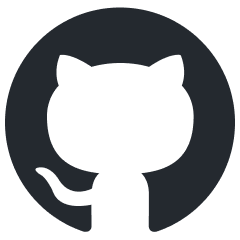
Agently
[AI Agent Application Development Framework] - 🚀 Build AI agent native application in very few code 💬 Easy to interact with AI agent in code using structure data and chained-calls syntax 🧩 Enhance AI Agent using plugins instead of rebuild a whole new agent
Stars: 1096
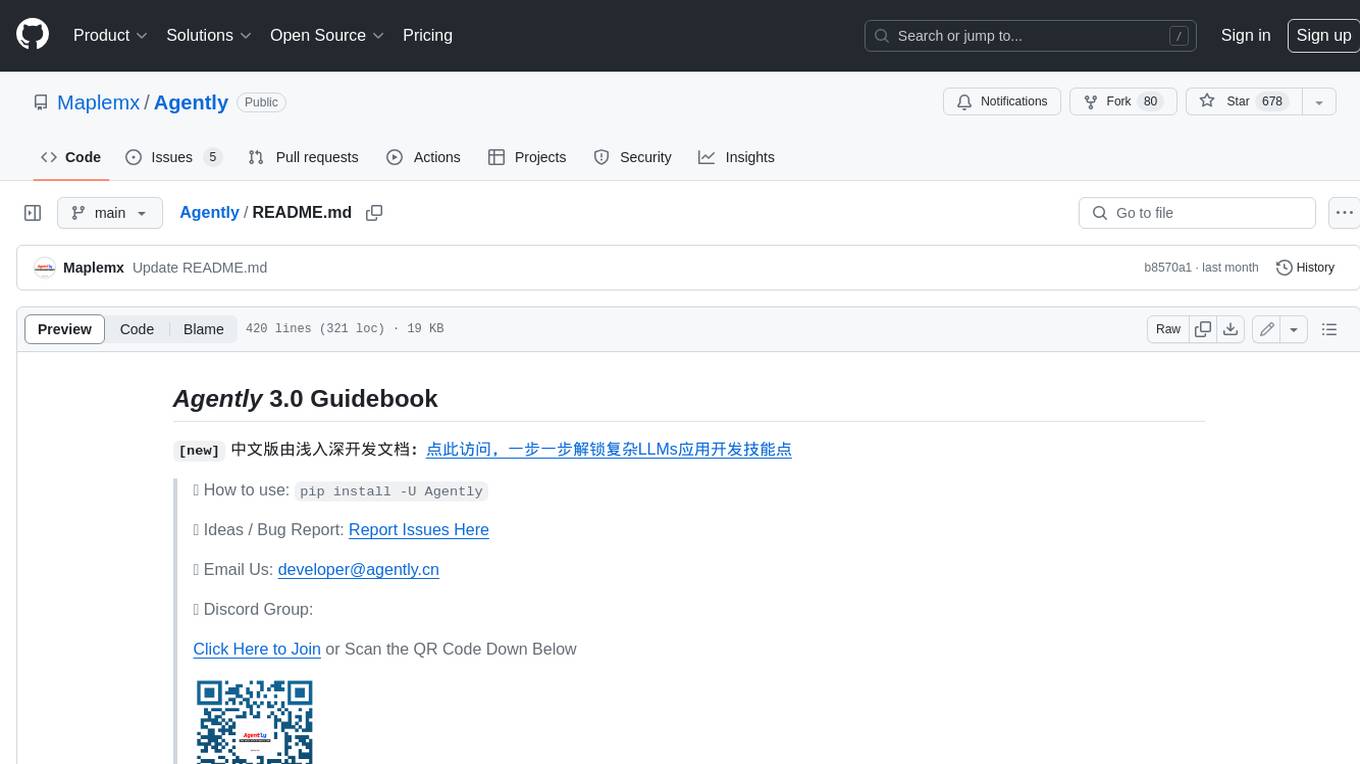
Agently is a development framework that helps developers build AI agent native application really fast. You can use and build AI agent in your code in an extremely simple way. You can create an AI agent instance then interact with it like calling a function in very few codes like this below. Click the run button below and witness the magic. It's just that simple: python # Import and Init Settings import Agently agent = Agently.create_agent() agent\ .set_settings("current_model", "OpenAI")\ .set_settings("model.OpenAI.auth", {"api_key": ""}) # Interact with the agent instance like calling a function result = agent\ .input("Give me 3 words")\ .output([("String", "one word")])\ .start() print(result) ['apple', 'banana', 'carrot'] And you may notice that when we print the value of `result`, the value is a `list` just like the format of parameter we put into the `.output()`. In Agently framework we've done a lot of work like this to make it easier for application developers to integrate Agent instances into their business code. This will allow application developers to focus on how to build their business logic instead of figure out how to cater to language models or how to keep models satisfied.
README:
[Important]
Agently AI开发框架中文首页改版已经完成,模型切换、AgenticRequest、Workflow全新教程文档全面更新,请访问:Agently.cn查看
[Important]
We'll rewrite repo homepage soon to tell you more about our recently work, please wait for it.
[Showcase Repo]Agently Daily News Collector: English | 新闻汇总报告生成器开源项目
[hot]
中文版由浅入深开发文档:点此访问,一步一步解锁复杂LLMs应用开发技能点
📥 How to use:
pip install -U Agently
💡 Ideas / Bug Report: Report Issues Here
📧 Email Us: [email protected]
👾 Discord Group:
Click Here to Join or Scan the QR Code Down Below
![]()
💬 WeChat Group(加入微信群):
Click Here to Apply or Scan the QR Code Down Below
![]()
If you like this project, please ⭐️, thanks.
Colab Documents:
- Introduction Guidebook
- Application Development Handbook
- Plugin Development Handbook(still working on it)
Code Examples:
To build agent in many different fields:
- Agent Instance Help You to Generate SQL from Natural Language according Meta Data of Database
- Survey Agent Asks Questions and Collecting Feedback from Customer According Form
- Teacher Agent for Kids with Search Ability
Or, to call agent instance abilities in code logic to help:
- Transform Long Text to Question & Answer Pairs
- Human Review and Maybe Step In before Response is Sent to User
- Simple Example for How to Call Functions
Explore More: Visit Demostration Playground
Install Agently Python Package:
pip install -U Agently
Then we are ready to go!
Agently is a development framework that helps developers build AI agent native application really fast.
You can use and build AI agent in your code in an extremely simple way.
You can create an AI agent instance then interact with it like calling a function in very few codes like this below.
Click the run button below and witness the magic. It's just that simple:
# Import and Init Settings
import Agently
agent = Agently.create_agent()
agent\
.set_settings("current_model", "OpenAI")\
.set_settings("model.OpenAI.auth", { "api_key": "" })
# Interact with the agent instance like calling a function
result = agent\
.input("Give me 3 words")\
.output([("String", "one word")])\
.start()
print(result)
['apple', 'banana', 'carrot']
And you may notice that when we print the value of result
, the value is a list
just like the format of parameter we put into the .output()
.
In Agently framework we've done a lot of work like this to make it easier for application developers to integrate Agent instances into their business code. This will allow application developers to focus on how to build their business logic instead of figure out how to cater to language models or how to keep models satisfied.
When we start using AI agent in code to help us handle business logic, we can easily sence that there must be some differences from the traditional software develop way. But what're the differences exactly?
I think the key point is to use an AI agent to solve the problem instead of man-made code logic.
In AI agent native application, we put an AI agent instance into our code, then we ask it to execute / to solve the problem with natural language or natural-language-like expressions.
"Ask-Get Response" takes place of traditional "Define Problem - Programme - Code to Make It Happen".
Can that be true and as easy as we say?
Sure! Agently framework provide the easy way to interact with AI agent instance will make application module development quick and easy.
Here down below are two CLI application demos that in two totally different domains but both be built by 64 lines of codes powered by Agently.
DEMO VIDEO
https://github.com/Maplemx/Agently/assets/4413155/b7d16592-5fdc-43c0-a14c-f2272c7900da
CODE
import Agently
agent_factory = Agently.AgentFactory(is_debug = False)
agent_factory\
.set_settings("current_model", "OpenAI")\
.set_settings("model.OpenAI.auth", { "api_key": "" })
agent = agent_factory.create_agent()
meta_data = {
"table_meta" : [
{
"table_name": "user",
"columns": [
{ "column_name": "user_id", "desc": "identity of user", "value type": "Number" },
{ "column_name": "gender", "desc": "gender of user", "value type": ["male", "female"] },
{ "column_name": "age", "desc": "age of user", "value type": "Number" },
{ "column_name": "customer_level", "desc": "level of customer account", "value type": [1,2,3,4,5] },
]
},
{
"table_name": "order",
"columns": [
{ "column_name": "order_id", "desc": "identity of order", "value type": "Number" },
{ "column_name": "customer_user_id", "desc": "identity of customer, same value as user_id", "value type": "Number" },
{ "column_name": "item_name", "desc": "item name of this order", "value type": "String" },
{ "column_name": "item_number", "desc": "how many items to buy in this order", "value type": "Number" },
{ "column_name": "price", "desc": "how much of each item", "value type": "Number" },
{ "column_name": "date", "desc": "what date did this order happend", "value type": "Date" },
]
},
]
}
is_finish = False
while not is_finish:
question = input("What do you want to know: ")
show_thinking = None
while str(show_thinking).lower() not in ("y", "n"):
show_thinking = input("Do you want to observe the thinking process? [Y/N]: ")
show_thinking = False if show_thinking.lower() == "n" else True
print("[Generating...]")
result = agent\
.input({
"table_meta": meta_data["table_meta"],
"question": question
})\
.instruct([
"output SQL to query the database according meta data:{table_meta} that can anwser the question:{question}",
"output language: English",
])\
.output({
"thinkings": ["String", "Your problem solving thinking step by step"],
"SQL": ("String", "final SQL only"),
})\
.start()
if show_thinking:
thinking_process = "\n".join(result["thinkings"])
print("[Thinking Process]\n", thinking_process)
print("[SQL]\n", result["SQL"])
while str(is_finish).lower() not in ("y", "n"):
is_finish = input("Do you want to quit?[Y to quit / N to continue]: ")
is_finish = False if is_finish.lower() == "n" else True
import Agently
agent_factory = Agently.AgentFactory(is_debug = False)
agent_factory\
.set_settings("current_model", "OpenAI")\
.set_settings("model.OpenAI.auth", { "api_key": "" })
writer_agent = agent_factory.create_agent()
roleplay_agent = agent_factory.create_agent()
# Create Character
character_desc = input("Describe the character you want to talk to with a few words: ")
is_accepted = ""
suggestions = ""
last_time_character_setting = {}
while is_accepted.lower() != "y":
is_accepted = ""
input_dict = { "character_desc": character_desc }
if suggestions != "":
input_dict.update({ "suggestions": suggestions })
input_dict.update({ "last_time_character_setting": last_time_character_setting })
setting_result = writer_agent\
.input(input_dict)\
.instruct([
"Design a character based on {input.character_desc}.",
"if {input.suggestions} exist, rewrite {input.last_time_character_setting} followed {input.suggestions}."
])\
.output({
"name": ("String",),
"age": ("Number",),
"character": ("String", "Descriptions about the role of this character, the actions he/she likes to take, his/her behaviour habbits, etc."),
"belief": ("String", "Belief or mottos of this character"),
"background_story": [("String", "one part of background story of this character")],
"response_examples": [{ "Question": ("String", "question that user may ask this character"), "Response": ("String", "short and quick response that this character will say.") }],
})\
.on_delta(lambda data: print(data, end=""))\
.start()
while is_accepted.lower() not in ("y", "n"):
is_accepted = input("Are you satisfied with this character role setting? [Y/N]: ")
if is_accepted.lower() == "n":
suggestions = input("Do you have some suggestions about this setting? (leave this empty will redo all the setting): ")
if suggestions != "":
last_time_character_settings = setting_result
print("[Start Loading Character Setting to Agent...]")
# Load Character to Agent then Chat with It
for key, value in setting_result.items():
roleplay_agent.set_role(key, value)
print("[Loading is Done. Let's Start Chatting](input '#exit' to quit)")
roleplay_agent.active_session()
chat_input = ""
while True:
chat_input = input("YOU: ")
if chat_input == "#exit":
break
print(f"{ setting_result['name'] }: ", end="")
roleplay_agent\
.input(chat_input)\
.instruct("Response {chat_input} follow your {ROLE} settings. Response like in a CHAT not a query or request!")\
.on_delta(lambda data: print(data, end=""))\
.start()
print("")
print("Bye👋~")
The post about LLM Powered Autonomous Agents by Lilian Weng from OpenAI has given a really good concept of the basic structure of AI agent. But the post did not give the explanation about how to build an AI agent.
Some awesome projects like LangChain and Camel-AI present their ideas about how to build AI agent. In these projects, agents are classified into many different type according the task of the agent or the thinking process of the agent.
But if we follow these ideas to build agents, that means we must build a whole new agent if we want to have a new agent to work in a different domain. Even though all the projects provide a ChatAgent basic class or something like that, still new agent sub-classes will be built and more and more specific types of agent will be produce. With the number of agent types increasing, one day, boom! There'll be too many types of agent for developer to choose and for agent platform to manage. They'll be hard to seach, hard to choose, hard to manage and hard to update.
So Agently team can not stop wondering if there's a better way to enhance agent and make all developers easy to participate in.
Also, AI agent's structure and components seems simple and easy to build at present. But if we look further ahead, each component shall be more complex (memory management for example) and more and more new components will be added in (sencors for example).
What if we stop building agent like an undivded whole but to seperate it into a center structure which is managing the runtime context data and runtime process and connect wiht different plugins to enhance its abilities in the runtime process to make it suitable for different usage scene? "Divide and conquer", just like the famous engineering motto said.
We make it happened in Agently 3.0 and when Agently 3.0 in its alpha test, we were happy to see this plugin-to-enhance design not only solved the problem about rebuild a whole new agent, but also helped each component developers focuing on the target and questions only that component care about without distraction. That makes component plugin develop really easy and code simple.
Here's an example that shows how to develop an agent component plugin in Agently framework. Because of the runtime context data management work has been done by the framework, plugin developers can use many runtime tools to help building the agent component plugin. That makes the work pretty easy.
⚠️ : The code below is an plugin code example, it works in the framework and can not be run seperately.
from .utils import ComponentABC
from Agently.utils import RuntimeCtxNamespace
# Create Plugin Class comply with Abstract Basic Class
class Role(ComponentABC):
def __init__(self, agent: object):
self.agent = agent
# Framework pass runtime_ctx and storage through and component can use them
self.role_runtime_ctx = RuntimeCtxNamespace("role", self.agent.agent_runtime_ctx)
self.role_storage = self.agent.global_storage.table("role")
# Defined methods of this component
# Update runtime_ctx which follow the agent instance lifetime circle
def set_name(self, name: str, *, target: str):
self.role_runtime_ctx.set("NAME", name)
return self.agent
def set(self, key: any, value: any=None, *, target: str):
if value is not None:
self.role_runtime_ctx.set(key, value)
else:
self.role_runtime_ctx.set("DESC", key)
return self.agent
def update(self, key: any, value: any=None, *, target: str):
if value is not None:
self.role_runtime_ctx.update(key, value)
else:
self.role_runtime_ctx.update("DESC", key)
return self.agent
def append(self, key: any, value: any=None, *, target: str):
if value is not None:
self.role_runtime_ctx.append(key, value)
else:
self.role_runtime_ctx.append("DESC", key)
return self.agent
def extend(self, key: any, value: any=None, *, target: str):
if value is not None:
self.role_runtime_ctx.extend(key, value)
else:
self.role_runtime_ctx.extend("DESC", key)
return self.agent
# Or save to / load from storage which keep the data in file storage or database
def save(self, role_name: str=None):
if role_name == None:
role_name = self.role_runtime_ctx.get("NAME")
if role_name != None and role_name != "":
self.role_storage\
.set(role_name, self.role_runtime_ctx.get())\
.save()
return self.agent
else:
raise Exception("[Agent Component: Role] Role attr 'NAME' must be stated before save. Use .set_role_name() to specific that.")
def load(self, role_name: str):
role_data = self.role_storage.get(role_name)
for key, value in role_data.items():
self.role_runtime_ctx.update(key, value)
return self.agent
# Pass the data to request standard slots on Prefix Stage
def _prefix(self):
return {
"role": self.role_runtime_ctx.get(),
}
# Export component plugin interface to be called in agent runtime process
def export(self):
return {
"early": None, # method to be called on Early Stage
"prefix": self._prefix, # method to be called on Prefix Stage
"suffix": None, # mothod to be called on Suffix Stage
# Alias that application developers can use in agent instance
# Example:
# "alias": { "set_role_name": { "func": self.set_name } }
# => agent.set_role_name("Alice")
"alias": {
"set_role_name": { "func": self.set_name },
"set_role": { "func": self.set },
"update_role": { "func": self.update },
"append_role": { "func": self.append },
"extend_role": { "func": self.extend },
"save_role": { "func": self.save },
"load_role": { "func": self.load },
},
}
# Export to Plugins Dir Auto Scaner
def export():
return ("Role", Role)
Agently framework also allows plugin developers pack their plugin outside the main package of framework and share their plugin package individually to other developers. Developers those who want to use a specific plugin can just download the plugin package, unpack the files into their working folder, then install the plugin easily.
These codes down below will present how easy this installation can be.
⚠️ : The code below is an plugin install example, it only works when you unpack an plugin folder in your working folder.
import Agently
# Import install method from plugin folder
from session_plugin import install
# Then install
install(Agently)
# That's all
# Now your agent can use new abilities enhanced by new plugin
Here's also a real case when Agently v3.0.1 had an issue that make Session component unavailable. We use plugin package update can fix the bug without update the whole framework package.
OK. That's the general introduction about Agently AI agent development framework.
If you want to dive deeper, you can also visit these documents/links:
- Agently 3.0 Application Development Handbook
- Agently 3.0 Plugin Development Handbook (Working on it)
- Agently 3.0 Demostration Playground
Don't forget ⭐️ this repo if you like our work.
Thanks and happy coding!
For Tasks:
Click tags to check more tools for each tasksFor Jobs:
Alternative AI tools for Agently
Similar Open Source Tools
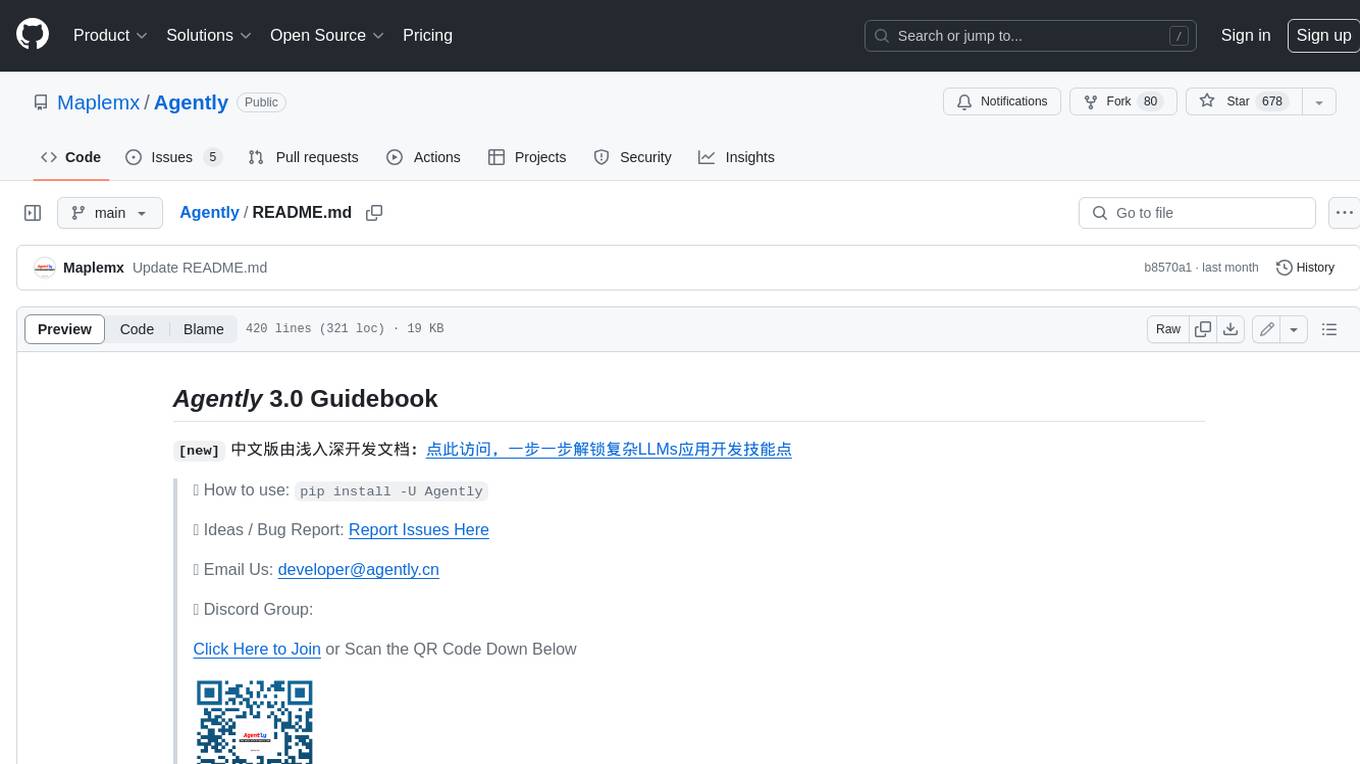
Agently
Agently is a development framework that helps developers build AI agent native application really fast. You can use and build AI agent in your code in an extremely simple way. You can create an AI agent instance then interact with it like calling a function in very few codes like this below. Click the run button below and witness the magic. It's just that simple: python # Import and Init Settings import Agently agent = Agently.create_agent() agent\ .set_settings("current_model", "OpenAI")\ .set_settings("model.OpenAI.auth", {"api_key": ""}) # Interact with the agent instance like calling a function result = agent\ .input("Give me 3 words")\ .output([("String", "one word")])\ .start() print(result) ['apple', 'banana', 'carrot'] And you may notice that when we print the value of `result`, the value is a `list` just like the format of parameter we put into the `.output()`. In Agently framework we've done a lot of work like this to make it easier for application developers to integrate Agent instances into their business code. This will allow application developers to focus on how to build their business logic instead of figure out how to cater to language models or how to keep models satisfied.
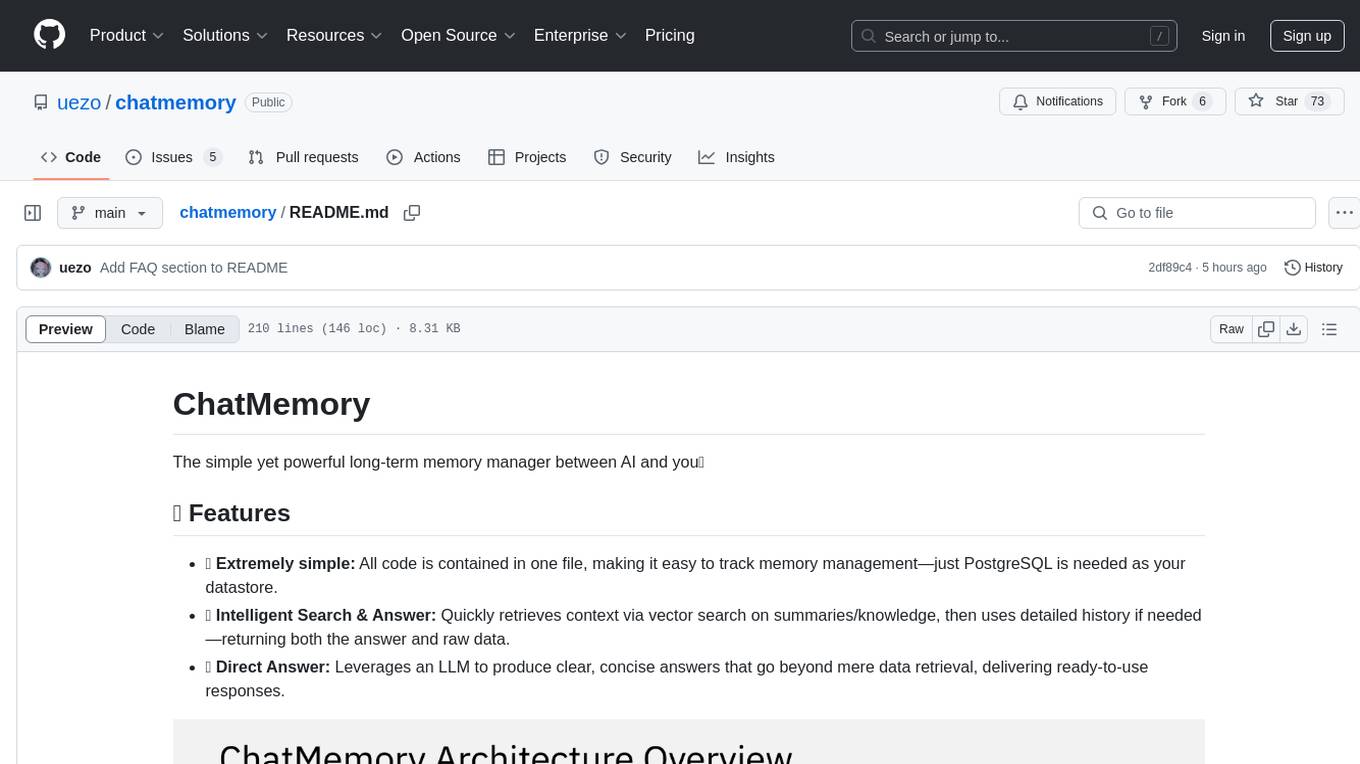
chatmemory
ChatMemory is a simple yet powerful long-term memory manager that facilitates communication between AI and users. It organizes conversation data into history, summary, and knowledge entities, enabling quick retrieval of context and generation of clear, concise answers. The tool leverages vector search on summaries/knowledge and detailed history to provide accurate responses. It balances speed and accuracy by using lightweight retrieval and fallback detailed search mechanisms, ensuring efficient memory management and response generation beyond mere data retrieval.
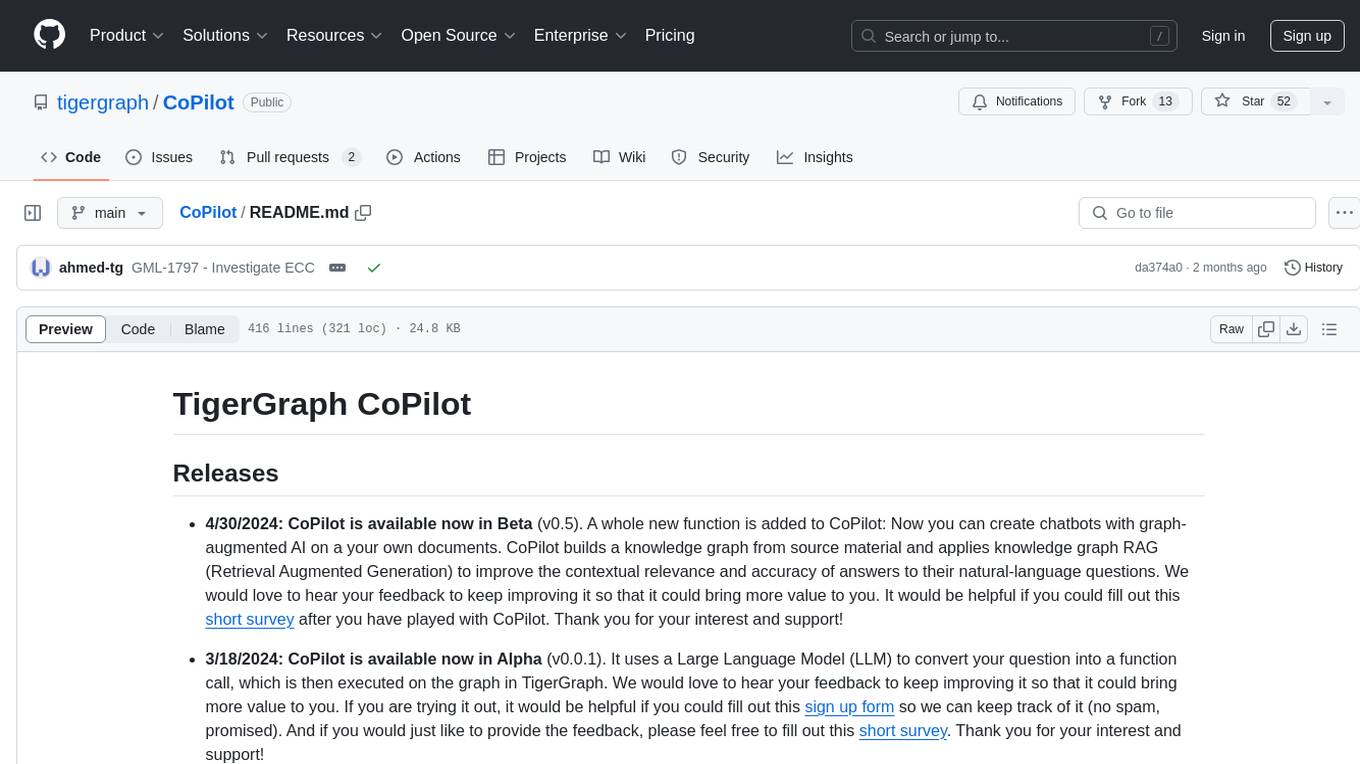
CoPilot
TigerGraph CoPilot is an AI assistant that combines graph databases and generative AI to enhance productivity across various business functions. It includes three core component services: InquiryAI for natural language assistance, SupportAI for knowledge Q&A, and QueryAI for GSQL code generation. Users can interact with CoPilot through a chat interface on TigerGraph Cloud and APIs. CoPilot requires LLM services for beta but will support TigerGraph's LLM in future releases. It aims to improve contextual relevance and accuracy of answers to natural-language questions by building knowledge graphs and using RAG. CoPilot is extensible and can be configured with different LLM providers, graph schemas, and LangChain tools.
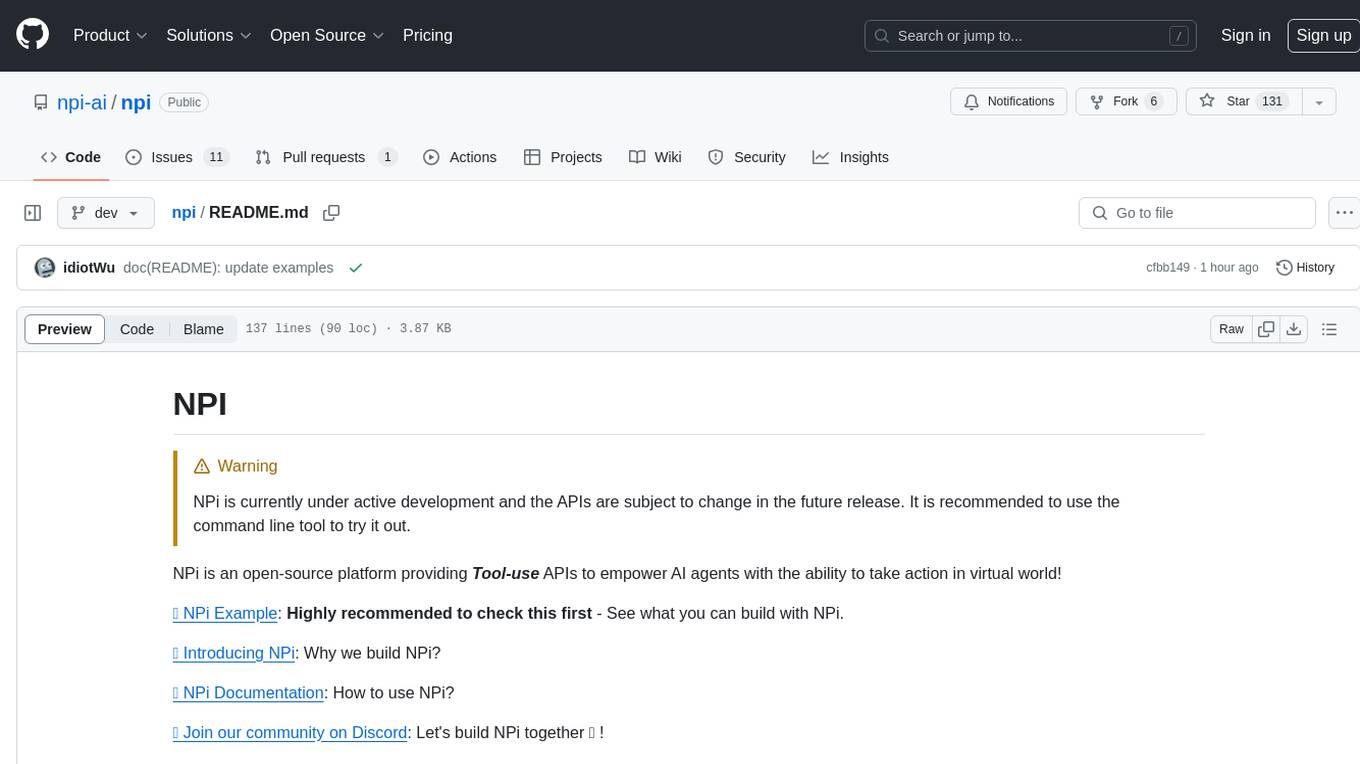
npi
NPi is an open-source platform providing Tool-use APIs to empower AI agents with the ability to take action in the virtual world. It is currently under active development, and the APIs are subject to change in future releases. NPi offers a command line tool for installation and setup, along with a GitHub app for easy access to repositories. The platform also includes a Python SDK and examples like Calendar Negotiator and Twitter Crawler. Join the NPi community on Discord to contribute to the development and explore the roadmap for future enhancements.
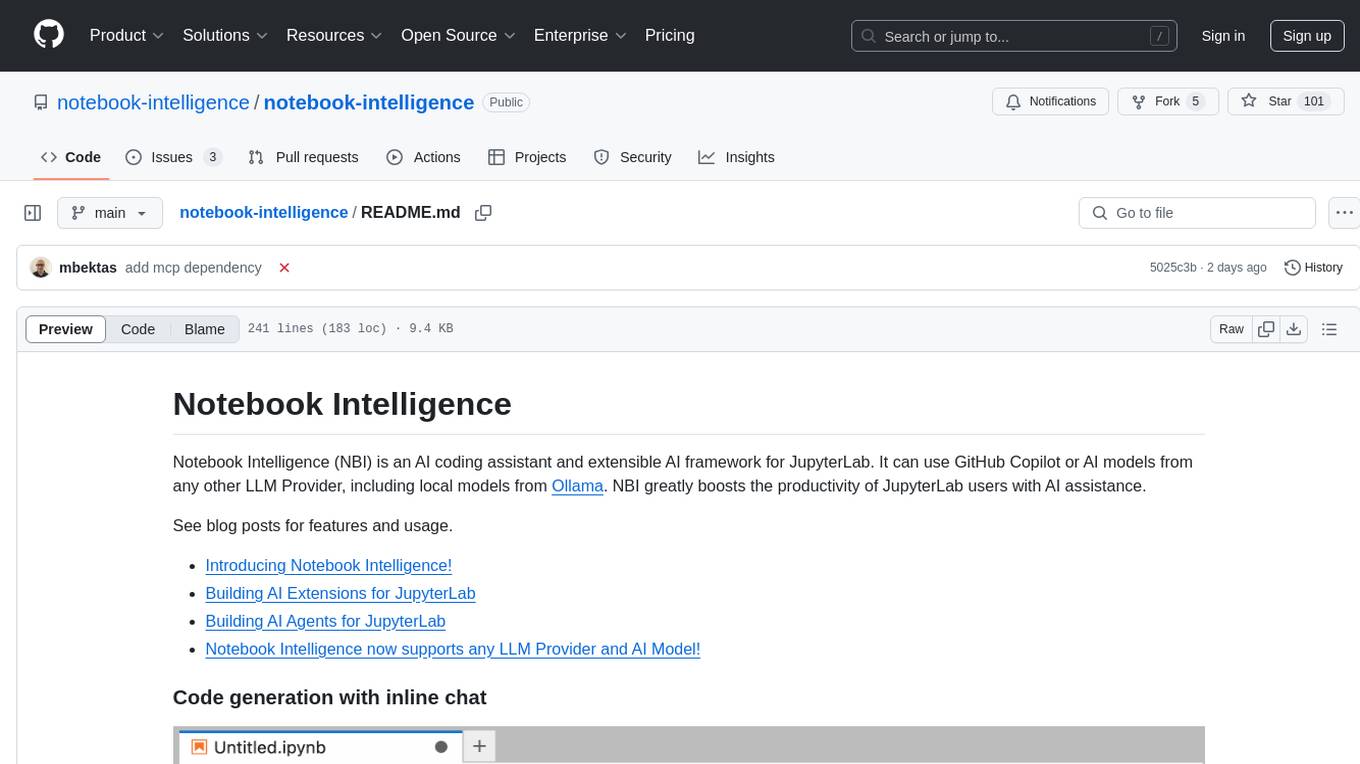
notebook-intelligence
Notebook Intelligence (NBI) is an AI coding assistant and extensible AI framework for JupyterLab. It greatly boosts the productivity of JupyterLab users with AI assistance by providing features such as code generation with inline chat, auto-complete, and chat interface. NBI supports various LLM Providers and AI Models, including local models from Ollama. Users can configure model provider and model options, remember GitHub Copilot login, and save configuration files. NBI seamlessly integrates with Model Context Protocol (MCP) servers, supporting both Standard Input/Output (stdio) and Server-Sent Events (SSE) transports. Users can easily add MCP servers to NBI, auto-approve tools, set environment variables, and group servers based on functionality. Additionally, NBI allows access to built-in tools from an MCP participant, enhancing the user experience and productivity.
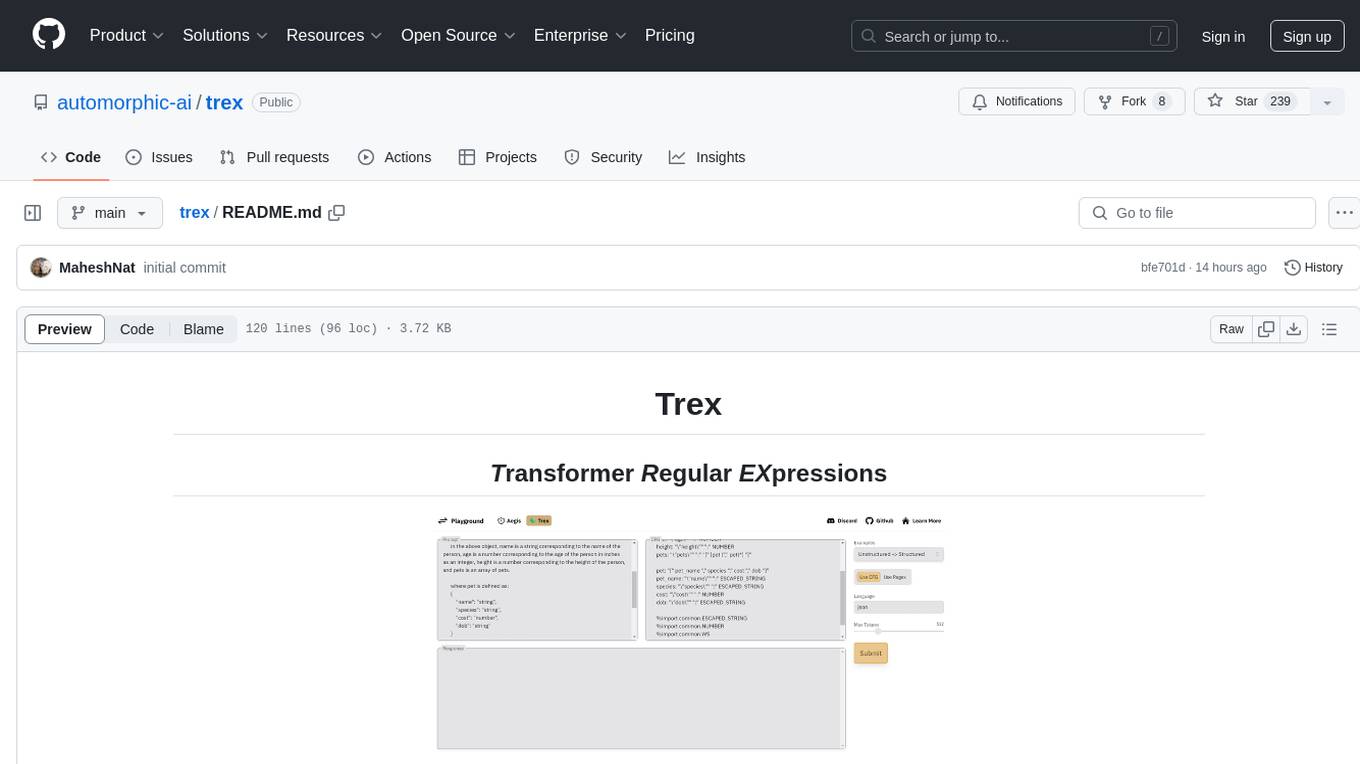
trex
Trex is a tool that transforms unstructured data into structured data by specifying a regex or context-free grammar. It intelligently restructures data to conform to the defined schema. It offers a Python client for installation and requires an API key obtained by signing up at automorphic.ai. The tool supports generating structured JSON objects based on user-defined schemas and prompts. Trex aims to provide significant speed improvements, structured custom CFG and regex generation, and generation from JSON schema. Future plans include auto-prompt generation for unstructured ETL and more intelligent models.
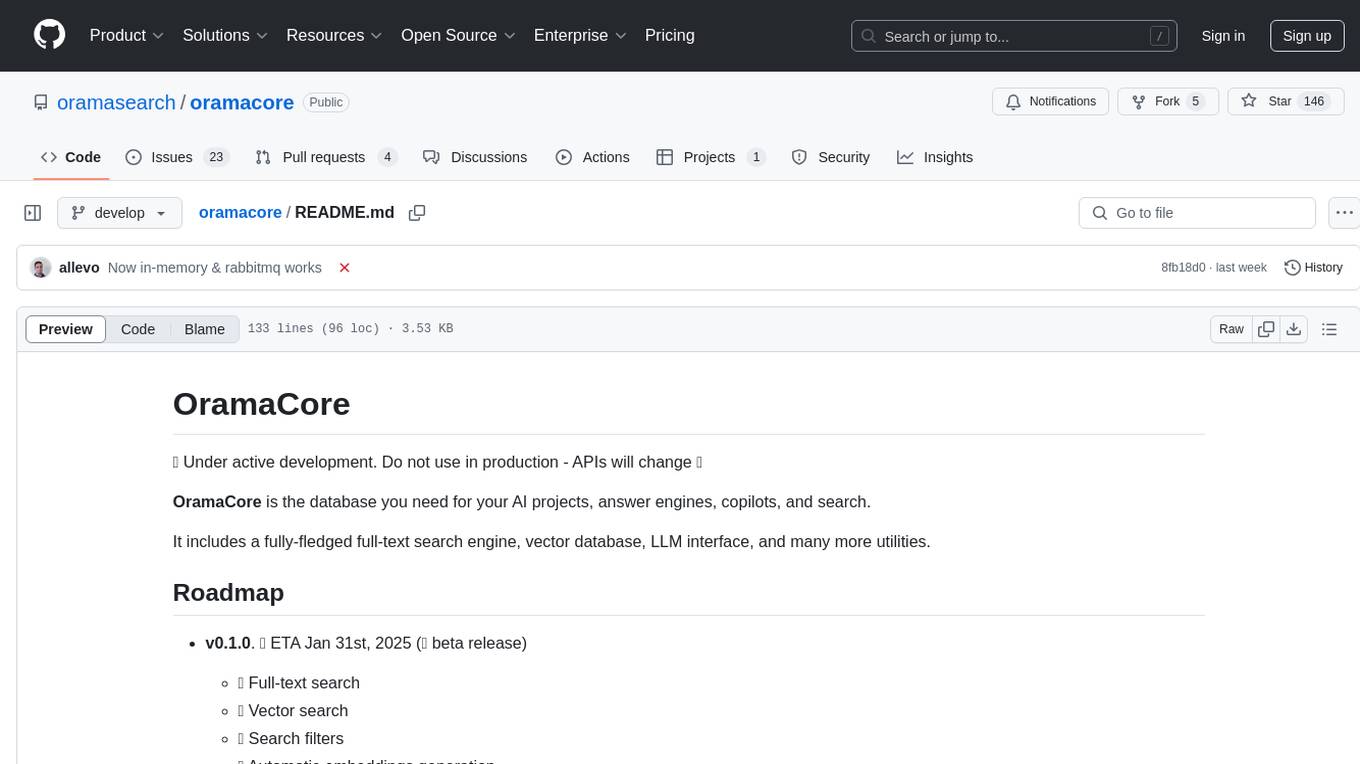
oramacore
OramaCore is a database designed for AI projects, answer engines, copilots, and search functionalities. It offers features such as a full-text search engine, vector database, LLM interface, and various utilities. The tool is currently under active development and not recommended for production use due to potential API changes. OramaCore aims to provide a comprehensive solution for managing data and enabling advanced search capabilities in AI applications.
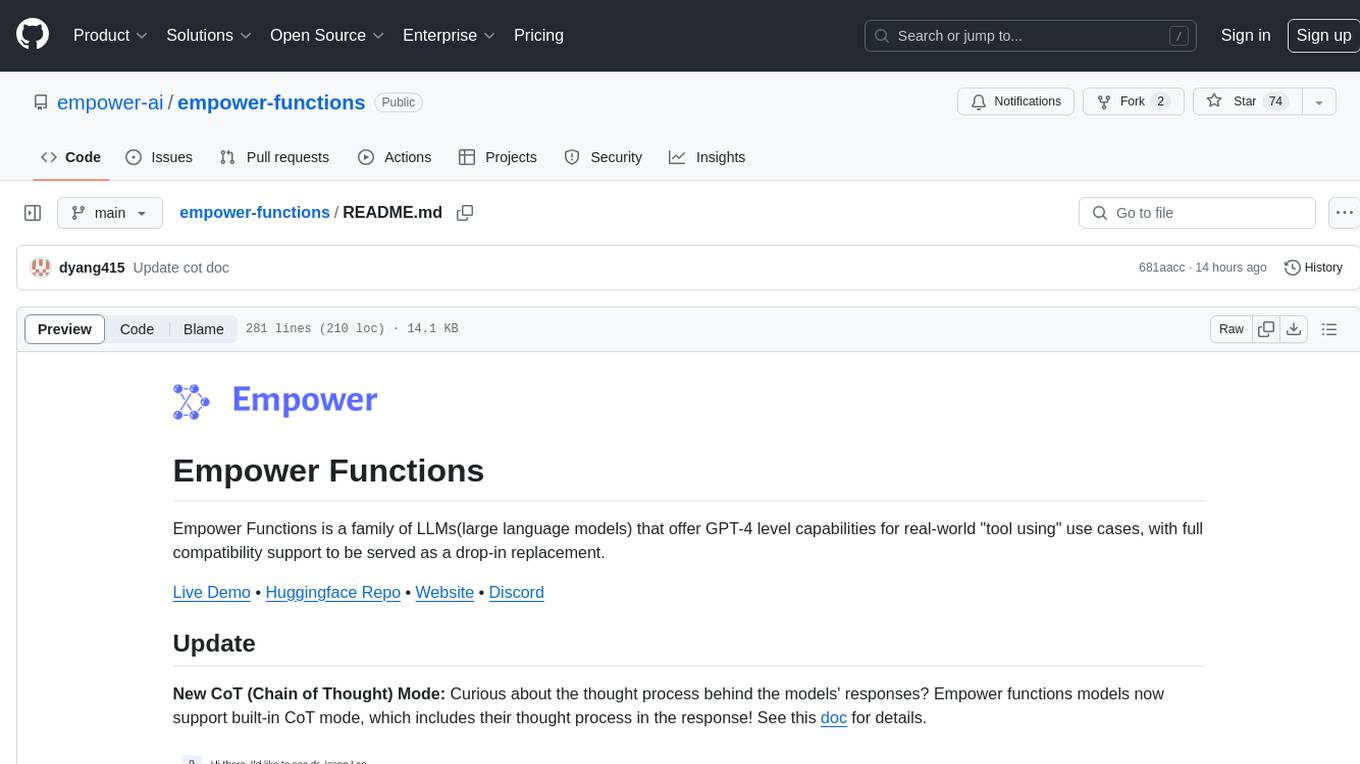
empower-functions
Empower Functions is a family of large language models (LLMs) that provide GPT-4 level capabilities for real-world 'tool using' use cases. These models offer compatibility support to be used as drop-in replacements, enabling interactions with external APIs by recognizing when a function needs to be called and generating JSON containing necessary arguments based on user inputs. This capability is crucial for building conversational agents and applications that convert natural language into API calls, facilitating tasks such as weather inquiries, data extraction, and interactions with knowledge bases. The models can handle multi-turn conversations, choose between tools or standard dialogue, ask for clarification on missing parameters, integrate responses with tool outputs in a streaming fashion, and efficiently execute multiple functions either in parallel or sequentially with dependencies.
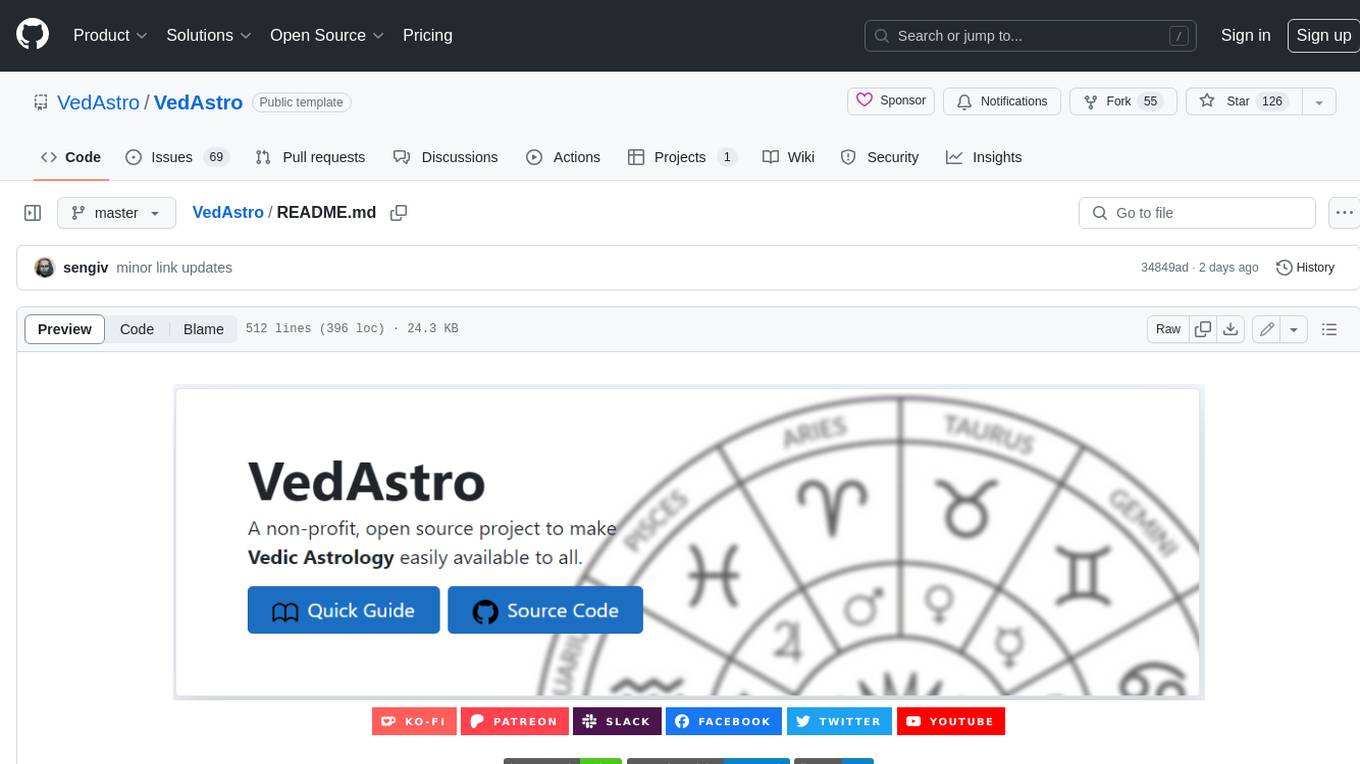
VedAstro
VedAstro is an open-source Vedic astrology tool that provides accurate astrological predictions and data. It offers a user-friendly website, a chat API, an open API, a JavaScript SDK, a Swiss Ephemeris API, and a machine learning table generator. VedAstro is free to use and is constantly being updated with new features and improvements.
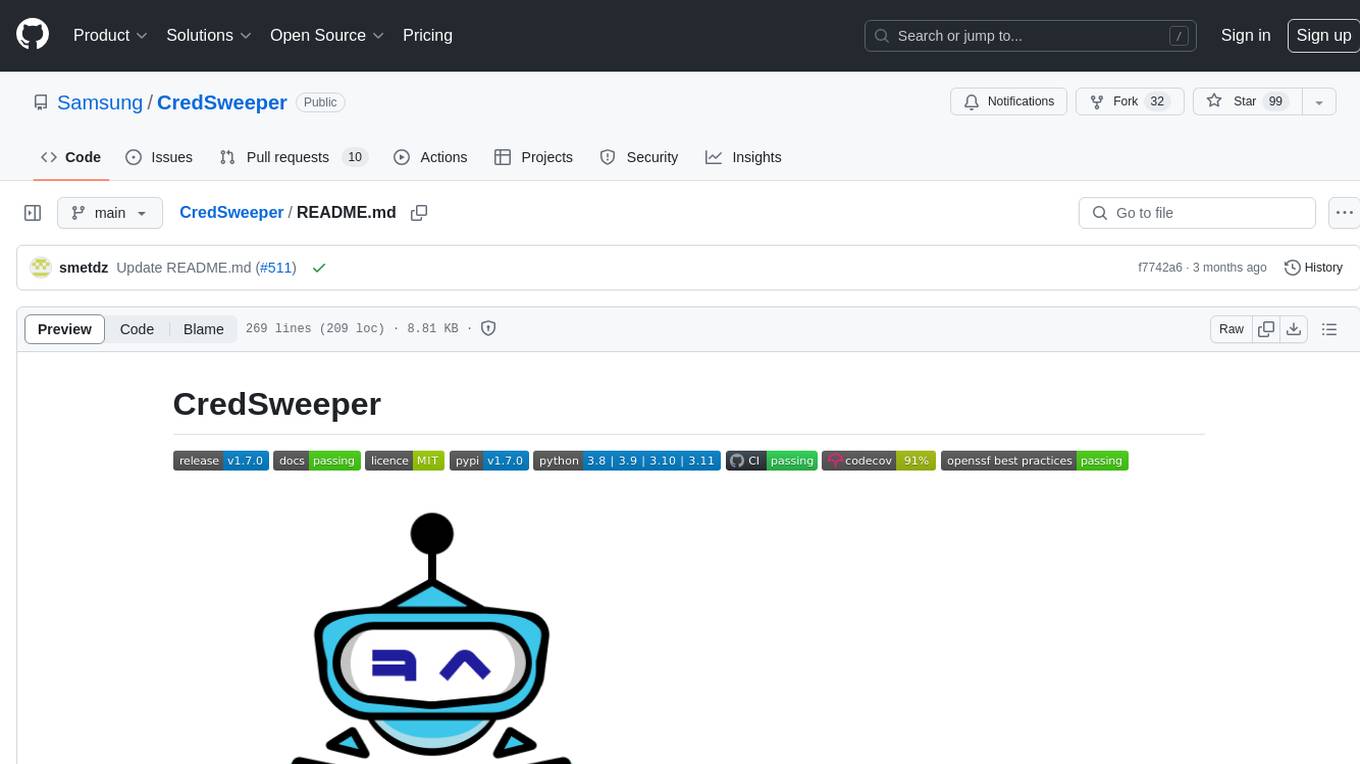
CredSweeper
CredSweeper is a tool designed to detect credentials like tokens, passwords, and API keys in directories or files. It helps users identify potential exposure of sensitive information by scanning lines, filtering, and utilizing an AI model. The tool reports lines containing possible credentials, their location, and the expected type of credential.
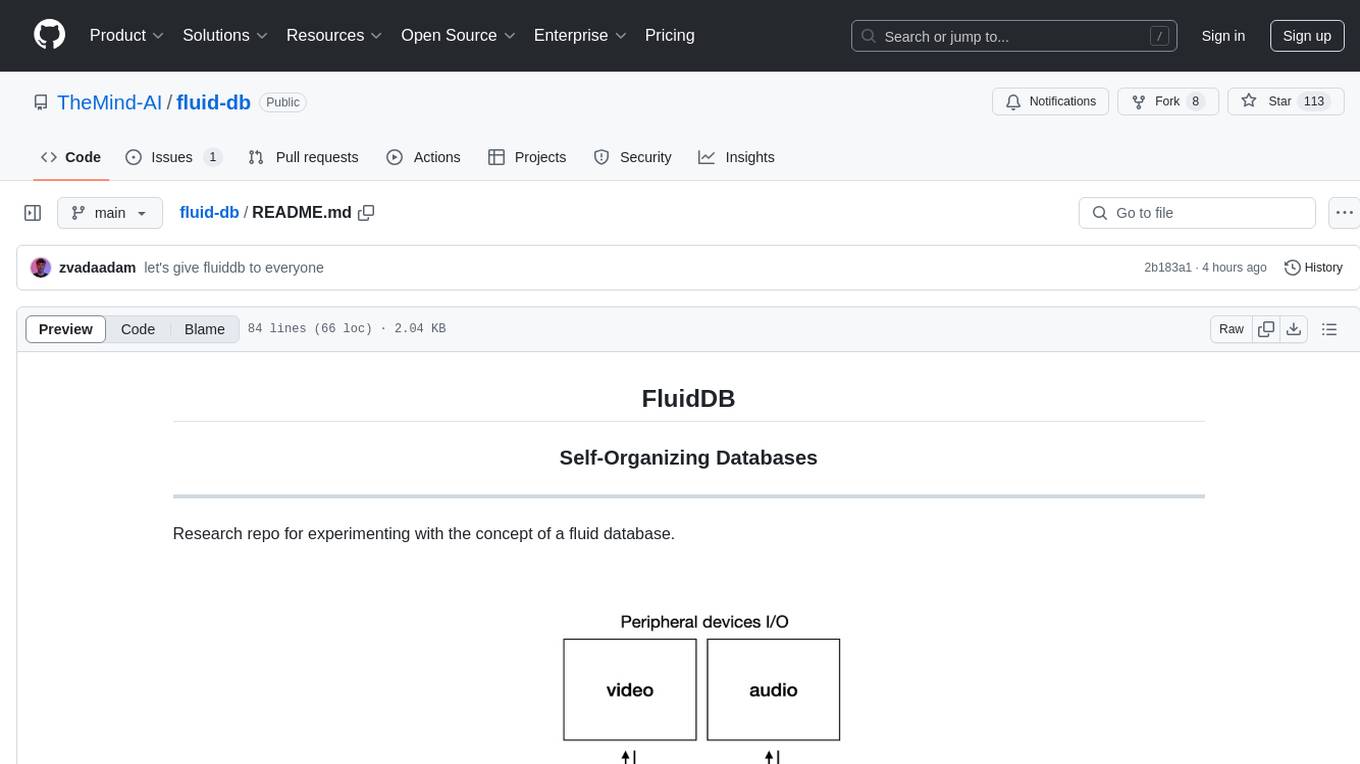
fluid-db
FluidDB is a research repository focusing on the concept of a fluid database that dynamically updates its schema based on ingested data. It enables the creation of personalized AI agents with features like adaptive schema, flexible querying, and versatile data input. The tool allows for storing unstructured data in a structured form and supports natural language queries. It aims to revolutionize database management by providing a dynamic and intuitive approach to data storage and retrieval.
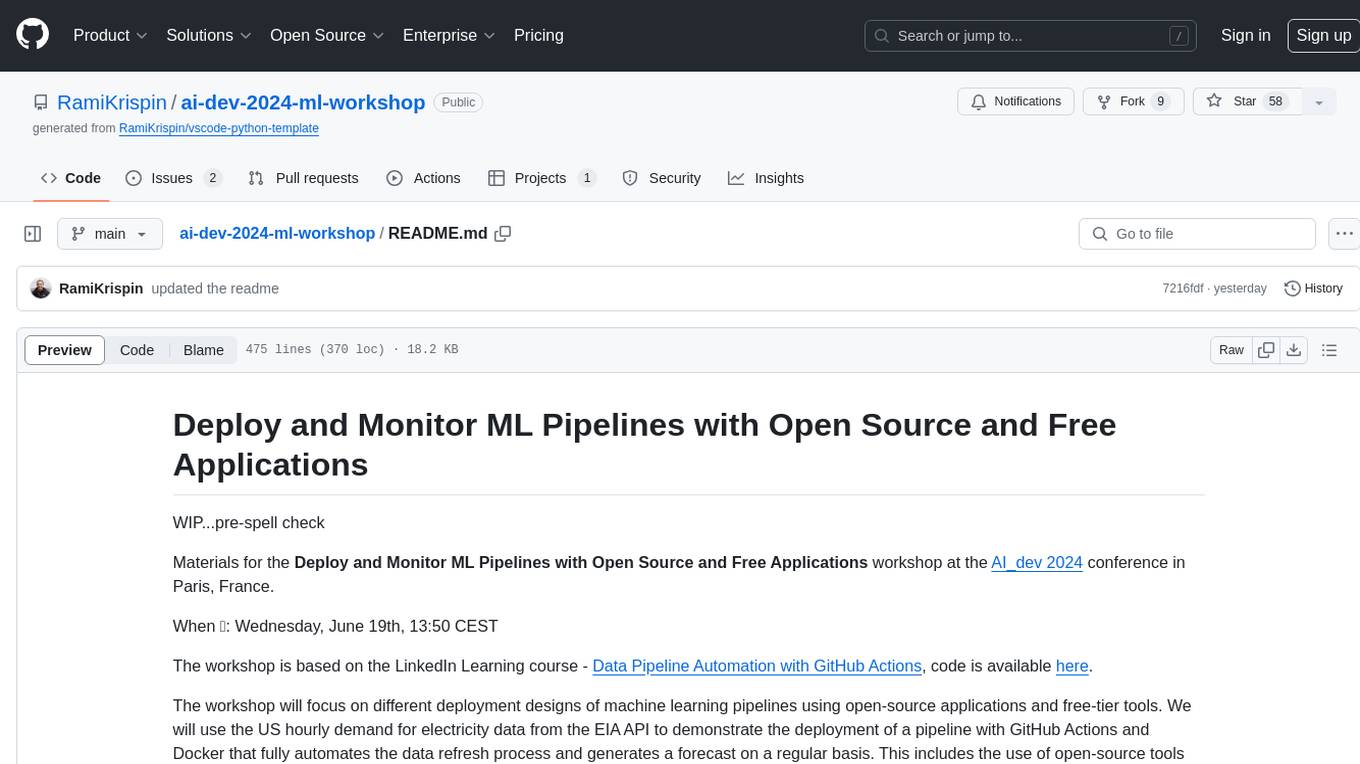
ai-dev-2024-ml-workshop
The 'ai-dev-2024-ml-workshop' repository contains materials for the Deploy and Monitor ML Pipelines workshop at the AI_dev 2024 conference in Paris, focusing on deployment designs of machine learning pipelines using open-source applications and free-tier tools. It demonstrates automating data refresh and forecasting using GitHub Actions and Docker, monitoring with MLflow and YData Profiling, and setting up a monitoring dashboard with Quarto doc on GitHub Pages.
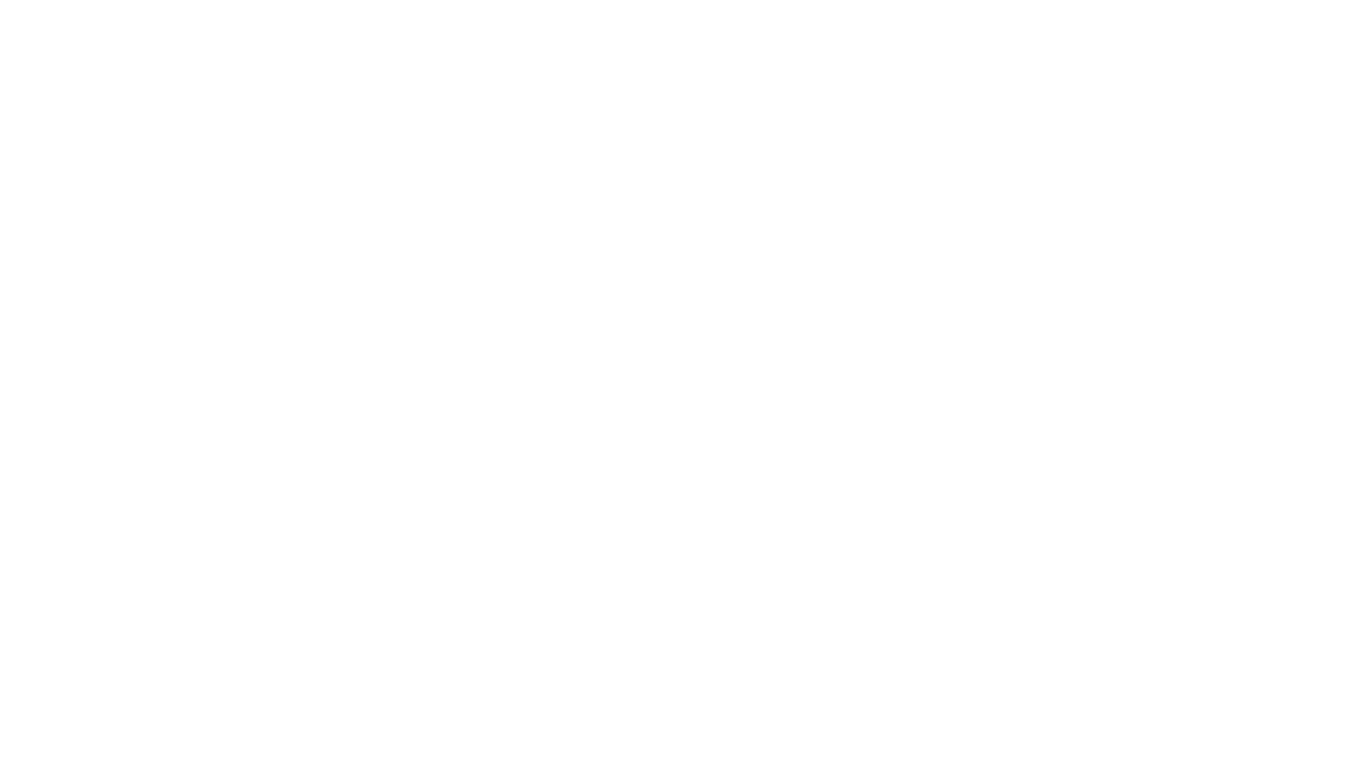
motorhead
Motorhead is a memory and information retrieval server for LLMs. It provides three simple APIs to assist with memory handling in chat applications using LLMs. The first API, GET /sessions/:id/memory, returns messages up to a maximum window size. The second API, POST /sessions/:id/memory, allows you to send an array of messages to Motorhead for storage. The third API, DELETE /sessions/:id/memory, deletes the session's message list. Motorhead also features incremental summarization, where it processes half of the maximum window size of messages and summarizes them when the maximum is reached. Additionally, it supports searching by text query using vector search. Motorhead is configurable through environment variables, including the maximum window size, whether to enable long-term memory, the model used for incremental summarization, the server port, your OpenAI API key, and the Redis URL.
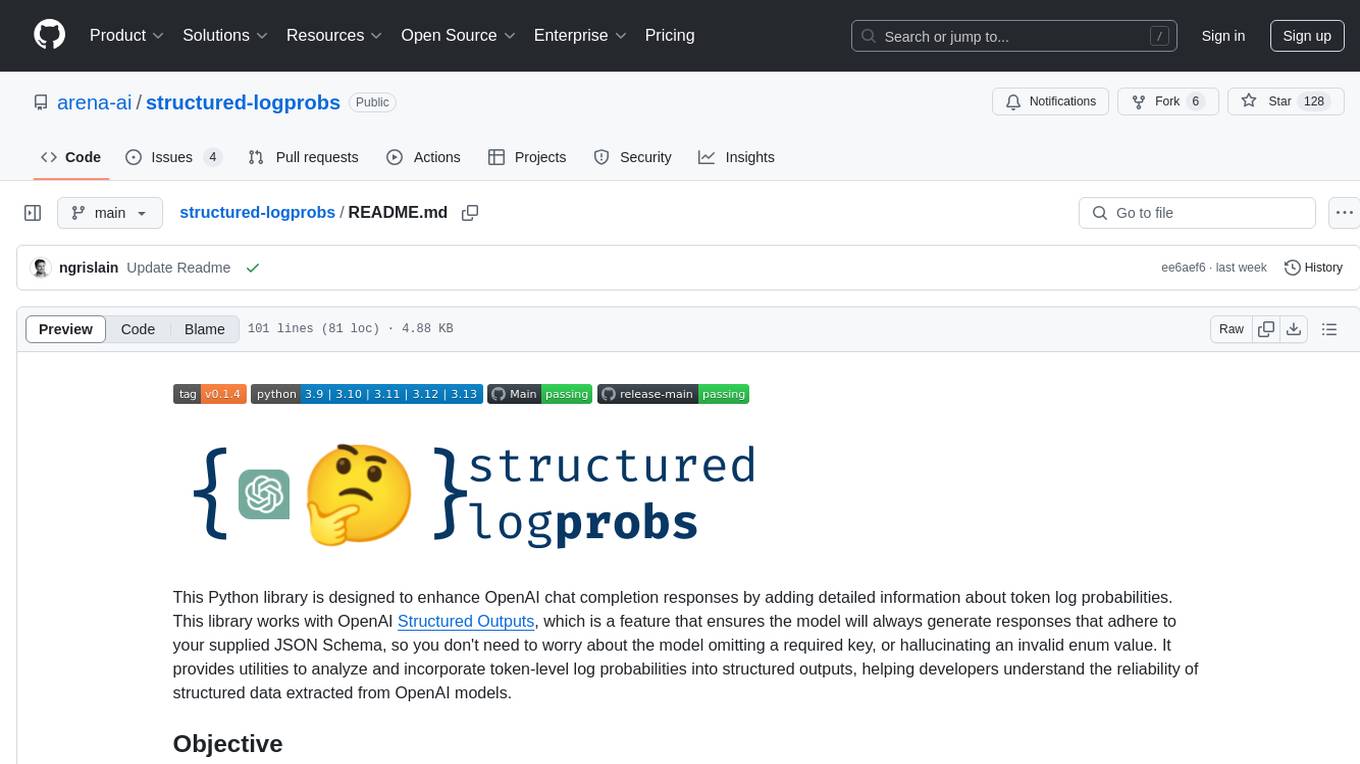
structured-logprobs
This Python library enhances OpenAI chat completion responses by providing detailed information about token log probabilities. It works with OpenAI Structured Outputs to ensure model-generated responses adhere to a JSON Schema. Developers can analyze and incorporate token-level log probabilities to understand the reliability of structured data extracted from OpenAI models.
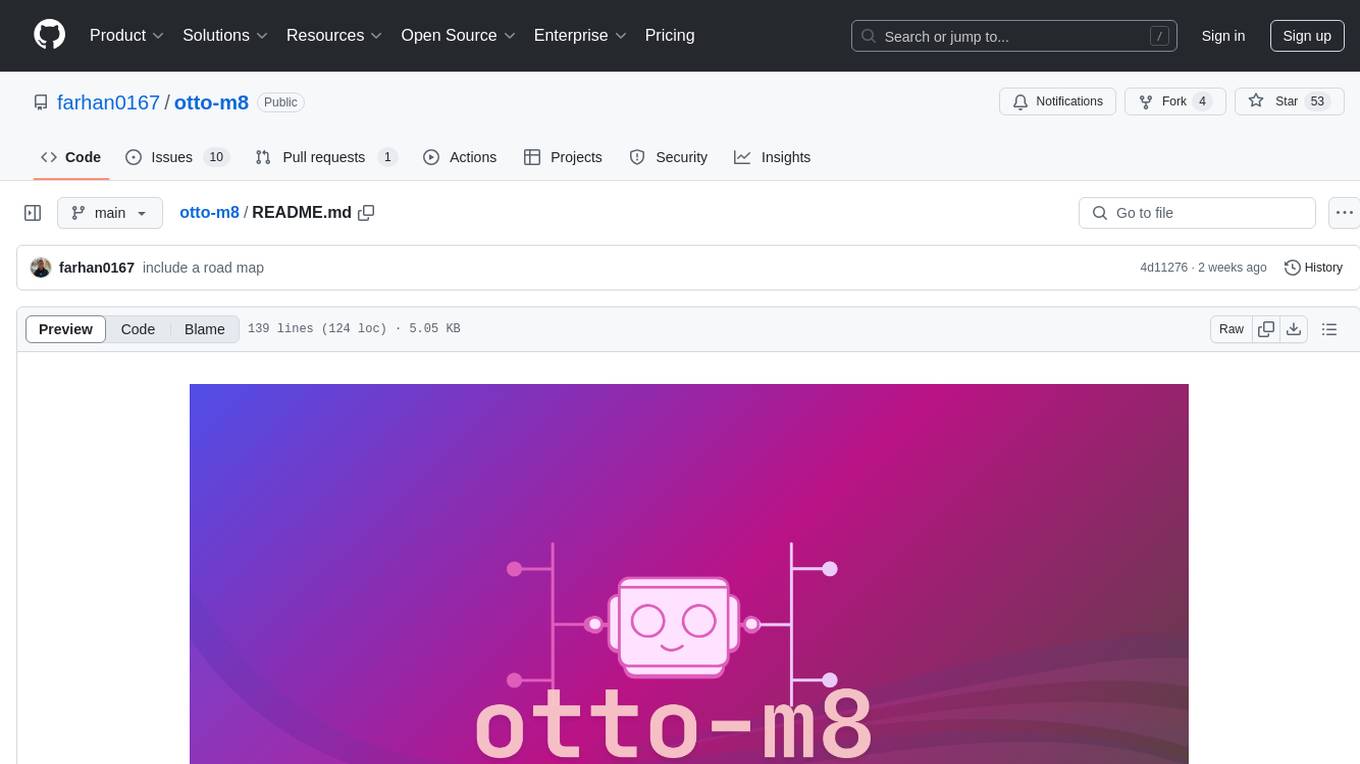
otto-m8
otto-m8 is a flowchart based automation platform designed to run deep learning workloads with minimal to no code. It provides a user-friendly interface to spin up a wide range of AI models, including traditional deep learning models and large language models. The tool deploys Docker containers of workflows as APIs for integration with existing workflows, building AI chatbots, or standalone applications. Otto-m8 operates on an Input, Process, Output paradigm, simplifying the process of running AI models into a flowchart-like UI.
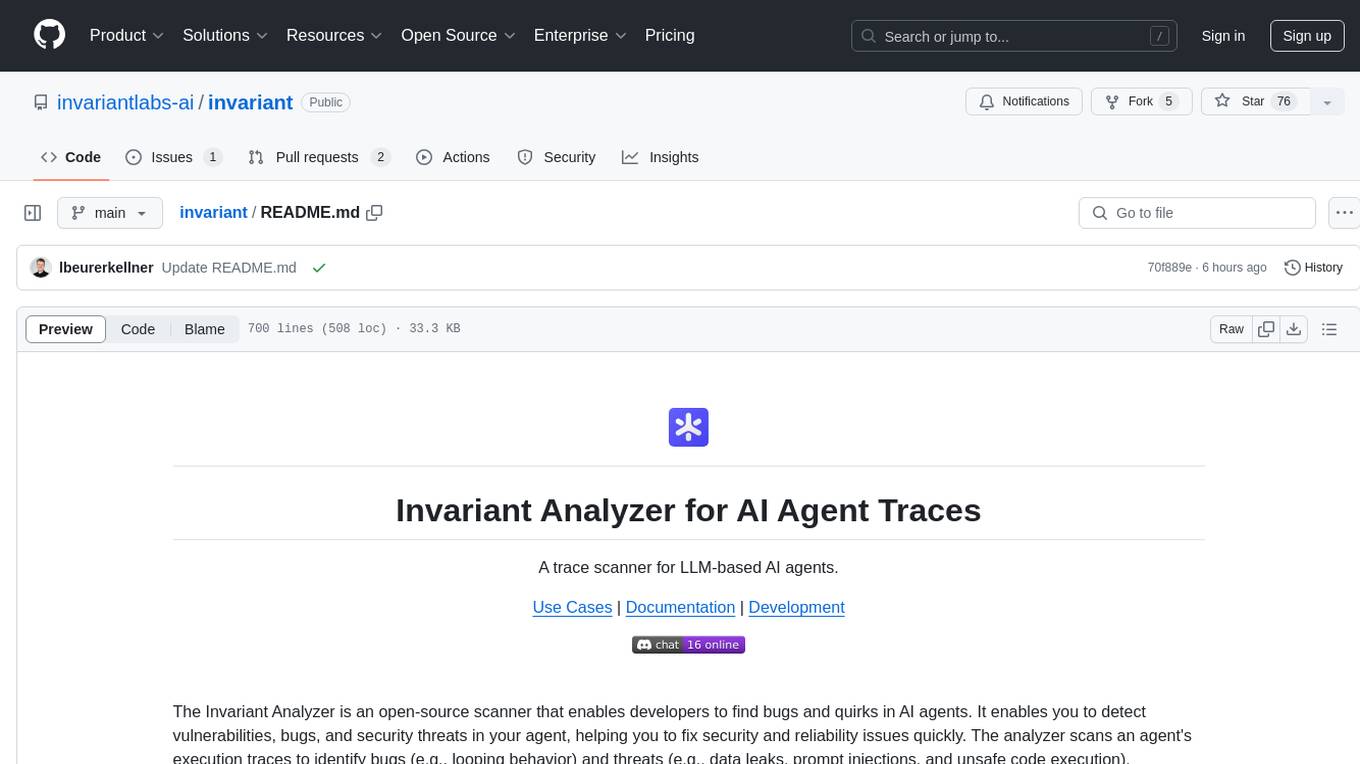
invariant
Invariant Analyzer is an open-source scanner designed for LLM-based AI agents to find bugs, vulnerabilities, and security threats. It scans agent execution traces to identify issues like looping behavior, data leaks, prompt injections, and unsafe code execution. The tool offers a library of built-in checkers, an expressive policy language, data flow analysis, real-time monitoring, and extensible architecture for custom checkers. It helps developers debug AI agents, scan for security violations, and prevent security issues and data breaches during runtime. The analyzer leverages deep contextual understanding and a purpose-built rule matching engine for security policy enforcement.
For similar tasks
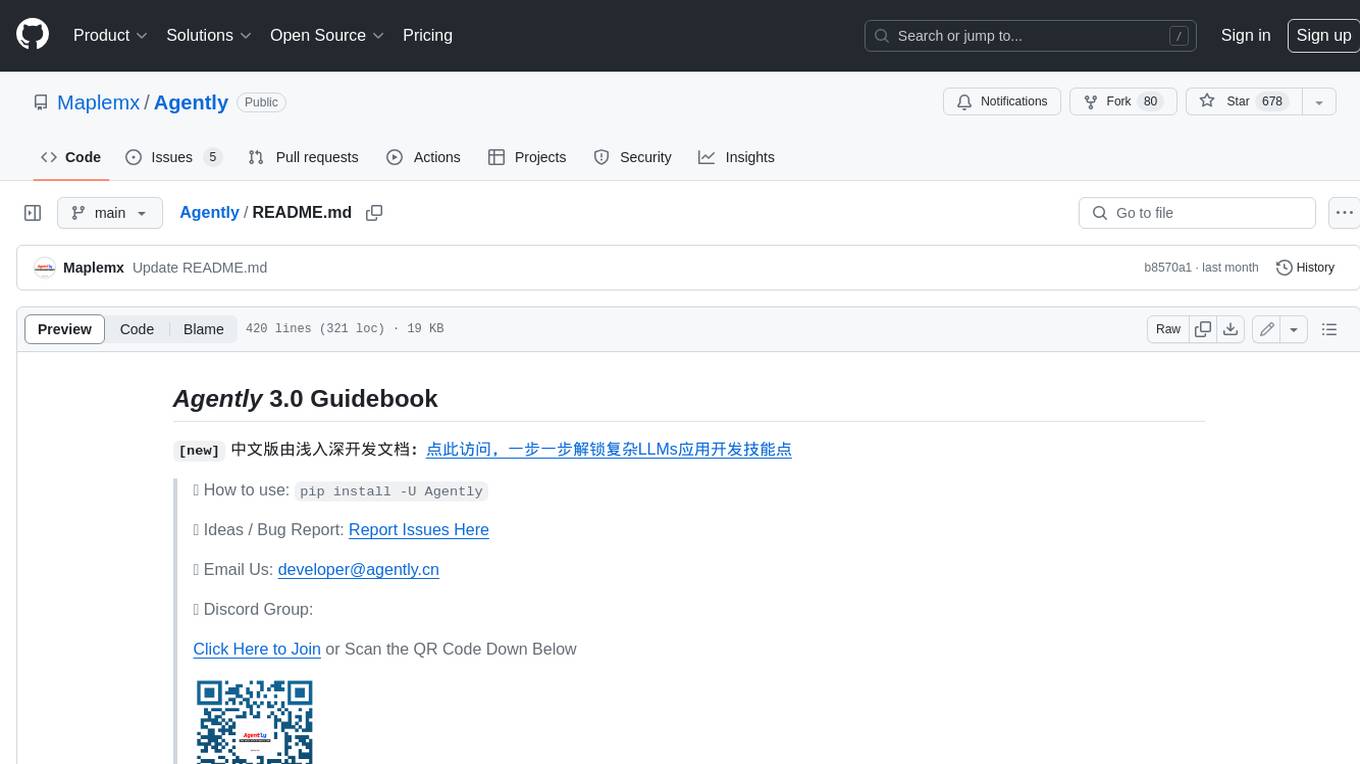
Agently
Agently is a development framework that helps developers build AI agent native application really fast. You can use and build AI agent in your code in an extremely simple way. You can create an AI agent instance then interact with it like calling a function in very few codes like this below. Click the run button below and witness the magic. It's just that simple: python # Import and Init Settings import Agently agent = Agently.create_agent() agent\ .set_settings("current_model", "OpenAI")\ .set_settings("model.OpenAI.auth", {"api_key": ""}) # Interact with the agent instance like calling a function result = agent\ .input("Give me 3 words")\ .output([("String", "one word")])\ .start() print(result) ['apple', 'banana', 'carrot'] And you may notice that when we print the value of `result`, the value is a `list` just like the format of parameter we put into the `.output()`. In Agently framework we've done a lot of work like this to make it easier for application developers to integrate Agent instances into their business code. This will allow application developers to focus on how to build their business logic instead of figure out how to cater to language models or how to keep models satisfied.
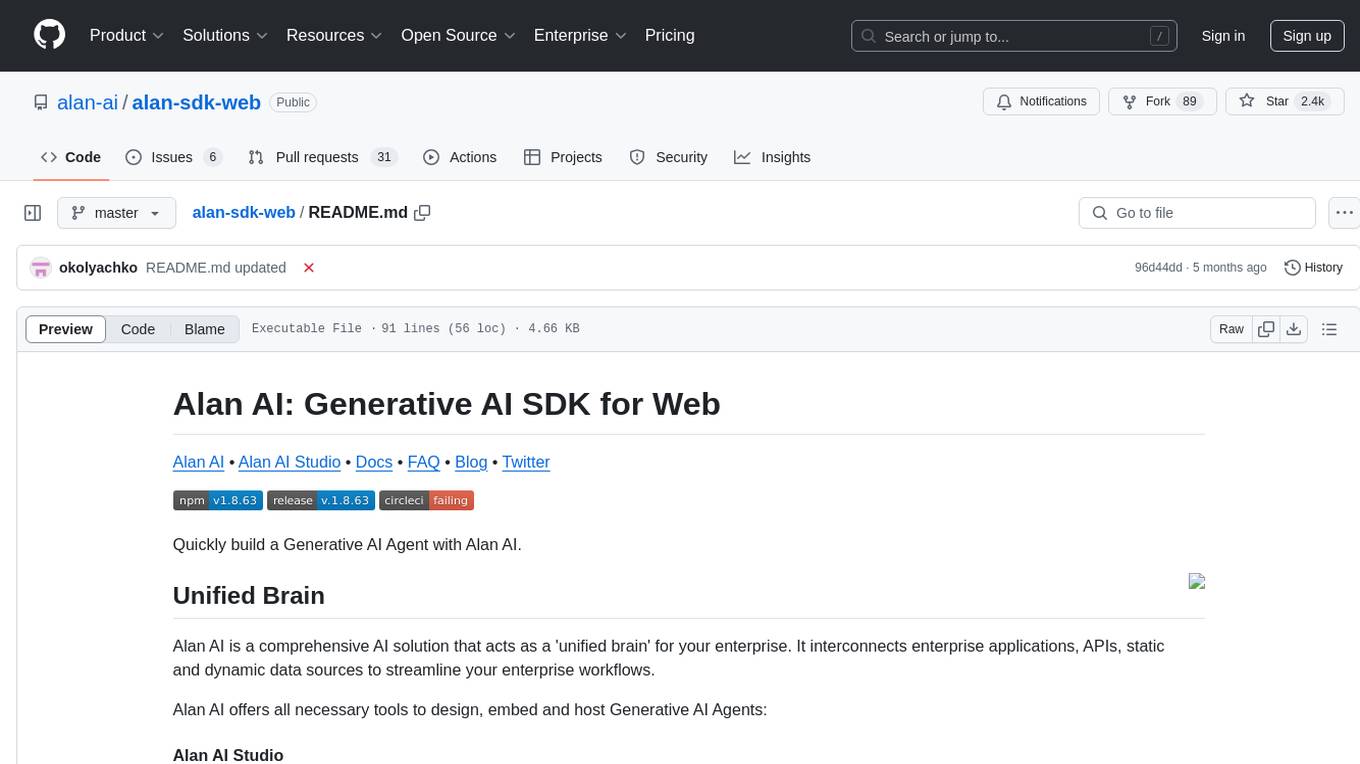
alan-sdk-web
Alan AI is a comprehensive AI solution that acts as a 'unified brain' for enterprises, interconnecting applications, APIs, and data sources to streamline workflows. It offers tools like Alan AI Studio for designing dialog scenarios, lightweight SDKs for embedding AI Agents, and a backend powered by advanced AI technologies. With Alan AI, users can create conversational experiences with minimal UI changes, benefit from a serverless environment, receive on-the-fly updates, and access dialog testing and analytics tools. The platform supports various frameworks like JavaScript, React, Angular, Vue, Ember, and Electron, and provides example web apps for different platforms. Users can also explore Alan AI SDKs for iOS, Android, Flutter, Ionic, Apache Cordova, and React Native.
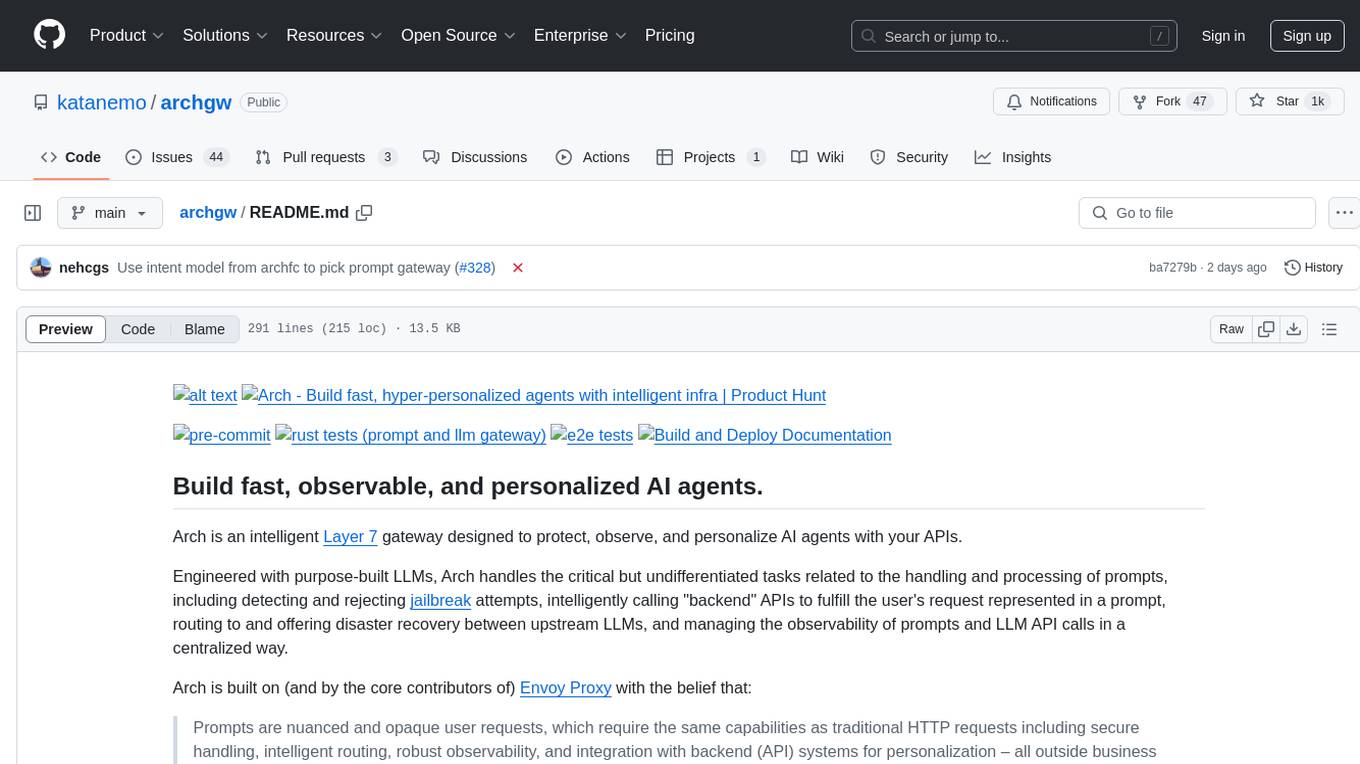
archgw
Arch is an intelligent Layer 7 gateway designed to protect, observe, and personalize AI agents with APIs. It handles tasks related to prompts, including detecting jailbreak attempts, calling backend APIs, routing between LLMs, and managing observability. Built on Envoy Proxy, it offers features like function calling, prompt guardrails, traffic management, and observability. Users can build fast, observable, and personalized AI agents using Arch to improve speed, security, and personalization of GenAI apps.
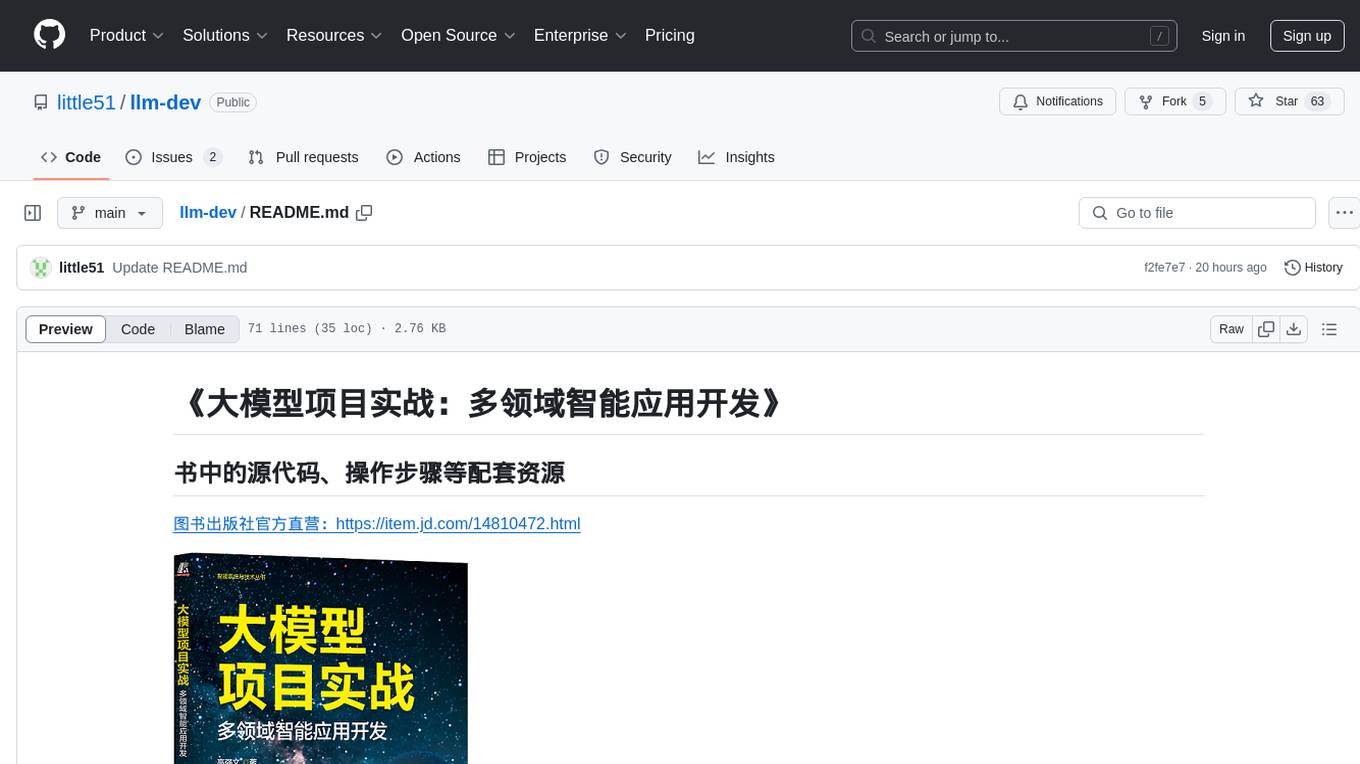
llm-dev
The 'llm-dev' repository contains source code and resources for the book 'Practical Projects of Large Models: Multi-Domain Intelligent Application Development'. It covers topics such as language model basics, application architecture, working modes, environment setup, model installation, fine-tuning, quantization, multi-modal model applications, chat applications, programming large model applications, VS Code plugin development, enhanced generation applications, translation applications, intelligent agent applications, speech model applications, digital human applications, model training applications, and AI town applications.
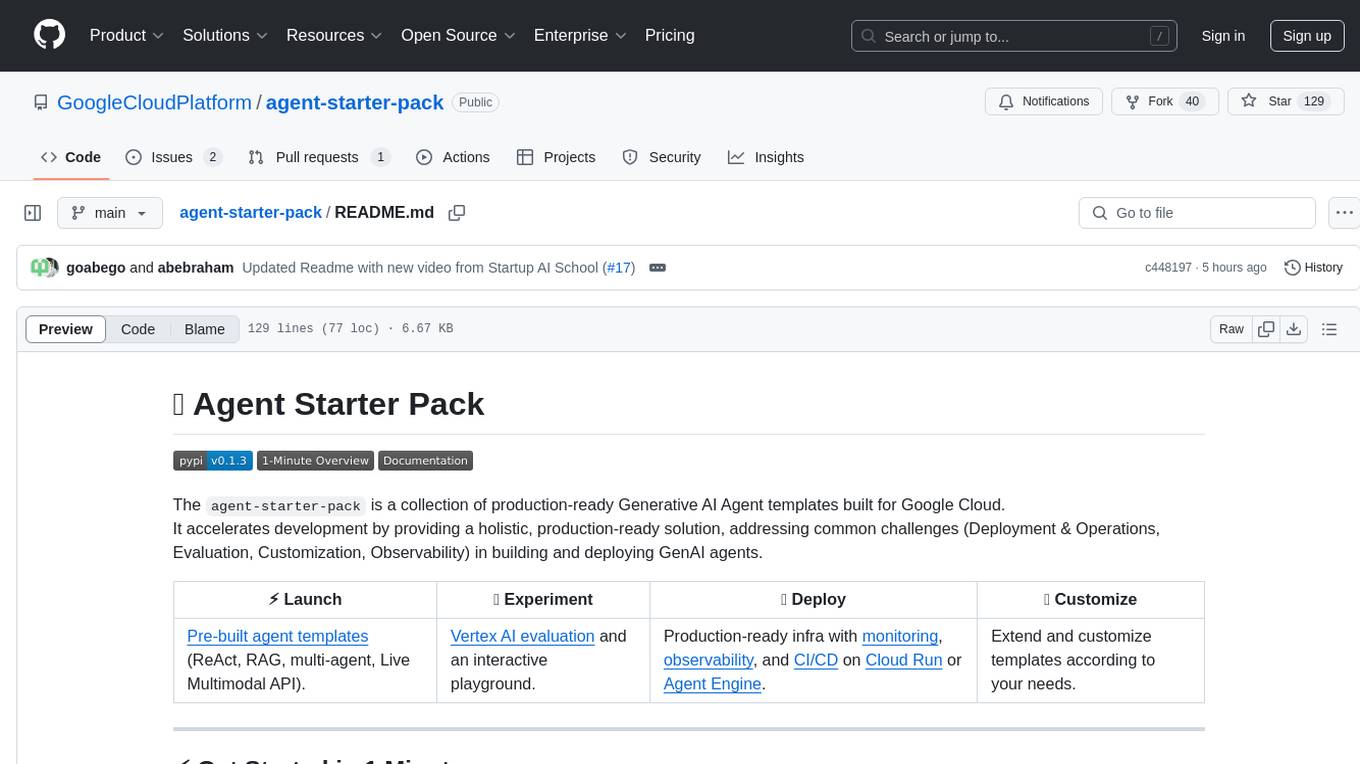
agent-starter-pack
The agent-starter-pack is a collection of production-ready Generative AI Agent templates built for Google Cloud. It accelerates development by providing a holistic, production-ready solution, addressing common challenges in building and deploying GenAI agents. The tool offers pre-built agent templates, evaluation tools, production-ready infrastructure, and customization options. It also provides CI/CD automation and data pipeline integration for RAG agents. The starter pack covers all aspects of agent development, from prototyping and evaluation to deployment and monitoring. It is designed to simplify project creation, template selection, and deployment for agent development on Google Cloud.
For similar jobs
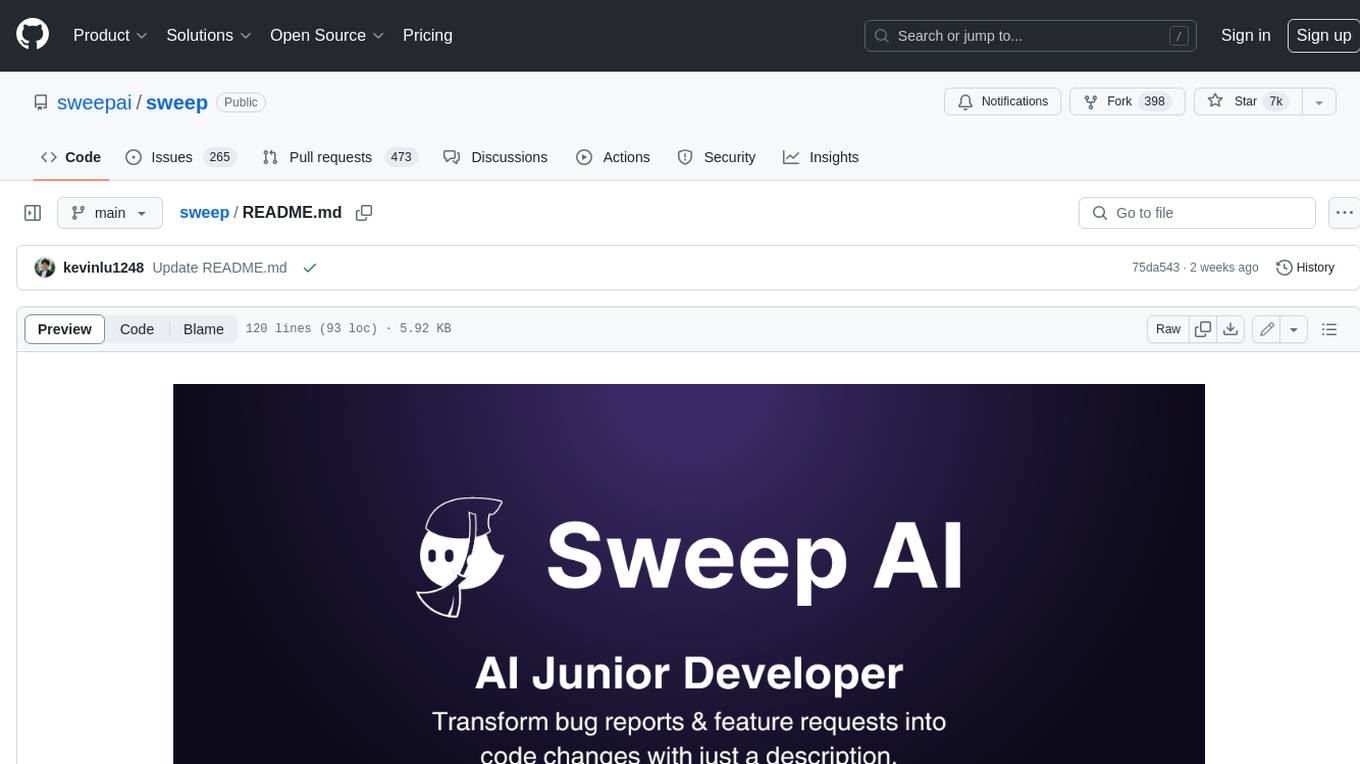
sweep
Sweep is an AI junior developer that turns bugs and feature requests into code changes. It automatically handles developer experience improvements like adding type hints and improving test coverage.
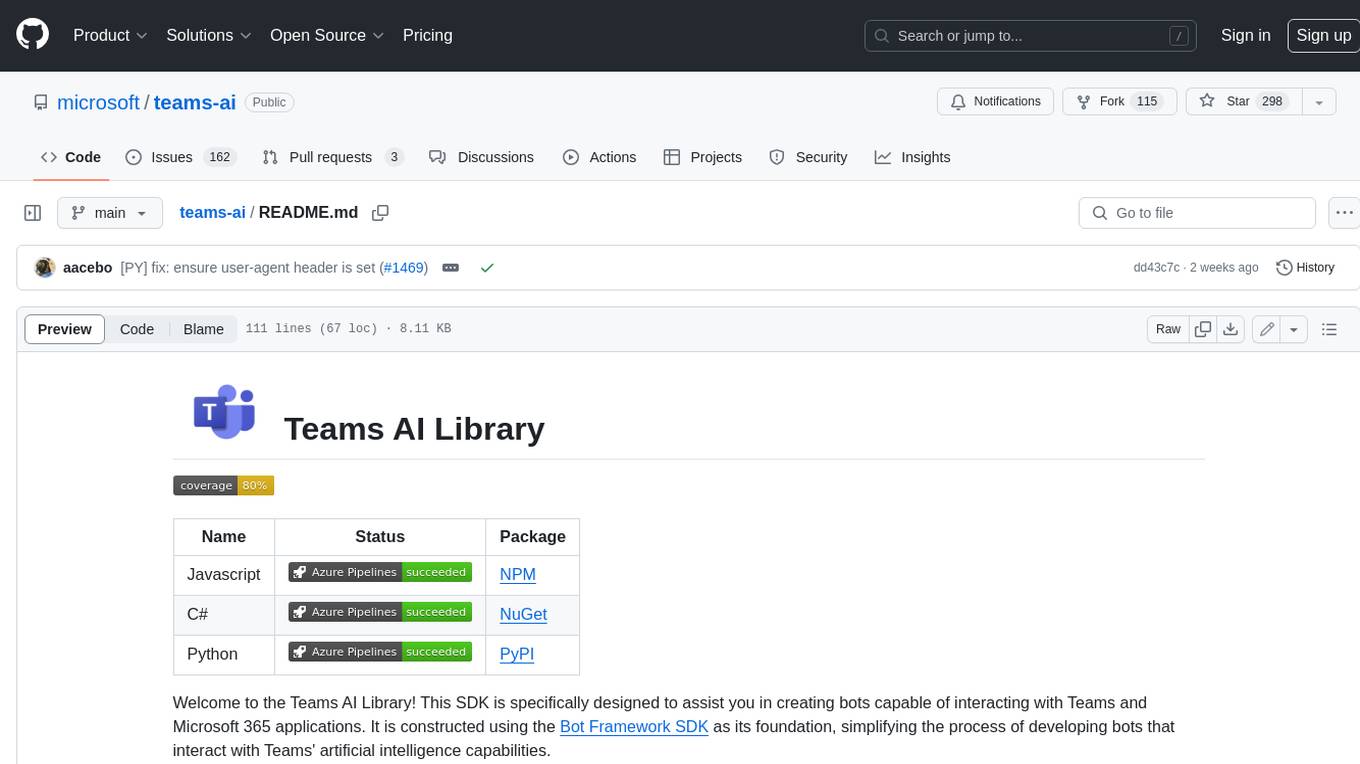
teams-ai
The Teams AI Library is a software development kit (SDK) that helps developers create bots that can interact with Teams and Microsoft 365 applications. It is built on top of the Bot Framework SDK and simplifies the process of developing bots that interact with Teams' artificial intelligence capabilities. The SDK is available for JavaScript/TypeScript, .NET, and Python.
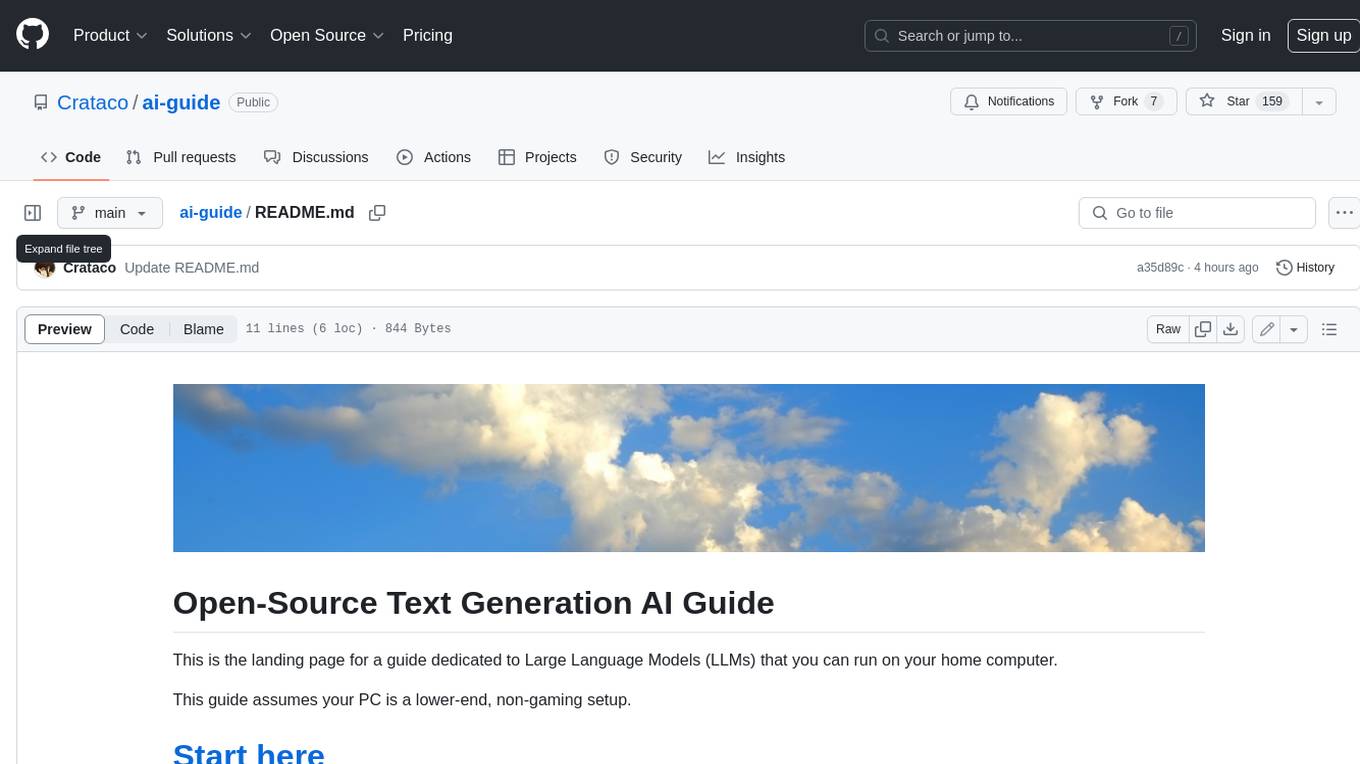
ai-guide
This guide is dedicated to Large Language Models (LLMs) that you can run on your home computer. It assumes your PC is a lower-end, non-gaming setup.
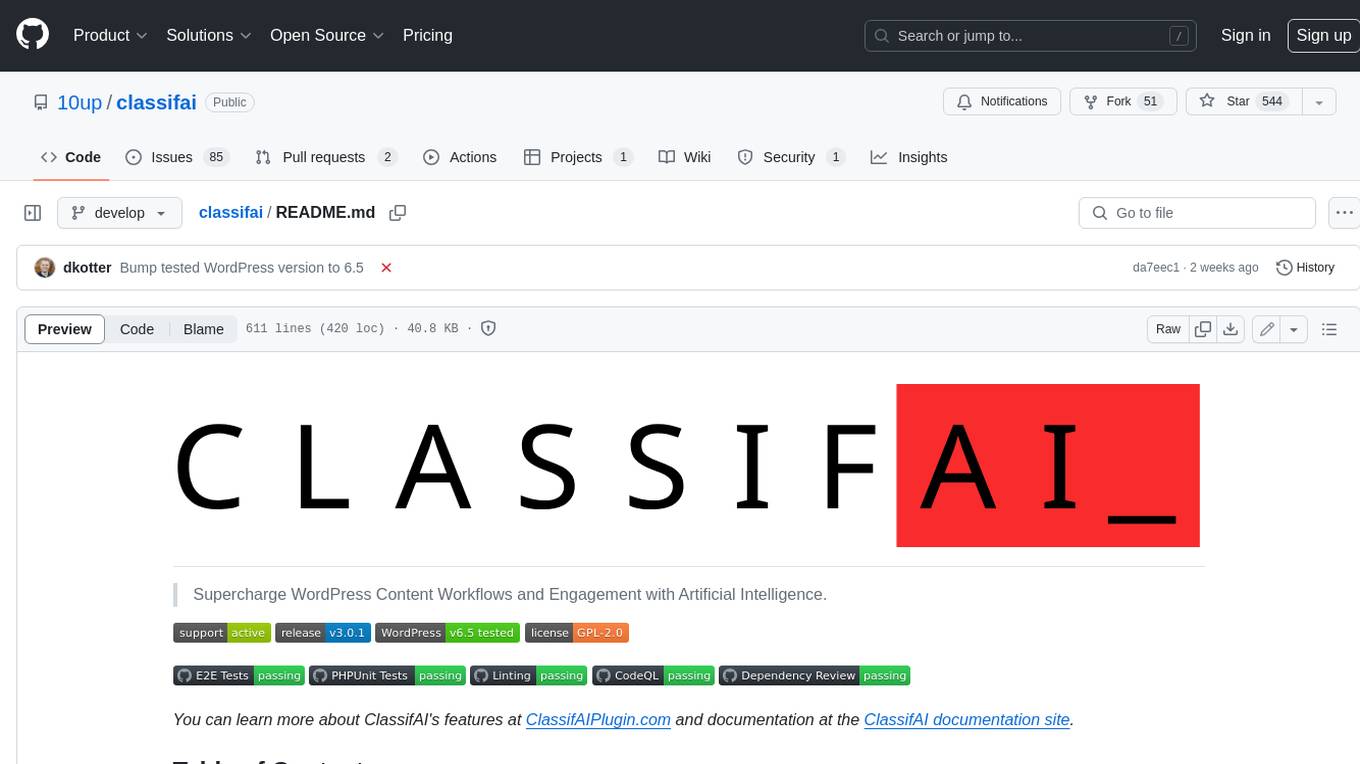
classifai
Supercharge WordPress Content Workflows and Engagement with Artificial Intelligence. Tap into leading cloud-based services like OpenAI, Microsoft Azure AI, Google Gemini and IBM Watson to augment your WordPress-powered websites. Publish content faster while improving SEO performance and increasing audience engagement. ClassifAI integrates Artificial Intelligence and Machine Learning technologies to lighten your workload and eliminate tedious tasks, giving you more time to create original content that matters.
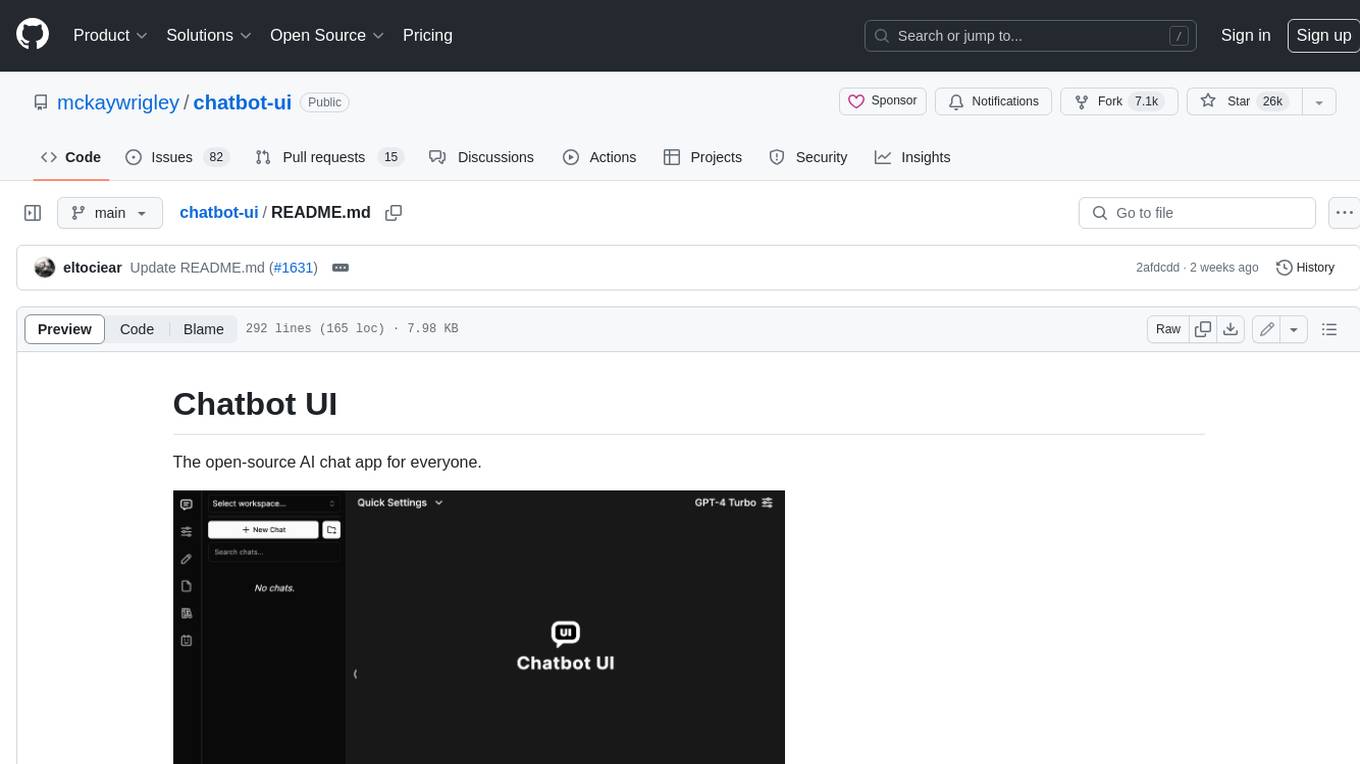
chatbot-ui
Chatbot UI is an open-source AI chat app that allows users to create and deploy their own AI chatbots. It is easy to use and can be customized to fit any need. Chatbot UI is perfect for businesses, developers, and anyone who wants to create a chatbot.
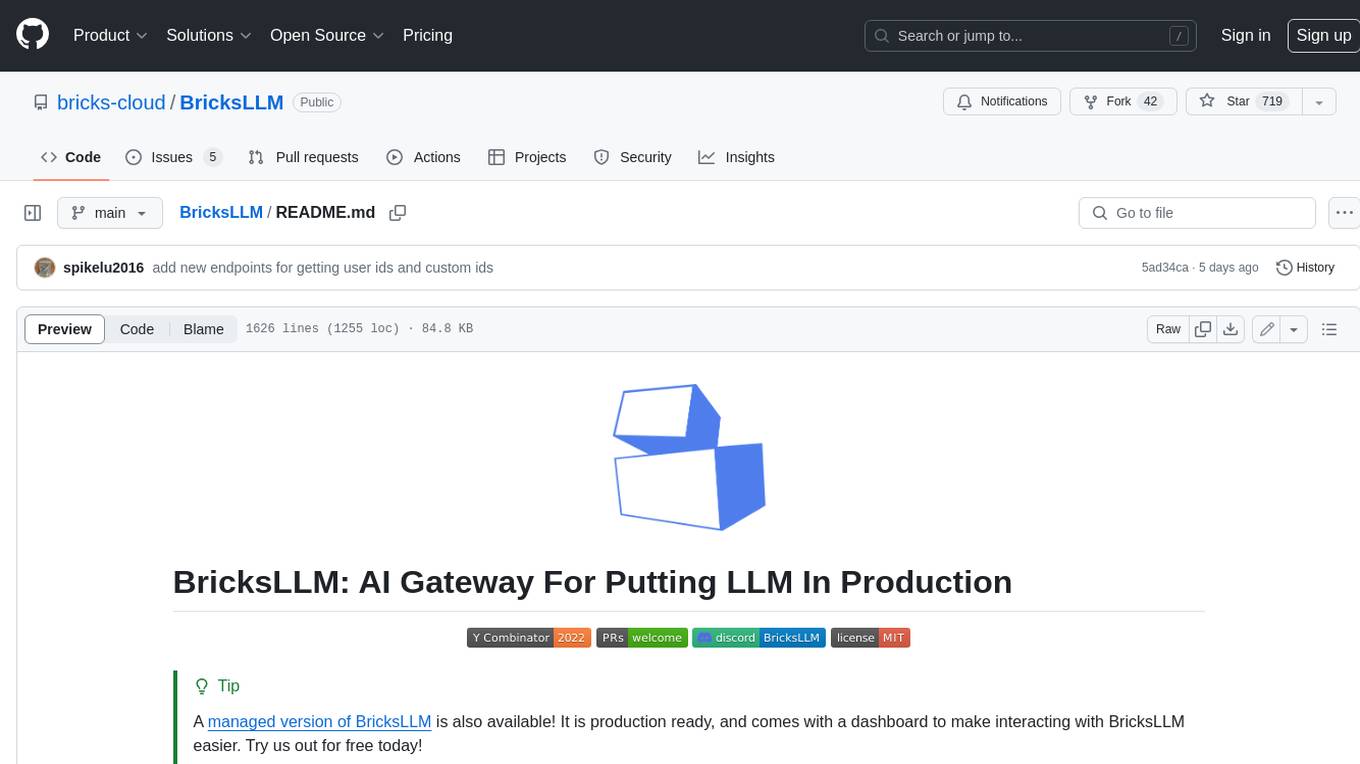
BricksLLM
BricksLLM is a cloud native AI gateway written in Go. Currently, it provides native support for OpenAI, Anthropic, Azure OpenAI and vLLM. BricksLLM aims to provide enterprise level infrastructure that can power any LLM production use cases. Here are some use cases for BricksLLM: * Set LLM usage limits for users on different pricing tiers * Track LLM usage on a per user and per organization basis * Block or redact requests containing PIIs * Improve LLM reliability with failovers, retries and caching * Distribute API keys with rate limits and cost limits for internal development/production use cases * Distribute API keys with rate limits and cost limits for students
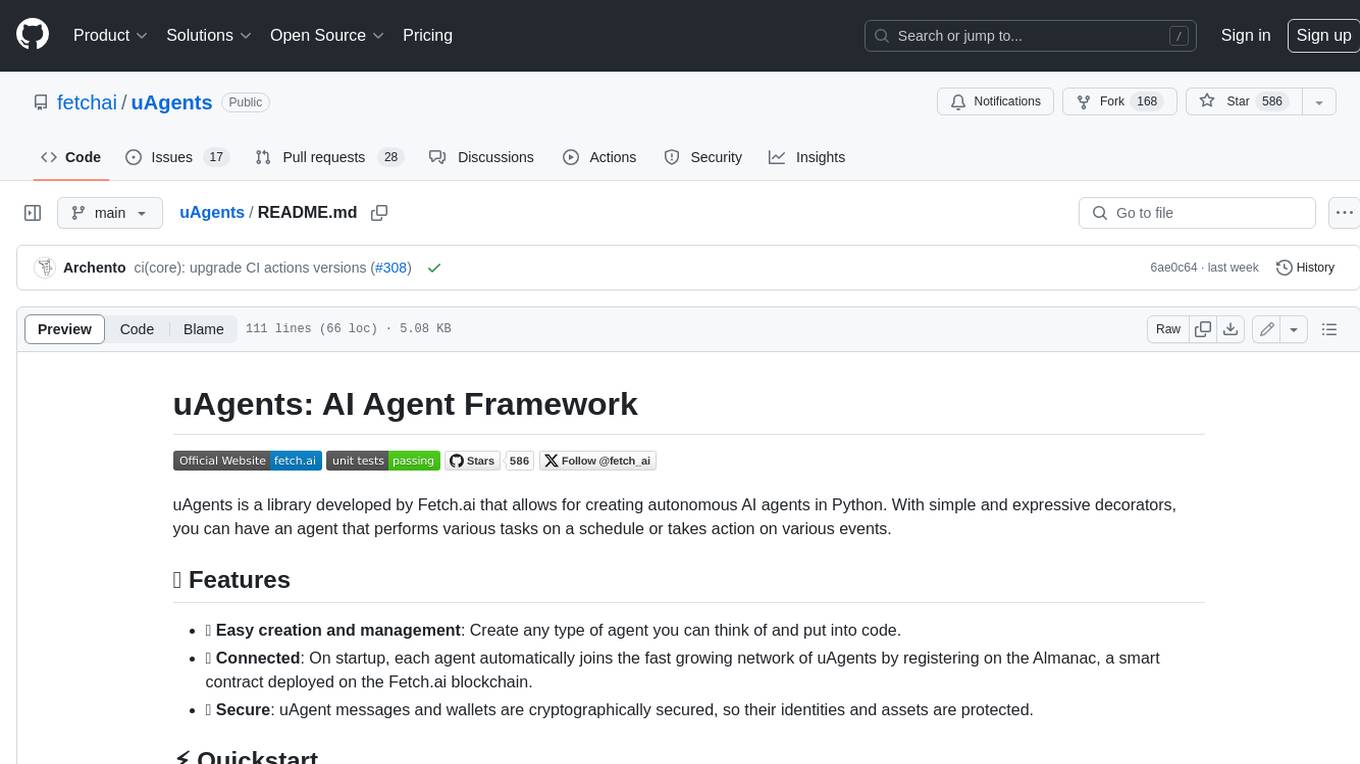
uAgents
uAgents is a Python library developed by Fetch.ai that allows for the creation of autonomous AI agents. These agents can perform various tasks on a schedule or take action on various events. uAgents are easy to create and manage, and they are connected to a fast-growing network of other uAgents. They are also secure, with cryptographically secured messages and wallets.
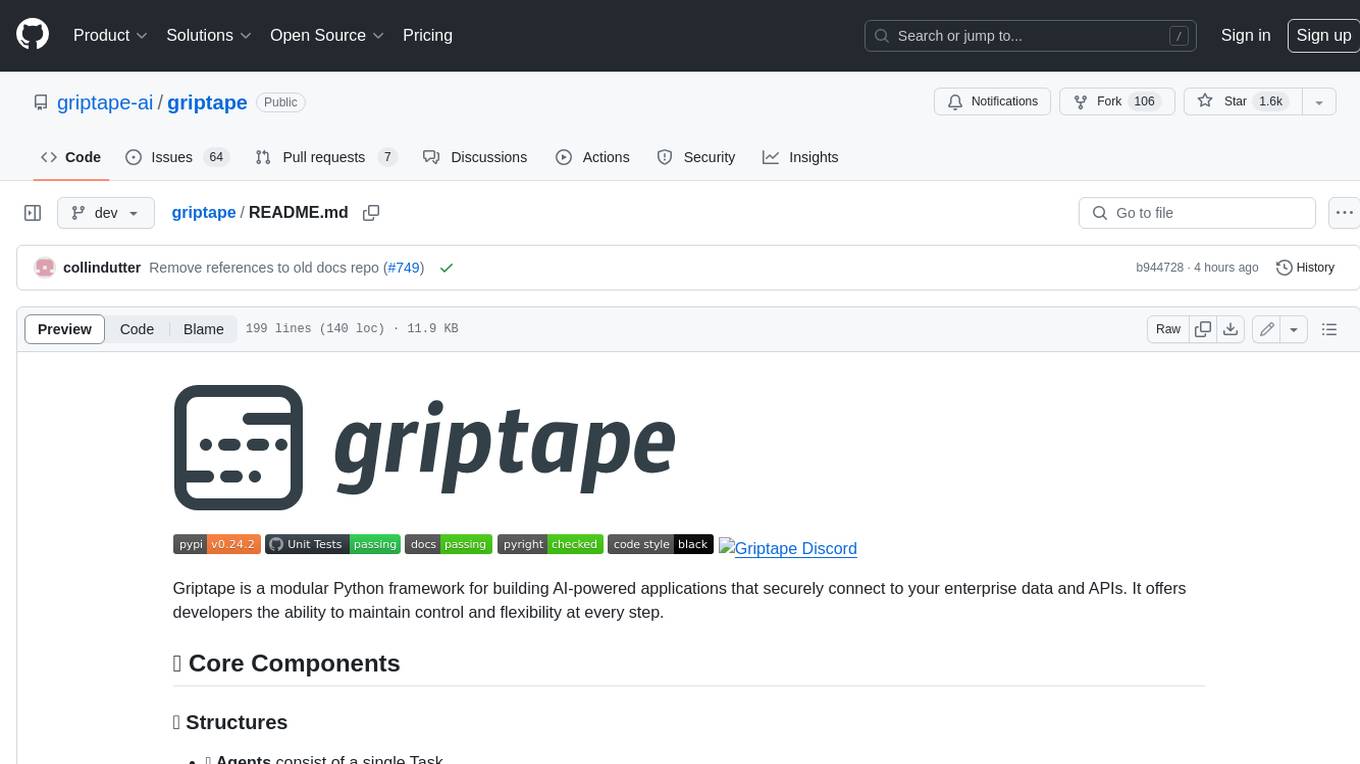
griptape
Griptape is a modular Python framework for building AI-powered applications that securely connect to your enterprise data and APIs. It offers developers the ability to maintain control and flexibility at every step. Griptape's core components include Structures (Agents, Pipelines, and Workflows), Tasks, Tools, Memory (Conversation Memory, Task Memory, and Meta Memory), Drivers (Prompt and Embedding Drivers, Vector Store Drivers, Image Generation Drivers, Image Query Drivers, SQL Drivers, Web Scraper Drivers, and Conversation Memory Drivers), Engines (Query Engines, Extraction Engines, Summary Engines, Image Generation Engines, and Image Query Engines), and additional components (Rulesets, Loaders, Artifacts, Chunkers, and Tokenizers). Griptape enables developers to create AI-powered applications with ease and efficiency.