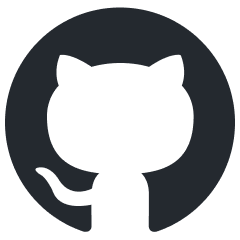
Conference-Acceptance-Rate
Acceptance rates for the major AI conferences
Stars: 4015
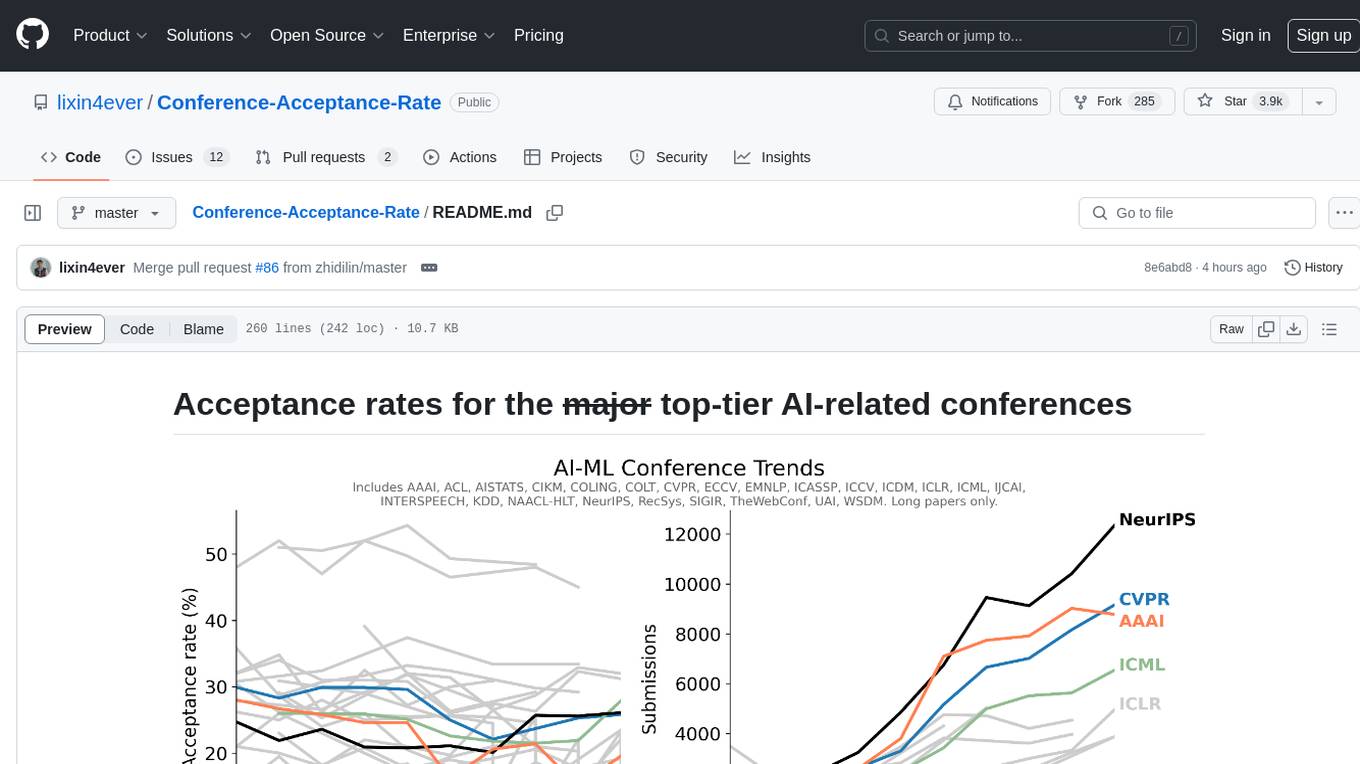
The 'Conference-Acceptance-Rate' repository provides acceptance rates for top-tier AI-related conferences in the fields of Natural Language Processing, Computational Linguistics, Computer Vision, Pattern Recognition, Machine Learning, Learning Theory, Artificial Intelligence, Data Mining, Information Retrieval, Speech Processing, and Signal Processing. The data includes acceptance rates for long papers and short papers over several years for each conference, allowing researchers to track trends and make informed decisions about where to submit their work.
README:
Conference | Long Paper | Short Paper |
---|---|---|
ACL'14 | 26.2% (146/572) | 26.1% (139/551) |
ACL'15 | 25.0% (173/692) | 22.4% (145/648) |
ACL'16 | 28.0% (231/825) | 21.0% (97/463) |
ACL'17 | 25.0% (195/751) | 18.9% (107/567) |
ACL'18 | 25.3% (258/1018) | 24.0% (126/526) |
ACL'19 | 25.7% (447/1737) | 18.2% (213/1168) |
ACL'20 | 25.4% (571/2244) | 17.6% (208/1185) |
ACL'21 | 24.5% (571/2327) | 13.6% (139/1023) |
ACL'21 Findings | 14.6% (339/2327) | 11.5% (118/1023) |
ACL'22 | ? (604/?) | ? (97/?) |
ACL'22 Findings | ? (361/?) | ? (361/?) |
ACL'23 | 23.5% (910/3872) | 16.5% (164/992) |
ACL'23 Findings | 18.4% (712/3872) | 19.1% (189/992) |
EMNLP'14 | 30.4% (155/510) | 27.8% (70/252) |
EMNLP'15 | 26.2% (157/600) | 22.1% (155/700) |
EMNLP'16 | 25.8% (177/687) | 21.8% (87/400) |
EMNLP'17 | 25.8% (216/836) | 18.4% (107/582) |
EMNLP'18 | 25.5% (351/1376) | 23.2% (198/855) |
EMNLP'19 | 25.6% (465/1813) | 20.5% (218/1063) |
EMNLP'20 | 24.5% (602/2455) | 16.6% (150/904) |
EMNLP'20 Findings | 13.5% (332/2455) | 12.7% (115/904) |
EMNLP'21 | 25.6% (650/2540) | 17.9% (190/1060) |
EMNLP'21 Findings | 11.8% (300/2540) | 11.2% (119/1060) |
EMNLP'22 | 22.1% (715/3242) | 12.0% (114/948) |
EMNLP'22 Findings | 14.0% (453/3242) | 10.1% (96/948) |
EMNLP'23 | 23.3% (901/3868) | 14.0% (146/1041) |
EMNLP'23 Findings | 22.9% (886/3868) | 19.5% (203/1041) |
NAACL-HLT'13 | 30.0% (88/293) | 32.1% (51/162) |
NAACL-HLT'15 | 29.1% (117/402) | 22.1% (69/312) |
NAACL-HLT'16 | 25.3% (100/396) | 28.9% (82/284) |
NAACL-HLT'18 | 32.0% (207/647) | 29.4% (125/425) |
NAACL-HLT'19 | 26.3% (281/1067) | 21.3% (142/666) |
NAACL-HLT'21 | 29.2% (366/1254) | 22.6% (123/544) |
NAACL-HLT'22 | ? (358/?) | ? (84/?) |
NAACL-HLT'22 Findings | ? (183/?) | ? (26/?) |
COLING'12 | 27% (311/1000+) | - |
COLING'14 | 30.8% (217/705) | - |
COLING'16 | 32.4% (337/1039) | - |
COLING'18 | 37.4% (332/888) | - |
COLING'20 | 33.4% (622/1862) | - |
COLING'22 | 33.4% (522/1563) | 24.2% (112/463) |
Conference | Long Paper | Short Paper |
---|---|---|
CVPR'14 | 29.9% (540/1807) (104 orals and 436 posters) | - |
CVPR'15 | 28.3% (602/2123) (71 orals and 531 posters) | - |
CVPR'16 | 29.9% (643/2145) (83 orals, 123 spotlights and 437 posters) | - |
CVPR'17 | 29.9% (783/2620) (71 orals, 144 spotlights and 568 posters) | - |
CVPR'18 | 29.6% (979/3303) (70 orals, 224 spotlights and 685 posters) | - |
CVPR'19 | 25.0% (1294/5160) (288 short orals and 1294 posters) | - |
CVPR'20 | 22.1% (1470/6656) | - |
CVPR'21 | 23.7% (1661/7015) (295 orals and 1366 posters) | - |
CVPR'22 | 25.3% (2067/8161) | - |
CVPR'23 | 25.8% (2360/9155) | - |
CVPR'24 | 23.6% (2719/11532) (90 orals, 324 Highlight, 2305 posters) | - |
ICCV'13 | 27.9% (454/1629) (41 orals and 413 posters) | - |
ICCV'15 | 30.9% (525/1698) | - |
ICCV'17 | 29.0% (621/2143) (45 orals, 56 spotlights and 520 posters) | - |
ICCV'19 | 25.0% (1077/4304) (187 short orals and 1077 posters) | - |
ECCV'14 | 27.9% (363/1444) (38 orals and 325 posters) | - |
ECCV'16 | 26.6% (415/1561) (28 orals, 45 spotlights and 342 posters) | - |
ECCV'18 | 31.8% (776/2439) (59 orals and 717 posters) | - |
ECCV'20 | 27.1% (1361/5025) (104 orals, 161 spotlights and 1096 posters) | - |
Conference | Long Paper | Short Paper |
---|---|---|
ICML'14 | 15.0% (Cycle I), 22.0% (Cycle II) | - |
ICML'15 | 26.0% (270/1037) | - |
ICML'16 | 24.0% (322/?) | - |
ICML'17 | 25.9% (434/1676) | - |
ICML'18 | 25.1% (621/2473) | - |
ICML'19 | 22.6% (773/3424) | - |
ICML'20 | 21.8% (1088/4990) | - |
ICML'21 | 21.5% (1184/5513) (166 long talks, 1018 short talks) | - |
ICML'22 | 21.9% (1235/5630) (118 long talks, 1117 short talks) | - |
ICML'23 | 27.9% (1827/6538) (158 live orals, 1669 virtual orals with posters) | - |
ICML'24 | 27.5% (2610/9473) (144 orals, 191 spotlights and 2275 posters) | - |
NeurIPS'14 | 24.7% (414/1678) | - |
NeurIPS'15 | 21.9% (403/1838) | - |
NeurIPS'16 | 23.6% (569/2403) | - |
NeurIPS'17 | 20.9% (678/3240) (40 orals, 112 spotlights and 526 posters) | - |
NeurIPS'18 | 20.8% (1011/4856) (30 orals, 168 spotlights and 813 posters) | - |
NeurIPS'19 | 21.1% (1428/6743) (36 orals, 164 spotlights and 1228 posters) | - |
NeurIPS'20 | 20.1% (1900/9454) (105 orals, 280 spotlights and 1515 posters) | - |
NeurIPS'21 | 25.7% (2344/9122) (55 orals, 260 spotlights and 2029 posters) | - |
NeurIPS'22 | 25.6% (?/10411) (? orals, ? spotlights and ? posters) | - |
NeurIPS'23 | 26.1% (3218/12343) (67 orals, 378 spotlights and 2773 posters) | - |
ICLR'14 | - | - |
ICLR'15 | - | - |
ICLR'16 | - | - |
ICLR'17 | 39.1% (198/507) (15 orals and 183 posters) | - |
ICLR'18 | 32.0% (314/981) (23 orals and 291 posters) | - |
ICLR'19 | 31.4% (500/1591) (24 orals and 476 posters) | - |
ICLR'20 | 26.5% (687/2594) (48 orals, 107 spotlights and 532 posters) | - |
ICLR'21 | 28.7% (860/2997) (53 orals, 114 spotlights and 693 posters) | - |
ICLR'22 | 32.9% (1095/3328) (54 orals, 176 spotlights and 865 posters) | - |
ICLR'23 | 32.0% (1574/4956) (91 orals, 280 spotlights and 1203 posters) | - |
ICLR'24 | 30.81% (2250/7304) (85 orals, 366 spotlights and 1799 posters) | - |
COLT'14 | 32.1% (45/140) | - |
COLT'15 | 34.8% (62/178) | - |
COLT'16 | 26.1% (53/203) | - |
COLT'17 | 32.5% (74/228) | - |
COLT'18 | 27.2% (91/335) | - |
COLT'19 | 30.0% (118/393) | - |
COLT'20 | 30.9% (120/388) | - |
UAI'14 | 32.0% (94/292) | - |
UAI'15 | 34.0% (99/291) | - |
UAI'16 | 31.0% (85/275) | - |
UAI'17 | 31.0% (87/282) | - |
UAI'18 | 30.8% (104/337) | - |
UAI'19 | 26.0% (118/450) | - |
UAI'20 | 27.5% (142/515) | - |
UAI'21 | 26.3% (205/777) | - |
UAI'22 | 32.3% (230/712) (36 orals and 194 posters) | - |
UAI'23 | 31.2% (243/778) | - |
AISTATS'14 | 35.8% (120/335) | - |
AISTATS'15 | 28.7% (127/442) | - |
AISTATS'16 | 30.7% (165/537) | - |
AISTATS'17 | 31.7% (168/530) | - |
AISTATS'18 | 33.2% (214/645) | - |
AISTATS'19 | 32.4% (360/1111) | - |
AISTATS'20 | - | - |
AISTATS'21 | 29.8% (455/1527) (48 orals) | - |
AISTATS'22 | 29.2% (493/1685) | - |
AISTATS'23 | 29.4% (496/1686) | - |
AISTATS'24 | 27.6% (546/1980) | - |
Conference | Long Paper | Short Paper |
---|---|---|
AAAI'14 | 28.0% (398/1406) | - |
AAAI'15 | 26.7% (531/1991) | - |
AAAI'16 | 25.8% (549/2132) | - |
AAAI'17 | 24.6% (638/2590) | - |
AAAI'18 | 24.6% (933/3800) | - |
AAAI'19 | 16.2% (1150/7095) | - |
AAAI'20 | 20.6% (1591/7737) | - |
AAAI'21 | 21.4% (1692/7911) | - |
AAAI'22 | 15.0% (1349/9020) | - |
AAAI'23 | 19.6% (1721/8777) | - |
AAAI'24 | 23.75% (2342/9862) | - |
IJCAI'13 | 28.0% (413/1473) | - |
IJCAI'15 | 28.6% (572/1996) | - |
IJCAI'16 | 24.0% (551/2294) | - |
IJCAI'17 | 26.0% (660/2540) | - |
IJCAI'18 | 20.5% (710/3470) | - |
IJCAI'19 | 17.9% (850/4752) | - |
IJCAI'20 | 12.6% (592/4717) | - |
IJCAI'21 | 13.9% (587/4204) | - |
IJCAI'22 | 14.9% (679/4535) | - |
Conference | Long Paper | Short Paper |
---|---|---|
KDD'14 | 14.6% (151/1036) | - |
KDD'15 | 19.5% (160/819) | - |
KDD'16 | 13.7% (142/1115) | - |
KDD'17 | 17.4% (130/748) | - |
KDD'18 | 18.4% (181/983) (107 orals and 74 posters) | - |
KDD'19 | 14.2% (170/1200) (110 orals and 60 posters) | - |
KDD'20 | 16.9% (216/1279) | - |
KDD'22 | 15.0% (254/1695) | - |
KDD'23 | 22.1% (313/1416) | - |
SIGIR'14 | 21.0% (82/387) | 40.0% (104/263) |
SIGIR'15 | 20.0% (70/351) | 31.3% (79/252) |
SIGIR'16 | 18.0% (62/341) | 30.6% (104/339) |
SIGIR'17 | 22.0% (78/362) | 30.0% (121/398) |
SIGIR'18 | 21.0% (86/409) | 30.0% (98/327) |
SIGIR'19 | 19.7% (84/426) | 24.4% (108/443) |
SIGIR'20 | 26.5% (147/555) | 30.2% (153/507) |
SIGIR'21 | 21.0% (151/720) | 27.6% (145/526) |
SIGIR'22 | 20.3% (161/794) | 24.7% (165/667) |
TheWebConf'14 | 13.0% (84/645) | - |
TheWebConf'15 | 14.0% (131/929) | - |
TheWebConf'16 | 16.0% (115/727) | - |
TheWebConf'17 | 17.0% (164/966) | - |
TheWebConf'18 | 15.0% (171/1140) | - |
TheWebConf'19 | 18.0% (225/1247) | 19.9% (72/361) |
TheWebConf'20 | 19.2% (217/1129) | 24.7% (98/397) |
TheWebConf'21 | 20.6% (357/1736) | - |
TheWebConf'22 | 17.7% (323/1822) | - |
TheWebConf'23 | 19.2% (365/1900) | - |
WSDM'14 | 18.0% (64/355) | - |
WSDM'15 | 16.4% (39/238) | - |
WSDM'16 | 18.2% (67/368) | - |
WSDM'17 | 15.8% (80/505) | - |
WSDM'18 | 16.1% (84/514) | - |
WSDM'19 | 16.4% (84/511) | - |
WSDM'20 | 14.8% (91/615) | - |
WSDM'21 | 18.6% (112/603) | - |
WSDM'22 | 15.8% (80/505) | - |
WSDM'23 | 17.8% (123/690) | - |
CIKM'14 | 21.0% (175/838) | 21.9% (57/260) |
CIKM'15 | 26.0% (165/646) | 25.0% (69/276) |
CIKM'16 | 23.0% (160/701) | 23.5% (55/234) |
CIKM'17 | 20.0% (171/855) | 28.4% (119/419) |
CIKM'18 | 17.0% (147/862) | 23.2% (96/413) |
CIKM'19 | 19.4% (200/1030) | 21.3% (100/470) |
CIKM'20 | 21.0% (193/920) | 25.9% (103/397) |
CIKM'21 | 21.7% (271/1251) | 28.3% (177/626) |
CIKM'22 | ?% (272/?) | ?% (196/?) |
ICDM'14 | 9.8% (71/727) | 9.8% (71/727) |
ICDM'15 | 8.4% (68/807) | 9.7% (78/807) |
ICDM'16 | 8.6% (78/904) | 11.0% (100/904) |
ICDM'17 | 9.3% (72/778) | 10.7% (83/778) |
ICDM'18 | 8.9% (84/948) | 11.1% (105/948) |
ICDM'19 | 9.1% (95/1046) | 9.5% (99/1046) |
ICDM'20 | 9.8% (91/930) | 9.9% (92/930) |
ICDM'21 | 9.9% (98/990) | 10.1% (100/990) |
RecSys'15 | 23.0% (35/152) | - |
RecSys'16 | 18.2% (29/159) | - |
RecSys'17 | 20.8% (26/125) | 16.4% (20/122) |
RecSys'18 | 17.7% (32/181) | - |
RecSys'19 | 19.0% (36/189) | - |
RecSys'20 | 17.9% (39/218) | - |
Conference | Long Paper | Short Paper |
---|---|---|
INTERSPEECH'14 | - | - |
INTERSPEECH'15 | 51.0% (~743/1458) | - |
INTERSPEECH'16 | 50.5% (779/1541) | - |
INTERSPEECH'17 | 52.0% (799/1582) | - |
INTERSPEECH'18 | 54.3% (749/1320) | - |
INTERSPEECH'19 | 49.3% (914/1855) | - |
INTERSPEECH'20 | ~47% (?/?) | - |
INTERSPEECH'21 | 48.4% (963/1990) | - |
ICASSP'14 | 48.0% (1709/3500) | - |
ICASSP'15 | 52.0% (1207/2322) | - |
ICASSP'16 | 47.0% (1265/2682) | - |
ICASSP'17 | 52.0% (1220/2518) | - |
ICASSP'18 | 49.7% (1406/2829) | - |
ICASSP'19 | 46.5% (1774/3815) | - |
ICASSP'21 | 48.0% (1734/3610) | - |
ICASSP'22 | 45.0% (1785/3967) | - |
ICASSP'23 | 45.1% (2765/6127) | - |
ICASSP'24 | 45.0% (~2812/5796) | - |
Note:
- For KDD and TheWebConf (formerly known as WWW), only the papers from research track are counted.
- For ICDM, submissions of short paper and those of long paper are in the same session and the decision of the paper type is made according to its quality.
For Tasks:
Click tags to check more tools for each tasksFor Jobs:
Alternative AI tools for Conference-Acceptance-Rate
Similar Open Source Tools
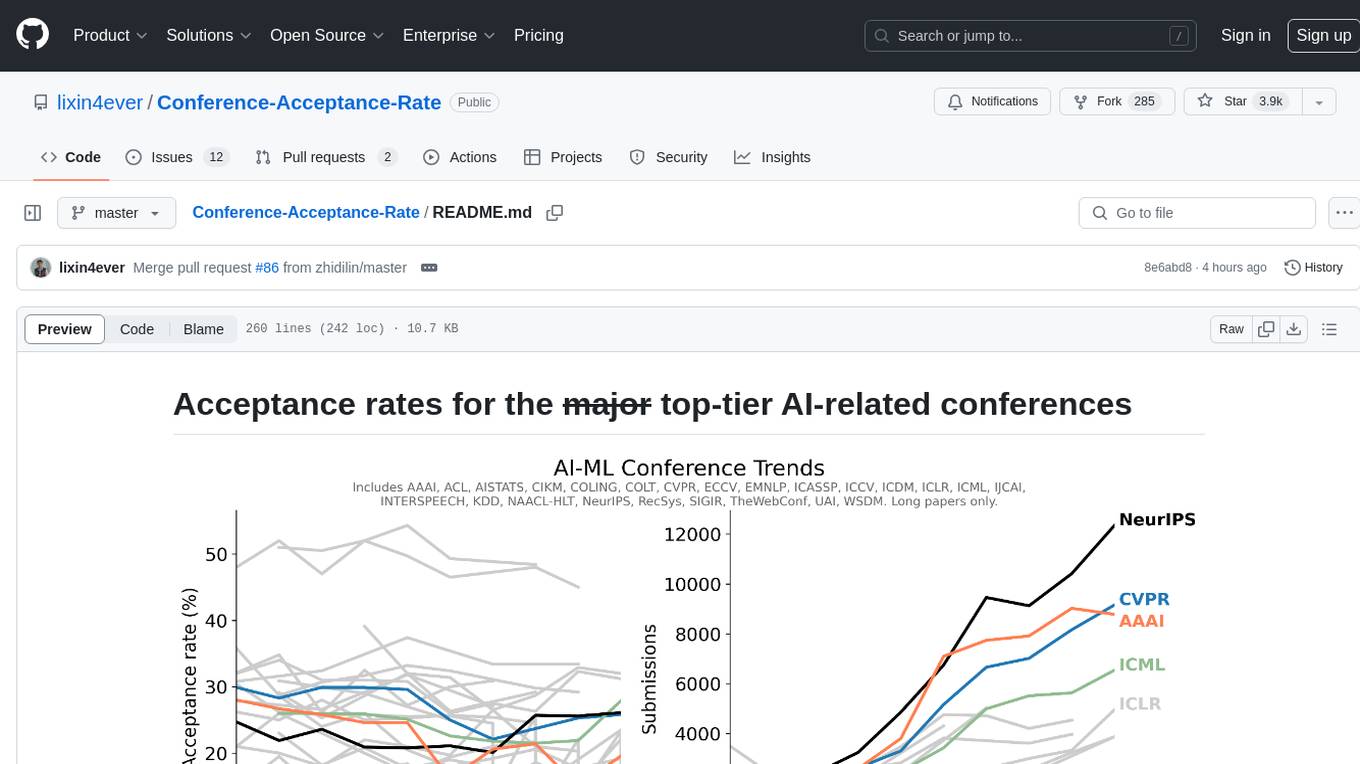
Conference-Acceptance-Rate
The 'Conference-Acceptance-Rate' repository provides acceptance rates for top-tier AI-related conferences in the fields of Natural Language Processing, Computational Linguistics, Computer Vision, Pattern Recognition, Machine Learning, Learning Theory, Artificial Intelligence, Data Mining, Information Retrieval, Speech Processing, and Signal Processing. The data includes acceptance rates for long papers and short papers over several years for each conference, allowing researchers to track trends and make informed decisions about where to submit their work.
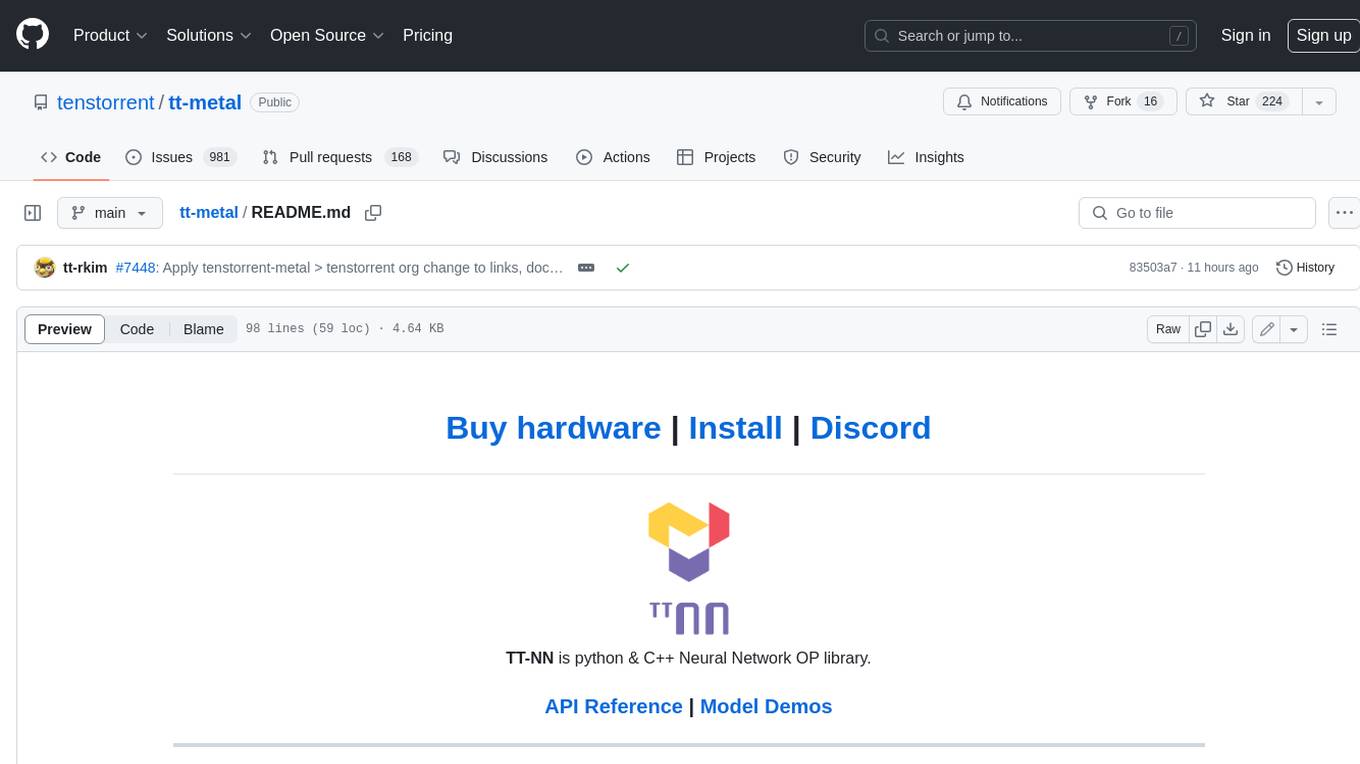
tt-metal
TT-NN is a python & C++ Neural Network OP library. It provides a low-level programming model, TT-Metalium, enabling kernel development for Tenstorrent hardware.
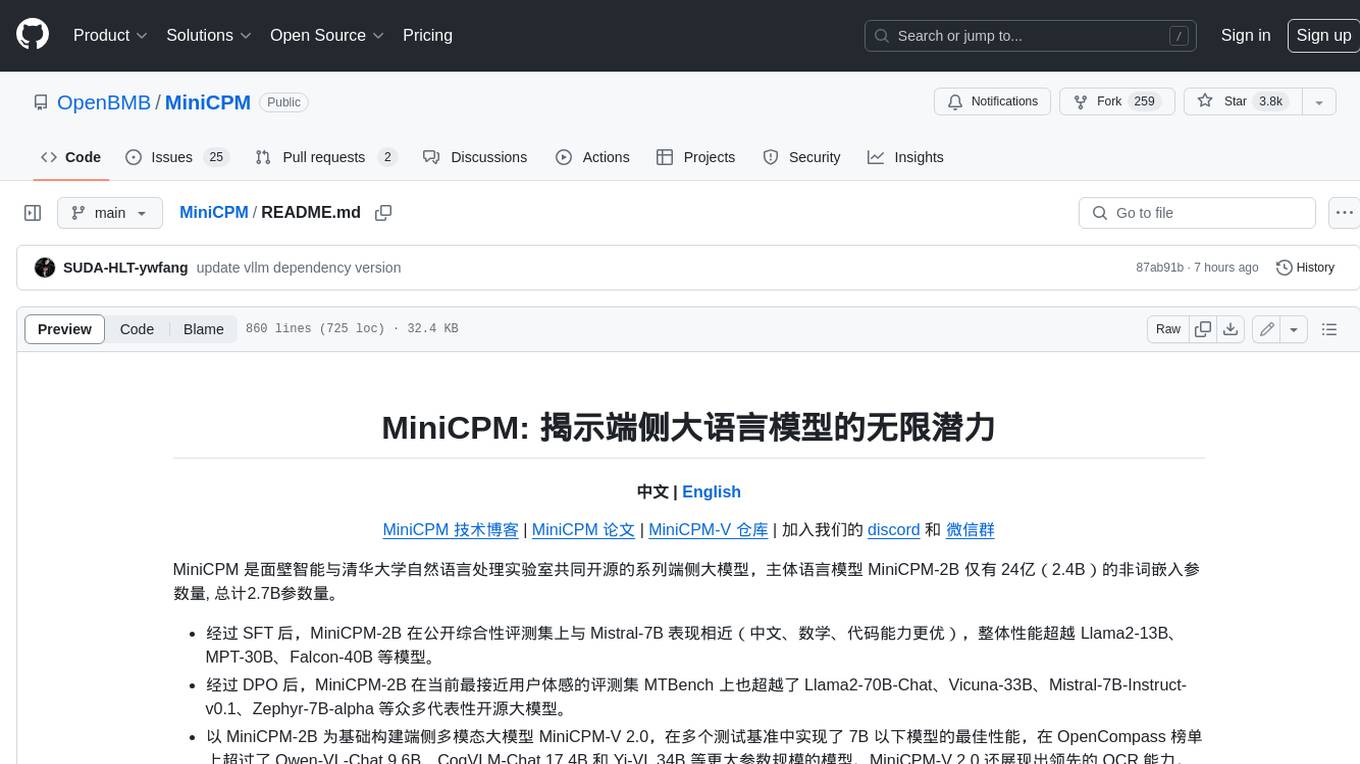
MiniCPM
MiniCPM is a series of open-source large models on the client side jointly developed by Face Intelligence and Tsinghua University Natural Language Processing Laboratory. The main language model MiniCPM-2B has only 2.4 billion (2.4B) non-word embedding parameters, with a total of 2.7B parameters. - After SFT, MiniCPM-2B performs similarly to Mistral-7B on public comprehensive evaluation sets (better in Chinese, mathematics, and code capabilities), and outperforms models such as Llama2-13B, MPT-30B, and Falcon-40B overall. - After DPO, MiniCPM-2B also surpasses many representative open-source large models such as Llama2-70B-Chat, Vicuna-33B, Mistral-7B-Instruct-v0.1, and Zephyr-7B-alpha on the current evaluation set MTBench, which is closest to the user experience. - Based on MiniCPM-2B, a multi-modal large model MiniCPM-V 2.0 on the client side is constructed, which achieves the best performance of models below 7B in multiple test benchmarks, and surpasses larger parameter scale models such as Qwen-VL-Chat 9.6B, CogVLM-Chat 17.4B, and Yi-VL 34B on the OpenCompass leaderboard. MiniCPM-V 2.0 also demonstrates leading OCR capabilities, approaching Gemini Pro in scene text recognition capabilities. - After Int4 quantization, MiniCPM can be deployed and inferred on mobile phones, with a streaming output speed slightly higher than human speech speed. MiniCPM-V also directly runs through the deployment of multi-modal large models on mobile phones. - A single 1080/2080 can efficiently fine-tune parameters, and a single 3090/4090 can fully fine-tune parameters. A single machine can continuously train MiniCPM, and the secondary development cost is relatively low.
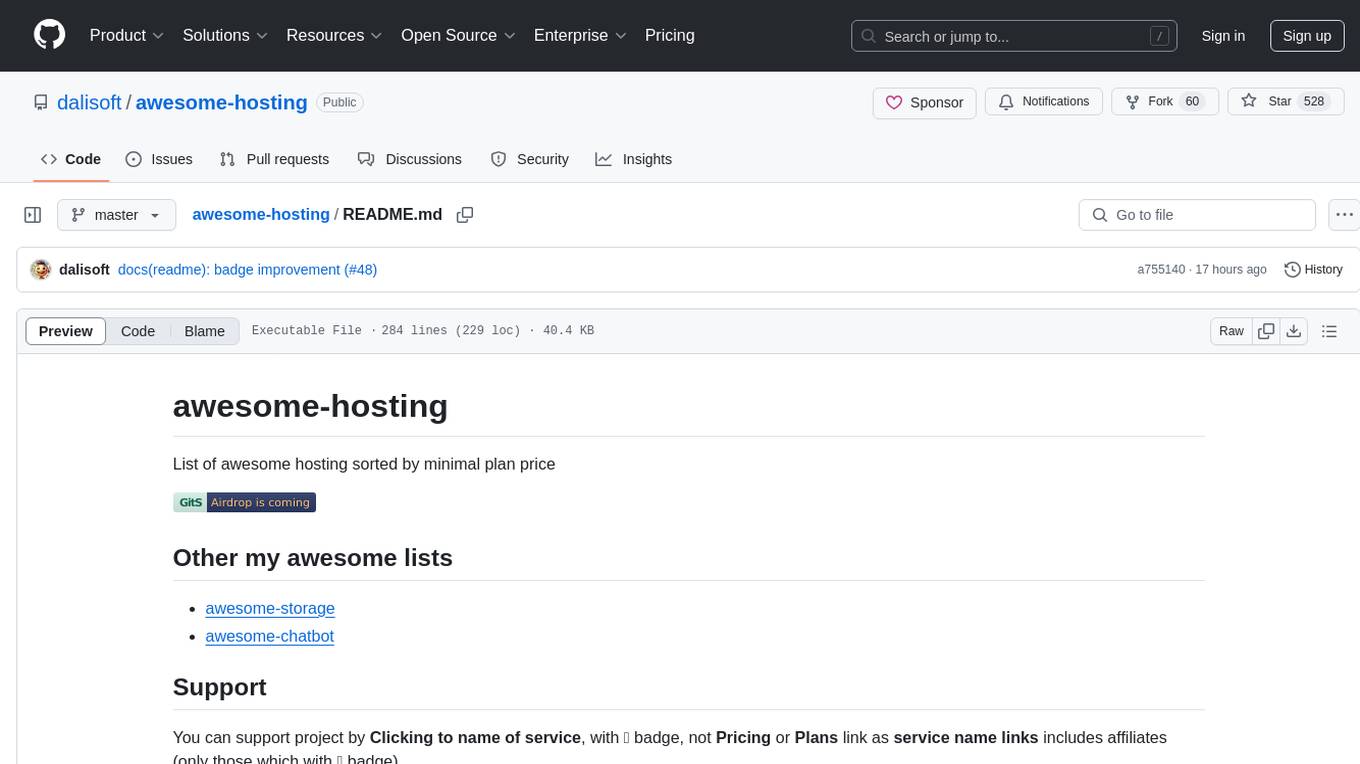
awesome-hosting
awesome-hosting is a curated list of hosting services sorted by minimal plan price. It includes various categories such as Web Services Platform, Backend-as-a-Service, Lambda, Node.js, Static site hosting, WordPress hosting, VPS providers, managed databases, GPU cloud services, and LLM/Inference API providers. Each category lists multiple service providers along with details on their minimal plan, trial options, free tier availability, open-source support, and specific features. The repository aims to help users find suitable hosting solutions based on their budget and requirements.
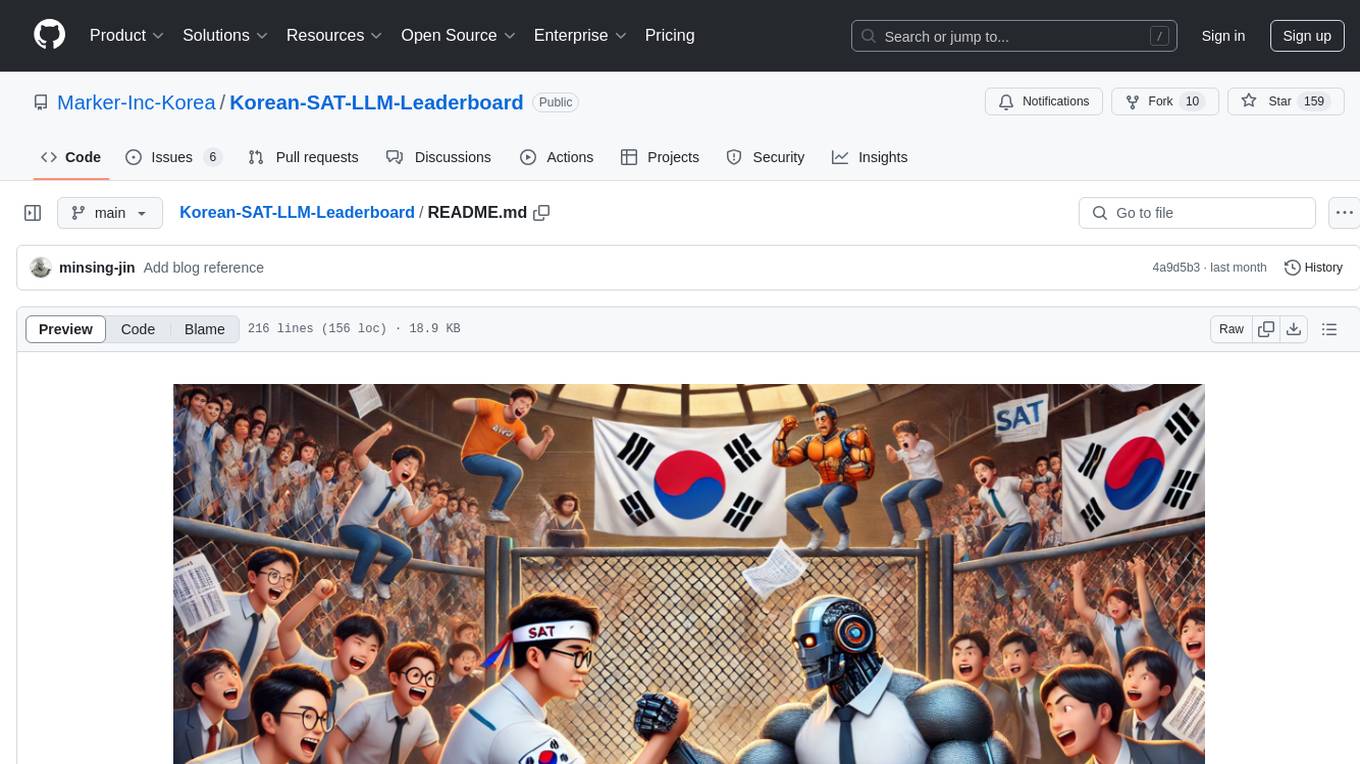
Korean-SAT-LLM-Leaderboard
The Korean SAT LLM Leaderboard is a benchmarking project that allows users to test their fine-tuned Korean language models on a 10-year dataset of the Korean College Scholastic Ability Test (CSAT). The project provides a platform to compare human academic ability with the performance of large language models (LLMs) on various question types to assess reading comprehension, critical thinking, and sentence interpretation skills. It aims to share benchmark data, utilize a reliable evaluation dataset curated by the Korea Institute for Curriculum and Evaluation, provide annual updates to prevent data leakage, and promote open-source LLM advancement for achieving top-tier performance on the Korean CSAT.
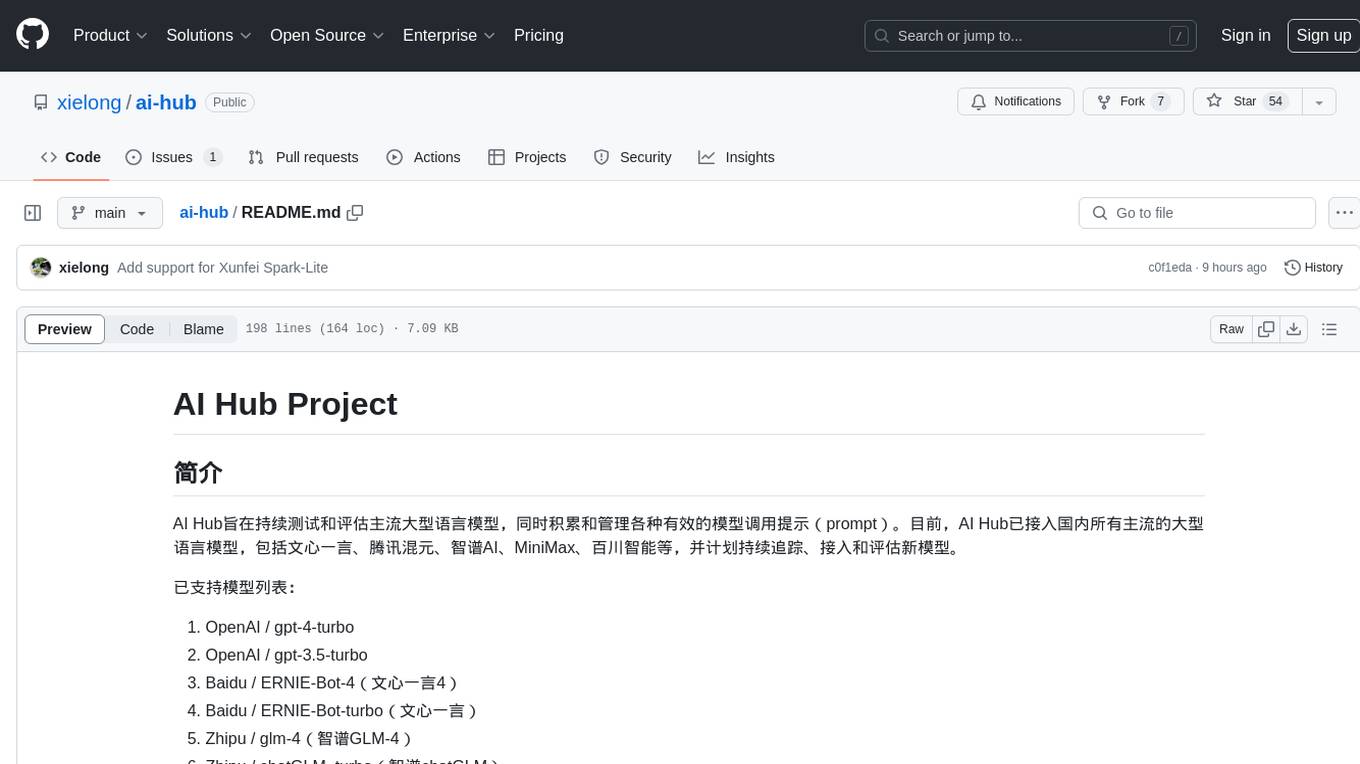
ai-hub
AI Hub Project aims to continuously test and evaluate mainstream large language models, while accumulating and managing various effective model invocation prompts. It has integrated all mainstream large language models in China, including OpenAI GPT-4 Turbo, Baidu ERNIE-Bot-4, Tencent ChatPro, MiniMax abab5.5-chat, and more. The project plans to continuously track, integrate, and evaluate new models. Users can access the models through REST services or Java code integration. The project also provides a testing suite for translation, coding, and benchmark testing.
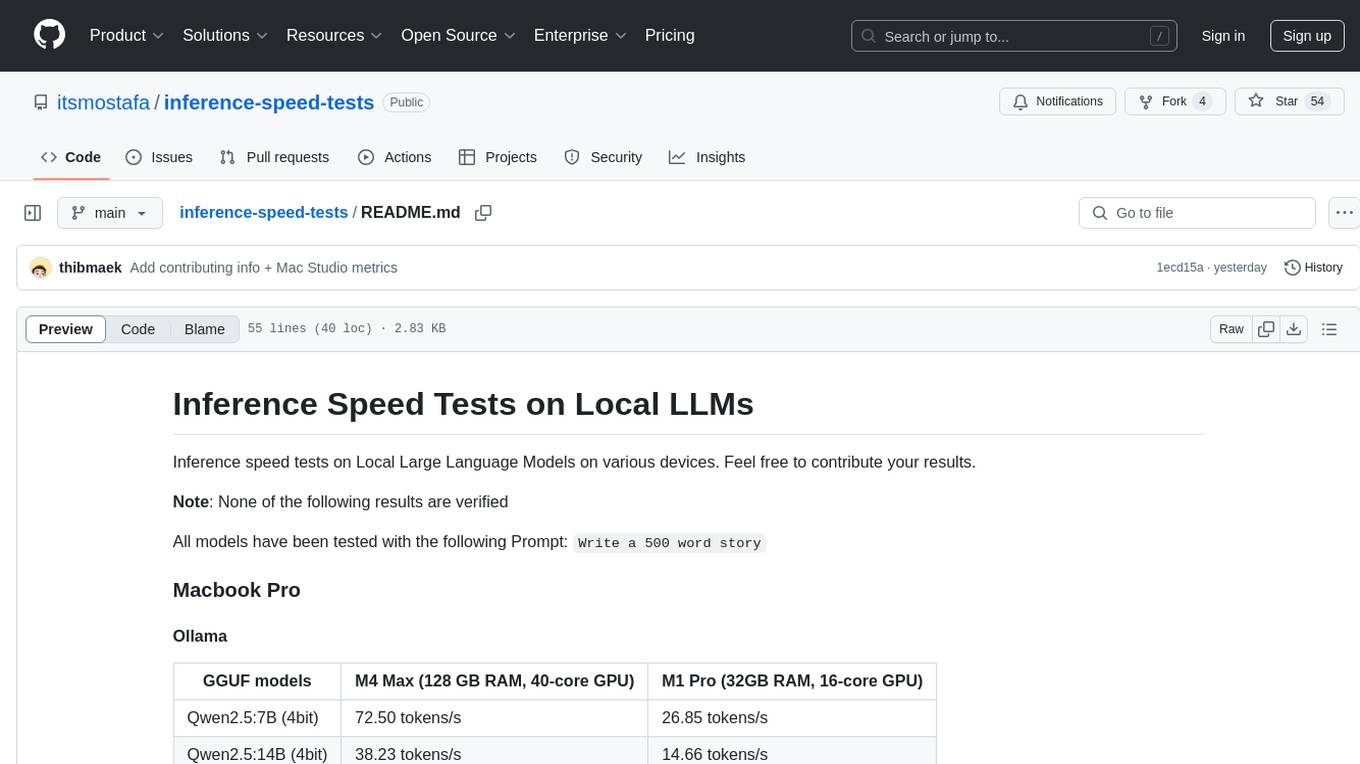
inference-speed-tests
This repository contains inference speed tests on Local Large Language Models on various devices. It provides results for different models tested on Macbook Pro and Mac Studio. Users can contribute their own results by running models with the provided prompt and adding the tokens-per-second output. Note that the results are not verified.
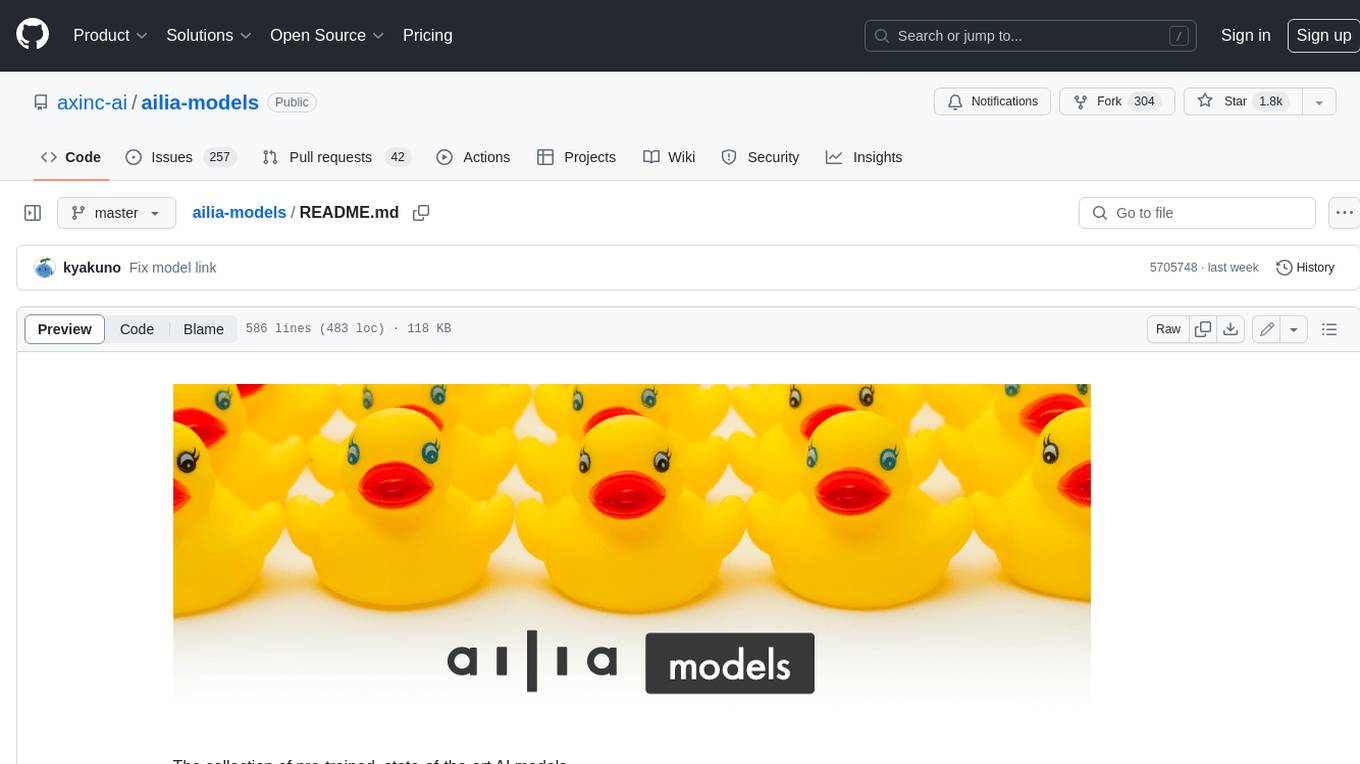
ailia-models
The collection of pre-trained, state-of-the-art AI models. ailia SDK is a self-contained, cross-platform, high-speed inference SDK for AI. The ailia SDK provides a consistent C++ API across Windows, Mac, Linux, iOS, Android, Jetson, and Raspberry Pi platforms. It also supports Unity (C#), Python, Rust, Flutter(Dart) and JNI for efficient AI implementation. The ailia SDK makes extensive use of the GPU through Vulkan and Metal to enable accelerated computing. # Supported models 323 models as of April 8th, 2024
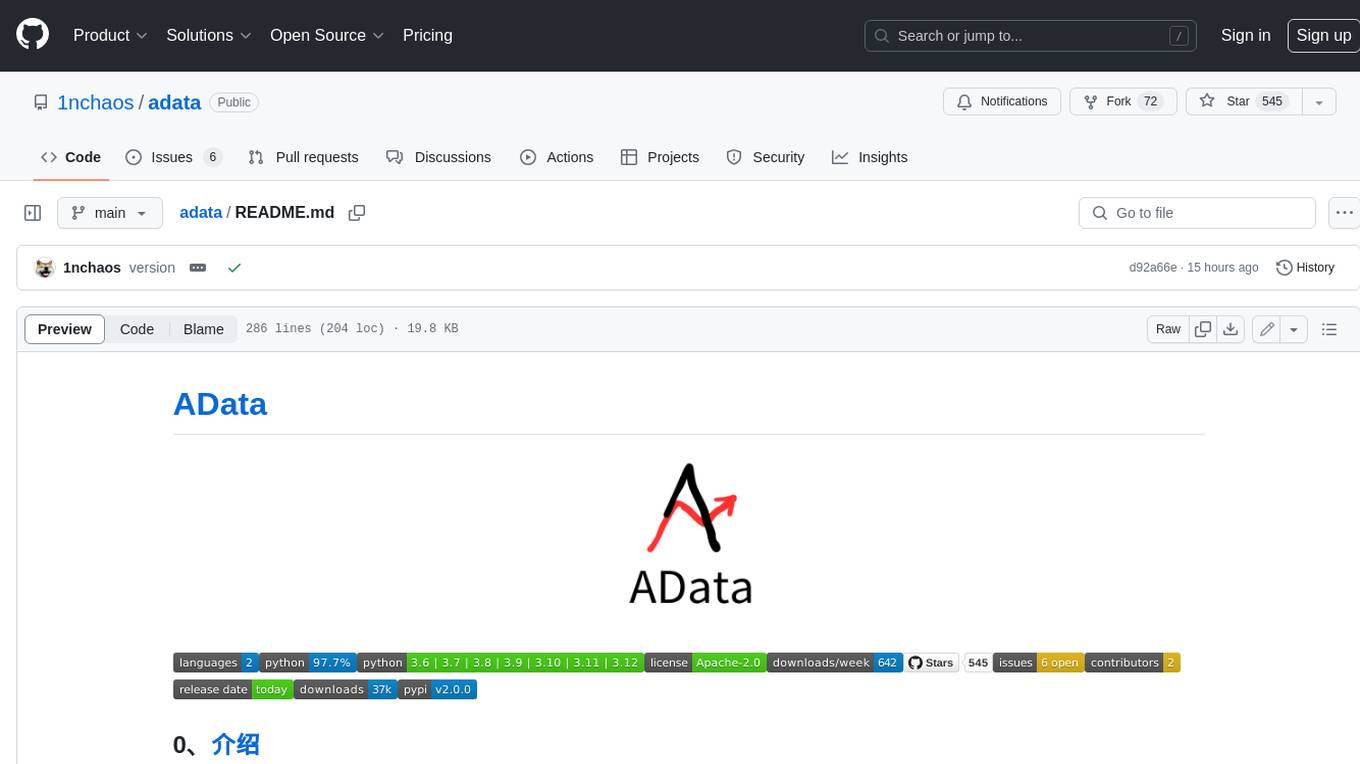
adata
AData is a free and open-source A-share database that focuses on transaction-related data. It provides comprehensive data on stocks, including basic information, market data, and sentiment analysis. AData is designed to be easy to use and integrate with other applications, making it a valuable tool for quantitative trading and AI training.
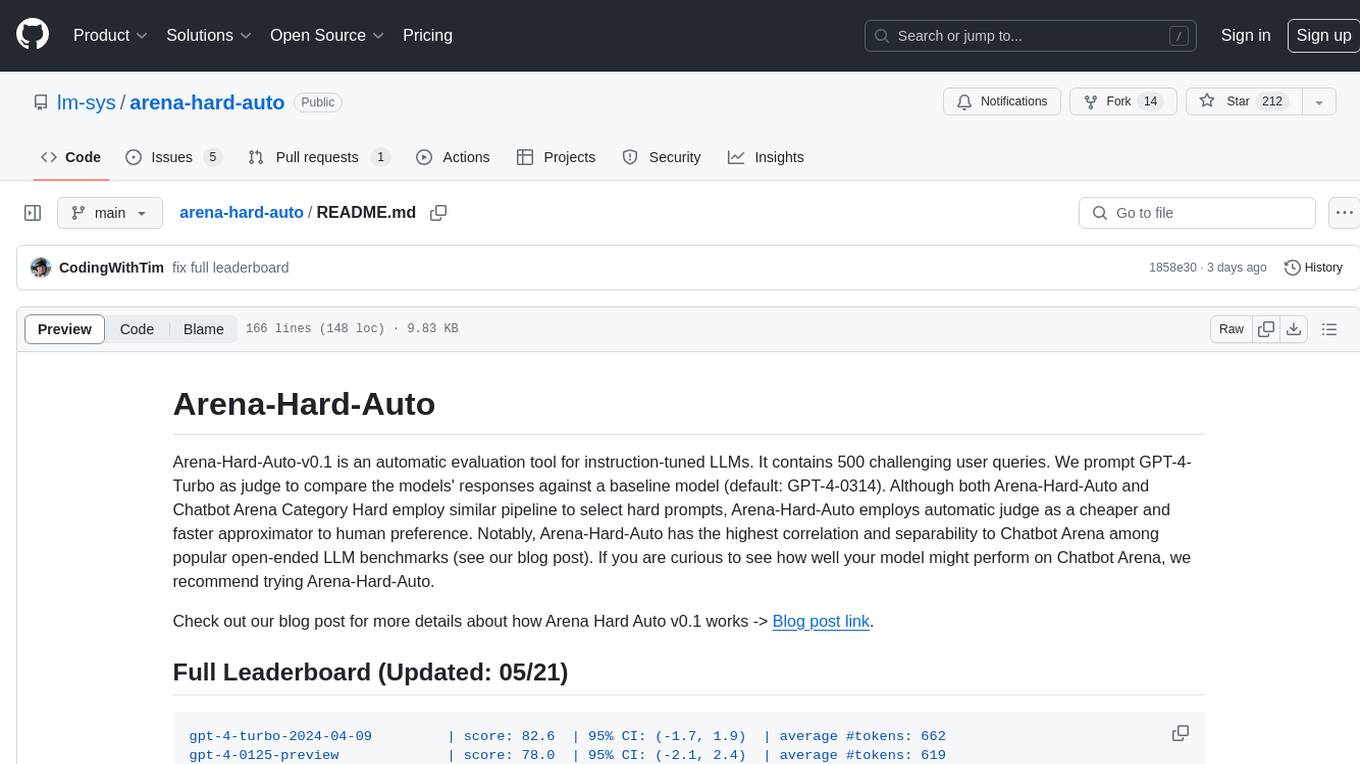
arena-hard-auto
Arena-Hard-Auto-v0.1 is an automatic evaluation tool for instruction-tuned LLMs. It contains 500 challenging user queries. The tool prompts GPT-4-Turbo as a judge to compare models' responses against a baseline model (default: GPT-4-0314). Arena-Hard-Auto employs an automatic judge as a cheaper and faster approximator to human preference. It has the highest correlation and separability to Chatbot Arena among popular open-ended LLM benchmarks. Users can evaluate their models' performance on Chatbot Arena by using Arena-Hard-Auto.
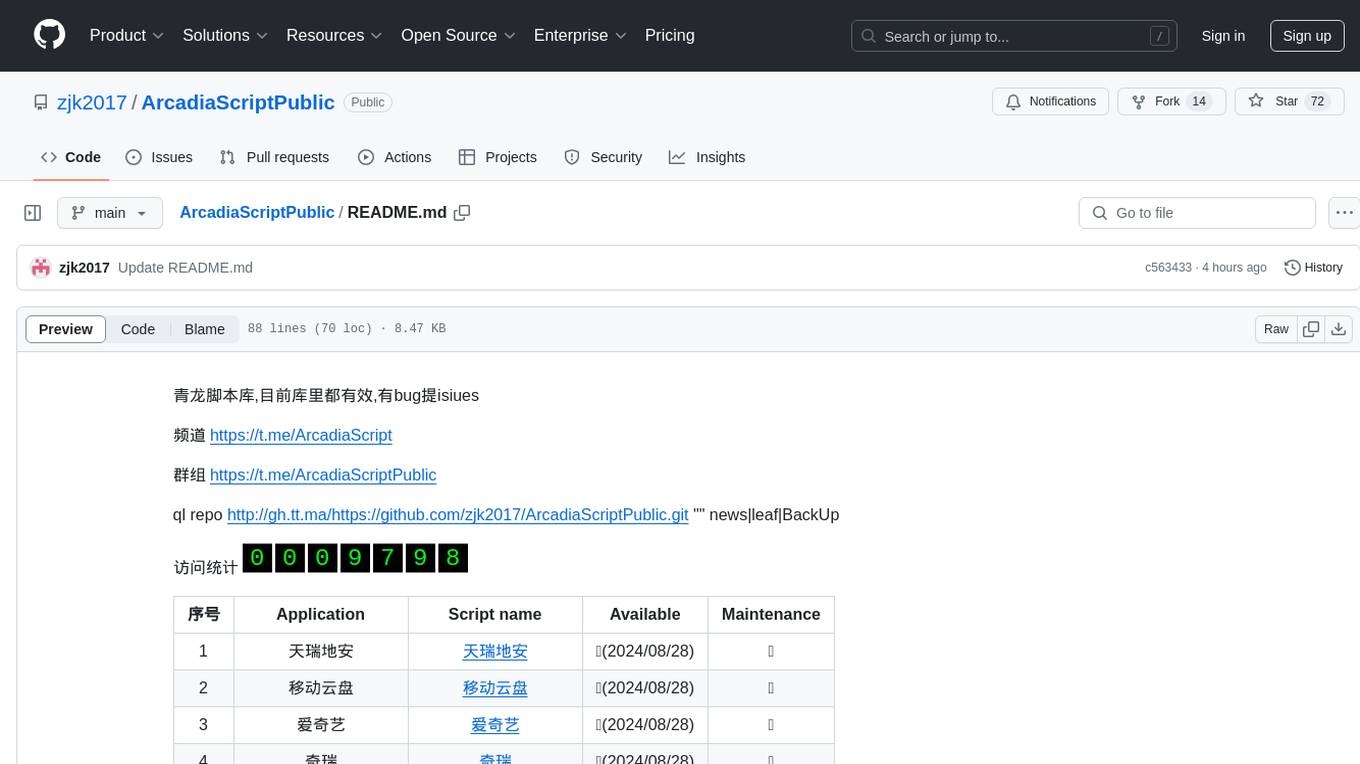
ArcadiaScriptPublic
ArcadiaScriptPublic is a repository containing various scripts for learning and practicing JavaScript, Python, and Shell scripting. It is intended for testing and educational purposes only, and not for commercial use. The repository does not guarantee the legality, accuracy, completeness, or effectiveness of the scripts, and users are advised to use them at their own discretion. No resources from the repository are allowed to be republished or redistributed by any public account or self-media. The repository owner disclaims any responsibility for script-related issues, including losses or damages resulting from script errors. Users indirectly utilizing the scripts, such as setting up VPS or engaging in activities that violate national/regional laws or regulations, are solely responsible for any privacy leaks or consequences. If any entity or individual believes that the scripts in the project may infringe upon their rights, they should promptly notify and provide proof of identity and ownership, upon which the relevant scripts will be removed after verification. Anyone viewing or using the scripts in this project should carefully read and accept the disclaimer provided by zjk2017/ArcadiaScriptPublic, as the repository reserves the right to change or supplement the disclaimer at any time. Users must completely delete the downloaded content from their computers or phones within 24 hours of downloading, and any form of profit chain generation is strictly prohibited.
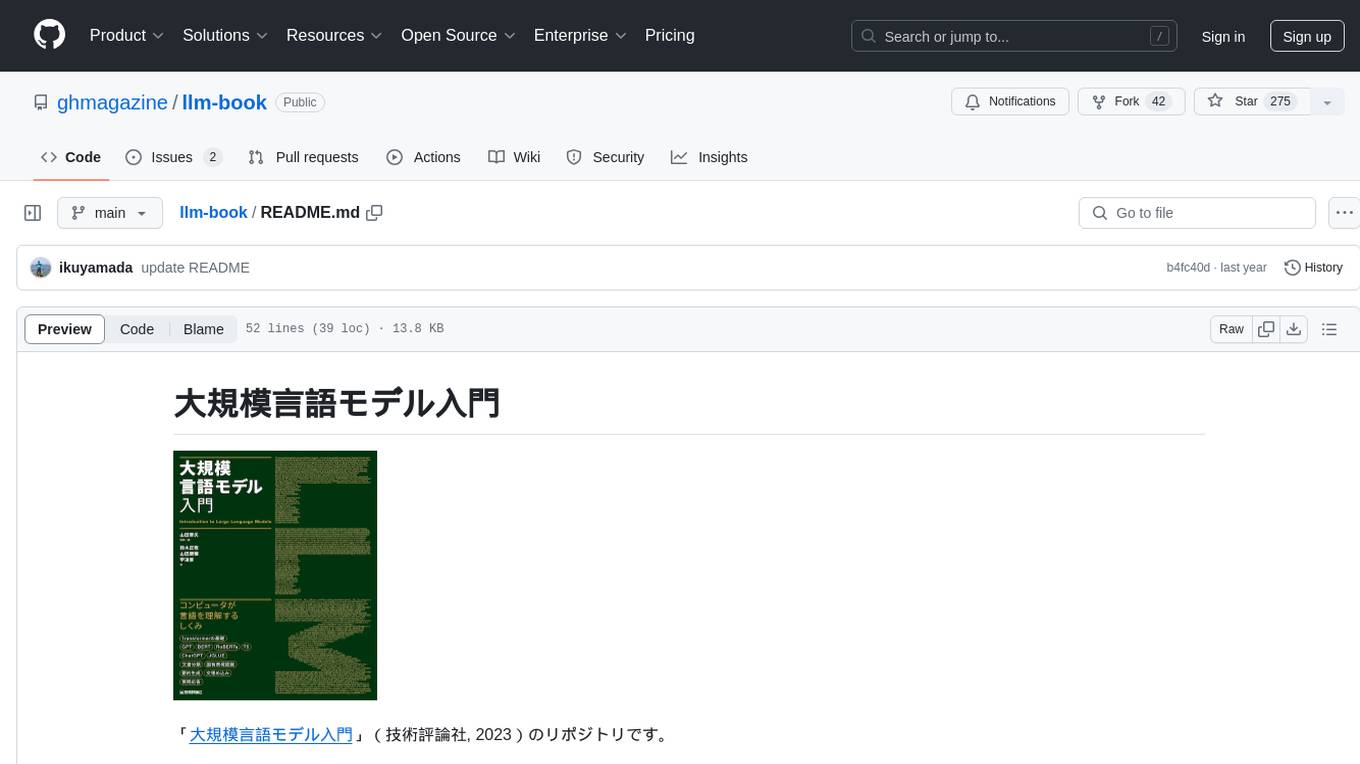
llm-book
The 'llm-book' repository is dedicated to the introduction of large-scale language models, focusing on natural language processing tasks. The code is designed to run on Google Colaboratory and utilizes datasets and models available on the Hugging Face Hub. Note that as of July 28, 2023, there are issues with the MARC-ja dataset links, but an alternative notebook using the WRIME Japanese sentiment analysis dataset has been added. The repository covers various chapters on topics such as Transformers, fine-tuning language models, entity recognition, summarization, document embedding, question answering, and more.
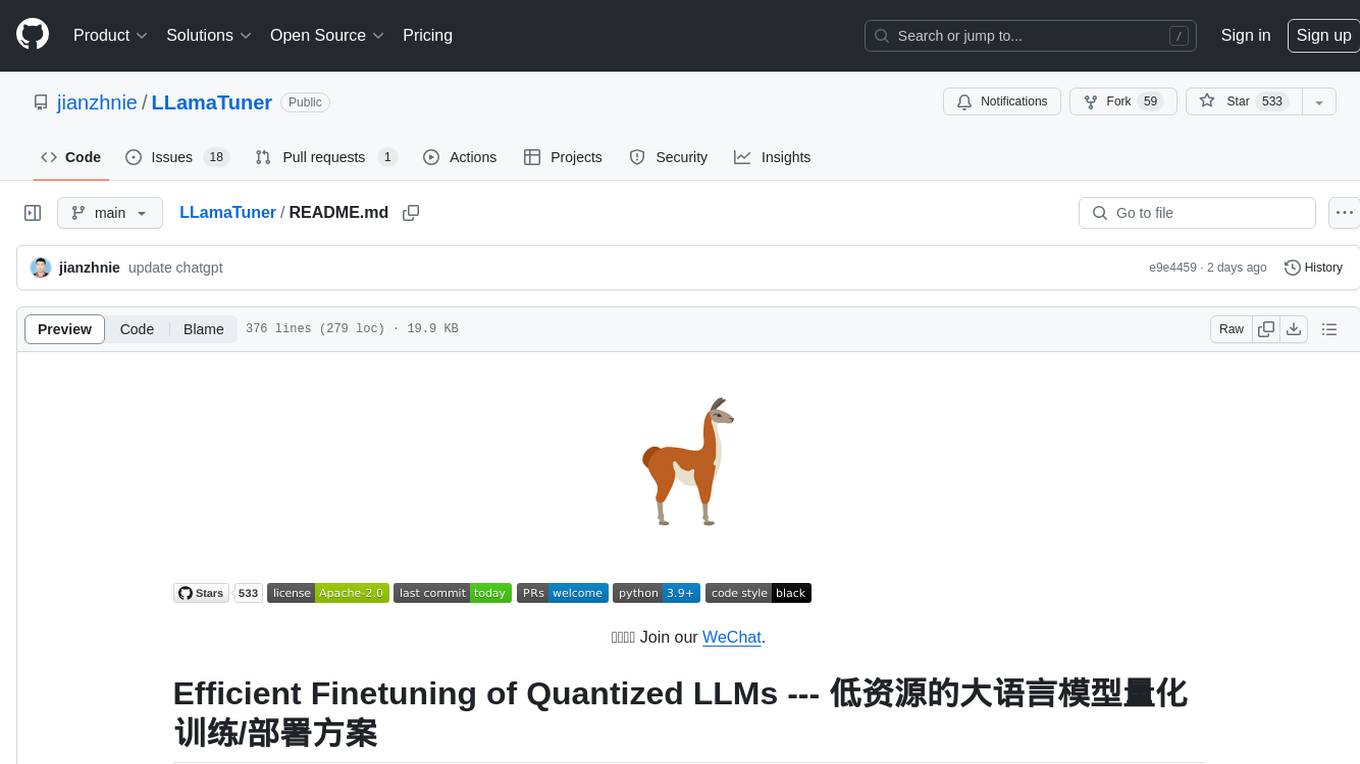
LLamaTuner
LLamaTuner is a repository for the Efficient Finetuning of Quantized LLMs project, focusing on building and sharing instruction-following Chinese baichuan-7b/LLaMA/Pythia/GLM model tuning methods. The project enables training on a single Nvidia RTX-2080TI and RTX-3090 for multi-round chatbot training. It utilizes bitsandbytes for quantization and is integrated with Huggingface's PEFT and transformers libraries. The repository supports various models, training approaches, and datasets for supervised fine-tuning, LoRA, QLoRA, and more. It also provides tools for data preprocessing and offers models in the Hugging Face model hub for inference and finetuning. The project is licensed under Apache 2.0 and acknowledges contributions from various open-source contributors.
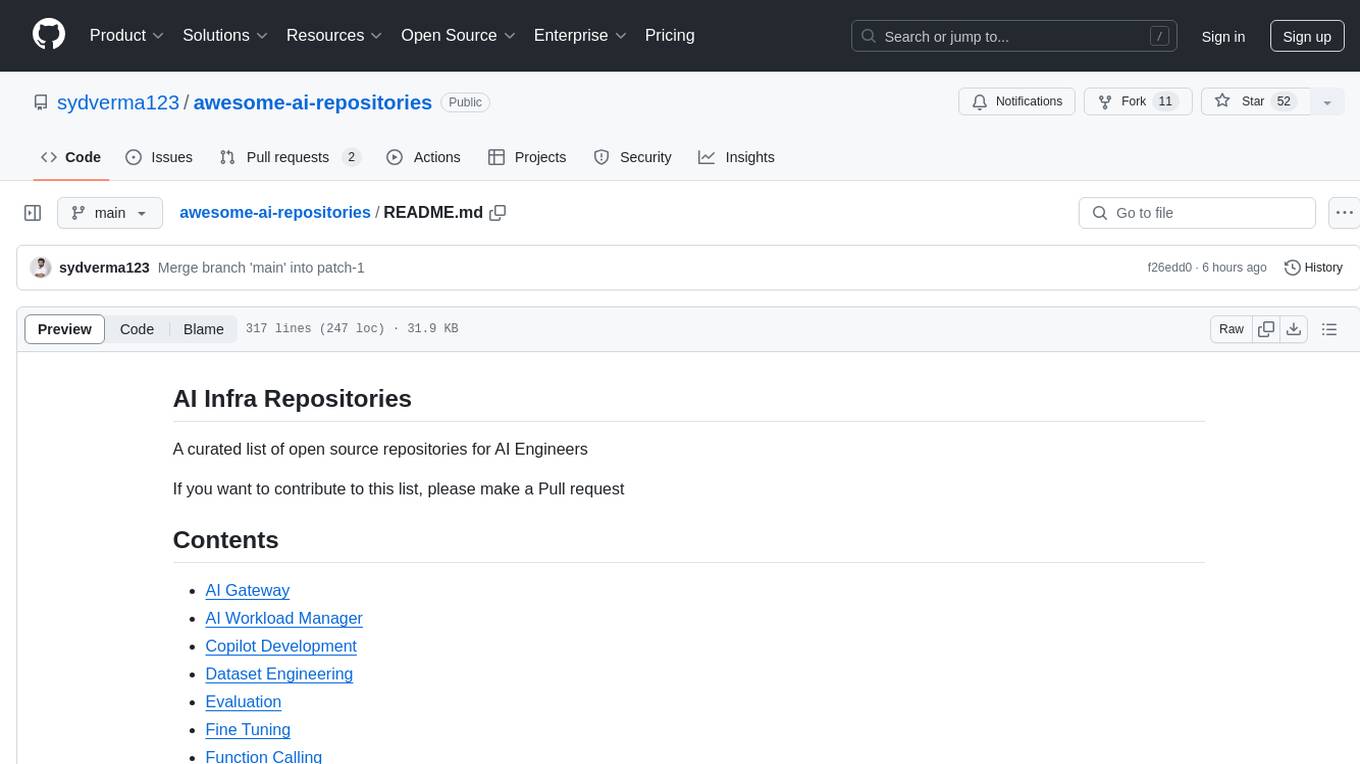
awesome-ai-repositories
A curated list of open source repositories for AI Engineers. The repository provides a comprehensive collection of tools and frameworks for various AI-related tasks such as AI Gateway, AI Workload Manager, Copilot Development, Dataset Engineering, Evaluation, Fine Tuning, Function Calling, Graph RAG, Guardrails, Local Model Inference, LLM Agent Framework, Model Serving, Observability, Pre Training, Prompt Engineering, RAG Framework, Security, Structured Extraction, Structured Generation, Vector DB, and Voice Agent.
For similar tasks
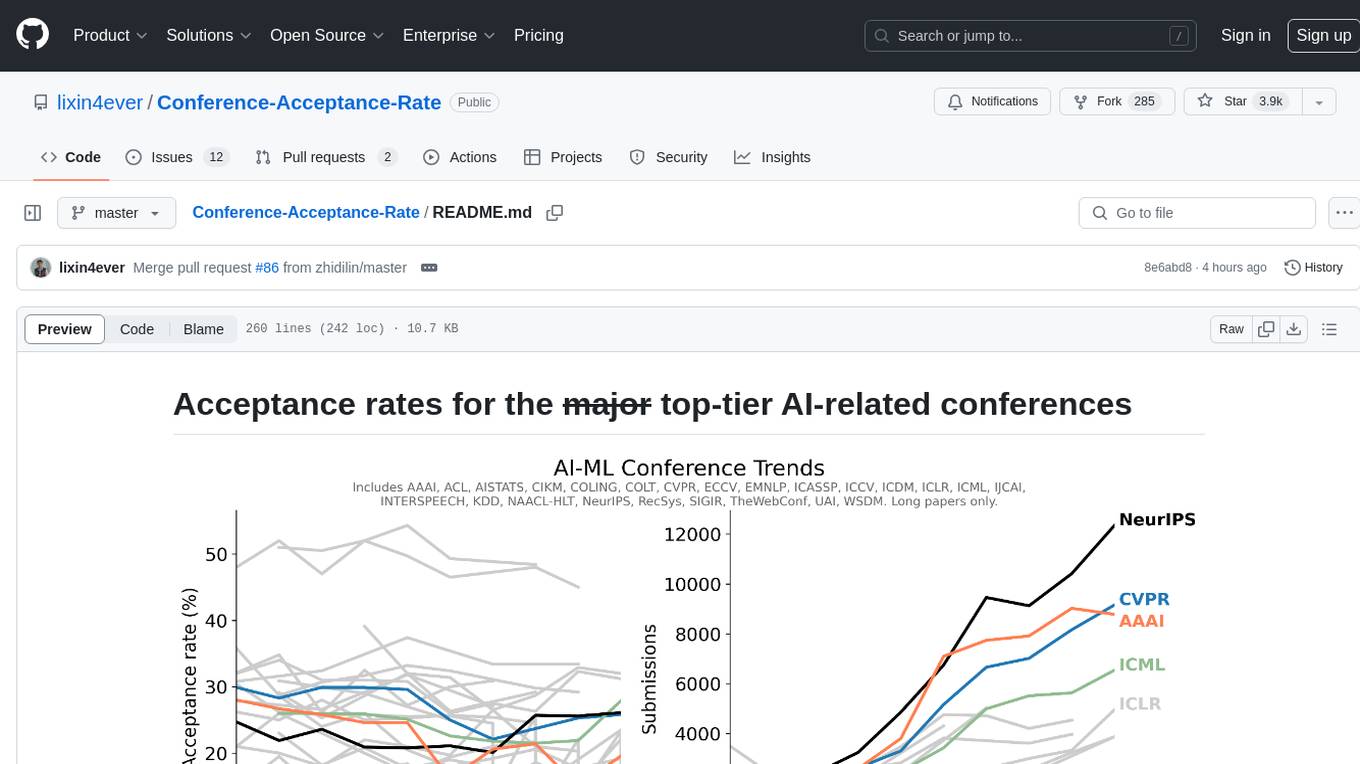
Conference-Acceptance-Rate
The 'Conference-Acceptance-Rate' repository provides acceptance rates for top-tier AI-related conferences in the fields of Natural Language Processing, Computational Linguistics, Computer Vision, Pattern Recognition, Machine Learning, Learning Theory, Artificial Intelligence, Data Mining, Information Retrieval, Speech Processing, and Signal Processing. The data includes acceptance rates for long papers and short papers over several years for each conference, allowing researchers to track trends and make informed decisions about where to submit their work.
For similar jobs
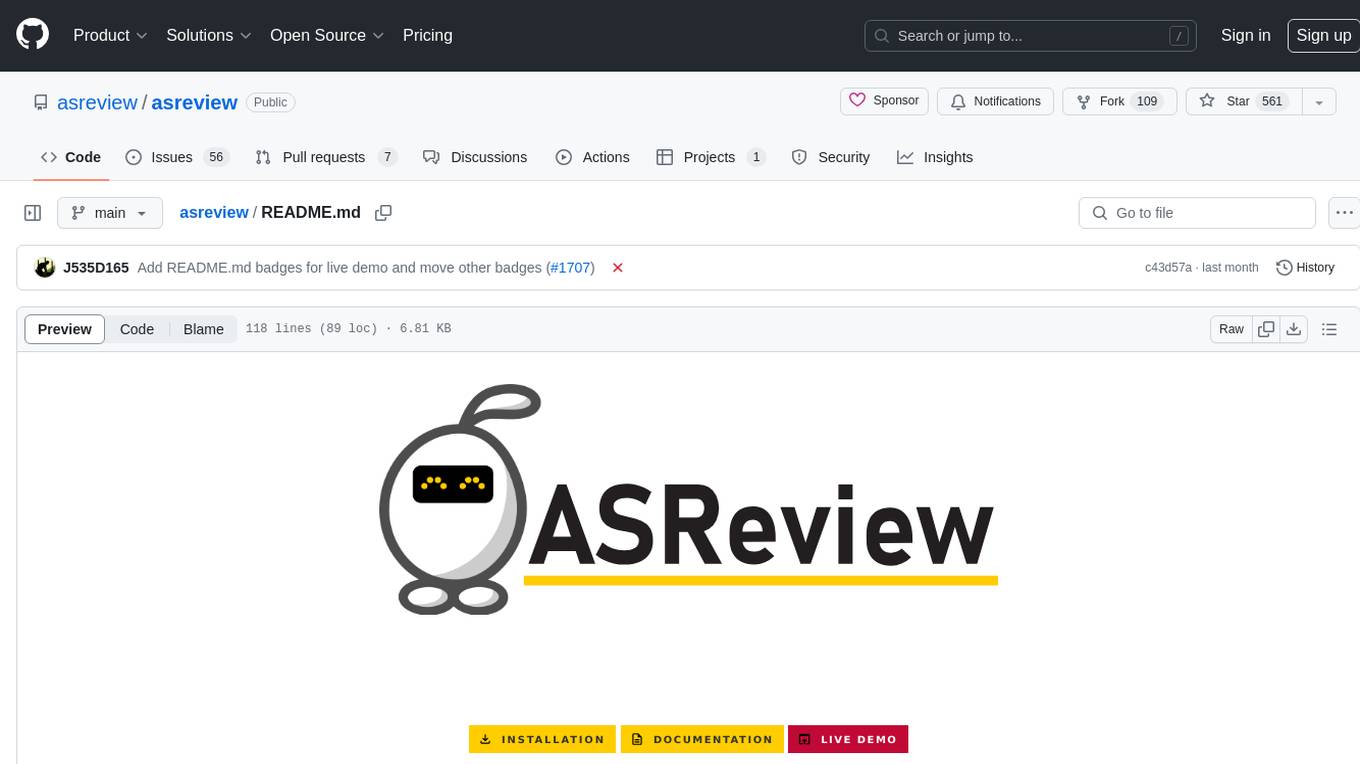
asreview
The ASReview project implements active learning for systematic reviews, utilizing AI-aided pipelines to assist in finding relevant texts for search tasks. It accelerates the screening of textual data with minimal human input, saving time and increasing output quality. The software offers three modes: Oracle for interactive screening, Exploration for teaching purposes, and Simulation for evaluating active learning models. ASReview LAB is designed to support decision-making in any discipline or industry by improving efficiency and transparency in screening large amounts of textual data.
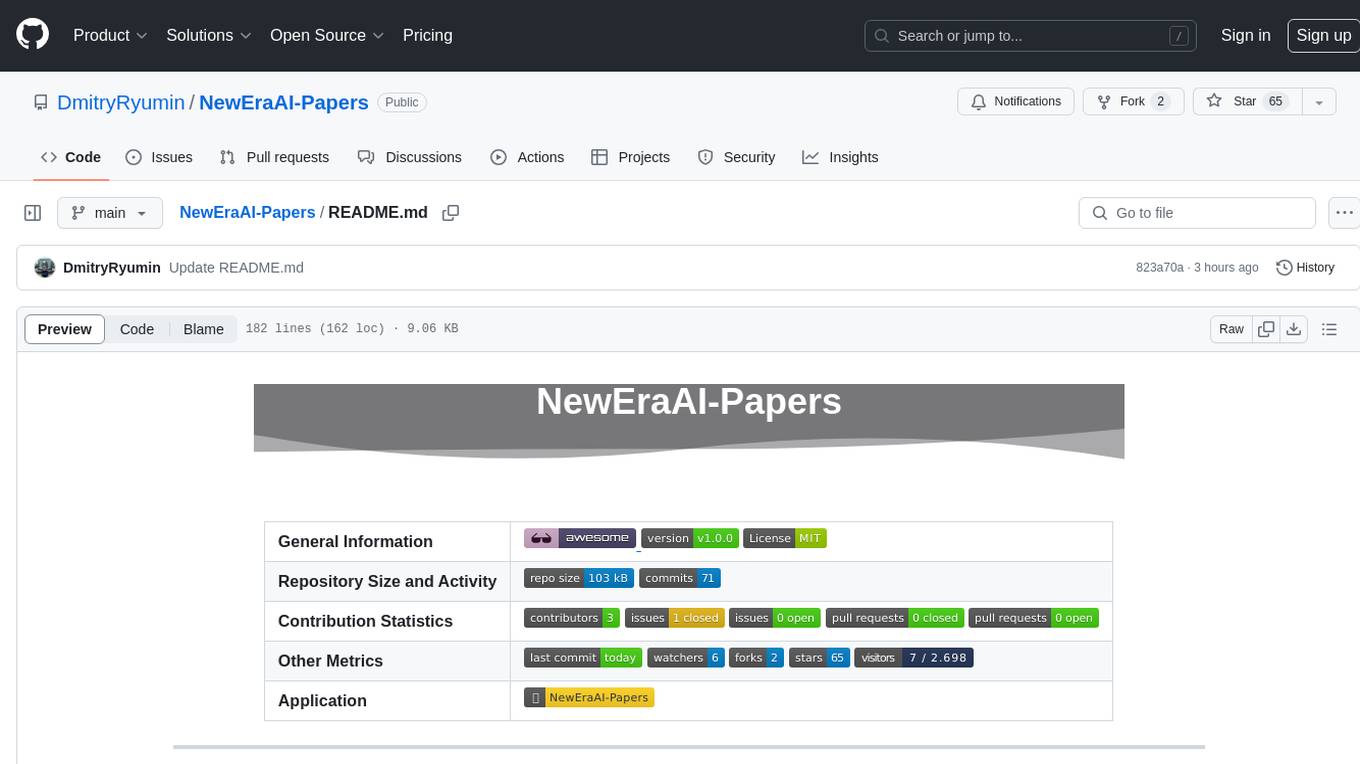
NewEraAI-Papers
The NewEraAI-Papers repository provides links to collections of influential and interesting research papers from top AI conferences, along with open-source code to promote reproducibility and provide detailed implementation insights beyond the scope of the article. Users can stay up to date with the latest advances in AI research by exploring this repository. Contributions to improve the completeness of the list are welcomed, and users can create pull requests, open issues, or contact the repository owner via email to enhance the repository further.
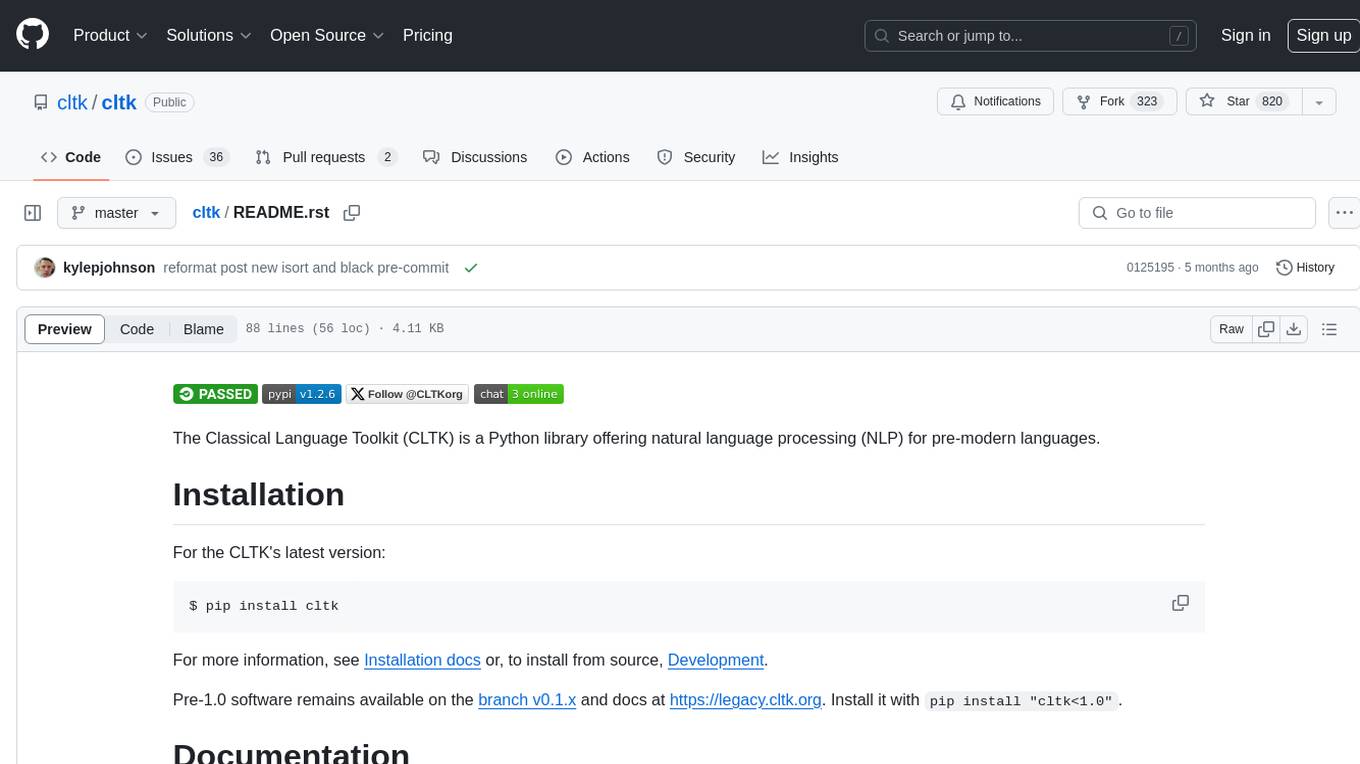
cltk
The Classical Language Toolkit (CLTK) is a Python library that provides natural language processing (NLP) capabilities for pre-modern languages. It offers a modular processing pipeline with pre-configured defaults and supports almost 20 languages. Users can install the latest version using pip and access detailed documentation on the official website. The toolkit is designed to meet the unique needs of researchers working with historical languages, filling a void in the NLP landscape that often neglects non-spoken languages and different research goals.
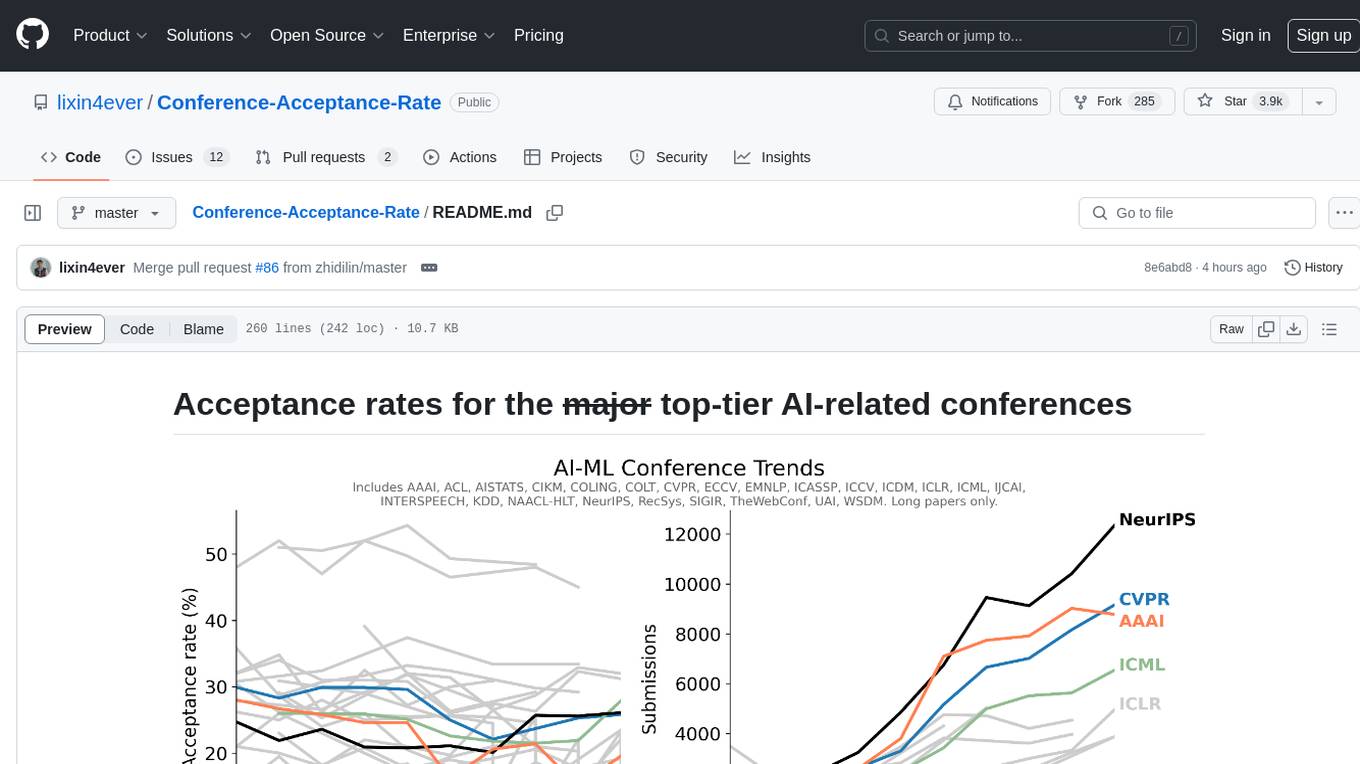
Conference-Acceptance-Rate
The 'Conference-Acceptance-Rate' repository provides acceptance rates for top-tier AI-related conferences in the fields of Natural Language Processing, Computational Linguistics, Computer Vision, Pattern Recognition, Machine Learning, Learning Theory, Artificial Intelligence, Data Mining, Information Retrieval, Speech Processing, and Signal Processing. The data includes acceptance rates for long papers and short papers over several years for each conference, allowing researchers to track trends and make informed decisions about where to submit their work.
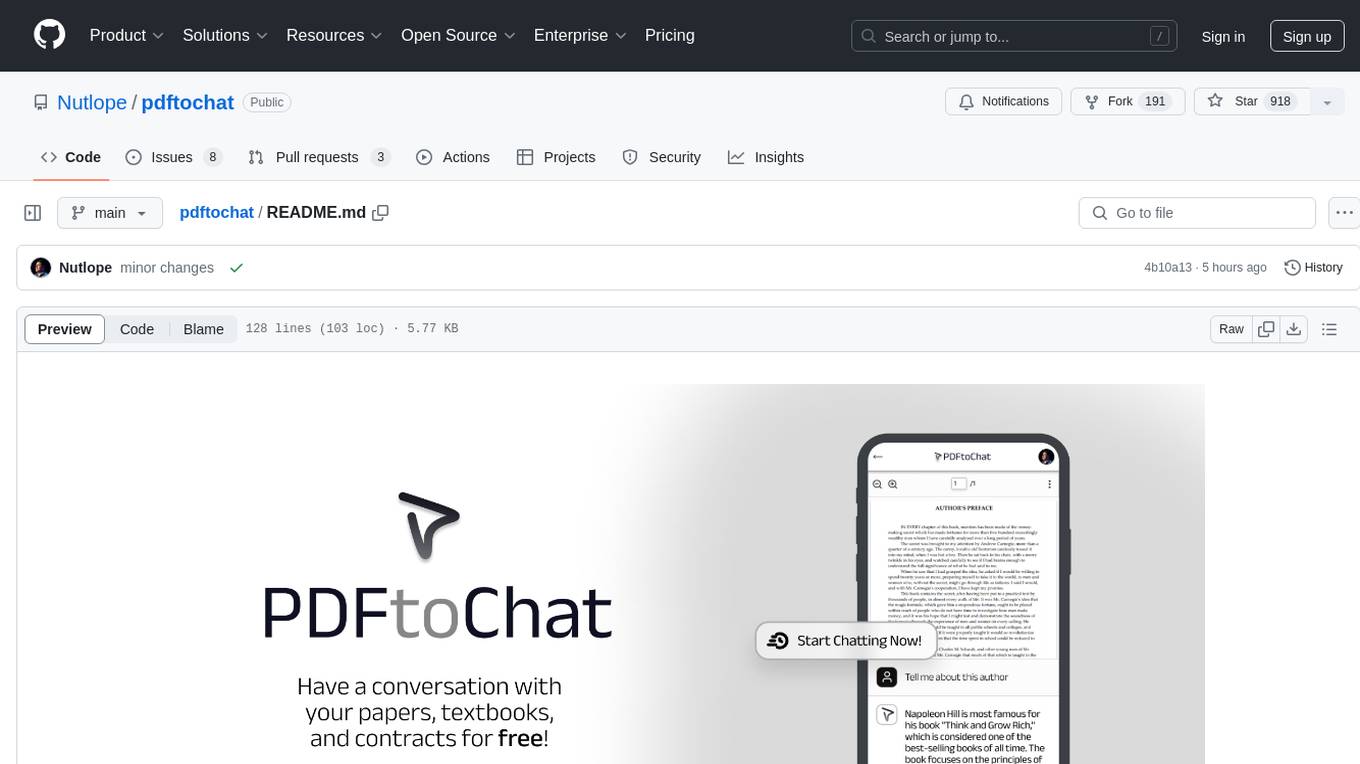
pdftochat
PDFToChat is a tool that allows users to chat with their PDF documents in seconds. It is powered by Together AI and Pinecone, utilizing a tech stack including Next.js, Mixtral, M2 Bert, LangChain.js, MongoDB Atlas, Bytescale, Vercel, Clerk, and Tailwind CSS. Users can deploy the tool to Vercel or any other host by setting up Together.ai, MongoDB Atlas database, Bytescale, Clerk, and Vercel. The tool enables users to interact with PDFs through chat, with future tasks including adding features like trash icon for deleting PDFs, exploring different embedding models, implementing auto scrolling, improving replies, benchmarking accuracy, researching chunking and retrieval best practices, adding demo video, upgrading to Next.js 14, adding analytics, customizing tailwind prose, saving chats in postgres DB, compressing large PDFs, implementing custom uploader, session tracking, error handling, and support for images in PDFs.
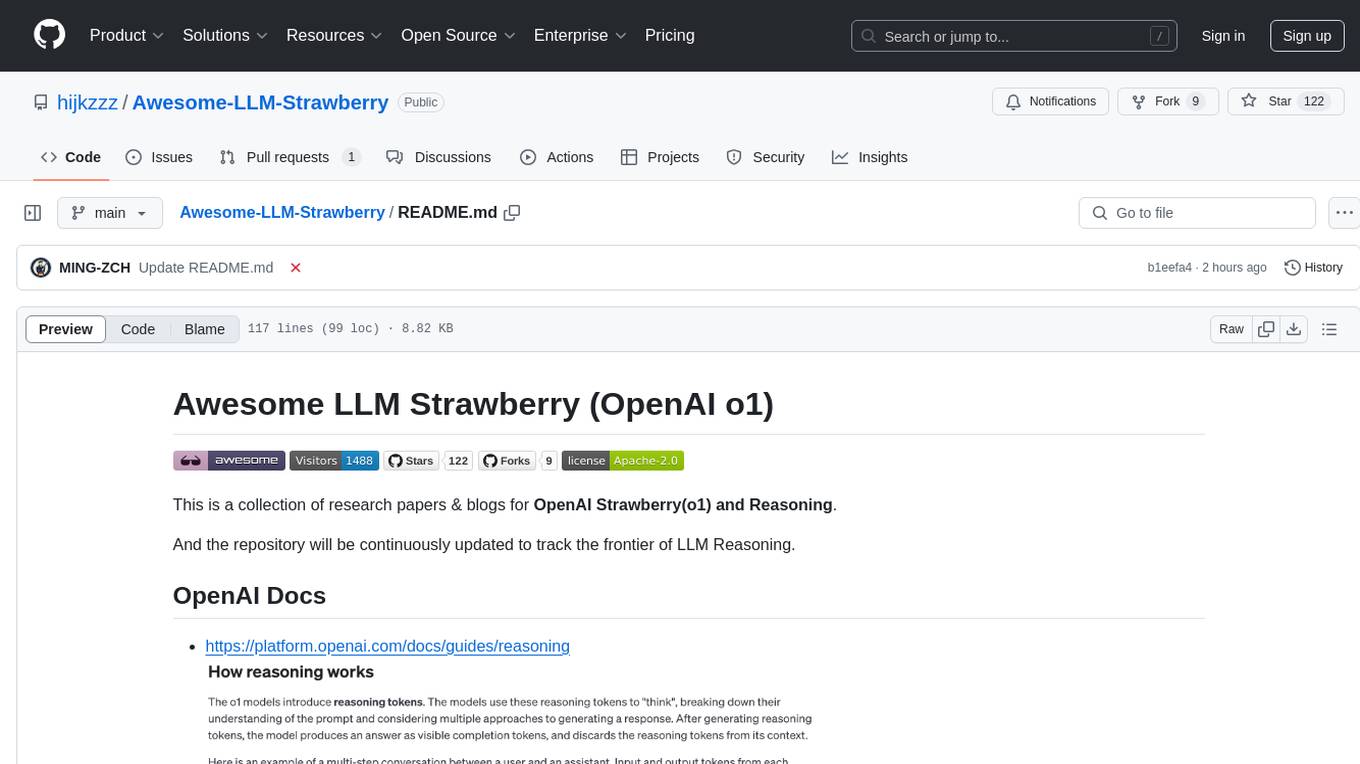
Awesome-LLM-Strawberry
Awesome LLM Strawberry is a collection of research papers and blogs related to OpenAI Strawberry(o1) and Reasoning. The repository is continuously updated to track the frontier of LLM Reasoning.
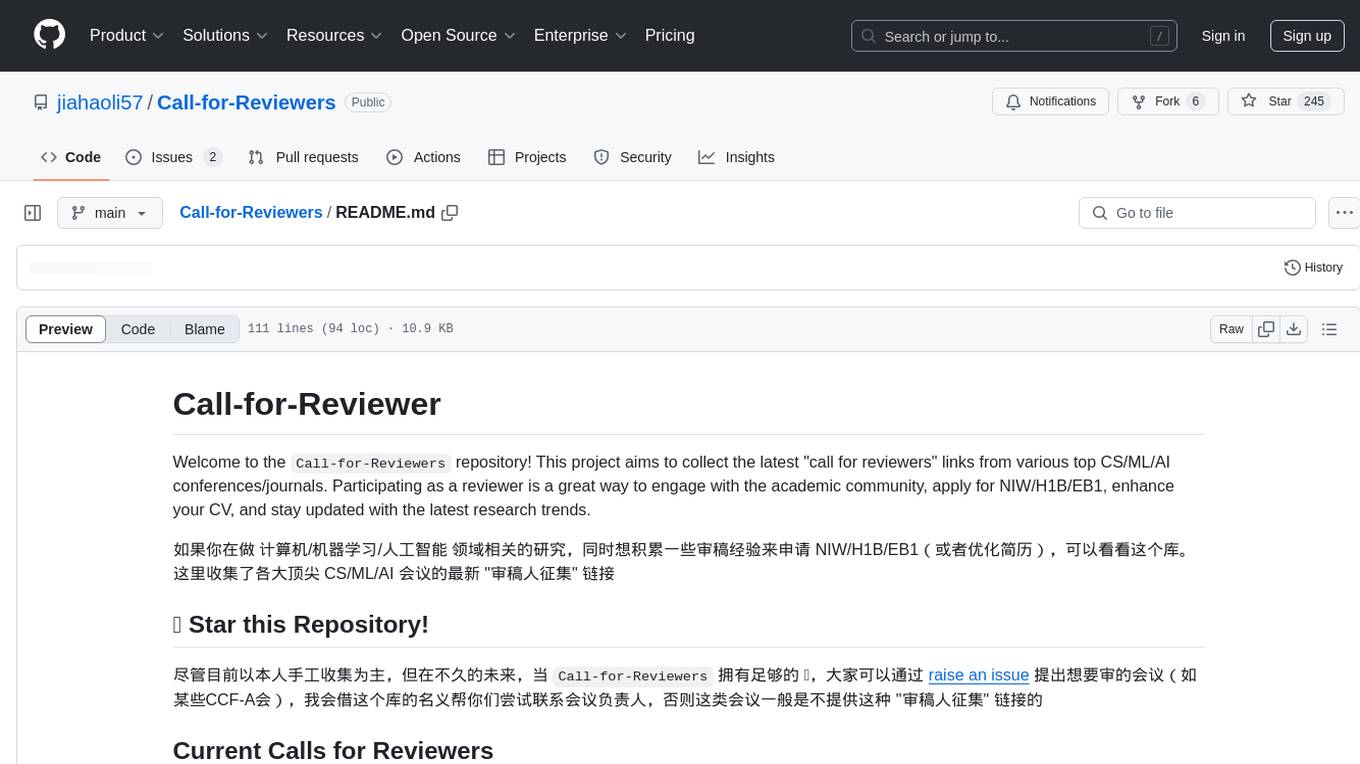
Call-for-Reviewers
The `Call-for-Reviewers` repository aims to collect the latest 'call for reviewers' links from various top CS/ML/AI conferences/journals. It provides an opportunity for individuals in the computer/ machine learning/ artificial intelligence fields to gain review experience for applying for NIW/H1B/EB1 or enhancing their CV. The repository helps users stay updated with the latest research trends and engage with the academic community.
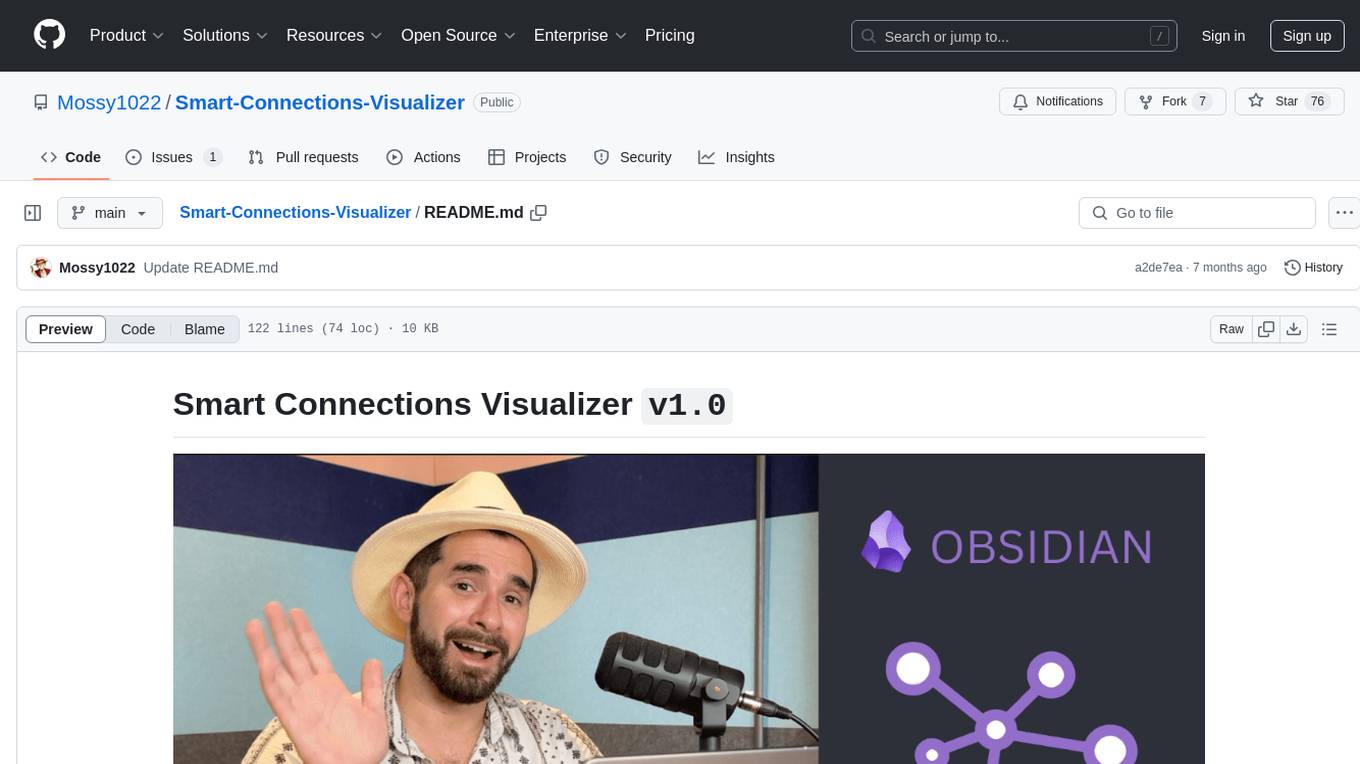
Smart-Connections-Visualizer
The Smart Connections Visualizer Plugin is a tool designed to enhance note-taking and information visualization by creating dynamic force-directed graphs that represent connections between notes or excerpts. Users can customize visualization settings, preview notes, and interact with the graph to explore relationships and insights within their notes. The plugin aims to revolutionize communication with AI and improve decision-making processes by visualizing complex information in a more intuitive and context-driven manner.