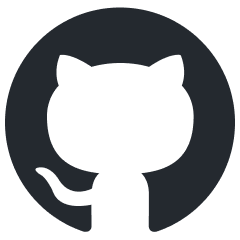
aikit
🏗️ Fine-tune, build, and deploy open-source LLMs easily!
Stars: 425
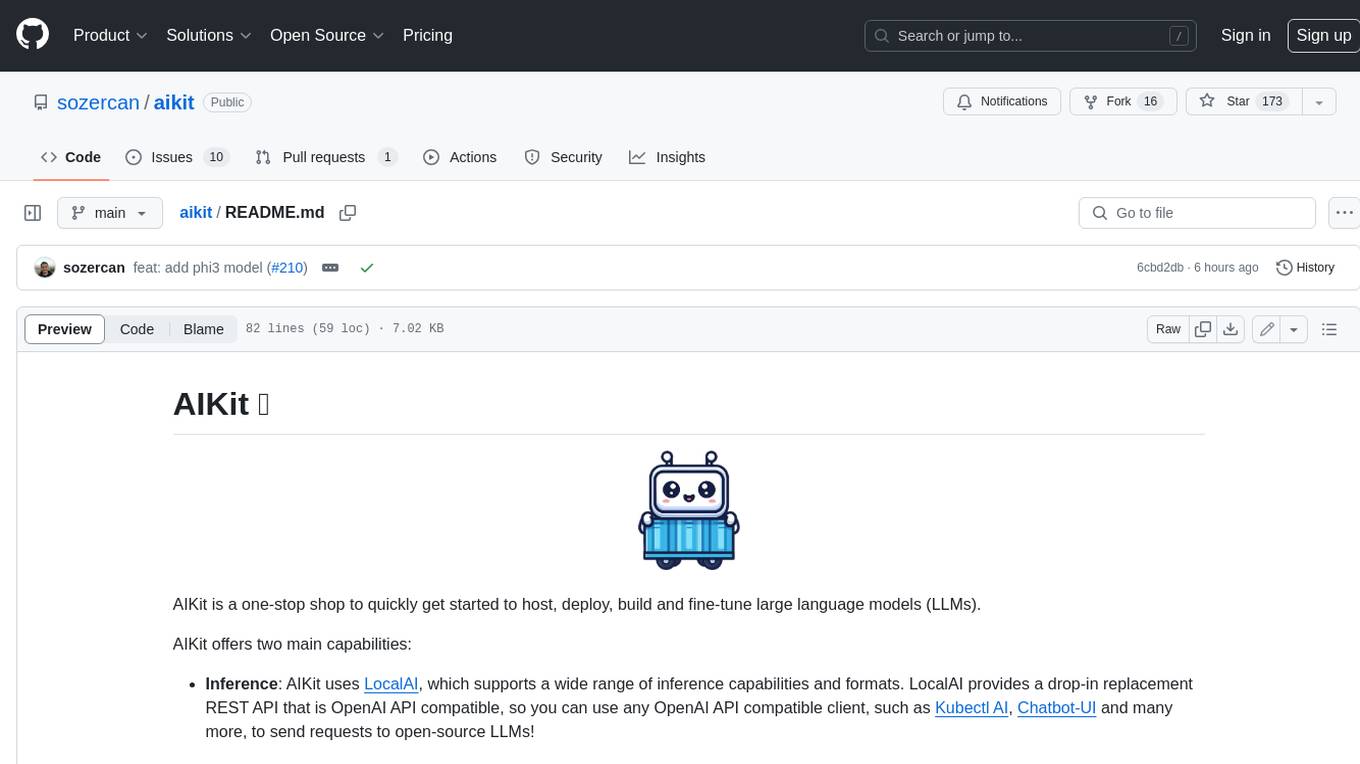
AIKit is a one-stop shop to quickly get started to host, deploy, build and fine-tune large language models (LLMs). AIKit offers two main capabilities: Inference: AIKit uses LocalAI, which supports a wide range of inference capabilities and formats. LocalAI provides a drop-in replacement REST API that is OpenAI API compatible, so you can use any OpenAI API compatible client, such as Kubectl AI, Chatbot-UI and many more, to send requests to open-source LLMs! Fine Tuning: AIKit offers an extensible fine tuning interface. It supports Unsloth for fast, memory efficient, and easy fine-tuning experience.
README:
AIKit is a comprehensive platform to quickly get started to host, deploy, build and fine-tune large language models (LLMs).
AIKit offers two main capabilities:
-
Inference: AIKit uses LocalAI, which supports a wide range of inference capabilities and formats. LocalAI provides a drop-in replacement REST API that is OpenAI API compatible, so you can use any OpenAI API compatible client, such as Kubectl AI, Chatbot-UI and many more, to send requests to open LLMs!
-
Fine-Tuning: AIKit offers an extensible fine-tuning interface. It supports Unsloth for fast, memory efficient, and easy fine-tuning experience.
👉 For full documentation, please see AIKit website!
- 🐳 No GPU, Internet access or additional tools needed except for Docker!
- 🤏 Minimal image size, resulting in less vulnerabilities and smaller attack surface with a custom distroless-based image
- 🎵 Fine-tune support
- 🚀 Easy to use declarative configuration for inference and fine-tuning
- ✨ OpenAI API compatible to use with any OpenAI API compatible client
- 📸 Multi-modal model support
- 🖼️ Image generation support
- 🦙 Support for GGUF (
llama
), GPTQ or EXL2 (exllama2
), and GGML (llama-ggml
) and Mamba models - 🚢 Kubernetes deployment ready
- 📦 Supports multiple models with a single image
- 🖥️ Supports AMD64 and ARM64 CPUs and GPU-accelerated inferencing with NVIDIA GPUs
- 🔐 Ensure supply chain security with SBOMs, Provenance attestations, and signed images
- 🌈 Supports air-gapped environments with self-hosted, local, or any remote container registries to store model images for inference on the edge.
You can get started with AIKit quickly on your local machine without a GPU!
docker run -d --rm -p 8080:8080 ghcr.io/sozercan/llama3.1:8b
After running this, navigate to http://localhost:8080/chat to access the WebUI!
AIKit provides an OpenAI API compatible endpoint, so you can use any OpenAI API compatible client to send requests to open LLMs!
curl http://localhost:8080/v1/chat/completions -H "Content-Type: application/json" -d '{
"model": "llama-3.1-8b-instruct",
"messages": [{"role": "user", "content": "explain kubernetes in a sentence"}]
}'
Output should be similar to:
{
// ...
"model": "llama-3.1-8b-instruct",
"choices": [
{
"index": 0,
"finish_reason": "stop",
"message": {
"role": "assistant",
"content": "Kubernetes is an open-source container orchestration system that automates the deployment, scaling, and management of applications and services, allowing developers to focus on writing code rather than managing infrastructure."
}
}
],
// ...
}
That's it! 🎉 API is OpenAI compatible so this is a drop-in replacement for any OpenAI API compatible client.
AIKit comes with pre-made models that you can use out-of-the-box!
If it doesn't include a specific model, you can always create your own images, and host in a container registry of your choice!
[!NOTE] AIKit supports both AMD64 and ARM64 CPUs. You can run the same command on either architecture, and Docker will automatically pull the correct image for your CPU.
Depending on your CPU capabilities, AIKit will automatically select the most optimized instruction set.
Model | Optimization | Parameters | Command | Model Name | License |
---|---|---|---|---|---|
🦙 Llama 3.2 | Instruct | 1B | docker run -d --rm -p 8080:8080 ghcr.io/sozercan/llama3.2:1b |
llama-3.2-1b-instruct |
Llama |
🦙 Llama 3.2 | Instruct | 3B | docker run -d --rm -p 8080:8080 ghcr.io/sozercan/llama3.2:3b |
llama-3.2-3b-instruct |
Llama |
🦙 Llama 3.1 | Instruct | 8B | docker run -d --rm -p 8080:8080 ghcr.io/sozercan/llama3.1:8b |
llama-3.1-8b-instruct |
Llama |
🦙 Llama 3.3 | Instruct | 70B | docker run -d --rm -p 8080:8080 ghcr.io/sozercan/llama3.3:70b |
llama-3.3-70b-instruct |
Llama |
Instruct | 8x7B | docker run -d --rm -p 8080:8080 ghcr.io/sozercan/mixtral:8x7b |
mixtral-8x7b-instruct |
Apache | |
Instruct | 3.8B | docker run -d --rm -p 8080:8080 ghcr.io/sozercan/phi3.5:3.8b |
phi-3.5-3.8b-instruct |
MIT | |
🔡 Gemma 2 | Instruct | 2B | docker run -d --rm -p 8080:8080 ghcr.io/sozercan/gemma2:2b |
gemma-2-2b-instruct |
Gemma |
⌨️ Codestral 0.1 | Code | 22B | docker run -d --rm -p 8080:8080 ghcr.io/sozercan/codestral:22b |
codestral-22b |
MNLP |
QwQ | 32B | docker run -d --rm -p 8080:8080 ghcr.io/sozercan/qwq:32b |
qwq-32b-preview |
Apache 2.0 |
[!NOTE] To enable GPU acceleration, please see GPU Acceleration.
Please note that only difference between CPU and GPU section is the
--gpus all
flag in the command to enable GPU acceleration.
Model | Optimization | Parameters | Command | Model Name | License |
---|---|---|---|---|---|
🦙 Llama 3.2 | Instruct | 1B | docker run -d --rm --gpus all -p 8080:8080 ghcr.io/sozercan/llama3.2:1b |
llama-3.2-1b-instruct |
Llama |
🦙 Llama 3.2 | Instruct | 3B | docker run -d --rm --gpus all -p 8080:8080 ghcr.io/sozercan/llama3.2:3b |
llama-3.2-3b-instruct |
Llama |
🦙 Llama 3.1 | Instruct | 8B | docker run -d --rm --gpus all -p 8080:8080 ghcr.io/sozercan/llama3.1:8b |
llama-3.1-8b-instruct |
Llama |
🦙 Llama 3.3 | Instruct | 70B | docker run -d --rm --gpus all -p 8080:8080 ghcr.io/sozercan/llama3.3:70b |
llama-3.3-70b-instruct |
Llama |
Instruct | 8x7B | docker run -d --rm --gpus all -p 8080:8080 ghcr.io/sozercan/mixtral:8x7b |
mixtral-8x7b-instruct |
Apache | |
Instruct | 3.8B | docker run -d --rm --gpus all -p 8080:8080 ghcr.io/sozercan/phi3.5:3.8b |
phi-3.5-3.8b-instruct |
MIT | |
🔡 Gemma 2 | Instruct | 2B | docker run -d --rm --gpus all -p 8080:8080 ghcr.io/sozercan/gemma2:2b |
gemma-2-2b-instruct |
Gemma |
⌨️ Codestral 0.1 | Code | 22B | docker run -d --rm --gpus all -p 8080:8080 ghcr.io/sozercan/codestral:22b |
codestral-22b |
MNLP |
QwQ | 32B | docker run -d --rm --gpus all -p 8080:8080 ghcr.io/sozercan/qwq:32b |
qwq-32b-preview |
Apache 2.0 | |
📸 Flux 1 Dev | Text to image | 12B | docker run -d --rm --gpus all -p 8080:8080 ghcr.io/sozercan/flux1:dev |
flux-1-dev |
FLUX.1 [dev] Non-Commercial License |
[!NOTE] To enable GPU acceleration on Apple Silicon, please see Podman Desktop documentation. For more information, please see GPU Acceleration.
Apple Silicon is an experimental runtime and it may change in the future. This runtime is specific to Apple Silicon only, and it will not work as expected on other architectures, including Intel Macs.
Only
gguf
models are supported on Apple Silicon.
Model | Optimization | Parameters | Command | Model Name | License |
---|---|---|---|---|---|
🦙 Llama 3.2 | Instruct | 1B | podman run -d --rm --device /dev/dri -p 8080:8080 ghcr.io/sozercan/applesilicon/llama3.2:1b |
llama-3.2-1b-instruct |
Llama |
🦙 Llama 3.2 | Instruct | 3B | podman run -d --rm --device /dev/dri -p 8080:8080 ghcr.io/sozercan/applesilicon/llama3.2:3b |
llama-3.2-3b-instruct |
Llama |
🦙 Llama 3.1 | Instruct | 8B | podman run -d --rm --device /dev/dri -p 8080:8080 ghcr.io/sozercan/applesilicon/llama3.1:8b |
llama-3.1-8b-instruct |
Llama |
Instruct | 3.8B | podman run -d --rm --device /dev/dri -p 8080:8080 ghcr.io/sozercan/applesilicon/phi3.5:3.8b |
phi-3.5-3.8b-instruct |
MIT | |
🔡 Gemma 2 | Instruct | 2B | podman run -d --rm --device /dev/dri -p 8080:8080 ghcr.io/sozercan/applesilicon/gemma2:2b |
gemma-2-2b-instruct |
Gemma |
👉 For more information and how to fine tune models or create your own images, please see AIKit website!
For Tasks:
Click tags to check more tools for each tasksFor Jobs:
Alternative AI tools for aikit
Similar Open Source Tools
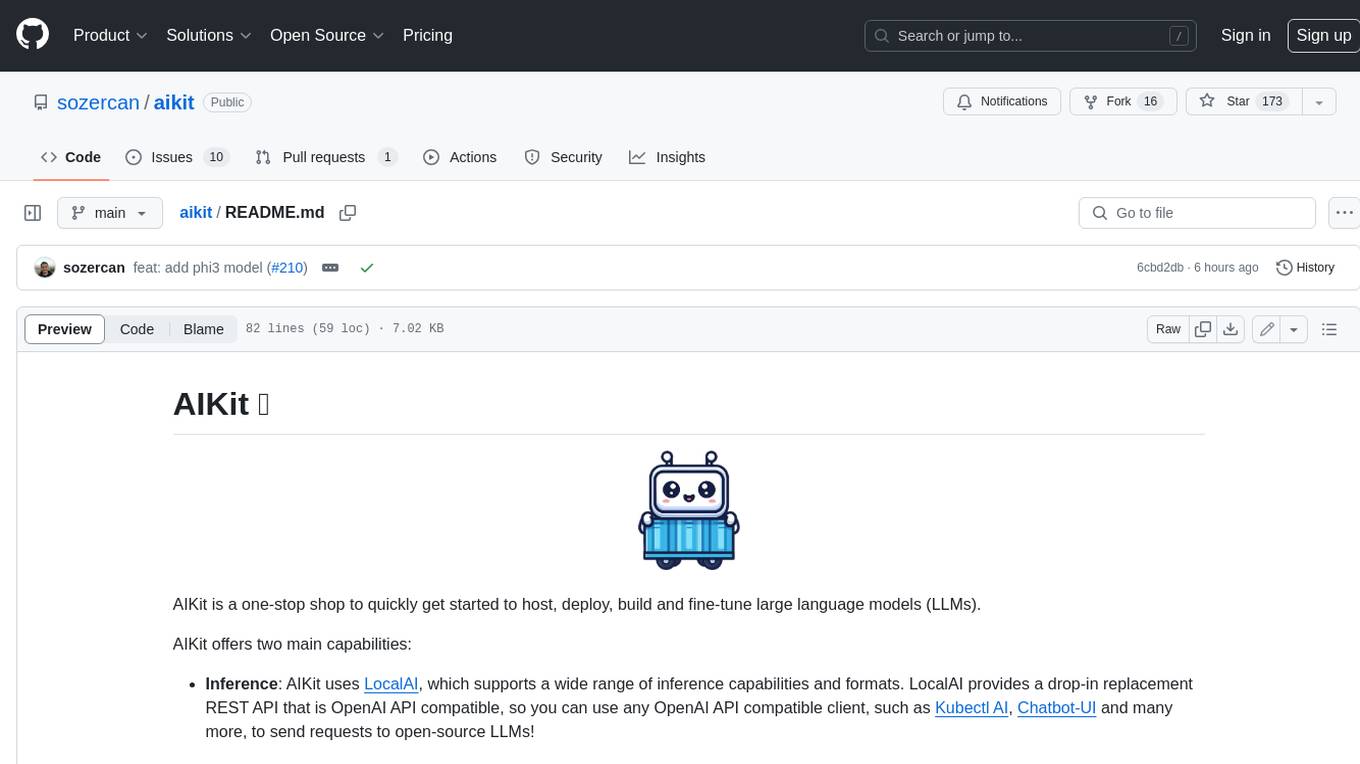
aikit
AIKit is a one-stop shop to quickly get started to host, deploy, build and fine-tune large language models (LLMs). AIKit offers two main capabilities: Inference: AIKit uses LocalAI, which supports a wide range of inference capabilities and formats. LocalAI provides a drop-in replacement REST API that is OpenAI API compatible, so you can use any OpenAI API compatible client, such as Kubectl AI, Chatbot-UI and many more, to send requests to open-source LLMs! Fine Tuning: AIKit offers an extensible fine tuning interface. It supports Unsloth for fast, memory efficient, and easy fine-tuning experience.
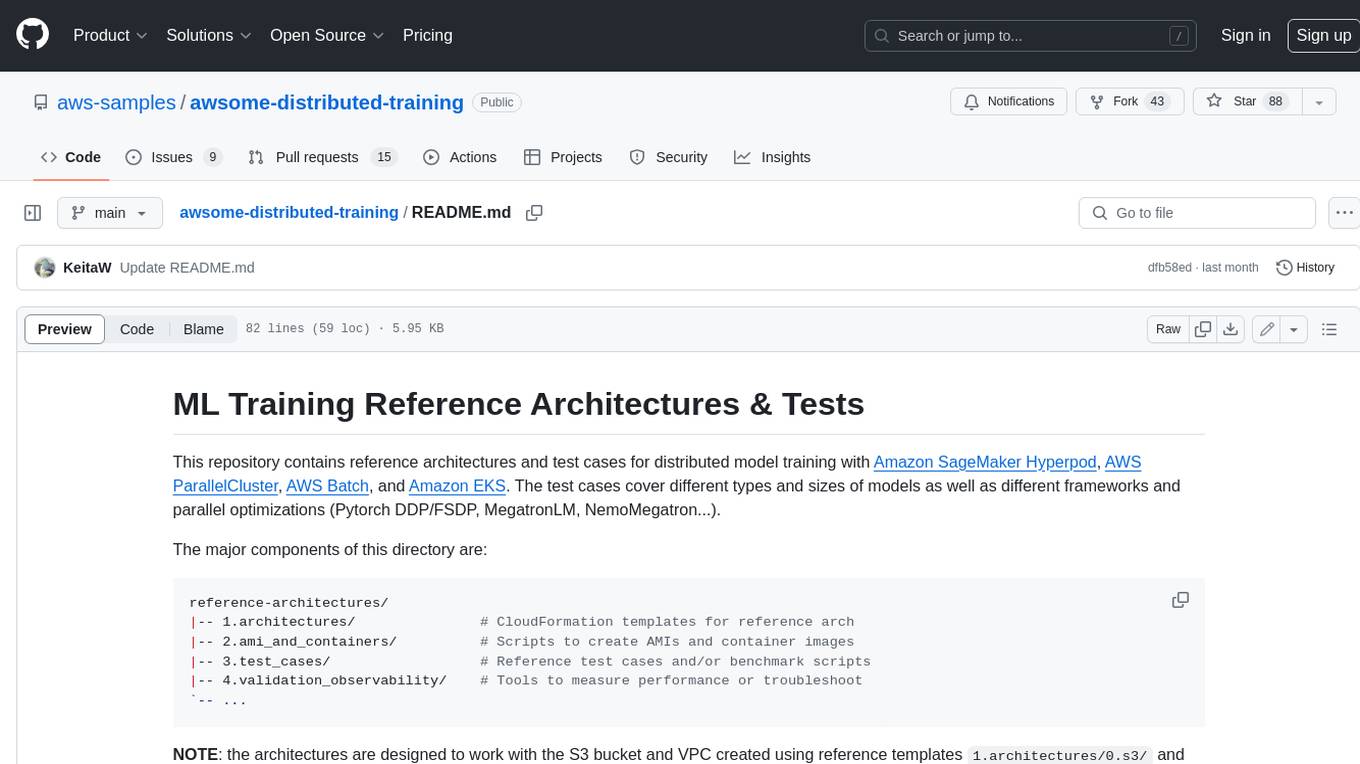
awsome-distributed-training
This repository contains reference architectures and test cases for distributed model training with Amazon SageMaker Hyperpod, AWS ParallelCluster, AWS Batch, and Amazon EKS. The test cases cover different types and sizes of models as well as different frameworks and parallel optimizations (Pytorch DDP/FSDP, MegatronLM, NemoMegatron...).
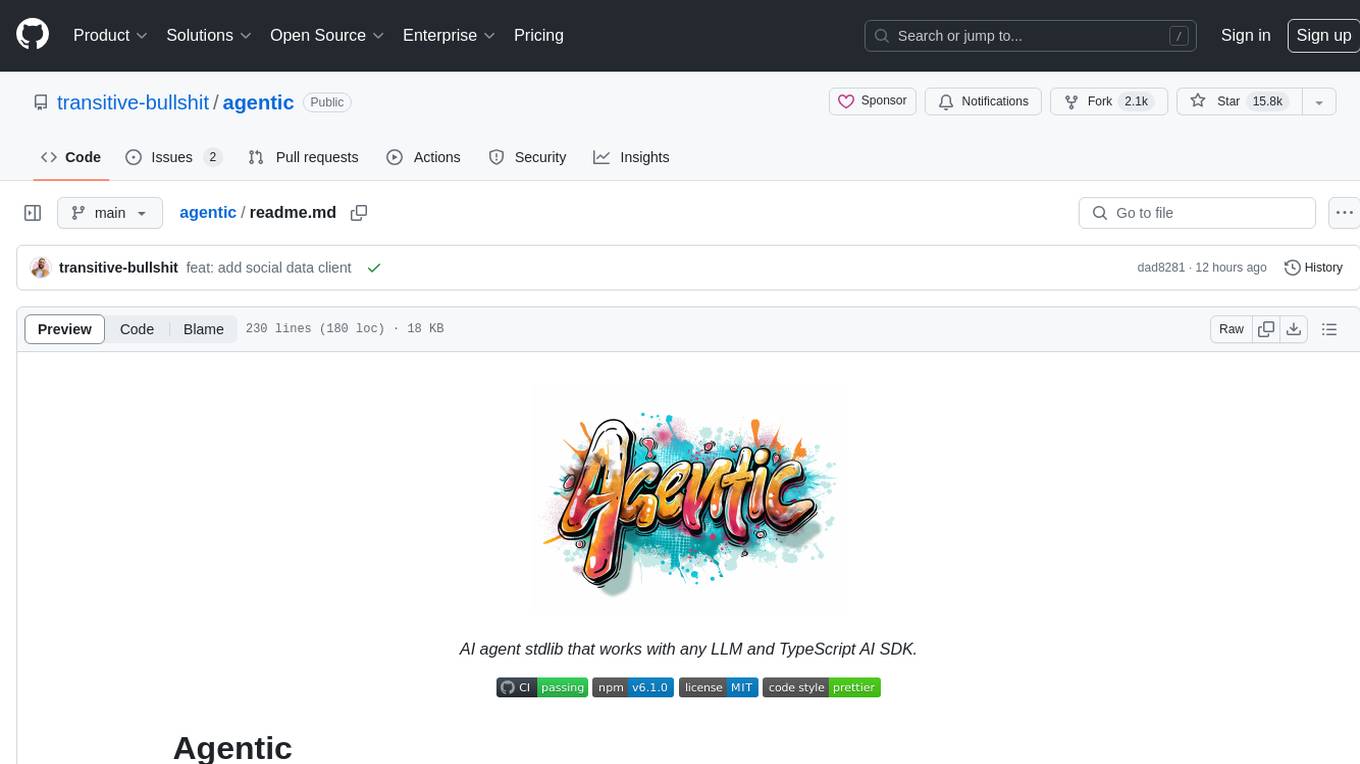
agentic
Agentic is a standard AI functions/tools library optimized for TypeScript and LLM-based apps, compatible with major AI SDKs. It offers a set of thoroughly tested AI functions that can be used with favorite AI SDKs without writing glue code. The library includes various clients for services like Bing web search, calculator, Clearbit data resolution, Dexa podcast questions, and more. It also provides compound tools like SearchAndCrawl and supports multiple AI SDKs such as OpenAI, Vercel AI SDK, LangChain, LlamaIndex, Firebase Genkit, and Dexa Dexter. The goal is to create minimal clients with strongly-typed TypeScript DX, composable AIFunctions via AIFunctionSet, and compatibility with major TS AI SDKs.
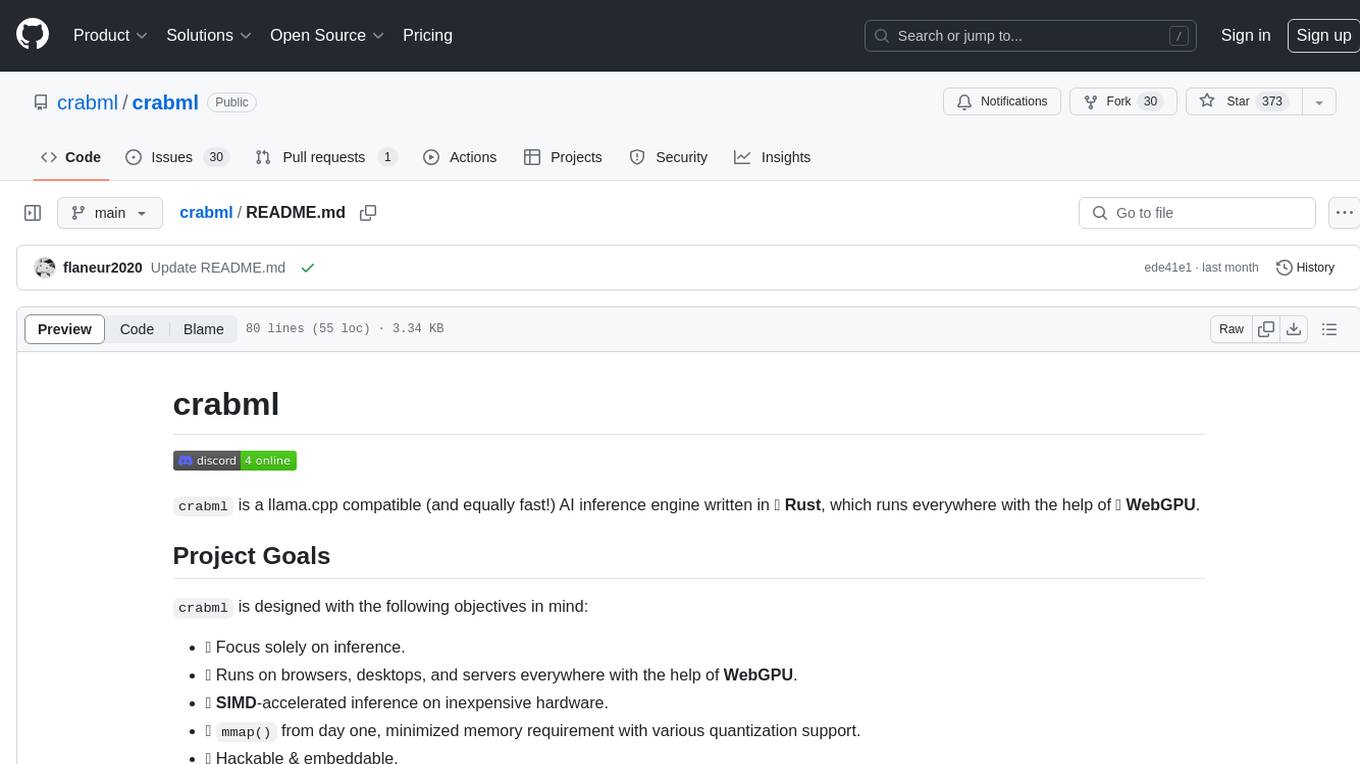
crabml
Crabml is a llama.cpp compatible AI inference engine written in Rust, designed for efficient inference on various platforms with WebGPU support. It focuses on running inference tasks with SIMD acceleration and minimal memory requirements, supporting multiple models and quantization methods. The project is hackable, embeddable, and aims to provide high-performance AI inference capabilities.
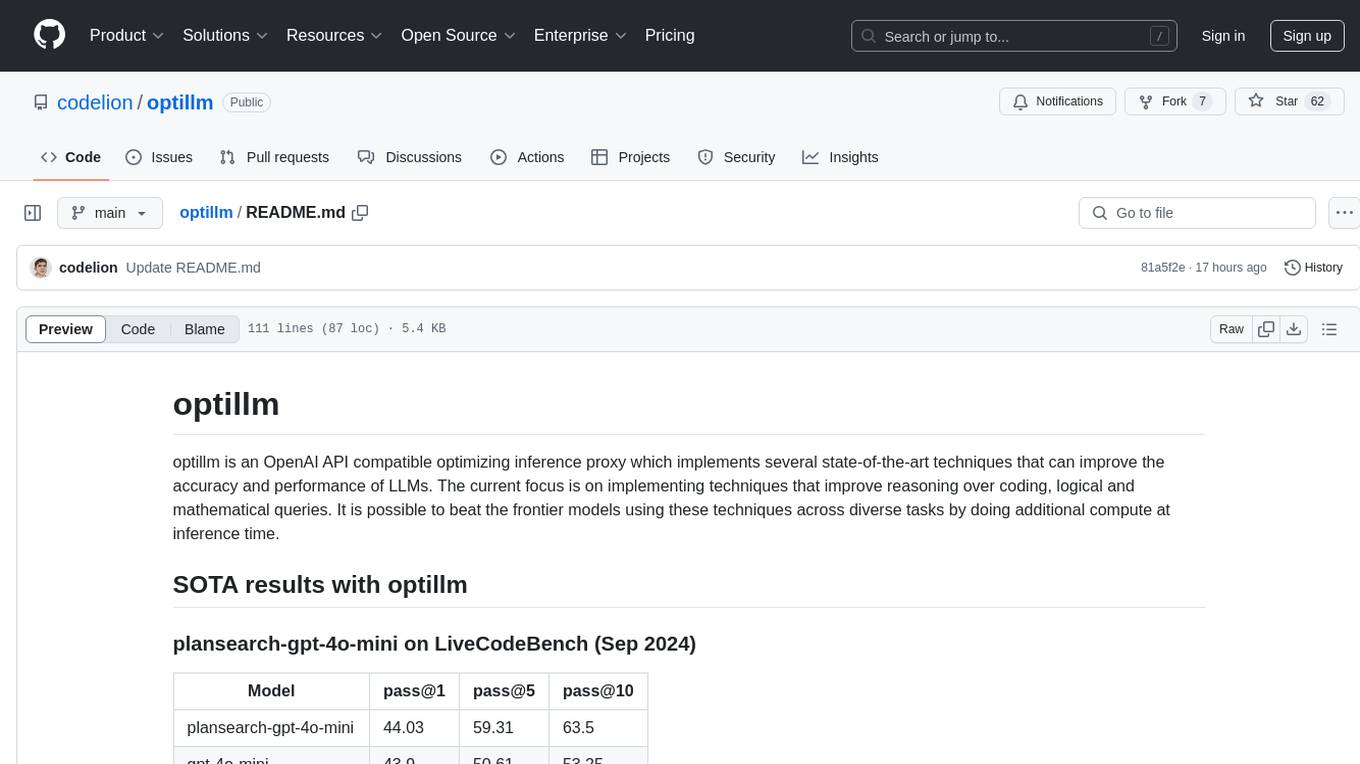
optillm
optillm is an OpenAI API compatible optimizing inference proxy implementing state-of-the-art techniques to enhance accuracy and performance of LLMs, focusing on reasoning over coding, logical, and mathematical queries. By leveraging additional compute at inference time, it surpasses frontier models across diverse tasks.
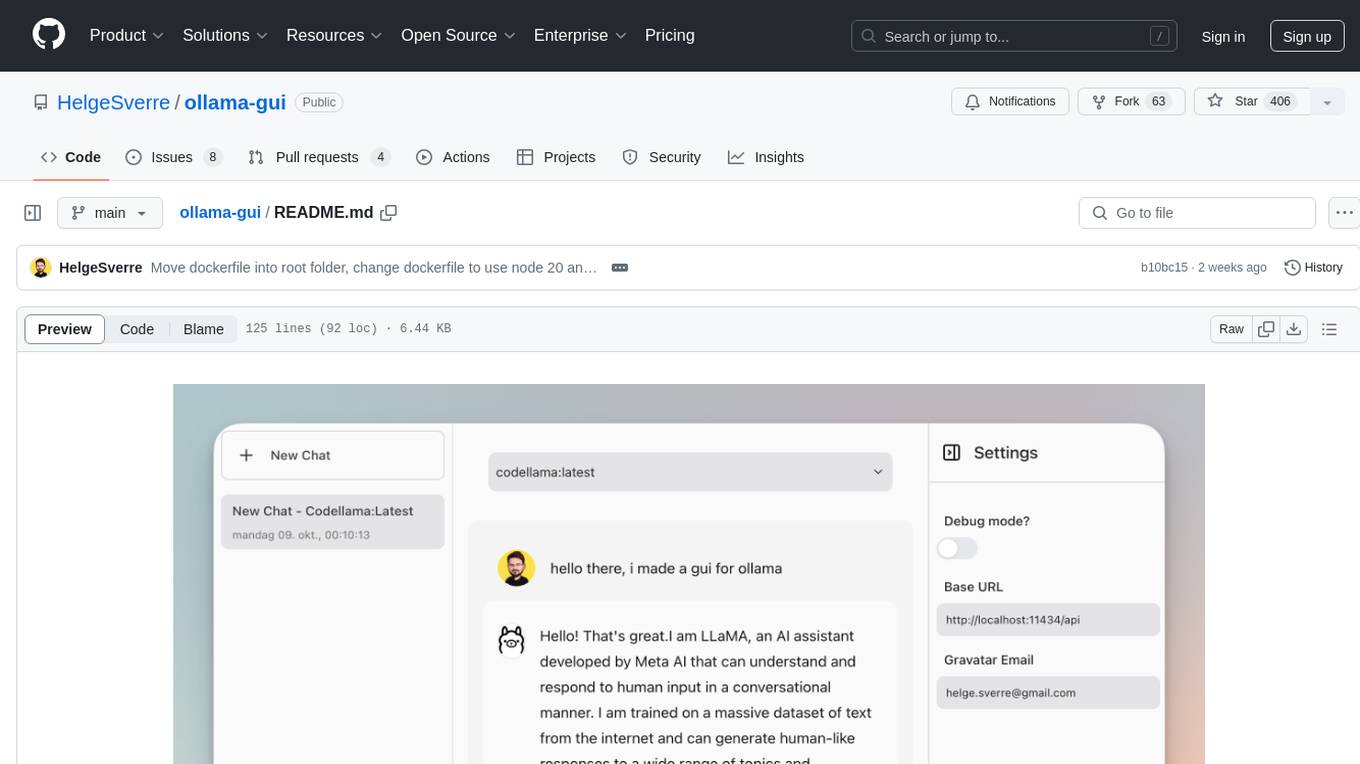
ollama-gui
Ollama GUI is a web interface for ollama.ai, a tool that enables running Large Language Models (LLMs) on your local machine. It provides a user-friendly platform for chatting with LLMs and accessing various models for text generation. Users can easily interact with different models, manage chat history, and explore available models through the web interface. The tool is built with Vue.js, Vite, and Tailwind CSS, offering a modern and responsive design for seamless user experience.
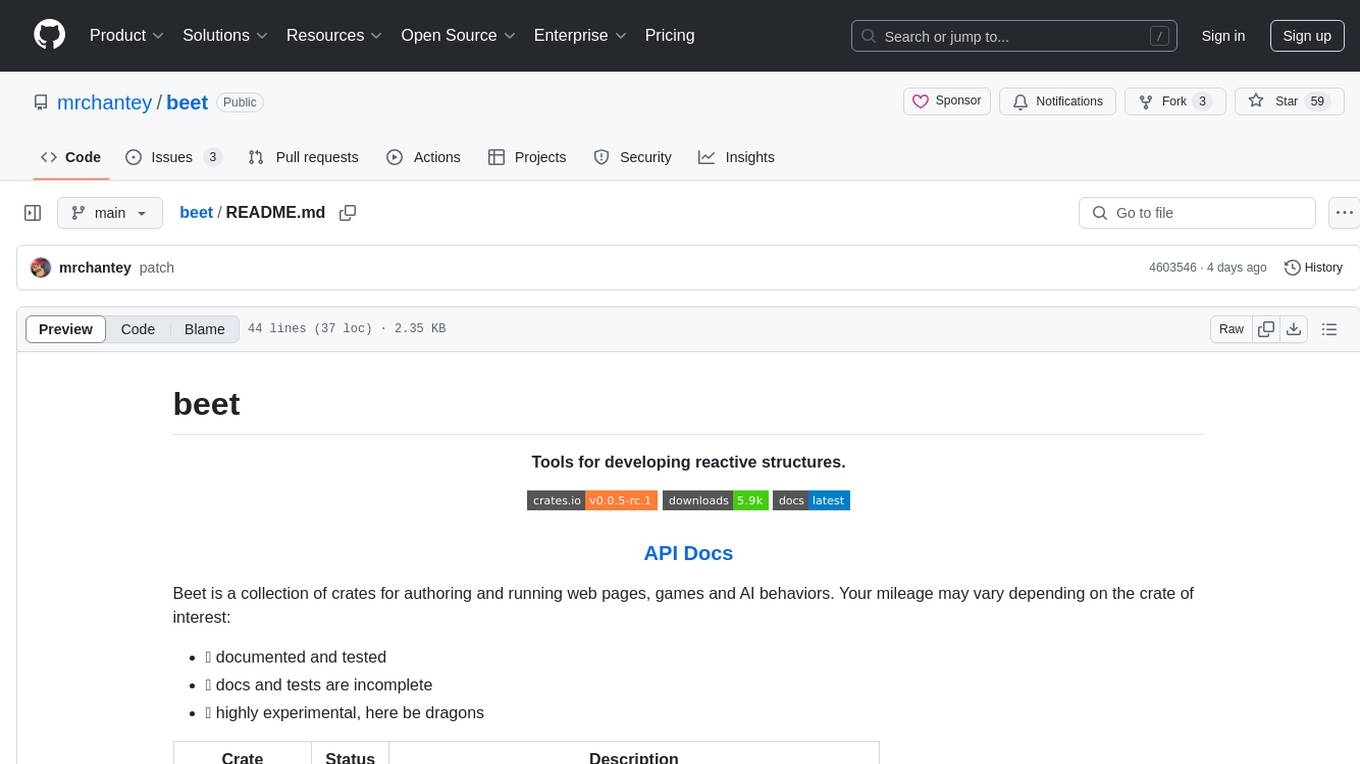
beet
Beet is a collection of crates for authoring and running web pages, games and AI behaviors. It includes crates like `beet_flow` for scenes-as-control-flow bevy library, `beet_spatial` for spatial behaviors, `beet_ml` for machine learning, `beet_sim` for simulation tooling, `beet_rsx` for authoring tools for html and bevy, and `beet_router` for file-based router for web docs. The `beet` crate acts as a base crate that re-exports sub-crates based on feature flags, similar to the `bevy` crate structure.
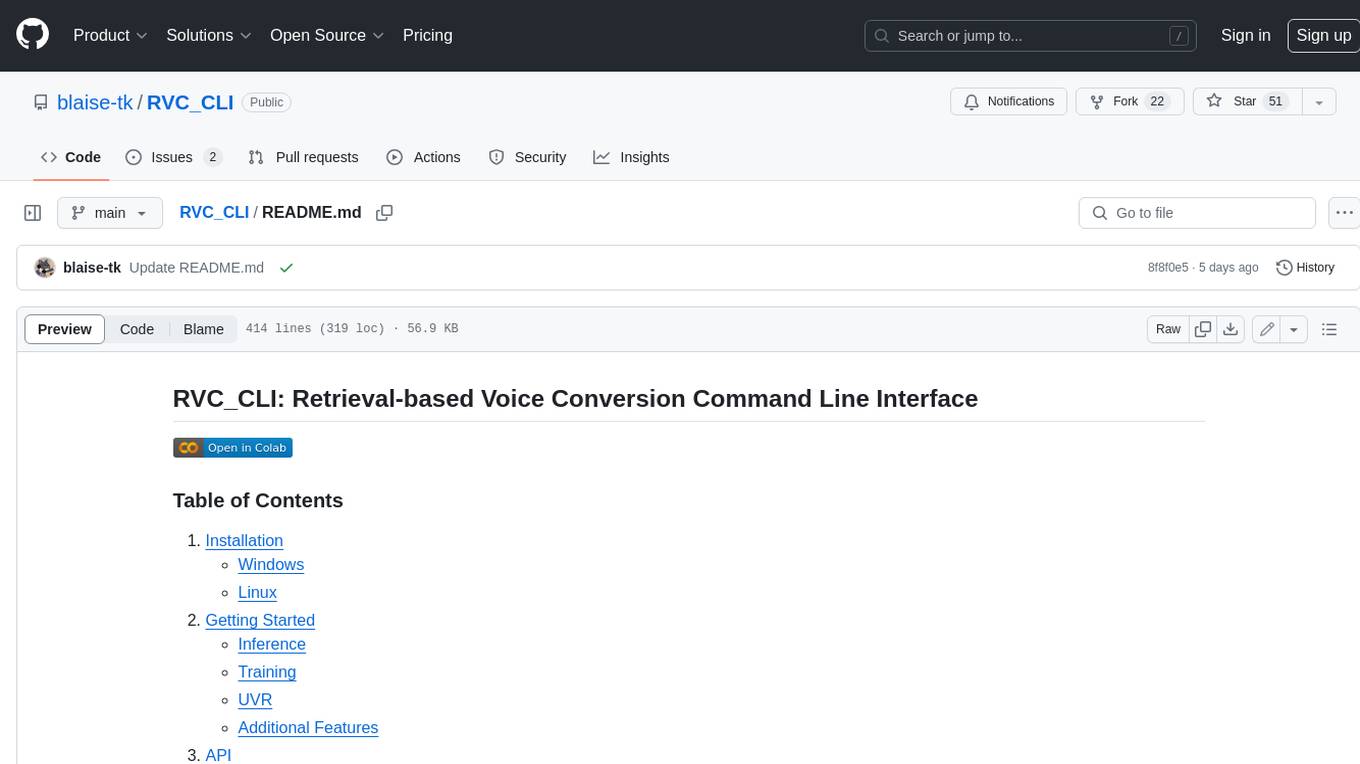
RVC_CLI
**RVC_CLI: Retrieval-based Voice Conversion Command Line Interface** This command-line interface (CLI) provides a comprehensive set of tools for voice conversion, enabling you to modify the pitch, timbre, and other characteristics of audio recordings. It leverages advanced machine learning models to achieve realistic and high-quality voice conversions. **Key Features:** * **Inference:** Convert the pitch and timbre of audio in real-time or process audio files in batch mode. * **TTS Inference:** Synthesize speech from text using a variety of voices and apply voice conversion techniques. * **Training:** Train custom voice conversion models to meet specific requirements. * **Model Management:** Extract, blend, and analyze models to fine-tune and optimize performance. * **Audio Analysis:** Inspect audio files to gain insights into their characteristics. * **API:** Integrate the CLI's functionality into your own applications or workflows. **Applications:** The RVC_CLI finds applications in various domains, including: * **Music Production:** Create unique vocal effects, harmonies, and backing vocals. * **Voiceovers:** Generate voiceovers with different accents, emotions, and styles. * **Audio Editing:** Enhance or modify audio recordings for podcasts, audiobooks, and other content. * **Research and Development:** Explore and advance the field of voice conversion technology. **For Jobs:** * Audio Engineer * Music Producer * Voiceover Artist * Audio Editor * Machine Learning Engineer **AI Keywords:** * Voice Conversion * Pitch Shifting * Timbre Modification * Machine Learning * Audio Processing **For Tasks:** * Convert Pitch * Change Timbre * Synthesize Speech * Train Model * Analyze Audio
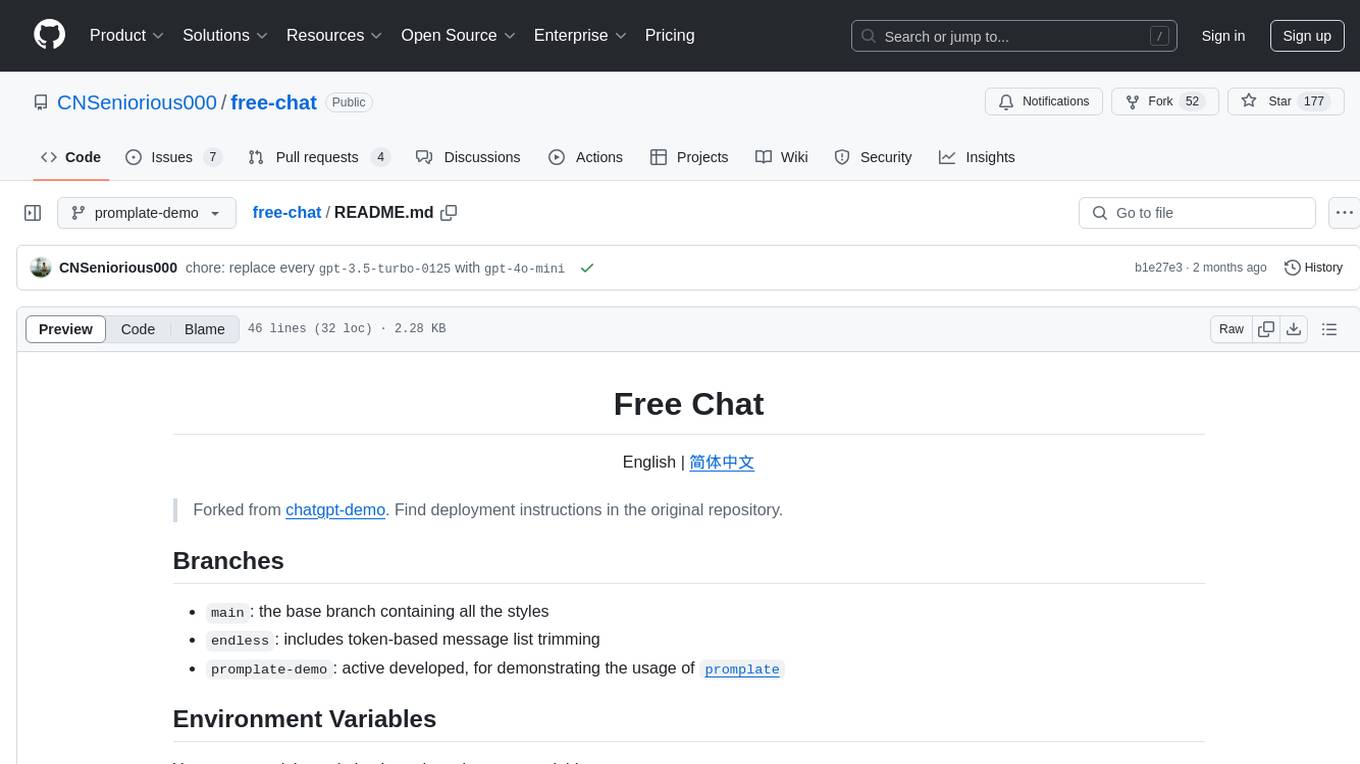
free-chat
Free Chat is a forked project from chatgpt-demo that allows users to deploy a chat application with various features. It provides branches for different functionalities like token-based message list trimming and usage demonstration of 'promplate'. Users can control the website through environment variables, including setting OpenAI API key, temperature parameter, proxy, base URL, and more. The project welcomes contributions and acknowledges supporters. It is licensed under MIT by Muspi Merol.
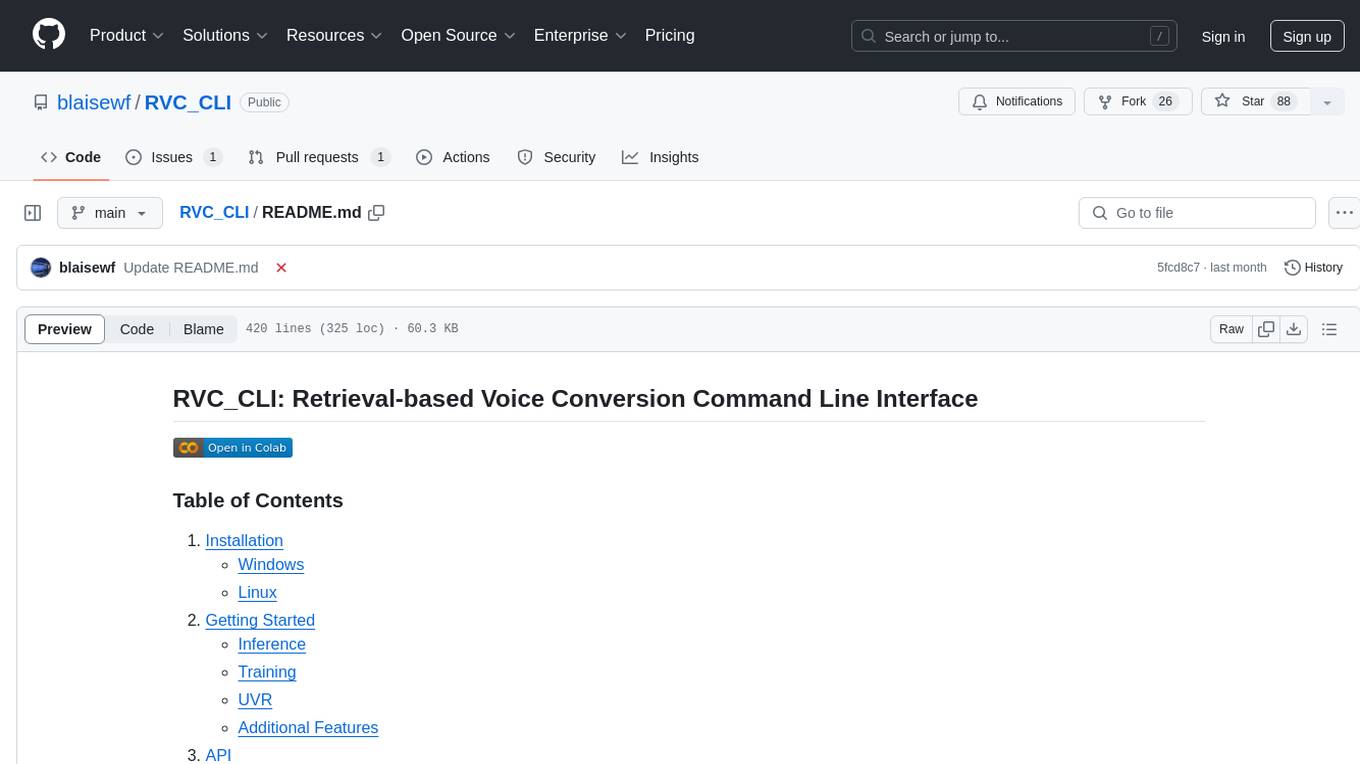
RVC_CLI
RVC_CLI is a command line interface tool for retrieval-based voice conversion. It provides functionalities for installation, getting started, inference, training, UVR, additional features, and API integration. Users can perform tasks like single inference, batch inference, TTS inference, preprocess dataset, extract features, start training, generate index file, model extract, model information, model blender, launch TensorBoard, download models, audio analyzer, and prerequisites download. The tool is built on various projects like ContentVec, HIFIGAN, audio-slicer, python-audio-separator, RMVPE, FCPE, VITS, So-Vits-SVC, Harmonify, and others.
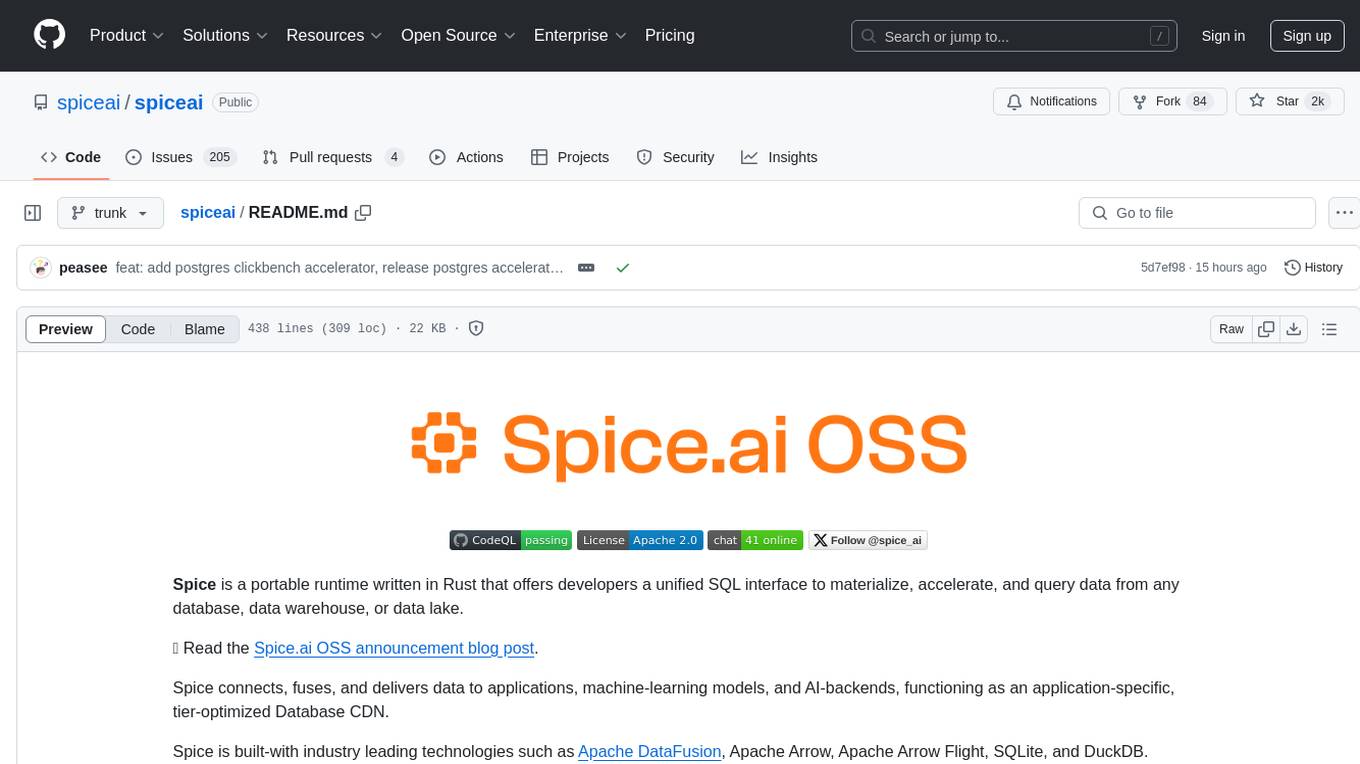
spiceai
Spice is a portable runtime written in Rust that offers developers a unified SQL interface to materialize, accelerate, and query data from any database, data warehouse, or data lake. It connects, fuses, and delivers data to applications, machine-learning models, and AI-backends, functioning as an application-specific, tier-optimized Database CDN. Built with industry-leading technologies such as Apache DataFusion, Apache Arrow, Apache Arrow Flight, SQLite, and DuckDB. Spice makes it fast and easy to query data from one or more sources using SQL, co-locating a managed dataset with applications or machine learning models, and accelerating it with Arrow in-memory, SQLite/DuckDB, or attached PostgreSQL for fast, high-concurrency, low-latency queries.
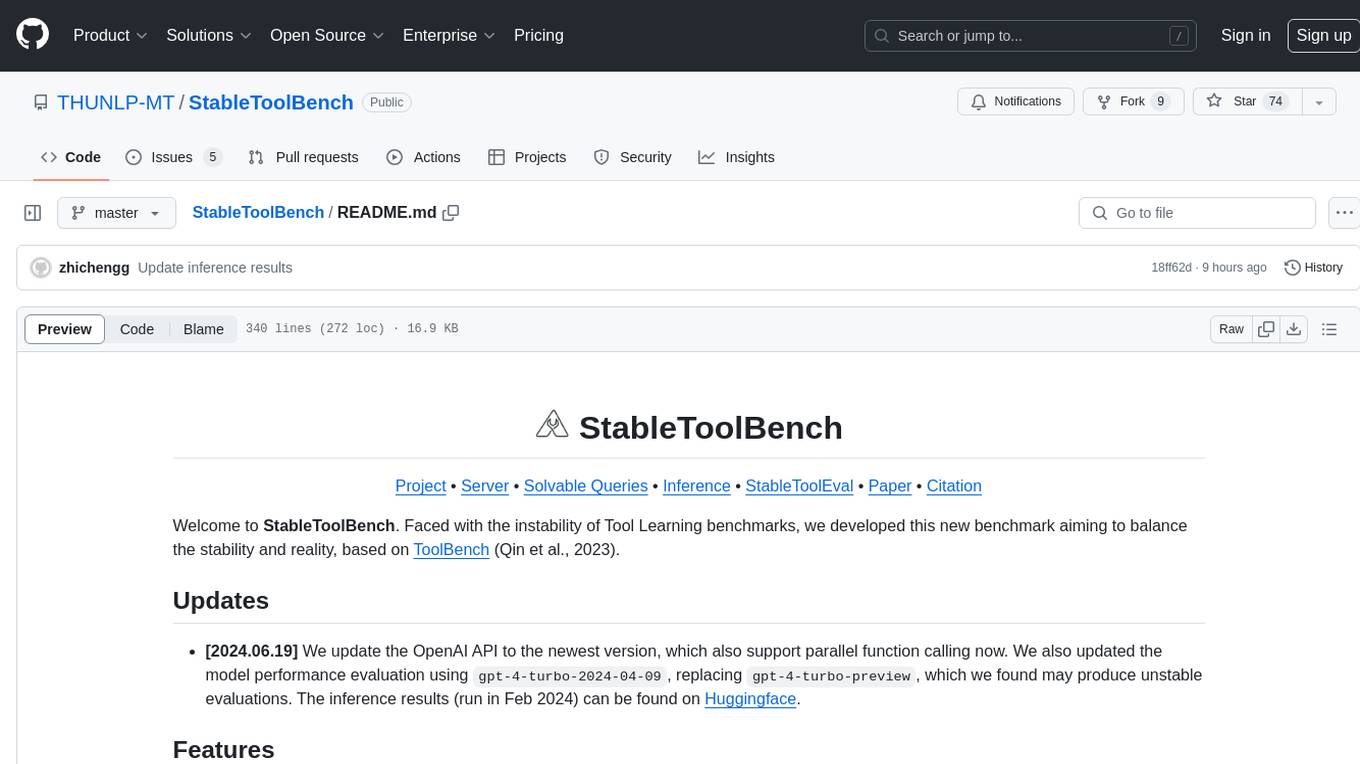
StableToolBench
StableToolBench is a new benchmark developed to address the instability of Tool Learning benchmarks. It aims to balance stability and reality by introducing features like Virtual API System, Solvable Queries, and Stable Evaluation System. The benchmark ensures consistency through a caching system and API simulators, filters queries based on solvability using LLMs, and evaluates model performance using GPT-4 with metrics like Solvable Pass Rate and Solvable Win Rate.
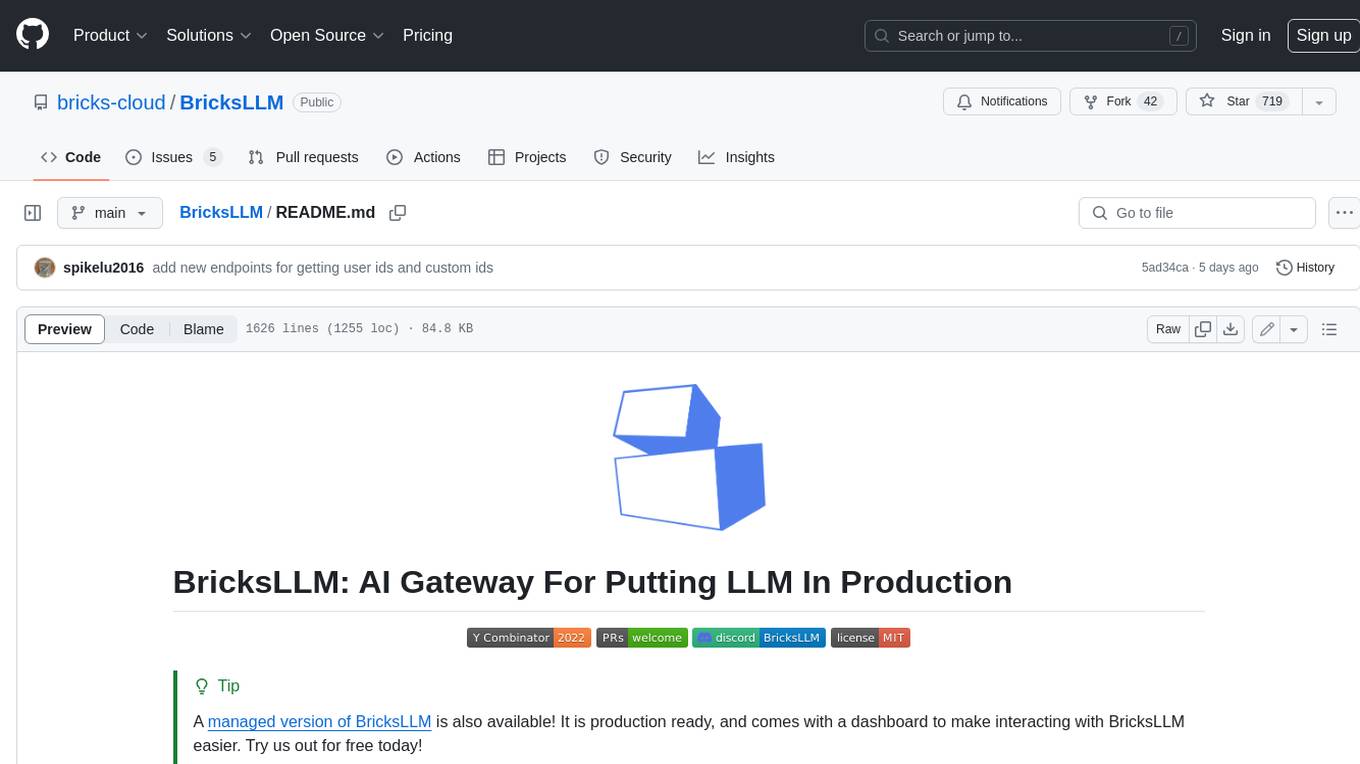
BricksLLM
BricksLLM is a cloud native AI gateway written in Go. Currently, it provides native support for OpenAI, Anthropic, Azure OpenAI and vLLM. BricksLLM aims to provide enterprise level infrastructure that can power any LLM production use cases. Here are some use cases for BricksLLM: * Set LLM usage limits for users on different pricing tiers * Track LLM usage on a per user and per organization basis * Block or redact requests containing PIIs * Improve LLM reliability with failovers, retries and caching * Distribute API keys with rate limits and cost limits for internal development/production use cases * Distribute API keys with rate limits and cost limits for students
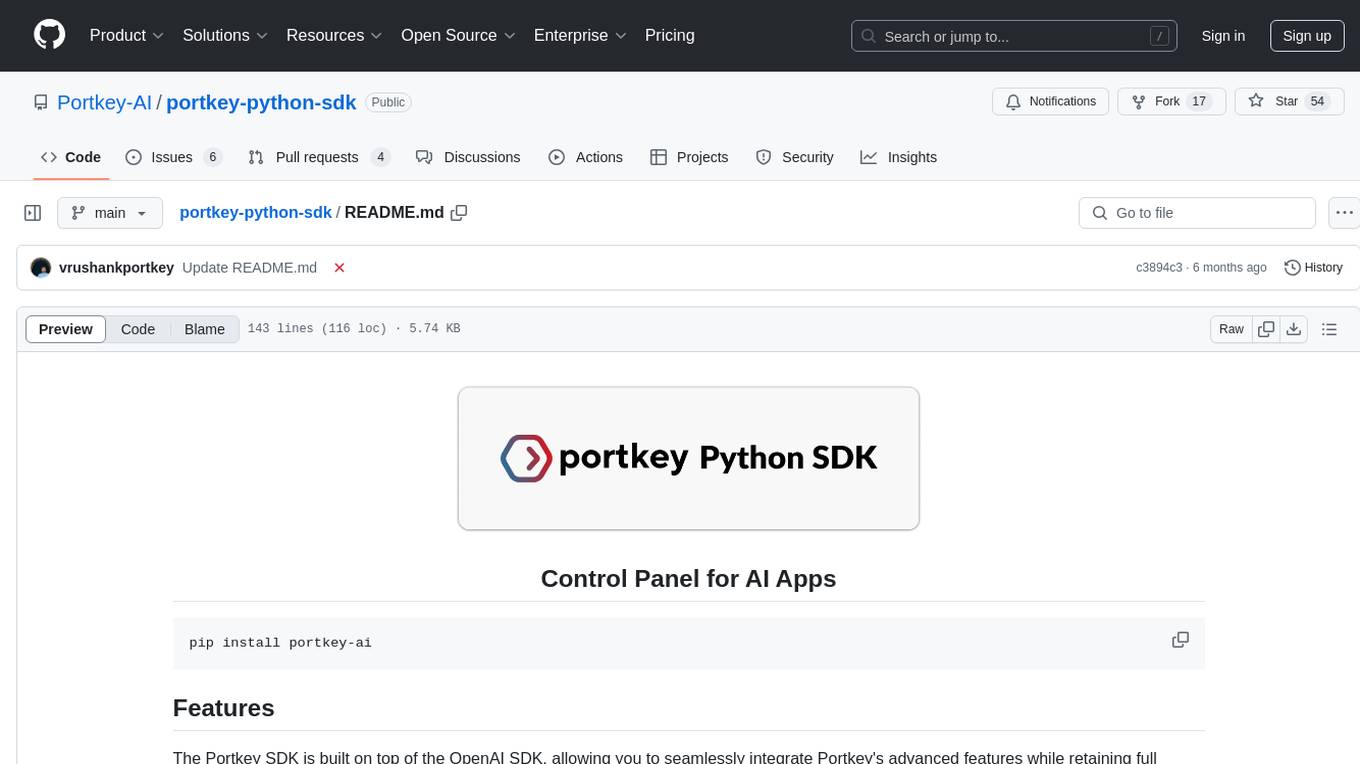
portkey-python-sdk
The Portkey Python SDK is a control panel for AI apps that allows seamless integration of Portkey's advanced features with OpenAI methods. It provides features such as AI gateway for unified API signature, interoperability, automated fallbacks & retries, load balancing, semantic caching, virtual keys, request timeouts, observability with logging, requests tracing, custom metadata, feedback collection, and analytics. Users can make requests to OpenAI using Portkey SDK and also use async functionality. The SDK is compatible with OpenAI SDK methods and offers Portkey-specific methods like feedback and prompts. It supports various providers and encourages contributions through Github issues or direct contact via email or Discord.
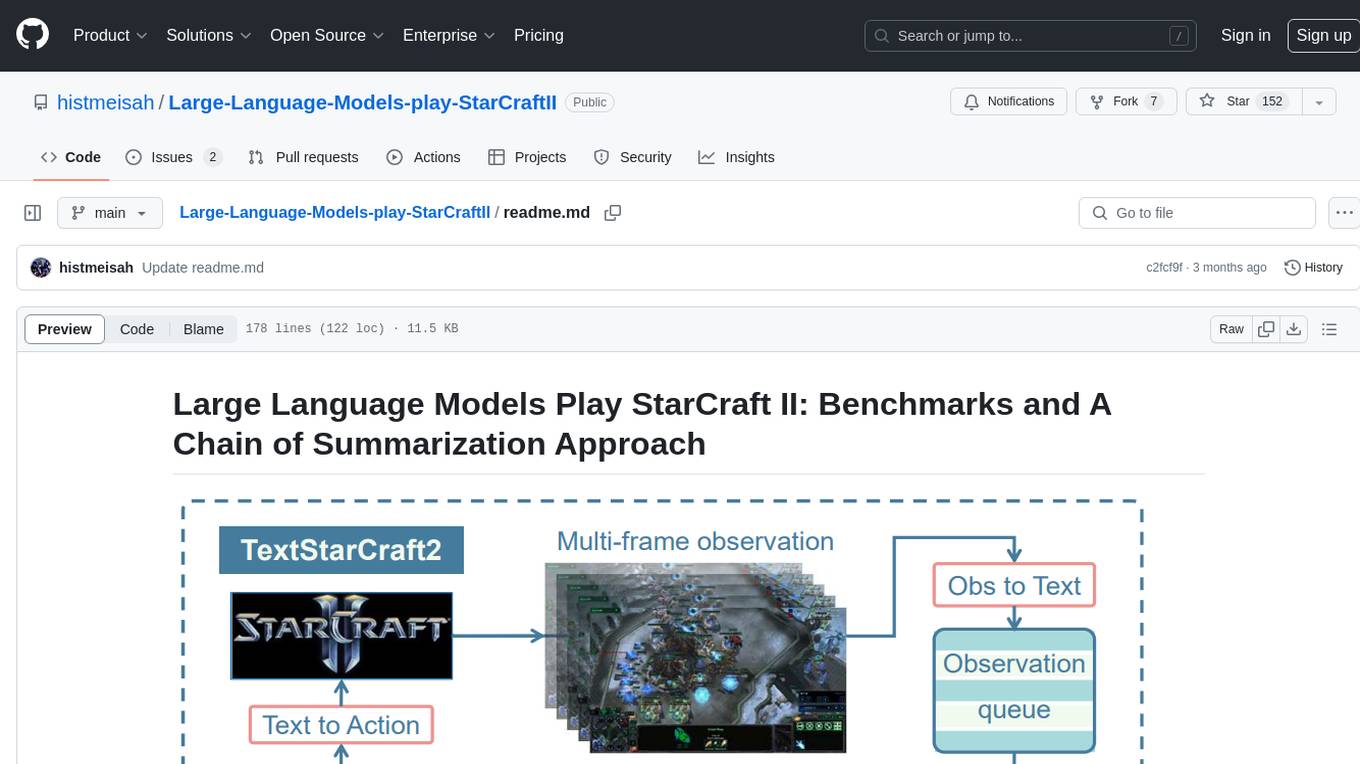
Large-Language-Models-play-StarCraftII
Large Language Models Play StarCraft II is a project that explores the capabilities of large language models (LLMs) in playing the game StarCraft II. The project introduces TextStarCraft II, a textual environment for the game, and a Chain of Summarization method for analyzing game information and making strategic decisions. Through experiments, the project demonstrates that LLM agents can defeat the built-in AI at a challenging difficulty level. The project provides benchmarks and a summarization approach to enhance strategic planning and interpretability in StarCraft II gameplay.
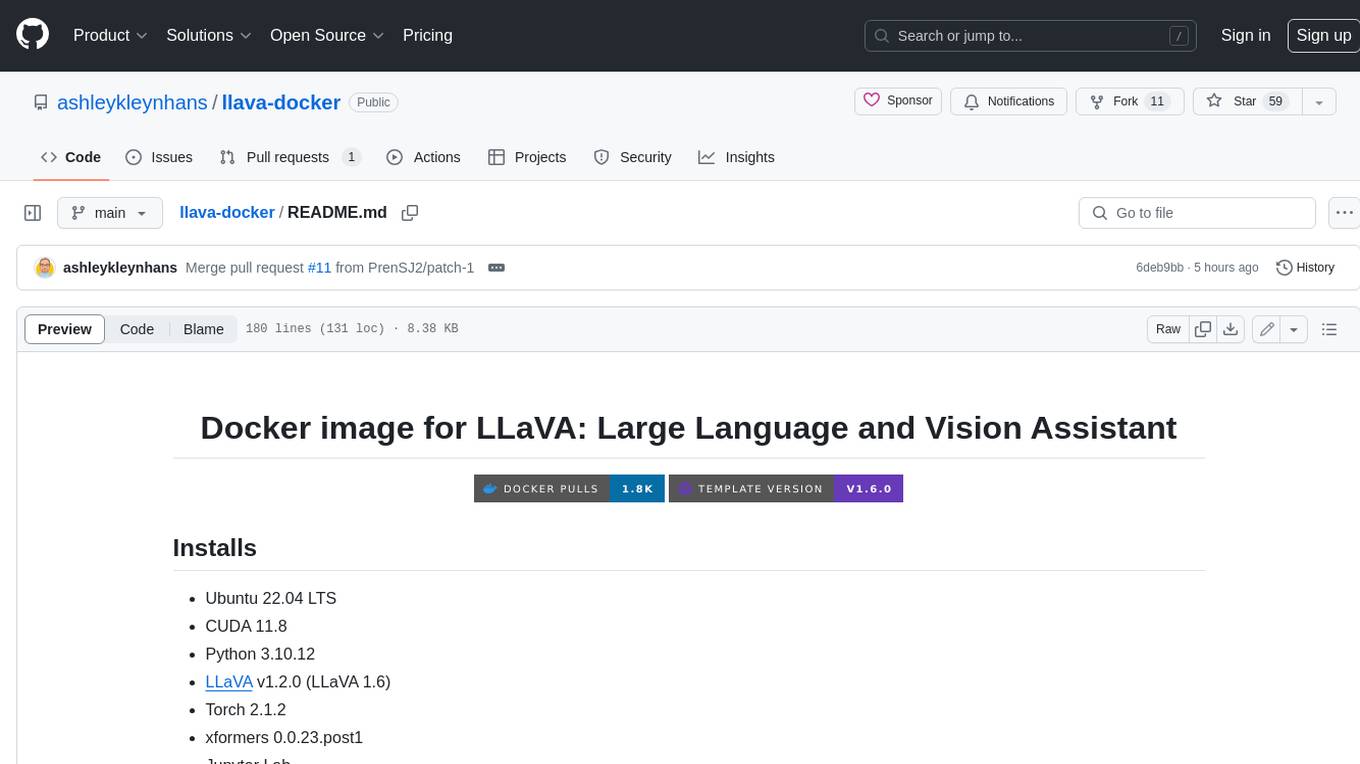
llava-docker
This Docker image for LLaVA (Large Language and Vision Assistant) provides a convenient way to run LLaVA locally or on RunPod. LLaVA is a powerful AI tool that combines natural language processing and computer vision capabilities. With this Docker image, you can easily access LLaVA's functionalities for various tasks, including image captioning, visual question answering, text summarization, and more. The image comes pre-installed with LLaVA v1.2.0, Torch 2.1.2, xformers 0.0.23.post1, and other necessary dependencies. You can customize the model used by setting the MODEL environment variable. The image also includes a Jupyter Lab environment for interactive development and exploration. Overall, this Docker image offers a comprehensive and user-friendly platform for leveraging LLaVA's capabilities.
For similar tasks
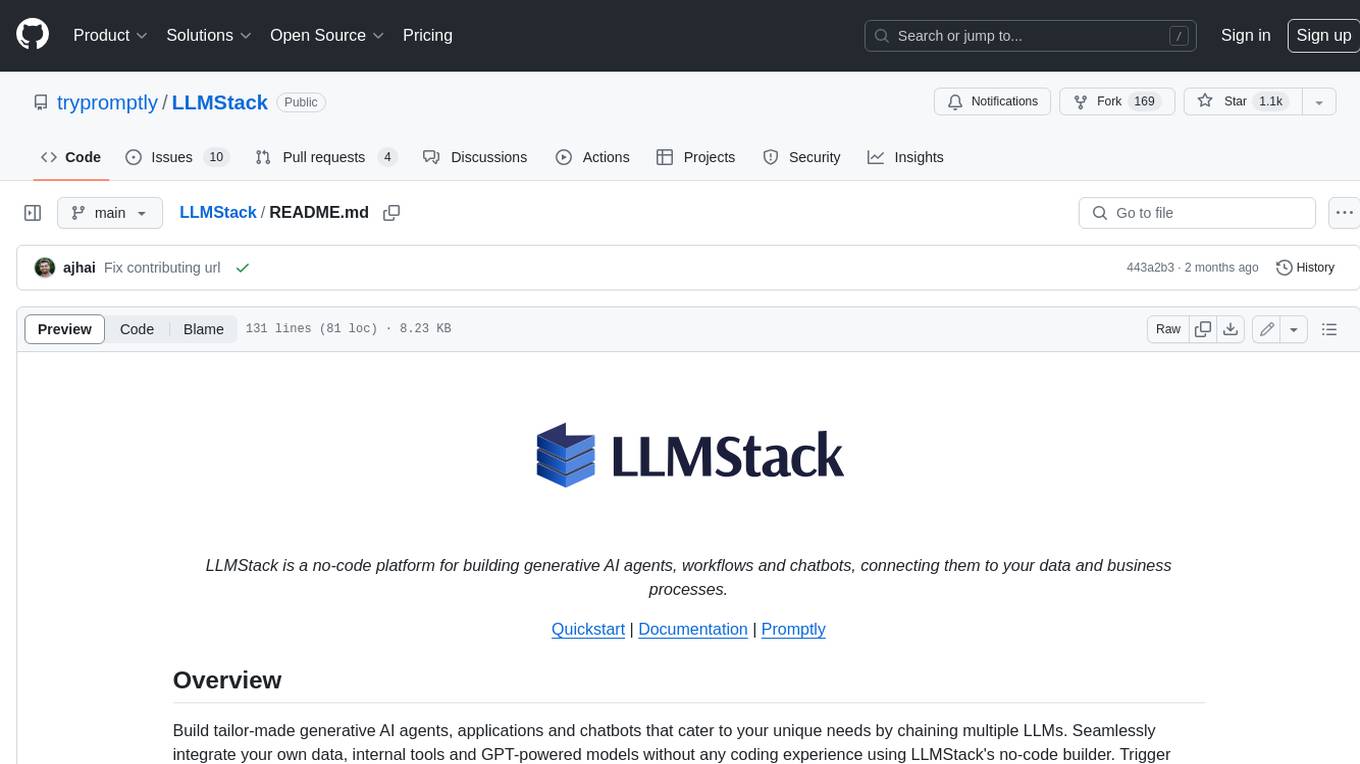
LLMStack
LLMStack is a no-code platform for building generative AI agents, workflows, and chatbots. It allows users to connect their own data, internal tools, and GPT-powered models without any coding experience. LLMStack can be deployed to the cloud or on-premise and can be accessed via HTTP API or triggered from Slack or Discord.
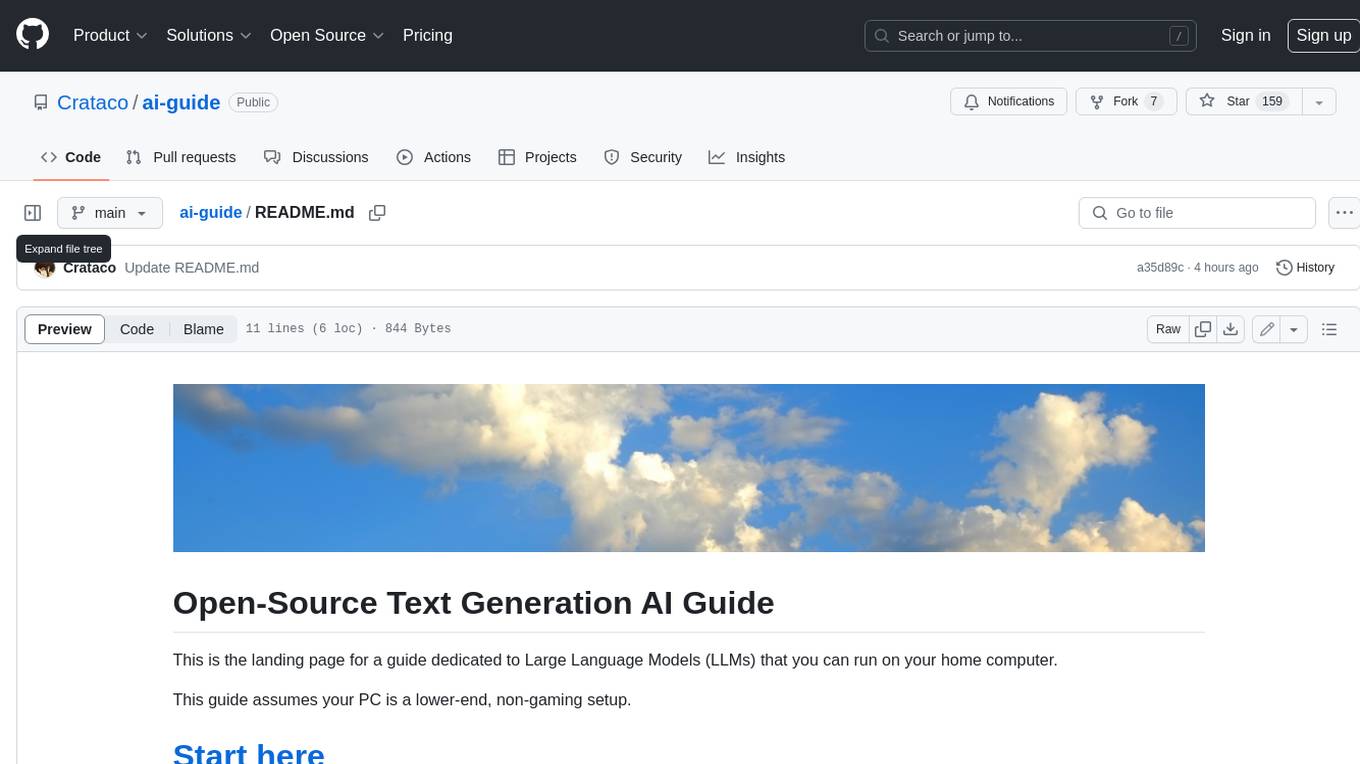
ai-guide
This guide is dedicated to Large Language Models (LLMs) that you can run on your home computer. It assumes your PC is a lower-end, non-gaming setup.
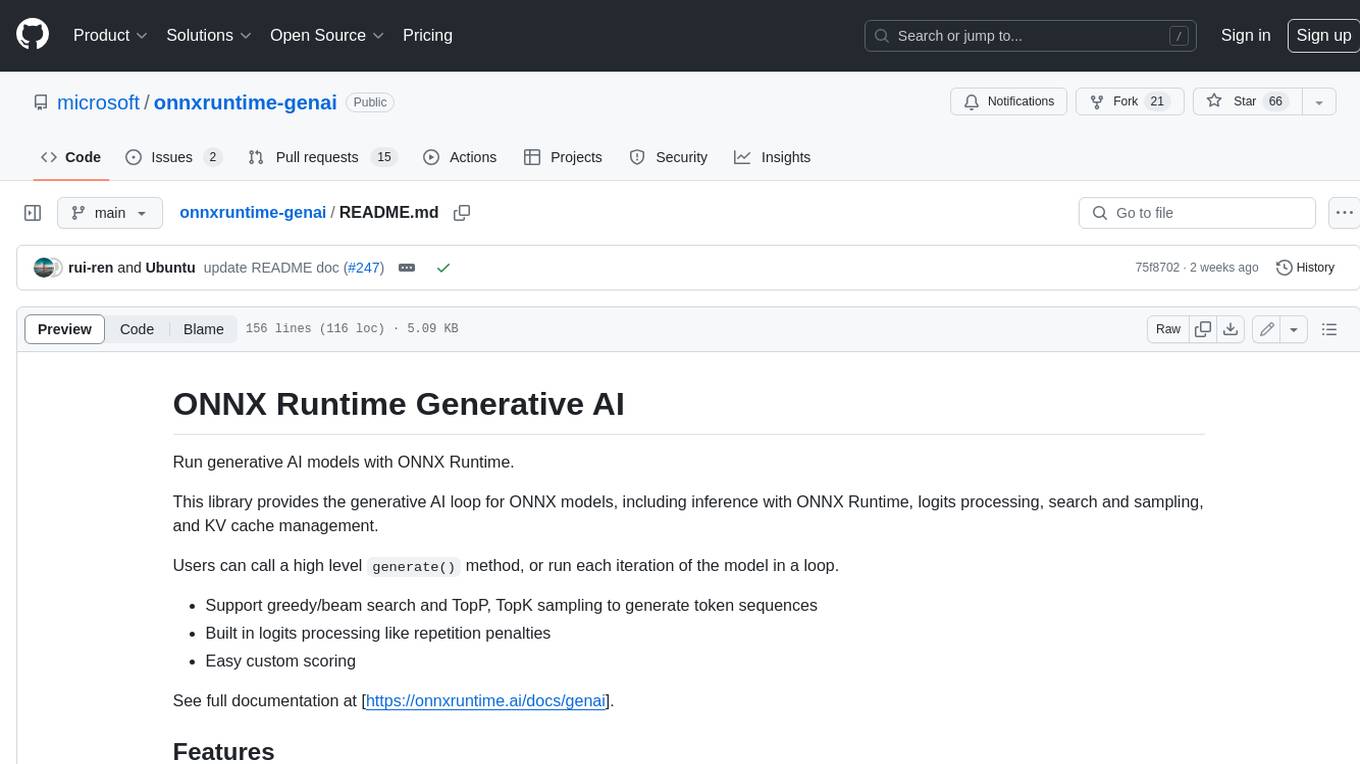
onnxruntime-genai
ONNX Runtime Generative AI is a library that provides the generative AI loop for ONNX models, including inference with ONNX Runtime, logits processing, search and sampling, and KV cache management. Users can call a high level `generate()` method, or run each iteration of the model in a loop. It supports greedy/beam search and TopP, TopK sampling to generate token sequences, has built in logits processing like repetition penalties, and allows for easy custom scoring.
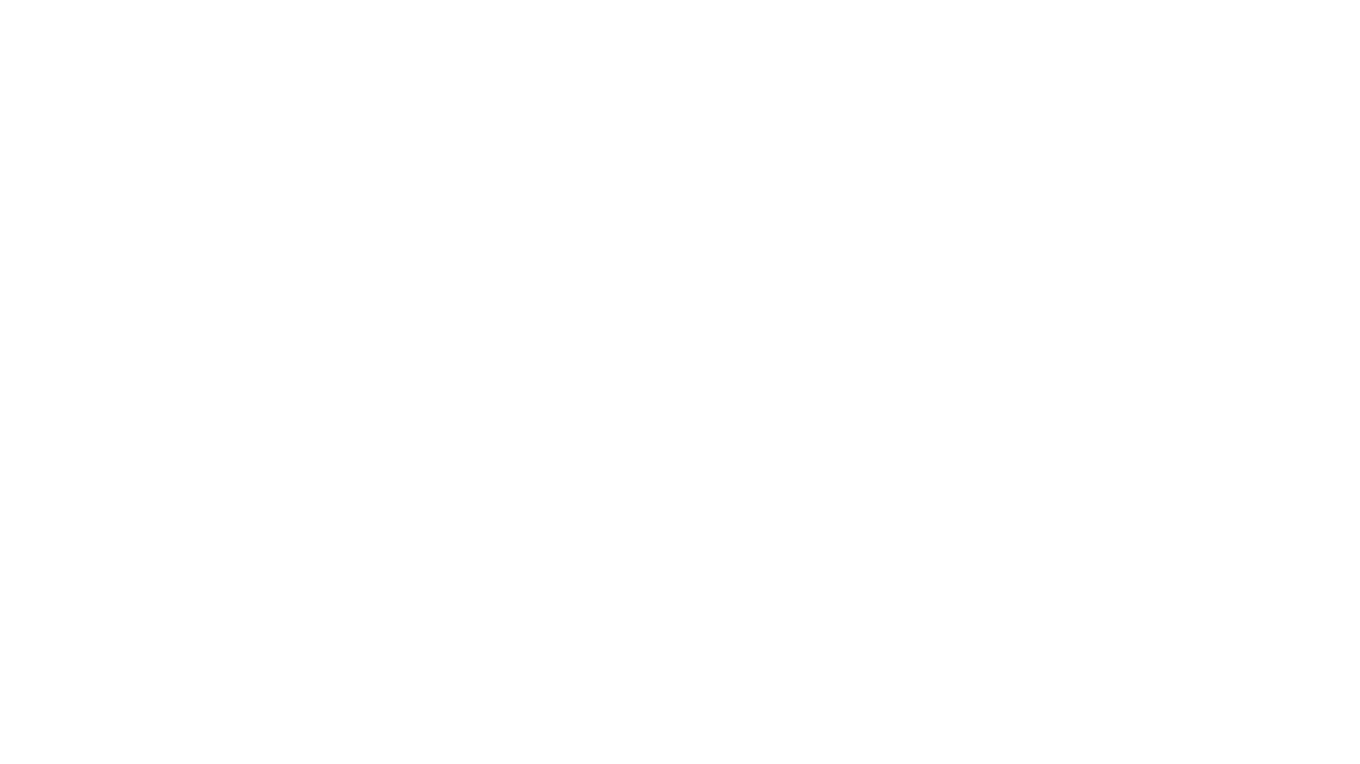
jupyter-ai
Jupyter AI connects generative AI with Jupyter notebooks. It provides a user-friendly and powerful way to explore generative AI models in notebooks and improve your productivity in JupyterLab and the Jupyter Notebook. Specifically, Jupyter AI offers: * An `%%ai` magic that turns the Jupyter notebook into a reproducible generative AI playground. This works anywhere the IPython kernel runs (JupyterLab, Jupyter Notebook, Google Colab, Kaggle, VSCode, etc.). * A native chat UI in JupyterLab that enables you to work with generative AI as a conversational assistant. * Support for a wide range of generative model providers, including AI21, Anthropic, AWS, Cohere, Gemini, Hugging Face, NVIDIA, and OpenAI. * Local model support through GPT4All, enabling use of generative AI models on consumer grade machines with ease and privacy.
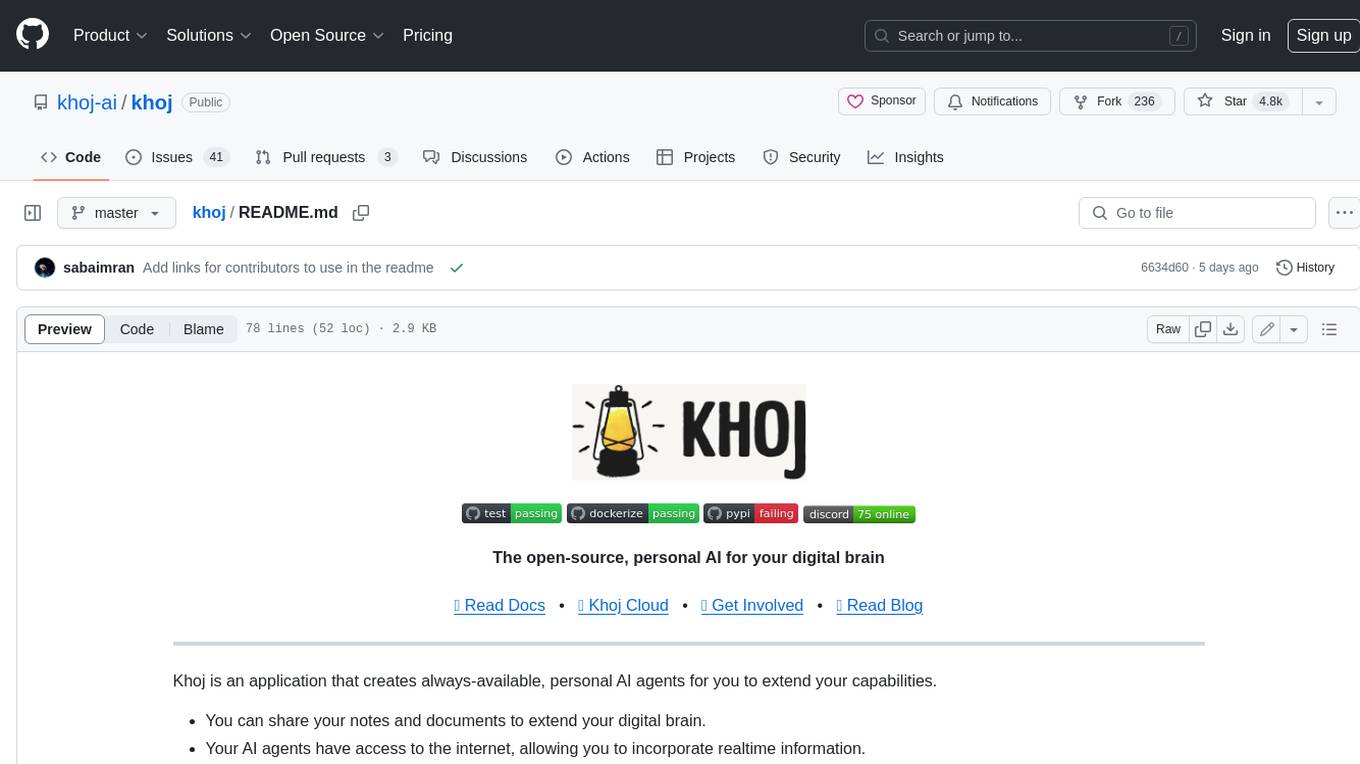
khoj
Khoj is an open-source, personal AI assistant that extends your capabilities by creating always-available AI agents. You can share your notes and documents to extend your digital brain, and your AI agents have access to the internet, allowing you to incorporate real-time information. Khoj is accessible on Desktop, Emacs, Obsidian, Web, and Whatsapp, and you can share PDF, markdown, org-mode, notion files, and GitHub repositories. You'll get fast, accurate semantic search on top of your docs, and your agents can create deeply personal images and understand your speech. Khoj is self-hostable and always will be.
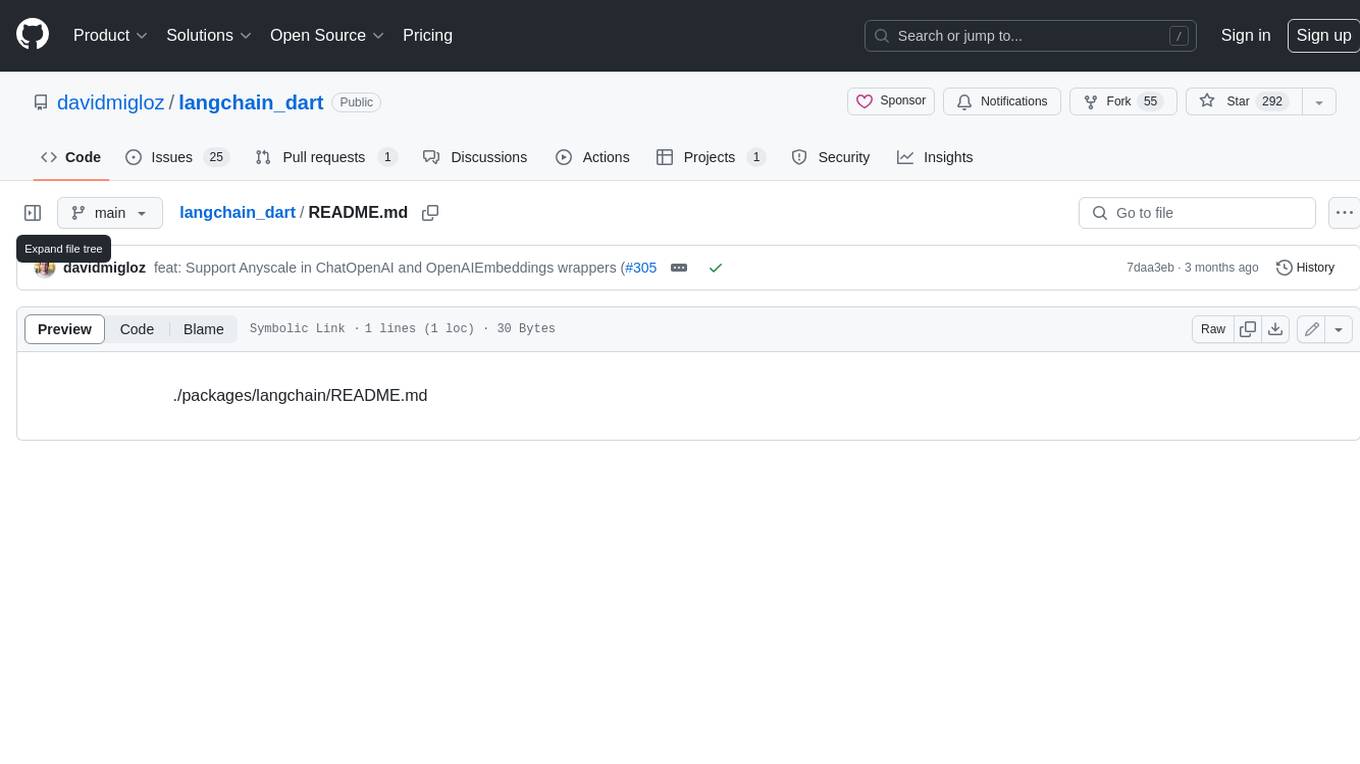
langchain_dart
LangChain.dart is a Dart port of the popular LangChain Python framework created by Harrison Chase. LangChain provides a set of ready-to-use components for working with language models and a standard interface for chaining them together to formulate more advanced use cases (e.g. chatbots, Q&A with RAG, agents, summarization, extraction, etc.). The components can be grouped into a few core modules: * **Model I/O:** LangChain offers a unified API for interacting with various LLM providers (e.g. OpenAI, Google, Mistral, Ollama, etc.), allowing developers to switch between them with ease. Additionally, it provides tools for managing model inputs (prompt templates and example selectors) and parsing the resulting model outputs (output parsers). * **Retrieval:** assists in loading user data (via document loaders), transforming it (with text splitters), extracting its meaning (using embedding models), storing (in vector stores) and retrieving it (through retrievers) so that it can be used to ground the model's responses (i.e. Retrieval-Augmented Generation or RAG). * **Agents:** "bots" that leverage LLMs to make informed decisions about which available tools (such as web search, calculators, database lookup, etc.) to use to accomplish the designated task. The different components can be composed together using the LangChain Expression Language (LCEL).
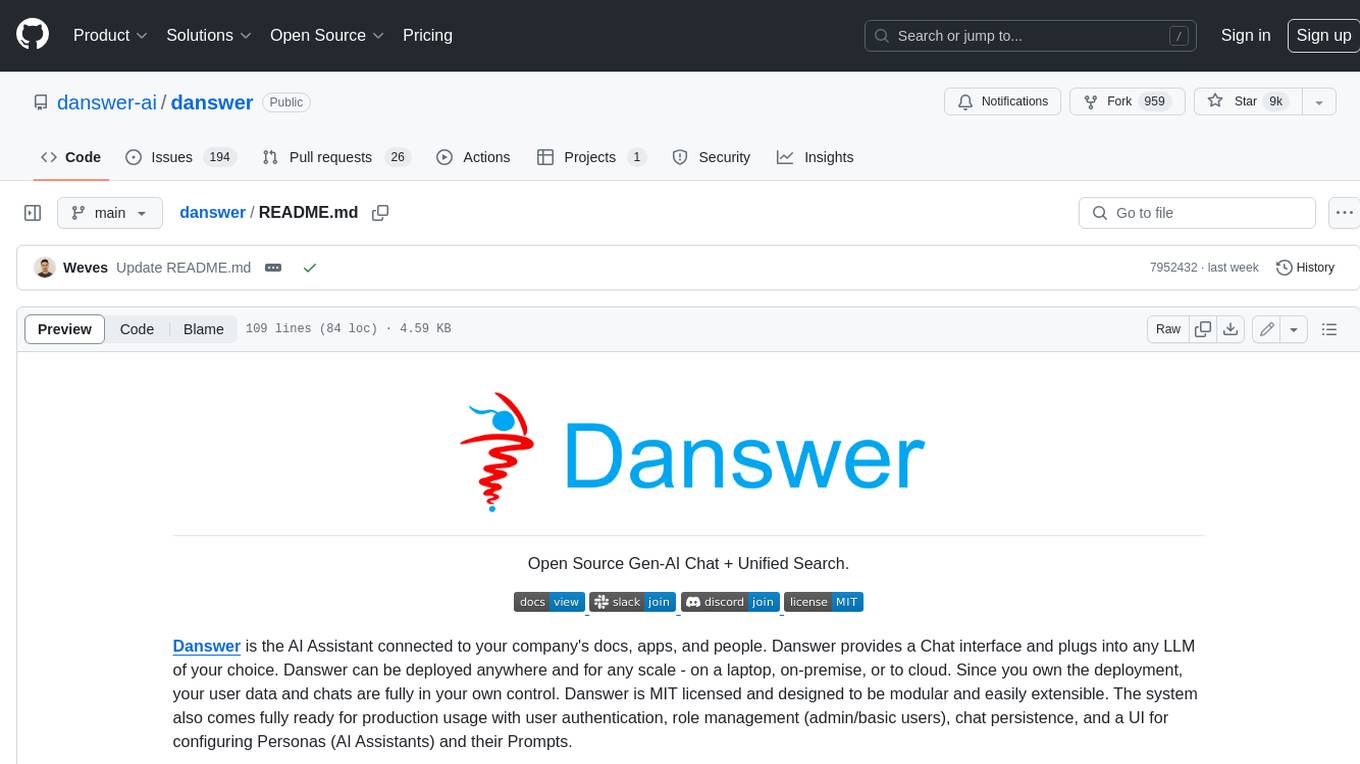
danswer
Danswer is an open-source Gen-AI Chat and Unified Search tool that connects to your company's docs, apps, and people. It provides a Chat interface and plugs into any LLM of your choice. Danswer can be deployed anywhere and for any scale - on a laptop, on-premise, or to cloud. Since you own the deployment, your user data and chats are fully in your own control. Danswer is MIT licensed and designed to be modular and easily extensible. The system also comes fully ready for production usage with user authentication, role management (admin/basic users), chat persistence, and a UI for configuring Personas (AI Assistants) and their Prompts. Danswer also serves as a Unified Search across all common workplace tools such as Slack, Google Drive, Confluence, etc. By combining LLMs and team specific knowledge, Danswer becomes a subject matter expert for the team. Imagine ChatGPT if it had access to your team's unique knowledge! It enables questions such as "A customer wants feature X, is this already supported?" or "Where's the pull request for feature Y?"
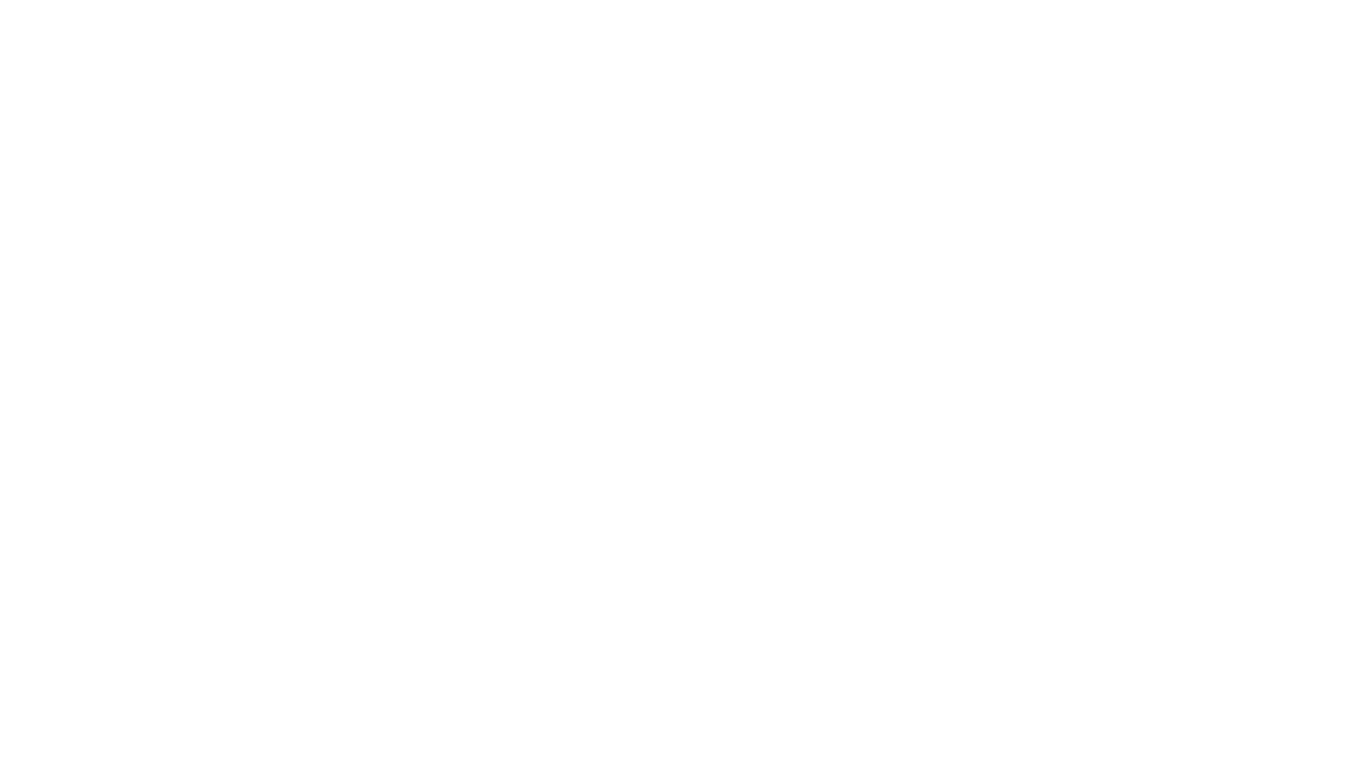
infinity
Infinity is an AI-native database designed for LLM applications, providing incredibly fast full-text and vector search capabilities. It supports a wide range of data types, including vectors, full-text, and structured data, and offers a fused search feature that combines multiple embeddings and full text. Infinity is easy to use, with an intuitive Python API and a single-binary architecture that simplifies deployment. It achieves high performance, with 0.1 milliseconds query latency on million-scale vector datasets and up to 15K QPS.
For similar jobs
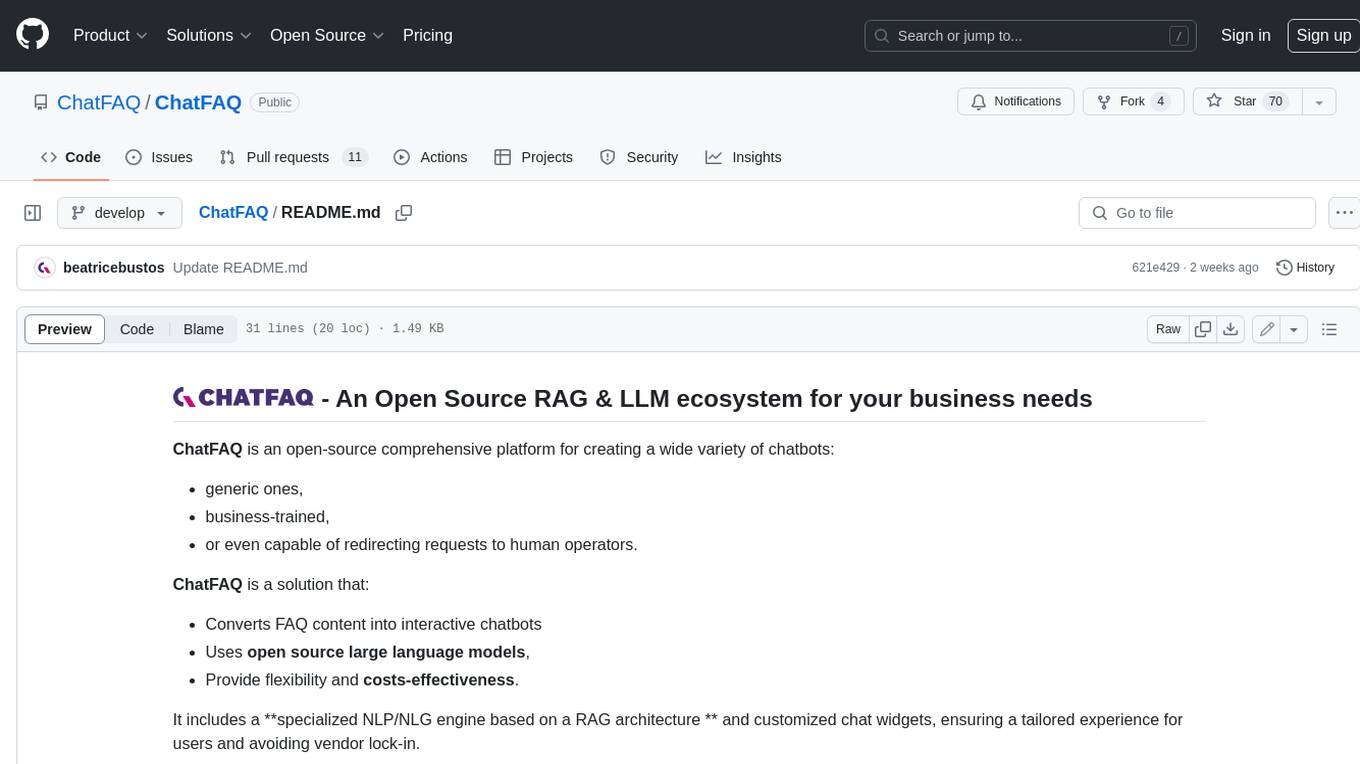
ChatFAQ
ChatFAQ is an open-source comprehensive platform for creating a wide variety of chatbots: generic ones, business-trained, or even capable of redirecting requests to human operators. It includes a specialized NLP/NLG engine based on a RAG architecture and customized chat widgets, ensuring a tailored experience for users and avoiding vendor lock-in.
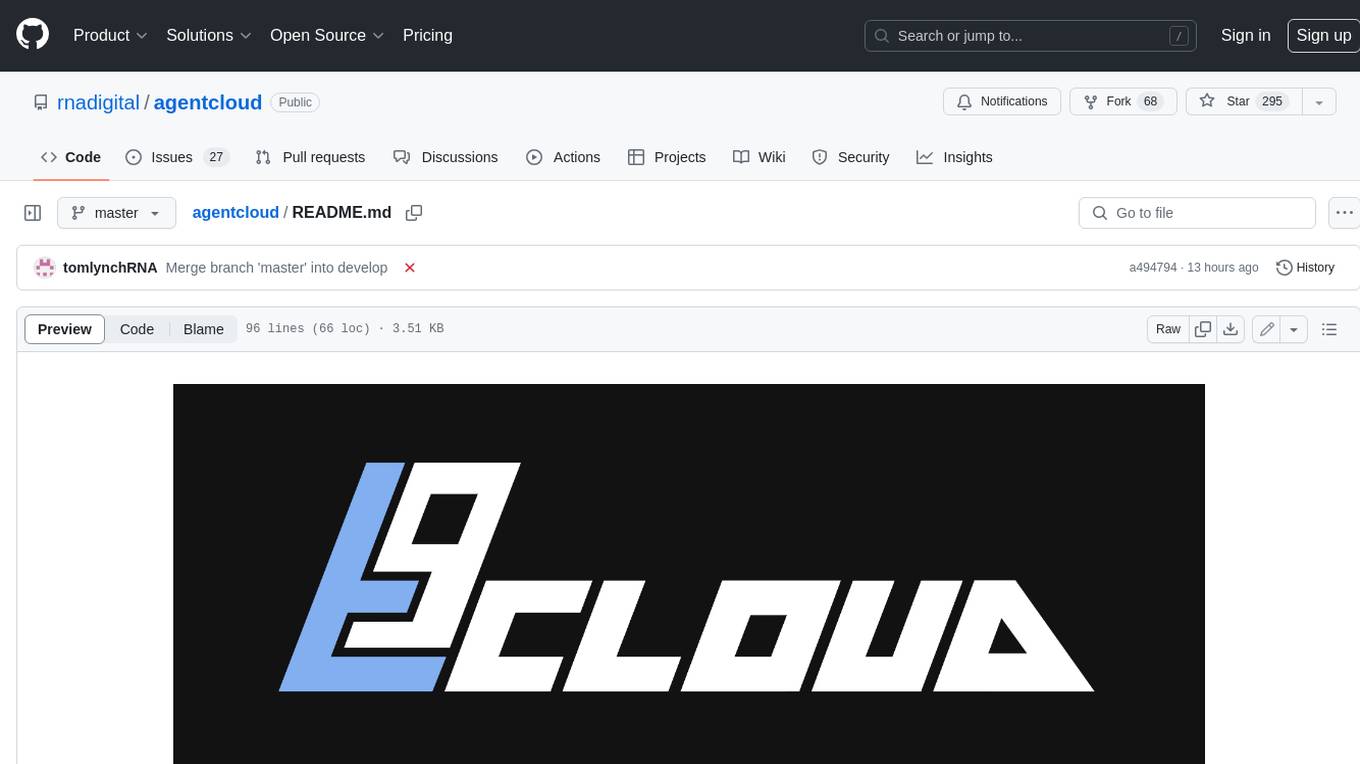
agentcloud
AgentCloud is an open-source platform that enables companies to build and deploy private LLM chat apps, empowering teams to securely interact with their data. It comprises three main components: Agent Backend, Webapp, and Vector Proxy. To run this project locally, clone the repository, install Docker, and start the services. The project is licensed under the GNU Affero General Public License, version 3 only. Contributions and feedback are welcome from the community.
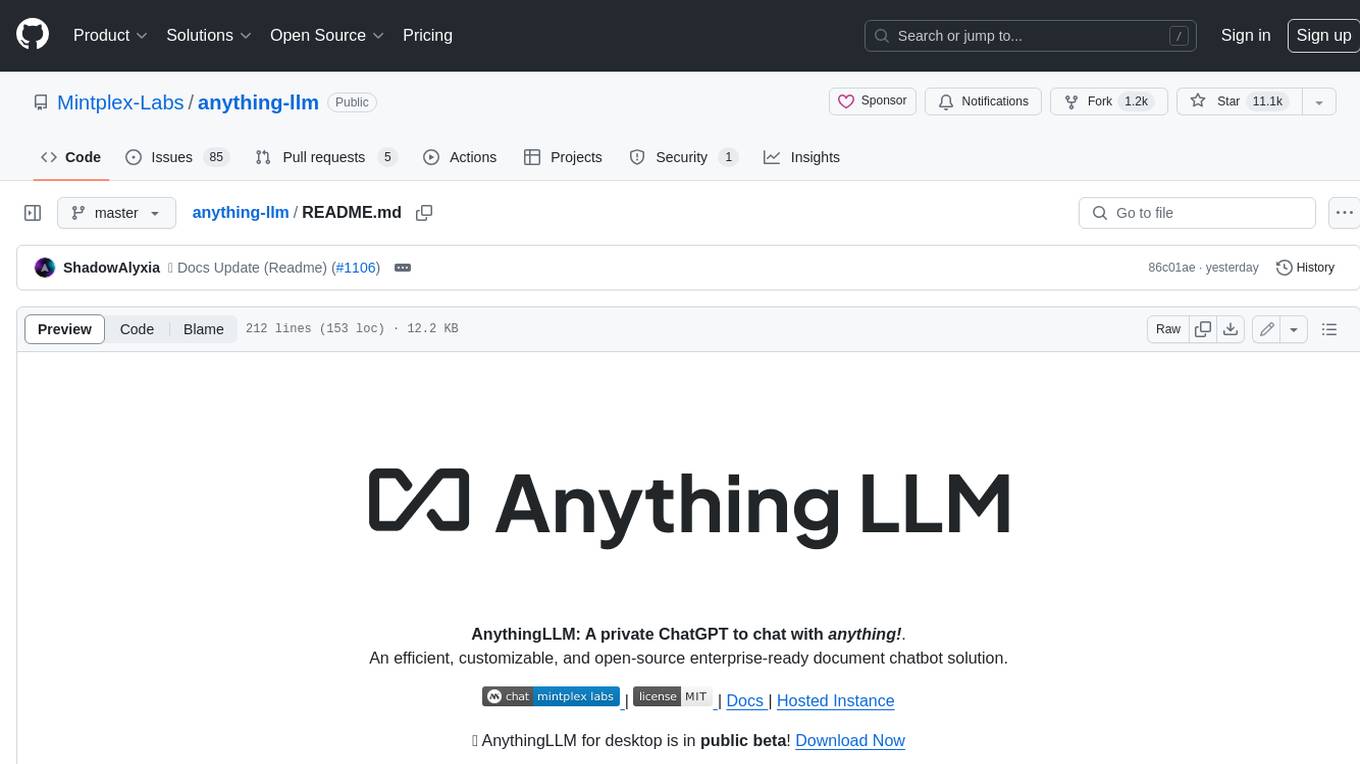
anything-llm
AnythingLLM is a full-stack application that enables you to turn any document, resource, or piece of content into context that any LLM can use as references during chatting. This application allows you to pick and choose which LLM or Vector Database you want to use as well as supporting multi-user management and permissions.
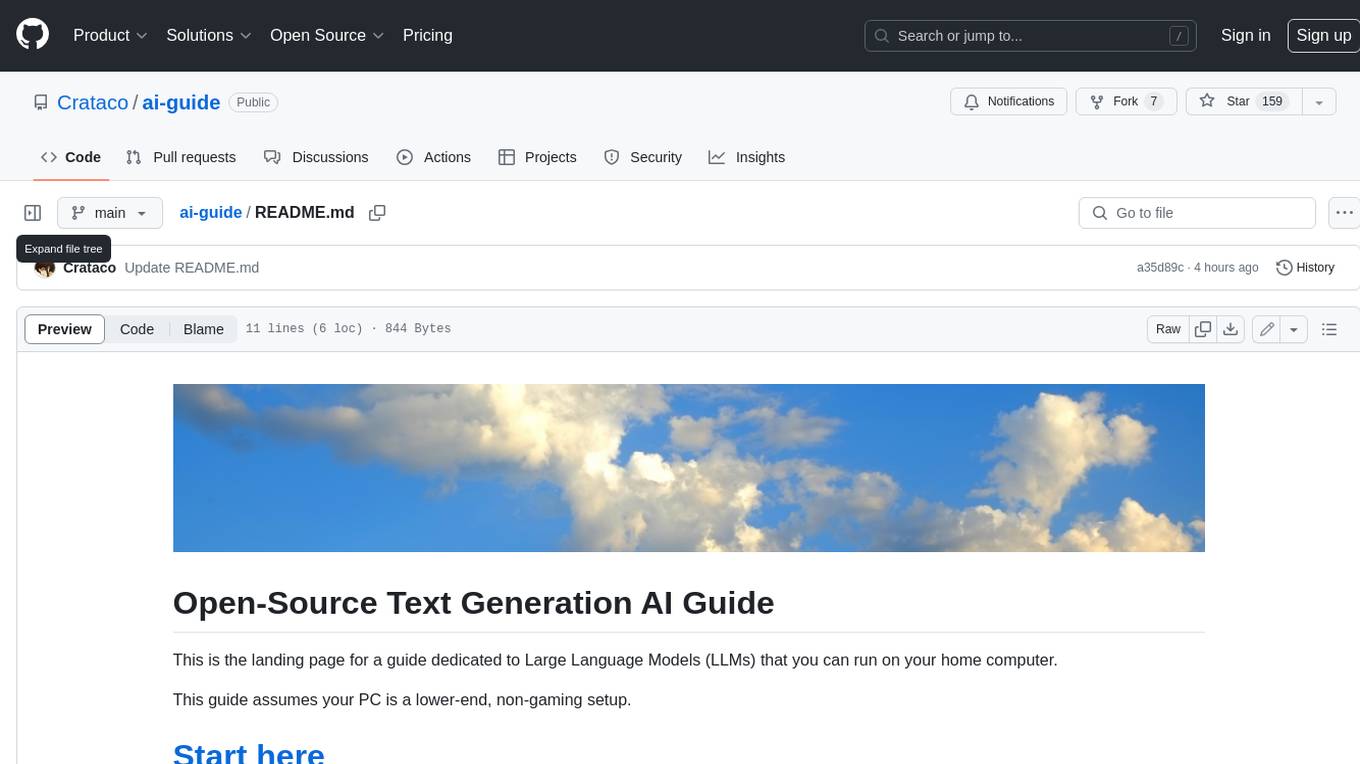
ai-guide
This guide is dedicated to Large Language Models (LLMs) that you can run on your home computer. It assumes your PC is a lower-end, non-gaming setup.
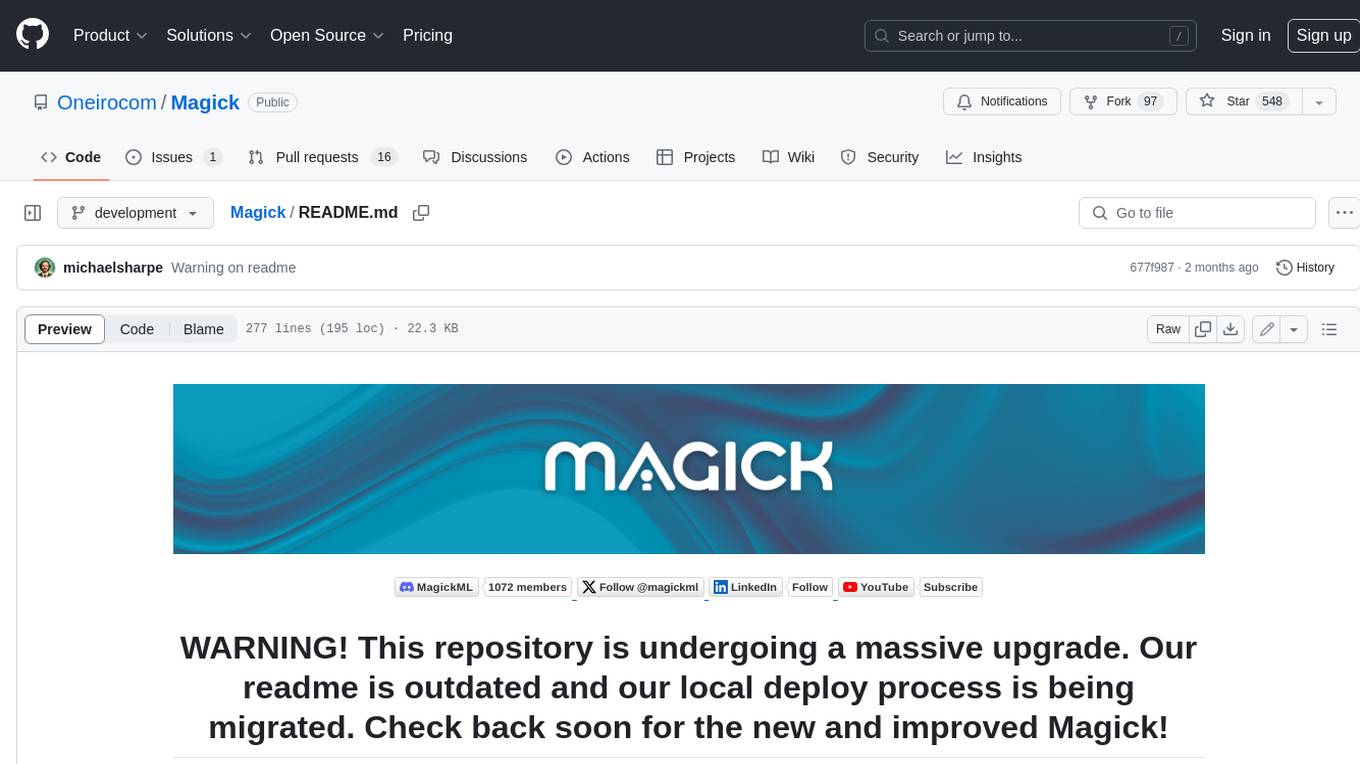
Magick
Magick is a groundbreaking visual AIDE (Artificial Intelligence Development Environment) for no-code data pipelines and multimodal agents. Magick can connect to other services and comes with nodes and templates well-suited for intelligent agents, chatbots, complex reasoning systems and realistic characters.
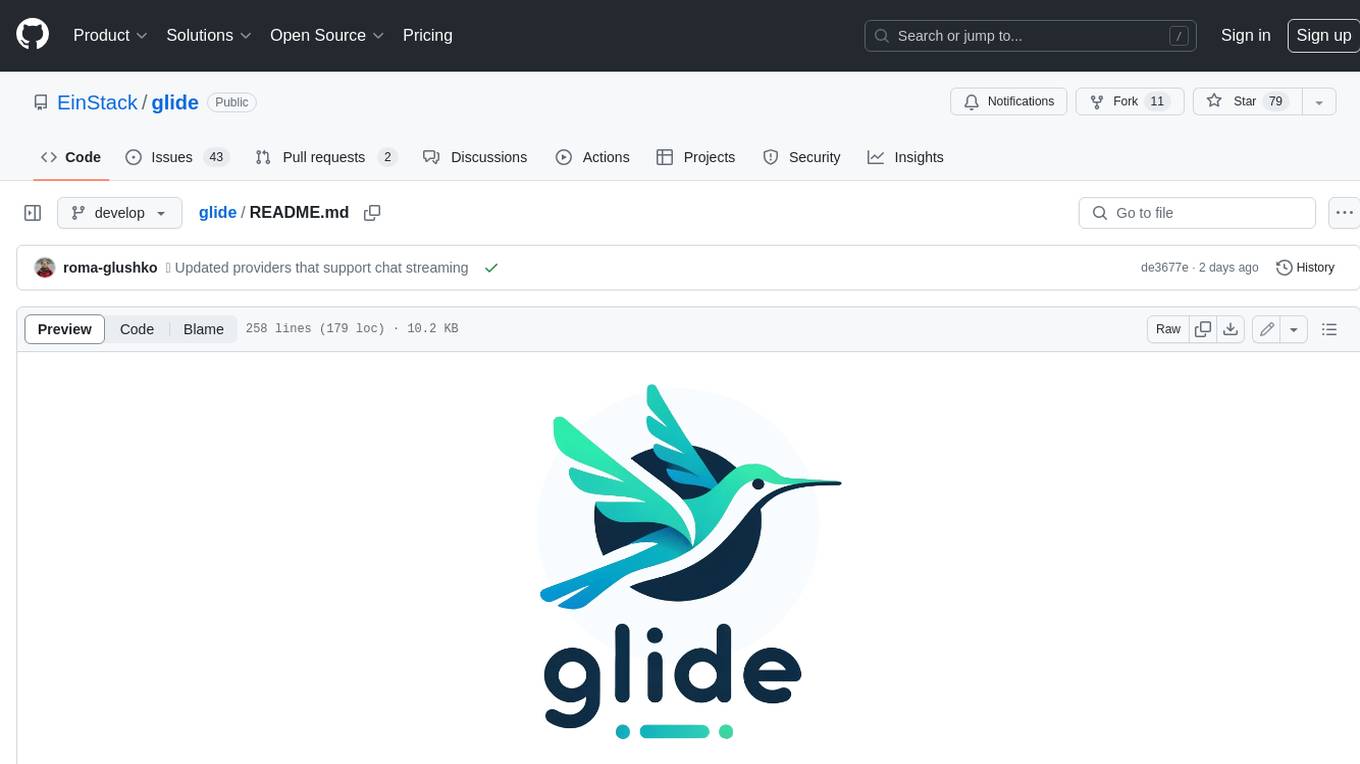
glide
Glide is a cloud-native LLM gateway that provides a unified REST API for accessing various large language models (LLMs) from different providers. It handles LLMOps tasks such as model failover, caching, key management, and more, making it easy to integrate LLMs into applications. Glide supports popular LLM providers like OpenAI, Anthropic, Azure OpenAI, AWS Bedrock (Titan), Cohere, Google Gemini, OctoML, and Ollama. It offers high availability, performance, and observability, and provides SDKs for Python and NodeJS to simplify integration.
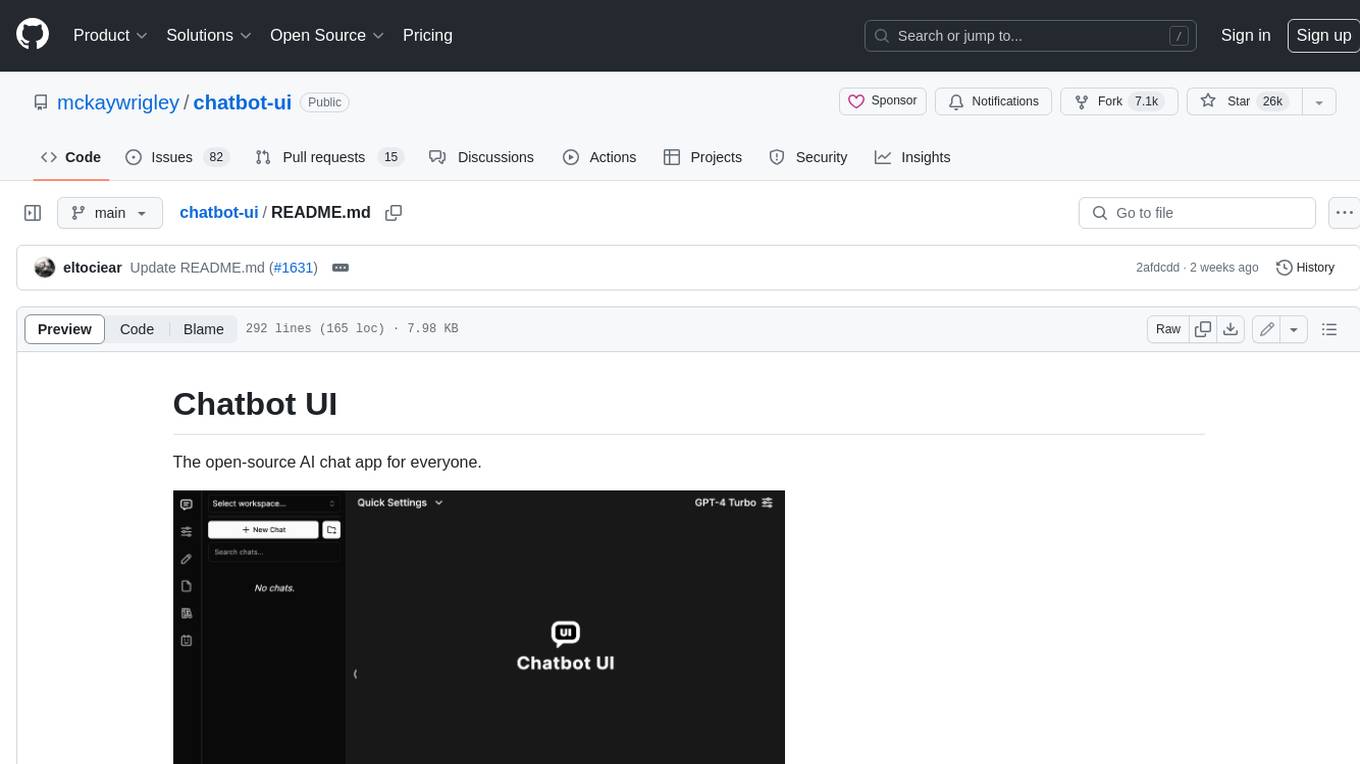
chatbot-ui
Chatbot UI is an open-source AI chat app that allows users to create and deploy their own AI chatbots. It is easy to use and can be customized to fit any need. Chatbot UI is perfect for businesses, developers, and anyone who wants to create a chatbot.
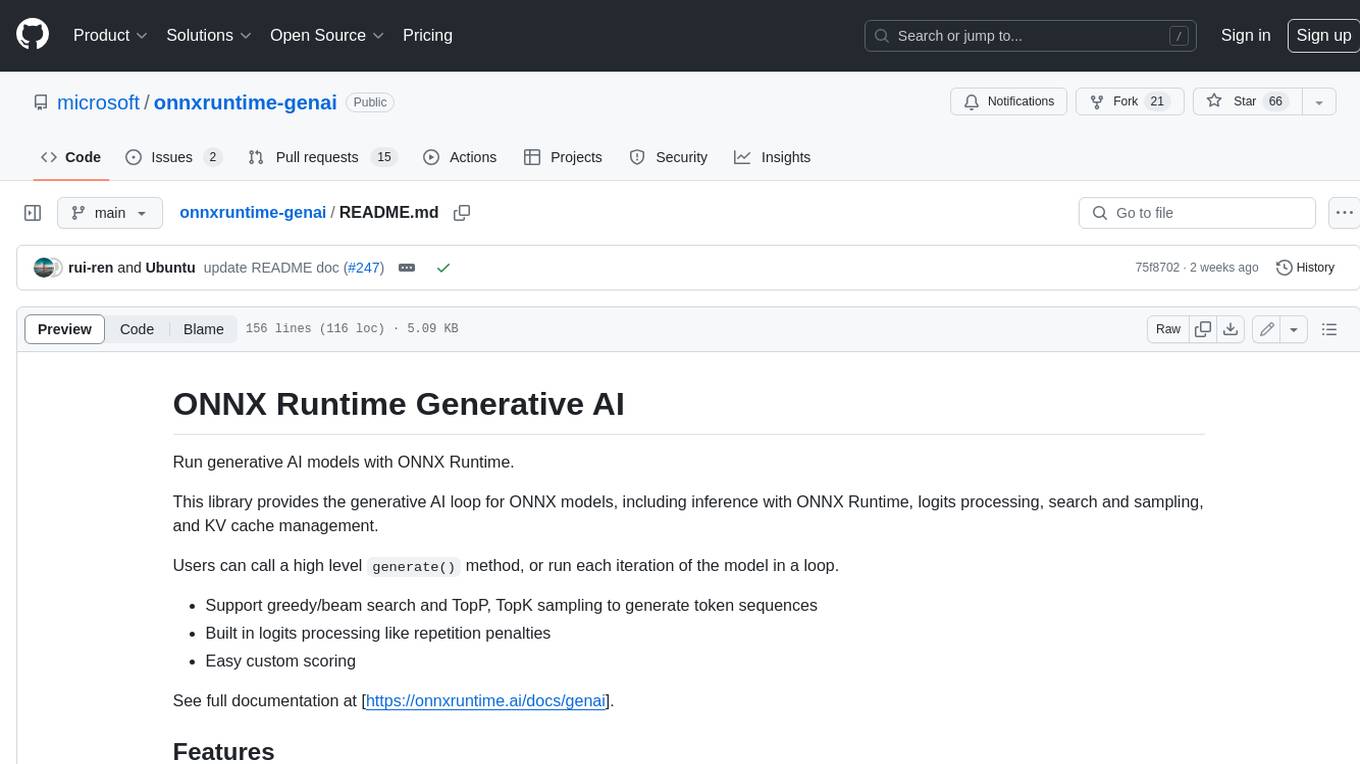
onnxruntime-genai
ONNX Runtime Generative AI is a library that provides the generative AI loop for ONNX models, including inference with ONNX Runtime, logits processing, search and sampling, and KV cache management. Users can call a high level `generate()` method, or run each iteration of the model in a loop. It supports greedy/beam search and TopP, TopK sampling to generate token sequences, has built in logits processing like repetition penalties, and allows for easy custom scoring.