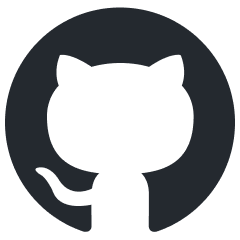
llm-structured-output
None
Stars: 52
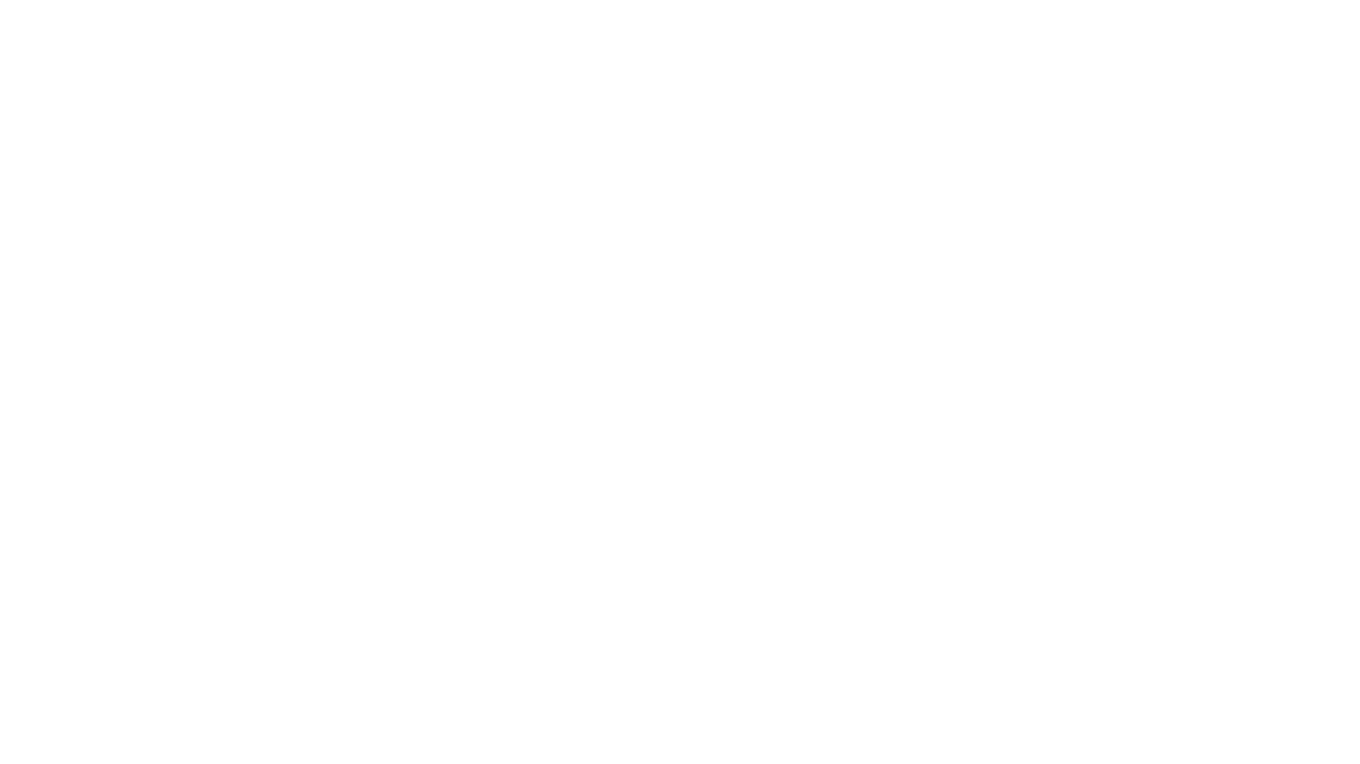
This repository contains a library for constraining LLM generation to structured output, enforcing a JSON schema for precise data types and property names. It includes an acceptor/state machine framework, JSON acceptor, and JSON schema acceptor for guiding decoding in LLMs. The library provides reference implementations using Apple's MLX library and examples for function calling tasks. The tool aims to improve LLM output quality by ensuring adherence to a schema, reducing unnecessary output, and enhancing performance through pre-emptive decoding. Evaluations show performance benchmarks and comparisons with and without schema constraints.
README:
This repository contains a library to constrain LLM generation to structured output, such as function calling a.k.a. tool use.
We include examples of application implementations using the MLX library.
Differences with other approaches:
-
"JSON mode": this library constrains output to be valid JSON, but goes beyond JSON mode in also enforcing a JSON schema. This enables much tighter steeing: specifying data types, property names, etc.
-
GBNF translation: rather than converting the JSON schema to a formal grammar, we steer the output directly using the schema, which enables more flexible and deeper control with lower overhead. For example, expressing minimum and maximum array or string lengths in GBNF can lead to very large set of production rules, and certain JSON schema features are simply not possible.
-
Fine-tuning: our approach is complementary to fine-tuning an LLM to produce structured output. While fine-tuning currently can enhance but not guarantee adherence to a schema, our system introduces strong guarantees on the output.
Without a schema, Mistral 7B Instruct 0.2 solves the data extraction task but, despite our instructions to the contrary, it adds a lot of additional output that's not necessary, is hard to parse, and wastes time.
With the schema, the generation is precisely the output we require.
You'll find:
-
A framefork and set of acceptors for constraining LLM output, which are application-independent.
-
Reference implementations and examples using Apple's MLX library.
-
An acceptor/state machine framework which progresses all valid states of a given graph simultaneously. This minimizes the need for backtracking, which is expensive for LLMs as it would require re-computing past tokens. In this sense, the concept is similar to a chart parser or Earley-style recognizer and shares a similar motivation. In practice, it's quite different because we're dealing with token-level input. We implemented several optimizations to minimize combinatorial explosion: we use a trie to traverse the token vocabulary in logarithmic time, and collapse the trie branches when multiple options are equivalent. We also prune the chart by removing equivalent states arrived at by different paths. See acceptor.py.
-
A JSON acceptor based on the framework above that accepts valid JSON. See json_acceptor.py.
-
A JSON schema acceptor based on both items above that accepts valid JSON that conforms to a JSON schema. See json_schema_acceptor.py. Please note that most but not all JSON schema directives are implemented. Please open an issue if one that you need is not.
-
An example of using the acceptors above to guide decoding in an LLM using Apple's MLX framework. See llm_schema.py. This example includes several decoding techniques, including pre-emptive evaluation, which is a way to use the acceptor to anticipate the tokens that can be generated according to the schema, and use that to evaluate two tokens at a time instead of one, sometimes leading to noticeable performance improvements.
-
A server example that implements an OpenAI-compatible API including tools / function calling. Unlike OpenAI's, this implementation always generates valid JSON, and does not return hallucinated parameters not defined in your function schema (but it may still hallucinate their values). See server.py.
Clone this repo:
git clone https://github.com/otriscon/llm-structured-output.git
cd llm-structured-output
Optional, but recommended: create and activate a virtual environment with your favorite tool of choice, e.g.
python -m venv .venv
source .venv/bin/activate
Move into the examples folder and install the requirements, then move back:
cd src/examples
pip install -r requirements.txt
cd ..
Run the llm_schema example:
MODEL=mistralai/Mistral-7B-Instruct-v0.2
LLM_PROMPT='[INST] Parse the following address into a JSON object: "27 Barrow St, New York, NY 10014". Your answer should be only a JSON object according to this schema: {"type": "object", "properties": {"streetNumber": {"type": "number"}, "streetName": {"type": "string"}, "city": {"type": {"string"}}, "state": {"type": "string"}, "zipCode": {"type": "number"}}}. Do not explain the result, just output it. Do not add any additional information. [/INST]'
LLM_SCHEMA='{"type": "object", "properties": {"streetNumber": {"type": "number"}, "streetName": {"type": "string"}, "city": {"type": "string"}, "state": {"type": "string"}, "zipCode": {"type": "number"}}}'
python3 -m examples.llm_schema --model-path $MODEL --prompt "$LLM_PROMPT" --schema "$LLM_SCHEMA" --max-tokens 1000 --repeat-prompt
Run the server example:
MODEL_PATH=mistralai/Mistral-7B-Instruct-v0.2 uvicorn examples.server:app --port 8080 --reload
Try calling the server with this example adapted from the OpenAI documentation (click on the example request titled Functions):
curl http://localhost:8080/v1/chat/completions \
-H "Content-Type: application/json" \
-d '{
"model": "ignored",
"messages": [
{
"role": "user",
"content": "What'\''s the weather like in Boston today?"
}
],
"tools": [
{
"type": "function",
"function": {
"name": "get_current_weather",
"description": "Get the current weather in a given location",
"parameters": {
"type": "object",
"properties": {
"location": {
"type": "string",
"description": "The city and state, e.g. San Francisco, CA"
},
"unit": {
"type": "string",
"enum": ["celsius", "fahrenheit"]
}
},
"required": ["location"]
}
}
}
],
"tool_choice": "auto"
}'
Install in your project with pip install llm-structured-output
and
use a JsonSchemaAcceptorDriver
within your normal generation loop:
import json
import mlx.core as mx
from mlx_lm.utils import load # Needs pip import mlx_lm
from llm_structured_output import JsonSchemaAcceptorDriver, HuggingfaceTokenizerHelper, bias_logits
MODEL_PATH = "mistralai/Mistral-7B-Instruct-v0.2"
SCHEMA = {
"type": "object",
"properties": {
"streetNumber": {"type": "number"},
"streetName": {"type": "string"},
"city": {"type": "string"},
"state": {"type": "string"},
"zipCode": {"type": "number"},
},
}
PROMPT = f'''
[INST] Parse the following address into a JSON object: "27 Barrow St, New York, NY 10014".
Your answer should be only a JSON object according to this schema: {json.dumps(SCHEMA)}
Do not explain the result, just output it. Do not add any additional information. [/INST]
'''
# Load the model as usual.
model, tokenizer = load(MODEL_PATH)
# Instantiate a token acceptor
tokenizer_helper = HuggingfaceTokenizerHelper(tokenizer)
vocabulary, eos_id = tokenizer_helper.extract_vocabulary()
token_acceptor_factory = JsonSchemaAcceptorDriver.driver_factory_for_model(vocabulary, eos_id)
token_acceptor = token_acceptor_factory(SCHEMA)
cache = None
tokens = tokenizer_helper.encode_prompt(PROMPT)
while tokens[-1] != eos_id:
# Evaluate the model as usual.
logits, cache = model(mx.array(tokens)[None], cache)
# Set probability to -inf for invalid tokens.
accepted_token_bitmap = token_acceptor.select_valid_tokens()
logits = bias_logits(mx, logits[0, -1, :], accepted_token_bitmap)
# Sample as usual, e.g.:
token = mx.argmax(logits, axis=-1).item()
if token == eos_id:
break
# Store or use the generated token.
tokens = [token]
text = tokenizer_helper.no_strip_decode(tokens)
print(text, end="")
# Advance the acceptor to the next state.
token_acceptor.advance_token(token)
Constraining the output of an LLM to follow a schema doesn't magically make the LLM great at producing output that solves a particular task.
If an LLM that is not prompted or fine-tuned correctly to solve the task, it will produce syntactically valid output but the values inside won't necessarily constitute a good solution. As with any other technique, proper LLM prompting and/or n-shot examples are crucial to avoid getting nice-looking, well-formatted, schema-compliant nonsense.
In particular, it's crucial to instruct the LLM regarding the desired output format, including making the desired schema part of the prompt. Here's an example of a prompt that includes the schema:
Parse the following address into a JSON object: "27 Barrow St, New York, NY 10014".
Your answer should be only a JSON object according to this schema: {"type": "object", "properties": {"streetNumber": {"type": "number"}, "streetName": {"type": "string"}, "city": {"type": {"string"}}, "state": {"type": "string"}, "zipCode": {"type": "number"}}}.
Do not explain the result, just output it. Do not add any additional information.
In order to give the LLM a scratch-pad prior to JSON generation for e.g.
chain-of-thought reasoning, we have included an option for the acceptor to kick in
only on output within a section delimited by the lines ```json
and ```
,
with the prior output treated as free text. This is enabled with the is_encapsulated_json
option of the JsonSchemaAcceptorDriver
constructor. Here's an example of a
prompt that produces encapsulated JSON:
Your mission is to parse the following address into a JSON object: "27 Barrow St, New York, NY 10014".
Your answer should be a JSON object according to this schema: {"type": "object", "properties": {"streetNumber": {"type": "number"}, "streetName": {"type": "string"}, "city": {"type": {"string"}}, "state": {"type": "string"}, "zipCode": {"type": "number"}}}.
First, think through the task step by step, and then output a JSON object wrapped between the lines ```json and ```.
In our OpenAI-compatible server example, when the request specifies tool_calls
or a
legacy function_call
, we automatically prepend a system message to the prompt with
the schema and instructions for the LLM to use the tools provided. If your prompt already
includes these instructions (because e.g. you want to customize them), this can be disabled
with a non-standard option in the request payload: "tool_options": { "no_prompt_steering": true }
The library has been tested with the following datasets:
-
ALU.AI's table extraction evaluation dataset (not yet open-source)
We're starting to perform evaluations to understand how well different LLMs perform in function calling tasks. The tools and data can be found in the src/tests folder.
Environment:
- llm_structured_output v0.0.15
- mlx 0.14.1
- 2023 Mac Studio M2 Ultra 24 cores (16 performance and 8 efficiency) 192 GB RAM running macOS Sonoma 14.5
- LLM: mlx-community/Meta-Llama-3-8B-Instruct-4bit
- Benchmarking LLM: gpt-4o-2024-05-13
Results:
Since we need to select the acceptable tokens prior to sampling, constraining the output according to a schema introduces a delay for every token, which depends on the complexity of the schema. On the other hand, since the output is guaranteed to be valid JSON and to conform to the schema, it can reduce the number of tokens generated and reduce or eliminate the number of retries required to solve the task.
As an experiment to improve performance, we implement the option to use pre-emptive decoding: when the range of tokens that can be accepted after the current one is small, as often happens with structured output, we submit to the LLM a batch of two-token continuations where the first token is the one that was to be evaluated anyway, and the second token in each item in the batch is one of the possible continuations predicted according to the schema. We can then sample two tokens instead of one. We find that this approach can occasionally produce considerable increases in token generation speed, but in general it can also considerably slow it down, depending on model and quantization. We found that it works better with no fp16 models (no quantization), but batching performance degrades vastly in quantized models making pre-emptive decoding not worth it for those models.
-
The following tests were perfomed on an Apple Studio with an M2 Ultra (24 core) with 192GB of RAM using MLX version 0.9.0, with models converted to MLX format.
-
The results are the average of 5 runs on a simple data extraction task with a 127-token prompt.
-
Pre-emptive decoding was tested in two different forms: with a constant batch size, where we always sent the same size matrices for evaluation, and variable- size batching, where we made the batch large or shorter depending on the numer of possible follow-up tokens.
Mistral-7B-v0.2-Instruct (fp16) | Prompt tps | Generation tps | Generation tokens |
---|---|---|---|
No schema | 305.82 | 34.76 | 321 |
Schema | 307.00 | 31.70 | 42 |
Pre-emptive constant batch =5 | 211.72 | 33.16 | 42 |
Pre-emptive variable batch <=5 | 321.85 | 36.53 | 42 |
Notes:
- Pre-emptive decoding accelerates generation even over schemaless generation.
Mistral-7B-v0.2-Instruct (q4) | Prompt tps | Generation tps | Generation tokens |
---|---|---|---|
No schema | 487.19 | 86.36 | 137 |
Schema | 487.83 | 67.60 | 42 |
Pre-emptive constant batch =5 | 139.61 | 27.16 | 42 |
Pre-emptive variable batch <=5 | 488.88 | 36.25 | 42 |
Notes:
- Pre-emptive decoding is vastly slower, with the only change being quantization.
Mixtral-8x7B-Instruct-v0.1 (fp16) | Prompt tps | Generation tps | Generation tokens |
---|---|---|---|
No schema | 3.48 | 2.23 | 50 |
Schema | 3.49 | 2.21 | 50 |
Pre-emptive constant batch =5 | 2.36 | 1.16 | 50 |
Pre-emptive variable batch <=5 | 3.18 | 1.68 | 50 |
Notes:
-
This is the only tested model that outputs schema-conforming output without a schema.
-
Pre-emptive decoding is a lot slower again.
Mixtral-8x7B-Instruct-v0.1 (q4) | Prompt tps | Generation tps | Generation tokens |
---|---|---|---|
No schema | 15.02 | 32.21 | 165 |
Schema | 14.94 | 23.75 | 50 |
Pre-emptive constant batch =5 | 9.29 | 11.28 | 50 |
Pre-emptive variable batch <=5 | 15.02 | 17.94 | 50 |
-
Extend JSON schema support as needed (see TODOs in code). Please, feel free to open an issue if you need a feature that not supported at the moment. Also open to implement additional schemas such as YAML and reference implementations for other LLMs.
-
Add formal test cases.
-
Reference implementation for the Transformers library.
-
Port to C++ and reference implementation for llama.cpp
For Tasks:
Click tags to check more tools for each tasksFor Jobs:
Alternative AI tools for llm-structured-output
Similar Open Source Tools
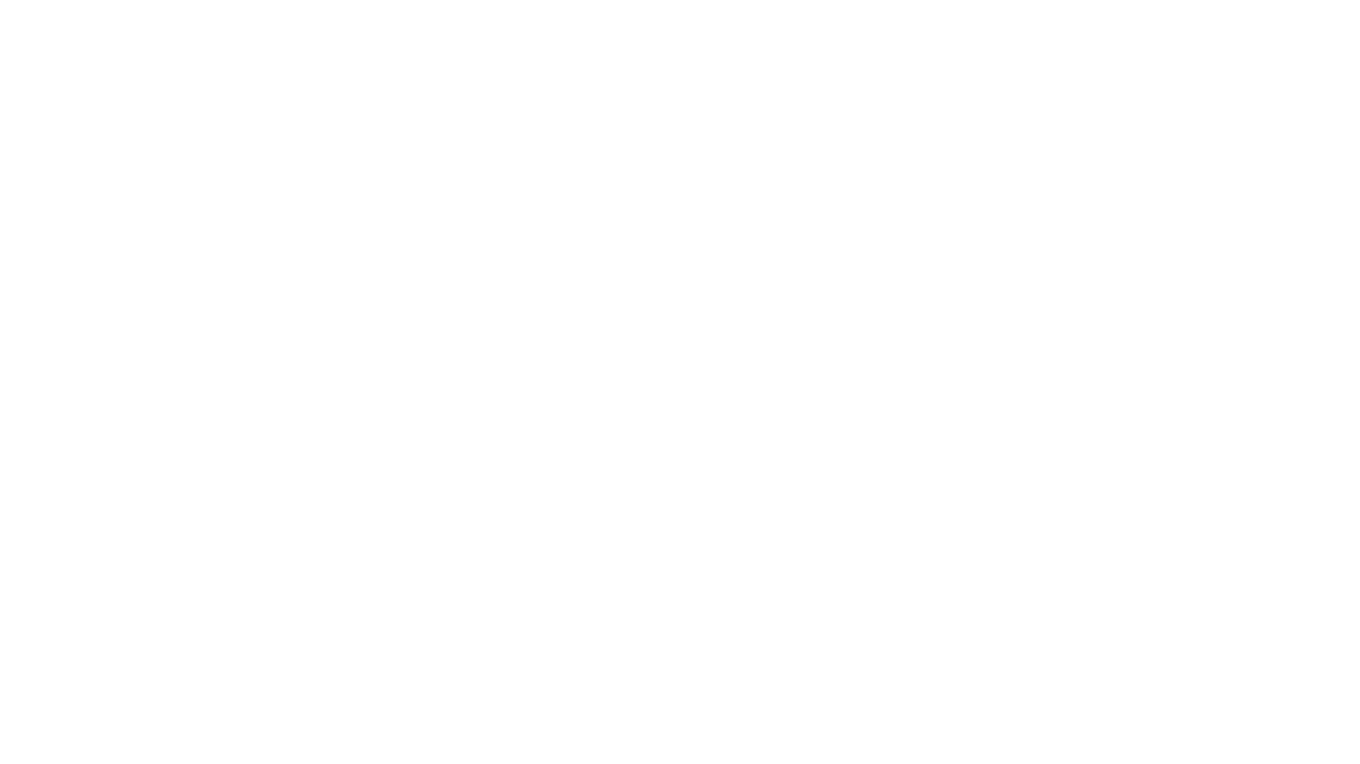
llm-structured-output
This repository contains a library for constraining LLM generation to structured output, enforcing a JSON schema for precise data types and property names. It includes an acceptor/state machine framework, JSON acceptor, and JSON schema acceptor for guiding decoding in LLMs. The library provides reference implementations using Apple's MLX library and examples for function calling tasks. The tool aims to improve LLM output quality by ensuring adherence to a schema, reducing unnecessary output, and enhancing performance through pre-emptive decoding. Evaluations show performance benchmarks and comparisons with and without schema constraints.
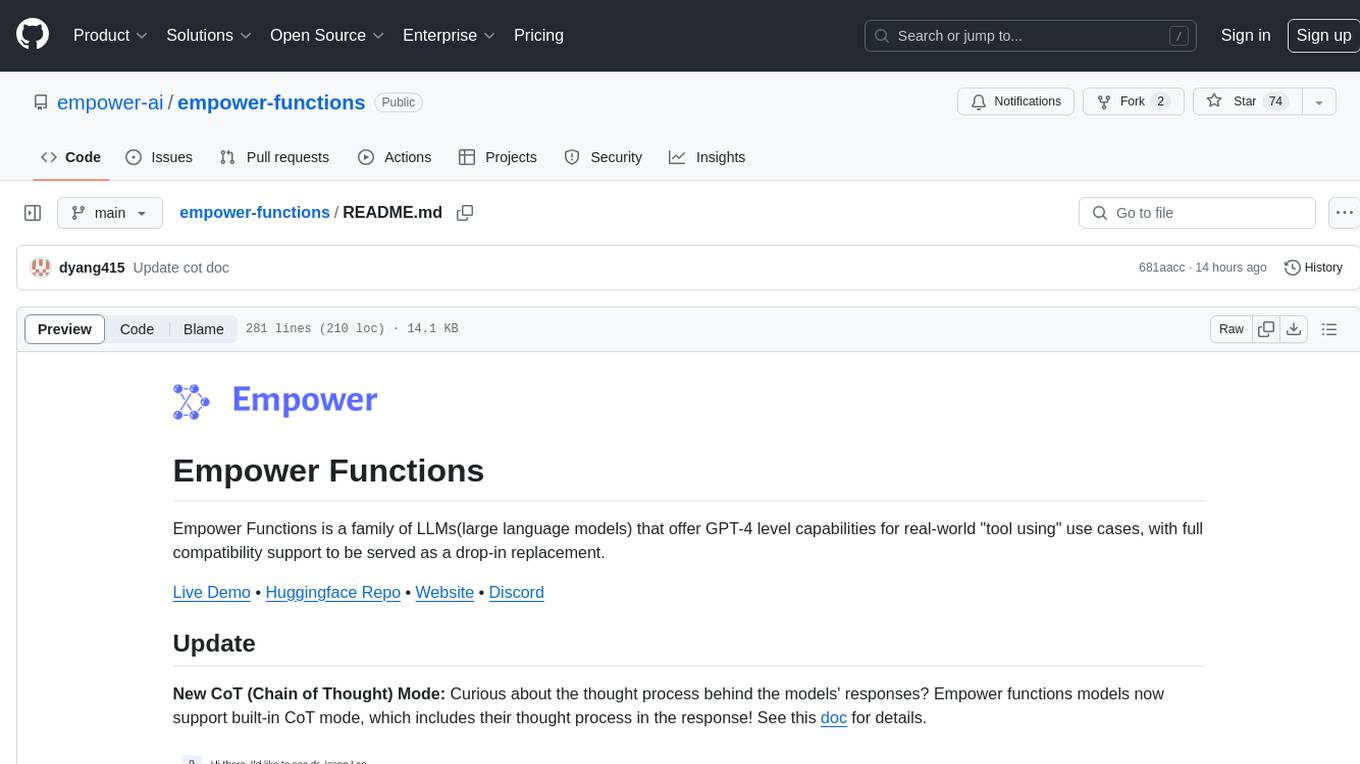
empower-functions
Empower Functions is a family of large language models (LLMs) that provide GPT-4 level capabilities for real-world 'tool using' use cases. These models offer compatibility support to be used as drop-in replacements, enabling interactions with external APIs by recognizing when a function needs to be called and generating JSON containing necessary arguments based on user inputs. This capability is crucial for building conversational agents and applications that convert natural language into API calls, facilitating tasks such as weather inquiries, data extraction, and interactions with knowledge bases. The models can handle multi-turn conversations, choose between tools or standard dialogue, ask for clarification on missing parameters, integrate responses with tool outputs in a streaming fashion, and efficiently execute multiple functions either in parallel or sequentially with dependencies.
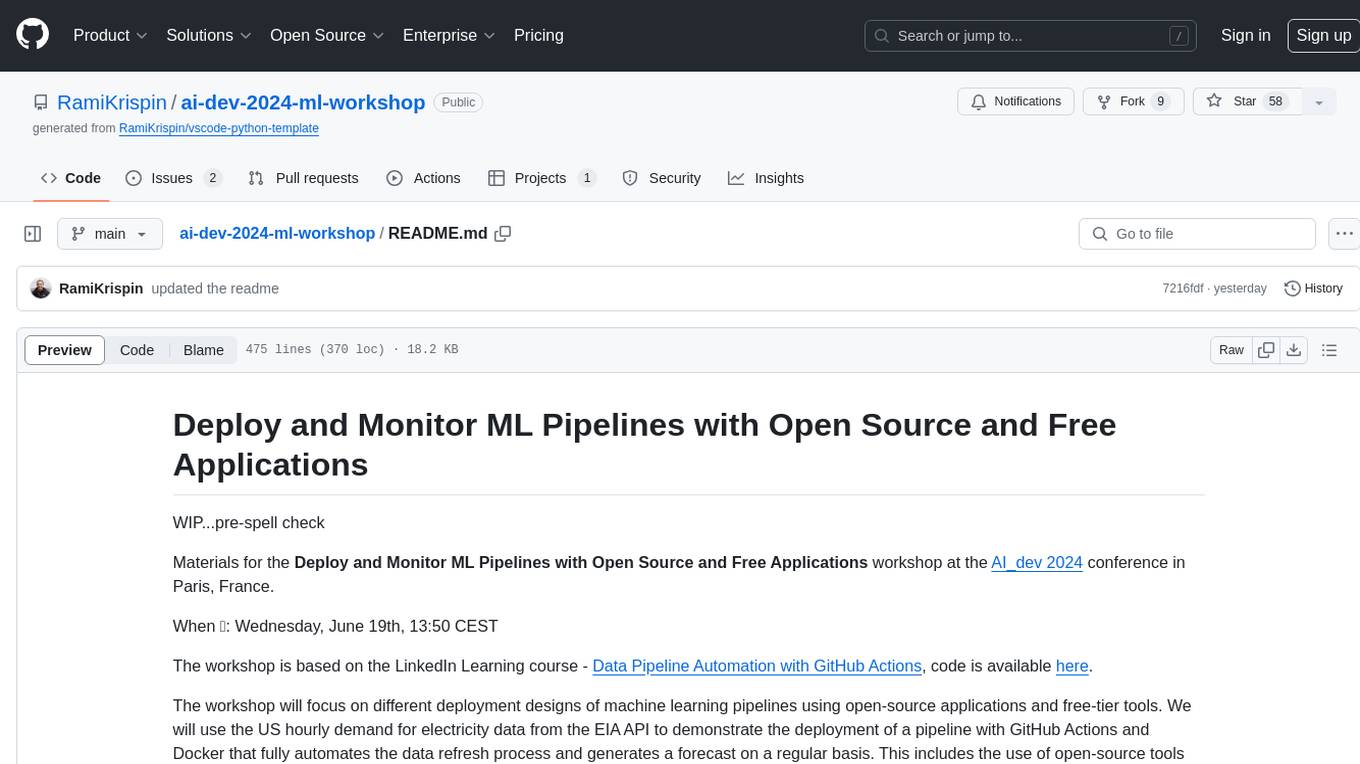
ai-dev-2024-ml-workshop
The 'ai-dev-2024-ml-workshop' repository contains materials for the Deploy and Monitor ML Pipelines workshop at the AI_dev 2024 conference in Paris, focusing on deployment designs of machine learning pipelines using open-source applications and free-tier tools. It demonstrates automating data refresh and forecasting using GitHub Actions and Docker, monitoring with MLflow and YData Profiling, and setting up a monitoring dashboard with Quarto doc on GitHub Pages.
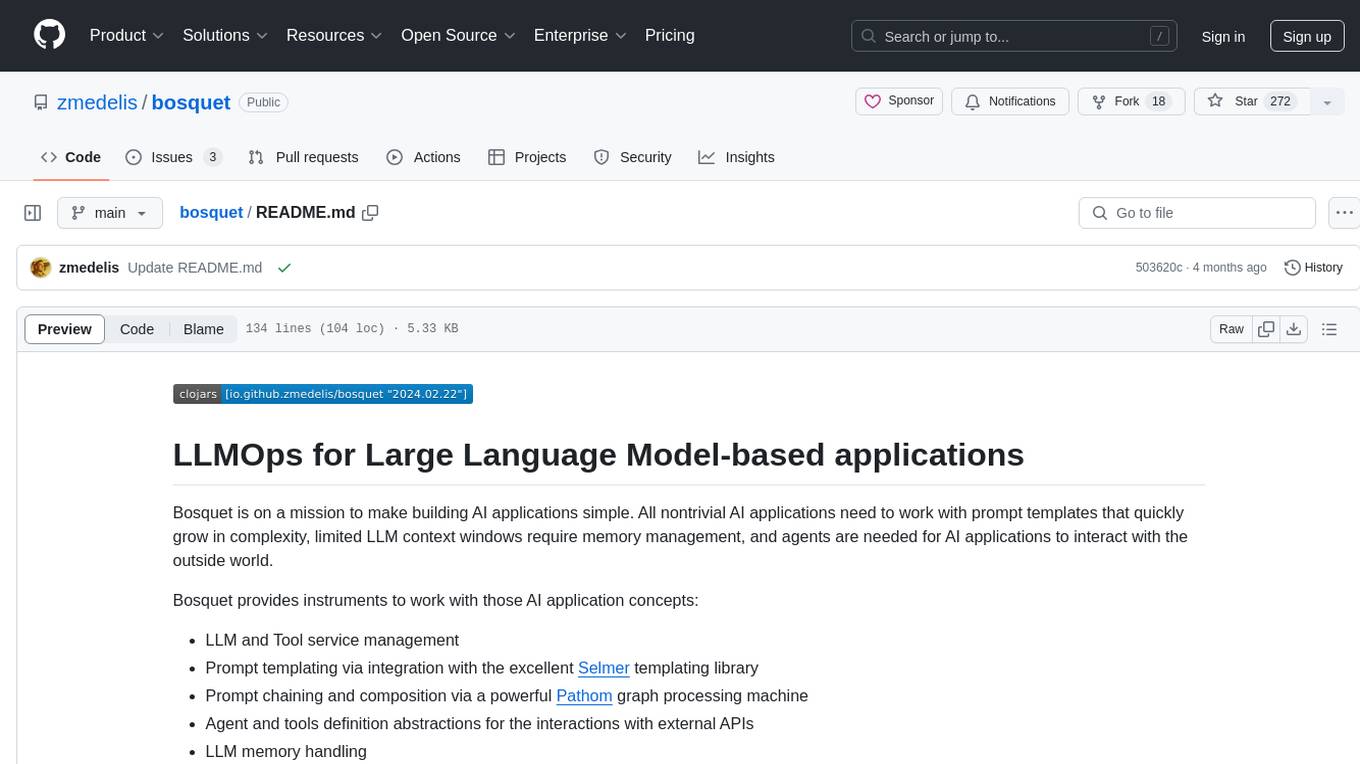
bosquet
Bosquet is a tool designed for LLMOps in large language model-based applications. It simplifies building AI applications by managing LLM and tool services, integrating with Selmer templating library for prompt templating, enabling prompt chaining and composition with Pathom graph processing, defining agents and tools for external API interactions, handling LLM memory, and providing features like call response caching. The tool aims to streamline the development process for AI applications that require complex prompt templates, memory management, and interaction with external systems.
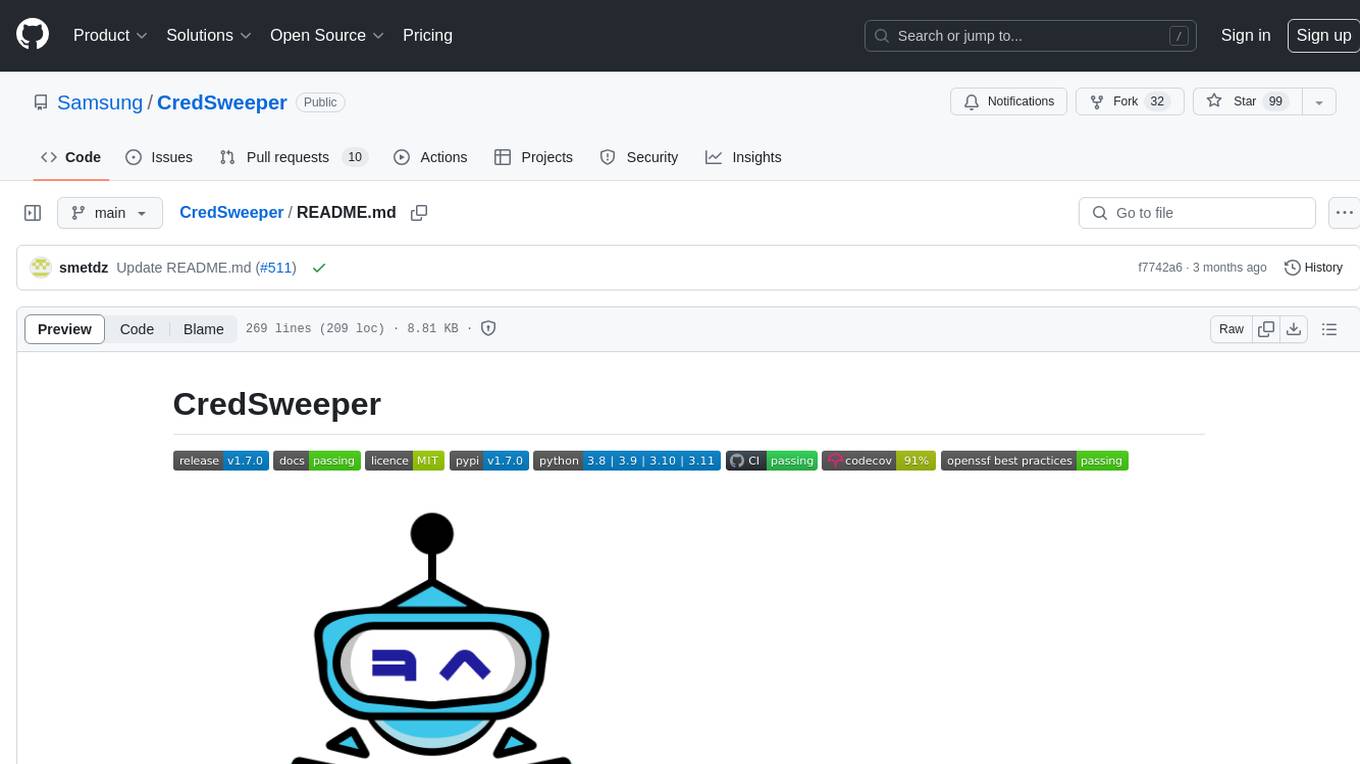
CredSweeper
CredSweeper is a tool designed to detect credentials like tokens, passwords, and API keys in directories or files. It helps users identify potential exposure of sensitive information by scanning lines, filtering, and utilizing an AI model. The tool reports lines containing possible credentials, their location, and the expected type of credential.
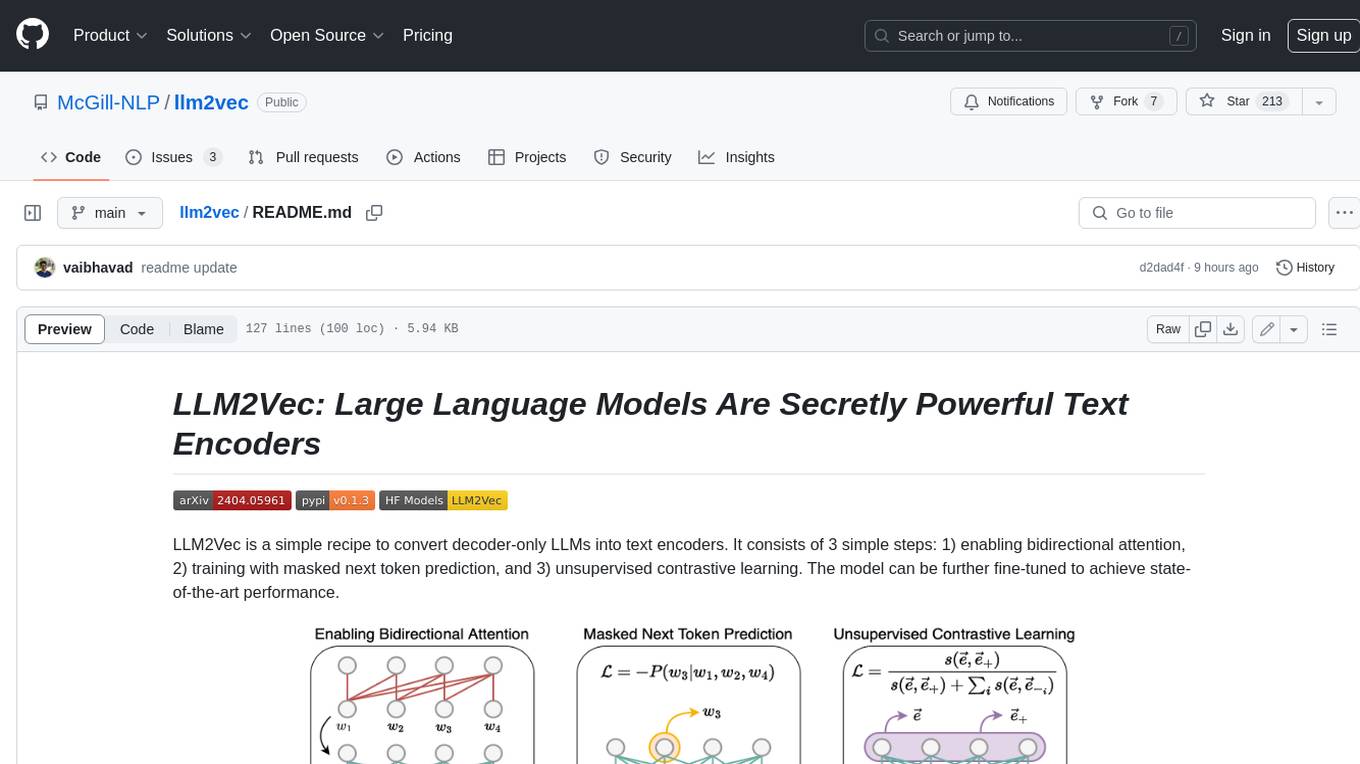
llm2vec
LLM2Vec is a simple recipe to convert decoder-only LLMs into text encoders. It consists of 3 simple steps: 1) enabling bidirectional attention, 2) training with masked next token prediction, and 3) unsupervised contrastive learning. The model can be further fine-tuned to achieve state-of-the-art performance.
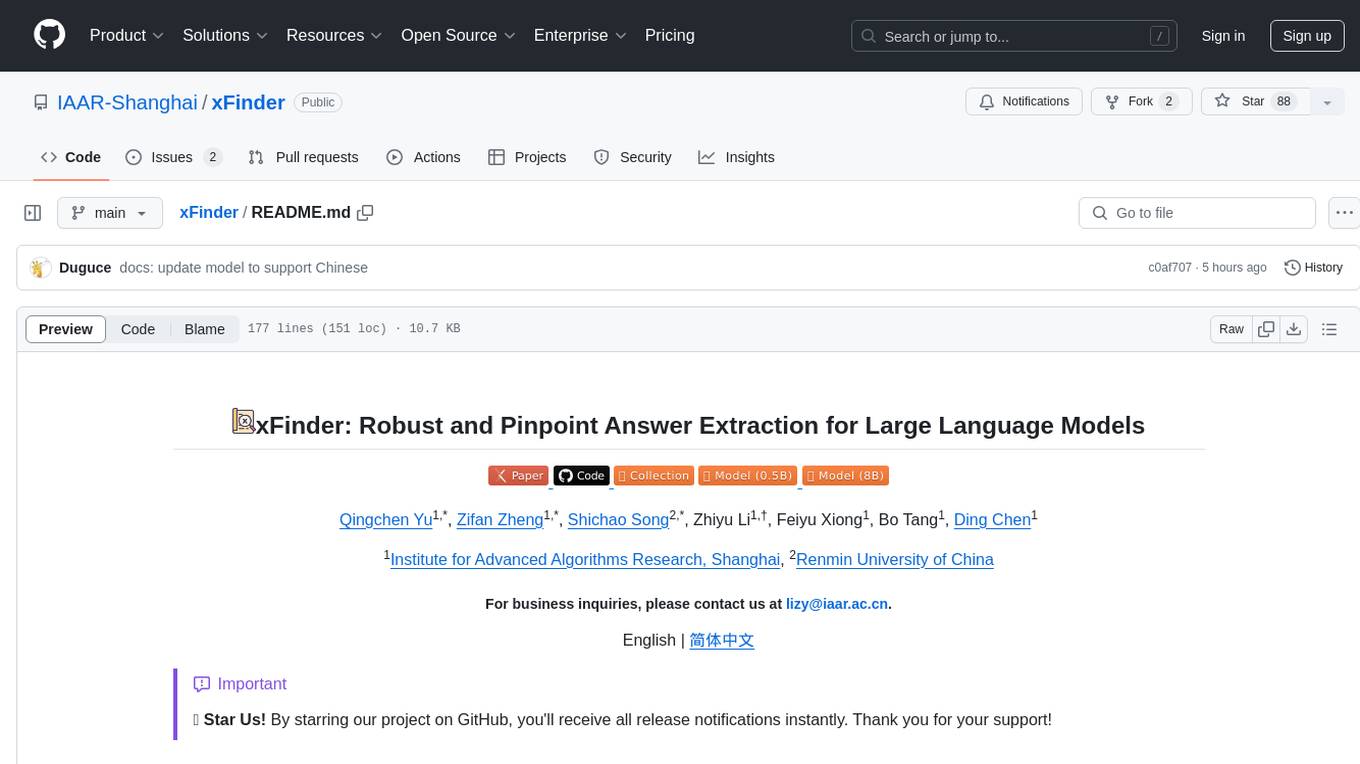
xFinder
xFinder is a model specifically designed for key answer extraction from large language models (LLMs). It addresses the challenges of unreliable evaluation methods by optimizing the key answer extraction module. The model achieves high accuracy and robustness compared to existing frameworks, enhancing the reliability of LLM evaluation. It includes a specialized dataset, the Key Answer Finder (KAF) dataset, for effective training and evaluation. xFinder is suitable for researchers and developers working with LLMs to improve answer extraction accuracy.
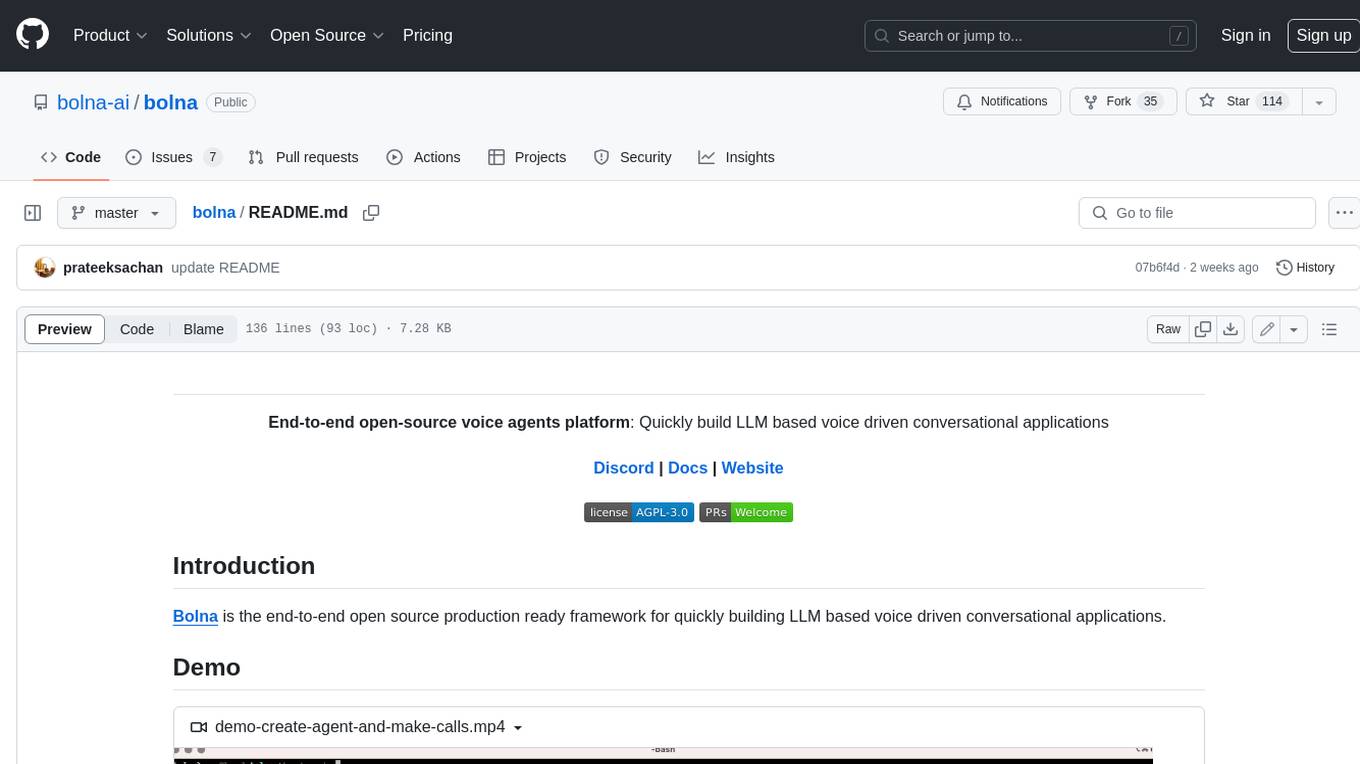
bolna
Bolna is an open-source platform for building voice-driven conversational applications using large language models (LLMs). It provides a comprehensive set of tools and integrations to handle various aspects of voice-based interactions, including telephony, transcription, LLM-based conversation handling, and text-to-speech synthesis. Bolna simplifies the process of creating voice agents that can perform tasks such as initiating phone calls, transcribing conversations, generating LLM-powered responses, and synthesizing speech. It supports multiple providers for each component, allowing users to customize their setup based on their specific needs. Bolna is designed to be easy to use, with a straightforward local setup process and well-documented APIs. It is also extensible, enabling users to integrate with other telephony providers or add custom functionality.
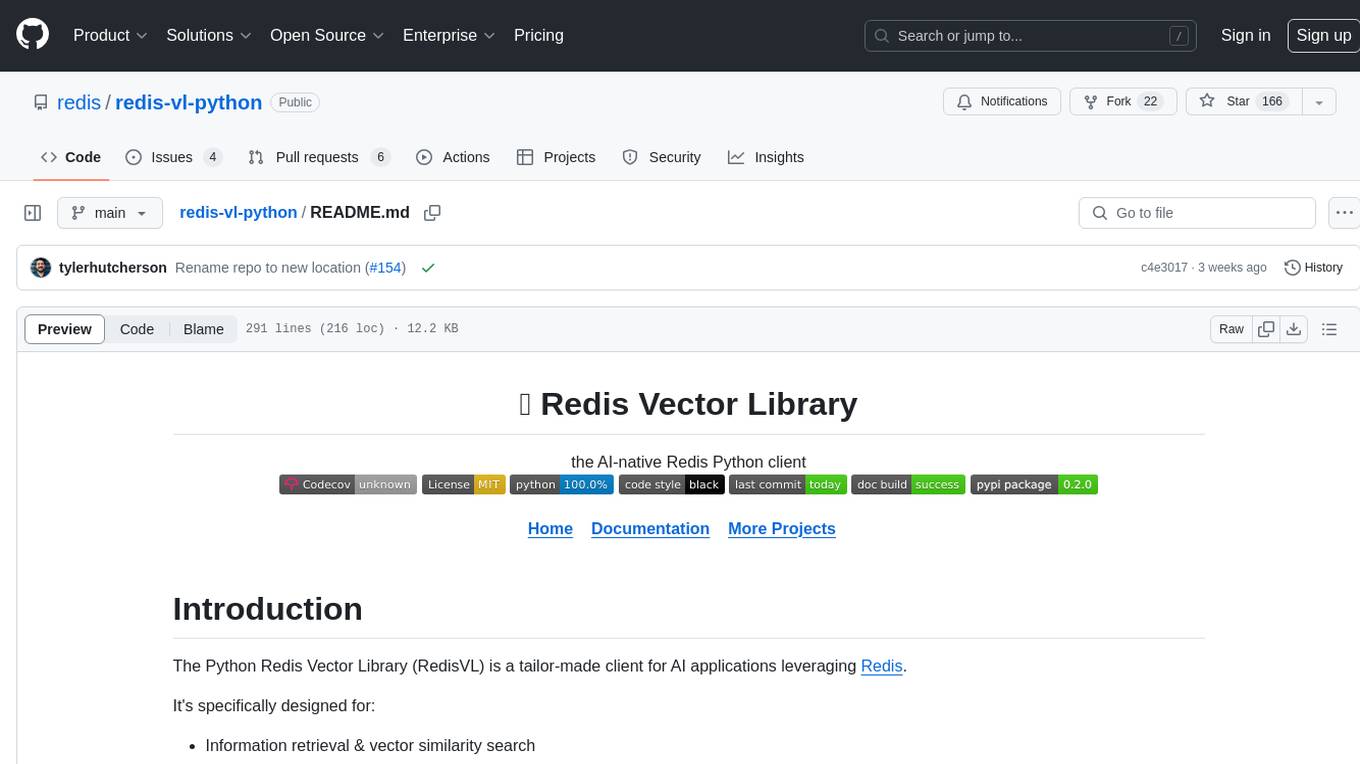
redis-vl-python
The Python Redis Vector Library (RedisVL) is a tailor-made client for AI applications leveraging Redis. It enhances applications with Redis' speed, flexibility, and reliability, incorporating capabilities like vector-based semantic search, full-text search, and geo-spatial search. The library bridges the gap between the emerging AI-native developer ecosystem and the capabilities of Redis by providing a lightweight, elegant, and intuitive interface. It abstracts the features of Redis into a grammar that is more aligned to the needs of today's AI/ML Engineers or Data Scientists.
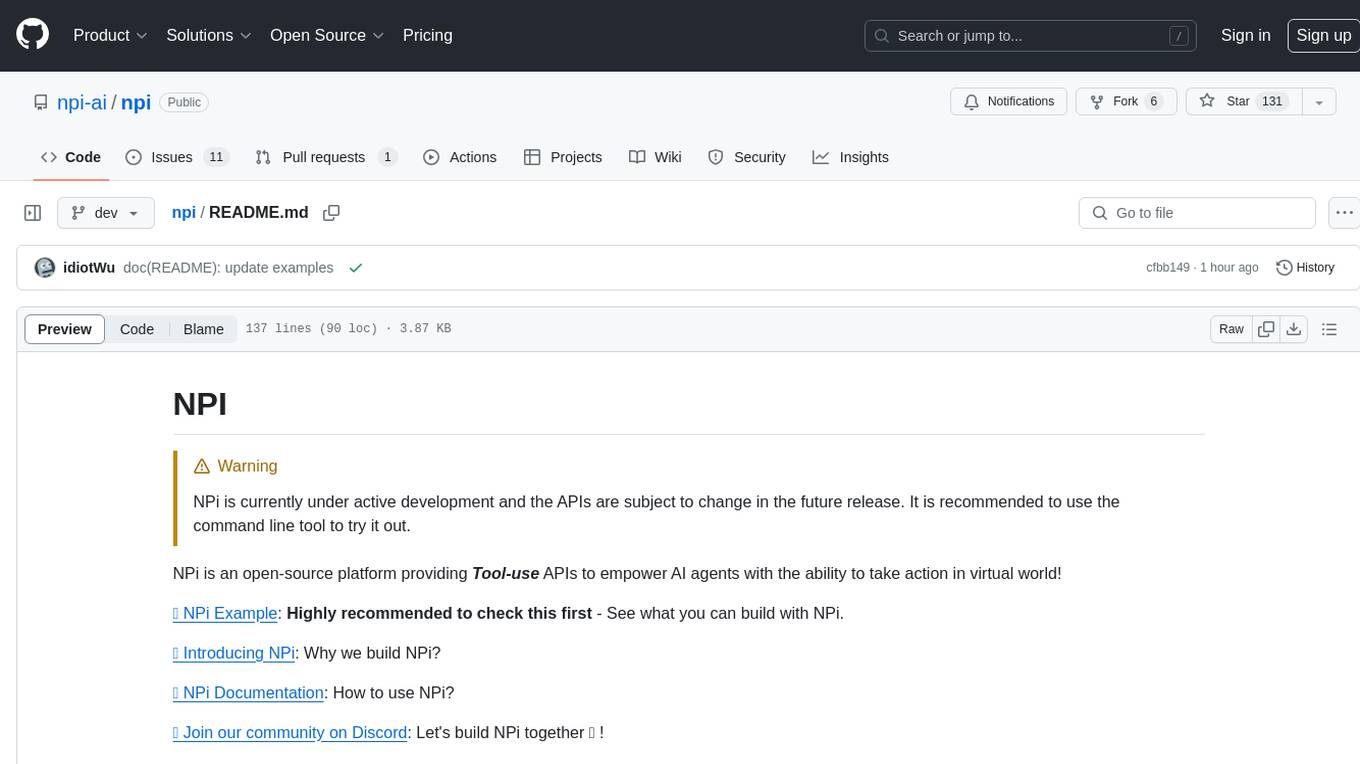
npi
NPi is an open-source platform providing Tool-use APIs to empower AI agents with the ability to take action in the virtual world. It is currently under active development, and the APIs are subject to change in future releases. NPi offers a command line tool for installation and setup, along with a GitHub app for easy access to repositories. The platform also includes a Python SDK and examples like Calendar Negotiator and Twitter Crawler. Join the NPi community on Discord to contribute to the development and explore the roadmap for future enhancements.
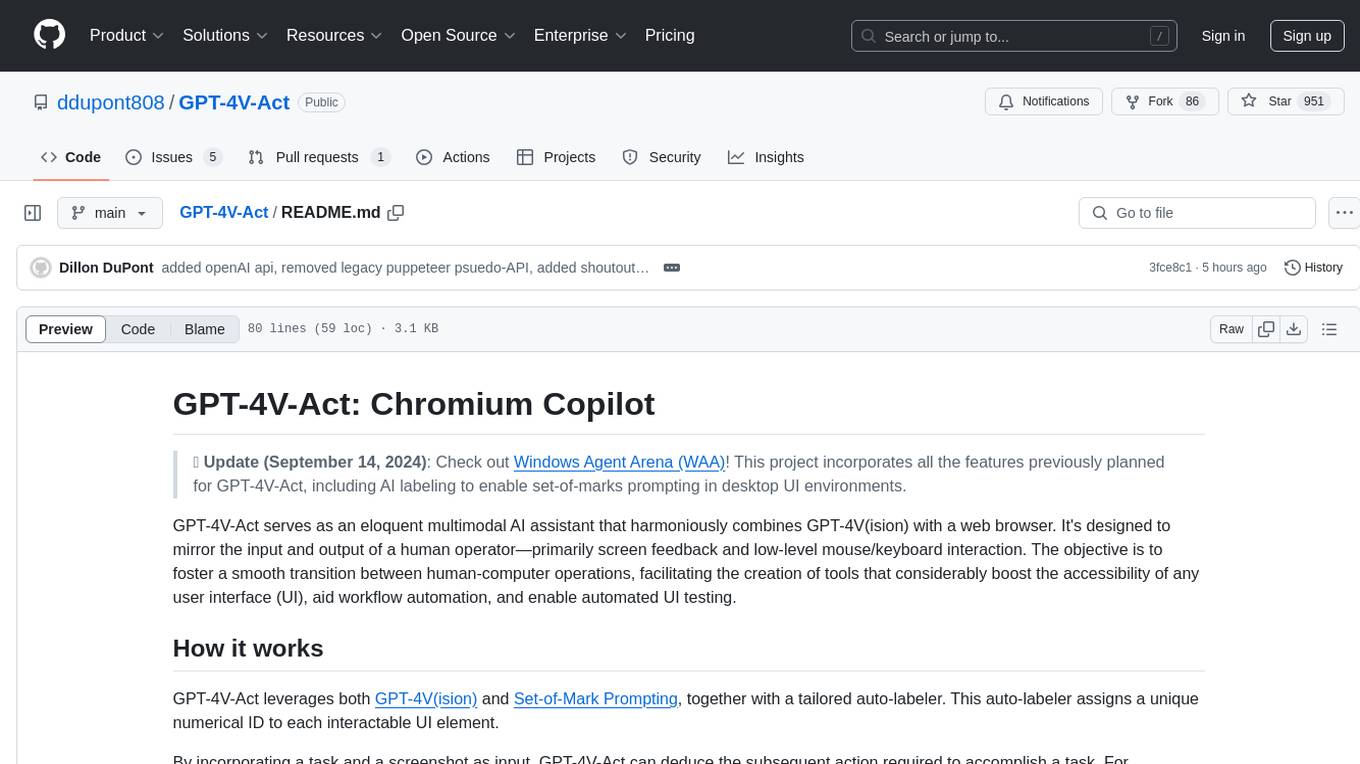
GPT-4V-Act
GPT-4V-Act is a multimodal AI assistant that combines GPT-4V(ision) with a web browser to mirror human operator input and output. It facilitates human-computer operations, boosts UI accessibility, aids workflow automation, and enables automated UI testing through AI labeling and set-of-marks prompting.
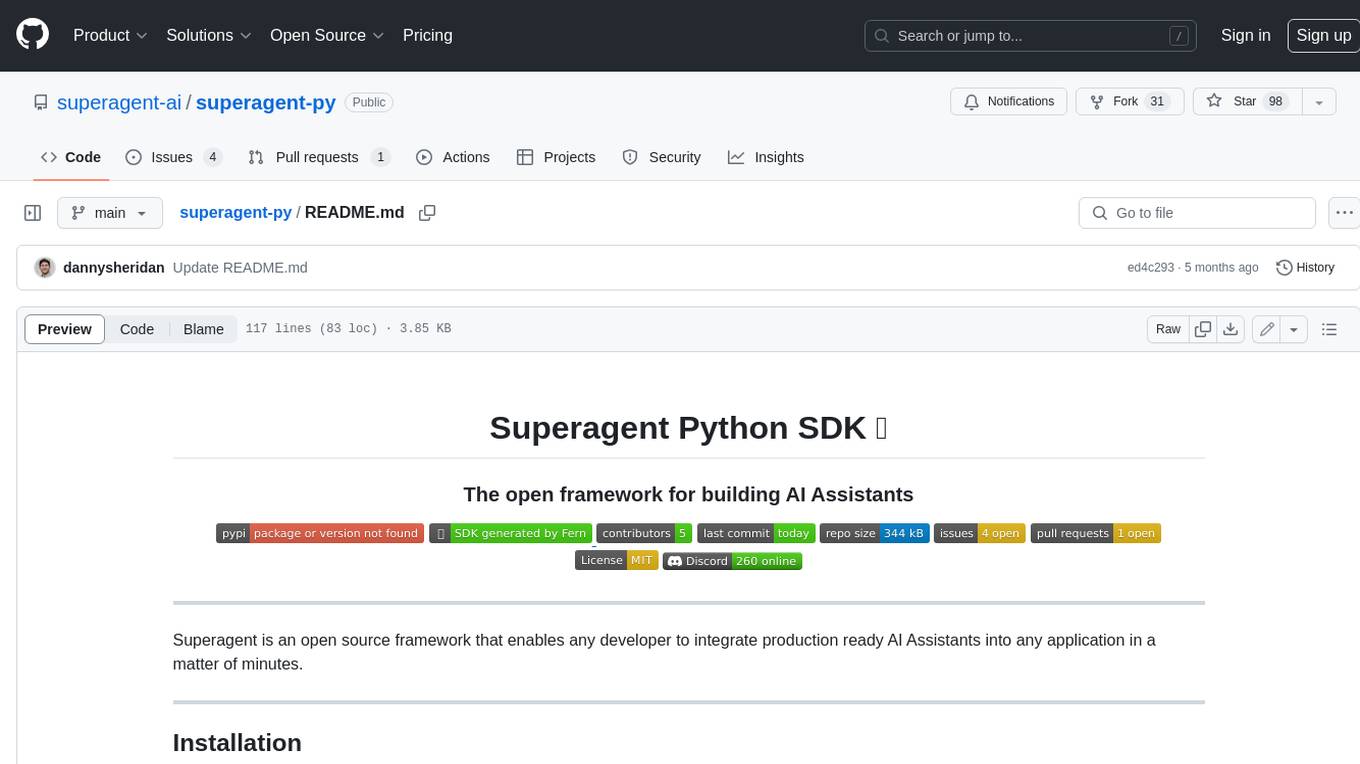
superagent-py
Superagent is an open-source framework that enables developers to integrate production-ready AI assistants into any application quickly and easily. It provides a Python SDK for interacting with the Superagent API, allowing developers to create, manage, and invoke AI agents. The SDK simplifies the process of building AI-powered applications, making it accessible to developers of all skill levels.
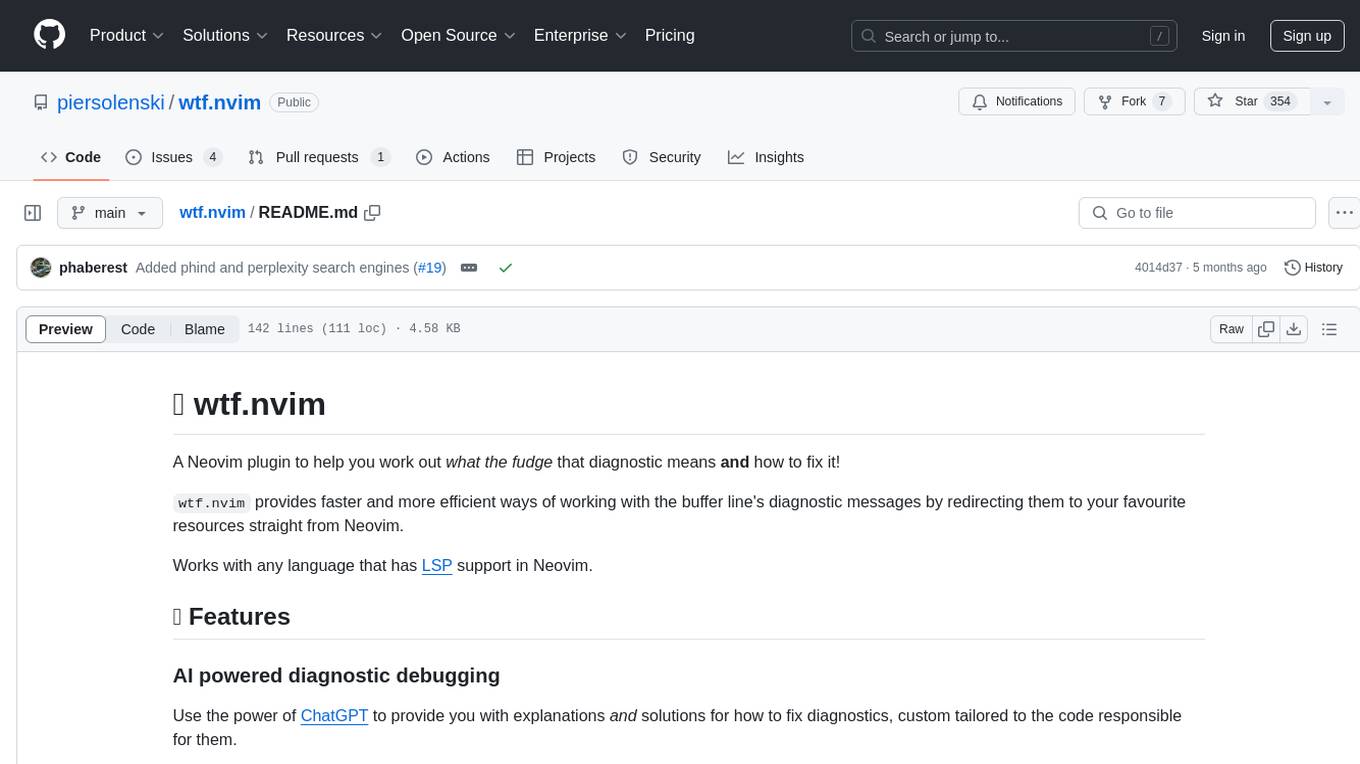
wtf.nvim
wtf.nvim is a Neovim plugin that enhances diagnostic debugging by providing explanations and solutions for code issues using ChatGPT. It allows users to search the web for answers directly from Neovim, making the debugging process faster and more efficient. The plugin works with any language that has LSP support in Neovim, offering AI-powered diagnostic assistance and seamless integration with various resources for resolving coding problems.
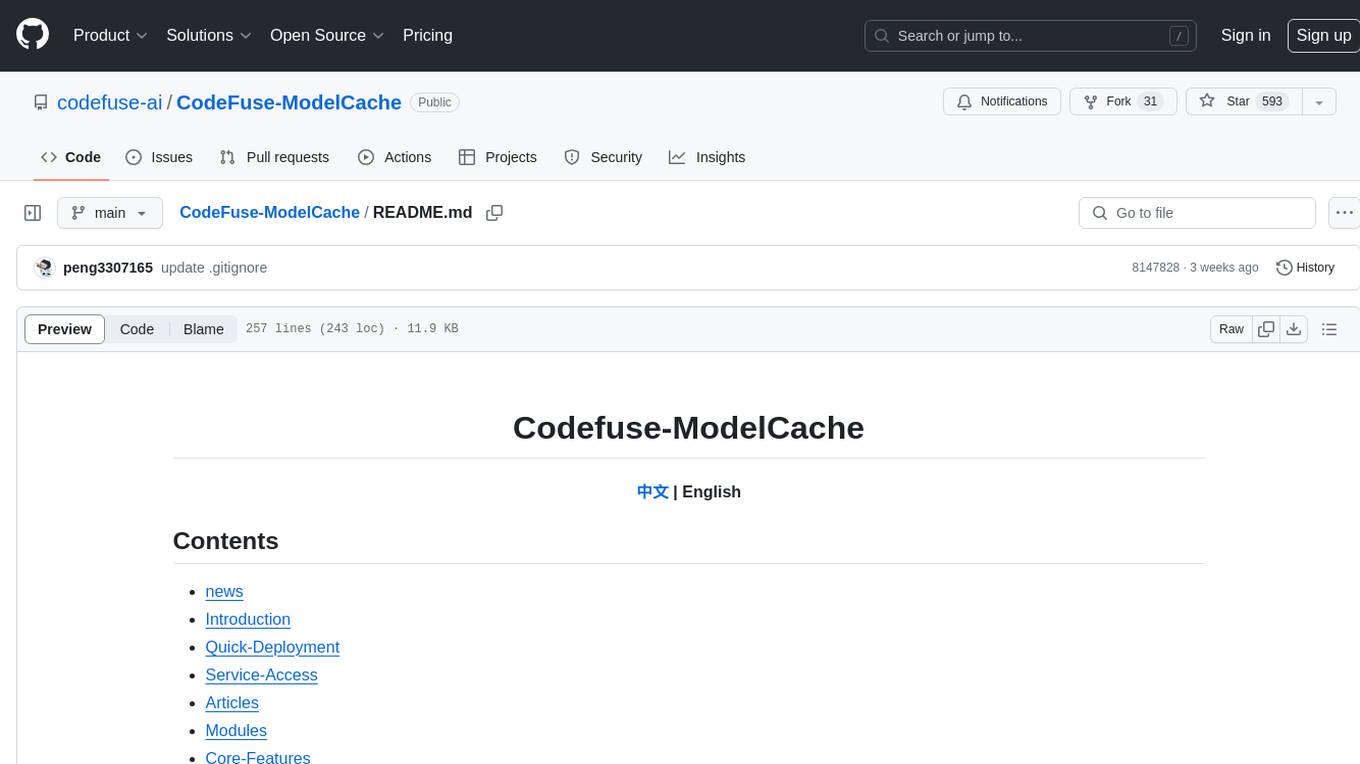
CodeFuse-ModelCache
Codefuse-ModelCache is a semantic cache for large language models (LLMs) that aims to optimize services by introducing a caching mechanism. It helps reduce the cost of inference deployment, improve model performance and efficiency, and provide scalable services for large models. The project caches pre-generated model results to reduce response time for similar requests and enhance user experience. It integrates various embedding frameworks and local storage options, offering functionalities like cache-writing, cache-querying, and cache-clearing through RESTful API. The tool supports multi-tenancy, system commands, and multi-turn dialogue, with features for data isolation, database management, and model loading schemes. Future developments include data isolation based on hyperparameters, enhanced system prompt partitioning storage, and more versatile embedding models and similarity evaluation algorithms.
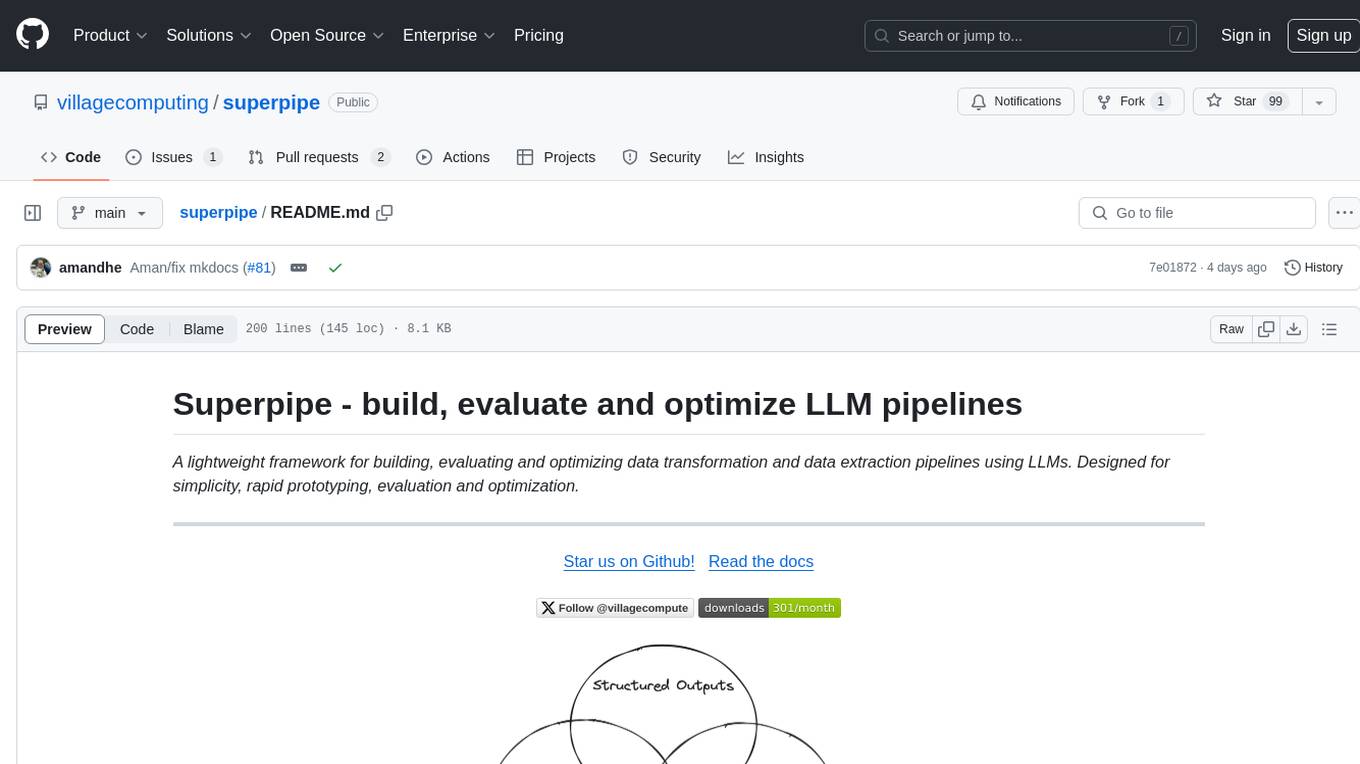
superpipe
Superpipe is a lightweight framework designed for building, evaluating, and optimizing data transformation and data extraction pipelines using LLMs. It allows users to easily combine their favorite LLM libraries with Superpipe's building blocks to create pipelines tailored to their unique data and use cases. The tool facilitates rapid prototyping, evaluation, and optimization of end-to-end pipelines for tasks such as classification and evaluation of job departments based on work history. Superpipe also provides functionalities for evaluating pipeline performance, optimizing parameters for cost, accuracy, and speed, and conducting grid searches to experiment with different models and prompts.
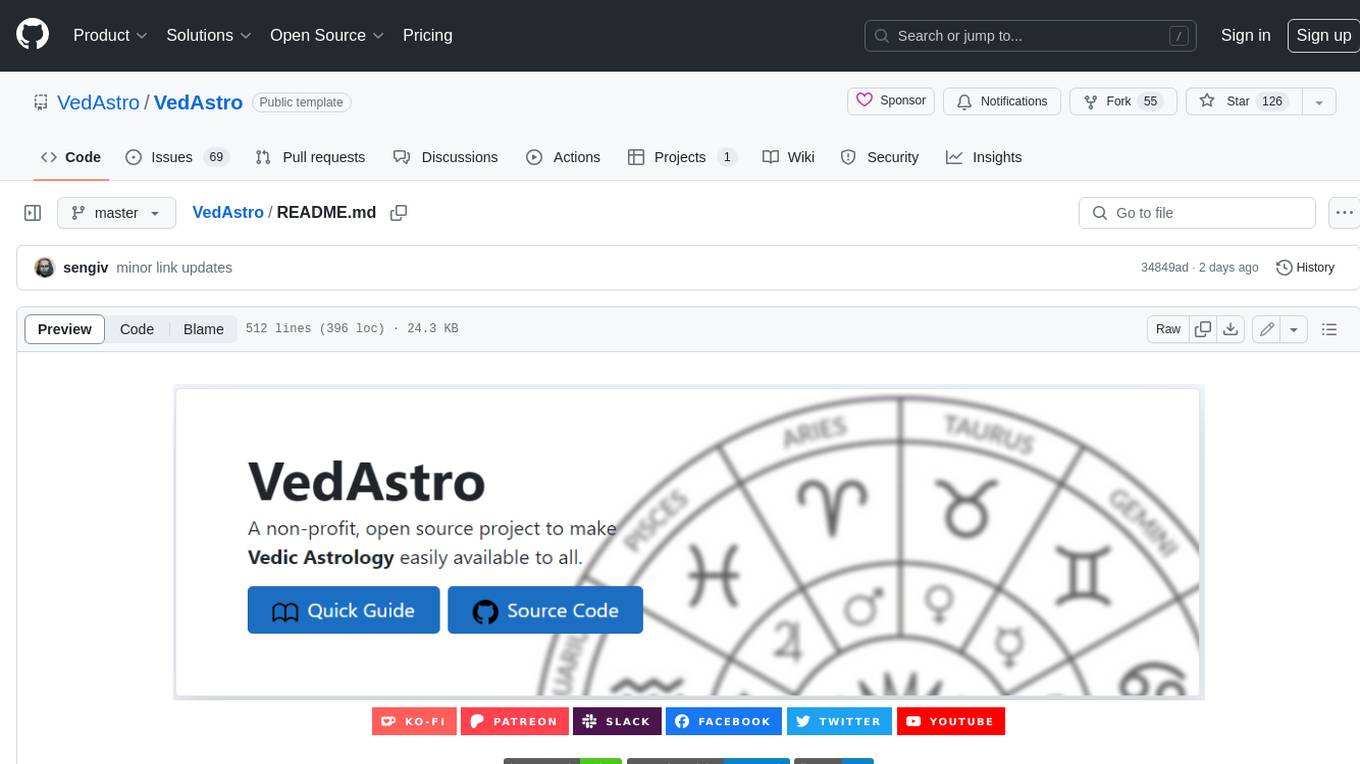
VedAstro
VedAstro is an open-source Vedic astrology tool that provides accurate astrological predictions and data. It offers a user-friendly website, a chat API, an open API, a JavaScript SDK, a Swiss Ephemeris API, and a machine learning table generator. VedAstro is free to use and is constantly being updated with new features and improvements.
For similar tasks
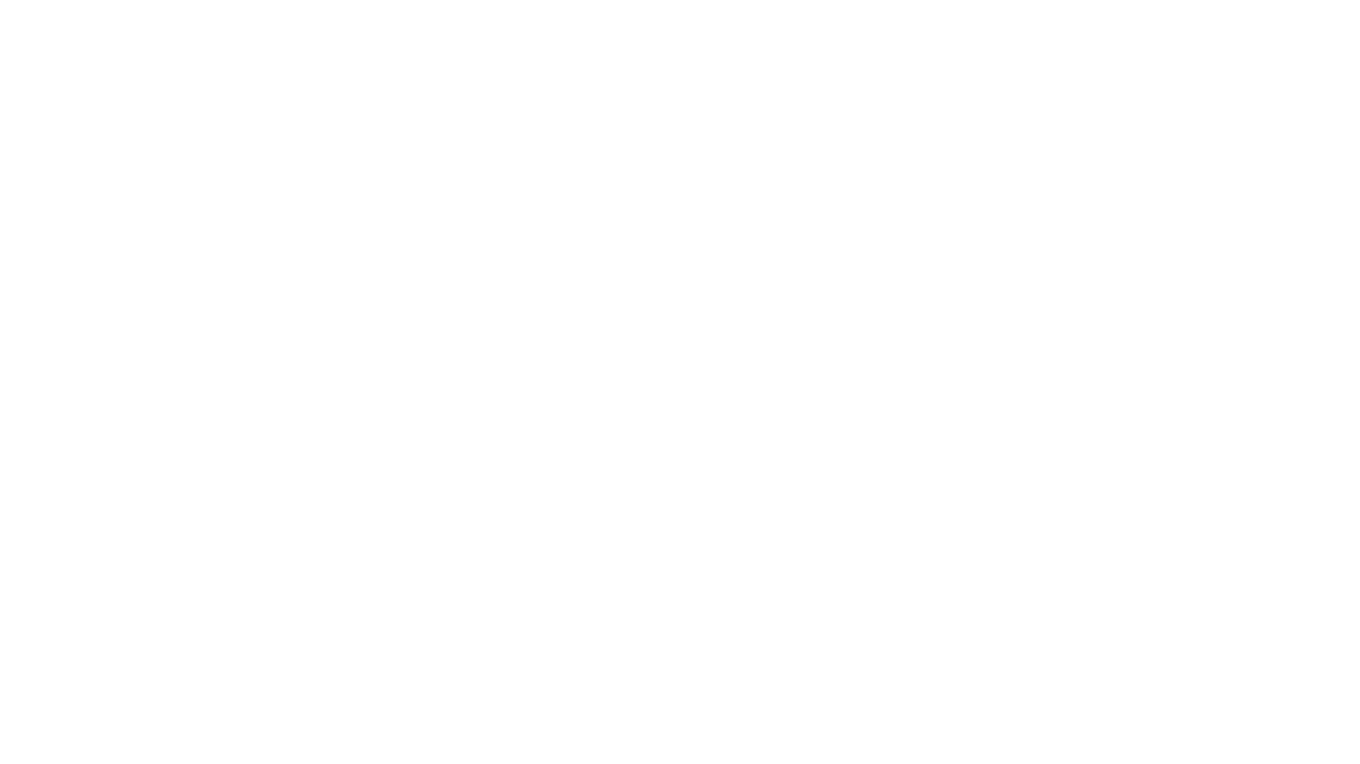
llm-structured-output
This repository contains a library for constraining LLM generation to structured output, enforcing a JSON schema for precise data types and property names. It includes an acceptor/state machine framework, JSON acceptor, and JSON schema acceptor for guiding decoding in LLMs. The library provides reference implementations using Apple's MLX library and examples for function calling tasks. The tool aims to improve LLM output quality by ensuring adherence to a schema, reducing unnecessary output, and enhancing performance through pre-emptive decoding. Evaluations show performance benchmarks and comparisons with and without schema constraints.
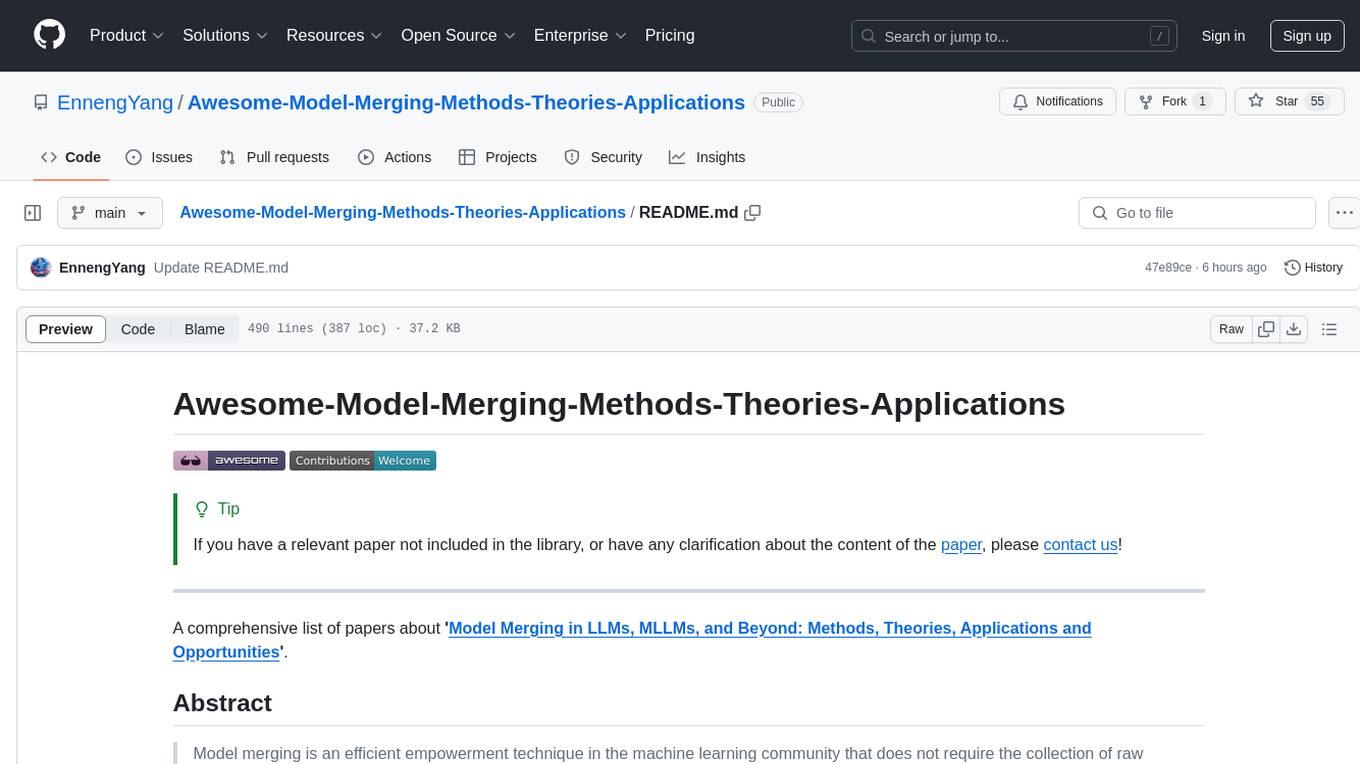
Awesome-Model-Merging-Methods-Theories-Applications
A comprehensive repository focusing on 'Model Merging in LLMs, MLLMs, and Beyond', providing an exhaustive overview of model merging methods, theories, applications, and future research directions. The repository covers various advanced methods, applications in foundation models, different machine learning subfields, and tasks like pre-merging methods, architecture transformation, weight alignment, basic merging methods, and more.
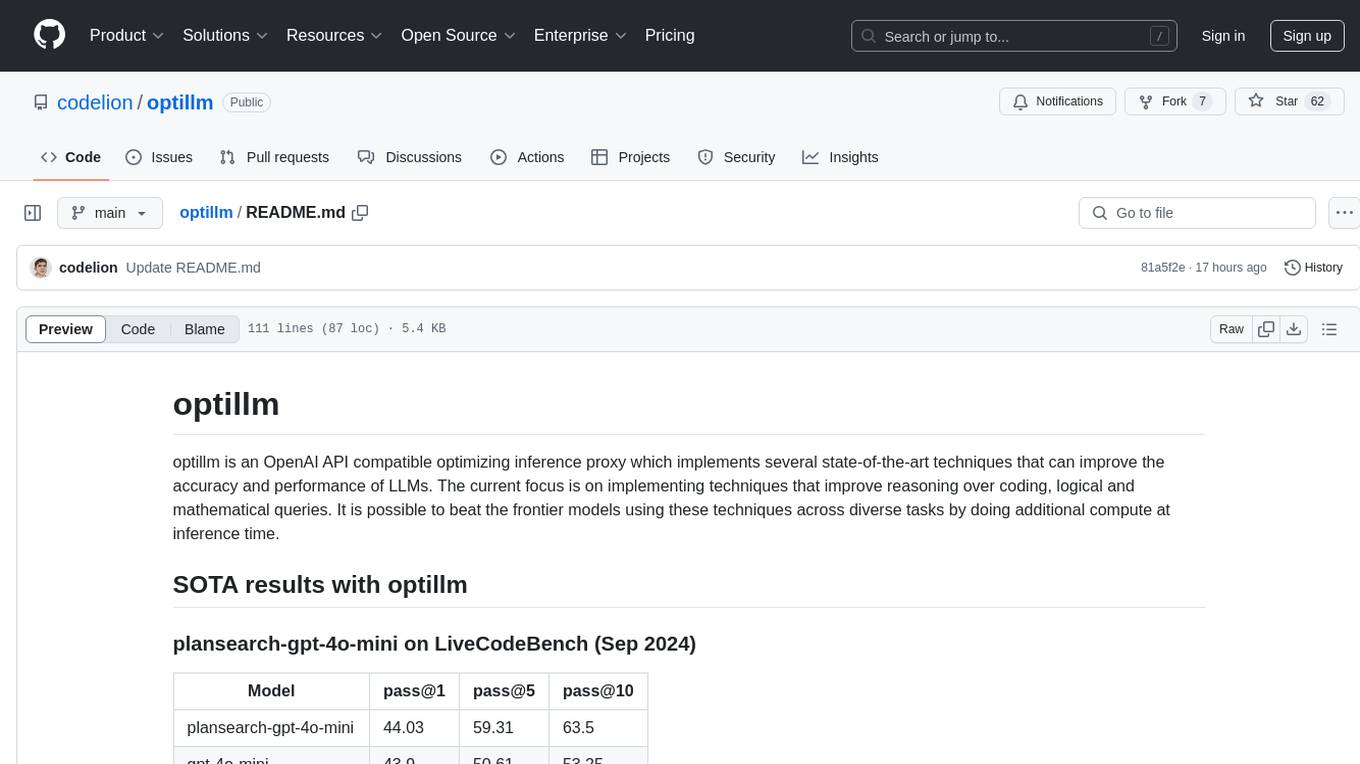
optillm
optillm is an OpenAI API compatible optimizing inference proxy implementing state-of-the-art techniques to enhance accuracy and performance of LLMs, focusing on reasoning over coding, logical, and mathematical queries. By leveraging additional compute at inference time, it surpasses frontier models across diverse tasks.
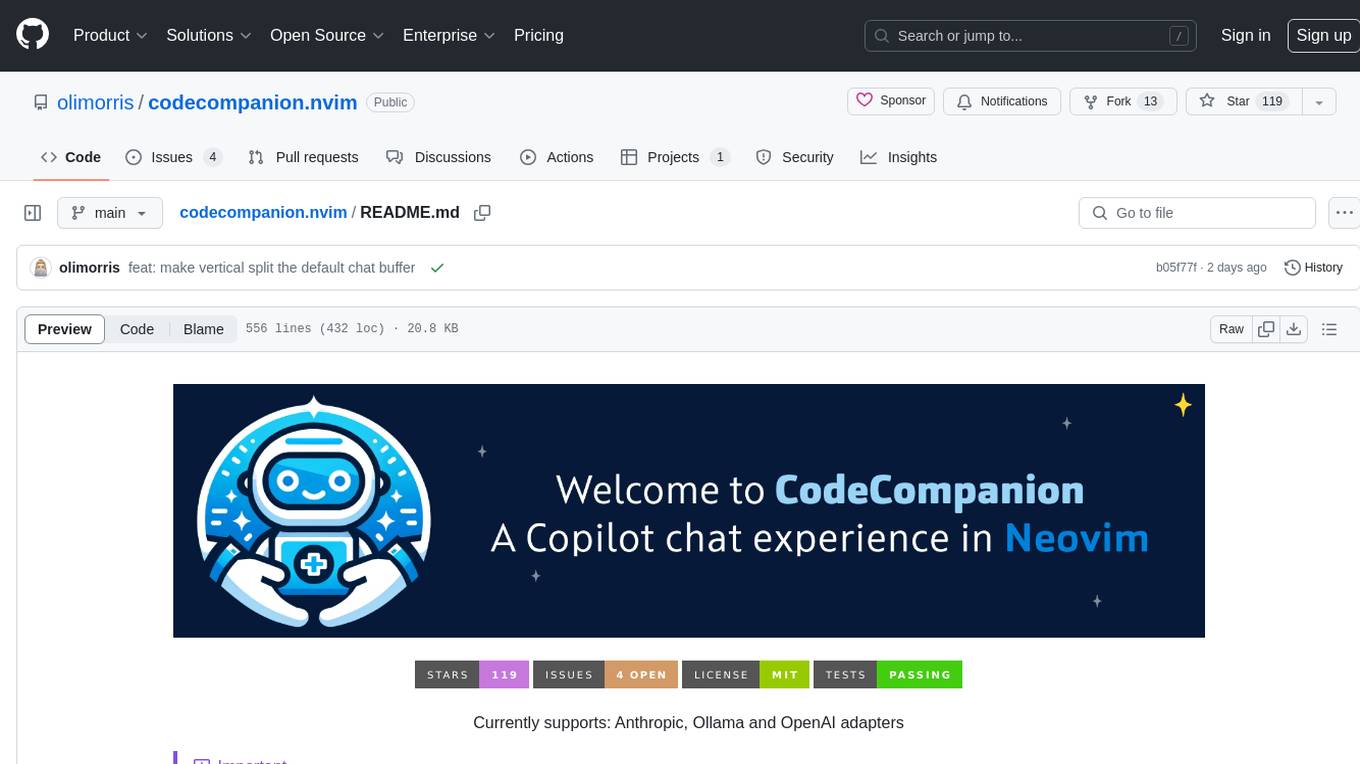
codecompanion.nvim
CodeCompanion.nvim is a Neovim plugin that provides a Copilot Chat experience, adapter support for various LLMs, agentic workflows, inline code creation and modification, built-in actions for language prompts and error fixes, custom actions creation, async execution, and more. It supports Anthropic, Ollama, and OpenAI adapters. The plugin is primarily developed for personal workflows with no guarantees of regular updates or support. Users can customize the plugin to their needs by forking the project.
For similar jobs
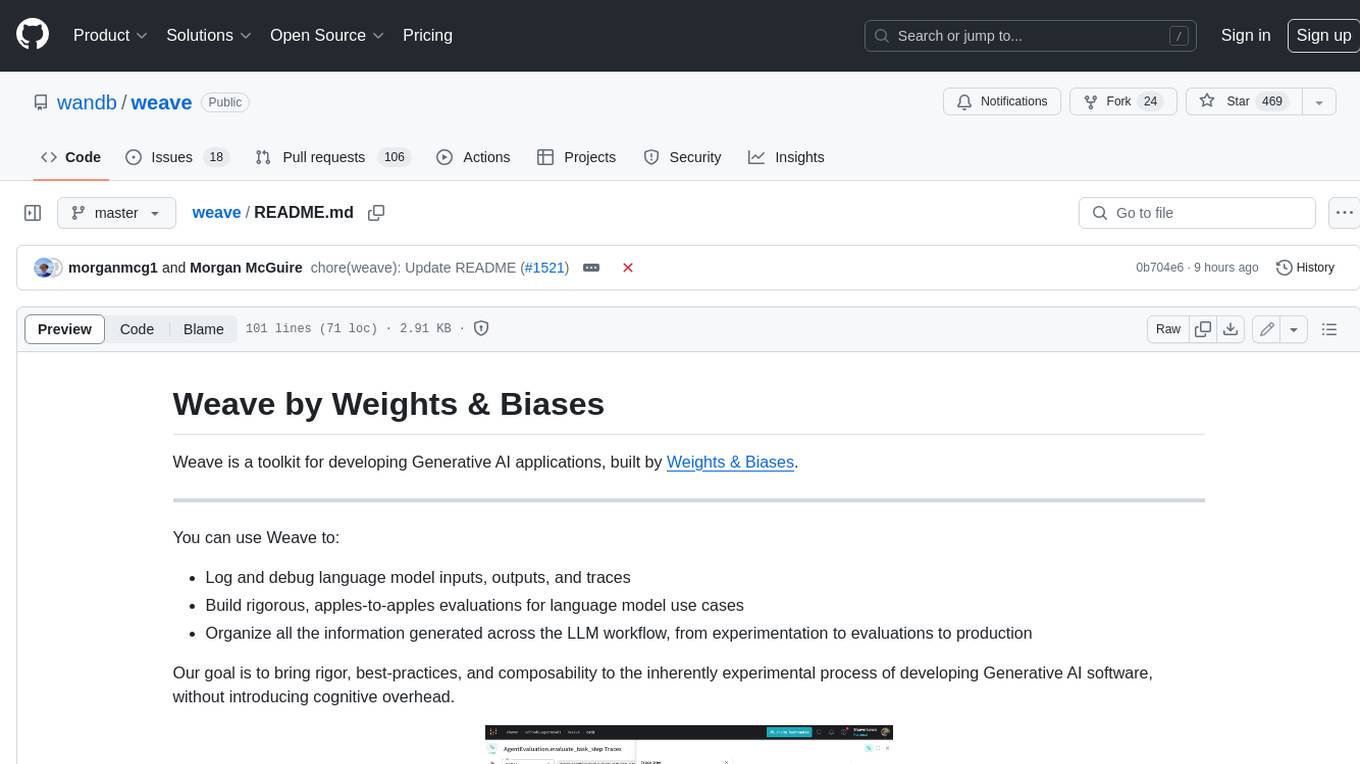
weave
Weave is a toolkit for developing Generative AI applications, built by Weights & Biases. With Weave, you can log and debug language model inputs, outputs, and traces; build rigorous, apples-to-apples evaluations for language model use cases; and organize all the information generated across the LLM workflow, from experimentation to evaluations to production. Weave aims to bring rigor, best-practices, and composability to the inherently experimental process of developing Generative AI software, without introducing cognitive overhead.
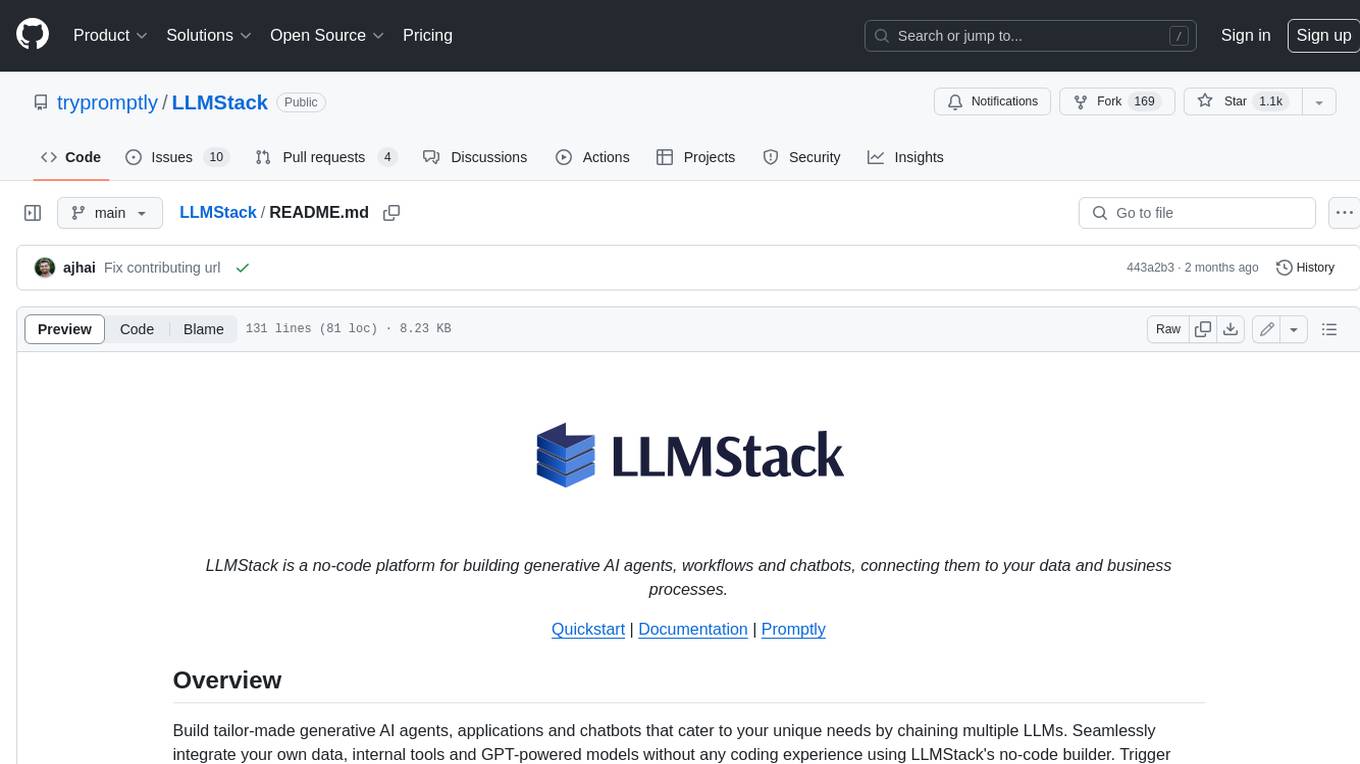
LLMStack
LLMStack is a no-code platform for building generative AI agents, workflows, and chatbots. It allows users to connect their own data, internal tools, and GPT-powered models without any coding experience. LLMStack can be deployed to the cloud or on-premise and can be accessed via HTTP API or triggered from Slack or Discord.
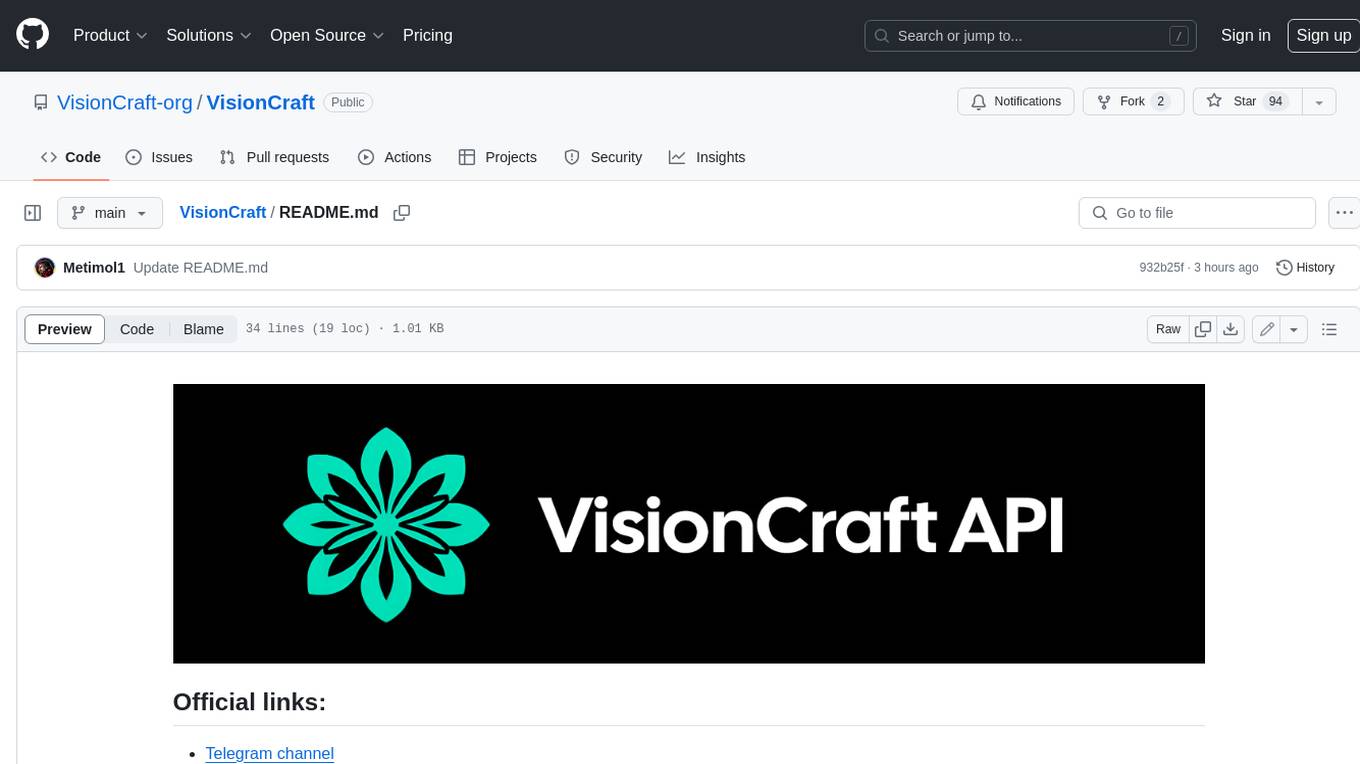
VisionCraft
The VisionCraft API is a free API for using over 100 different AI models. From images to sound.
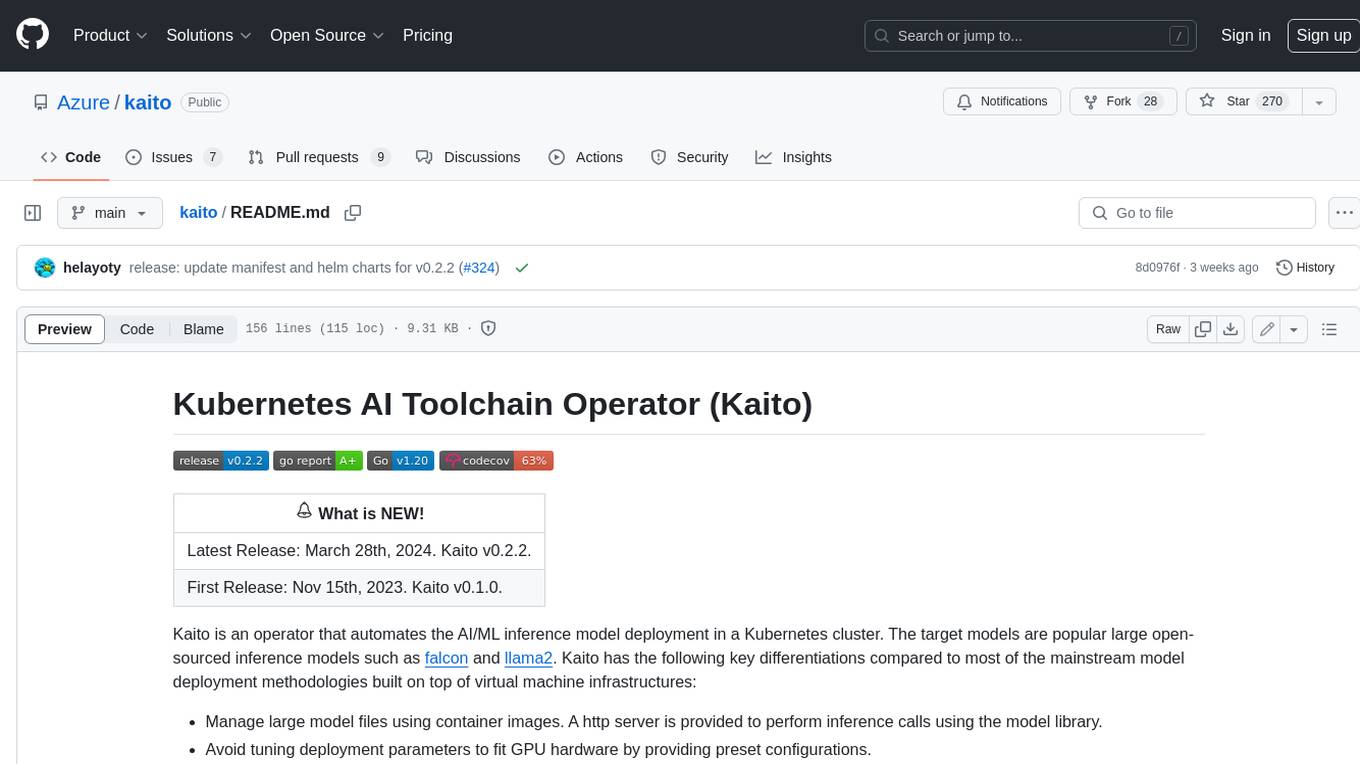
kaito
Kaito is an operator that automates the AI/ML inference model deployment in a Kubernetes cluster. It manages large model files using container images, avoids tuning deployment parameters to fit GPU hardware by providing preset configurations, auto-provisions GPU nodes based on model requirements, and hosts large model images in the public Microsoft Container Registry (MCR) if the license allows. Using Kaito, the workflow of onboarding large AI inference models in Kubernetes is largely simplified.
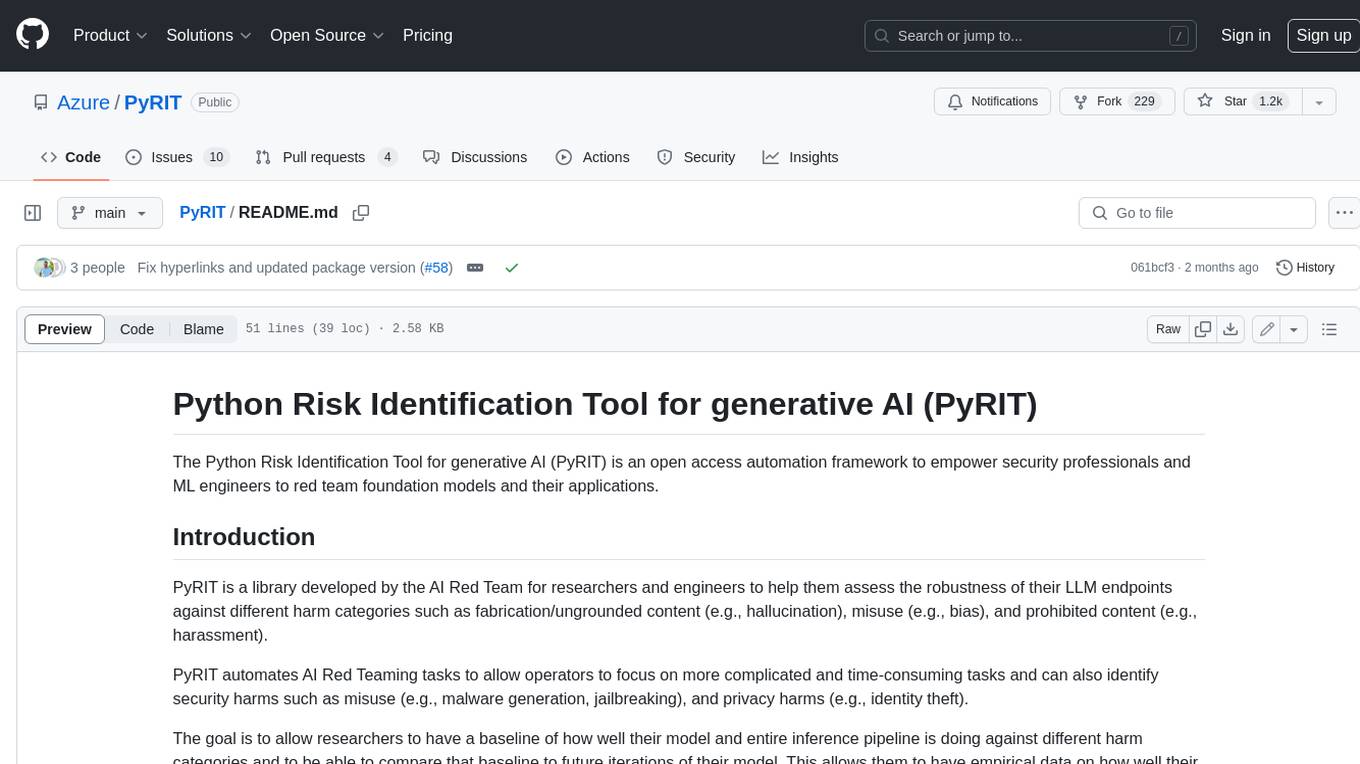
PyRIT
PyRIT is an open access automation framework designed to empower security professionals and ML engineers to red team foundation models and their applications. It automates AI Red Teaming tasks to allow operators to focus on more complicated and time-consuming tasks and can also identify security harms such as misuse (e.g., malware generation, jailbreaking), and privacy harms (e.g., identity theft). The goal is to allow researchers to have a baseline of how well their model and entire inference pipeline is doing against different harm categories and to be able to compare that baseline to future iterations of their model. This allows them to have empirical data on how well their model is doing today, and detect any degradation of performance based on future improvements.
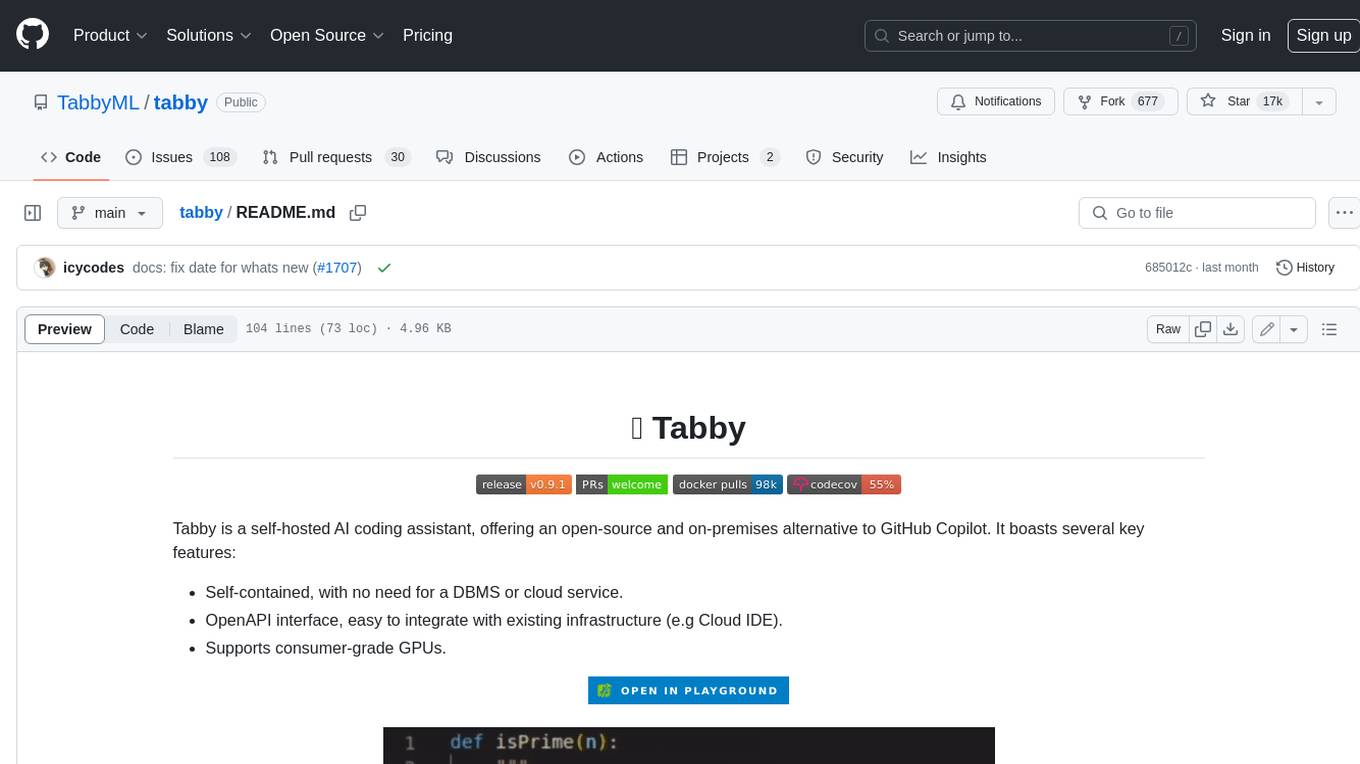
tabby
Tabby is a self-hosted AI coding assistant, offering an open-source and on-premises alternative to GitHub Copilot. It boasts several key features: * Self-contained, with no need for a DBMS or cloud service. * OpenAPI interface, easy to integrate with existing infrastructure (e.g Cloud IDE). * Supports consumer-grade GPUs.
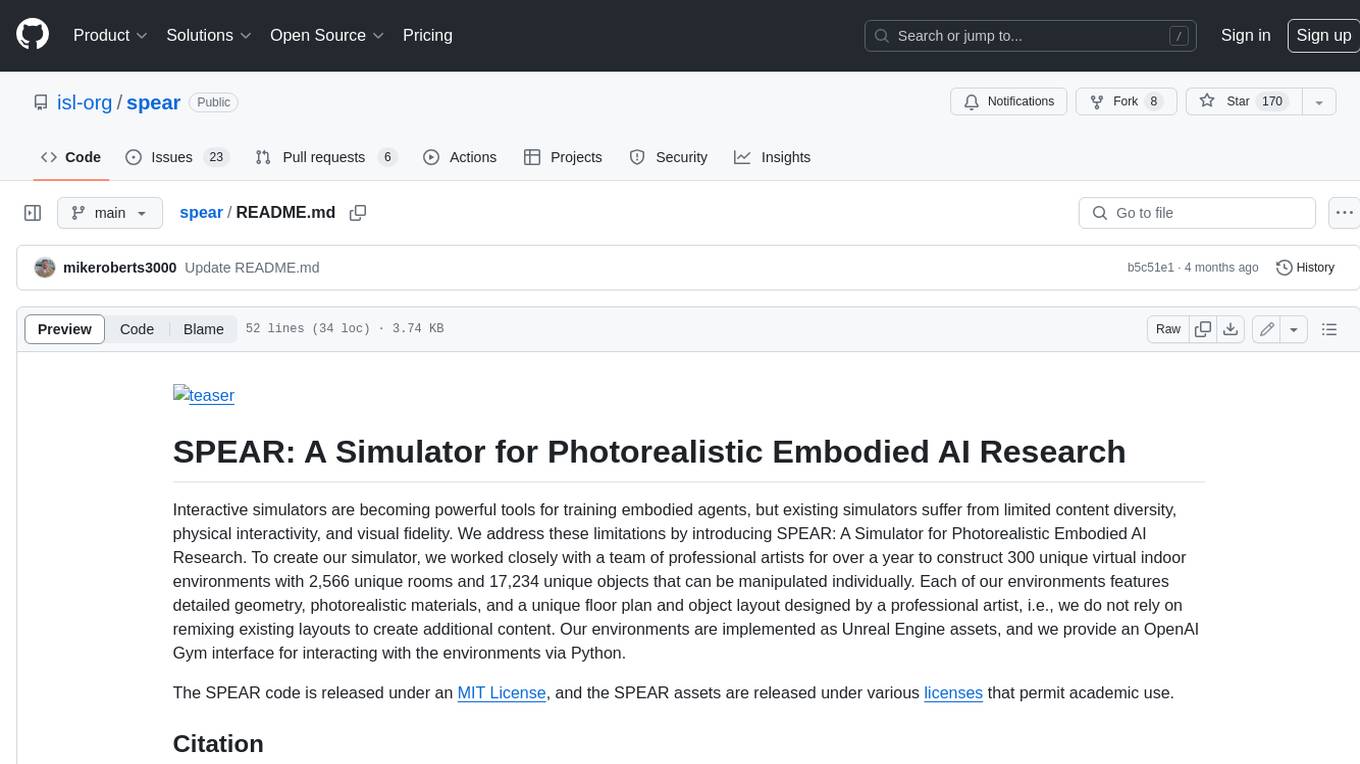
spear
SPEAR (Simulator for Photorealistic Embodied AI Research) is a powerful tool for training embodied agents. It features 300 unique virtual indoor environments with 2,566 unique rooms and 17,234 unique objects that can be manipulated individually. Each environment is designed by a professional artist and features detailed geometry, photorealistic materials, and a unique floor plan and object layout. SPEAR is implemented as Unreal Engine assets and provides an OpenAI Gym interface for interacting with the environments via Python.
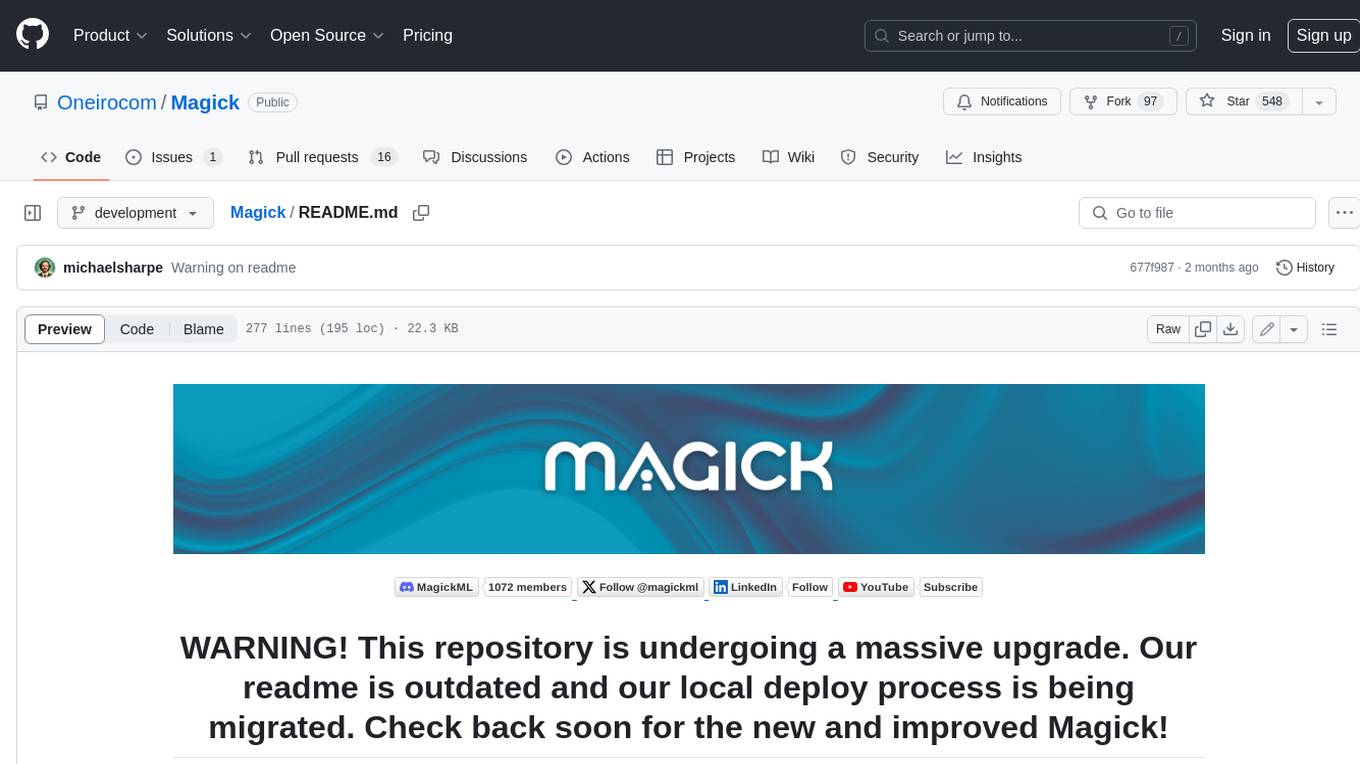
Magick
Magick is a groundbreaking visual AIDE (Artificial Intelligence Development Environment) for no-code data pipelines and multimodal agents. Magick can connect to other services and comes with nodes and templates well-suited for intelligent agents, chatbots, complex reasoning systems and realistic characters.