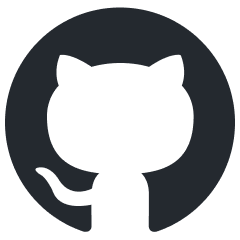
mystic
constrained nonlinear optimization for scientific machine learning, UQ, and AI
Stars: 473
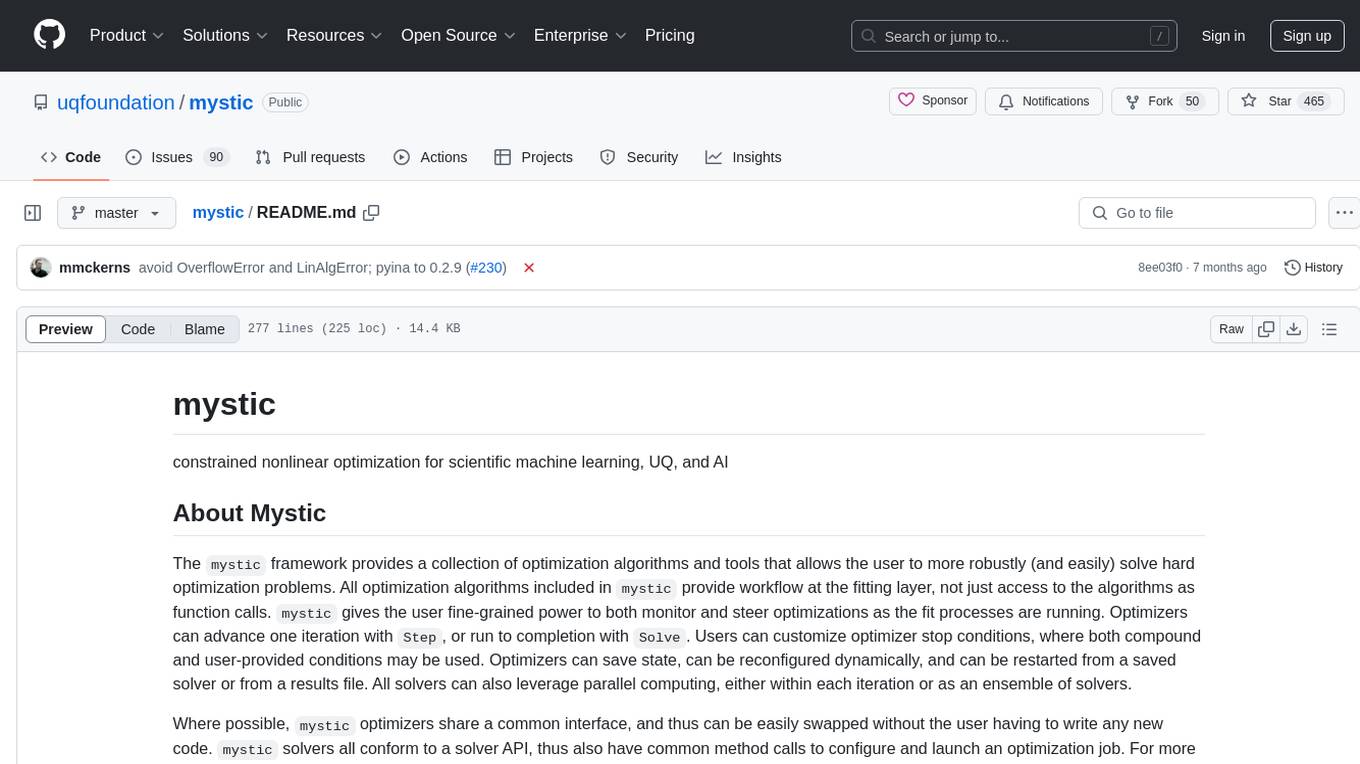
The `mystic` framework provides a collection of optimization algorithms and tools that allow the user to robustly solve hard optimization problems. It offers fine-grained power to monitor and steer optimizations during the fit processes. Optimizers can advance one iteration or run to completion, with customizable stop conditions. `mystic` optimizers share a common interface for easy swapping without writing new code. The framework supports parameter constraints, including soft and hard constraints, and provides tools for scientific machine learning, uncertainty quantification, adaptive sampling, nonlinear interpolation, and artificial intelligence. `mystic` is actively developed and welcomes user feedback and contributions.
README:
constrained nonlinear optimization for scientific machine learning, UQ, and AI
The mystic
framework provides a collection of optimization algorithms
and tools that allows the user to more robustly (and easily) solve hard
optimization problems. All optimization algorithms included in mystic
provide workflow at the fitting layer, not just access to the algorithms
as function calls. mystic
gives the user fine-grained power to both
monitor and steer optimizations as the fit processes are running.
Optimizers can advance one iteration with Step
, or run to completion
with Solve
. Users can customize optimizer stop conditions, where both
compound and user-provided conditions may be used. Optimizers can save
state, can be reconfigured dynamically, and can be restarted from a
saved solver or from a results file. All solvers can also leverage
parallel computing, either within each iteration or as an ensemble of
solvers.
Where possible, mystic
optimizers share a common interface, and thus can
be easily swapped without the user having to write any new code. mystic
solvers all conform to a solver API, thus also have common method calls
to configure and launch an optimization job. For more details, see
mystic.abstract_solver
. The API also makes it easy to bind a favorite
3rd party solver into the mystic
framework.
Optimization algorithms in mystic
can accept parameter constraints,
as "soft constraints" (i.e. penalties
, which "penalize" regions of
solution space that violate the constraints), or as "hard constraints"
(i.e. constraints
, which constrain the solver to only search in regions
of space where the constraints are respected), or both. mystic
provides
a large selection of constraints, including probabistic and dimensionally
reducing constraints. By providing a robust interface designed to
enable the user to easily configure and control solvers, mystic
greatly reduces the barrier to solving hard optimization problems.
Sampling, interpolation, and statistics in mystic
are all designed
to seamlessly couple with constrained optimization to facilitate
scientific machine learning, uncertainty quantification, adaptive
sampling, nonlinear interpolation, and artificial intelligence.
mystic
can convert systems of equalities and inequalities to
hard or soft constraints using methods in mystic.symbolic
.
With mystic.constraints.vectorize
, constraints can be converted
to kernel transforms for use in machine learning. Similarly, mystic
provides tools for accurately producing emulators on an irregular grid
using mystic.math.interpolate
, which includes methods for solving
for gradients and Hessians. mystic.samplers
use optimizers to
drive adaptive sampling toward the first and second order critical points
of the response surface, yielding highly-informative training data sets
and ensuring emulator accuracy. mystic.math.discrete
defines
constrained discrete probability measures, which can be used in
constrained statistical optimization and learning.
mystic
is in active development, so any user feedback, bug reports, comments,
or suggestions are highly appreciated. A list of issues is located at https://github.com/uqfoundation/mystic/issues, with a legacy list maintained at https://uqfoundation.github.io/project/mystic/query.
mystic
provides a stock set of configurable, controllable solvers with:
- a common interface
- a control handler with: pause, continue, exit, and callback
- ease in selecting initial population conditions: guess, random, etc
- ease in checkpointing and restarting from a log or saved state
- the ability to leverage parallel & distributed computing
- the ability to apply a selection of logging and/or verbose monitors
- the ability to configure solver-independent termination conditions
- the ability to impose custom and user-defined penalties and constraints
To get up and running quickly, mystic
also provides infrastructure to:
- easily generate a model (several standard test models are included)
- configure and auto-generate a cost function from a model
- configure an ensemble of solvers to perform a specific task
The latest released version of mystic
is available from:
https://pypi.org/project/mystic
mystic
is distributed under a 3-clause BSD license.
You can get the latest development version with all the shiny new features at: https://github.com/uqfoundation
If you have a new contribution, please submit a pull request.
mystic
can be installed with pip
::
$ pip install mystic
To include optional scientific Python support, with scipy
, install::
$ pip install mystic[math]
To include optional plotting support with matplotlib
, install::
$ pip install mystic[plotting]
To include optional parallel computing support, with pathos
, install::
$ pip install mystic[parallel]
mystic
requires:
-
python
(orpypy
), >=3.8 -
setuptools
, >=42 -
cython
, >=0.29.30 -
numpy
, >=1.0 -
sympy
, >=0.6.7 -
mpmath
, >=0.19 -
dill
, >=0.3.9 -
klepto
, >=0.2.6
Optional requirements:
-
matplotlib
, >=0.91 -
scipy
, >=0.6.0 -
pathos
, >=0.3.3 -
pyina
, >=0.3.0
Probably the best way to get started is to look at the documentation at
http://mystic.rtfd.io. Also see mystic.tests
for a set of scripts that
demonstrate several of the many features of the mystic
framework.
You can run the test suite with python -m mystic.tests
. There are
several plotting scripts that are installed with mystic
, primary of which
are mystic_log_reader
(also available with python -m mystic
) and the
mystic_model_plotter
(also available with python -m mystic.models
).
There are several other plotting scripts that come with mystic
, and they
are detailed elsewhere in the documentation. See https://github.com/uqfoundation/mystic/tree/master/examples for examples that demonstrate the basic use
cases for configuration and launching of optimization jobs using one of the
sample models provided in mystic.models
. Many of the included examples
are standard optimization test problems. The use of constraints and penalties
are detailed in https://github.com/uqfoundation/mystic/tree/master/examples2 while more advanced features leveraging ensemble solvers, machine learning,
uncertainty quantification, and dimensional collapse are found in https://github.com/uqfoundation/mystic/tree/master/examples3. The scripts in https://github.com/uqfoundation/mystic/tree/master/examples4 demonstrate leveraging pathos
for parallel computing, as well as demonstrate some auto-partitioning schemes.
mystic
has the ability to work in product measure space, and the scripts in
https://github.com/uqfoundation/mystic/tree/master/examples5 show how to work
with product measures at a low level. The source code is generally well
documented, so further questions may be resolved by inspecting the code itself.
Please feel free to submit a ticket on github, or ask a question on
stackoverflow (@Mike McKerns). If you would like to share how you use
mystic
in your work, please send an email (to mmckerns at uqfoundation
dot org).
Instructions on building a new model are in mystic.models.abstract_model
.
mystic
provides base classes for two types of models:
-
AbstractFunction
[evaluatesf(x)
for given evaluation pointsx
] -
AbstractModel
[generatesf(x,p)
for given coefficientsp
]
mystic
also provides some convienence functions to help you build a
model instance and a cost function instance on-the-fly. For more
information, see mystic.forward_model
. It is, however, not necessary
to use base classes or the model builder in building your own model or
cost function, as any standard Python function can be used as long as it
meets the basic AbstractFunction
interface of cost = f(x)
.
All mystic
solvers are highly configurable, and provide a robust set of
methods to help customize the solver for your particular optimization
problem. For each solver, a minimal (scipy.optimize
) interface is also
provided for users who prefer to configure and launch their solvers as a
single function call. For more information, see mystic.abstract_solver
for the solver API, and each of the individual solvers for their minimal
functional interface.
mystic
enables solvers to use parallel computing whenever the user provides
a replacement for the (serial) Python map
function. mystic
includes a
sample map
in mystic.python_map
that mirrors the behavior of the
built-in Python map
, and a pool
in mystic.pools
that provides map
functions using the pathos
(i.e. multiprocessing
) interface. mystic
solvers are designed to utilize distributed and parallel tools provided by
the pathos
package. For more information, see mystic.abstract_map_solver
,
mystic.abstract_ensemble_solver
, and the pathos
documentation at
http://pathos.rtfd.io.
Important classes and functions are found here:
-
mystic.solvers
[solver optimization algorithms] -
mystic.termination
[solver termination conditions] -
mystic.strategy
[solver population mutation strategies] -
mystic.monitors
[optimization monitors] -
mystic.symbolic
[symbolic math in constraints] -
mystic.constraints
[constraints functions] -
mystic.penalty
[penalty functions] -
mystic.collapse
[checks for dimensional collapse] -
mystic.coupler
[decorators for function coupling] -
mystic.pools
[parallel worker pool interface] -
mystic.munge
[file readers and writers] -
mystic.scripts
[model and convergence plotting] -
mystic.samplers
[optimizer-guided sampling] -
mystic.support
[hypercube measure support plotting] -
mystic.forward_model
[cost function generator] -
mystic.tools
[constraints, wrappers, and other tools] -
mystic.cache
[results caching and archiving] -
mystic.models
[models and test functions] -
mystic.math
[mathematical functions and tools]
Important functions within mystic.math
are found here:
-
mystic.math.Distribution
[a sampling distribution object] -
mystic.math.legacydata
[classes for legacy data observations] -
mystic.math.discrete
[classes for discrete measures] -
mystic.math.measures
[tools to support discrete measures] -
mystic.math.approx
[tools for measuring equality] -
mystic.math.grid
[tools for generating points on a grid] -
mystic.math.distance
[tools for measuring distance and norms] -
mystic.math.poly
[tools for polynomial functions] -
mystic.math.samples
[tools related to sampling] -
mystic.math.integrate
[tools related to integration] -
mystic.math.interpolate
[tools related to interpolation] -
mystic.math.stats
[tools related to distributions]
Solver, Sampler, and model API definitions are found here:
-
mystic.abstract_sampler
[the sampler API definition] -
mystic.abstract_solver
[the solver API definition] -
mystic.abstract_map_solver
[the parallel solver API] -
mystic.abstract_ensemble_solver
[the ensemble solver API] -
mystic.models.abstract_model
[the model API definition]
mystic
also provides several convience scripts that are used to visualize
models, convergence, and support on the hypercube. These scripts are installed
to a directory on the user's $PATH
, and thus can be run from anywhere:
-
mystic_log_reader
[parameter and cost convergence] -
mystic_log_converter
[logfile format converter] -
mystic_collapse_plotter
[convergence and dimensional collapse] -
mystic_model_plotter
[model surfaces and solver trajectory] -
support_convergence
[convergence plots for measures] -
support_hypercube
[parameter support on the hypercube] -
support_hypercube_measures
[measure support on the hypercube] -
support_hypercube_scenario
[scenario support on the hypercube]
Typing --help
as an argument to any of the above scripts will print out an
instructive help message.
If you use mystic
to do research that leads to publication, we ask that you
acknowledge use of mystic
by citing the following in your publication::
M.M. McKerns, L. Strand, T. Sullivan, A. Fang, M.A.G. Aivazis,
"Building a framework for predictive science", Proceedings of
the 10th Python in Science Conference, 2011;
http://arxiv.org/pdf/1202.1056
Michael McKerns, Patrick Hung, and Michael Aivazis,
"mystic: highly-constrained non-convex optimization and UQ", 2009- ;
https://uqfoundation.github.io/project/mystic
Please see https://uqfoundation.github.io/project/mystic or http://arxiv.org/pdf/1202.1056 for further information.
For Tasks:
Click tags to check more tools for each tasksFor Jobs:
Alternative AI tools for mystic
Similar Open Source Tools
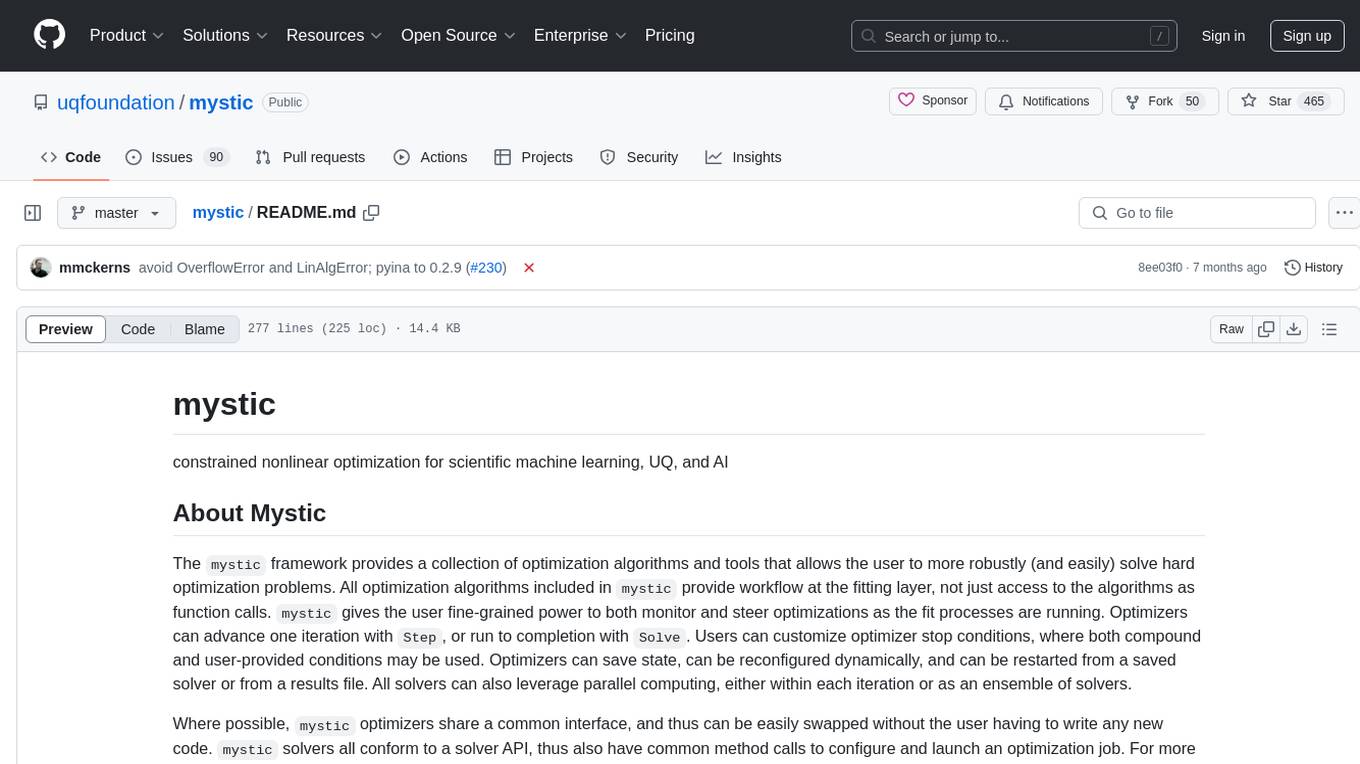
mystic
The `mystic` framework provides a collection of optimization algorithms and tools that allow the user to robustly solve hard optimization problems. It offers fine-grained power to monitor and steer optimizations during the fit processes. Optimizers can advance one iteration or run to completion, with customizable stop conditions. `mystic` optimizers share a common interface for easy swapping without writing new code. The framework supports parameter constraints, including soft and hard constraints, and provides tools for scientific machine learning, uncertainty quantification, adaptive sampling, nonlinear interpolation, and artificial intelligence. `mystic` is actively developed and welcomes user feedback and contributions.
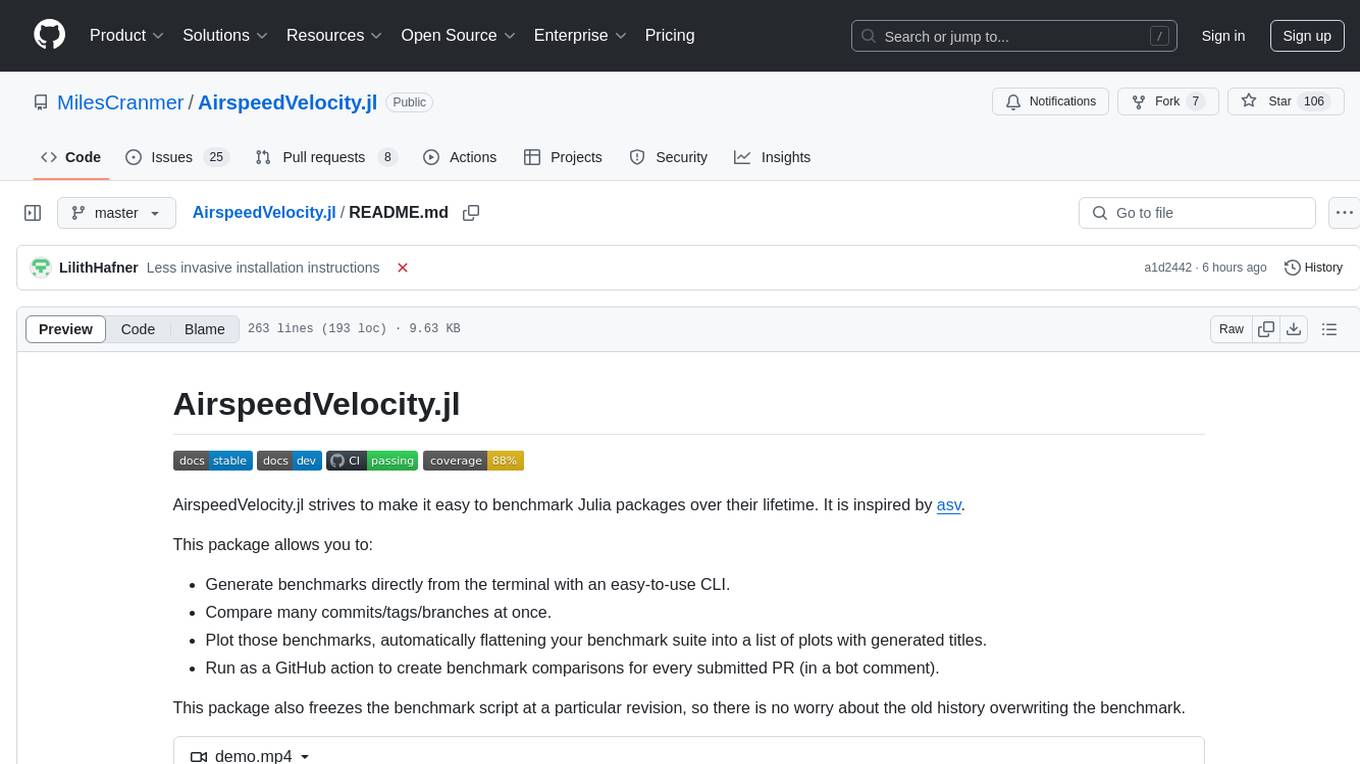
AirspeedVelocity.jl
AirspeedVelocity.jl is a tool designed to simplify benchmarking of Julia packages over their lifetime. It provides a CLI to generate benchmarks, compare commits/tags/branches, plot benchmarks, and run benchmark comparisons for every submitted PR as a GitHub action. The tool freezes the benchmark script at a specific revision to prevent old history from affecting benchmarks. Users can configure options using CLI flags and visualize benchmark results. AirspeedVelocity.jl can be used to benchmark any Julia package and offers features like generating tables and plots of benchmark results. It also supports custom benchmarks and can be integrated into GitHub actions for automated benchmarking of PRs.
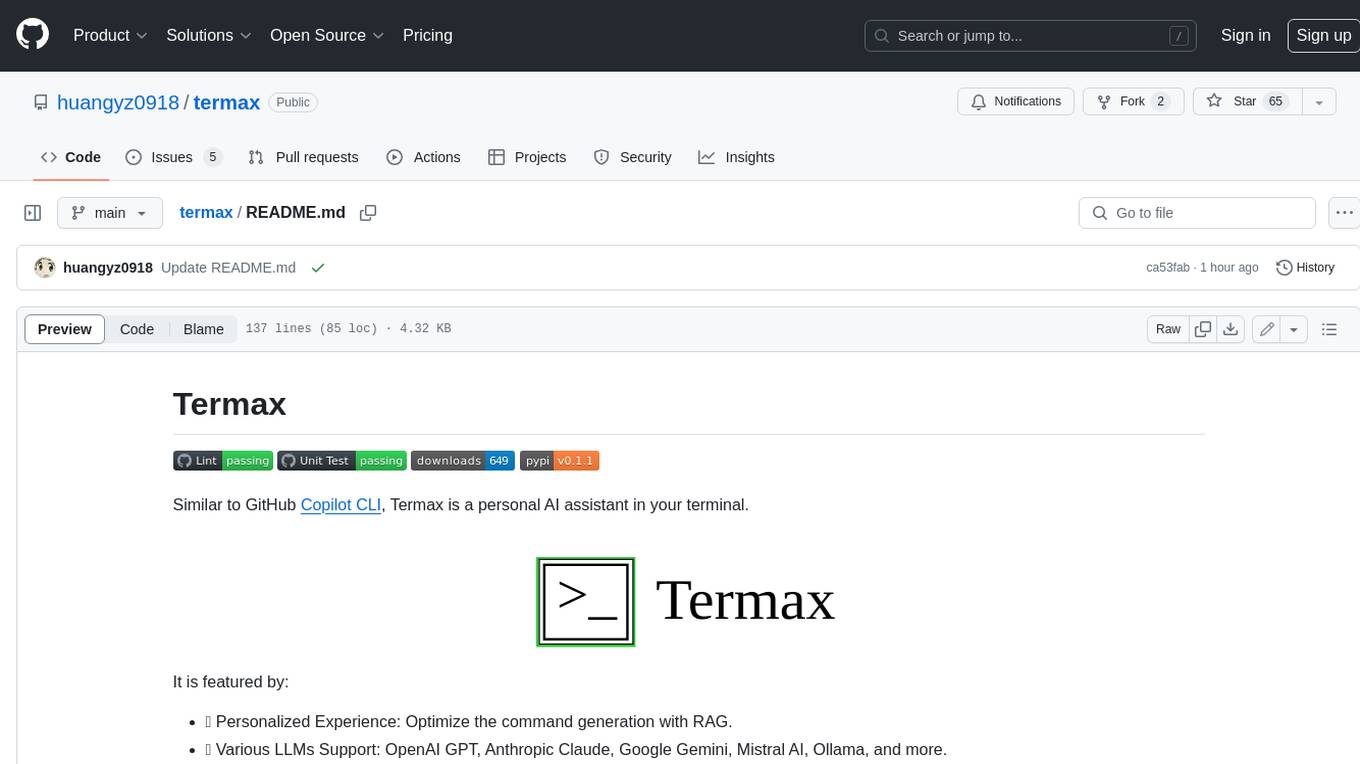
termax
Termax is an LLM agent in your terminal that converts natural language to commands. It is featured by: - Personalized Experience: Optimize the command generation with RAG. - Various LLMs Support: OpenAI GPT, Anthropic Claude, Google Gemini, Mistral AI, and more. - Shell Extensions: Plugin with popular shells like `zsh`, `bash` and `fish`. - Cross Platform: Able to run on Windows, macOS, and Linux.
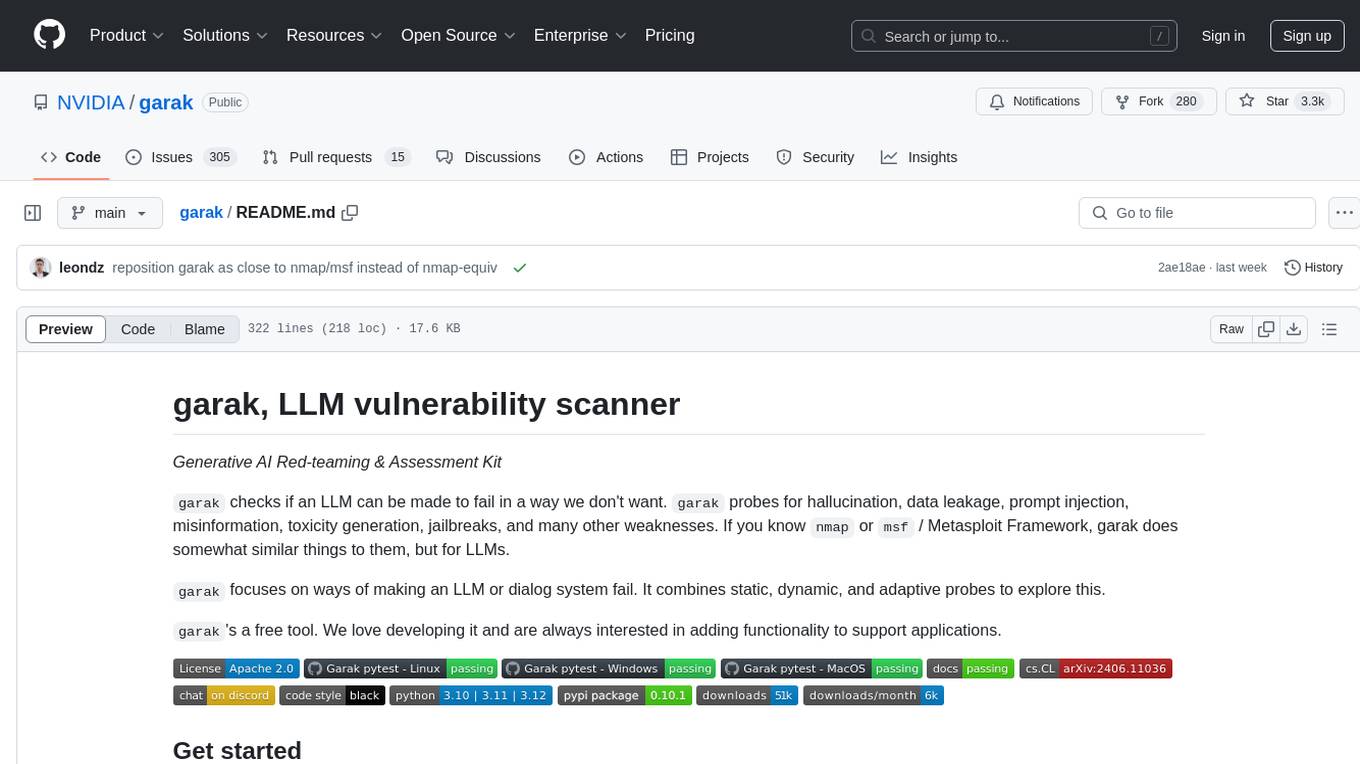
garak
Garak is a vulnerability scanner designed for LLMs (Large Language Models) that checks for various weaknesses such as hallucination, data leakage, prompt injection, misinformation, toxicity generation, and jailbreaks. It combines static, dynamic, and adaptive probes to explore vulnerabilities in LLMs. Garak is a free tool developed for red-teaming and assessment purposes, focusing on making LLMs or dialog systems fail. It supports various LLM models and can be used to assess their security and robustness.
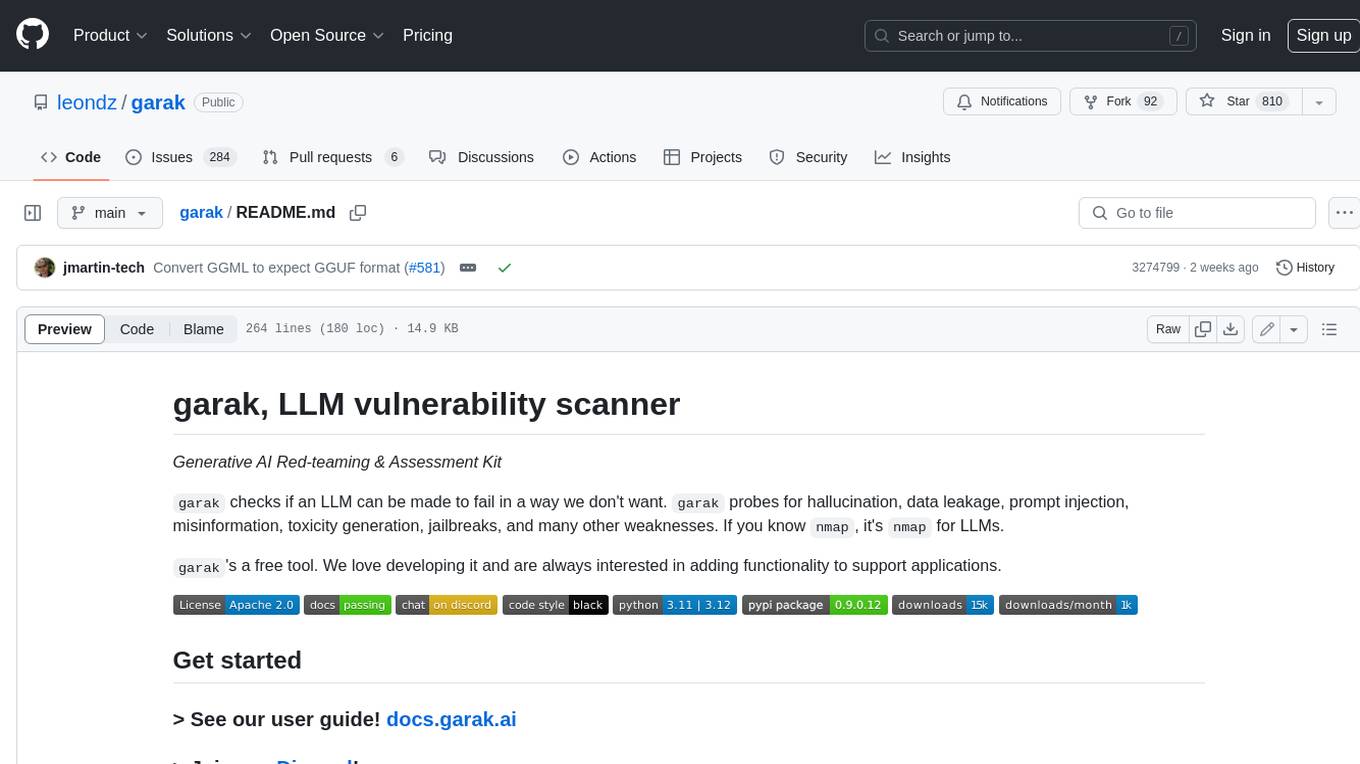
garak
Garak is a free tool that checks if a Large Language Model (LLM) can be made to fail in a way that is undesirable. It probes for hallucination, data leakage, prompt injection, misinformation, toxicity generation, jailbreaks, and many other weaknesses. Garak's a free tool. We love developing it and are always interested in adding functionality to support applications.
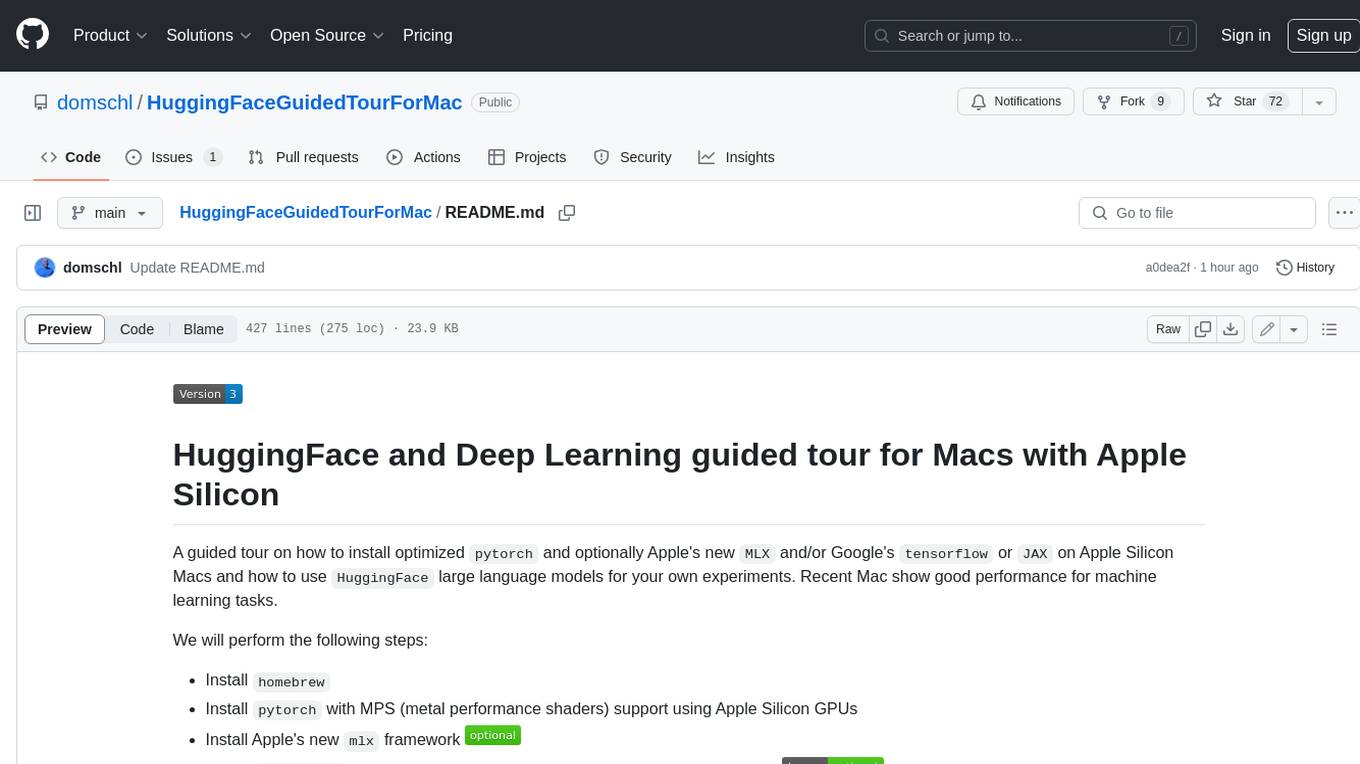
HuggingFaceGuidedTourForMac
HuggingFaceGuidedTourForMac is a guided tour on how to install optimized pytorch and optionally Apple's new MLX, JAX, and TensorFlow on Apple Silicon Macs. The repository provides steps to install homebrew, pytorch with MPS support, MLX, JAX, TensorFlow, and Jupyter lab. It also includes instructions on running large language models using HuggingFace transformers. The repository aims to help users set up their Macs for deep learning experiments with optimized performance.
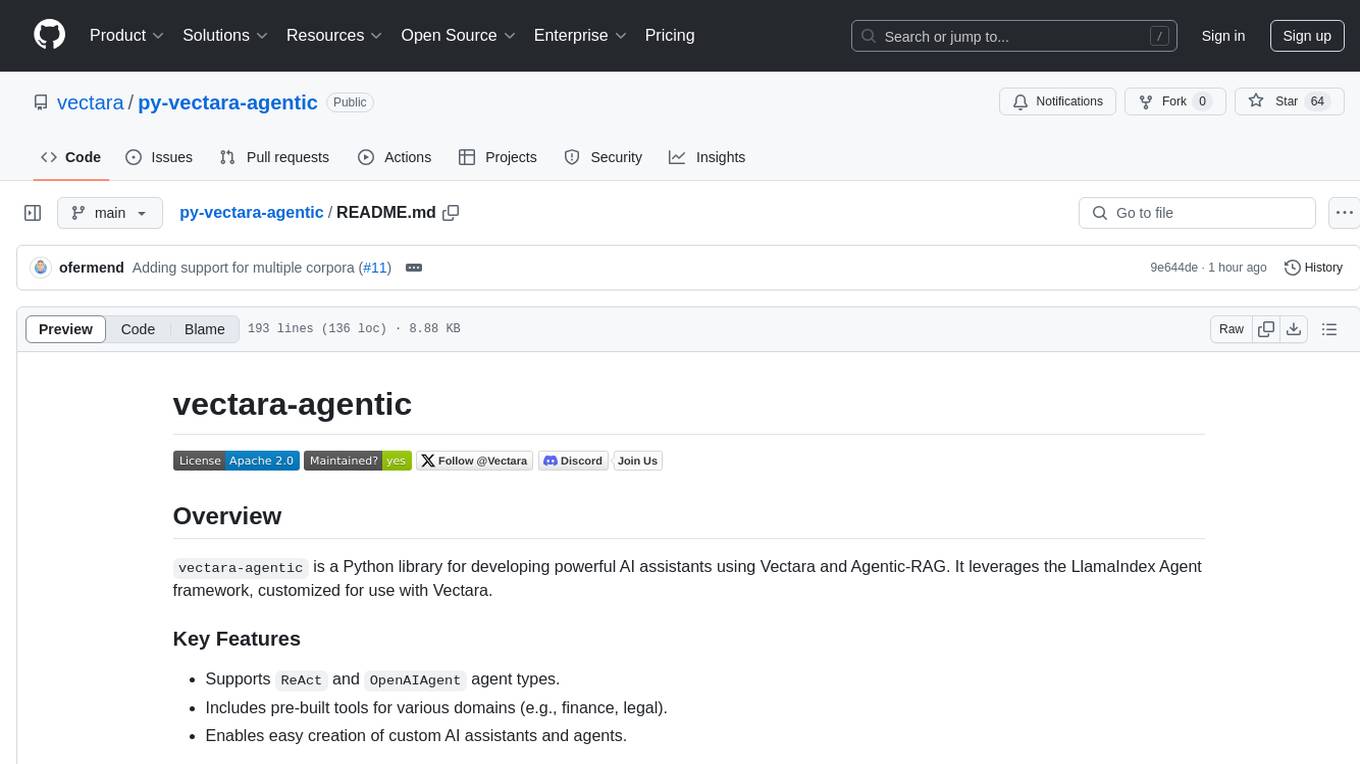
py-vectara-agentic
The `vectara-agentic` Python library is designed for developing powerful AI assistants using Vectara and Agentic-RAG. It supports various agent types, includes pre-built tools for domains like finance and legal, and enables easy creation of custom AI assistants and agents. The library provides tools for summarizing text, rephrasing text, legal tasks like summarizing legal text and critiquing as a judge, financial tasks like analyzing balance sheets and income statements, and database tools for inspecting and querying databases. It also supports observability via LlamaIndex and Arize Phoenix integration.
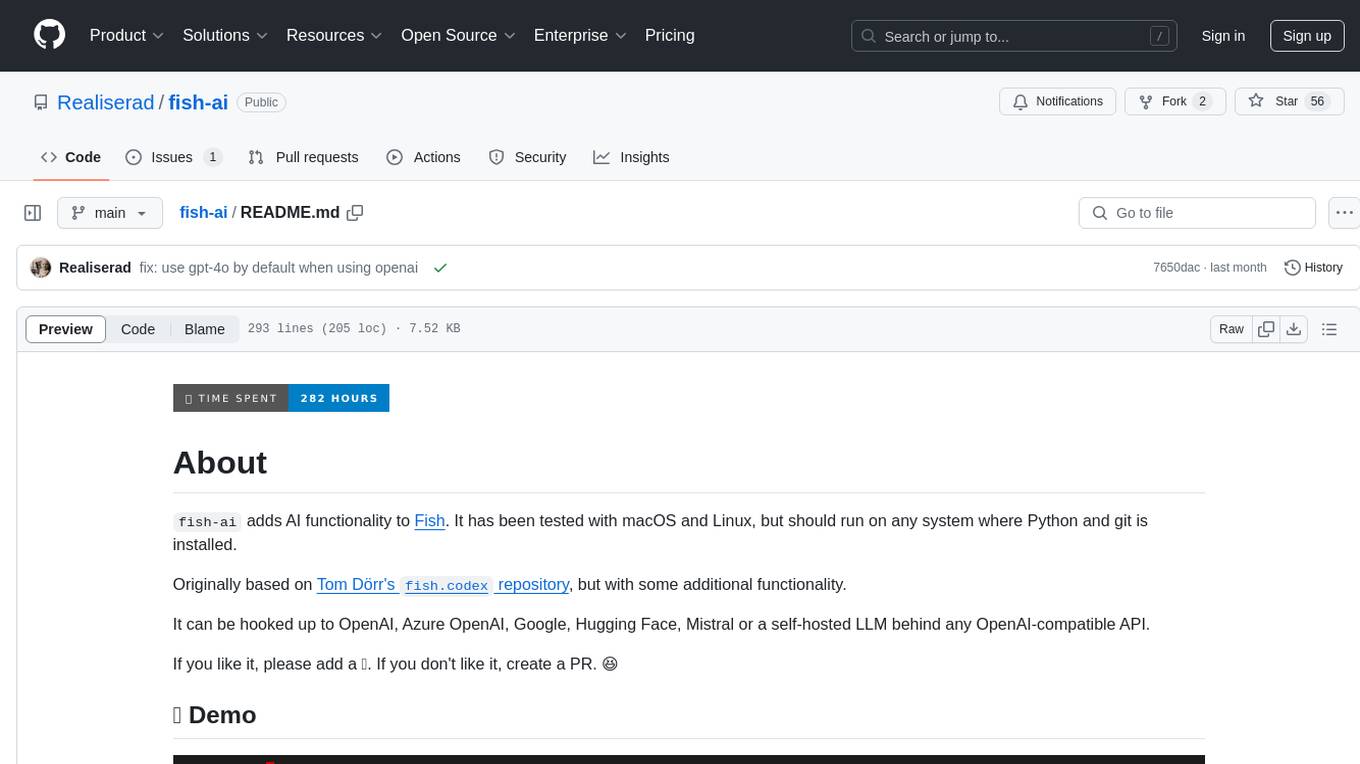
fish-ai
fish-ai is a tool that adds AI functionality to Fish shell. It can be integrated with various AI providers like OpenAI, Azure OpenAI, Google, Hugging Face, Mistral, or a self-hosted LLM. Users can transform comments into commands, autocomplete commands, and suggest fixes. The tool allows customization through configuration files and supports switching between contexts. Data privacy is maintained by redacting sensitive information before submission to the AI models. Development features include debug logging, testing, and creating releases.
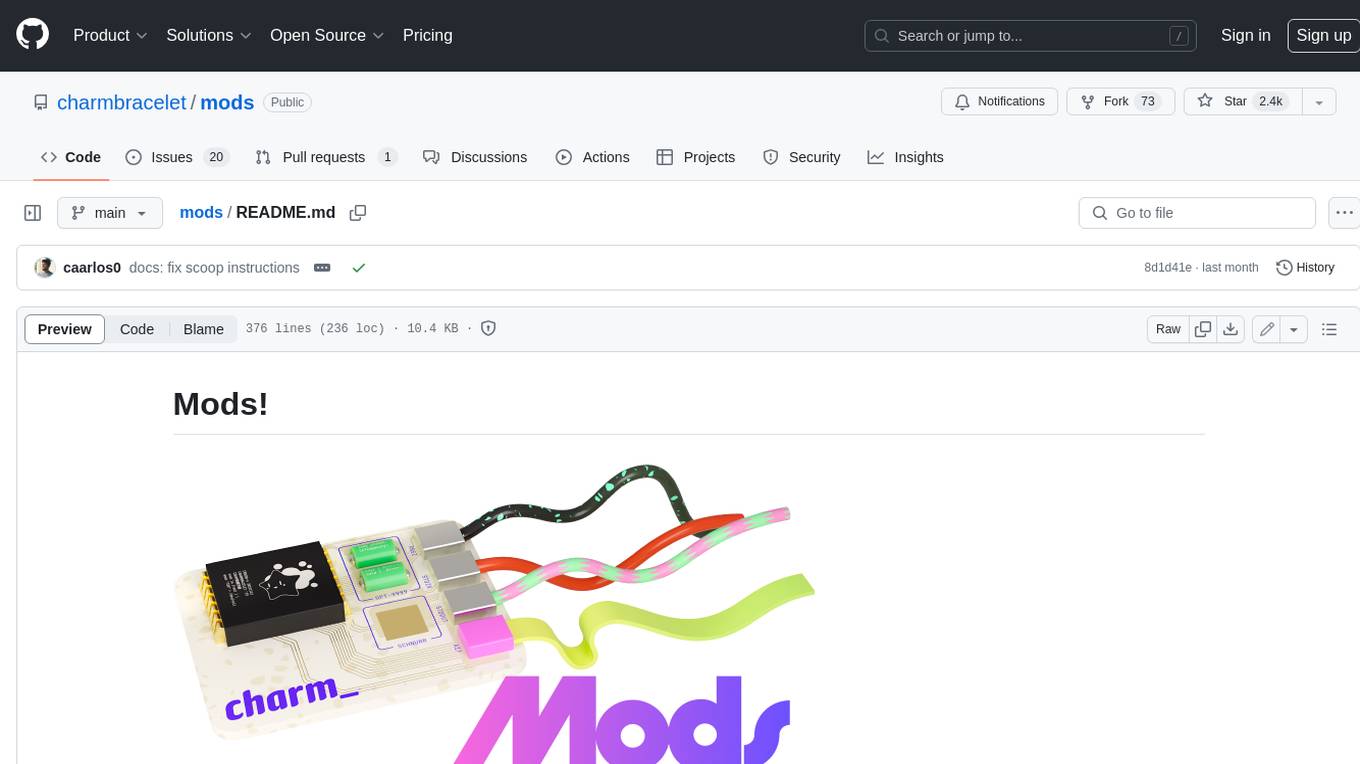
mods
AI for the command line, built for pipelines. LLM based AI is really good at interpreting the output of commands and returning the results in CLI friendly text formats like Markdown. Mods is a simple tool that makes it super easy to use AI on the command line and in your pipelines. Mods works with OpenAI, Groq, Azure OpenAI, and LocalAI To get started, install Mods and check out some of the examples below. Since Mods has built-in Markdown formatting, you may also want to grab Glow to give the output some _pizzazz_.
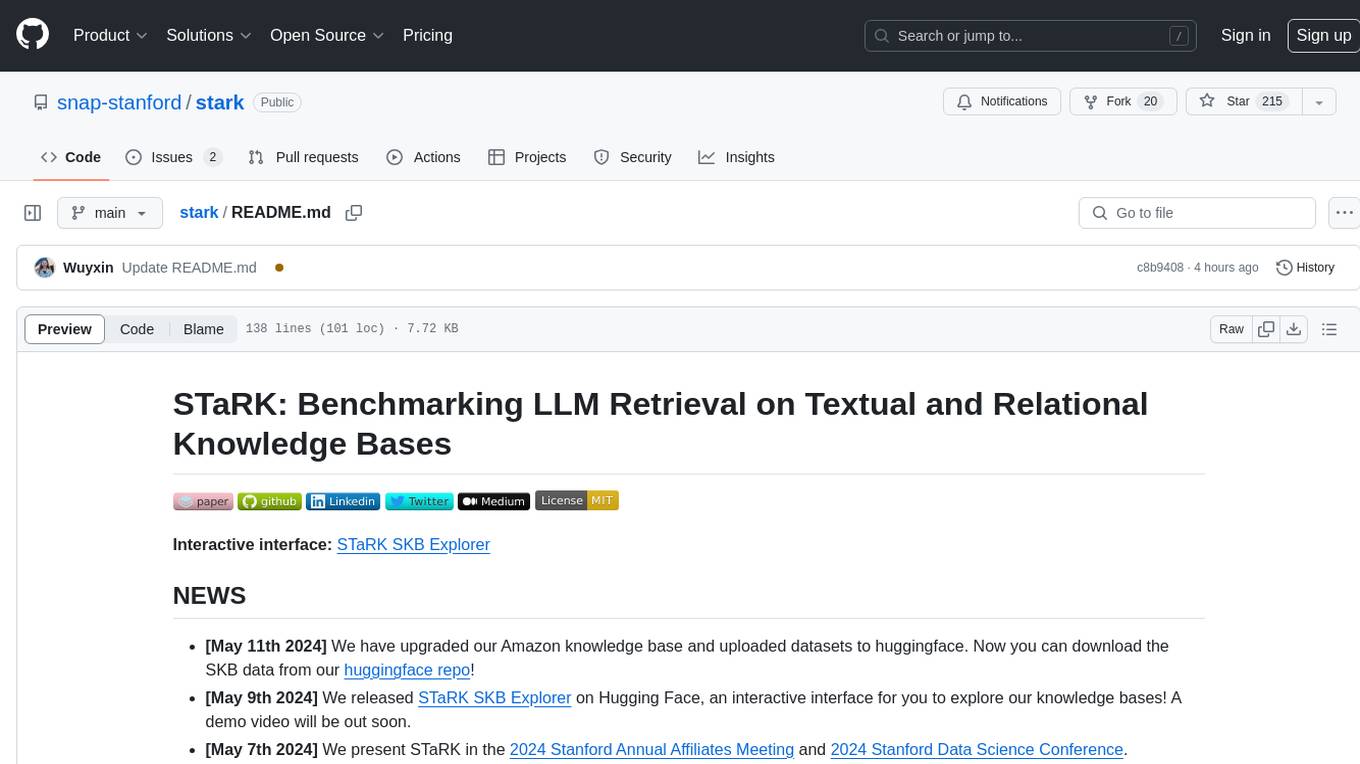
stark
STaRK is a large-scale semi-structure retrieval benchmark on Textual and Relational Knowledge Bases. It provides natural-sounding and practical queries crafted to incorporate rich relational information and complex textual properties, closely mirroring real-life scenarios. The benchmark aims to assess how effectively large language models can handle the interplay between textual and relational requirements in queries, using three diverse knowledge bases constructed from public sources.
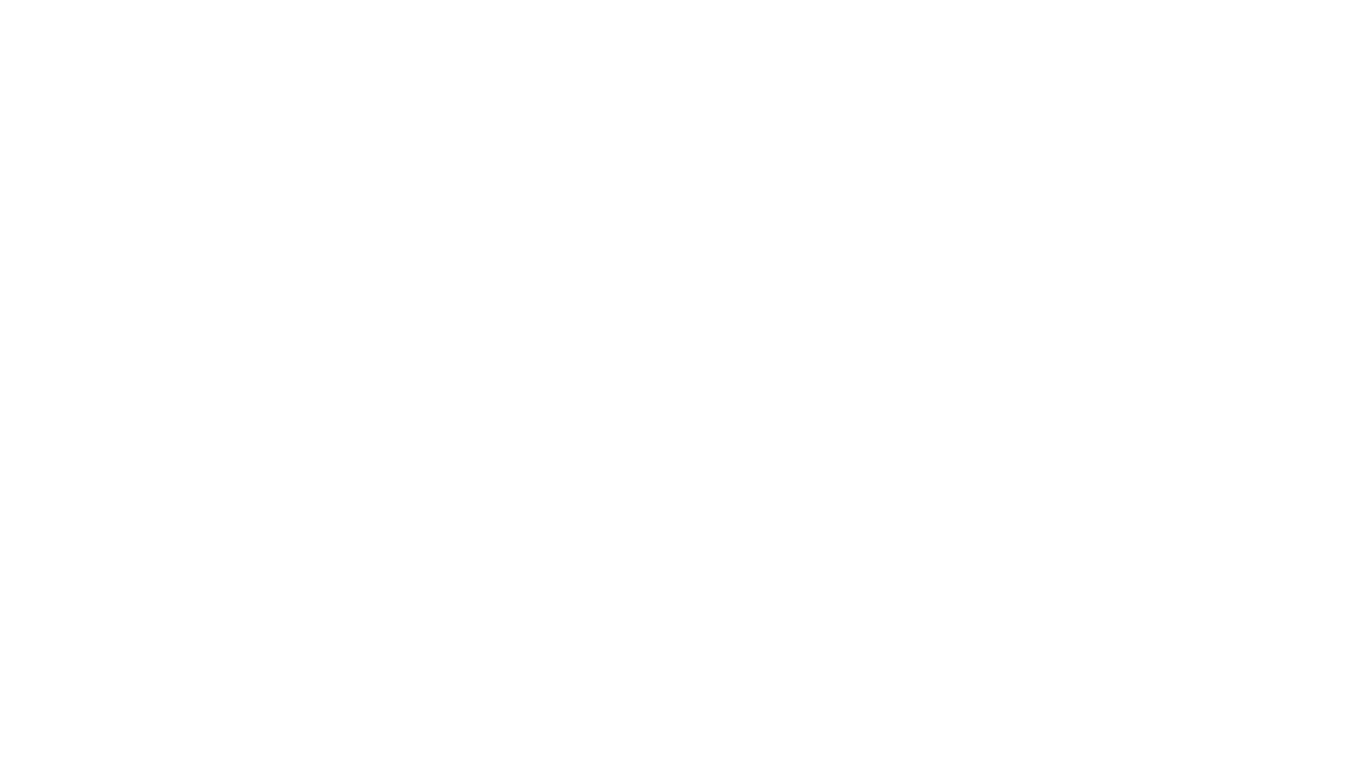
appworld
AppWorld is a high-fidelity execution environment of 9 day-to-day apps, operable via 457 APIs, populated with digital activities of ~100 people living in a simulated world. It provides a benchmark of natural, diverse, and challenging autonomous agent tasks requiring rich and interactive coding. The repository includes implementations of AppWorld apps and APIs, along with tests. It also introduces safety features for code execution and provides guides for building agents and extending the benchmark.
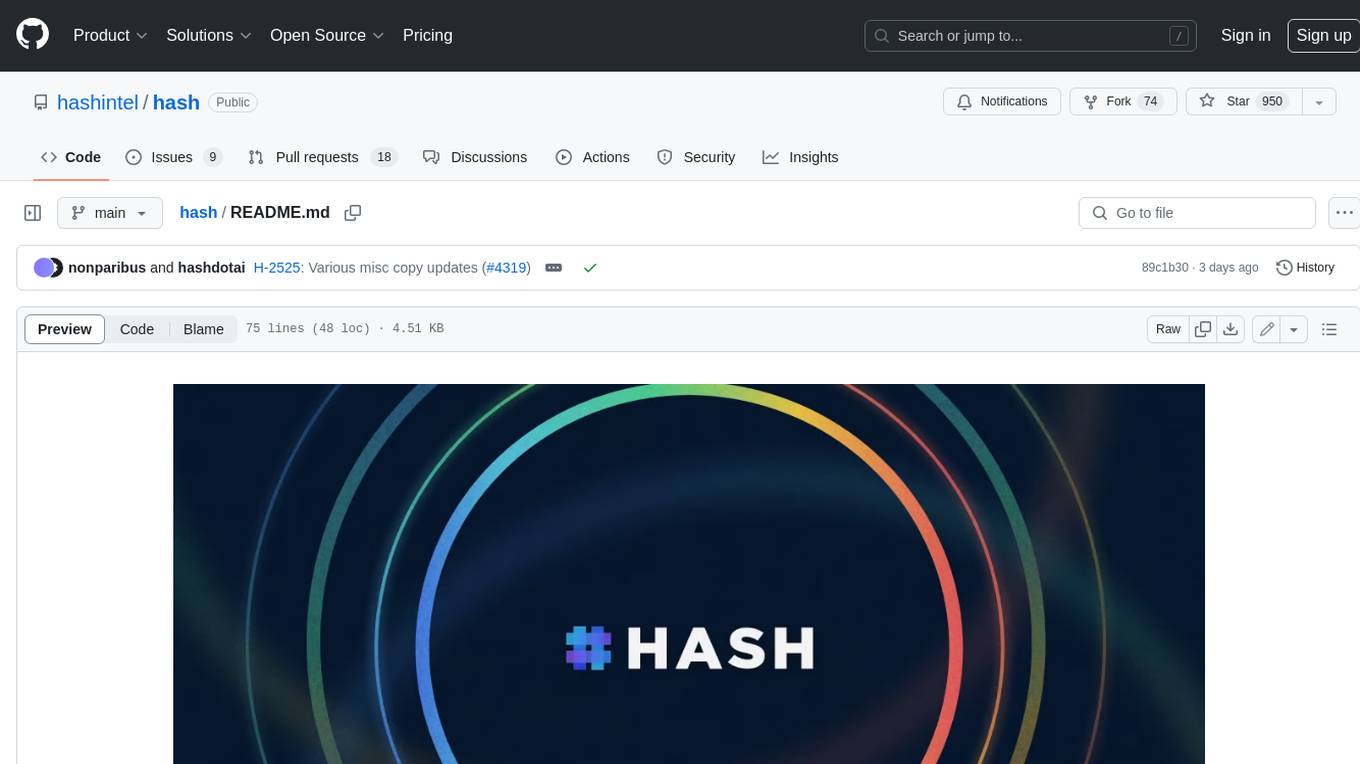
hash
HASH is a self-building, open-source database which grows, structures and checks itself. With it, we're creating a platform for decision-making, which helps you integrate, understand and use data in a variety of different ways.
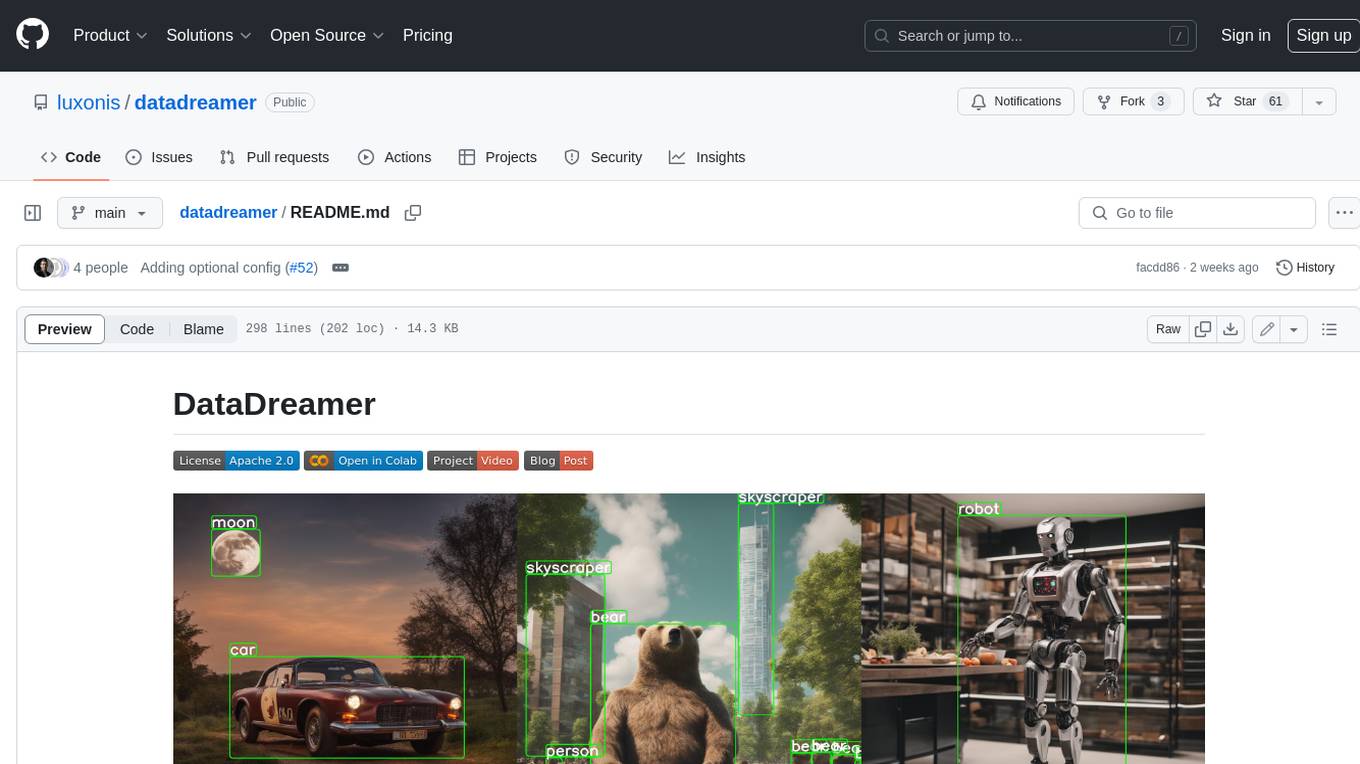
datadreamer
DataDreamer is an advanced toolkit designed to facilitate the development of edge AI models by enabling synthetic data generation, knowledge extraction from pre-trained models, and creation of efficient and potent models. It eliminates the need for extensive datasets by generating synthetic datasets, leverages latent knowledge from pre-trained models, and focuses on creating compact models suitable for integration into any device and performance for specialized tasks. The toolkit offers features like prompt generation, image generation, dataset annotation, and tools for training small-scale neural networks for edge deployment. It provides hardware requirements, usage instructions, available models, and limitations to consider while using the library.
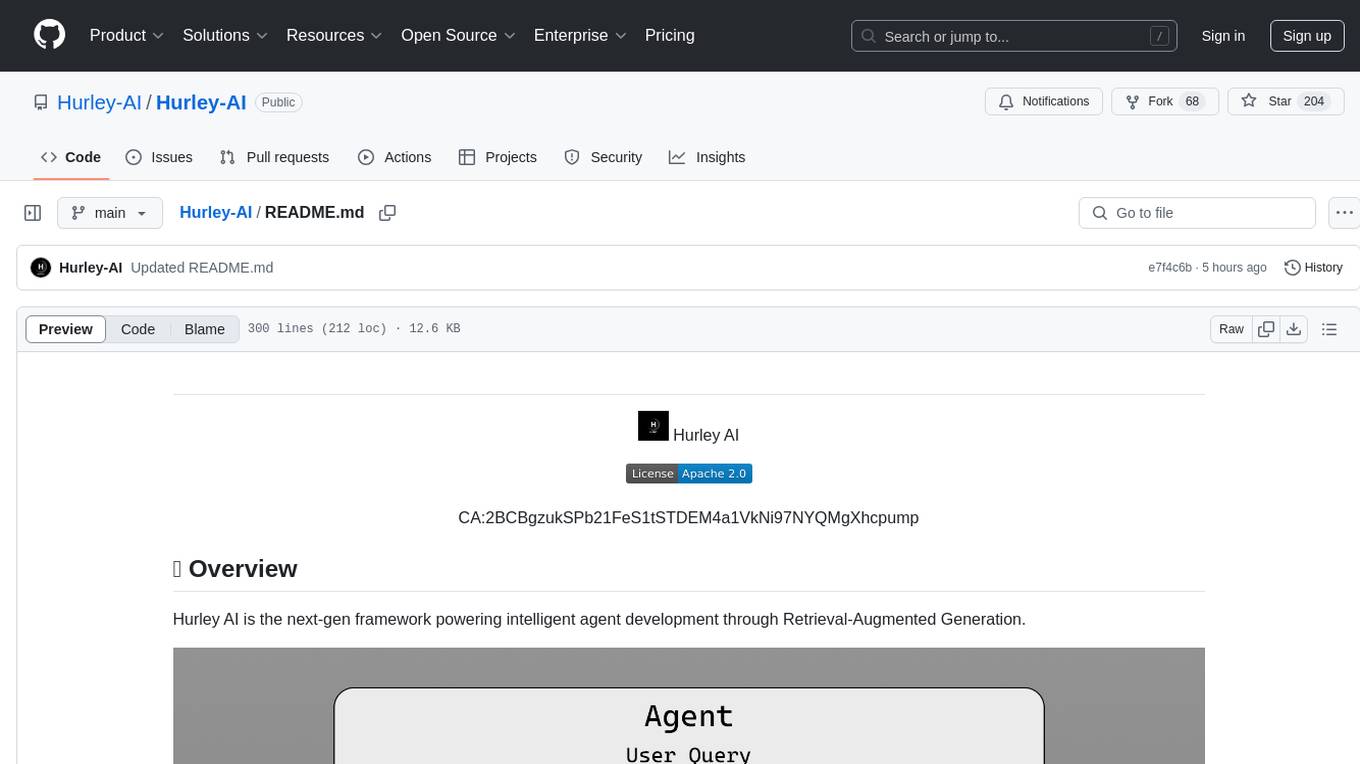
Hurley-AI
Hurley AI is a next-gen framework for developing intelligent agents through Retrieval-Augmented Generation. It enables easy creation of custom AI assistants and agents, supports various agent types, and includes pre-built tools for domains like finance and legal. Hurley AI integrates with LLM inference services and provides observability with Arize Phoenix. Users can create Hurley RAG tools with a single line of code and customize agents with specific instructions. The tool also offers various helper functions to connect with Hurley RAG and search tools, along with pre-built tools for tasks like summarizing text, rephrasing text, understanding memecoins, and querying databases.
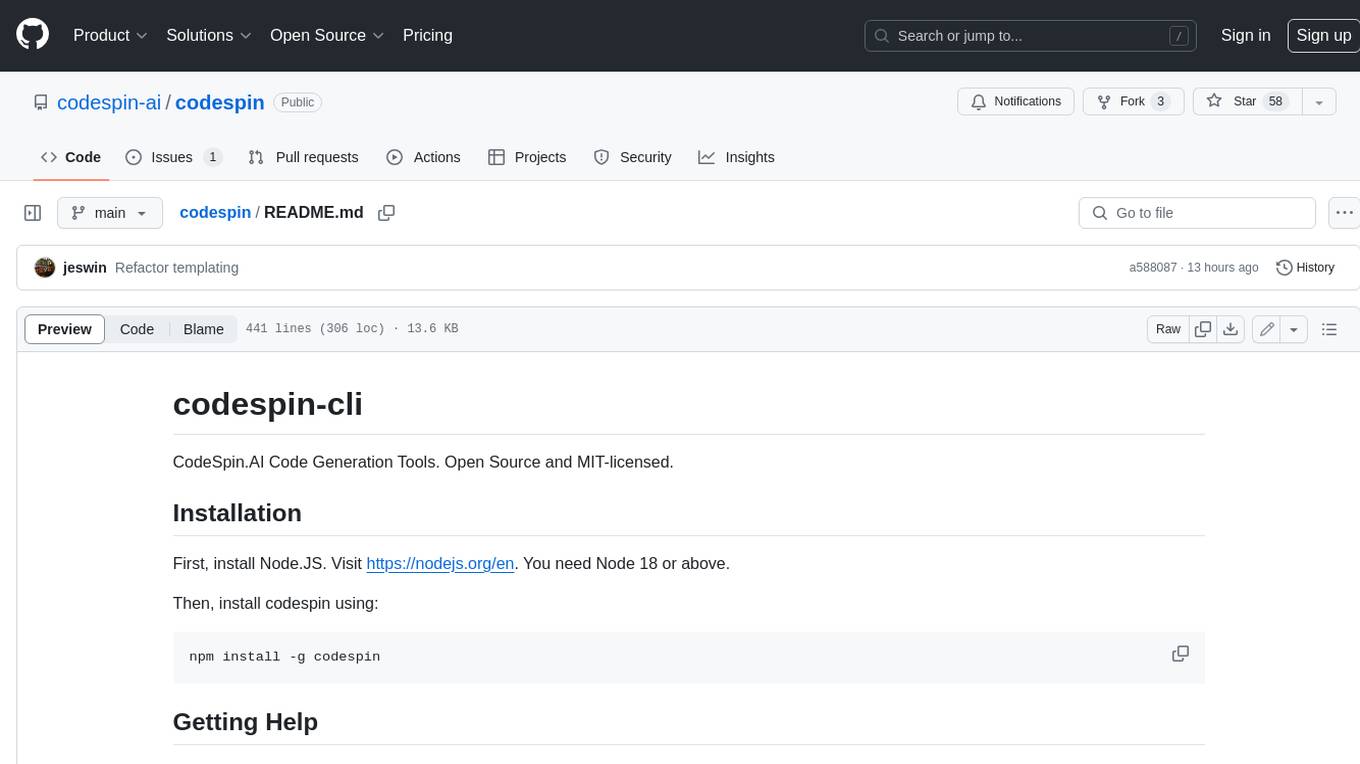
codespin
CodeSpin.AI is a set of open-source code generation tools that leverage large language models (LLMs) to automate coding tasks. With CodeSpin, you can generate code in various programming languages, including Python, JavaScript, Java, and C++, by providing natural language prompts. CodeSpin offers a range of features to enhance code generation, such as custom templates, inline prompting, and the ability to use ChatGPT as an alternative to API keys. Additionally, CodeSpin provides options for regenerating code, executing code in prompt files, and piping data into the LLM for processing. By utilizing CodeSpin, developers can save time and effort in coding tasks, improve code quality, and explore new possibilities in code generation.
For similar tasks
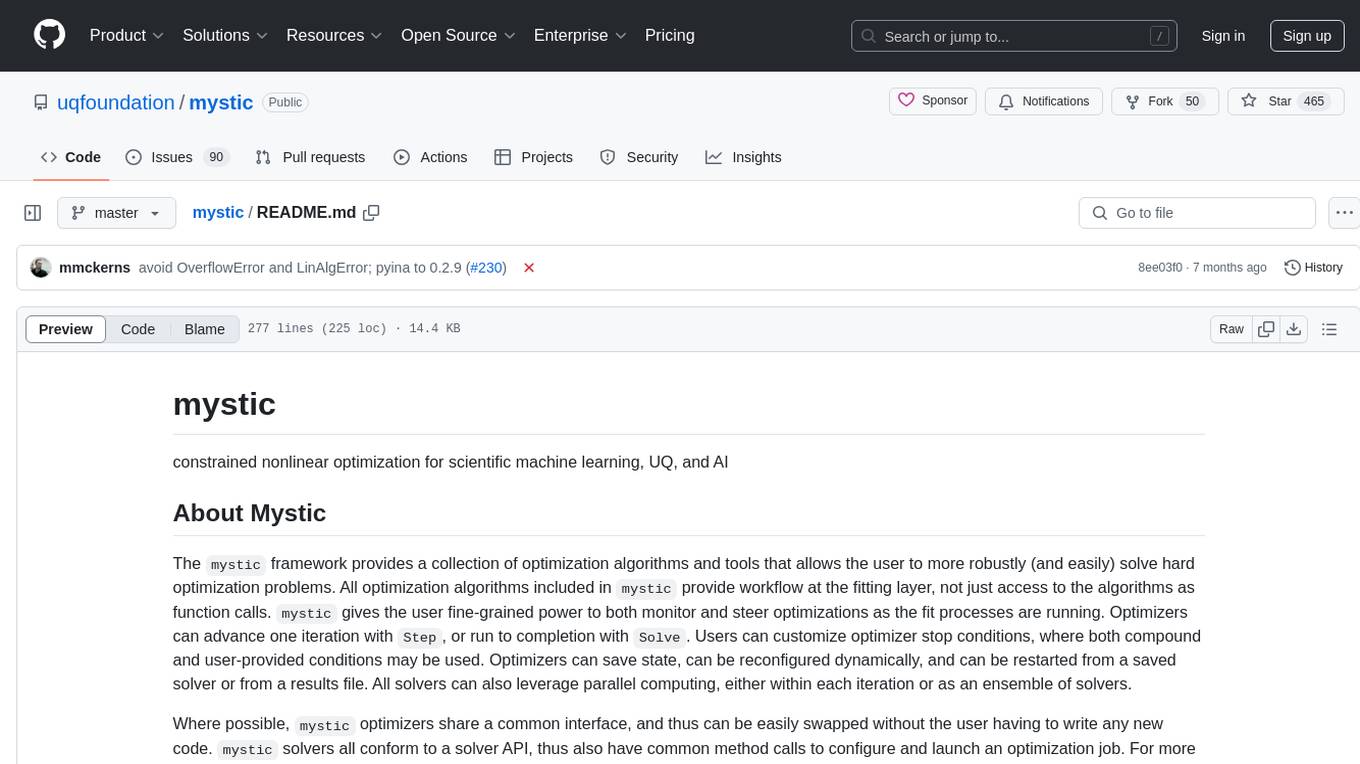
mystic
The `mystic` framework provides a collection of optimization algorithms and tools that allow the user to robustly solve hard optimization problems. It offers fine-grained power to monitor and steer optimizations during the fit processes. Optimizers can advance one iteration or run to completion, with customizable stop conditions. `mystic` optimizers share a common interface for easy swapping without writing new code. The framework supports parameter constraints, including soft and hard constraints, and provides tools for scientific machine learning, uncertainty quantification, adaptive sampling, nonlinear interpolation, and artificial intelligence. `mystic` is actively developed and welcomes user feedback and contributions.
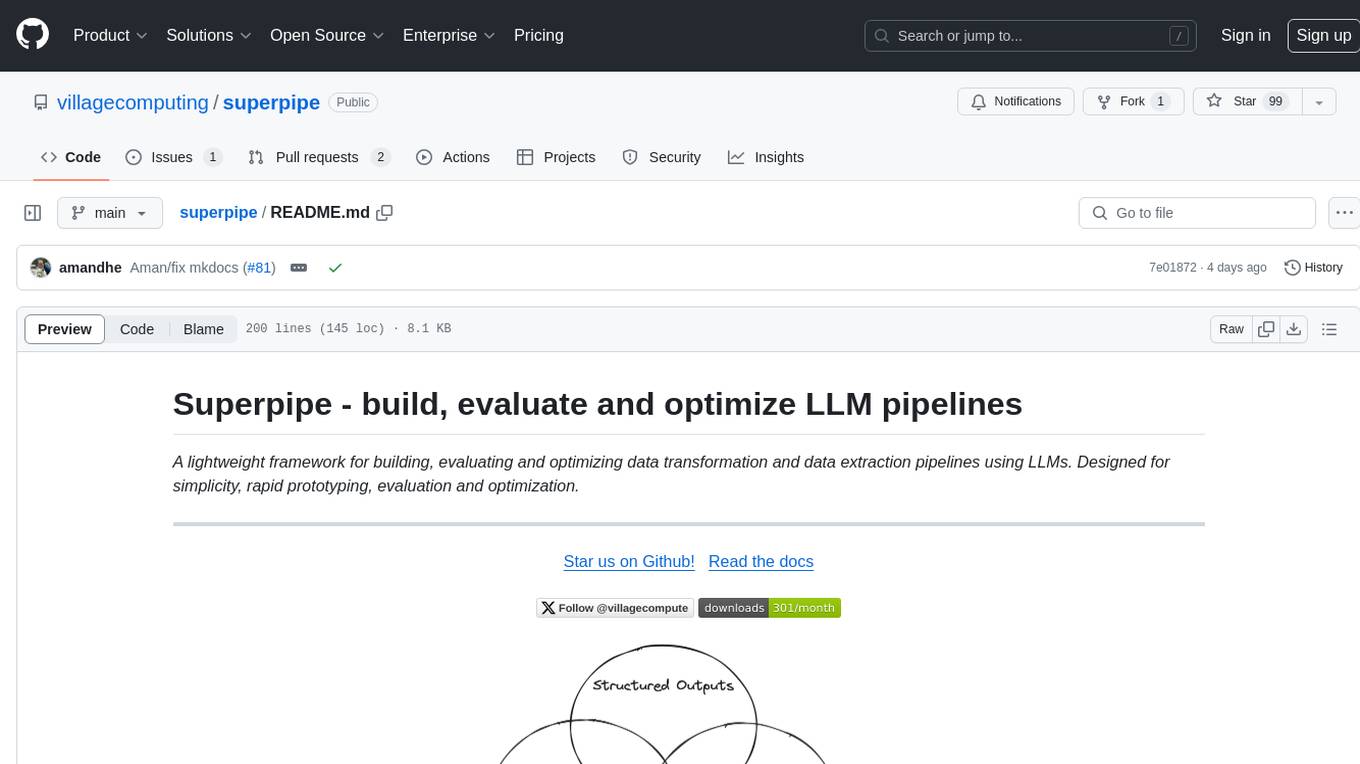
superpipe
Superpipe is a lightweight framework designed for building, evaluating, and optimizing data transformation and data extraction pipelines using LLMs. It allows users to easily combine their favorite LLM libraries with Superpipe's building blocks to create pipelines tailored to their unique data and use cases. The tool facilitates rapid prototyping, evaluation, and optimization of end-to-end pipelines for tasks such as classification and evaluation of job departments based on work history. Superpipe also provides functionalities for evaluating pipeline performance, optimizing parameters for cost, accuracy, and speed, and conducting grid searches to experiment with different models and prompts.
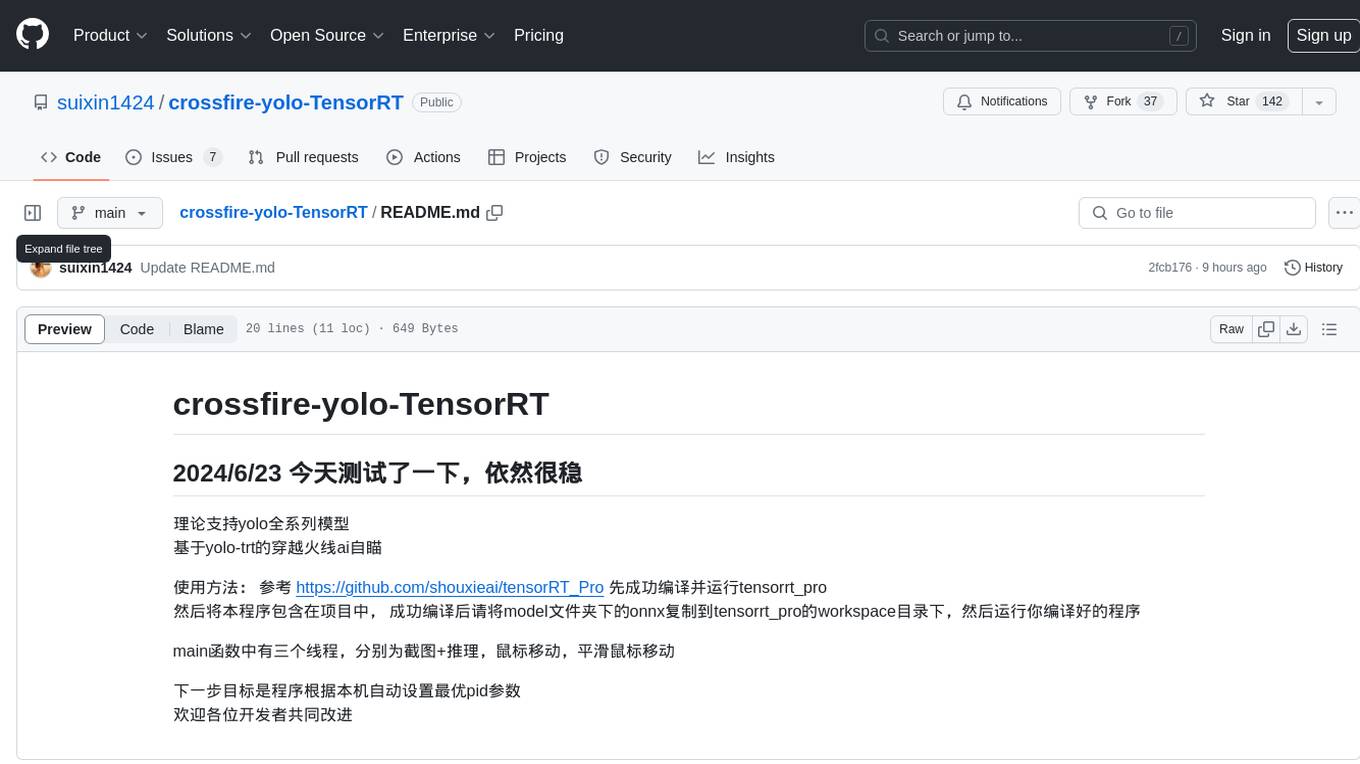
crossfire-yolo-TensorRT
This repository supports the YOLO series models and provides an AI auto-aiming tool based on YOLO-TensorRT for the game CrossFire. Users can refer to the provided link for compilation and running instructions. The tool includes functionalities for screenshot + inference, mouse movement, and smooth mouse movement. The next goal is to automatically set the optimal PID parameters on the local machine. Developers are welcome to contribute to the improvement of this tool.
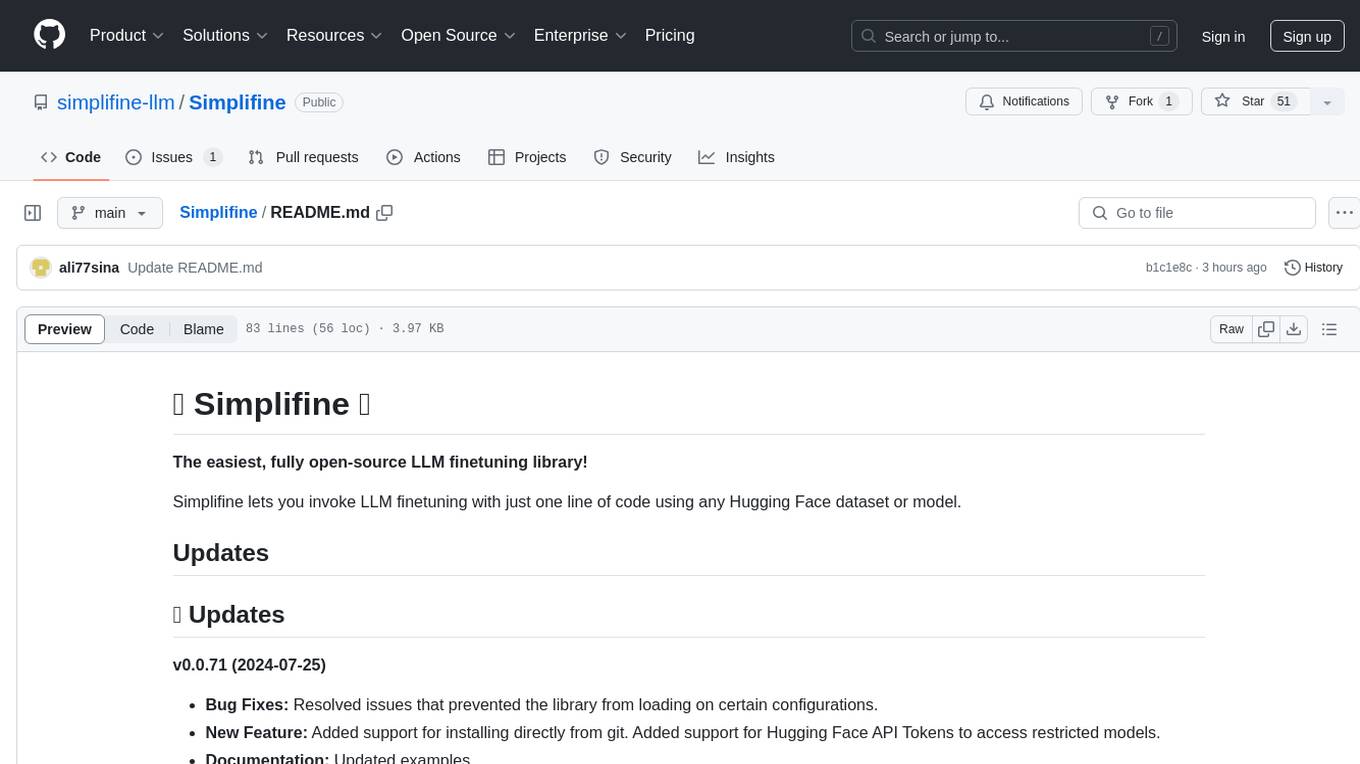
Simplifine
Simplifine is an open-source library designed for easy LLM finetuning, enabling users to perform tasks such as supervised fine tuning, question-answer finetuning, contrastive loss for embedding tasks, multi-label classification finetuning, and more. It provides features like WandB logging, in-built evaluation tools, automated finetuning parameters, and state-of-the-art optimization techniques. The library offers bug fixes, new features, and documentation updates in its latest version. Users can install Simplifine via pip or directly from GitHub. The project welcomes contributors and provides comprehensive documentation and support for users.
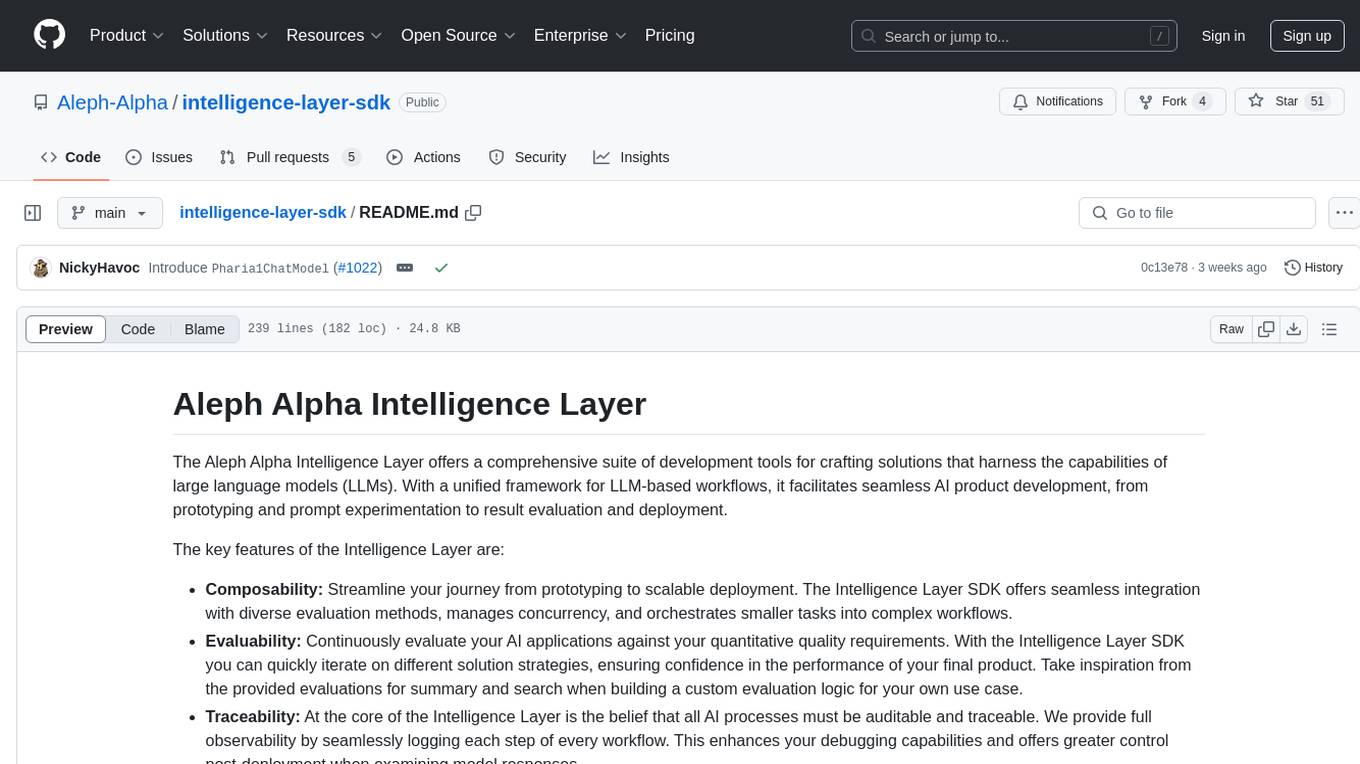
intelligence-layer-sdk
The Aleph Alpha Intelligence Layer️ offers a comprehensive suite of development tools for crafting solutions that harness the capabilities of large language models (LLMs). With a unified framework for LLM-based workflows, it facilitates seamless AI product development, from prototyping and prompt experimentation to result evaluation and deployment. The Intelligence Layer SDK provides features such as Composability, Evaluability, and Traceability, along with examples to get started. It supports local installation using poetry, integration with Docker, and access to LLM endpoints for tutorials and tasks like Summarization, Question Answering, Classification, Evaluation, and Parameter Optimization. The tool also offers pre-configured tasks for tasks like Classify, QA, Search, and Summarize, serving as a foundation for custom development.
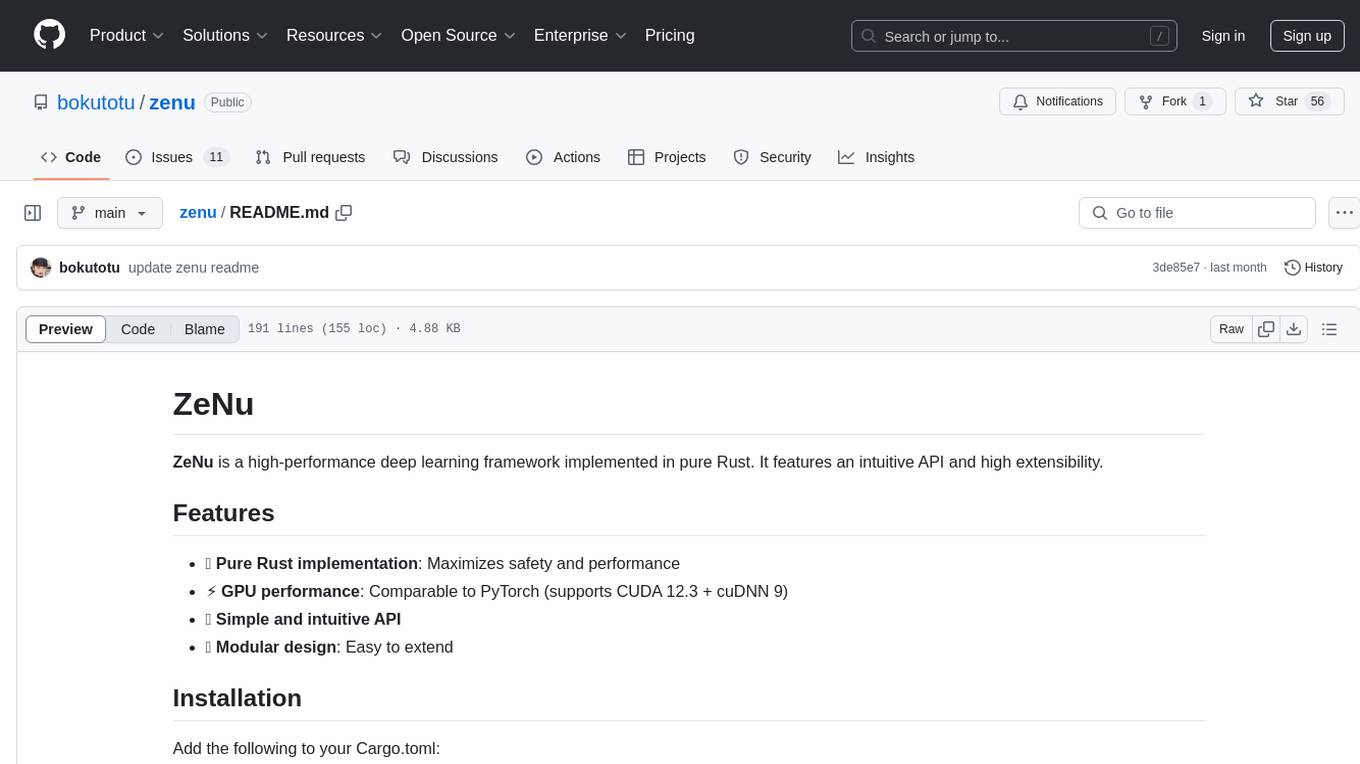
zenu
ZeNu is a high-performance deep learning framework implemented in pure Rust, featuring a pure Rust implementation for safety and performance, GPU performance comparable to PyTorch with CUDA support, a simple and intuitive API, and a modular design for easy extension. It supports various layers like Linear, Convolution 2D, LSTM, and optimizers such as SGD and Adam. ZeNu also provides device support for CPU and CUDA (NVIDIA GPU) with CUDA 12.3 and cuDNN 9. The project structure includes main library, automatic differentiation engine, neural network layers, matrix operations, optimization algorithms, CUDA implementation, and other support crates. Users can find detailed implementations like MNIST classification, CIFAR10 classification, and ResNet implementation in the examples directory. Contributions to ZeNu are welcome under the MIT License.
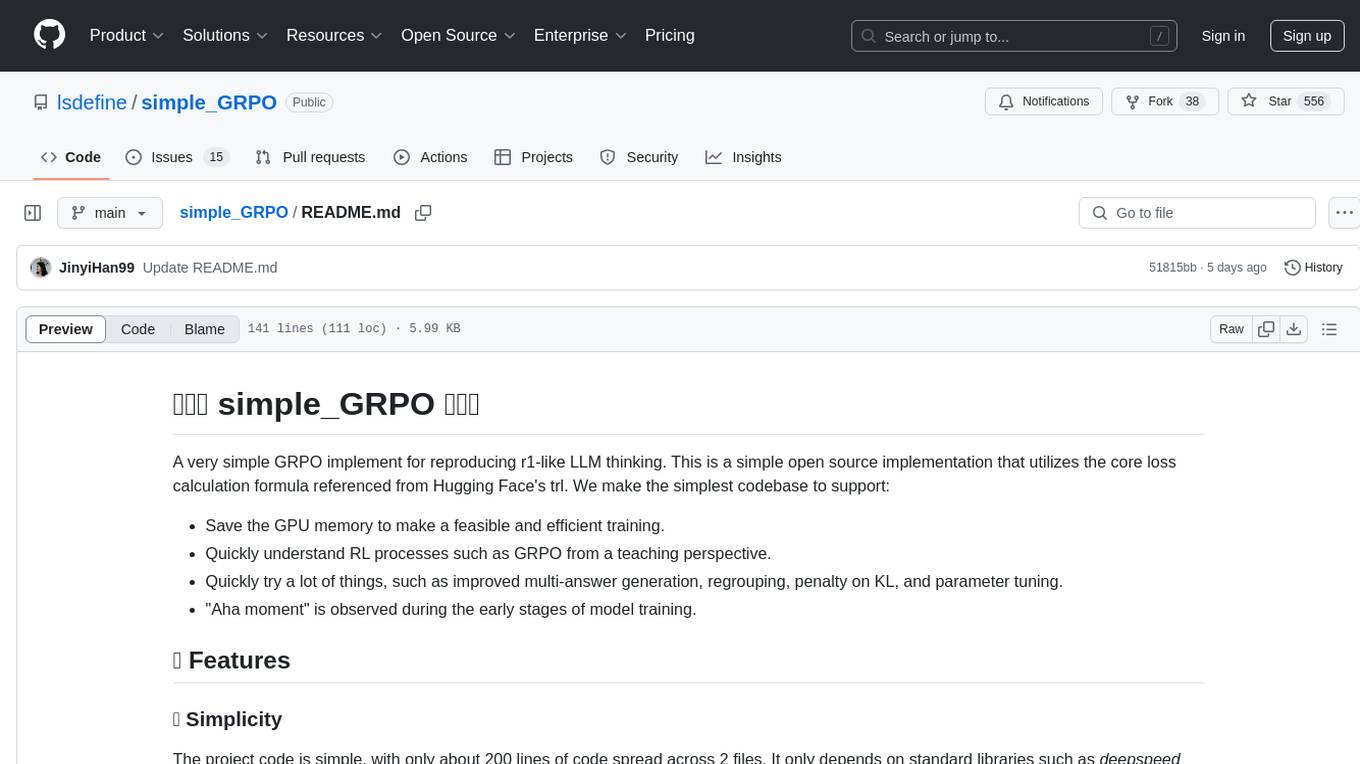
simple_GRPO
simple_GRPO is a very simple implementation of the GRPO algorithm for reproducing r1-like LLM thinking. It provides a codebase that supports saving GPU memory, understanding RL processes, trying various improvements like multi-answer generation, regrouping, penalty on KL, and parameter tuning. The project focuses on simplicity, performance, and core loss calculation based on Hugging Face's trl. It offers a straightforward setup with minimal dependencies and efficient training on multiple GPUs.
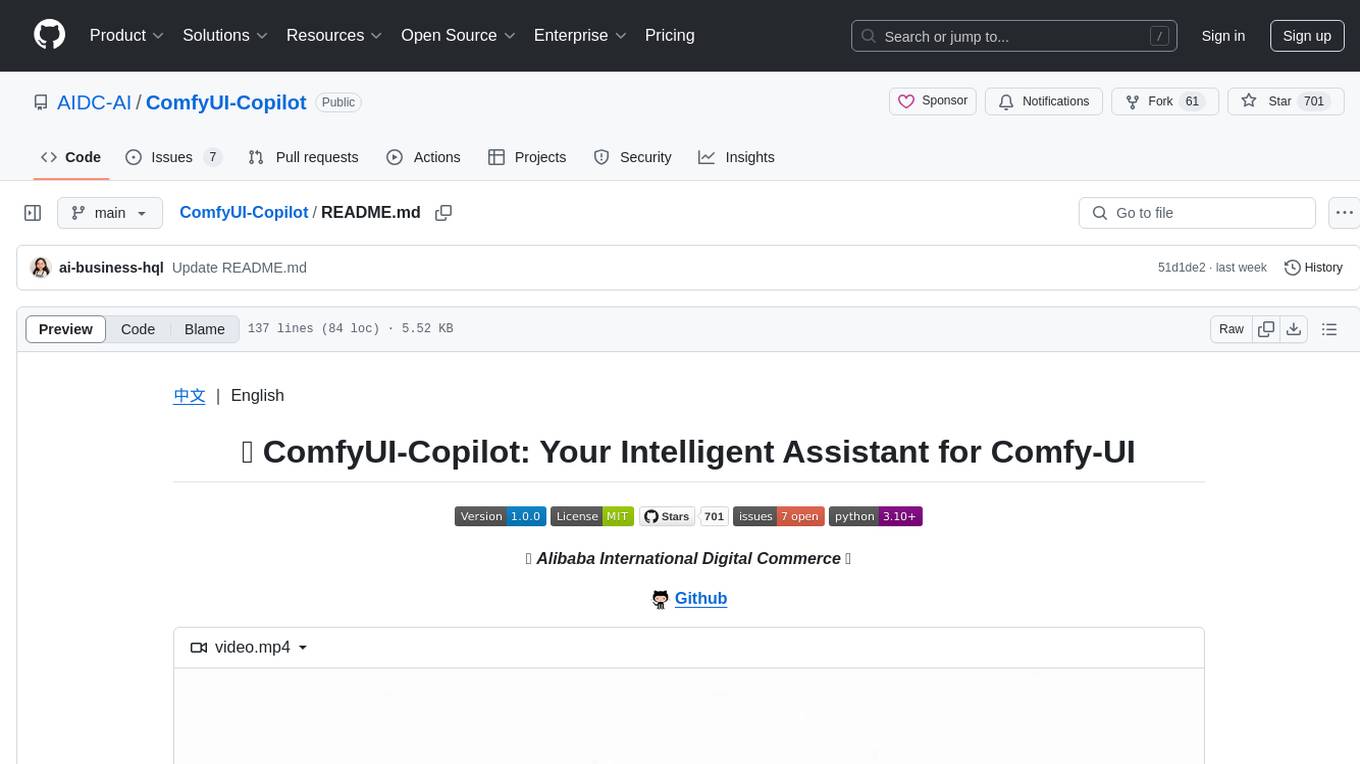
ComfyUI-Copilot
ComfyUI-Copilot is an intelligent assistant built on the Comfy-UI framework that simplifies and enhances the AI algorithm debugging and deployment process through natural language interactions. It offers intuitive node recommendations, workflow building aids, and model querying services to streamline development processes. With features like interactive Q&A bot, natural language node suggestions, smart workflow assistance, and model querying, ComfyUI-Copilot aims to lower the barriers to entry for beginners, boost development efficiency with AI-driven suggestions, and provide real-time assistance for developers.
For similar jobs
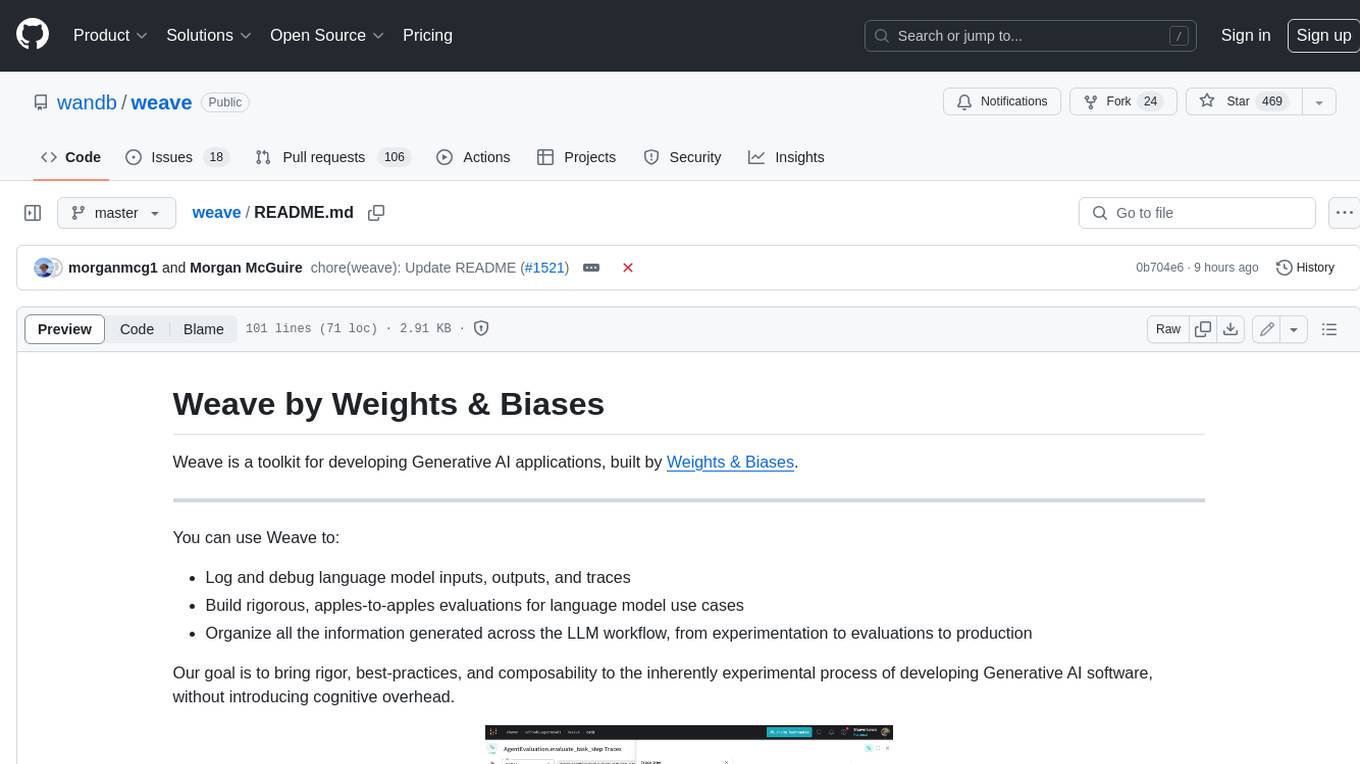
weave
Weave is a toolkit for developing Generative AI applications, built by Weights & Biases. With Weave, you can log and debug language model inputs, outputs, and traces; build rigorous, apples-to-apples evaluations for language model use cases; and organize all the information generated across the LLM workflow, from experimentation to evaluations to production. Weave aims to bring rigor, best-practices, and composability to the inherently experimental process of developing Generative AI software, without introducing cognitive overhead.
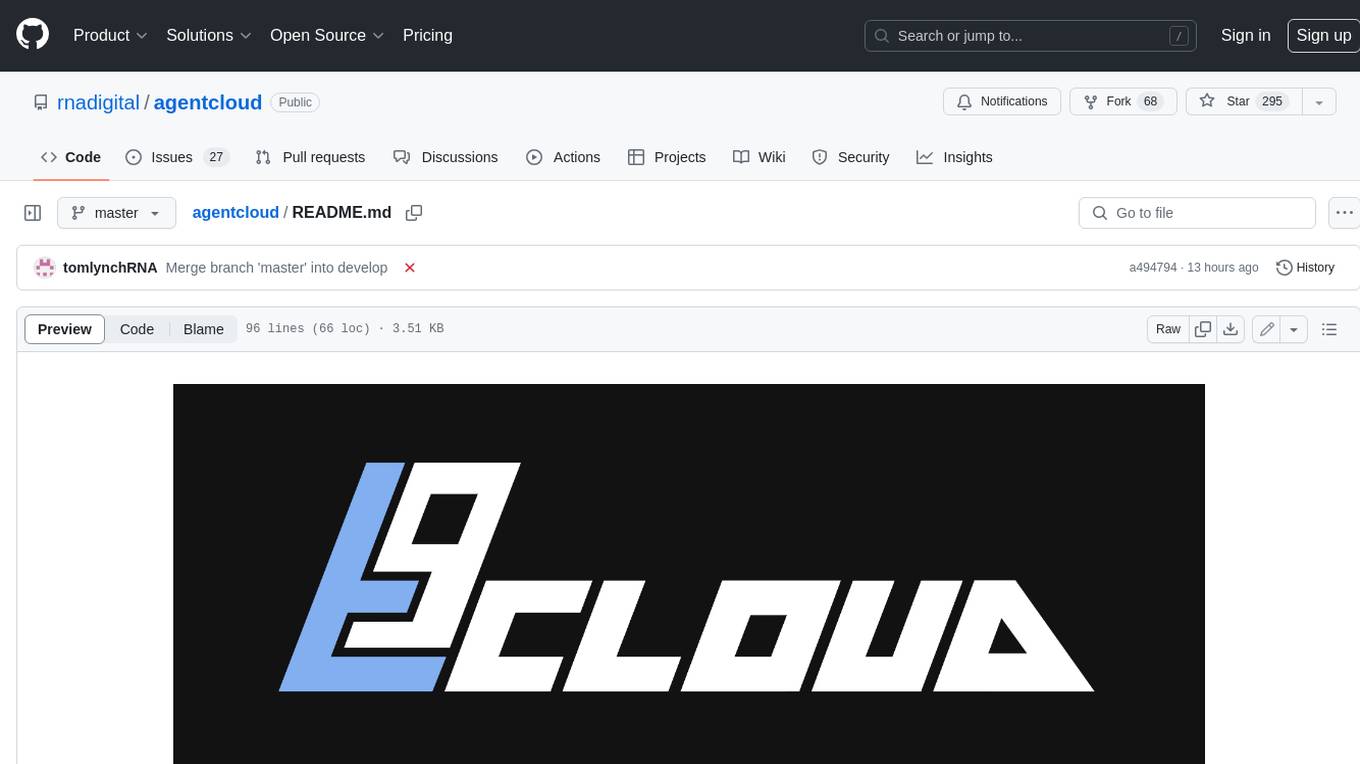
agentcloud
AgentCloud is an open-source platform that enables companies to build and deploy private LLM chat apps, empowering teams to securely interact with their data. It comprises three main components: Agent Backend, Webapp, and Vector Proxy. To run this project locally, clone the repository, install Docker, and start the services. The project is licensed under the GNU Affero General Public License, version 3 only. Contributions and feedback are welcome from the community.
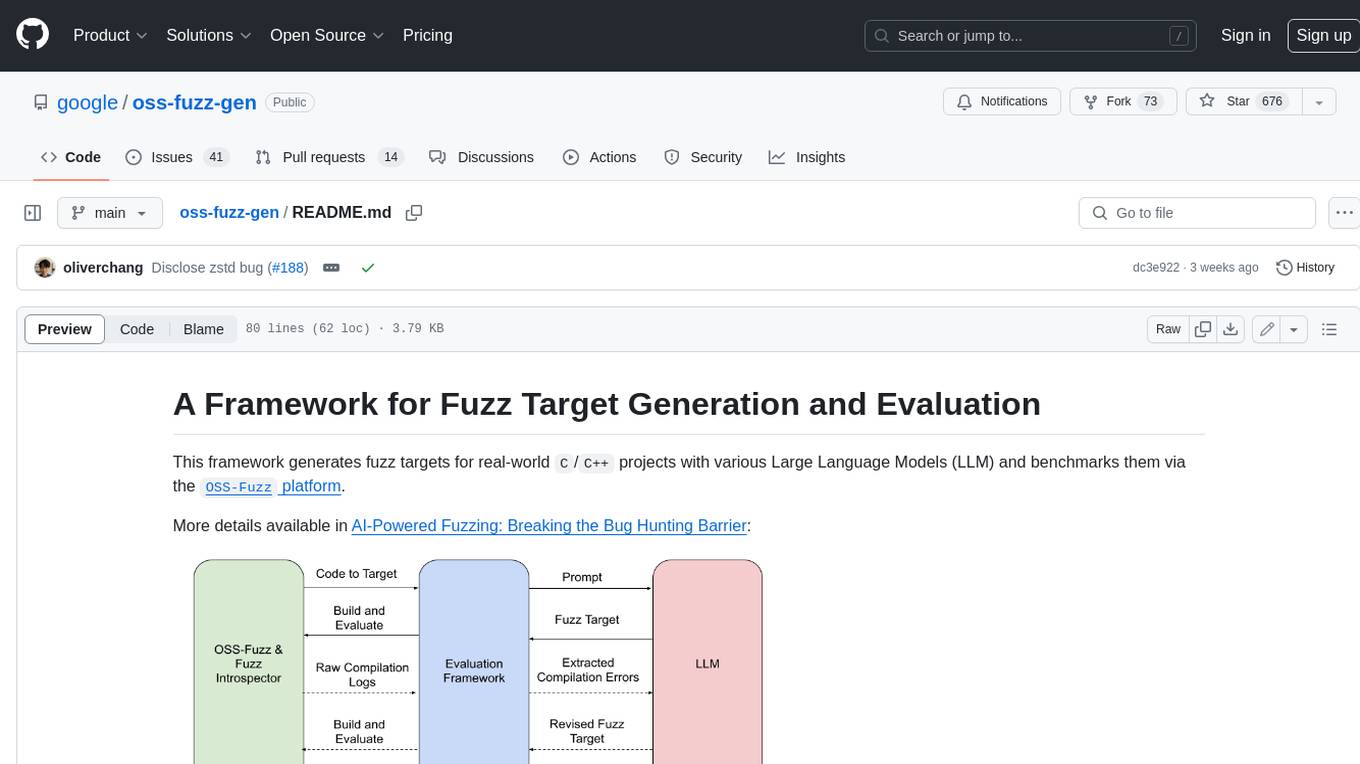
oss-fuzz-gen
This framework generates fuzz targets for real-world `C`/`C++` projects with various Large Language Models (LLM) and benchmarks them via the `OSS-Fuzz` platform. It manages to successfully leverage LLMs to generate valid fuzz targets (which generate non-zero coverage increase) for 160 C/C++ projects. The maximum line coverage increase is 29% from the existing human-written targets.
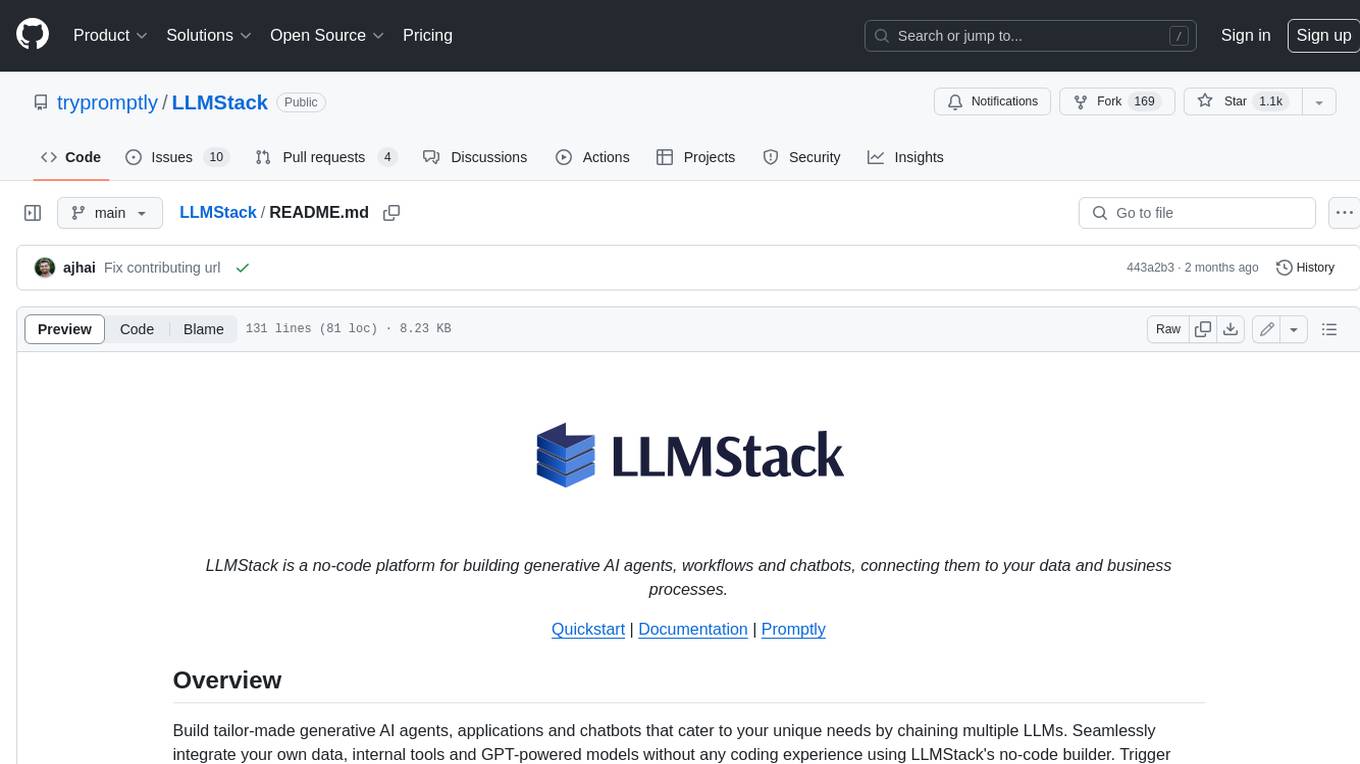
LLMStack
LLMStack is a no-code platform for building generative AI agents, workflows, and chatbots. It allows users to connect their own data, internal tools, and GPT-powered models without any coding experience. LLMStack can be deployed to the cloud or on-premise and can be accessed via HTTP API or triggered from Slack or Discord.
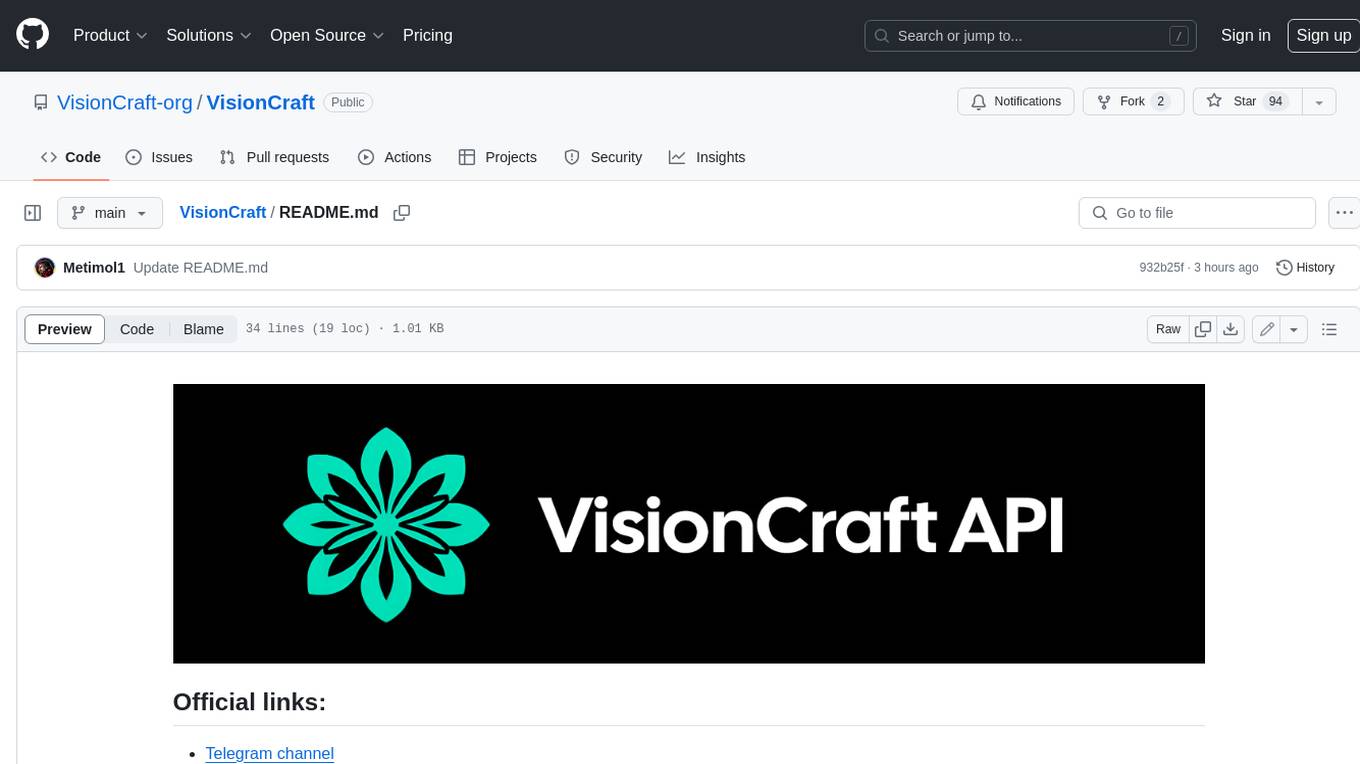
VisionCraft
The VisionCraft API is a free API for using over 100 different AI models. From images to sound.
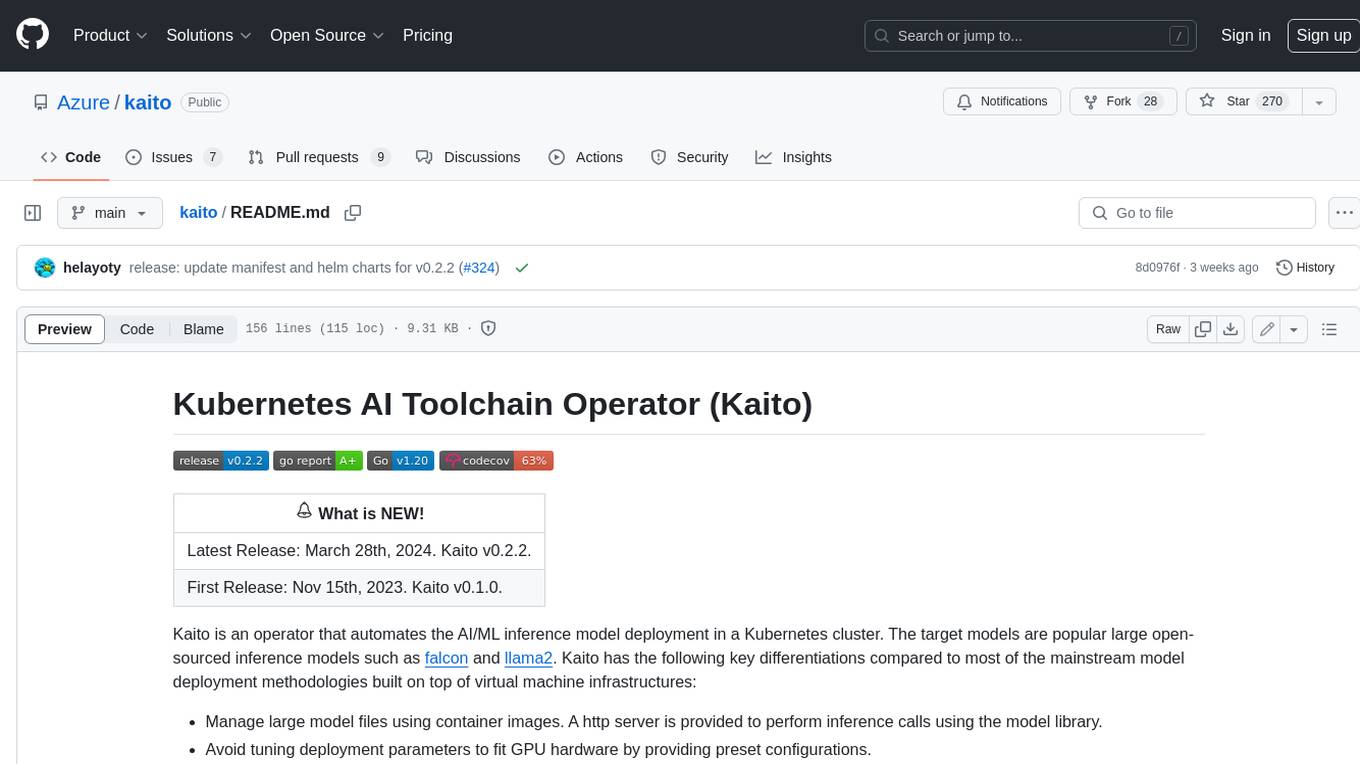
kaito
Kaito is an operator that automates the AI/ML inference model deployment in a Kubernetes cluster. It manages large model files using container images, avoids tuning deployment parameters to fit GPU hardware by providing preset configurations, auto-provisions GPU nodes based on model requirements, and hosts large model images in the public Microsoft Container Registry (MCR) if the license allows. Using Kaito, the workflow of onboarding large AI inference models in Kubernetes is largely simplified.
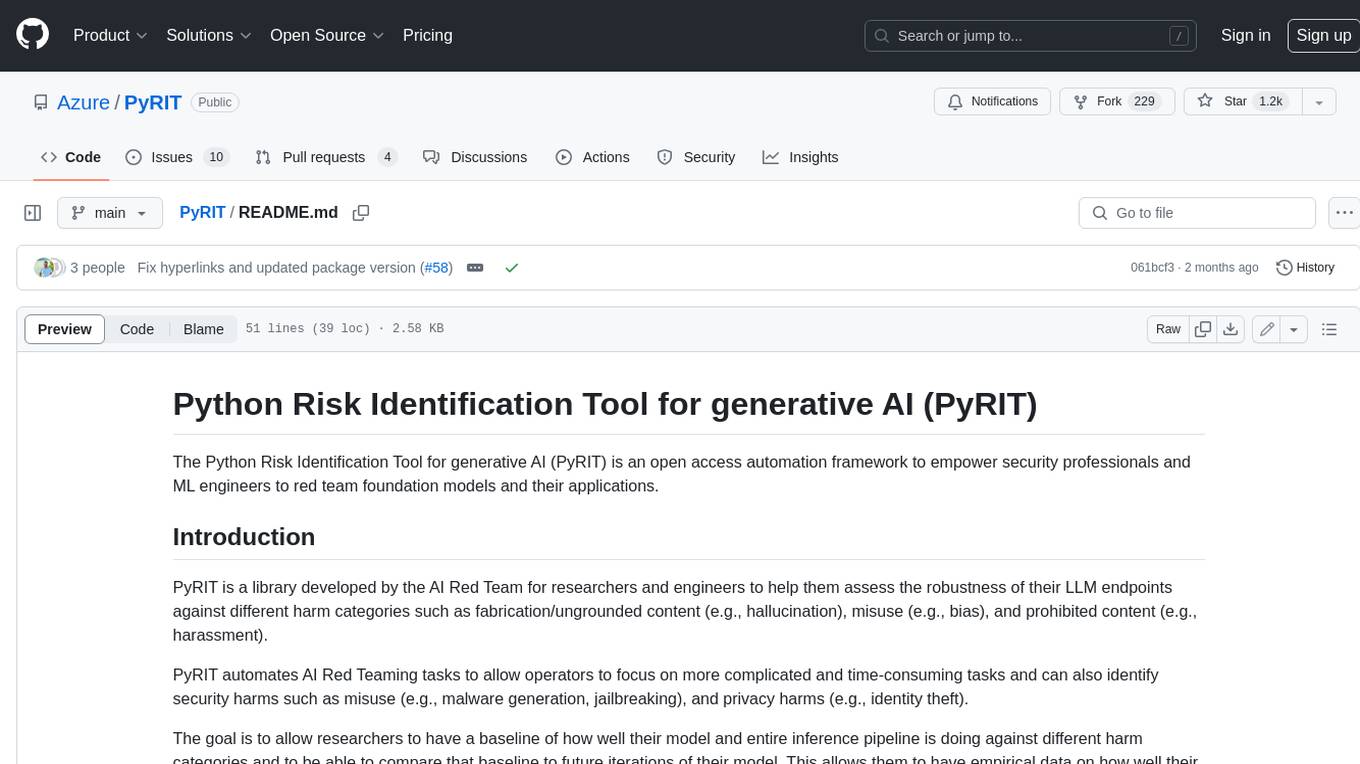
PyRIT
PyRIT is an open access automation framework designed to empower security professionals and ML engineers to red team foundation models and their applications. It automates AI Red Teaming tasks to allow operators to focus on more complicated and time-consuming tasks and can also identify security harms such as misuse (e.g., malware generation, jailbreaking), and privacy harms (e.g., identity theft). The goal is to allow researchers to have a baseline of how well their model and entire inference pipeline is doing against different harm categories and to be able to compare that baseline to future iterations of their model. This allows them to have empirical data on how well their model is doing today, and detect any degradation of performance based on future improvements.
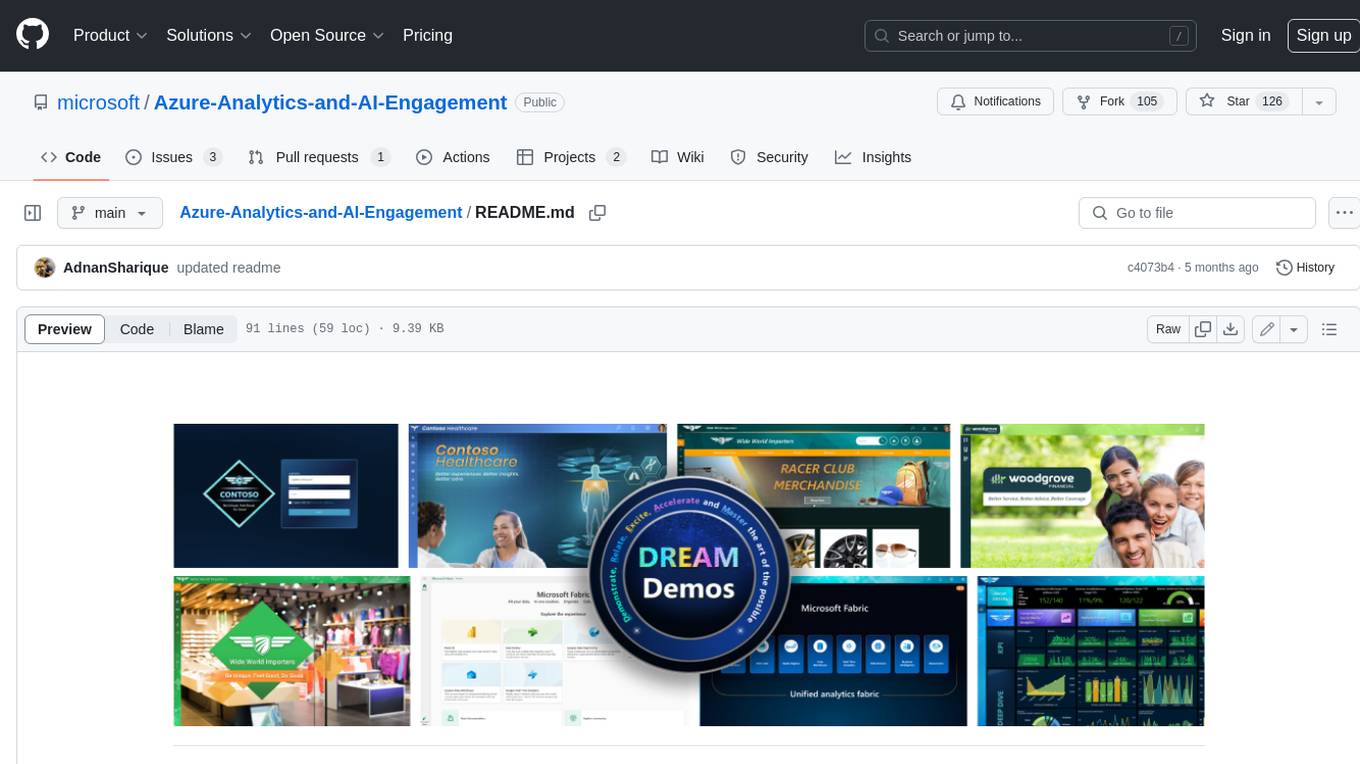
Azure-Analytics-and-AI-Engagement
The Azure-Analytics-and-AI-Engagement repository provides packaged Industry Scenario DREAM Demos with ARM templates (Containing a demo web application, Power BI reports, Synapse resources, AML Notebooks etc.) that can be deployed in a customer’s subscription using the CAPE tool within a matter of few hours. Partners can also deploy DREAM Demos in their own subscriptions using DPoC.