Best AI tools for< Dataset Preparation >
20 - AI tool Sites
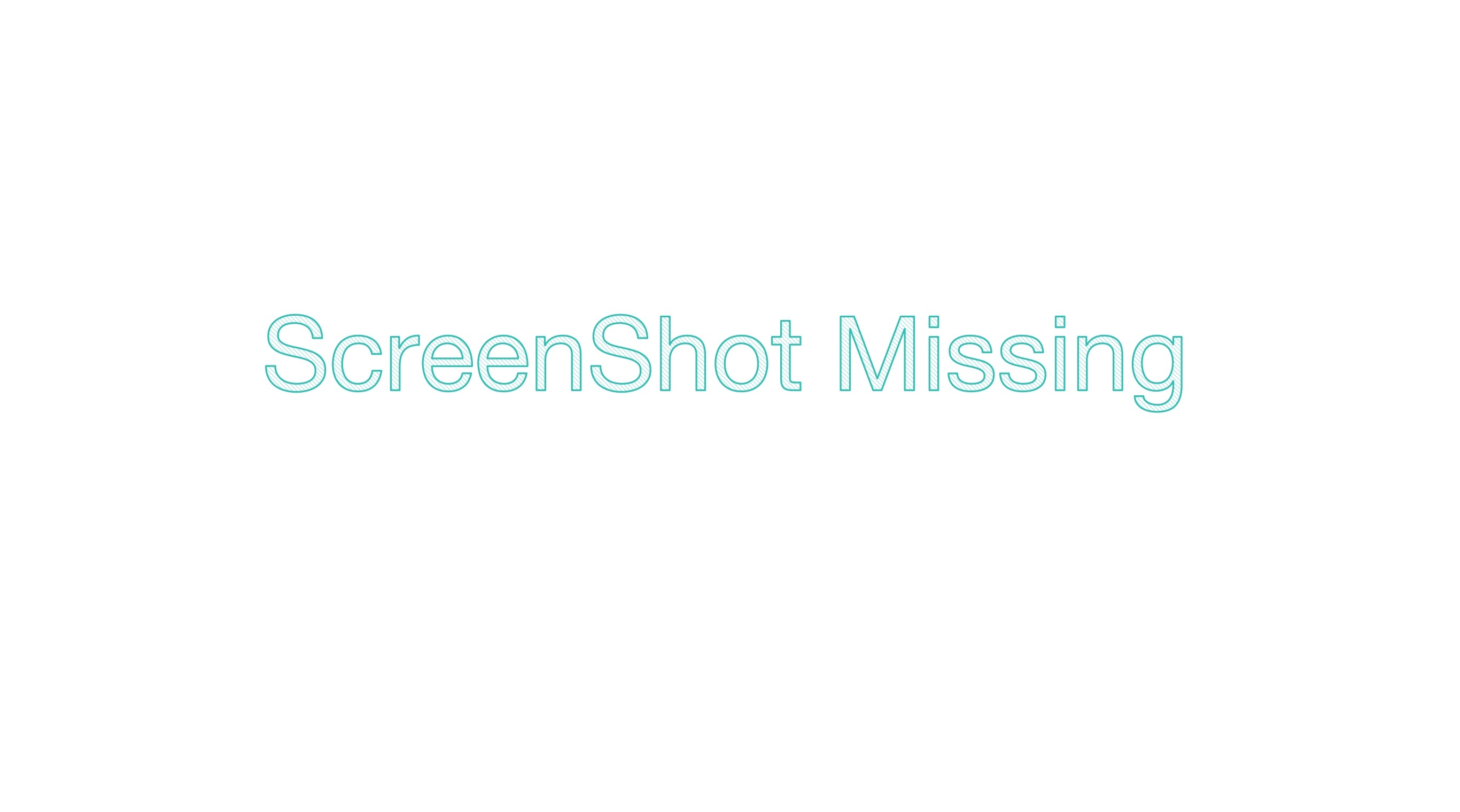
Unsloth
Unsloth is an AI tool designed to make finetuning large language models like Llama-3, Mistral, Phi-3, and Gemma 2x faster, use 70% less memory, and with no degradation in accuracy. The tool provides documentation to help users navigate through training their custom models, covering essentials such as installing and updating Unsloth, creating datasets, running, and deploying models. Users can also integrate third-party tools and utilize platforms like Google Colab.
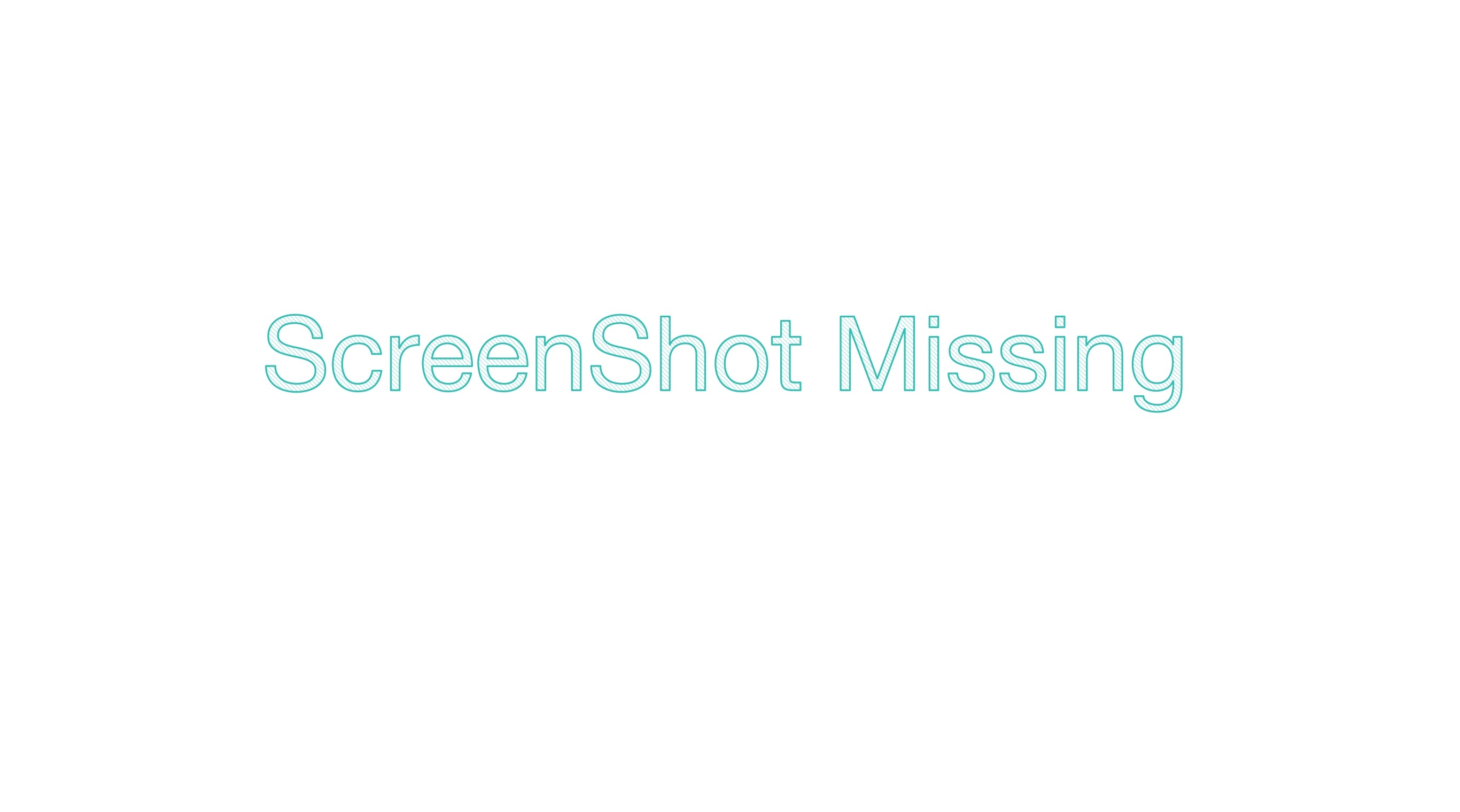
dataset.macgence
dataset.macgence is an AI-powered data analysis tool that helps users extract valuable insights from their datasets. It offers a user-friendly interface for uploading, cleaning, and analyzing data, making it suitable for both beginners and experienced data analysts. With advanced algorithms and visualization capabilities, dataset.macgence enables users to uncover patterns, trends, and correlations in their data, leading to informed decision-making. Whether you're a business professional, researcher, or student, dataset.macgence can streamline your data analysis process and enhance your data-driven strategies.
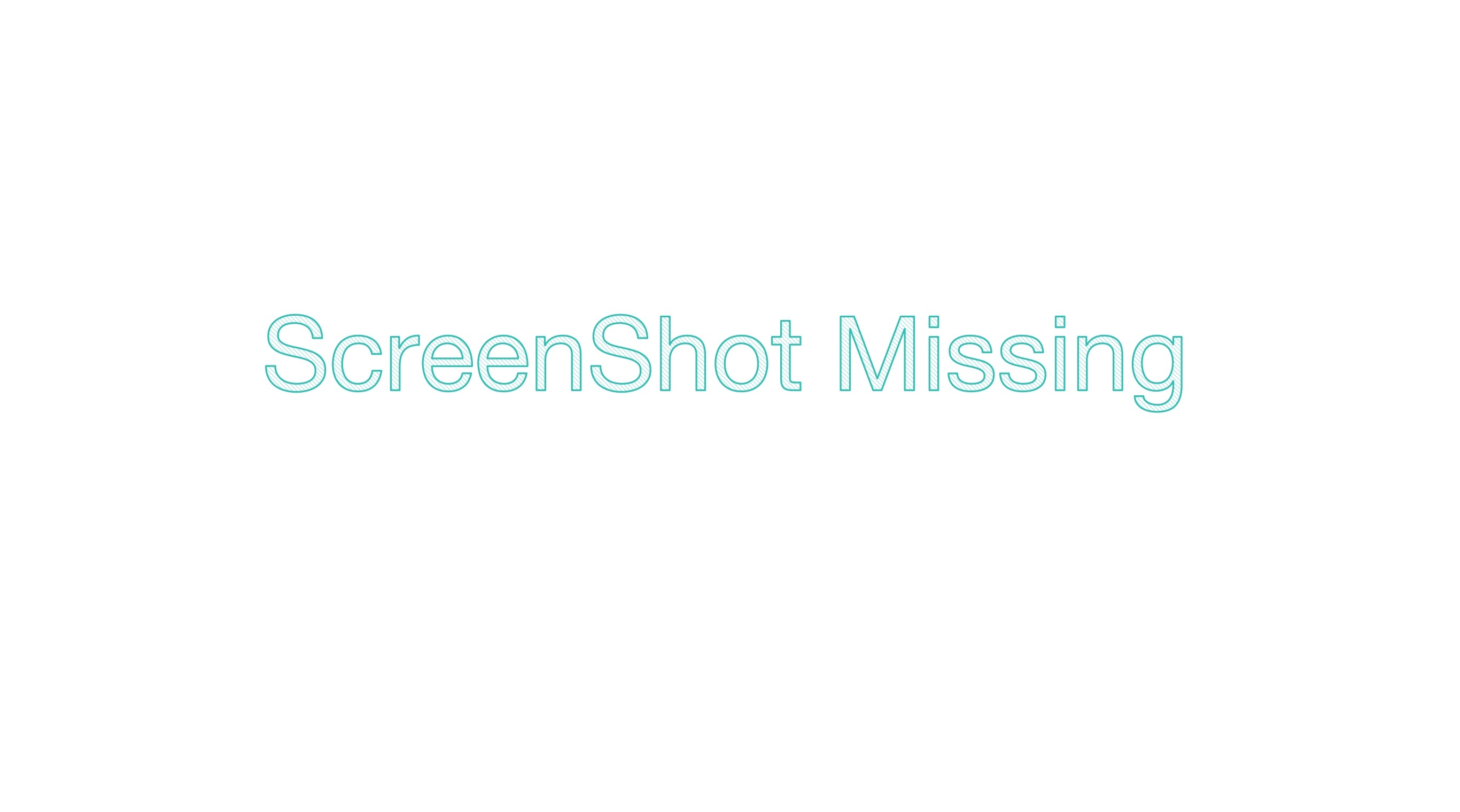
Deepfake Detection Challenge Dataset
The Deepfake Detection Challenge Dataset is a project initiated by Facebook AI to accelerate the development of new ways to detect deepfake videos. The dataset consists of over 100,000 videos and was created in collaboration with industry leaders and academic experts. It includes two versions: a preview dataset with 5k videos and a full dataset with 124k videos, each featuring facial modification algorithms. The dataset was used in a Kaggle competition to create better models for detecting manipulated media. The top-performing models achieved high accuracy on the public dataset but faced challenges when tested against the black box dataset, highlighting the importance of generalization in deepfake detection. The project aims to encourage the research community to continue advancing in detecting harmful manipulated media.
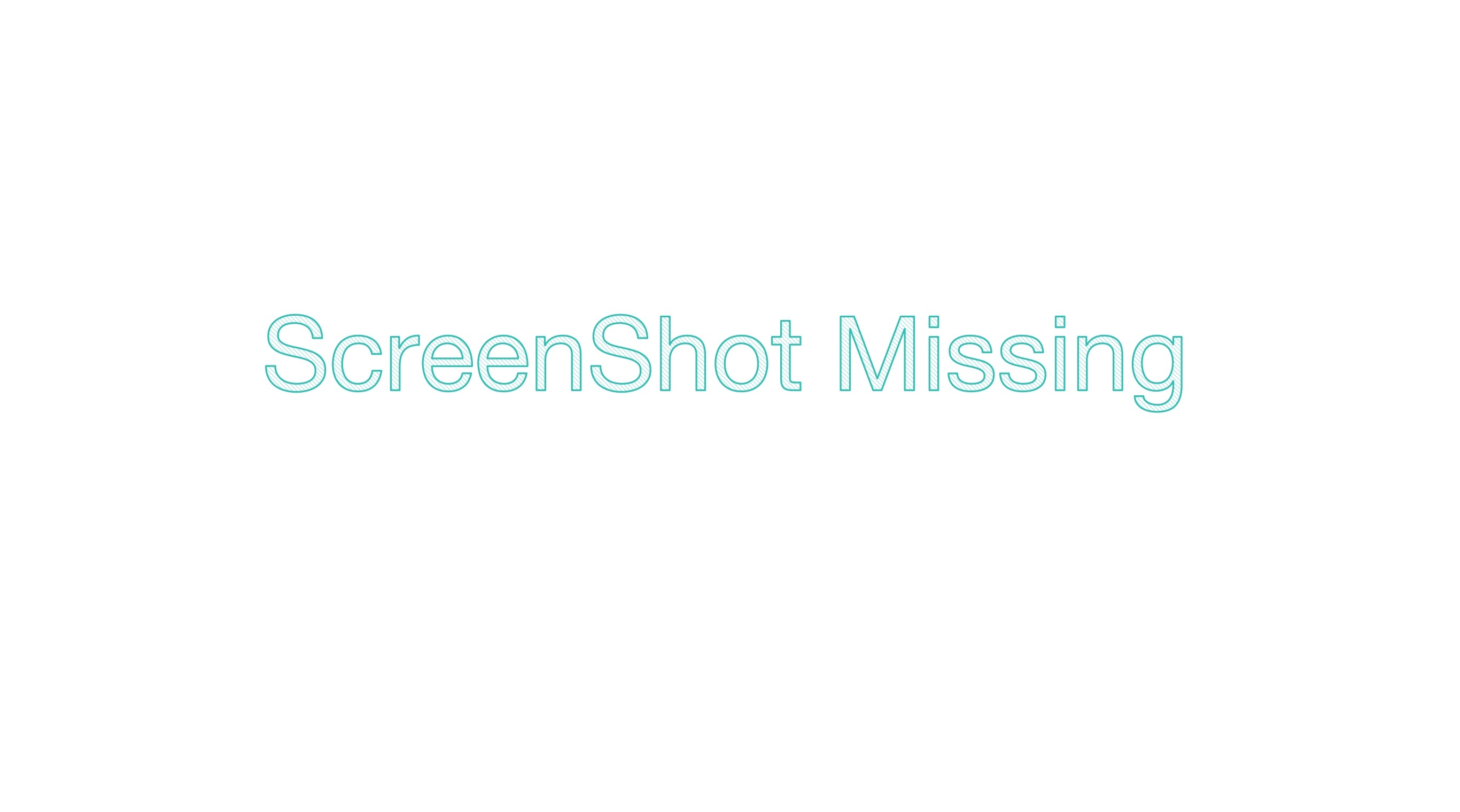
Kiln
Kiln is an AI tool designed for fine-tuning LLM models, generating synthetic data, and facilitating collaboration on datasets. It offers intuitive desktop apps, zero-code fine-tuning for various models, interactive visual tools for data generation, Git-based version control for datasets, and the ability to generate various prompts from data. Kiln supports a wide range of models and providers, provides an open-source library and API, prioritizes privacy, and allows structured data tasks in JSON format. The tool is free to use and focuses on rapid AI prototyping and dataset collaboration.
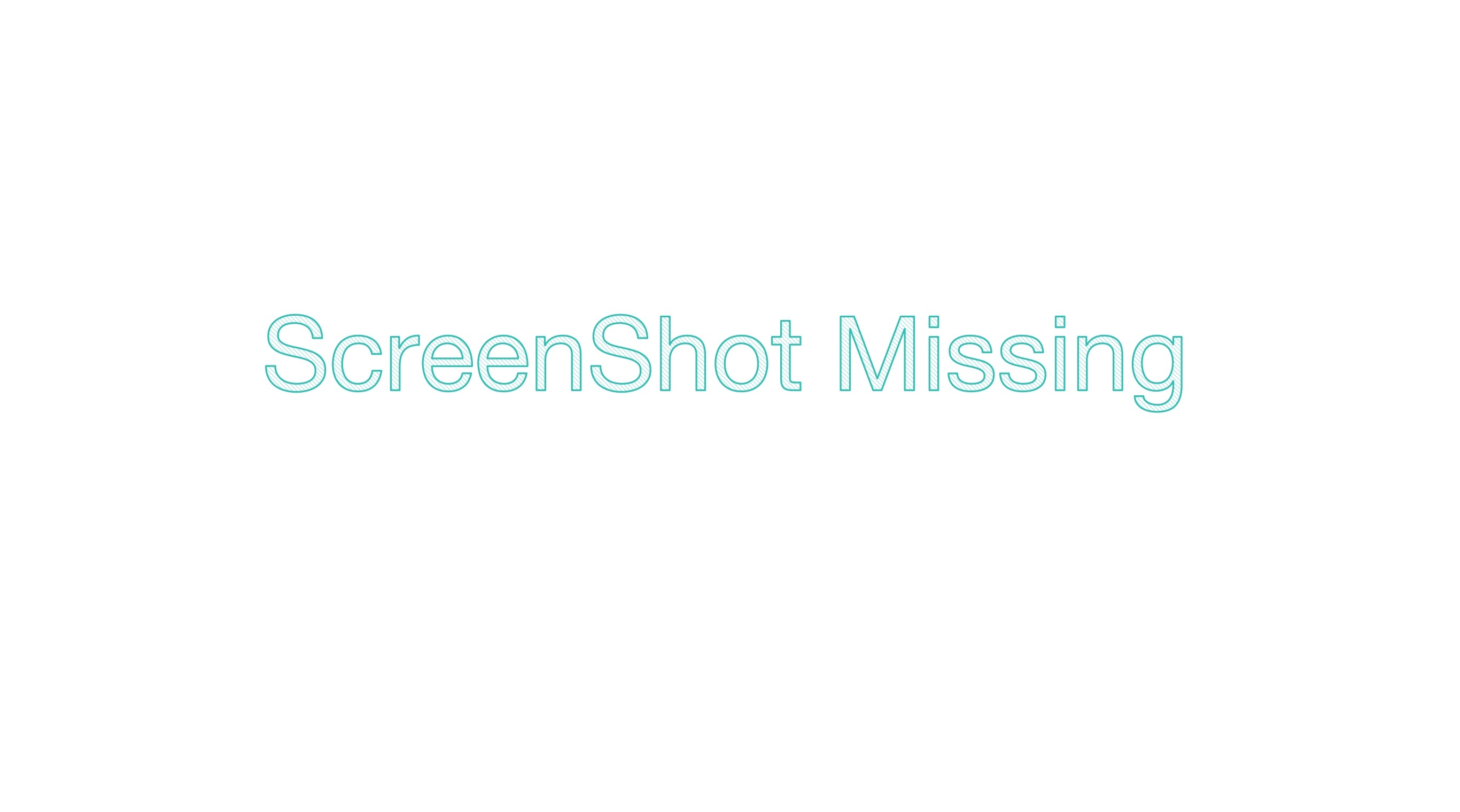
Cogitotech
Cogitotech is an AI tool that specializes in data annotation and labeling expertise. The platform offers a comprehensive suite of services tailored to meet training data needs for computer vision models and AI applications. With a decade-long industry exposure, Cogitotech provides high-quality training data for industries like healthcare, financial services, security, and more. The platform helps minimize biases in AI algorithms and ensures accurate and reliable training data solutions for deploying AI in real-life systems.
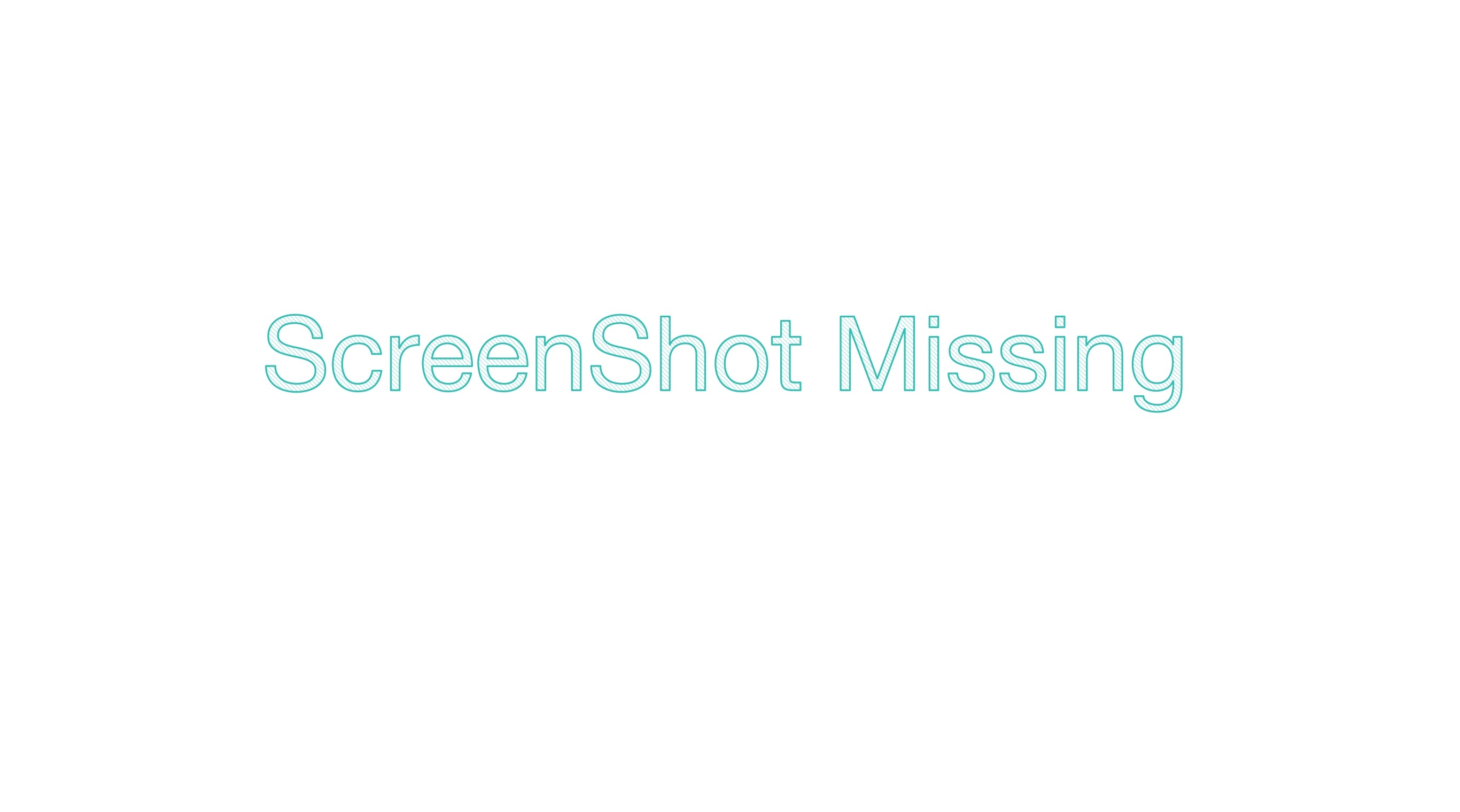
Datature
Datature is an all-in-one platform for building and deploying computer vision models. It provides tools for data management, annotation, training, and deployment, making it easy to develop and implement computer vision solutions. Datature is used by a variety of industries, including healthcare, retail, manufacturing, and agriculture.
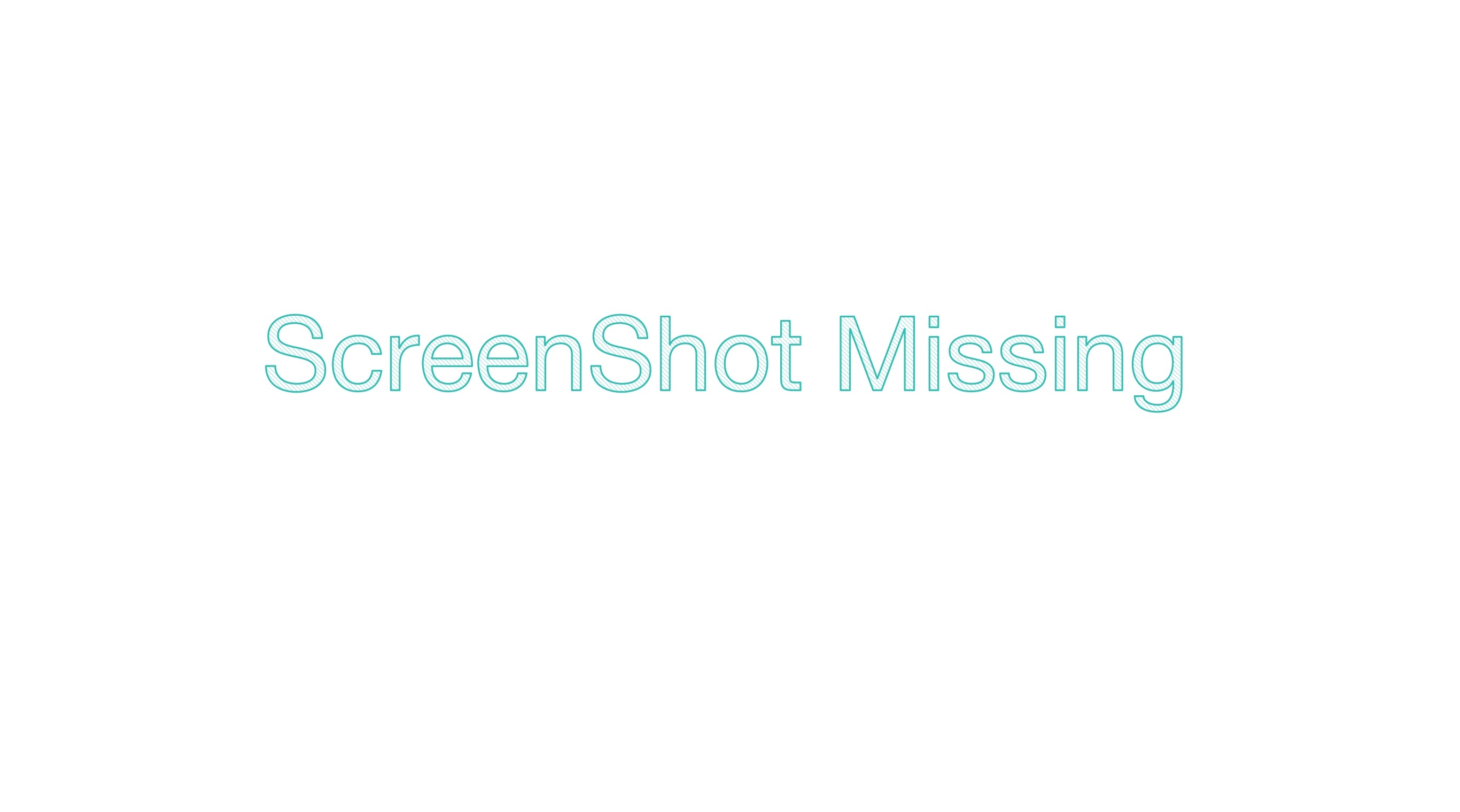
Education Data Center
The Education Data Center (EDC) Version 2.0 is a platform dedicated to providing clear and timely access to education data for researchers and education stakeholders. It offers a State Assessment Data Repository, a leading database of state assessment data in the United States. Users can download data files, utilize a custom-made AI tool to query the data, and access information about the EDC. The platform aims to support evidence-based decision-making to enhance the educational support for the nation's students.
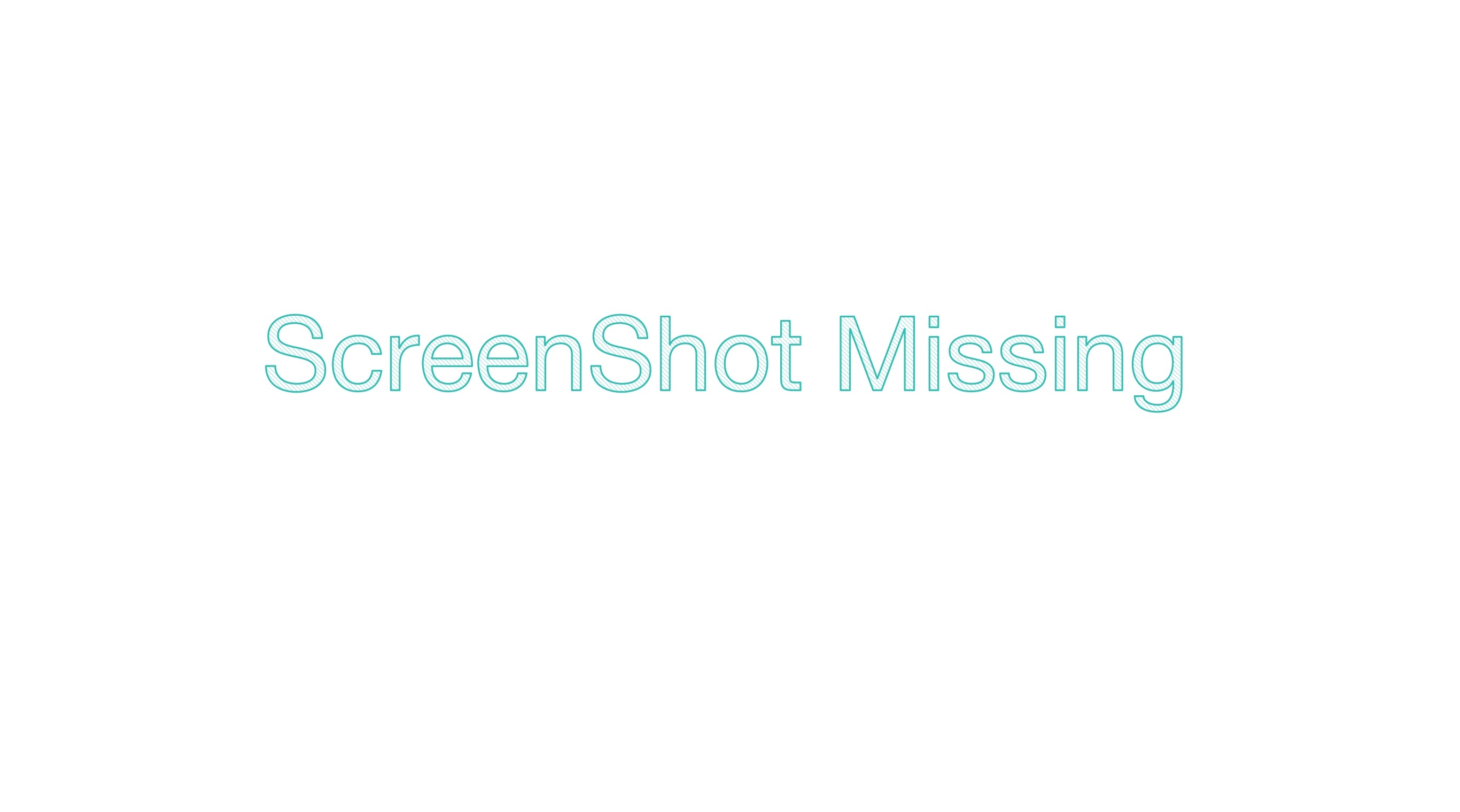
Clearbit
Clearbit is a B2B marketing intelligence platform that provides data enrichment, scoring, routing, and buying intent signals. It is powered by artificial intelligence and is the first HubSpot Native Data Provider. Clearbit's data foundation is built on public data from the web, proprietary data, and the power of LLMs to convert unstructured information into precise and standardized data sets. This data can be used to enrich leads, contacts, and accounts, and to identify hidden buying intent. Clearbit also offers a variety of features to help businesses score and route leads, and to create better converting forms.
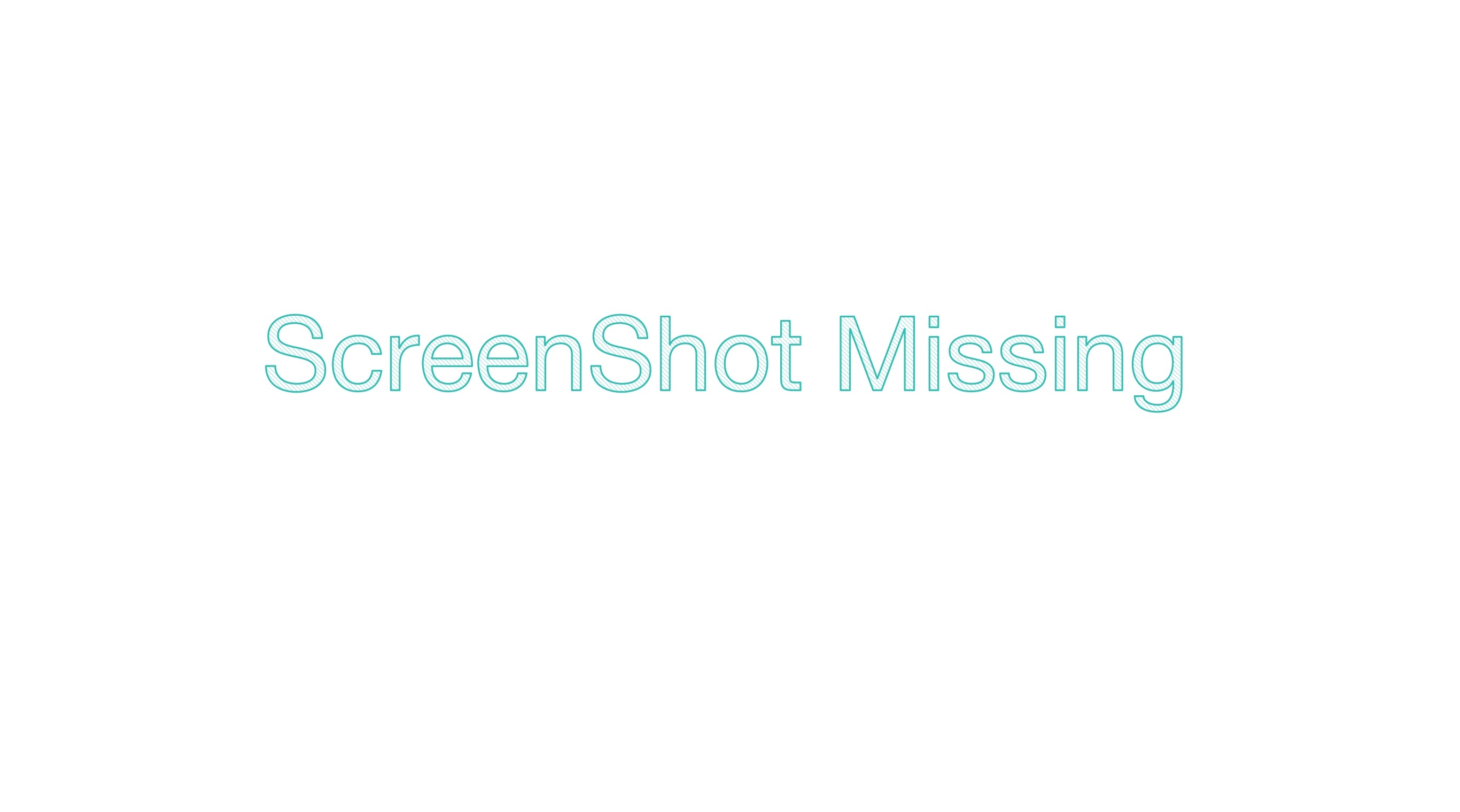
Create AI Characters and Chat with AI
This website allows users to create AI characters and chat with them. Users can customize their characters' appearance, personality, and interests. They can also choose from a variety of topics to chat about. The website uses artificial intelligence to generate the characters' responses, which are designed to be realistic and engaging.
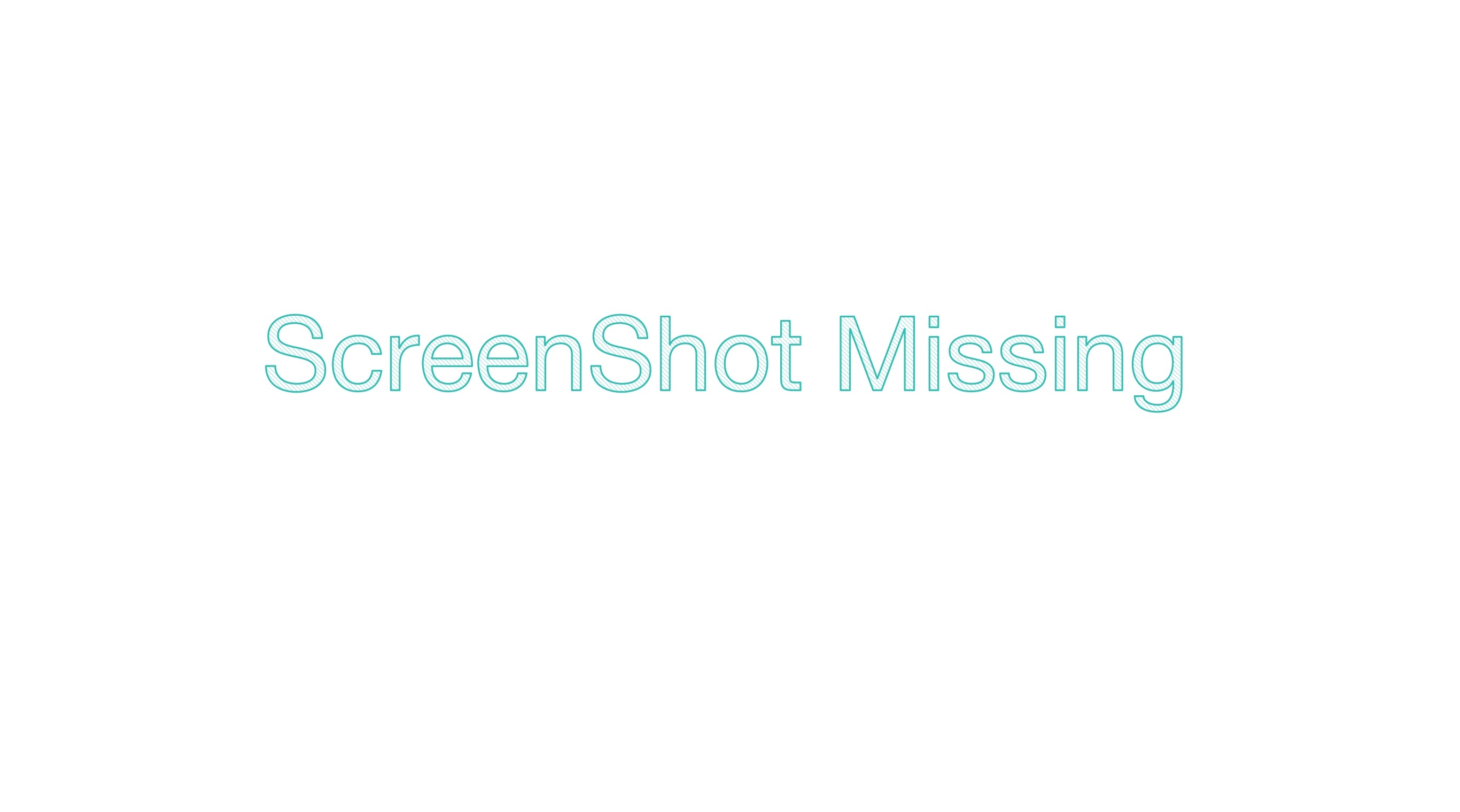
LINQ Me Up
LINQ Me Up is an AI-powered tool designed to boost .Net productivity by generating and converting LINQ code queries. It allows users to effortlessly convert SQL queries to LINQ code, transform LINQ code into SQL queries, and generate tailored LINQ queries for various datasets. The tool supports C# and Visual Basic code, Method and Query syntax, and provides AI-powered analysis for optimized results. LINQ Me Up aims to supercharge productivity by simplifying query conversion processes and saving valuable time and costs for developers.
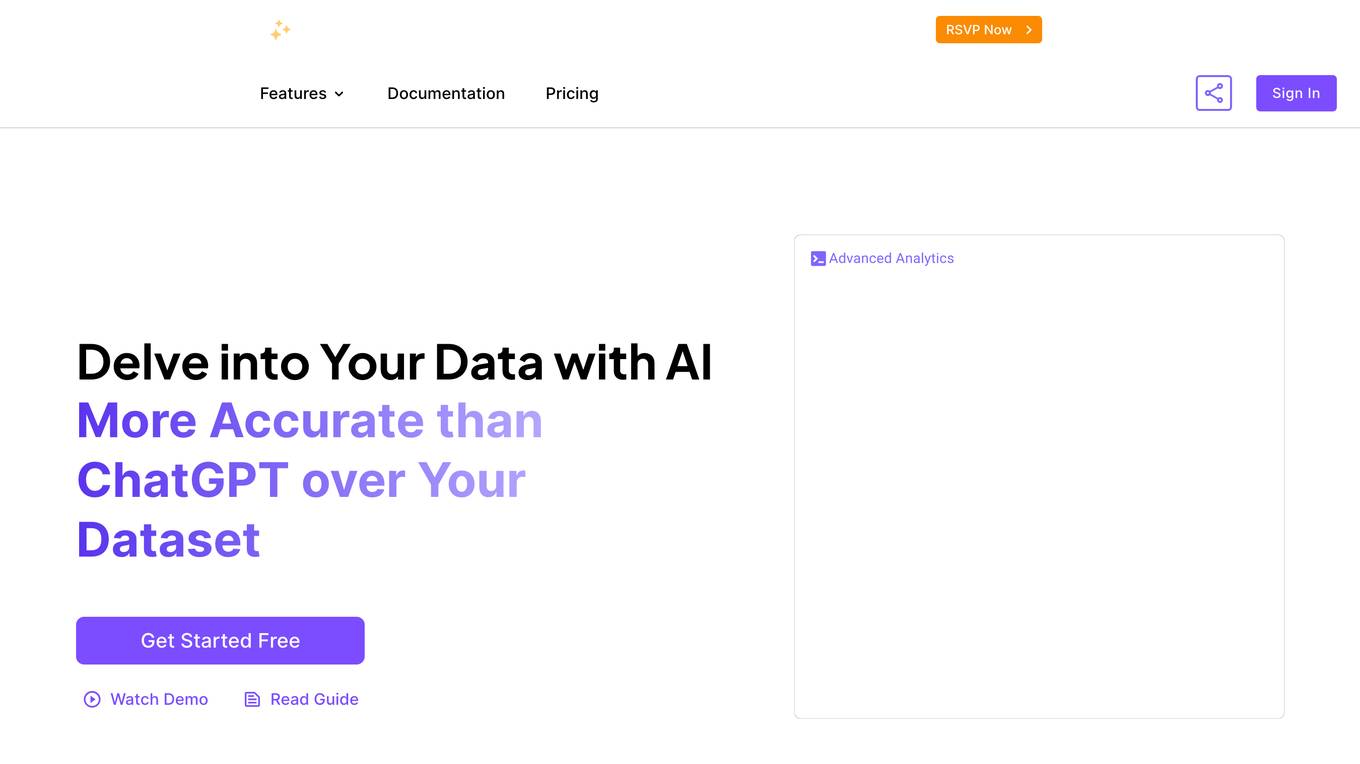
Powerdrill
Powerdrill is a platform that provides swift insights from knowledge and data. It offers a range of features such as discovering datasets, creating BI dashboards, accessing various apps, resources, blogs, documentation, and changelogs. The platform is available in English and fosters a community through its affiliate program. Users can sign up for a basic plan to start utilizing the tools and services offered by Powerdrill.
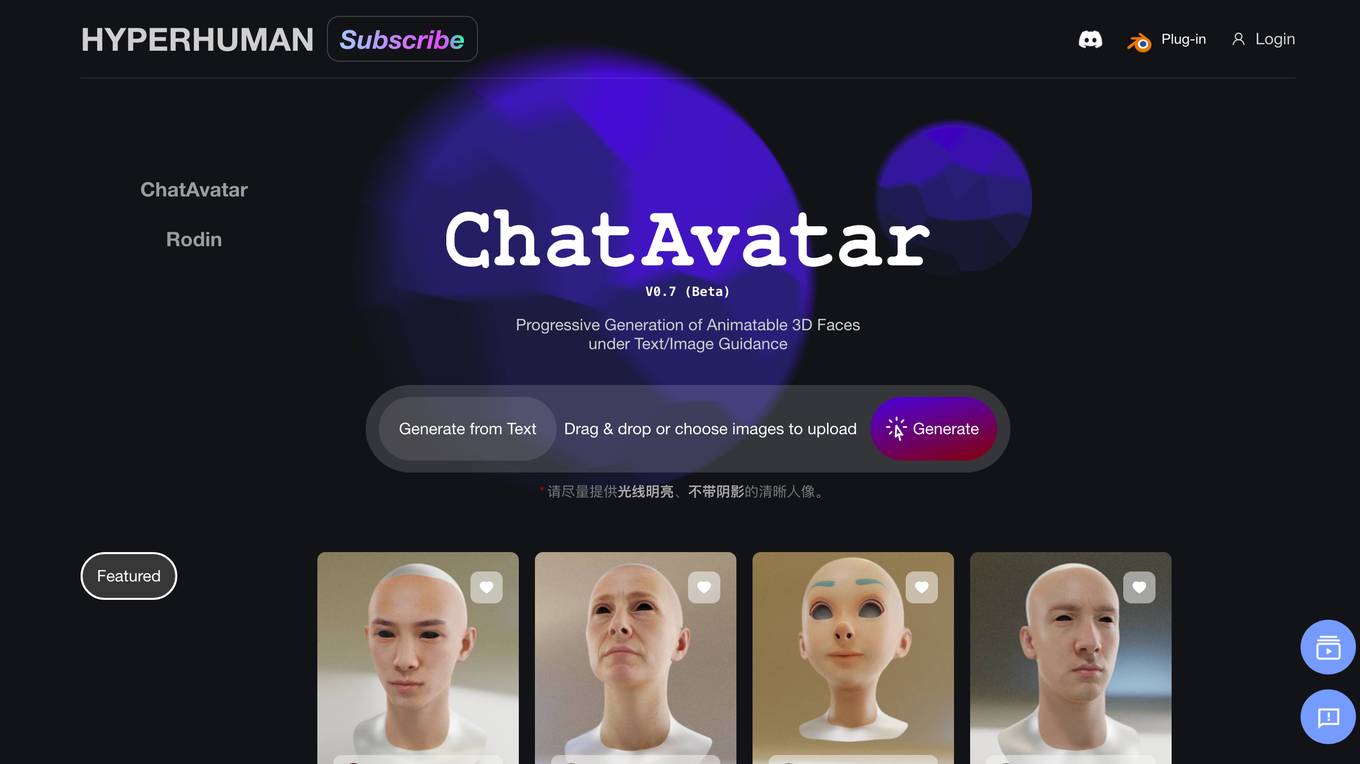
Rodin
Rodin is a free AI 3D model generator that allows users to create high-quality 3D assets from images. Users can subscribe to different plans based on their needs, such as Creator, Business, Education, and Enterprise. The tool offers features like generating 3D models from single or multi-view images, rotating models for better results, uploading photos from any angle, and unlocking multi-image fusion for subscribers. Additionally, users can share stories to earn credits and access various tools like Texture Generator, Mesh Editor, Model Viewer, and Format Convertor. Rodin also provides tutorials and tips for users to enhance their 3D modeling experience.
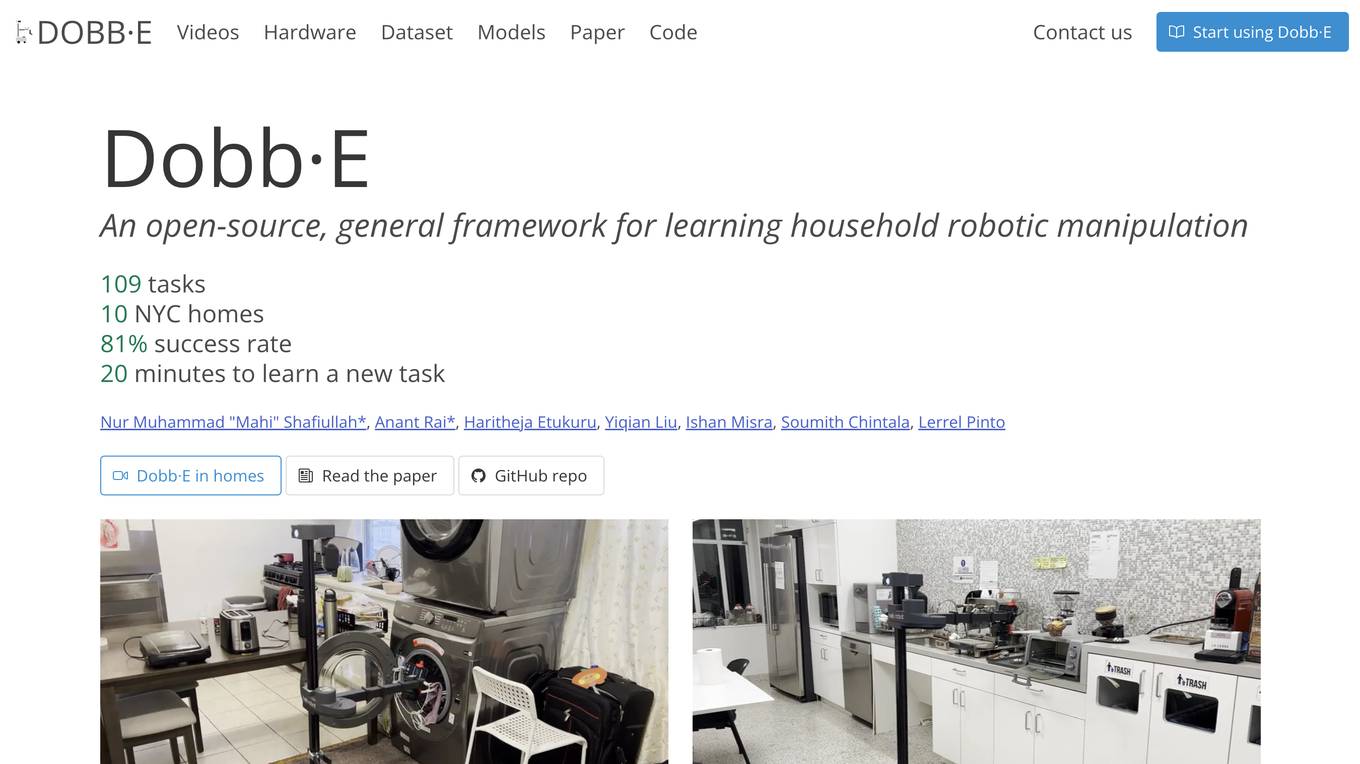
Dobb·E
Dobb·E is an open-source, general framework for learning household robotic manipulation. It aims to create a 'generalist machine' for homes, a domestic assistant that can adapt and learn various tasks cost-effectively. Dobb·E can learn a new task with just five minutes of demonstration, achieving an 81% success rate in 10 NYC homes. The system is designed to accelerate research on home robots and eventually enable robot butlers in every home.
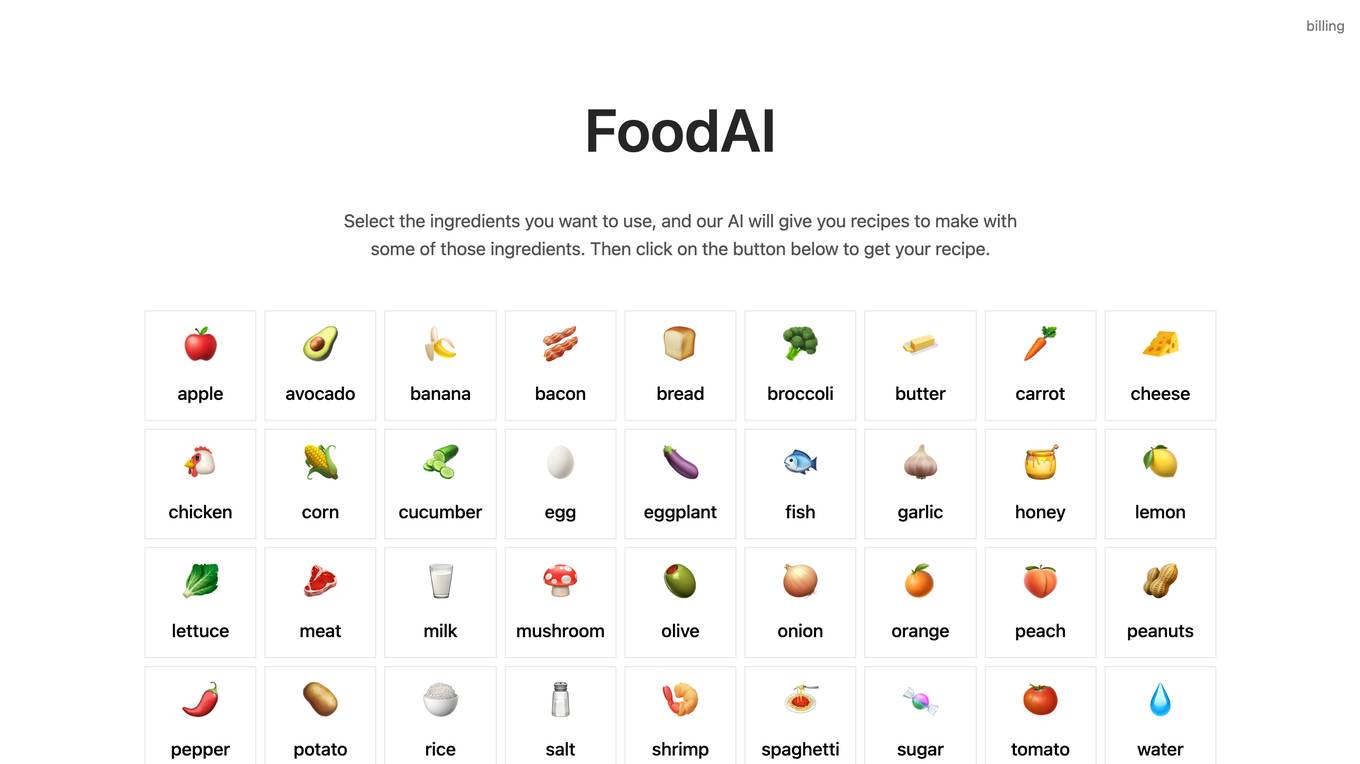
foodai.app
foodai.app is a website domain that may be for sale. It does not currently offer any AI tool or application. The website seems to be focused on the sale of the domain name and provides contact information for inquiries. It is a simple webpage with limited content and no interactive features.
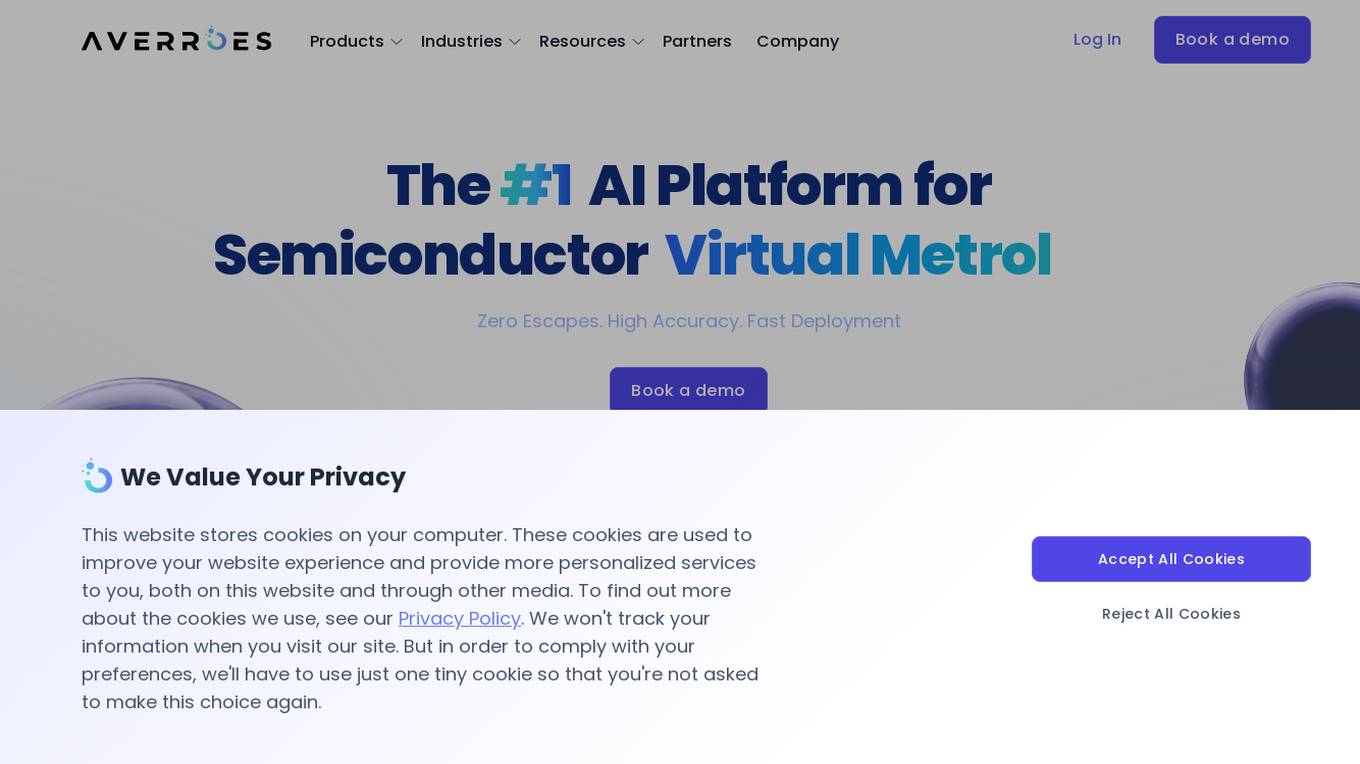
Averroes
Averroes is the #1 AI Automated Visual Inspection Software designed for various industries such as Oil and Gas, Food and Beverage, Pharma, Semiconductor, and Electronics. It offers an end-to-end AI visual inspection platform that allows users to effortlessly train and deploy custom AI models for defect classification, object detection, and segmentation. Averroes provides advanced solutions for quality assurance, including automated defect classification, submicron defect detection, defect segmentation, defect review, and defect monitoring. The platform ensures labeling consistency, offers flexible deployment options, and has shown remarkable improvements in defect detection and productivity for semiconductor OEMs.
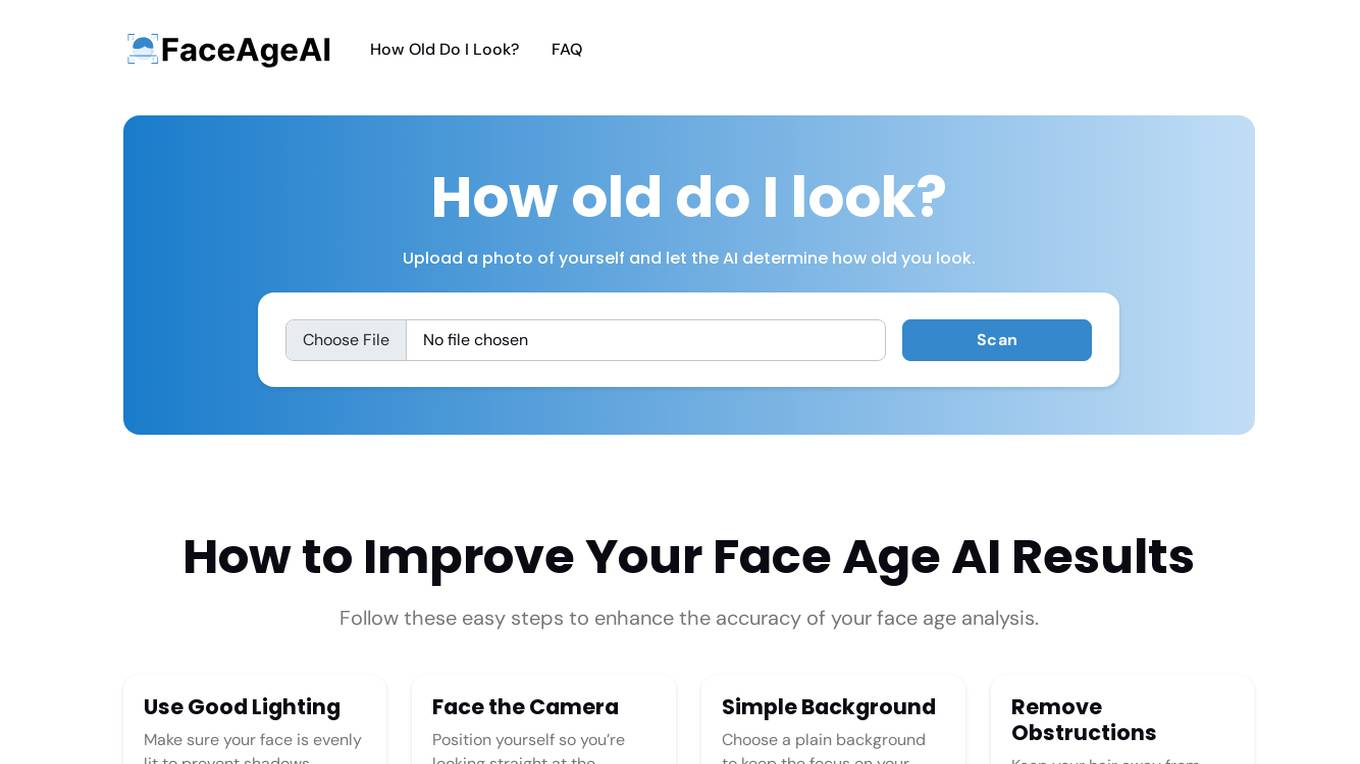
How Old Do I Look?
This AI-powered age detection tool analyzes your photo to estimate how old you look. It utilizes advanced artificial intelligence technology to assess facial characteristics such as wrinkles, skin texture, and facial features, comparing them against a vast dataset to provide an approximation of your age. The tool is free to use and ensures privacy by automatically deleting uploaded photos after analysis.
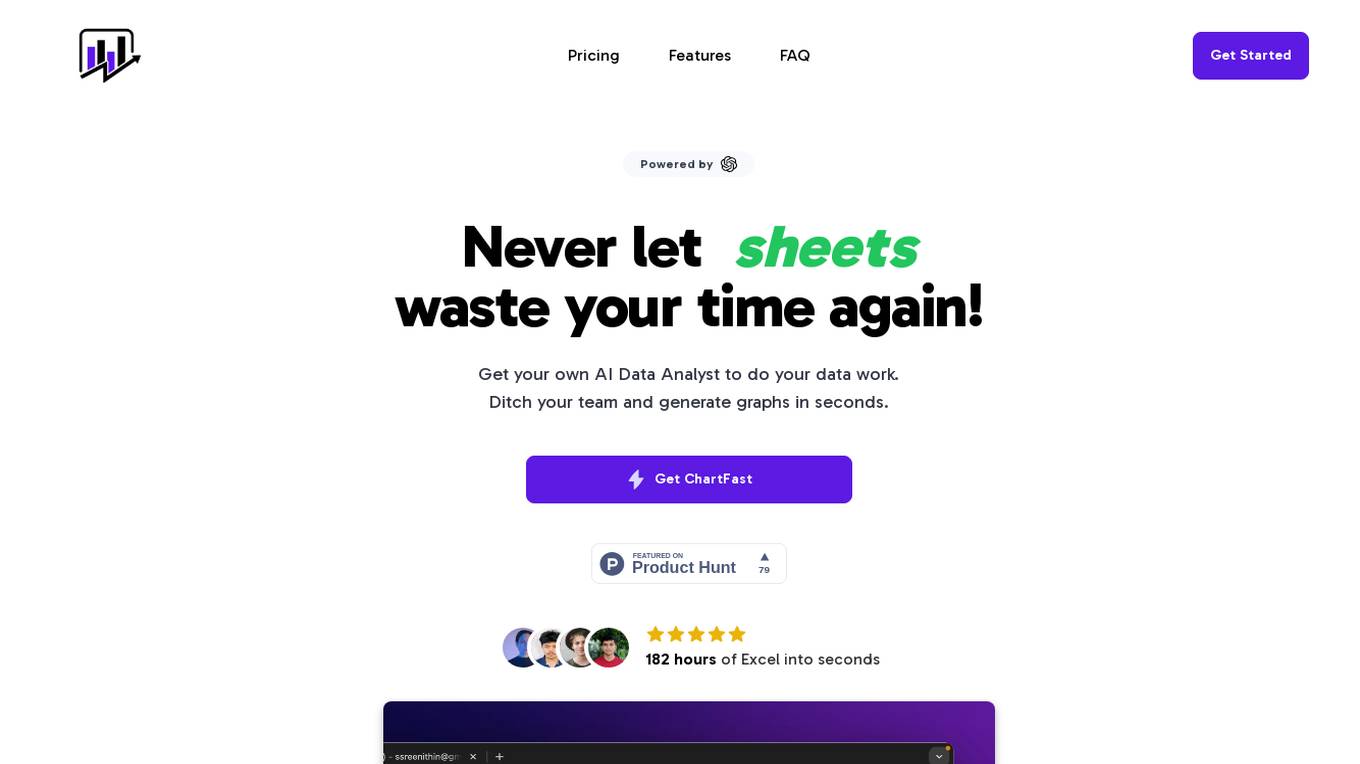
ChartFast
ChartFast is an AI Data Analyzer tool that automates data visualization and analysis tasks, powered by GPT-4 technology. It allows users to generate precise and sleek graphs in seconds, process vast amounts of data, and provide interactive data queries and quick exports. With features like specialized internal libraries for complex graph generation, customizable visualization code, and instant data export, ChartFast aims to streamline data work and enhance data analysis efficiency.
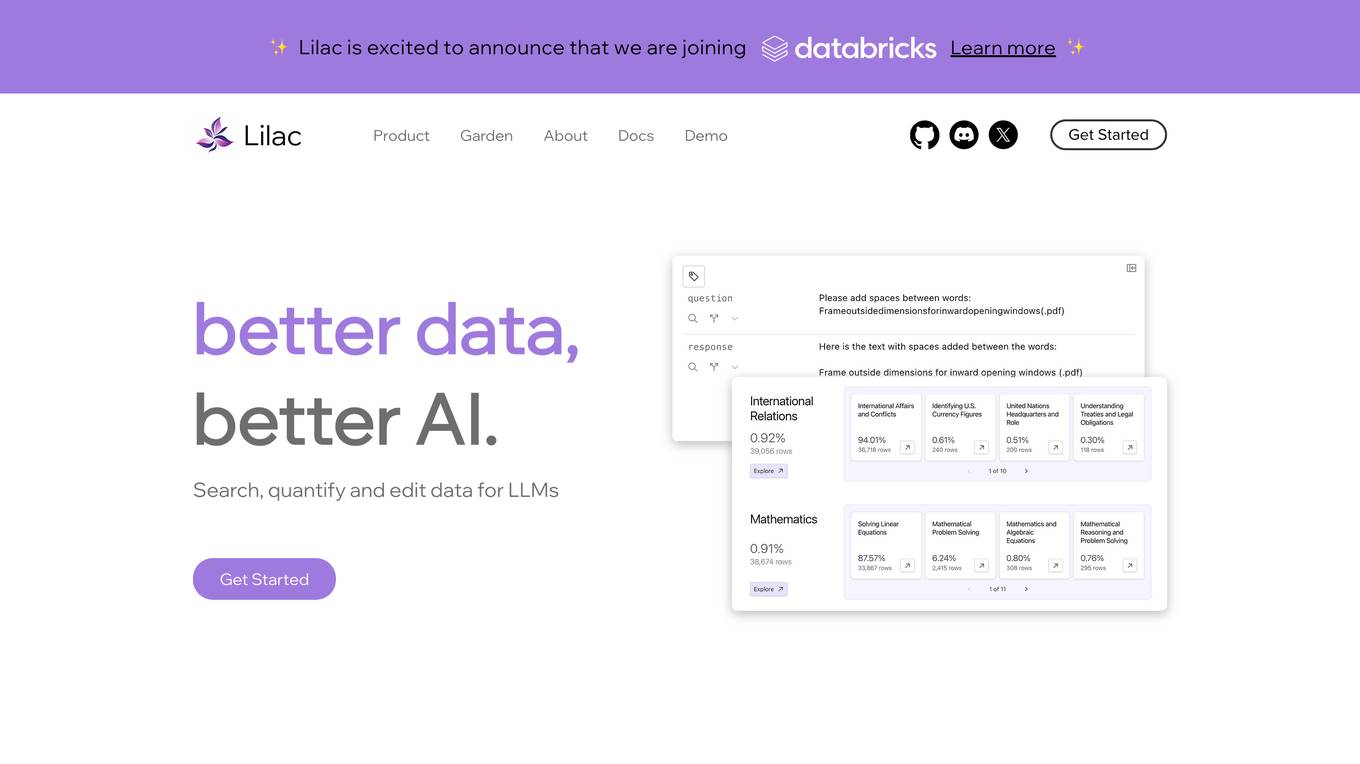
Lilac
Lilac is an AI tool designed to enhance data quality and exploration for AI applications. It offers features such as data search, quantification, editing, clustering, semantic search, field comparison, and fuzzy-concept search. Lilac enables users to accelerate dataset computations and transformations, making it a valuable asset for data scientists and AI practitioners. The tool is trusted by Alignment Lab and is recommended for working with LLM datasets.
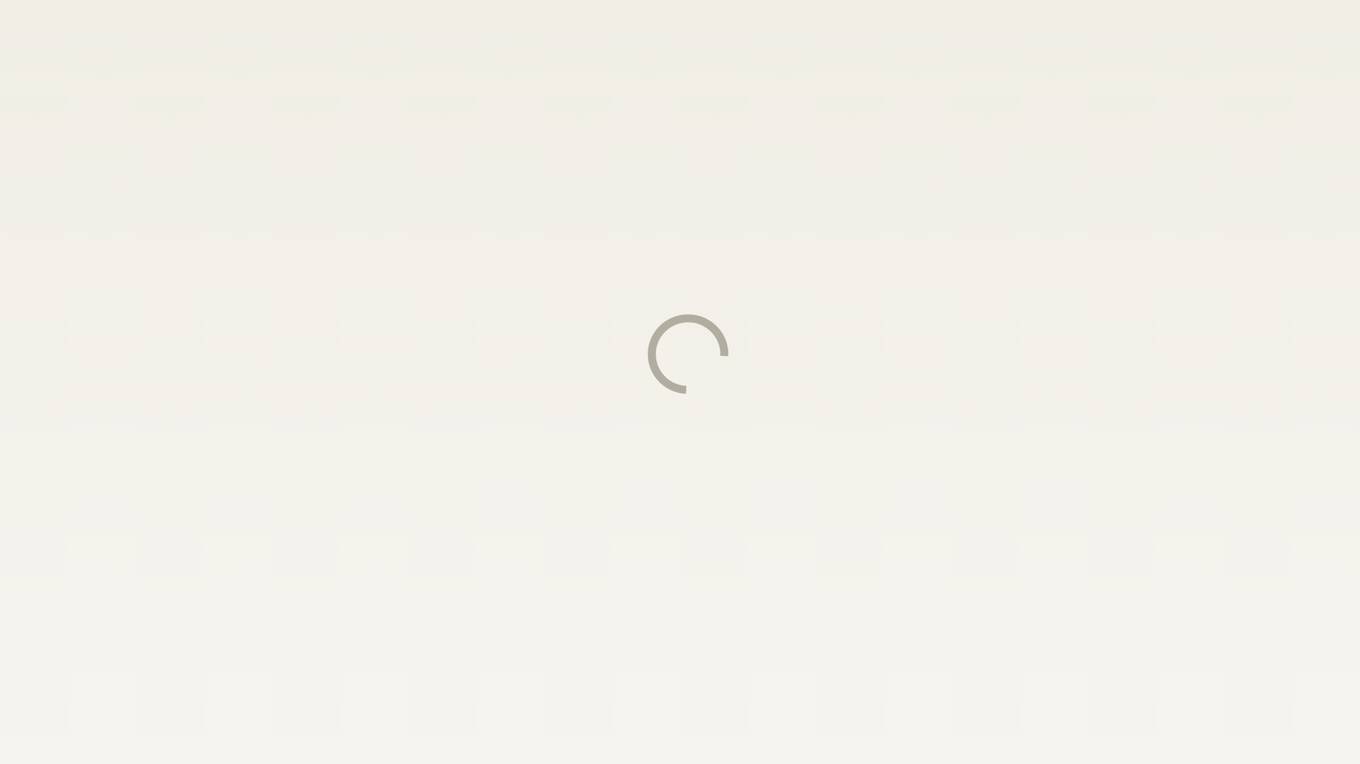
Claude
Claude is a large multi-modal model, trained by Google. It is similar to GPT-3, but it is trained on a larger dataset and with more advanced techniques. Claude is capable of generating human-like text, translating languages, answering questions, and writing different kinds of creative content.
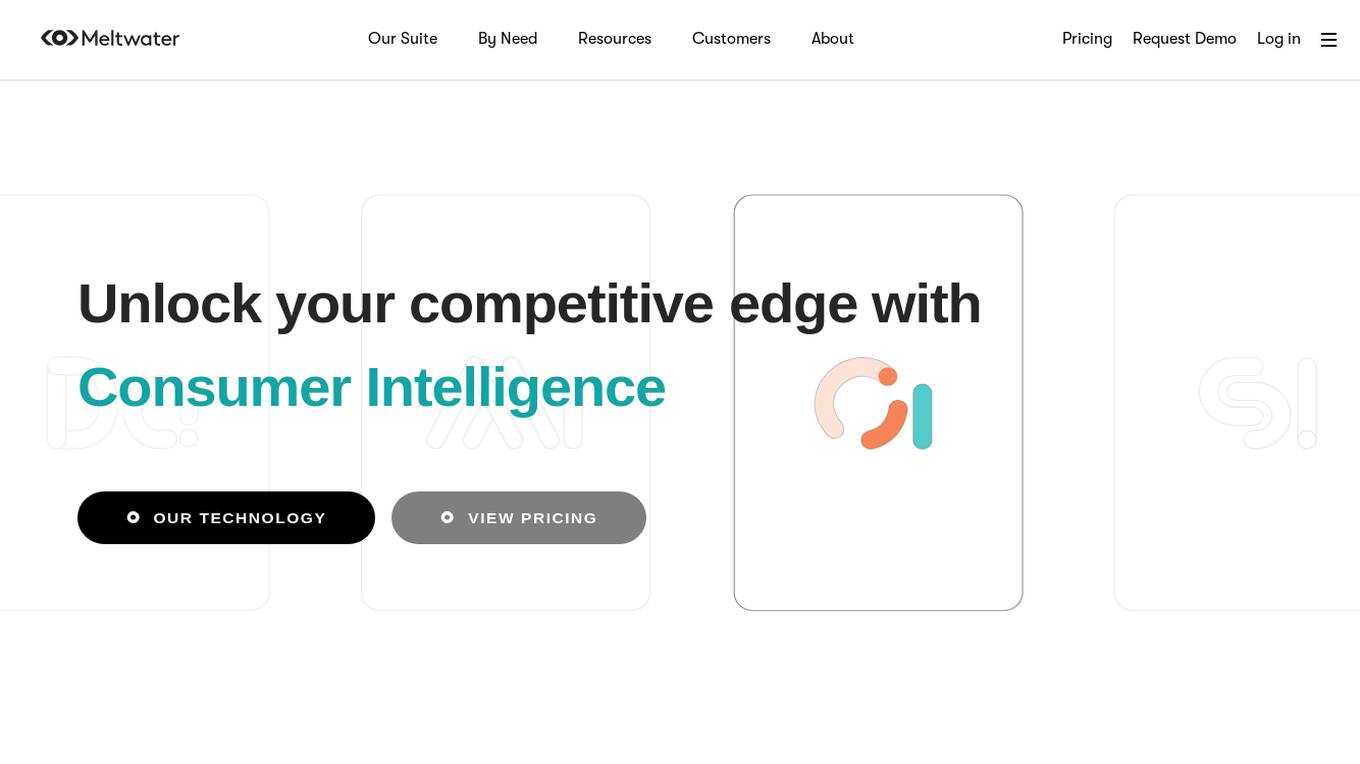
Meltwater
Meltwater is an AI-powered media intelligence platform that helps businesses gain competitive insights by analyzing media, social, and consumer trends. With a robust dataset and powerful AI capabilities, Meltwater empowers teams to uncover actionable insights for PR, marketing, and sales strategies. The platform offers tools for media monitoring, social listening, influencer marketing, and more, enabling users to make data-driven decisions and measure the impact of their efforts.
20 - Open Source AI Tools
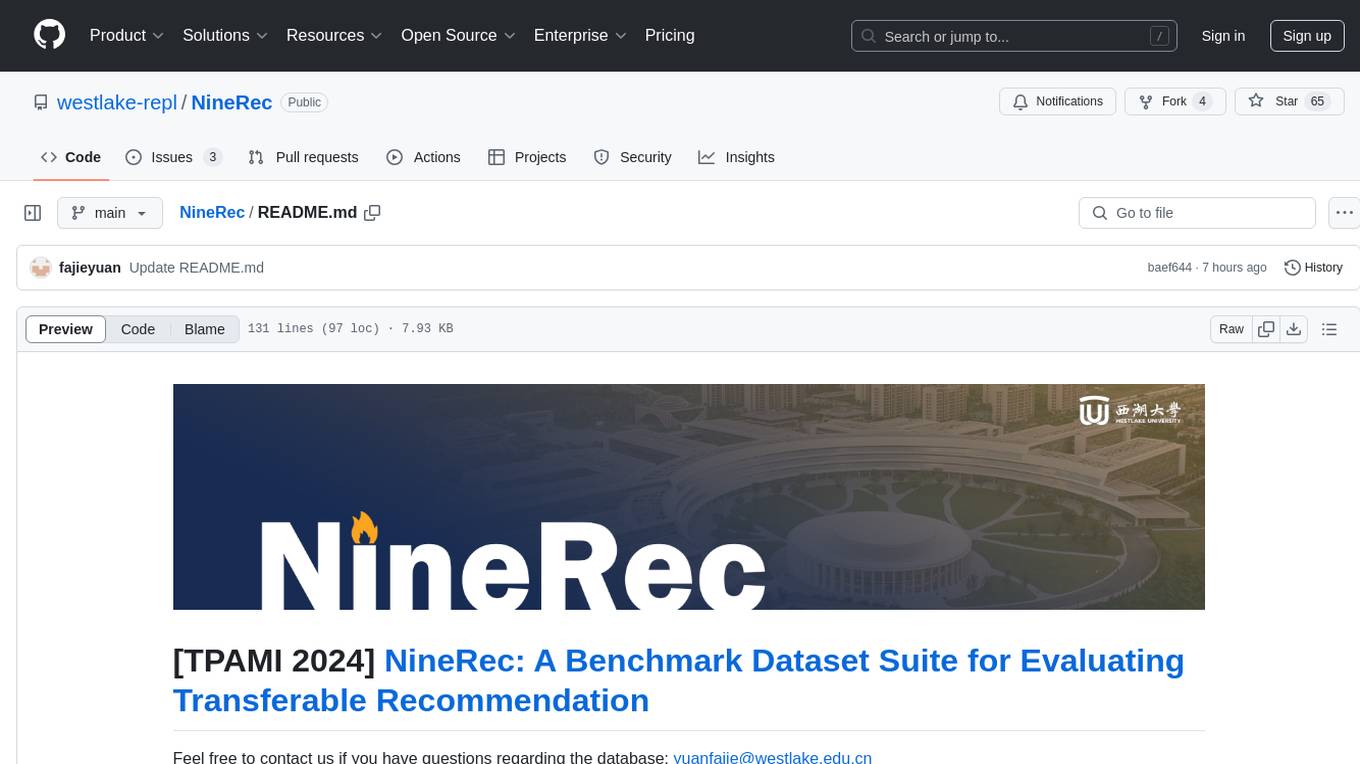
NineRec
NineRec is a benchmark dataset suite for evaluating transferable recommendation models. It provides datasets for pre-training and transfer learning in recommender systems, focusing on multimodal and foundation model tasks. The dataset includes user-item interactions, item texts in multiple languages, item URLs, and raw images. Researchers can use NineRec to develop more effective and efficient methods for pre-training recommendation models beyond end-to-end training. The dataset is accompanied by code for dataset preparation, training, and testing in PyTorch environment.
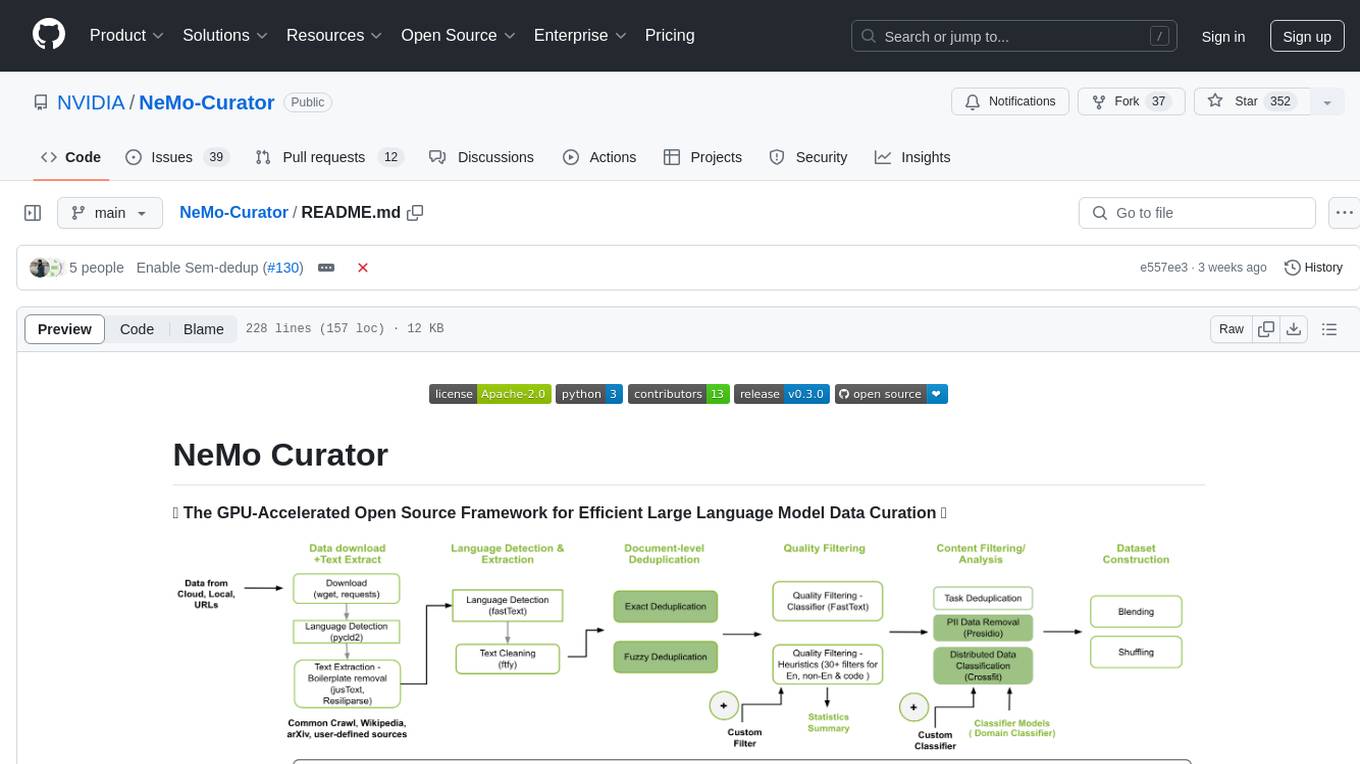
NeMo-Curator
NeMo Curator is a GPU-accelerated open-source framework designed for efficient large language model data curation. It provides scalable dataset preparation for tasks like foundation model pretraining, domain-adaptive pretraining, supervised fine-tuning, and parameter-efficient fine-tuning. The library leverages GPUs with Dask and RAPIDS to accelerate data curation, offering customizable and modular interfaces for pipeline expansion and model convergence. Key features include data download, text extraction, quality filtering, deduplication, downstream-task decontamination, distributed data classification, and PII redaction. NeMo Curator is suitable for curating high-quality datasets for large language model training.
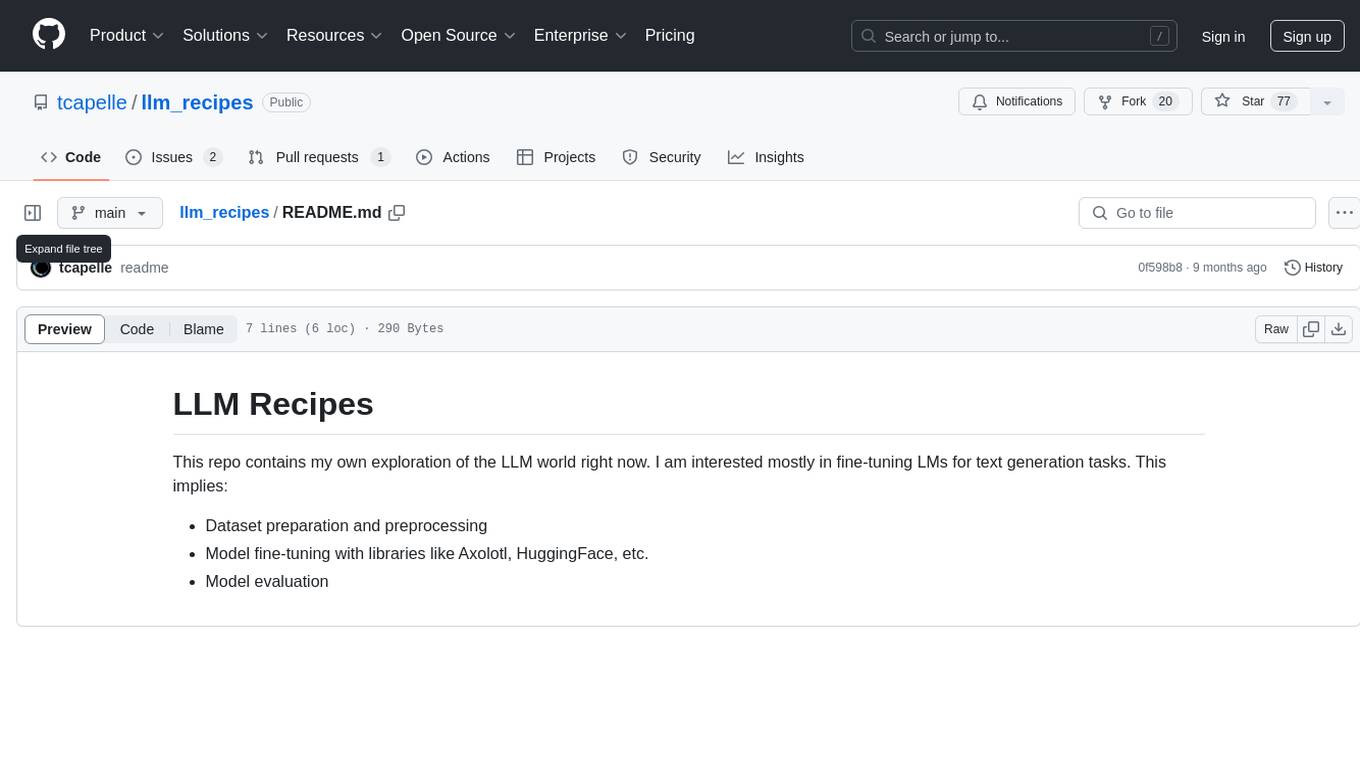
llm_recipes
This repository showcases the author's experiments with Large Language Models (LLMs) for text generation tasks. It includes dataset preparation, preprocessing, model fine-tuning using libraries such as Axolotl and HuggingFace, and model evaluation.
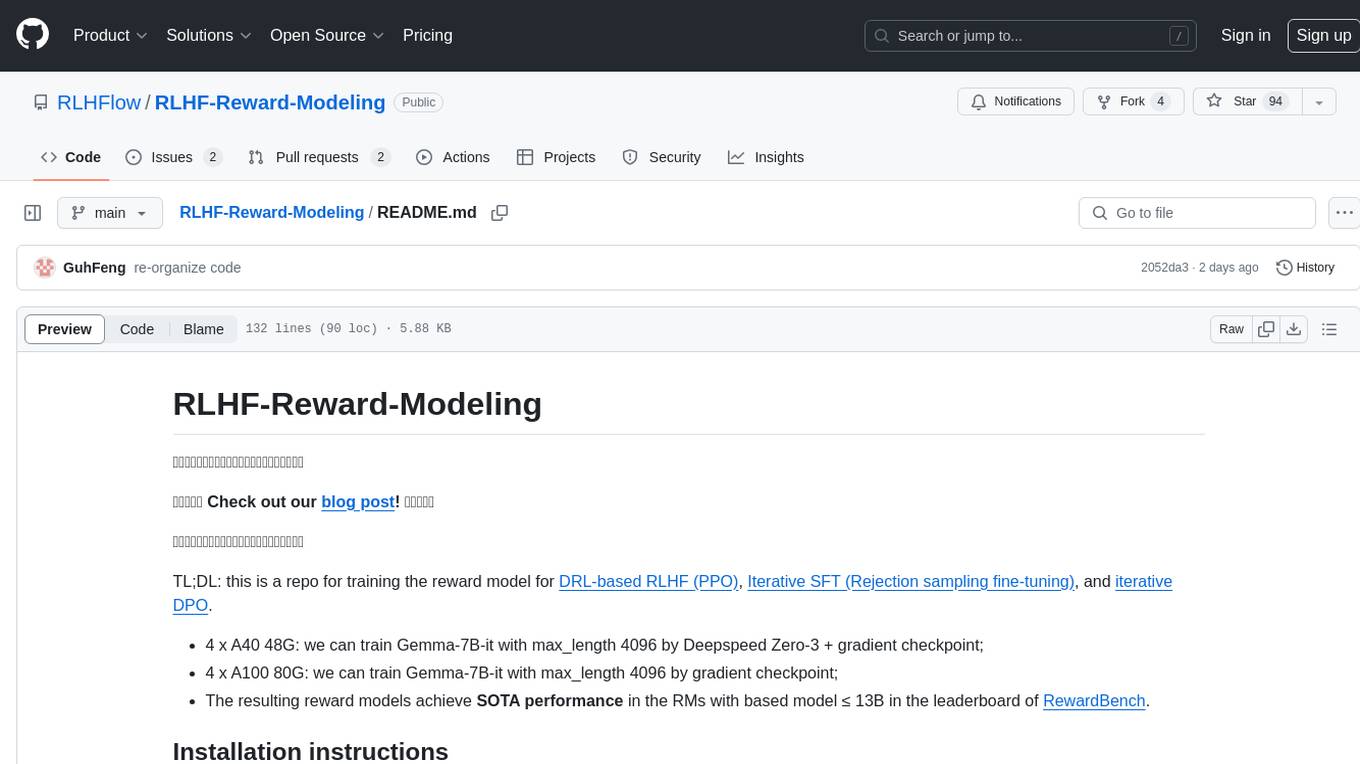
RLHF-Reward-Modeling
This repository, RLHF-Reward-Modeling, is dedicated to training reward models for DRL-based RLHF (PPO), Iterative SFT, and iterative DPO. It provides state-of-the-art performance in reward models with a base model size of up to 13B. The installation instructions involve setting up the environment and aligning the handbook. Dataset preparation requires preprocessing conversations into a standard format. The code can be run with Gemma-2b-it, and evaluation results can be obtained using provided datasets. The to-do list includes various reward models like Bradley-Terry, preference model, regression-based reward model, and multi-objective reward model. The repository is part of iterative rejection sampling fine-tuning and iterative DPO.
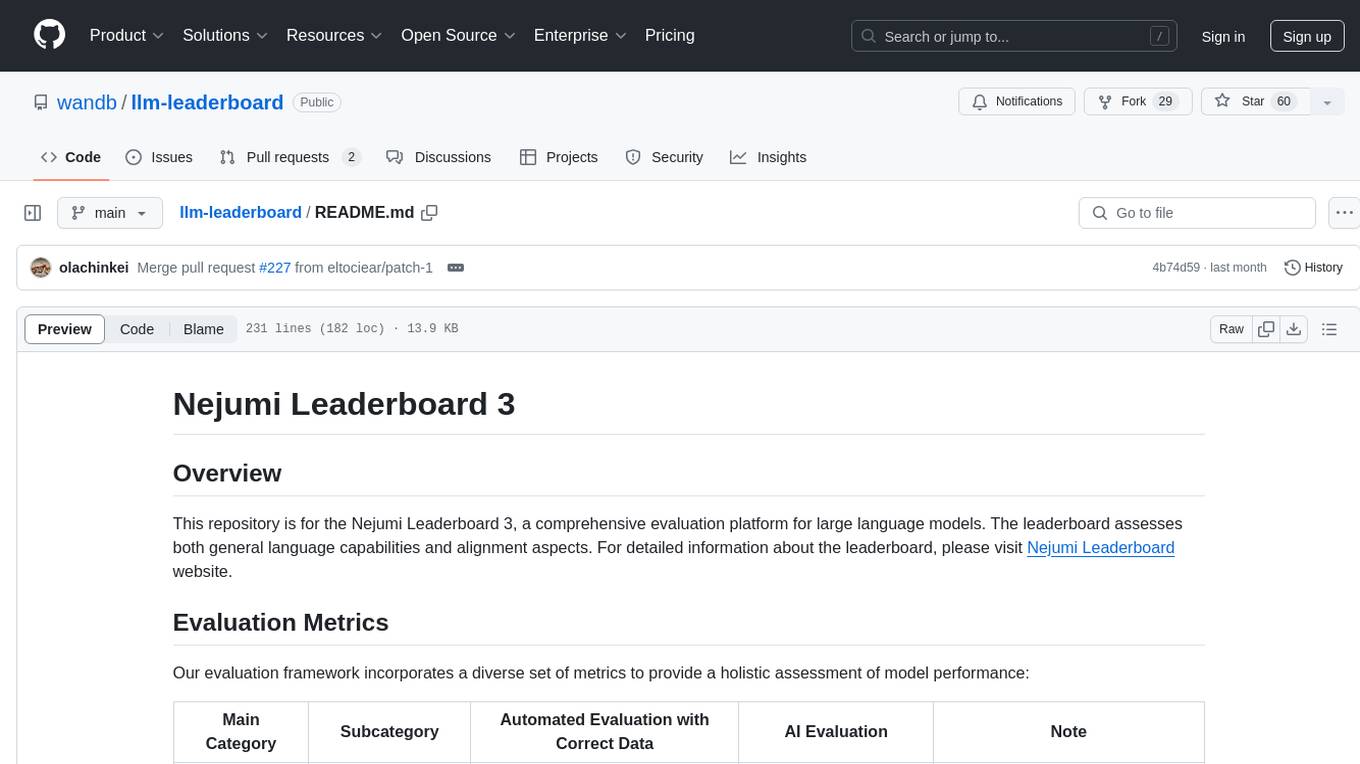
llm-leaderboard
Nejumi Leaderboard 3 is a comprehensive evaluation platform for large language models, assessing general language capabilities and alignment aspects. The evaluation framework includes metrics for language processing, translation, summarization, information extraction, reasoning, mathematical reasoning, entity extraction, knowledge/question answering, English, semantic analysis, syntactic analysis, alignment, ethics/moral, toxicity, bias, truthfulness, and robustness. The repository provides an implementation guide for environment setup, dataset preparation, configuration, model configurations, and chat template creation. Users can run evaluation processes using specified configuration files and log results to the Weights & Biases project.
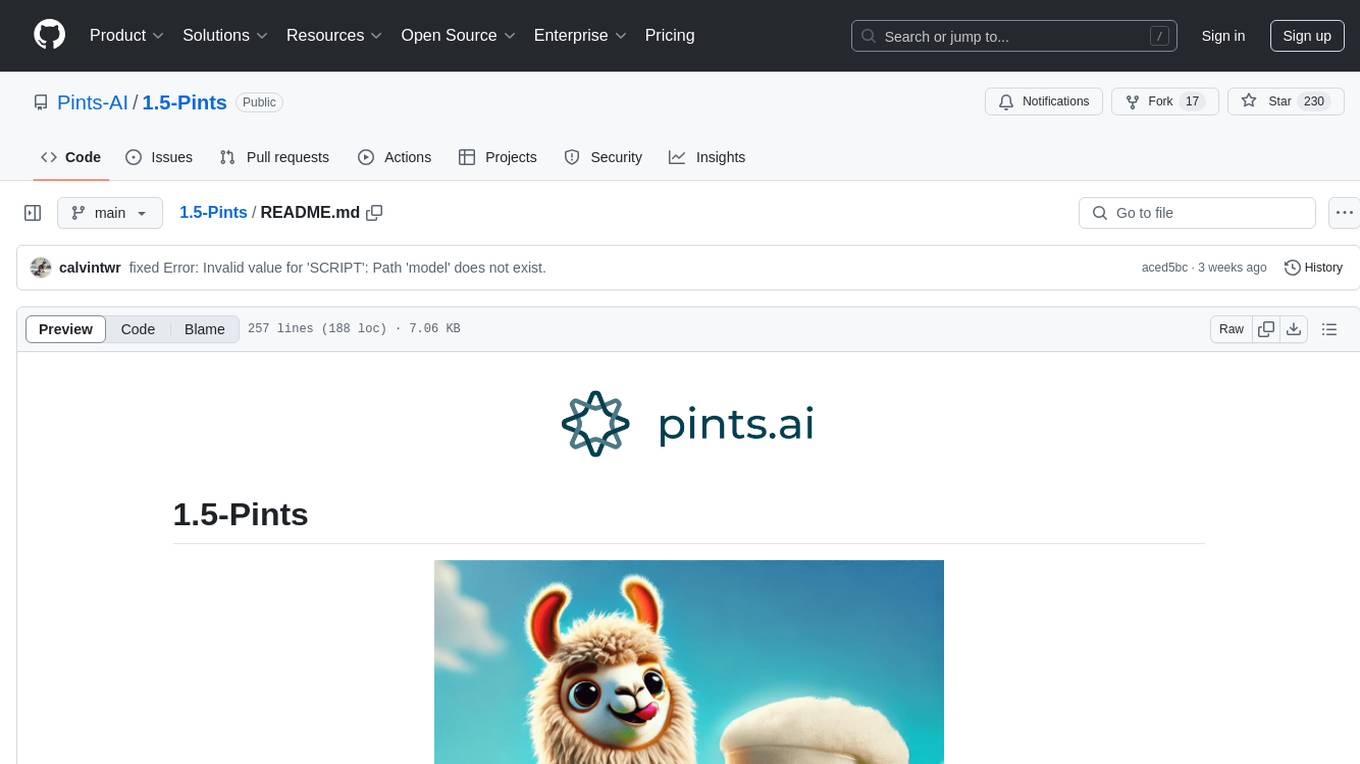
1.5-Pints
1.5-Pints is a repository that provides a recipe to pre-train models in 9 days, aiming to create AI assistants comparable to Apple OpenELM and Microsoft Phi. It includes model architecture, training scripts, and utilities for 1.5-Pints and 0.12-Pint developed by Pints.AI. The initiative encourages replication, experimentation, and open-source development of Pint by sharing the model's codebase and architecture. The repository offers installation instructions, dataset preparation scripts, model training guidelines, and tools for model evaluation and usage. Users can also find information on finetuning models, converting lit models to HuggingFace models, and running Direct Preference Optimization (DPO) post-finetuning. Additionally, the repository includes tests to ensure code modifications do not disrupt the existing functionality.
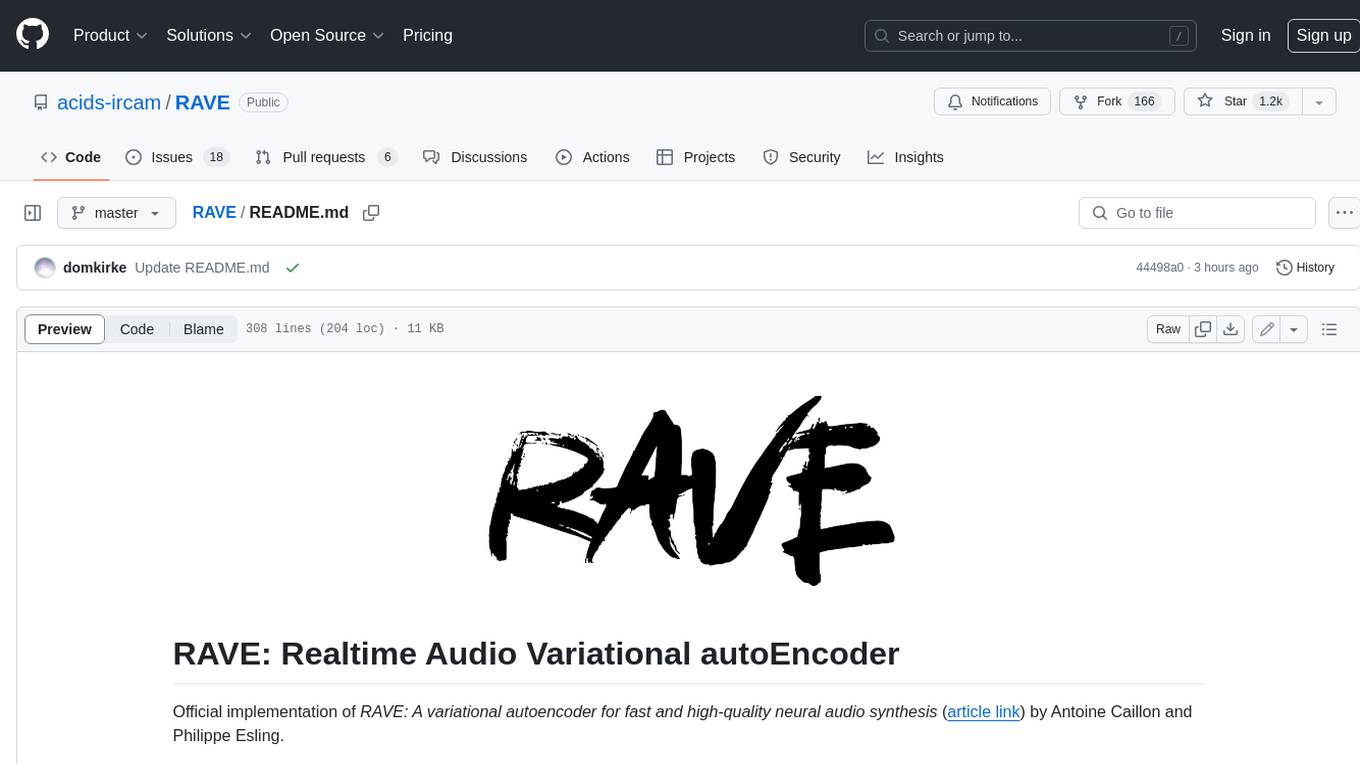
RAVE
RAVE is a variational autoencoder for fast and high-quality neural audio synthesis. It can be used to generate new audio samples from a given dataset, or to modify the style of existing audio samples. RAVE is easy to use and can be trained on a variety of audio datasets. It is also computationally efficient, making it suitable for real-time applications.
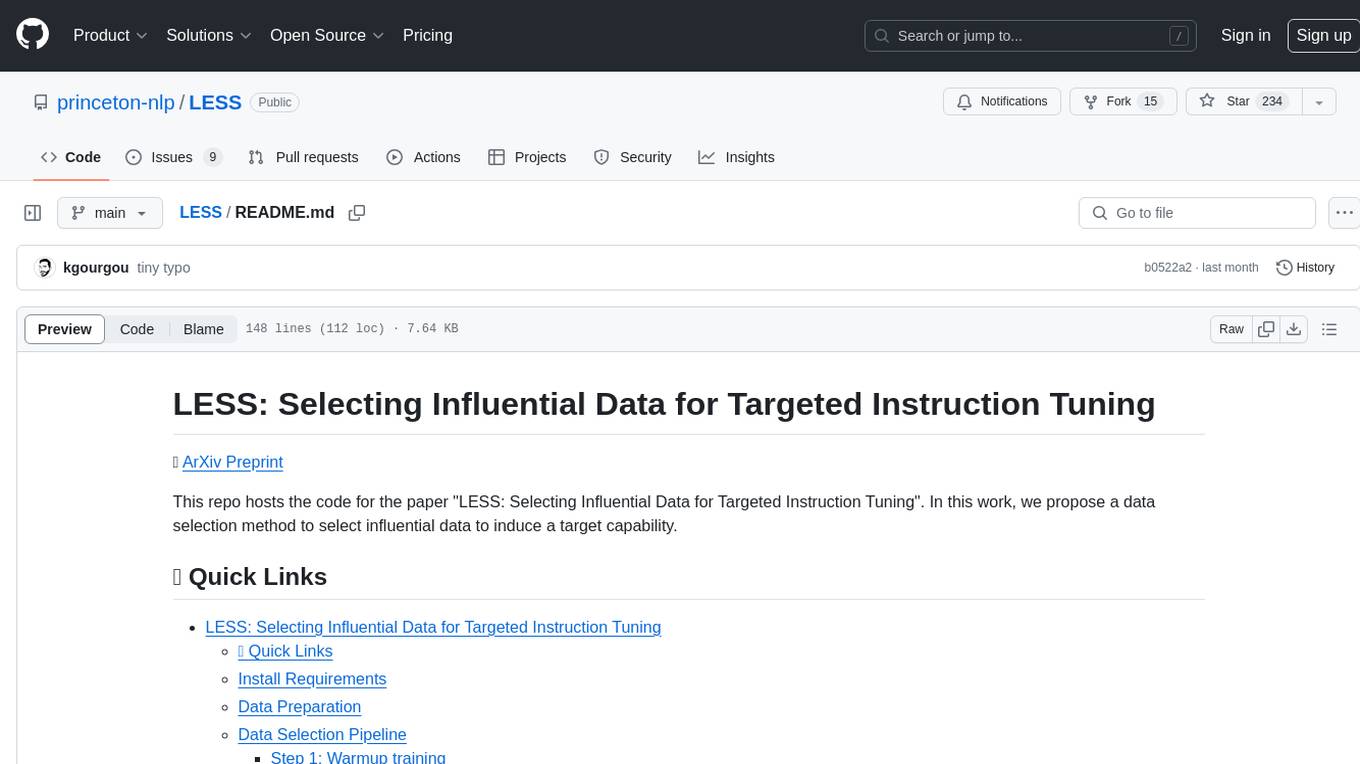
LESS
This repository contains the code for the paper 'LESS: Selecting Influential Data for Targeted Instruction Tuning'. The work proposes a data selection method to choose influential data for inducing a target capability. It includes steps for warmup training, building the gradient datastore, selecting data for a task, and training with the selected data. The repository provides tools for data preparation, data selection pipeline, and evaluation of the model trained on the selected data.
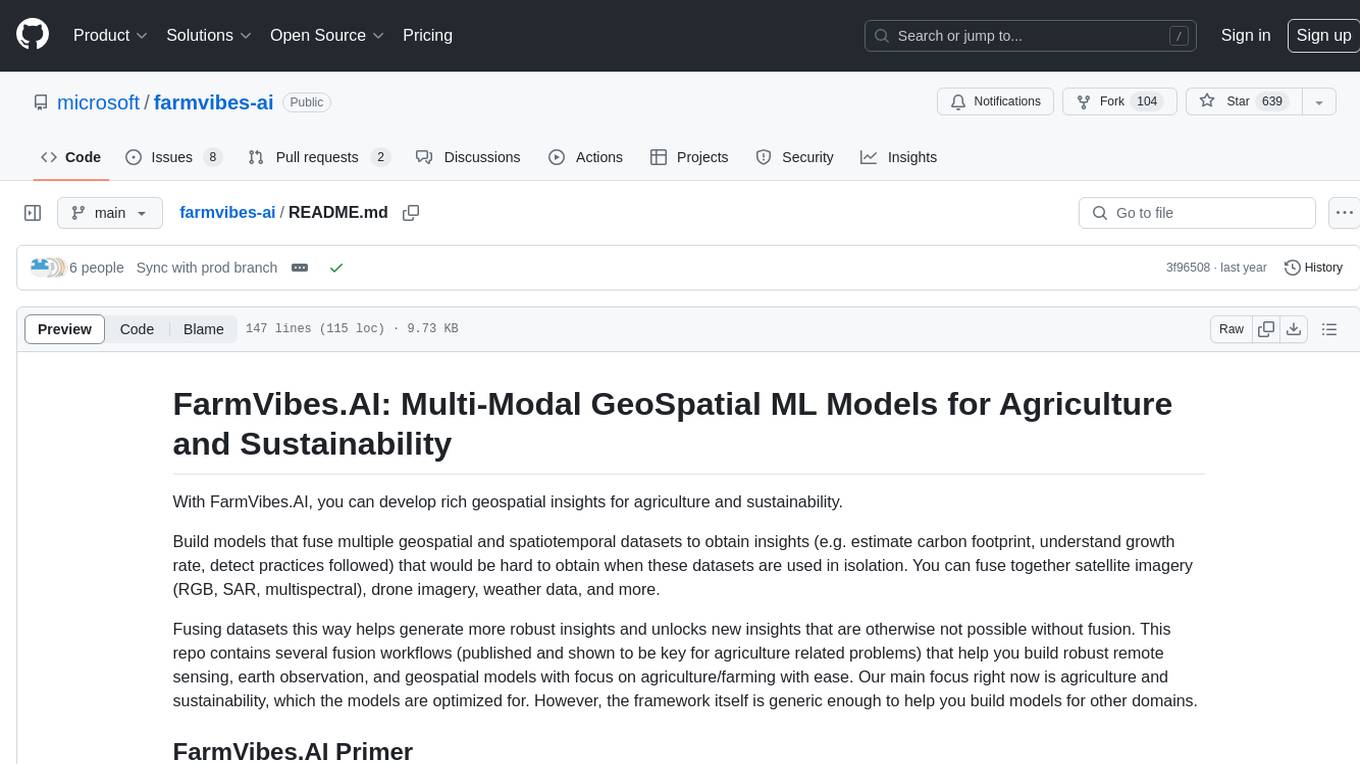
farmvibes-ai
FarmVibes.AI is a repository focused on developing multi-modal geospatial machine learning models for agriculture and sustainability. It enables users to fuse various geospatial and spatiotemporal datasets, such as satellite imagery, drone imagery, and weather data, to generate robust insights for agriculture-related problems. The repository provides fusion workflows, data preparation tools, model training notebooks, and an inference engine to facilitate the creation of geospatial models tailored for agriculture and farming. Users can interact with the tools via a local cluster, REST API, or a Python client, and the repository includes documentation and notebook examples to guide users in utilizing FarmVibes.AI for tasks like harvest date detection, climate impact estimation, micro climate prediction, and crop identification.
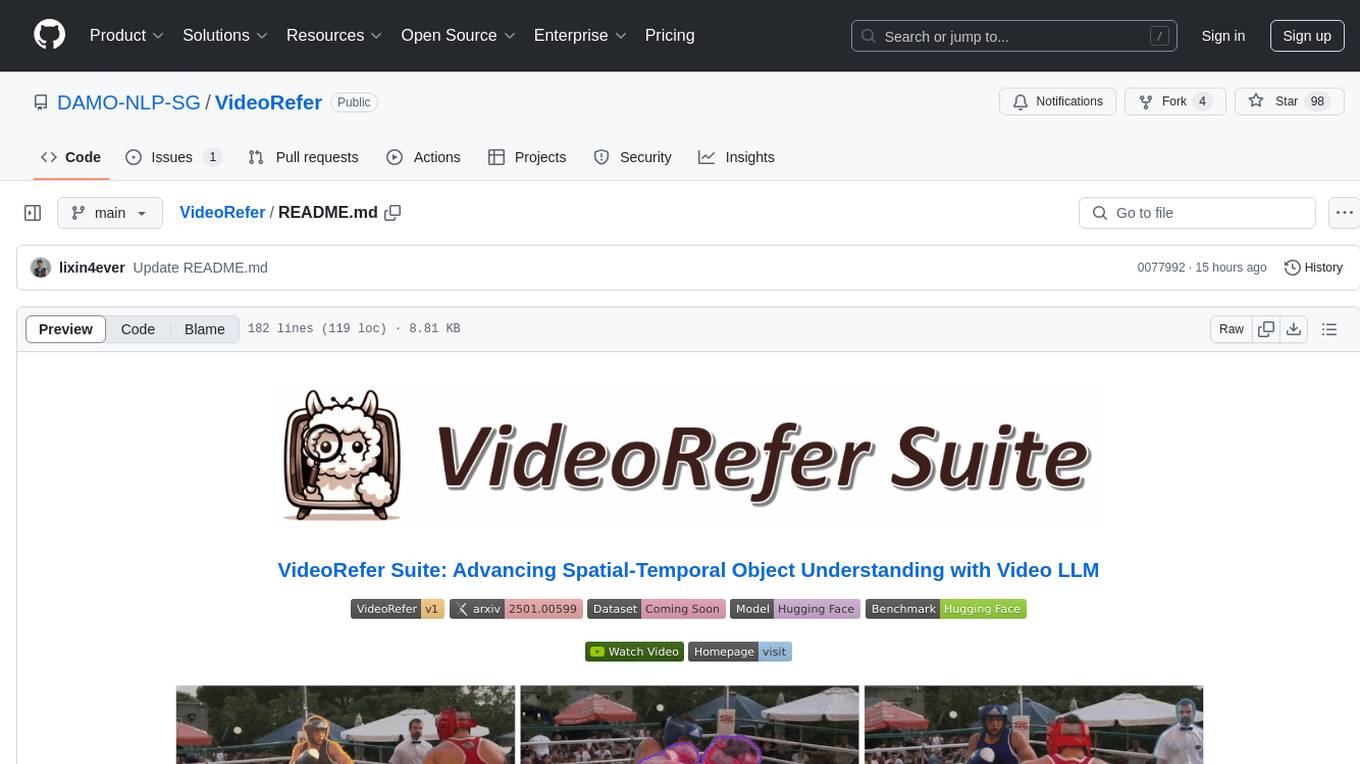
VideoRefer
VideoRefer Suite is a tool designed to enhance the fine-grained spatial-temporal understanding capabilities of Video Large Language Models (Video LLMs). It consists of three primary components: Model (VideoRefer) for perceiving, reasoning, and retrieval for user-defined regions at any specified timestamps, Dataset (VideoRefer-700K) for high-quality object-level video instruction data, and Benchmark (VideoRefer-Bench) to evaluate object-level video understanding capabilities. The tool can understand any object within a video.
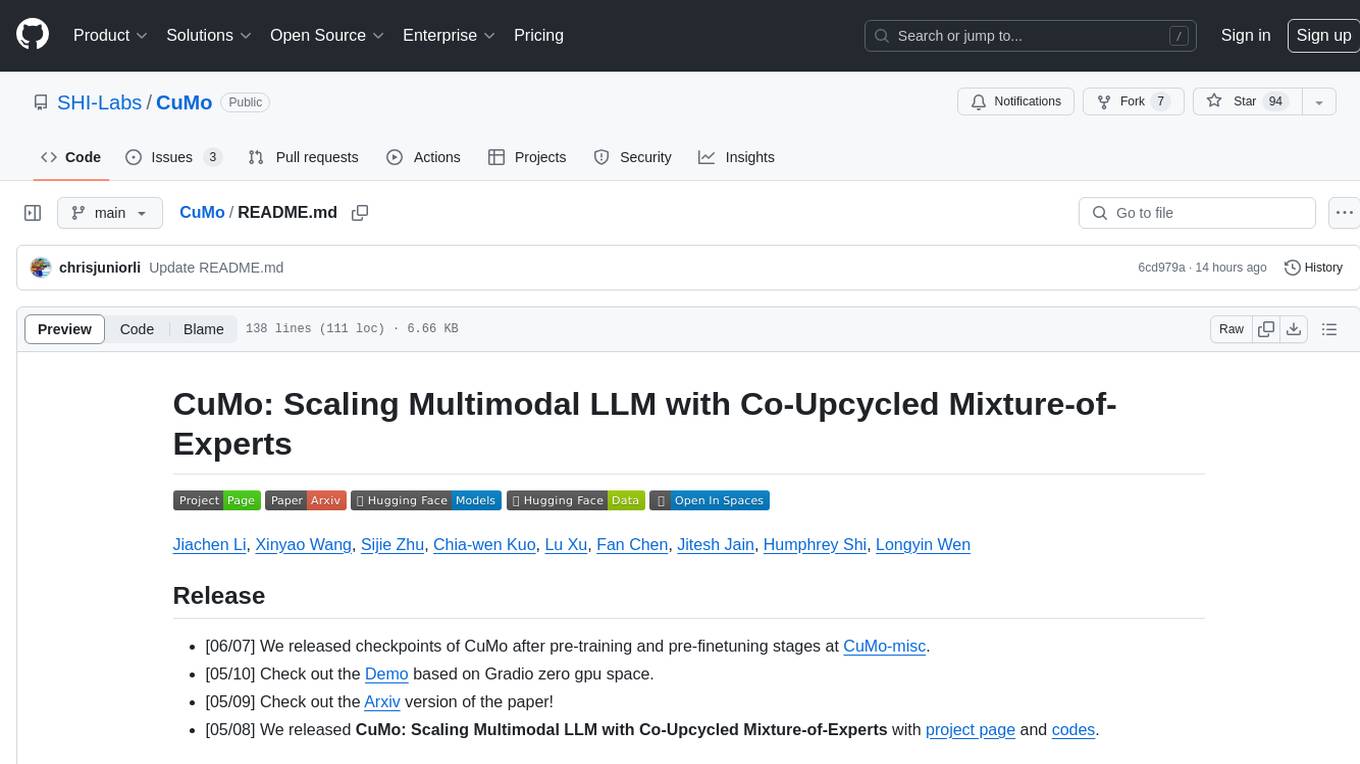
CuMo
CuMo is a project focused on scaling multimodal Large Language Models (LLMs) with Co-Upcycled Mixture-of-Experts. It introduces CuMo, which incorporates Co-upcycled Top-K sparsely-gated Mixture-of-experts blocks into the vision encoder and the MLP connector, enhancing the capabilities of multimodal LLMs. The project adopts a three-stage training approach with auxiliary losses to stabilize the training process and maintain a balanced loading of experts. CuMo achieves comparable performance to other state-of-the-art multimodal LLMs on various Visual Question Answering (VQA) and visual-instruction-following benchmarks.
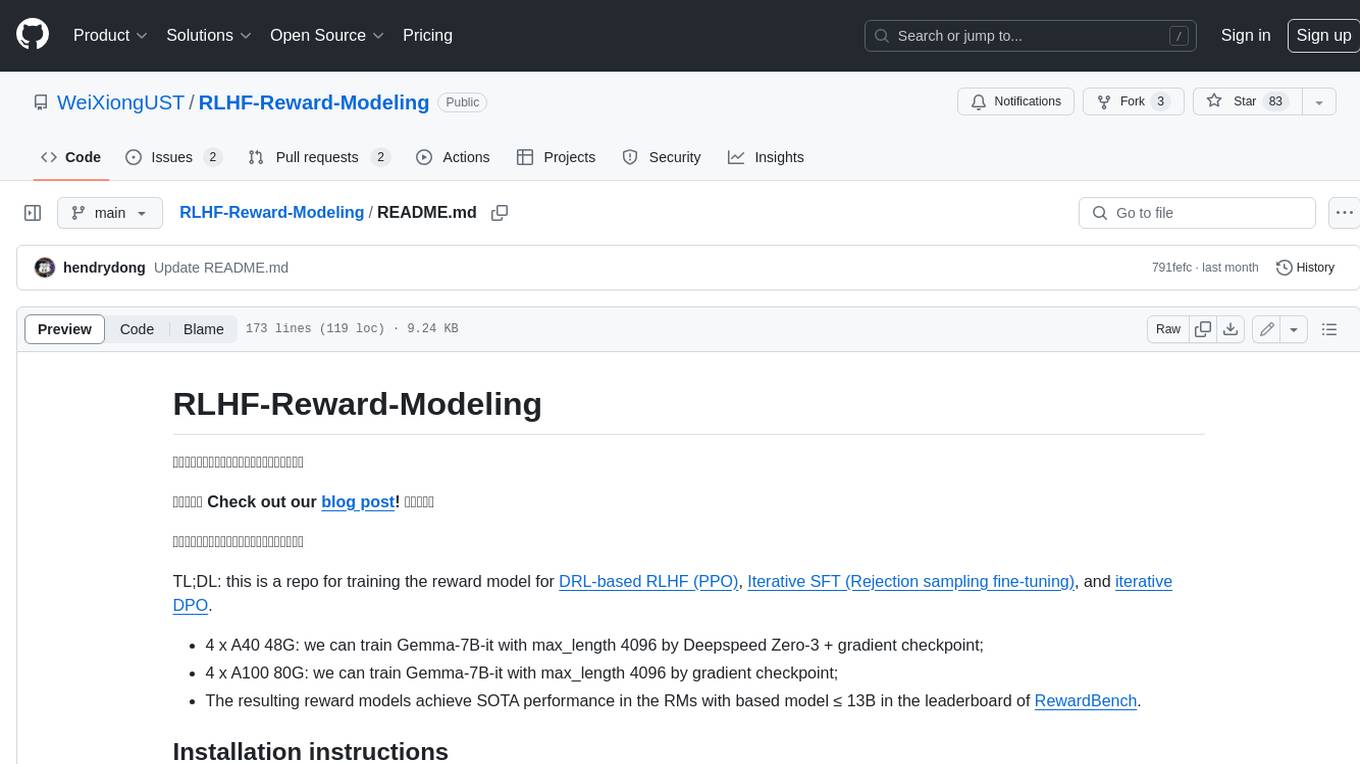
RLHF-Reward-Modeling
This repository contains code for training reward models for Deep Reinforcement Learning-based Reward-modulated Hierarchical Fine-tuning (DRL-based RLHF), Iterative Selection Fine-tuning (Rejection sampling fine-tuning), and iterative Decision Policy Optimization (DPO). The reward models are trained using a Bradley-Terry model based on the Gemma and Mistral language models. The resulting reward models achieve state-of-the-art performance on the RewardBench leaderboard for reward models with base models of up to 13B parameters.
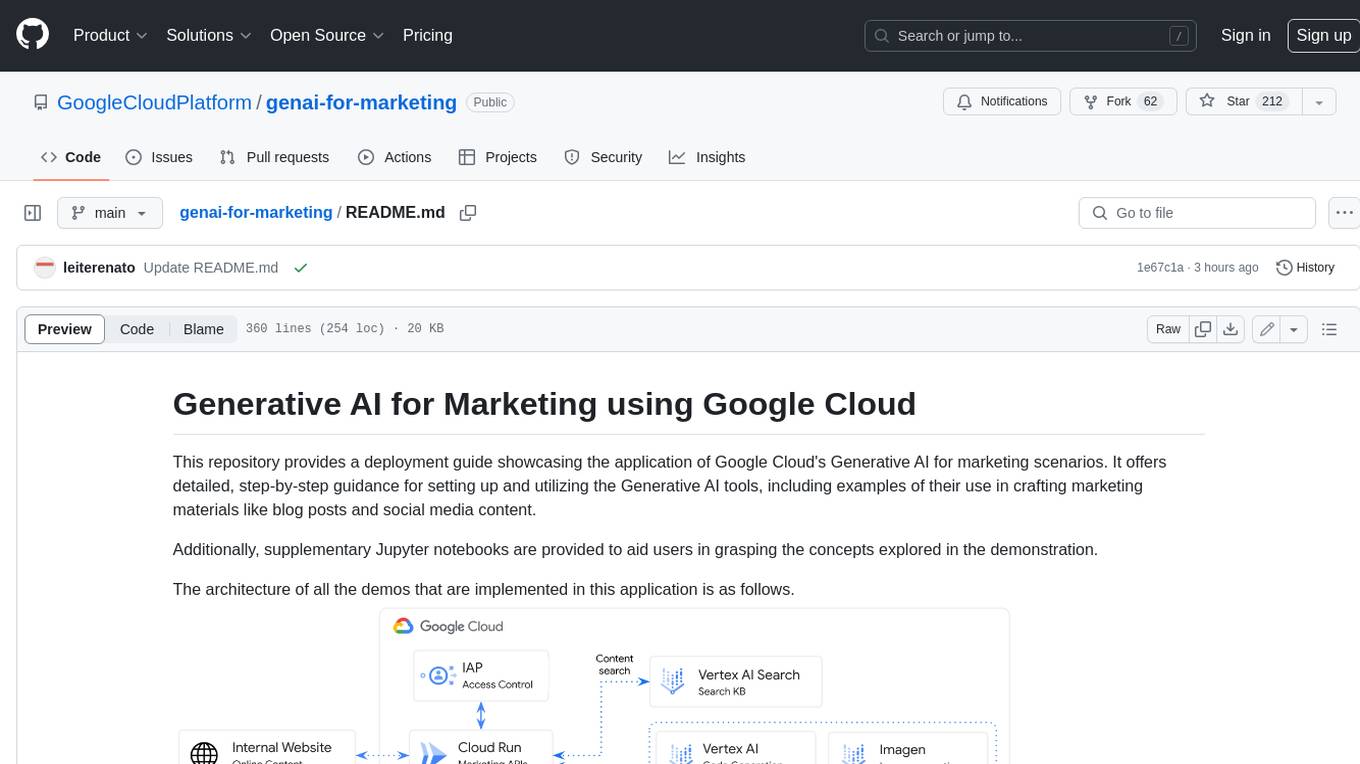
genai-for-marketing
This repository provides a deployment guide for utilizing Google Cloud's Generative AI tools in marketing scenarios. It includes step-by-step instructions, examples of crafting marketing materials, and supplementary Jupyter notebooks. The demos cover marketing insights, audience analysis, trendspotting, content search, content generation, and workspace integration. Users can access and visualize marketing data, analyze trends, improve search experience, and generate compelling content. The repository structure includes backend APIs, frontend code, sample notebooks, templates, and installation scripts.
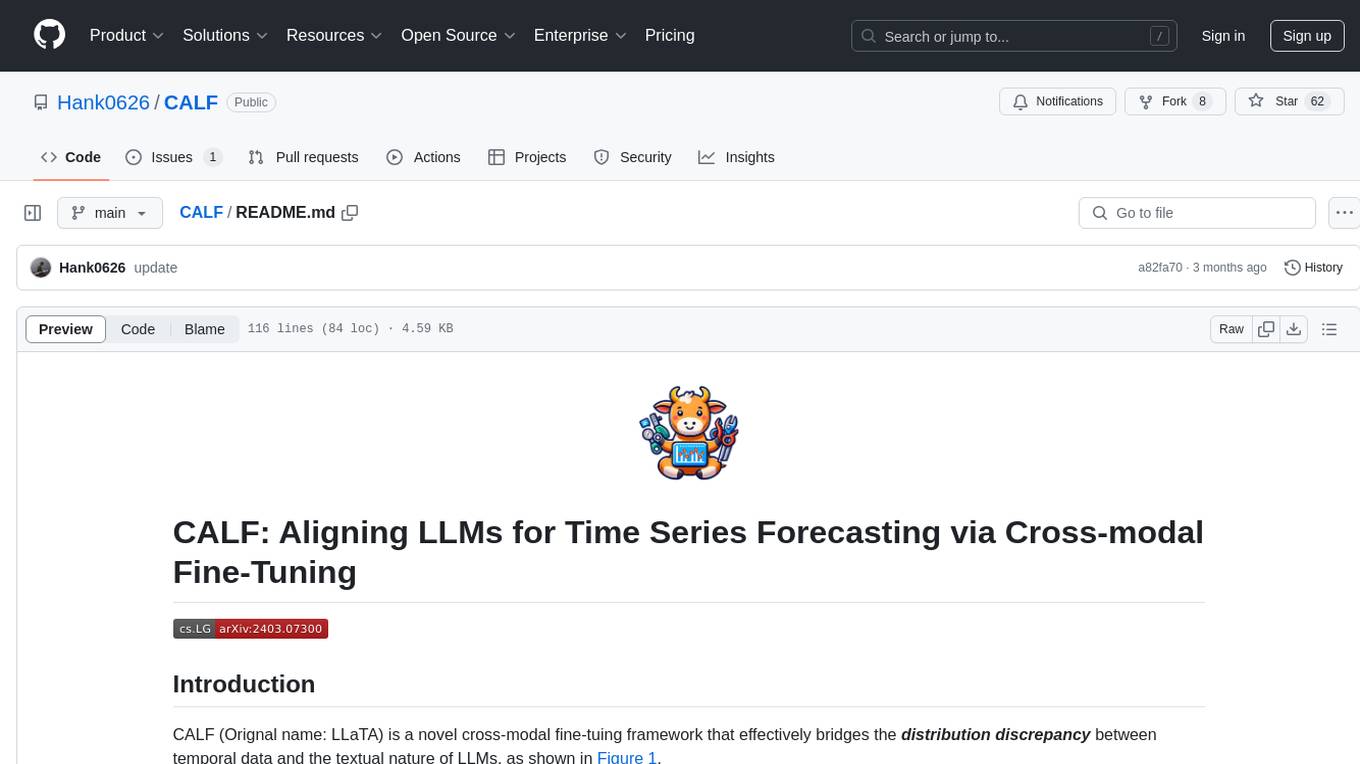
CALF
CALF (LLaTA) is a cross-modal fine-tuning framework that bridges the distribution discrepancy between temporal data and the textual nature of LLMs. It introduces three cross-modal fine-tuning techniques: Cross-Modal Match Module, Feature Regularization Loss, and Output Consistency Loss. The framework aligns time series and textual inputs, ensures effective weight updates, and maintains consistent semantic context for time series data. CALF provides scripts for long-term and short-term forecasting, requires Python 3.9, and utilizes word token embeddings for model training.
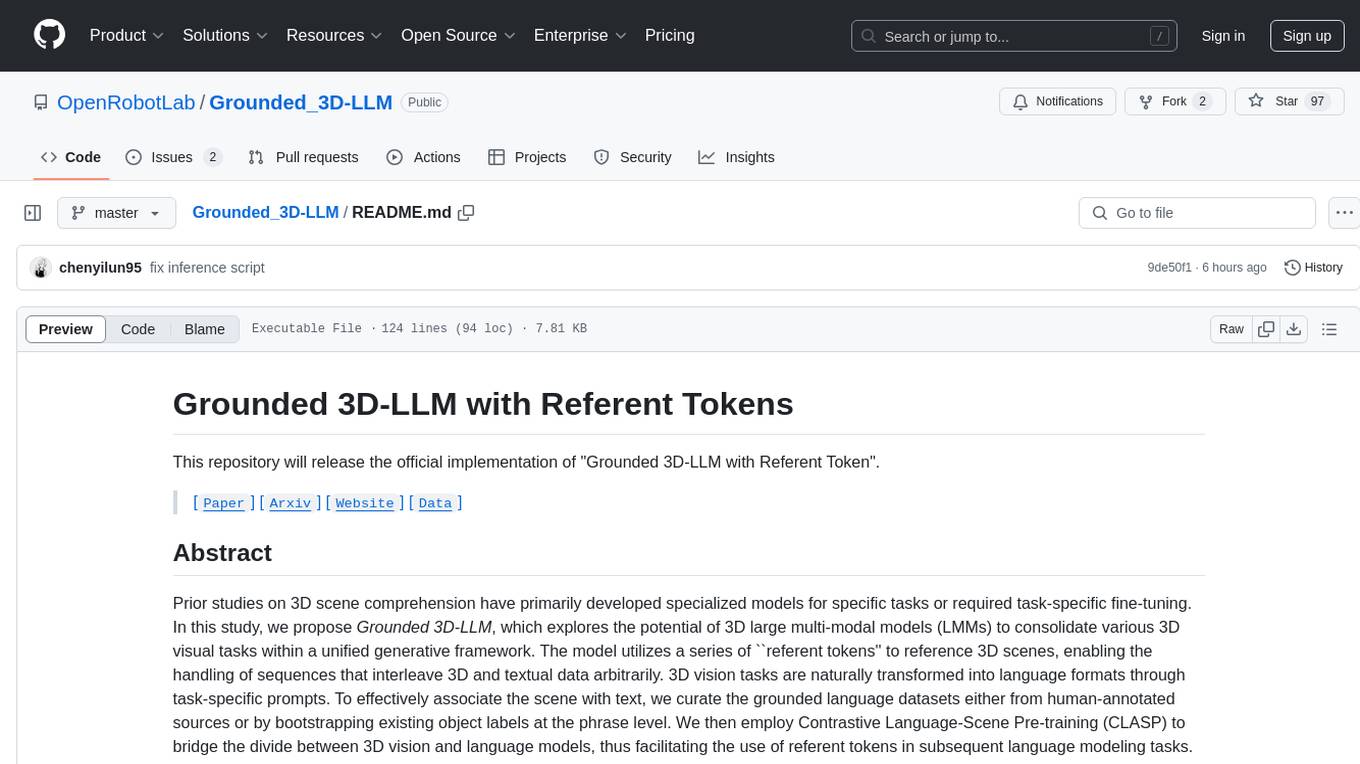
Grounded_3D-LLM
Grounded 3D-LLM is a unified generative framework that utilizes referent tokens to reference 3D scenes, enabling the handling of sequences that interleave 3D and textual data. It transforms 3D vision tasks into language formats through task-specific prompts, curating grounded language datasets and employing Contrastive Language-Scene Pre-training (CLASP) to bridge the gap between 3D vision and language models. The model covers tasks like 3D visual question answering, dense captioning, object detection, and language grounding.
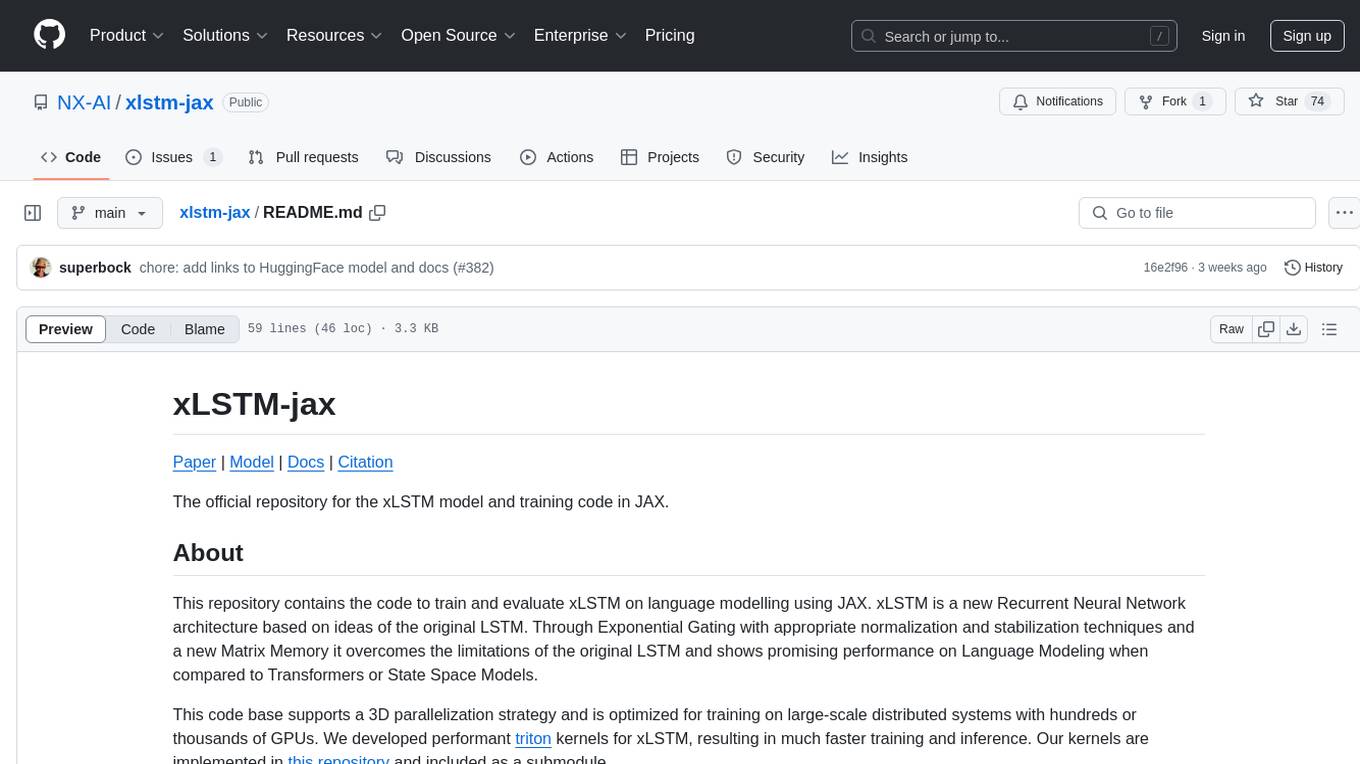
xlstm-jax
The xLSTM-jax repository contains code for training and evaluating the xLSTM model on language modeling using JAX. xLSTM is a Recurrent Neural Network architecture that improves upon the original LSTM through Exponential Gating, normalization, stabilization techniques, and a Matrix Memory. It is optimized for large-scale distributed systems with performant triton kernels for faster training and inference.
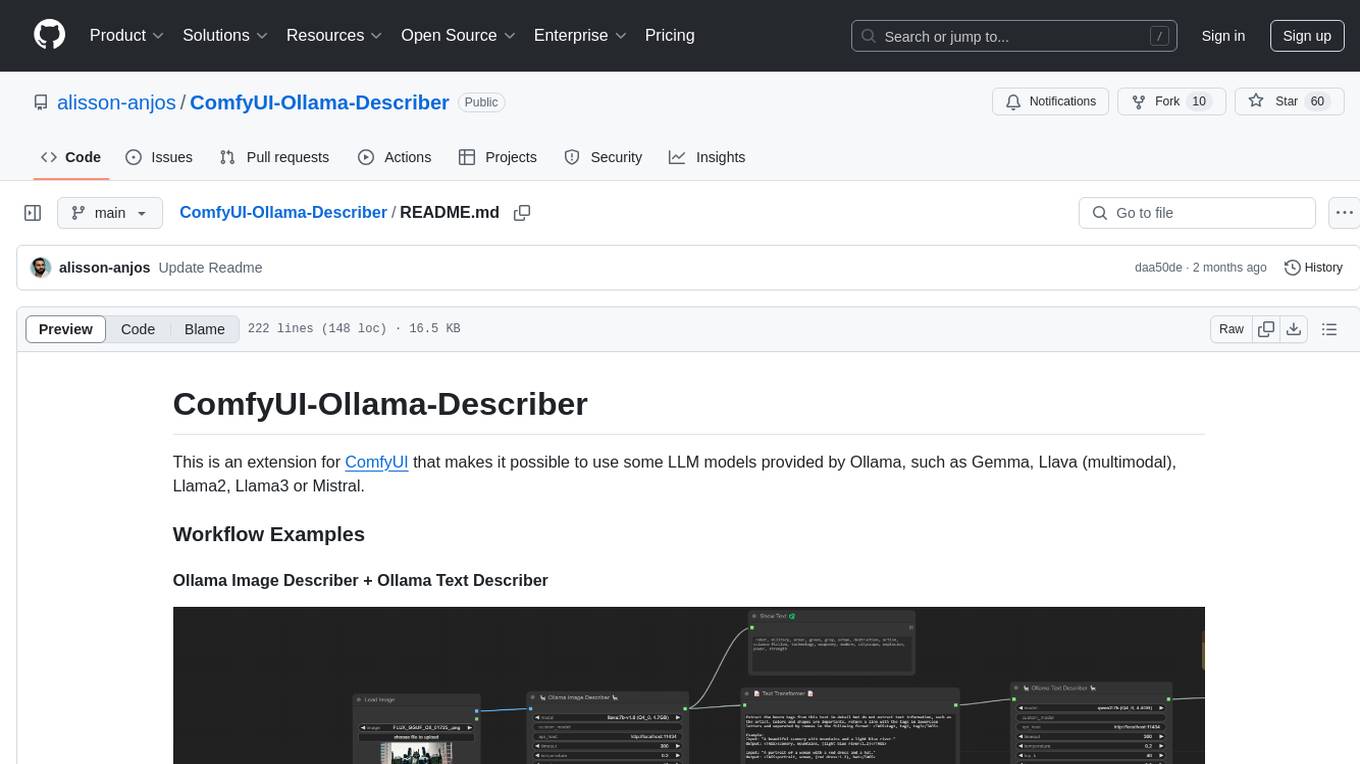
ComfyUI-Ollama-Describer
ComfyUI-Ollama-Describer is an extension for ComfyUI that enables the use of LLM models provided by Ollama, such as Gemma, Llava (multimodal), Llama2, Llama3, or Mistral. It requires the Ollama library for interacting with large-scale language models, supporting GPUs using CUDA and AMD GPUs on Windows, Linux, and Mac. The extension allows users to run Ollama through Docker and utilize NVIDIA GPUs for faster processing. It provides nodes for image description, text description, image captioning, and text transformation, with various customizable parameters for model selection, API communication, response generation, and model memory management.
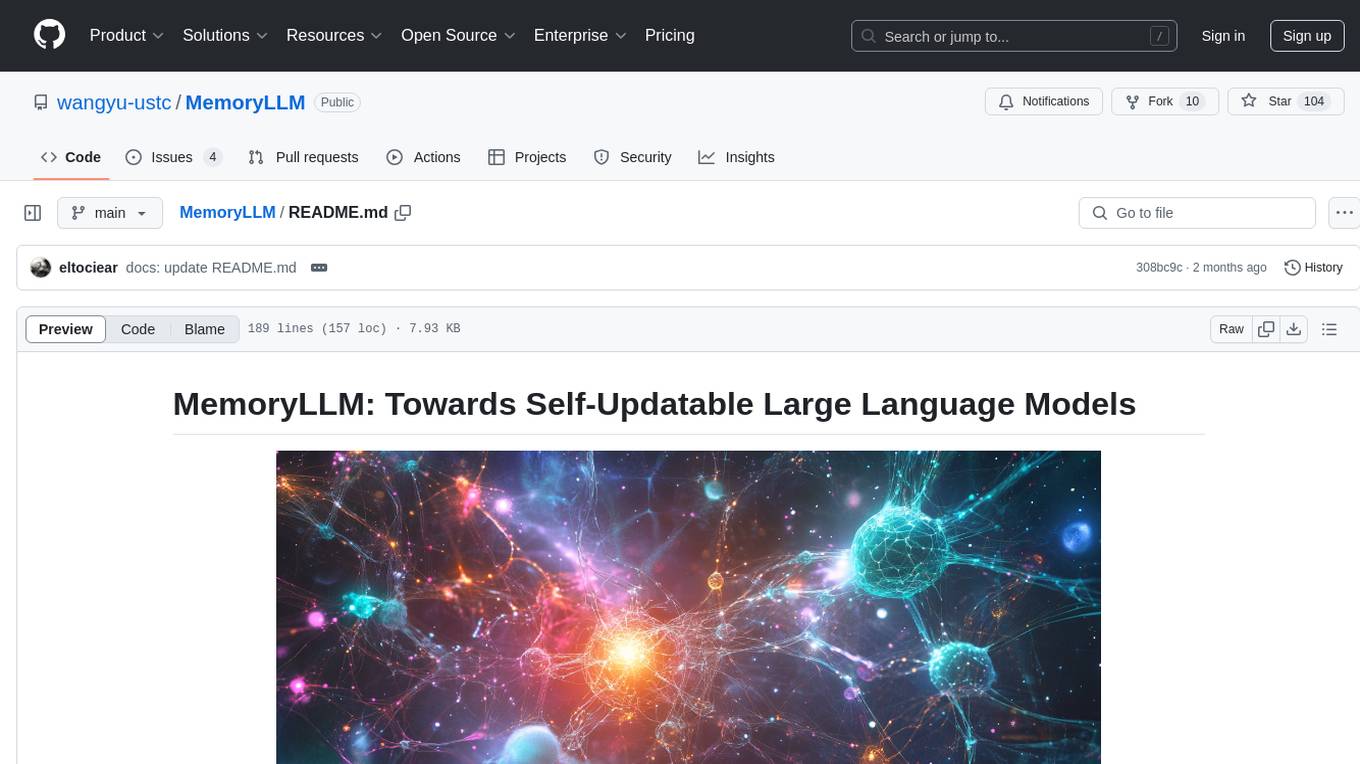
MemoryLLM
MemoryLLM is a large language model designed for self-updating capabilities. It offers pretrained models with different memory capacities and features, such as chat models. The repository provides training code, evaluation scripts, and datasets for custom experiments. MemoryLLM aims to enhance knowledge retention and performance on various natural language processing tasks.
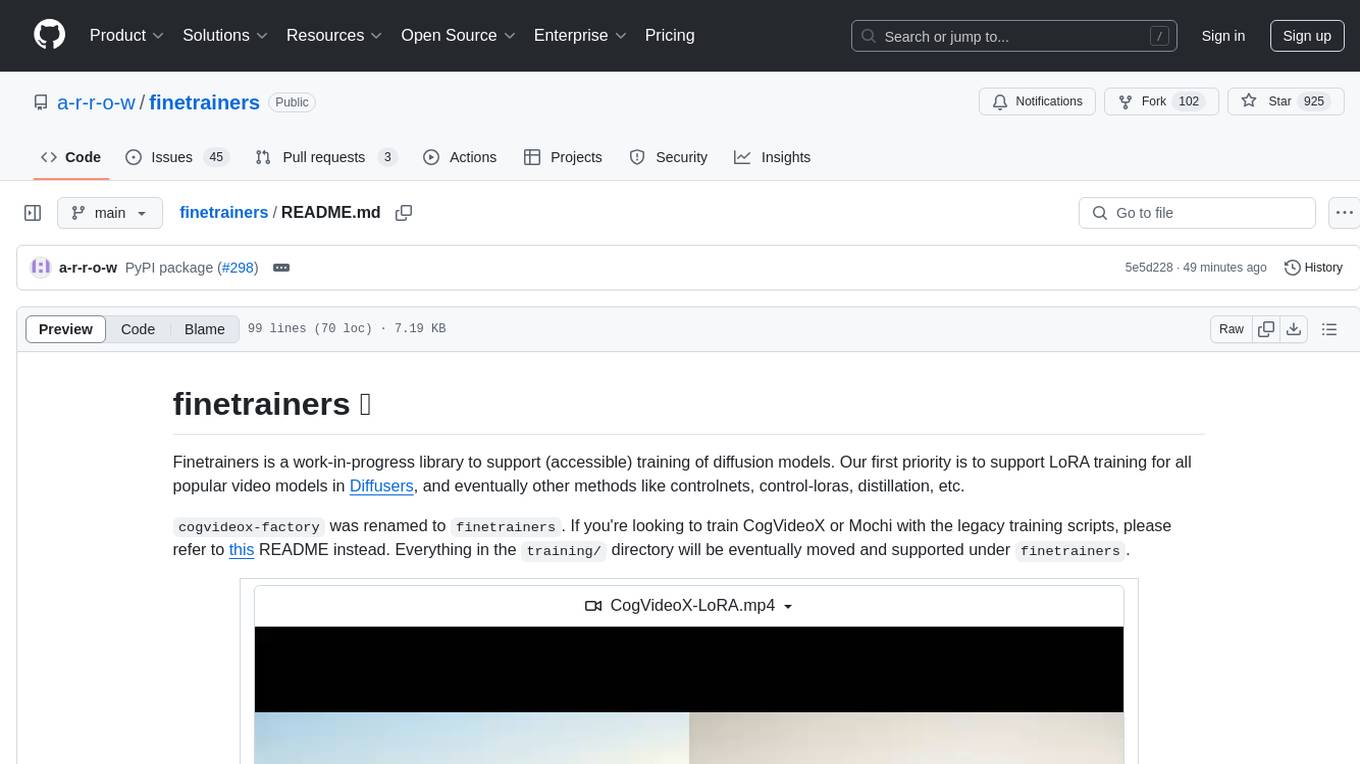
finetrainers
FineTrainers is a work-in-progress library designed to support the training of video models, with a focus on LoRA training for popular video models in Diffusers. It aims to eventually extend support to other methods like controlnets, control-loras, distillation, etc. The library provides tools for training custom models, handling big datasets, and supporting multi-backend distributed training. It also offers tooling for curating small and high-quality video datasets for fine-tuning.
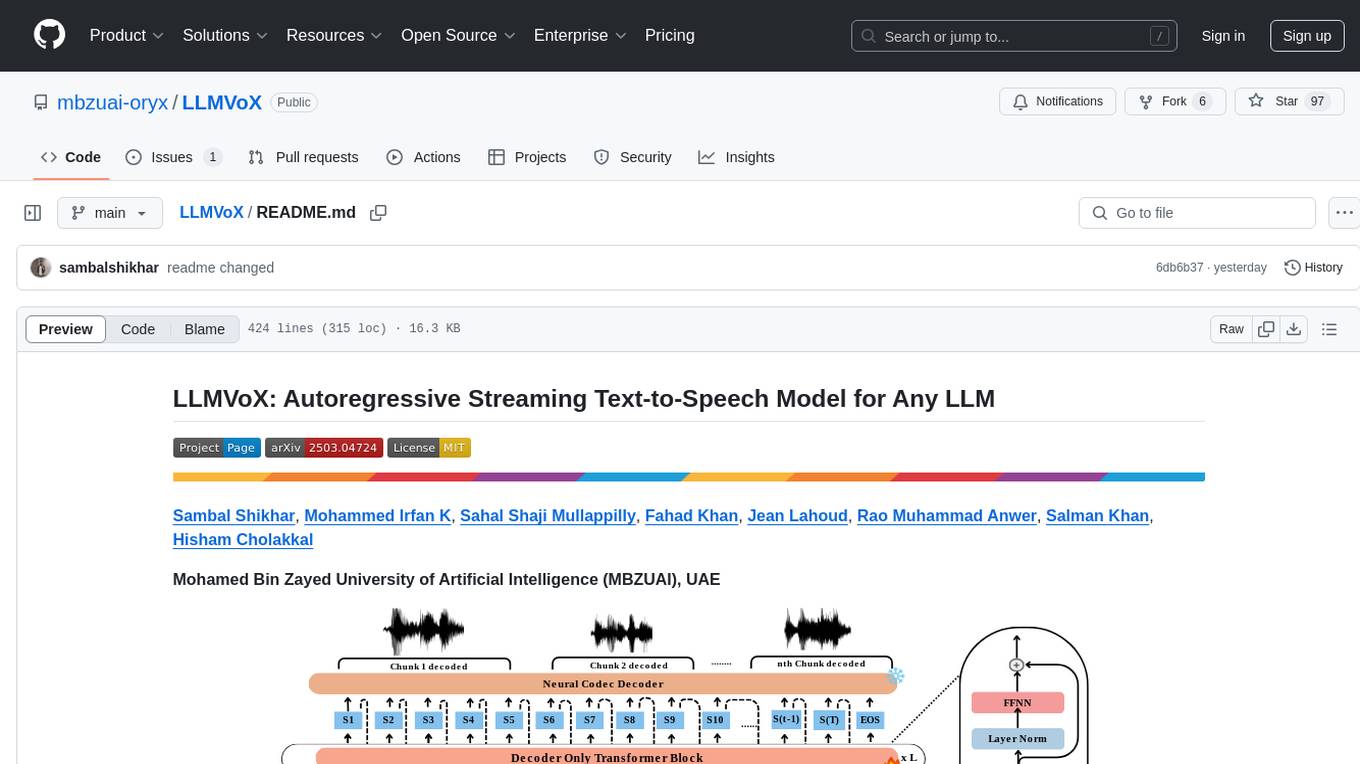
LLMVoX
LLMVoX is a lightweight 30M-parameter, LLM-agnostic, autoregressive streaming Text-to-Speech (TTS) system designed to convert text outputs from Large Language Models into high-fidelity streaming speech with low latency. It achieves significantly lower Word Error Rate compared to speech-enabled LLMs while operating at comparable latency and speech quality. Key features include being lightweight & fast with only 30M parameters, LLM-agnostic for easy integration with existing models, multi-queue streaming for continuous speech generation, and multilingual support for easy adaptation to new languages.
15 - OpenAI Gpts
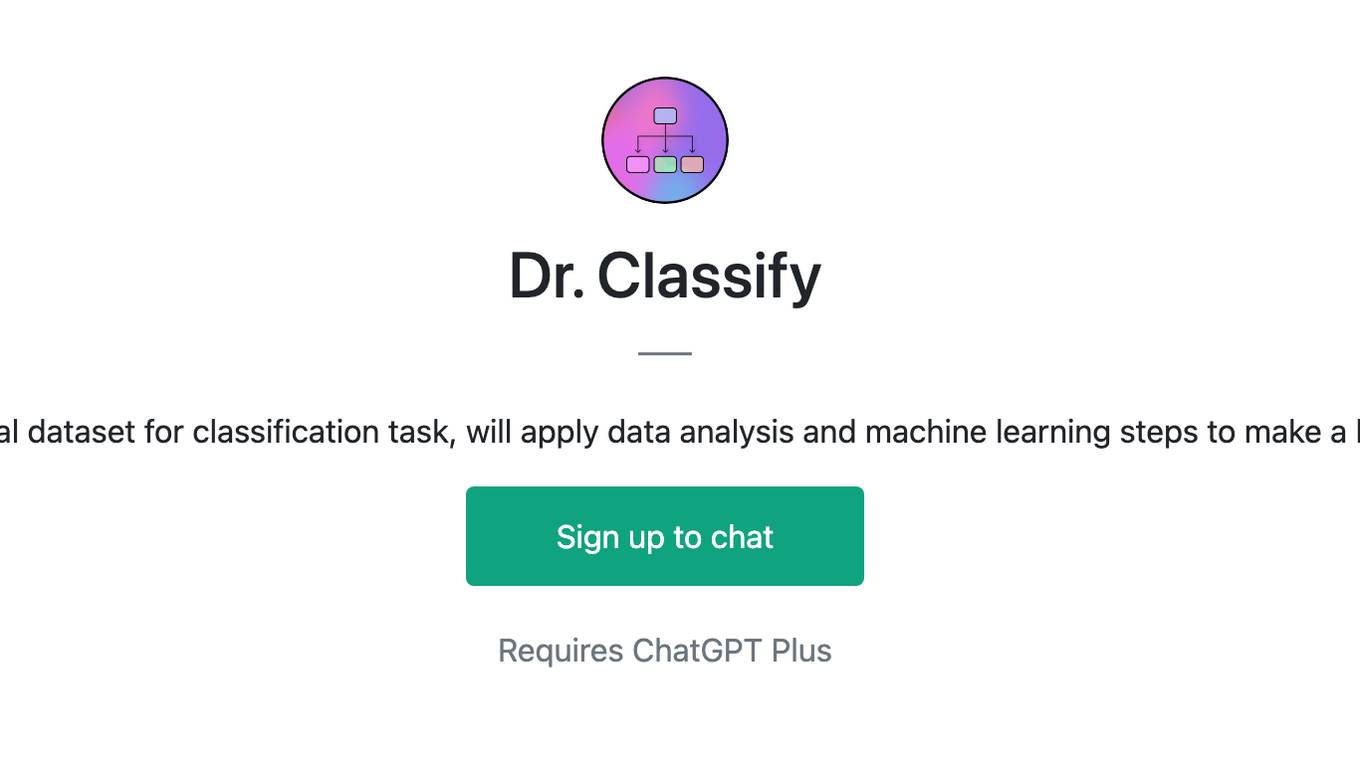
Dr. Classify
Just upload a numerical dataset for classification task, will apply data analysis and machine learning steps to make a best model possible.
![VitalsGPT [V0.0.2.2] Screenshot](/screenshots_gpts/g-cL1rJdm11.jpg)
VitalsGPT [V0.0.2.2]
Simple CustomGPT built on Vitals Inquiry Case in Malta, aimed to help journalists and citizens navigate the inquiry's large dataset in a neutral, informative fashion. Always cross-reference replies to actual data. Do not rely solely on this LLM for verification of facts.
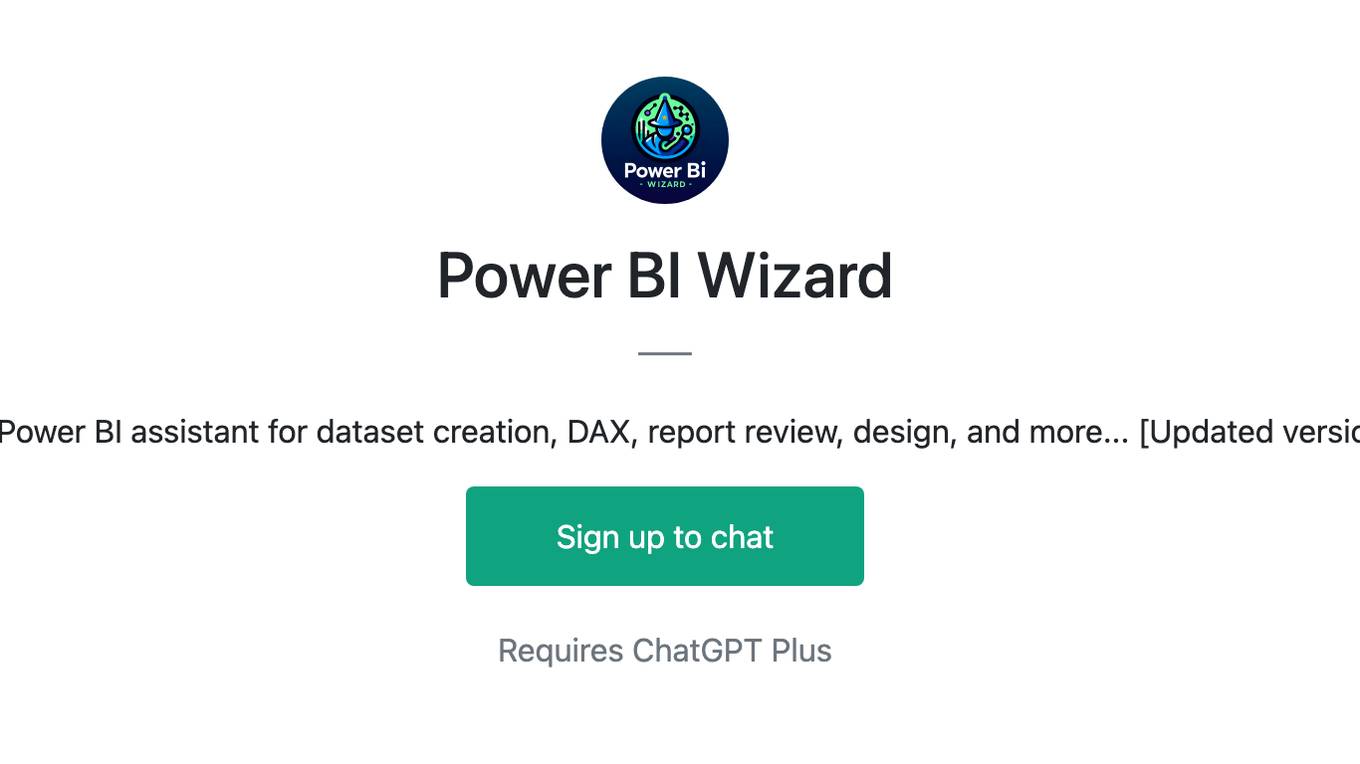
Power BI Wizard
Your Power BI assistant for dataset creation, DAX, report review, design, and more... [Updated version].
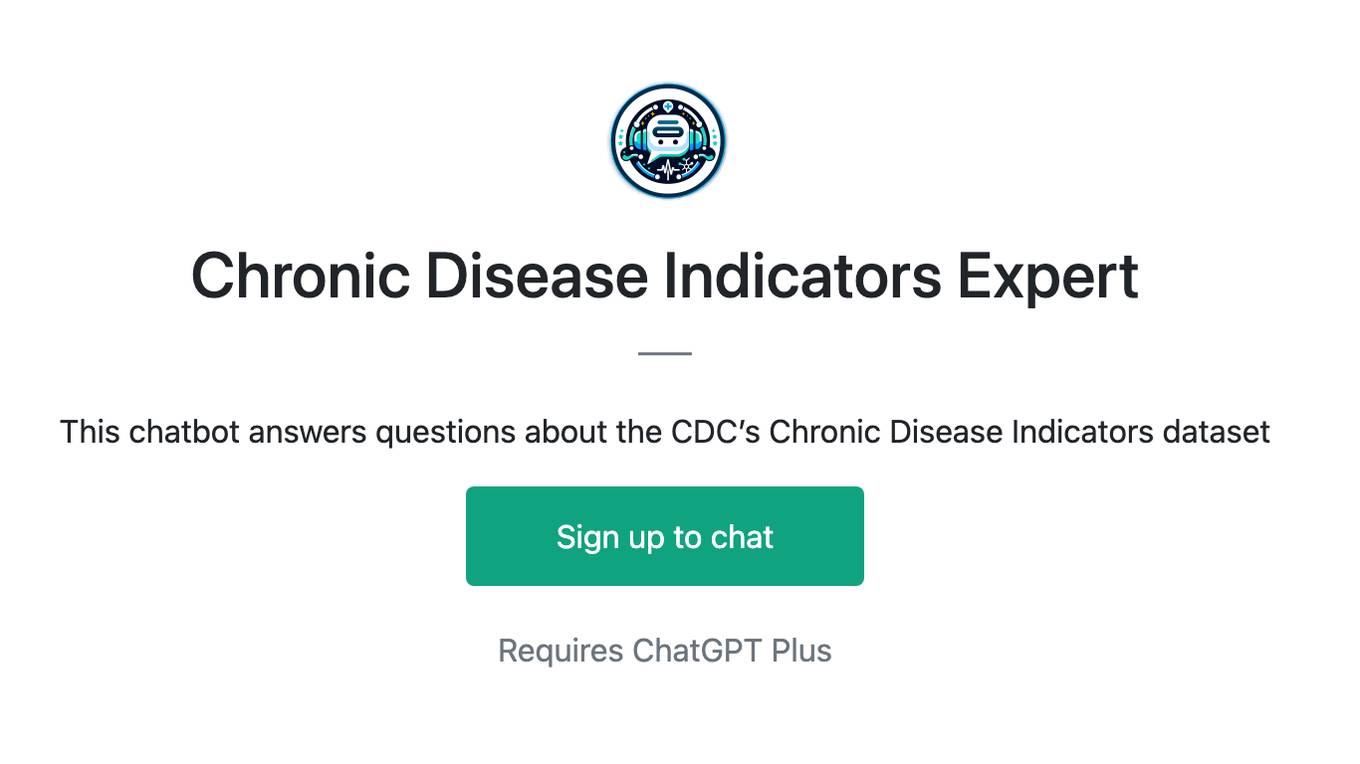
Chronic Disease Indicators Expert
This chatbot answers questions about the CDC’s Chronic Disease Indicators dataset
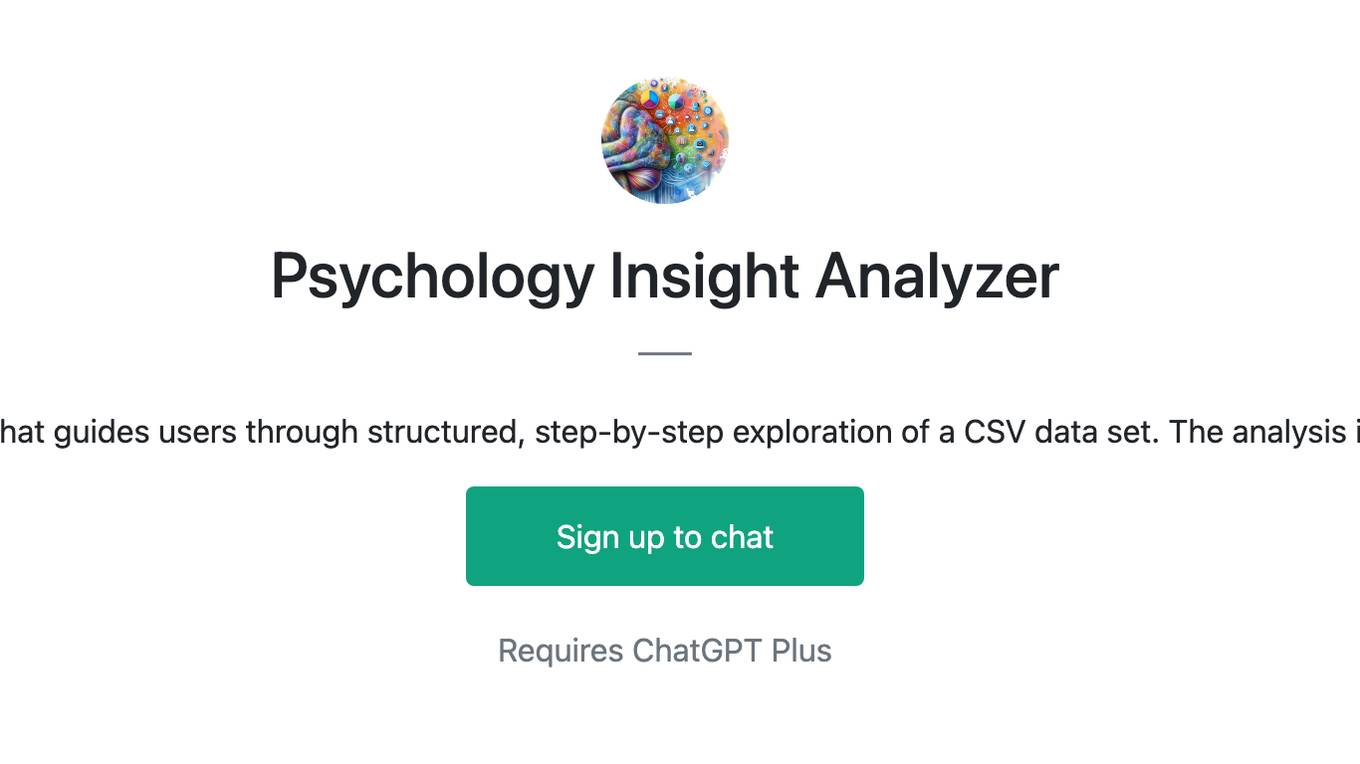
Psychology Insight Analyzer
Psychology data analysis expert that guides users through structured, step-by-step exploration of a CSV data set. The analysis is based on research questions.
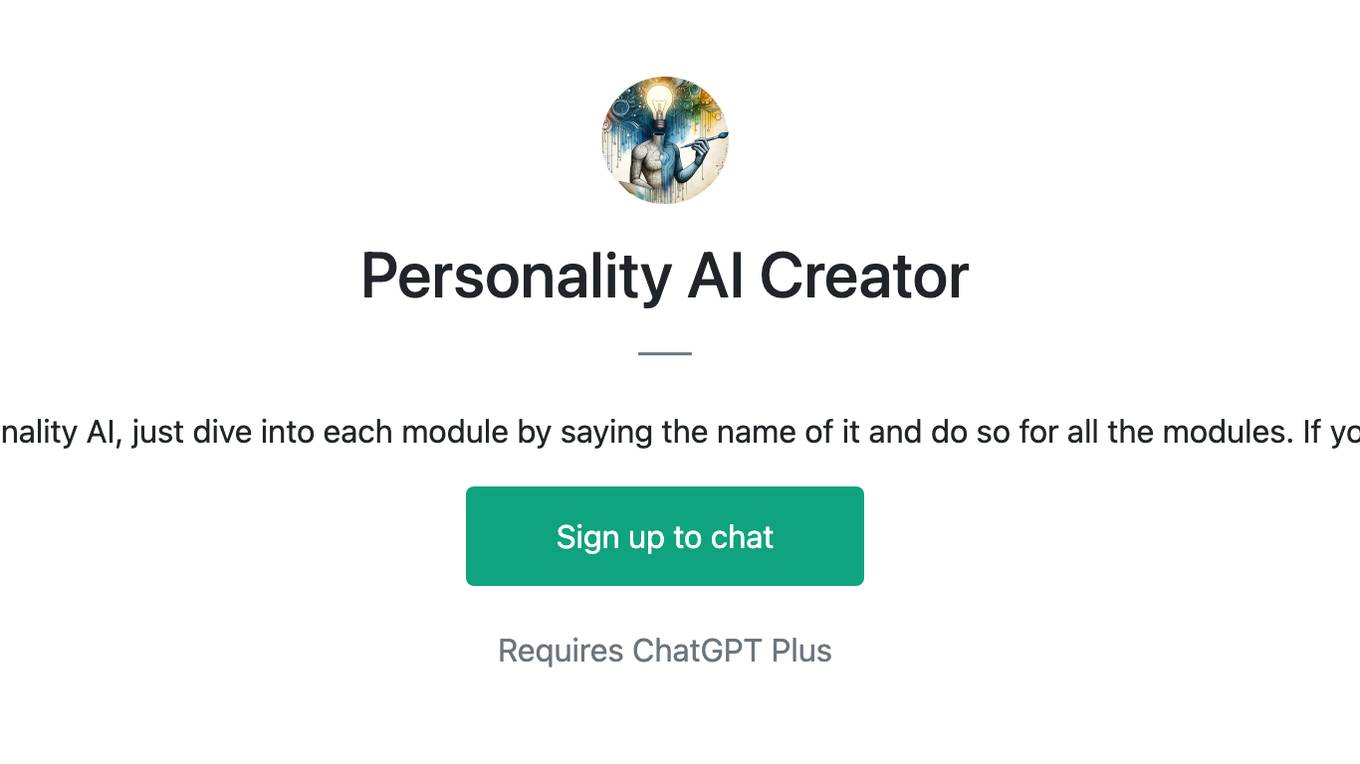
Personality AI Creator
I will create a quality data set for a personality AI, just dive into each module by saying the name of it and do so for all the modules. If you find it useful, share it to your friends
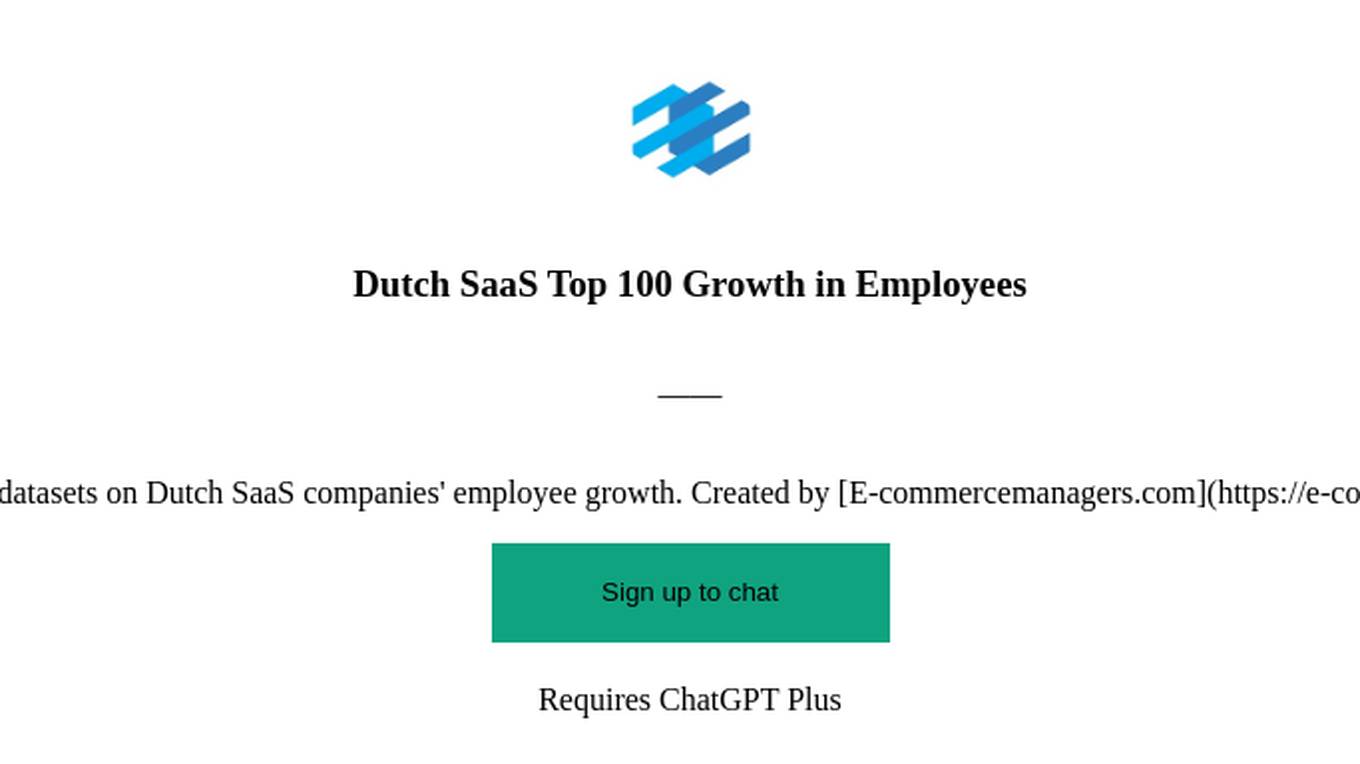
Dutch SaaS Top 100 Growth in Employees
Analyze and interpret datasets on Dutch SaaS companies' employee growth. Created by [E-commercemanagers.com](https://e-commercemanagers.com).
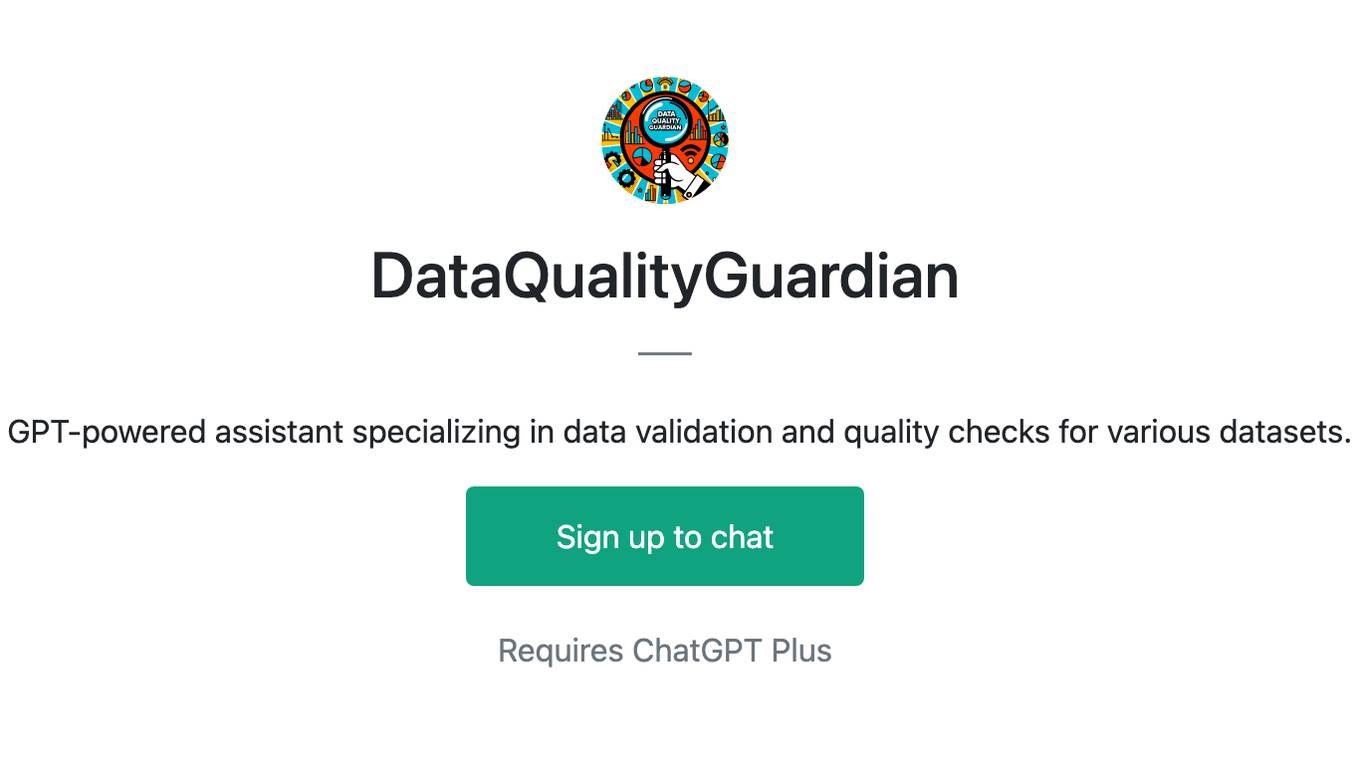
DataQualityGuardian
A GPT-powered assistant specializing in data validation and quality checks for various datasets.
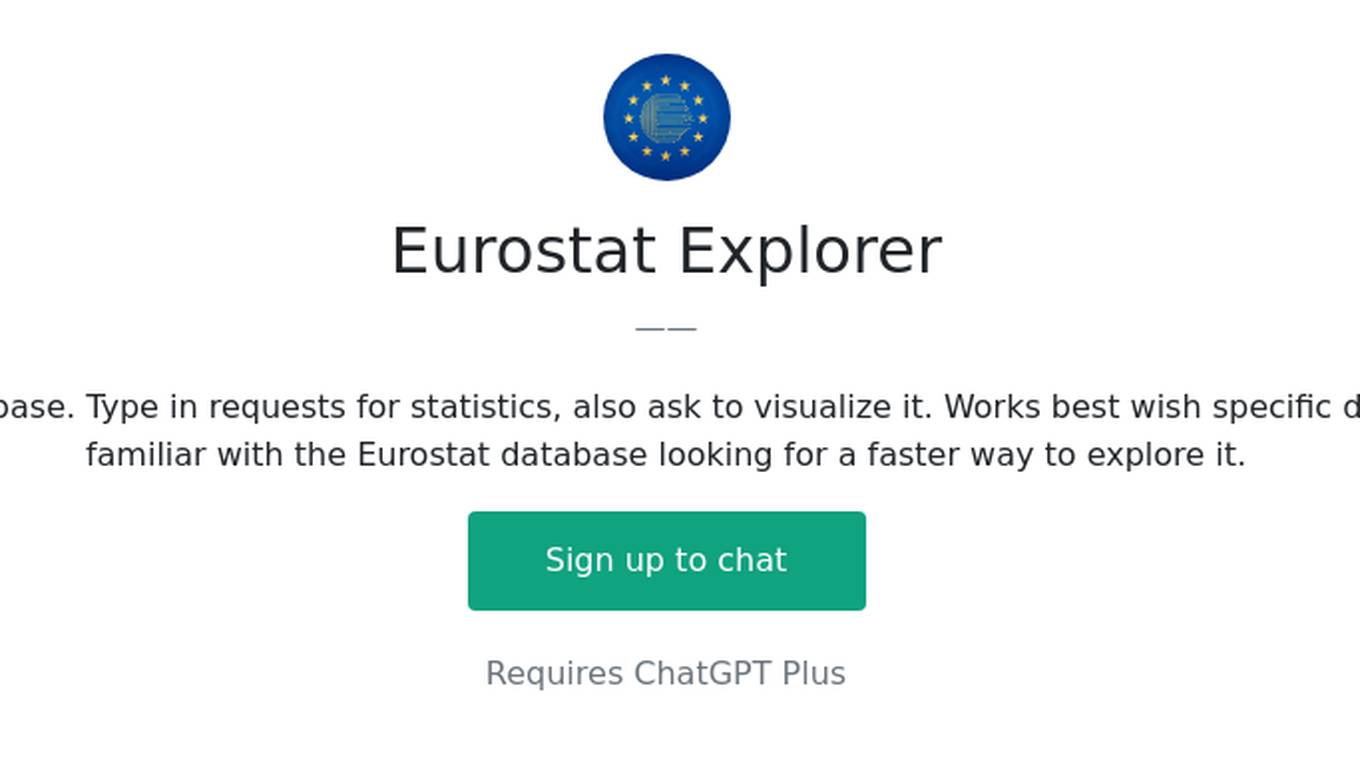
Eurostat Explorer
Explore & interpret the Eurostat database. Type in requests for statistics, also ask to visualize it. Works best wish specific datasets. It's meant for professionals familiar with the Eurostat database looking for a faster way to explore it.
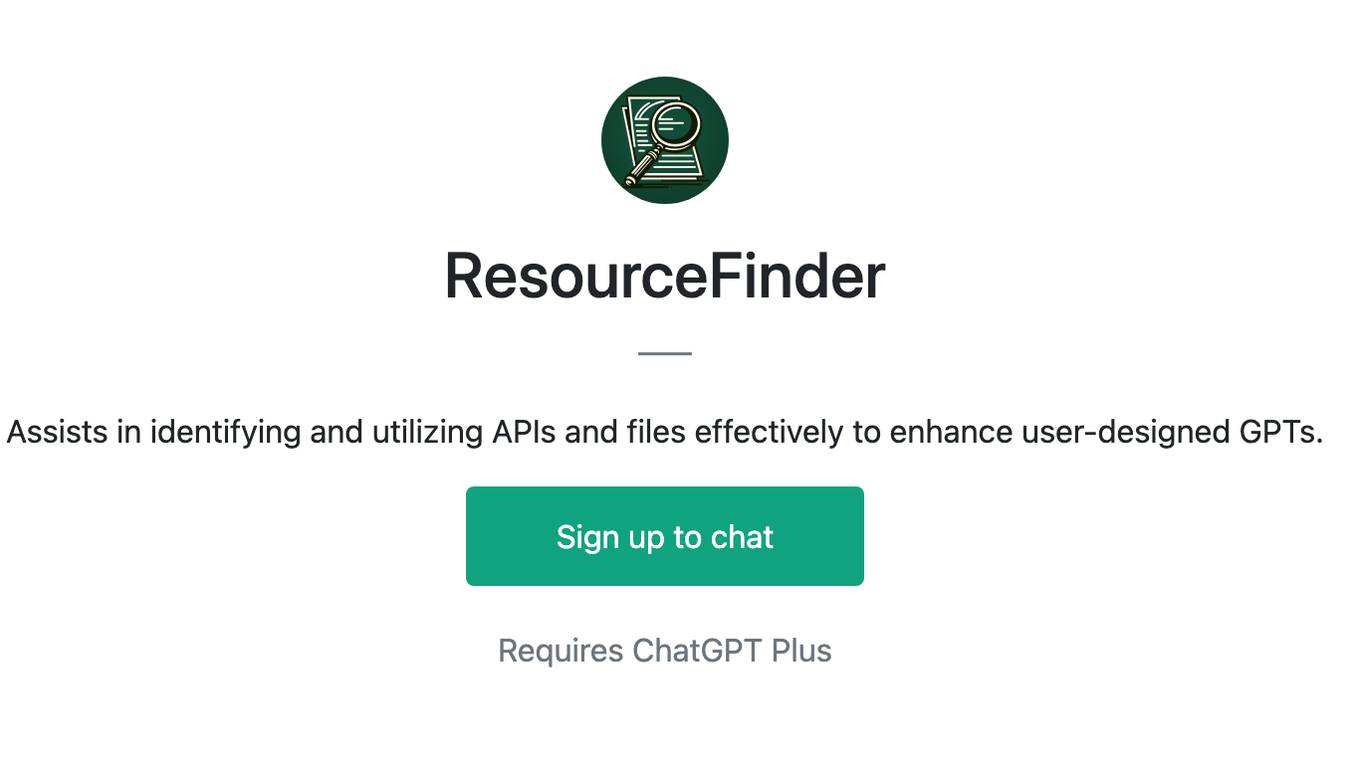
ResourceFinder
Assists in identifying and utilizing APIs and files effectively to enhance user-designed GPTs.
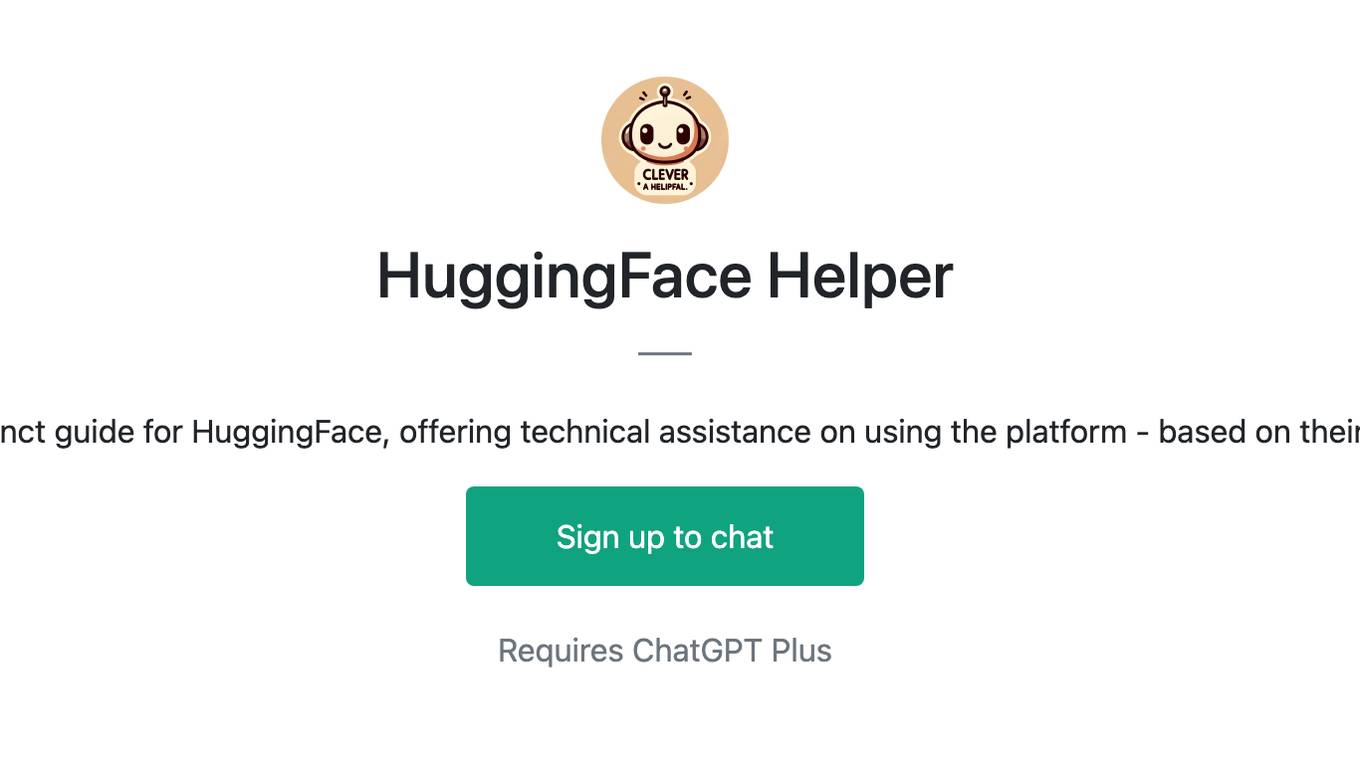
HuggingFace Helper
A witty yet succinct guide for HuggingFace, offering technical assistance on using the platform - based on their Learning Hub