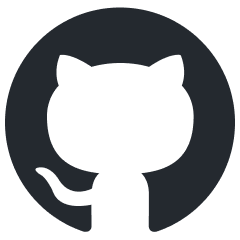
AmigaGPT
AmigaOS 3.1/4.1 and MorphOS application for chatting with ChatGPT or generating images with DALL-E
Stars: 57
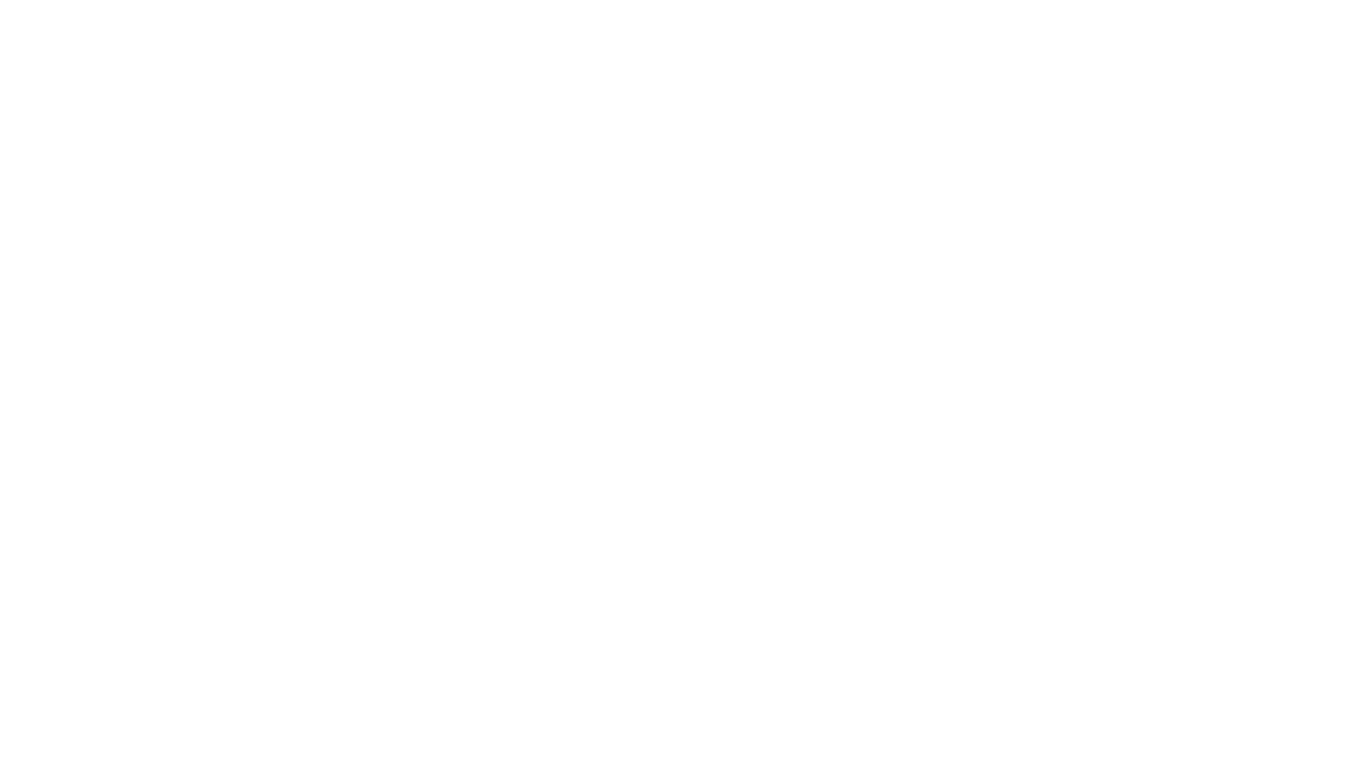
AmigaGPT is a versatile ChatGPT client for AmigaOS 3.x, 4.1, and MorphOS. It brings the capabilities of OpenAI’s GPT to Amiga systems, enabling text generation, question answering, and creative exploration. AmigaGPT can generate images using DALL-E, supports speech output, and seamlessly integrates with AmigaOS. Users can customize the UI, choose fonts and colors, and enjoy a native user experience. The tool requires specific system requirements and offers features like state-of-the-art language models, AI image generation, speech capability, and UI customization.
README:
AmigaGPT is a versatile ChatGPT client for AmigaOS 3.x, 4.1 and MorphOS. This powerful tool brings the capabilities of OpenAI's GPT to your Amiga system, enabling text generation, question answering, and creative exploration. AmigaGPT can also generate stunning images using DALL-E and includes support for speech output, making it easier than ever to interact with AI on your Amiga. Designed to integrate seamlessly with your system, AmigaGPT delivers modern AI technology while embracing the timeless Amiga experience.
AmigaGPT uses the o1, GPT-4o, GPT-4 and GPT-3.5 models developed by OpenAI to generate coherent, context-aware responses to your input.
AmigaGPT can access the powerful DALL-E models to generate images from a prompt. You can view and save the images right inside the app.
AmigaGPT takes full advantage of the MUI framework to provide a smooth, native user experience that is responsive and easy to use.
You can customise the look and feel of the application, including the ability to choose the fonts, colours and a choice of opening in the Workbench screen or a custom screen.
AmigaGPT has support for OpenAI's high quality 16 bit voices. For AmigaOS 3, AmigaGPT can use the Amiga's speech synthesis capability to read the generated text aloud with support for switching between the old Workbench 1.x v34 and the Workbench 2.0 v37 speech synthesisers. For AmigaOS 4.1, it has support for flite.device
.
AmigaGPT includes ARexx support, allowing you to control the application programmatically from external scripts or other applications. You can send prompts, generate images, and utilize speech synthesis through simple ARexx commands.
Ensure you have the necessary system requirements:
- An OCS/ECS/AGA Amiga or a PowerPC machine capable of running MorphOS
- AmigaOS 3.1 or higher, AmigaOS 4.1 or MorphOS
- Motorola 68020 or higher CPU or PowerPC for AmigaOS 4/MorphOS
- Internet access using a TCP/IP stack such as Roadshow (http://roadshow.apc-tcp.de/index-en.php)
- For AmigaOS 3 & 4: AmiSSL 5.18 or higher (https://aminet.net/package/util/libs/AmiSSL-v5-OS3) for OS3 and (https://aminet.net/package/util/libs/AmiSSL-v5-OS4) for OS4
- MUI 3 minimum but MUI 5 recommended for all features (https://github.com/amiga-mui/muidev/releases)
- MCC_Guigfx MUI custom class for displaying images (http://aminet.net/package/dev/mui/MCC_Guigfx)
- MCC_NList MUI custom class for lists (http://aminet.net/package/dev/mui/MCC_NList-0.128)
- codesets.library 6.22 or higher (http://aminet.net/package/util/libs/codesets-6.22)
- An OpenAI account with an active API key
-
Optional: AmigaOS 3 only: A copy of the Workbench 1.x disk to install
narrator.device
v34 and a copy of the Workbench 2.0 disk to installnarrator.device
v37 - Optional: AmigaOS 4 only: Flite device (http://aminet.net/package/mus/misc/flite_device)
- Optional: For OpenAI voices, AHI needs to be installed (http://aminet.net/package/driver/audio/ahiusr_4.18)
- For AmigaOS 3 & 4, Install AmiSSL and a TCP/IP stack if not already done so
- Download and install MUI. Version 5 recommended, version 3 minimum. Reboot.
- Download and install MCC_Guigfx, MCC_NList and codesets.library
- Download the latest release of AmigaGPT
- Extract the
amigagpt.lha
archive to a temporary location - Run the provided installer
AmigaGPT supports reading the output aloud. How AmigaGPT does this depends on whether you are using AmigaOS 3 or 4. Or for OpenAI voices, this works on every system.
If your OS does not come with AHI installed, you can get it from https://aminet.net/package/driver/audio/ahiusr_4.18
AmigaGPT supports reading the output aloud. This requires a file called narrator.device
which cannot be included with AmigaGPT because it is still under copyright. Therefore, you must copy this file legally from your Workbench disks so that AmigaGPT will be able to synthesise speech. There are 2 versions of narrator.device
supported, v34 and v37.
v34 is the original version that came with Workbench 1.x. v37 was an updated version included with Workbench 2.0.x. It has more features and sounds more natural, however it does sound quite different which is why AmigaGPT supports you installing both versions and your choice of version to be used can be selected in the Speech menu in the app.
Regardless of which version of narrator.device
you choose to install (or both), AmigaGPT requires that you install the free third party translator.library
v43. This works with both versions of narrator.device
.
Since translator.library
v43 is not available as a standalone install, you will need to install v42 and then patch it to v43.
- Download http://aminet.net/util/libs/translator42.lha and extract the archive to any convenient location on your Amiga such as
RAM:
- Navigate to that directory and double click the
Install
program - Run the installer using all the default settings
- Download http://aminet.net/util/libs/Tran43pch.lha and once again extract it to a location of your choice
- Navigate to that directory and double click the
Install
program - Run the installer using all the default settings
- Reboot your Amiga - It will not work until the system is restarted
- Insert your Workbench 1.x disk and copy
df0:devs/narrator.device
to{AmigaGPTProgramDirectory}/devs/speech/34
- Insert your Workbench 2.0.x (you cannot use 2.1 because the speech libraries were removed after version 2.0.4) disk and copy
df0:devs/narrator.device
to{AmigaGPTProgramDirectory}/devs/speech/37
- AmigaGPT for AmigaOS 4 uses the Flite device to provide speech synthesis. Download it from http://aminet.net/package/mus/misc/flite_device.
- Extract the archive and run the installer
- Launch the application by double-clicking the AmigaGPT icon
- You may also launch the app in the command line but before you do, run the command
STACK 32768
to give the program 32kb of stack since the default stack size for apps launched from the shell is 4kb and this is not enough for AmigaGPT and will cause random crashes due to stack overflow. This is not required when you launch the app by double clicking the icon since the stack size is saved in the icon
When launched, AmigaGPT presents you with a choice of opening the app in a new screen or opening in Workbench. If you open in a new screen you have the ability to create a screen for the app to open in. AmigaGPT supports anything from 320x200 all the way up to 4k resolution if using a video card for RTG. Bear in mind text will appear very tiny in resolutions above 1080p so you may want to increase the font size settings from the View menu when the app opens.
When launching for the first time you will need to enter your OpenAI API key before you can start chatting. If you haven't already done so, create an OpenAI account and navigate to https://platform.openai.com/account/api-keys to generate an API key for use with AmigaGPT.
There are 2 main modes of operation: Chat and Image Generation. You can switch between them via the tabs in the top left corner.
When the app has opened, you are presented with a text input box. You can type any prompt into this box and press "Send" to see the GPT model's response. The generated text appears in the box above the input. You can choose to have this text read aloud using the "Speech" menu option. You can also select which model for OpenAI to use in the "OpenAI" menu option.
To the left of the chat box is a conversation list which you can use to go to another saved conversation. New conversations can be created with the "New chat" button and conversations can be removed with the "Delete chat" button.
To generate images, simply select your desired image generation model from the "OpenAI" menu then type your prompt in the text box then hit the "Create Image" button. When it has been downloaded to your Amiga, you are then able to open the image to your desired scale, or save a copy of the file to a new location on your Amiga. Do note however that AmigaGPT will automatially save all your generated images until you delete them. This is just in case you would like to create a copy elsewhere.
The "Project" menu includes an "About" option, which displays information about the program.
In the "Edit" menu, you'll find basic text editing commands like Cut, Copy, Paste and Clear.
The "View" menu allows you to change the appearance of the app.
The "Connection" menu allows you to connect via a proxy server. It supports both HTTP and HTTPS proxy servers but if you use an unecrypted HTTP proxy server you can improve the performance of AmigaGPT by removing the need for the encryption of the OpenAI traffic to be done on the system running AmigaGPT. For an easy proxy server you can run on your local network you can try out https://mitmproxy.org
AmigaGPT now includes ARexx support, allowing you to control the application from external ARexx scripts or other applications. This powerful feature enables seamless integration with your Amiga workflow and automation of repetitive tasks.
The following ARexx commands are available:
Sends a message to the OpenAI API and returns the response.
SENDMESSAGE M=MODEL/K,S=SYSTEM/K,K=APIKEY/K,P=PROMPT/F
-
M=MODEL
- Optional, the chat model to use (use LISTCHATMODELS to see available models) -
S=SYSTEM
- Optional, system message to include -
K=APIKEY
- Optional, your OpenAI API key (uses the saved key if not specified) -
P=PROMPT
- Required, the prompt or question to send
Generates an image using the specified model.
CREATEIMAGE M=MODEL/K,S=SIZE/K,K=APIKEY/K,D=DESTINATION/K,P=PROMPT/F
-
M=MODEL
- Optional, the image model to use (use LISTIMAGEMODELS to see available models) -
S=SIZE
- Optional, image size (use LISTIMAGESIZES to see available sizes) -
K=APIKEY
- Optional, your OpenAI API key -
D=DESTINATION
- Optional, the path where the image will be saved -
P=PROMPT
- Required, description of the image to generate
Uses text-to-speech to speak the specified text.
SPEAKTEXT M=MODEL/K,V=VOICE/K,I=INSTRUCTIONS/K,K=APIKEY/K,P=PROMPT/F
-
M=MODEL
- Optional, the voice model to use (use LISTVOICEMODELS to see available models) -
V=VOICE
- Optional, the voice to use (use LISTVOICES to see available voices) -
I=INSTRUCTIONS
- Optional, special instructions for the voice -
K=APIKEY
- Optional, your OpenAI API key -
P=PROMPT
- Required, the text to speak
-
LISTCHATMODELS
- Lists all available chat models -
LISTIMAGEMODELS
- Lists all available image models -
LISTIMAGESIZES
- Lists all available image sizes -
LISTVOICEMODELS
- Lists all available TTS models -
LISTVOICES
- Lists all available TTS voices
-
?
- Displays a list of available commands
/* Simple ARexx script for AmigaGPT */
OPTIONS RESULTS
ADDRESS 'AMIGAGPT'
/* Send a message to GPT and get a response */
'SENDMESSAGE P=What is the capital of France?'
SAY RESULT
/* Generate an image */
'CREATEIMAGE P=A beautiful Amiga computer on a desk'
SAY 'Image saved to:' RESULT
/* Speak some text */
'SPEAKTEXT P=Hello from ARexx!'
EXIT
For the latest information on the models you can use in AmigaGPT, please refer to OpenAI's documentation at https://platform.openai.com/docs/models
You can either compile the code natively or with the Docker container.
If you would like to build this project from source you will need Bebbo's amiga-gcc toolchain here https://github.com/bebbo/amiga-gcc
Once installed, get the required other SDK's (AmiSSL, Translator, json-c) from https://github.com/sacredbanana/AmigaSDK-gcc and put these in your Amiga dev environment created in the above step.
Get this toolchain set up https://github.com/sba1/adtools
Once installed, get the required other SDK's (AmiSSL, Translator, json-c) from https://github.com/sacredbanana/AmigaSDK-gcc and put these in your Amiga dev environment created in the above step.
You may use pre-prepared Docker images that are able to compile both the AmigaOS 3 and AmigaOS 4 versions of the app.
Just install Docker on your machine and run the build_os3.sh
or build_os4.sh
scripts depending on which version of the app you want to build. If you want to perform a clean build, you can set the environment variable CLEAN=1
for example you can run CLEAN=1 ./build_os3.sh
.
The build app will be saved to the /out
directory.
AmigaGPT is licensed under the MIT License.
We welcome contributions to AmigaGPT! If you have a bug to report, a feature to suggest, or a change you'd like to make to the code, please open a new issue or submit a pull request.
- Cameron Armstrong (sacredbanana/Nightfox) https://github.com/sacredbanana/
- Mauricio Sandoval - Icon design
- Mauricio Sandoval - Spanish
- Tobias Baeumer - German
- Bebbo for creating the Amiga GCC toolchain https://github.com/bebbo
- OpenAI for making this all possible https://openai.com
- EAB and everyone in it for answering my questions https://eab.abime.net/
- Ján Zahurančík for all the thorough testing, bundling AmigaGPT into AmiKit and for all the moral support https://www.amikit.amiga.sk
- CoffinOS for bundling AmigaGPT into CoffinOS https://getcoffin.net
- Amiga Future Magazine for reviewing AmigaGPT and publishing several of its updates in the News from Aminet section https://www.amigafuture.de/
- WhatIFF? Magaine for reviewing AmigaGPT and interviewing me in issue 14 https://www.whatiff.info
- Dan Wood for reviewing AmigaGPT on his YouTube channel https://www.youtube.com/watch?v=-OA28r8Up5U
- Proteque-CBN for reviewing AmigaGPT on his YouTube channel https://www.youtube.com/watch?v=t3q8HQ6wrnw
- AmigaBill for covering AmigaGPT in the Amiga News section on his Twitch streams and allowing me to join his stream to promote it https://www.twitch.tv/amigabill
- Les Docs for making a video review and giving a tutorial on how to add support for the French accent https://www.youtube.com/watch?v=BV5Fq1PresE
For Tasks:
Click tags to check more tools for each tasksFor Jobs:
Alternative AI tools for AmigaGPT
Similar Open Source Tools
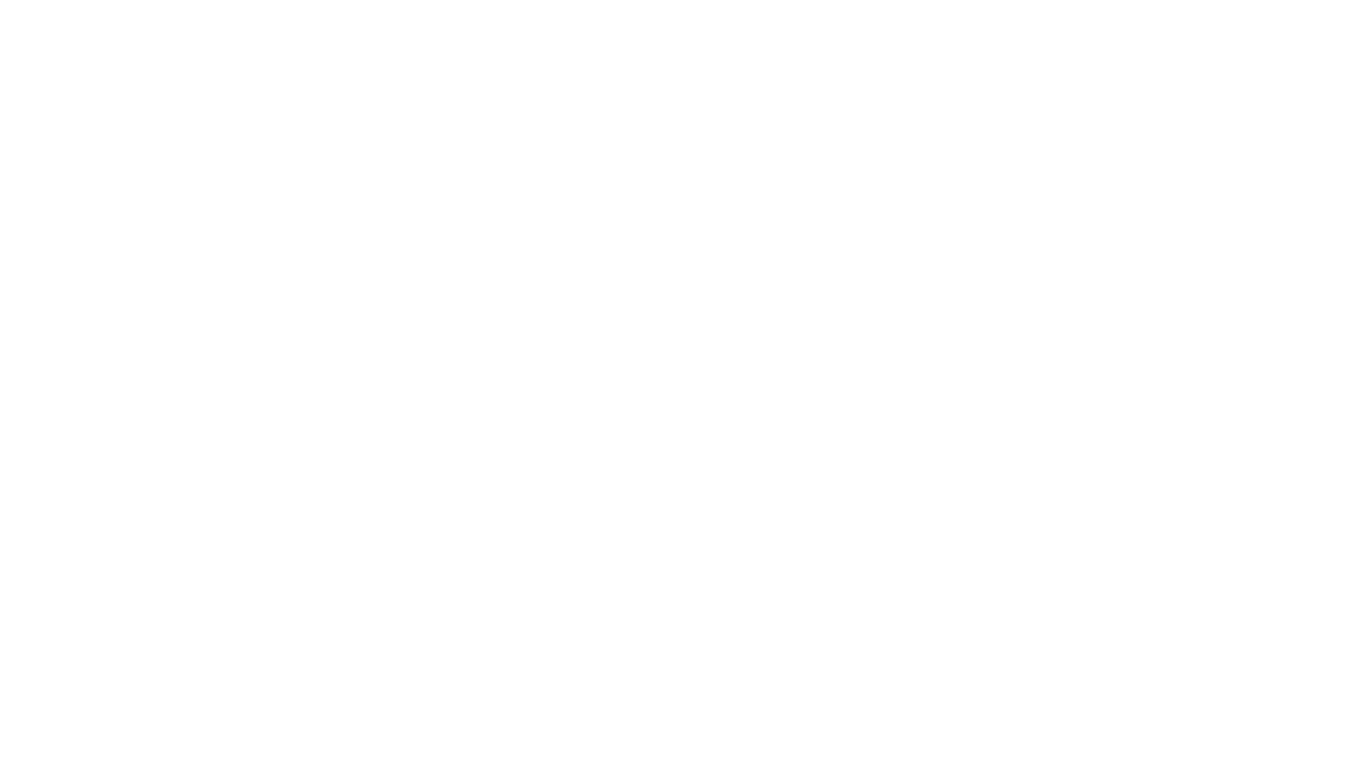
AmigaGPT
AmigaGPT is a versatile ChatGPT client for AmigaOS 3.x, 4.1, and MorphOS. It brings the capabilities of OpenAI’s GPT to Amiga systems, enabling text generation, question answering, and creative exploration. AmigaGPT can generate images using DALL-E, supports speech output, and seamlessly integrates with AmigaOS. Users can customize the UI, choose fonts and colors, and enjoy a native user experience. The tool requires specific system requirements and offers features like state-of-the-art language models, AI image generation, speech capability, and UI customization.
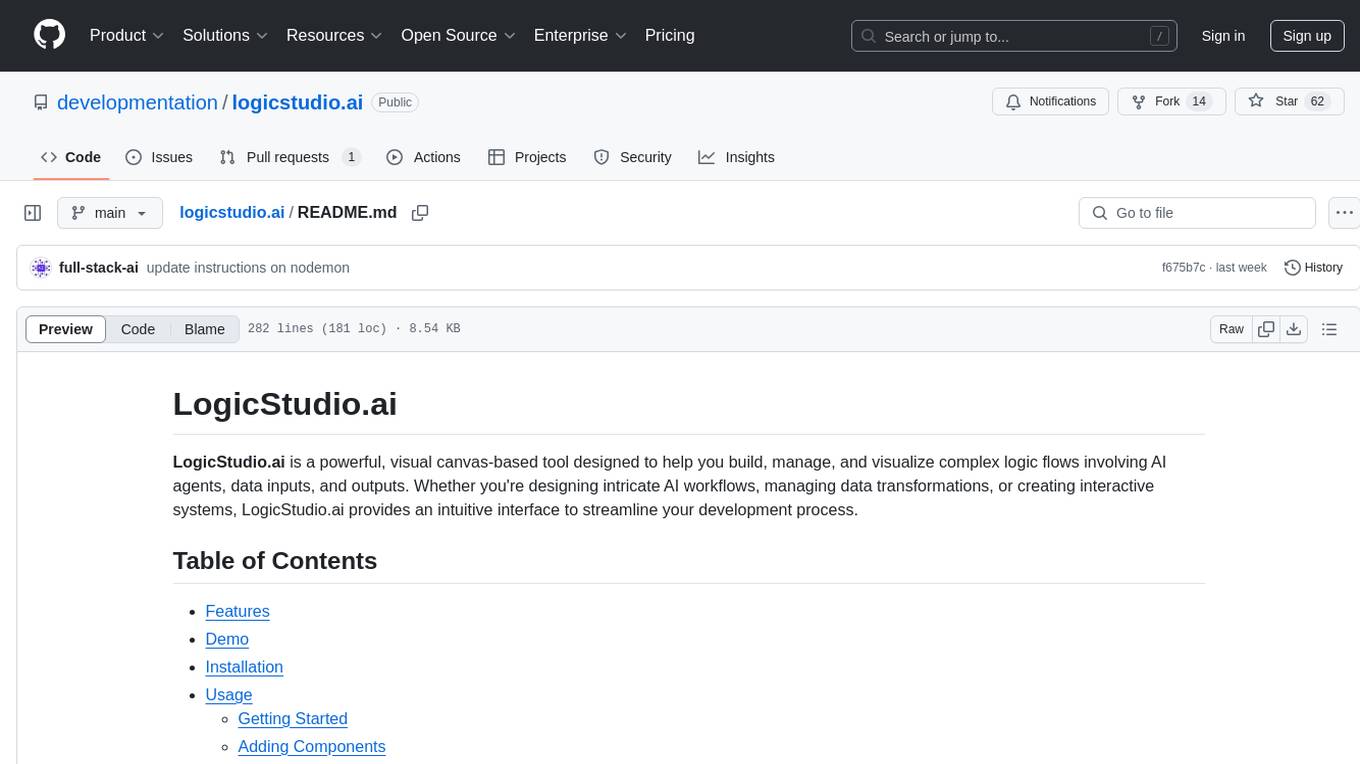
logicstudio.ai
LogicStudio.ai is a powerful visual canvas-based tool for building, managing, and visualizing complex logic flows involving AI agents, data inputs, and outputs. It provides an intuitive interface to streamline development processes by offering features like drag-and-drop canvas design, dynamic components, real-time connections, import/export capabilities, zoom & pan controls, file management, AI integration, editable views, and various output formats. Users can easily add, connect, configure, and manage components to create interactive systems and workflows.
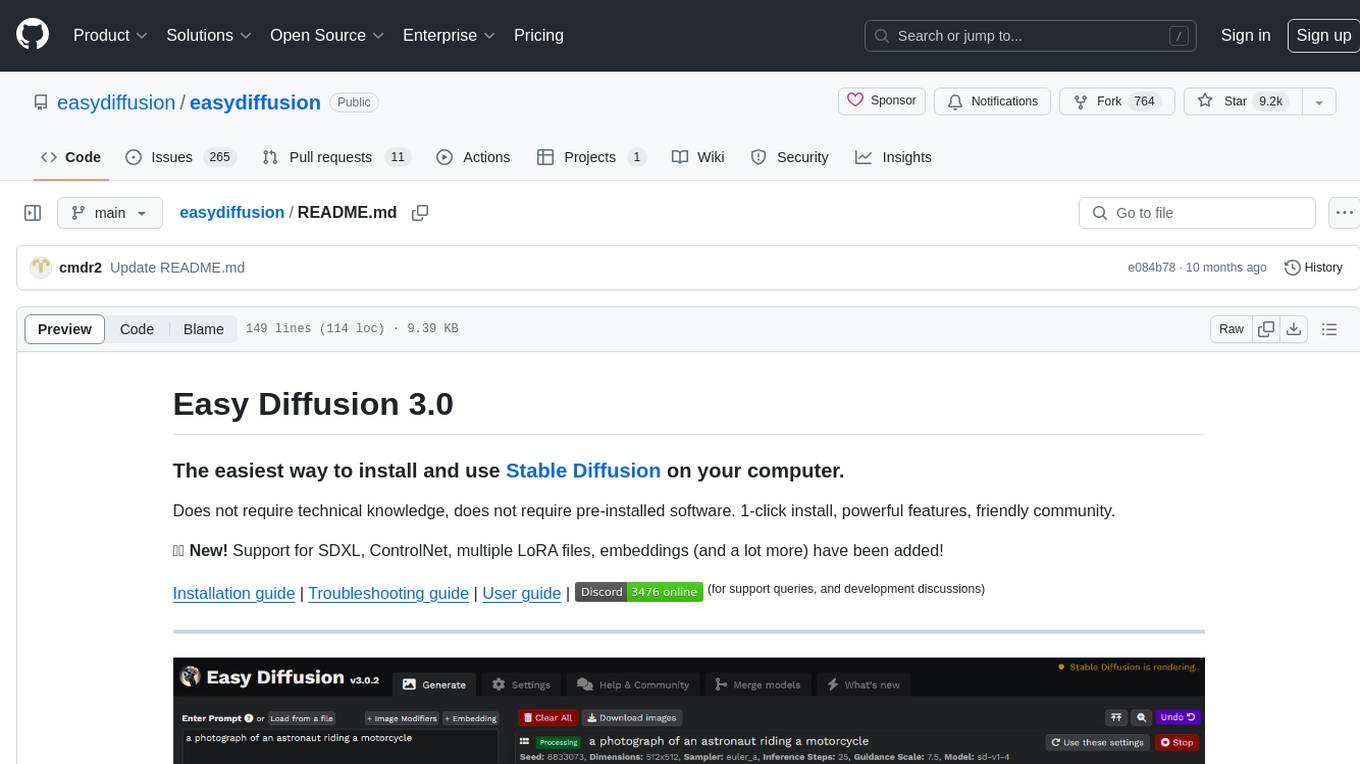
easydiffusion
Easy Diffusion 3.0 is a user-friendly tool for installing and using Stable Diffusion on your computer. It offers hassle-free installation, clutter-free UI, task queue, intelligent model detection, live preview, image modifiers, multiple prompts file, saving generated images, UI themes, searchable models dropdown, and supports various image generation tasks like 'Text to Image', 'Image to Image', and 'InPainting'. The tool also provides advanced features such as custom models, merge models, custom VAE models, multi-GPU support, auto-updater, developer console, and more. It is designed for both new users and advanced users looking for powerful AI image generation capabilities.
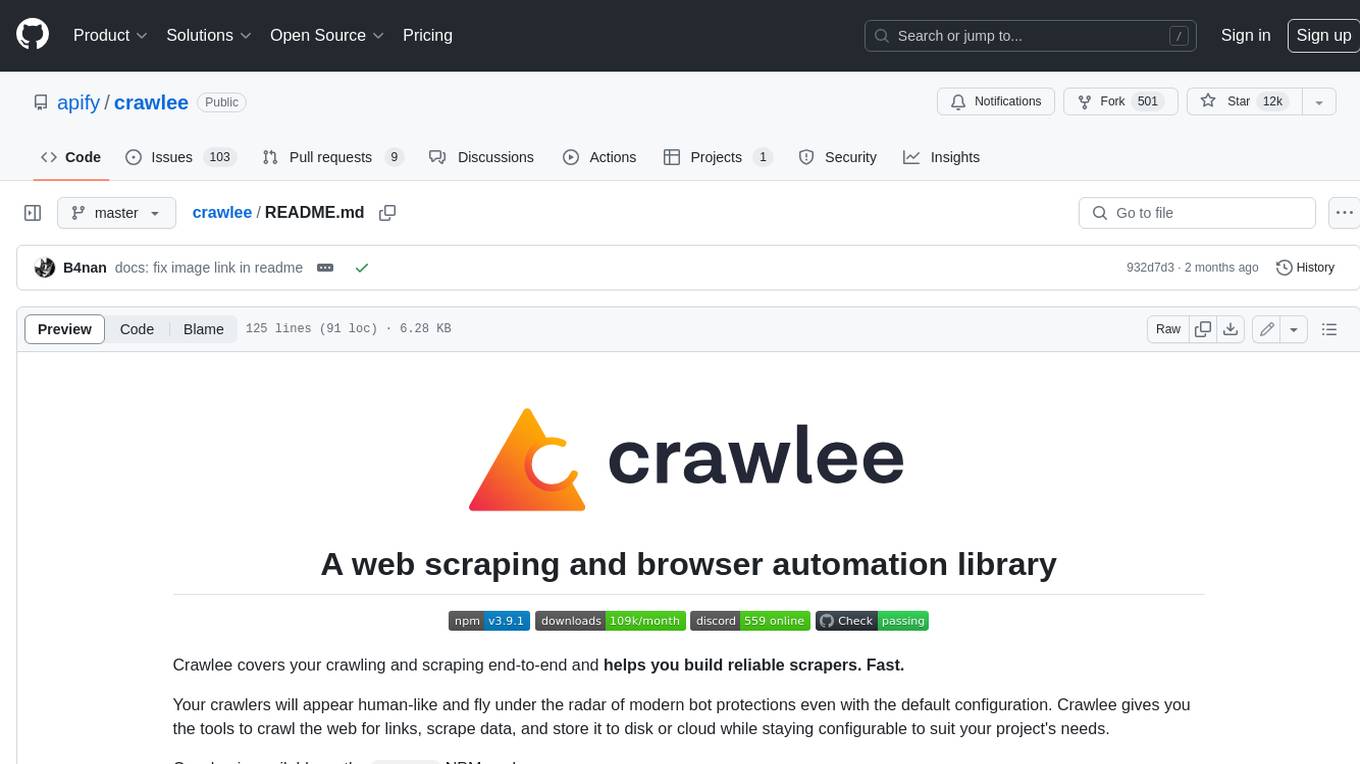
crawlee
Crawlee is a web scraping and browser automation library that helps you build reliable scrapers quickly. Your crawlers will appear human-like and fly under the radar of modern bot protections even with the default configuration. Crawlee gives you the tools to crawl the web for links, scrape data, and store it to disk or cloud while staying configurable to suit your project's needs.
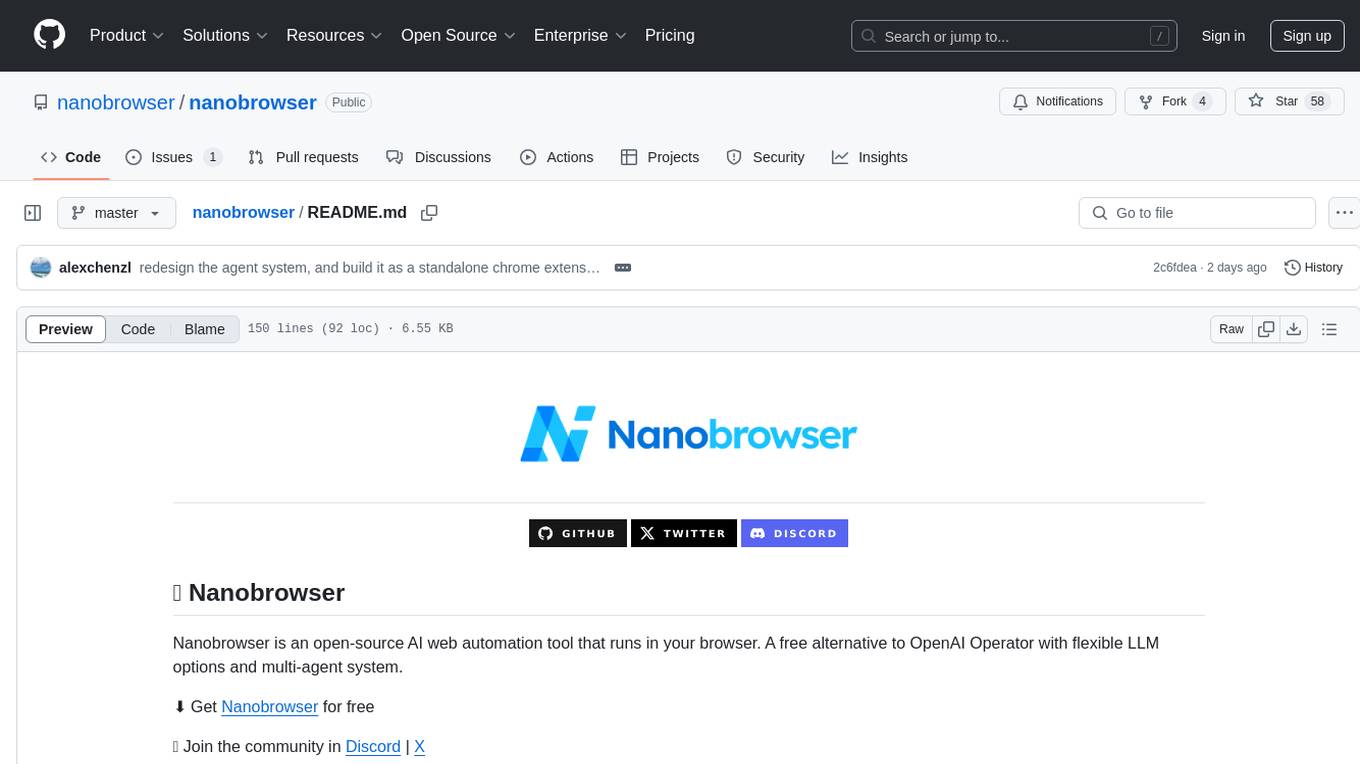
nanobrowser
Nanobrowser is an open-source AI web automation tool that runs in your browser. It is a free alternative to OpenAI Operator with flexible LLM options and a multi-agent system. Nanobrowser offers premium web automation capabilities while keeping users in complete control, with features like a multi-agent system, interactive side panel, task automation, follow-up questions, and multiple LLM support. Users can easily download and install Nanobrowser as a Chrome extension, configure agent models, and accomplish tasks such as news summary, GitHub research, and shopping research with just a sentence. The tool uses a specialized multi-agent system powered by large language models to understand and execute complex web tasks. Nanobrowser is actively developed with plans to expand LLM support, implement security measures, optimize memory usage, enable session replay, and develop specialized agents for domain-specific tasks. Contributions from the community are welcome to improve Nanobrowser and build the future of web automation.
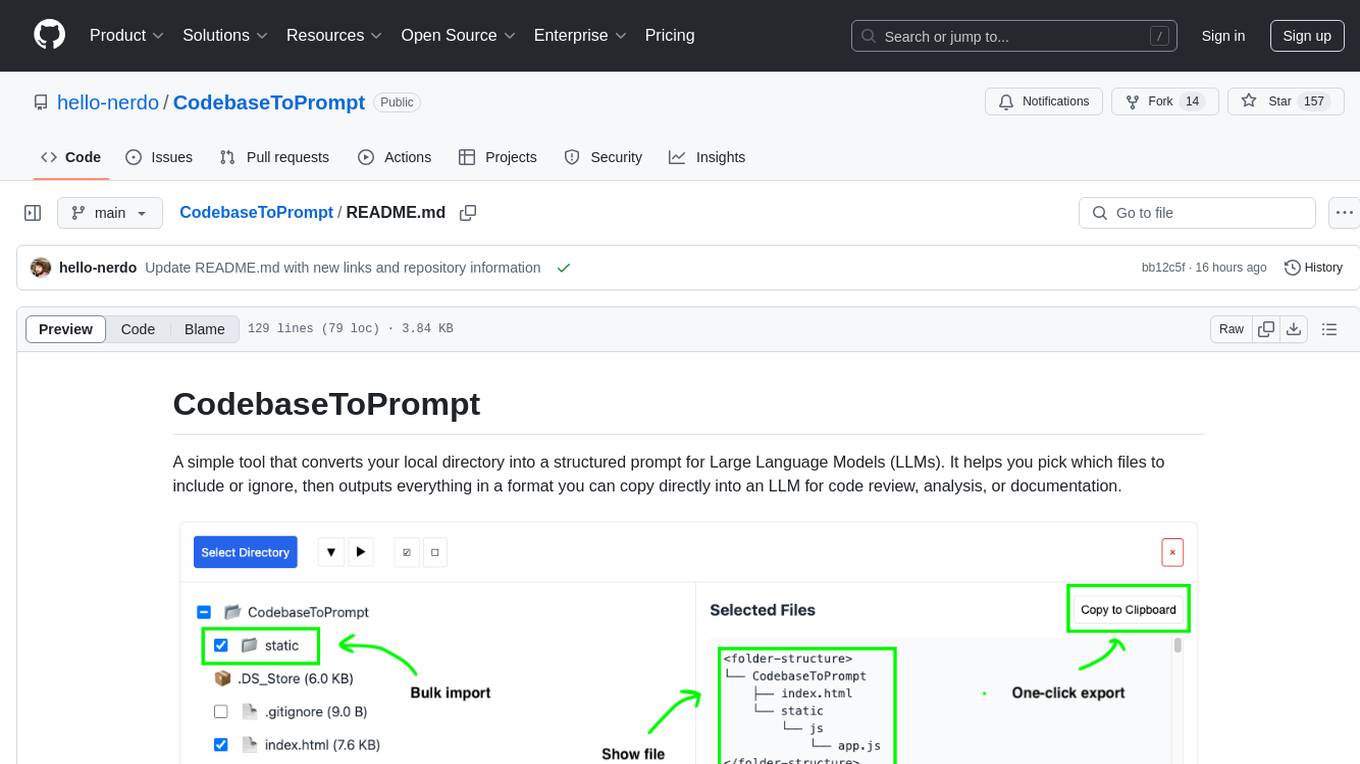
CodebaseToPrompt
CodebaseToPrompt is a tool that converts a local directory into a structured prompt for Large Language Models (LLMs). It allows users to select specific files for code review, analysis, or documentation by exploring and filtering through the file tree in an interactive interface. The tool generates a formatted output that can be directly used with LLMs, estimates token count, and supports flexible text selection. Users can deploy the tool using Docker for self-contained usage and can contribute to the project by opening issues or submitting pull requests.
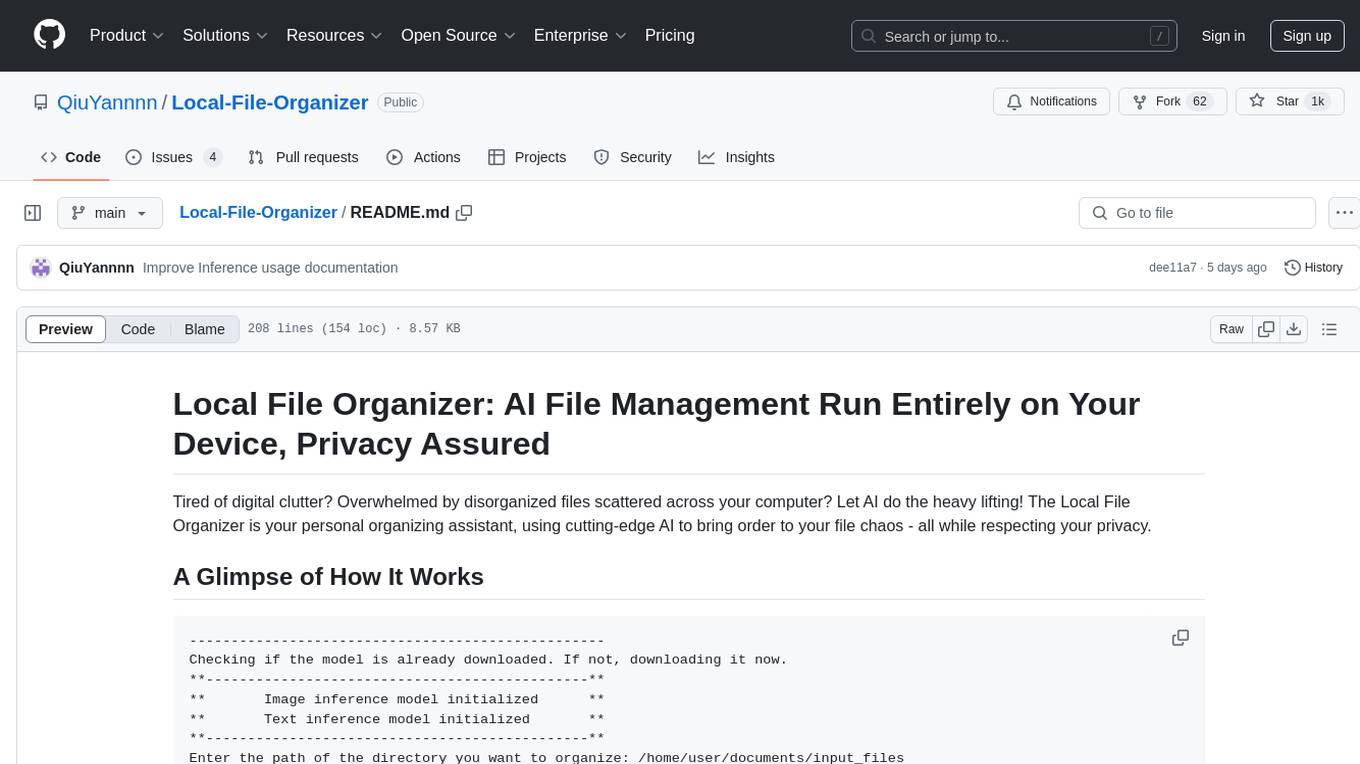
Local-File-Organizer
The Local File Organizer is an AI-powered tool designed to help users organize their digital files efficiently and securely on their local device. By leveraging advanced AI models for text and visual content analysis, the tool automatically scans and categorizes files, generates relevant descriptions and filenames, and organizes them into a new directory structure. All AI processing occurs locally using the Nexa SDK, ensuring privacy and security. With support for multiple file types and customizable prompts, this tool aims to simplify file management and bring order to users' digital lives.
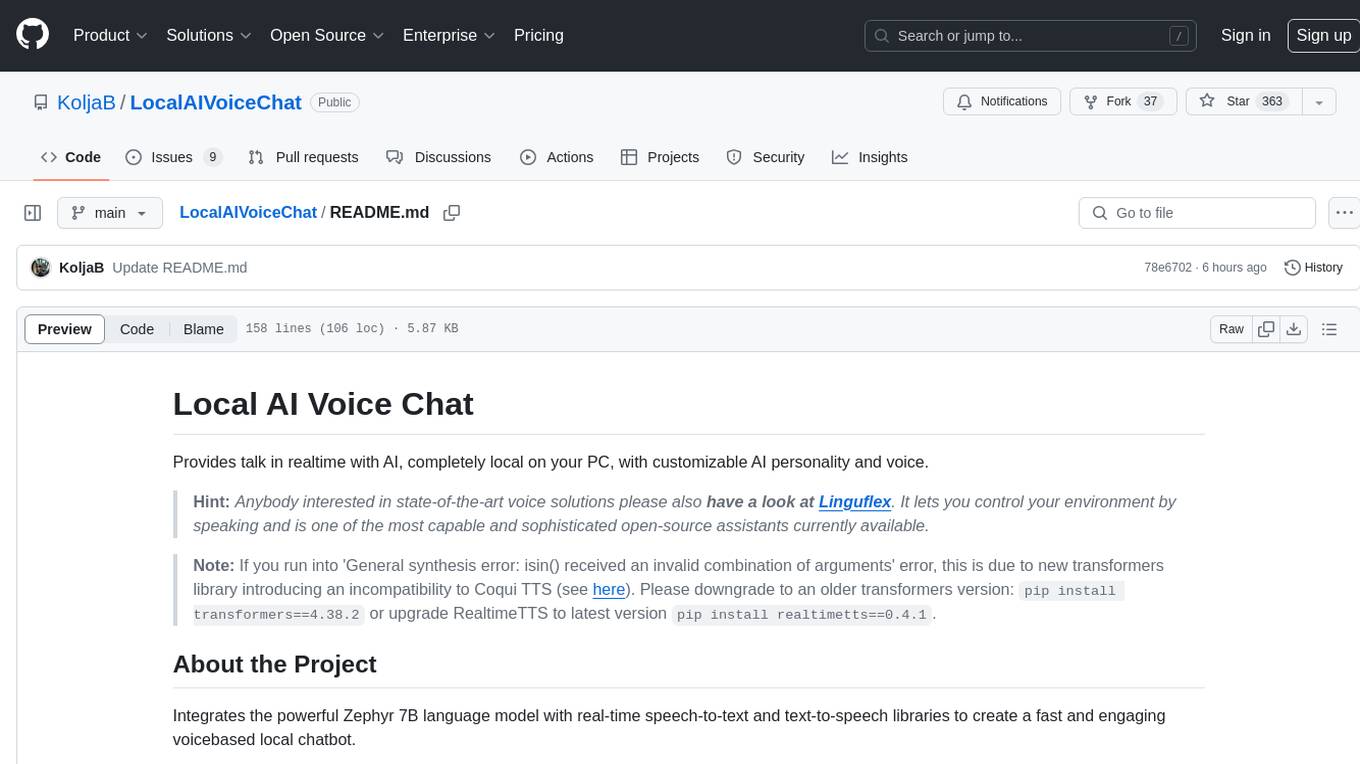
LocalAIVoiceChat
LocalAIVoiceChat is an experimental alpha software that enables real-time voice chat with a customizable AI personality and voice on your PC. It integrates Zephyr 7B language model with speech-to-text and text-to-speech libraries. The tool is designed for users interested in state-of-the-art voice solutions and provides an early version of a local real-time chatbot.
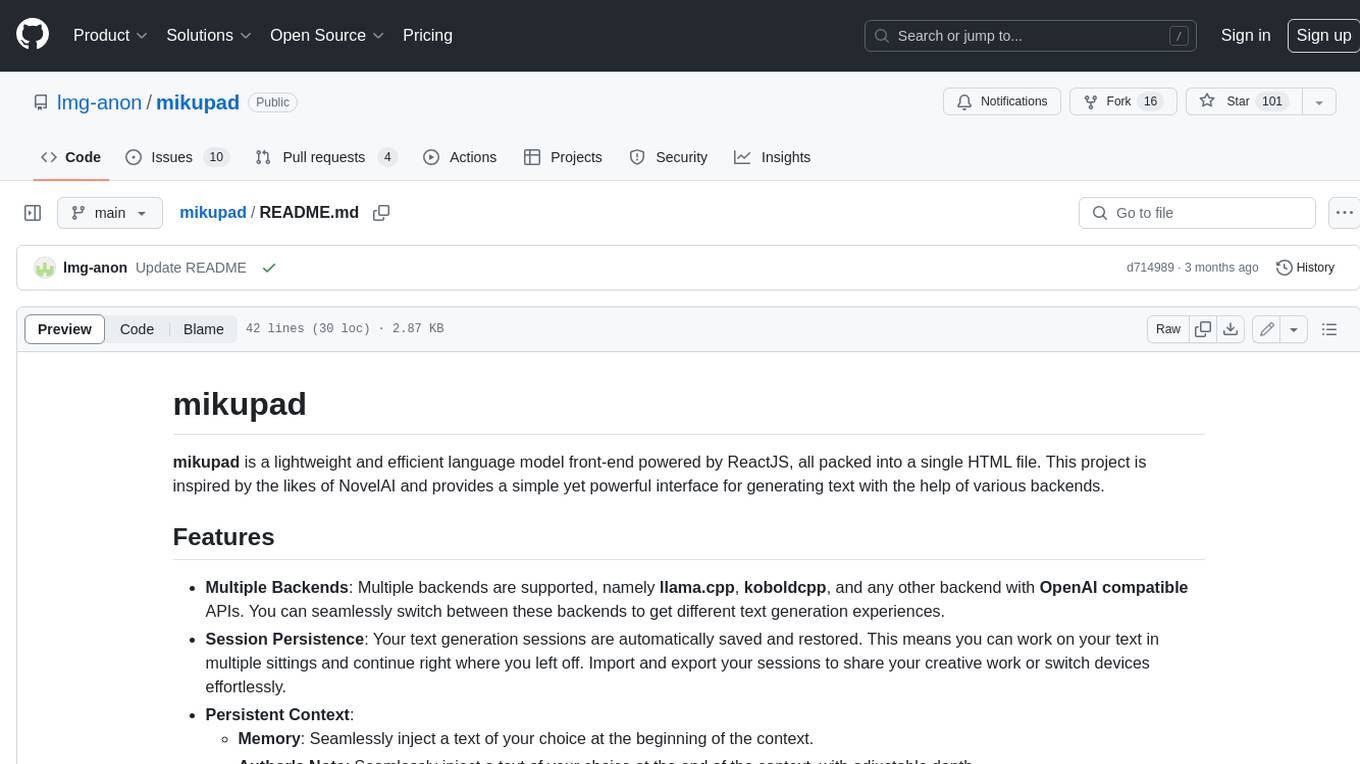
mikupad
mikupad is a lightweight and efficient language model front-end powered by ReactJS, all packed into a single HTML file. Inspired by the likes of NovelAI, it provides a simple yet powerful interface for generating text with the help of various backends.
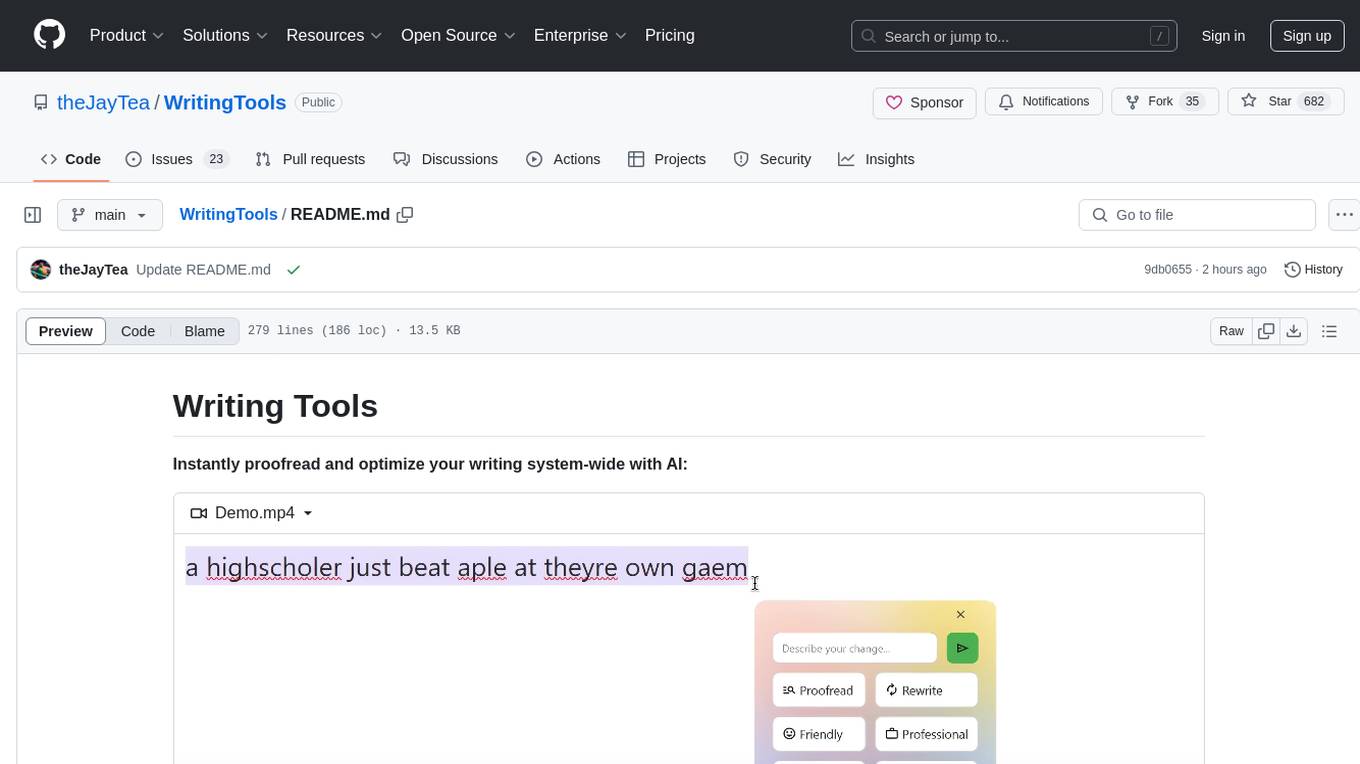
WritingTools
Writing Tools is an Apple Intelligence-inspired application for Windows, Linux, and macOS that supercharges your writing with an AI LLM. It allows users to instantly proofread, optimize text, and summarize content from webpages, YouTube videos, documents, etc. The tool is privacy-focused, open-source, and supports multiple languages. It offers powerful features like grammar correction, content summarization, and LLM chat mode, making it a versatile writing assistant for various tasks.
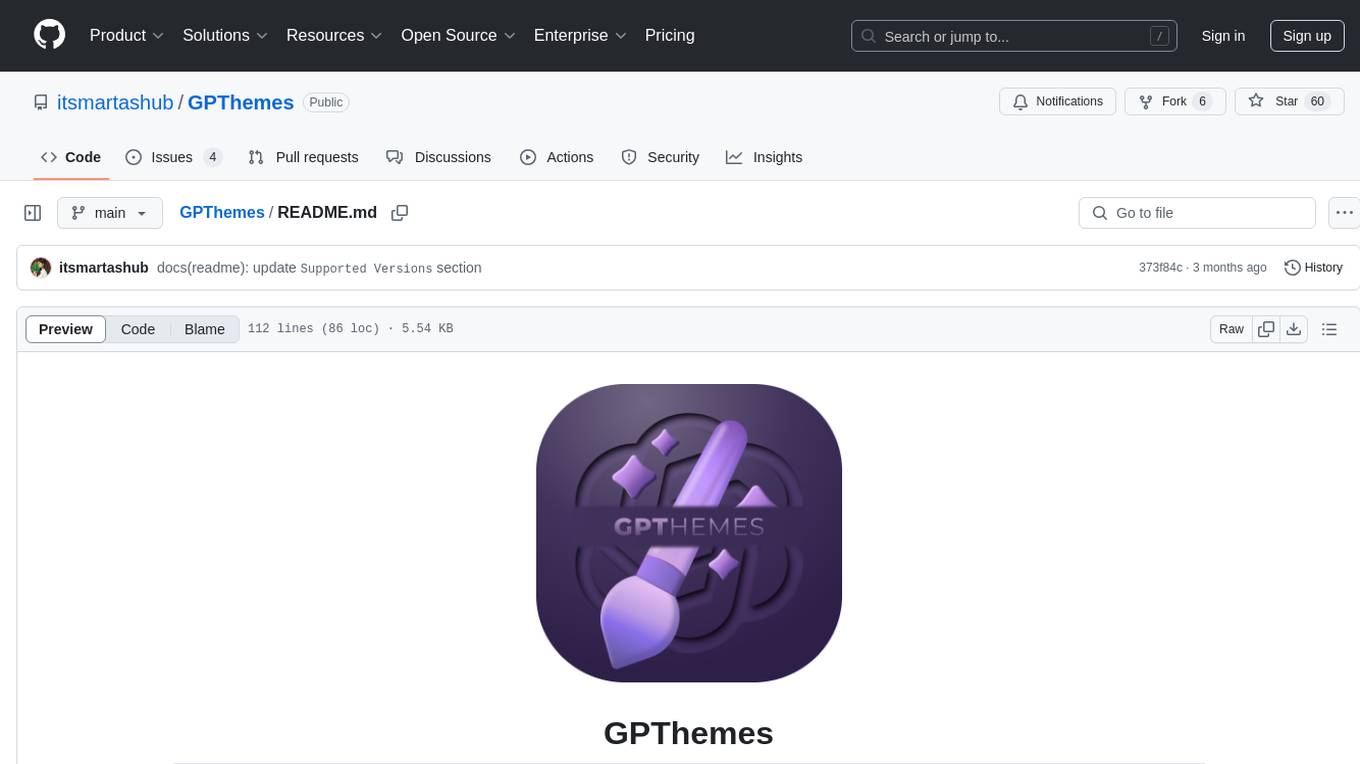
GPThemes
GPThemes is a GitHub repository that provides a collection of customizable themes for various programming languages and text editors. It offers a wide range of color schemes and styling options to enhance the visual appearance of code editors and terminals. Users can easily browse through the available themes and apply them to their preferred development environment to personalize the coding experience. With GPThemes, developers can quickly switch between different themes to find the one that best suits their preferences and workflow, making coding more enjoyable and visually appealing.
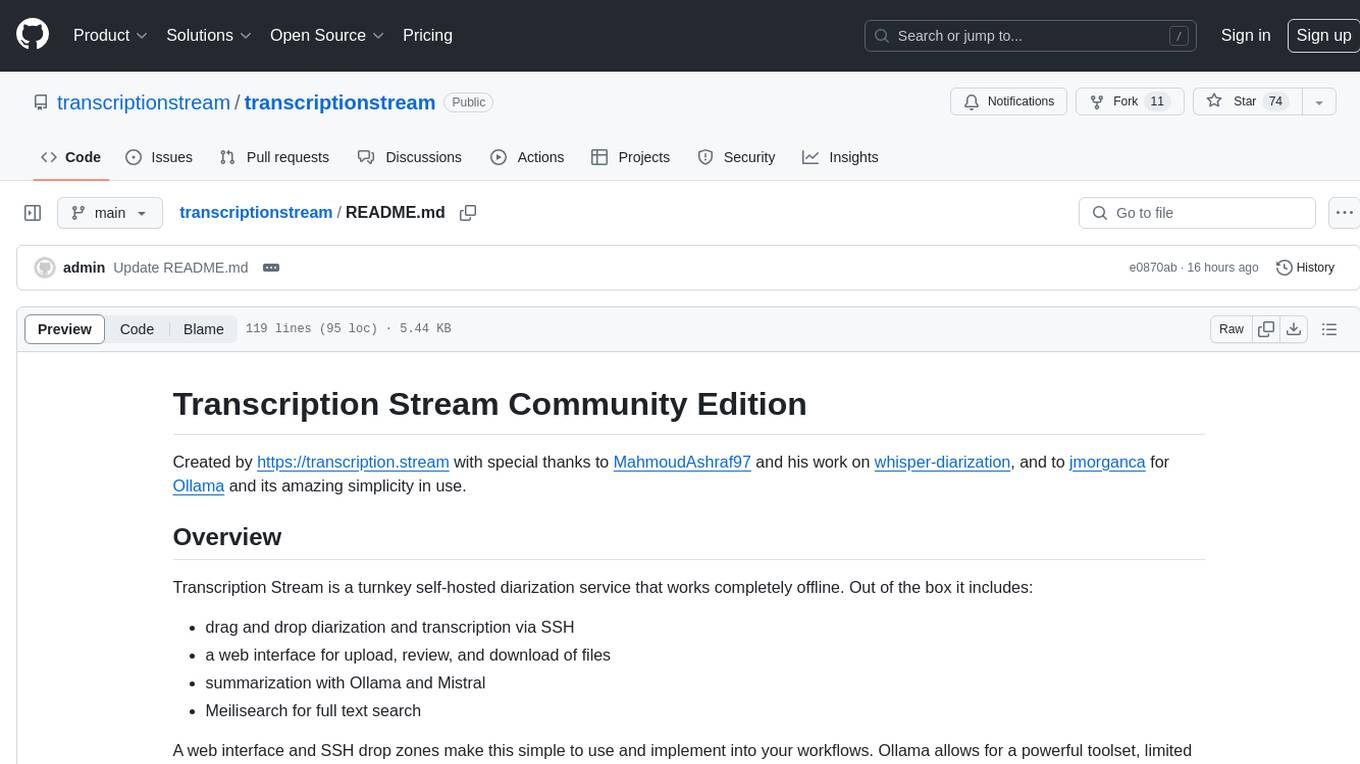
transcriptionstream
Transcription Stream is a self-hosted diarization service that works offline, allowing users to easily transcribe and summarize audio files. It includes a web interface for file management, Ollama for complex operations on transcriptions, and Meilisearch for fast full-text search. Users can upload files via SSH or web interface, with output stored in named folders. The tool requires a NVIDIA GPU and provides various scripts for installation and running. Ports for SSH, HTTP, Ollama, and Meilisearch are specified, along with access details for SSH server and web interface. Customization options and troubleshooting tips are provided in the documentation.
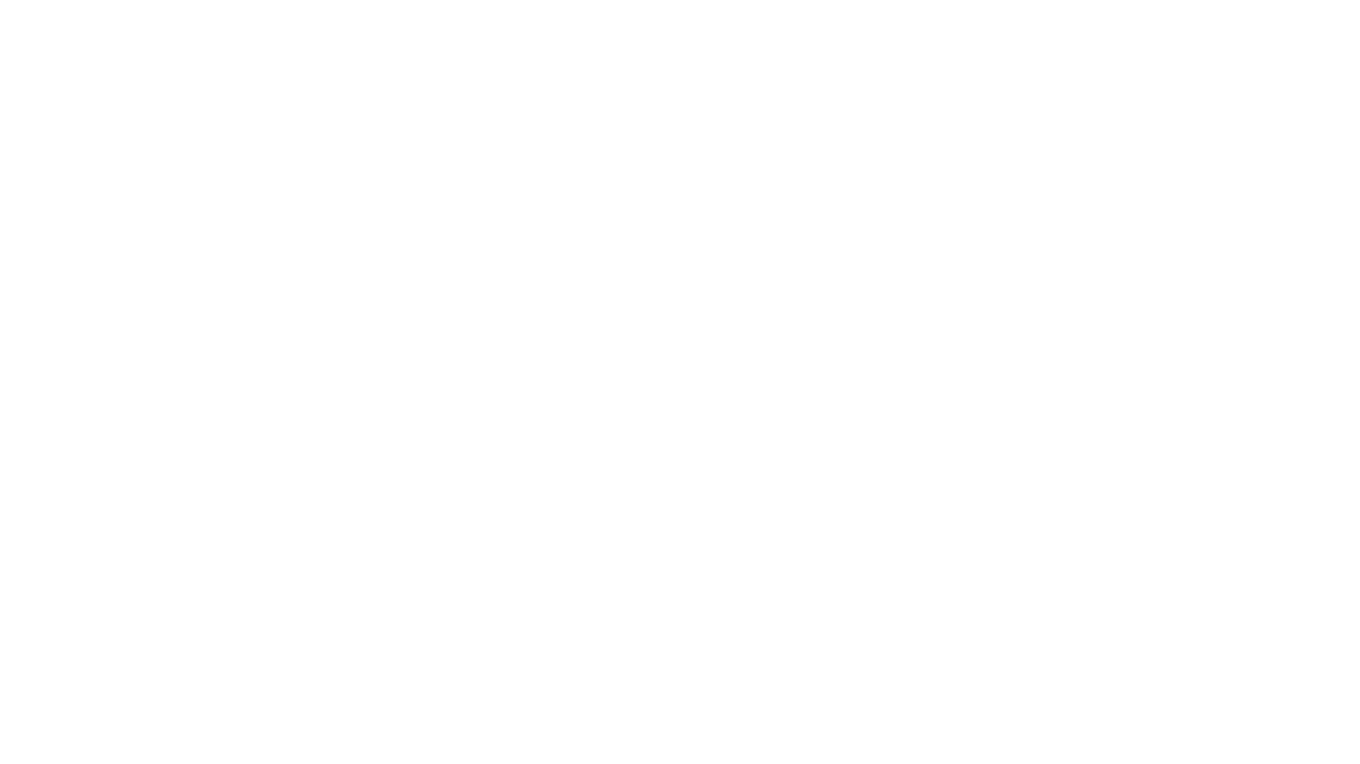
CodebaseToPrompt
CodebaseToPrompt is a simple tool that converts a local directory into a structured prompt for Large Language Models (LLMs). It allows users to select specific files for code review, analysis, or documentation by exploring and filtering through the file tree in a browser-based interface. The tool generates a formatted output that can be directly used with AI tools, provides token count estimates, and supports local storage for saving selections. Users can easily copy the selected files in the desired format for further use.
For similar tasks
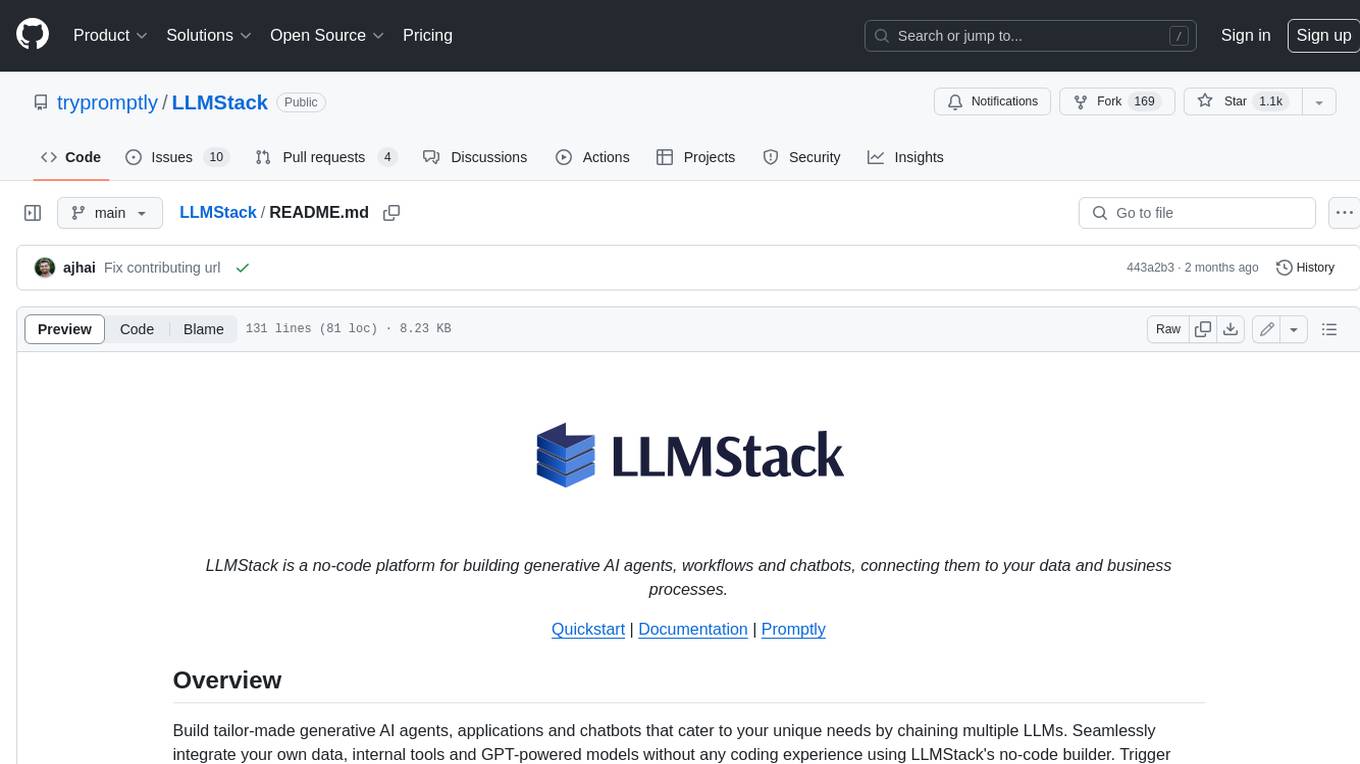
LLMStack
LLMStack is a no-code platform for building generative AI agents, workflows, and chatbots. It allows users to connect their own data, internal tools, and GPT-powered models without any coding experience. LLMStack can be deployed to the cloud or on-premise and can be accessed via HTTP API or triggered from Slack or Discord.
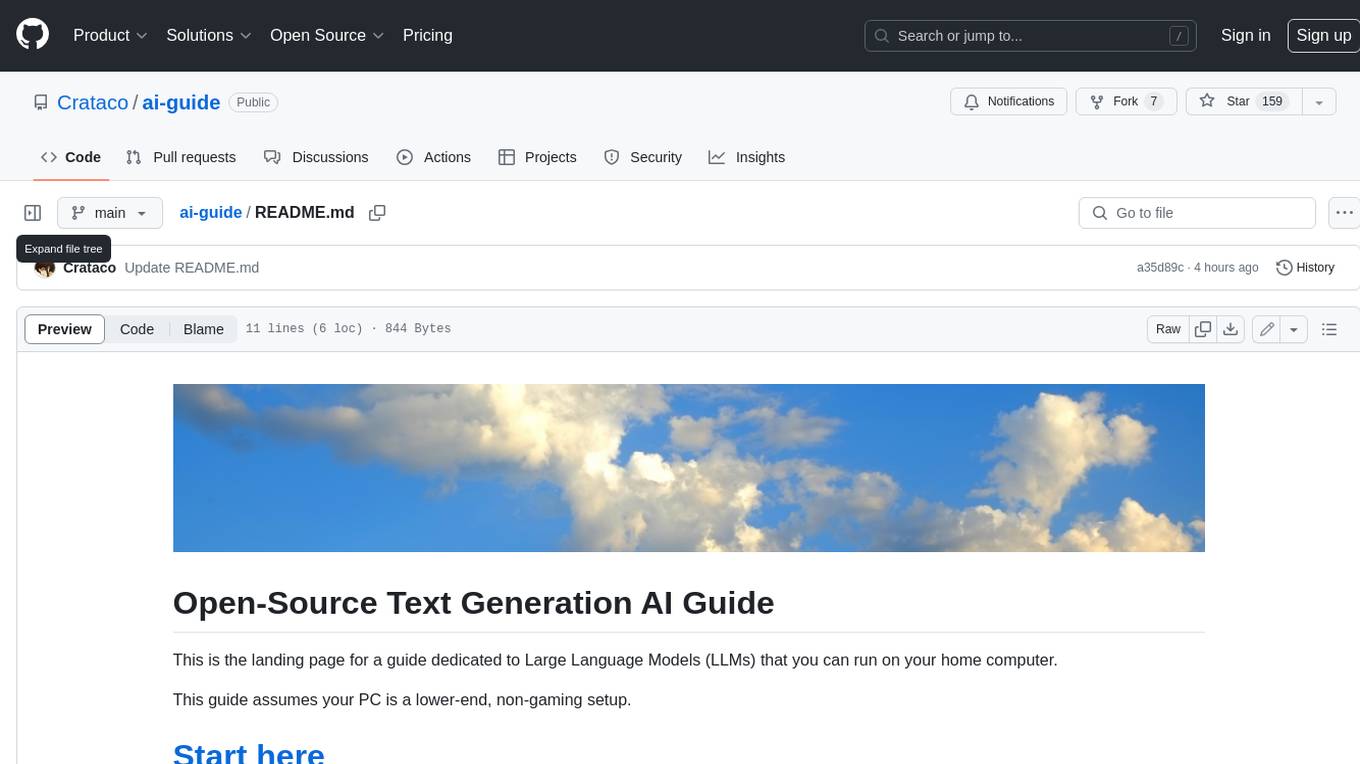
ai-guide
This guide is dedicated to Large Language Models (LLMs) that you can run on your home computer. It assumes your PC is a lower-end, non-gaming setup.
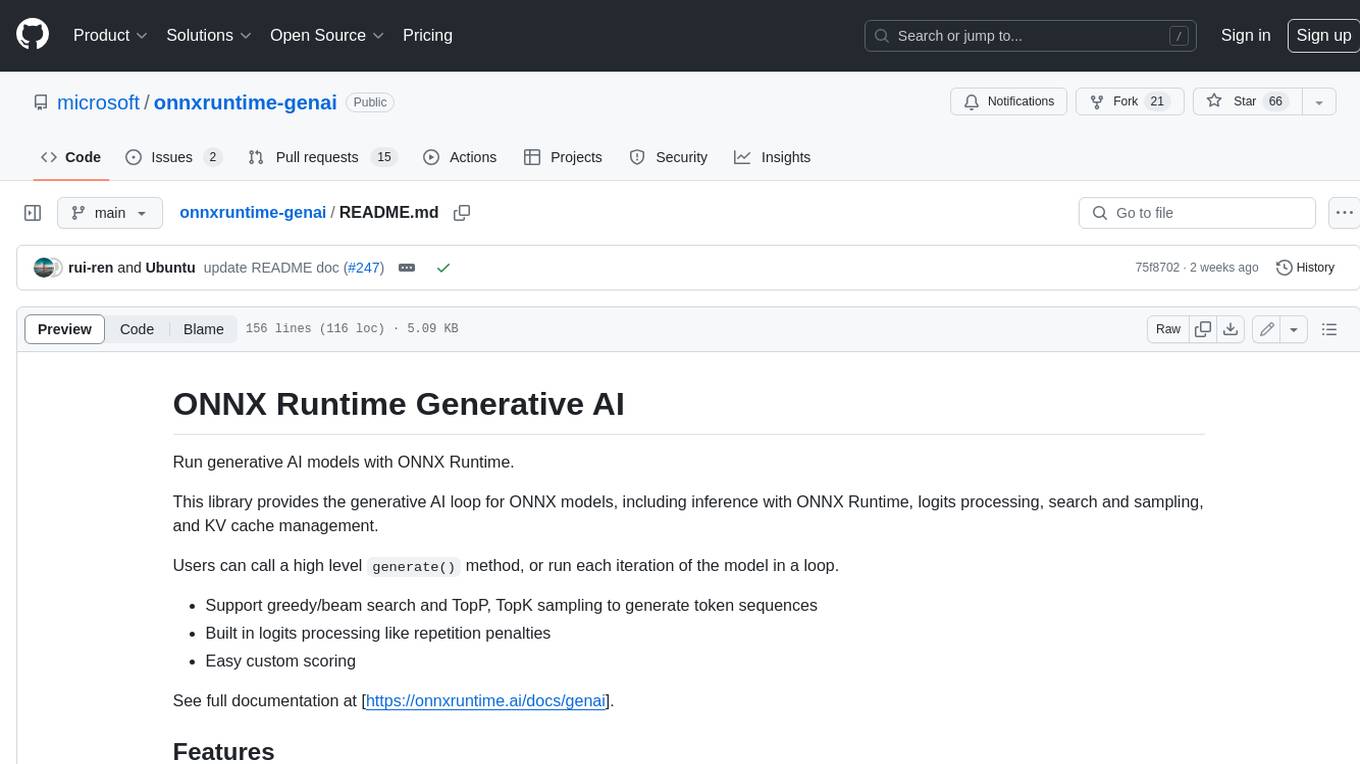
onnxruntime-genai
ONNX Runtime Generative AI is a library that provides the generative AI loop for ONNX models, including inference with ONNX Runtime, logits processing, search and sampling, and KV cache management. Users can call a high level `generate()` method, or run each iteration of the model in a loop. It supports greedy/beam search and TopP, TopK sampling to generate token sequences, has built in logits processing like repetition penalties, and allows for easy custom scoring.
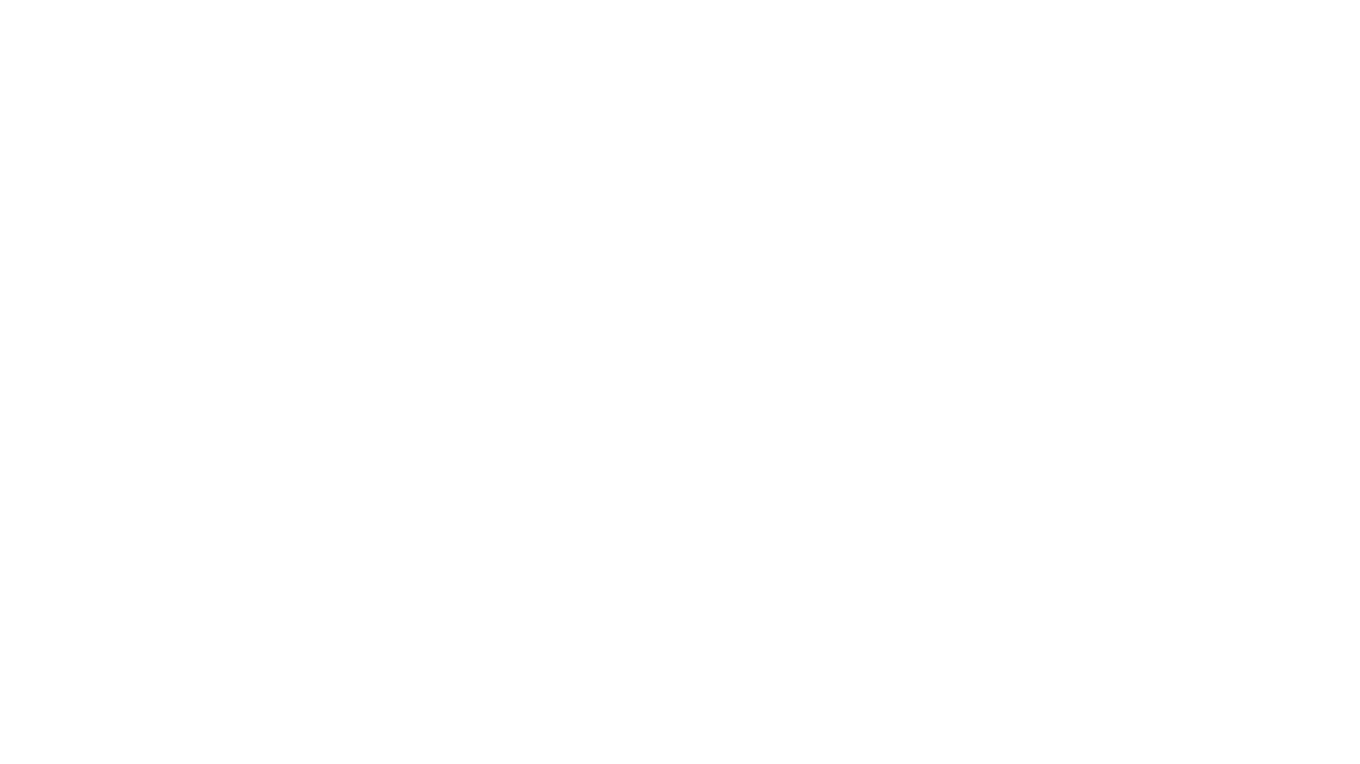
jupyter-ai
Jupyter AI connects generative AI with Jupyter notebooks. It provides a user-friendly and powerful way to explore generative AI models in notebooks and improve your productivity in JupyterLab and the Jupyter Notebook. Specifically, Jupyter AI offers: * An `%%ai` magic that turns the Jupyter notebook into a reproducible generative AI playground. This works anywhere the IPython kernel runs (JupyterLab, Jupyter Notebook, Google Colab, Kaggle, VSCode, etc.). * A native chat UI in JupyterLab that enables you to work with generative AI as a conversational assistant. * Support for a wide range of generative model providers, including AI21, Anthropic, AWS, Cohere, Gemini, Hugging Face, NVIDIA, and OpenAI. * Local model support through GPT4All, enabling use of generative AI models on consumer grade machines with ease and privacy.
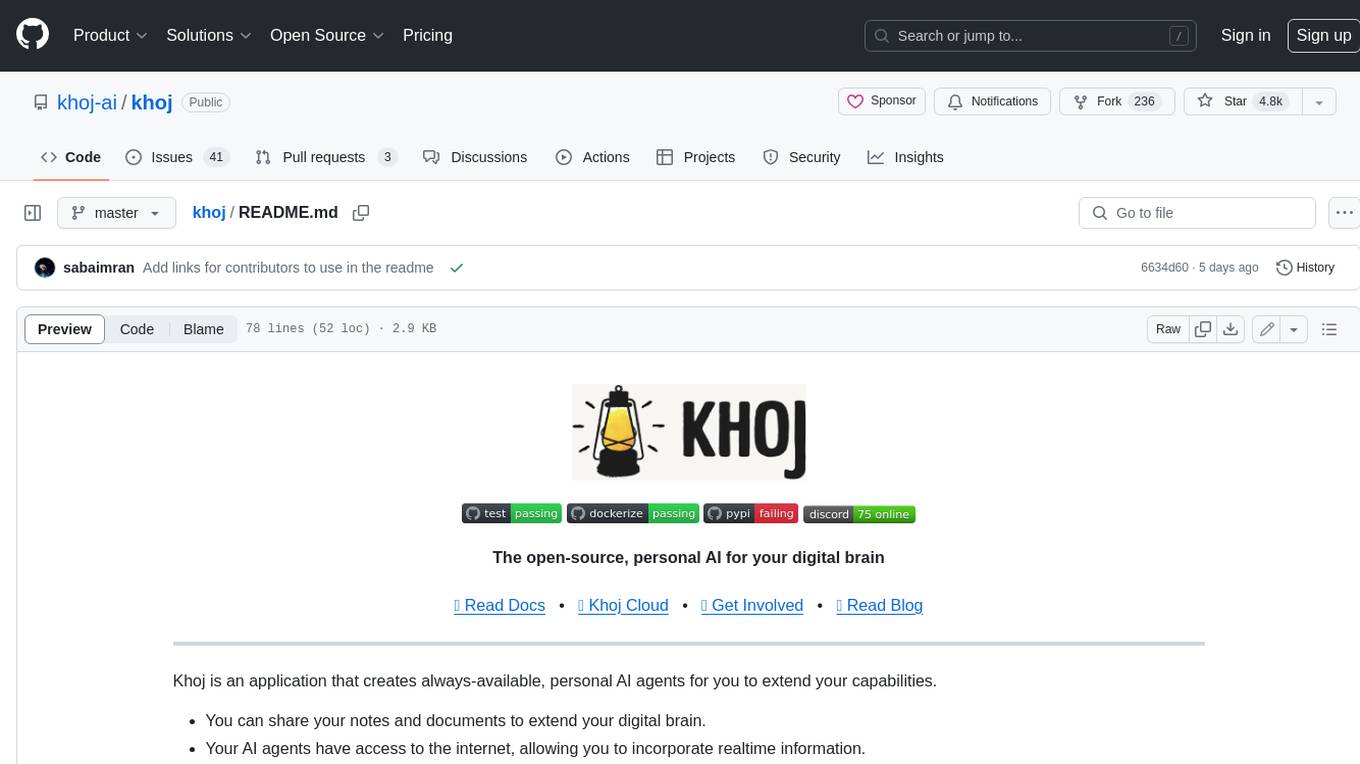
khoj
Khoj is an open-source, personal AI assistant that extends your capabilities by creating always-available AI agents. You can share your notes and documents to extend your digital brain, and your AI agents have access to the internet, allowing you to incorporate real-time information. Khoj is accessible on Desktop, Emacs, Obsidian, Web, and Whatsapp, and you can share PDF, markdown, org-mode, notion files, and GitHub repositories. You'll get fast, accurate semantic search on top of your docs, and your agents can create deeply personal images and understand your speech. Khoj is self-hostable and always will be.
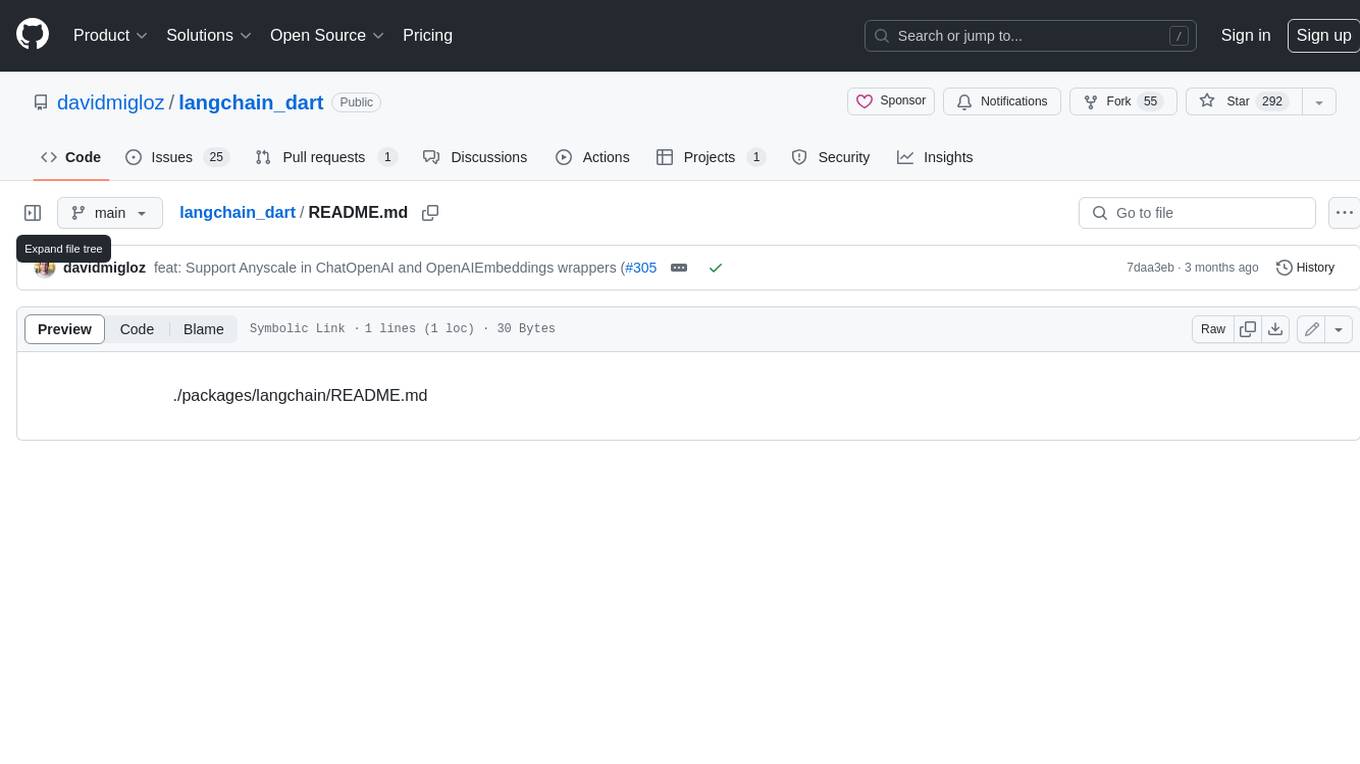
langchain_dart
LangChain.dart is a Dart port of the popular LangChain Python framework created by Harrison Chase. LangChain provides a set of ready-to-use components for working with language models and a standard interface for chaining them together to formulate more advanced use cases (e.g. chatbots, Q&A with RAG, agents, summarization, extraction, etc.). The components can be grouped into a few core modules: * **Model I/O:** LangChain offers a unified API for interacting with various LLM providers (e.g. OpenAI, Google, Mistral, Ollama, etc.), allowing developers to switch between them with ease. Additionally, it provides tools for managing model inputs (prompt templates and example selectors) and parsing the resulting model outputs (output parsers). * **Retrieval:** assists in loading user data (via document loaders), transforming it (with text splitters), extracting its meaning (using embedding models), storing (in vector stores) and retrieving it (through retrievers) so that it can be used to ground the model's responses (i.e. Retrieval-Augmented Generation or RAG). * **Agents:** "bots" that leverage LLMs to make informed decisions about which available tools (such as web search, calculators, database lookup, etc.) to use to accomplish the designated task. The different components can be composed together using the LangChain Expression Language (LCEL).
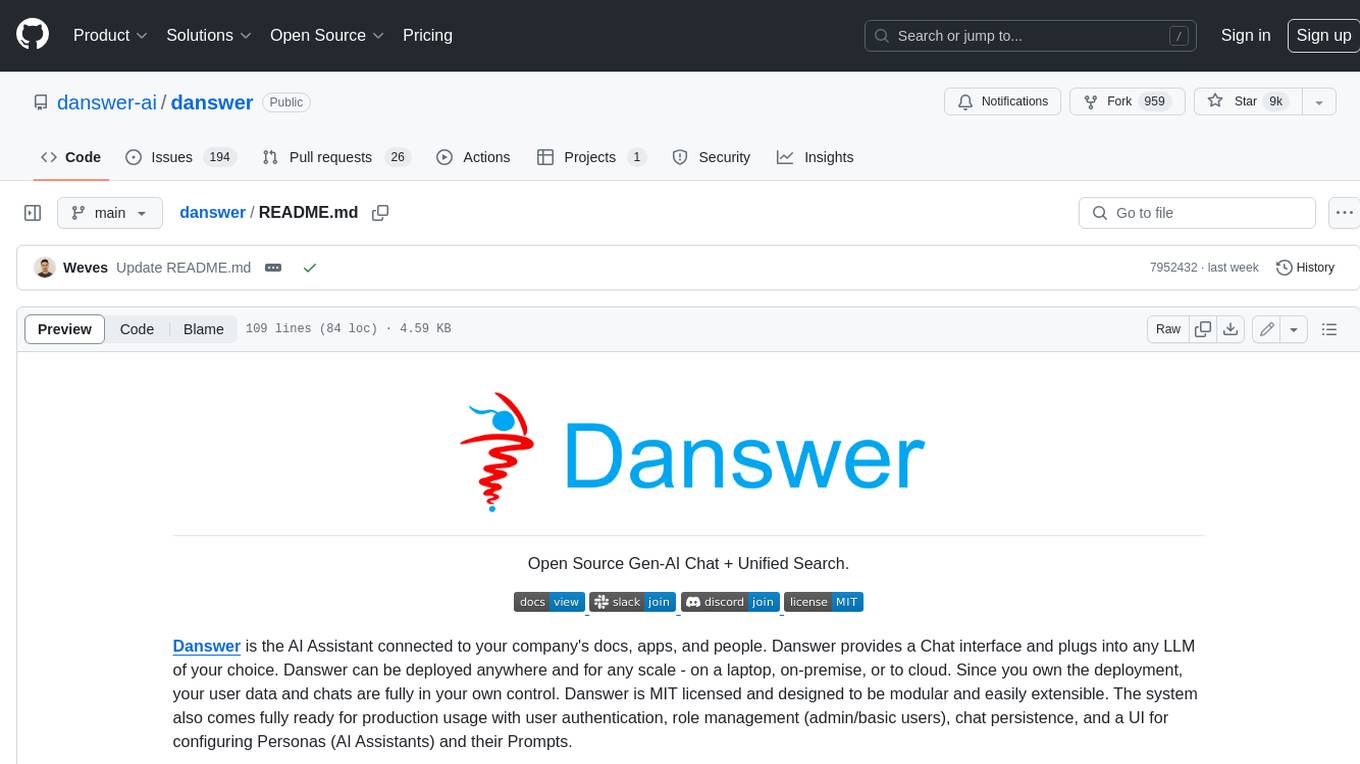
danswer
Danswer is an open-source Gen-AI Chat and Unified Search tool that connects to your company's docs, apps, and people. It provides a Chat interface and plugs into any LLM of your choice. Danswer can be deployed anywhere and for any scale - on a laptop, on-premise, or to cloud. Since you own the deployment, your user data and chats are fully in your own control. Danswer is MIT licensed and designed to be modular and easily extensible. The system also comes fully ready for production usage with user authentication, role management (admin/basic users), chat persistence, and a UI for configuring Personas (AI Assistants) and their Prompts. Danswer also serves as a Unified Search across all common workplace tools such as Slack, Google Drive, Confluence, etc. By combining LLMs and team specific knowledge, Danswer becomes a subject matter expert for the team. Imagine ChatGPT if it had access to your team's unique knowledge! It enables questions such as "A customer wants feature X, is this already supported?" or "Where's the pull request for feature Y?"
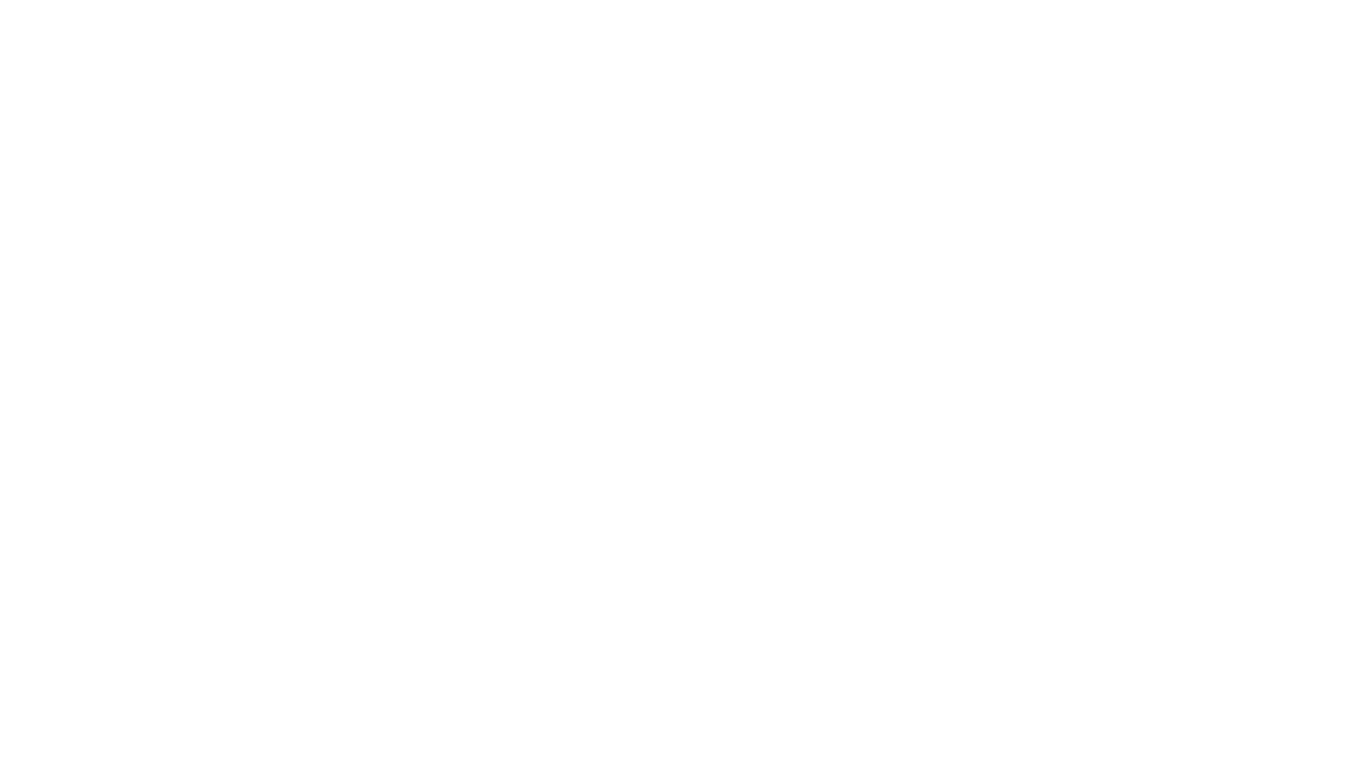
infinity
Infinity is an AI-native database designed for LLM applications, providing incredibly fast full-text and vector search capabilities. It supports a wide range of data types, including vectors, full-text, and structured data, and offers a fused search feature that combines multiple embeddings and full text. Infinity is easy to use, with an intuitive Python API and a single-binary architecture that simplifies deployment. It achieves high performance, with 0.1 milliseconds query latency on million-scale vector datasets and up to 15K QPS.
For similar jobs
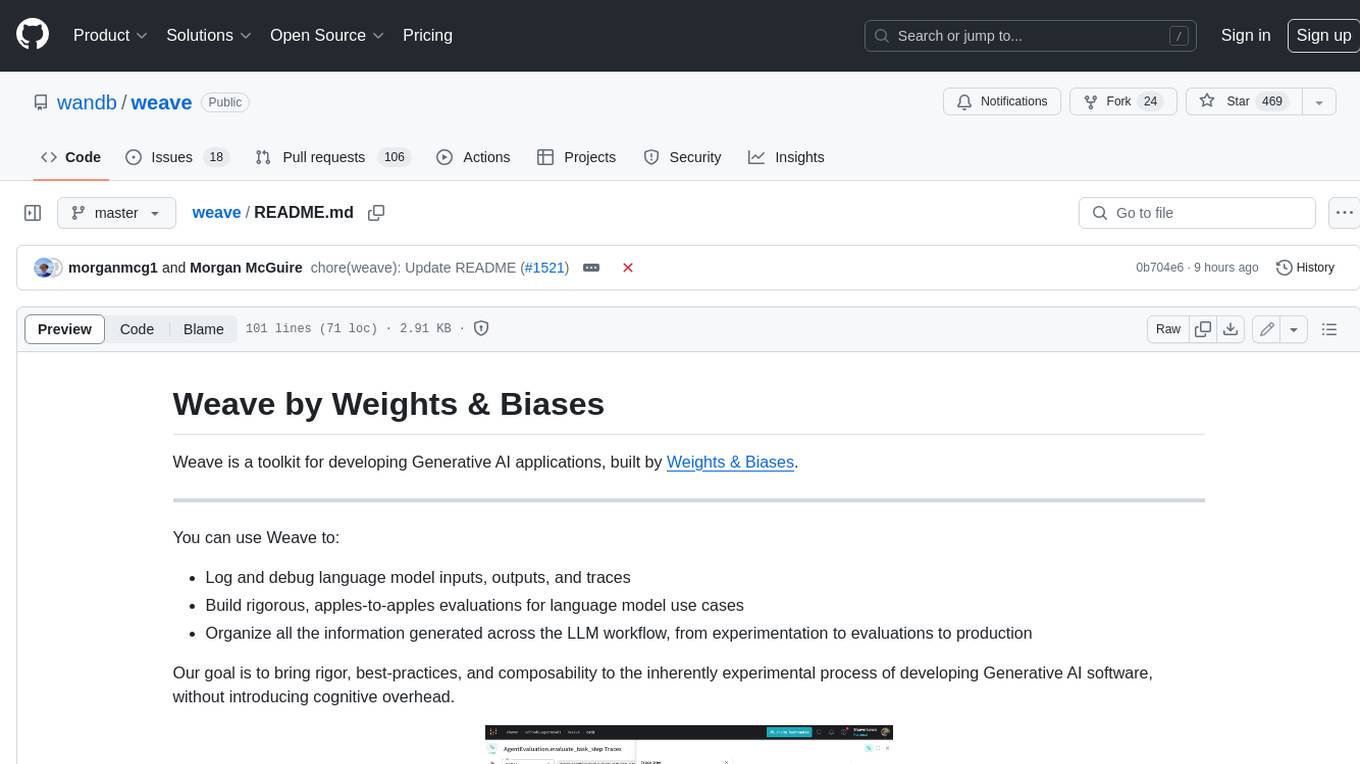
weave
Weave is a toolkit for developing Generative AI applications, built by Weights & Biases. With Weave, you can log and debug language model inputs, outputs, and traces; build rigorous, apples-to-apples evaluations for language model use cases; and organize all the information generated across the LLM workflow, from experimentation to evaluations to production. Weave aims to bring rigor, best-practices, and composability to the inherently experimental process of developing Generative AI software, without introducing cognitive overhead.
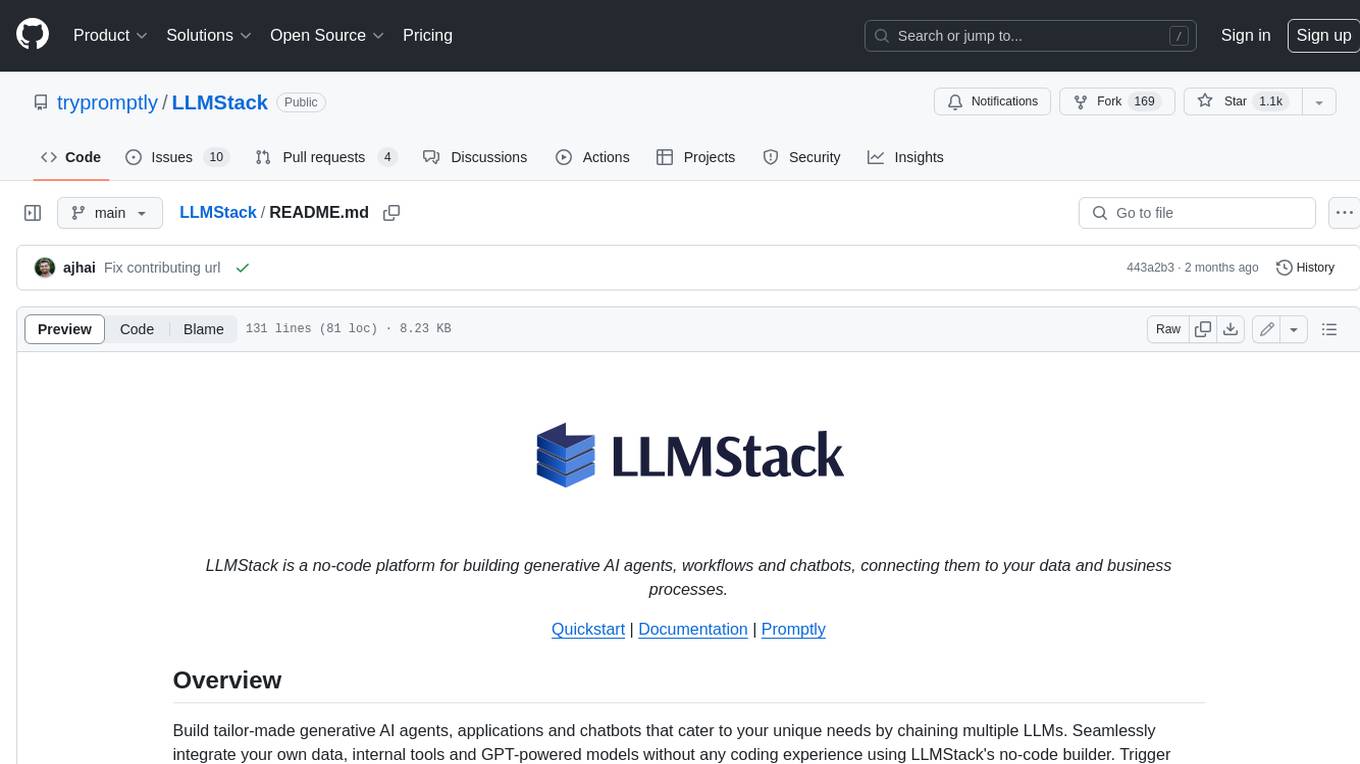
LLMStack
LLMStack is a no-code platform for building generative AI agents, workflows, and chatbots. It allows users to connect their own data, internal tools, and GPT-powered models without any coding experience. LLMStack can be deployed to the cloud or on-premise and can be accessed via HTTP API or triggered from Slack or Discord.
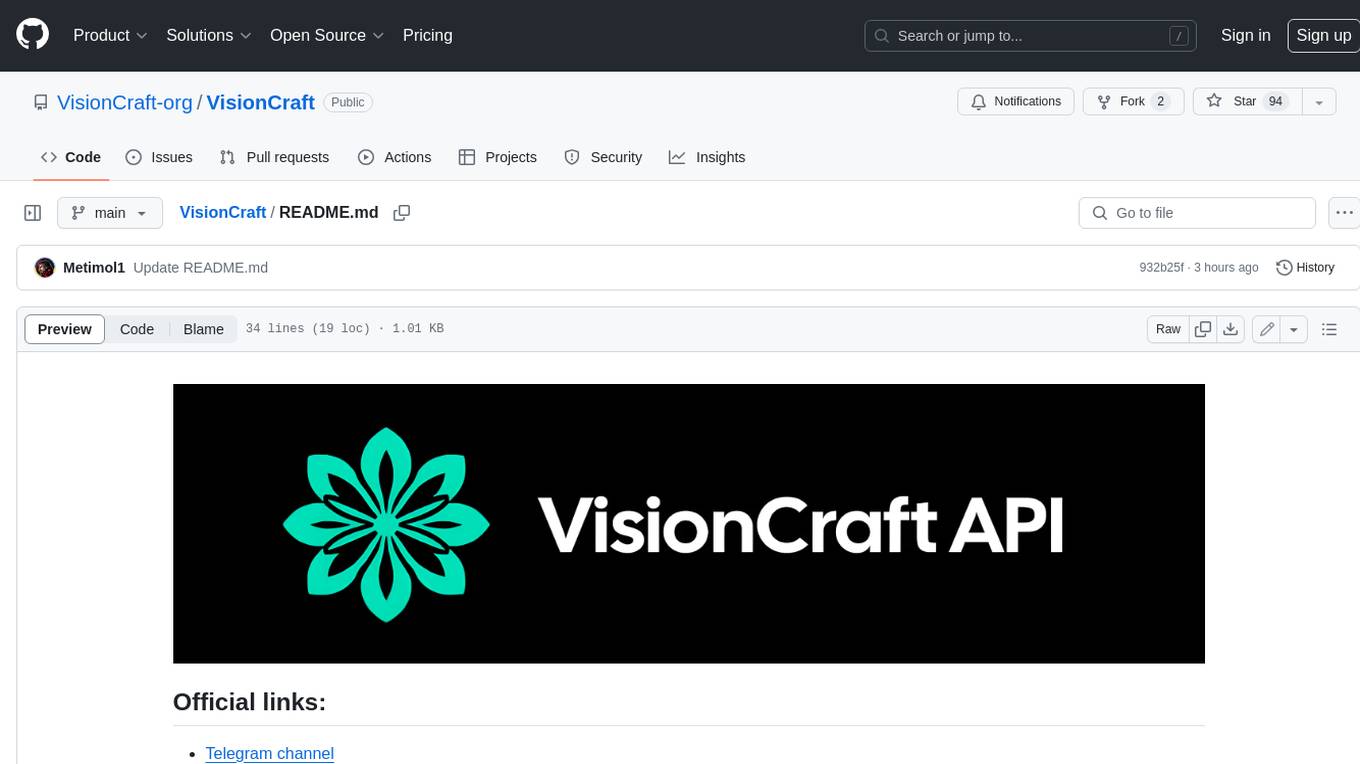
VisionCraft
The VisionCraft API is a free API for using over 100 different AI models. From images to sound.
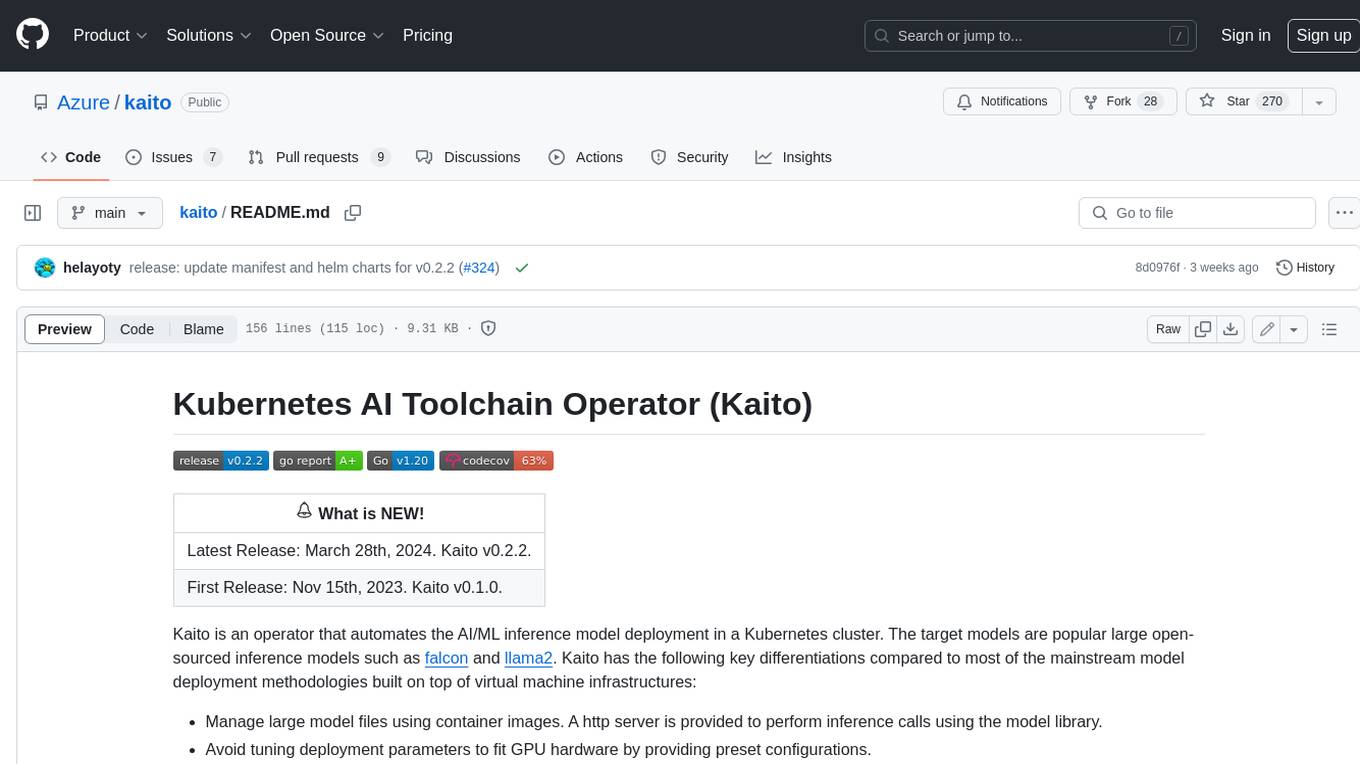
kaito
Kaito is an operator that automates the AI/ML inference model deployment in a Kubernetes cluster. It manages large model files using container images, avoids tuning deployment parameters to fit GPU hardware by providing preset configurations, auto-provisions GPU nodes based on model requirements, and hosts large model images in the public Microsoft Container Registry (MCR) if the license allows. Using Kaito, the workflow of onboarding large AI inference models in Kubernetes is largely simplified.
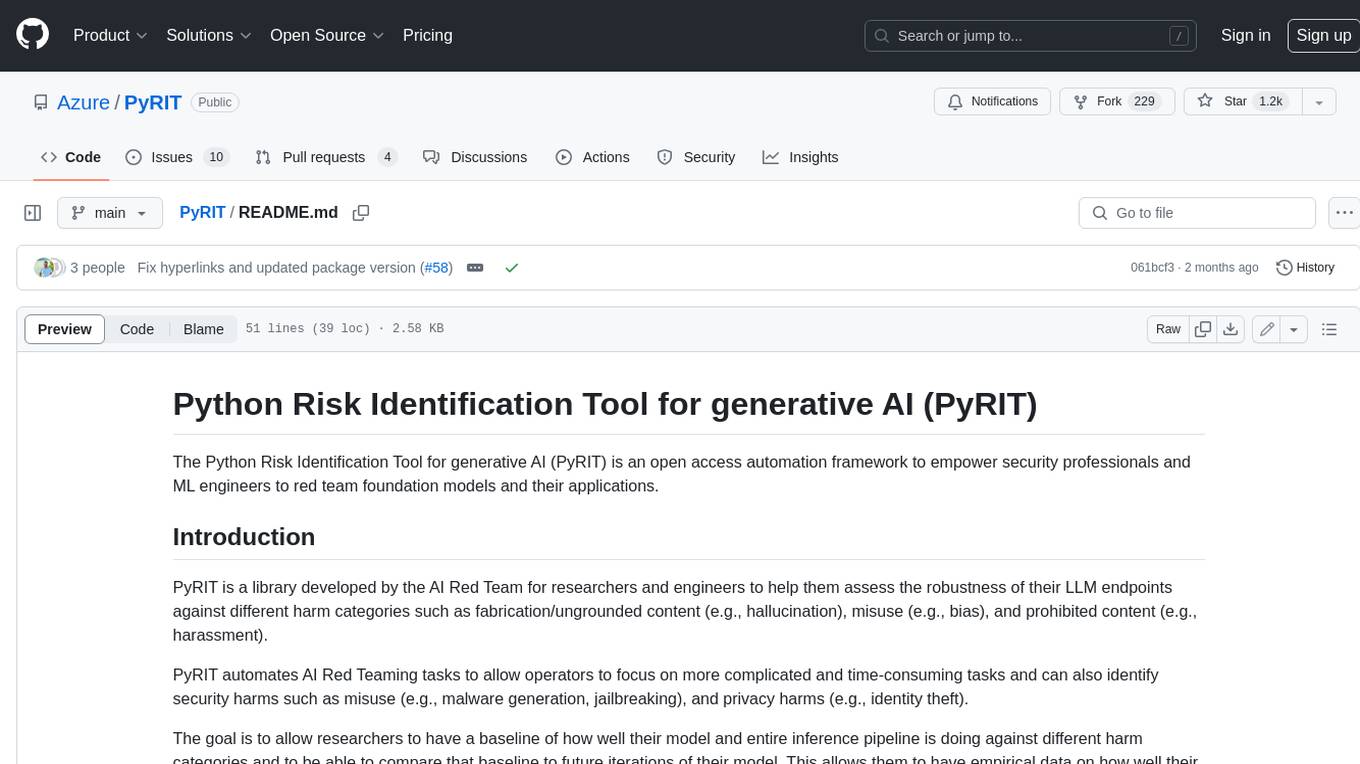
PyRIT
PyRIT is an open access automation framework designed to empower security professionals and ML engineers to red team foundation models and their applications. It automates AI Red Teaming tasks to allow operators to focus on more complicated and time-consuming tasks and can also identify security harms such as misuse (e.g., malware generation, jailbreaking), and privacy harms (e.g., identity theft). The goal is to allow researchers to have a baseline of how well their model and entire inference pipeline is doing against different harm categories and to be able to compare that baseline to future iterations of their model. This allows them to have empirical data on how well their model is doing today, and detect any degradation of performance based on future improvements.
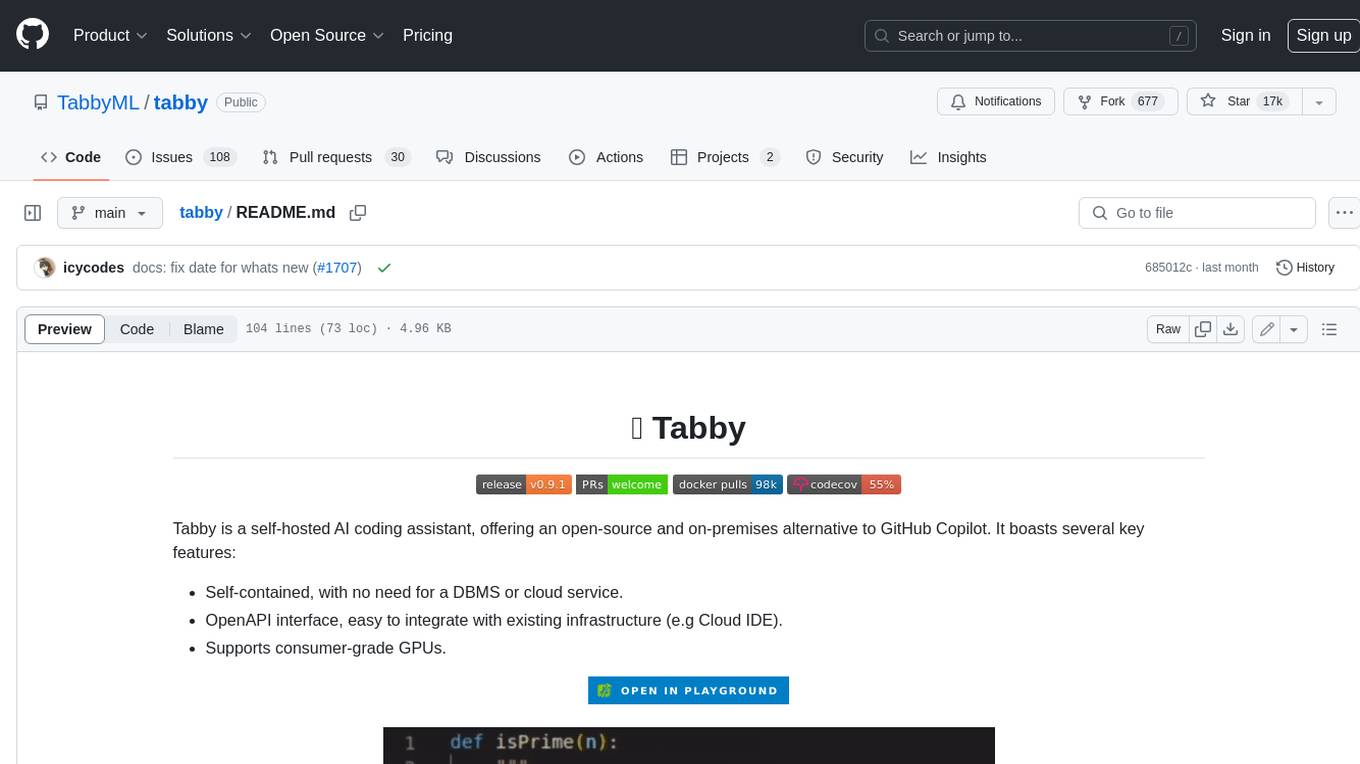
tabby
Tabby is a self-hosted AI coding assistant, offering an open-source and on-premises alternative to GitHub Copilot. It boasts several key features: * Self-contained, with no need for a DBMS or cloud service. * OpenAPI interface, easy to integrate with existing infrastructure (e.g Cloud IDE). * Supports consumer-grade GPUs.
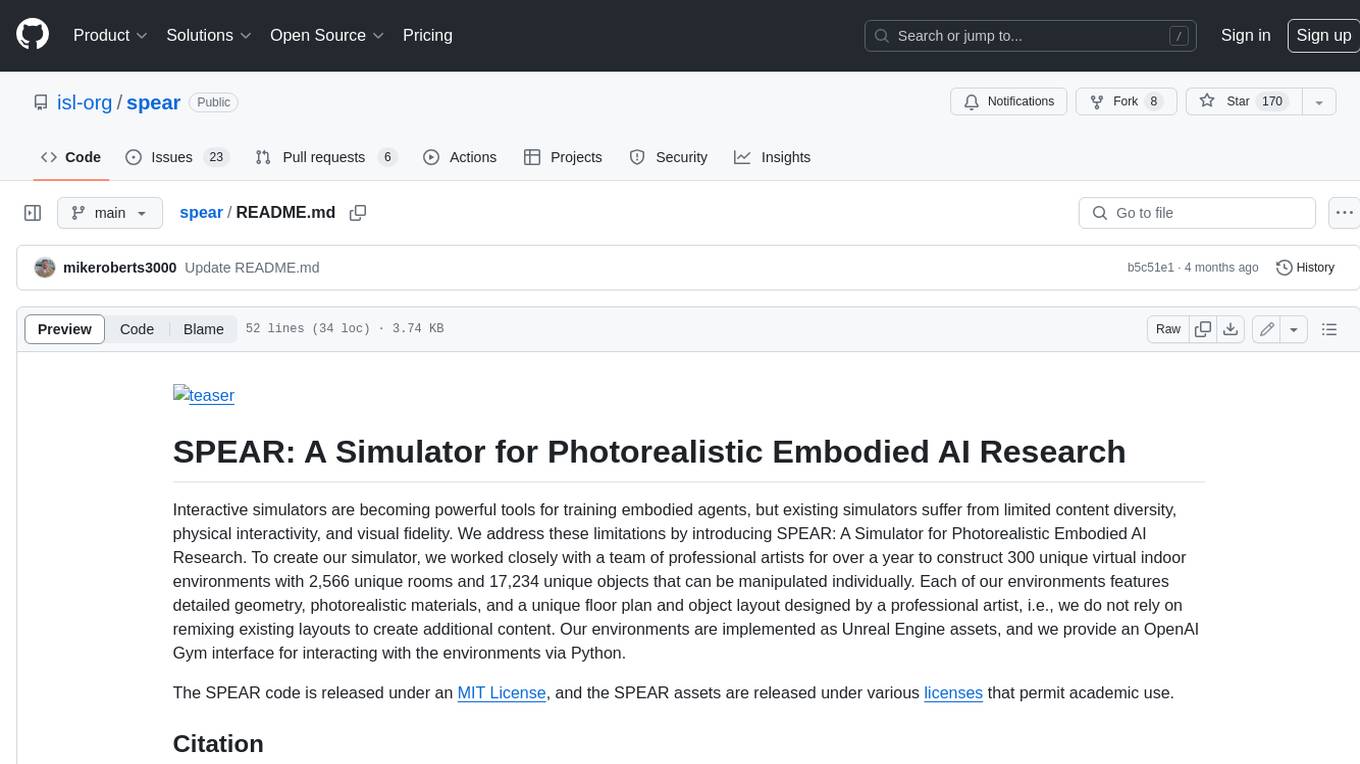
spear
SPEAR (Simulator for Photorealistic Embodied AI Research) is a powerful tool for training embodied agents. It features 300 unique virtual indoor environments with 2,566 unique rooms and 17,234 unique objects that can be manipulated individually. Each environment is designed by a professional artist and features detailed geometry, photorealistic materials, and a unique floor plan and object layout. SPEAR is implemented as Unreal Engine assets and provides an OpenAI Gym interface for interacting with the environments via Python.
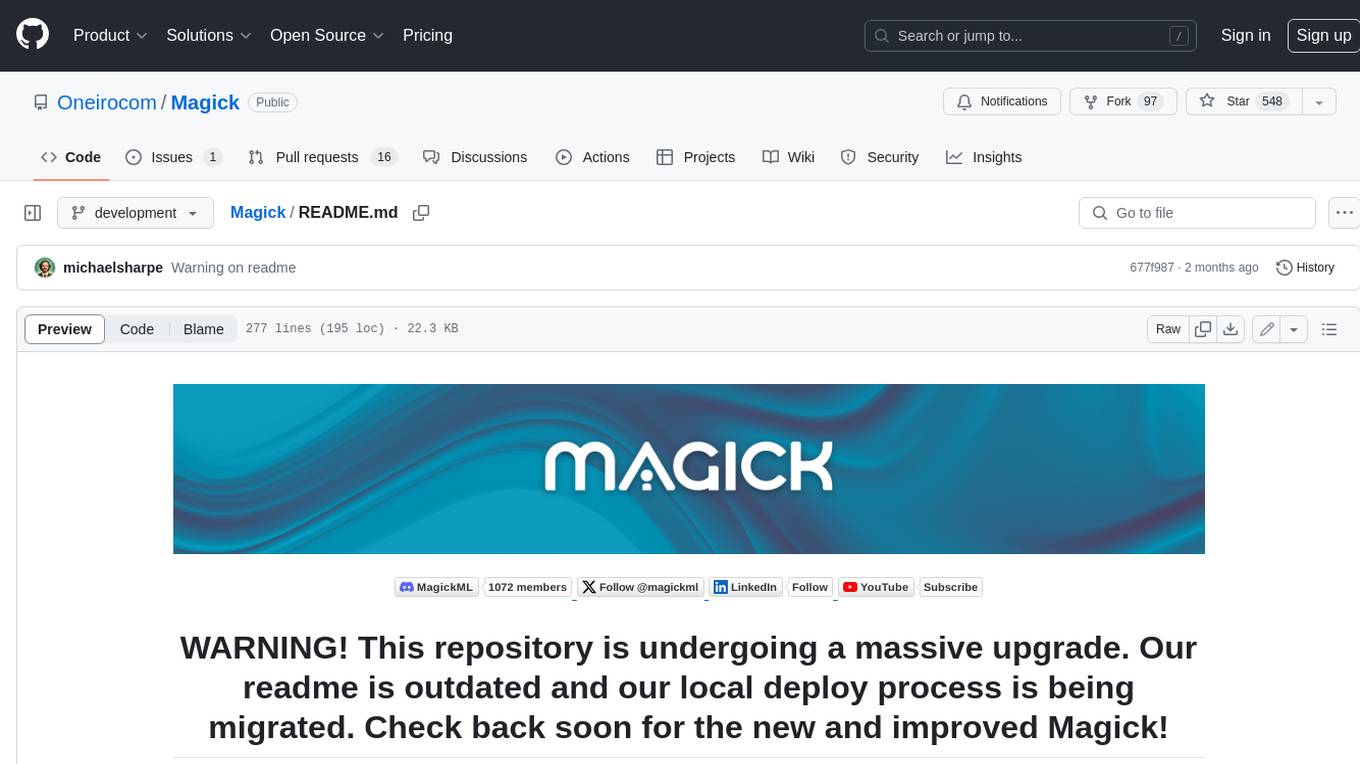
Magick
Magick is a groundbreaking visual AIDE (Artificial Intelligence Development Environment) for no-code data pipelines and multimodal agents. Magick can connect to other services and comes with nodes and templates well-suited for intelligent agents, chatbots, complex reasoning systems and realistic characters.