Best AI tools for< Tokenize Sequences >
8 - AI tool Sites
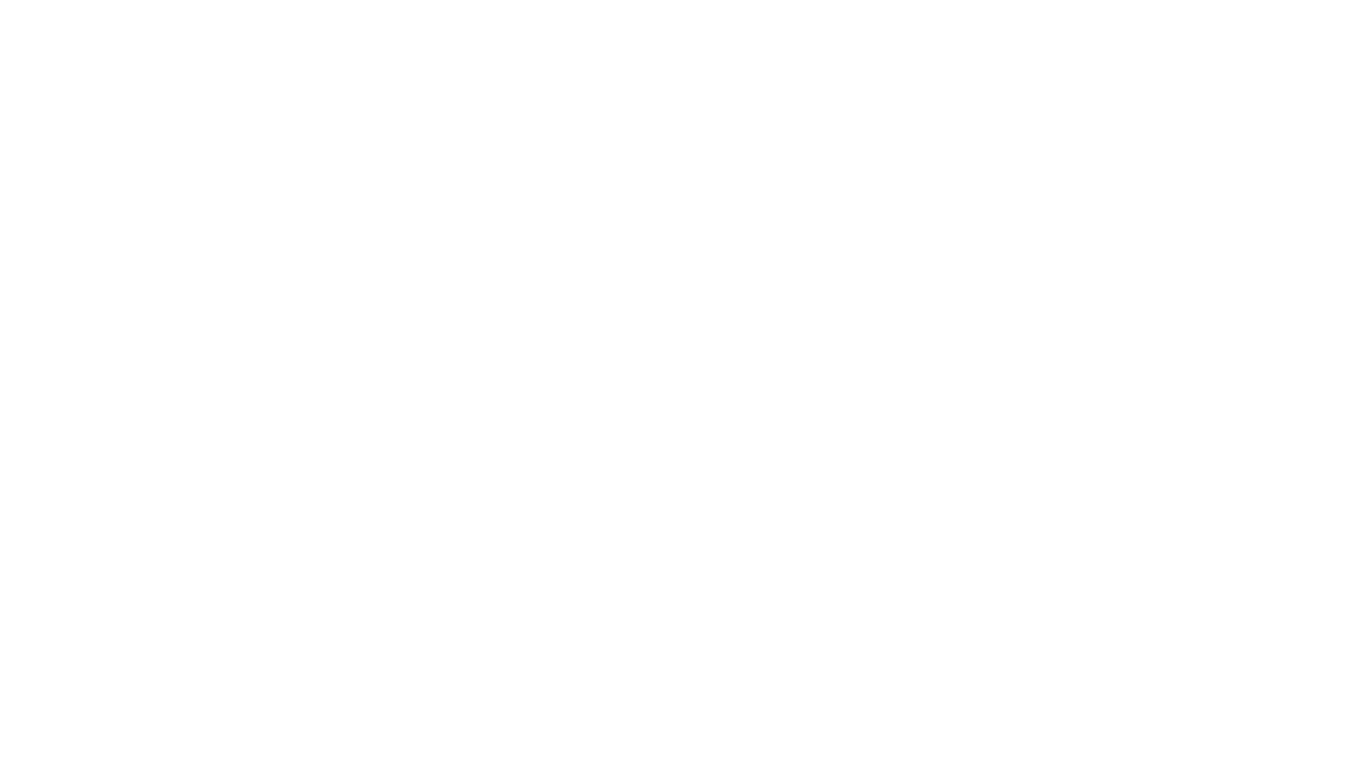
NLTK
NLTK (Natural Language Toolkit) is a leading platform for building Python programs to work with human language data. It provides easy-to-use interfaces to over 50 corpora and lexical resources such as WordNet, along with a suite of text processing libraries for classification, tokenization, stemming, tagging, parsing, and semantic reasoning, wrappers for industrial-strength NLP libraries, and an active discussion forum. Thanks to a hands-on guide introducing programming fundamentals alongside topics in computational linguistics, plus comprehensive API documentation, NLTK is suitable for linguists, engineers, students, educators, researchers, and industry users alike.
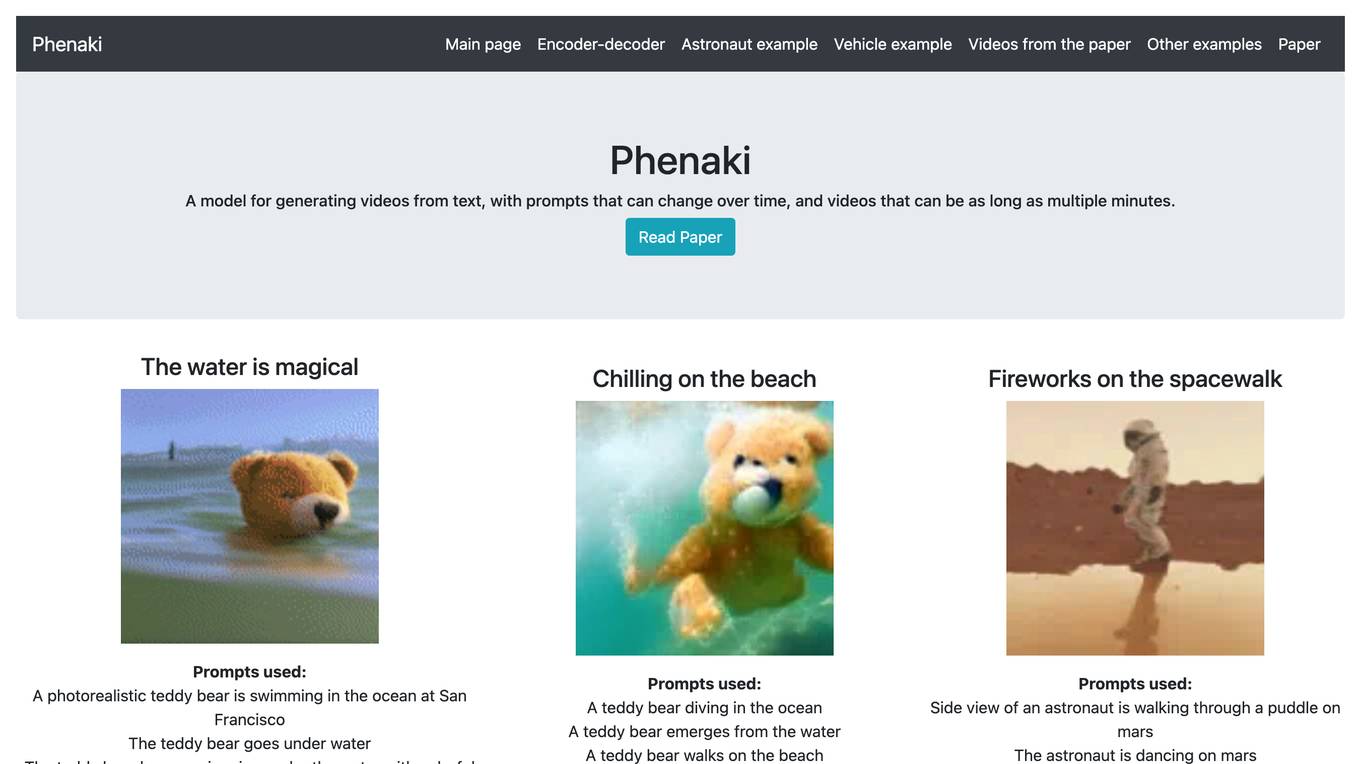
Phenaki
Phenaki is a model capable of generating realistic videos from a sequence of textual prompts. It is particularly challenging to generate videos from text due to the computational cost, limited quantities of high-quality text-video data, and variable length of videos. To address these issues, Phenaki introduces a new causal model for learning video representation, which compresses the video to a small representation of discrete tokens. This tokenizer uses causal attention in time, which allows it to work with variable-length videos. To generate video tokens from text, Phenaki uses a bidirectional masked transformer conditioned on pre-computed text tokens. The generated video tokens are subsequently de-tokenized to create the actual video. To address data issues, Phenaki demonstrates how joint training on a large corpus of image-text pairs as well as a smaller number of video-text examples can result in generalization beyond what is available in the video datasets. Compared to previous video generation methods, Phenaki can generate arbitrarily long videos conditioned on a sequence of prompts (i.e., time-variable text or a story) in an open domain. To the best of our knowledge, this is the first time a paper studies generating videos from time-variable prompts. In addition, the proposed video encoder-decoder outperforms all per-frame baselines currently used in the literature in terms of spatio-temporal quality and the number of tokens per video.
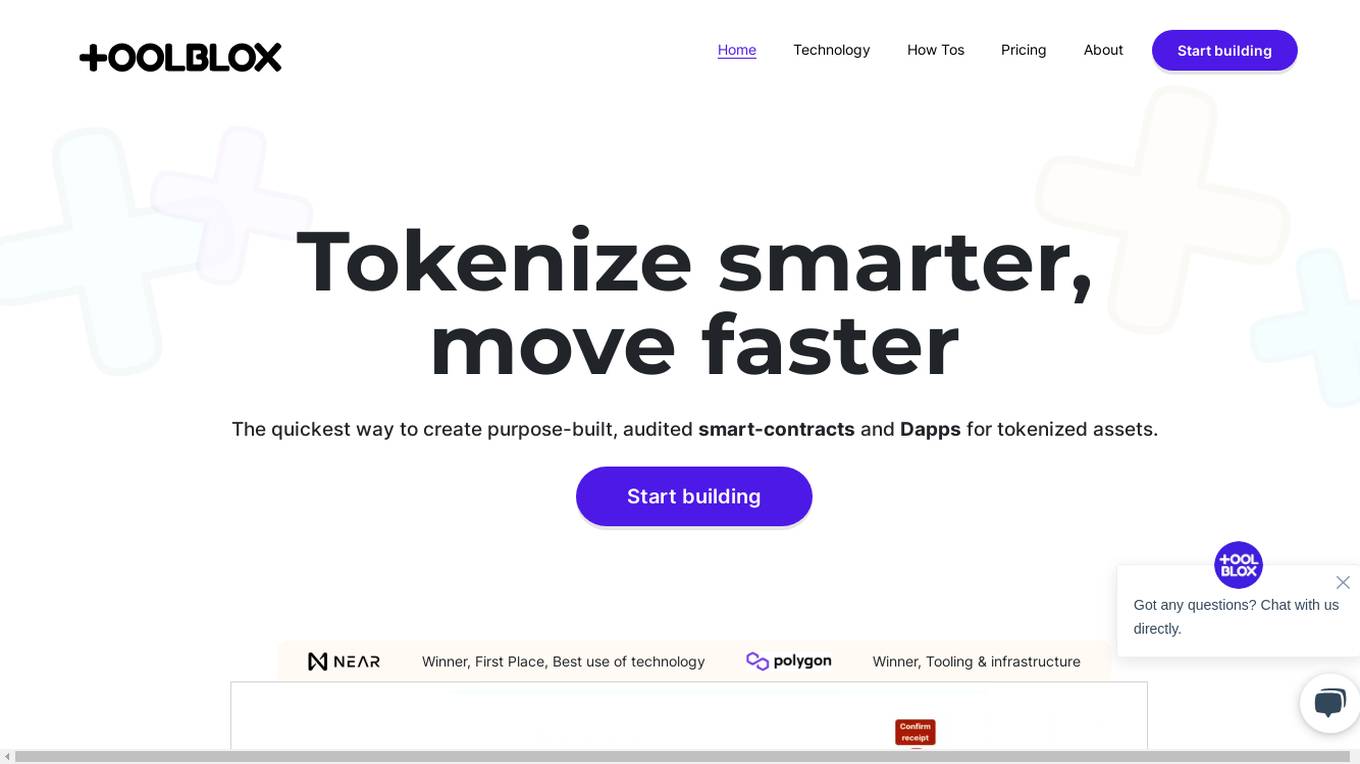
Toolblox
Toolblox is an AI-powered platform that enables users to create purpose-built, audited smart-contracts and Dapps for tokenized assets quickly and efficiently. It offers a no-code solution for turning ideas into smart-contracts, visualizing workflows, and creating tokenization solutions. With pre-audited smart-contracts, examples, and an AI assistant, Toolblox simplifies the process of building and launching decentralized applications. The platform caters to founders, agencies, and businesses looking to streamline their operations and leverage blockchain technology.
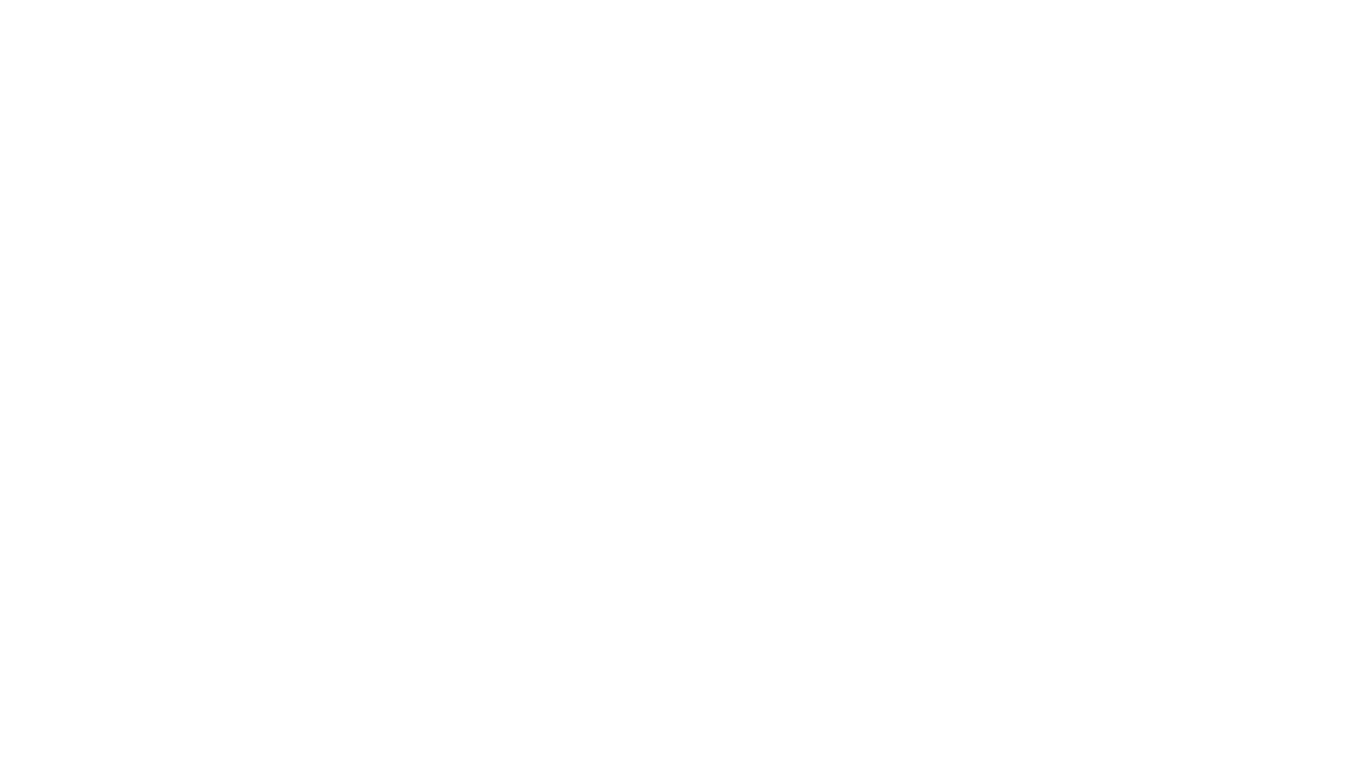
Basis Theory
Basis Theory is a token orchestration platform that helps businesses route transactions through multiple PSPs and partners or unlock seamless subscription payments while ensuring PCI compliance. It offers flexible payment solutions, secure data handling, and industry-tailored payment flows to boost sales and reduce friction. The platform allows users to stand up a vault in minutes, customize payment flows, and work with various partners without service limitations. Basis Theory caters to high-risk merchants, subscription platforms, marketplaces, fintechs, and more, providing tailored payment experiences and compliance solutions.
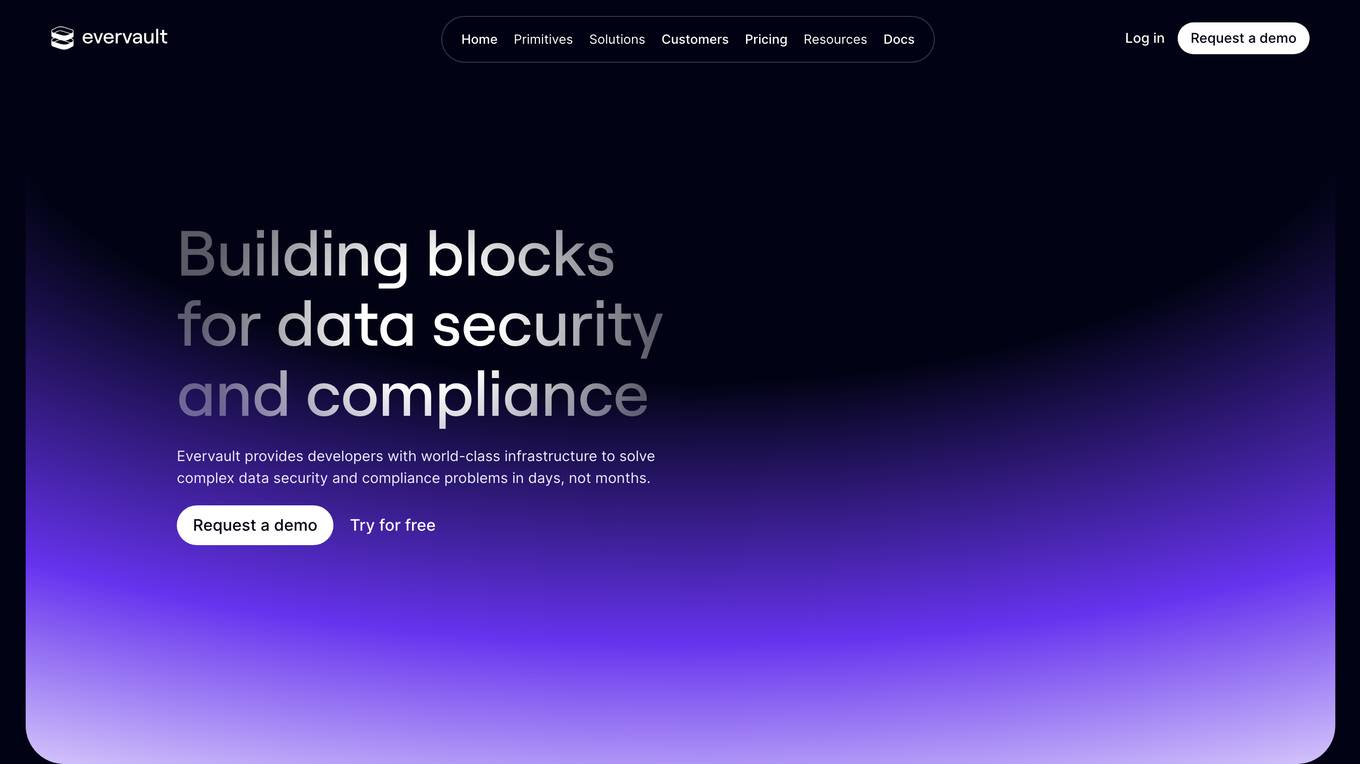
Evervault
Evervault is a platform that provides flexible payments security solutions for businesses. It offers tools to tokenize cards, optimize margins, comply with PCI standards, avoid gateway lock-in, and set up card issuing programs. Evervault helps businesses secure sensitive payment data and accelerate their payment processes with a focus on security, compliance, and performance.
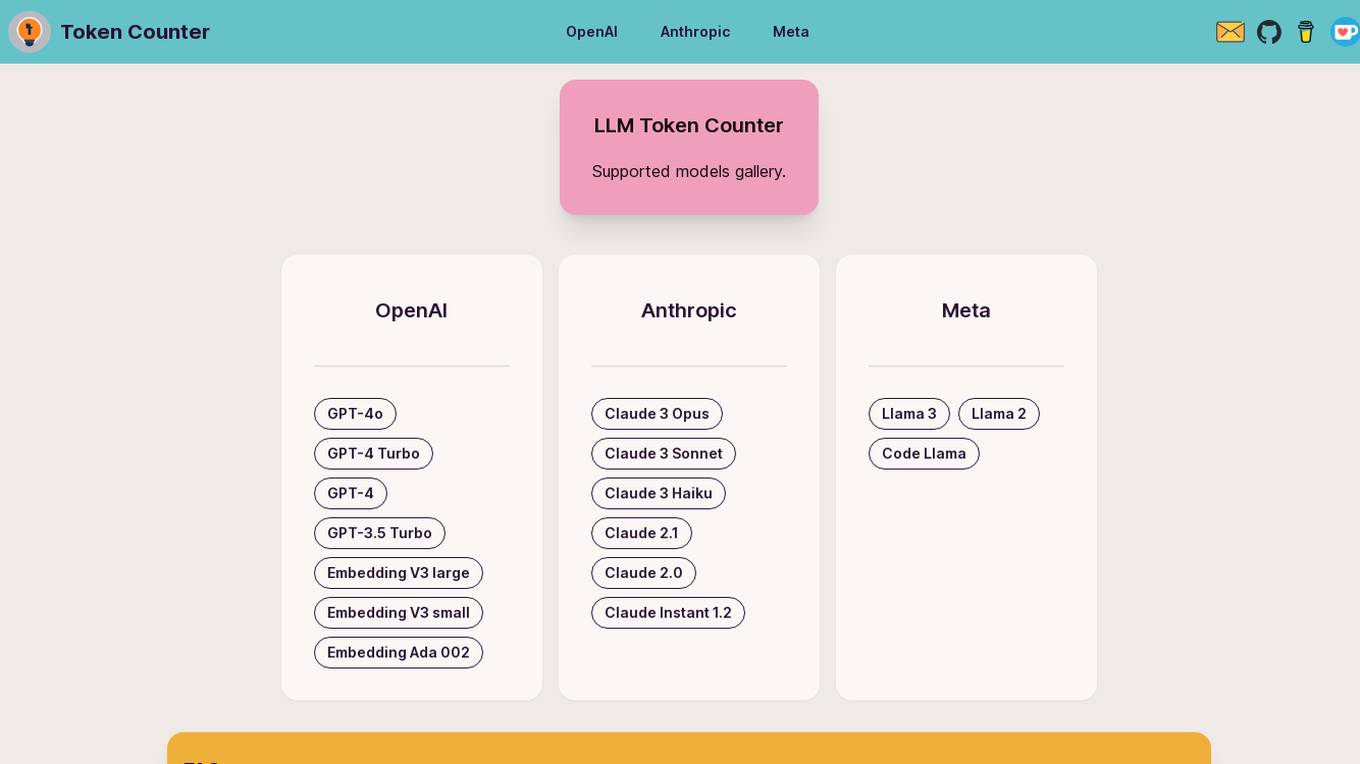
LLM Token Counter
The LLM Token Counter is a sophisticated tool designed to help users effectively manage token limits for various Language Models (LLMs) like GPT-3.5, GPT-4, Claude-3, Llama-3, and more. It utilizes Transformers.js, a JavaScript implementation of the Hugging Face Transformers library, to calculate token counts client-side. The tool ensures data privacy by not transmitting prompts to external servers.
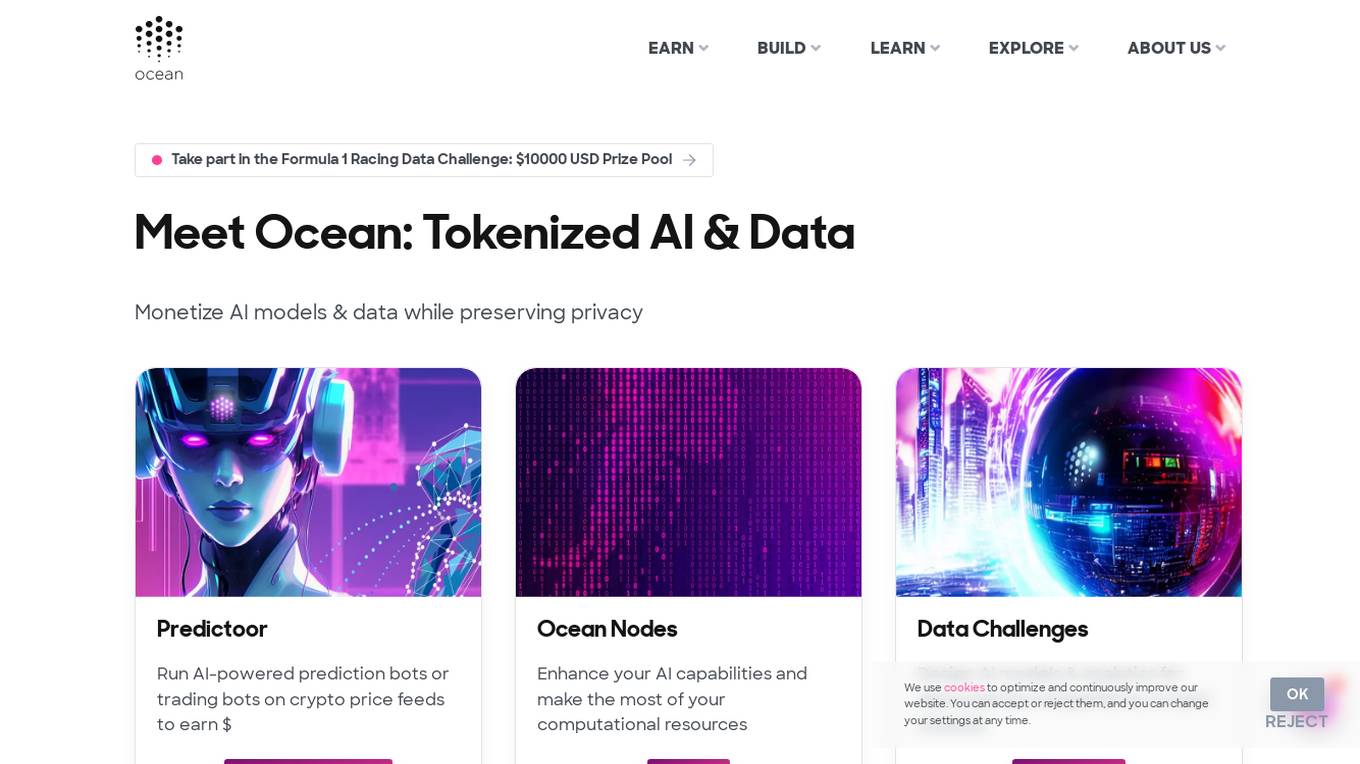
Ocean Protocol
Ocean Protocol is a tokenized AI and data platform that enables users to monetize AI models and data while maintaining privacy. It offers tools like Predictoor for running AI-powered prediction bots, Ocean Nodes for enhancing AI capabilities, and features like Data NFTs and Datatokens for protecting intellectual property and controlling data access. The platform focuses on decentralized AI, privacy, and modular architecture to empower users in the AI and data science domains.
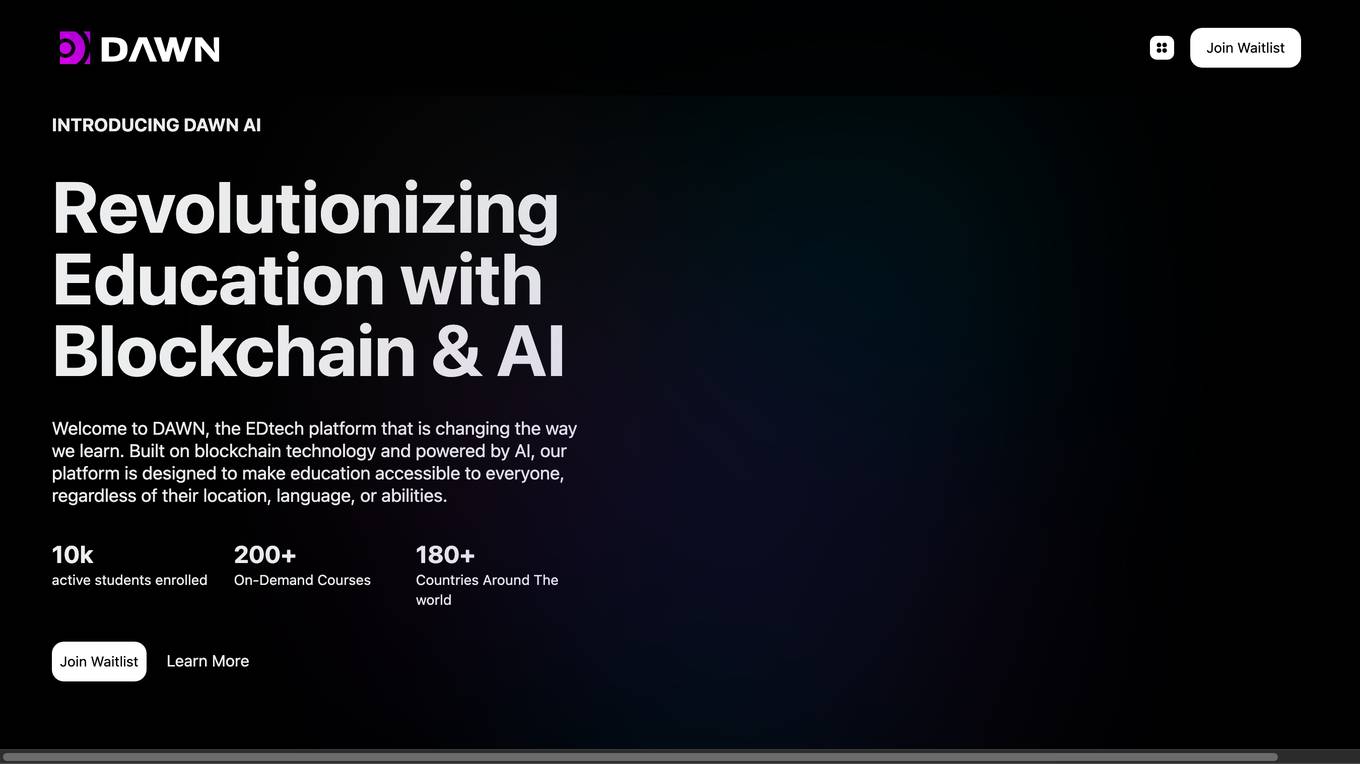
DAWN AI
DAWN AI is an EDtech platform that is revolutionizing education with blockchain and AI. It is designed to make education accessible to everyone, regardless of their location, language, or abilities. DAWN offers a complete suite of blockchain-scaling solutions, including course transcription, AI recruitment services, a dyslexia-friendly platform, closed captioning and sign language interpretation, and tokenized affiliate marketing. It also has a Learn and Earn program in the metaverse, where learners can earn tokens by completing educational challenges and tasks in virtual worlds.
20 - Open Source AI Tools
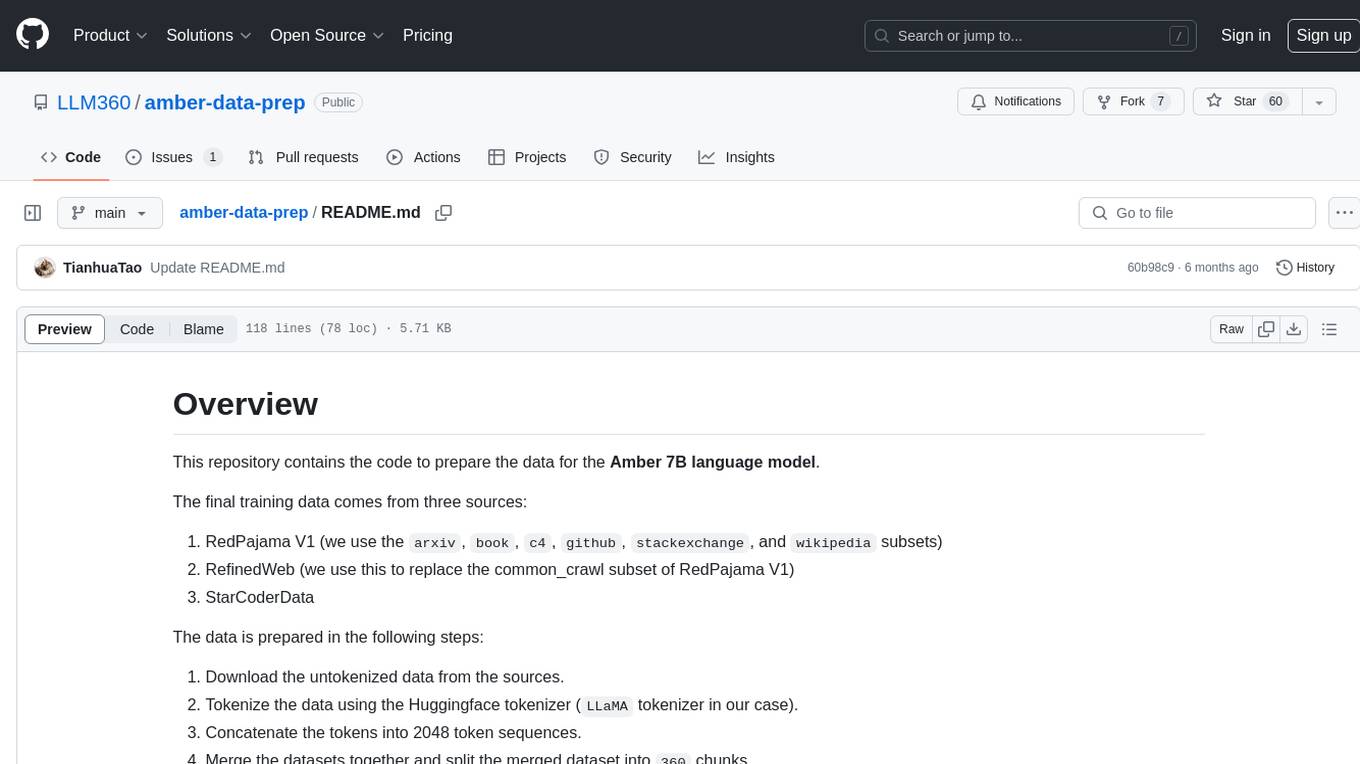
amber-data-prep
This repository contains the code to prepare the data for the Amber 7B language model. The final training data comes from three sources: RedPajama V1, RefinedWeb, and StarCoderData. The data preparation involves downloading untokenized data, tokenizing the data using the Huggingface tokenizer, concatenating tokens into 2048 token sequences, merging datasets, and splitting the merged dataset into 360 chunks. Each tokenized data chunk is a jsonl file containing samples with 2049 tokens. The repository provides scripts for downloading datasets, tokenizing and concatenating sequences, validating data, and merging subsets into chunks.
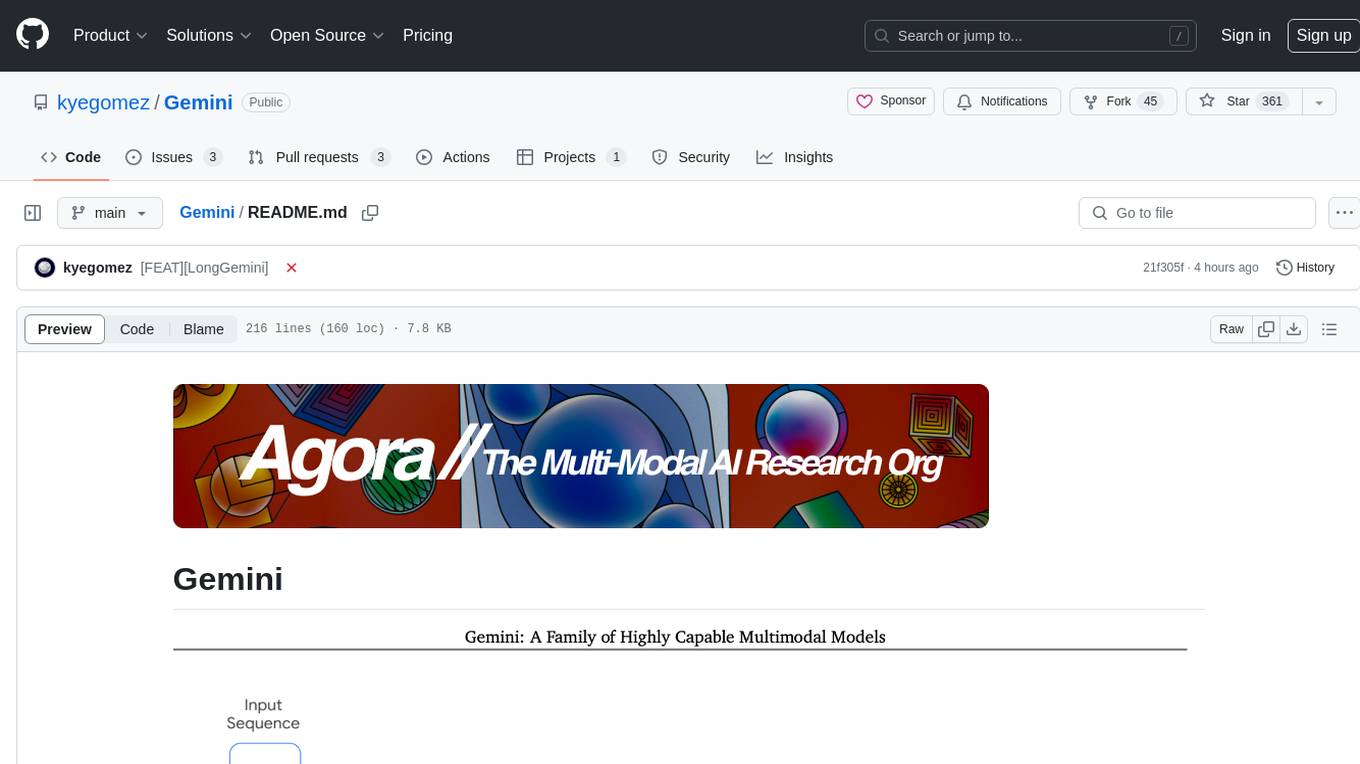
Gemini
Gemini is an open-source model designed to handle multiple modalities such as text, audio, images, and videos. It utilizes a transformer architecture with special decoders for text and image generation. The model processes input sequences by transforming them into tokens and then decoding them to generate image outputs. Gemini differs from other models by directly feeding image embeddings into the transformer instead of using a visual transformer encoder. The model also includes a component called Codi for conditional generation. Gemini aims to effectively integrate image, audio, and video embeddings to enhance its performance.
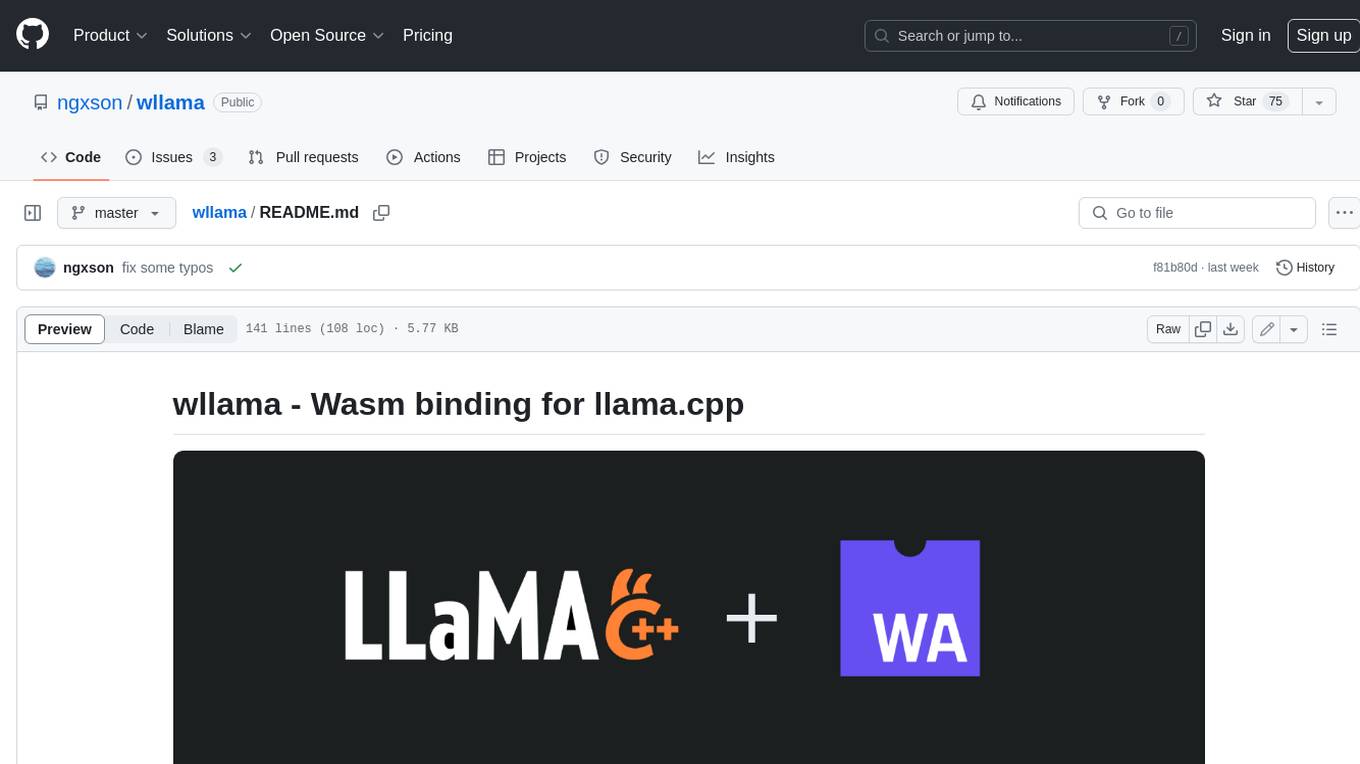
wllama
Wllama is a WebAssembly binding for llama.cpp, a high-performance and lightweight language model library. It enables you to run inference directly on the browser without the need for a backend or GPU. Wllama provides both high-level and low-level APIs, allowing you to perform various tasks such as completions, embeddings, tokenization, and more. It also supports model splitting, enabling you to load large models in parallel for faster download. With its Typescript support and pre-built npm package, Wllama is easy to integrate into your React Typescript projects.
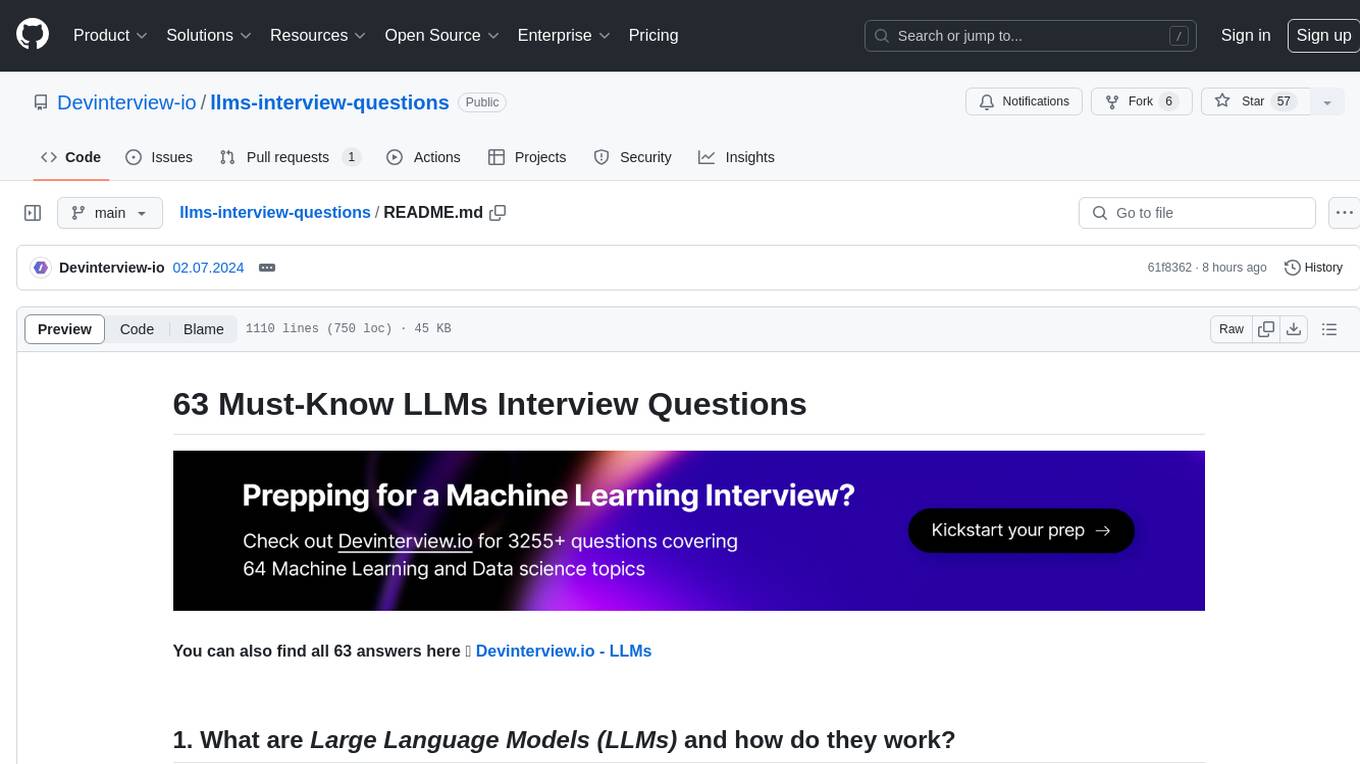
llms-interview-questions
This repository contains a comprehensive collection of 63 must-know Large Language Models (LLMs) interview questions. It covers topics such as the architecture of LLMs, transformer models, attention mechanisms, training processes, encoder-decoder frameworks, differences between LLMs and traditional statistical language models, handling context and long-term dependencies, transformers for parallelization, applications of LLMs, sentiment analysis, language translation, conversation AI, chatbots, and more. The readme provides detailed explanations, code examples, and insights into utilizing LLMs for various tasks.
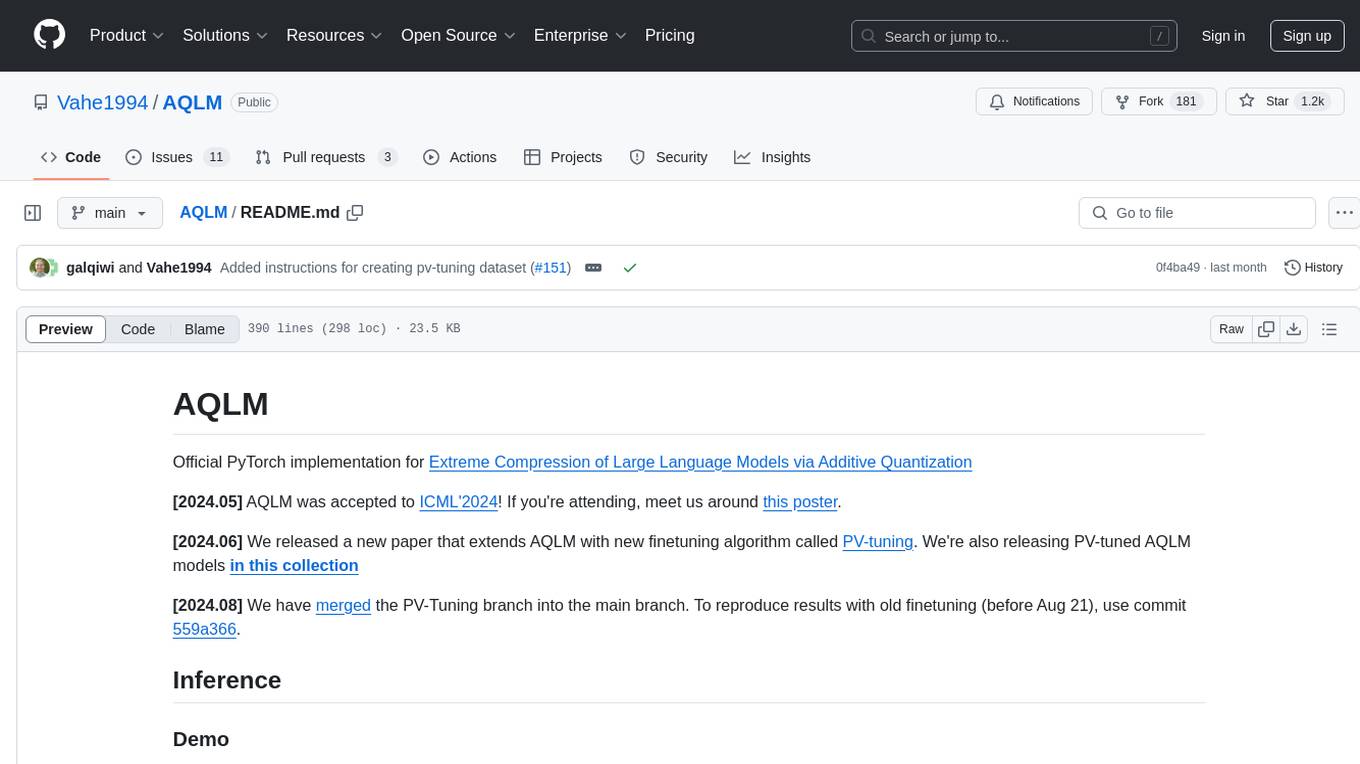
AQLM
AQLM is the official PyTorch implementation for Extreme Compression of Large Language Models via Additive Quantization. It includes prequantized AQLM models without PV-Tuning and PV-Tuned models for LLaMA, Mistral, and Mixtral families. The repository provides inference examples, model details, and quantization setups. Users can run prequantized models using Google Colab examples, work with different model families, and install the necessary inference library. The repository also offers detailed instructions for quantization, fine-tuning, and model evaluation. AQLM quantization involves calibrating models for compression, and users can improve model accuracy through finetuning. Additionally, the repository includes information on preparing models for inference and contributing guidelines.
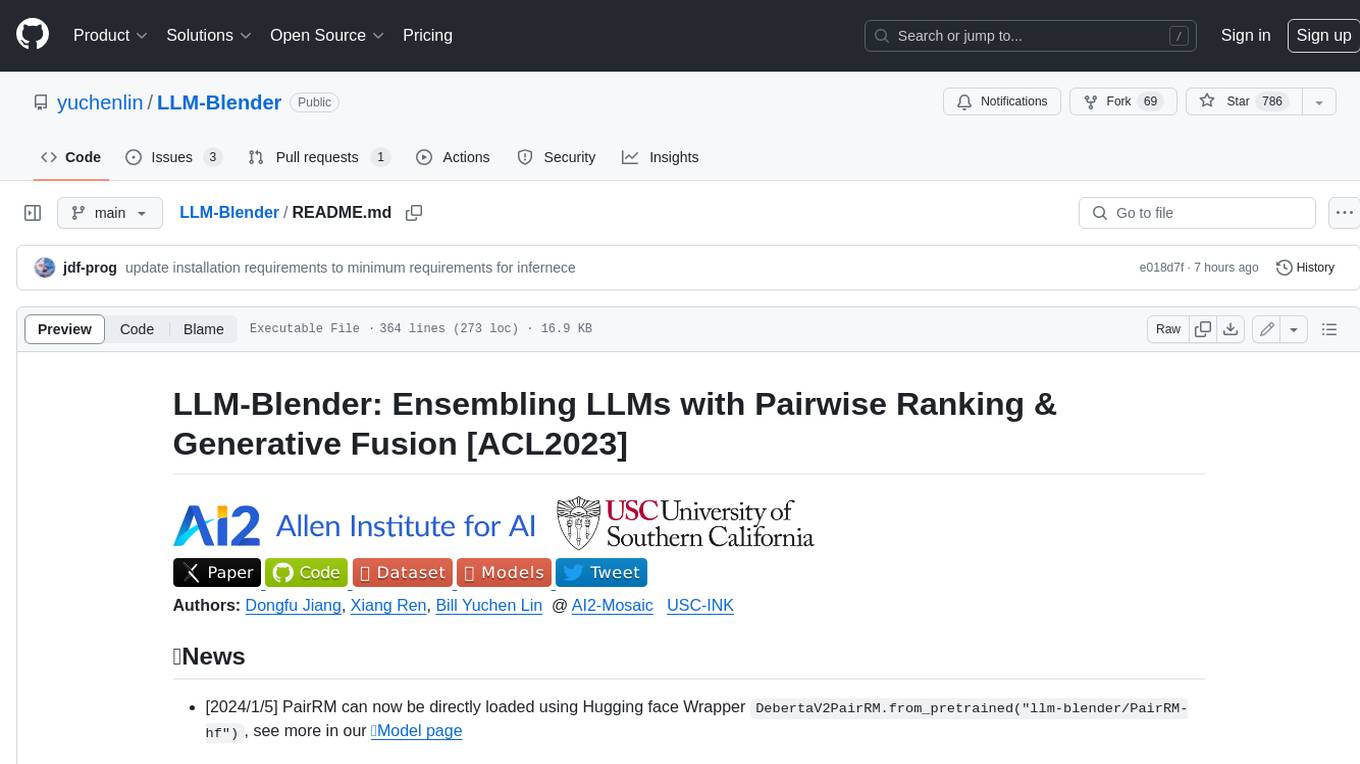
LLM-Blender
LLM-Blender is a framework for ensembling large language models (LLMs) to achieve superior performance. It consists of two modules: PairRanker and GenFuser. PairRanker uses pairwise comparisons to distinguish between candidate outputs, while GenFuser merges the top-ranked candidates to create an improved output. LLM-Blender has been shown to significantly surpass the best LLMs and baseline ensembling methods across various metrics on the MixInstruct benchmark dataset.
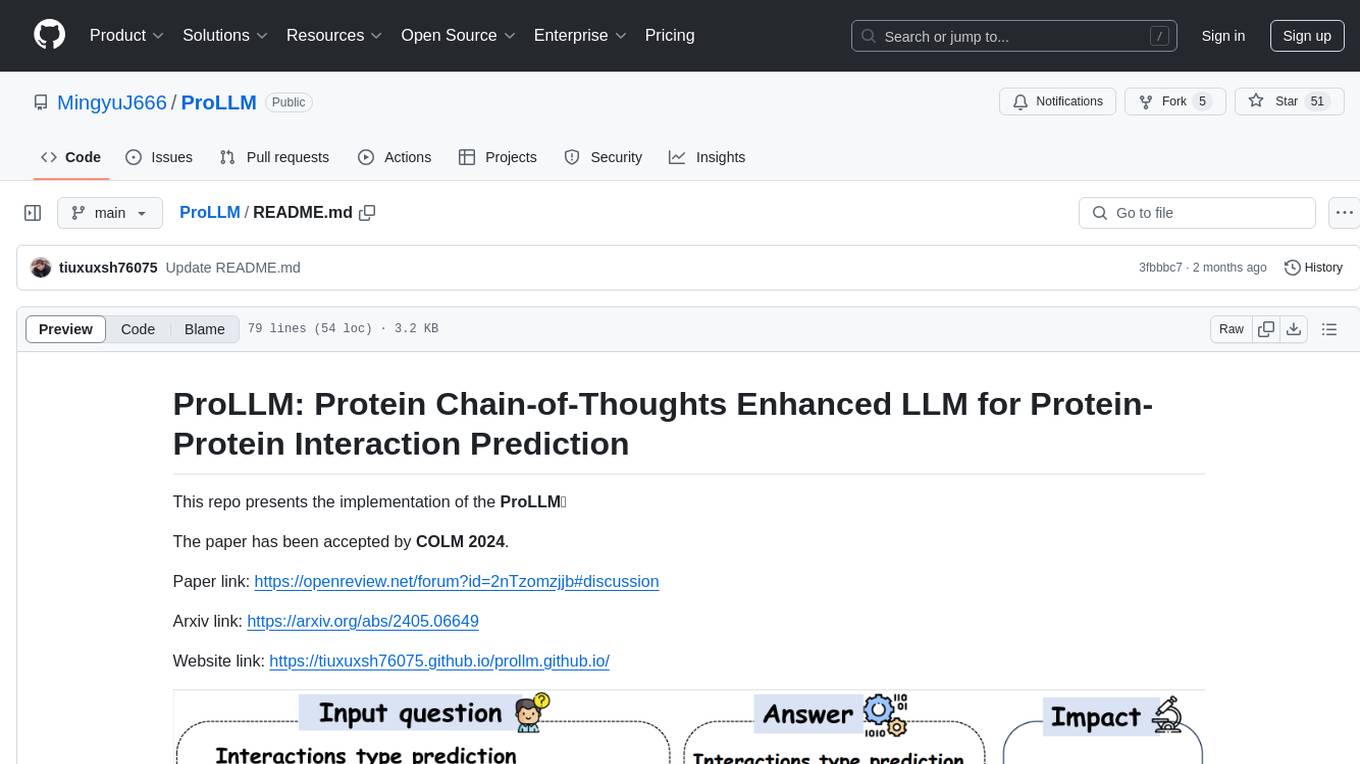
ProLLM
ProLLM is a framework that leverages Large Language Models to interpret and analyze protein sequences and interactions through natural language processing. It introduces the Protein Chain of Thought (ProCoT) method to transform complex protein interaction data into intuitive prompts, enhancing predictive accuracy by incorporating protein-specific embeddings and fine-tuning on domain-specific datasets.
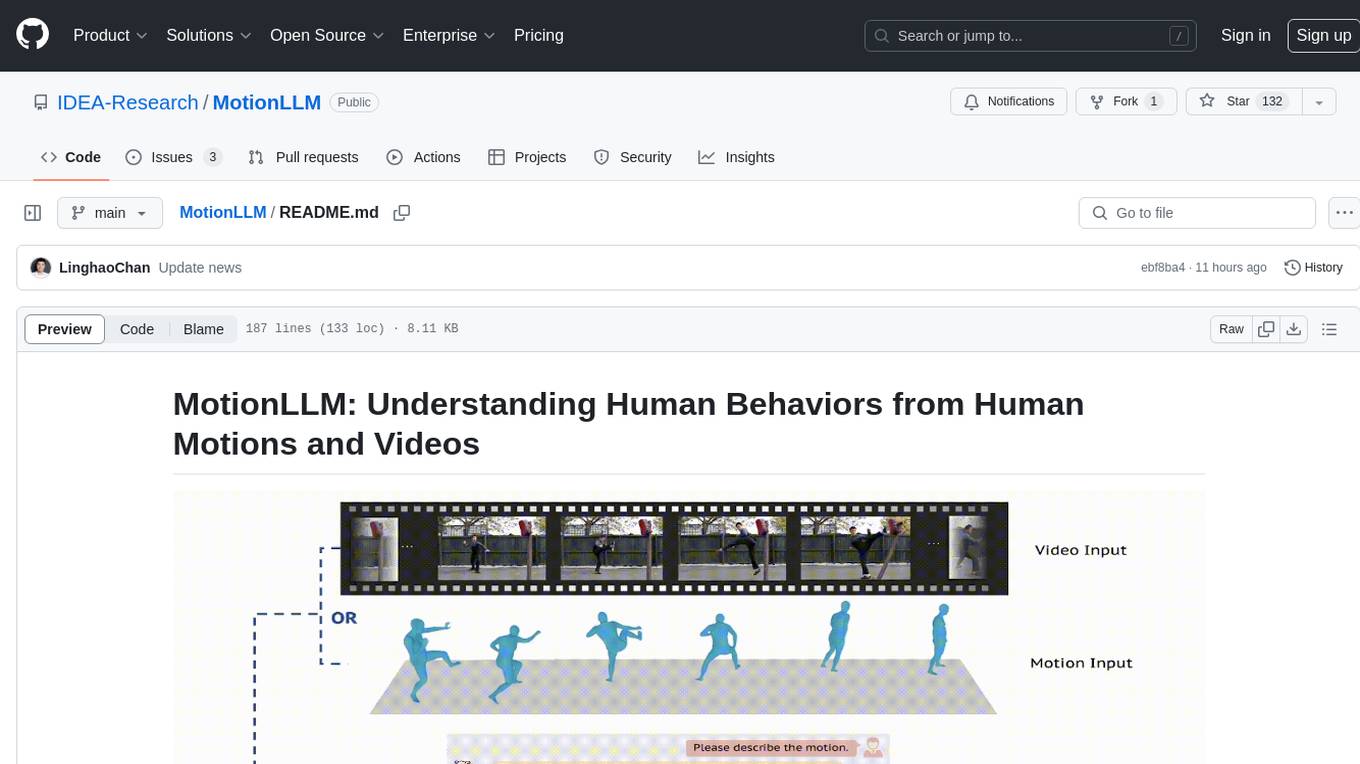
MotionLLM
MotionLLM is a framework for human behavior understanding that leverages Large Language Models (LLMs) to jointly model videos and motion sequences. It provides a unified training strategy, dataset MoVid, and MoVid-Bench for evaluating human behavior comprehension. The framework excels in captioning, spatial-temporal comprehension, and reasoning abilities.
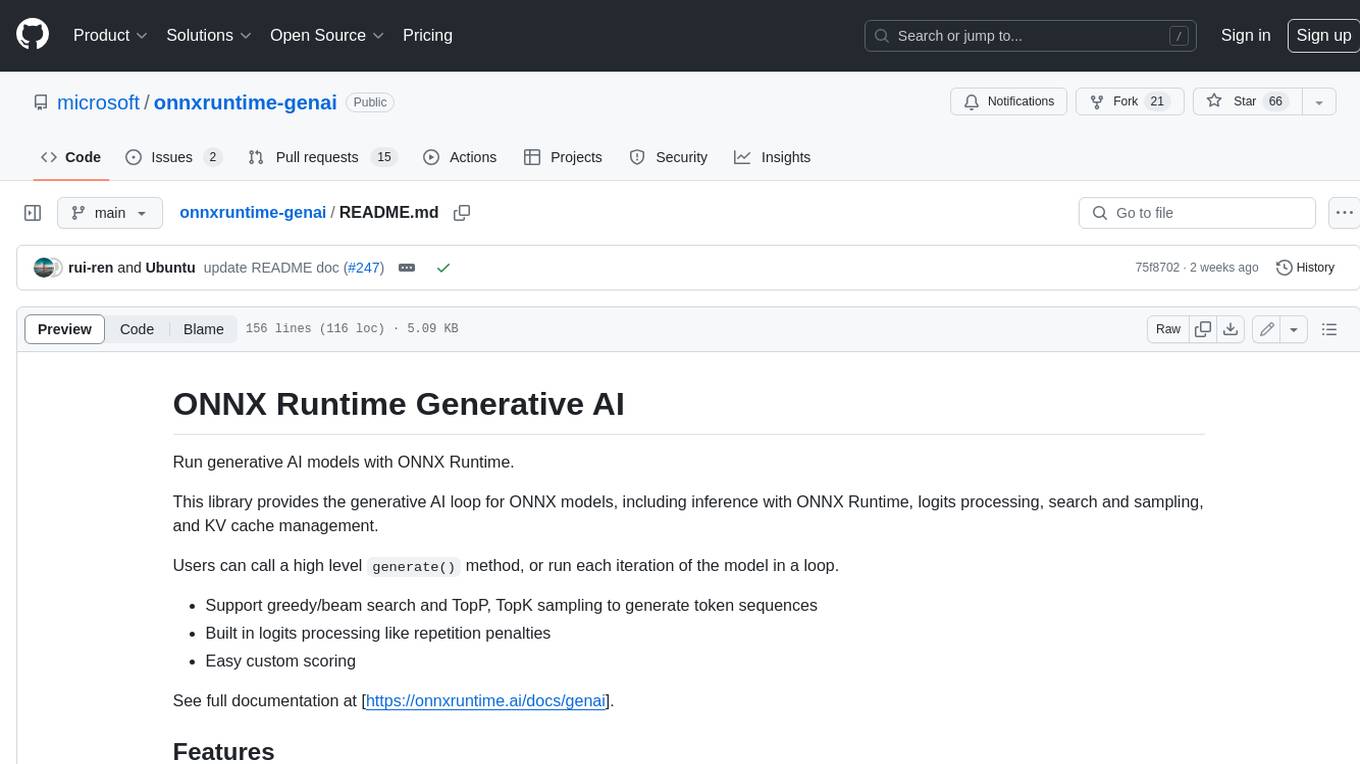
onnxruntime-genai
ONNX Runtime Generative AI is a library that provides the generative AI loop for ONNX models, including inference with ONNX Runtime, logits processing, search and sampling, and KV cache management. Users can call a high level `generate()` method, or run each iteration of the model in a loop. It supports greedy/beam search and TopP, TopK sampling to generate token sequences, has built in logits processing like repetition penalties, and allows for easy custom scoring.
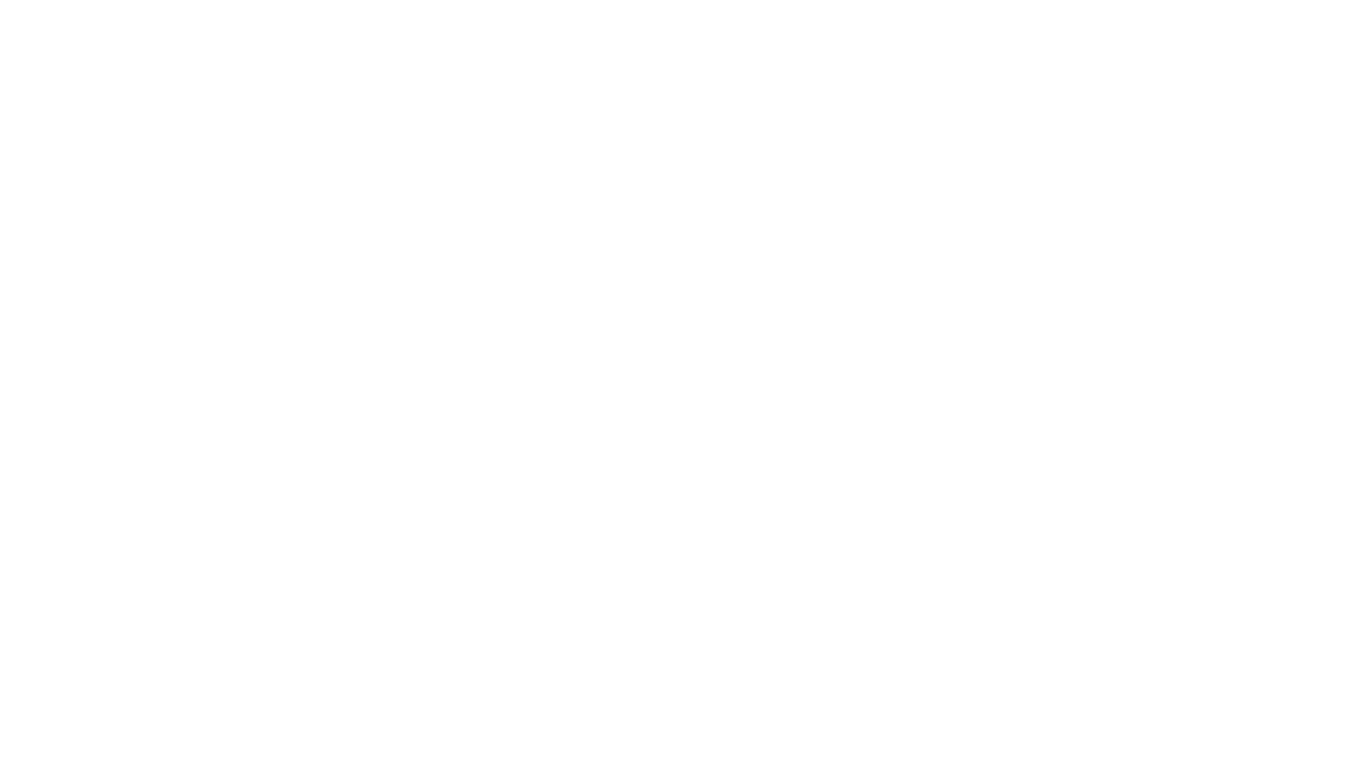
instructor-php
Instructor for PHP is a library designed for structured data extraction in PHP, powered by Large Language Models (LLMs). It simplifies the process of extracting structured, validated data from unstructured text or chat sequences. Instructor enhances workflow by providing a response model, validation capabilities, and max retries for requests. It supports classes as response models and provides features like partial results, string input, extracting scalar and enum values, and specifying data models using PHP type hints or DocBlock comments. The library allows customization of validation and provides detailed event notifications during request processing. Instructor is compatible with PHP 8.2+ and leverages PHP reflection, Symfony components, and SaloonPHP for communication with LLM API providers.
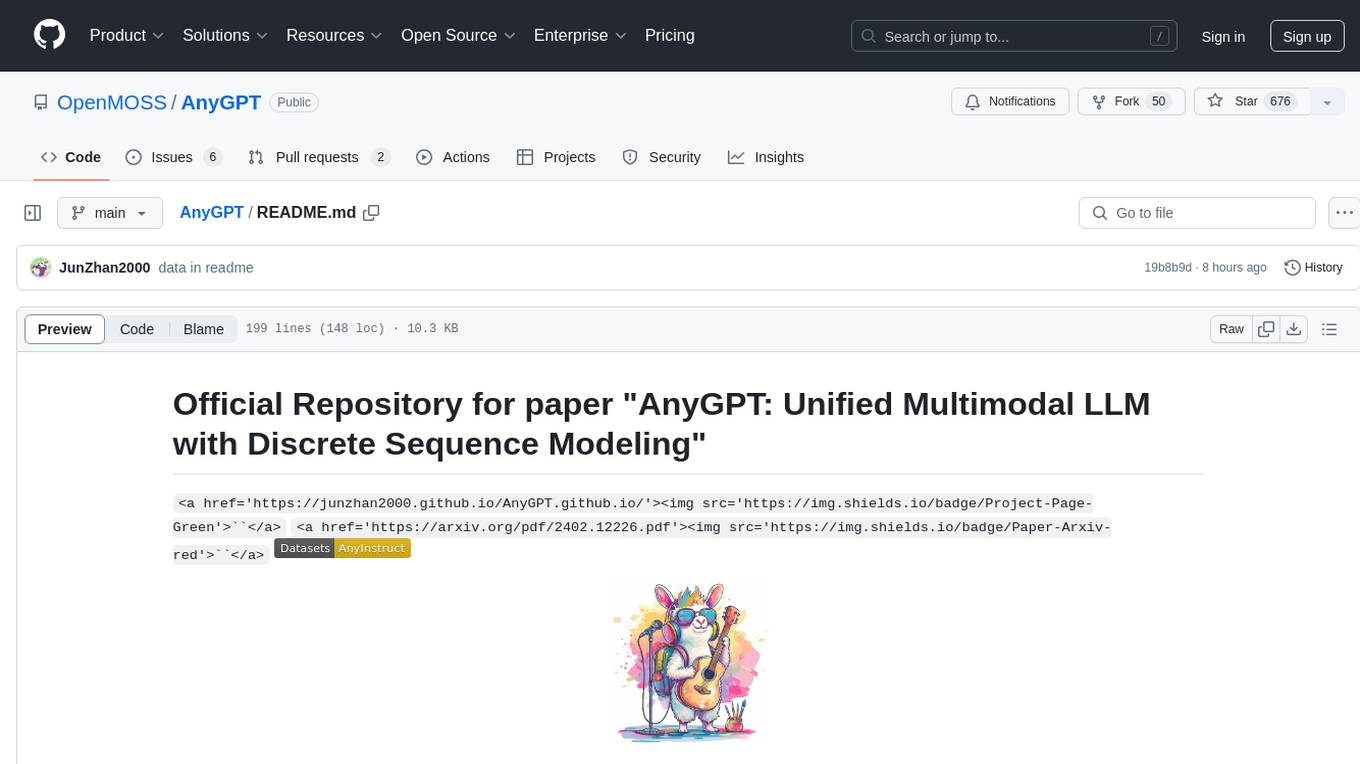
AnyGPT
AnyGPT is a unified multimodal language model that utilizes discrete representations for processing various modalities like speech, text, images, and music. It aligns the modalities for intermodal conversions and text processing. AnyInstruct dataset is constructed for generative models. The model proposes a generative training scheme using Next Token Prediction task for training on a Large Language Model (LLM). It aims to compress vast multimodal data on the internet into a single model for emerging capabilities. The tool supports tasks like text-to-image, image captioning, ASR, TTS, text-to-music, and music captioning.
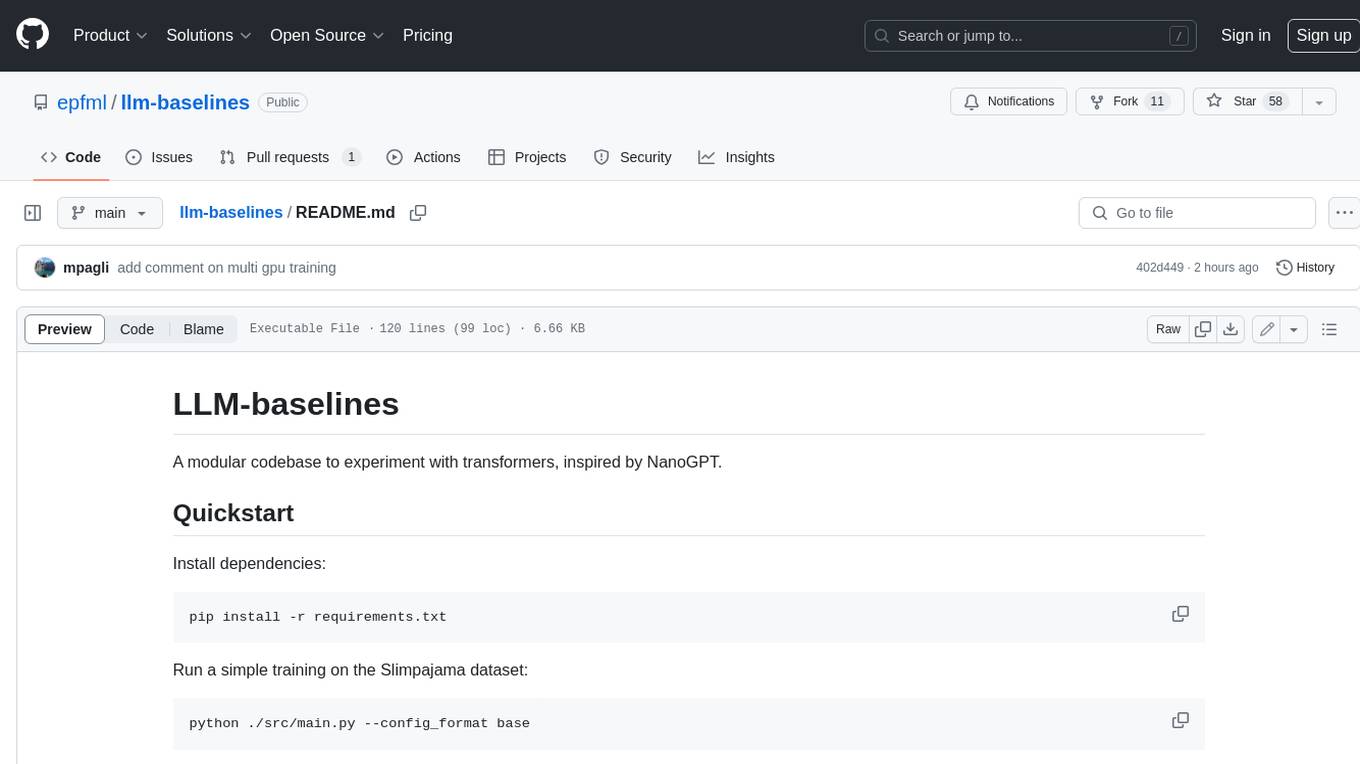
llm-baselines
LLM-baselines is a modular codebase to experiment with transformers, inspired from NanoGPT. It provides a quick and easy way to train and evaluate transformer models on a variety of datasets. The codebase is well-documented and easy to use, making it a great resource for researchers and practitioners alike.
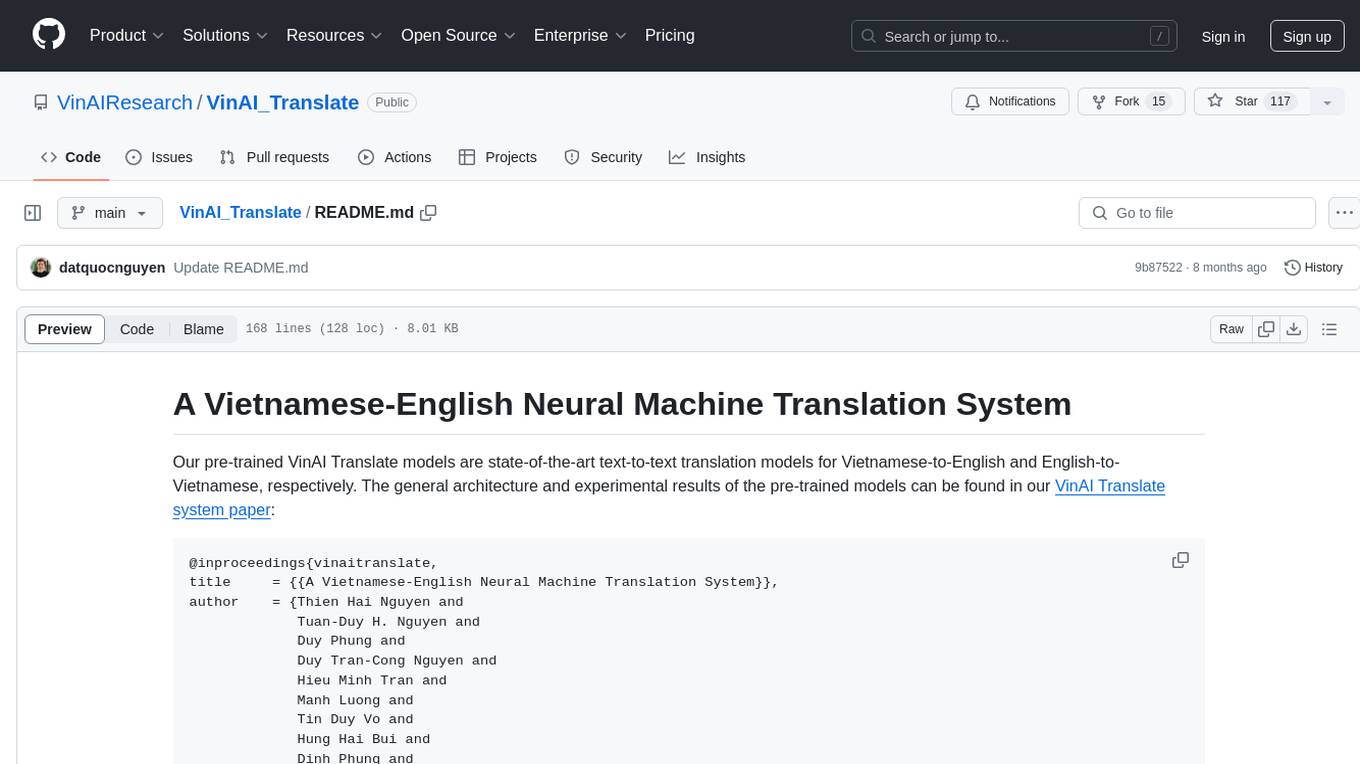
VinAI_Translate
VinAI_Translate is a Vietnamese-English Neural Machine Translation System offering state-of-the-art text-to-text translation models for Vietnamese-to-English and English-to-Vietnamese. The system includes pre-trained models with different configurations and parameters, allowing for further fine-tuning. Users can interact with the models through the VinAI Translate system website or the HuggingFace space 'VinAI Translate'. Evaluation scripts are available for assessing the translation quality. The tool can be used in the 'transformers' library for Vietnamese-to-English and English-to-Vietnamese translations, supporting both GPU-based batch translation and CPU-based sequence translation examples.
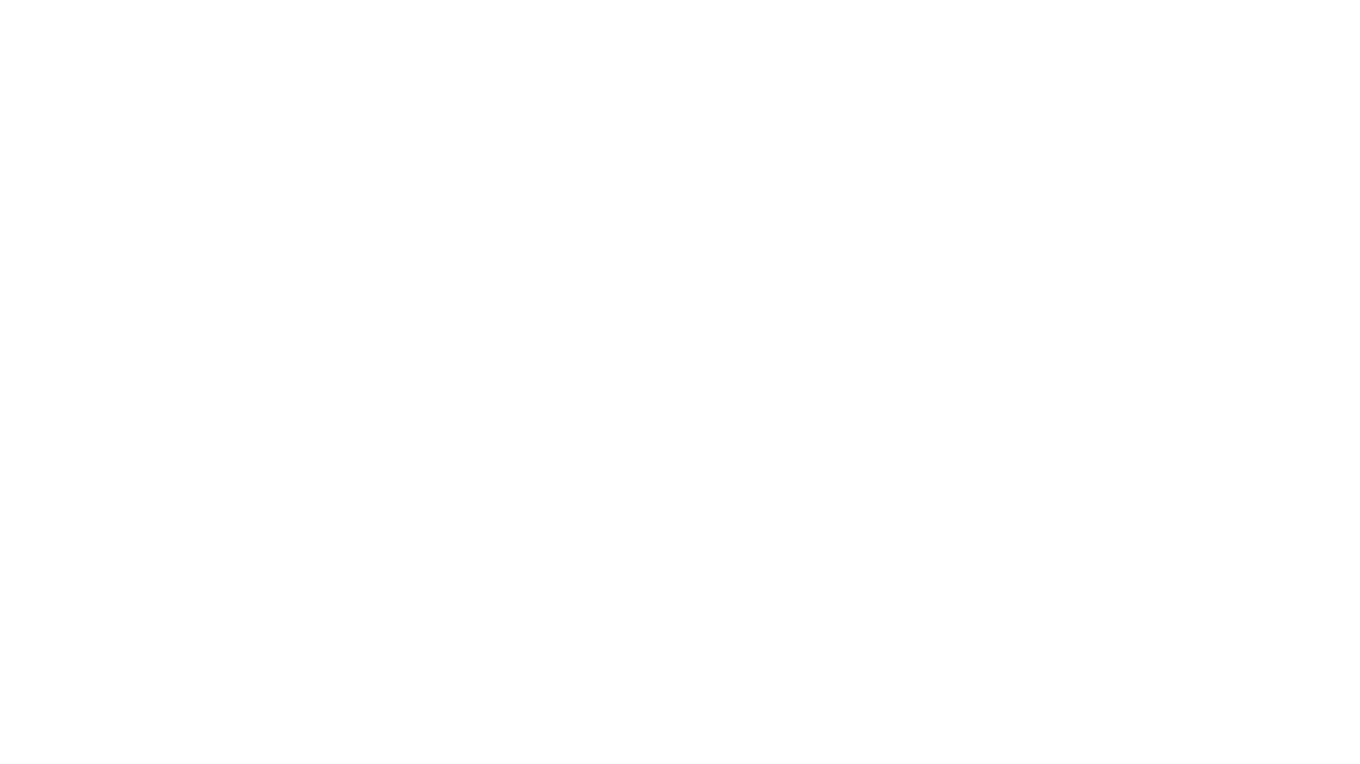
starcoder2-self-align
StarCoder2-Instruct is an open-source pipeline that introduces StarCoder2-15B-Instruct-v0.1, a self-aligned code Large Language Model (LLM) trained with a fully permissive and transparent pipeline. It generates instruction-response pairs to fine-tune StarCoder-15B without human annotations or data from proprietary LLMs. The tool is primarily finetuned for Python code generation tasks that can be verified through execution, with potential biases and limitations. Users can provide response prefixes or one-shot examples to guide the model's output. The model may have limitations with other programming languages and out-of-domain coding tasks.
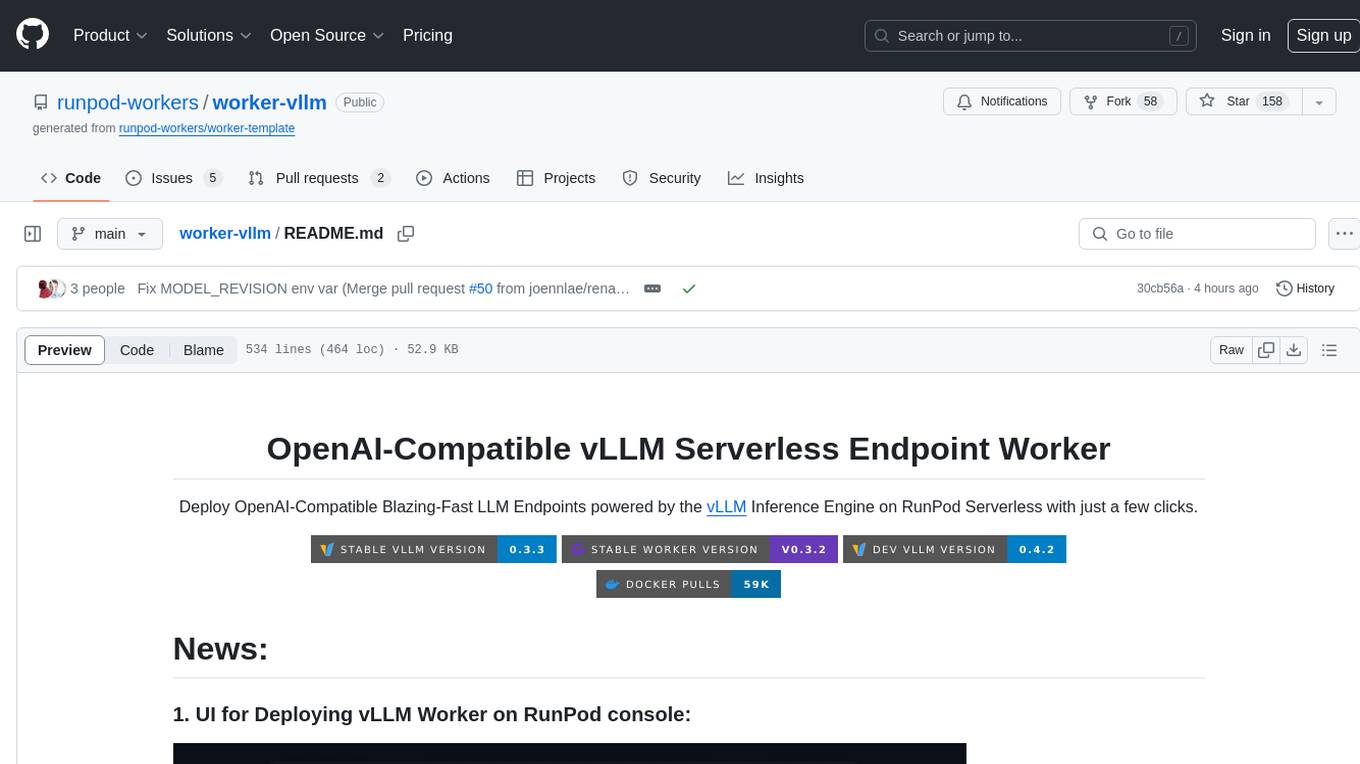
worker-vllm
The worker-vLLM repository provides a serverless endpoint for deploying OpenAI-compatible vLLM models with blazing-fast performance. It supports deploying various model architectures, such as Aquila, Baichuan, BLOOM, ChatGLM, Command-R, DBRX, DeciLM, Falcon, Gemma, GPT-2, GPT BigCode, GPT-J, GPT-NeoX, InternLM, Jais, LLaMA, MiniCPM, Mistral, Mixtral, MPT, OLMo, OPT, Orion, Phi, Phi-3, Qwen, Qwen2, Qwen2MoE, StableLM, Starcoder2, Xverse, and Yi. Users can deploy models using pre-built Docker images or build custom images with specified arguments. The repository also supports OpenAI compatibility for chat completions, completions, and models, with customizable input parameters. Users can modify their OpenAI codebase to use the deployed vLLM worker and access a list of available models for deployment.
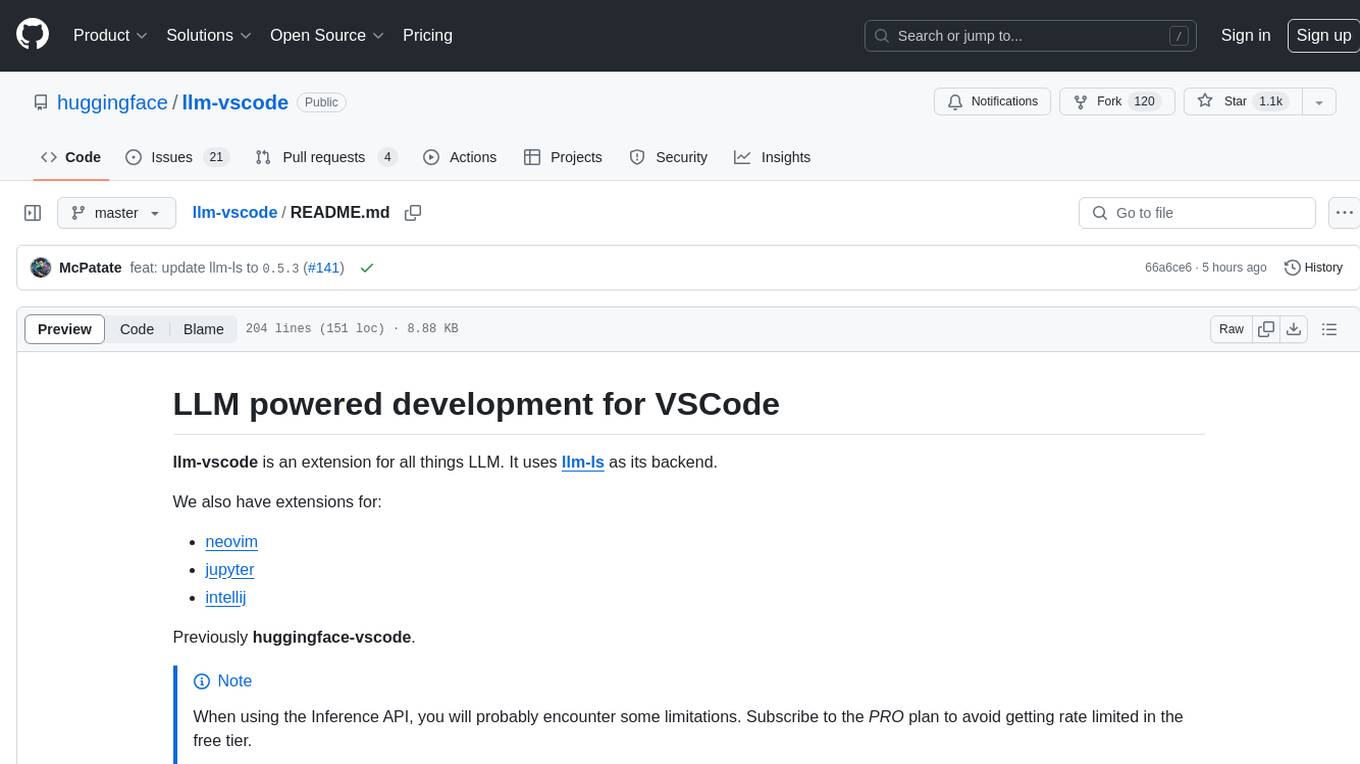
llm-vscode
llm-vscode is an extension designed for all things LLM, utilizing llm-ls as its backend. It offers features such as code completion with 'ghost-text' suggestions, the ability to choose models for code generation via HTTP requests, ensuring prompt size fits within the context window, and code attribution checks. Users can configure the backend, suggestion behavior, keybindings, llm-ls settings, and tokenization options. Additionally, the extension supports testing models like Code Llama 13B, Phind/Phind-CodeLlama-34B-v2, and WizardLM/WizardCoder-Python-34B-V1.0. Development involves cloning llm-ls, building it, and setting up the llm-vscode extension for use.
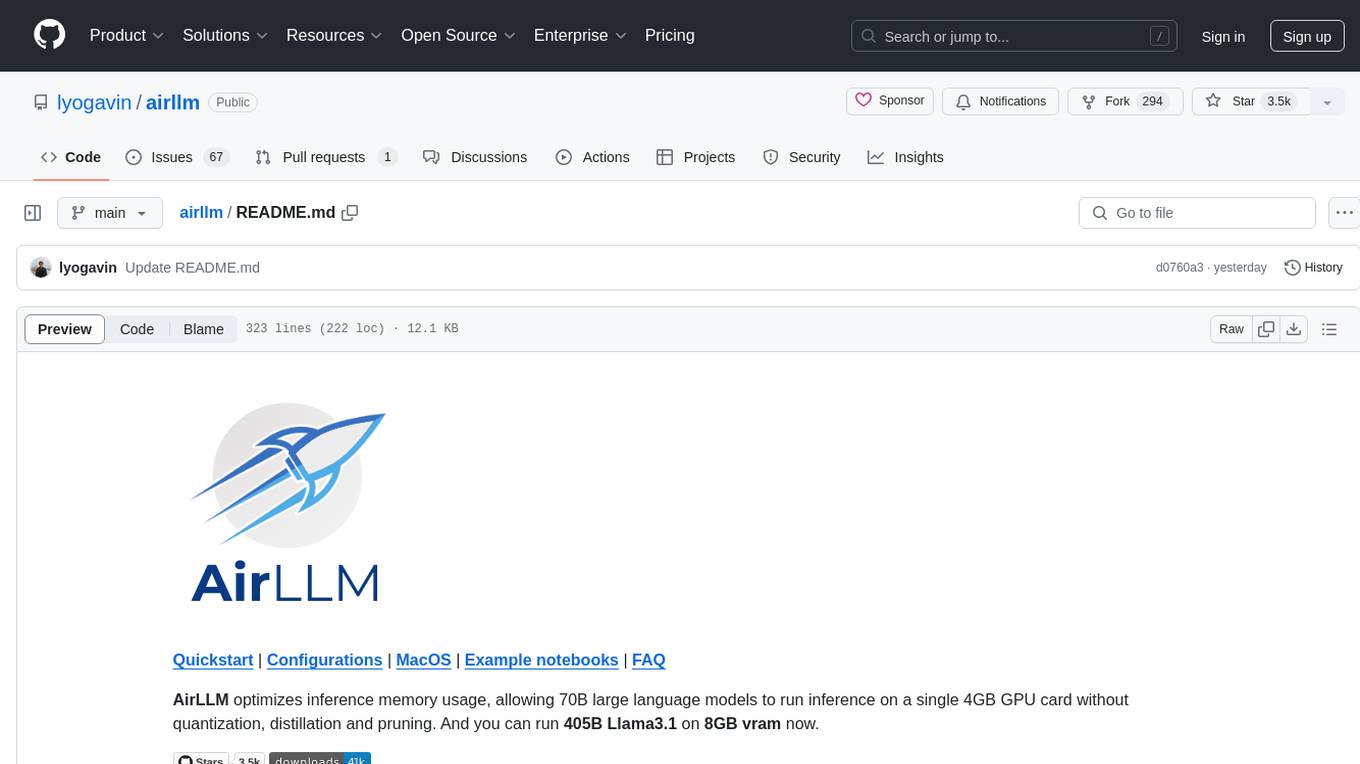
airllm
AirLLM is a tool that optimizes inference memory usage, enabling large language models to run on low-end GPUs without quantization, distillation, or pruning. It supports models like Llama3.1 on 8GB VRAM. The tool offers model compression for up to 3x inference speedup with minimal accuracy loss. Users can specify compression levels, profiling modes, and other configurations when initializing models. AirLLM also supports prefetching and disk space management. It provides examples and notebooks for easy implementation and usage.