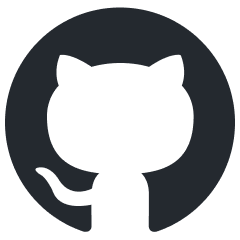
yolo-ios-app
Ultralytics YOLO iOS App source code for running YOLOv8 in your own iOS apps 🌟
Stars: 186
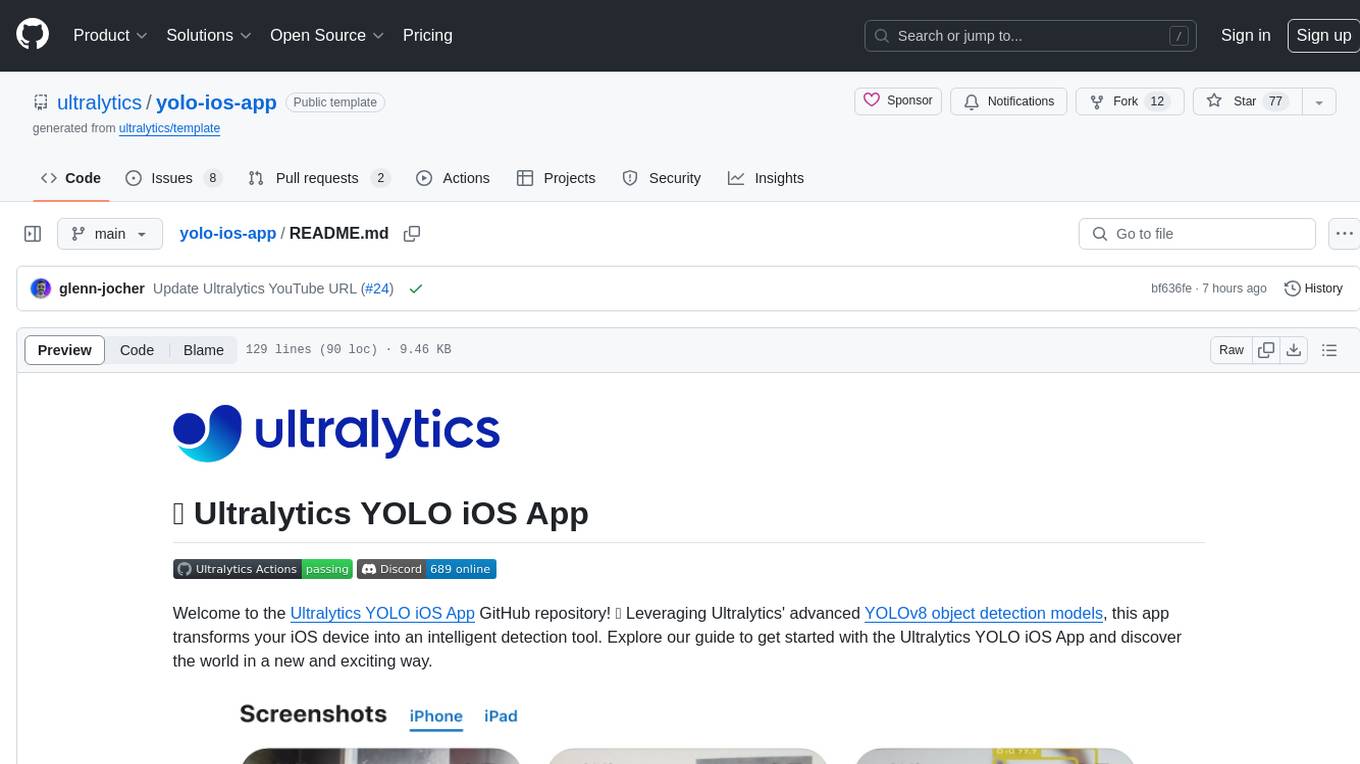
The Ultralytics YOLO iOS App GitHub repository offers an advanced object detection tool leveraging YOLOv8 models for iOS devices. Users can transform their devices into intelligent detection tools to explore the world in a new and exciting way. The app provides real-time detection capabilities with multiple AI models to choose from, ranging from 'nano' to 'x-large'. Contributors are welcome to participate in this open-source project, and licensing options include AGPL-3.0 for open-source use and an Enterprise License for commercial integration. Users can easily set up the app by following the provided steps, including cloning the repository, adding YOLOv8 models, and running the app on their iOS devices.
README:
Welcome to the Ultralytics YOLO iOS App GitHub repository! đź“– Leveraging Ultralytics' advanced YOLO11 object detection models, this app transforms your iOS device into an intelligent detection tool. Explore our guide to get started with the Ultralytics YOLO iOS App and discover the world in a new and exciting way.
Getting started with the Ultralytics YOLO iOS App is straightforward. Follow these steps to install the app on your iOS device.
Ensure you have the following before you start:
-
Xcode: The Ultralytics YOLO iOS App requires Xcode installed on your macOS machine. Download it from the Mac App Store.
-
An iOS Device: For testing the app, you'll need an iPhone or iPad running iOS 14.0 or later.
-
An Apple Developer Account: A free Apple Developer account will suffice for device testing. Sign up here if you haven't already.
-
Clone the Repository:
git clone https://github.com/ultralytics/yolo-ios-app.git
-
Open the Project in Xcode:
Navigate to the cloned directory and open the
YOLO.xcodeproj
file.In Xcode, go to the project's target settings and choose your Apple Developer account under the "Signing & Capabilities" tab.
-
Add YOLO11 Models to the Project:
Export CoreML INT8 models using the
ultralytics
Python package (withpip install ultralytics
), or download them from our GitHub release assets. You should have 5 YOLO11 models in total. Place these in theYOLO/Models
directory as seen in the Xcode screenshot below.from ultralytics import YOLO # Loop through all YOLO11 model sizes for size in ("n", "s", "m", "l", "x"): # Load a YOLO11 PyTorch model model = YOLO(f"yolo11{size}.pt") # Export the PyTorch model to CoreML INT8 format with NMS layers model.export(format="coreml", int8=True, nms=True, imgsz=[640, 384])
-
Run the Ultralytics YOLO iOS App:
Connect your iOS device and select it as the run target. Press the Run button to install the app on your device.
The Ultralytics YOLO iOS App is designed to be intuitive:
- Real-Time Detection: Launch the app and aim your camera at objects to detect them instantly.
- Multiple AI Models: Select from a range of Ultralytics YOLO11 models, from YOLO11n 'nano' to YOLO11x 'x-large'.
We warmly welcome your contributions to Ultralytics' open-source projects! Your support and contributions significantly impact. Get involved by reviewing our Contributing Guide, and share your feedback through our Survey. A massive thank you 🙏 to everyone who contributes!

Ultralytics offers two licensing options:
-
AGPL-3.0 License: An OSI-approved open-source license, perfect for academics, researchers, and enthusiasts. It encourages sharing knowledge and collaboration. See the LICENSE file for details.
-
Enterprise License: Designed for commercial use, this license permits integrating Ultralytics software into proprietary products and services. For commercial use, please contact us through Ultralytics Licensing.
- Submit Ultralytics bug reports and feature requests via GitHub Issues.
- Join our Discord for assistance, questions, and discussions with the community and team!
For Tasks:
Click tags to check more tools for each tasksFor Jobs:
Alternative AI tools for yolo-ios-app
Similar Open Source Tools
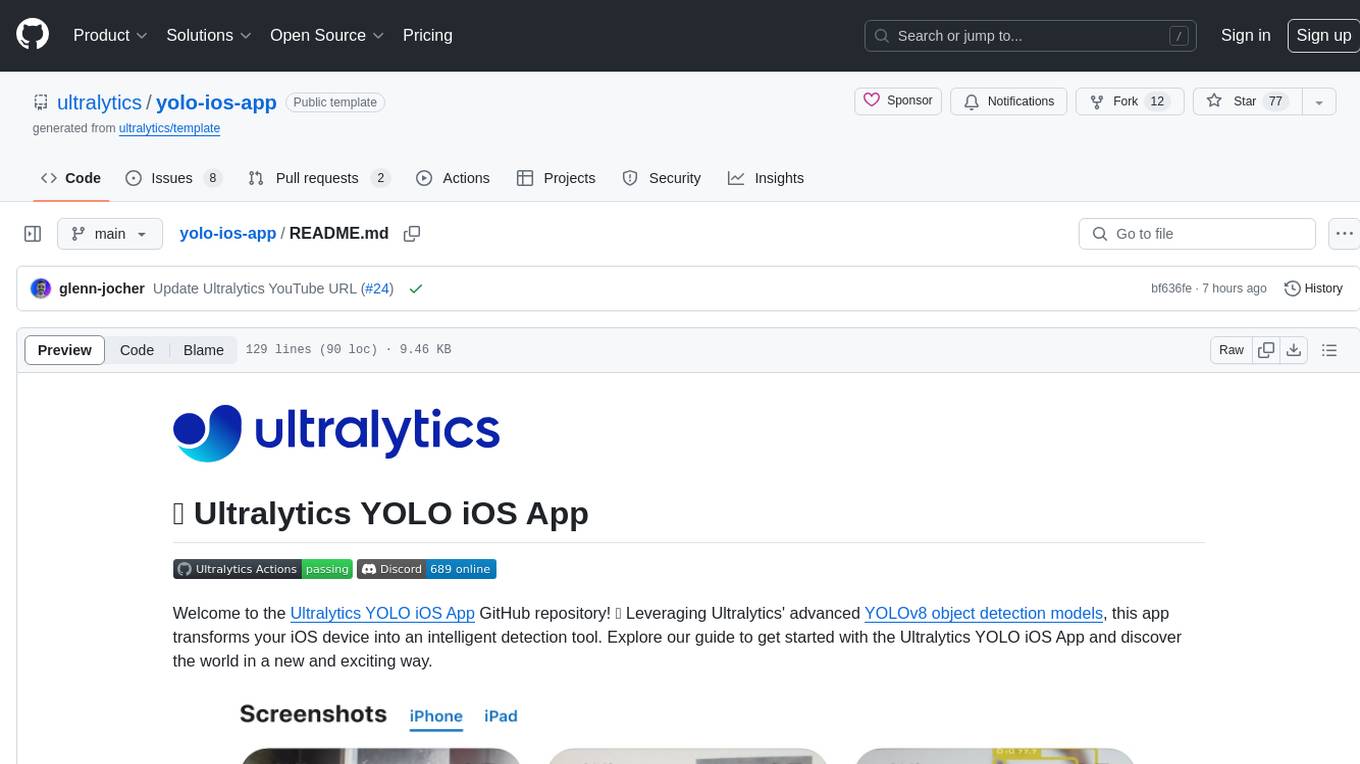
yolo-ios-app
The Ultralytics YOLO iOS App GitHub repository offers an advanced object detection tool leveraging YOLOv8 models for iOS devices. Users can transform their devices into intelligent detection tools to explore the world in a new and exciting way. The app provides real-time detection capabilities with multiple AI models to choose from, ranging from 'nano' to 'x-large'. Contributors are welcome to participate in this open-source project, and licensing options include AGPL-3.0 for open-source use and an Enterprise License for commercial integration. Users can easily set up the app by following the provided steps, including cloning the repository, adding YOLOv8 models, and running the app on their iOS devices.
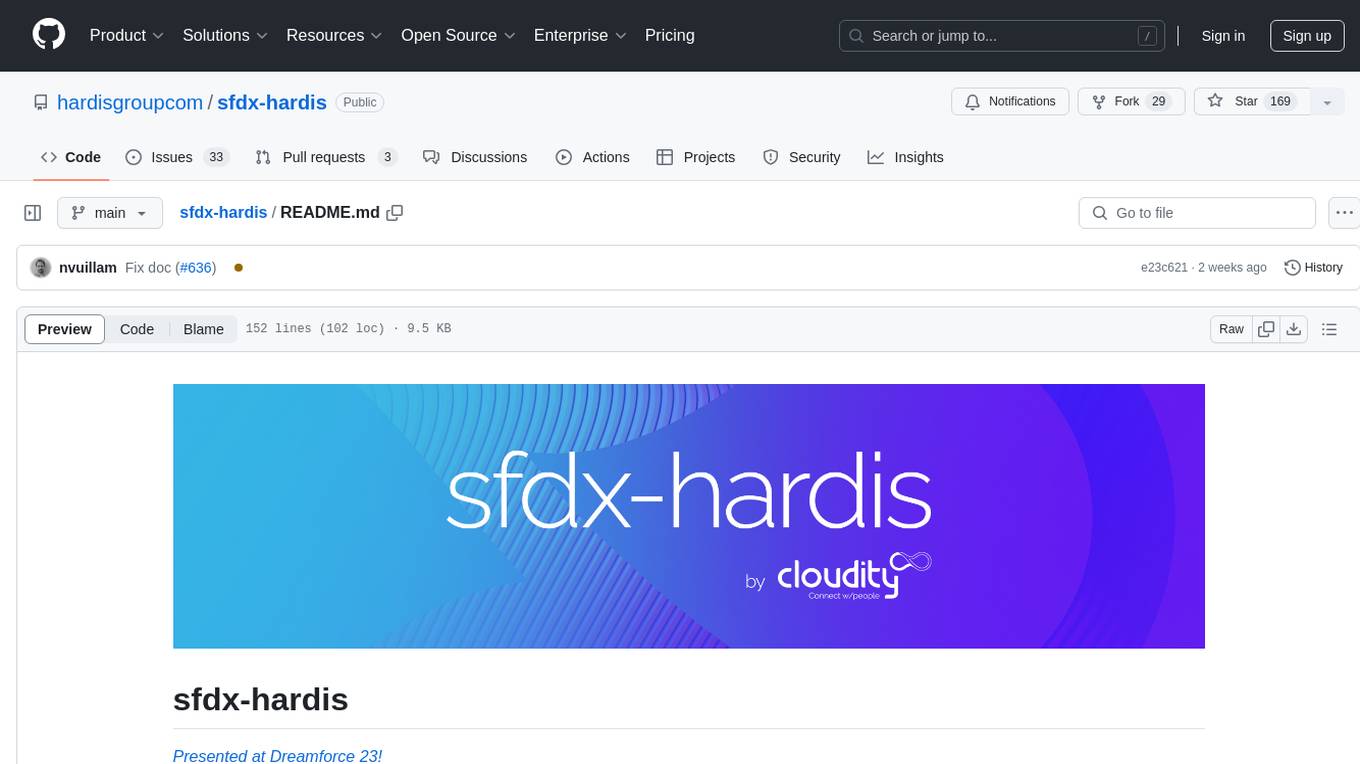
sfdx-hardis
sfdx-hardis is a toolbox for Salesforce DX, developed by Cloudity, that simplifies tasks which would otherwise take minutes or hours to complete manually. It enables users to define complete CI/CD pipelines for Salesforce projects, backup metadata, and monitor any Salesforce org. The tool offers a wide range of commands that can be accessed via the command line interface or through a Visual Studio Code extension. Additionally, sfdx-hardis provides Docker images for easy integration into CI workflows. The tool is designed to be natively compliant with various platforms and tools, making it a versatile solution for Salesforce developers.
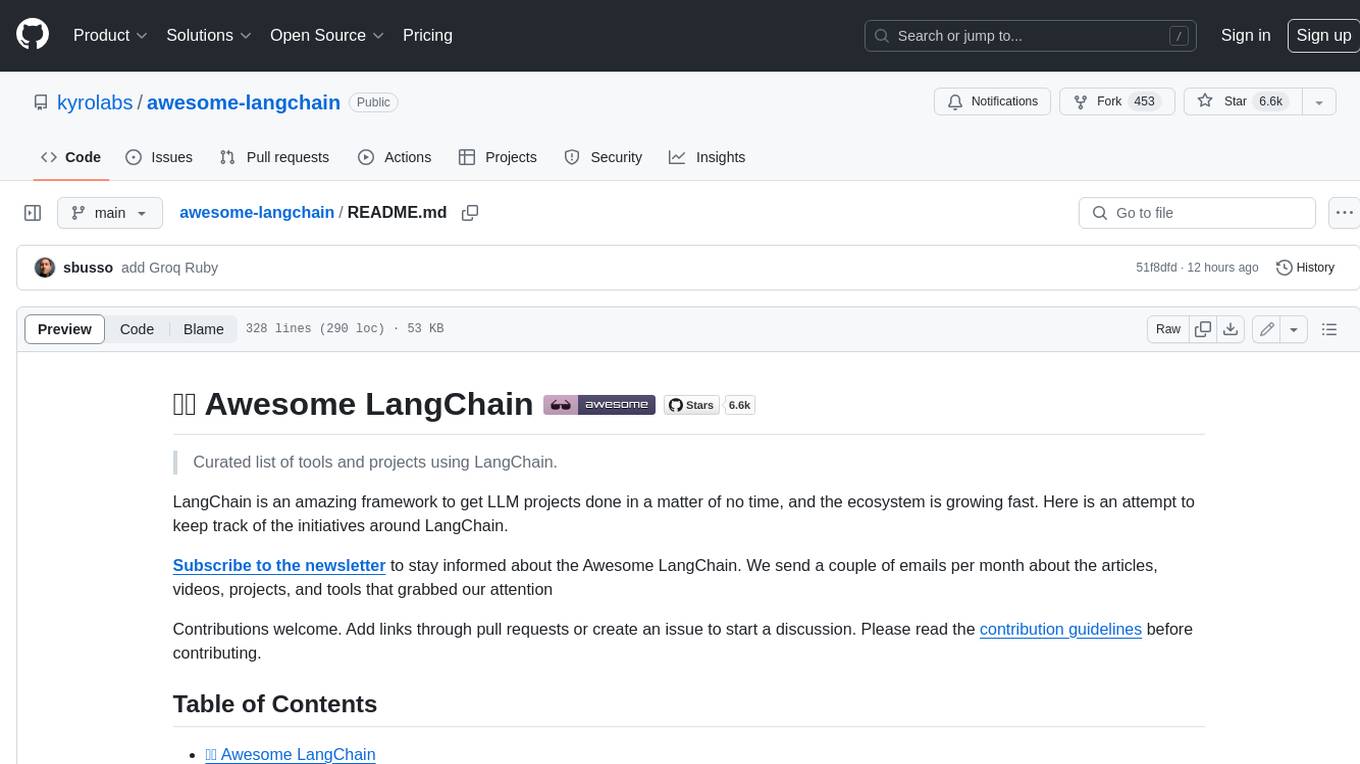
awesome-langchain
LangChain is an amazing framework to get LLM projects done in a matter of no time, and the ecosystem is growing fast. Here is an attempt to keep track of the initiatives around LangChain. Subscribe to the newsletter to stay informed about the Awesome LangChain. We send a couple of emails per month about the articles, videos, projects, and tools that grabbed our attention Contributions welcome. Add links through pull requests or create an issue to start a discussion. Please read the contribution guidelines before contributing.
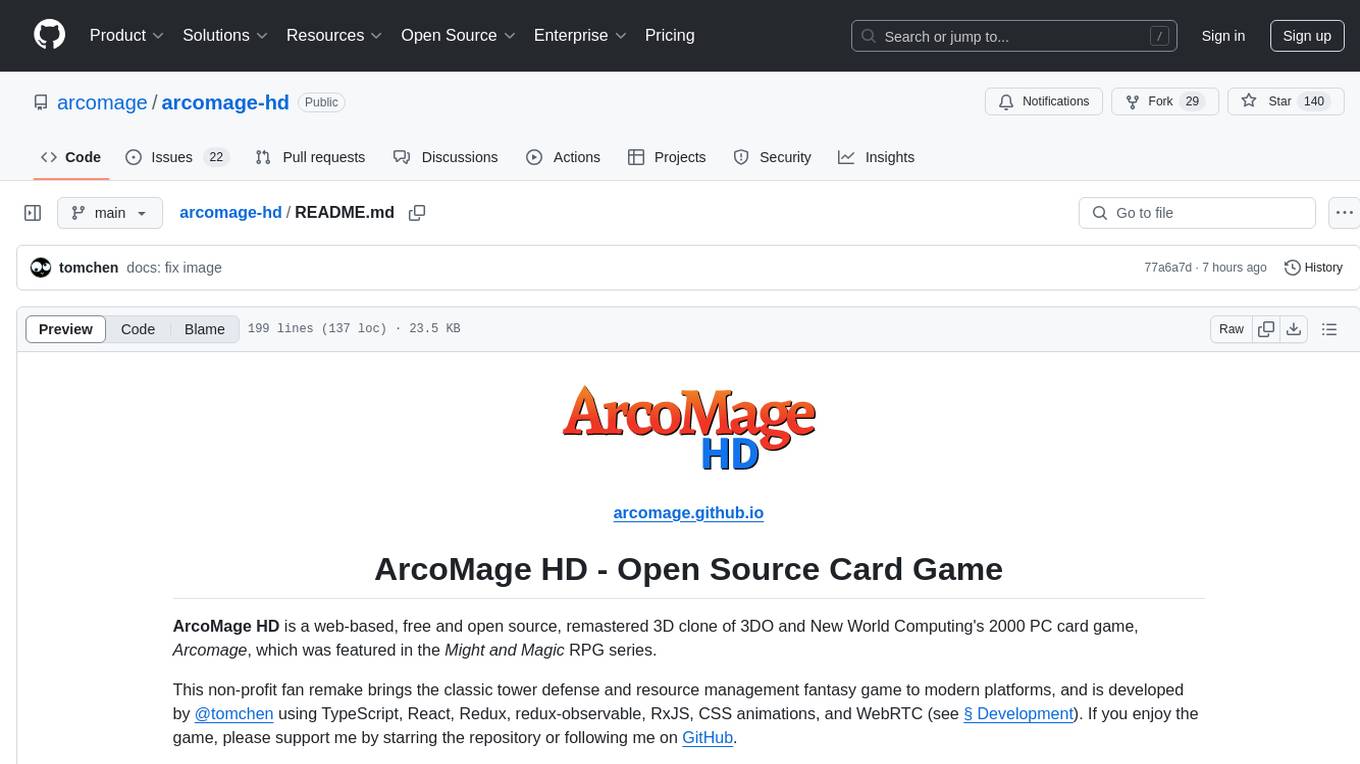
arcomage-hd
ArcoMage HD is a web-based, free and open source, remastered 3D clone of 3DO and New World Computing's 2000 PC card game, Arcomage, featured in the Might and Magic RPG series. This non-profit fan remake brings the classic tower defense and resource management fantasy game to modern platforms. It is fully responsive, supporting desktop, tablet, and mobile devices, and includes comprehensive accessibility features for users with disabilities. The game is playable offline as a PWA and offers both Single Player Mode against computer AI and Multiplayer Mode. Developed by @tomchen using TypeScript, React, Redux, redux-observable, RxJS, CSS animations, and WebRTC.
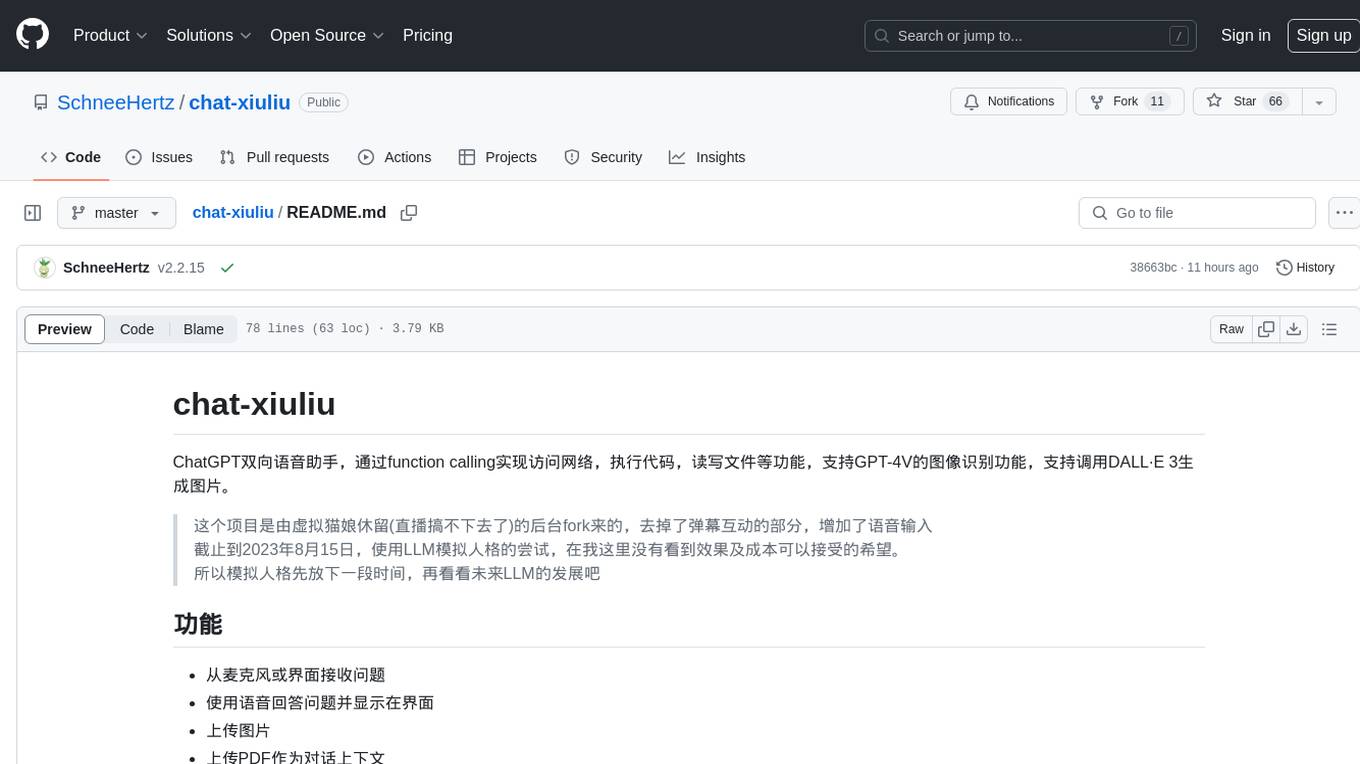
chat-xiuliu
Chat-xiuliu is a bidirectional voice assistant powered by ChatGPT, capable of accessing the internet, executing code, reading/writing files, and supporting GPT-4V's image recognition feature. It can also call DALL·E 3 to generate images. The project is a fork from a background of a virtual cat girl named Xiuliu, with removed live chat interaction and added voice input. It can receive questions from microphone or interface, answer them vocally, upload images and PDFs, process tasks through function calls, remember conversation content, search the web, generate images using DALL·E 3, read/write local files, execute JavaScript code in a sandbox, open local files or web pages, customize the cat girl's speaking style, save conversation screenshots, and support Azure OpenAI and other API endpoints in openai format. It also supports setting proxies and various AI models like GPT-4, GPT-3.5, and DALL·E 3.
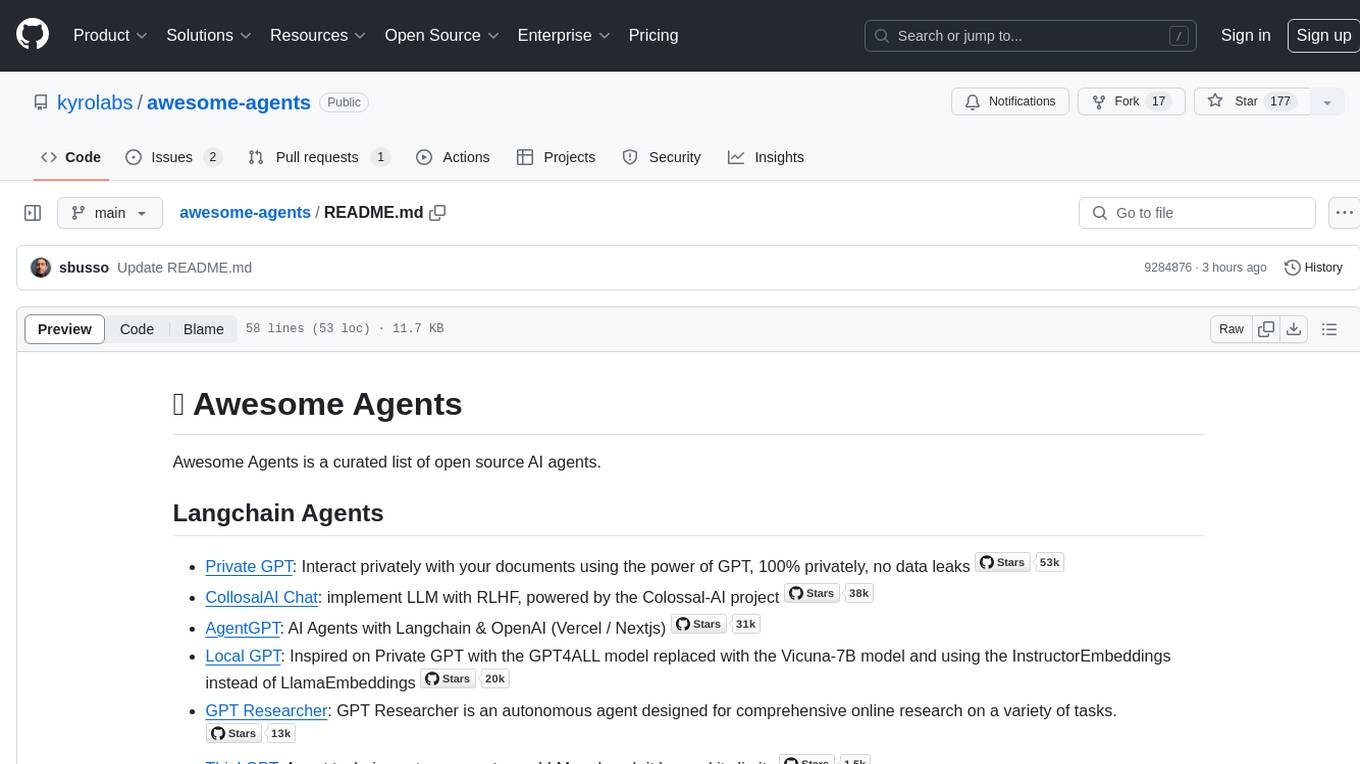
awesome-agents
Awesome Agents is a curated list of open source AI agents designed for various tasks such as private interactions with documents, chat implementations, autonomous research, human-behavior simulation, code generation, HR queries, domain-specific research, and more. The agents leverage Large Language Models (LLMs) and other generative AI technologies to provide solutions for complex tasks and projects. The repository includes a diverse range of agents for different use cases, from conversational chatbots to AI coding engines, and from autonomous HR assistants to vision task solvers.
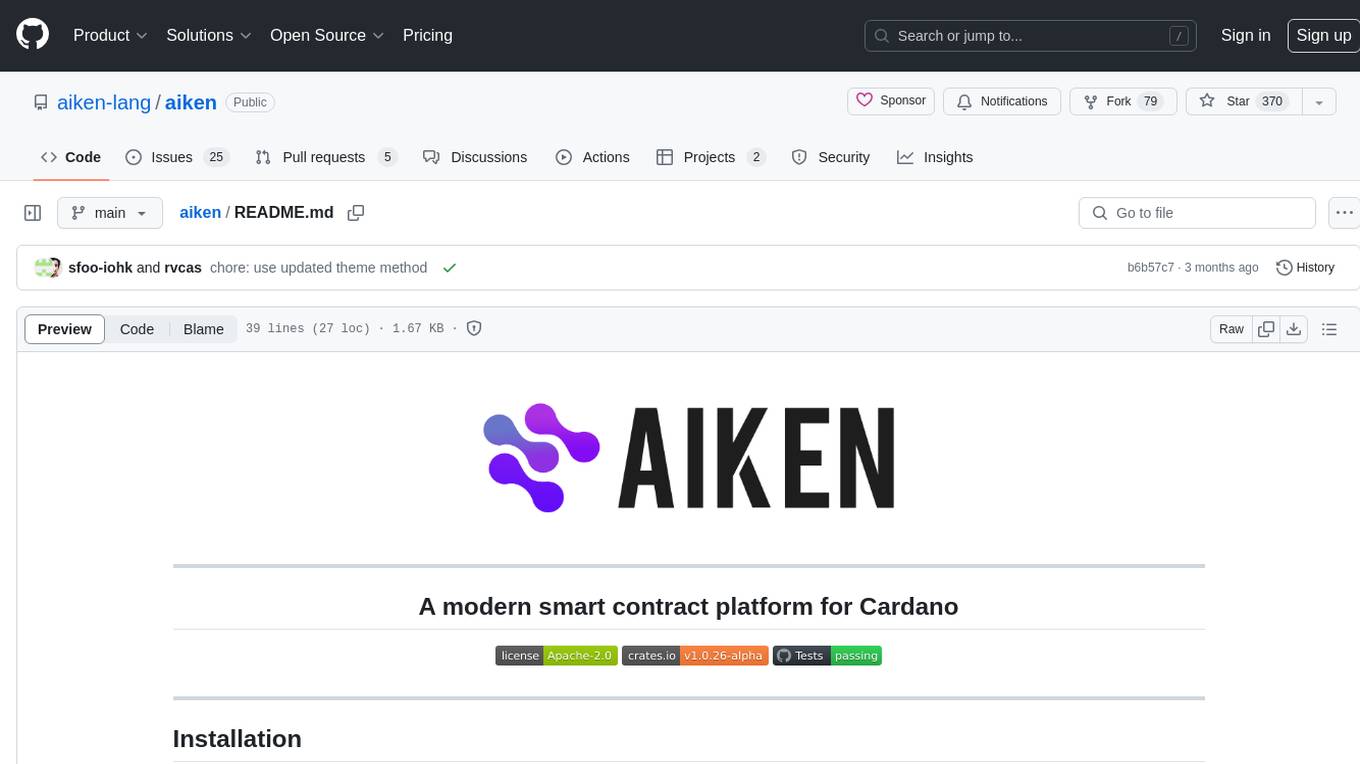
aiken
Aiken is a modern smart contract platform for Cardano, providing a user-friendly environment for developing and deploying smart contracts. It supports Linux, MacOS, and Windows operating systems. Aiken is designed to simplify the process of creating smart contracts on the Cardano blockchain, offering a seamless experience for developers. The platform is named after Howard Aiken, an American physicist and computing pioneer.
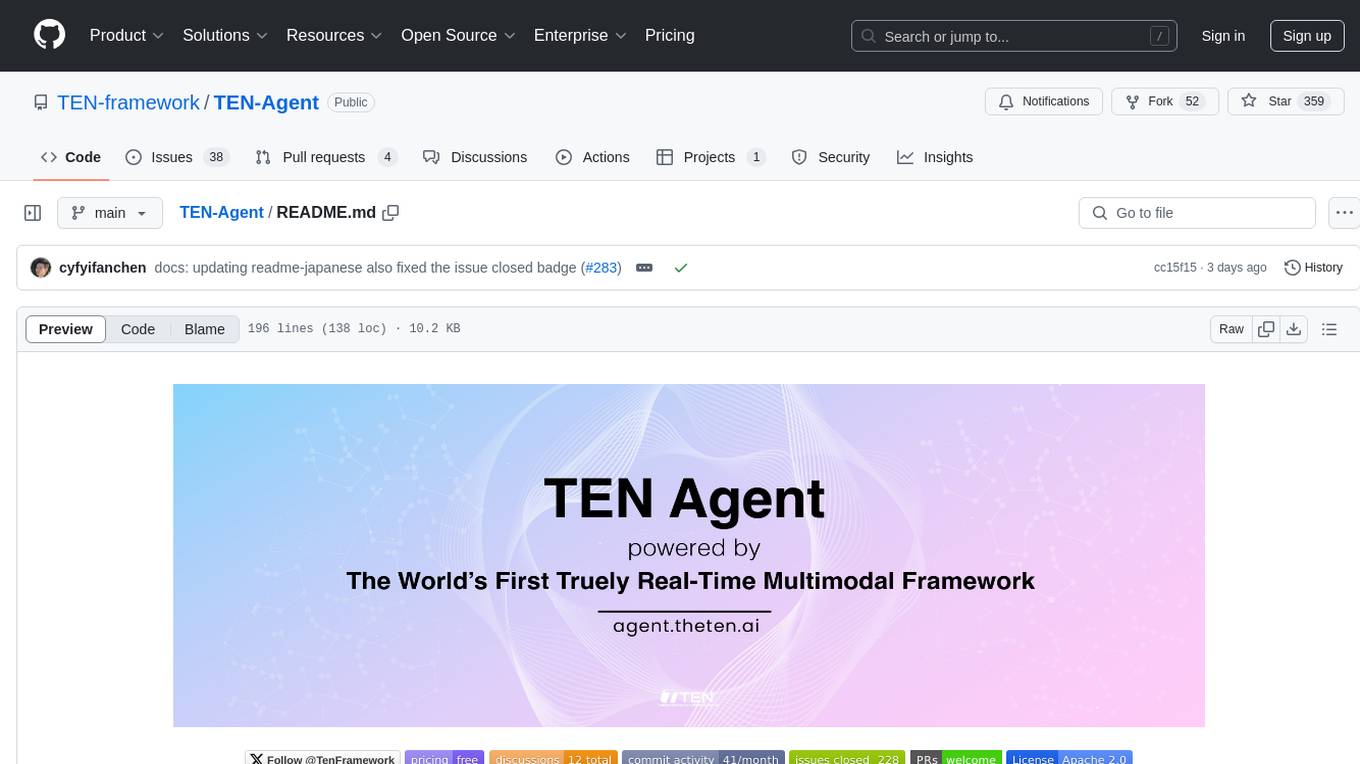
TEN-Agent
TEN Agent is an open-source multimodal agent powered by the world’s first real-time multimodal framework, TEN Framework. It offers high-performance real-time multimodal interactions, multi-language and multi-platform support, edge-cloud integration, flexibility beyond model limitations, and real-time agent state management. Users can easily build complex AI applications through drag-and-drop programming, integrating audio-visual tools, databases, RAG, and more.
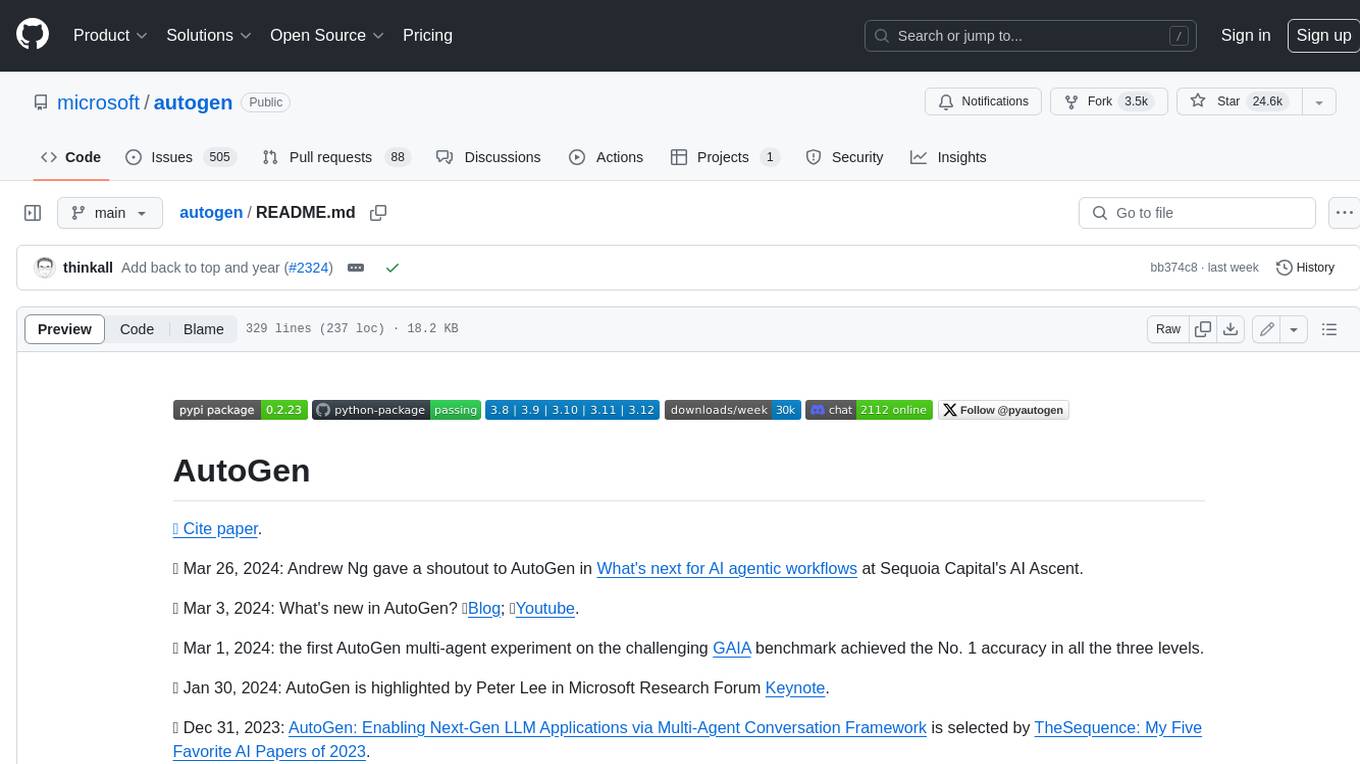
autogen
AutoGen is a framework that enables the development of LLM applications using multiple agents that can converse with each other to solve tasks. AutoGen agents are customizable, conversable, and seamlessly allow human participation. They can operate in various modes that employ combinations of LLMs, human inputs, and tools.
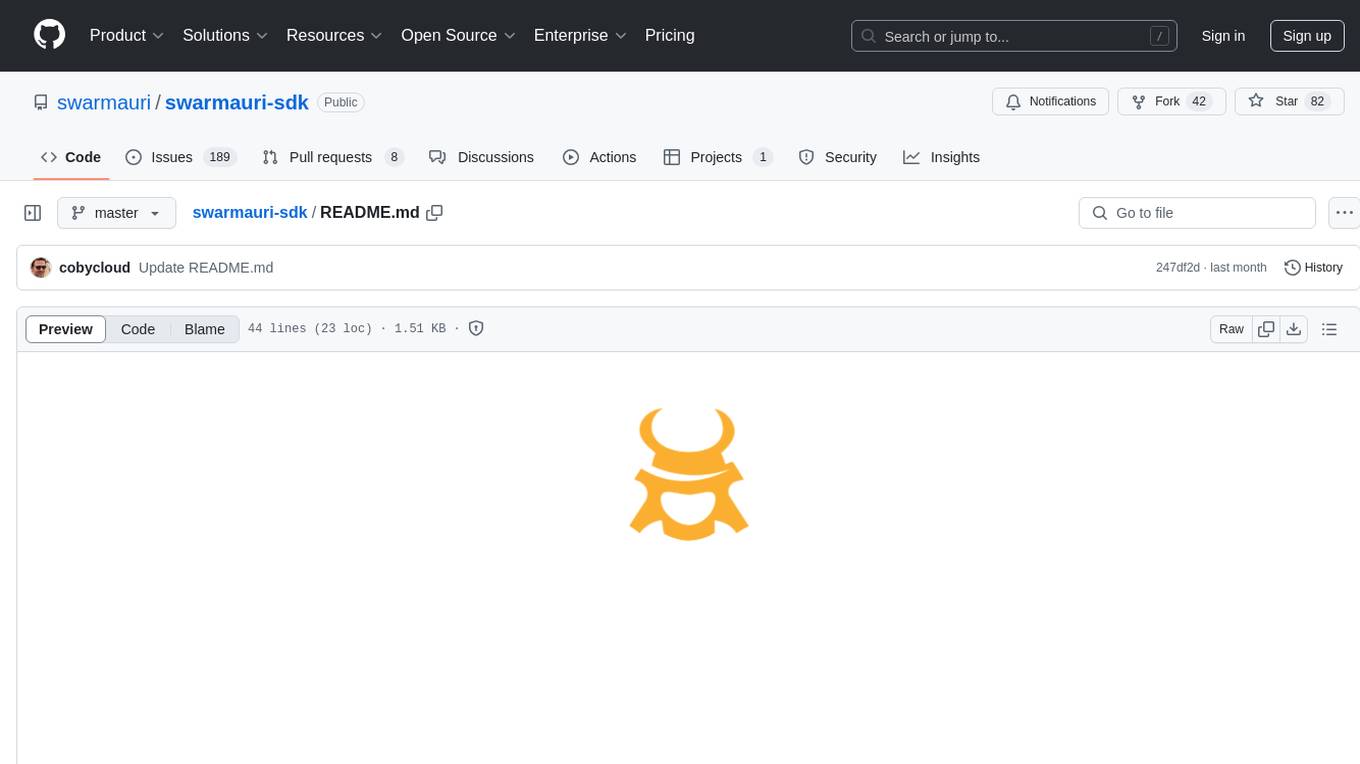
swarmauri-sdk
Swarmauri SDK is a repository containing core interfaces, standard ABCs, and standard concrete references of the SwarmaURI Framework. It provides a set of tools and functionalities for developers to work with the SwarmaURI ecosystem. The SDK aims to streamline the development process and enhance the interoperability of applications within the framework. Developers can easily integrate SwarmaURI features into their projects by leveraging the resources available in this repository.
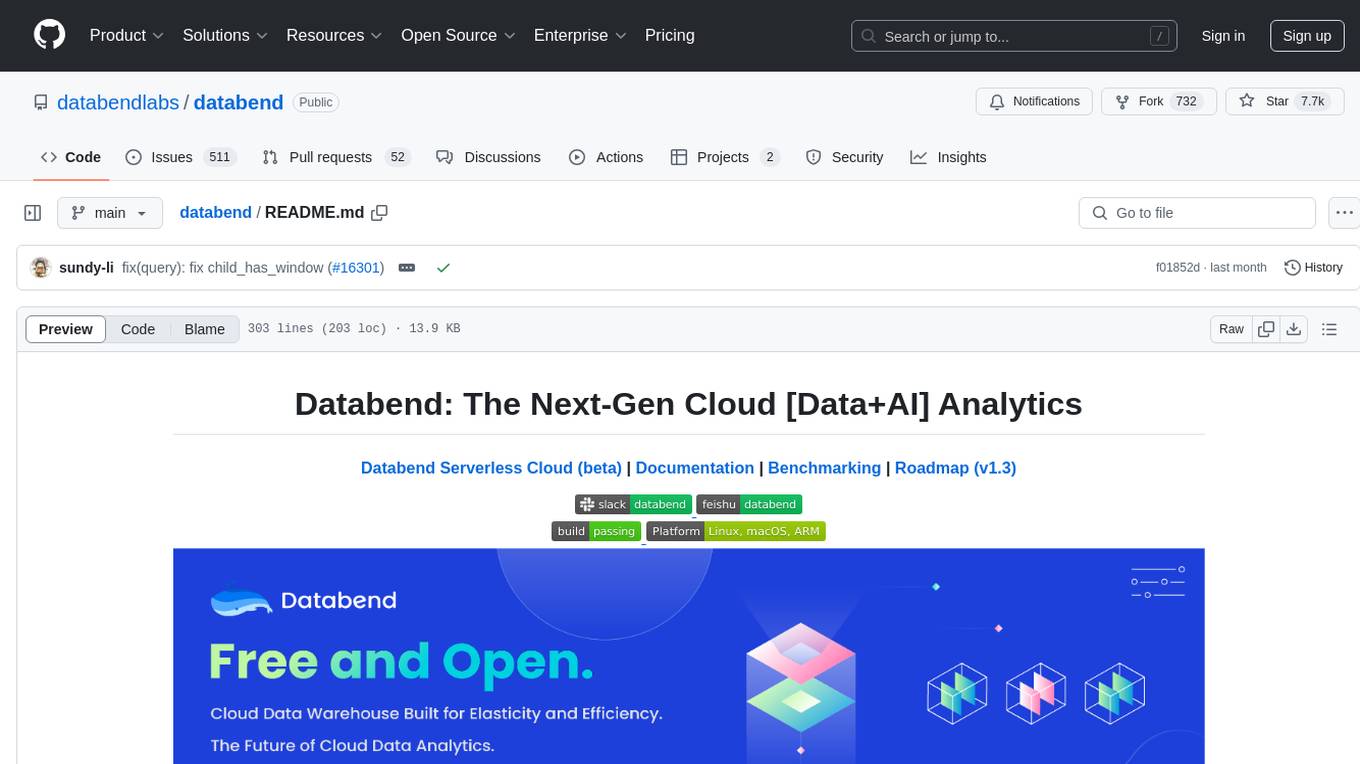
databend
Databend is an open-source cloud data warehouse built in Rust, offering fast query execution and data ingestion for complex analysis of large datasets. It integrates with major cloud platforms, provides high performance with AI-powered analytics, supports multiple data formats, ensures data integrity with ACID transactions, offers flexible indexing options, and features community-driven development. Users can try Databend through a serverless cloud or Docker installation, and perform tasks such as data import/export, querying semi-structured data, managing users/databases/tables, and utilizing AI functions.
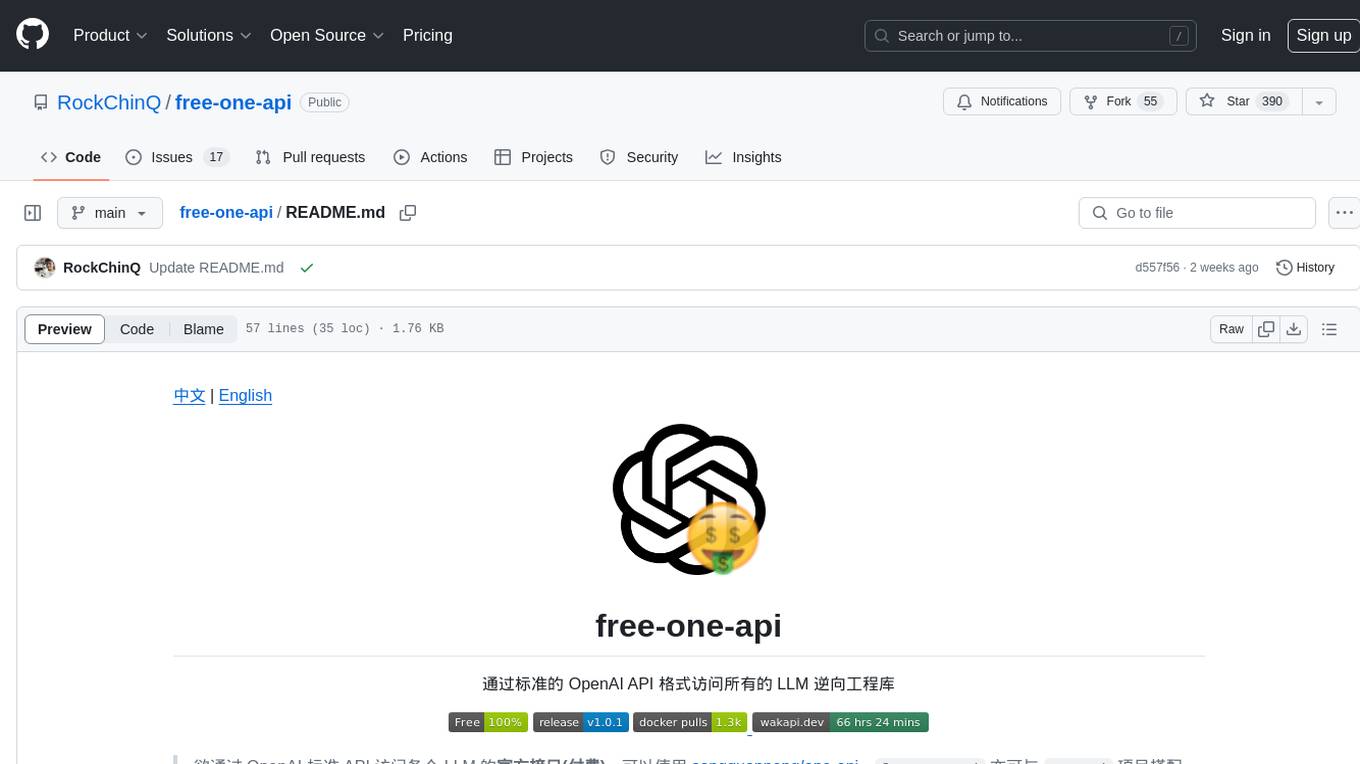
free-one-api
Free-one-api is a tool that allows access to all LLM reverse engineering libraries in a standard OpenAI API format. It supports automatic load balancing, Web UI, stream mode, multiple LLM reverse libraries, heartbeat detection mechanism, automatic disabling of unavailable channels, and runtime log recording. The tool is designed to work with the 'one-api' project and 'songquanpeng/one-api' for accessing official interfaces of various LLMs (paid). Contributors are needed to test adapters, find new reverse engineering libraries, and submit PRs.
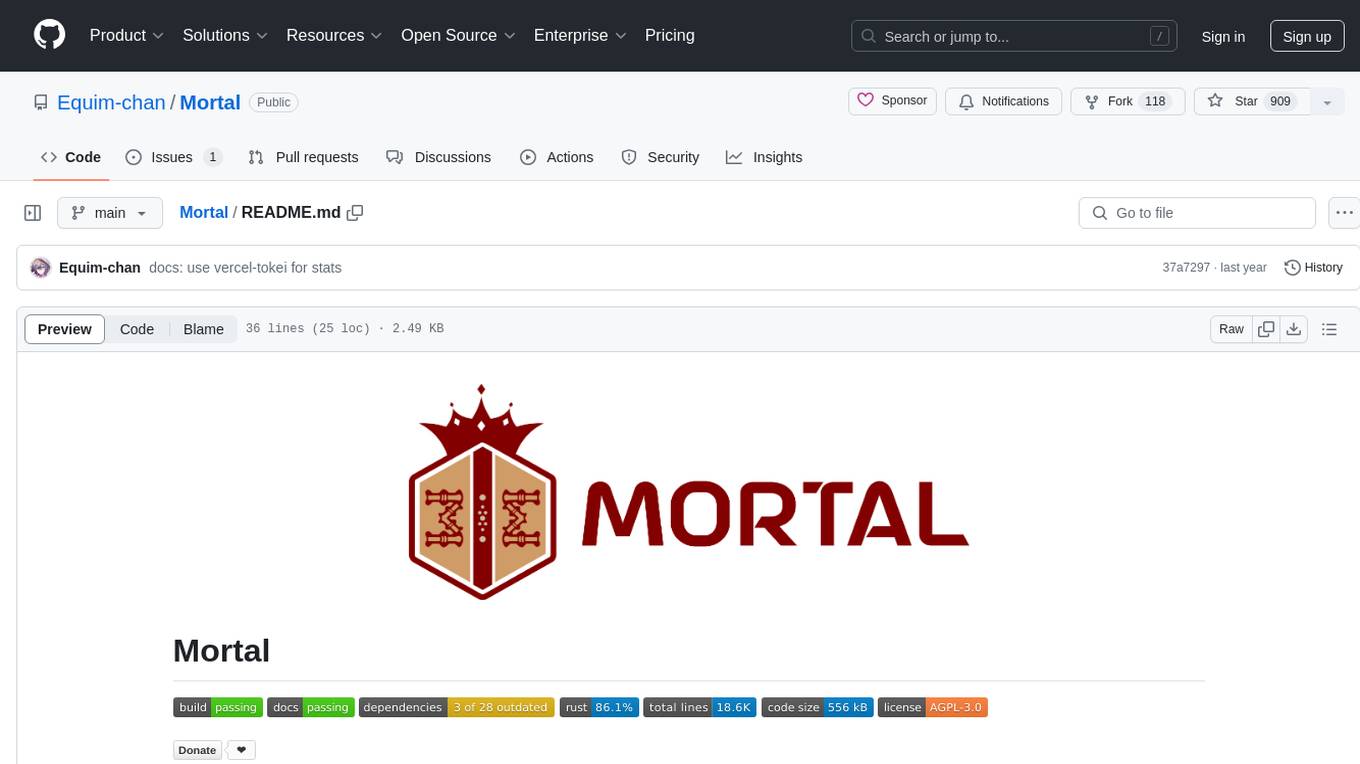
Mortal
Mortal (凡夫) is a free and open source AI for Japanese mahjong, powered by deep reinforcement learning. It provides a comprehensive solution for playing Japanese mahjong with AI assistance. The project focuses on utilizing deep reinforcement learning techniques to enhance gameplay and decision-making in Japanese mahjong. Mortal offers a user-friendly interface and detailed documentation to assist users in understanding and utilizing the AI effectively. The project is actively maintained and welcomes contributions from the community to further improve the AI's capabilities and performance.
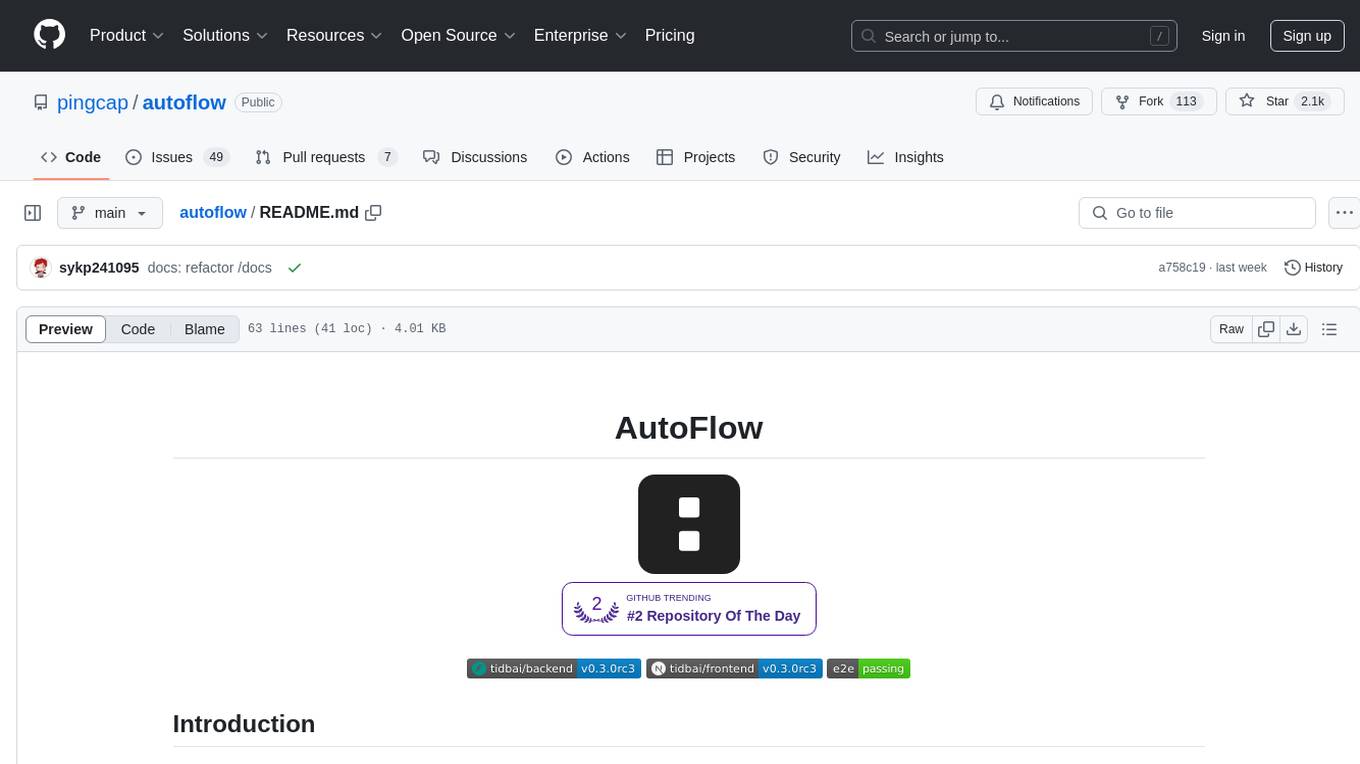
autoflow
AutoFlow is an open source graph rag based knowledge base tool built on top of TiDB Vector and LlamaIndex and DSPy. It features a Perplexity-style Conversational Search page and an Embeddable JavaScript Snippet for easy integration into websites. The tool allows for comprehensive coverage and streamlined search processes through sitemap URL scraping.
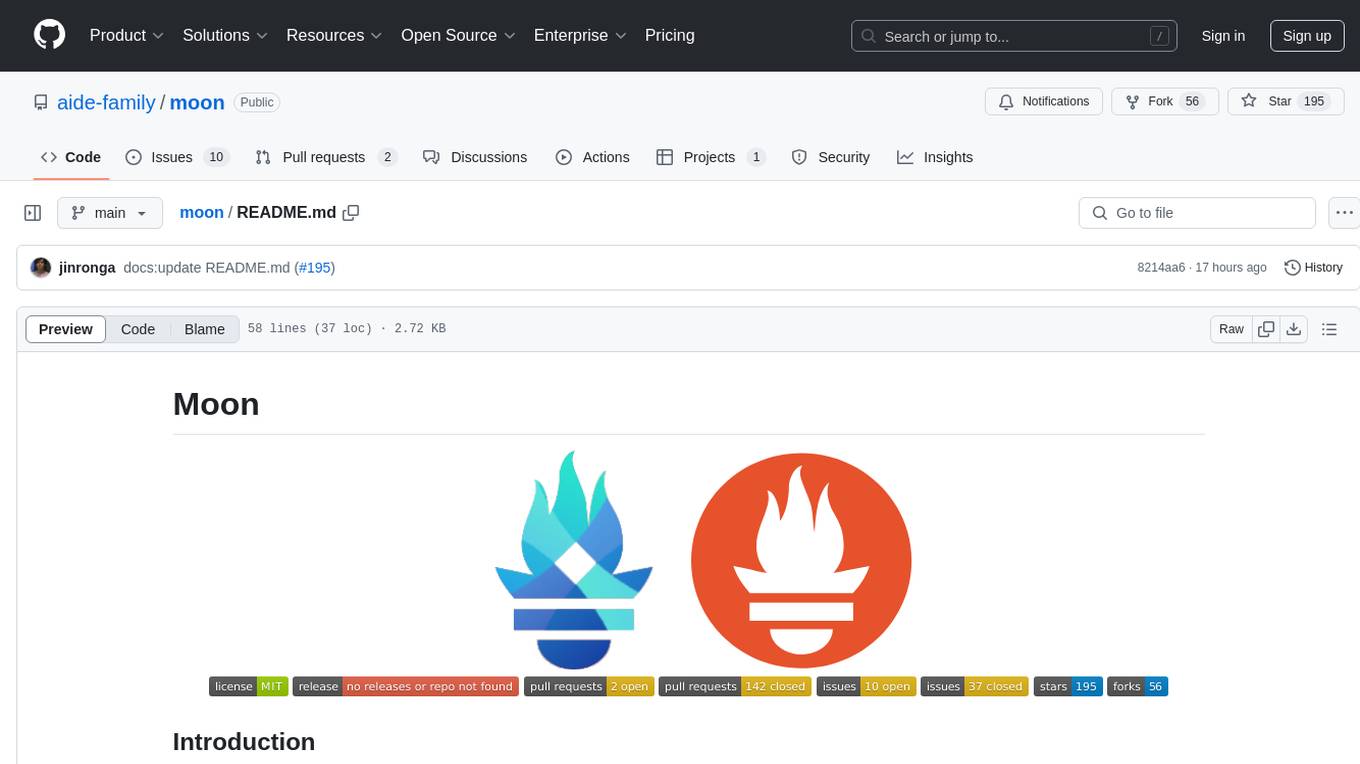
moon
Moon is a monitoring and alerting platform suitable for multiple domains, supporting various application scenarios such as cloud-native, Internet of Things (IoT), and Artificial Intelligence (AI). It simplifies operational work of cloud-native monitoring, boasts strong IoT and AI support capabilities, and meets diverse monitoring needs across industries. Capable of real-time data monitoring, intelligent alerts, and fault response for various fields.
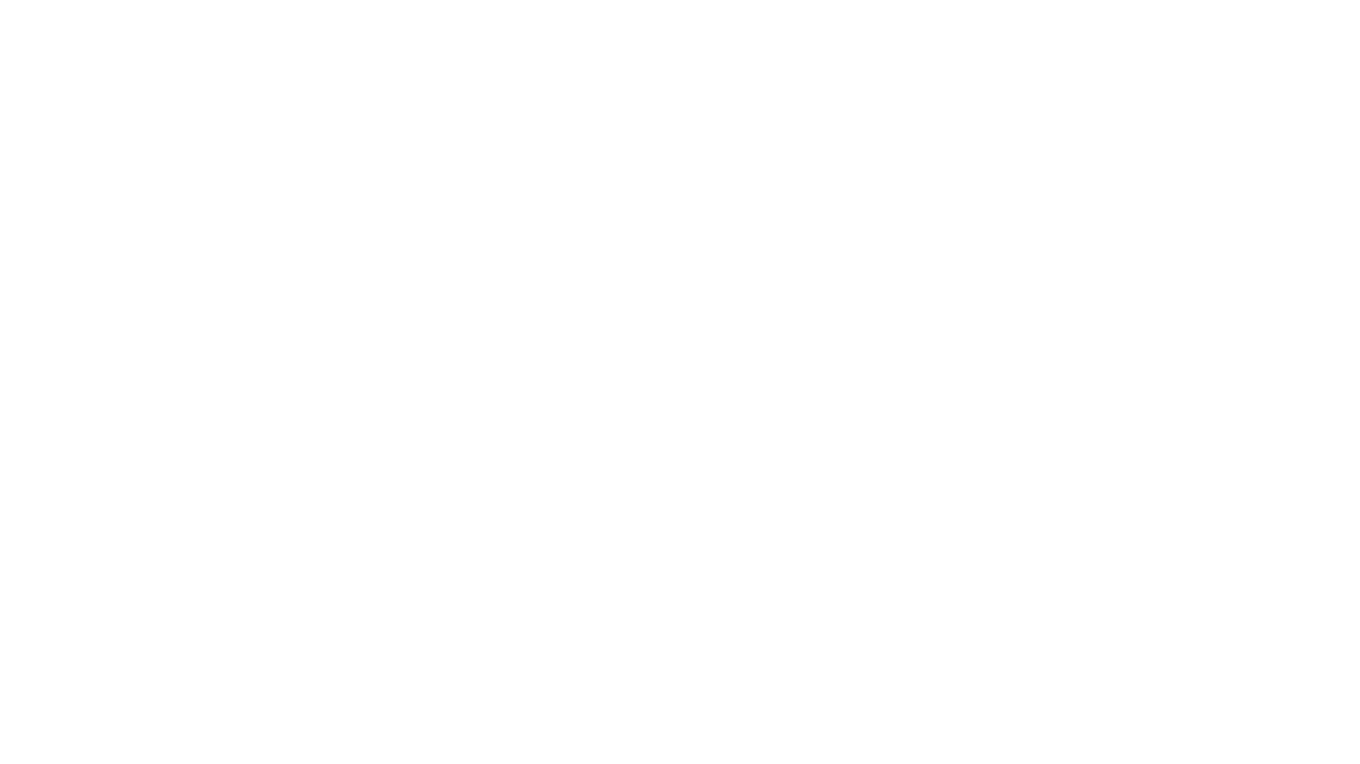
postiz-app
Postiz is an ultimate AI social media scheduling tool that offers everything you need to manage your social media posts, build an audience, capture leads, and grow your business. It allows you to schedule posts with AI features, measure work with analytics, collaborate with team members, and invite others to comment and schedule posts. The tech stack includes NX (Monorepo), NextJS (React), NestJS, Prisma, Redis, and Resend for email notifications.
For similar tasks
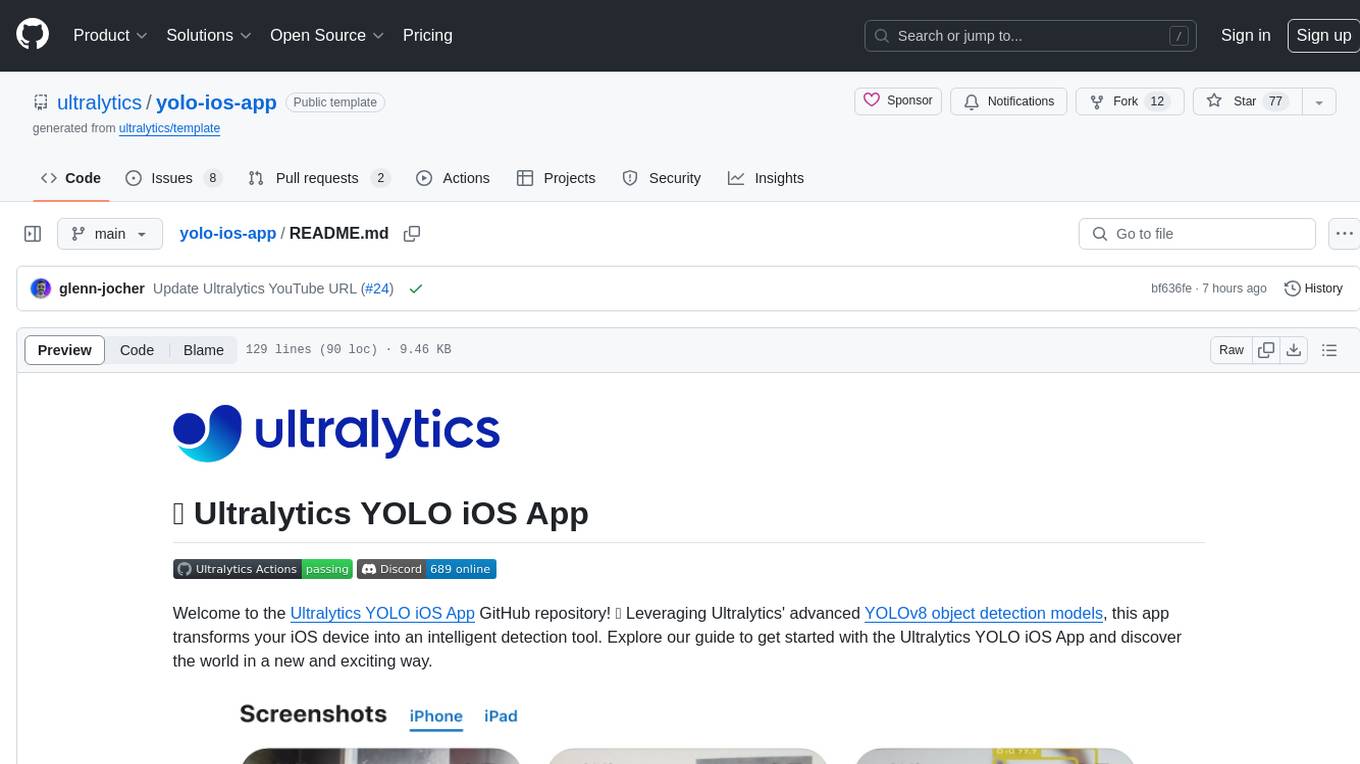
yolo-ios-app
The Ultralytics YOLO iOS App GitHub repository offers an advanced object detection tool leveraging YOLOv8 models for iOS devices. Users can transform their devices into intelligent detection tools to explore the world in a new and exciting way. The app provides real-time detection capabilities with multiple AI models to choose from, ranging from 'nano' to 'x-large'. Contributors are welcome to participate in this open-source project, and licensing options include AGPL-3.0 for open-source use and an Enterprise License for commercial integration. Users can easily set up the app by following the provided steps, including cloning the repository, adding YOLOv8 models, and running the app on their iOS devices.
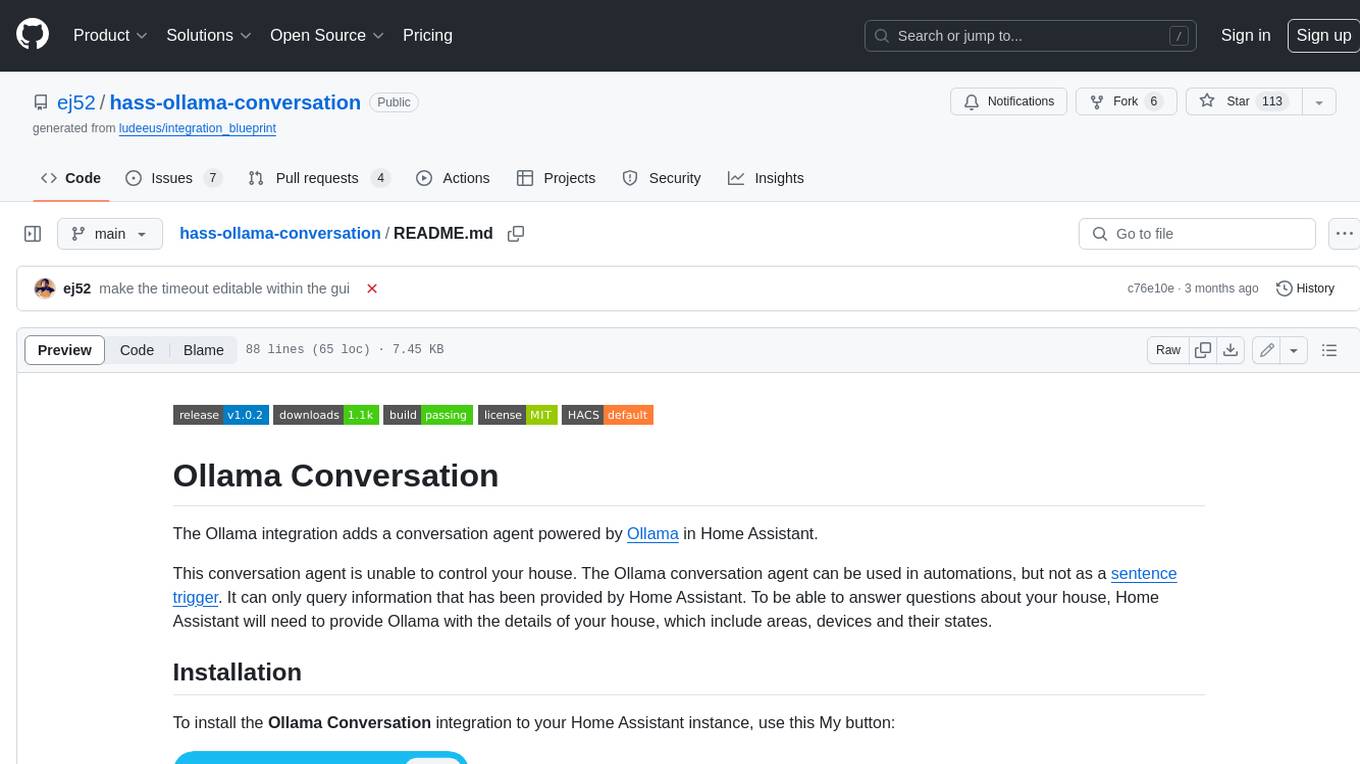
hass-ollama-conversation
The Ollama Conversation integration adds a conversation agent powered by Ollama in Home Assistant. This agent can be used in automations to query information provided by Home Assistant about your house, including areas, devices, and their states. Users can install the integration via HACS and configure settings such as API timeout, model selection, context size, maximum tokens, and other parameters to fine-tune the responses generated by the AI language model. Contributions to the project are welcome, and discussions can be held on the Home Assistant Community platform.
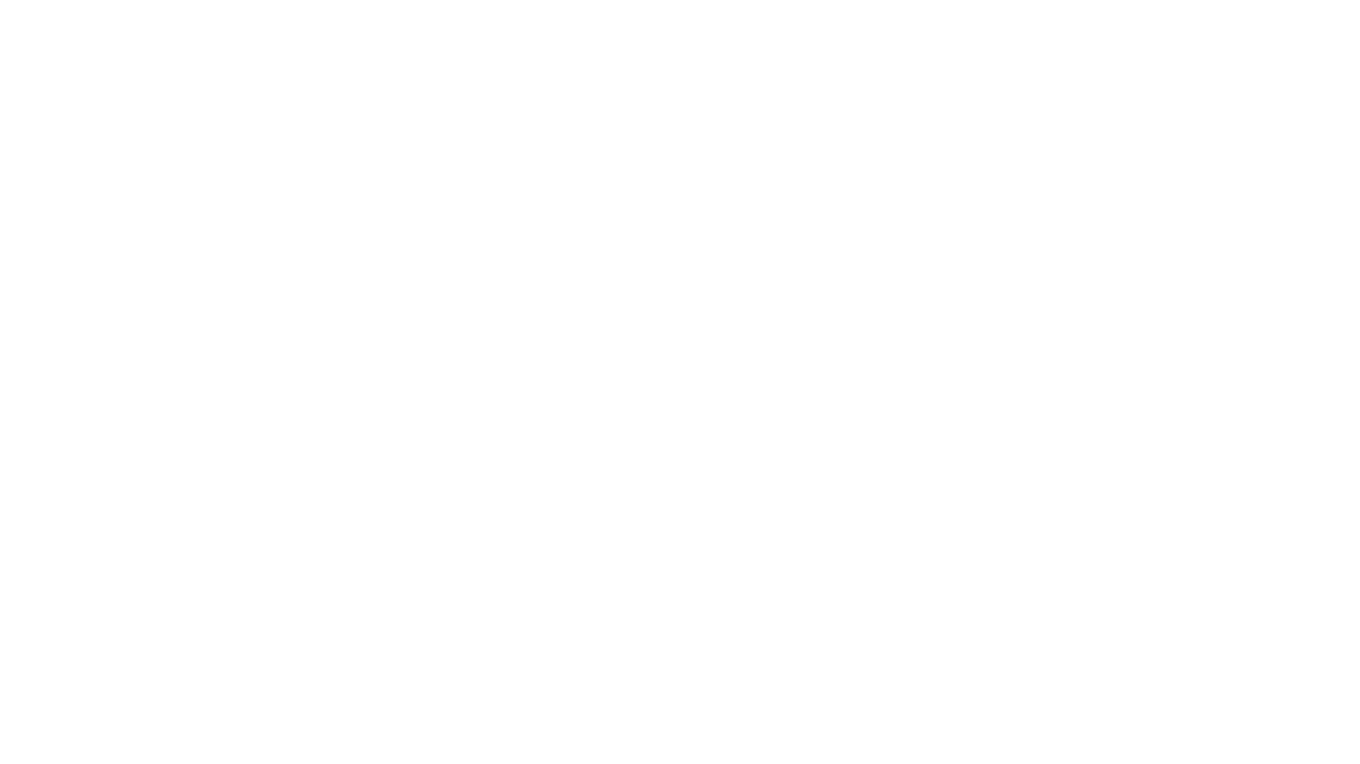
rclip
rclip is a command-line photo search tool powered by the OpenAI's CLIP neural network. It allows users to search for images using text queries, similar image search, and combining multiple queries. The tool extracts features from photos to enable searching and indexing, with options for previewing results in supported terminals or custom viewers. Users can install rclip on Linux, macOS, and Windows using different installation methods. The repository follows the Conventional Commits standard and welcomes contributions from the community.
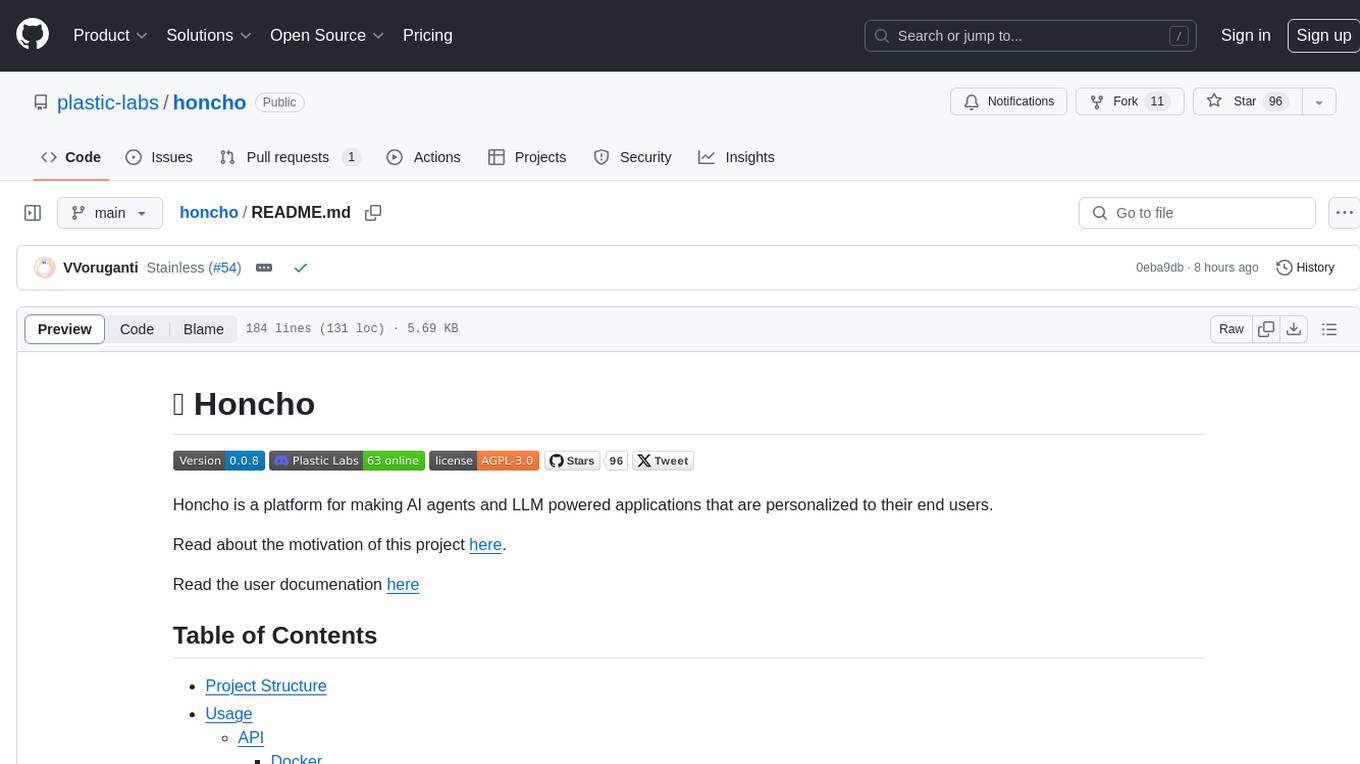
honcho
Honcho is a platform for creating personalized AI agents and LLM powered applications for end users. The repository is a monorepo containing the server/API for managing database interactions and storing application state, along with a Python SDK. It utilizes FastAPI for user context management and Poetry for dependency management. The API can be run using Docker or manually by setting environment variables. The client SDK can be installed using pip or Poetry. The project is open source and welcomes contributions, following a fork and PR workflow. Honcho is licensed under the AGPL-3.0 License.
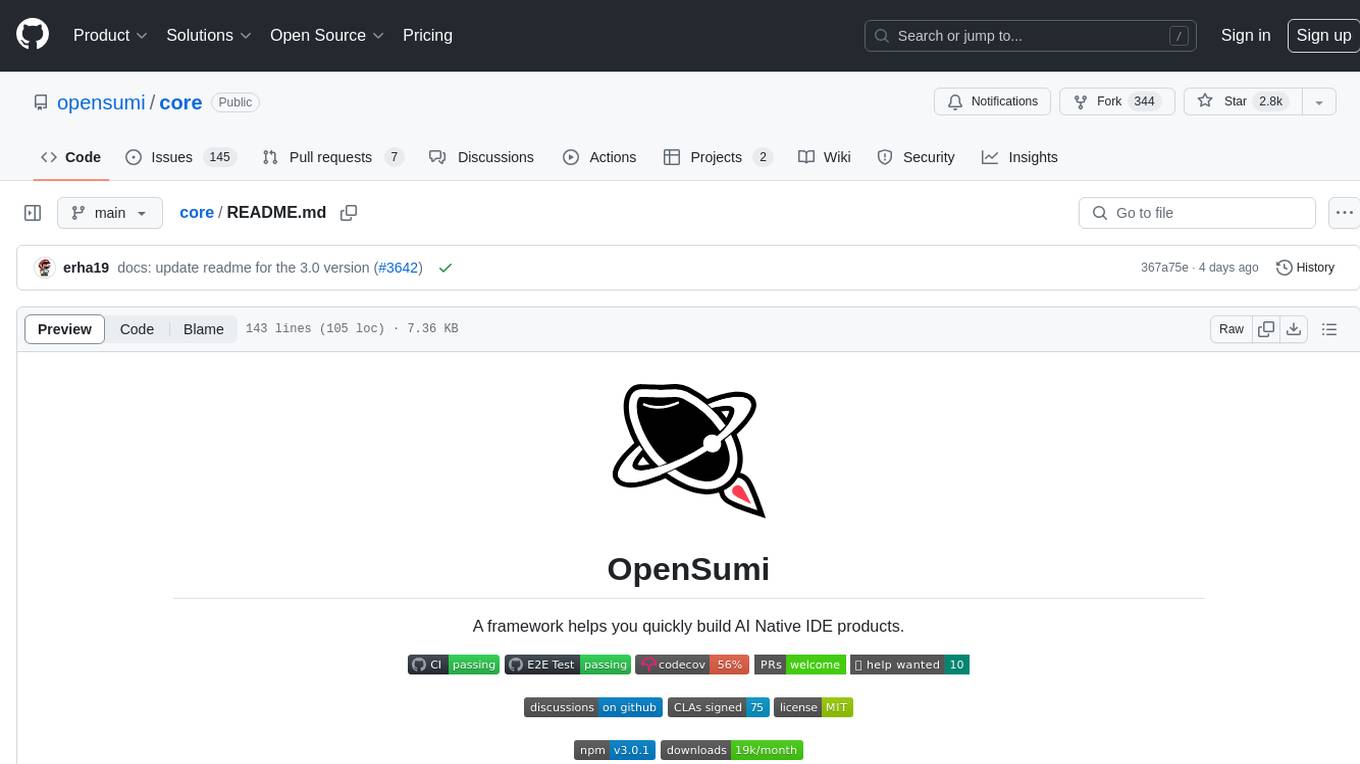
core
OpenSumi is a framework designed to help users quickly build AI Native IDE products. It provides a set of tools and templates for creating Cloud IDEs, Desktop IDEs based on Electron, CodeBlitz web IDE Framework, Lite Web IDE on the Browser, and Mini-App liked IDE. The framework also offers documentation for users to refer to and a detailed guide on contributing to the project. OpenSumi encourages contributions from the community and provides a platform for users to report bugs, contribute code, or improve documentation. The project is licensed under the MIT license and contains third-party code under other open source licenses.
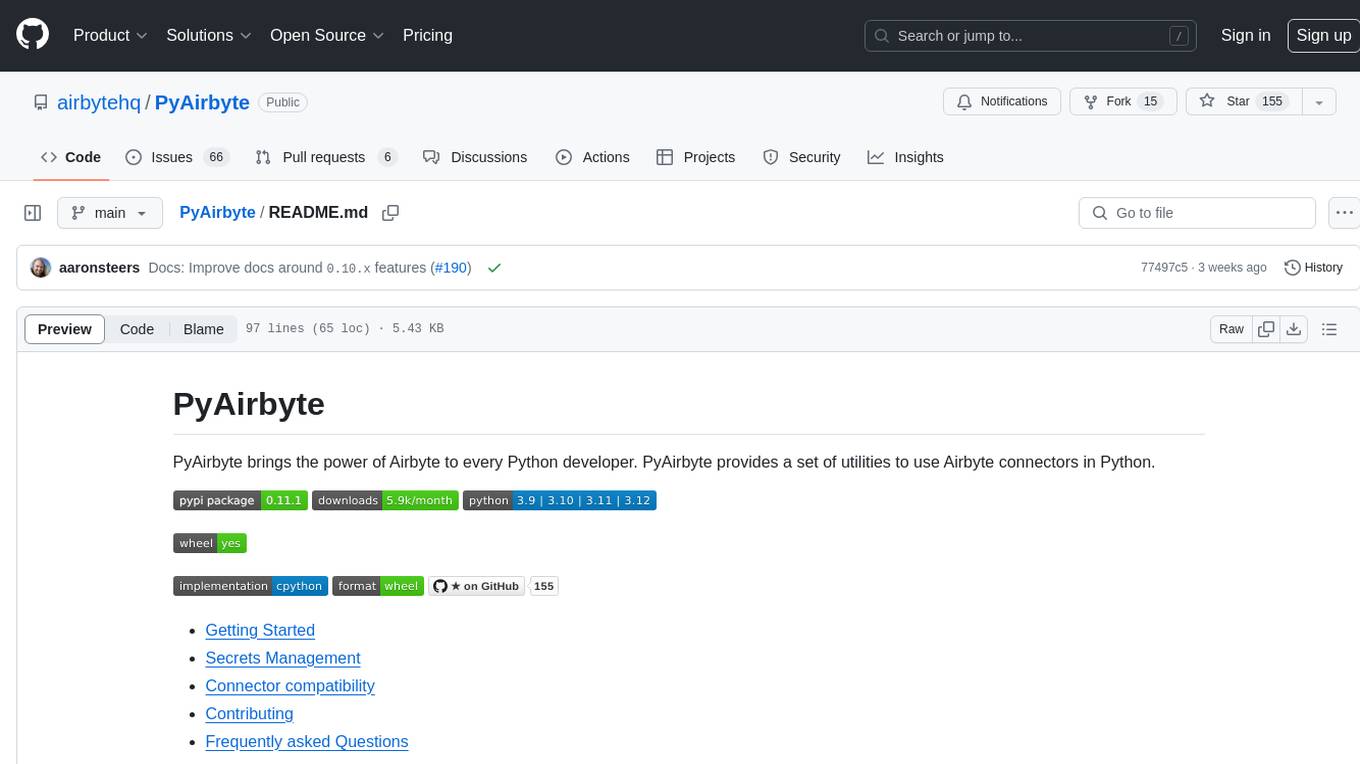
PyAirbyte
PyAirbyte brings the power of Airbyte to every Python developer by providing a set of utilities to use Airbyte connectors in Python. It enables users to easily manage secrets, work with various connectors like GitHub, Shopify, and Postgres, and contribute to the project. PyAirbyte is not a replacement for Airbyte but complements it, supporting data orchestration frameworks like Airflow and Snowpark. Users can develop ETL pipelines and import connectors from local directories. The tool simplifies data integration tasks for Python developers.
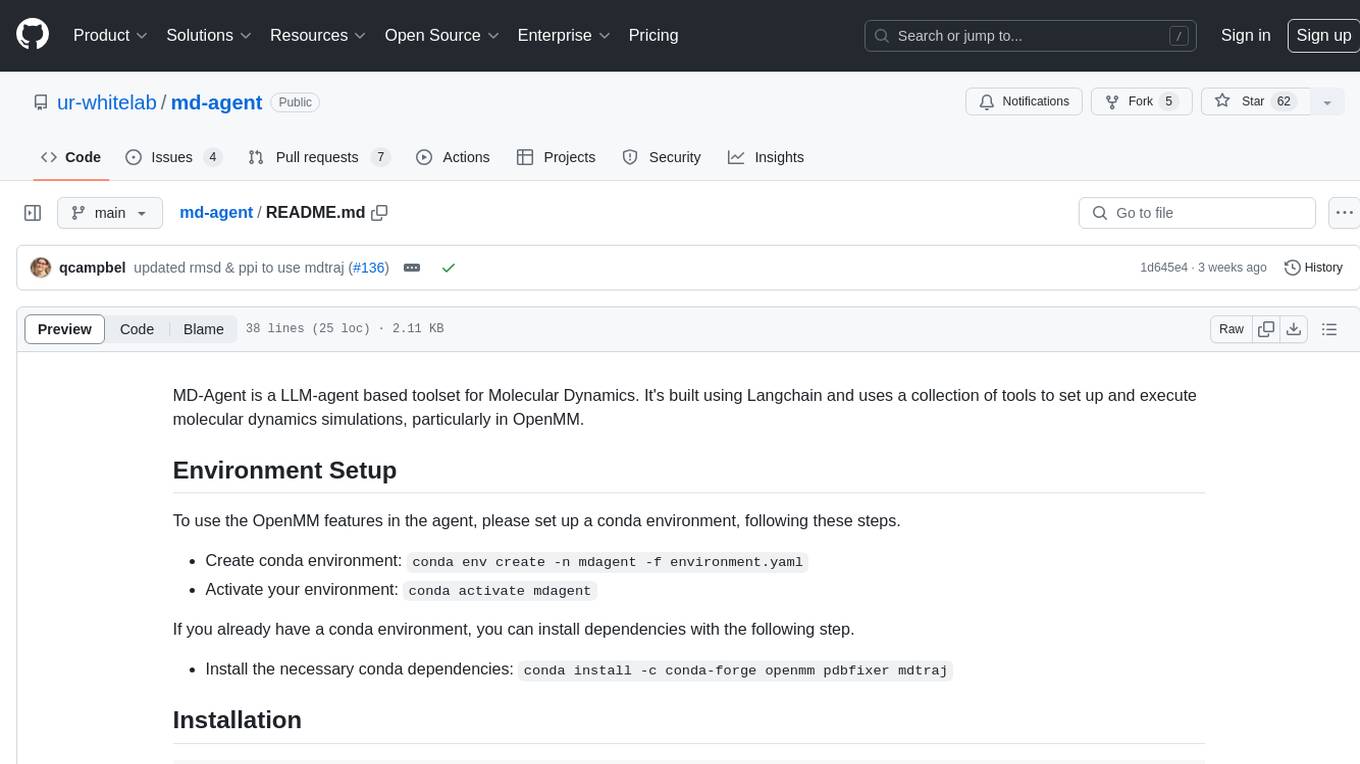
md-agent
MD-Agent is a LLM-agent based toolset for Molecular Dynamics. It uses Langchain and a collection of tools to set up and execute molecular dynamics simulations, particularly in OpenMM. The tool assists in environment setup, installation, and usage by providing detailed steps. It also requires API keys for certain functionalities, such as OpenAI and paper-qa for literature searches. Contributions to the project are welcome, with a detailed Contributor's Guide available for interested individuals.
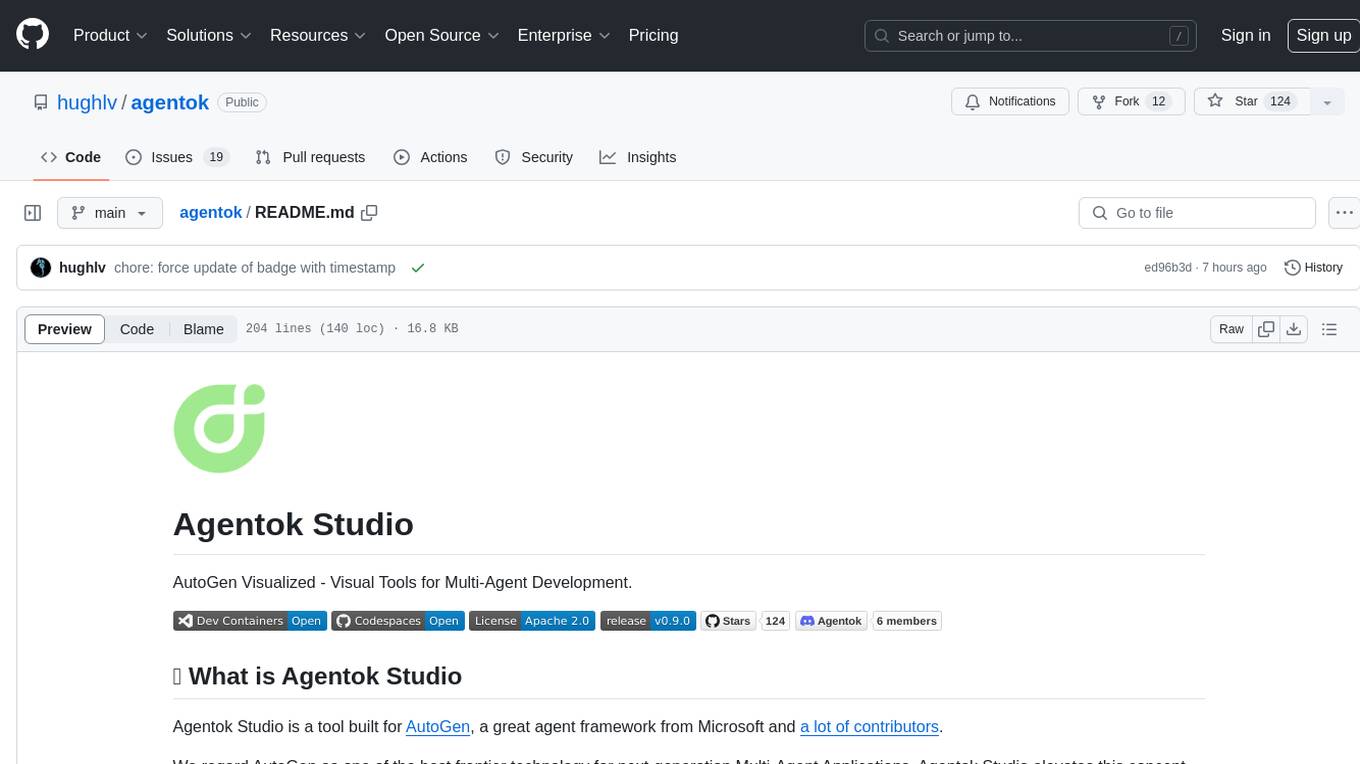
flowgen
FlowGen is a tool built for AutoGen, a great agent framework from Microsoft and a lot of contributors. It provides intuitive visual tools that streamline the construction and oversight of complex agent-based workflows, simplifying the process for creators and developers. Users can create Autoflows, chat with agents, and share flow templates. The tool is fully dockerized and supports deployment on Railway.app. Contributions to the project are welcome, and the platform uses semantic-release for versioning and releases.
For similar jobs
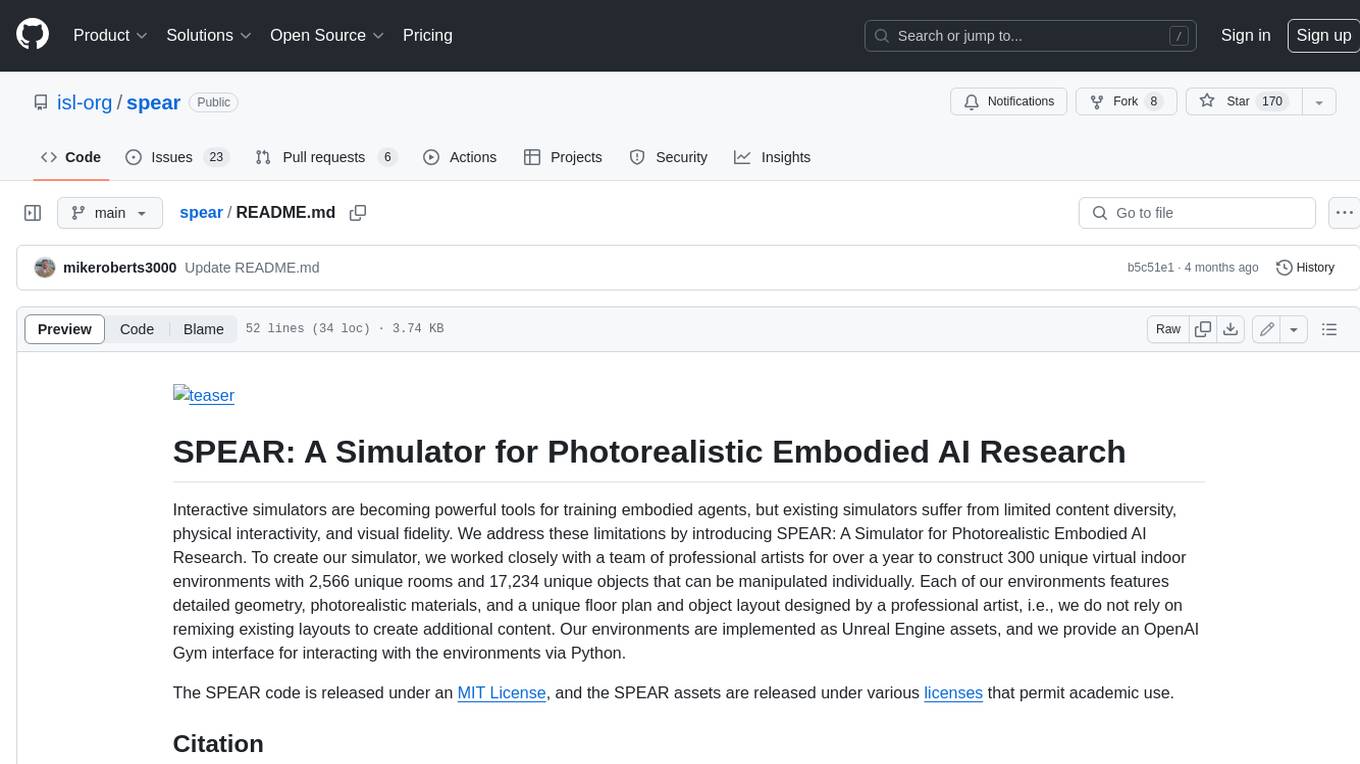
spear
SPEAR (Simulator for Photorealistic Embodied AI Research) is a powerful tool for training embodied agents. It features 300 unique virtual indoor environments with 2,566 unique rooms and 17,234 unique objects that can be manipulated individually. Each environment is designed by a professional artist and features detailed geometry, photorealistic materials, and a unique floor plan and object layout. SPEAR is implemented as Unreal Engine assets and provides an OpenAI Gym interface for interacting with the environments via Python.
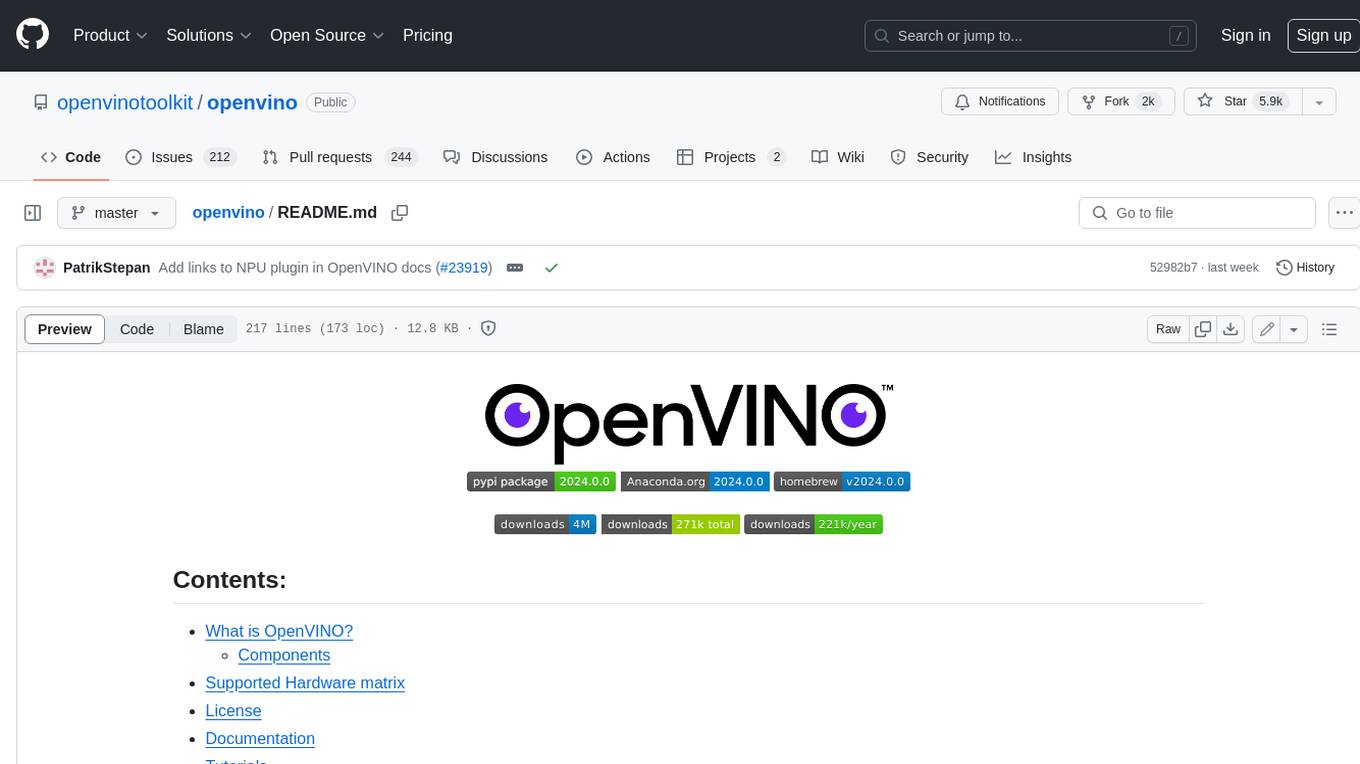
openvino
OpenVINO™ is an open-source toolkit for optimizing and deploying AI inference. It provides a common API to deliver inference solutions on various platforms, including CPU, GPU, NPU, and heterogeneous devices. OpenVINO™ supports pre-trained models from Open Model Zoo and popular frameworks like TensorFlow, PyTorch, and ONNX. Key components of OpenVINO™ include the OpenVINO™ Runtime, plugins for different hardware devices, frontends for reading models from native framework formats, and the OpenVINO Model Converter (OVC) for adjusting models for optimal execution on target devices.
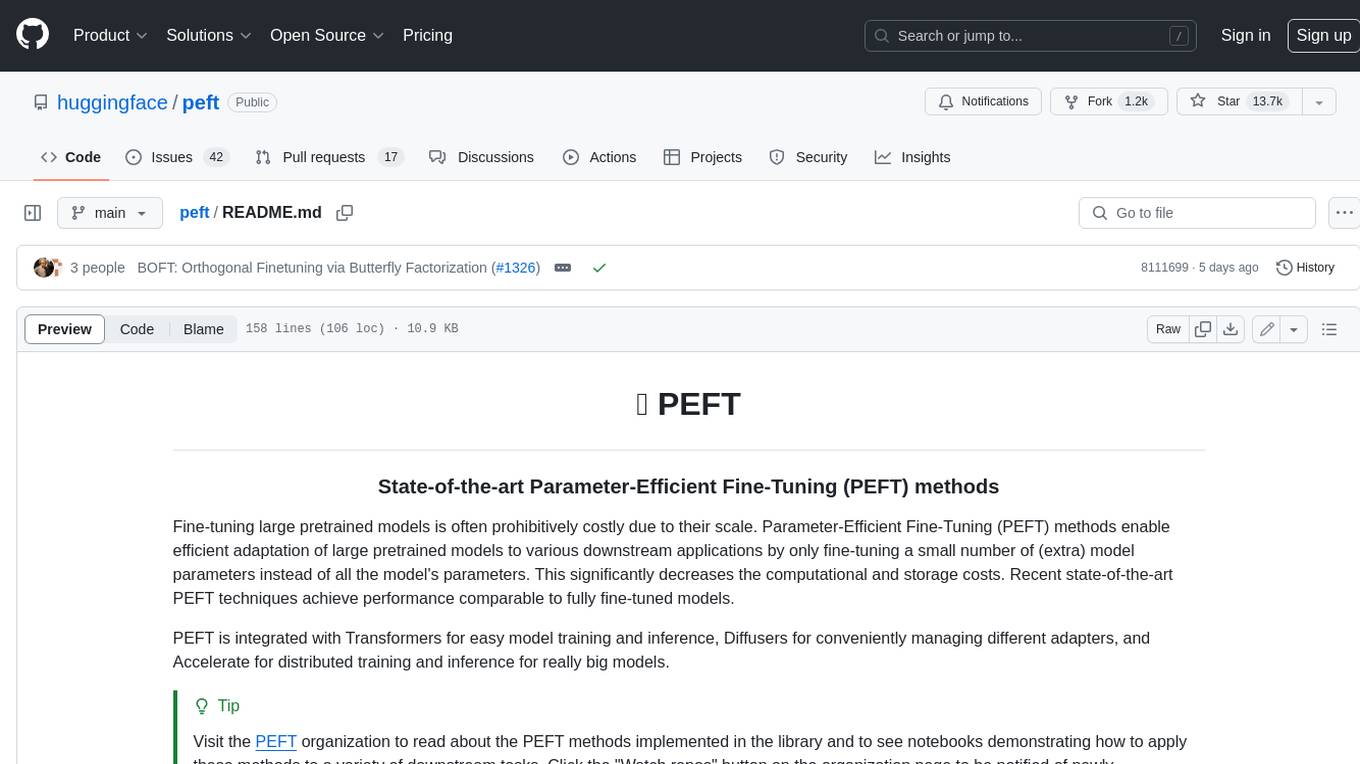
peft
PEFT (Parameter-Efficient Fine-Tuning) is a collection of state-of-the-art methods that enable efficient adaptation of large pretrained models to various downstream applications. By only fine-tuning a small number of extra model parameters instead of all the model's parameters, PEFT significantly decreases the computational and storage costs while achieving performance comparable to fully fine-tuned models.
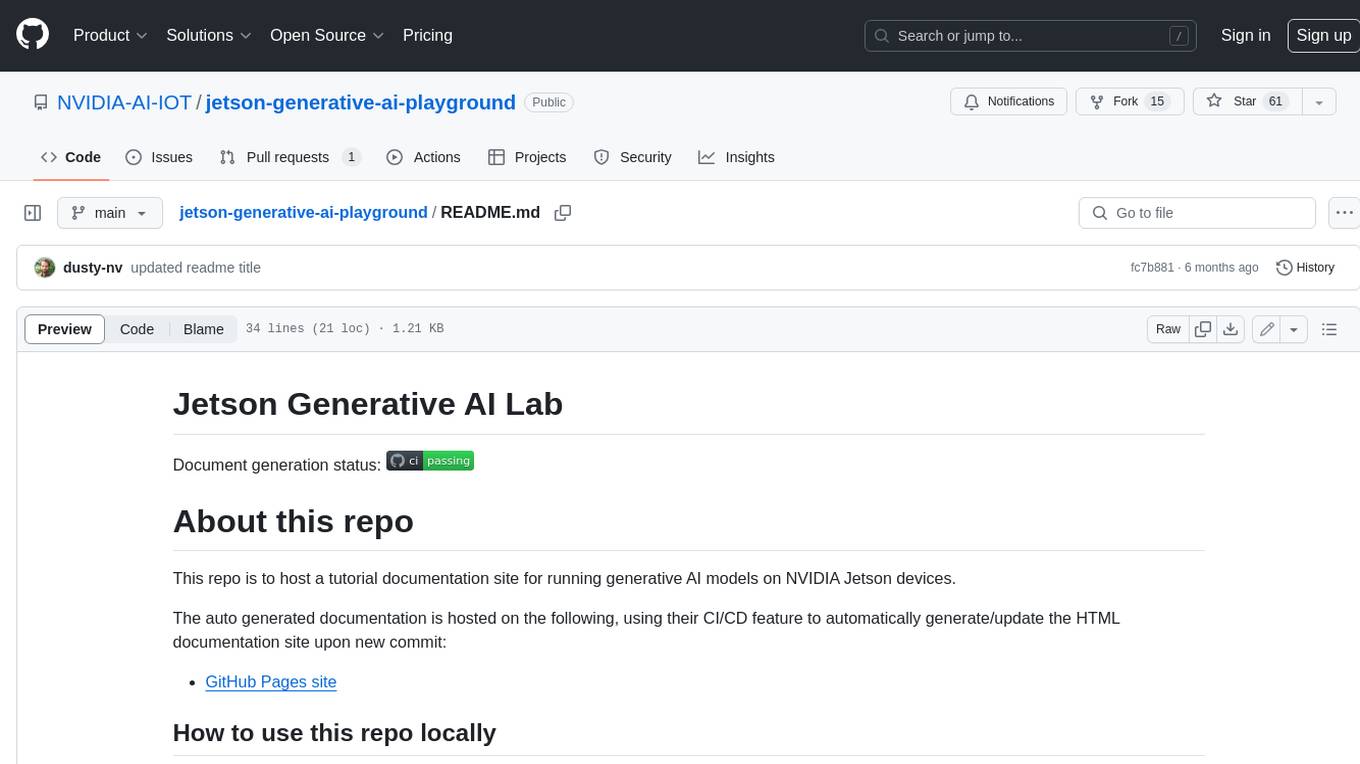
jetson-generative-ai-playground
This repo hosts tutorial documentation for running generative AI models on NVIDIA Jetson devices. The documentation is auto-generated and hosted on GitHub Pages using their CI/CD feature to automatically generate/update the HTML documentation site upon new commits.
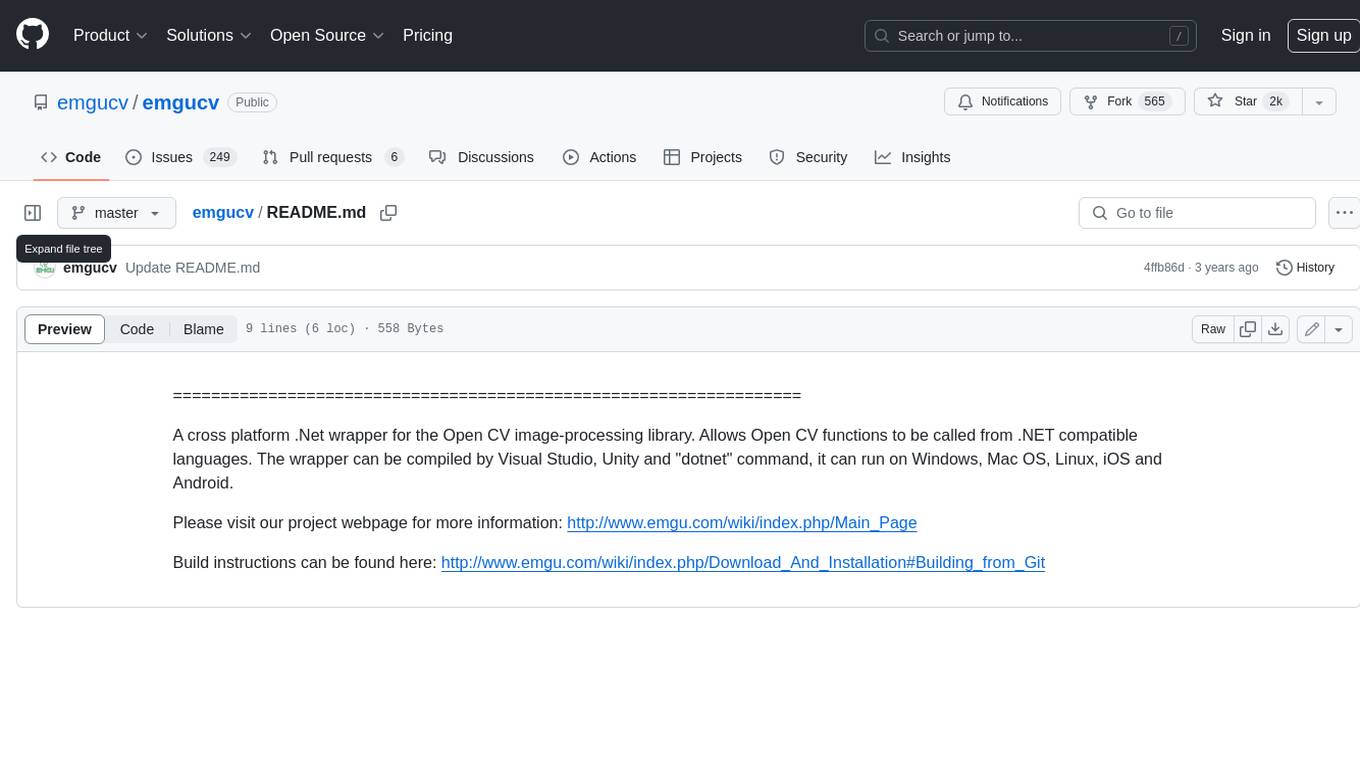
emgucv
Emgu CV is a cross-platform .Net wrapper for the OpenCV image-processing library. It allows OpenCV functions to be called from .NET compatible languages. The wrapper can be compiled by Visual Studio, Unity, and "dotnet" command, and it can run on Windows, Mac OS, Linux, iOS, and Android.
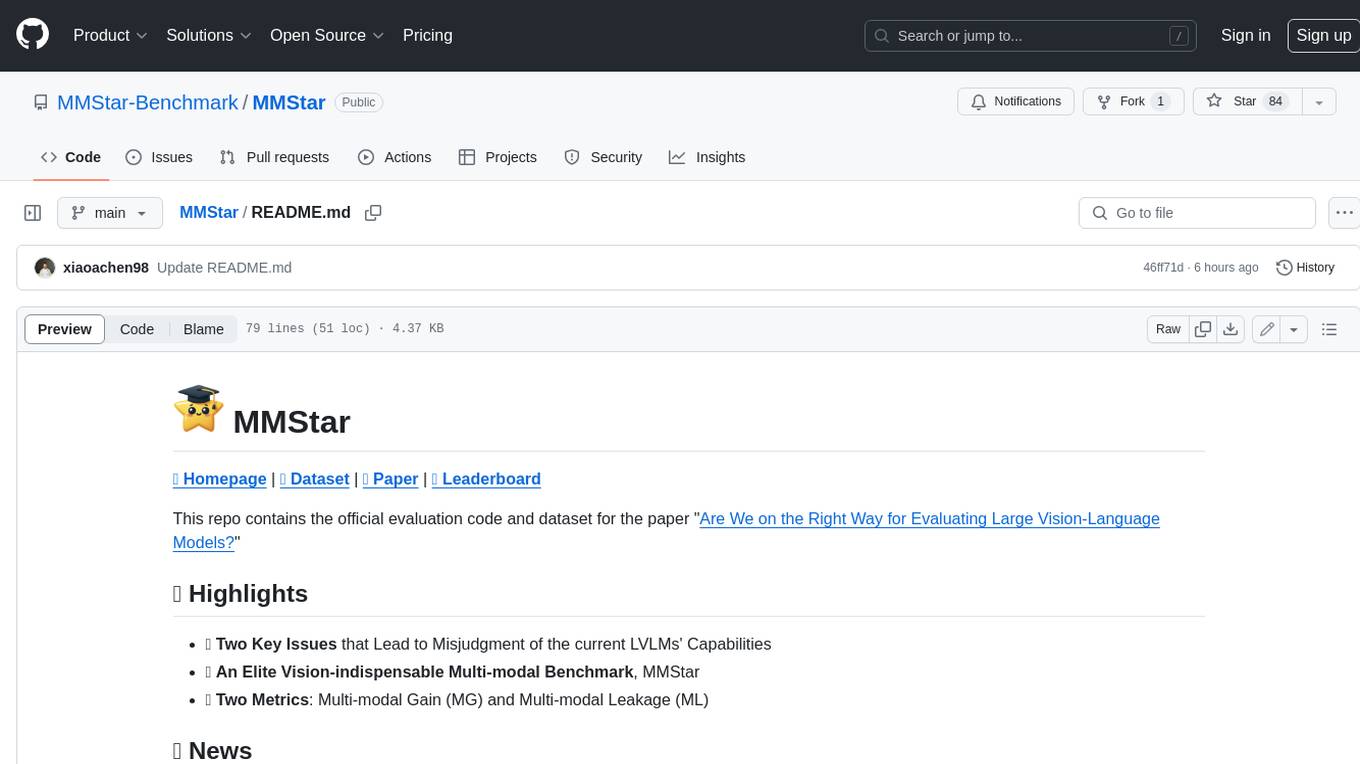
MMStar
MMStar is an elite vision-indispensable multi-modal benchmark comprising 1,500 challenge samples meticulously selected by humans. It addresses two key issues in current LLM evaluation: the unnecessary use of visual content in many samples and the existence of unintentional data leakage in LLM and LVLM training. MMStar evaluates 6 core capabilities across 18 detailed axes, ensuring a balanced distribution of samples across all dimensions.
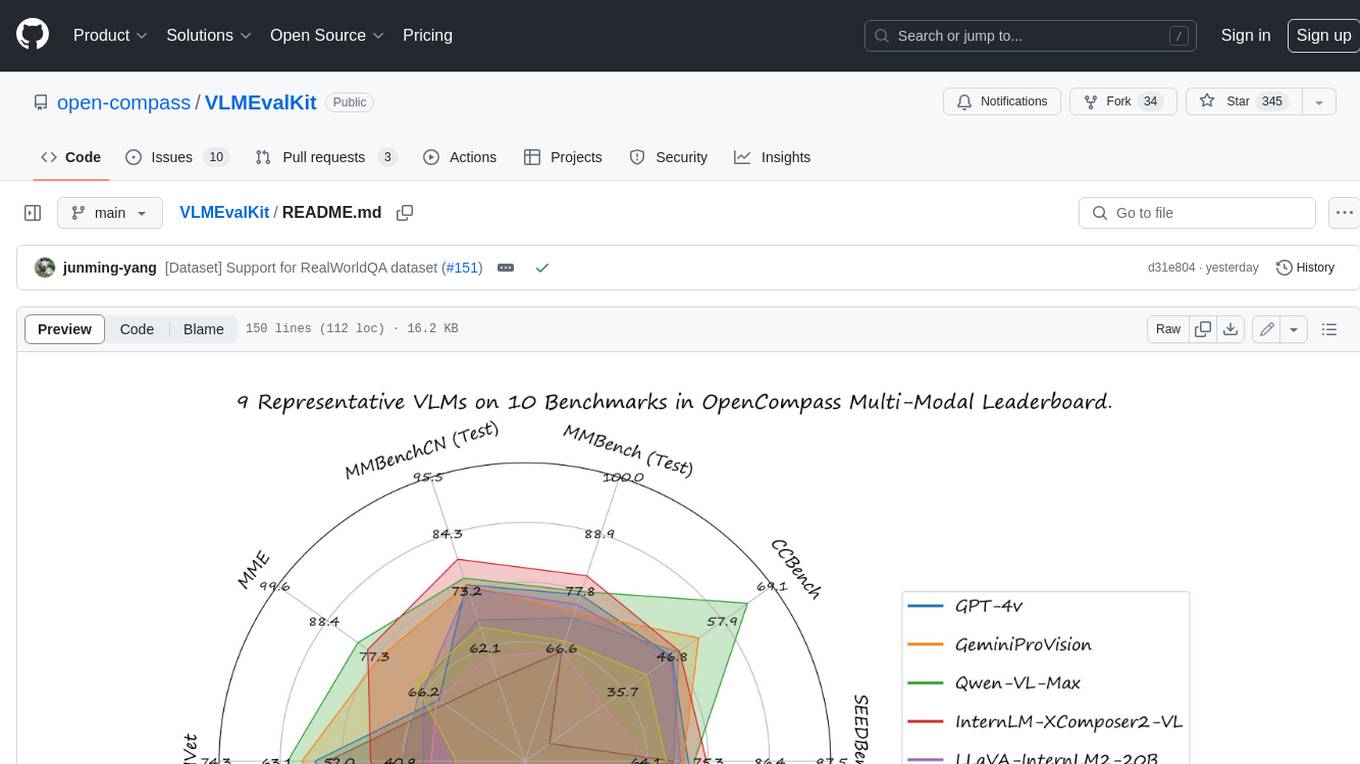
VLMEvalKit
VLMEvalKit is an open-source evaluation toolkit of large vision-language models (LVLMs). It enables one-command evaluation of LVLMs on various benchmarks, without the heavy workload of data preparation under multiple repositories. In VLMEvalKit, we adopt generation-based evaluation for all LVLMs, and provide the evaluation results obtained with both exact matching and LLM-based answer extraction.
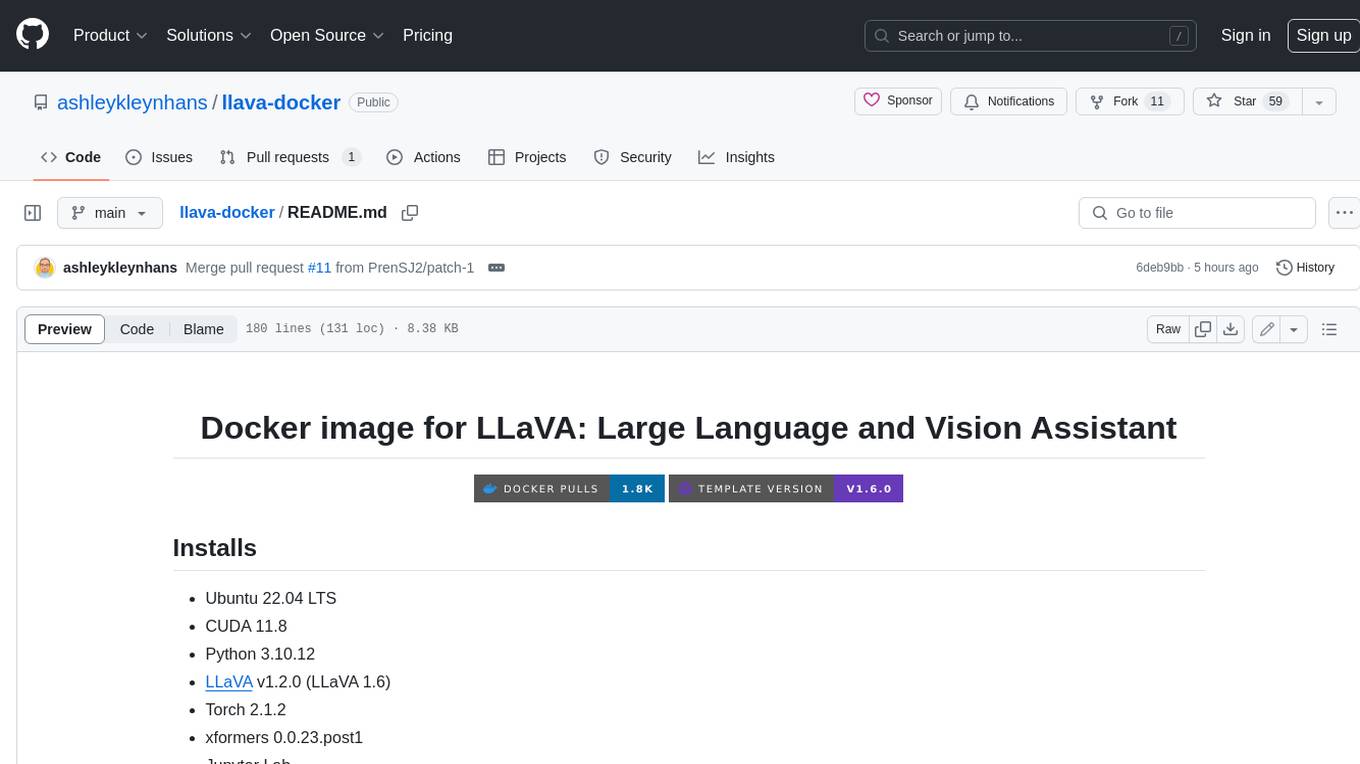
llava-docker
This Docker image for LLaVA (Large Language and Vision Assistant) provides a convenient way to run LLaVA locally or on RunPod. LLaVA is a powerful AI tool that combines natural language processing and computer vision capabilities. With this Docker image, you can easily access LLaVA's functionalities for various tasks, including image captioning, visual question answering, text summarization, and more. The image comes pre-installed with LLaVA v1.2.0, Torch 2.1.2, xformers 0.0.23.post1, and other necessary dependencies. You can customize the model used by setting the MODEL environment variable. The image also includes a Jupyter Lab environment for interactive development and exploration. Overall, this Docker image offers a comprehensive and user-friendly platform for leveraging LLaVA's capabilities.