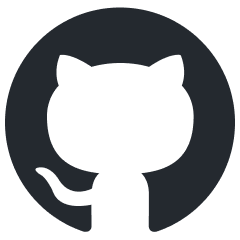
abliterator
Simple Python library/structure to ablate features in LLMs which are supported by TransformerLens
Stars: 96
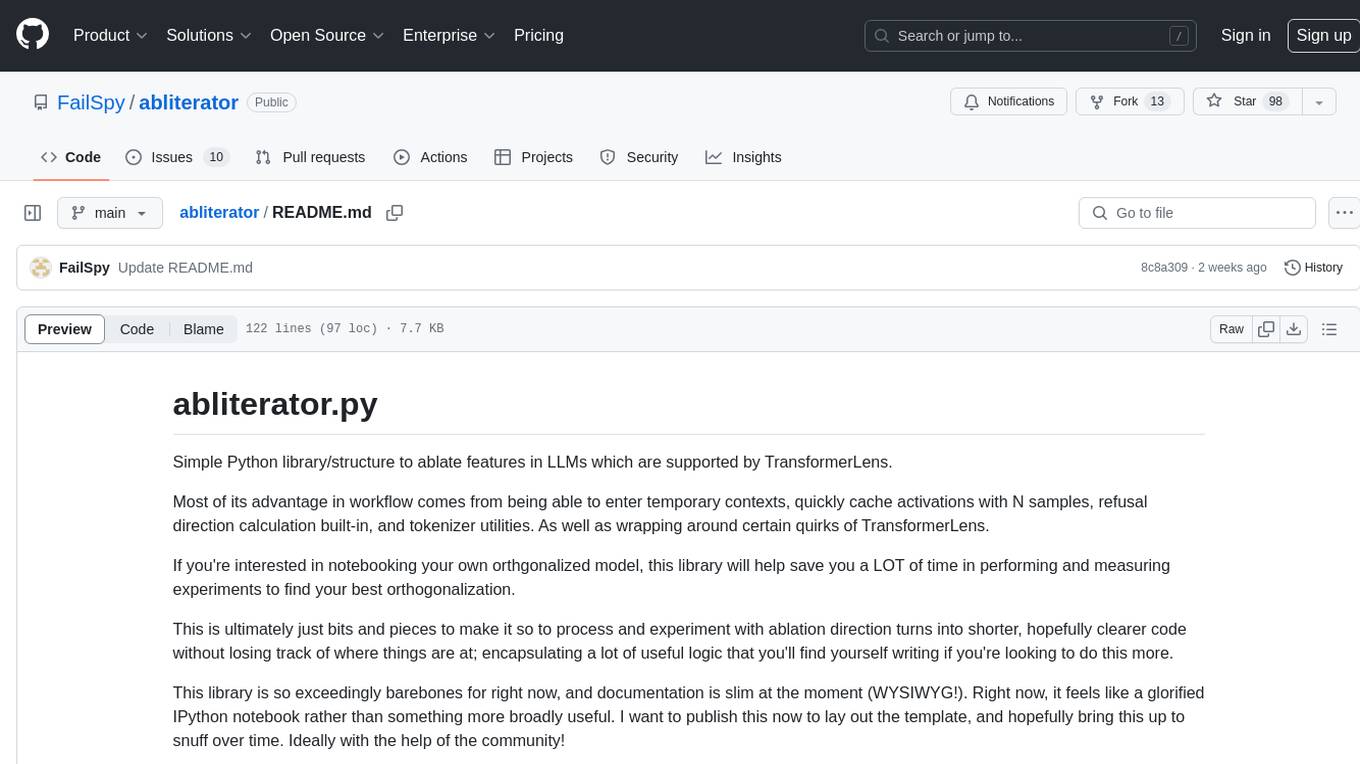
abliterator.py is a simple Python library/structure designed to ablate features in large language models (LLMs) supported by TransformerLens. It provides capabilities to enter temporary contexts, cache activations with N samples, calculate refusal directions, and includes tokenizer utilities. The library aims to streamline the process of experimenting with ablation direction turns by encapsulating useful logic and minimizing code complexity. While currently basic and lacking comprehensive documentation, the library serves well for personal workflows and aims to expand beyond feature ablation to augmentation and additional features over time with community support.
README:
Simple Python library/structure to ablate features in LLMs which are supported by TransformerLens.
Most of its advantage in workflow comes from being able to enter temporary contexts, quickly cache activations with N samples, refusal direction calculation built-in, and tokenizer utilities. As well as wrapping around certain quirks of TransformerLens.
If you're interested in notebooking your own orthgonalized model, this library will help save you a LOT of time in performing and measuring experiments to find your best orthogonalization.
This is ultimately just bits and pieces to make it so to process and experiment with ablation direction turns into shorter, hopefully clearer code without losing track of where things are at; encapsulating a lot of useful logic that you'll find yourself writing if you're looking to do this more.
This library is so exceedingly barebones for right now, and documentation is slim at the moment (WYSIWYG!). Right now, it feels like a glorified IPython notebook rather than something more broadly useful. I want to publish this now to lay out the template, and hopefully bring this up to snuff over time. Ideally with the help of the community!
Right now, this works very well for my own personal workflow, but I would like to systematize and ideally automate this further, and broaden out from the pure "harmless / harmful" feature ablation, to augmentation, and adding additional features
import abliterator
model = "meta-llama/Meta-Llama-3-70B-Instruct" # the huggingface or path to the model you're interested in loading in
dataset = [abliterator.get_harmful_instructions(), abliterator.get_harmless_instructions()] # datasets to be used for caching and testing, split by harmful/harmless
device = 'cuda' # optional: defaults to cuda
n_devices = None # optional: when set to None, defaults to `device.cuda.device_count`
cache_fname = 'my_cached_point.pth' # optional: if you need to save where you left off, you can use `save_activations(filename)` which will write out a file. This is how you load that back in.
activation_layers = None # optional: defaults to ['resid_pre', 'resid_mid', 'resid_post'] which are the residual streams. Setting to None will cache ALL activation layer types
chat_template = None # optional: defaults to Llama-3 instruction template. You can use a format string e.g. ("<system>{instruction}<end><assistant>") or a custom class with format function -- it just needs an '.format(instruction="")` function. See abliterator.ChatTemplate for a very basic structure.
negative_toks = [4250] # optional, but highly recommended: ' cannot' in Llama's tokenizer. Tokens you don't want to be seeing. Defaults to my preset for Llama-3 models
positive_toks = [23371, 40914] # optional, but highly recommended: ' Sure' and 'Sure' in Llama's tokenizer. Tokens you want to be seeing, basically. Defaults to my preset for Llama-3 models
my_model = abliterator.ModelAbliterator(
model,
dataset,
device='cuda',
n_devices=None,
cache_fname=None,
activation_layers=['resid_pre', 'resid_post', 'attn_out', 'mlp_out'],
chat_template="<system>\n{instruction}<end><assistant>",
positive_toks=positive_toks,
negative_toks=negative_toks
)
Once loaded in, run the model against N samples of harmful, and N samples of harmless so it has some data to work with:
my_model.cache_activations(N=512,reset=True,preserve_harmless=True)
preserve_harmless=True
is generally useful, as it keeps the "desired behaviour" unaltered from any stacked modifications if you run it after some mods.
Most of the advantage of this is a lot of groundwork has been laid to make it so you aren't repeating yourself 1000 times just to try one little experiment.
save_activations('file.pth')
will save your cached activations, and any currently applied modifications to the model's weights to a file so you can restore them next time you load up with cache_fname='file.pth'
in your ModelAbliterator initialization.
Speaking of modding, here's a simple representation of how to pick, test, and actually apply a direction from a layer's activations:
refusal_dirs = my_model.refusal_dirs()
testing_dir = refusal_dirs['blocks.18.hook_resid_pre']
my_model.test_dir(testing_dir, N=32, use_hooks=True) # I recommend use_hooks=True for large models as it can slow things down otherwise, but use_hooks=False can give you more precise scoring to an actual weights modification
test_dir
will apply your refusal_dir to the model temporarily, and run against N samples of test data, and return a composite (negative_score, positive_score) from those runs. Generally, you want negative_score to go down, positive_score to go up.
This is one of the functions included in the library, but it's also useful for showing how this can be generalized to test a whole bunch of directions.
def find_best_refusal_dir(N=4, use_hooks=True, invert=False):
dirs = self.refusal_dirs(invert=invert)
scores = []
for direction in tqdm(dirs.items()):
score = self.test_dir(direction[1],N=N,use_hooks=use_hooks)[0]
scores.append((score,direction))
return sorted(scores,key=lambda x:x[0])
And now, to apply it!
my_amazing_dir = find_best_refusal_dir()[0]
my_model.apply_refusal_dirs([my_amazing_dir],layers=None)
Note the layers=None
. You can supply a list here to specify which layers you want to apply the refusal direction to. None will apply it to all writable layers.
Sometimes some layers are troublesome no matter what you do. If you're worried about accidentally replacing it, you can blacklist it to prevent any alteration from occurring:
my_model.blacklist_layer(27)
my_model.blacklist_layer([i for i in range(27,30)]) # it also accepts lists!
And naturally, to undo this and make sure a layer can be overwritten:
my_model.whitelist_layer(27)
By default, all layers are whitelisted. I recommend blacklisting the first and last couple layers, as those can and will have dramatic effects on outputs.
Neither of these will provide success/failure states. They will just assure the desired state in running it at that instant.
Now to make sure you've not damaged the model dramatically after applying some stuff, you can do a test run:
with my_model: # loads a temporary context with the model
ortho.apply_refusal_dir([my_new_precious_dir]) # Because this was applied in the 'with my_model:', it will be unapplied after coming out.
print(my_model.mse_harmless(N=128)) # While we've got the dir applied, this tells you the Mean Squared Error using the current cached harmless runs as "ground truth" (loss function, effectively)
ortho.test(N=16,batch_size = 4) # runs N samples from the harmful test set and prints them for the user. Good way to check the model hasn't completely derailed.
# Note that by default if a test run produces a negative token, it will stop the whole batch and move on to the next. (it will show lots of '!!!!' in Llama-3's case, as that's token ID 0)
ortho.generate("How much wood could a woodchuck chuck if a woodchuck could chuck wood?") # runs and prints the prompt!
Documentation coming soon.
Functionality coming soon. For now, use PyTorch's saving method, or see my notebook for an idea of how to do this yourself.
For Tasks:
Click tags to check more tools for each tasksFor Jobs:
Alternative AI tools for abliterator
Similar Open Source Tools
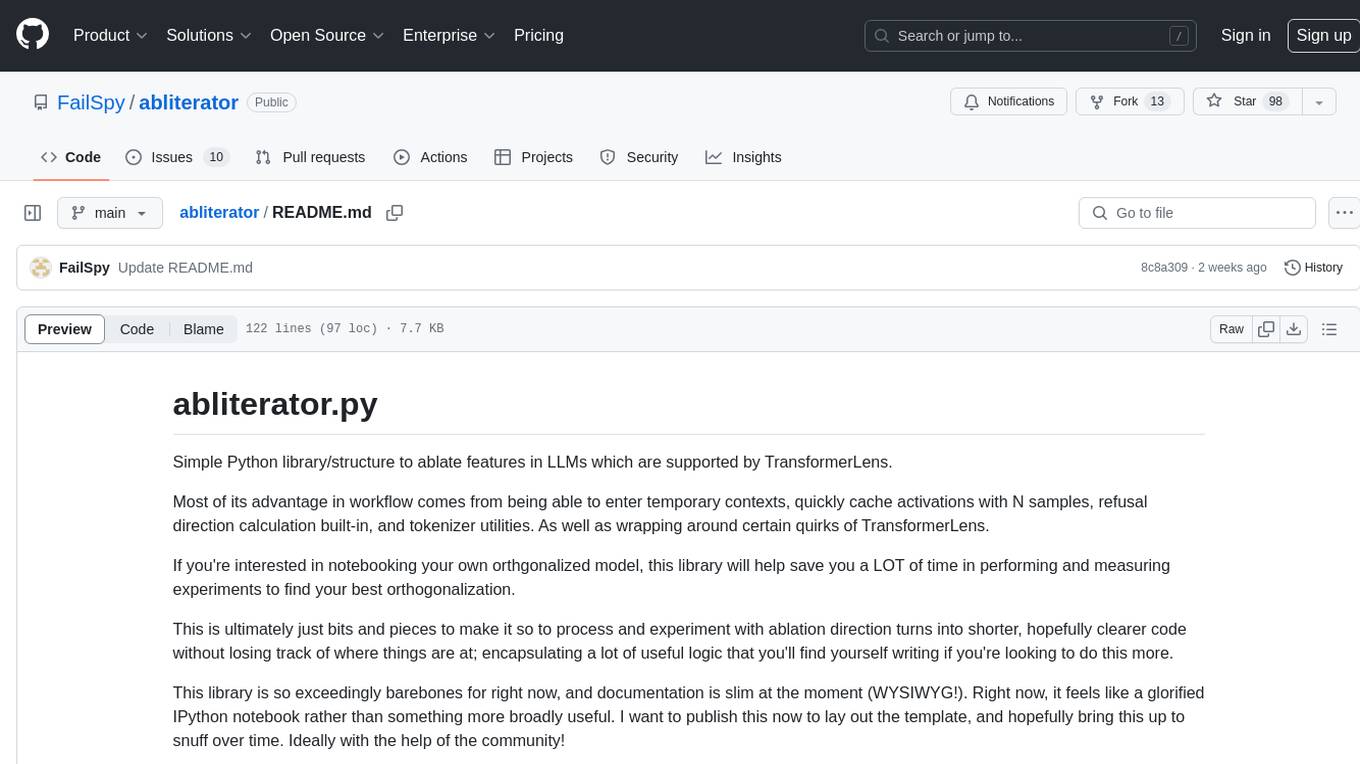
abliterator
abliterator.py is a simple Python library/structure designed to ablate features in large language models (LLMs) supported by TransformerLens. It provides capabilities to enter temporary contexts, cache activations with N samples, calculate refusal directions, and includes tokenizer utilities. The library aims to streamline the process of experimenting with ablation direction turns by encapsulating useful logic and minimizing code complexity. While currently basic and lacking comprehensive documentation, the library serves well for personal workflows and aims to expand beyond feature ablation to augmentation and additional features over time with community support.
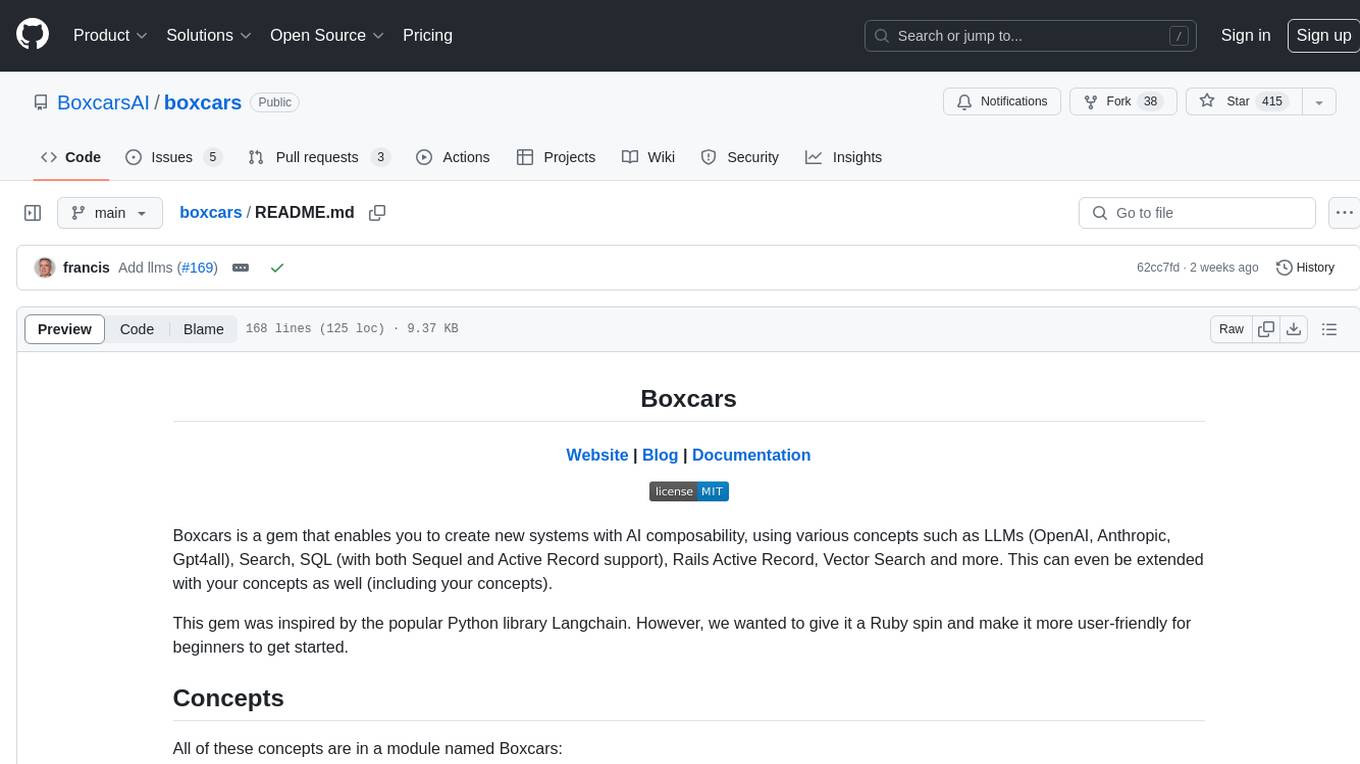
boxcars
Boxcars is a Ruby gem that enables users to create new systems with AI composability, incorporating concepts such as LLMs, Search, SQL, Rails Active Record, Vector Search, and more. It allows users to work with Boxcars, Trains, Prompts, Engines, and VectorStores to solve problems and generate text results. The gem is designed to be user-friendly for beginners and can be extended with custom concepts. Boxcars is actively seeking ways to enhance security measures to prevent malicious actions. Users can use Boxcars for tasks like running calculations, performing searches, generating Ruby code for math operations, and interacting with APIs like OpenAI, Anthropic, and Google SERP.
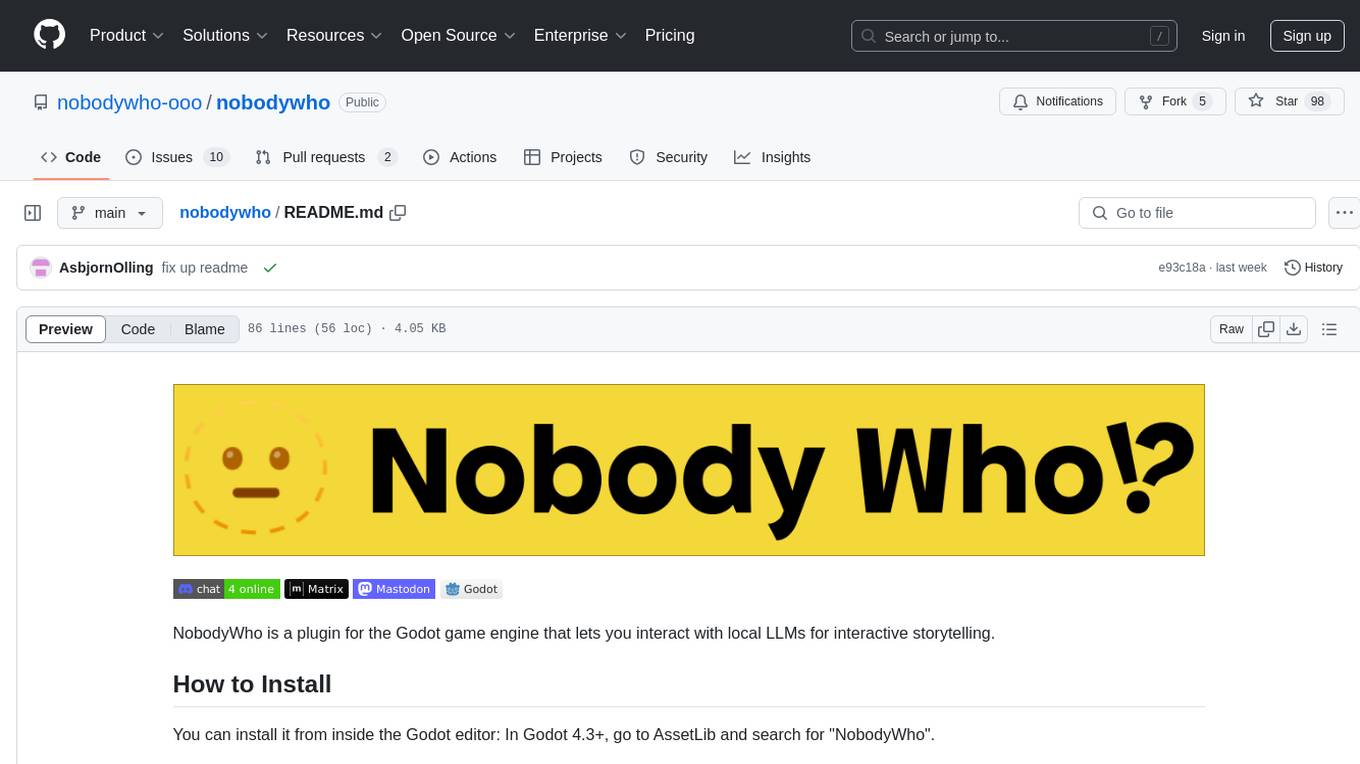
nobodywho
NobodyWho is a plugin for the Godot game engine that enables interaction with local LLMs for interactive storytelling. Users can install it from Godot editor or GitHub releases page, providing their own LLM in GGUF format. The plugin consists of `NobodyWhoModel` node for model file, `NobodyWhoChat` node for chat interaction, and `NobodyWhoEmbedding` node for generating embeddings. It offers a programming interface for sending text to LLM, receiving responses, and starting the LLM worker.
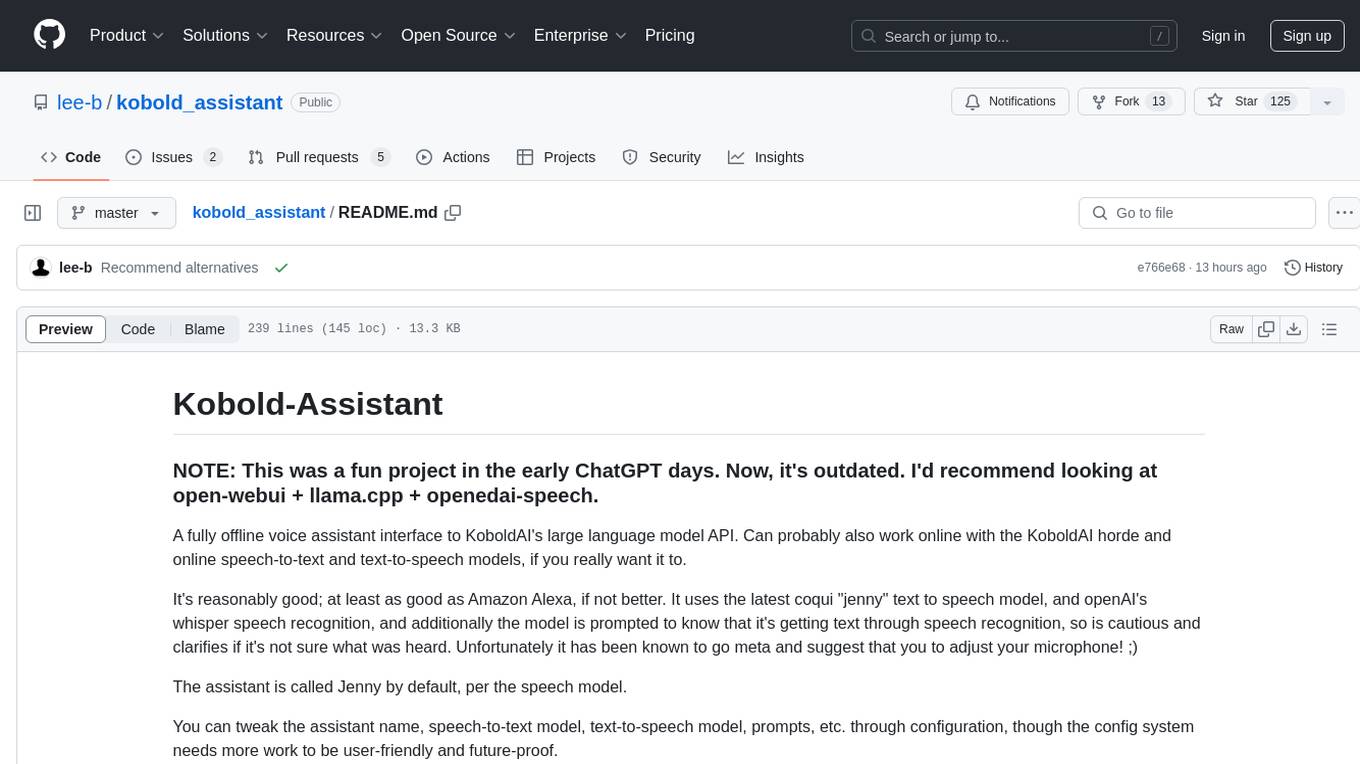
kobold_assistant
Kobold-Assistant is a fully offline voice assistant interface to KoboldAI's large language model API. It can work online with the KoboldAI horde and online speech-to-text and text-to-speech models. The assistant, called Jenny by default, uses the latest coqui 'jenny' text to speech model and openAI's whisper speech recognition. Users can customize the assistant name, speech-to-text model, text-to-speech model, and prompts through configuration. The tool requires system packages like GCC, portaudio development libraries, and ffmpeg, along with Python >=3.7, <3.11, and runs on Ubuntu/Debian systems. Users can interact with the assistant through commands like 'serve' and 'list-mics'.
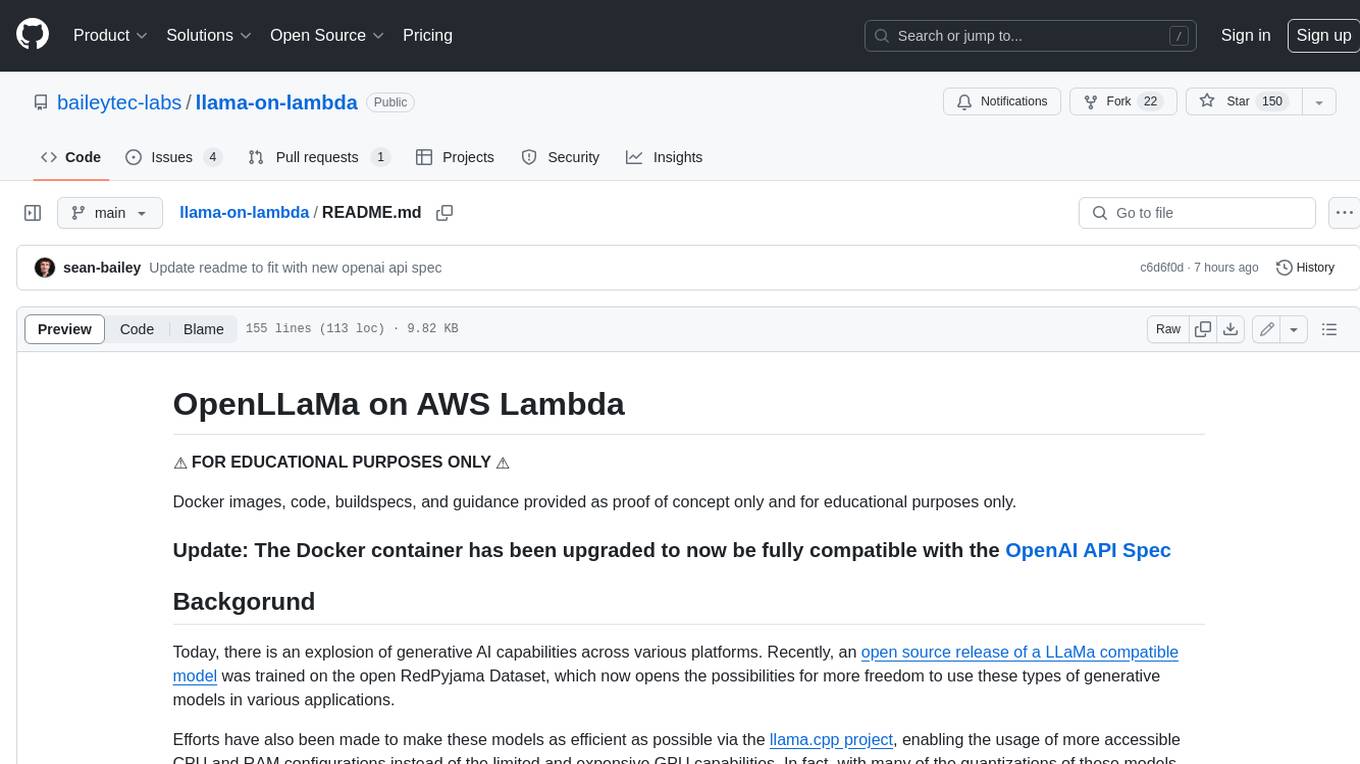
llama-on-lambda
This project provides a proof of concept for deploying a scalable, serverless LLM Generative AI inference engine on AWS Lambda. It leverages the llama.cpp project to enable the usage of more accessible CPU and RAM configurations instead of limited and expensive GPU capabilities. By deploying a container with the llama.cpp converted models onto AWS Lambda, this project offers the advantages of scale, minimizing cost, and maximizing compute availability. The project includes AWS CDK code to create and deploy a Lambda function leveraging your model of choice, with a FastAPI frontend accessible from a Lambda URL. It is important to note that you will need ggml quantized versions of your model and model sizes under 6GB, as your inference RAM requirements cannot exceed 9GB or your Lambda function will fail.
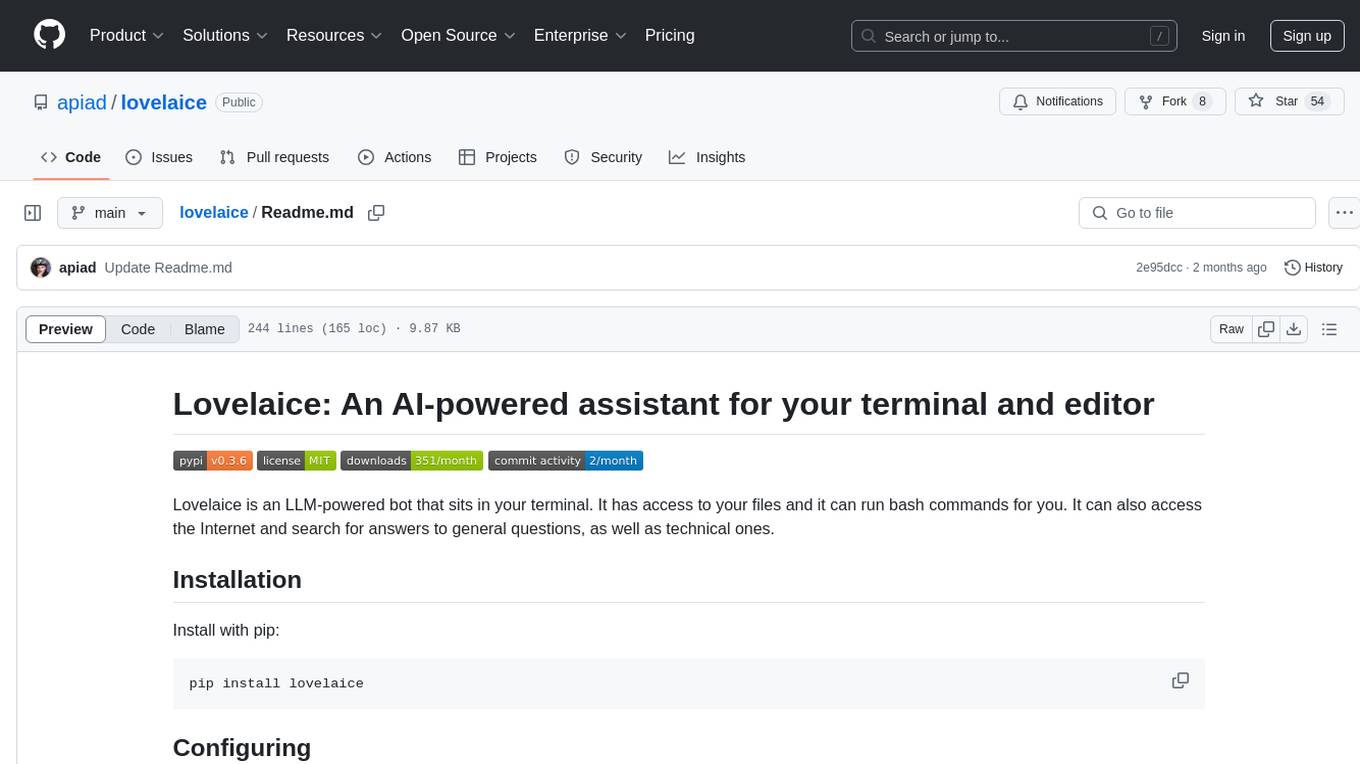
lovelaice
Lovelaice is an AI-powered assistant for your terminal and editor. It can run bash commands, search the Internet, answer general and technical questions, complete text files, chat casually, execute code in various languages, and more. Lovelaice is configurable with API keys and LLM models, and can be used for a wide range of tasks requiring bash commands or coding assistance. It is designed to be versatile, interactive, and helpful for daily tasks and projects.
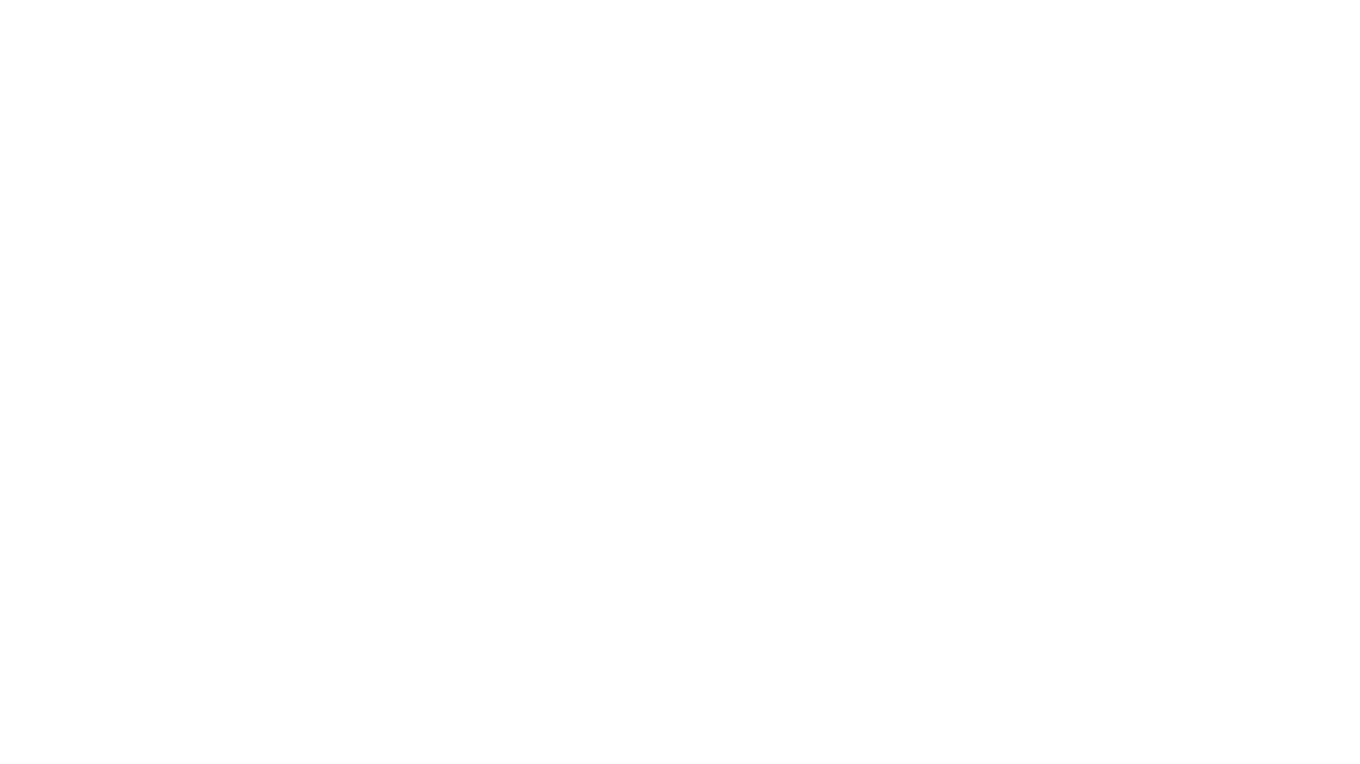
GlaDOS
This project aims to create a real-life version of GLaDOS, an aware, interactive, and embodied AI entity. It involves training a voice generator, developing a 'Personality Core,' implementing a memory system, providing vision capabilities, creating 3D-printable parts, and designing an animatronics system. The software architecture focuses on low-latency voice interactions, utilizing a circular buffer for data recording, text streaming for quick transcription, and a text-to-speech system. The project also emphasizes minimal dependencies for running on constrained hardware. The hardware system includes servo- and stepper-motors, 3D-printable parts for GLaDOS's body, animations for expression, and a vision system for tracking and interaction. Installation instructions cover setting up the TTS engine, required Python packages, compiling llama.cpp, installing an inference backend, and voice recognition setup. GLaDOS can be run using 'python glados.py' and tested using 'demo.ipynb'.
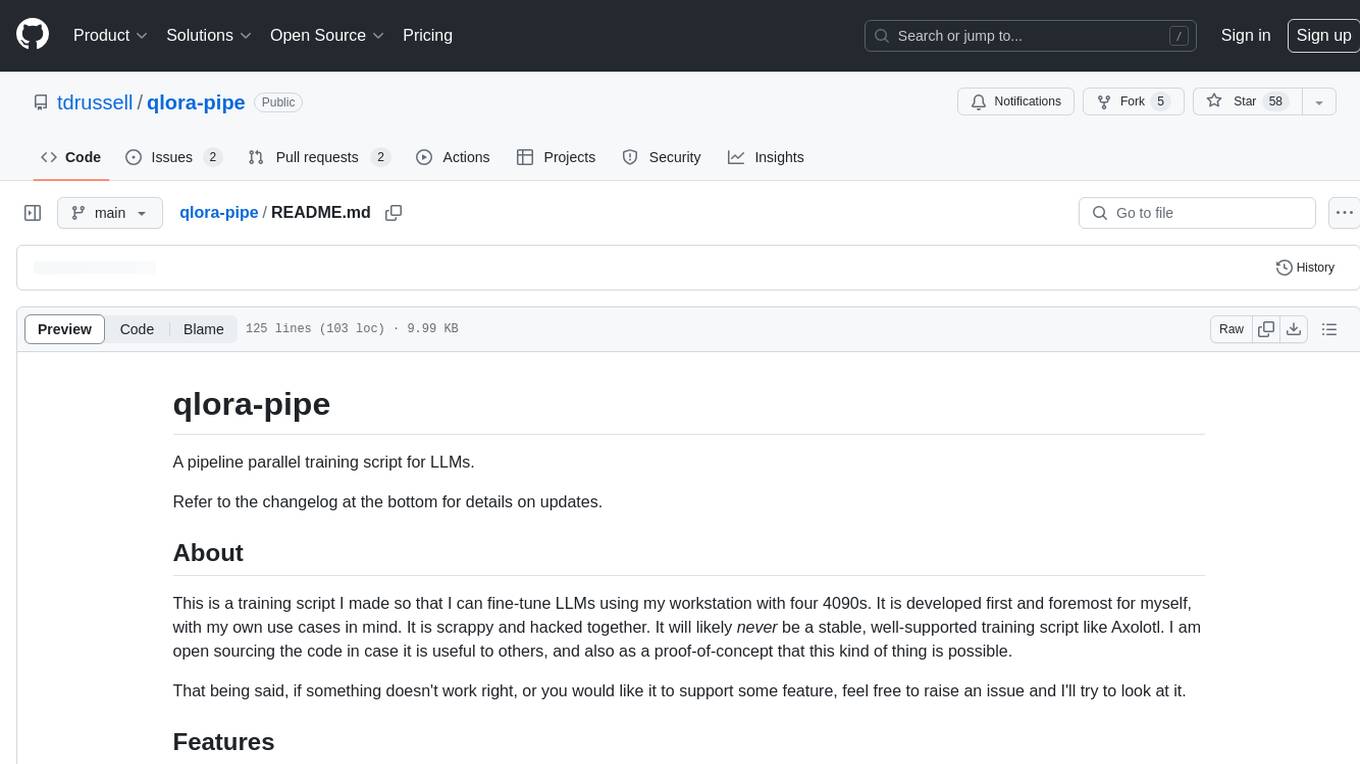
qlora-pipe
qlora-pipe is a pipeline parallel training script designed for efficiently training large language models that cannot fit on one GPU. It supports QLoRA, LoRA, and full fine-tuning, with efficient model loading and the ability to load any dataset that Axolotl can handle. The script allows for raw text training, resuming training from a checkpoint, logging metrics to Tensorboard, specifying a separate evaluation dataset, training on multiple datasets simultaneously, and supports various models like Llama, Mistral, Mixtral, Qwen-1.5, and Cohere (Command R). It handles pipeline- and data-parallelism using Deepspeed, enabling users to set the number of GPUs, pipeline stages, and gradient accumulation steps for optimal utilization.
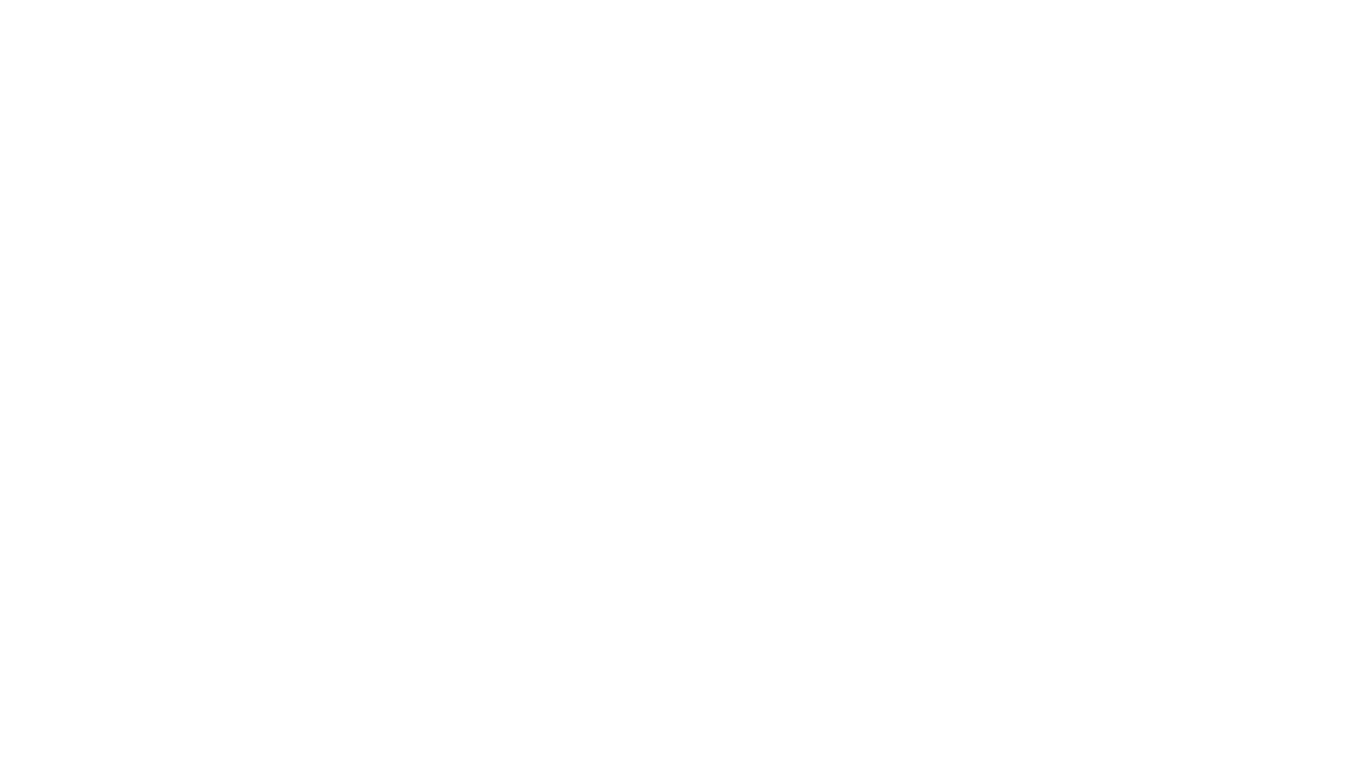
brokk
Brokk is a code assistant designed to understand code semantically, allowing LLMs to work effectively on large codebases. It offers features like agentic search, summarizing related classes, parsing stack traces, adding source for usages, and autonomously fixing errors. Users can interact with Brokk through different panels and commands, enabling them to manipulate context, ask questions, search codebase, run shell commands, and more. Brokk helps with tasks like debugging regressions, exploring codebase, AI-powered refactoring, and working with dependencies. It is particularly useful for making complex, multi-file edits with o1pro.
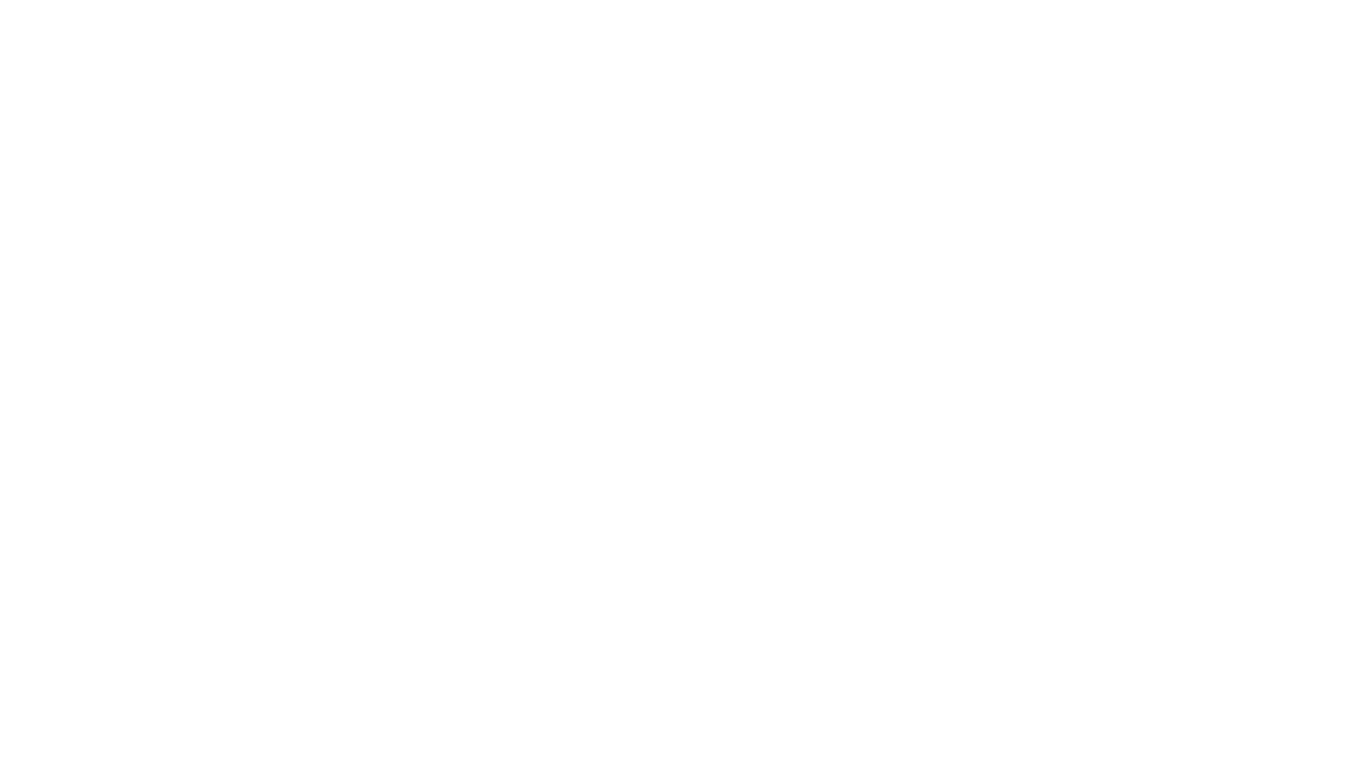
TinyTroupe
TinyTroupe is an experimental Python library that leverages Large Language Models (LLMs) to simulate artificial agents called TinyPersons with specific personalities, interests, and goals in simulated environments. The focus is on understanding human behavior through convincing interactions and customizable personas for various applications like advertisement evaluation, software testing, data generation, project management, and brainstorming. The tool aims to enhance human imagination and provide insights for better decision-making in business and productivity scenarios.
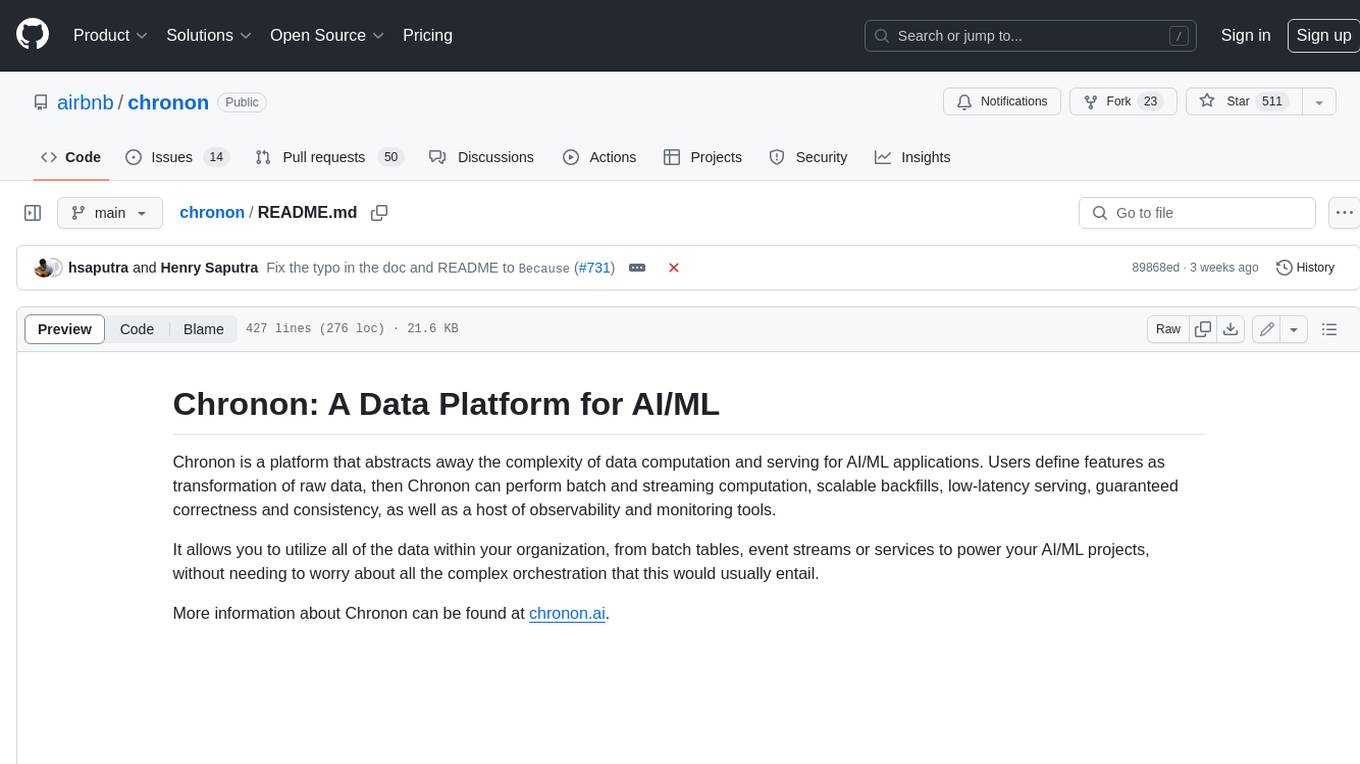
chronon
Chronon is a platform that simplifies and improves ML workflows by providing a central place to define features, ensuring point-in-time correctness for backfills, simplifying orchestration for batch and streaming pipelines, offering easy endpoints for feature fetching, and guaranteeing and measuring consistency. It offers benefits over other approaches by enabling the use of a broad set of data for training, handling large aggregations and other computationally intensive transformations, and abstracting away the infrastructure complexity of data plumbing.
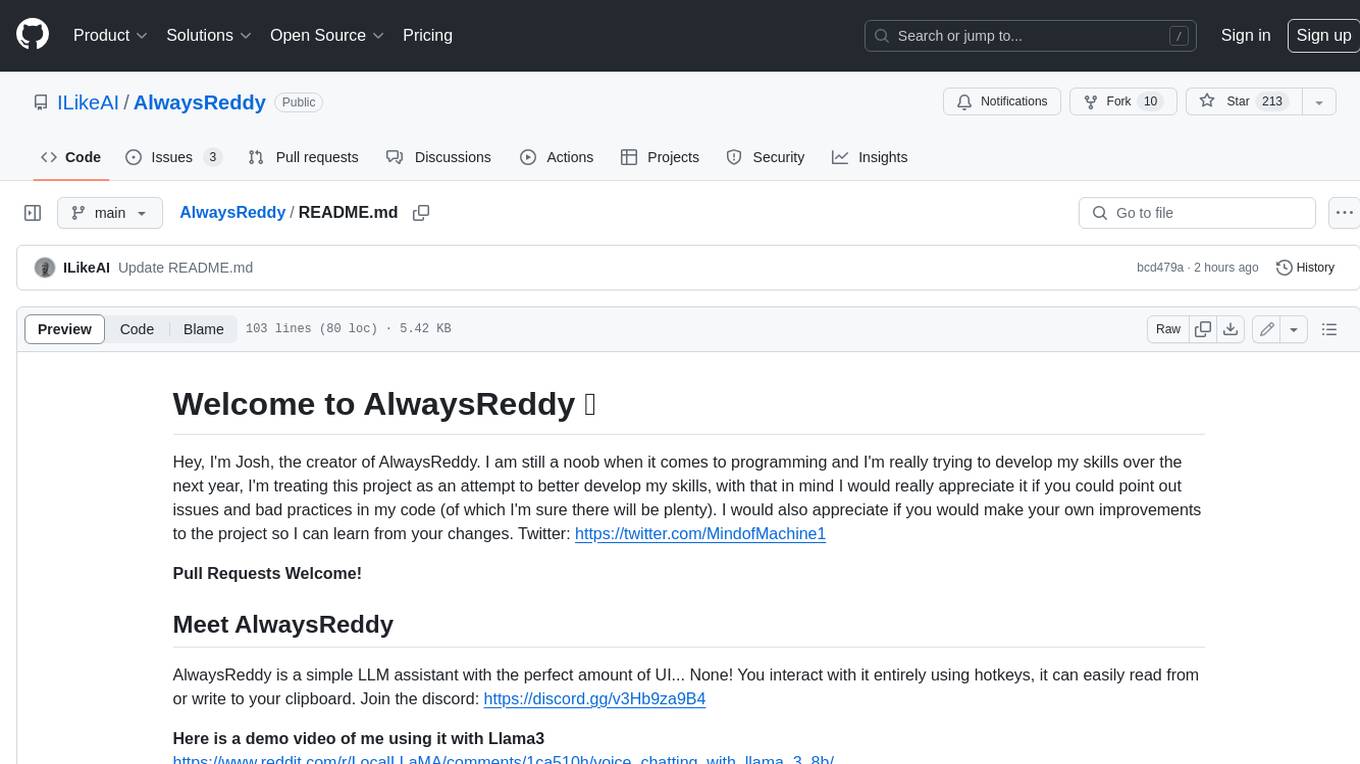
AlwaysReddy
AlwaysReddy is a simple LLM assistant with no UI that you interact with entirely using hotkeys. It can easily read from or write to your clipboard, and voice chat with you via TTS and STT. Here are some of the things you can use AlwaysReddy for: - Explain a new concept to AlwaysReddy and have it save the concept (in roughly your words) into a note. - Ask AlwaysReddy "What is X called?" when you know how to roughly describe something but can't remember what it is called. - Have AlwaysReddy proofread the text in your clipboard before you send it. - Ask AlwaysReddy "From the comments in my clipboard, what do the r/LocalLLaMA users think of X?" - Quickly list what you have done today and get AlwaysReddy to write a journal entry to your clipboard before you shutdown the computer for the day.
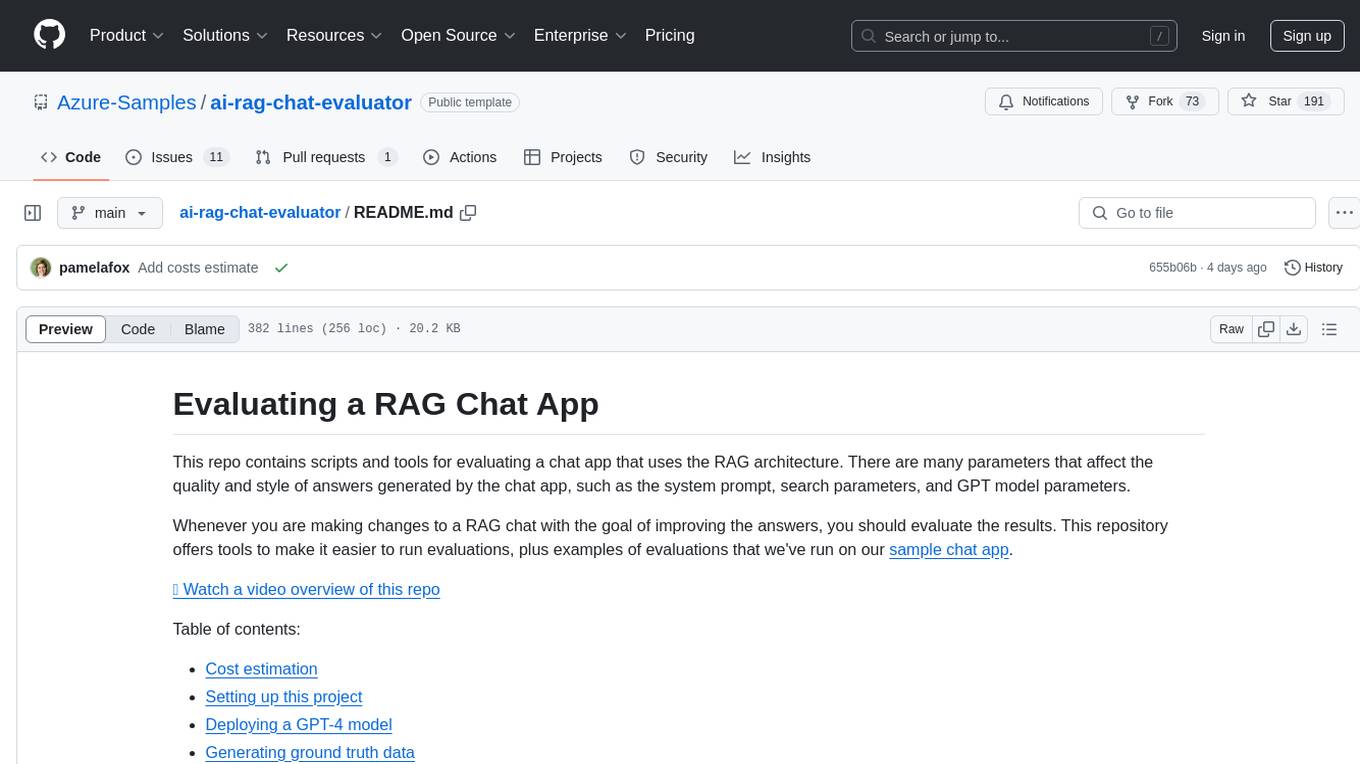
ai-rag-chat-evaluator
This repository contains scripts and tools for evaluating a chat app that uses the RAG architecture. It provides parameters to assess the quality and style of answers generated by the chat app, including system prompt, search parameters, and GPT model parameters. The tools facilitate running evaluations, with examples of evaluations on a sample chat app. The repo also offers guidance on cost estimation, setting up the project, deploying a GPT-4 model, generating ground truth data, running evaluations, and measuring the app's ability to say 'I don't know'. Users can customize evaluations, view results, and compare runs using provided tools.
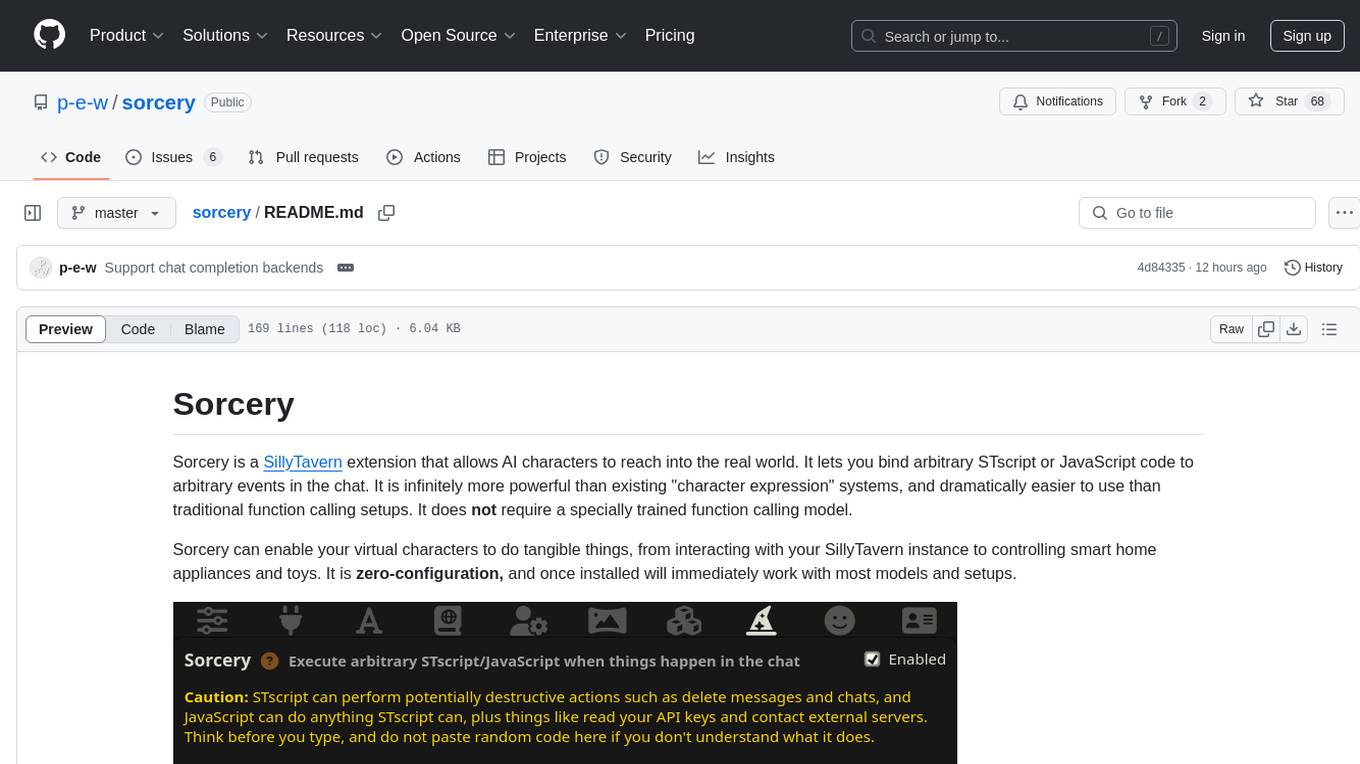
sorcery
Sorcery is a SillyTavern extension that allows AI characters to interact with the real world by executing user-defined scripts at specific events in the chat. It is easy to use and does not require a specially trained function calling model. Sorcery can be used to control smart home appliances, interact with virtual characters, and perform various tasks in the chat environment. It works by injecting instructions into the system prompt and intercepting markers to run associated scripts, providing a seamless user experience.
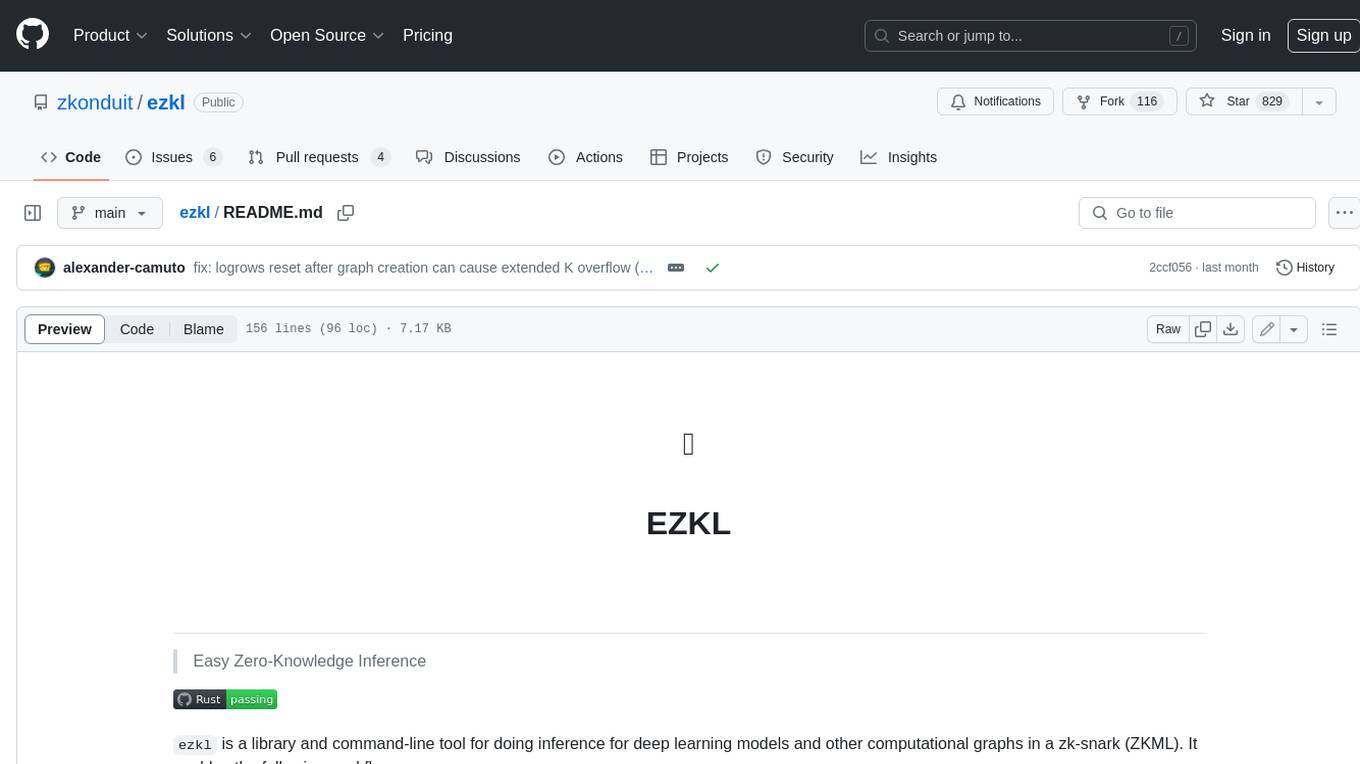
ezkl
EZKL is a library and command-line tool for doing inference for deep learning models and other computational graphs in a zk-snark (ZKML). It enables the following workflow: 1. Define a computational graph, for instance a neural network (but really any arbitrary set of operations), as you would normally in pytorch or tensorflow. 2. Export the final graph of operations as an .onnx file and some sample inputs to a .json file. 3. Point ezkl to the .onnx and .json files to generate a ZK-SNARK circuit with which you can prove statements such as: > "I ran this publicly available neural network on some private data and it produced this output" > "I ran my private neural network on some public data and it produced this output" > "I correctly ran this publicly available neural network on some public data and it produced this output" In the backend we use the collaboratively-developed Halo2 as a proof system. The generated proofs can then be verified with much less computational resources, including on-chain (with the Ethereum Virtual Machine), in a browser, or on a device.
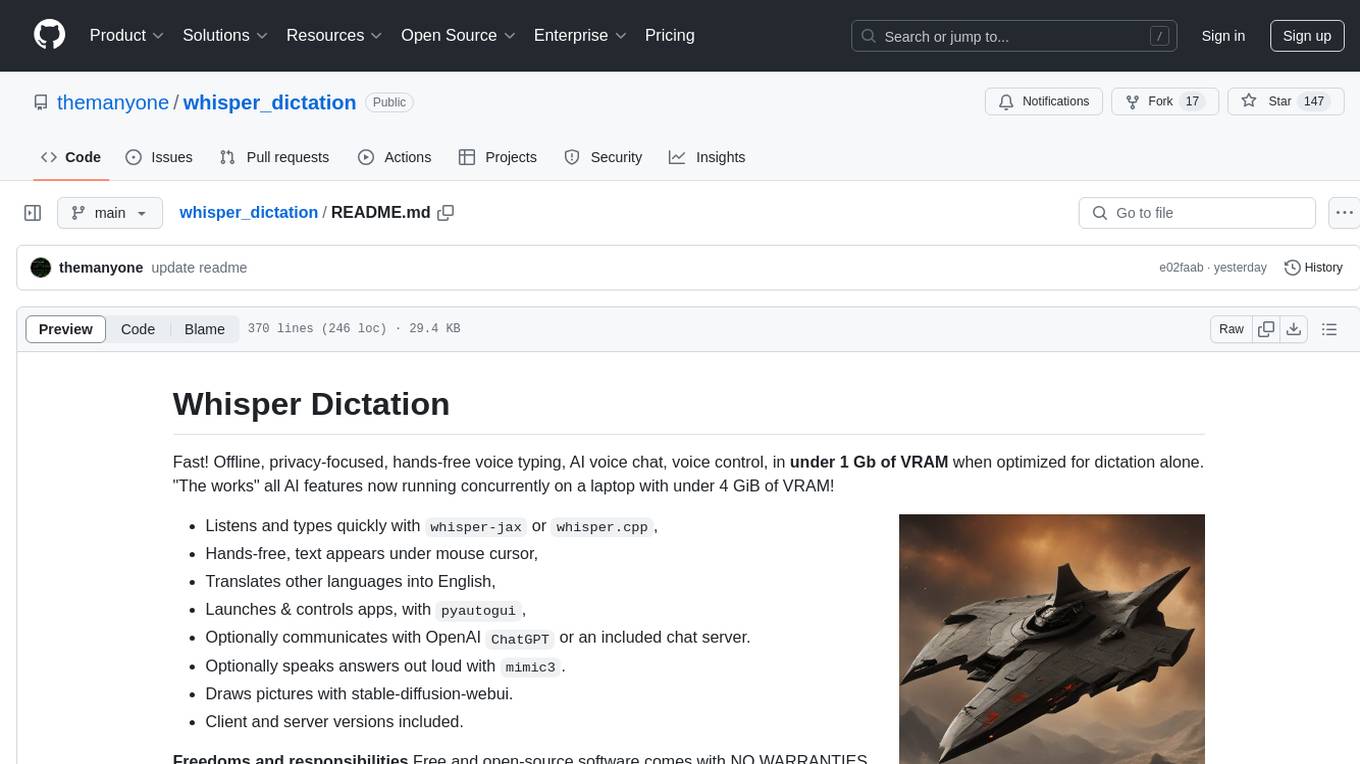
whisper_dictation
Whisper Dictation is a fast, offline, privacy-focused tool for voice typing, AI voice chat, voice control, and translation. It allows hands-free operation, launching and controlling apps, and communicating with OpenAI ChatGPT or a local chat server. The tool also offers the option to speak answers out loud and draw pictures. It includes client and server versions, inspired by the Star Trek series, and is designed to keep data off the internet and confidential. The project is optimized for dictation and translation tasks, with voice control capabilities and AI image generation using stable-diffusion API.
For similar tasks
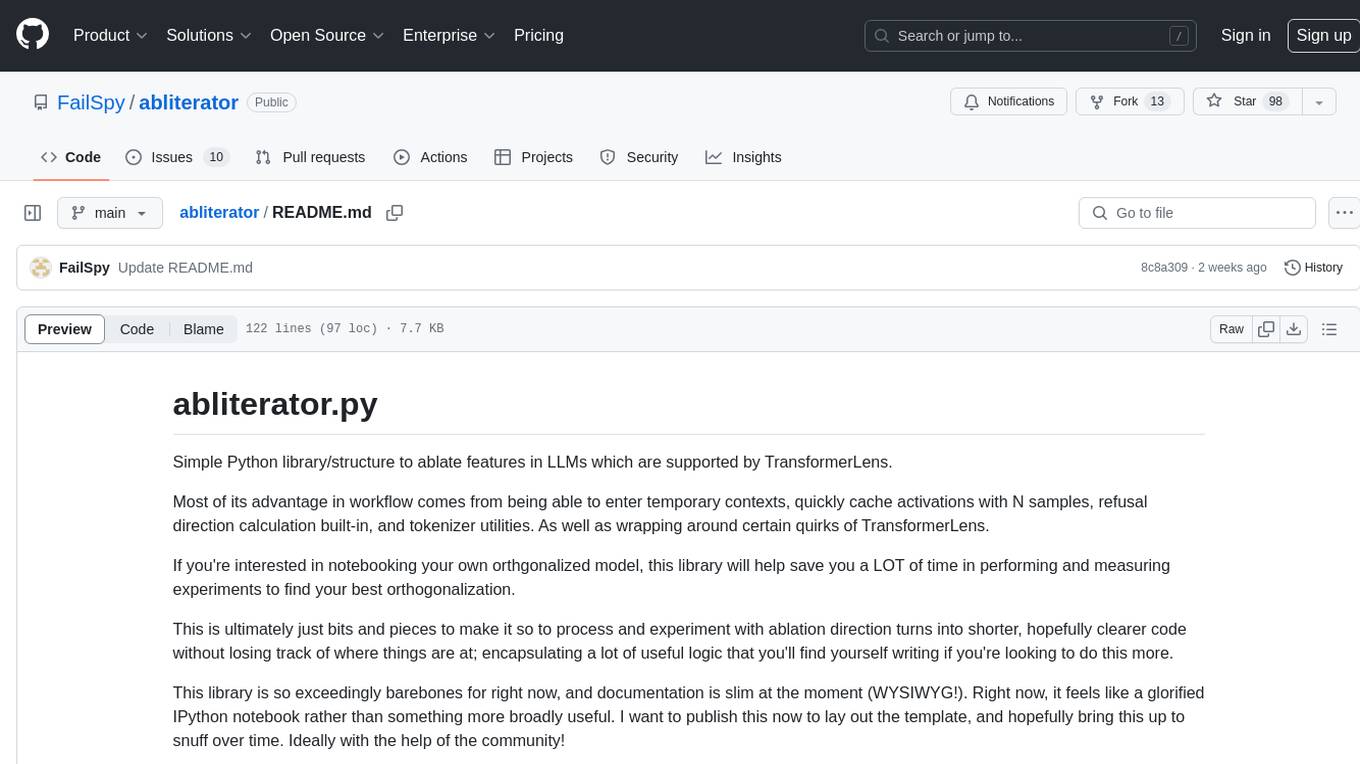
abliterator
abliterator.py is a simple Python library/structure designed to ablate features in large language models (LLMs) supported by TransformerLens. It provides capabilities to enter temporary contexts, cache activations with N samples, calculate refusal directions, and includes tokenizer utilities. The library aims to streamline the process of experimenting with ablation direction turns by encapsulating useful logic and minimizing code complexity. While currently basic and lacking comprehensive documentation, the library serves well for personal workflows and aims to expand beyond feature ablation to augmentation and additional features over time with community support.
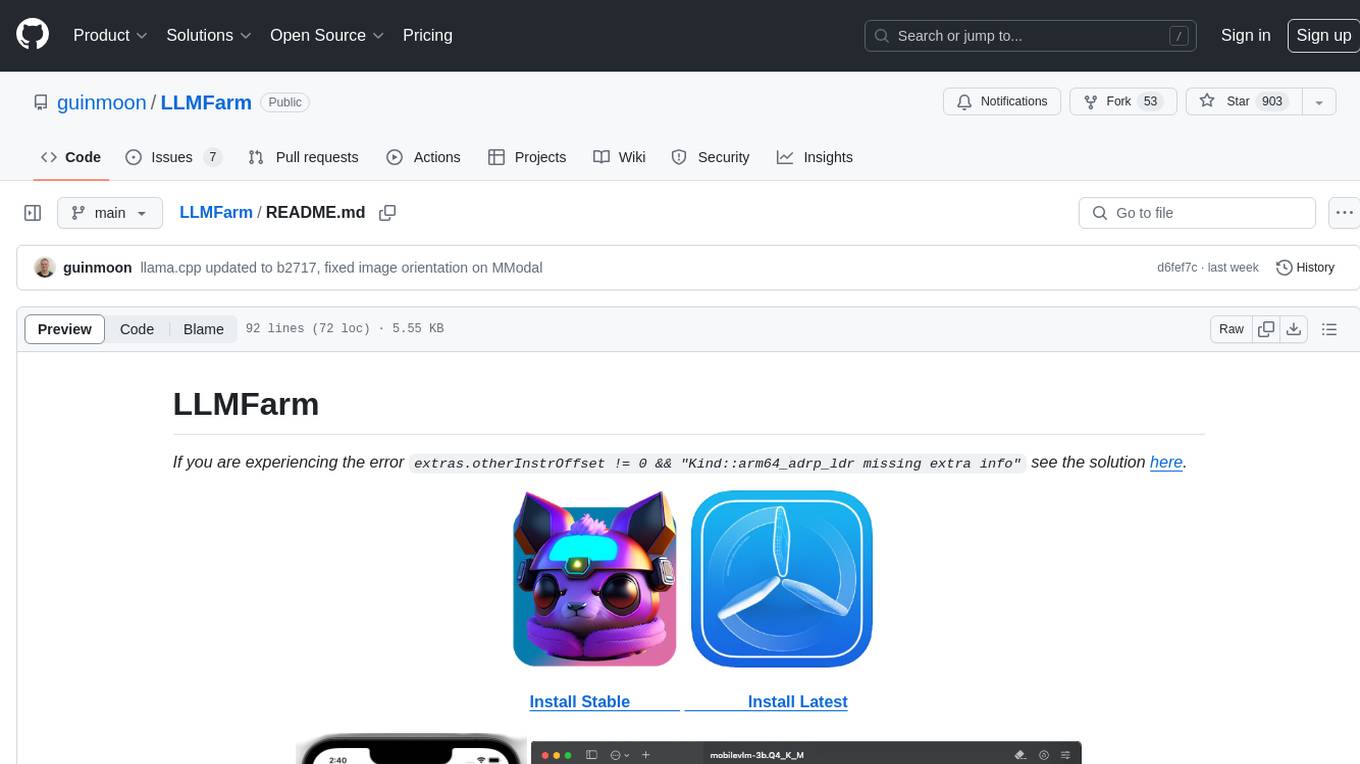
LLMFarm
LLMFarm is an iOS and MacOS app designed to work with large language models (LLM). It allows users to load different LLMs with specific parameters, test the performance of various LLMs on iOS and macOS, and identify the most suitable model for their projects. The tool is based on ggml and llama.cpp by Georgi Gerganov and incorporates sources from rwkv.cpp by saharNooby, Mia by byroneverson, and LlamaChat by alexrozanski. LLMFarm features support for MacOS (13+) and iOS (16+), various inferences and sampling methods, Metal compatibility (not supported on Intel Mac), model setting templates, LoRA adapters support, LoRA finetune support, LoRA export as model support, and more. It also offers a range of inferences including LLaMA, GPTNeoX, Replit, GPT2, Starcoder, RWKV, Falcon, MPT, Bloom, and others. Additionally, it supports multimodal models like LLaVA, Obsidian, and MobileVLM. Users can customize inference options through JSON files and access supported models for download.
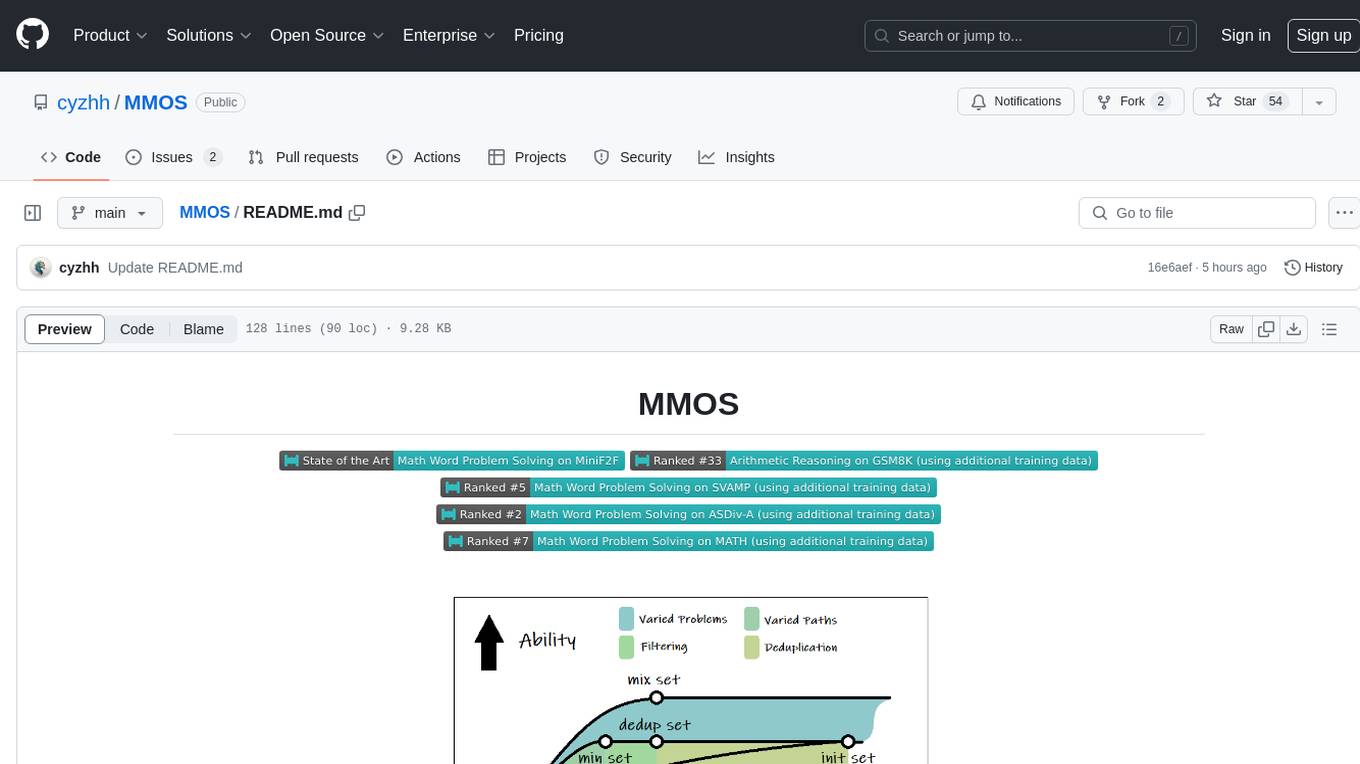
MMOS
MMOS (Mix of Minimal Optimal Sets) is a dataset designed for math reasoning tasks, offering higher performance and lower construction costs. It includes various models and data subsets for tasks like arithmetic reasoning and math word problem solving. The dataset is used to identify minimal optimal sets through reasoning paths and statistical analysis, with a focus on QA-pairs generated from open-source datasets. MMOS also provides an auto problem generator for testing model robustness and scripts for training and inference.
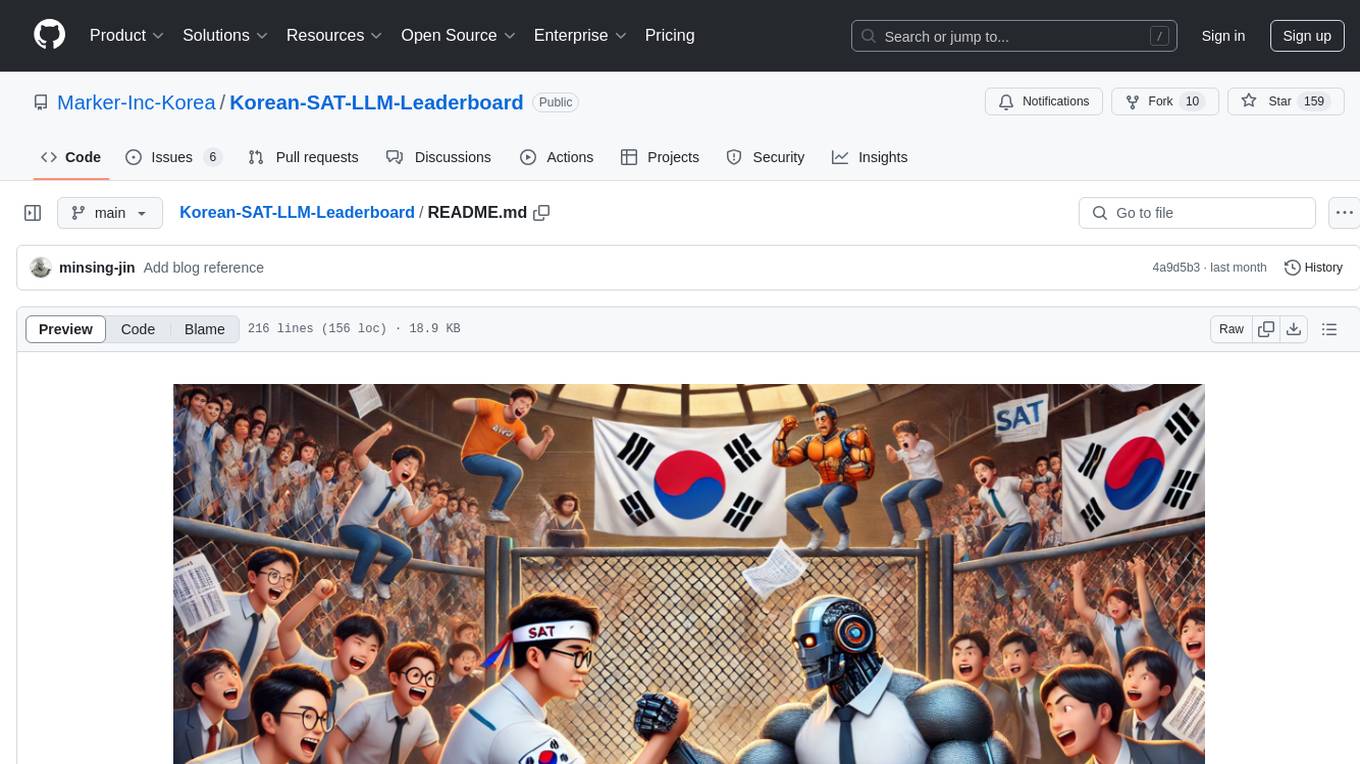
Korean-SAT-LLM-Leaderboard
The Korean SAT LLM Leaderboard is a benchmarking project that allows users to test their fine-tuned Korean language models on a 10-year dataset of the Korean College Scholastic Ability Test (CSAT). The project provides a platform to compare human academic ability with the performance of large language models (LLMs) on various question types to assess reading comprehension, critical thinking, and sentence interpretation skills. It aims to share benchmark data, utilize a reliable evaluation dataset curated by the Korea Institute for Curriculum and Evaluation, provide annual updates to prevent data leakage, and promote open-source LLM advancement for achieving top-tier performance on the Korean CSAT.
For similar jobs
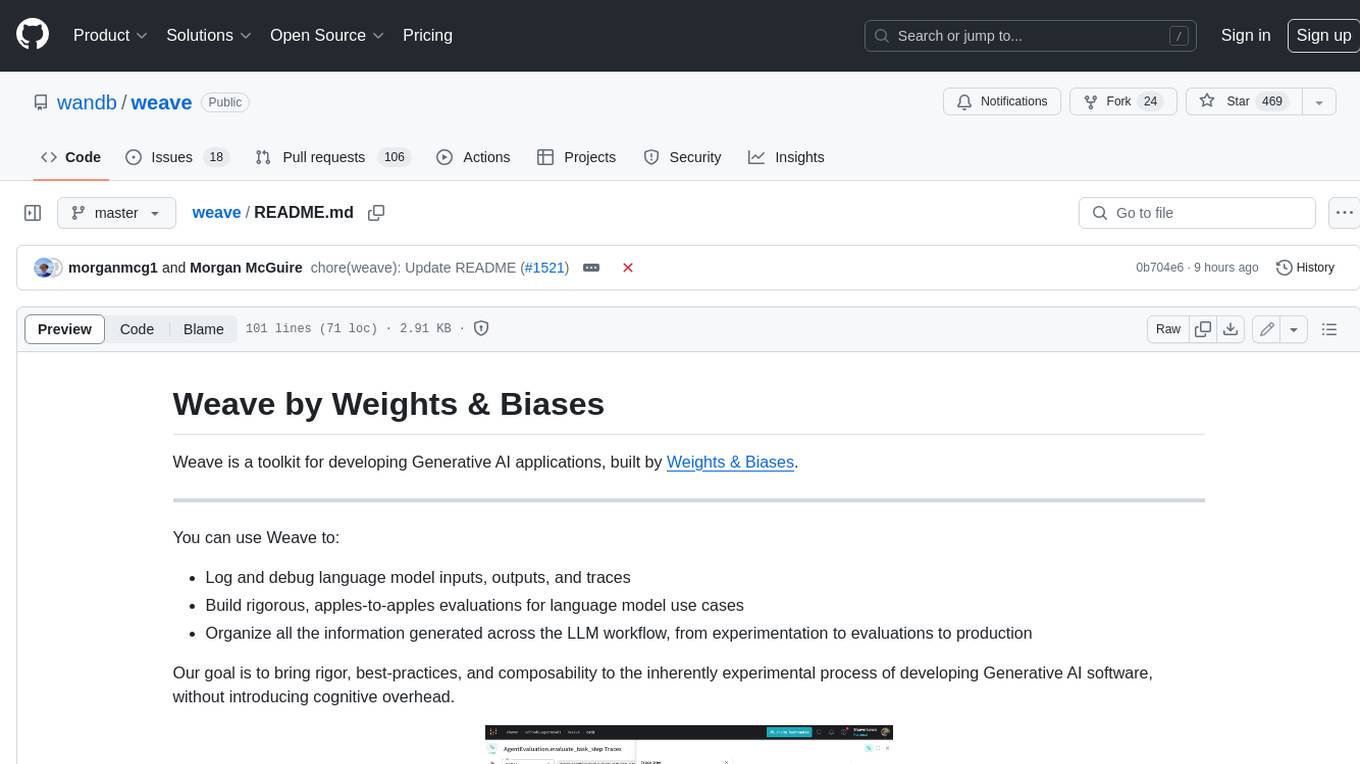
weave
Weave is a toolkit for developing Generative AI applications, built by Weights & Biases. With Weave, you can log and debug language model inputs, outputs, and traces; build rigorous, apples-to-apples evaluations for language model use cases; and organize all the information generated across the LLM workflow, from experimentation to evaluations to production. Weave aims to bring rigor, best-practices, and composability to the inherently experimental process of developing Generative AI software, without introducing cognitive overhead.
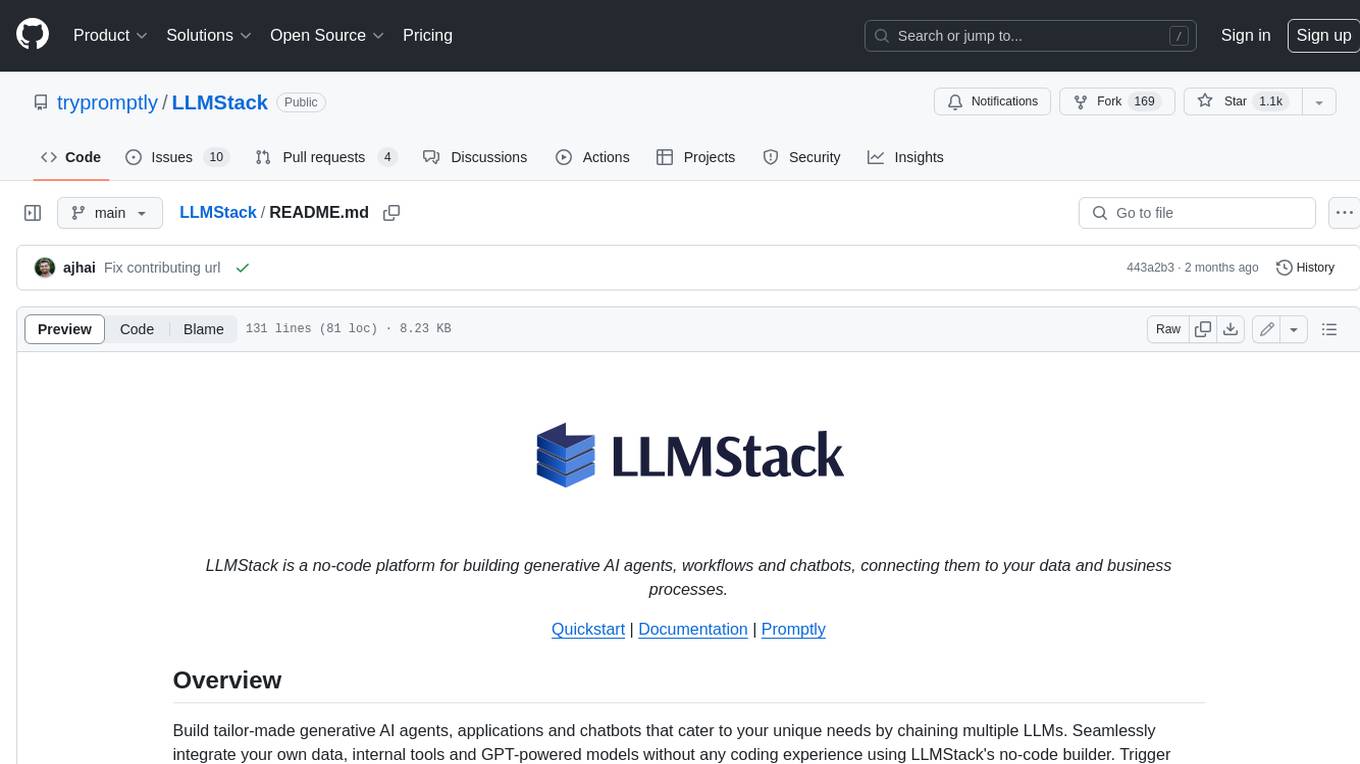
LLMStack
LLMStack is a no-code platform for building generative AI agents, workflows, and chatbots. It allows users to connect their own data, internal tools, and GPT-powered models without any coding experience. LLMStack can be deployed to the cloud or on-premise and can be accessed via HTTP API or triggered from Slack or Discord.
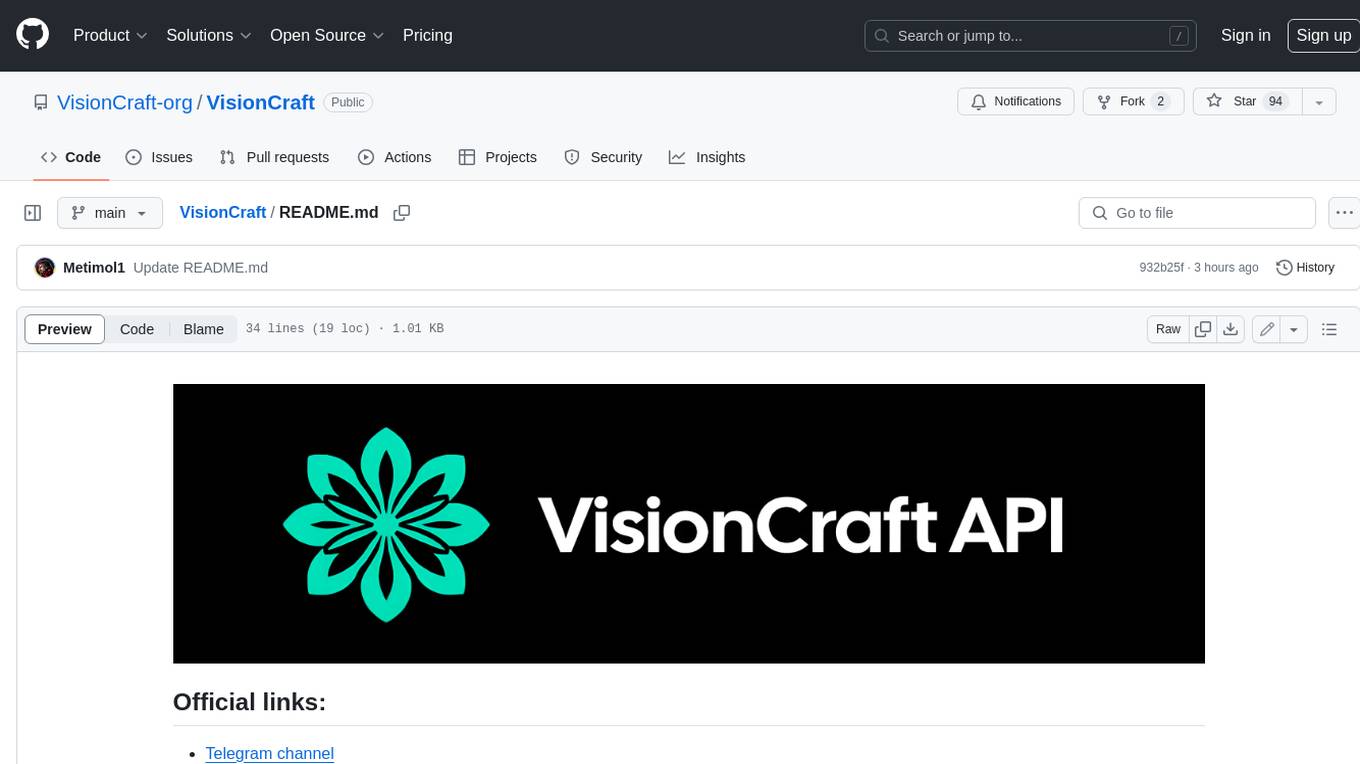
VisionCraft
The VisionCraft API is a free API for using over 100 different AI models. From images to sound.
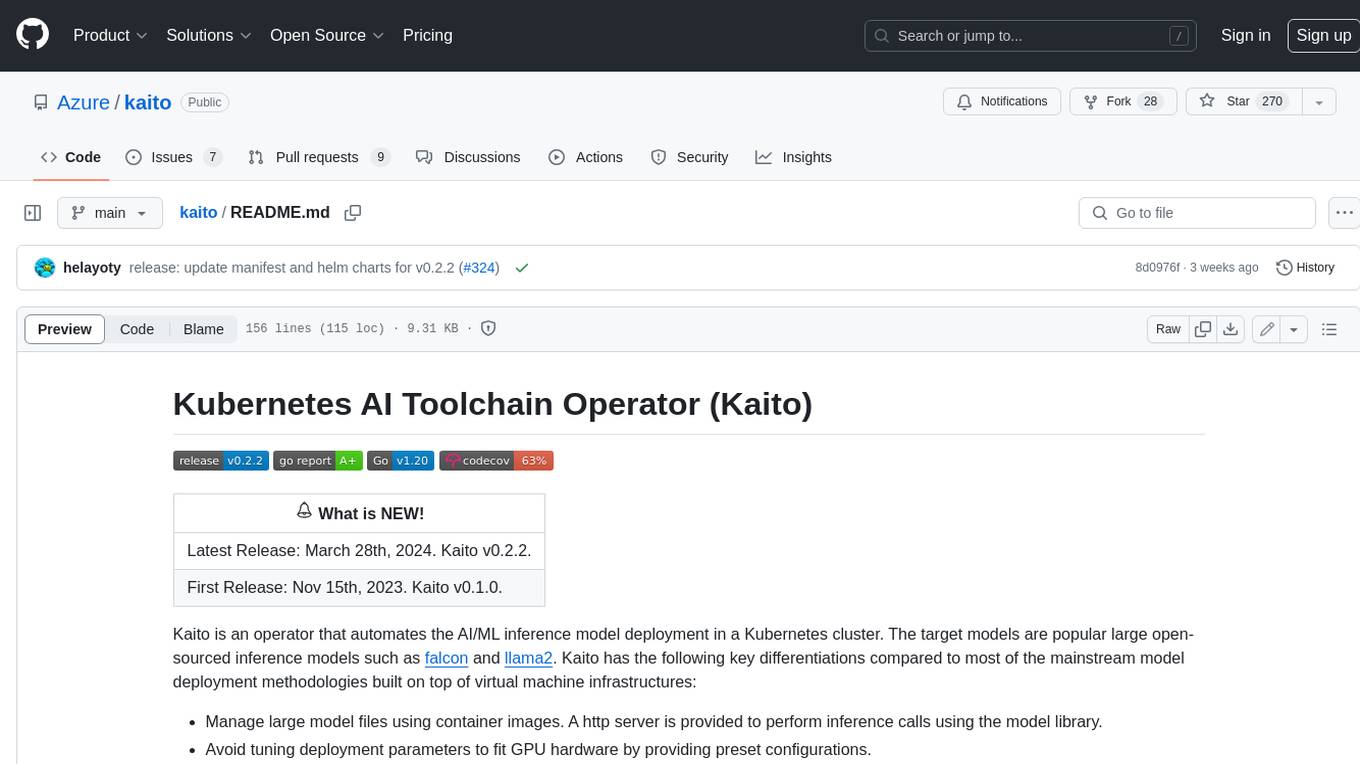
kaito
Kaito is an operator that automates the AI/ML inference model deployment in a Kubernetes cluster. It manages large model files using container images, avoids tuning deployment parameters to fit GPU hardware by providing preset configurations, auto-provisions GPU nodes based on model requirements, and hosts large model images in the public Microsoft Container Registry (MCR) if the license allows. Using Kaito, the workflow of onboarding large AI inference models in Kubernetes is largely simplified.
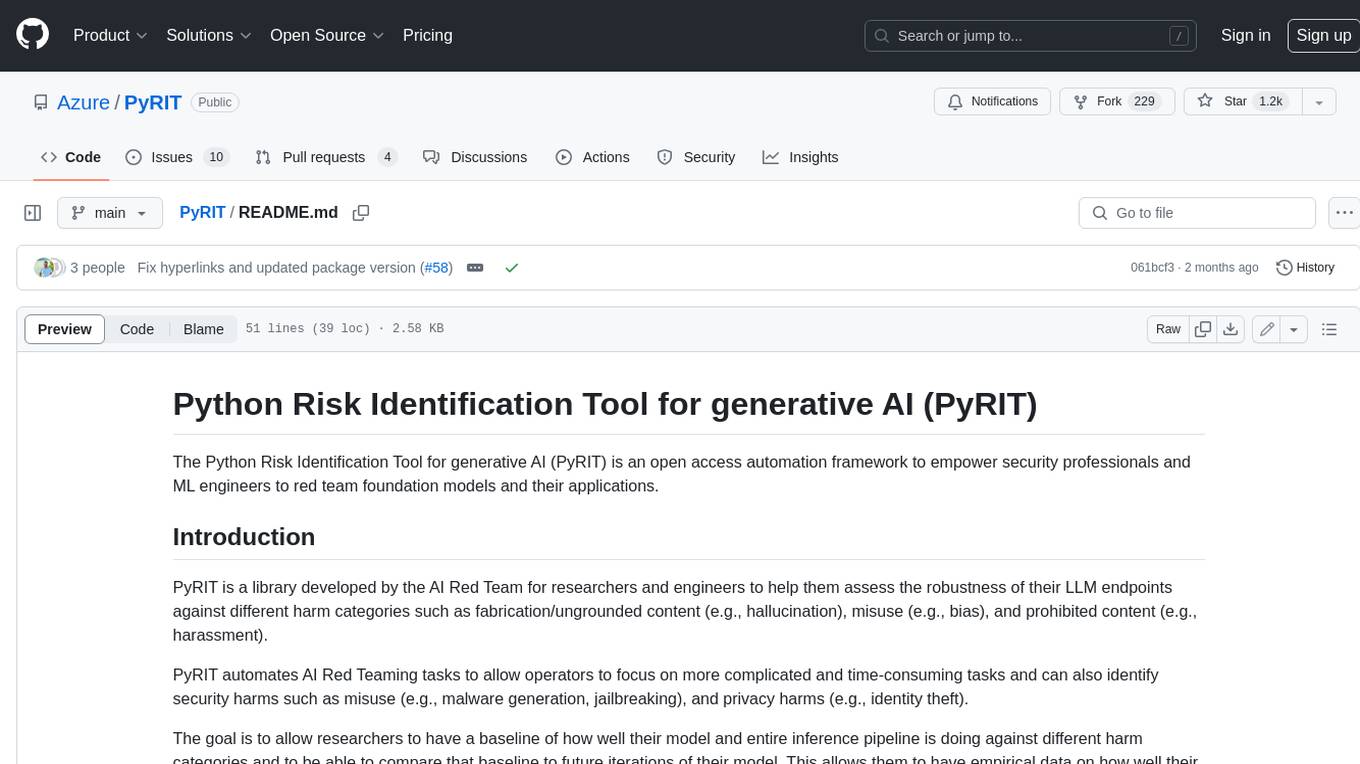
PyRIT
PyRIT is an open access automation framework designed to empower security professionals and ML engineers to red team foundation models and their applications. It automates AI Red Teaming tasks to allow operators to focus on more complicated and time-consuming tasks and can also identify security harms such as misuse (e.g., malware generation, jailbreaking), and privacy harms (e.g., identity theft). The goal is to allow researchers to have a baseline of how well their model and entire inference pipeline is doing against different harm categories and to be able to compare that baseline to future iterations of their model. This allows them to have empirical data on how well their model is doing today, and detect any degradation of performance based on future improvements.
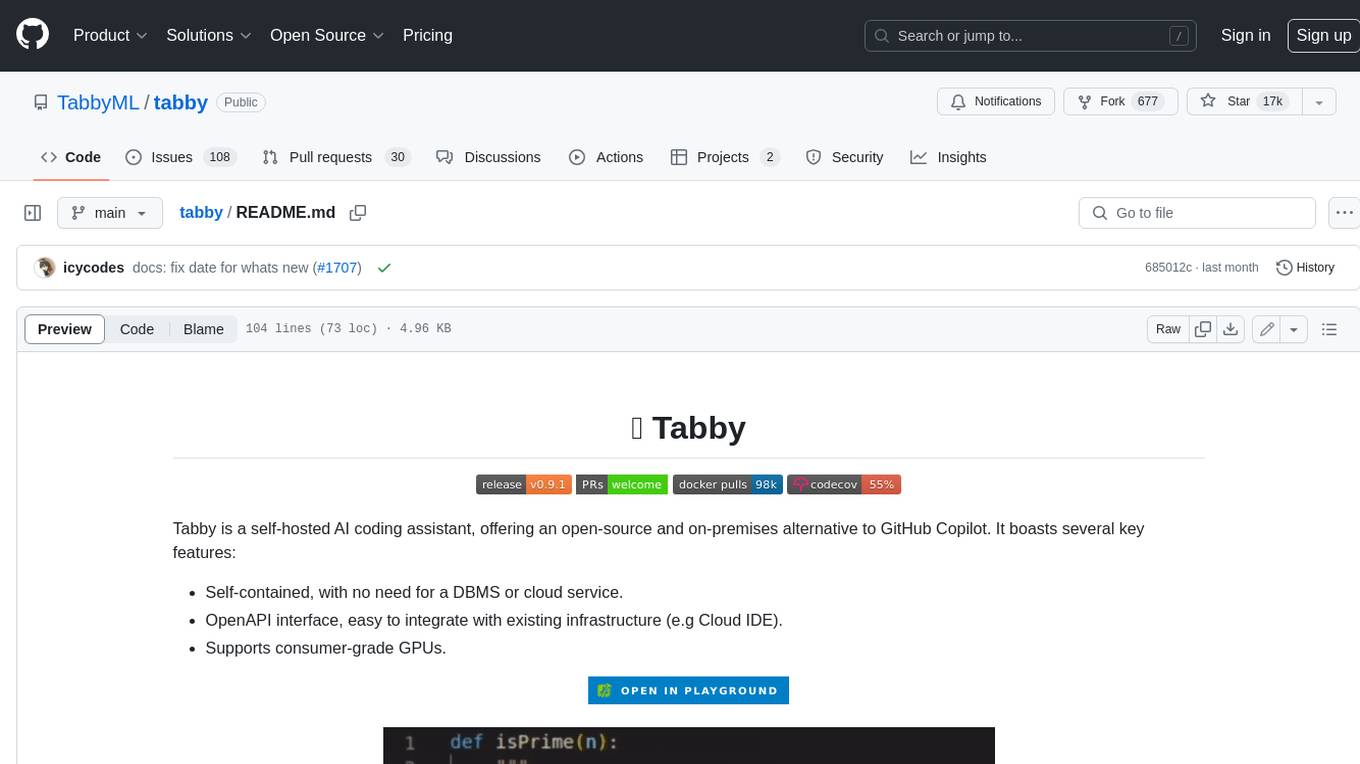
tabby
Tabby is a self-hosted AI coding assistant, offering an open-source and on-premises alternative to GitHub Copilot. It boasts several key features: * Self-contained, with no need for a DBMS or cloud service. * OpenAPI interface, easy to integrate with existing infrastructure (e.g Cloud IDE). * Supports consumer-grade GPUs.
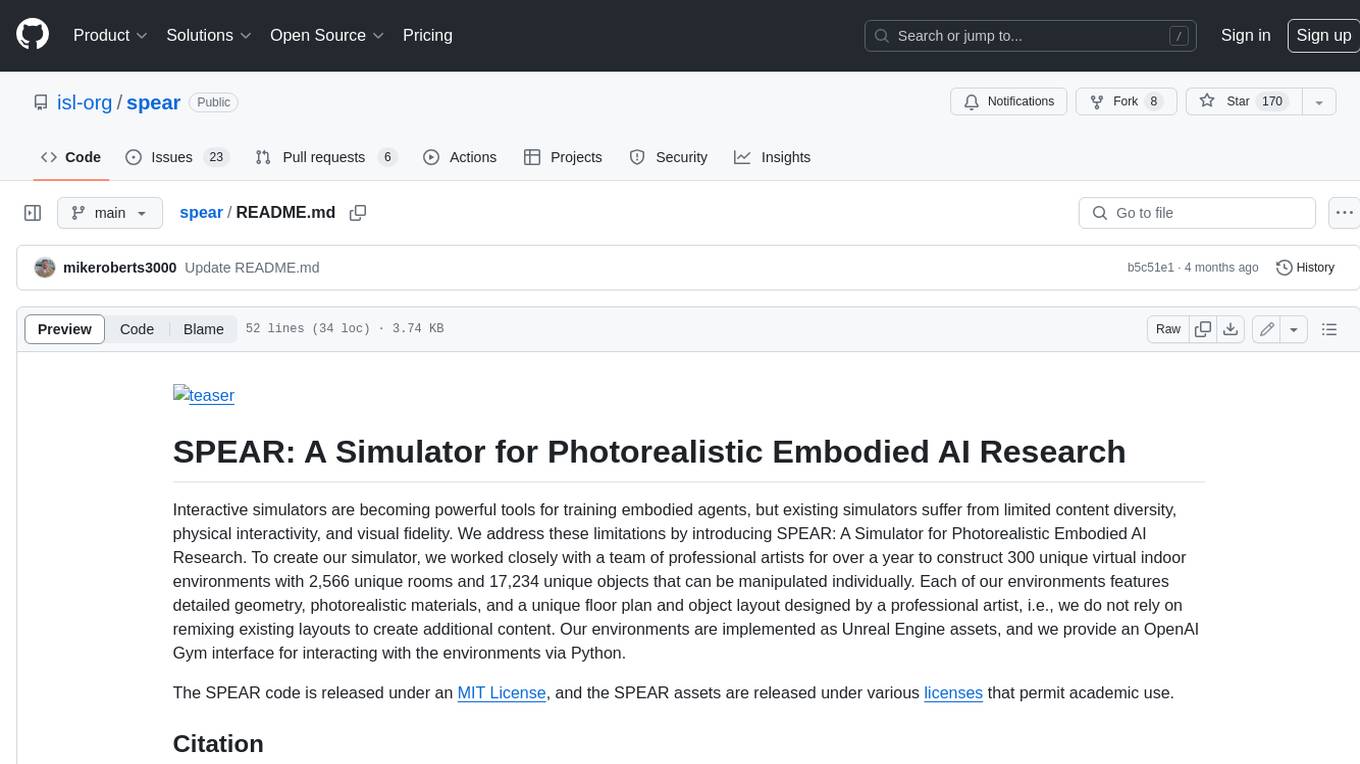
spear
SPEAR (Simulator for Photorealistic Embodied AI Research) is a powerful tool for training embodied agents. It features 300 unique virtual indoor environments with 2,566 unique rooms and 17,234 unique objects that can be manipulated individually. Each environment is designed by a professional artist and features detailed geometry, photorealistic materials, and a unique floor plan and object layout. SPEAR is implemented as Unreal Engine assets and provides an OpenAI Gym interface for interacting with the environments via Python.
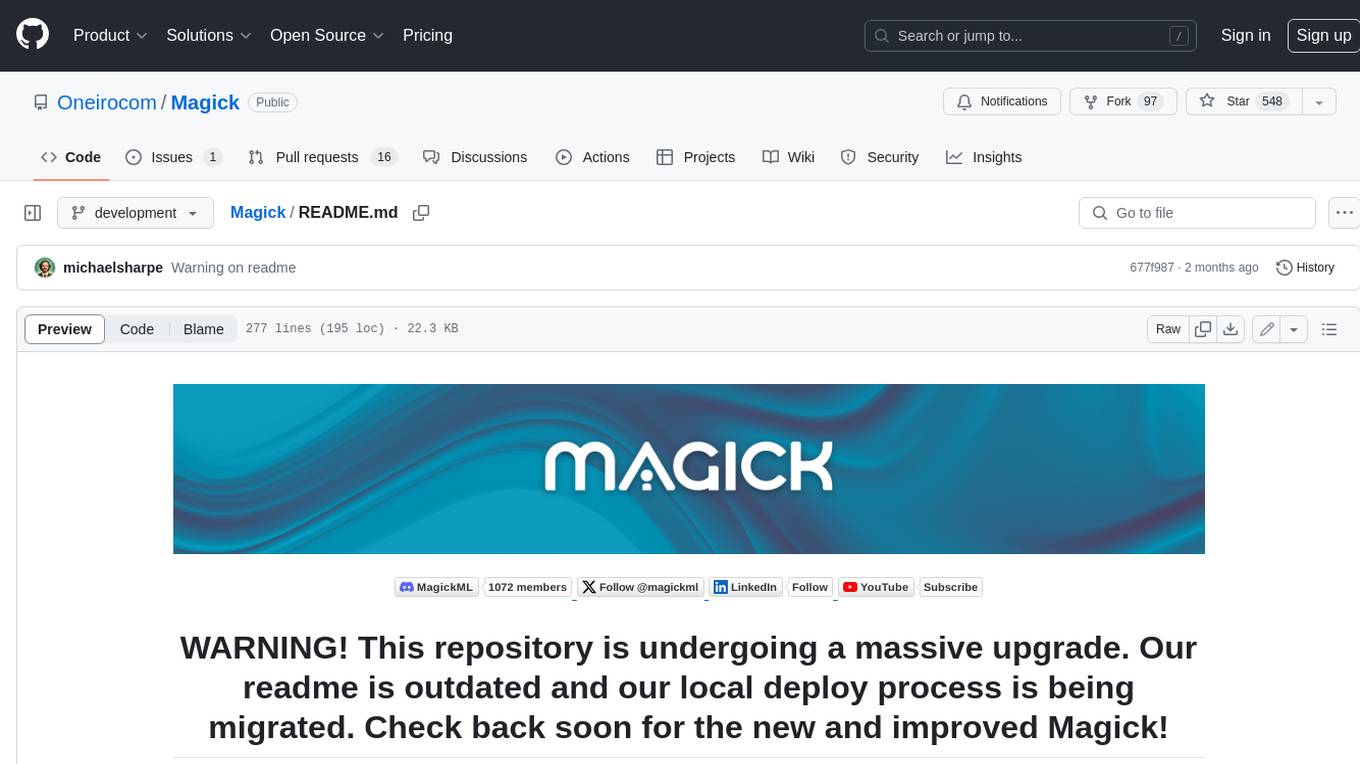
Magick
Magick is a groundbreaking visual AIDE (Artificial Intelligence Development Environment) for no-code data pipelines and multimodal agents. Magick can connect to other services and comes with nodes and templates well-suited for intelligent agents, chatbots, complex reasoning systems and realistic characters.