDK User Guide website.
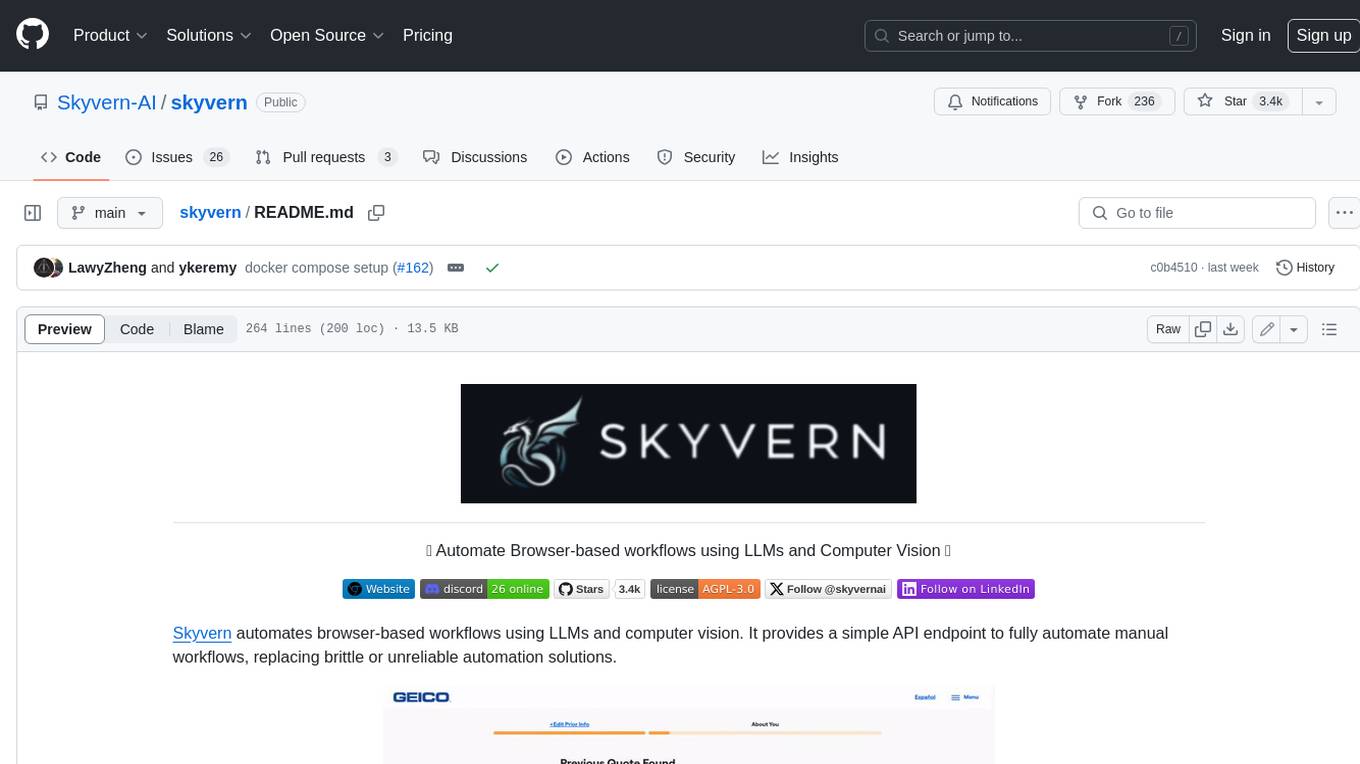
skyvern
Skyvern automates browser-based workflows using LLMs and computer vision. It provides a simple API endpoint to fully automate manual workflows, replacing brittle or unreliable automation solutions. Traditional approaches to browser automations required writing custom scripts for websites, often relying on DOM parsing and XPath-based interactions which would break whenever the website layouts changed. Instead of only relying on code-defined XPath interactions, Skyvern adds computer vision and LLMs to the mix to parse items in the viewport in real-time, create a plan for interaction and interact with them. This approach gives us a few advantages: 1. Skyvern can operate on websites it’s never seen before, as it’s able to map visual elements to actions necessary to complete a workflow, without any customized code 2. Skyvern is resistant to website layout changes, as there are no pre-determined XPaths or other selectors our system is looking for while trying to navigate 3. Skyvern leverages LLMs to reason through interactions to ensure we can cover complex situations. Examples include: 1. If you wanted to get an auto insurance quote from Geico, the answer to a common question “Were you eligible to drive at 18?” could be inferred from the driver receiving their license at age 16 2. If you were doing competitor analysis, it’s understanding that an Arnold Palmer 22 oz can at 7/11 is almost definitely the same product as a 23 oz can at Gopuff (even though the sizes are slightly different, which could be a rounding error!) Want to see examples of Skyvern in action? Jump to #real-world-examples-of- skyvern
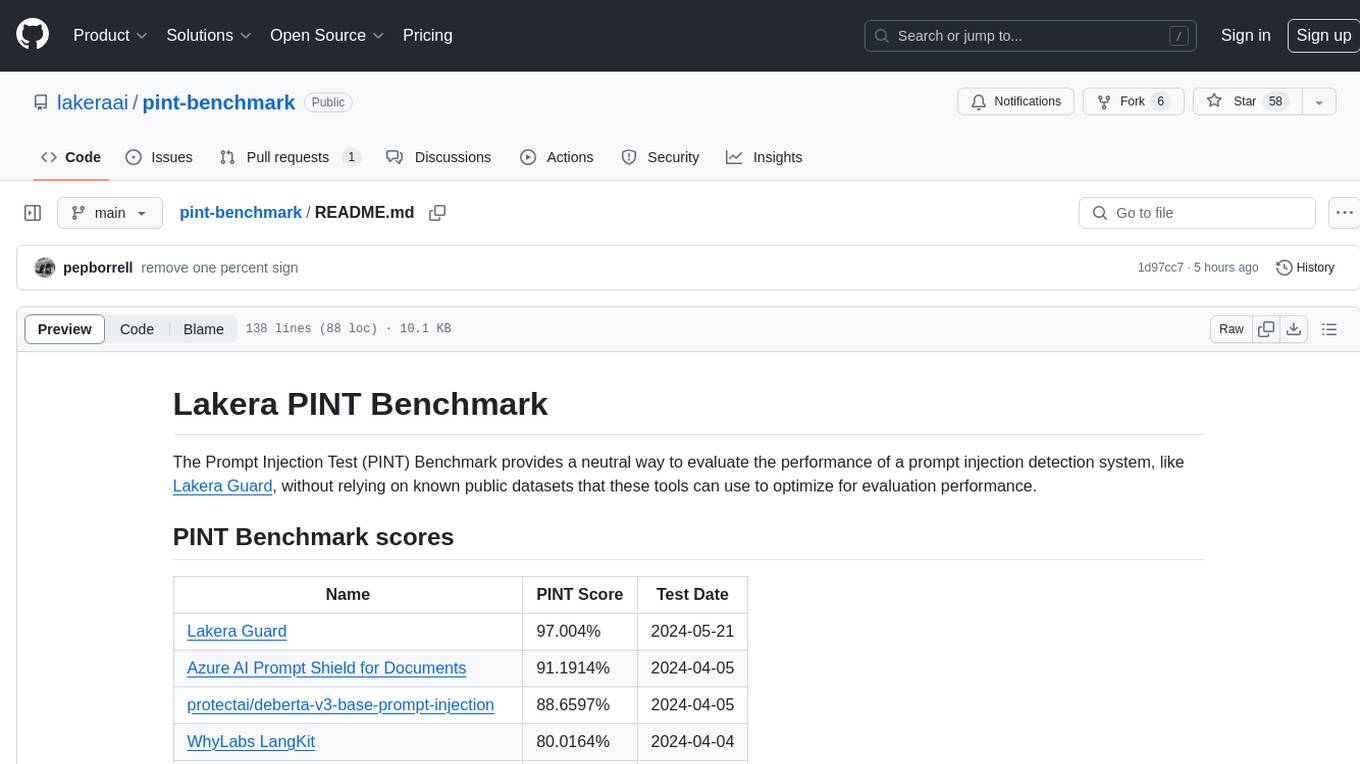
pint-benchmark
The Lakera PINT Benchmark provides a neutral evaluation method for prompt injection detection systems, offering a dataset of English inputs with prompt injections, jailbreaks, benign inputs, user-agent chats, and public document excerpts. The dataset is designed to be challenging and representative, with plans for future enhancements. The benchmark aims to be unbiased and accurate, welcoming contributions to improve prompt injection detection. Users can evaluate prompt injection detection systems using the provided Jupyter Notebook. The dataset structure is specified in YAML format, allowing users to prepare their datasets for benchmarking. Evaluation examples and resources are provided to assist users in evaluating prompt injection detection models and tools.
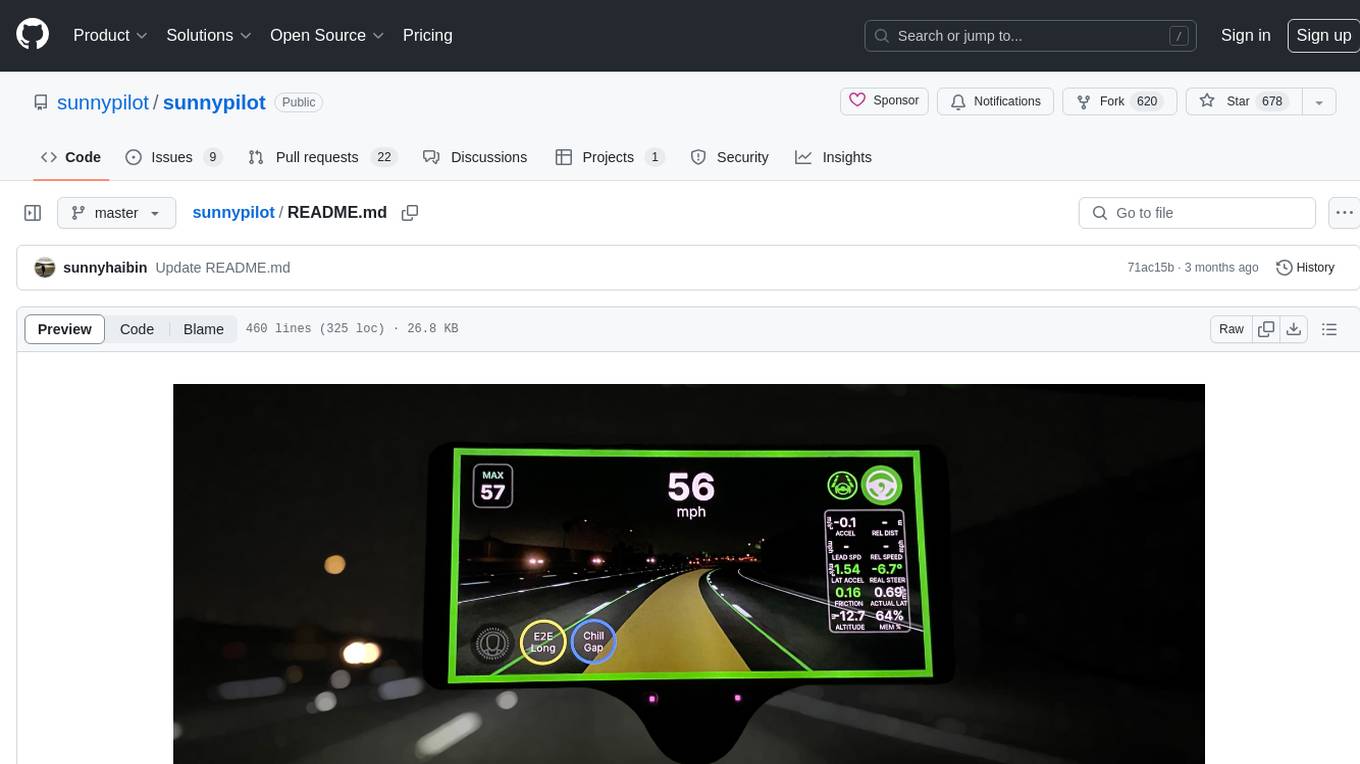
sunnypilot
Sunnypilot is a fork of comma.ai's openpilot, offering a unique driving experience for over 250+ supported car makes and models with modified behaviors of driving assist engagements. It complies with comma.ai's safety rules and provides features like Modified Assistive Driving Safety, Dynamic Lane Profile, Enhanced Speed Control, Gap Adjust Cruise, and more. Users can install it on supported devices and cars following detailed instructions, ensuring a safe and enhanced driving experience.
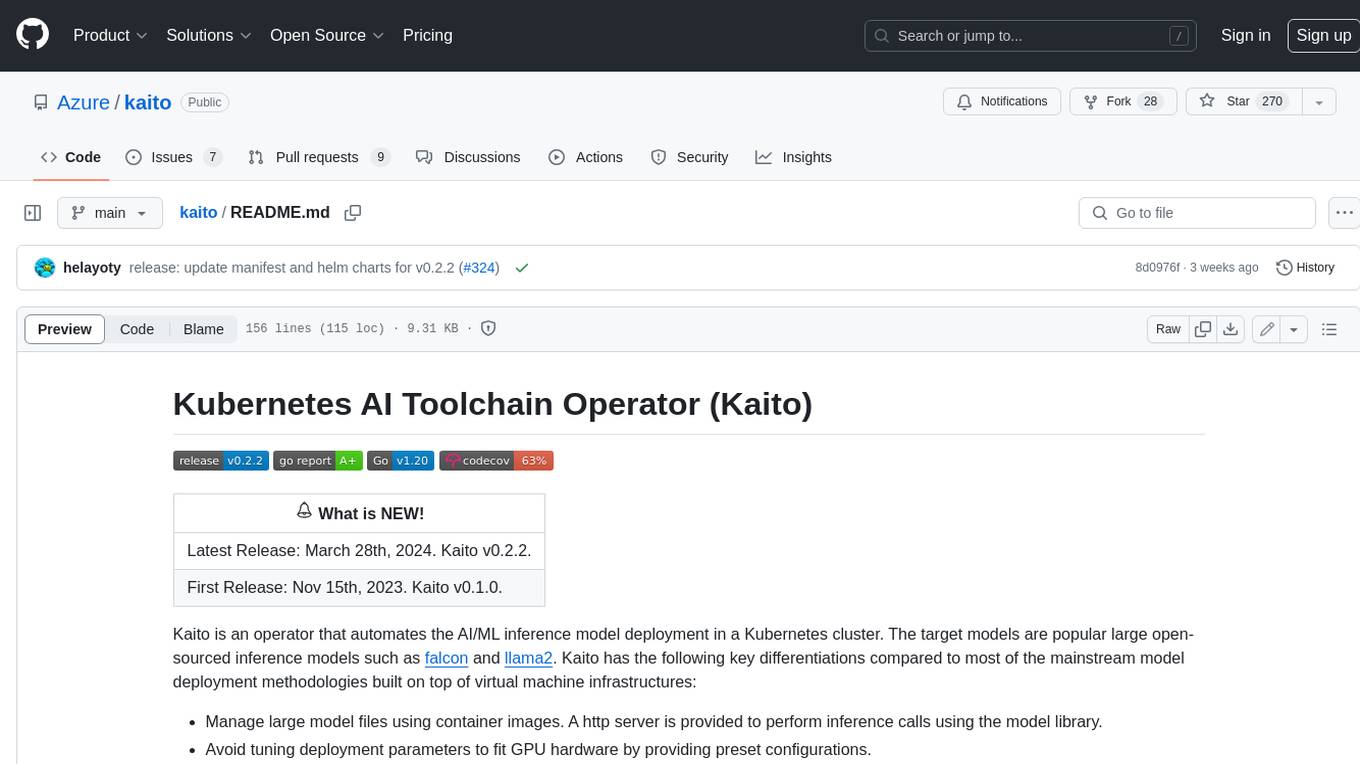
kaito
Kaito is an operator that automates the AI/ML inference model deployment in a Kubernetes cluster. It manages large model files using container images, avoids tuning deployment parameters to fit GPU hardware by providing preset configurations, auto-provisions GPU nodes based on model requirements, and hosts large model images in the public Microsoft Container Registry (MCR) if the license allows. Using Kaito, the workflow of onboarding large AI inference models in Kubernetes is largely simplified.
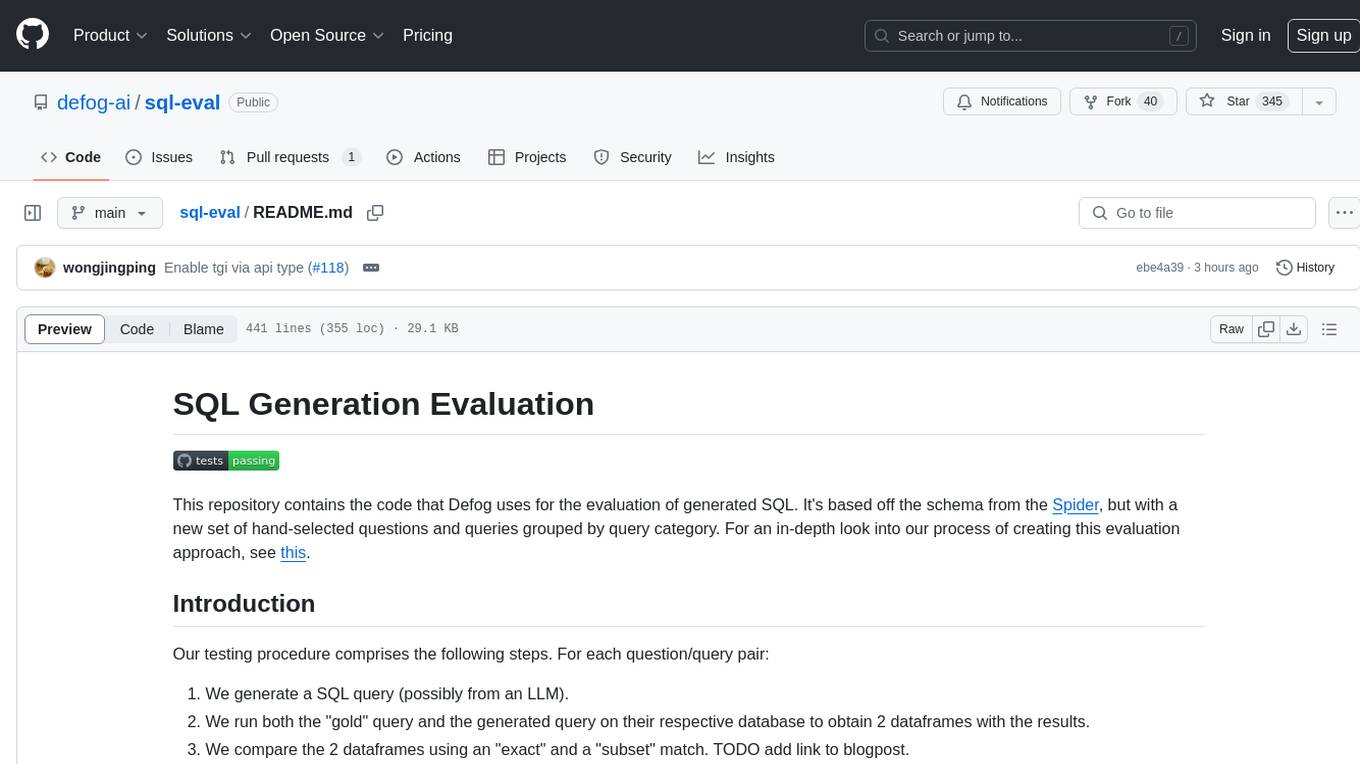
sql-eval
This repository contains the code that Defog uses for the evaluation of generated SQL. It's based off the schema from the Spider, but with a new set of hand-selected questions and queries grouped by query category. The testing procedure involves generating a SQL query, running both the 'gold' query and the generated query on their respective database to obtain dataframes with the results, comparing the dataframes using an 'exact' and a 'subset' match, logging these alongside other metrics of interest, and aggregating the results for reporting. The repository provides comprehensive instructions for installing dependencies, starting a Postgres instance, importing data into Postgres, importing data into Snowflake, using private data, implementing a query generator, and running the test with different runners.
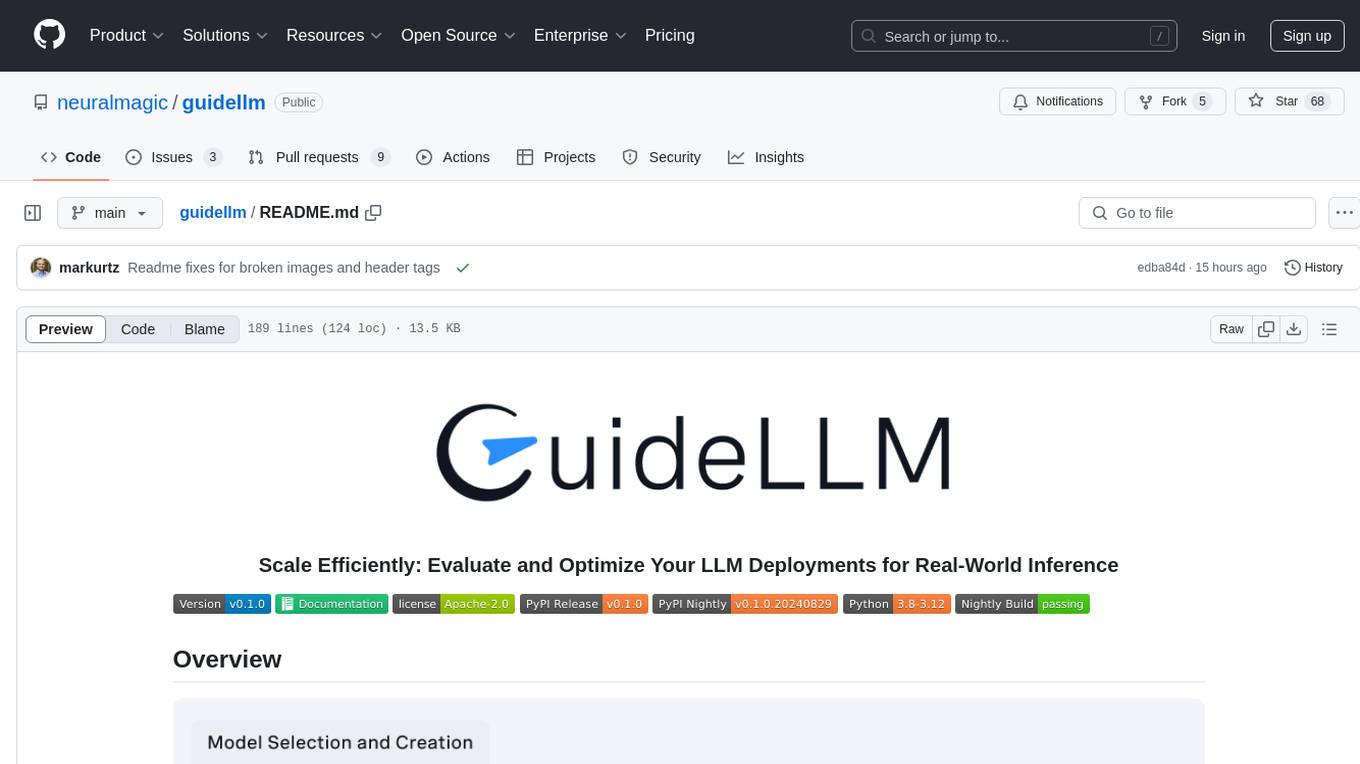
guidellm
GuideLLM is a powerful tool for evaluating and optimizing the deployment of large language models (LLMs). By simulating real-world inference workloads, GuideLLM helps users gauge the performance, resource needs, and cost implications of deploying LLMs on various hardware configurations. This approach ensures efficient, scalable, and cost-effective LLM inference serving while maintaining high service quality. Key features include performance evaluation, resource optimization, cost estimation, and scalability testing.
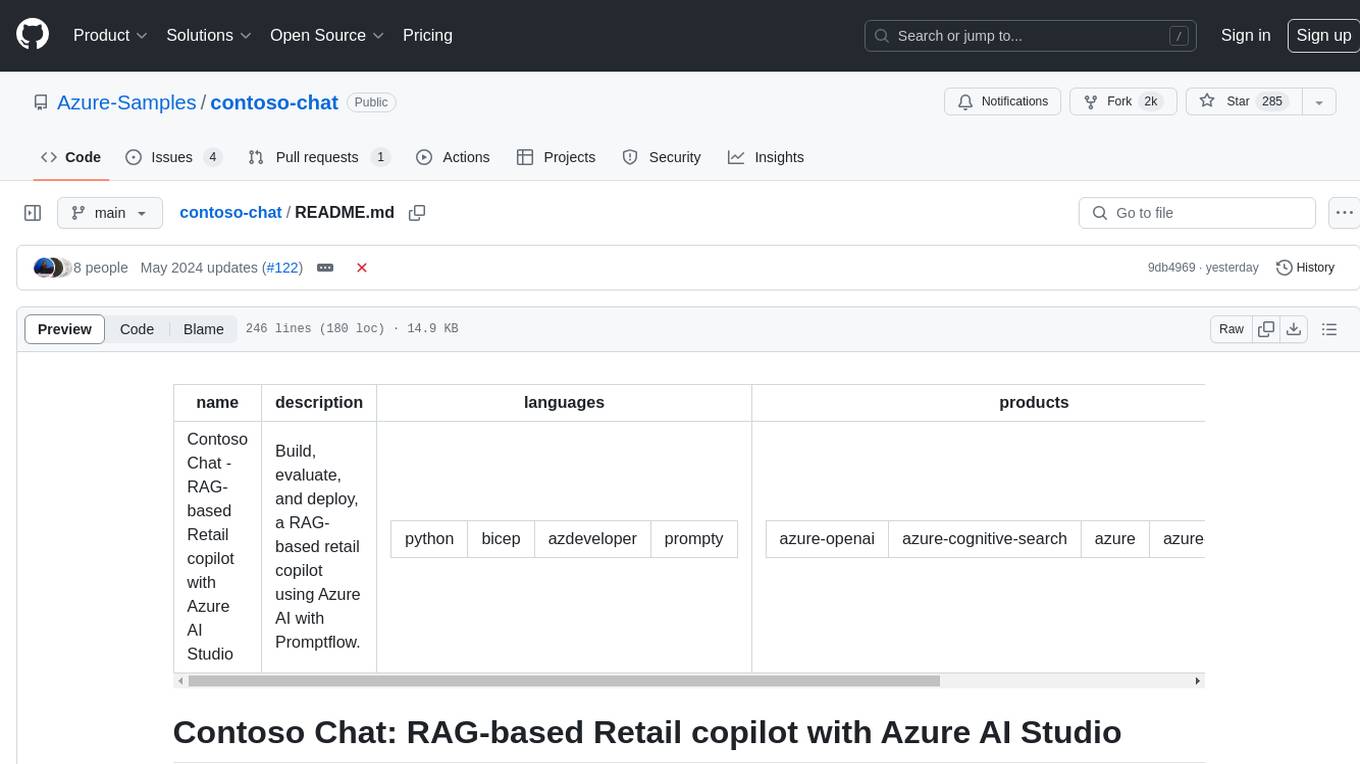
contoso-chat
Contoso Chat is a Python sample demonstrating how to build, evaluate, and deploy a retail copilot application with Azure AI Studio using Promptflow with Prompty assets. The sample implements a Retrieval Augmented Generation approach to answer customer queries based on the company's product catalog and customer purchase history. It utilizes Azure AI Search, Azure Cosmos DB, Azure OpenAI, text-embeddings-ada-002, and GPT models for vectorizing user queries, AI-assisted evaluation, and generating chat responses. By exploring this sample, users can learn to build a retail copilot application, define prompts using Prompty, design, run & evaluate a copilot using Promptflow, provision and deploy the solution to Azure using the Azure Developer CLI, and understand Responsible AI practices for evaluation and content safety.
For similar tasks
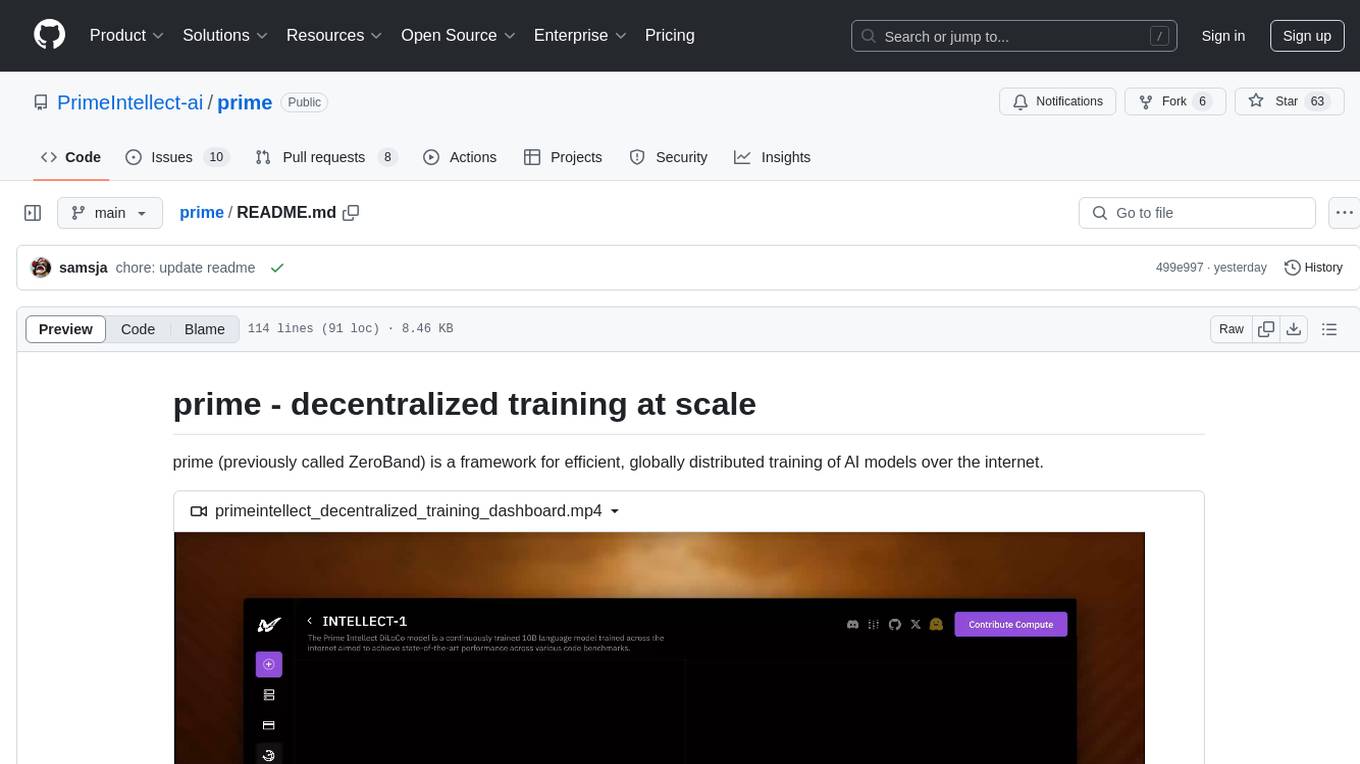
prime
Prime is a framework for efficient, globally distributed training of AI models over the internet. It includes features such as fault-tolerant training with ElasticDeviceMesh, asynchronous distributed checkpointing, live checkpoint recovery, custom Int8 All-Reduce Kernel, maximizing bandwidth utilization, PyTorch FSDP2/DTensor ZeRO-3 implementation, and CPU off-loading. The framework aims to optimize communication, checkpointing, and bandwidth utilization for large-scale AI model training.
For similar jobs
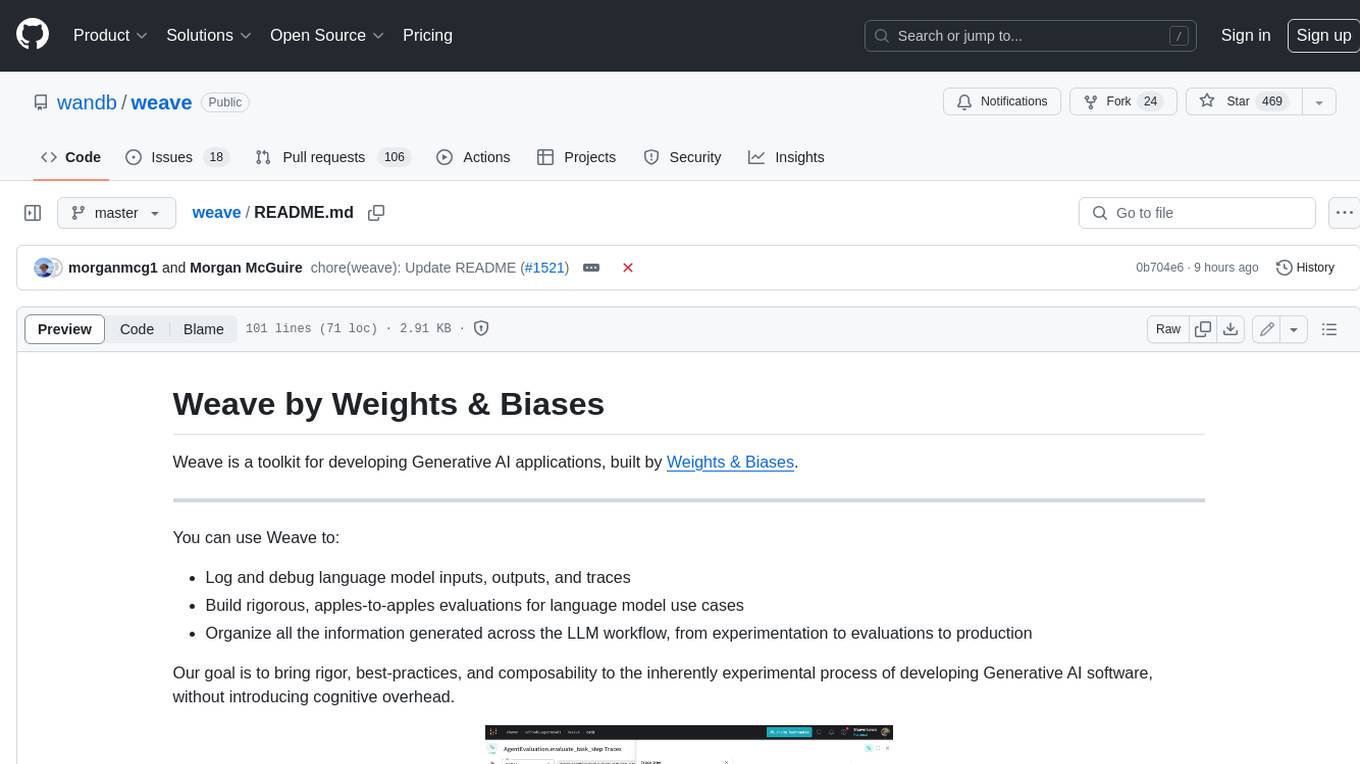
weave
Weave is a toolkit for developing Generative AI applications, built by Weights & Biases. With Weave, you can log and debug language model inputs, outputs, and traces; build rigorous, apples-to-apples evaluations for language model use cases; and organize all the information generated across the LLM workflow, from experimentation to evaluations to production. Weave aims to bring rigor, best-practices, and composability to the inherently experimental process of developing Generative AI software, without introducing cognitive overhead.
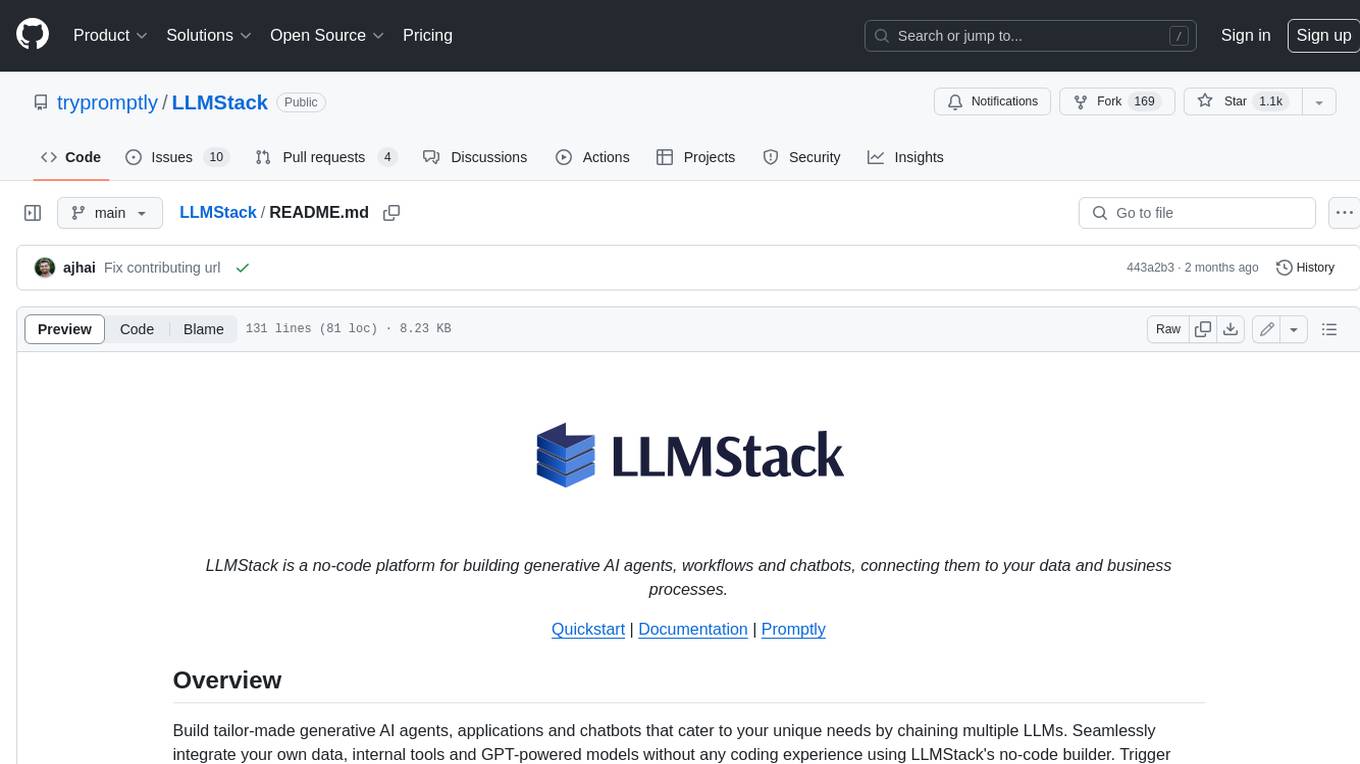
LLMStack
LLMStack is a no-code platform for building generative AI agents, workflows, and chatbots. It allows users to connect their own data, internal tools, and GPT-powered models without any coding experience. LLMStack can be deployed to the cloud or on-premise and can be accessed via HTTP API or triggered from Slack or Discord.
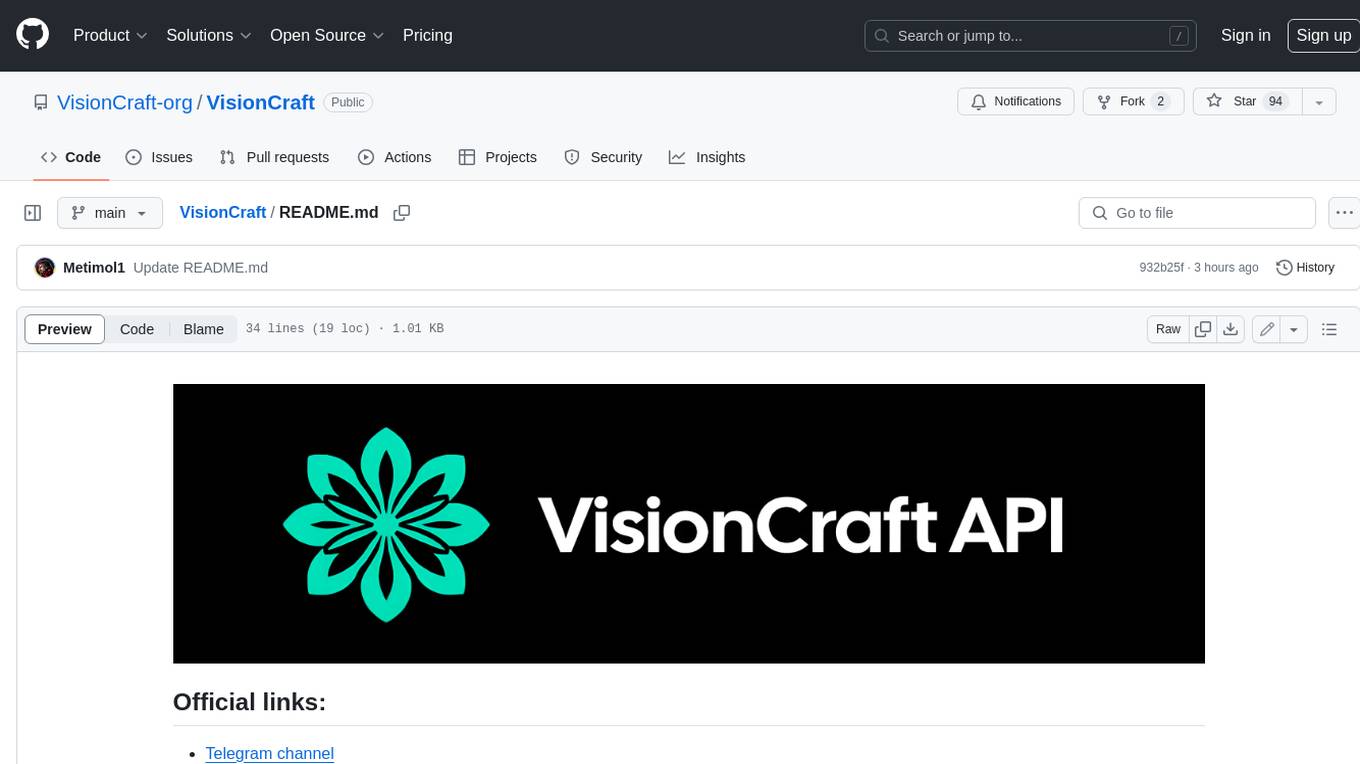
VisionCraft
The VisionCraft API is a free API for using over 100 different AI models. From images to sound.
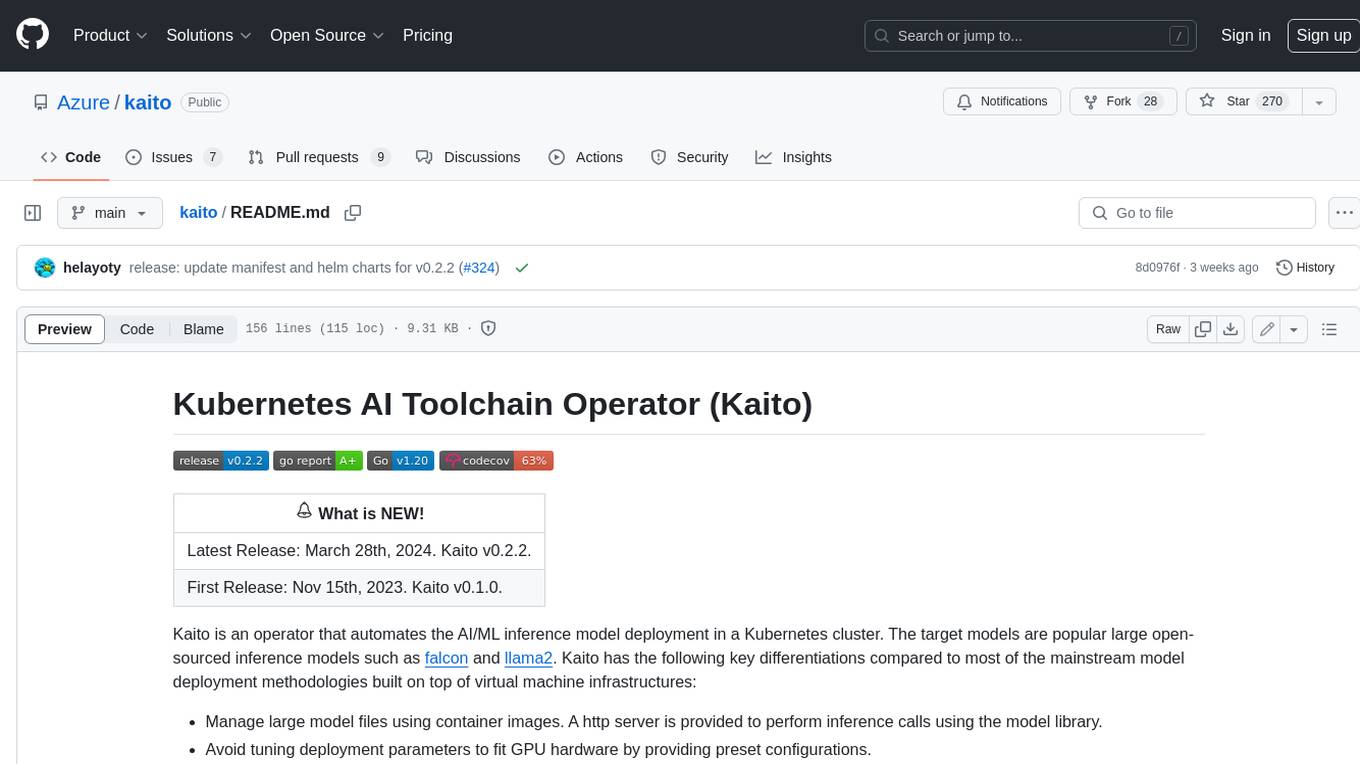
kaito
Kaito is an operator that automates the AI/ML inference model deployment in a Kubernetes cluster. It manages large model files using container images, avoids tuning deployment parameters to fit GPU hardware by providing preset configurations, auto-provisions GPU nodes based on model requirements, and hosts large model images in the public Microsoft Container Registry (MCR) if the license allows. Using Kaito, the workflow of onboarding large AI inference models in Kubernetes is largely simplified.
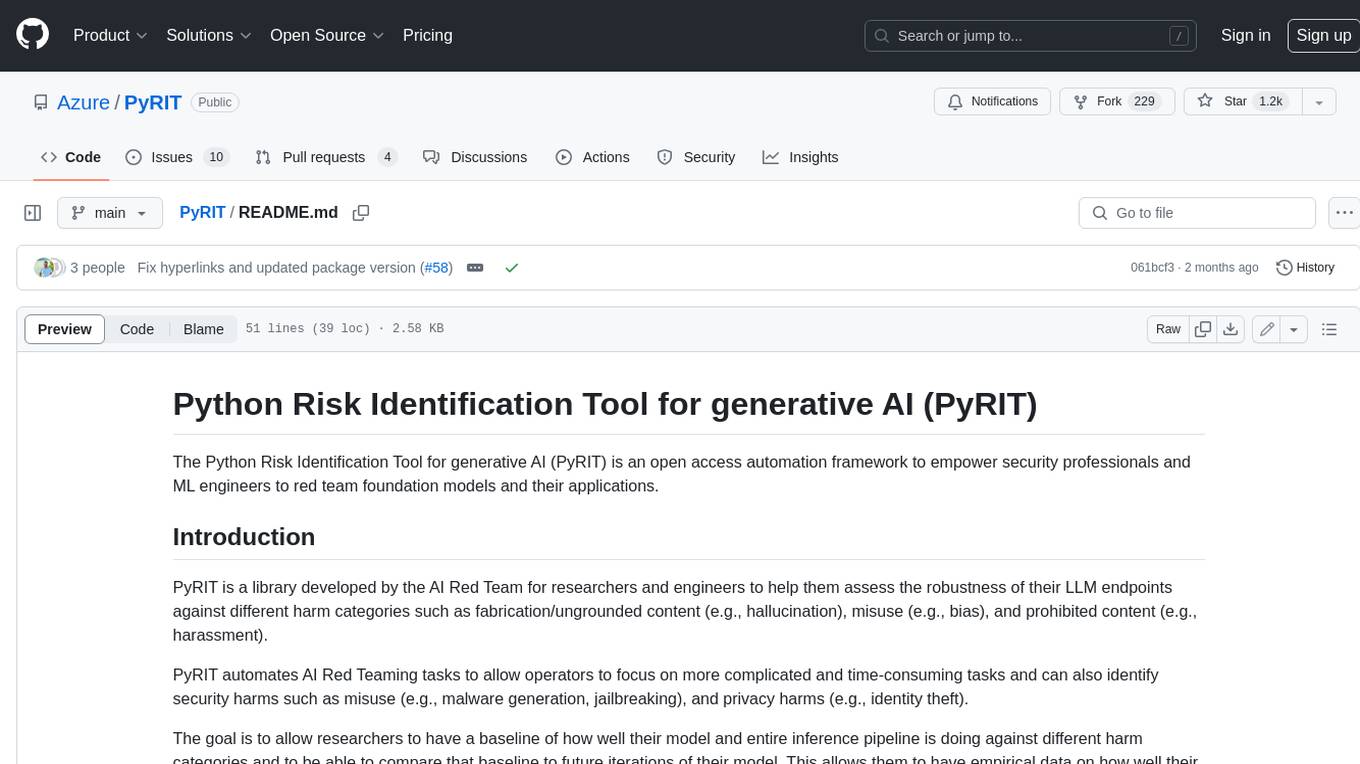
PyRIT
PyRIT is an open access automation framework designed to empower security professionals and ML engineers to red team foundation models and their applications. It automates AI Red Teaming tasks to allow operators to focus on more complicated and time-consuming tasks and can also identify security harms such as misuse (e.g., malware generation, jailbreaking), and privacy harms (e.g., identity theft). The goal is to allow researchers to have a baseline of how well their model and entire inference pipeline is doing against different harm categories and to be able to compare that baseline to future iterations of their model. This allows them to have empirical data on how well their model is doing today, and detect any degradation of performance based on future improvements.
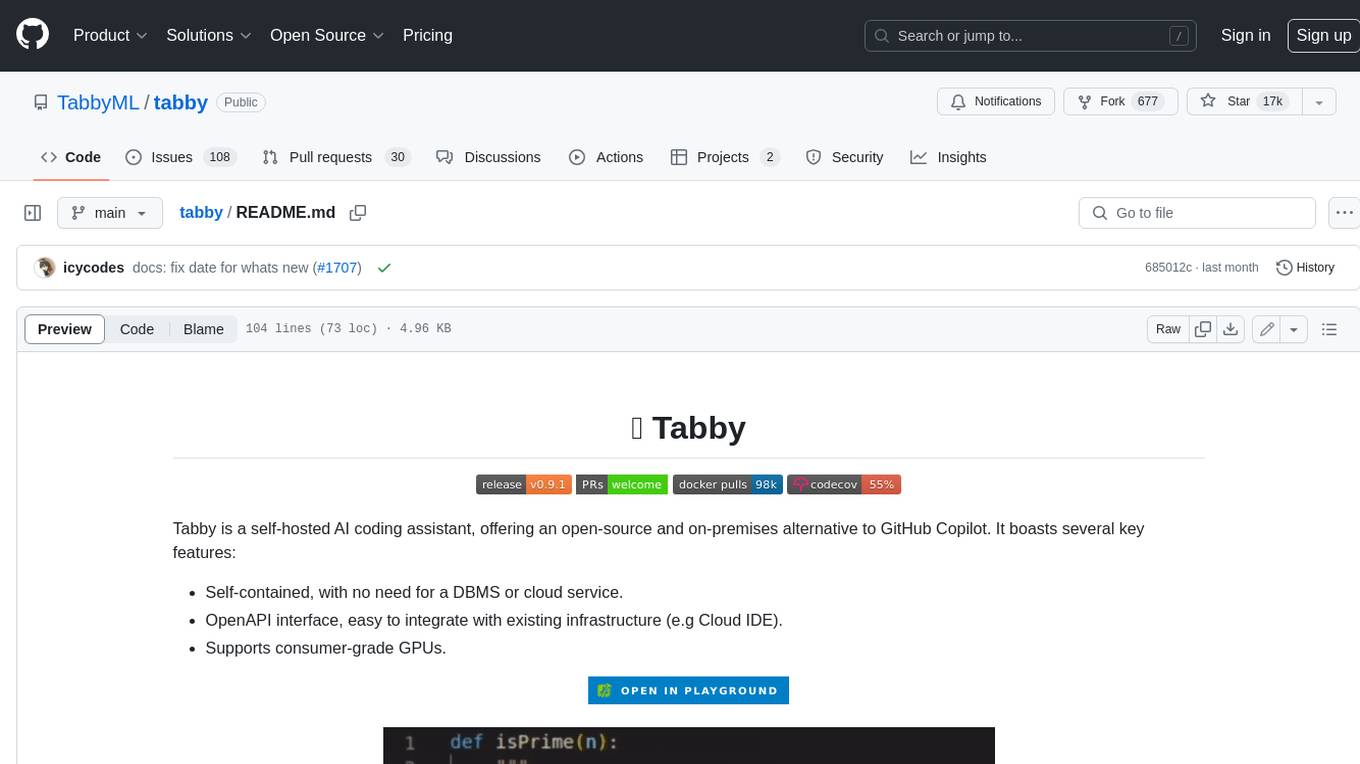
tabby
Tabby is a self-hosted AI coding assistant, offering an open-source and on-premises alternative to GitHub Copilot. It boasts several key features: * Self-contained, with no need for a DBMS or cloud service. * OpenAPI interface, easy to integrate with existing infrastructure (e.g Cloud IDE). * Supports consumer-grade GPUs.
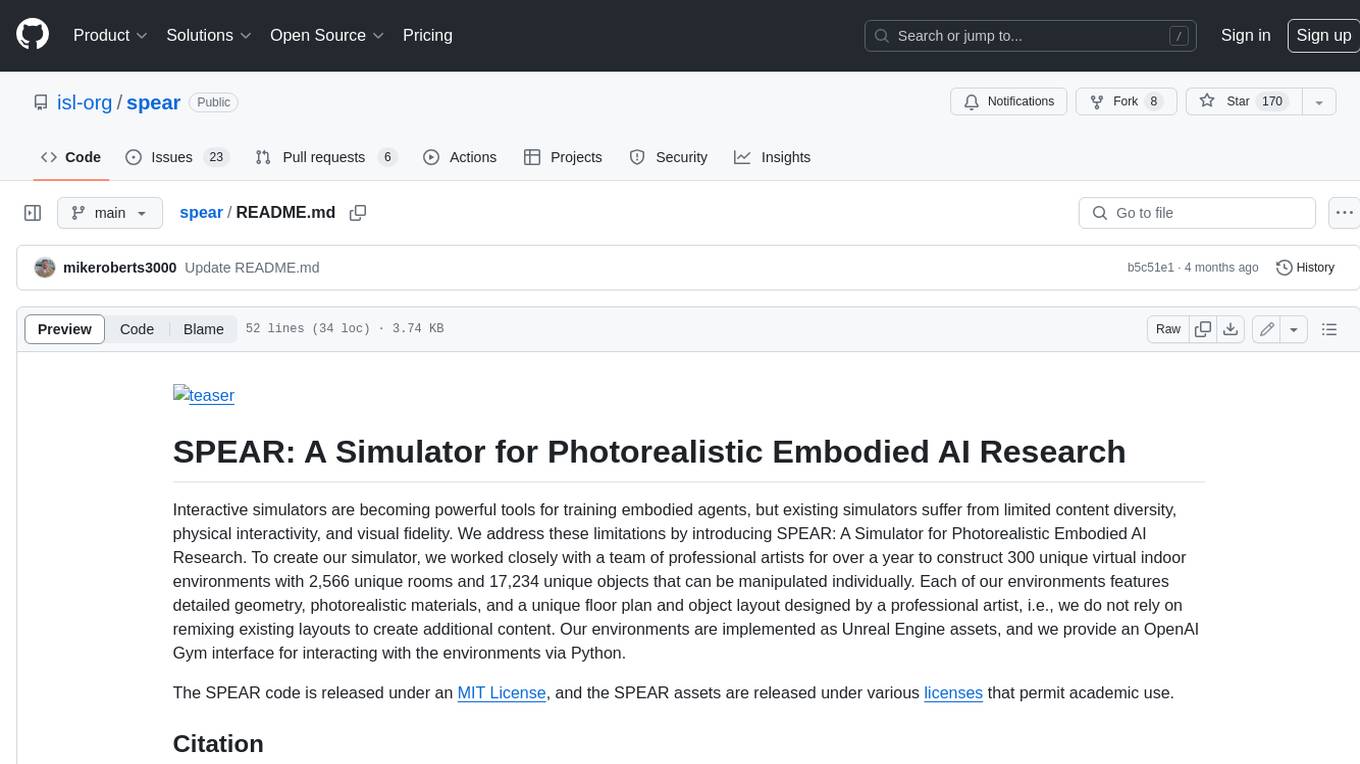
spear
SPEAR (Simulator for Photorealistic Embodied AI Research) is a powerful tool for training embodied agents. It features 300 unique virtual indoor environments with 2,566 unique rooms and 17,234 unique objects that can be manipulated individually. Each environment is designed by a professional artist and features detailed geometry, photorealistic materials, and a unique floor plan and object layout. SPEAR is implemented as Unreal Engine assets and provides an OpenAI Gym interface for interacting with the environments via Python.
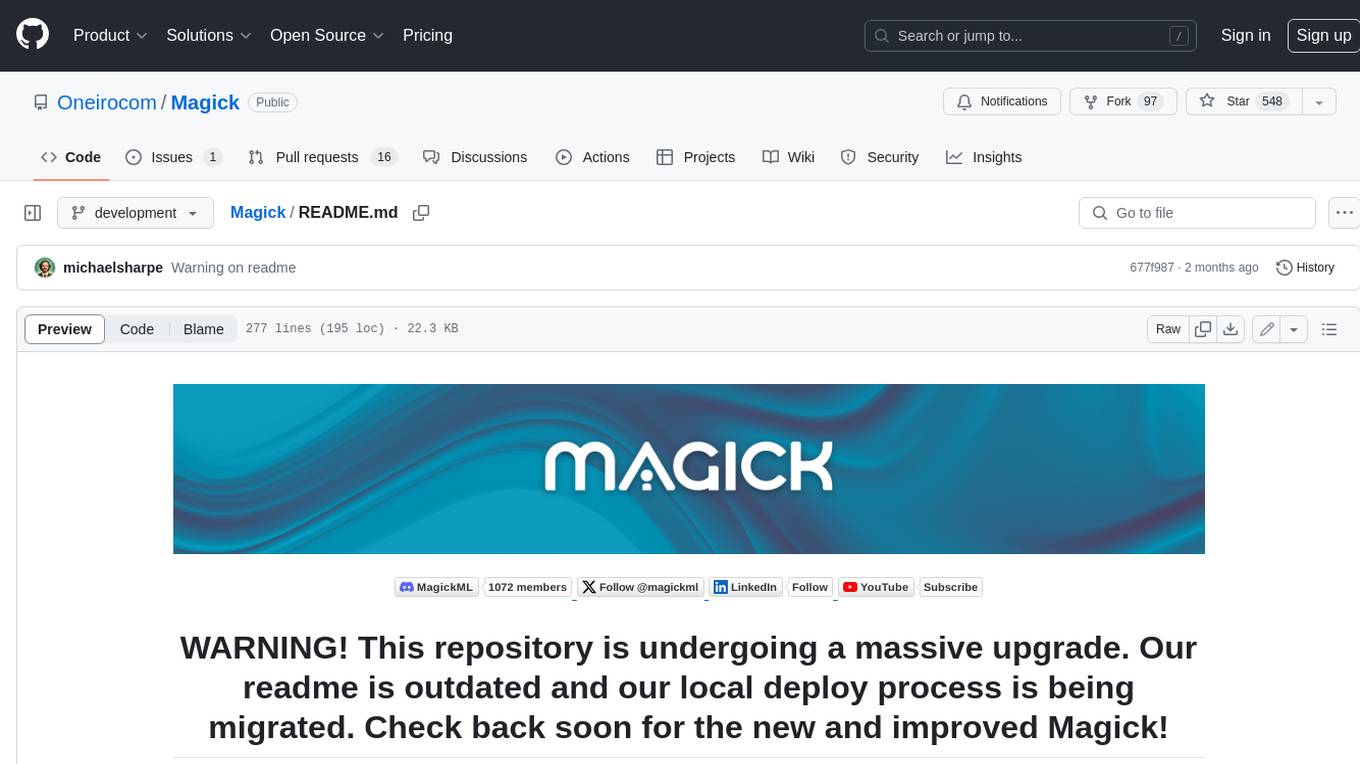
Magick
Magick is a groundbreaking visual AIDE (Artificial Intelligence Development Environment) for no-code data pipelines and multimodal agents. Magick can connect to other services and comes with nodes and templates well-suited for intelligent agents, chatbots, complex reasoning systems and realistic characters.