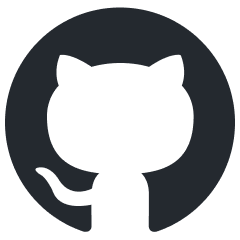
pdftochat
Chat with your PDFs with AI
Stars: 916
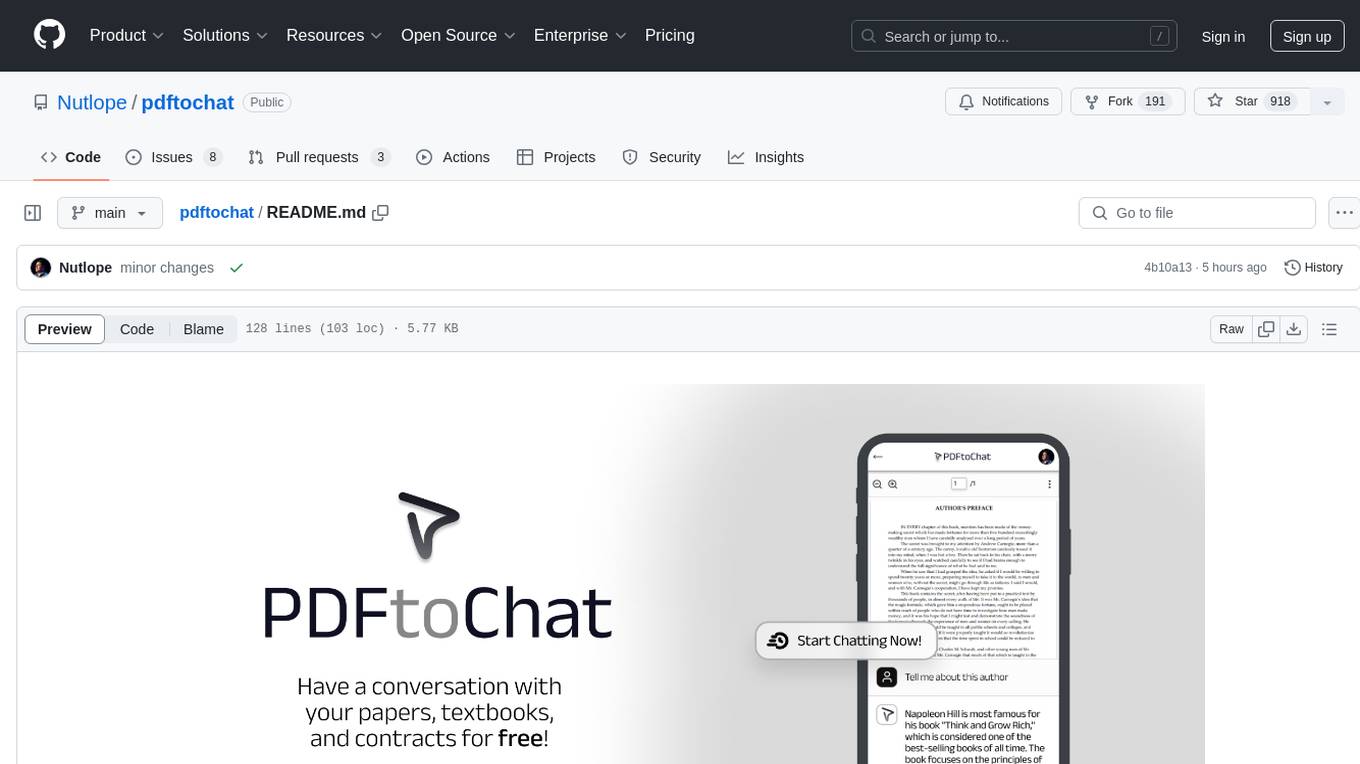
PDFToChat is a tool that allows users to chat with their PDF documents in seconds. It is powered by Together AI and Pinecone, utilizing a tech stack including Next.js, Mixtral, M2 Bert, LangChain.js, MongoDB Atlas, Bytescale, Vercel, Clerk, and Tailwind CSS. Users can deploy the tool to Vercel or any other host by setting up Together.ai, MongoDB Atlas database, Bytescale, Clerk, and Vercel. The tool enables users to interact with PDFs through chat, with future tasks including adding features like trash icon for deleting PDFs, exploring different embedding models, implementing auto scrolling, improving replies, benchmarking accuracy, researching chunking and retrieval best practices, adding demo video, upgrading to Next.js 14, adding analytics, customizing tailwind prose, saving chats in postgres DB, compressing large PDFs, implementing custom uploader, session tracking, error handling, and support for images in PDFs.
README:
Chat with your PDFs in seconds. Powered by Together AI and Pinecone.
Tech Stack · Deploy Your Own · Common Errors · Credits · Future Tasks
- Next.js App Router for the framework
- Mixtral through Together AI inference for the LLM
- M2 Bert 80M through Together AI for embeddings
- LangChain.js for the RAG code
- MongoDB Atlas for the vector database
- Bytescale for the PDF storage
- Vercel for hosting and for the postgres DB
- Clerk for user authentication
- Tailwind CSS for styling
You can deploy this template to Vercel or any other host. Note that you'll need to:
- Set up Together.ai
- Set up a MongoDB Atlas Atlas database with 768 dimensions
- See instructions below for MongoDB
- Set up Bytescale
- Set up Clerk
- Set up Vercel
- (Optional) Set up LangSmith for tracing.
See the .example.env for a list of all the required environment variables.
You will also need to prepare your database schema by running npx prisma db push
.
To set up a MongoDB Atlas database as the backing vectorstore, you will need to perform the following steps:
- Sign up on their website, then create a database cluster. Find it under the
Database
sidebar tab. - Create a collection by switching to
Collections
the tab and creating a blank collection. - Create an index by switching to the
Atlas Search
tab and clickingCreate Search Index
. - Make sure you select
Atlas Vector Search - JSON Editor
, select the appropriate database and collection, and paste the following into the textbox:
{
"fields": [
{
"numDimensions": 768,
"path": "embedding",
"similarity": "euclidean",
"type": "vector"
},
{
"path": "docstore_document_id",
"type": "filter"
}
]
}
Note that the numDimensions
is 768 dimensions to match the embeddings model we're using, and that we have another index on docstore_document_id
. This allows us to filter later.
You may call the index whatever you wish, just make a note of it!
- Finally, retrieve and set the following environment variables:
NEXT_PUBLIC_VECTORSTORE=mongodb # Set MongoDB Atlas as your vectorstore
MONGODB_ATLAS_URI= # Connection string for your database.
MONGODB_ATLAS_DB_NAME= # The name of your database.
MONGODB_ATLAS_COLLECTION_NAME= # The name of your collection.
MONGODB_ATLAS_INDEX_NAME= # The name of the index you just created.
- Check that you've created an
.env
file that contains your valid (and working) API keys, environment and index name. - Check that you've set the vector dimensions to
768
and thatindex
matched your specified field in the.env variable
. - Check that you've added a credit card on Together AI if you're hitting rate limiting issues due to the free tier
- Youssef for the design of the app
- Mayo for the original RAG repo and inspiration
- Jacob for the LangChain help
- Together AI, Bytescale, Pinecone, and Clerk for sponsoring
These are some future tasks that I have planned. Contributions are welcome!
- [ ] Add a trash icon for folks to delete PDFs from the dashboard and implement delete functionality
- [ ] Try different embedding models like UAE-large-v1 to see if it improves accuracy
- [ ] Explore best practices for auto scrolling based on other chat apps like chatGPT
- [ ] Do some prompt engineering for Mixtral to make replies as good as possible
- [ ] Protect API routes by making sure users are signed in before executing chats
- [ ] Run an initial benchmark on how accurate chunking / retrieval are
- [ ] Research best practices for chunking and retrieval and play around with them – ideally run benchmarks
- [ ] Try out Langsmith for more observability into how the RAG app runs
- [ ] Add demo video to the homepage to demonstrate functionality more easily
- [ ] Upgrade to Next.js 14 and fix any issues with that
- [ ] Implement sources like perplexity to be clickable with more info
- [ ] Add analytics to track the number of chats & errors
- [ ] Make some changes to the default tailwind
prose
to decrease padding - [ ] Add an initial message with sample questions or just add them as bubbles on the page
- [ ] Add an option to get answers as markdown or in regular paragraphs
- [ ] Implement something like SWR to automatically revalidate data
- [ ] Save chats for each user to get back to later in the postgres DB
- [ ] Bring up a message to direct folks to compress PDFs if they're beyond 10MB
- [ ] Use a self-designed custom uploader
- [ ] Use a session tracking tool to better understand how folks are using the site
- [ ] Add better error handling overall with appropriate toasts when actions fail
- [ ] Add support for images in PDFs with something like Nougat
For Tasks:
Click tags to check more tools for each tasksFor Jobs:
Alternative AI tools for pdftochat
Similar Open Source Tools
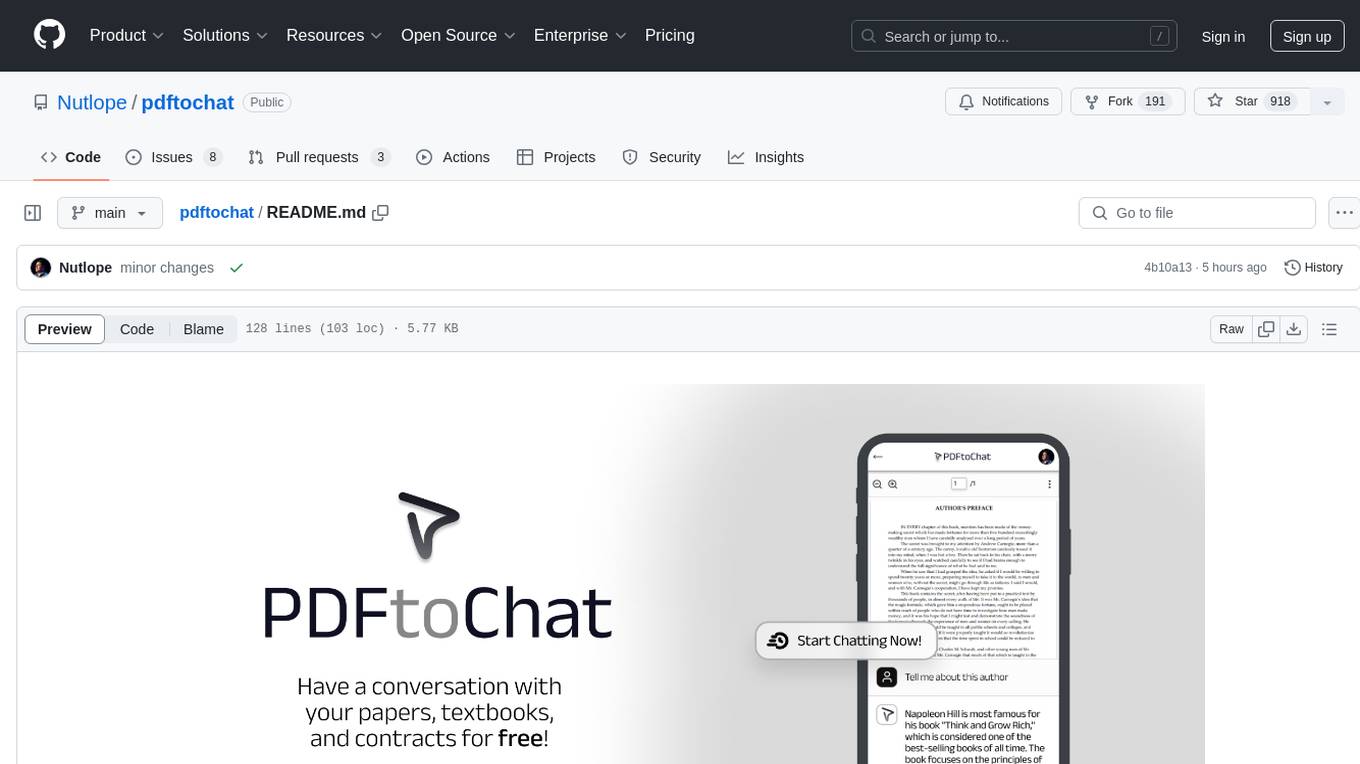
pdftochat
PDFToChat is a tool that allows users to chat with their PDF documents in seconds. It is powered by Together AI and Pinecone, utilizing a tech stack including Next.js, Mixtral, M2 Bert, LangChain.js, MongoDB Atlas, Bytescale, Vercel, Clerk, and Tailwind CSS. Users can deploy the tool to Vercel or any other host by setting up Together.ai, MongoDB Atlas database, Bytescale, Clerk, and Vercel. The tool enables users to interact with PDFs through chat, with future tasks including adding features like trash icon for deleting PDFs, exploring different embedding models, implementing auto scrolling, improving replies, benchmarking accuracy, researching chunking and retrieval best practices, adding demo video, upgrading to Next.js 14, adding analytics, customizing tailwind prose, saving chats in postgres DB, compressing large PDFs, implementing custom uploader, session tracking, error handling, and support for images in PDFs.
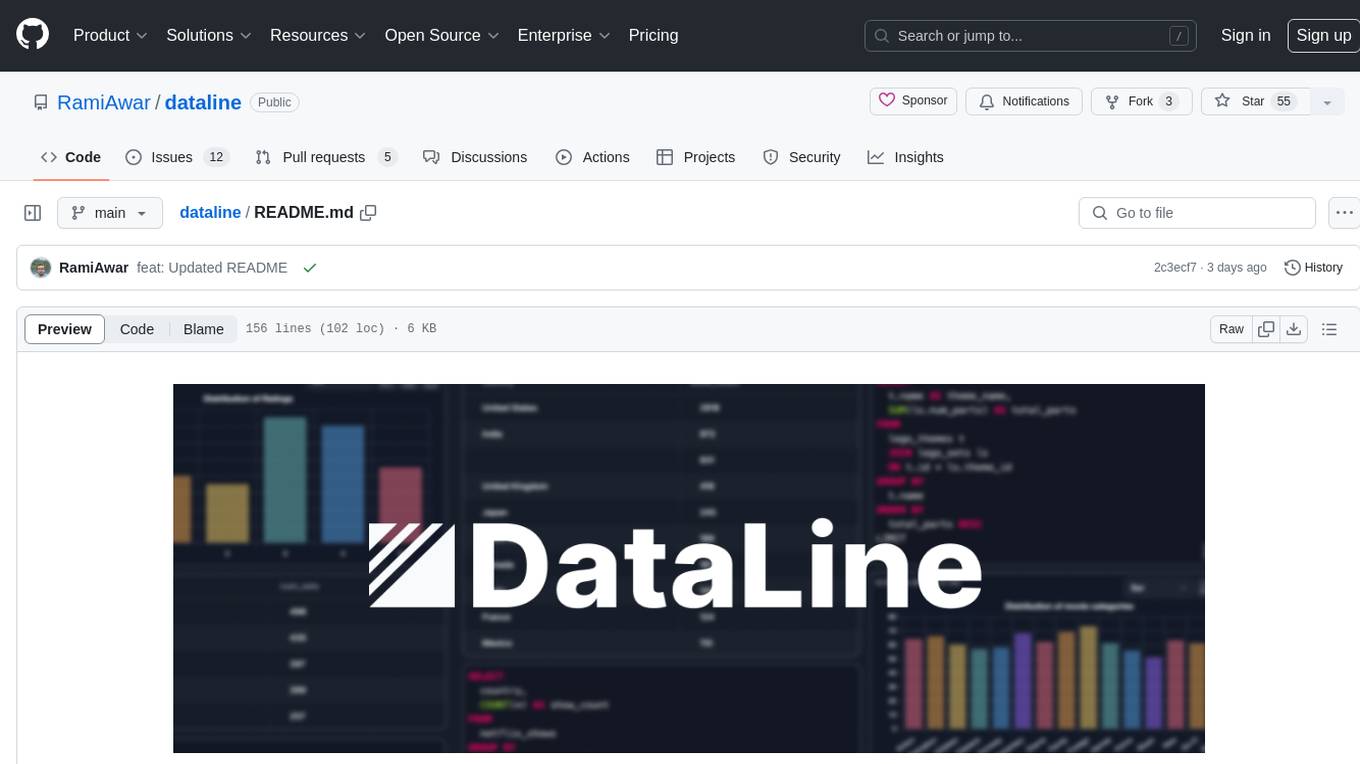
dataline
DataLine is an AI-driven data analysis and visualization tool designed for technical and non-technical users to explore data quickly. It offers privacy-focused data storage on the user's device, supports various data sources, generates charts, executes queries, and facilitates report building. The tool aims to speed up data analysis tasks for businesses and individuals by providing a user-friendly interface and natural language querying capabilities.
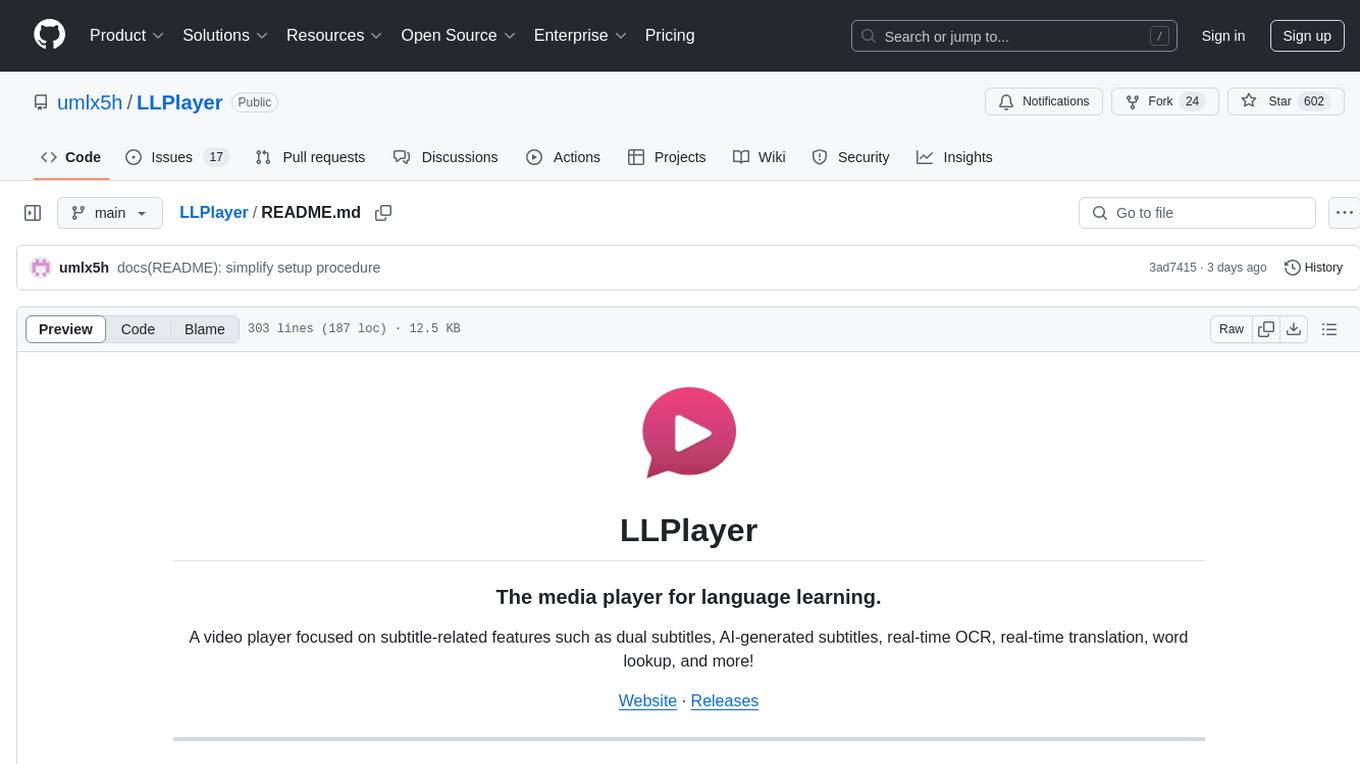
LLPlayer
LLPlayer is a specialized media player designed for language learning, offering unique features such as dual subtitles, AI-generated subtitles, real-time OCR, real-time translation, word lookup, and more. It supports multiple languages, online video playback, customizable settings, and integration with browser extensions. Written in C#/WPF, LLPlayer is free, open-source, and aims to enhance the language learning experience through innovative functionalities.
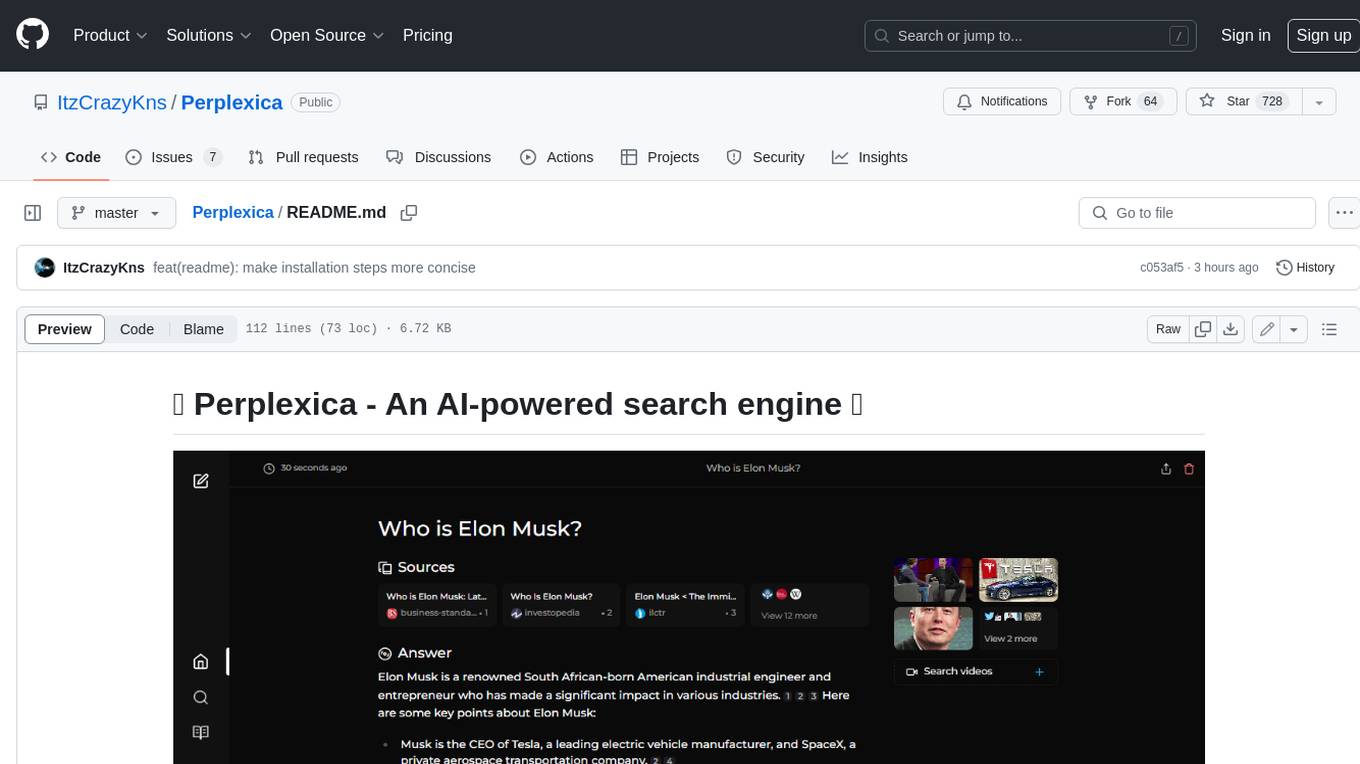
Perplexica
Perplexica is an open-source AI-powered search engine that utilizes advanced machine learning algorithms to provide clear answers with sources cited. It offers various modes like Copilot Mode, Normal Mode, and Focus Modes for specific types of questions. Perplexica ensures up-to-date information by using SearxNG metasearch engine. It also features image and video search capabilities and upcoming features include finalizing Copilot Mode and adding Discover and History Saving features.
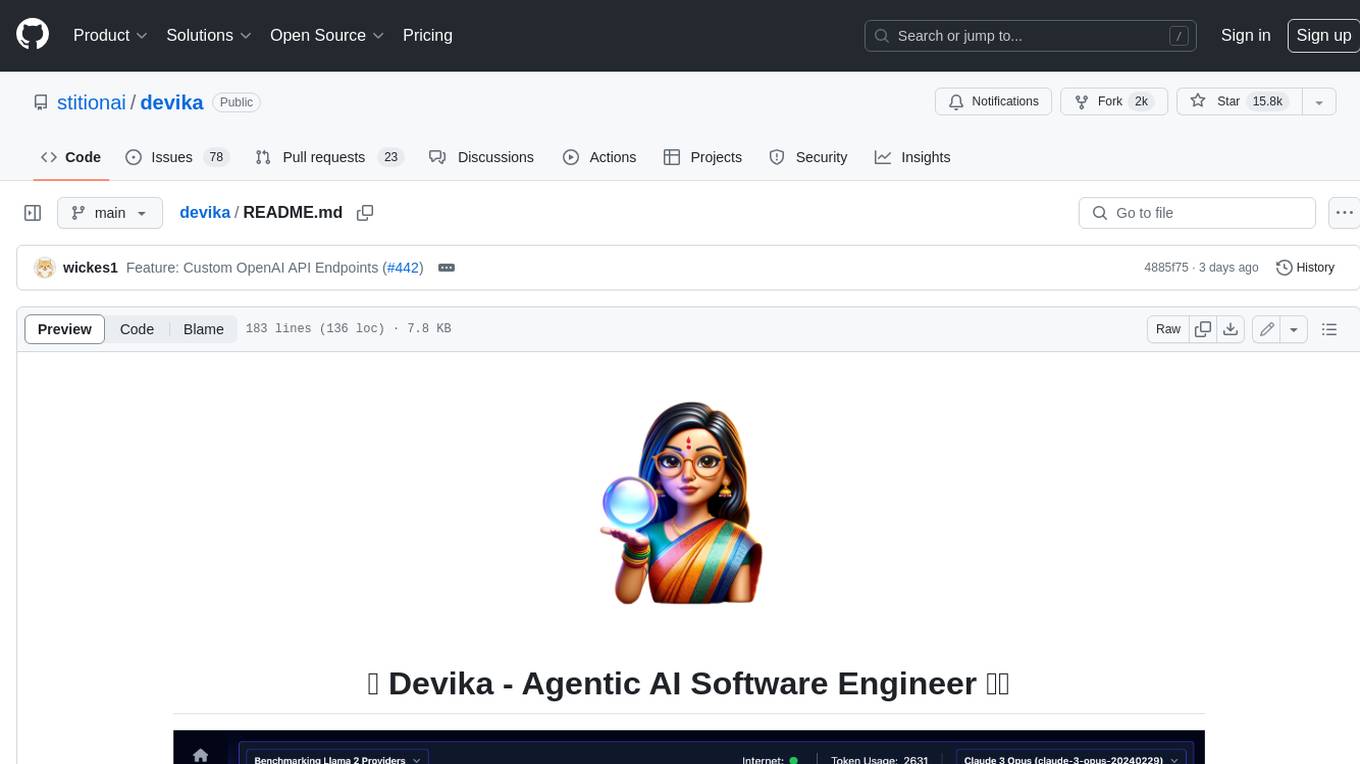
devika
Devika is an advanced AI software engineer that can understand high-level human instructions, break them down into steps, research relevant information, and write code to achieve the given objective. Devika utilizes large language models, planning and reasoning algorithms, and web browsing abilities to intelligently develop software. Devika aims to revolutionize the way we build software by providing an AI pair programmer who can take on complex coding tasks with minimal human guidance. Whether you need to create a new feature, fix a bug, or develop an entire project from scratch, Devika is here to assist you.
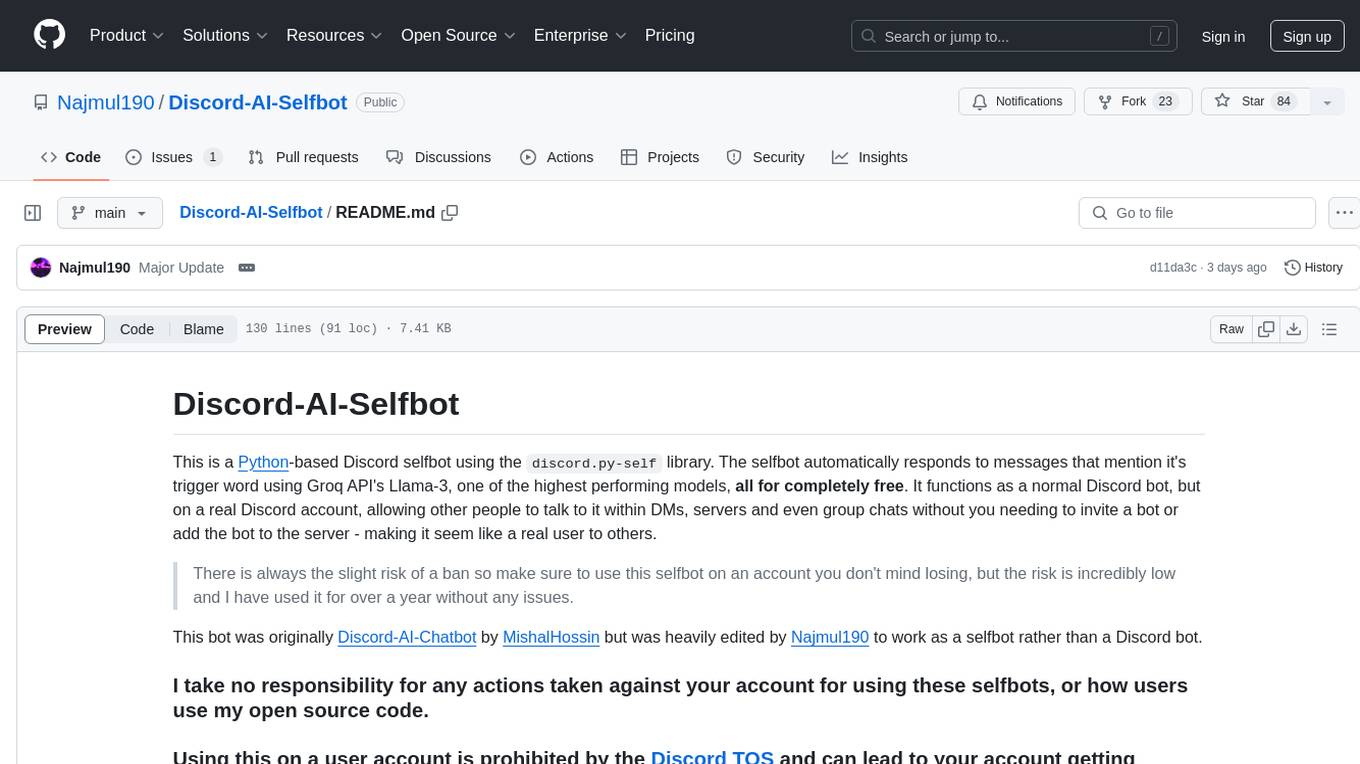
Discord-AI-Selfbot
Discord-AI-Selfbot is a Python-based Discord selfbot that uses the `discord.py-self` library to automatically respond to messages mentioning its trigger word using Groq API's Llama-3 model. It functions as a normal Discord bot on a real Discord account, enabling interactions in DMs, servers, and group chats without needing to invite a bot. The selfbot comes with features like custom AI instructions, free LLM model usage, mention and reply recognition, message handling, channel-specific responses, and a psychoanalysis command to analyze user messages for insights on personality.
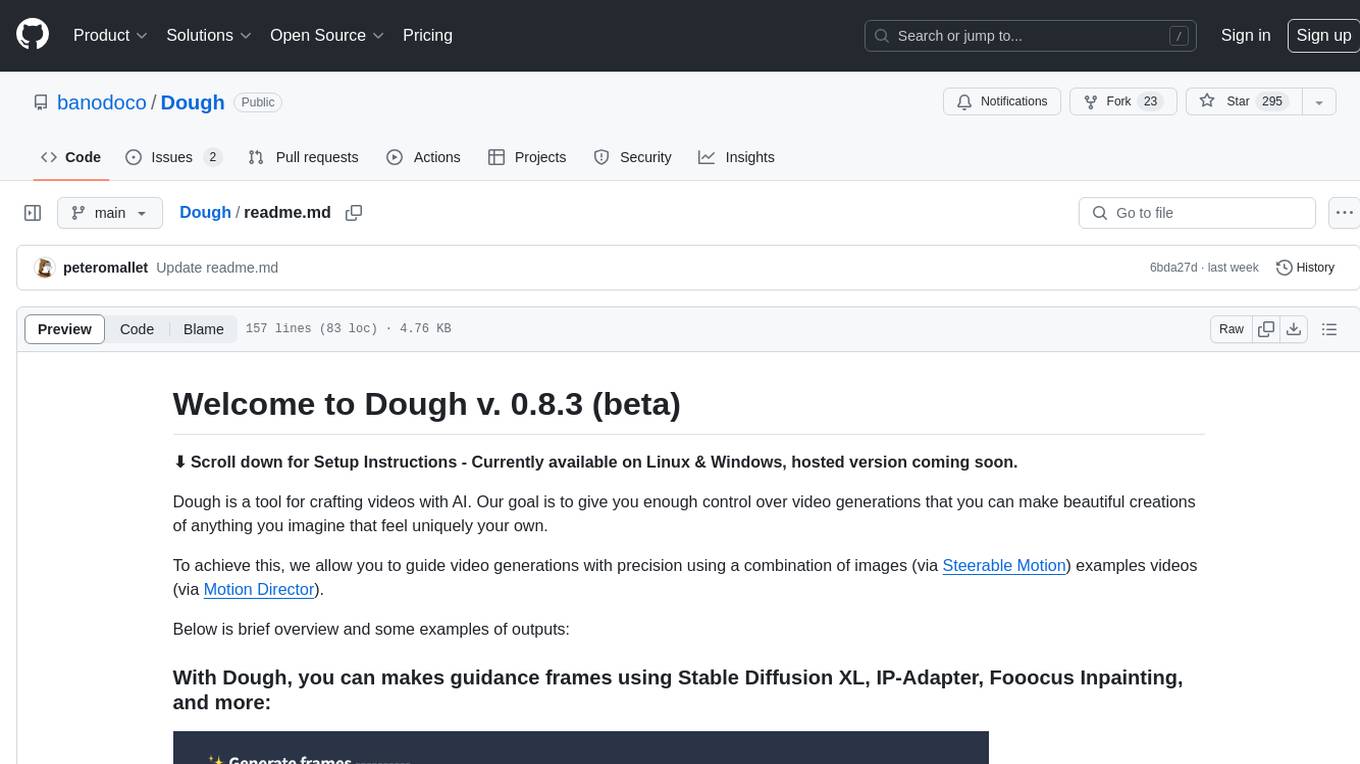
Dough
Dough is a tool for crafting videos with AI, allowing users to guide video generations with precision using images and example videos. Users can create guidance frames, assemble shots, and animate them by defining parameters and selecting guidance videos. The tool aims to help users make beautiful and unique video creations, providing control over the generation process. Setup instructions are available for Linux and Windows platforms, with detailed steps for installation and running the app.
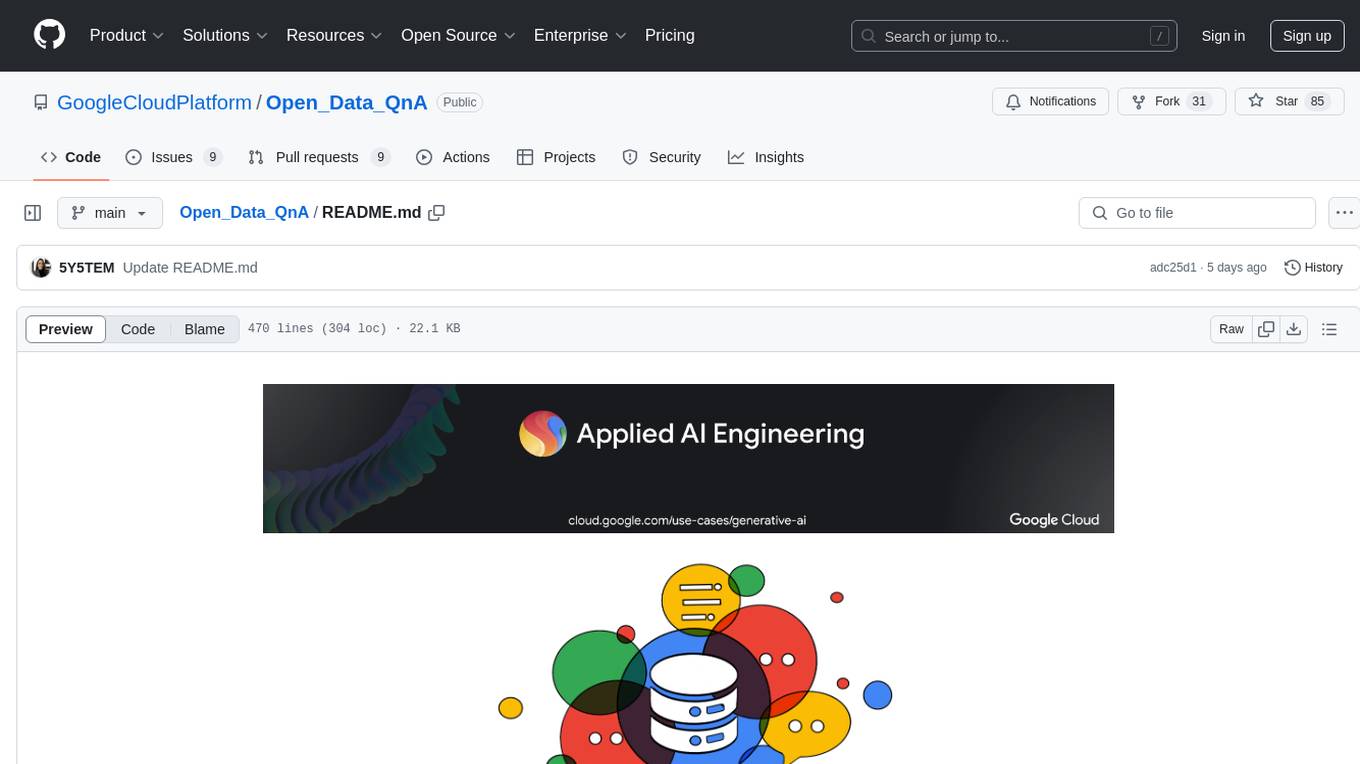
Open_Data_QnA
Open Data QnA is a Python library that allows users to interact with their PostgreSQL or BigQuery databases in a conversational manner, without needing to write SQL queries. The library leverages Large Language Models (LLMs) to bridge the gap between human language and database queries, enabling users to ask questions in natural language and receive informative responses. It offers features such as conversational querying with multiturn support, table grouping, multi schema/dataset support, SQL generation, query refinement, natural language responses, visualizations, and extensibility. The library is built on a modular design and supports various components like Database Connectors, Vector Stores, and Agents for SQL generation, validation, debugging, descriptions, embeddings, responses, and visualizations.
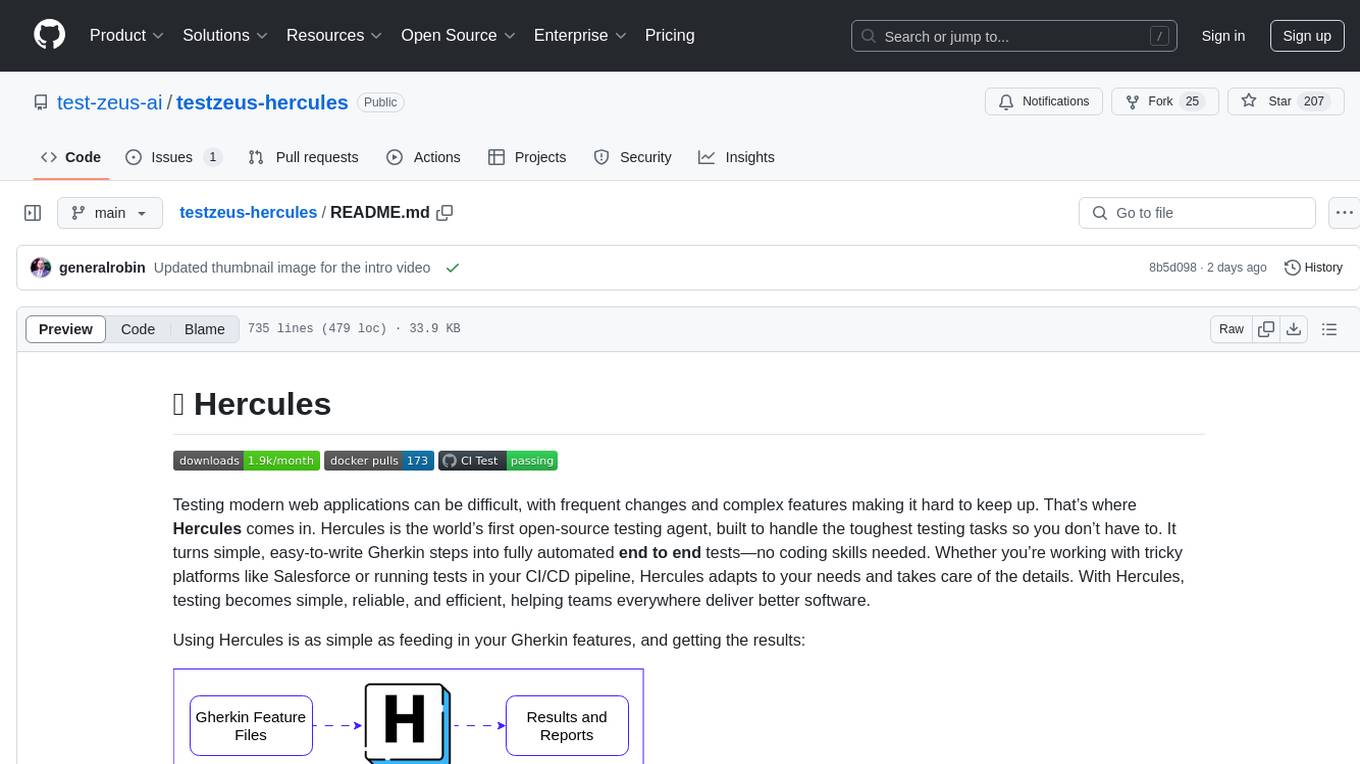
testzeus-hercules
Hercules is the world’s first open-source testing agent designed to handle the toughest testing tasks for modern web applications. It turns simple Gherkin steps into fully automated end-to-end tests, making testing simple, reliable, and efficient. Hercules adapts to various platforms like Salesforce and is suitable for CI/CD pipelines. It aims to democratize and disrupt test automation, making top-tier testing accessible to everyone. The tool is transparent, reliable, and community-driven, empowering teams to deliver better software. Hercules offers multiple ways to get started, including using PyPI package, Docker, or building and running from source code. It supports various AI models, provides detailed installation and usage instructions, and integrates with Nuclei for security testing and WCAG for accessibility testing. The tool is production-ready, open core, and open source, with plans for enhanced LLM support, advanced tooling, improved DOM distillation, community contributions, extensive documentation, and a bounty program.
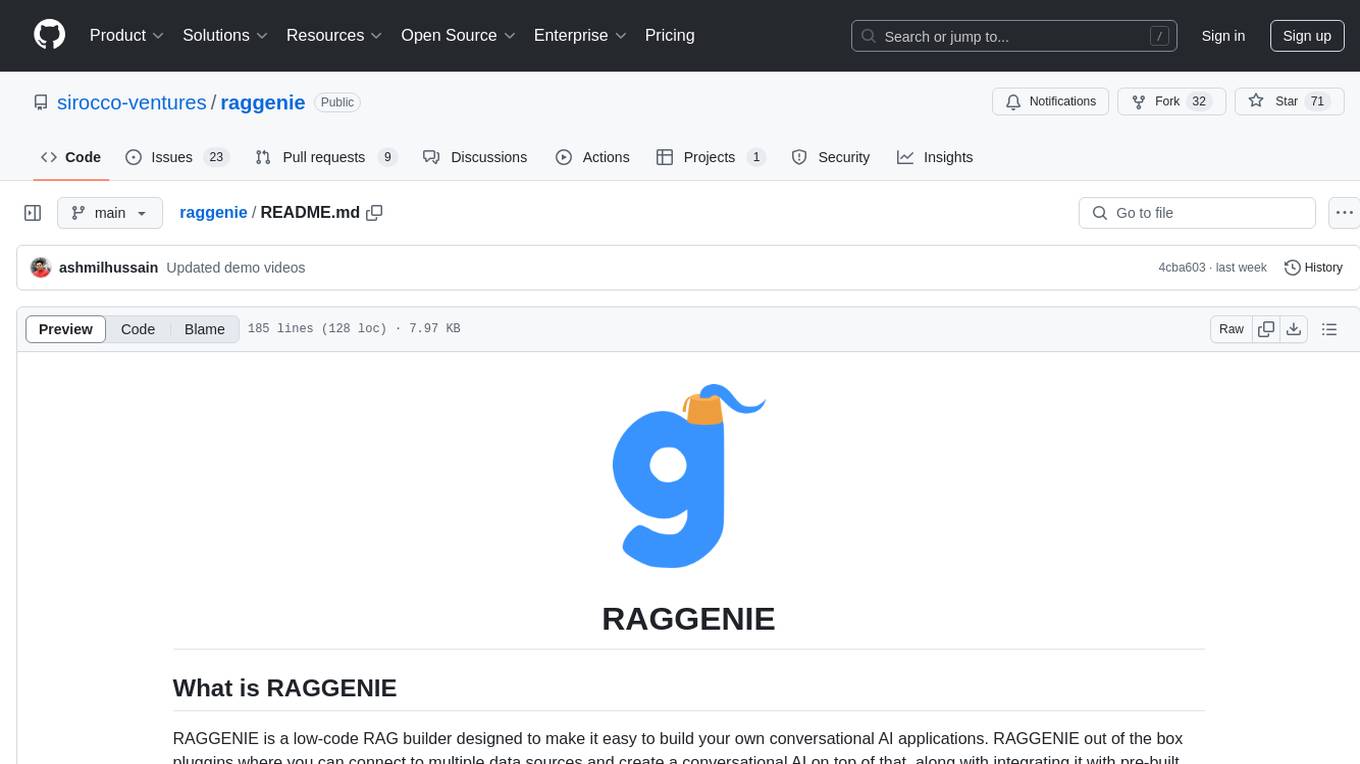
raggenie
RAGGENIE is a low-code RAG builder tool designed to simplify the creation of conversational AI applications. It offers out-of-the-box plugins for connecting to various data sources and building conversational AI on top of them, including integration with pre-built agents for actions. The tool is open-source under the MIT license, with a current focus on making it easy to build RAG applications and future plans for maintenance, monitoring, and transitioning applications from pilots to production.
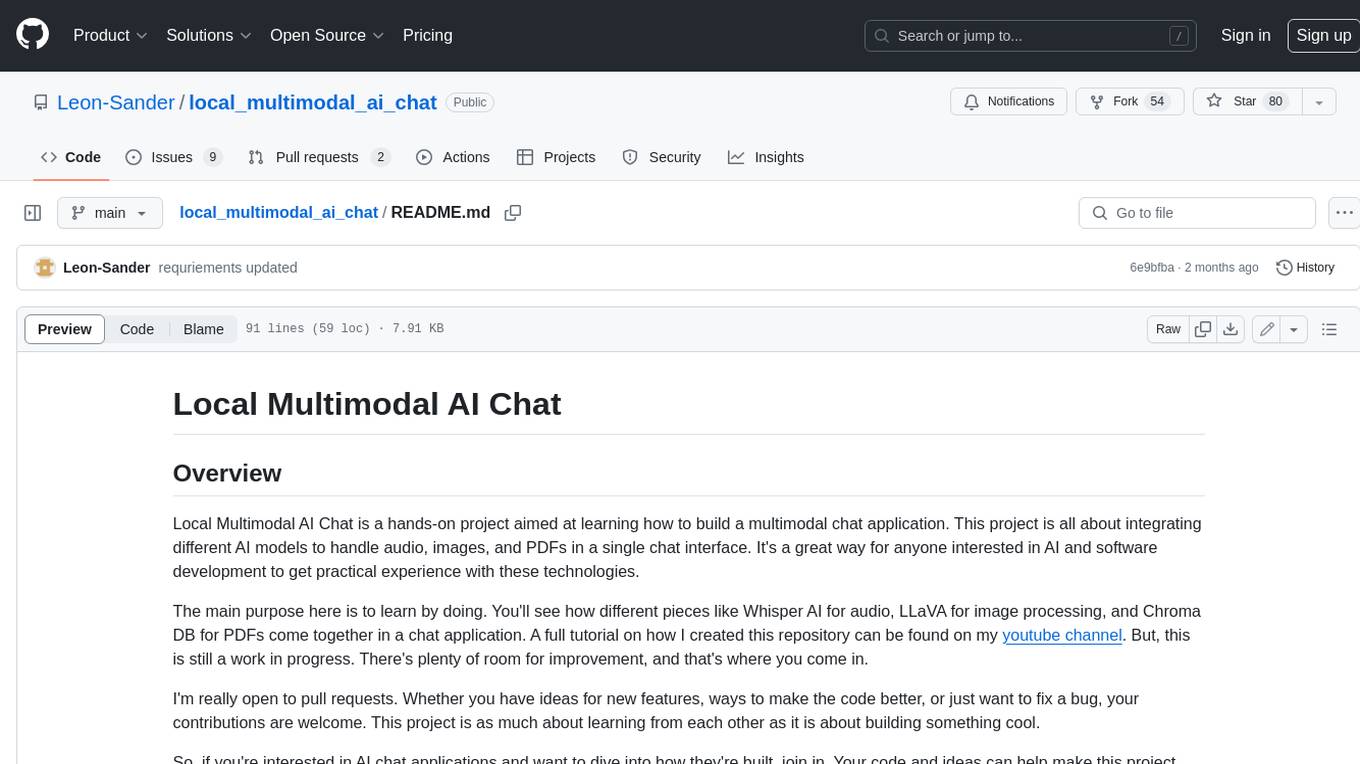
local_multimodal_ai_chat
Local Multimodal AI Chat is a hands-on project that teaches you how to build a multimodal chat application. It integrates different AI models to handle audio, images, and PDFs in a single chat interface. This project is perfect for anyone interested in AI and software development who wants to gain practical experience with these technologies.
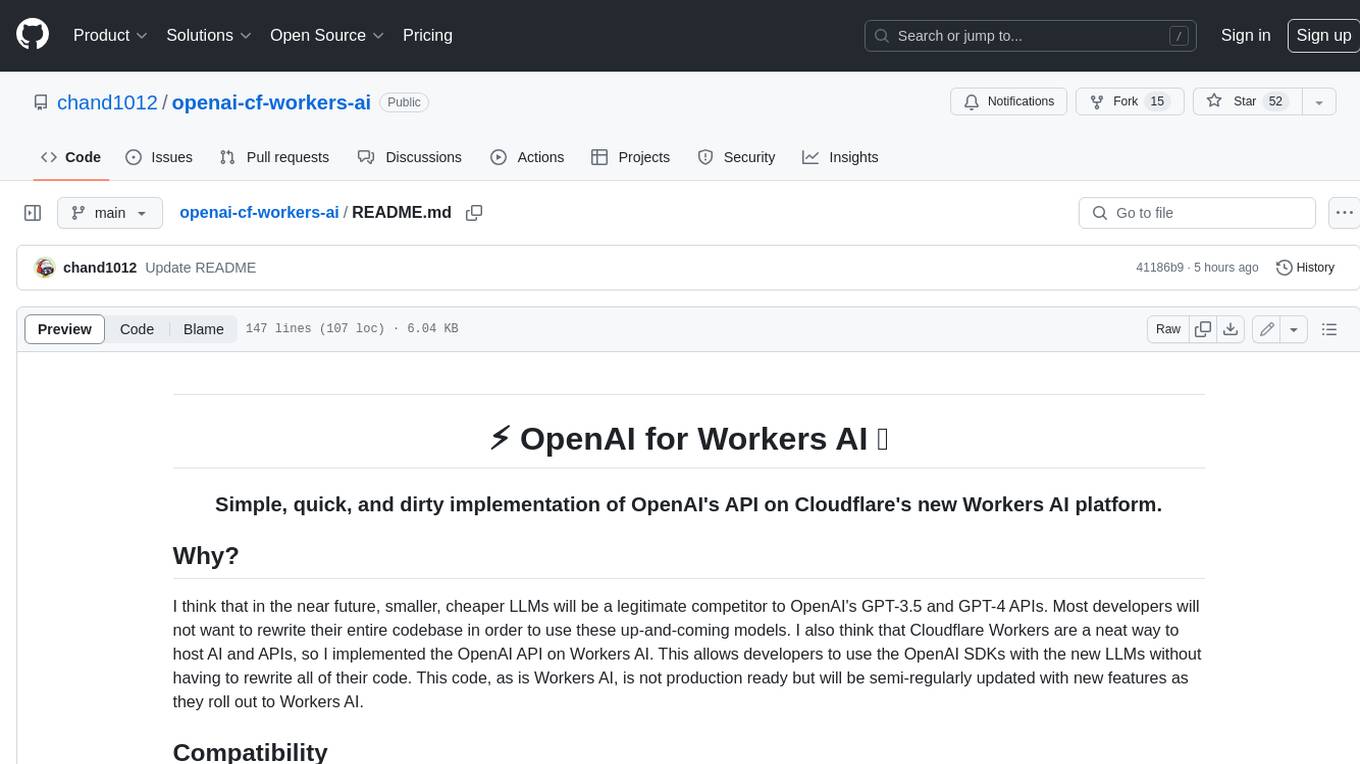
openai-cf-workers-ai
OpenAI for Workers AI is a simple, quick, and dirty implementation of OpenAI's API on Cloudflare's new Workers AI platform. It allows developers to use the OpenAI SDKs with the new LLMs without having to rewrite all of their code. The API currently supports completions, chat completions, audio transcription, embeddings, audio translation, and image generation. It is not production ready but will be semi-regularly updated with new features as they roll out to Workers AI.
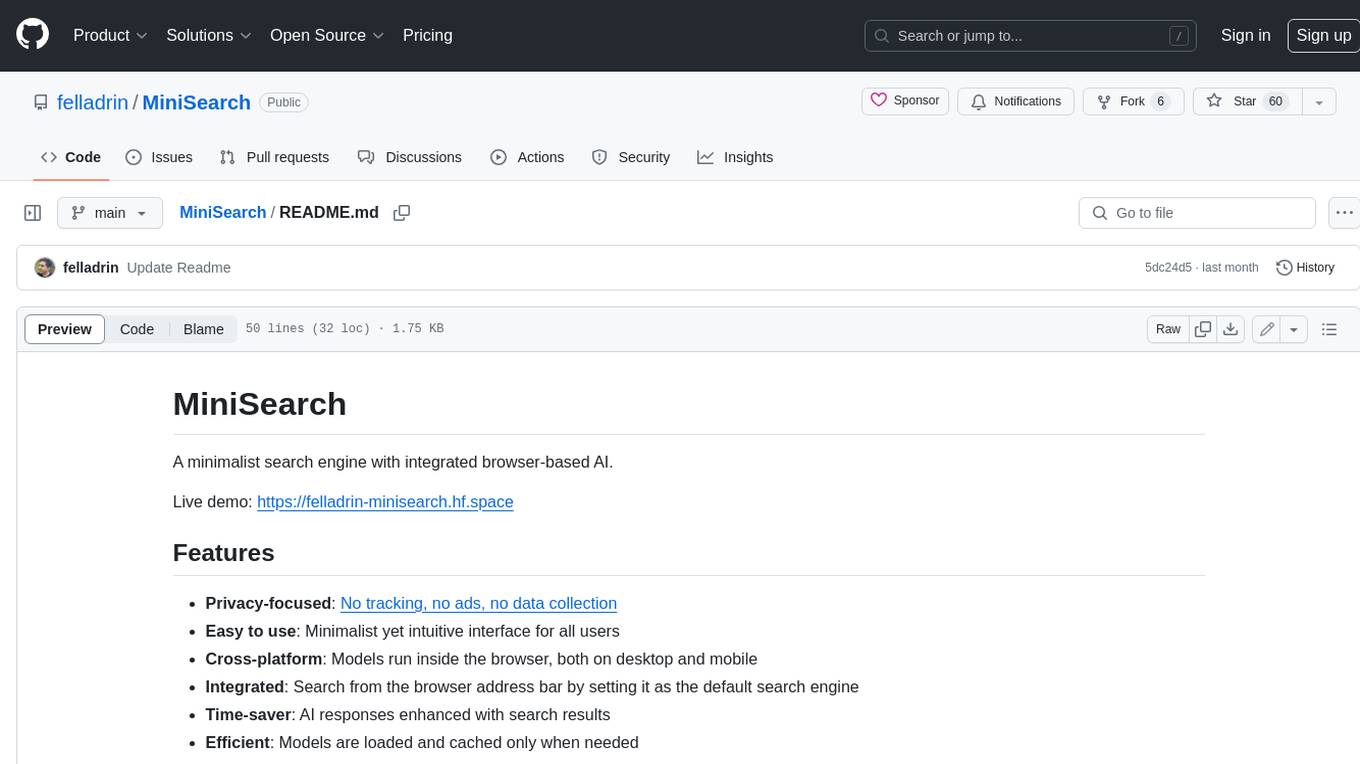
MiniSearch
MiniSearch is a minimalist search engine with integrated browser-based AI. It is privacy-focused, easy to use, cross-platform, integrated, time-saving, efficient, optimized, and open-source. MiniSearch can be used for a variety of tasks, including searching the web, finding files on your computer, and getting answers to questions. It is a great tool for anyone who wants a fast, private, and easy-to-use search engine.
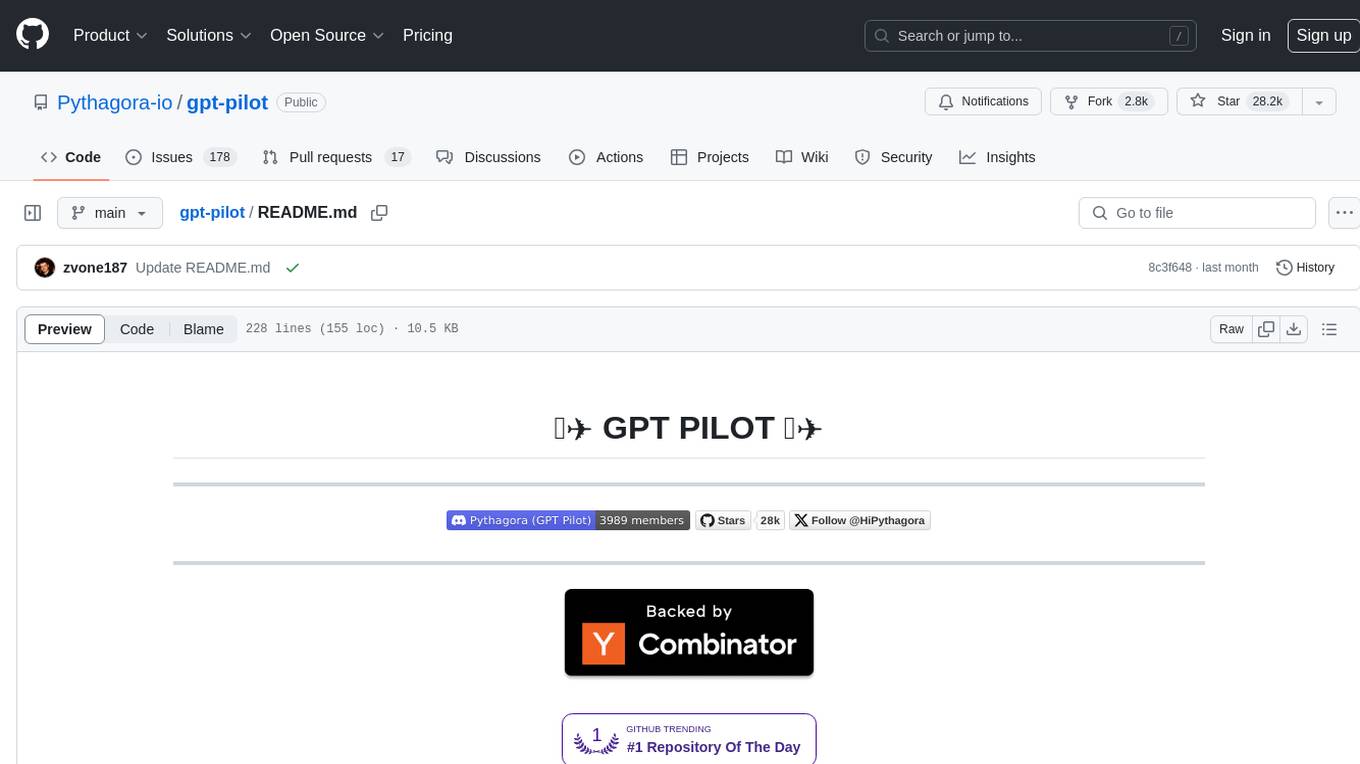
gpt-pilot
GPT Pilot is a core technology for the Pythagora VS Code extension, aiming to provide the first real AI developer companion. It goes beyond autocomplete, helping with writing full features, debugging, issue discussions, and reviews. The tool utilizes LLMs to generate production-ready apps, with developers overseeing the implementation. GPT Pilot works step by step like a developer, debugging issues as they arise. It can work at any scale, filtering out code to show only relevant parts to the AI during tasks. Contributions are welcome, with debugging and telemetry being key areas of focus for improvement.
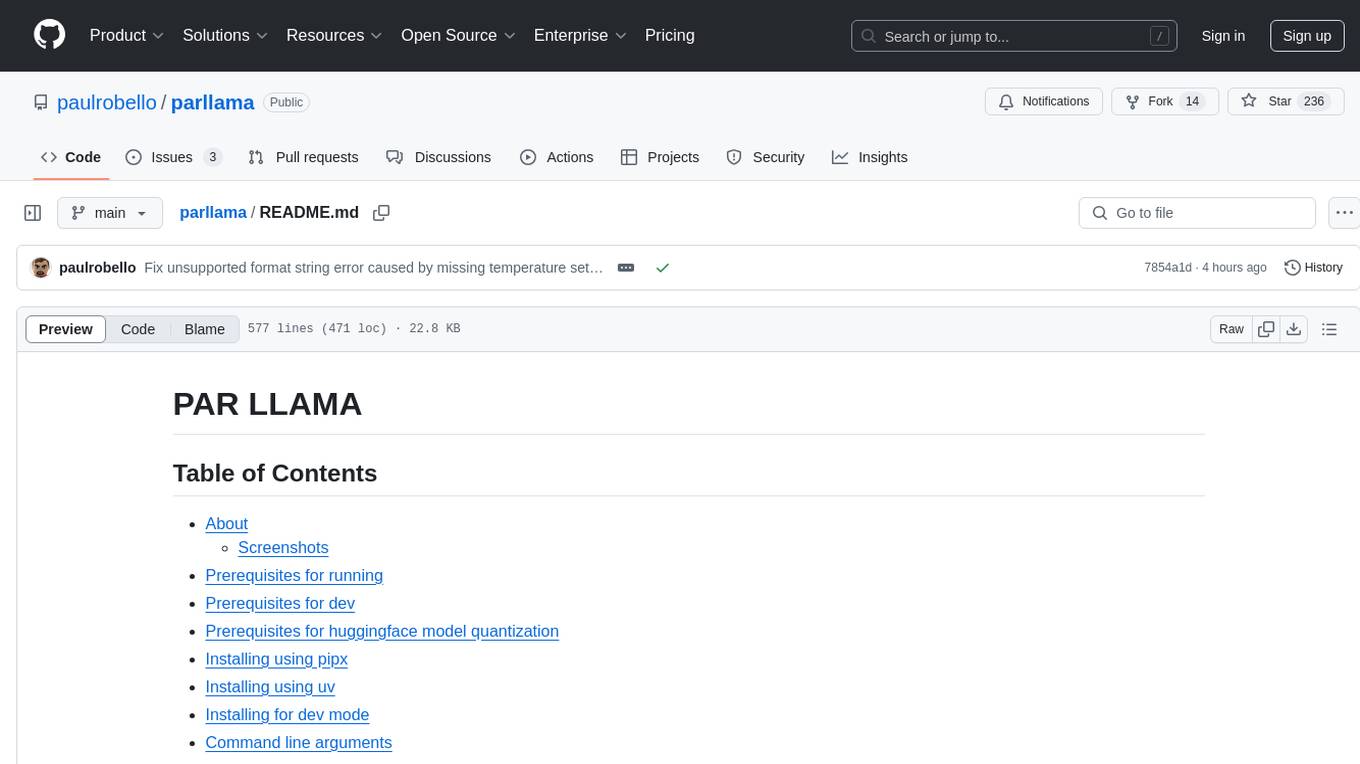
parllama
PAR LLAMA is a Text UI application for managing and using LLMs, designed with Textual and Rich and PAR AI Core. It runs on major OS's including Windows, Windows WSL, Mac, and Linux. Supports Dark and Light mode, custom themes, and various workflows like Ollama chat, image chat, and OpenAI provider chat. Offers features like custom prompts, themes, environment variables configuration, and remote instance connection. Suitable for managing and using LLMs efficiently.
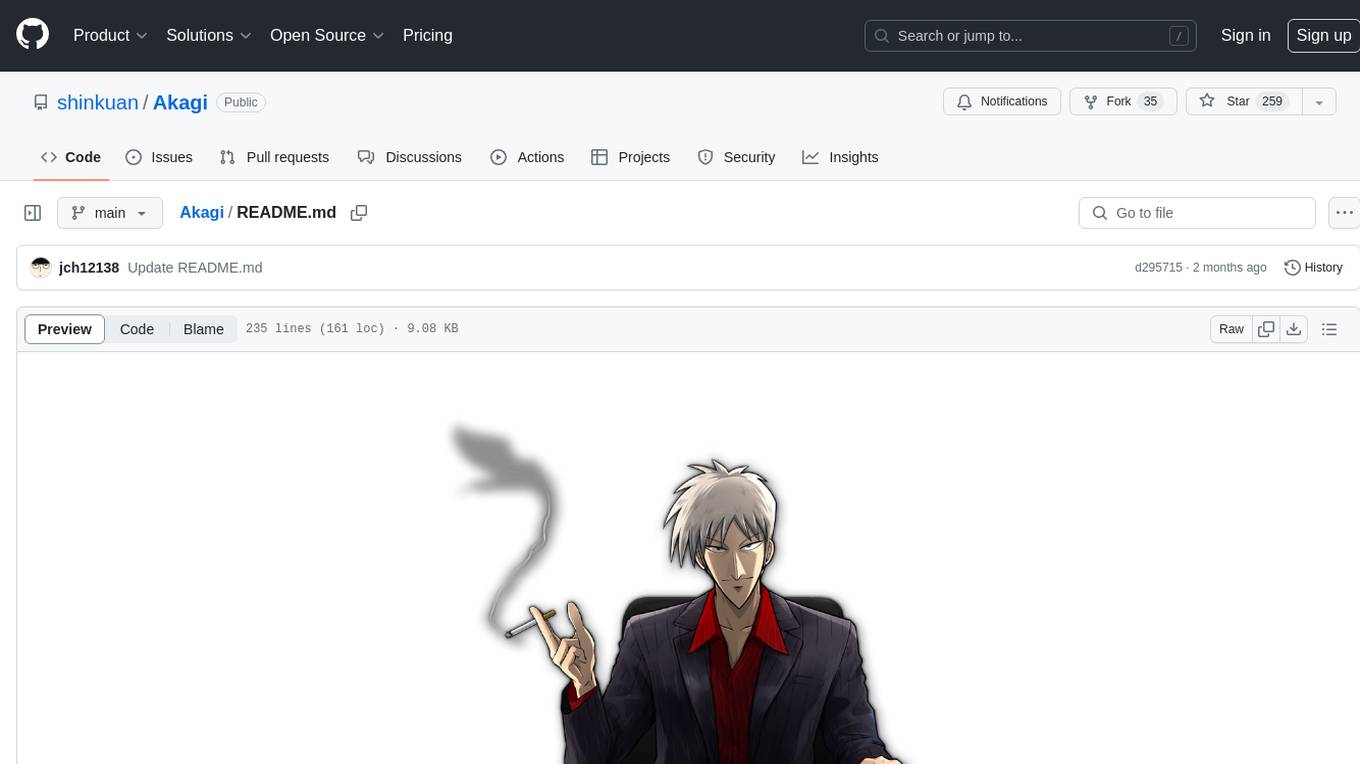
Akagi
Akagi is a project designed to help users understand and improve their performance in Majsoul game matches in real-time. It provides educational insights and tools for analyzing gameplay. Users can install Akagi on Windows or Mac systems and follow the setup instructions to enhance their gaming experience. The project aims to offer features like Autoplay, Auto Ron, and integration with MajsoulUnlocker. It also focuses on enhancing user safety by providing guidelines to minimize the risk of account suspension. Akagi is a tool that combines MITM interception, AI decision-making, and user interaction to optimize gameplay strategies and performance.
For similar tasks
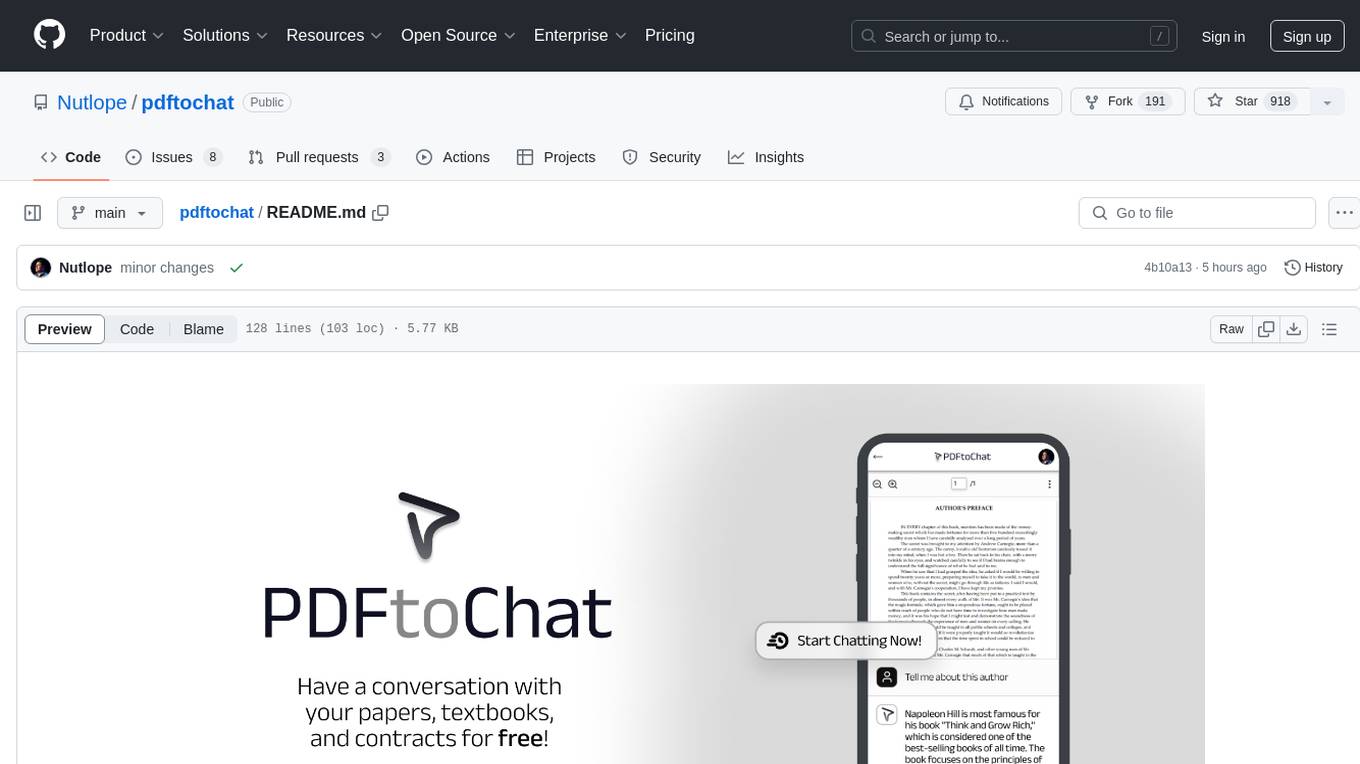
pdftochat
PDFToChat is a tool that allows users to chat with their PDF documents in seconds. It is powered by Together AI and Pinecone, utilizing a tech stack including Next.js, Mixtral, M2 Bert, LangChain.js, MongoDB Atlas, Bytescale, Vercel, Clerk, and Tailwind CSS. Users can deploy the tool to Vercel or any other host by setting up Together.ai, MongoDB Atlas database, Bytescale, Clerk, and Vercel. The tool enables users to interact with PDFs through chat, with future tasks including adding features like trash icon for deleting PDFs, exploring different embedding models, implementing auto scrolling, improving replies, benchmarking accuracy, researching chunking and retrieval best practices, adding demo video, upgrading to Next.js 14, adding analytics, customizing tailwind prose, saving chats in postgres DB, compressing large PDFs, implementing custom uploader, session tracking, error handling, and support for images in PDFs.
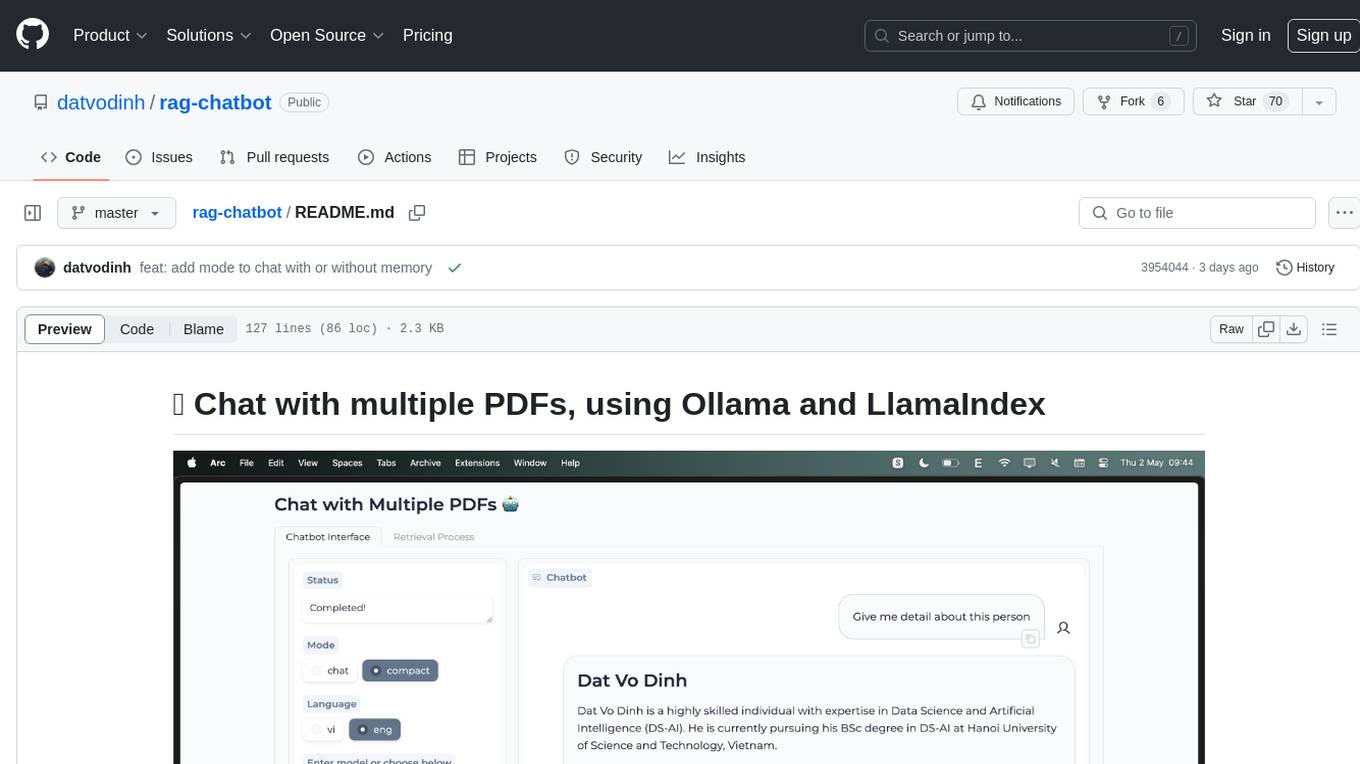
rag-chatbot
rag-chatbot is a tool that allows users to chat with multiple PDFs using Ollama and LlamaIndex. It provides an easy setup for running on local machines or Kaggle notebooks. Users can leverage models from Huggingface and Ollama, process multiple PDF inputs, and chat in multiple languages. The tool offers a simple UI with Gradio, supporting chat with history and QA modes. Setup instructions are provided for both Kaggle and local environments, including installation steps for Docker, Ollama, Ngrok, and the rag_chatbot package. Users can run the tool locally and access it via a web interface. Future enhancements include adding evaluation, better embedding models, knowledge graph support, improved document processing, MLX model integration, and Corrective RAG.
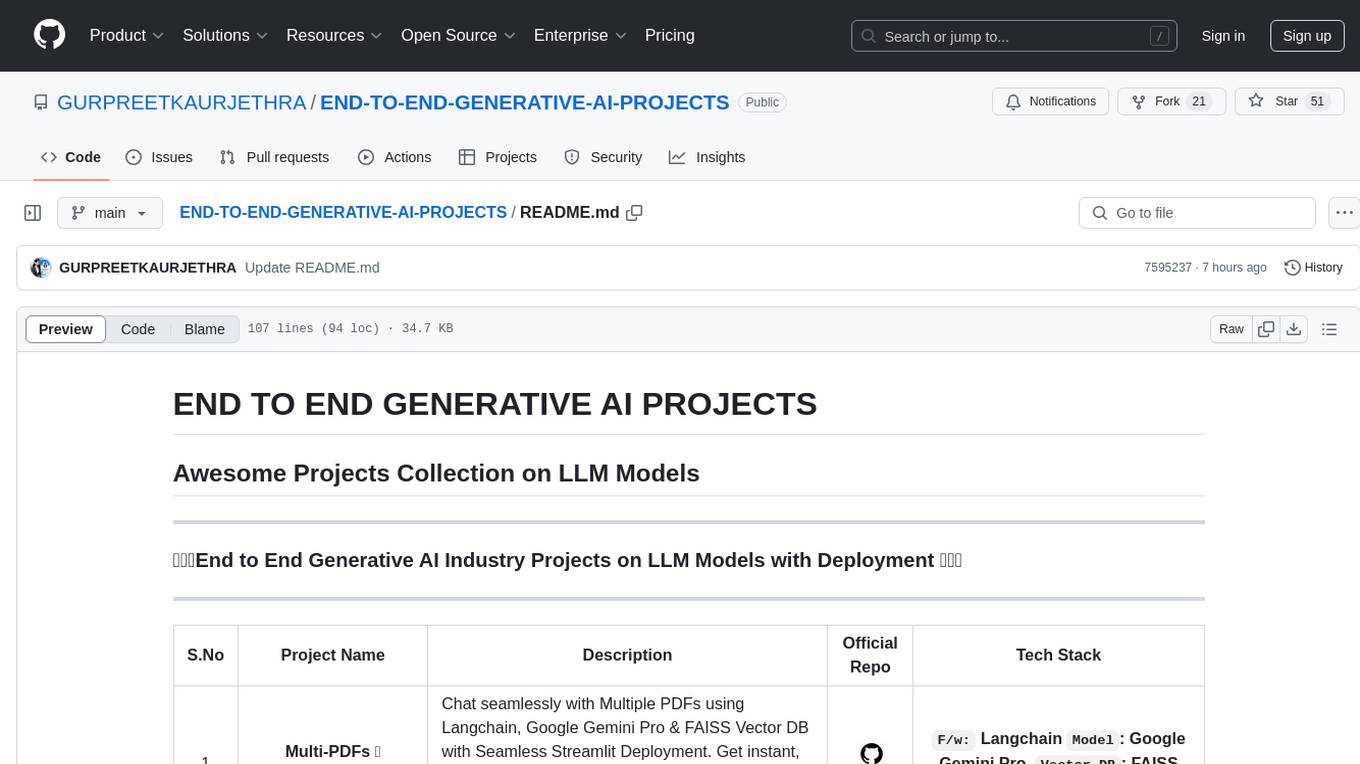
END-TO-END-GENERATIVE-AI-PROJECTS
The 'END TO END GENERATIVE AI PROJECTS' repository is a collection of awesome industry projects utilizing Large Language Models (LLM) for various tasks such as chat applications with PDFs, image to speech generation, video transcribing and summarizing, resume tracking, text to SQL conversion, invoice extraction, medical chatbot, financial stock analysis, and more. The projects showcase the deployment of LLM models like Google Gemini Pro, HuggingFace Models, OpenAI GPT, and technologies such as Langchain, Streamlit, LLaMA2, LLaMAindex, and more. The repository aims to provide end-to-end solutions for different AI applications.
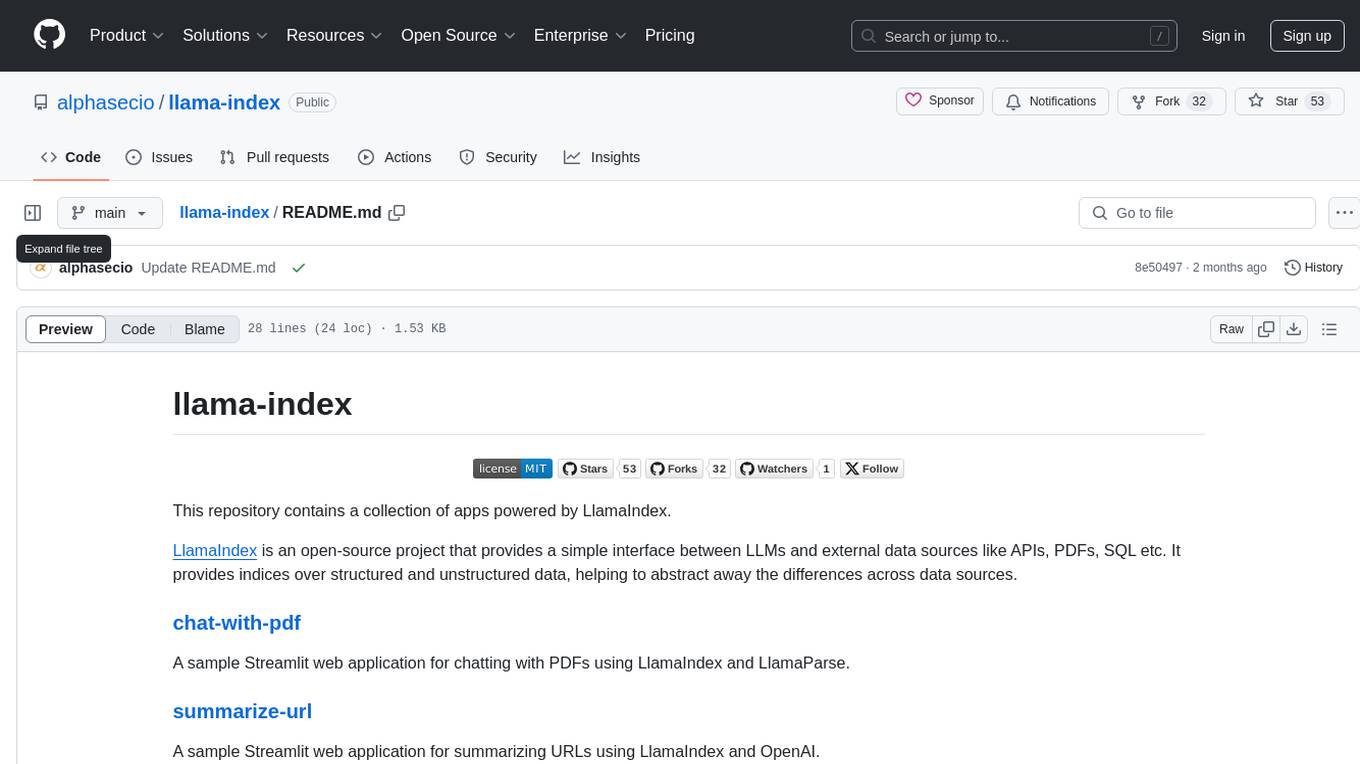
llama-index
This repository, llama-index, contains a collection of apps powered by LlamaIndex. LlamaIndex is an open-source project that provides a simple interface between LLMs and external data sources like APIs, PDFs, SQL etc. It provides indices over structured and unstructured data, helping to abstract away the differences across data sources. The repository includes apps like chat-with-pdf and summarize-url, showcasing the capabilities of LlamaIndex in interacting with PDFs and summarizing URLs.
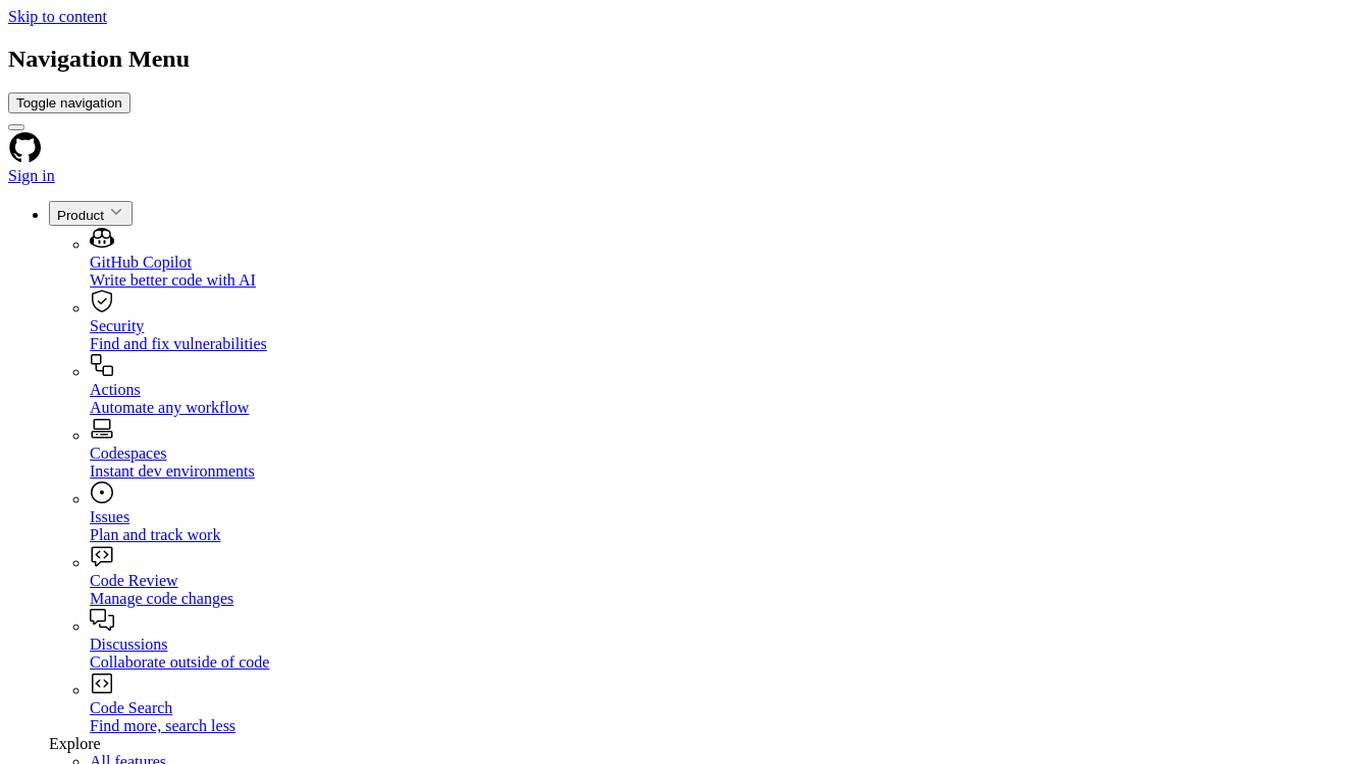
papersgpt-for-zotero
PapersGPT For Zotero is an AI plugin that enhances papers reading and research efficiency by integrating cutting-edge LLMs and offering seamless Zotero integration. Users can ask questions, extract insights, and converse with PDFs directly, making it a powerful research assistant for scholars, researchers, and anyone dealing with large amounts of text in PDF format. The plugin ensures privacy and data safety by using locally stored models and modules, with the ability to switch between different models easily. It provides a user-friendly interface for managing and chatting documents within Zotero, making research tasks more streamlined and productive.
For similar jobs
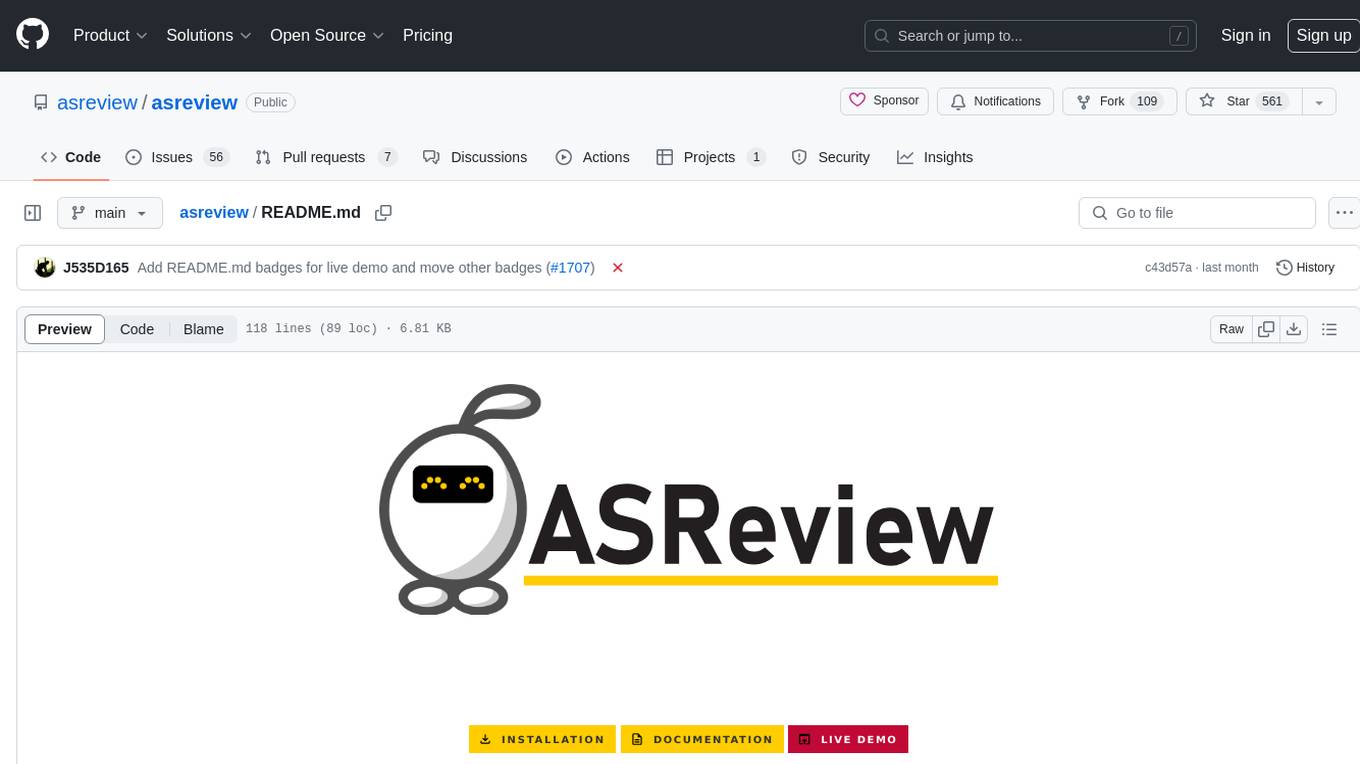
asreview
The ASReview project implements active learning for systematic reviews, utilizing AI-aided pipelines to assist in finding relevant texts for search tasks. It accelerates the screening of textual data with minimal human input, saving time and increasing output quality. The software offers three modes: Oracle for interactive screening, Exploration for teaching purposes, and Simulation for evaluating active learning models. ASReview LAB is designed to support decision-making in any discipline or industry by improving efficiency and transparency in screening large amounts of textual data.
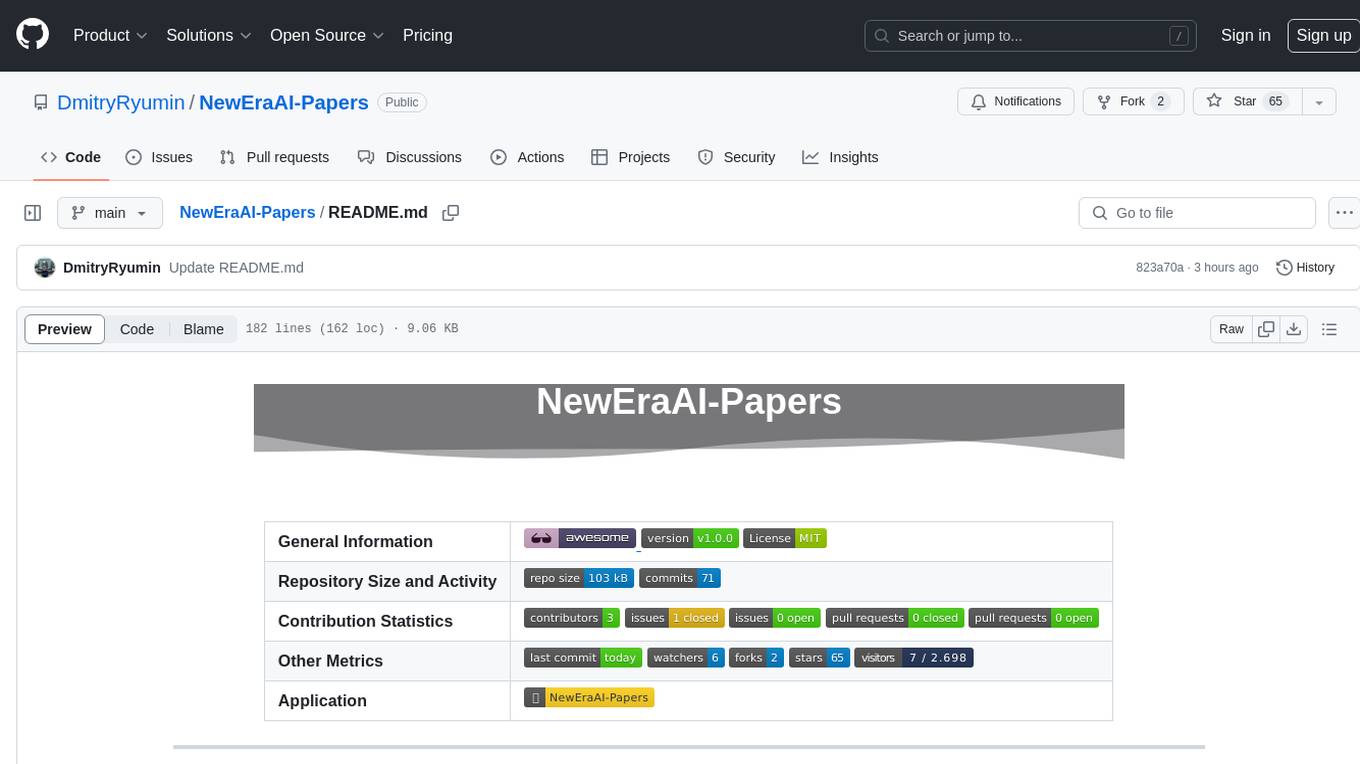
NewEraAI-Papers
The NewEraAI-Papers repository provides links to collections of influential and interesting research papers from top AI conferences, along with open-source code to promote reproducibility and provide detailed implementation insights beyond the scope of the article. Users can stay up to date with the latest advances in AI research by exploring this repository. Contributions to improve the completeness of the list are welcomed, and users can create pull requests, open issues, or contact the repository owner via email to enhance the repository further.
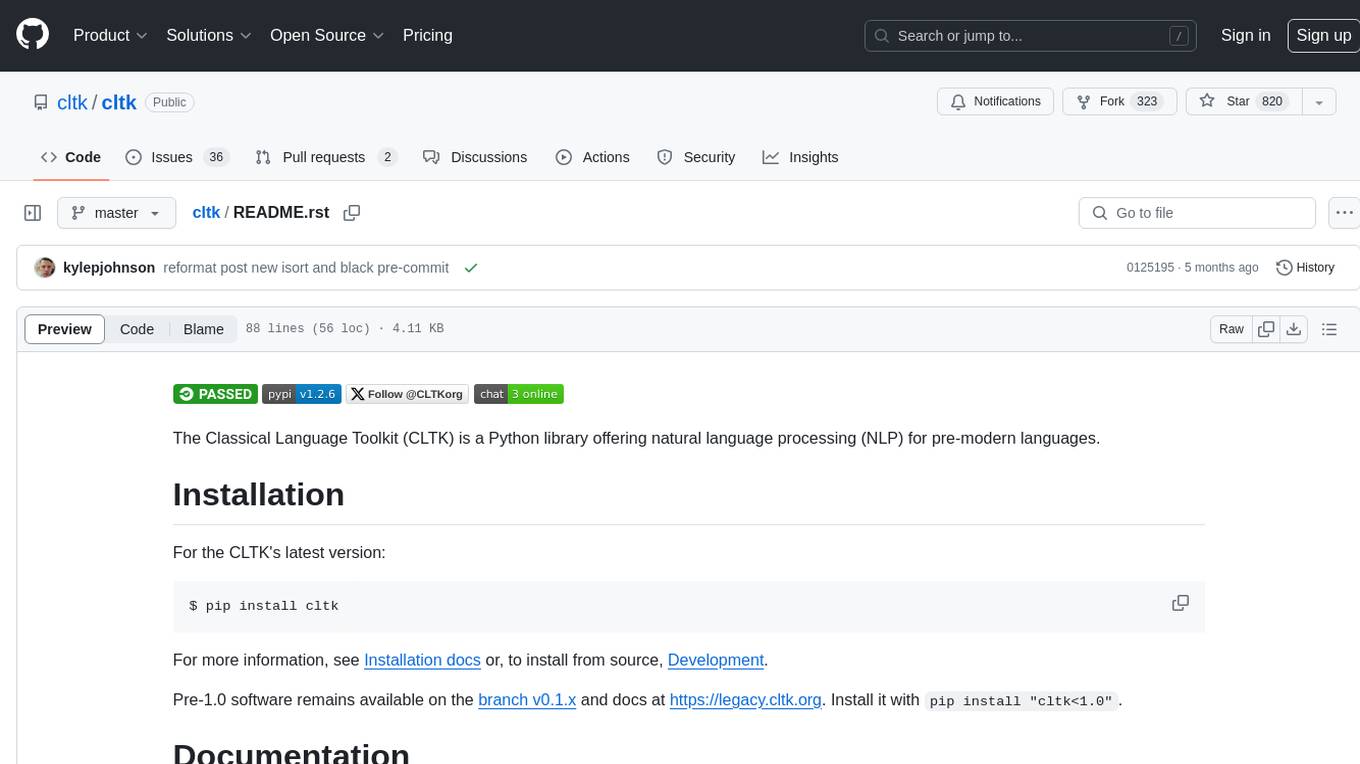
cltk
The Classical Language Toolkit (CLTK) is a Python library that provides natural language processing (NLP) capabilities for pre-modern languages. It offers a modular processing pipeline with pre-configured defaults and supports almost 20 languages. Users can install the latest version using pip and access detailed documentation on the official website. The toolkit is designed to meet the unique needs of researchers working with historical languages, filling a void in the NLP landscape that often neglects non-spoken languages and different research goals.
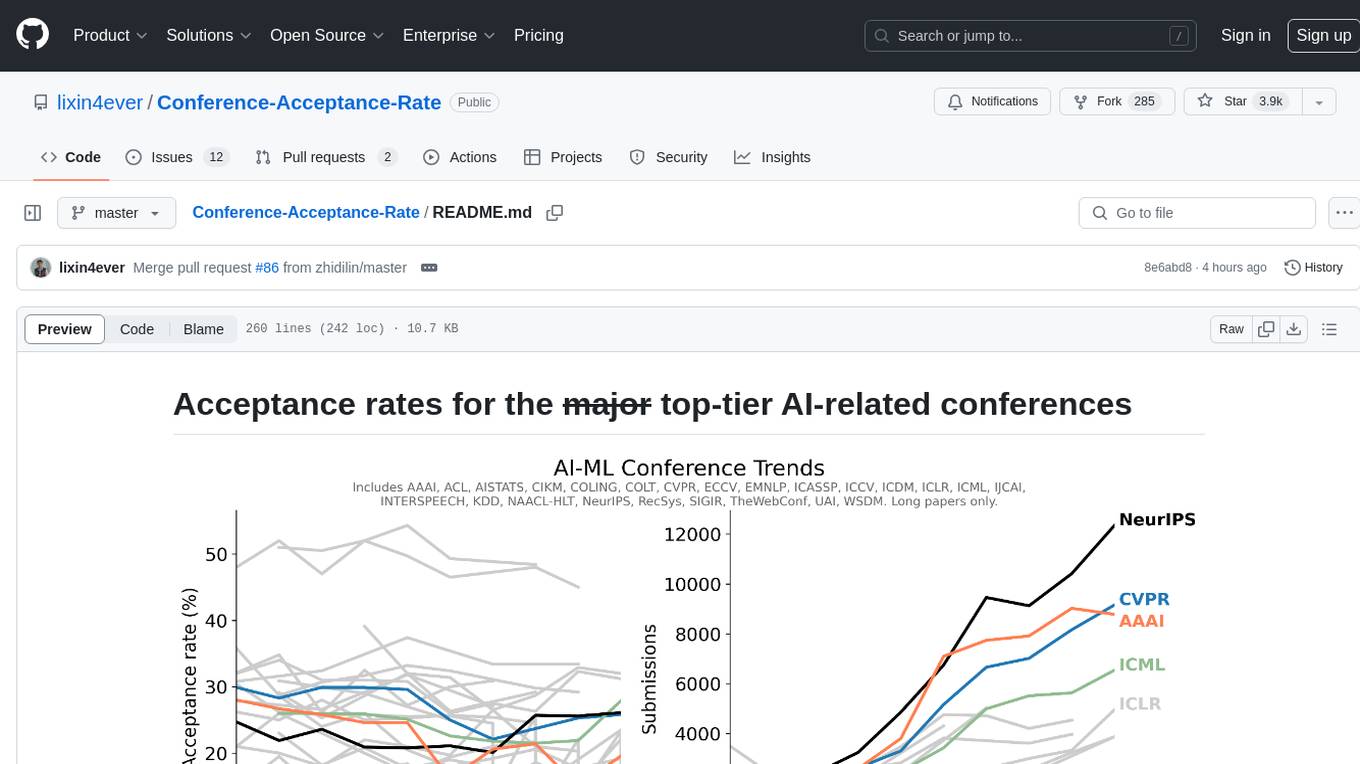
Conference-Acceptance-Rate
The 'Conference-Acceptance-Rate' repository provides acceptance rates for top-tier AI-related conferences in the fields of Natural Language Processing, Computational Linguistics, Computer Vision, Pattern Recognition, Machine Learning, Learning Theory, Artificial Intelligence, Data Mining, Information Retrieval, Speech Processing, and Signal Processing. The data includes acceptance rates for long papers and short papers over several years for each conference, allowing researchers to track trends and make informed decisions about where to submit their work.
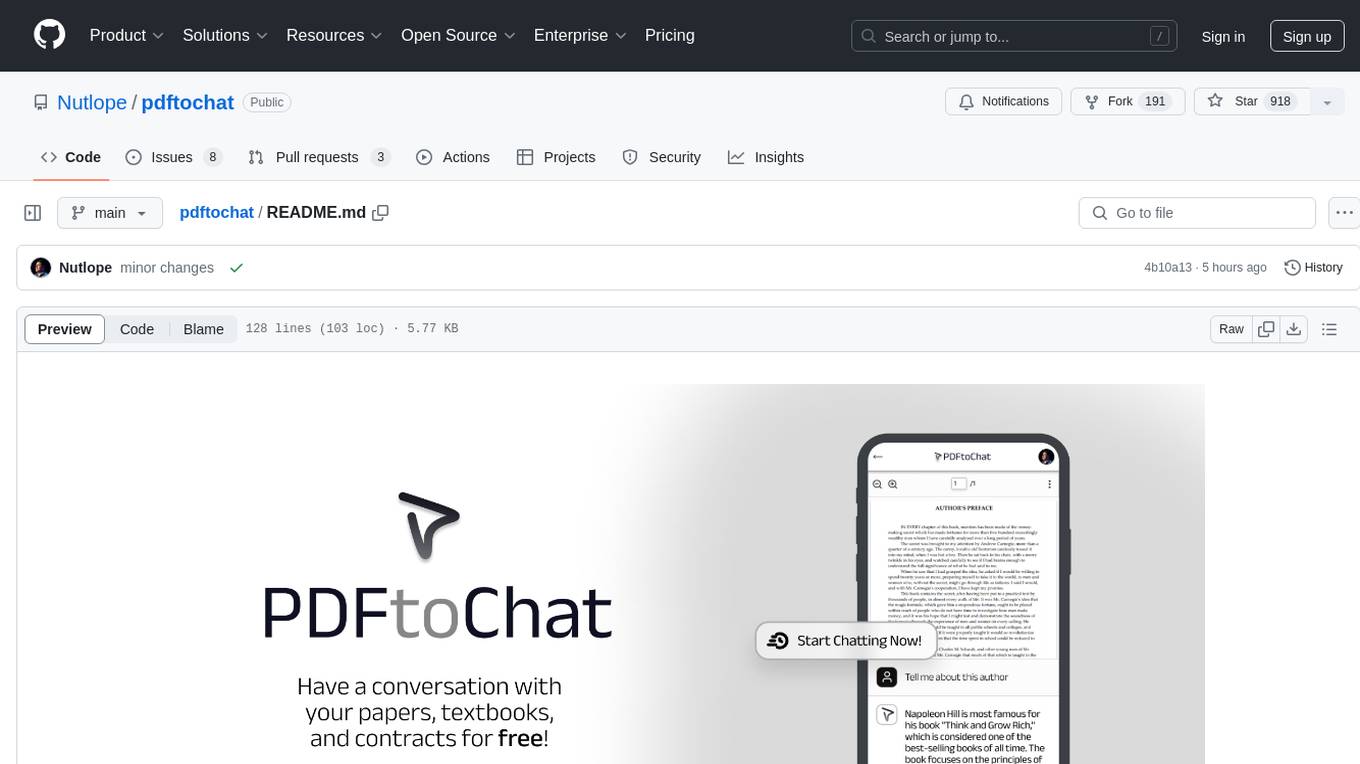
pdftochat
PDFToChat is a tool that allows users to chat with their PDF documents in seconds. It is powered by Together AI and Pinecone, utilizing a tech stack including Next.js, Mixtral, M2 Bert, LangChain.js, MongoDB Atlas, Bytescale, Vercel, Clerk, and Tailwind CSS. Users can deploy the tool to Vercel or any other host by setting up Together.ai, MongoDB Atlas database, Bytescale, Clerk, and Vercel. The tool enables users to interact with PDFs through chat, with future tasks including adding features like trash icon for deleting PDFs, exploring different embedding models, implementing auto scrolling, improving replies, benchmarking accuracy, researching chunking and retrieval best practices, adding demo video, upgrading to Next.js 14, adding analytics, customizing tailwind prose, saving chats in postgres DB, compressing large PDFs, implementing custom uploader, session tracking, error handling, and support for images in PDFs.
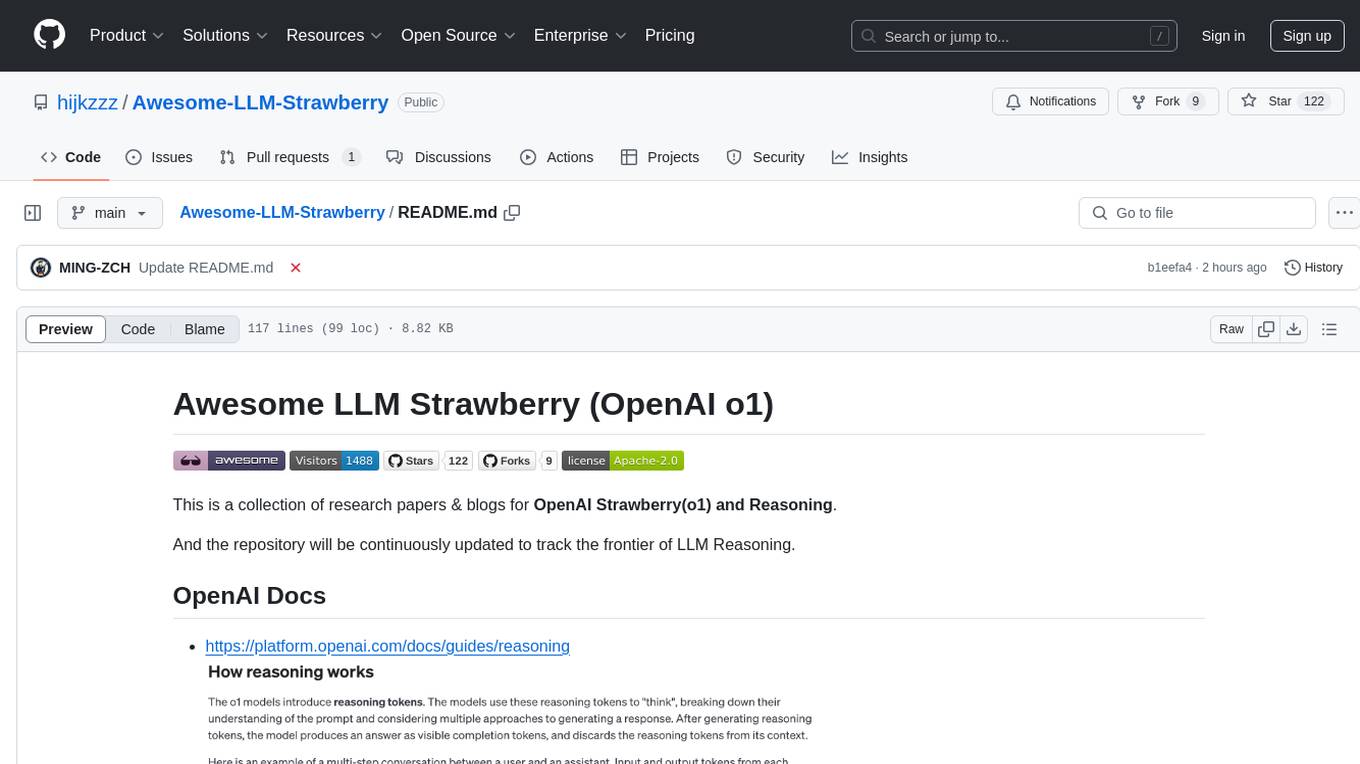
Awesome-LLM-Strawberry
Awesome LLM Strawberry is a collection of research papers and blogs related to OpenAI Strawberry(o1) and Reasoning. The repository is continuously updated to track the frontier of LLM Reasoning.
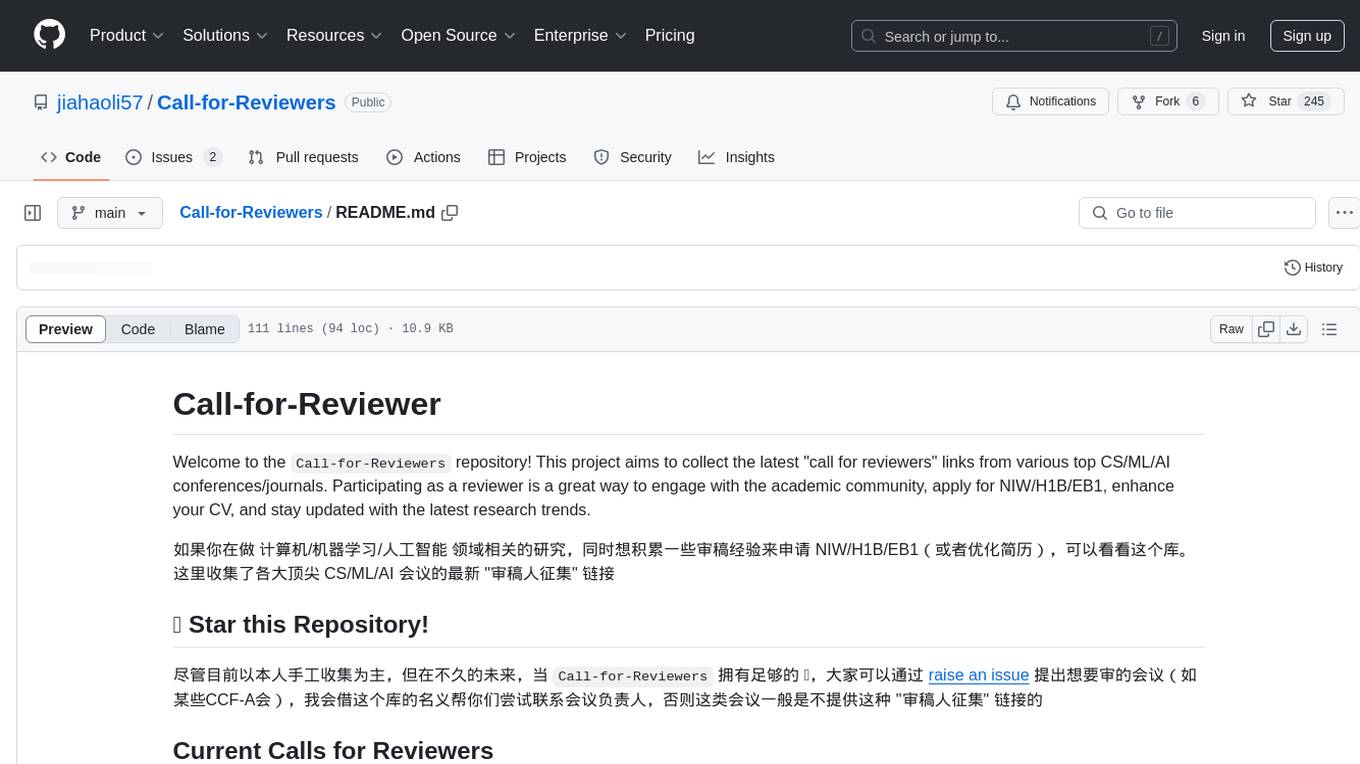
Call-for-Reviewers
The `Call-for-Reviewers` repository aims to collect the latest 'call for reviewers' links from various top CS/ML/AI conferences/journals. It provides an opportunity for individuals in the computer/ machine learning/ artificial intelligence fields to gain review experience for applying for NIW/H1B/EB1 or enhancing their CV. The repository helps users stay updated with the latest research trends and engage with the academic community.
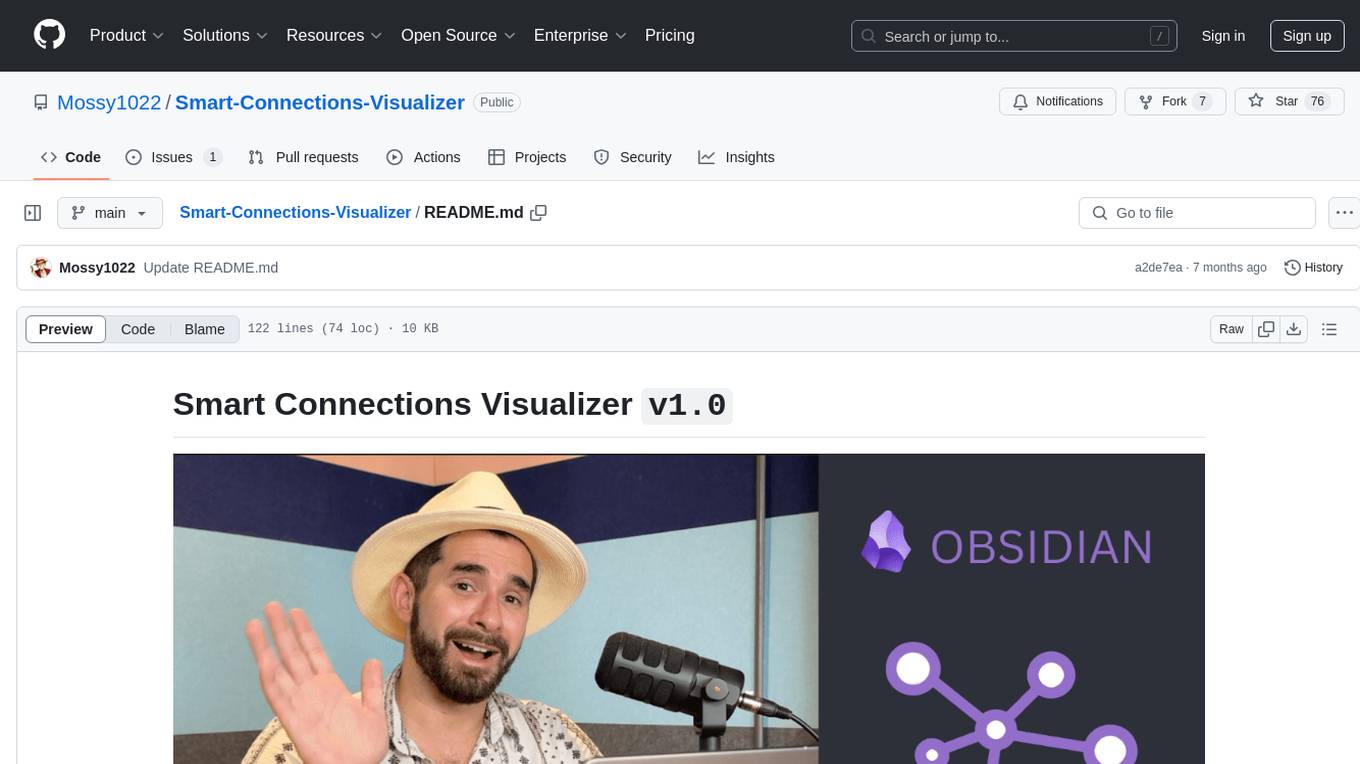
Smart-Connections-Visualizer
The Smart Connections Visualizer Plugin is a tool designed to enhance note-taking and information visualization by creating dynamic force-directed graphs that represent connections between notes or excerpts. Users can customize visualization settings, preview notes, and interact with the graph to explore relationships and insights within their notes. The plugin aims to revolutionize communication with AI and improve decision-making processes by visualizing complex information in a more intuitive and context-driven manner.