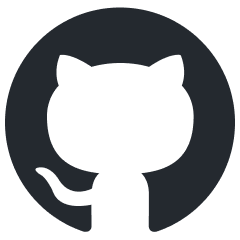
prajna
a program language for AI infrastructure
Stars: 87
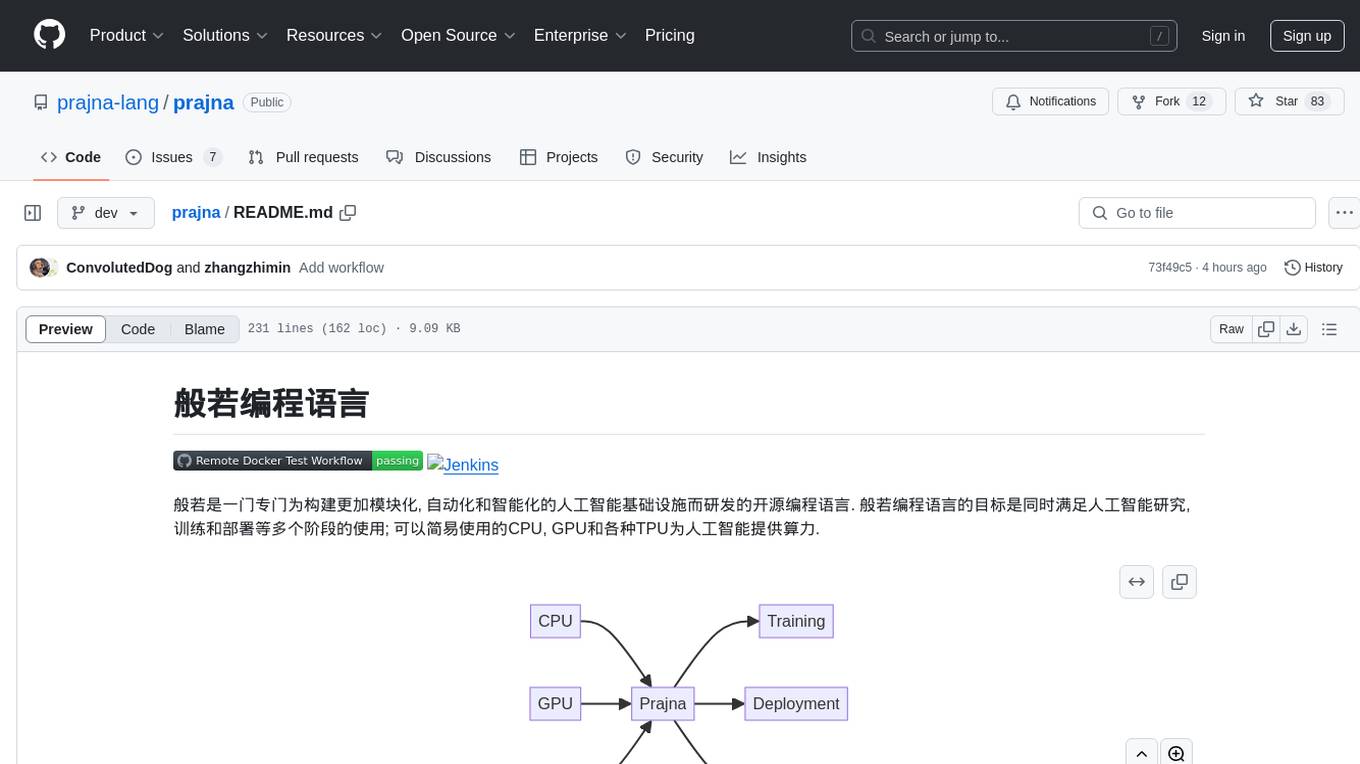
Prajna is an open-source programming language specifically developed for building more modular, automated, and intelligent artificial intelligence infrastructure. It aims to cater to various stages of AI research, training, and deployment by providing easy access to CPU, GPU, and various TPUs for AI computing. Prajna features just-in-time compilation, GPU/heterogeneous programming support, tensor computing, syntax improvements, and user-friendly interactions through main functions, Repl, and Jupyter, making it suitable for algorithm development and deployment in various scenarios.
README:
般若是一门专门为构建更加模块化, 自动化和智能化的人工智能基础设施而研发的开源编程语言. 般若编程语言的目标是同时满足人工智能研究, 训练和部署等多个阶段的使用; 可以简易使用的CPU, GPU和各种TPU为人工智能提供算力.
graph LR
CPU --> Prajna
GPU --> Prajna
TPU --> Prajna
Prajna --> Training
Prajna --> Deployment
Prajna --> Research
目前我们在建设人工智能基础设施时, 需要掌握C++, Python, CUDA和Triton等多门编程语言和技术, 这增加了软件开发的门槛和负担, 使得构建更加稳定和先进的人工智能基础设施尤为困难, 也阻碍了人工智能的进一步发展.
芯片行业研发了多种CPU, GPU, TPU和针对特定领域的加速卡, 这些处理器有着不同的硬件架构和软件生态, 进一步加剧了整个人工智能基础设施的碎片化.
对于人工智能基础设施研发效率低和碎片化严重的现状, 急需为人工智能基础设施专门设计一门编程语言, 围绕其重构我们的人工智能基础设施. 为此我们设计开发了般若编程语言.
般若采用即时编译方式,代码即程序, 无需事先编译为二进制可执行程序. 可以直接在X86, Arm和RiscV等各种指令集的芯片上直接运行. 采用LLVM作为后端, 所以会有着和C/C++一样的性能.
般若将同时提供对CPU, GPU和TPU的编程支持. 目前般若不止提供类似于CUDA的核函数编写, 还提供了gpu for等简单高效的并行编程范式, 会极大低降低异构/并行编程的复杂性. 后期会加大对各种芯片的支持力度
般若后面会集成类型于MLIR和TVM的张量优化技术, 提供高效, 并行乃至分布式计算的支持. 把张量计算相关的并行计算, 分布式计算的支持放在底层, 会非常有利于后续神经网络等框架的开发.
般若是属于类C语言, 借鉴了Rust的面向对象的设计, 移除不必要的语法特性, 例如引用等. 内存管理采用比较通用的引用计数.
般若支持main函数, Repl和Jupyter等多种交互方式, 适合算法研发和部署等多种场景.
use ::gpu::*;
use ::gpu::Tensor<f32, 2> as GpuMatrixf32;
@kernel
@target("nvptx")
func MatrixMultiply(A: GpuMatrixf32, B: GpuMatrixf32, C: GpuMatrixf32) {
var thread_x = ::gpu::ThreadIndex()[1];
var thread_y = ::gpu::ThreadIndex()[2];
var block_x = ::gpu::BlockIndex()[1];
var block_y = ::gpu::BlockIndex()[2];
var block_size = 32;
var global_x = block_x * block_size + thread_x;
var global_y = block_y * block_size + thread_y;
var sum = 0.0f32;
var step = A.Shape()[1] / block_size;
for i in 0 to step {
@shared
var local_a: Array<f32, 1024>;
@shared
var local_b: Array<f32, 1024>;
local_a[thread_x* 32 + thread_y] = A[global_x, thread_y + i * block_size];
local_b[thread_x* 32 + thread_y] = B[thread_x + i * block_size , global_y];
::gpu::BlockSynchronize();
for j in 0 to 32 {
sum = sum + local_a[thread_x * 32 + j] * local_b[j * 32 + thread_y];
}
::gpu::BlockSynchronize();
}
C[global_x, global_y] = sum;
}
@test
func Main() {
var block_size = 32;
var block_shape = [1, block_size, block_size]; // 注意和cuda的dim是相反的顺序, [z, y, x]
var a_shape = [10 * 32, 10 * 32];
var b_shape = [10 * 32, 20 * 32];
var grid_shape = [1, a_shape[0] / block_size, b_shape[1] / block_size];
var A = GpuMatrixf32::Create(a_shape);
var B = GpuMatrixf32::Create(b_shape);
var C = GpuMatrixf32::Create([a_shape[0], b_shape[1]]);
MatrixMultiply<|grid_shape, block_shape|>(A, B, C);
var epoch = 300;
var t0 = chrono::Clock();
for i in 0 to epoch {
MatrixMultiply<|grid_shape, block_shape|>(A, B, C);
}
gpu::Synchronize(); // 后面会改为更为通用的名字
var t1 = chrono::Clock();
t0.PrintLine();
t1.PrintLine();
var flops = 2 * a_shape[0] * a_shape[1] * b_shape[1];
var giga_flops = (flops.Cast<f32>() * 1.0e-9 * epoch.Cast<f32>()) / (t1 - t0);
giga_flops.Print();
"GFlop/s".PrintLine();
}
可以搜索*.prajna文件查看
下面是般若及其相关生态的路线图, 作者粗略地分为了下面的四个阶段.
timeline
title 般若生态路线图
般若编程语言: 编译器实现: GPU/异构编程: IDE
波罗蜜多运行时: 张量计算优化: 自动微分: 符号计算
框架: 数学库: 神经网络库: AutoML
应用: 视觉/语音/NPL: 自动驾驶: 多模态大模型
般若编程语言及其相关生态的建设是漫长和困难的. 般若编程语言的设计开发是整个生态的第一步, 也是最重要的一步, 目前已处于完善阶段.
下图是一个般若会涉及到的相关技术思维导图, 重构人工智能基础设施并非把现有的东西全部否定, 恰恰相反现有的相关开源项目依然占据重要位置. 般若社区扮演的更多的是设计,整合和改善的角色. 比如编译的实现, 我们只会去设计编程语言的语法, 而编译器的后端会使用LLVM项目. 而第二阶段的张量计算优化, 我们初期会直接使用MLIR/TVM等项目. 在IDE方面, 我们会增加VSCode和Jupyter的支持. 正是得益于这些开源项目, 般若生态路线图才能稳固快速的推进.
mindmap
root((Prajna))
Backend
LLVM
Nvptx
AMDGpu
Wasmtime
Paramita Runtime
Tensor Computing Optimization
Polyhedral Optimization
TVM
MLIR
Auto Diff
Symbol Compute
Framework
Nerual Networks
Mathmatics Tools
IDE
Vscode
ISP
Debug
Jupyter
PyWidgets
Notebook
因为研究,训练,部署三个阶段都可以使用Prajna, 我们不需要再把模型从Pytorch中抽离出来, 也不需要把python代码转换为C++代码去部署. 这使得我们的研发,训练和部署流程可以更快的迭代. Prajna本身改进了C++和Python的很多缺点, 也会使开发效率有所提升.
不同于Pytorch和Tensorflow在框架层去适配不同的硬件, Prajna会在编译层面去适配各种GPU和TPU. 甚至分布式计算也会由编译器自动处理, 这意味着Prajna的适配工作在编译器阶段就已经完成, 程序可以直接在CPU, GPU, TPU和集群上直接运行.
目前很多芯片厂商都在以工具链的形式去适配Pytorch, Tensorflow和Jax等框架, 这除了工作量巨大之外还不具备可维护性. 而在般若生态里, 硬件厂商只需要适配类似LLVM的后端即可, 这部分工作本身也是不可避免的.
除此之外, 还能使TPU可编程化, 这也使得我们的TPU能应用到更多场景.
般若编译器有着非常清晰的模块和层次, 人工智能不止可以生成Prajna的代码, 还可以操作Prajna的中间表示, 选择编译器优化策略等. 这些都直接提升般若生态的能力, 般若生态的提升也会进一步促进人工智能的发展.
般若生态的本质是为算力提供简单高效的使用方式, 很多行业都会因此而受益. 例如:
- 科学计算
- 有限元分析
- 办公统计软件
CUDA仅支持英伟达自己的GPU, 虽然目前般若也只支持英伟达GPU, 但Prajna后期会加入对其他GPU和TPU的支持.
本质上还是Python上的拓展, 无法避免Python本身的弊端, 也不利于自动驾驶, 物联网行业的部署.
Pytorch/Tensorflow项目代码过于庞大混乱, 基础架构和框架耦合在一起, 般若生态会着清晰的架构, 在合适的地方处理问题
可以查阅般若编程语言指南来进一步了解.
点击"launch binder"按钮来快速在线体验般若编程语言.
还可以直接下载已经安转Prajna的docker来直接体验.
docker pull matazure/prajna:0.1.0-cpu-ubuntu20.04
docker run -ti matazure/prajna:0.1.0-cpu-ubuntu20.04 prajna repl
For Tasks:
Click tags to check more tools for each tasksFor Jobs:
Alternative AI tools for prajna
Similar Open Source Tools
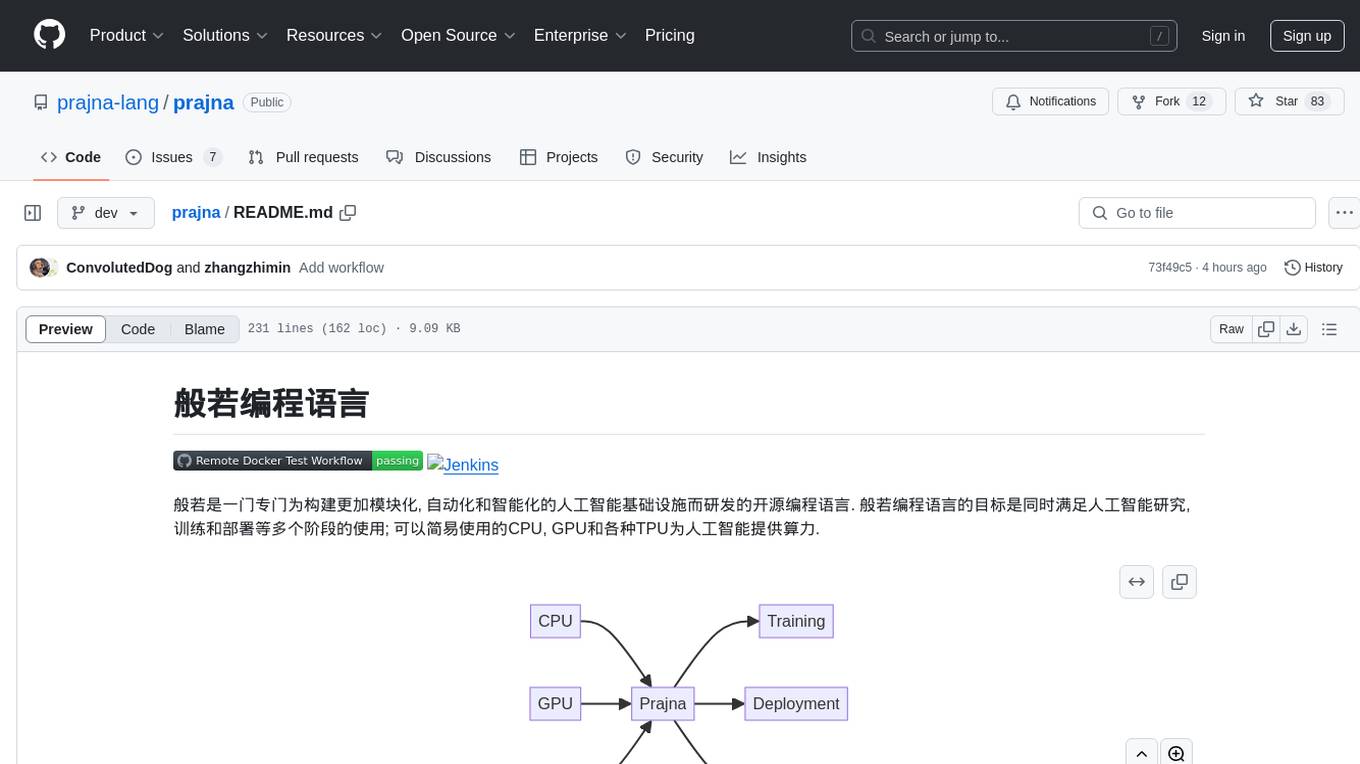
prajna
Prajna is an open-source programming language specifically developed for building more modular, automated, and intelligent artificial intelligence infrastructure. It aims to cater to various stages of AI research, training, and deployment by providing easy access to CPU, GPU, and various TPUs for AI computing. Prajna features just-in-time compilation, GPU/heterogeneous programming support, tensor computing, syntax improvements, and user-friendly interactions through main functions, Repl, and Jupyter, making it suitable for algorithm development and deployment in various scenarios.
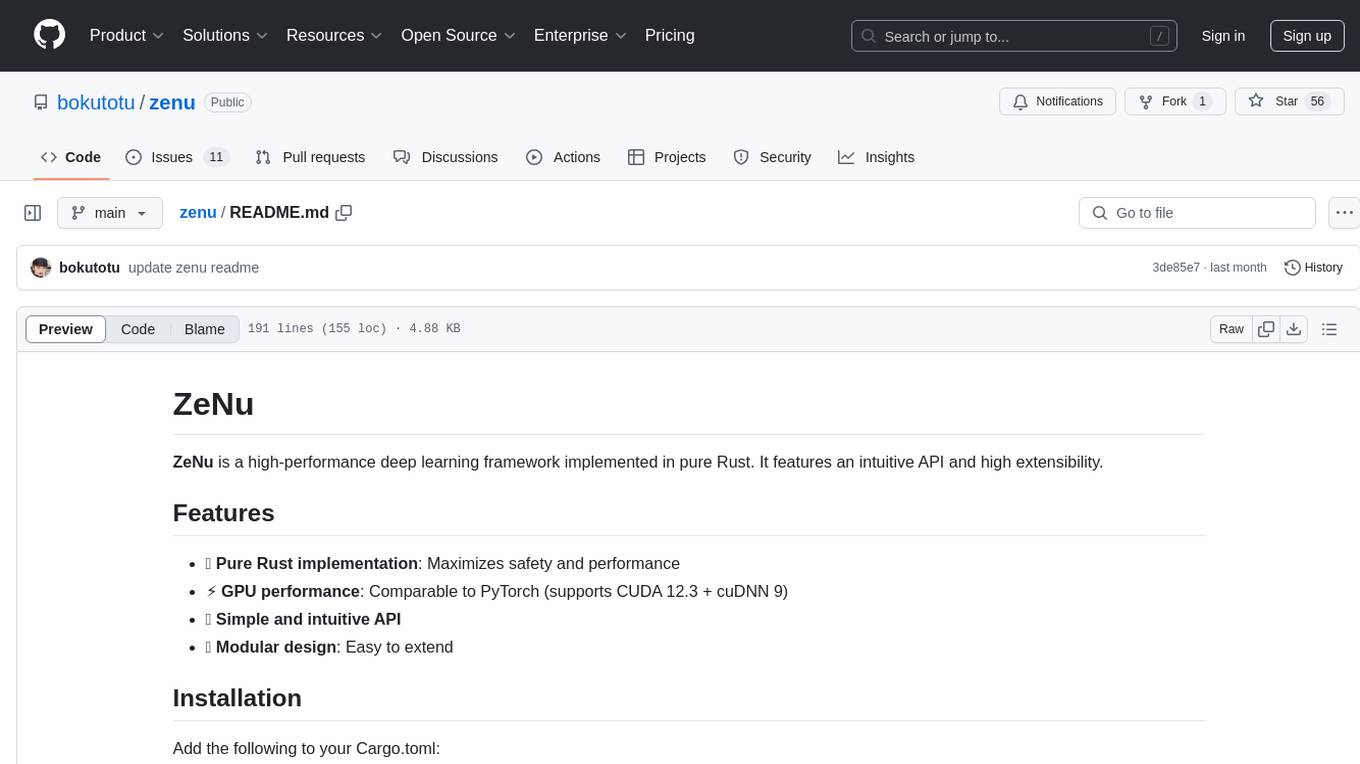
zenu
ZeNu is a high-performance deep learning framework implemented in pure Rust, featuring a pure Rust implementation for safety and performance, GPU performance comparable to PyTorch with CUDA support, a simple and intuitive API, and a modular design for easy extension. It supports various layers like Linear, Convolution 2D, LSTM, and optimizers such as SGD and Adam. ZeNu also provides device support for CPU and CUDA (NVIDIA GPU) with CUDA 12.3 and cuDNN 9. The project structure includes main library, automatic differentiation engine, neural network layers, matrix operations, optimization algorithms, CUDA implementation, and other support crates. Users can find detailed implementations like MNIST classification, CIFAR10 classification, and ResNet implementation in the examples directory. Contributions to ZeNu are welcome under the MIT License.
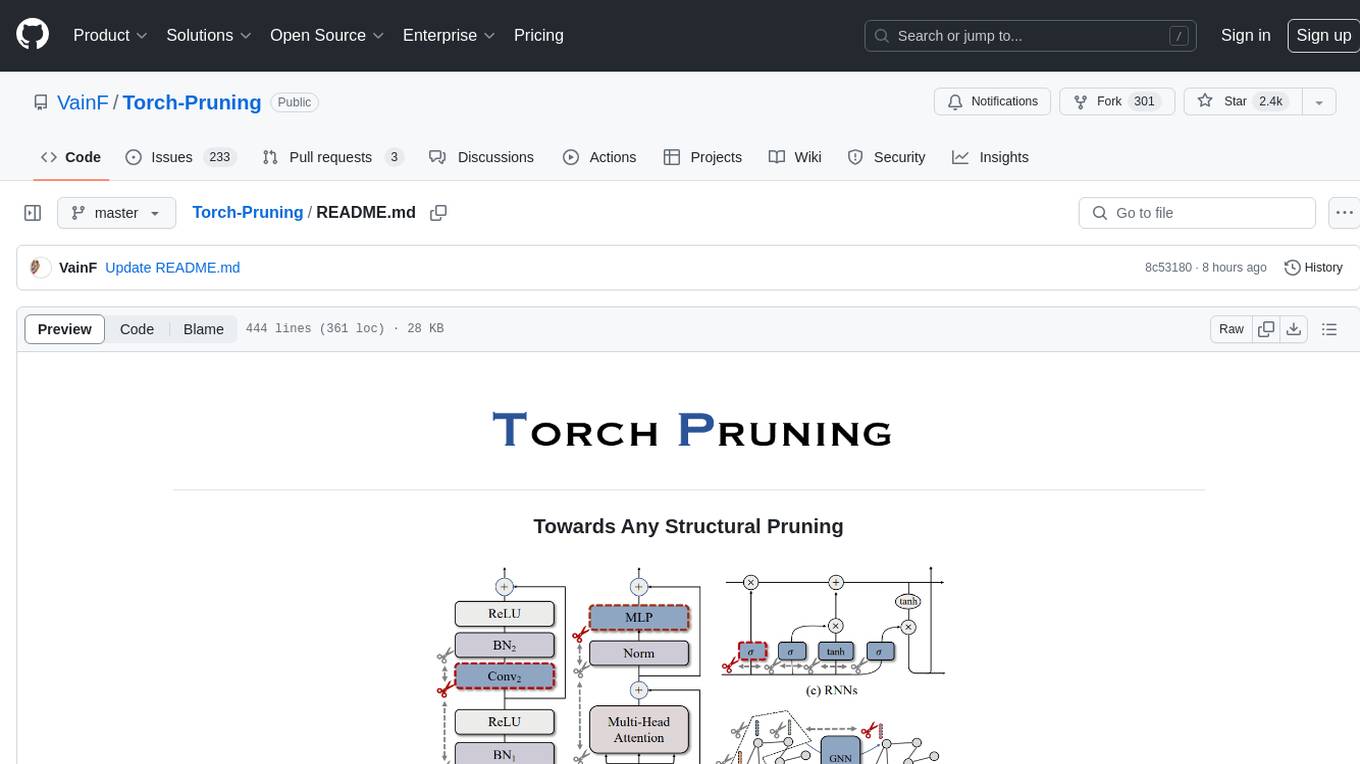
Torch-Pruning
Torch-Pruning (TP) is a library for structural pruning that enables pruning for a wide range of deep neural networks. It uses an algorithm called DepGraph to physically remove parameters. The library supports pruning off-the-shelf models from various frameworks and provides benchmarks for reproducing results. It offers high-level pruners, dependency graph for automatic pruning, low-level pruning functions, and supports various importance criteria and modules. Torch-Pruning is compatible with both PyTorch 1.x and 2.x versions.
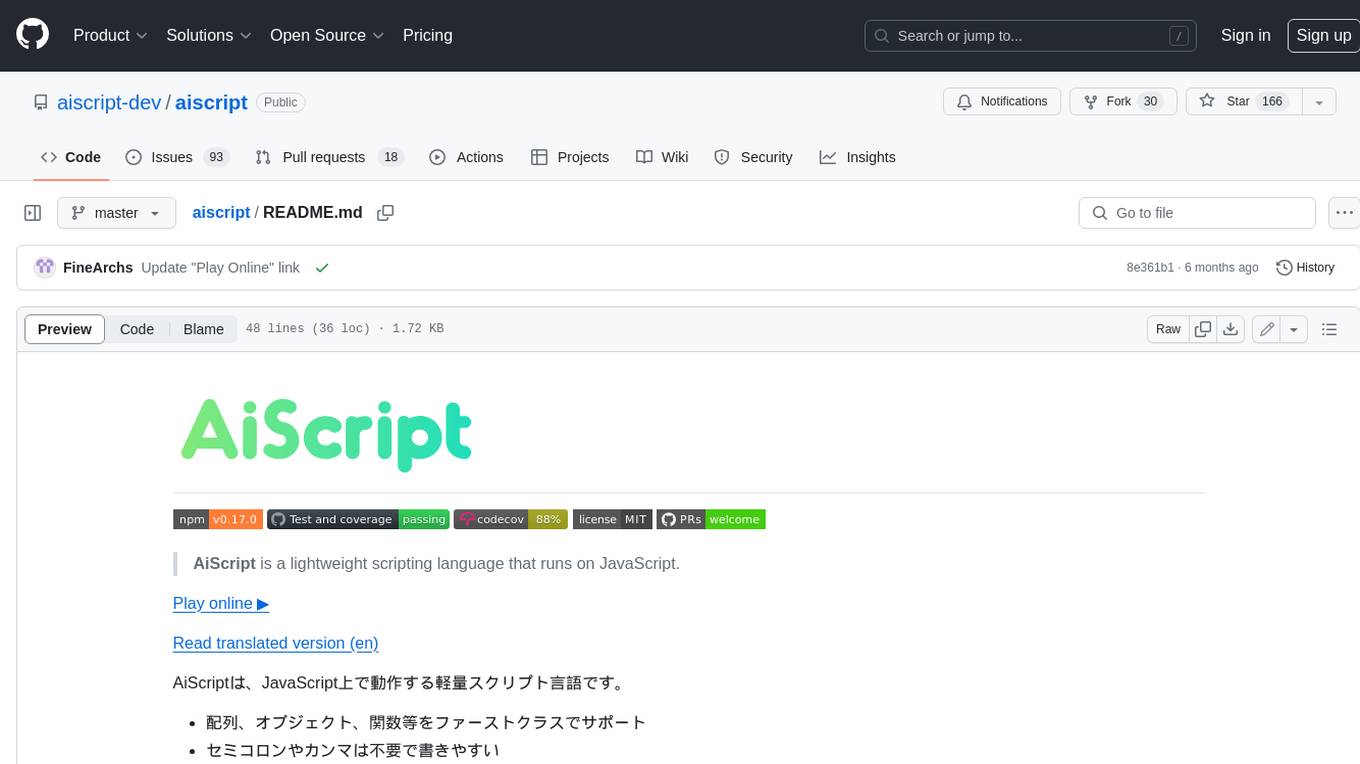
aiscript
AiScript is a lightweight scripting language that runs on JavaScript. It supports arrays, objects, and functions as first-class citizens, and is easy to write without the need for semicolons or commas. AiScript runs in a secure sandbox environment, preventing infinite loops from freezing the host. It also allows for easy provision of variables and functions from the host.
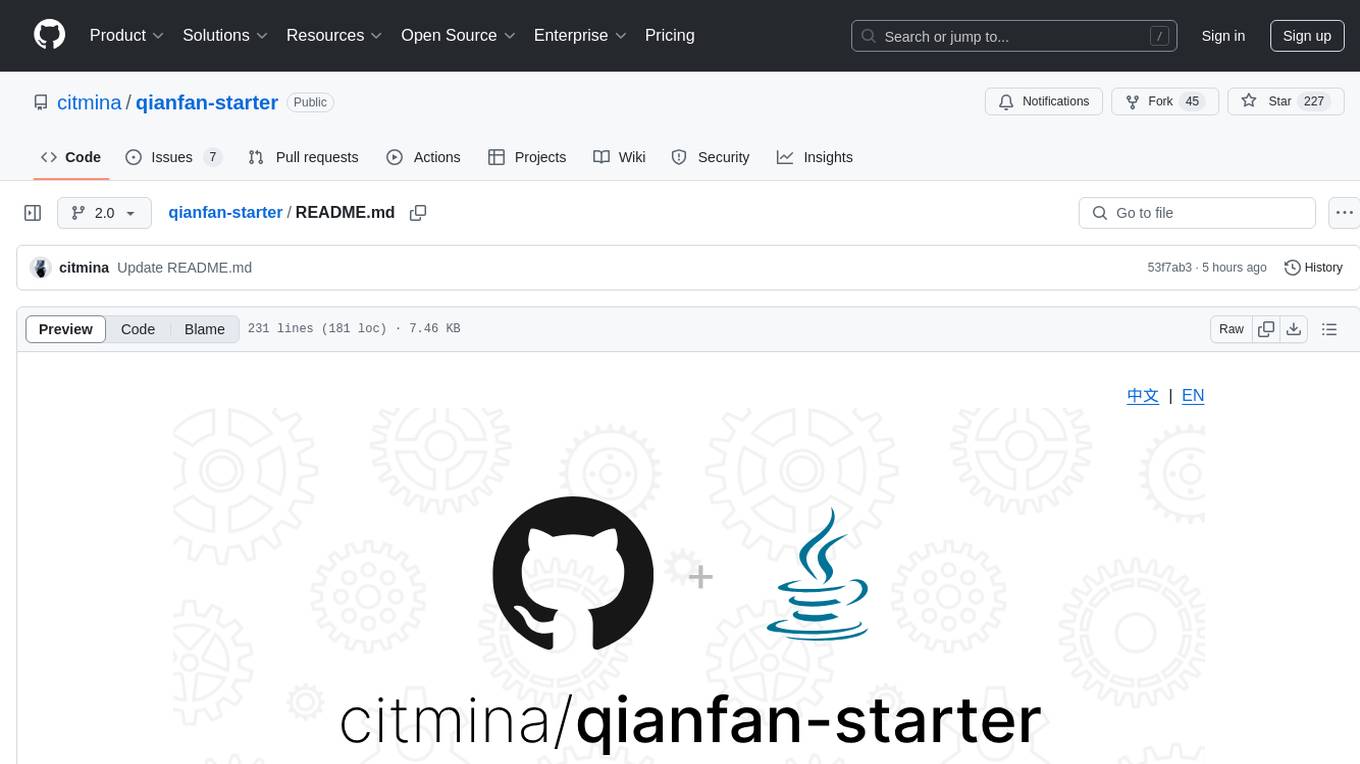
qianfan-starter
WenXin-Starter is a spring-boot-starter for Baidu's 'WenXin Workshop' large model, facilitating quick integration of Baidu's AI capabilities. It provides complete integration with WenXin Workshop's official API documentation, supports WenShengTu, built-in conversation memory, and supports conversation streaming. It also supports QPS control for individual models and queuing mechanism, with upcoming plugin support.
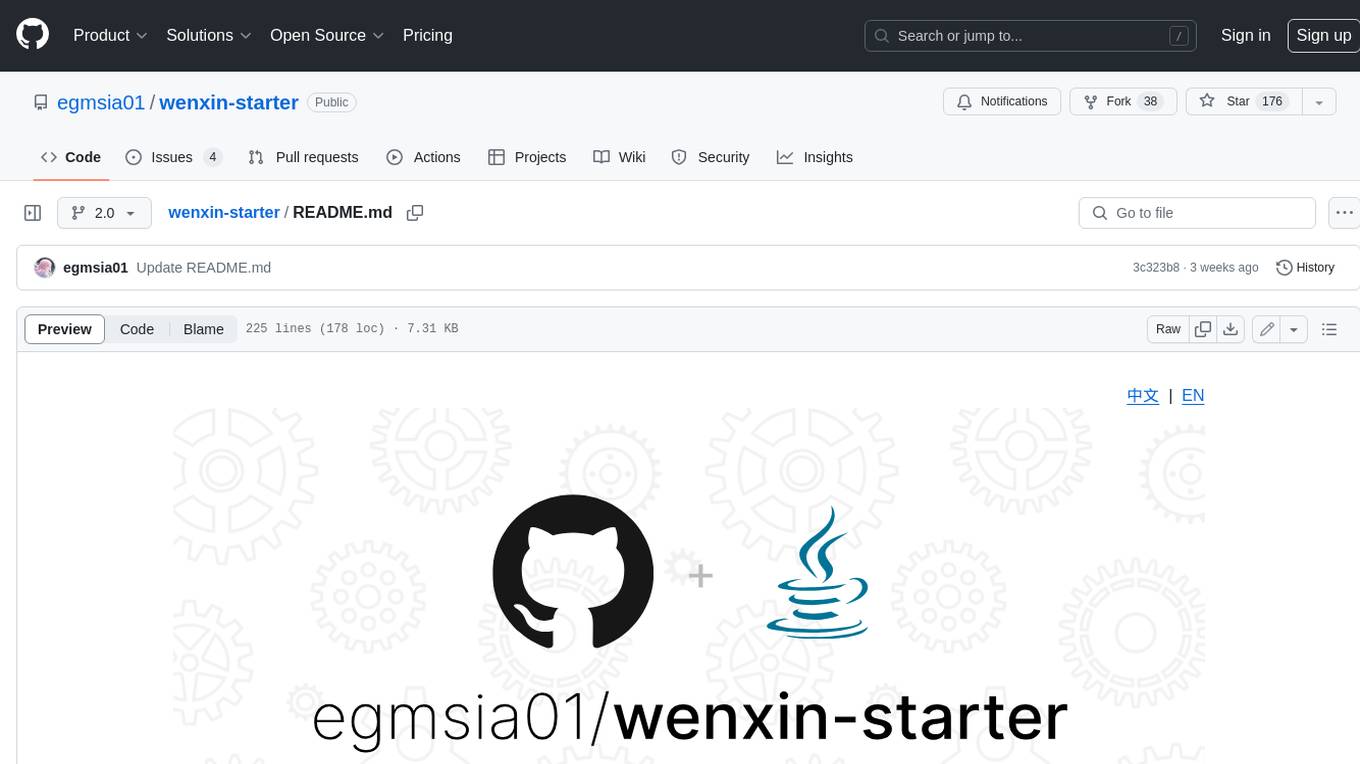
wenxin-starter
WenXin-Starter is a spring-boot-starter for Baidu's "Wenxin Qianfan WENXINWORKSHOP" large model, which can help you quickly access Baidu's AI capabilities. It fully integrates the official API documentation of Wenxin Qianfan. Supports text-to-image generation, built-in dialogue memory, and supports streaming return of dialogue. Supports QPS control of a single model and supports queuing mechanism. Plugins will be added soon.
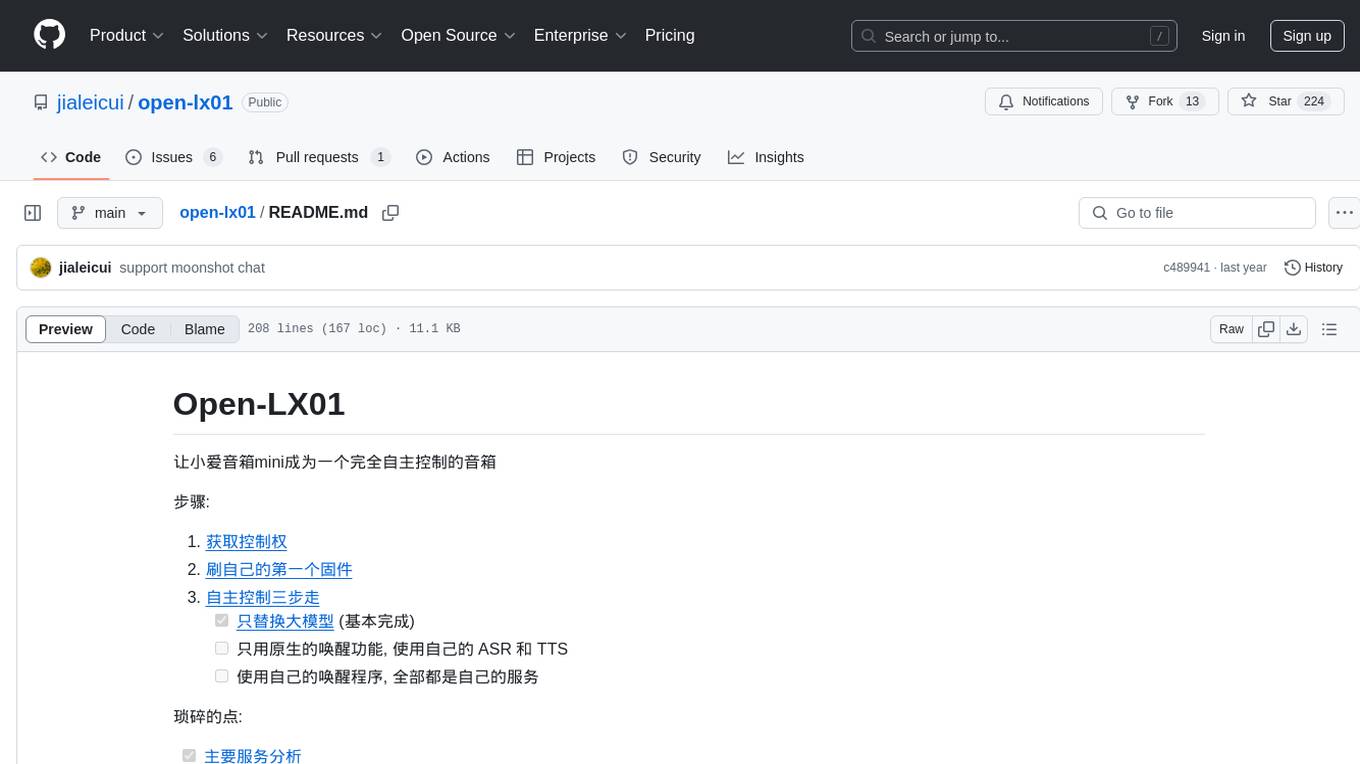
open-lx01
Open-LX01 is a project aimed at turning the Xiao Ai Mini smart speaker into a fully self-controlled device. The project involves steps such as gaining control, flashing custom firmware, and achieving autonomous control. It includes analysis of main services, reverse engineering methods, cross-compilation environment setup, customization of programs on the speaker, and setting up a web server. The project also covers topics like using custom ASR and TTS, developing a wake-up program, and creating a UI for various configurations. Additionally, it explores topics like gdb-server setup, open-mico-aivs-lab, and open-mipns-sai integration using Porcupine or Kaldi.
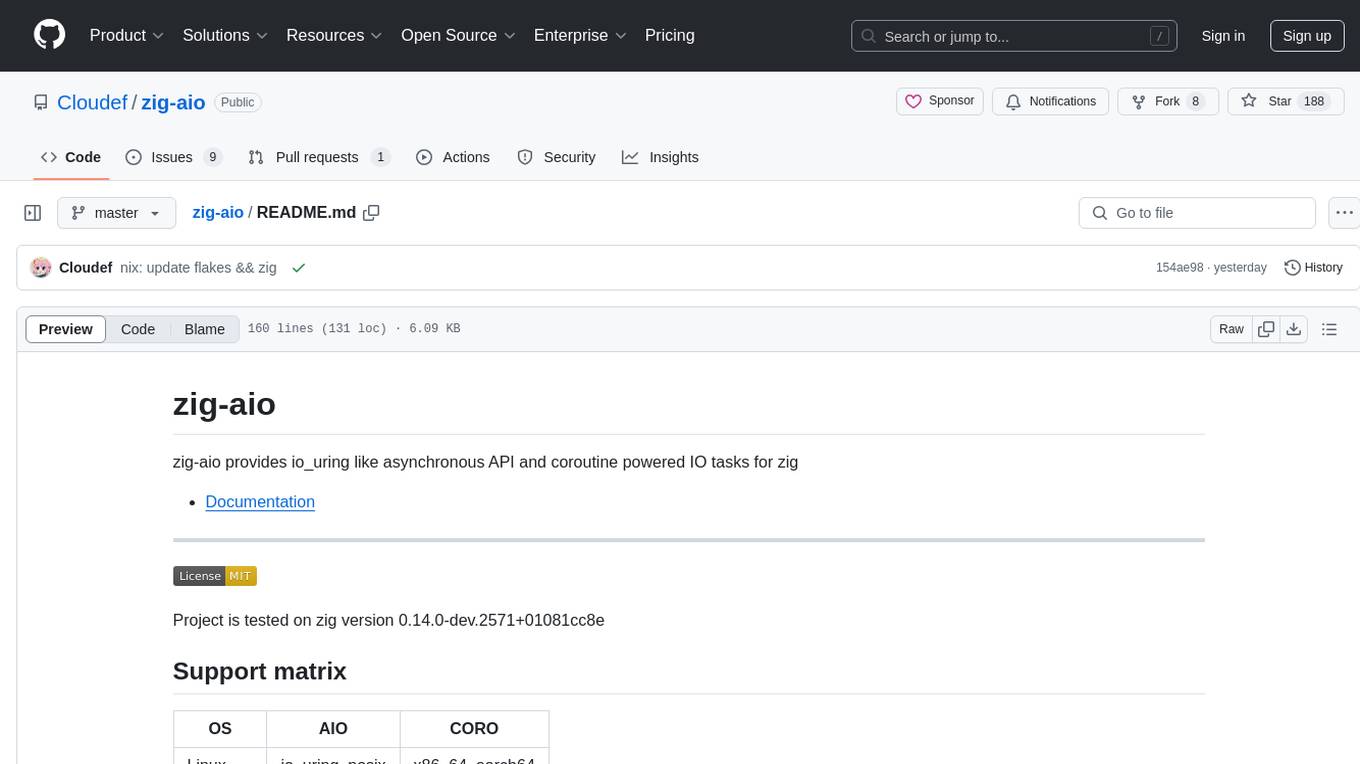
zig-aio
zig-aio is a library that provides an io_uring-like asynchronous API and coroutine-powered IO tasks for the Zig programming language. It offers support for different operating systems and backends, such as io_uring, iocp, and posix. The library aims to provide efficient IO operations by leveraging coroutines and async IO mechanisms. Users can create servers and clients with ease using the provided API functions for socket operations, sending and receiving data, and managing connections.
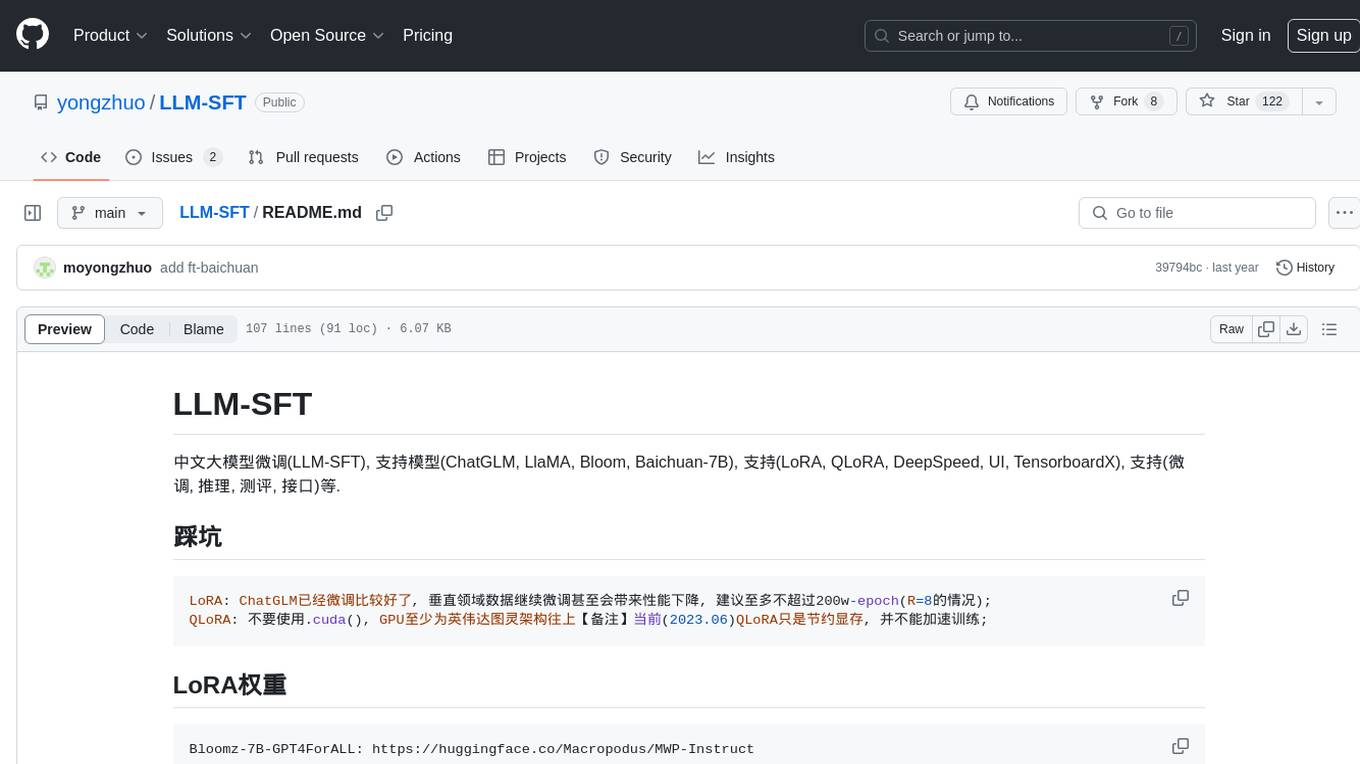
LLM-SFT
LLM-SFT is a Chinese large model fine-tuning tool that supports models such as ChatGLM, LlaMA, Bloom, Baichuan-7B, and frameworks like LoRA, QLoRA, DeepSpeed, UI, and TensorboardX. It facilitates tasks like fine-tuning, inference, evaluation, and API integration. The tool provides pre-trained weights for various models and datasets for Chinese language processing. It requires specific versions of libraries like transformers and torch for different functionalities.
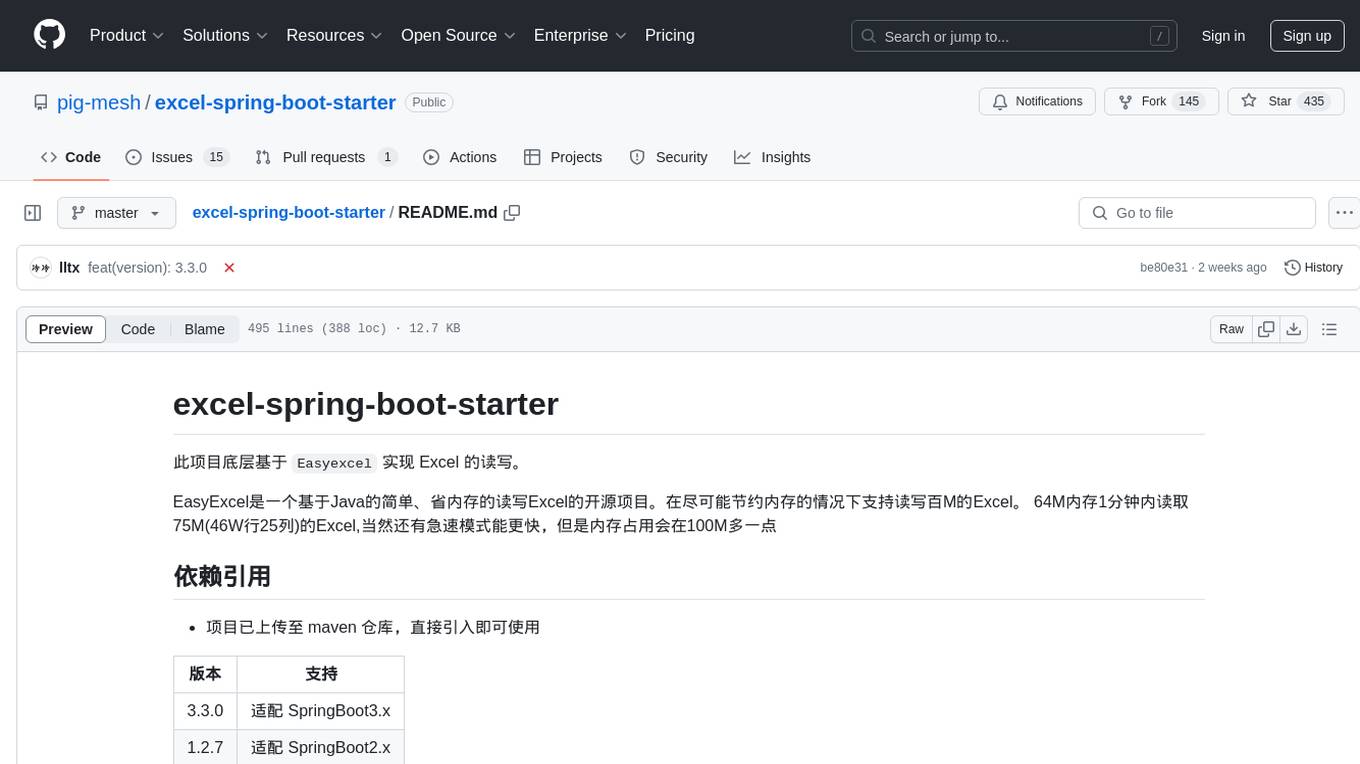
excel-spring-boot-starter
The excel-spring-boot-starter project is based on Easyexcel to implement reading and writing Excel files. EasyExcel is an open-source project for simple and memory-efficient reading and writing of Excel files in Java. It supports reading and writing Excel files up to 75M (46W rows 25 columns) in 1 minute with 64M memory, and there is a fast mode for even quicker performance but with slightly more memory consumption.
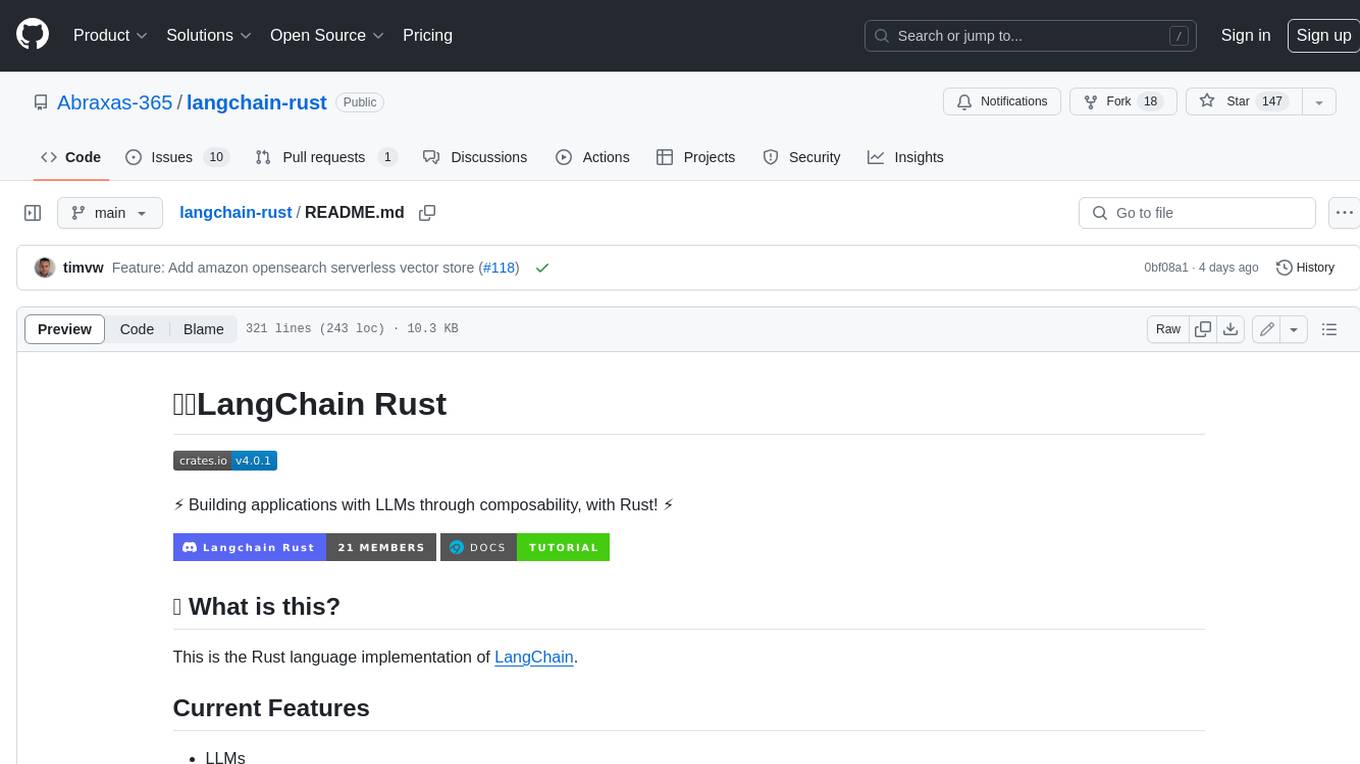
langchain-rust
LangChain Rust is a library for building applications with Large Language Models (LLMs) through composability. It provides a set of tools and components that can be used to create conversational agents, document loaders, and other applications that leverage LLMs. LangChain Rust supports a variety of LLMs, including OpenAI, Azure OpenAI, Ollama, and Anthropic Claude. It also supports a variety of embeddings, vector stores, and document loaders. LangChain Rust is designed to be easy to use and extensible, making it a great choice for developers who want to build applications with LLMs.
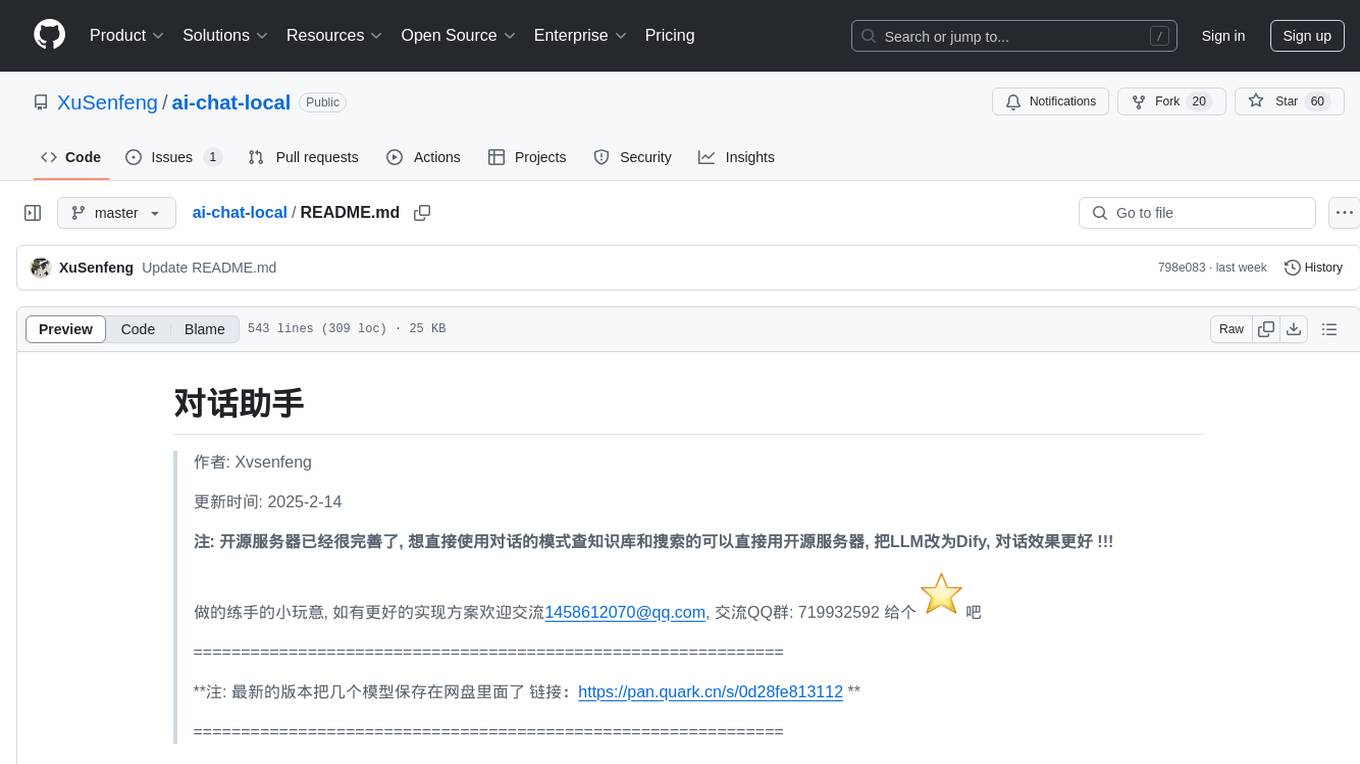
ai-chat-local
The ai-chat-local repository is a project that implements a local model customization using ollama, allowing data privacy and bypassing detection. It integrates with the esp32 development board to create a simple chat assistant. The project includes features like database integration for chat persistence, user recognition, and AI-based document processing. It also offers a graphical configuration tool for Dify.AI implementation.
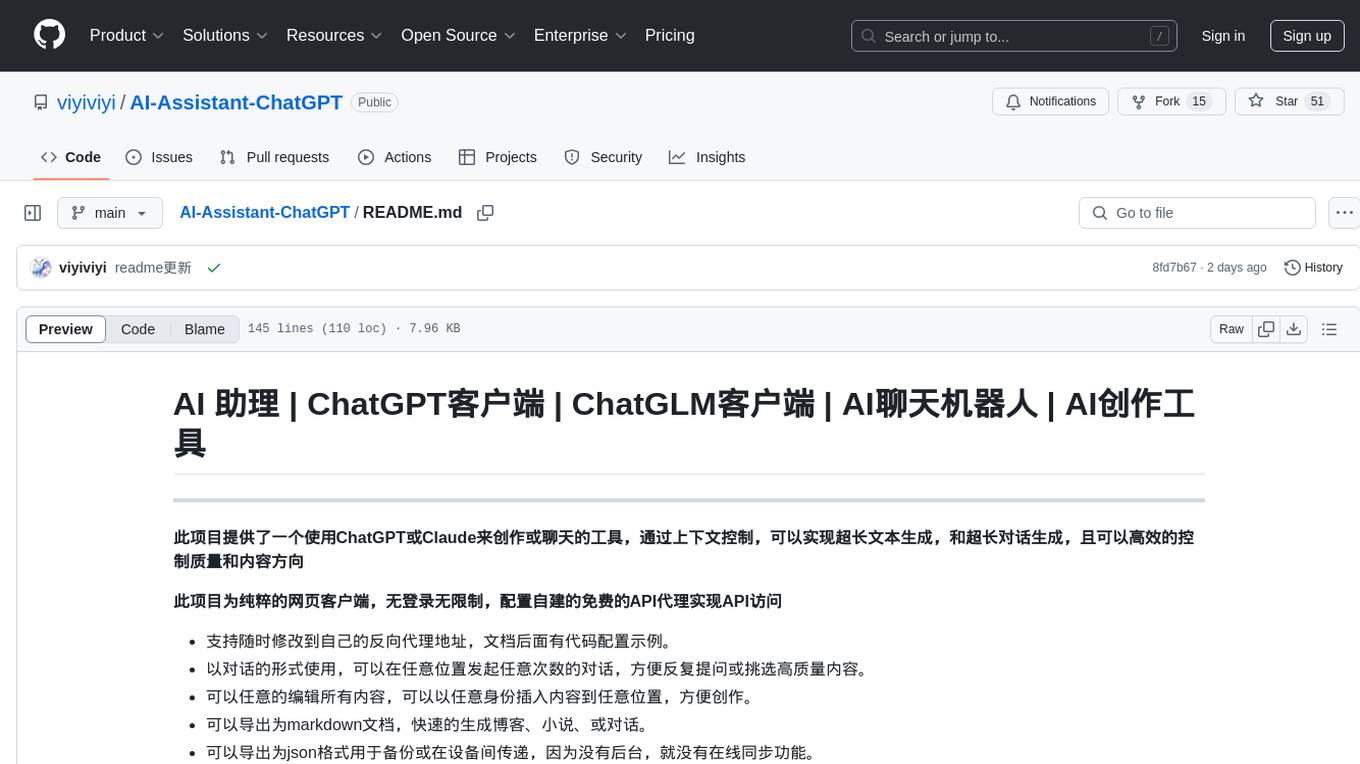
AI-Assistant-ChatGPT
AI Assistant ChatGPT is a web client tool that allows users to create or chat using ChatGPT or Claude. It enables generating long texts and conversations with efficient control over quality and content direction. The tool supports customization of reverse proxy address, conversation management, content editing, markdown document export, JSON backup, context customization, session-topic management, role customization, dynamic content navigation, and more. Users can access the tool directly at https://eaias.com or deploy it independently. It offers features for dialogue management, assistant configuration, session configuration, and more. The tool lacks data cloud storage and synchronization but provides guidelines for independent deployment. It is a frontend project that can be deployed using Cloudflare Pages and customized with backend modifications. The project is open-source under the MIT license.
For similar tasks
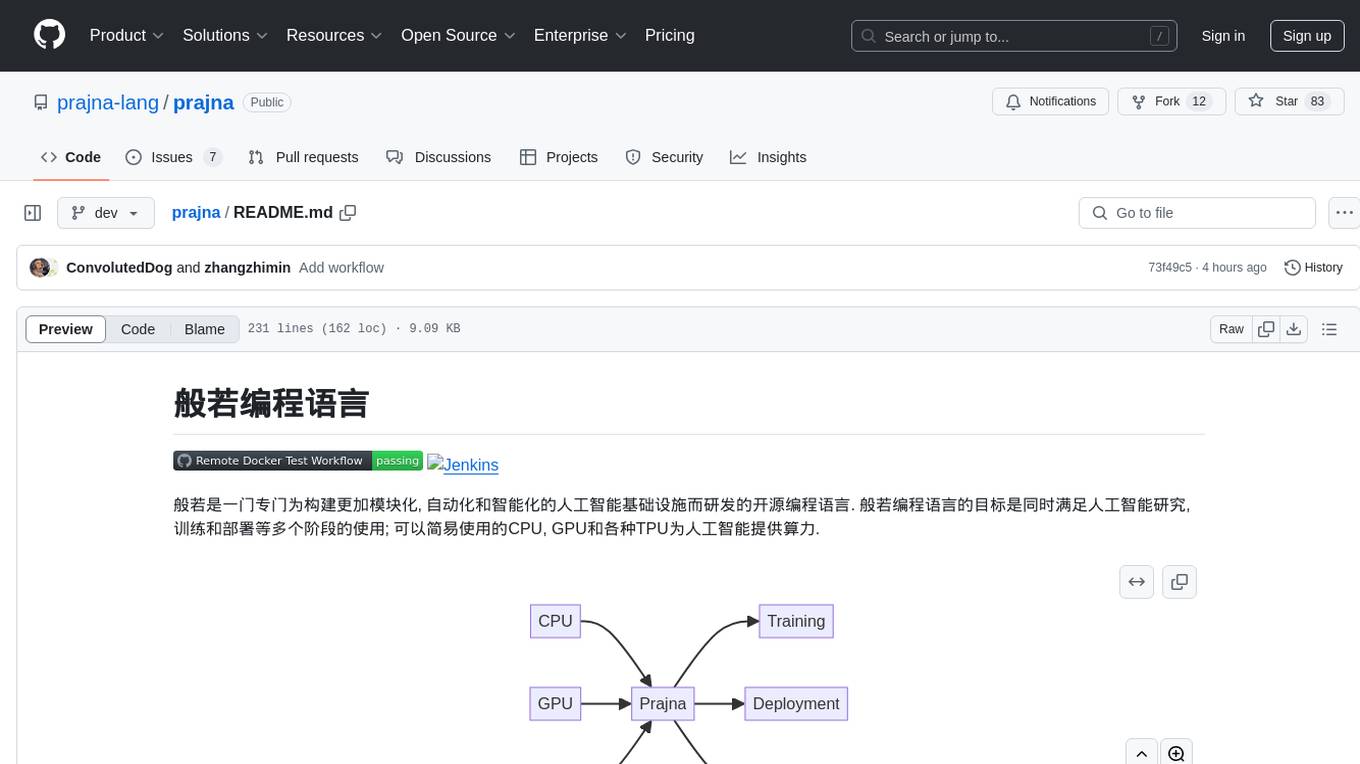
prajna
Prajna is an open-source programming language specifically developed for building more modular, automated, and intelligent artificial intelligence infrastructure. It aims to cater to various stages of AI research, training, and deployment by providing easy access to CPU, GPU, and various TPUs for AI computing. Prajna features just-in-time compilation, GPU/heterogeneous programming support, tensor computing, syntax improvements, and user-friendly interactions through main functions, Repl, and Jupyter, making it suitable for algorithm development and deployment in various scenarios.
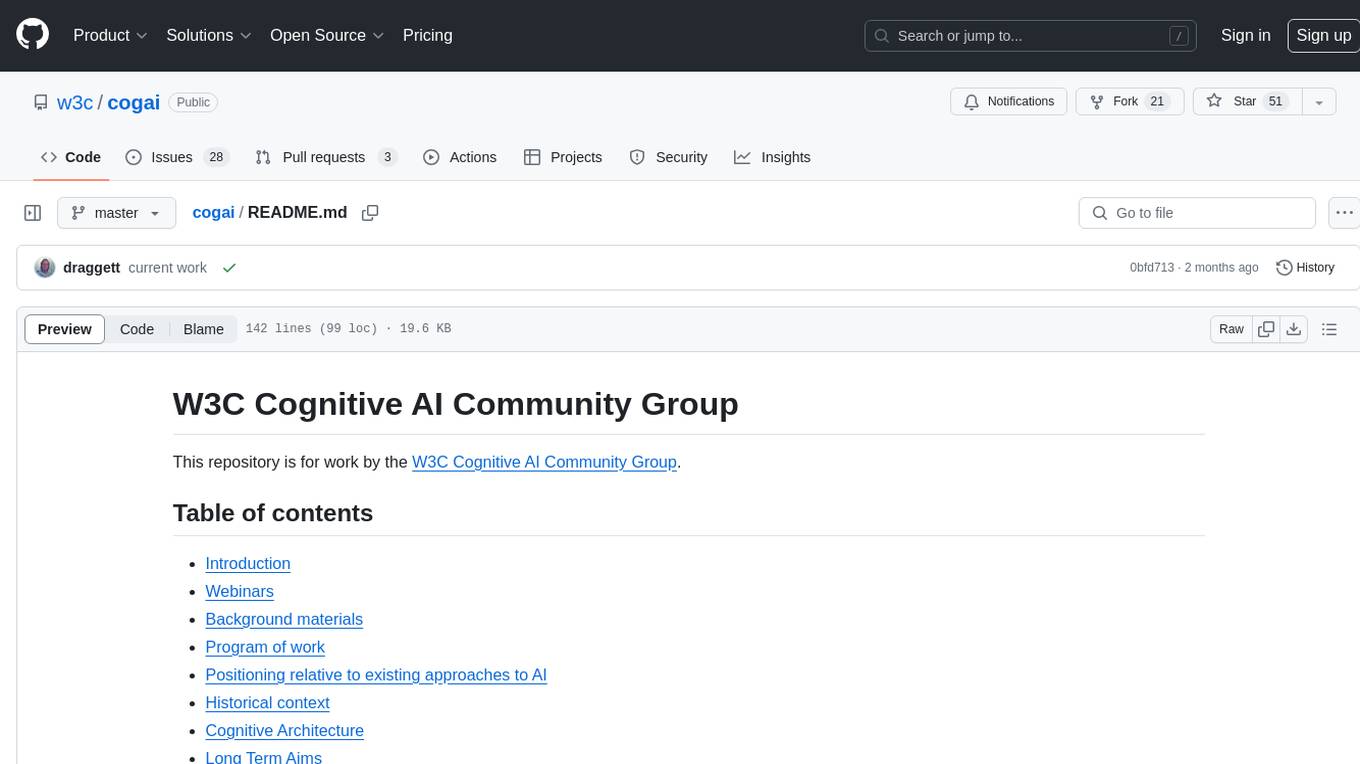
cogai
The W3C Cognitive AI Community Group focuses on advancing Cognitive AI through collaboration on defining use cases, open source implementations, and application areas. The group aims to demonstrate the potential of Cognitive AI in various domains such as customer services, healthcare, cybersecurity, online learning, autonomous vehicles, manufacturing, and web search. They work on formal specifications for chunk data and rules, plausible knowledge notation, and neural networks for human-like AI. The group positions Cognitive AI as a combination of symbolic and statistical approaches inspired by human thought processes. They address research challenges including mimicry, emotional intelligence, natural language processing, and common sense reasoning. The long-term goal is to develop cognitive agents that are knowledgeable, creative, collaborative, empathic, and multilingual, capable of continual learning and self-awareness.
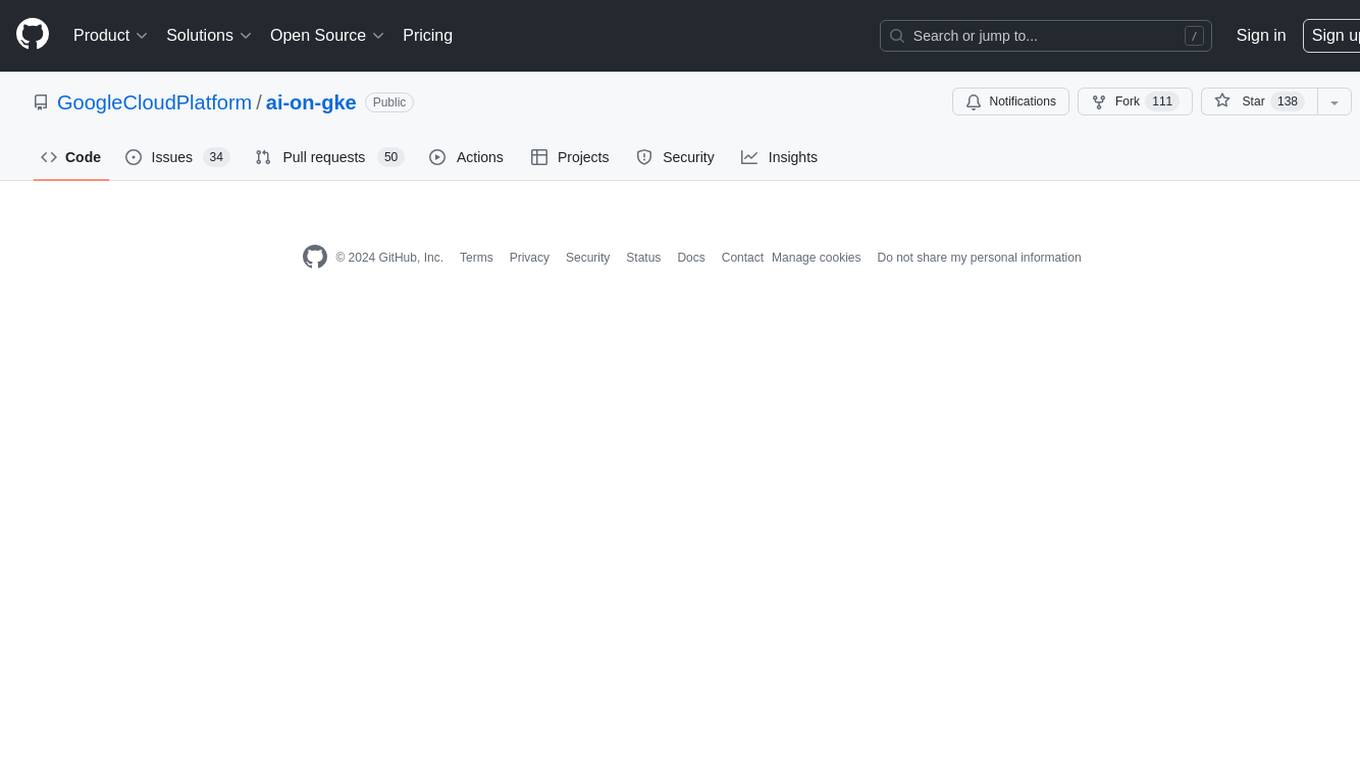
ai-on-gke
This repository contains assets related to AI/ML workloads on Google Kubernetes Engine (GKE). Run optimized AI/ML workloads with Google Kubernetes Engine (GKE) platform orchestration capabilities. A robust AI/ML platform considers the following layers: Infrastructure orchestration that support GPUs and TPUs for training and serving workloads at scale Flexible integration with distributed computing and data processing frameworks Support for multiple teams on the same infrastructure to maximize utilization of resources
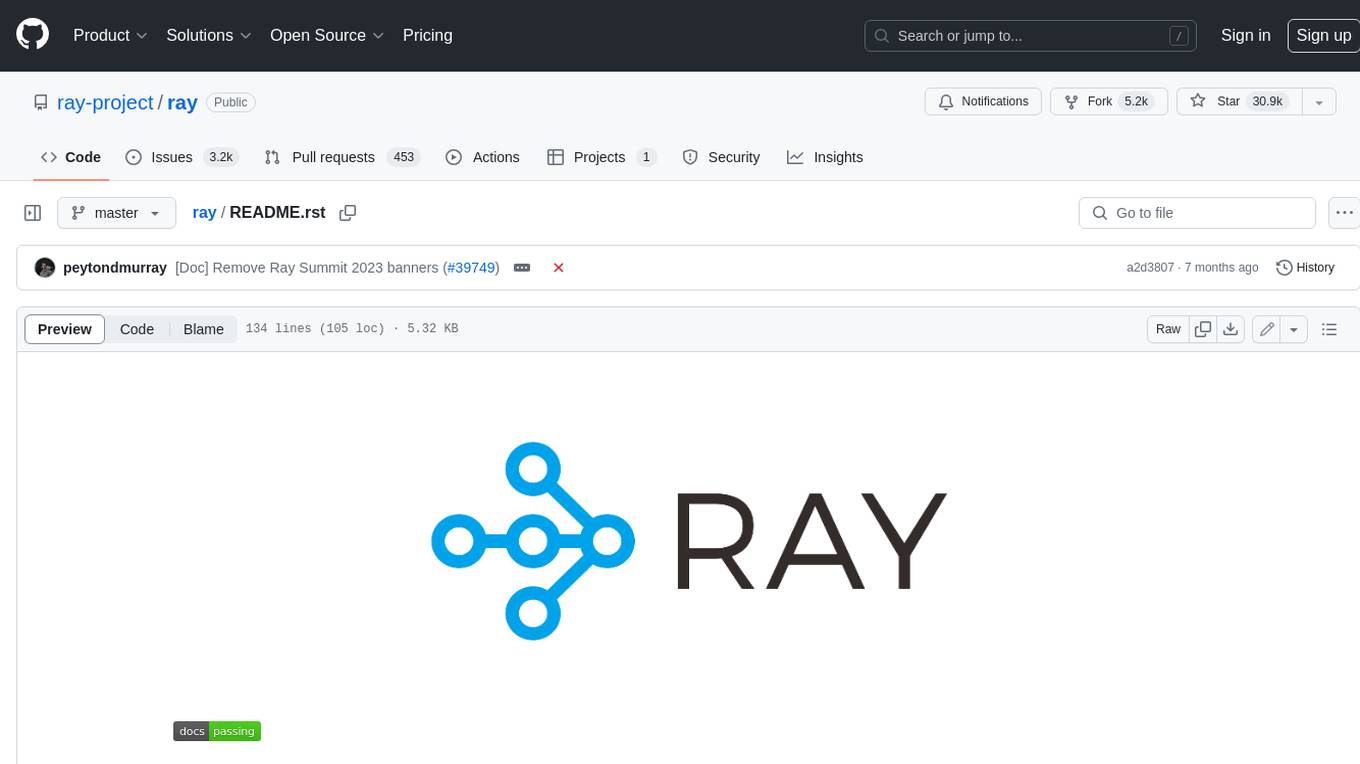
ray
Ray is a unified framework for scaling AI and Python applications. It consists of a core distributed runtime and a set of AI libraries for simplifying ML compute, including Data, Train, Tune, RLlib, and Serve. Ray runs on any machine, cluster, cloud provider, and Kubernetes, and features a growing ecosystem of community integrations. With Ray, you can seamlessly scale the same code from a laptop to a cluster, making it easy to meet the compute-intensive demands of modern ML workloads.
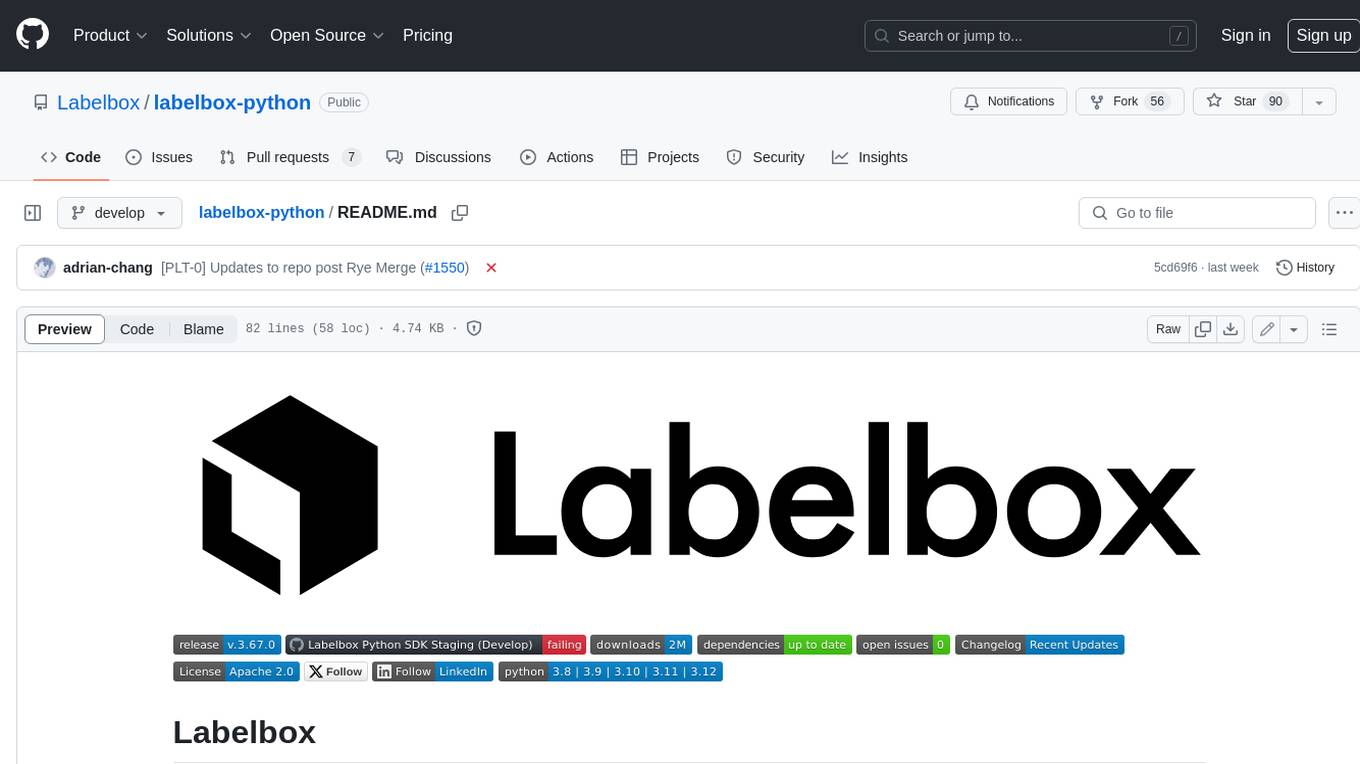
labelbox-python
Labelbox is a data-centric AI platform for enterprises to develop, optimize, and use AI to solve problems and power new products and services. Enterprises use Labelbox to curate data, generate high-quality human feedback data for computer vision and LLMs, evaluate model performance, and automate tasks by combining AI and human-centric workflows. The academic & research community uses Labelbox for cutting-edge AI research.
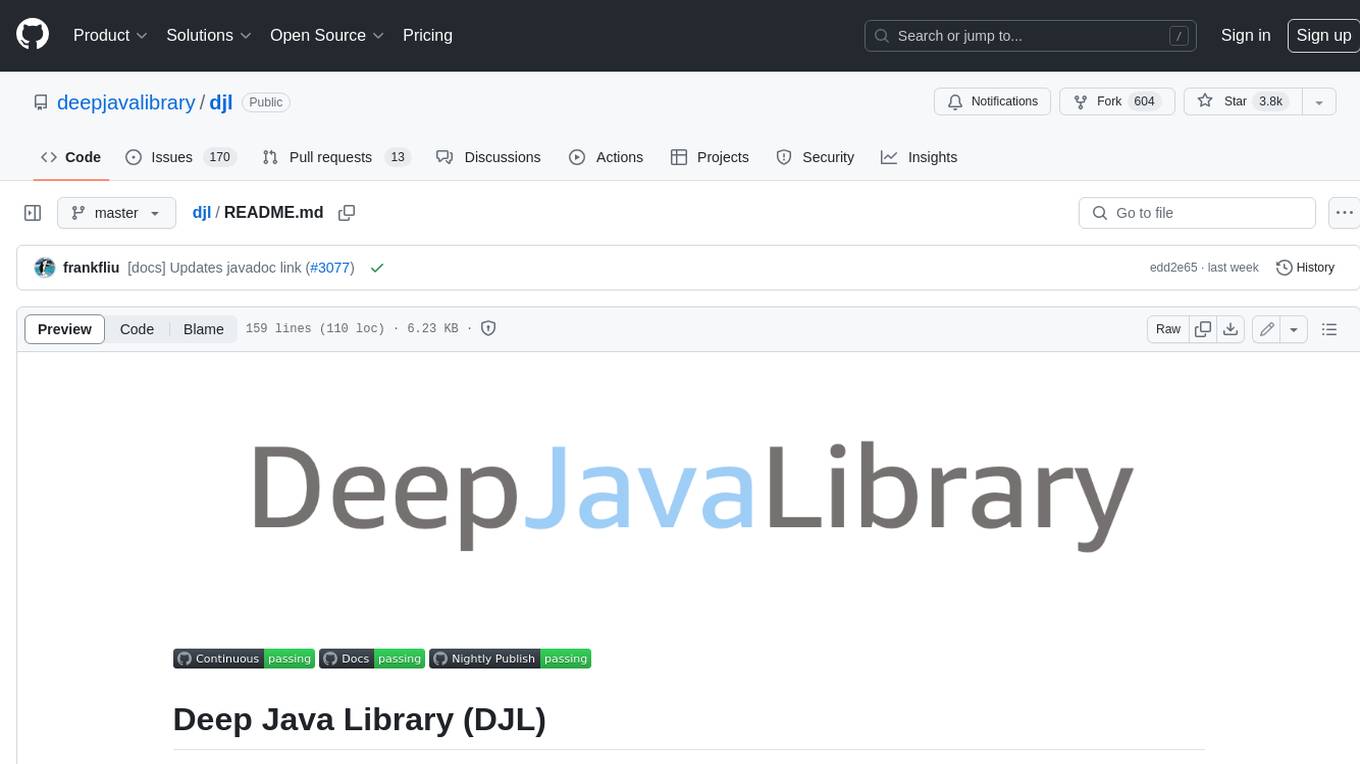
djl
Deep Java Library (DJL) is an open-source, high-level, engine-agnostic Java framework for deep learning. It is designed to be easy to get started with and simple to use for Java developers. DJL provides a native Java development experience and allows users to integrate machine learning and deep learning models with their Java applications. The framework is deep learning engine agnostic, enabling users to switch engines at any point for optimal performance. DJL's ergonomic API interface guides users with best practices to accomplish deep learning tasks, such as running inference and training neural networks.
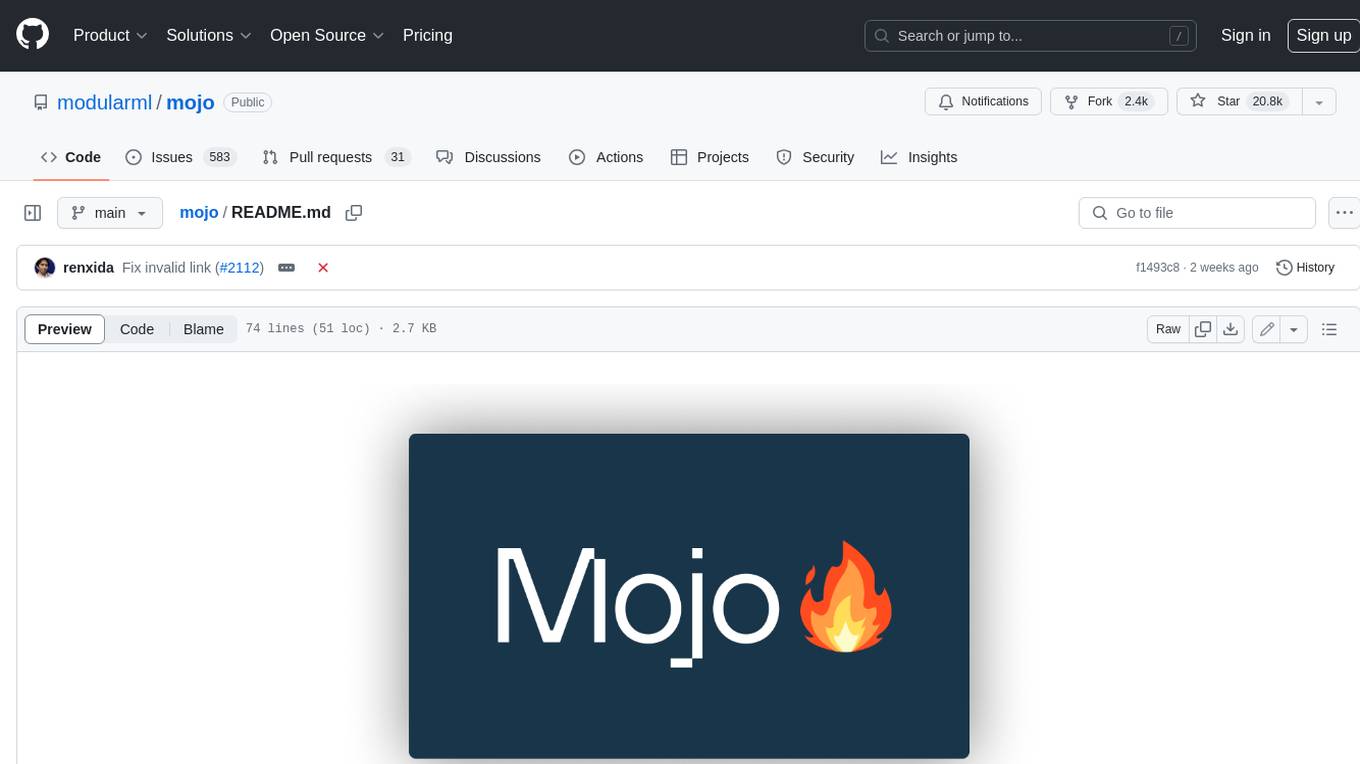
mojo
Mojo is a new programming language that bridges the gap between research and production by combining Python syntax and ecosystem with systems programming and metaprogramming features. Mojo is still young, but it is designed to become a superset of Python over time.
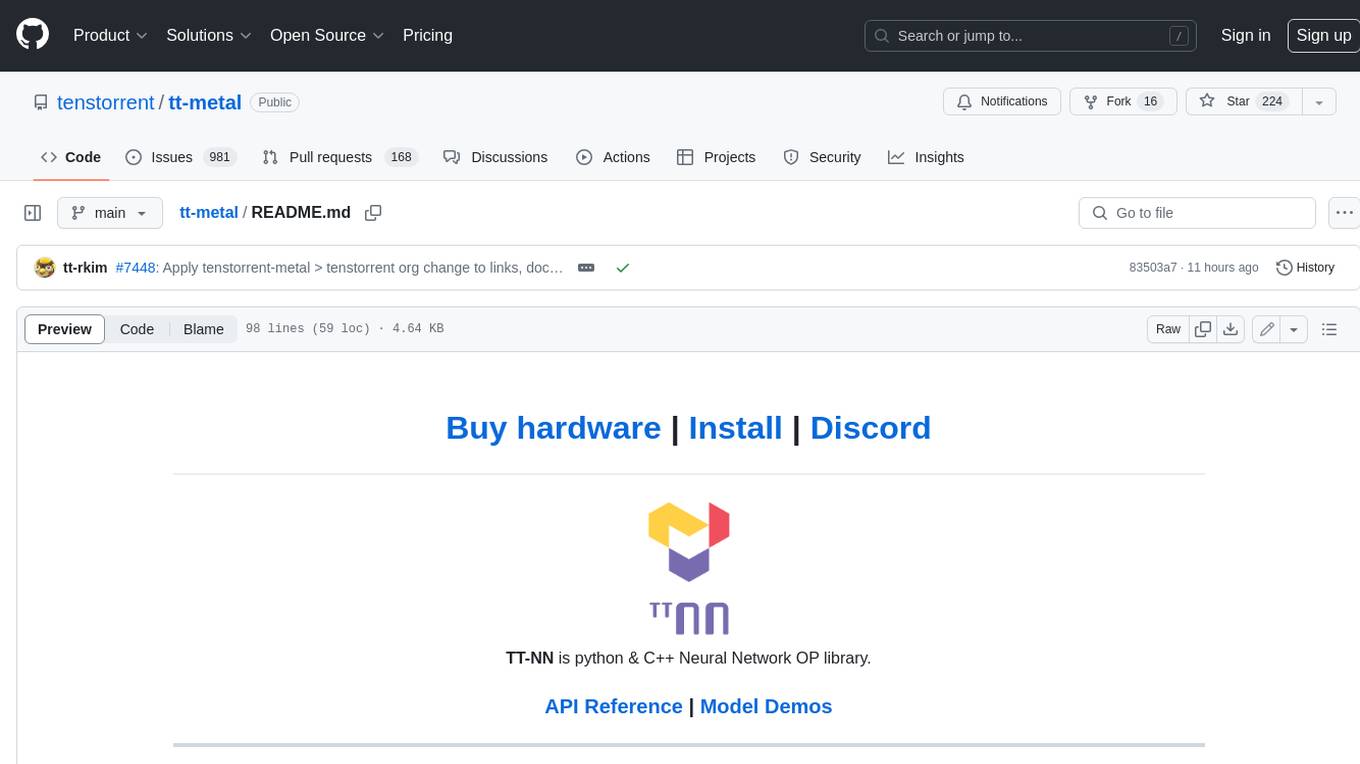
tt-metal
TT-NN is a python & C++ Neural Network OP library. It provides a low-level programming model, TT-Metalium, enabling kernel development for Tenstorrent hardware.
For similar jobs
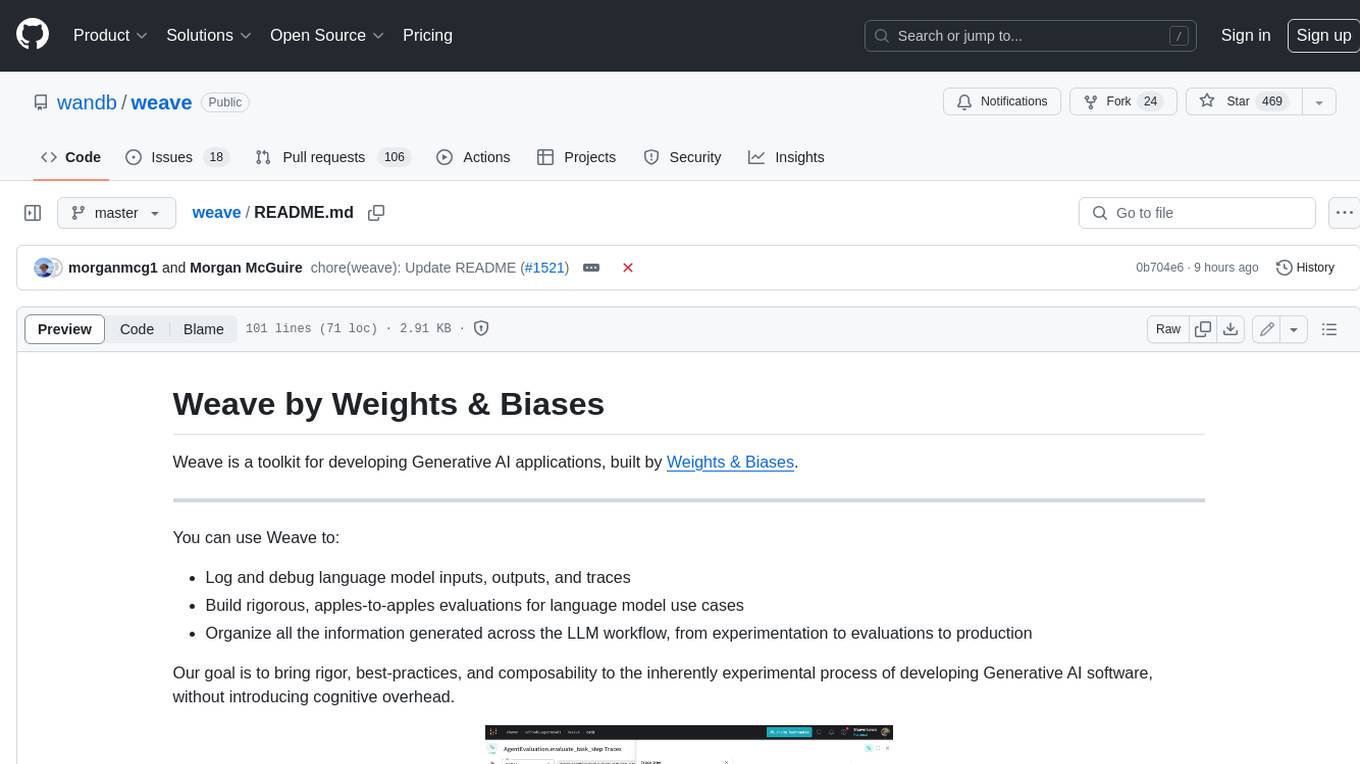
weave
Weave is a toolkit for developing Generative AI applications, built by Weights & Biases. With Weave, you can log and debug language model inputs, outputs, and traces; build rigorous, apples-to-apples evaluations for language model use cases; and organize all the information generated across the LLM workflow, from experimentation to evaluations to production. Weave aims to bring rigor, best-practices, and composability to the inherently experimental process of developing Generative AI software, without introducing cognitive overhead.
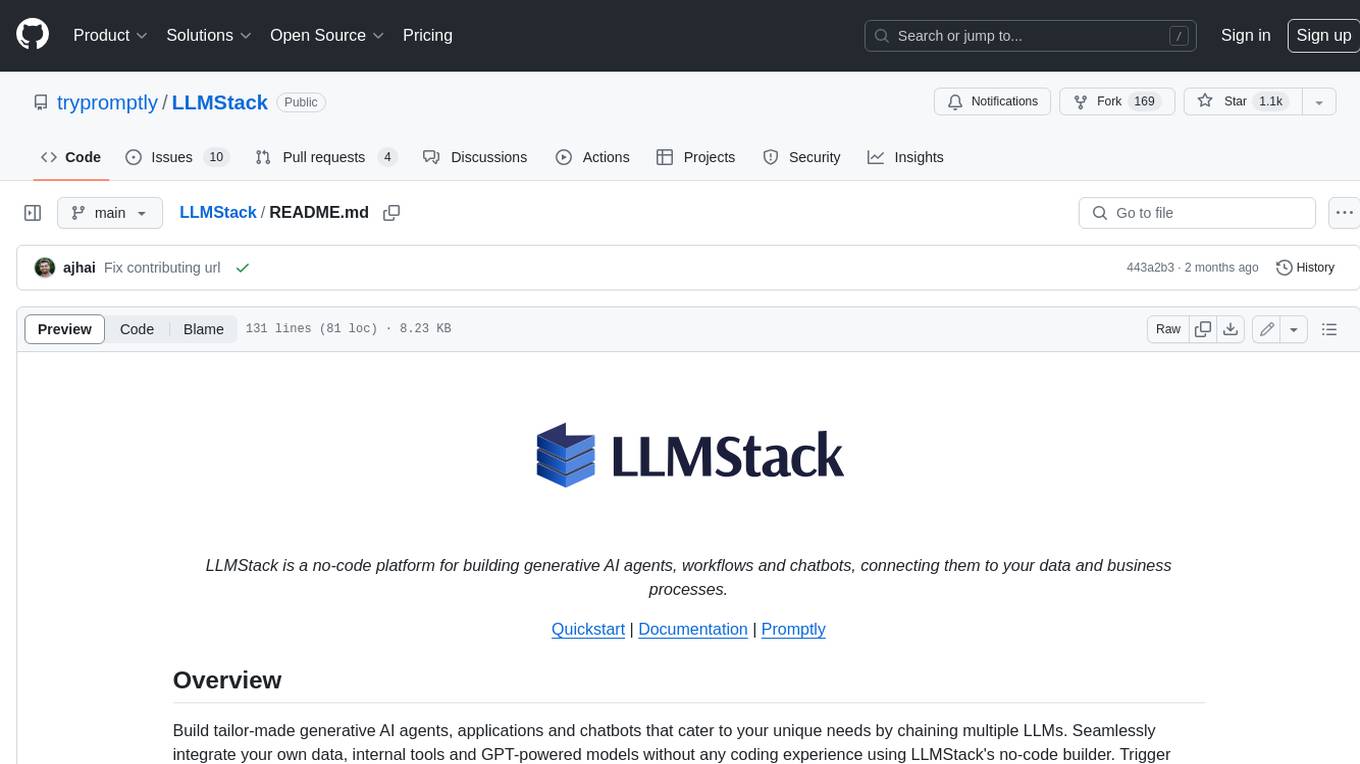
LLMStack
LLMStack is a no-code platform for building generative AI agents, workflows, and chatbots. It allows users to connect their own data, internal tools, and GPT-powered models without any coding experience. LLMStack can be deployed to the cloud or on-premise and can be accessed via HTTP API or triggered from Slack or Discord.
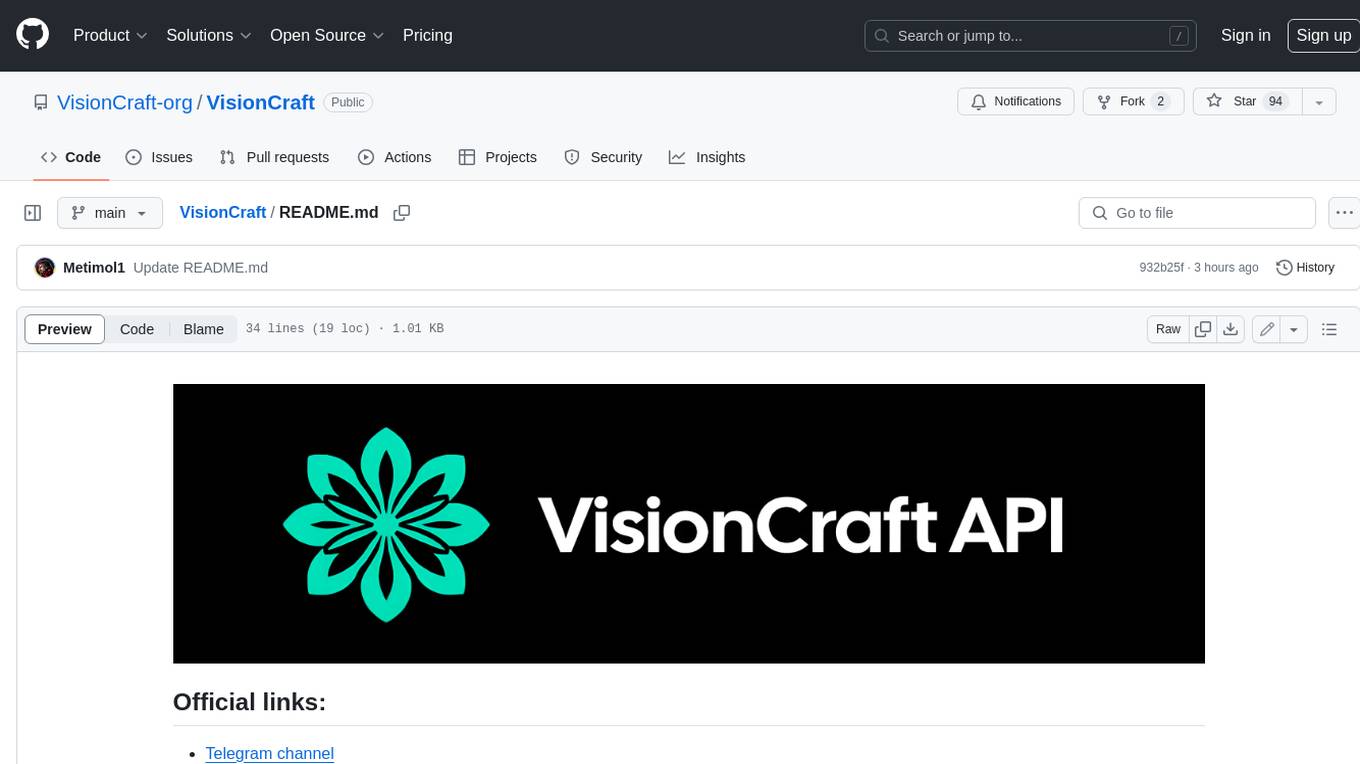
VisionCraft
The VisionCraft API is a free API for using over 100 different AI models. From images to sound.
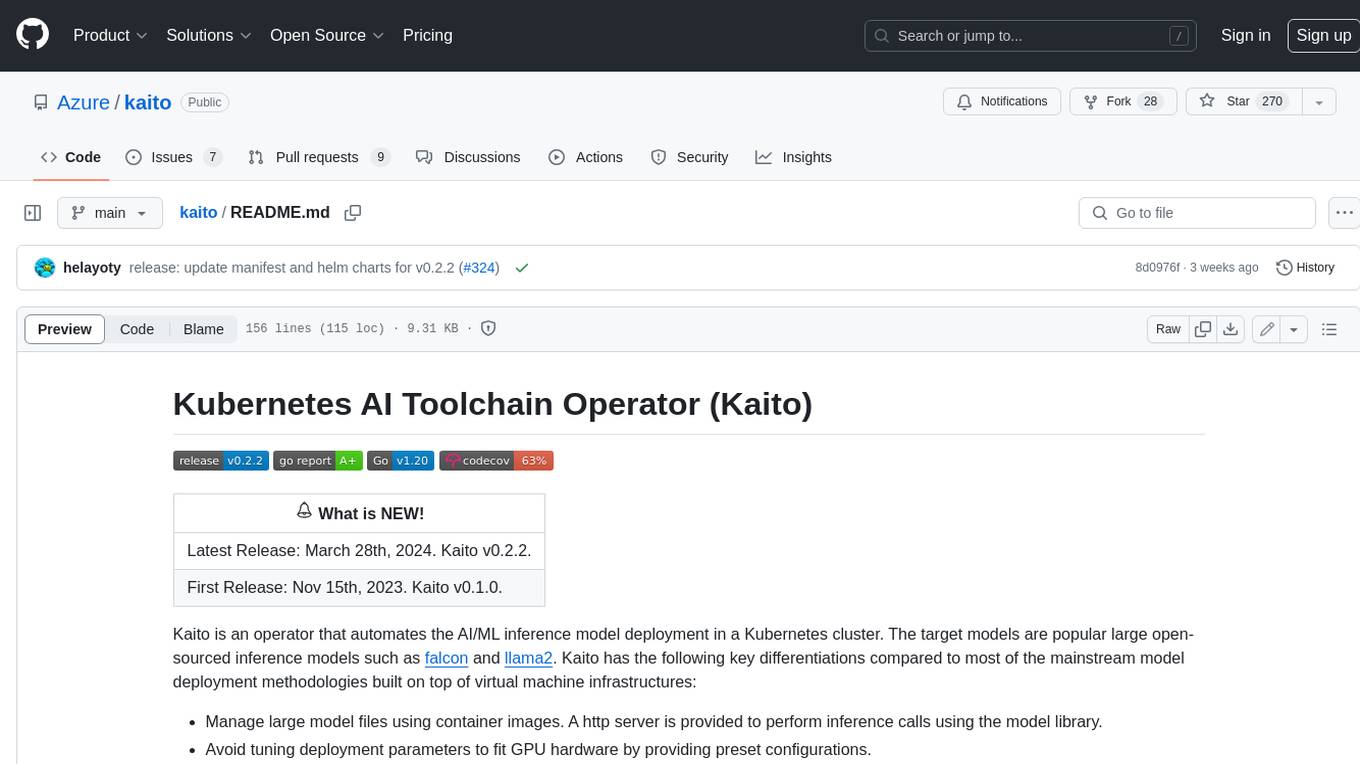
kaito
Kaito is an operator that automates the AI/ML inference model deployment in a Kubernetes cluster. It manages large model files using container images, avoids tuning deployment parameters to fit GPU hardware by providing preset configurations, auto-provisions GPU nodes based on model requirements, and hosts large model images in the public Microsoft Container Registry (MCR) if the license allows. Using Kaito, the workflow of onboarding large AI inference models in Kubernetes is largely simplified.
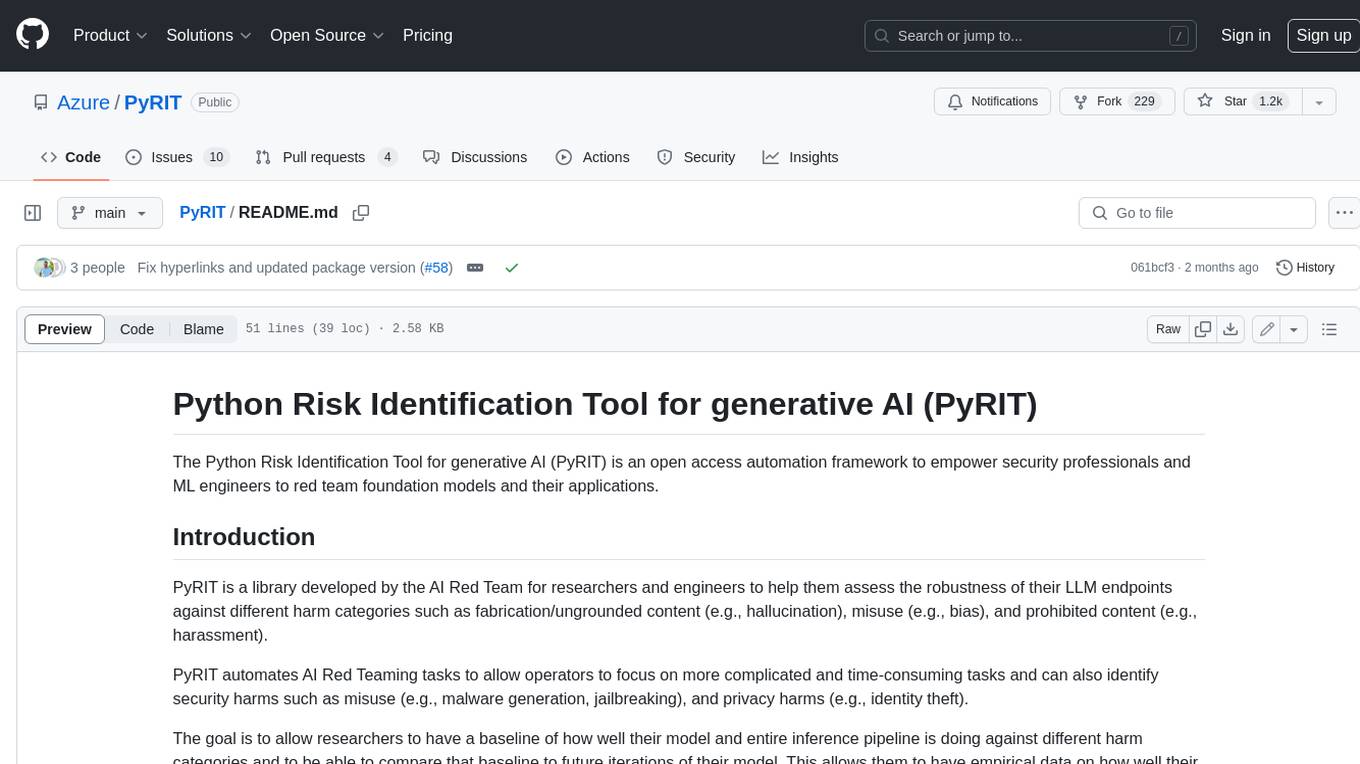
PyRIT
PyRIT is an open access automation framework designed to empower security professionals and ML engineers to red team foundation models and their applications. It automates AI Red Teaming tasks to allow operators to focus on more complicated and time-consuming tasks and can also identify security harms such as misuse (e.g., malware generation, jailbreaking), and privacy harms (e.g., identity theft). The goal is to allow researchers to have a baseline of how well their model and entire inference pipeline is doing against different harm categories and to be able to compare that baseline to future iterations of their model. This allows them to have empirical data on how well their model is doing today, and detect any degradation of performance based on future improvements.
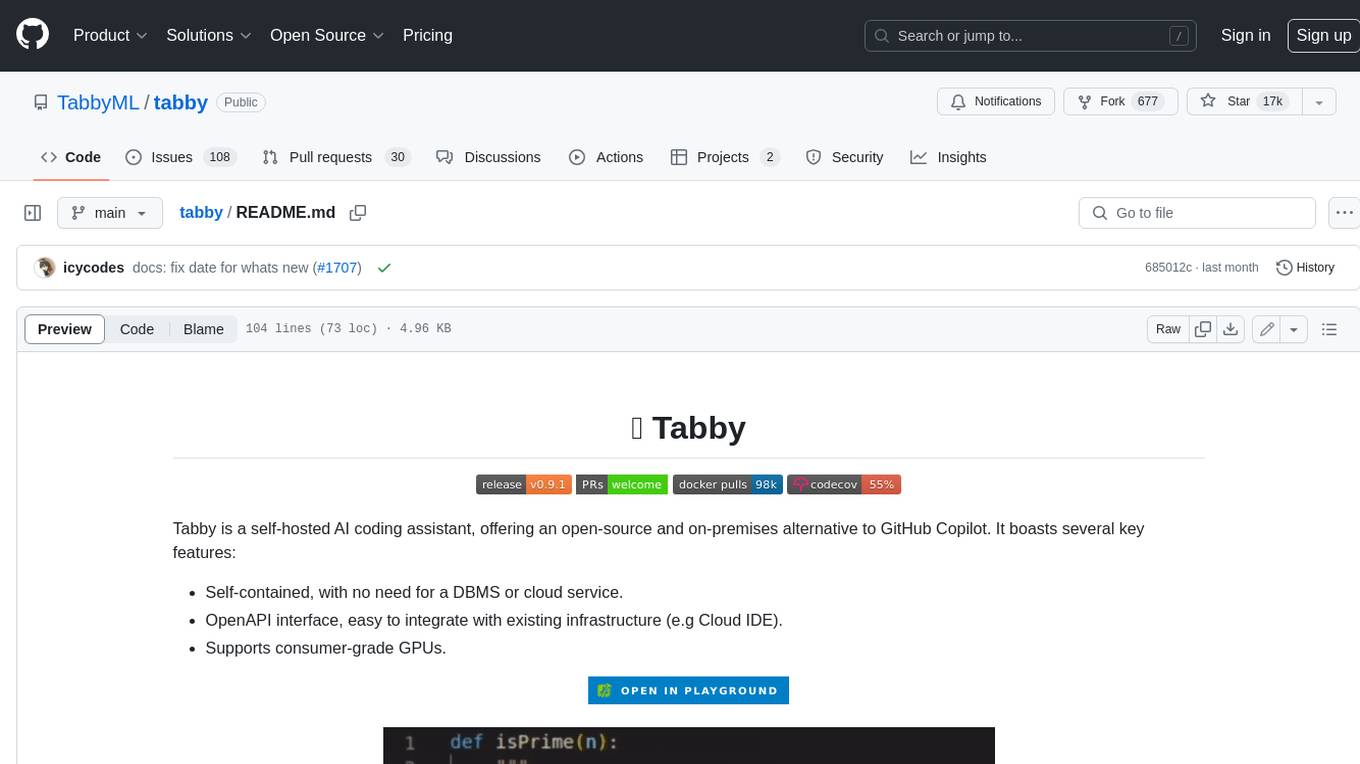
tabby
Tabby is a self-hosted AI coding assistant, offering an open-source and on-premises alternative to GitHub Copilot. It boasts several key features: * Self-contained, with no need for a DBMS or cloud service. * OpenAPI interface, easy to integrate with existing infrastructure (e.g Cloud IDE). * Supports consumer-grade GPUs.
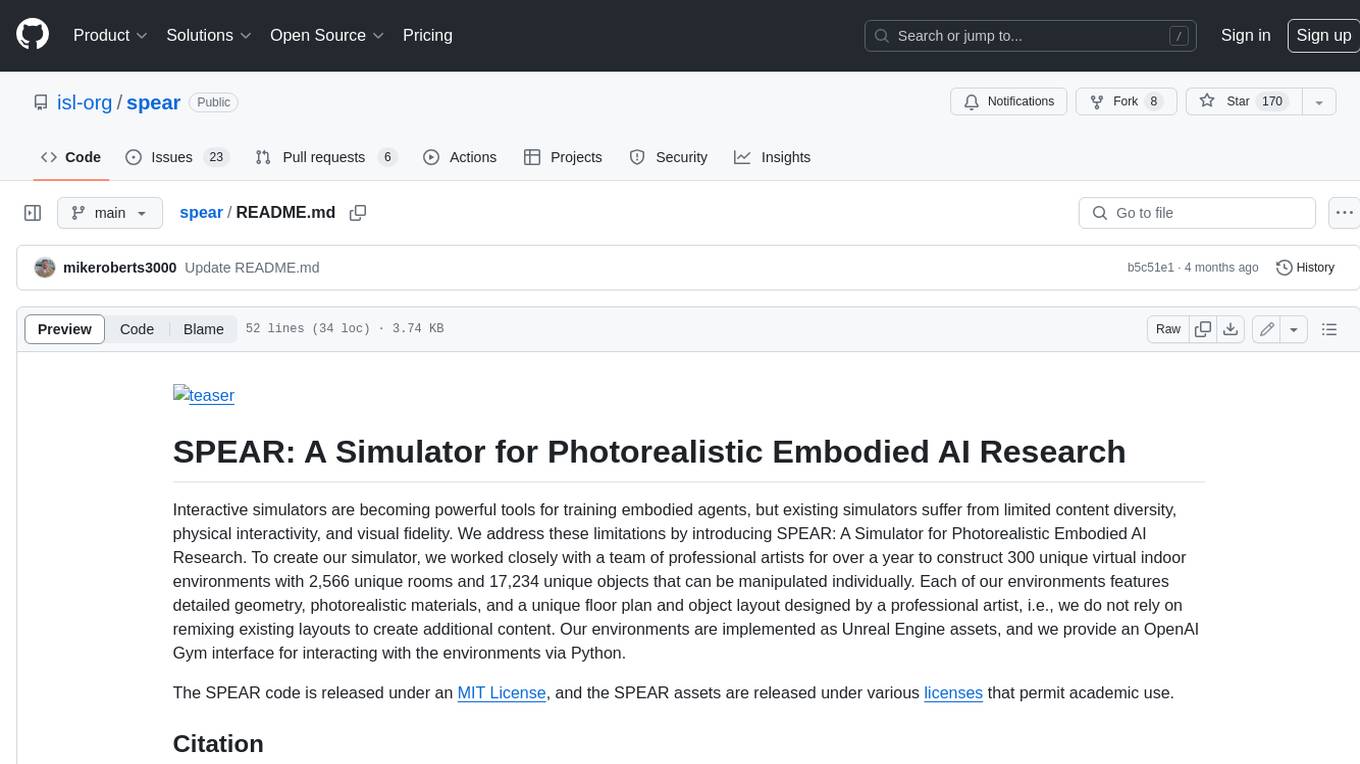
spear
SPEAR (Simulator for Photorealistic Embodied AI Research) is a powerful tool for training embodied agents. It features 300 unique virtual indoor environments with 2,566 unique rooms and 17,234 unique objects that can be manipulated individually. Each environment is designed by a professional artist and features detailed geometry, photorealistic materials, and a unique floor plan and object layout. SPEAR is implemented as Unreal Engine assets and provides an OpenAI Gym interface for interacting with the environments via Python.
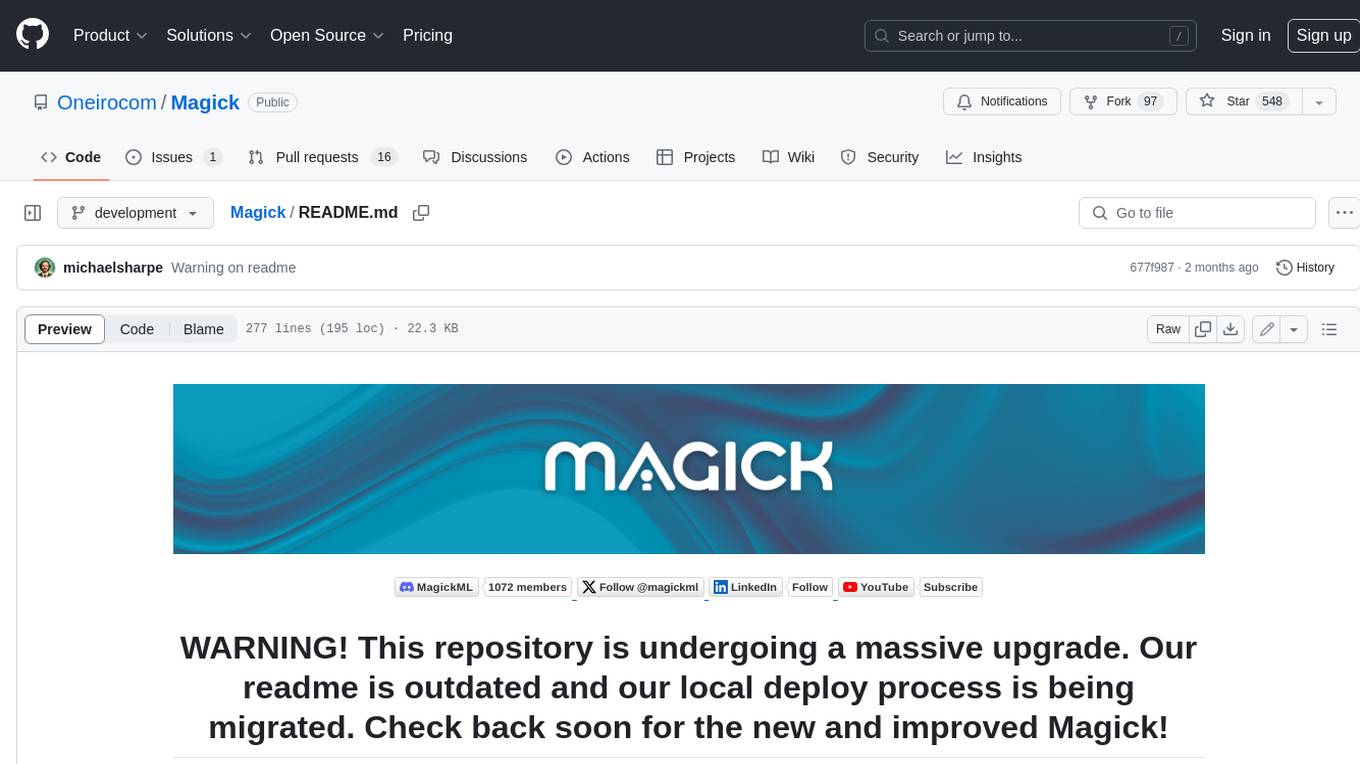
Magick
Magick is a groundbreaking visual AIDE (Artificial Intelligence Development Environment) for no-code data pipelines and multimodal agents. Magick can connect to other services and comes with nodes and templates well-suited for intelligent agents, chatbots, complex reasoning systems and realistic characters.