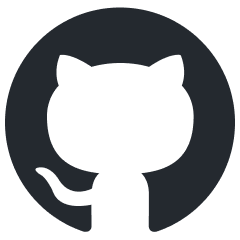
perplexity-mcp
A Model Context Protocol (MCP) server for research and documentation assistance using Perplexity AI. Won 1st @ Cline Hackathon
Stars: 150
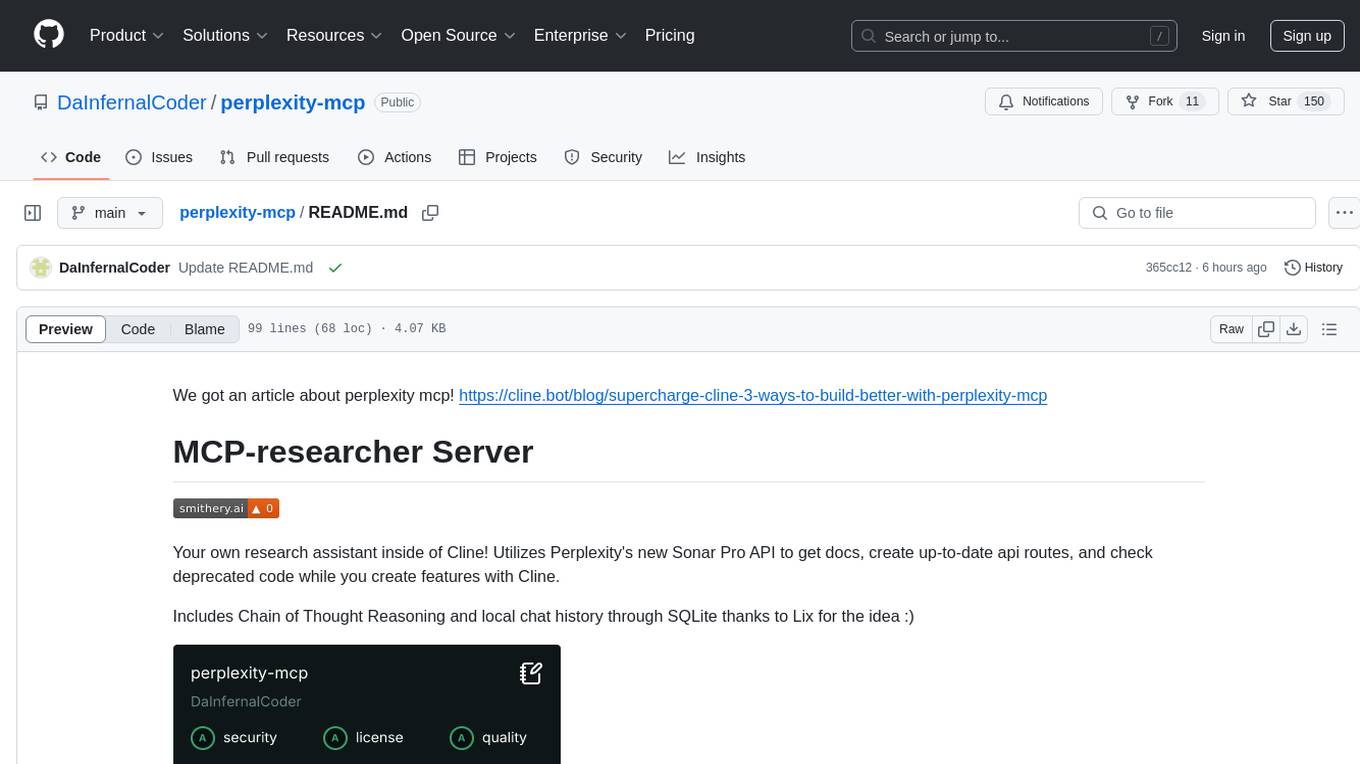
README:
We got an article about perplexity mcp! https://cline.bot/blog/supercharge-cline-3-ways-to-build-better-with-perplexity-mcp
Your own research assistant inside of Cline! Utilizes Perplexity's new Sonar Pro API to get docs, create up-to-date api routes, and check deprecated code while you create features with Cline.
Includes Chain of Thought Reasoning and local chat history through SQLite thanks to Lix for the idea :)
1. Search
Performs general search queries to get comprehensive information on any topic. The example shows how to use different detail levels (brief, normal, detailed) to get tailored responses.
Retrieves documentation and usage examples for specific technologies, libraries, or APIs. The example demonstrates getting comprehensive documentation for React hooks, including best practices and common pitfalls.
3. Find APIs
Discovers and evaluates APIs that could be integrated into a project. The example shows finding payment processing APIs with detailed analysis of features, pricing, and integration complexity.
Analyzes code for deprecated features or patterns, providing migration guidance. The example demonstrates checking React class components and lifecycle methods for modern alternatives.
To install MCP-researcher Server for Claude Desktop automatically via Smithery:
npx -y @smithery/cli install @DaInfernalCoder/perplexity-mcp --client claude
-
First install Node.js if not already installed (from nodejs.org)
-
Clone the repo
-
git clone https://github.com/DaInfernalCoder/researcher-mcp perplexity-server
-
cd perplexity-server
-
Install dependencies and build: npm install
-
Get a Perplexity API key from https://www.perplexity.ai/settings/api
-
Create the MCP settings file in the appropriate location for your OS: macOS: ~/Library/Application Support/Cursor/User/globalStorage/saoudrizwan.claude-dev/settings/cline_mcp_settings.json Windows: %APPDATA%\Cursor\User\globalStorage\saoudrizwan.claude-dev\settings\cline_mcp_settings.json Linux: ~/.config/Cursor/User/globalStorage/saoudrizwan.claude-dev/settings/cline_mcp_settings.json
-
To use with Claude Desktop, add the server config:
On MacOS: ~/Library/Application Support/Claude/claude_desktop_config.json
On Windows: %APPDATA%/Claude/claude_desktop_config.json
- To use with Cline, add into mcpServers:
{
"mcpServers": {
"perplexity-server": {
"command": "node",
"args": [
"[path/to/researcher-mcp/index.js]"
],
"env": {
"PERPLEXITY_API_KEY": ""
},
"disabled": false,
"autoApprove": [
"search",
"get_documentation",
"find_apis",
"check_deprecated_code"
]
}
}
}
- Build the server: npm run build
Make sure to:
- Replace /absolute/path/to with the actual path where you cloned the repository
- Replace your-api-key-here with your Perplexity API key
- Keep the same autoApprove settings for consistent behavior
For Tasks:
Click tags to check more tools for each tasksFor Jobs:
Alternative AI tools for perplexity-mcp
Similar Open Source Tools
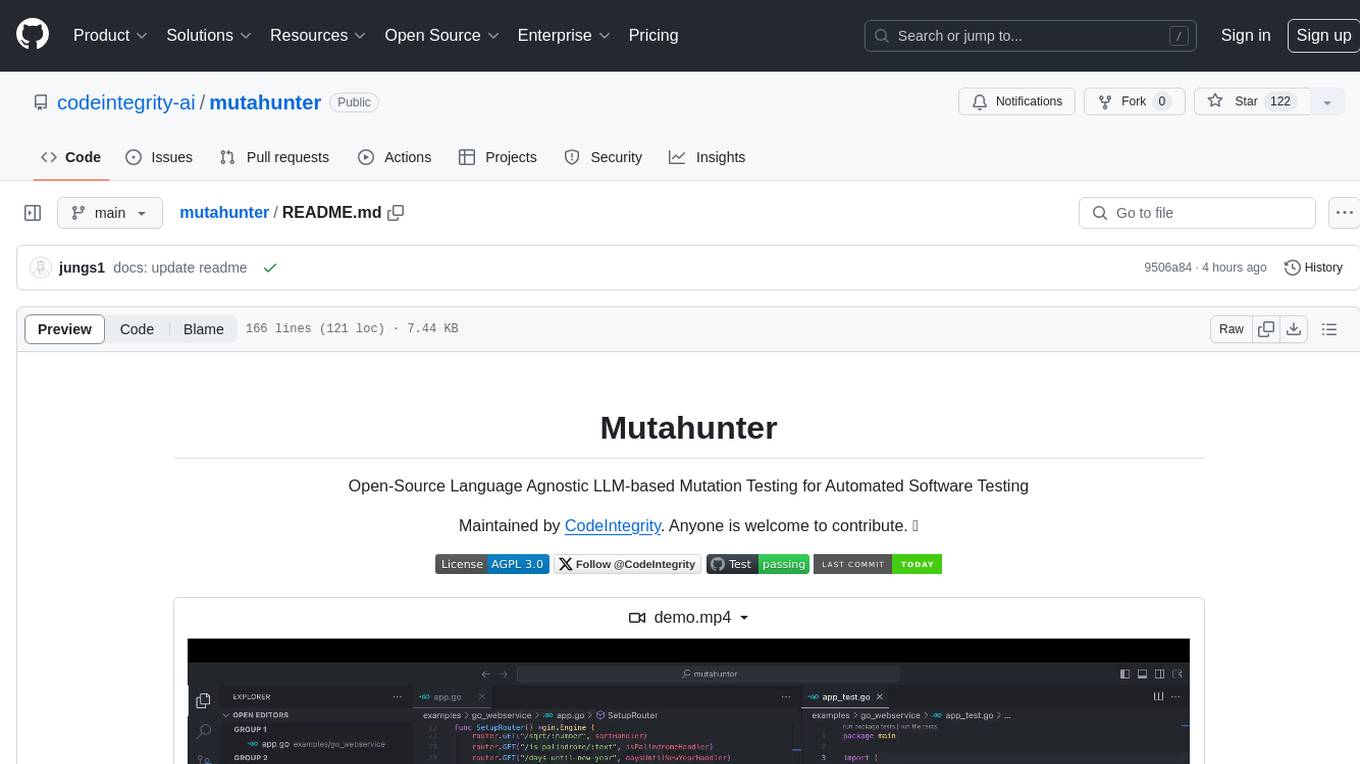
mutahunter
Mutahunter is an open-source language-agnostic mutation testing tool maintained by CodeIntegrity. It leverages LLM models to inject context-aware faults into codebase, ensuring comprehensive testing. The tool aims to empower companies and developers to enhance test suites and improve software quality by verifying the effectiveness of test cases through creating mutants in the code and checking if the test cases can catch these changes. Mutahunter provides detailed reports on mutation coverage, killed mutants, and survived mutants, enabling users to identify potential weaknesses in their test suites.
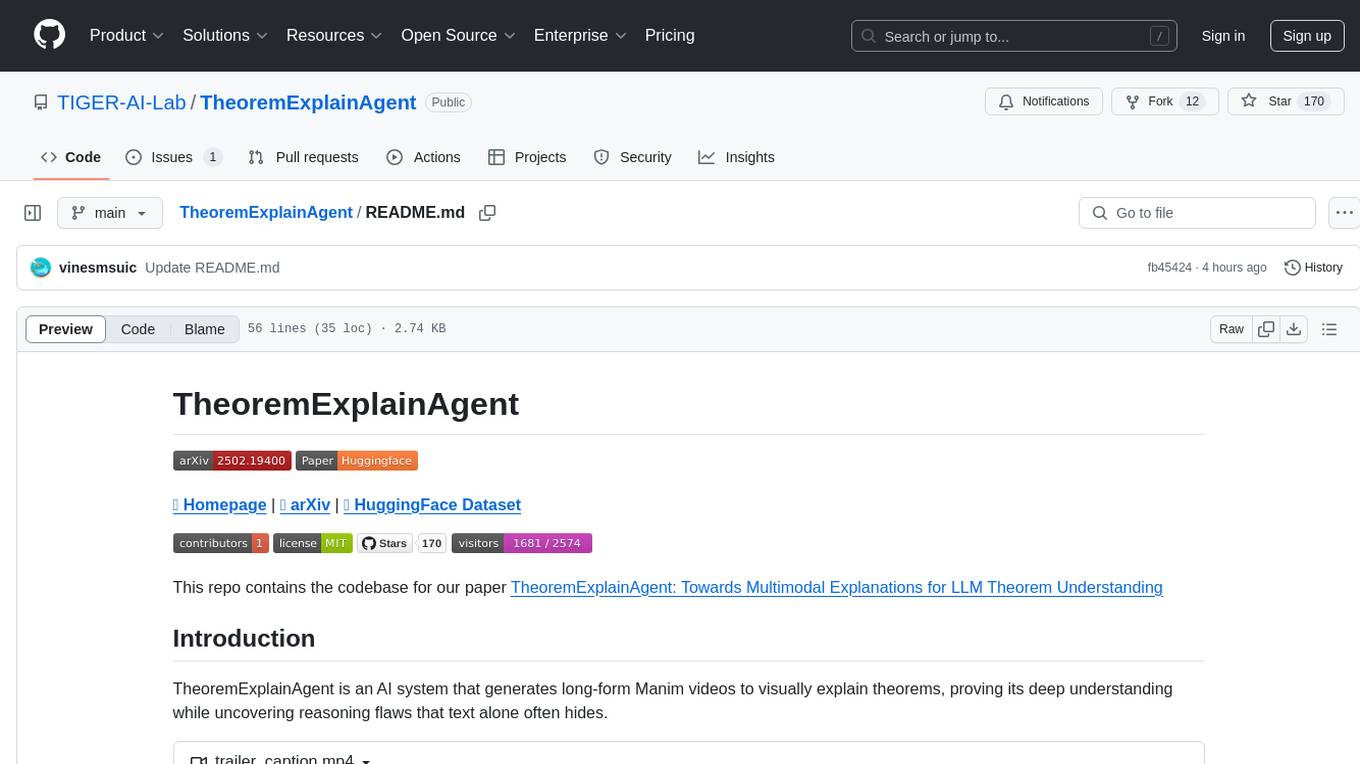
TheoremExplainAgent
TheoremExplainAgent is an AI system that generates long-form Manim videos to visually explain theorems, proving its deep understanding while uncovering reasoning flaws that text alone often hides. The codebase for the paper 'TheoremExplainAgent: Towards Multimodal Explanations for LLM Theorem Understanding' is available in this repository. It provides a tool for creating multimodal explanations for theorem understanding using AI technology.
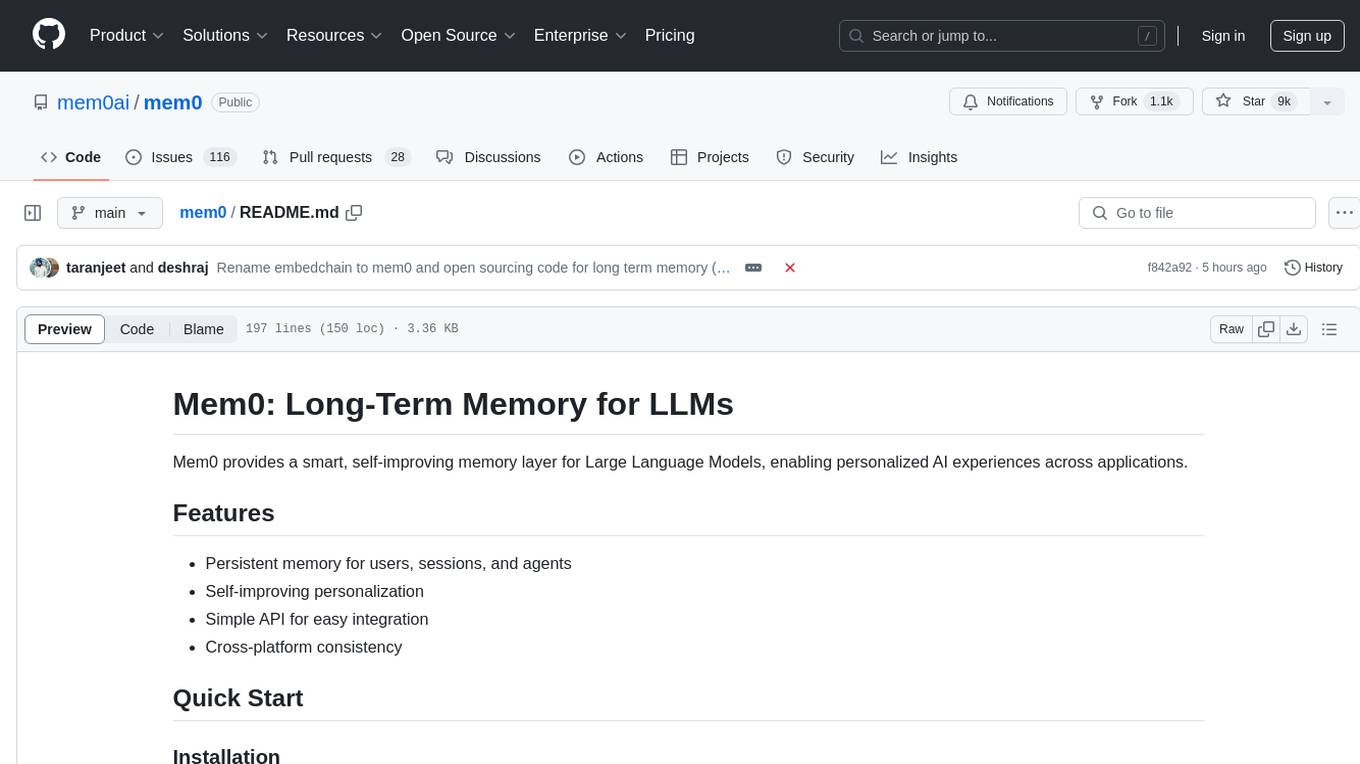
mem0
Mem0 is a tool that provides a smart, self-improving memory layer for Large Language Models, enabling personalized AI experiences across applications. It offers persistent memory for users, sessions, and agents, self-improving personalization, a simple API for easy integration, and cross-platform consistency. Users can store memories, retrieve memories, search for related memories, update memories, get the history of a memory, and delete memories using Mem0. It is designed to enhance AI experiences by enabling long-term memory storage and retrieval.
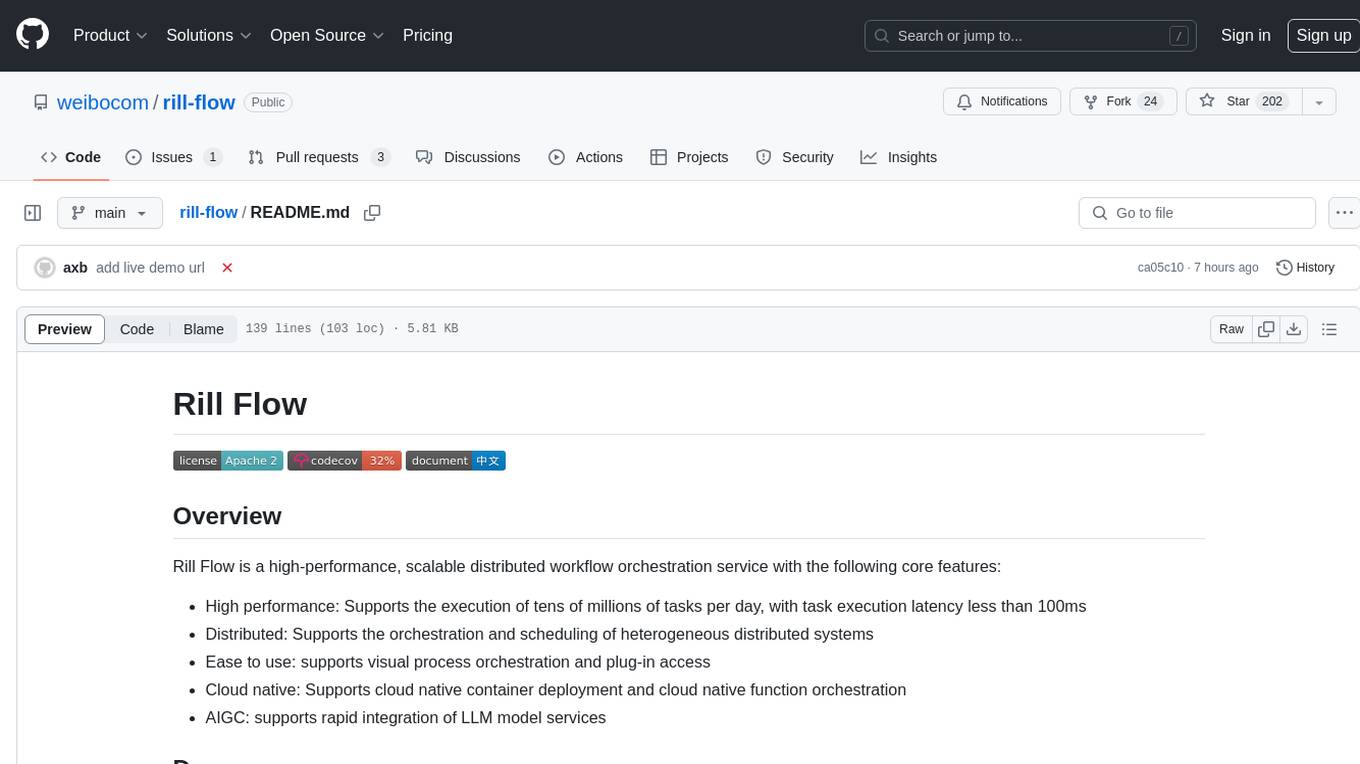
rill-flow
Rill Flow is a high-performance, scalable distributed workflow orchestration service that supports the execution of tens of millions of tasks per day with task execution latency less than 100ms. It is distributed and supports the orchestration and scheduling of heterogeneous distributed systems. Rill Flow is easy to use, supporting visual process orchestration and plug-in access. It is cloud native, allowing for cloud native container deployment and cloud native function orchestration. Additionally, Rill Flow supports rapid integration of LLM model services.
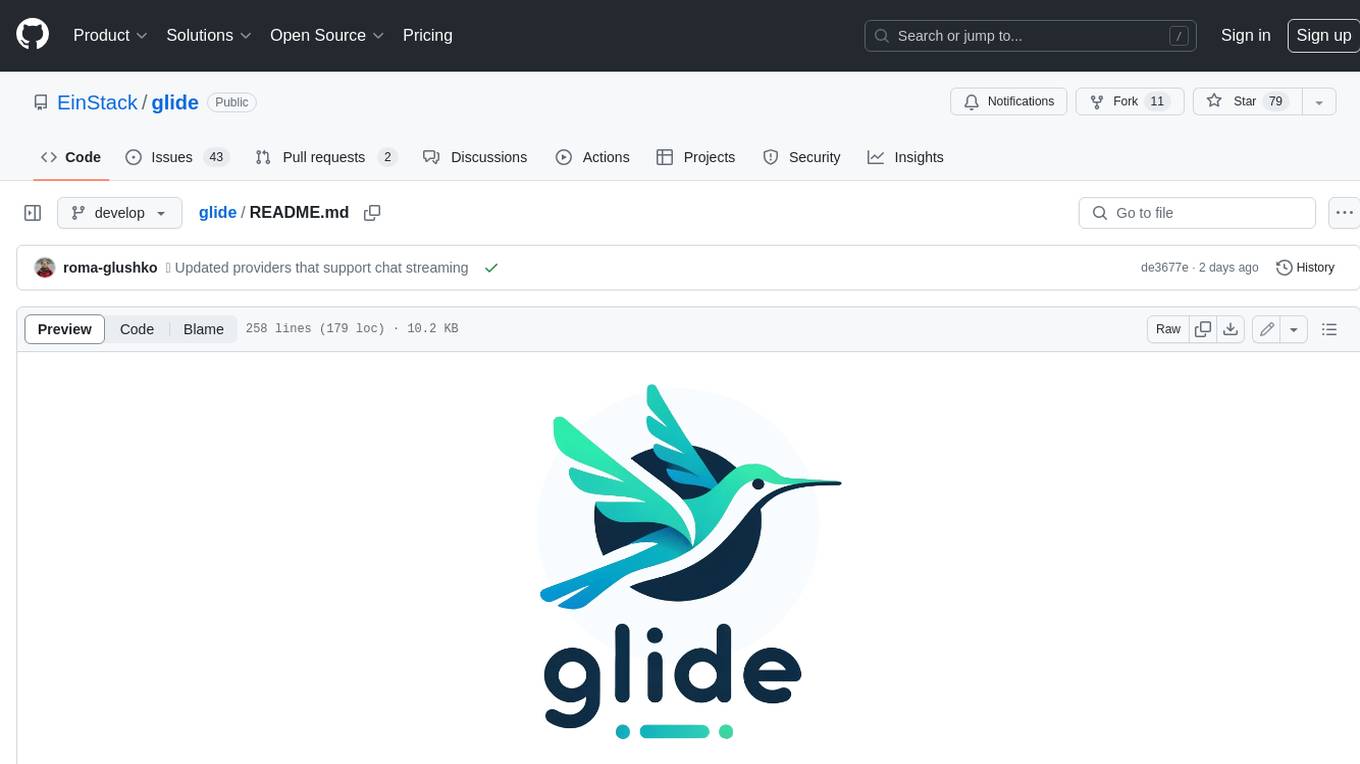
glide
Glide is a cloud-native LLM gateway that provides a unified REST API for accessing various large language models (LLMs) from different providers. It handles LLMOps tasks such as model failover, caching, key management, and more, making it easy to integrate LLMs into applications. Glide supports popular LLM providers like OpenAI, Anthropic, Azure OpenAI, AWS Bedrock (Titan), Cohere, Google Gemini, OctoML, and Ollama. It offers high availability, performance, and observability, and provides SDKs for Python and NodeJS to simplify integration.
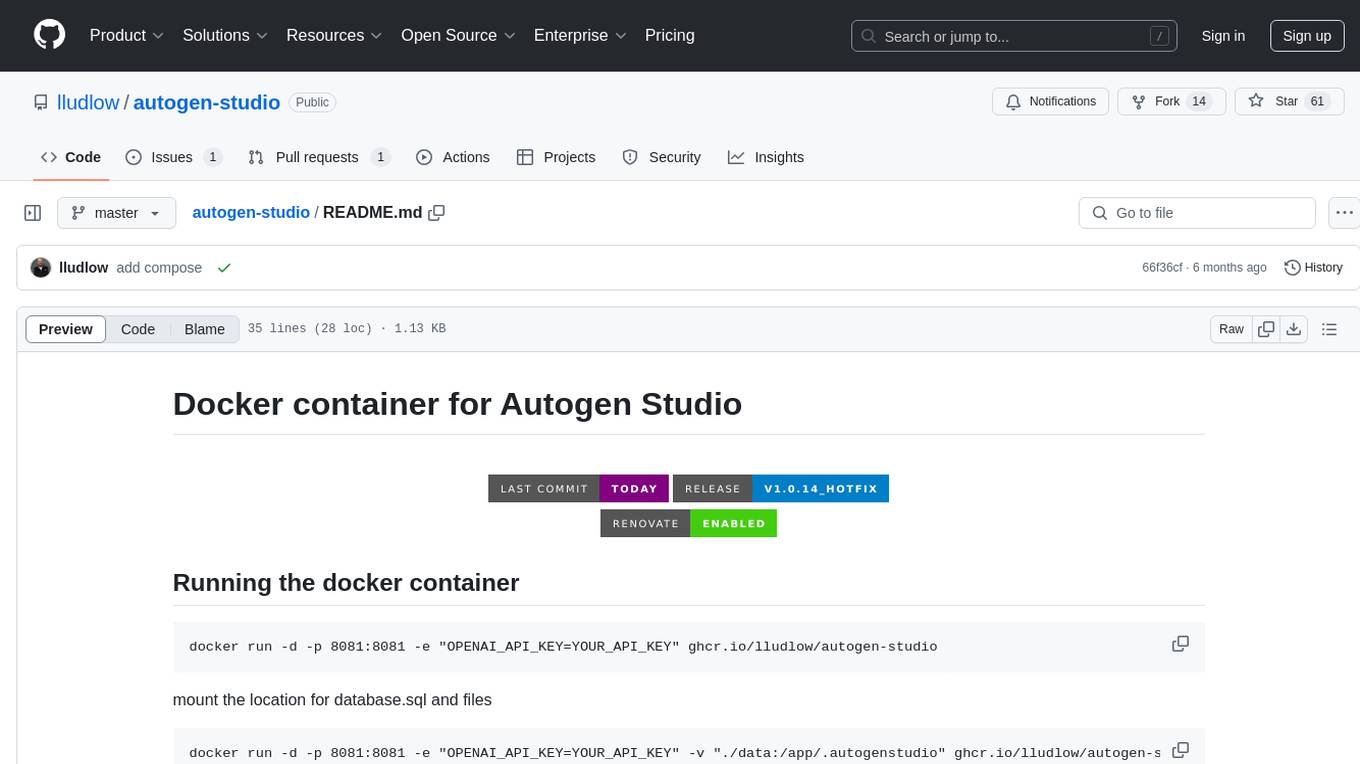
autogen-studio
Autogen Studio is a Docker container that provides a platform for running automated tasks using OpenAI API. It allows users to easily set up and manage a workspace for generating content and performing various tasks. The container can be run standalone or using Docker Compose, with options to mount data volumes and configure environment variables. Autogen Studio simplifies the process of leveraging AI capabilities for automating tasks and content generation.
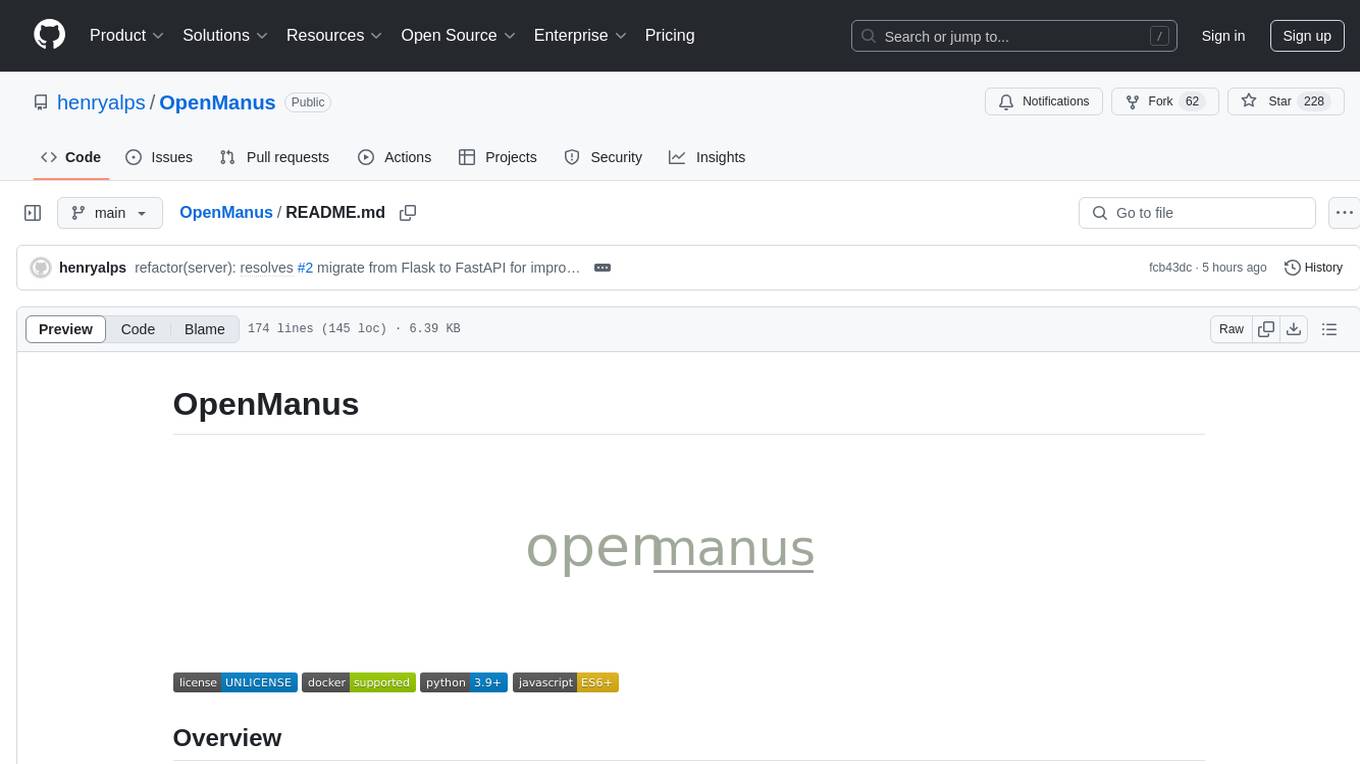
OpenManus
OpenManus is an open-source project aiming to replicate the capabilities of the Manus AI agent, known for autonomously executing complex tasks like travel planning and stock analysis. The project provides a modular, containerized framework using Docker, Python, and JavaScript, allowing developers to build, deploy, and experiment with a multi-agent AI system. Features include collaborative AI agents, Dockerized environment, task execution support, tool integration, modular design, and community-driven development. Users can interact with OpenManus via CLI, API, or web UI, and the project welcomes contributions to enhance its capabilities.
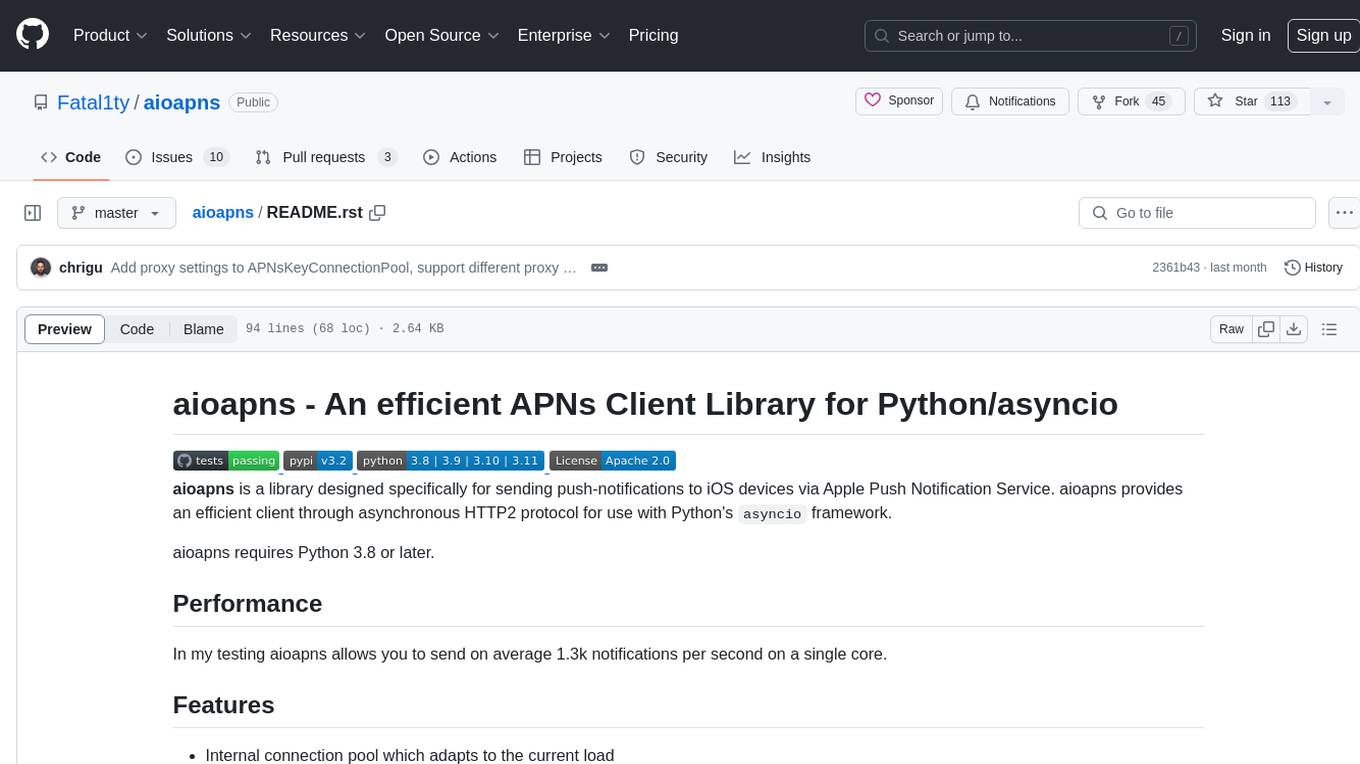
aioapns
aioapns is a Python library designed for sending push-notifications to iOS devices via Apple Push Notification Service. It provides an efficient client through asynchronous HTTP2 protocol for use with Python's asyncio framework. With features like internal connection pool, support for different types of connections, setting TTL and priority for notifications, and more, aioapns is a versatile tool for developers looking to send push notifications to iOS devices.
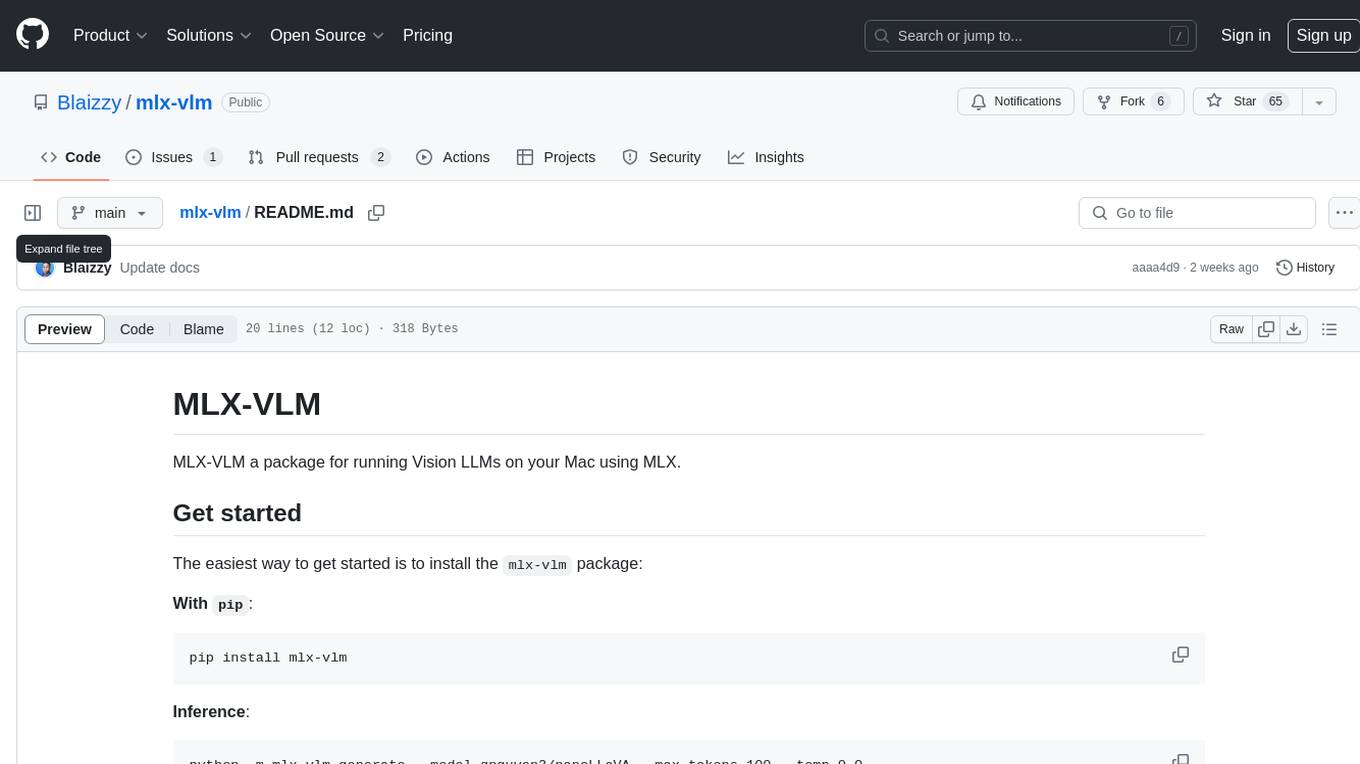
mlx-vlm
MLX-VLM is a package designed for running Vision LLMs on Mac systems using MLX. It provides a convenient way to install and utilize the package for processing large language models related to vision tasks. The tool simplifies the process of running LLMs on Mac computers, offering a seamless experience for users interested in leveraging MLX for vision-related projects.
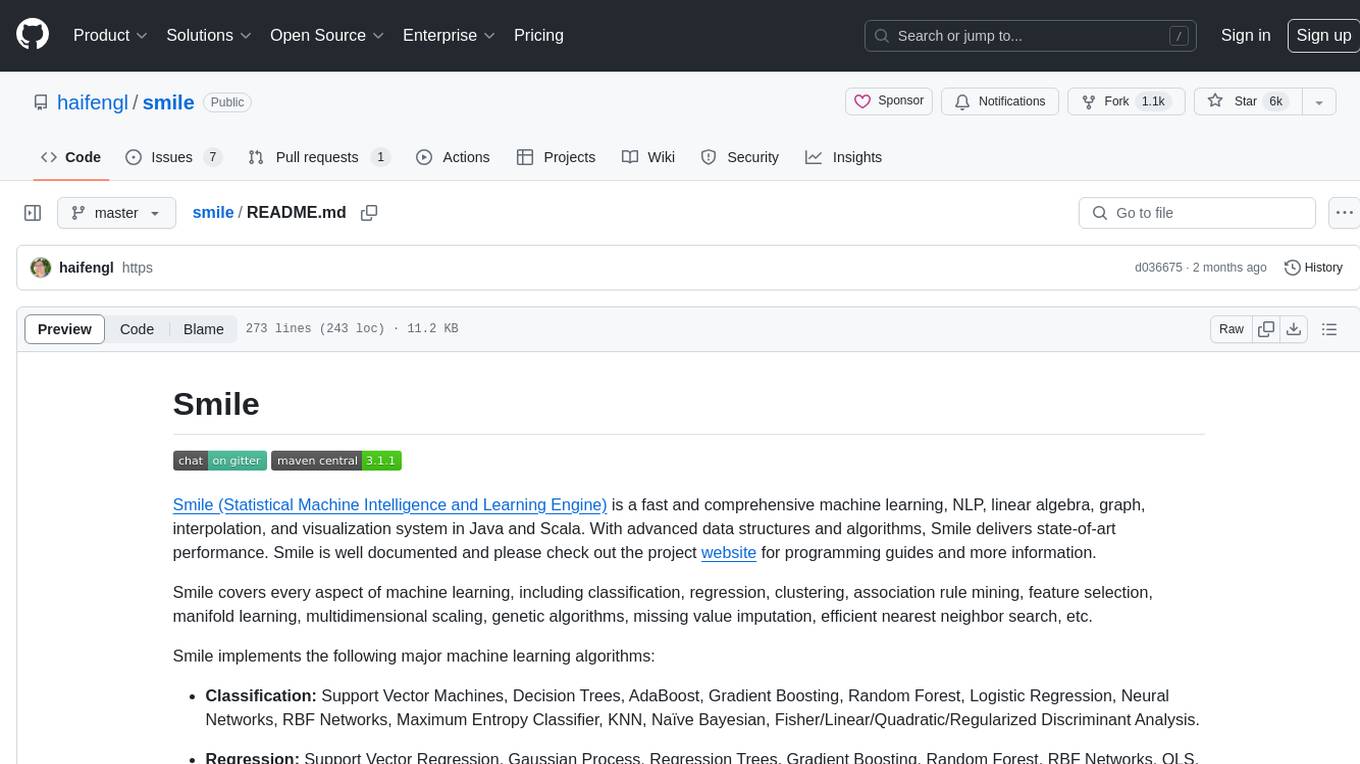
smile
Smile (Statistical Machine Intelligence and Learning Engine) is a comprehensive machine learning, NLP, linear algebra, graph, interpolation, and visualization system in Java and Scala. It covers every aspect of machine learning, including classification, regression, clustering, association rule mining, feature selection, manifold learning, multidimensional scaling, genetic algorithms, missing value imputation, efficient nearest neighbor search, etc. Smile implements major machine learning algorithms and provides interactive shells for Java, Scala, and Kotlin. It supports model serialization, data visualization using SmilePlot and declarative approach, and offers a gallery showcasing various algorithms and visualizations.
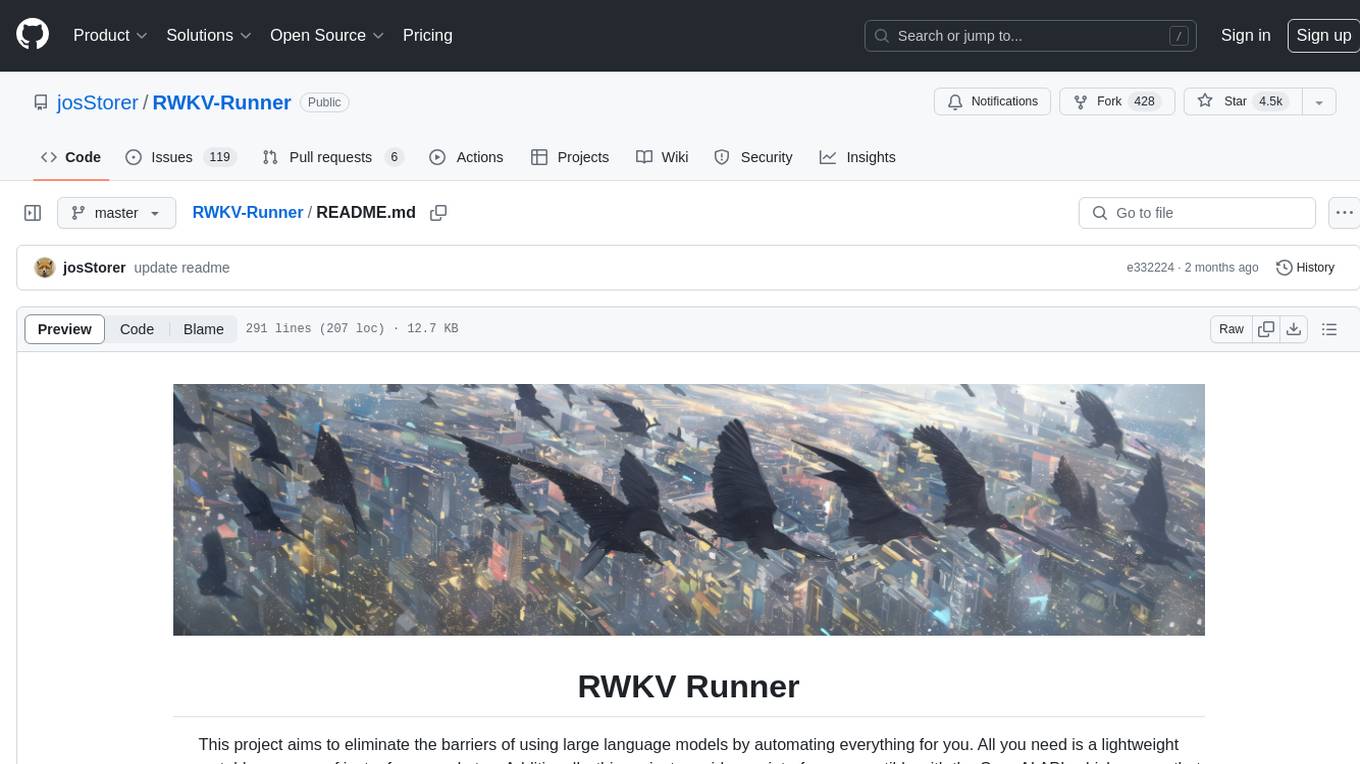
RWKV-Runner
RWKV Runner is a project designed to simplify the usage of large language models by automating various processes. It provides a lightweight executable program and is compatible with the OpenAI API. Users can deploy the backend on a server and use the program as a client. The project offers features like model management, VRAM configurations, user-friendly chat interface, WebUI option, parameter configuration, model conversion tool, download management, LoRA Finetune, and multilingual localization. It can be used for various tasks such as chat, completion, composition, and model inspection.
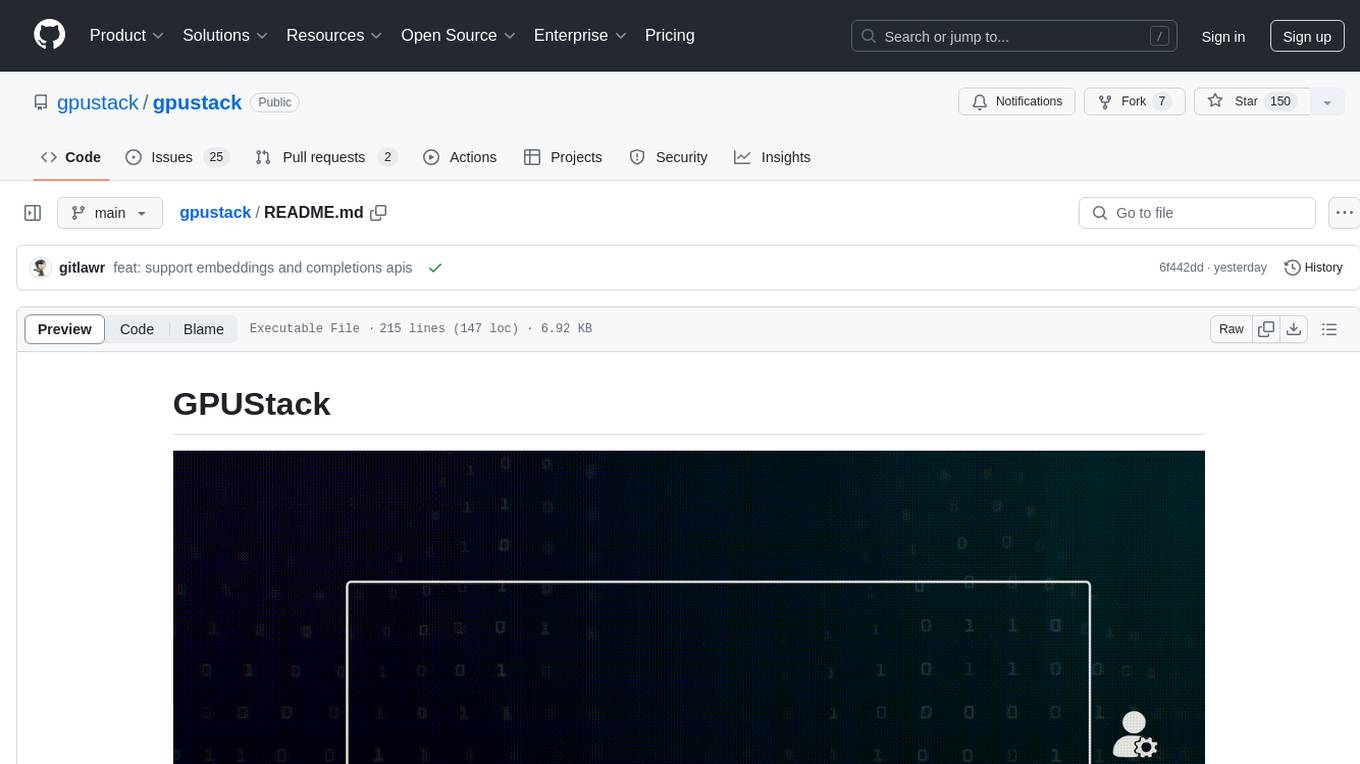
gpustack
GPUStack is an open-source GPU cluster manager designed for running large language models (LLMs). It supports a wide variety of hardware, scales with GPU inventory, offers lightweight Python package with minimal dependencies, provides OpenAI-compatible APIs, simplifies user and API key management, enables GPU metrics monitoring, and facilitates token usage and rate metrics tracking. The tool is suitable for managing GPU clusters efficiently and effectively.
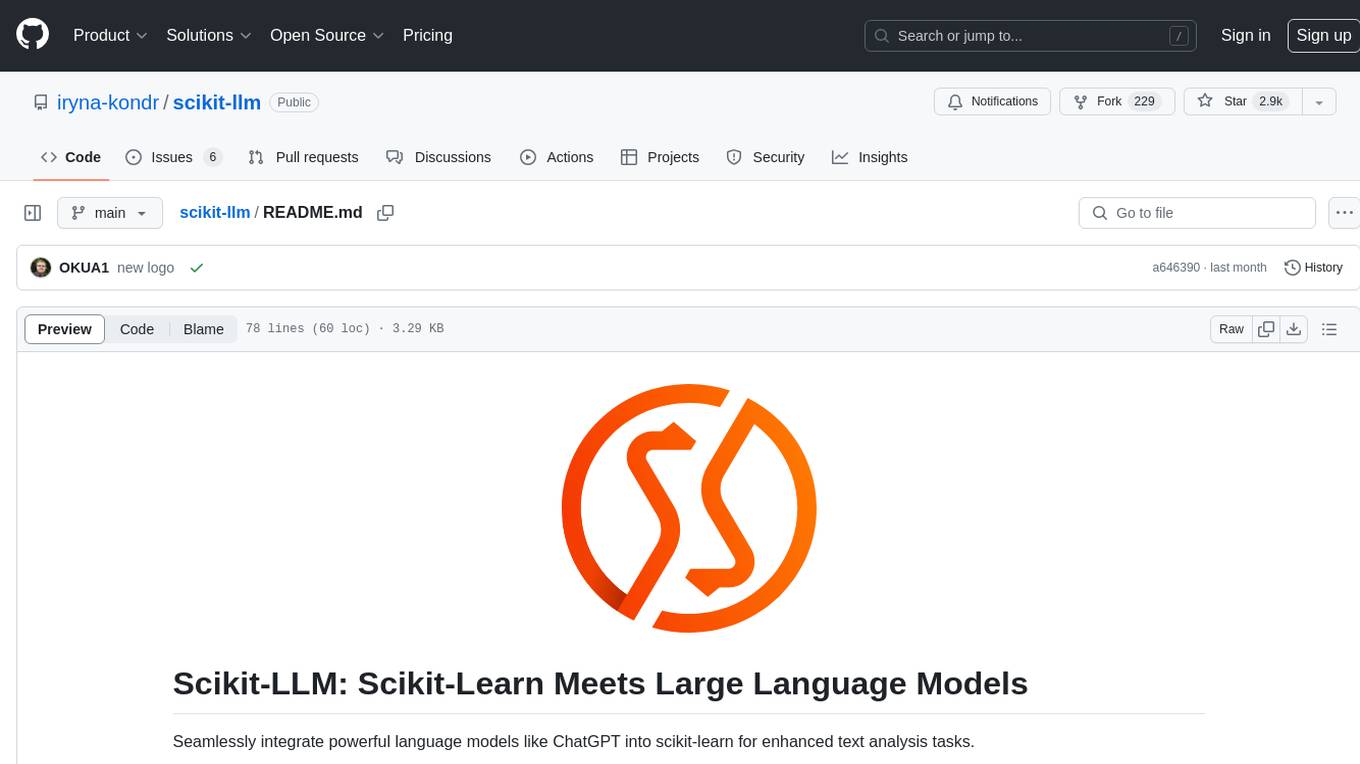
scikit-llm
Scikit-LLM is a tool that seamlessly integrates powerful language models like ChatGPT into scikit-learn for enhanced text analysis tasks. It allows users to leverage large language models for various text analysis applications within the familiar scikit-learn framework. The tool simplifies the process of incorporating advanced language processing capabilities into machine learning pipelines, enabling users to benefit from the latest advancements in natural language processing.
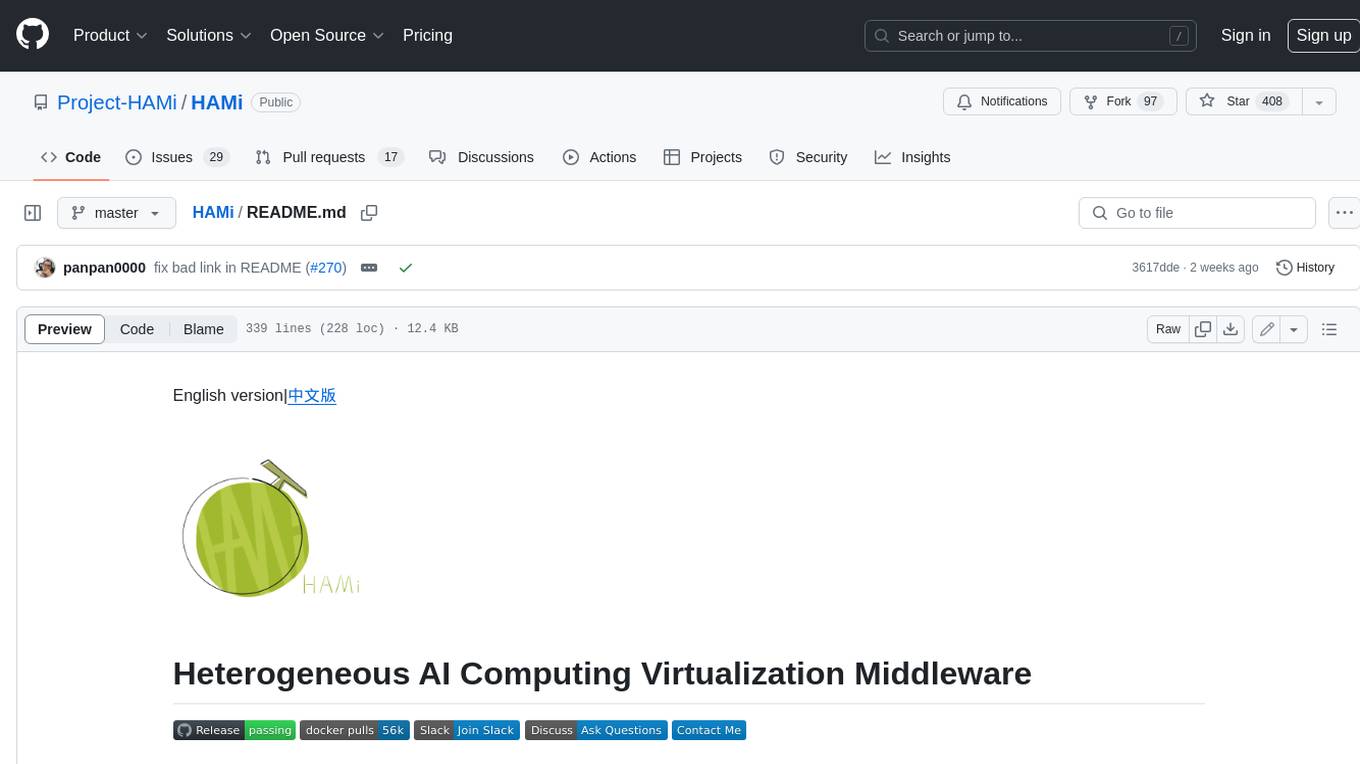
HAMi
HAMi is a Heterogeneous AI Computing Virtualization Middleware designed to manage Heterogeneous AI Computing Devices in a Kubernetes cluster. It allows for device sharing, device memory control, device type specification, and device UUID specification. The tool is easy to use and does not require modifying task YAML files. It includes features like hard limits on device memory, partial device allocation, streaming multiprocessor limits, and core usage specification. HAMi consists of components like a mutating webhook, scheduler extender, device plugins, and in-container virtualization techniques. It is suitable for scenarios requiring device sharing, specific device memory allocation, GPU balancing, low utilization optimization, and scenarios needing multiple small GPUs. The tool requires prerequisites like NVIDIA drivers, CUDA version, nvidia-docker, Kubernetes version, glibc version, and helm. Users can install, upgrade, and uninstall HAMi, submit tasks, and monitor cluster information. The tool's roadmap includes supporting additional AI computing devices, video codec processing, and Multi-Instance GPUs (MIG).
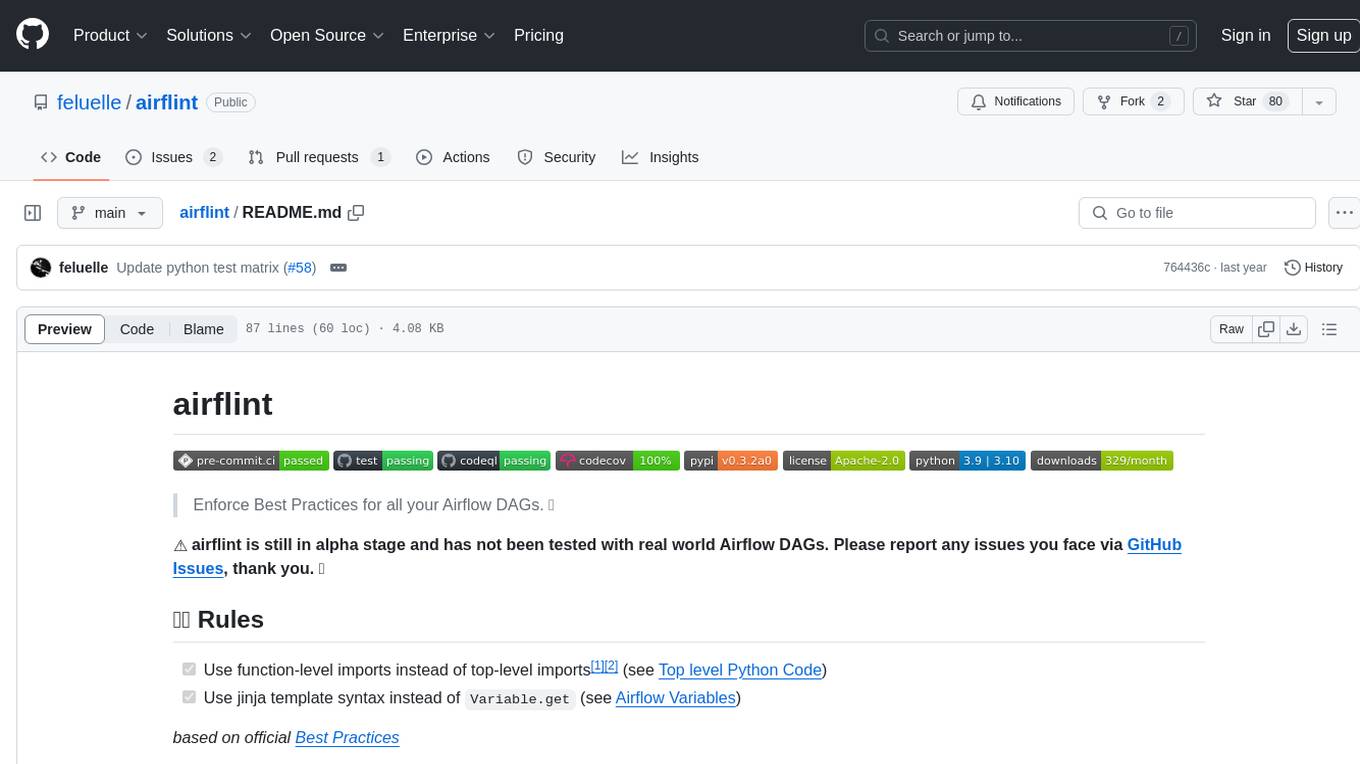
airflint
Airflint is a tool designed to enforce best practices for all your Airflow Directed Acyclic Graphs (DAGs). It is currently in the alpha stage and aims to help users adhere to recommended practices when working with Airflow. Users can install Airflint from PyPI and integrate it into their existing Airflow environment to improve DAG quality. The tool provides rules for function-level imports and jinja template syntax usage, among others, to enhance the development process of Airflow DAGs.