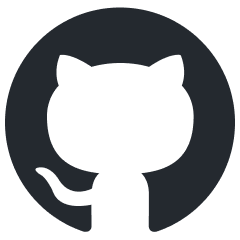
Phi-3CookBook
This is a Phi-3 book for getting started with Phi-3. Phi-3, a family of open sourced AI models developed by Microsoft. Phi-3 models are the most capable and cost-effective small language models (SLMs) available, outperforming models of the same size and next size up across a variety of language, reasoning, coding, and math benchmarks.
Stars: 2311
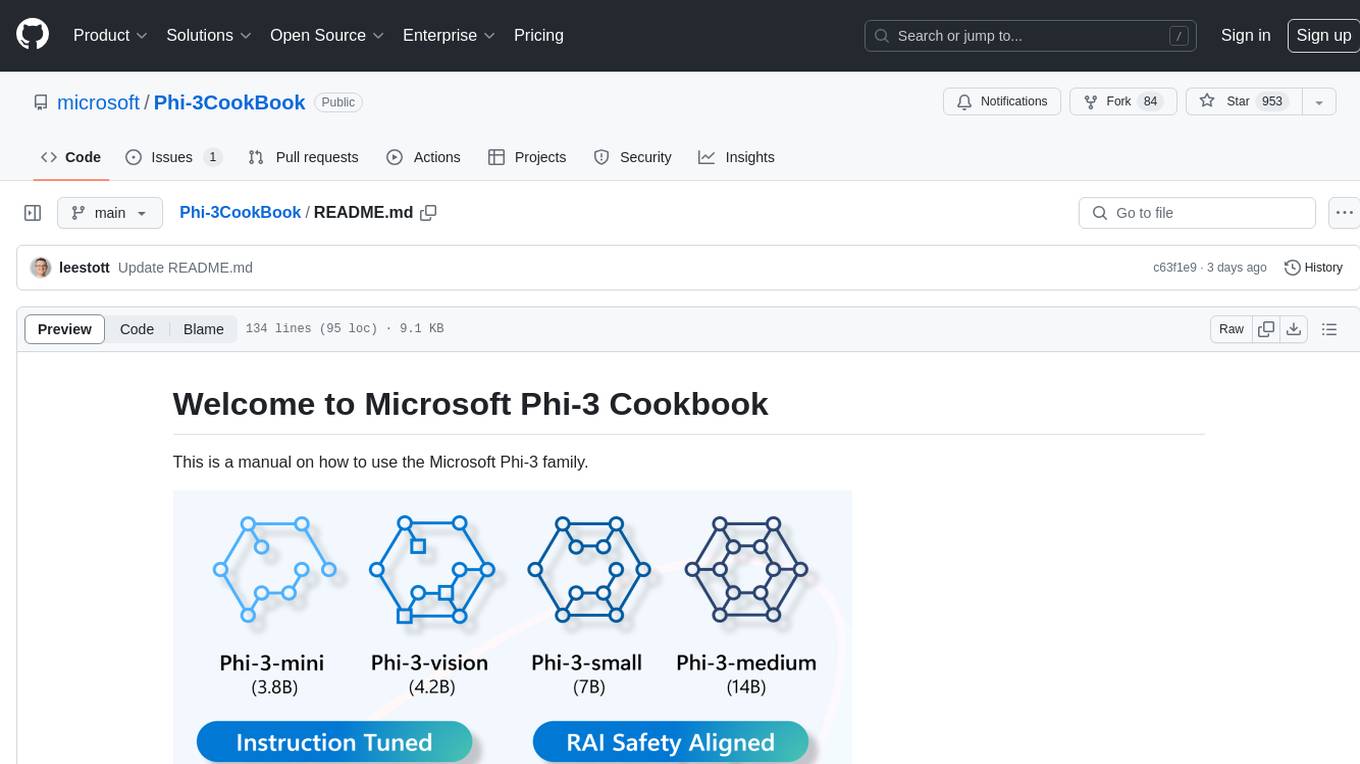
Phi-3CookBook is a manual on how to use the Microsoft Phi-3 family, which consists of open AI models developed by Microsoft. The Phi-3 models are highly capable and cost-effective small language models, outperforming models of similar and larger sizes across various language, reasoning, coding, and math benchmarks. The repository provides detailed information on different Phi-3 models, their performance, availability, and usage scenarios across different platforms like Azure AI Studio, Hugging Face, and Ollama. It also covers topics such as fine-tuning, evaluation, and end-to-end samples for Phi-3-mini and Phi-3-vision models, along with labs, workshops, and contributing guidelines.
README:
Phi, is a family of open AI models developed by Microsoft. Phi models are the most capable and cost-effective small language models (SLMs) available, outperforming models of the same size and next size up across a variety of language, reasoning, coding, and math benchmarks. The Phi-3 Family includes mini, small, medium and vision versions, trained based on different parameter amounts to serve various application scenarios. For more detailed information about Microsoft's Phi family, please visit the Welcome to the Phi Family page.
-
Introduction
-
Quick Start
- Using Phi-3 in GitHub Model Catalog(✅)
- Using Phi-3 in Hugging face(✅)
- Using Phi-3 with OpenAI SDK(✅)
- Using Phi-3 with Http Requests(✅)
- Using Phi-3 in Azure AI Studio(✅)
- Using Phi-3 Model Inference with Azure MaaS or MaaP(✅)
- Deploying Phi-3 models as serverless APIs in Azure AI Studio(✅)
- Using Phi-3 in Ollama(✅)
- Using Phi-3 in LM Studio(✅)
- Using Phi-3 in AI Toolkit VSCode(✅)
- Using Phi-3 and LiteLLM(✅)
-
- Inference Phi-3 in iOS(✅)
- Inference Phi-3 in Jetson(✅)
- Inference Phi-3 in AI PC(✅)
- Inference Phi-3 with Apple MLX Framework(✅)
- Inference Phi-3 in Local Server(✅)
- Inference Phi-3 in Remote Server using AI Toolkit(✅)
- Inference Phi-3 with Rust(✅)
- Inference Phi-3-Vision in Local(✅)
- Inference Phi-3 with Kaito AKS, Azure Containers(official support)(✅)
- Inference Your Fine-tuning ONNX Runtime Model(✅)
-
Fine-tuning Phi-3
- Downloading & Creating Sample Data Set(✅)
- Fine-tuning Scenarios(✅)
- Fine-tuning vs RAG(✅)
- Fine-tuning Let Phi-3 become an industry expert(✅)
- Fine-tuning Phi-3 with AI Toolkit for VS Code(✅)
- Fine-tuning Phi-3 with Azure Machine Learning Service(✅)
- Fine-tuning Phi-3 with Lora(✅)
- Fine-tuning Phi-3 with QLora(✅)
- Fine-tuning Phi-3 with Azure AI Studio(✅)
- Fine-tuning Phi-3 with Azure ML CLI/SDK(✅)
- Fine-tuning with Microsoft Olive(✅)
- Fine-tuning Phi-3-vision with Weights and Bias(✅)
- Fine-tuning Phi-3 with Apple MLX Framework(✅)
- Fine-tuning Phi-3-vision (official support)(✅)
- Fine-Tuning Phi-3 with Kaito AKS , Azure Containers(official Support)(✅)
- Fine-Tuning Phi-3 and 3.5 Vision(✅)
-
Evaluation Phi-3
-
E2E Samples for Phi-3-mini
- Introduction to End to End Samples(✅)
- Prepare your industry data(✅)
- Use Microsoft Olive to architect your projects(✅)
- Local Chatbot on Android with Phi-3, ONNXRuntime Mobile and ONNXRuntime Generate API(✅)
- Hugging Face Space WebGPU and Phi-3-mini Demo- Phi-3-mini provides the user with a private (and powerful) chatbot experience. You can try it out(✅)
- Local Chatbot in the browser using Phi3, ONNX Runtime Web and WebGPU(✅)
- OpenVino Chat(✅)
- Multi Model - Interactive Phi-3-mini and OpenAI Whisper(✅)
- MLFlow - Building a wrapper and using Phi-3 with MLFlow(✅)
- Model Optimization - How to optimize Phi-3-min model for ONNX Runtime Web with Olive(✅)
- WinUI3 App with Phi-3 mini-4k-instruct-onnx(✅)
- WinUI3 Multi Model AI Powered Notes App Sample(✅)
- Fine-tune and Integrate custom Phi-3 models with Prompt flow(✅)
- Fine-tune and Integrate custom Phi-3 models with Prompt flow in Azure AI Studio(✅)
- Evaluate the Fine-tuned Phi-3 / Phi-3.5 Model in Azure AI Studio Focusing on Microsoft's Responsible AI Principles(✅)
- Phi-3.5-mini-instruct language prediction sample (Chinese/English)(✅)
-
E2E Samples for Phi-3-vision
-
E2E Samples for Phi-3.5-MoE
-
Labs and workshops samples Phi-3
- C# .NET Labs(✅)
- Build your own Visual Studio Code GitHub Copilot Chat with Microsoft Phi-3 Family(✅)
- Local WebGPU Phi-3 Mini RAG Chatbot Samples with Local RAG File(✅)
- Phi-3 ONNX Tutorial(✅)
- Phi-3-vision ONNX Tutorial(✅)
- Run the Phi-3 models with the ONNX Runtime generate() API(✅)
- Phi-3 ONNX Multi Model LLM Chat UI, This is a chat demo(✅)
- C# Hello Phi-3 ONNX example Phi-3(✅)
- C# API Phi-3 ONNX example to support Phi3-Vision(✅)
- Run C# Phi-3 samples in a CodeSpace(✅)
- Using Phi-3 with Promptflow and Azure AI Search(✅)
- Windows AI-PC APIs with Windows Copilot Library
-
Learning Phi-3.5
- What's new Phi-3.5 Family(✅)
- Quantifying Phi-3.5 Family(✅)
- Phi-3.5 Application Samples
You can learn how to use Microsoft Phi-3 and how to build E2E solutions in your different hardware devices. To experience Phi-3 for yourself, start by playing with the model and customizing Phi-3 for your scenarios using the Azure AI Studio, Azure AI Model Catalog you can learn more at Getting Started with Azure AI Studio
Playground Each model has a dedicated playground to test the model Azure AI Playground.
You can learn how to use Microsoft Phi-3 and how to build E2E solutions in your different hardware devices. To experience Phi-3 for yourself, start by playing with the model and customizing Phi-3 for your scenarios using the GitHub Model Catalog you can learn more at Getting Started with GitHub Model Catalog
Playground Each model has a dedicated playground to test the model.
You can also find the model on the Hugging Face
Playground Hugging Chat playground
Note: These translations were automatically generated using the open-source co-op-translator and may contain errors or inaccuracies. For critical information, it is recommended to refer to the original or consult a professional human translation. If you'd like to add or update a translation, please refer to the co-op-translator repository, where you can easily contribute using simple commands.
Language | Code | Link to Translated README | Last Updated |
---|---|---|---|
Chinese (Simplified) | zh | Chinese Translation | 2024-10-04 |
Chinese (Traditional) | tw | Chinese Translation | 2024-10-04 |
French | fr | French Translation | 2024-10-04 |
Japanese | ja | Japanese Translation | 2024-10-04 |
Korean | ko | Korean Translation | 2024-10-04 |
Spanish | es | Spanish Translation | 2024-10-04 |
This project may contain trademarks or logos for projects, products, or services. Authorized use of Microsoft trademarks or logos is subject to and must follow Microsoft's Trademark & Brand Guidelines. Use of Microsoft trademarks or logos in modified versions of this project must not cause confusion or imply Microsoft sponsorship. Any use of third-party trademarks or logos are subject to those third-party's policies.
For Tasks:
Click tags to check more tools for each tasksFor Jobs:
Alternative AI tools for Phi-3CookBook
Similar Open Source Tools
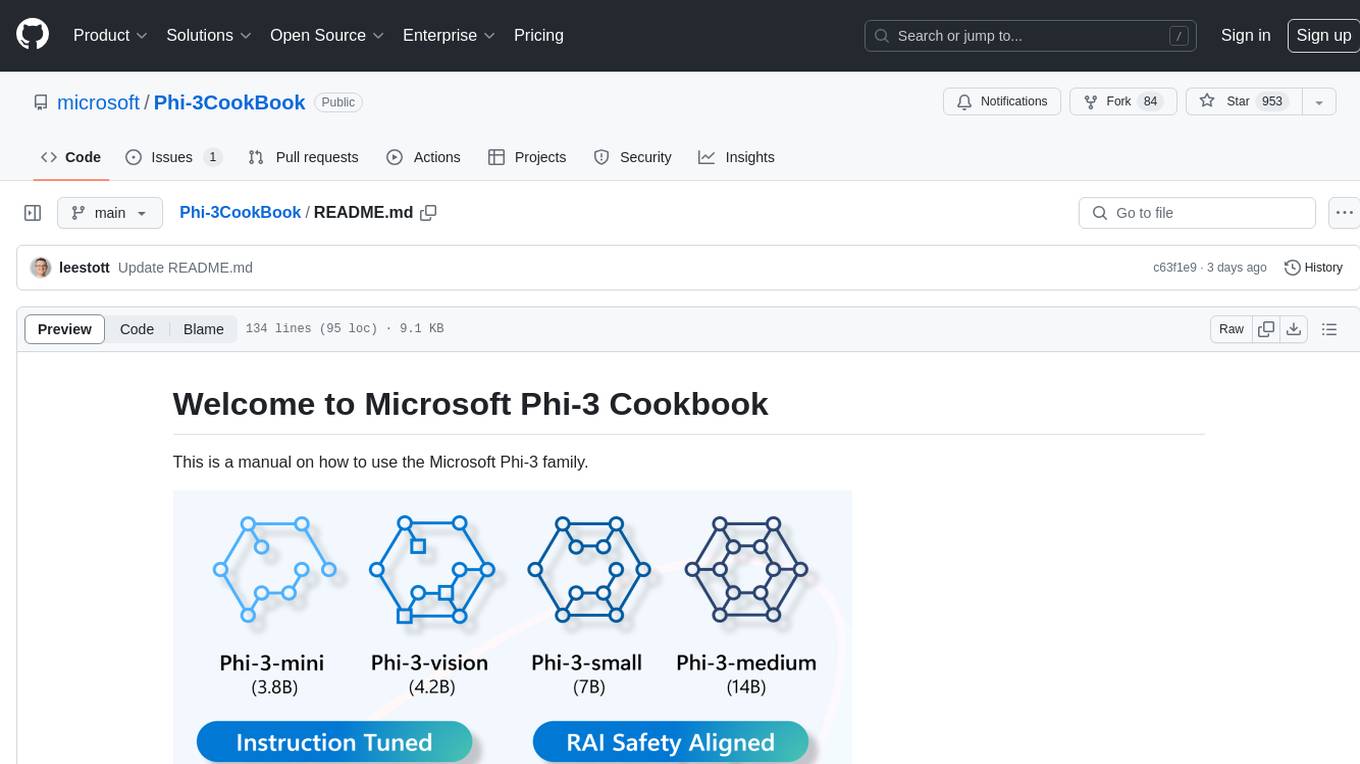
Phi-3CookBook
Phi-3CookBook is a manual on how to use the Microsoft Phi-3 family, which consists of open AI models developed by Microsoft. The Phi-3 models are highly capable and cost-effective small language models, outperforming models of similar and larger sizes across various language, reasoning, coding, and math benchmarks. The repository provides detailed information on different Phi-3 models, their performance, availability, and usage scenarios across different platforms like Azure AI Studio, Hugging Face, and Ollama. It also covers topics such as fine-tuning, evaluation, and end-to-end samples for Phi-3-mini and Phi-3-vision models, along with labs, workshops, and contributing guidelines.
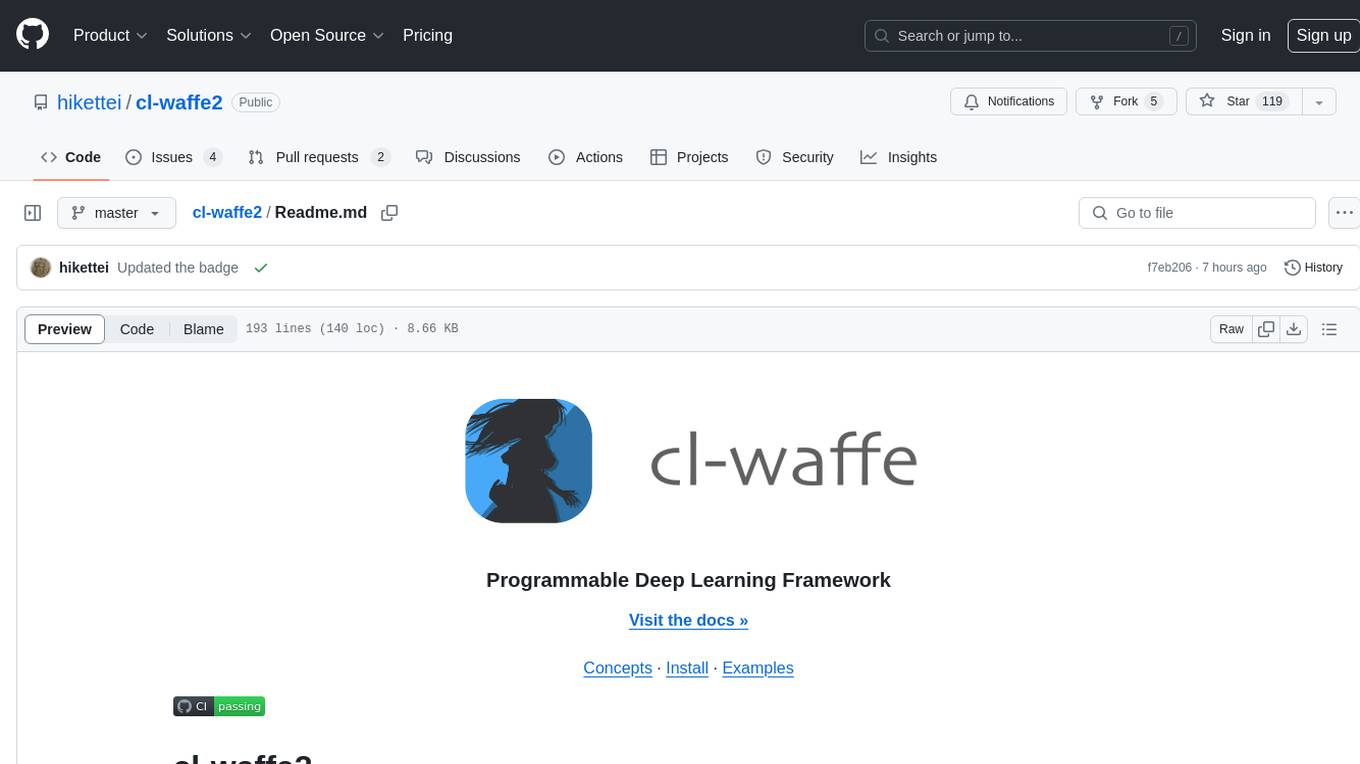
cl-waffe2
cl-waffe2 is an experimental deep learning framework in Common Lisp, providing fast, systematic, and customizable matrix operations, reverse mode tape-based Automatic Differentiation, and neural network model building and training features accelerated by a JIT Compiler. It offers abstraction layers, extensibility, inlining, graph-level optimization, visualization, debugging, systematic nodes, and symbolic differentiation. Users can easily write extensions and optimize their networks without overheads. The framework is designed to eliminate barriers between users and developers, allowing for easy customization and extension.
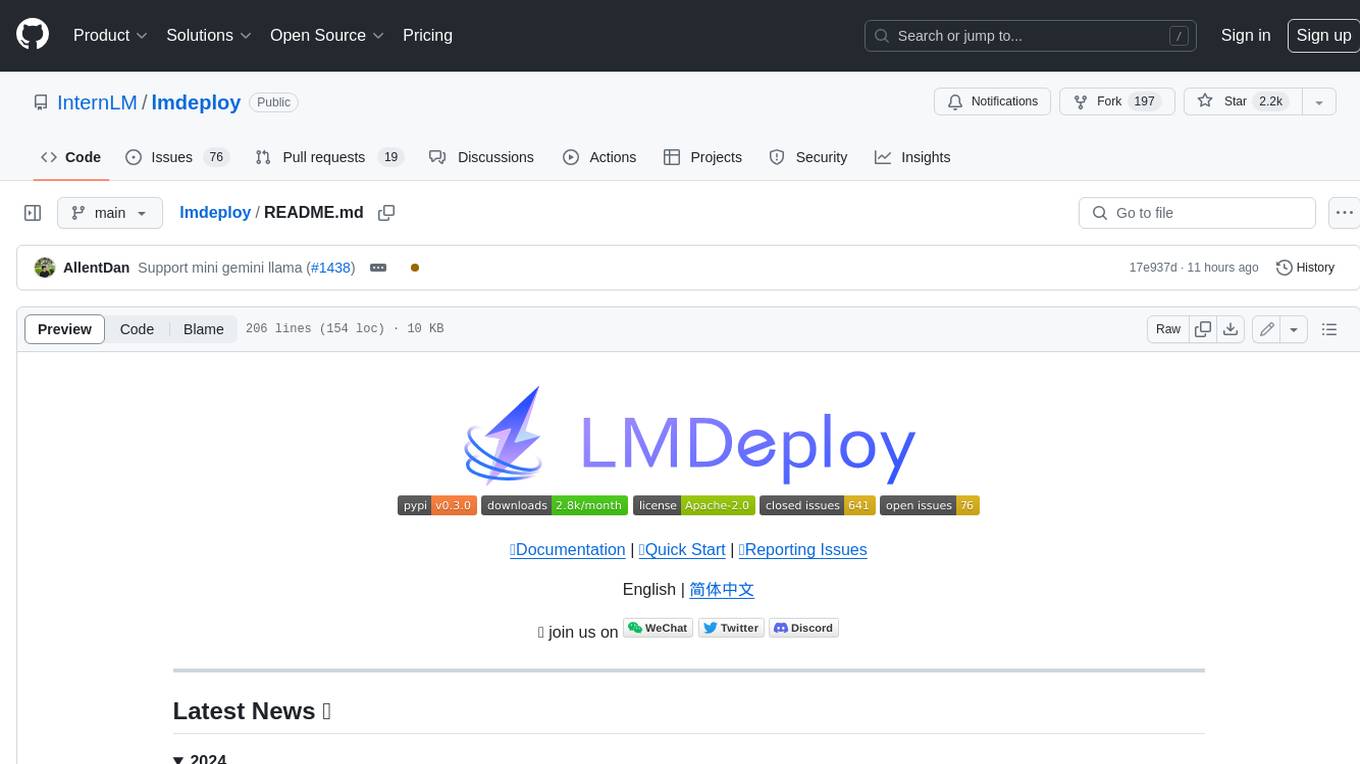
lmdeploy
LMDeploy is a toolkit for compressing, deploying, and serving LLM, developed by the MMRazor and MMDeploy teams. It has the following core features: * **Efficient Inference** : LMDeploy delivers up to 1.8x higher request throughput than vLLM, by introducing key features like persistent batch(a.k.a. continuous batching), blocked KV cache, dynamic split&fuse, tensor parallelism, high-performance CUDA kernels and so on. * **Effective Quantization** : LMDeploy supports weight-only and k/v quantization, and the 4-bit inference performance is 2.4x higher than FP16. The quantization quality has been confirmed via OpenCompass evaluation. * **Effortless Distribution Server** : Leveraging the request distribution service, LMDeploy facilitates an easy and efficient deployment of multi-model services across multiple machines and cards. * **Interactive Inference Mode** : By caching the k/v of attention during multi-round dialogue processes, the engine remembers dialogue history, thus avoiding repetitive processing of historical sessions.
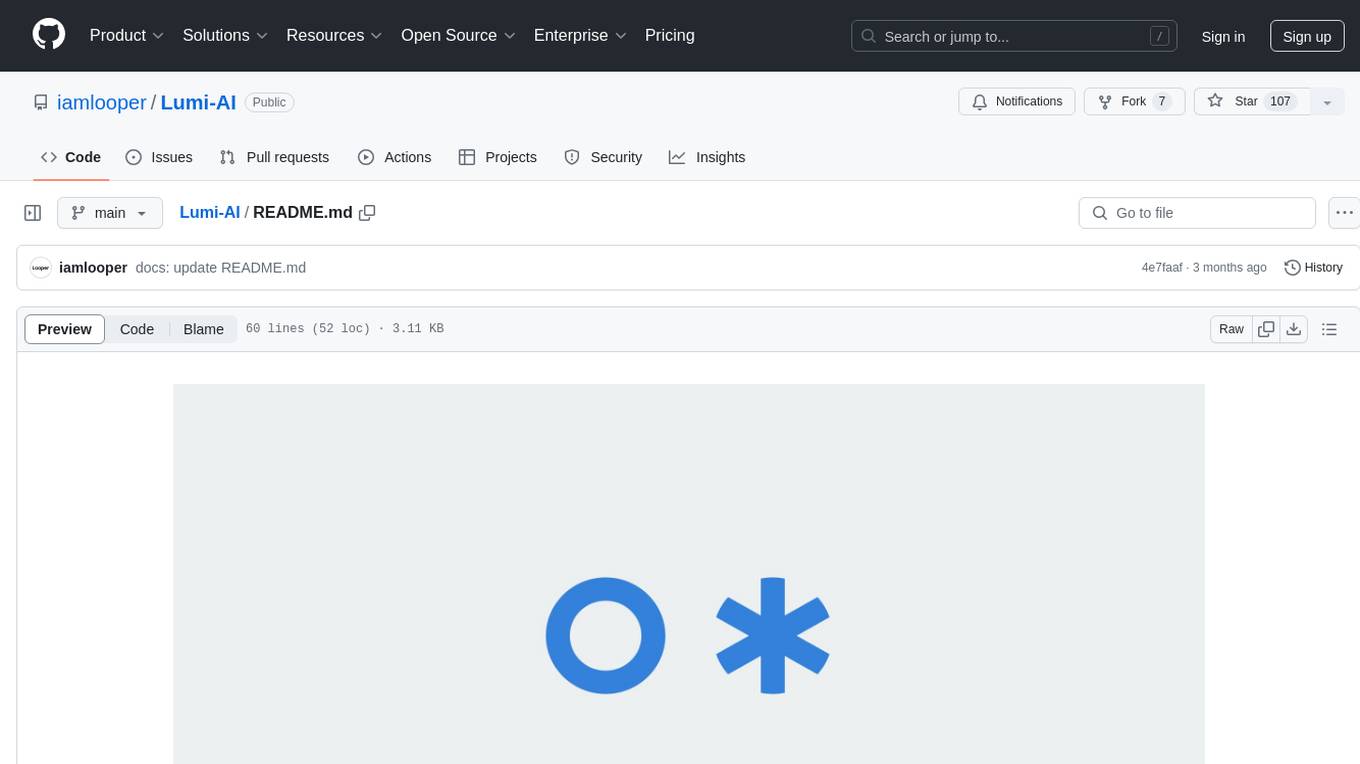
Lumi-AI
Lumi AI is a friendly AI sidekick with a human-like personality that offers features like file upload and analysis, web search, local chat storage, custom instructions, changeable conversational style, enhanced context retention, voice query input, and various tools. The project has been developed with contributions from a team of developers, designers, and testers, and is licensed under Apache 2.0 and MIT licenses.
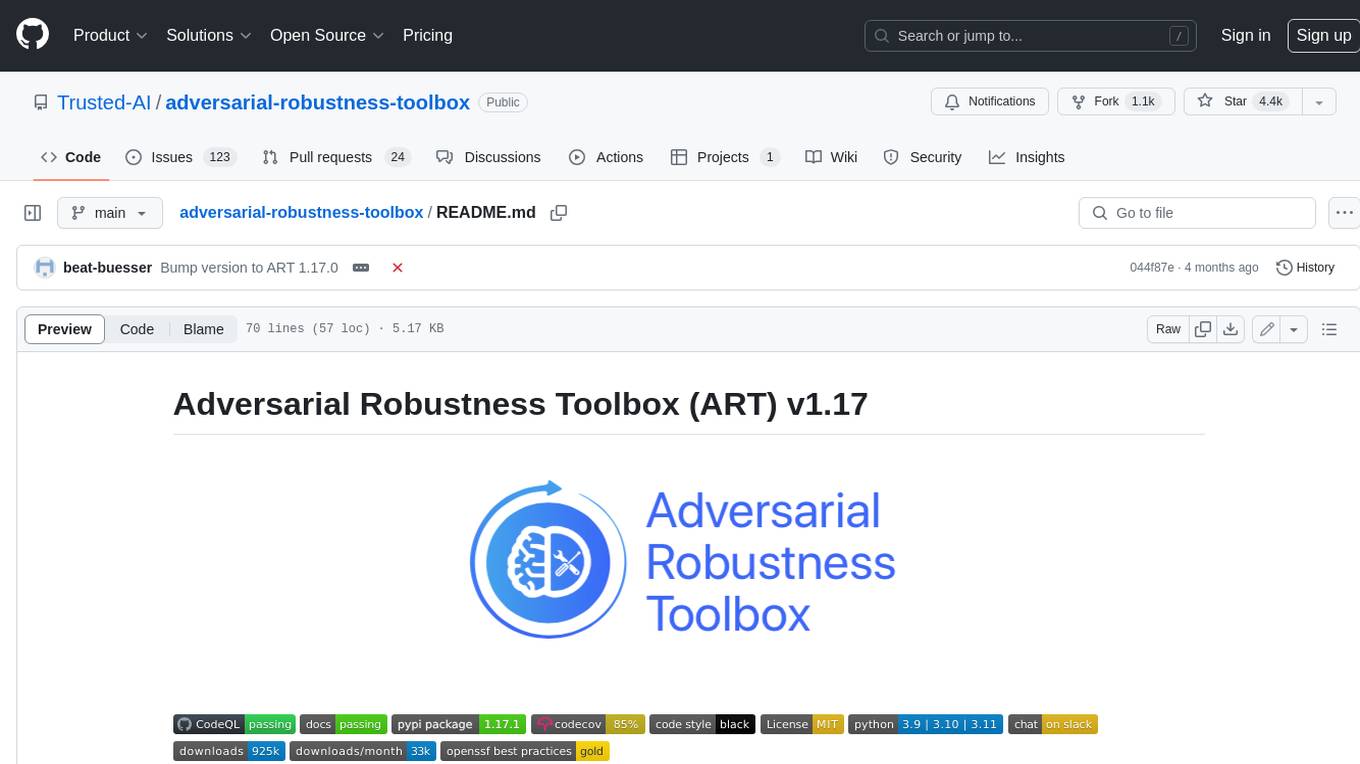
adversarial-robustness-toolbox
Adversarial Robustness Toolbox (ART) is a Python library for Machine Learning Security. ART provides tools that enable developers and researchers to defend and evaluate Machine Learning models and applications against the adversarial threats of Evasion, Poisoning, Extraction, and Inference. ART supports all popular machine learning frameworks (TensorFlow, Keras, PyTorch, MXNet, scikit-learn, XGBoost, LightGBM, CatBoost, GPy, etc.), all data types (images, tables, audio, video, etc.) and machine learning tasks (classification, object detection, speech recognition, generation, certification, etc.).
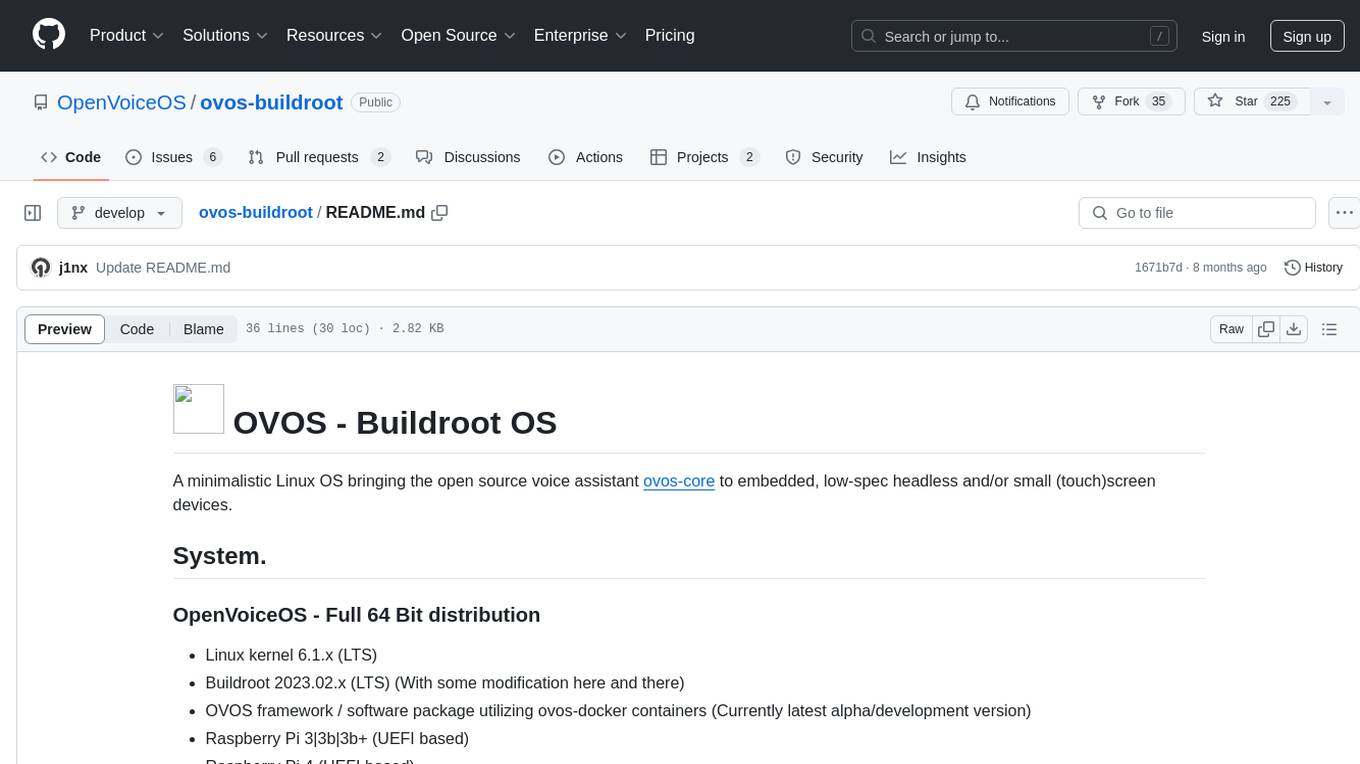
ovos-buildroot
OVOS - Buildroot OS is a minimalistic Linux OS designed to bring the open source voice assistant ovos-core to embedded, low-spec headless, and small touchscreen devices. It includes a full 64-bit distribution with Linux kernel 6.1.x, Buildroot 2023.02.x, and OVOS framework utilizing ovos-docker containers. The supported hardware includes Raspberry Pi 3, 3b, 3b+, Raspberry Pi 4, x86_64 Intel-based computers, and Open Virtual Appliance. The project is inspired by Mycroft AI, Buildroot, and HassOS, offering a platform for building voice assistant solutions on various devices.
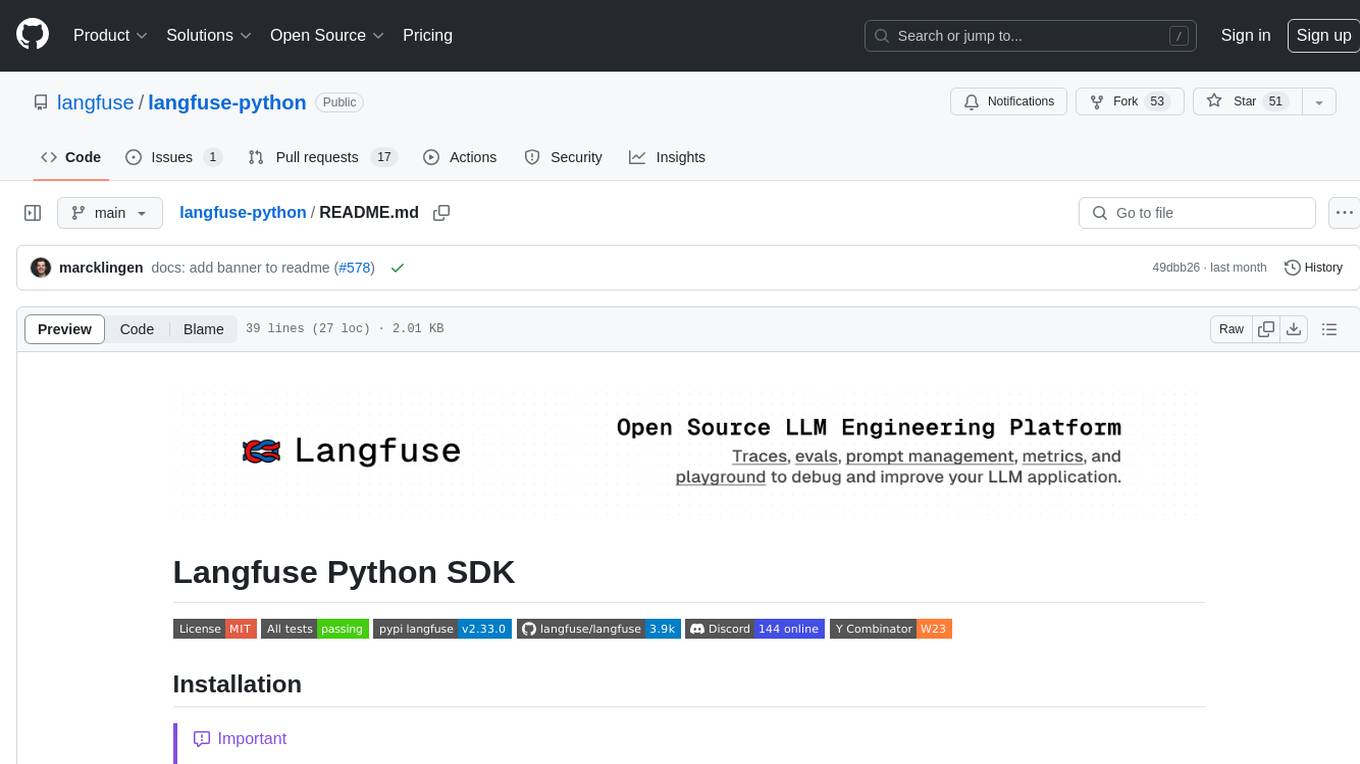
langfuse-python
Langfuse Python SDK is a software development kit that provides tools and functionalities for integrating with Langfuse's language processing services. It offers decorators for observing code behavior, low-level SDK for tracing, and wrappers for accessing Langfuse's public API. The SDK was recently rewritten in version 2, released on December 17, 2023, with detailed documentation available on the official website. It also supports integrations with OpenAI SDK, LlamaIndex, and LangChain for enhanced language processing capabilities.
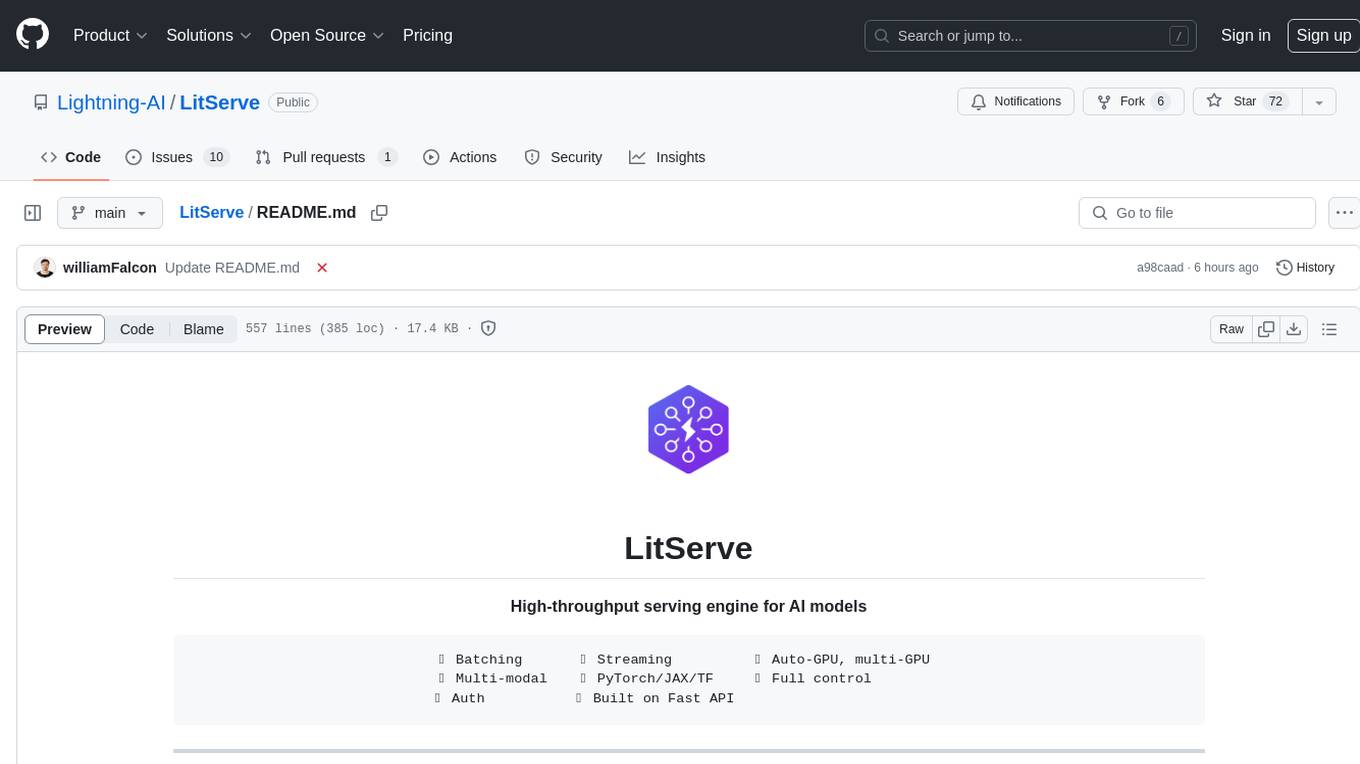
LitServe
LitServe is a high-throughput serving engine designed for deploying AI models at scale. It generates an API endpoint for models, handles batching, streaming, and autoscaling across CPU/GPUs. LitServe is built for enterprise scale with a focus on minimal, hackable code-base without bloat. It supports various model types like LLMs, vision, time-series, and works with frameworks like PyTorch, JAX, Tensorflow, and more. The tool allows users to focus on model performance rather than serving boilerplate, providing full control and flexibility.
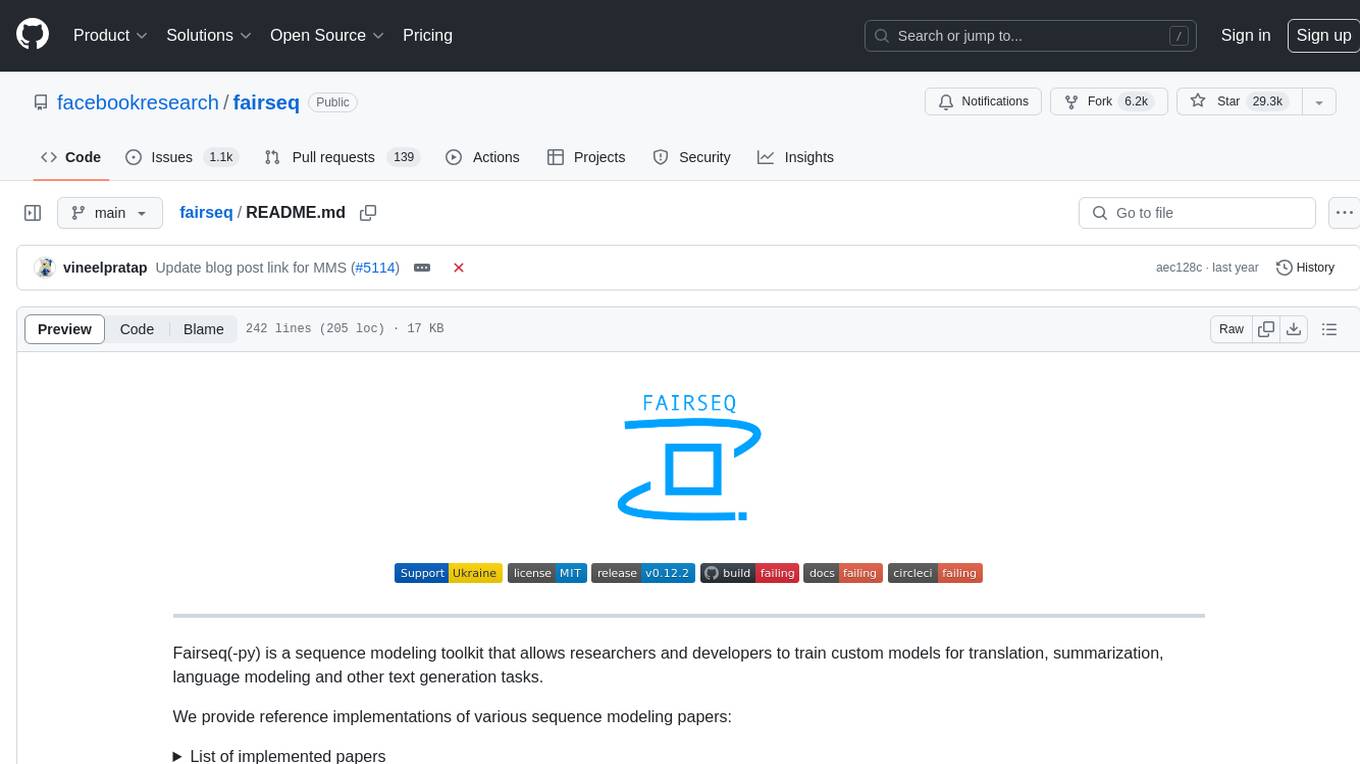
fairseq
Fairseq is a sequence modeling toolkit that enables researchers and developers to train custom models for translation, summarization, language modeling, and other text generation tasks. It provides reference implementations of various sequence modeling papers covering CNN, LSTM networks, Transformer networks, LightConv, DynamicConv models, Non-autoregressive Transformers, Finetuning, and more. The toolkit supports multi-GPU training, fast generation on CPU and GPU, mixed precision training, extensibility, flexible configuration based on Hydra, and full parameter and optimizer state sharding. Pre-trained models are available for translation and language modeling with a torch.hub interface. Fairseq also offers pre-trained models and examples for tasks like XLS-R, cross-lingual retrieval, wav2vec 2.0, unsupervised quality estimation, and more.
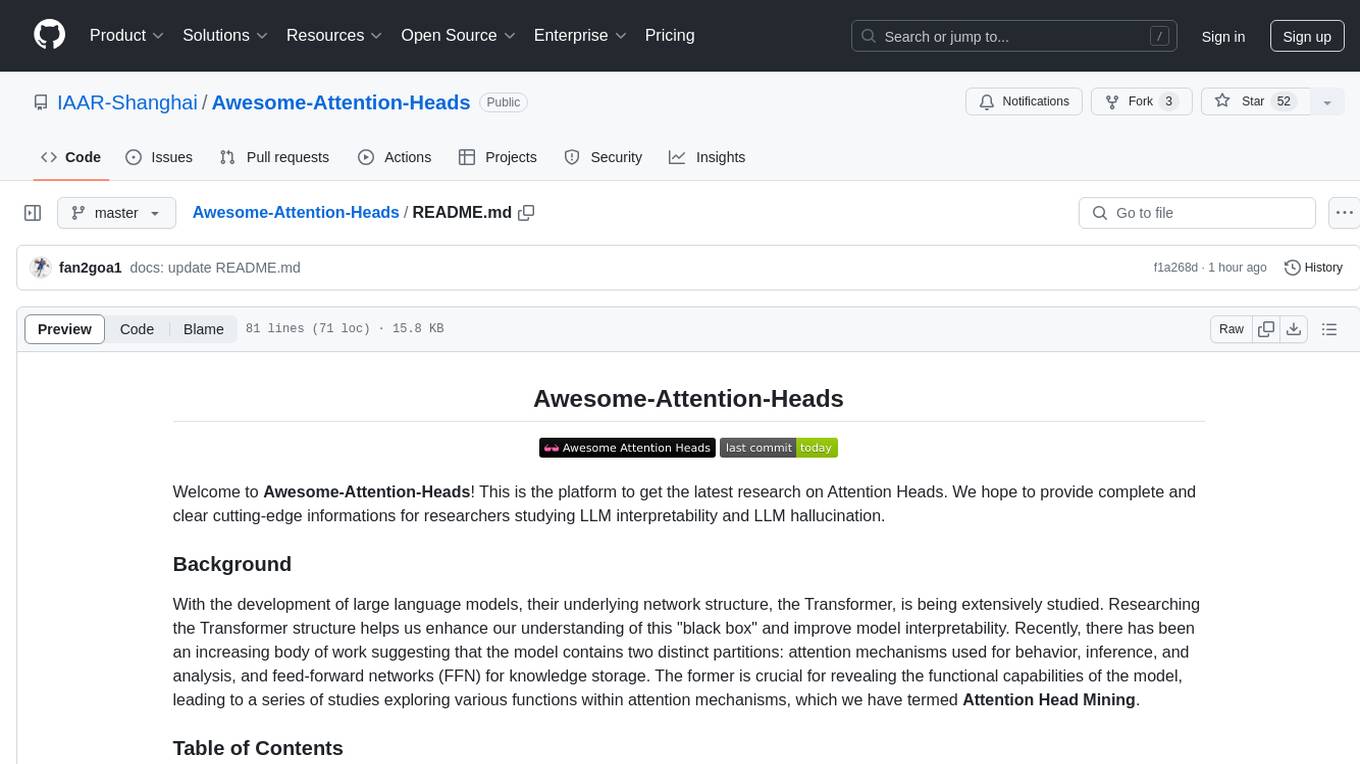
Awesome-Attention-Heads
Awesome-Attention-Heads is a platform providing the latest research on Attention Heads, focusing on enhancing understanding of Transformer structure for model interpretability. It explores attention mechanisms for behavior, inference, and analysis, alongside feed-forward networks for knowledge storage. The repository aims to support researchers studying LLM interpretability and hallucination by offering cutting-edge information on Attention Head Mining.
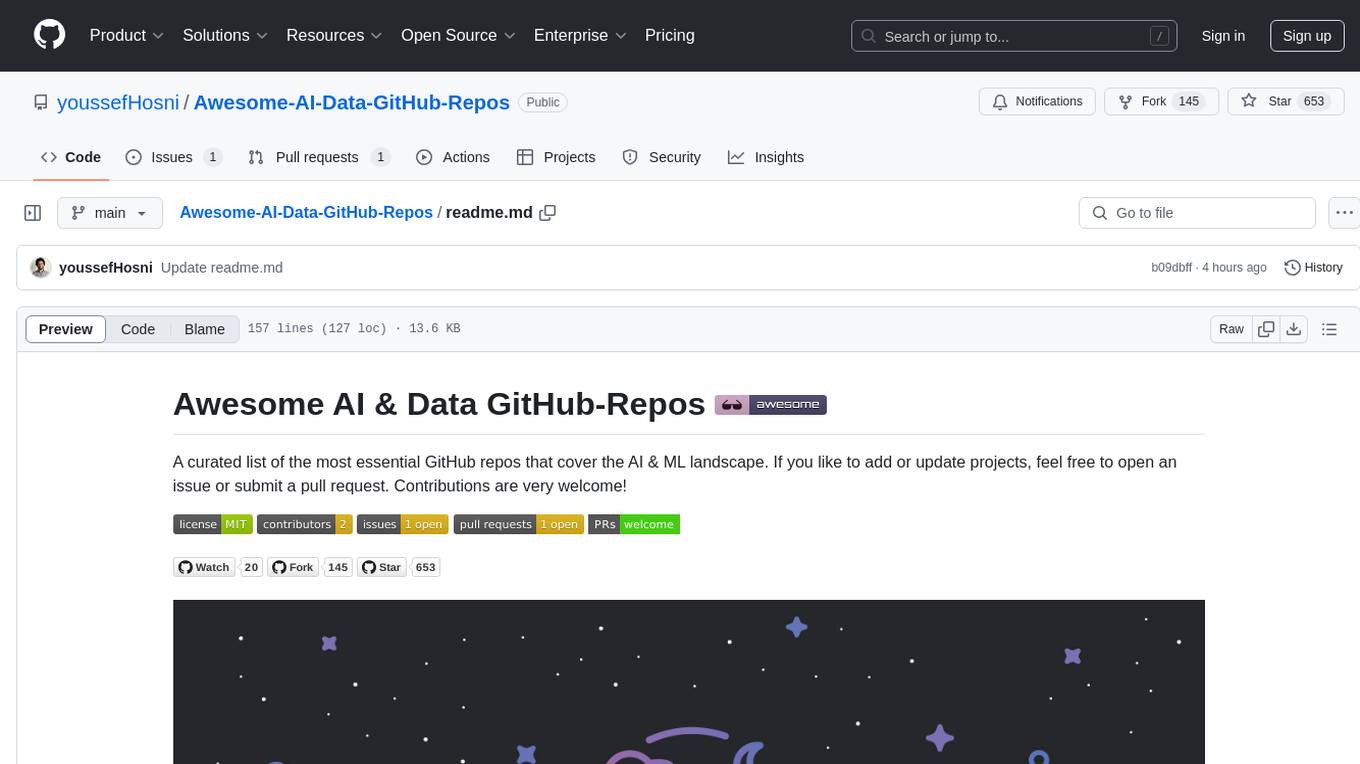
Awesome-AI-Data-GitHub-Repos
Awesome AI & Data GitHub-Repos is a curated list of essential GitHub repositories covering the AI & ML landscape. It includes resources for Natural Language Processing, Large Language Models, Computer Vision, Data Science, Machine Learning, MLOps, Data Engineering, SQL & Database, and Statistics. The repository aims to provide a comprehensive collection of projects and resources for individuals studying or working in the field of AI and data science.
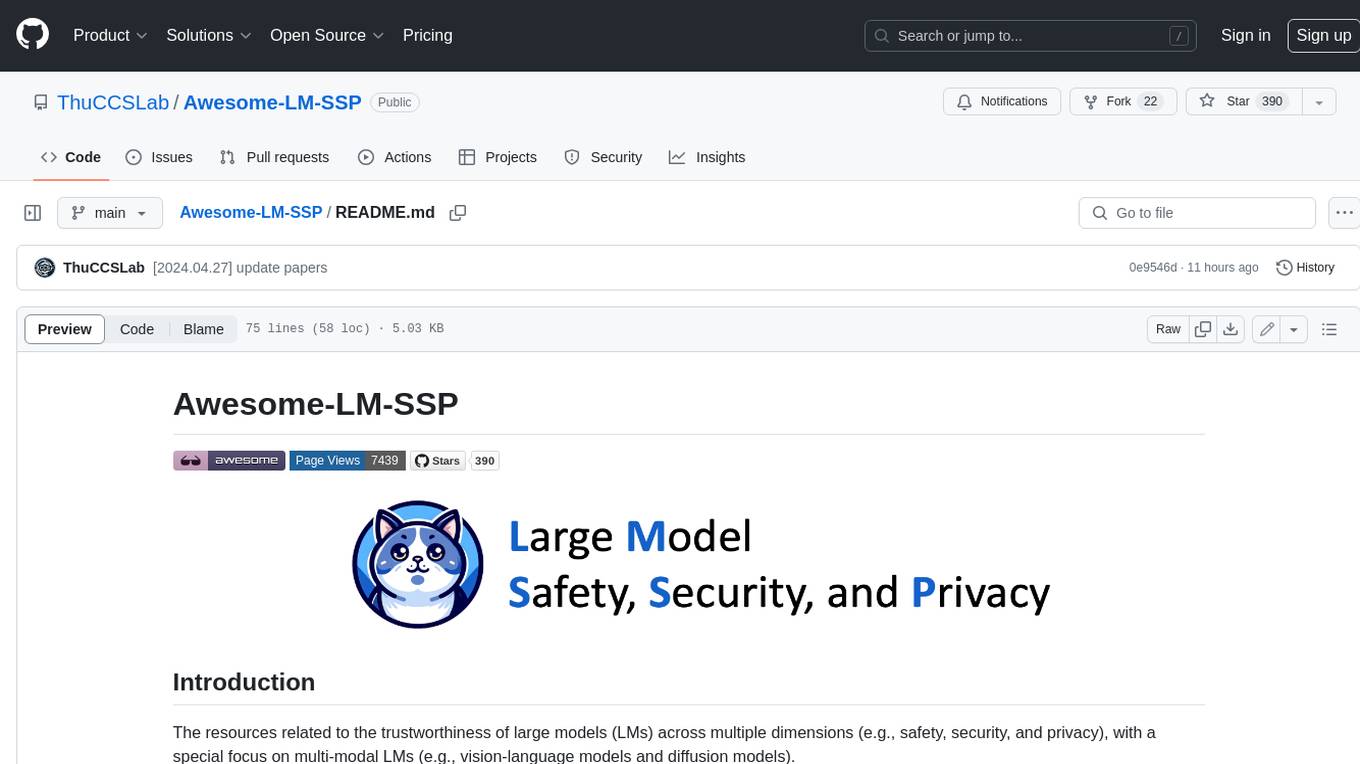
Awesome-LM-SSP
The Awesome-LM-SSP repository is a collection of resources related to the trustworthiness of large models (LMs) across multiple dimensions, with a special focus on multi-modal LMs. It includes papers, surveys, toolkits, competitions, and leaderboards. The resources are categorized into three main dimensions: safety, security, and privacy. Within each dimension, there are several subcategories. For example, the safety dimension includes subcategories such as jailbreak, alignment, deepfake, ethics, fairness, hallucination, prompt injection, and toxicity. The security dimension includes subcategories such as adversarial examples, poisoning, and system security. The privacy dimension includes subcategories such as contamination, copyright, data reconstruction, membership inference attacks, model extraction, privacy-preserving computation, and unlearning.
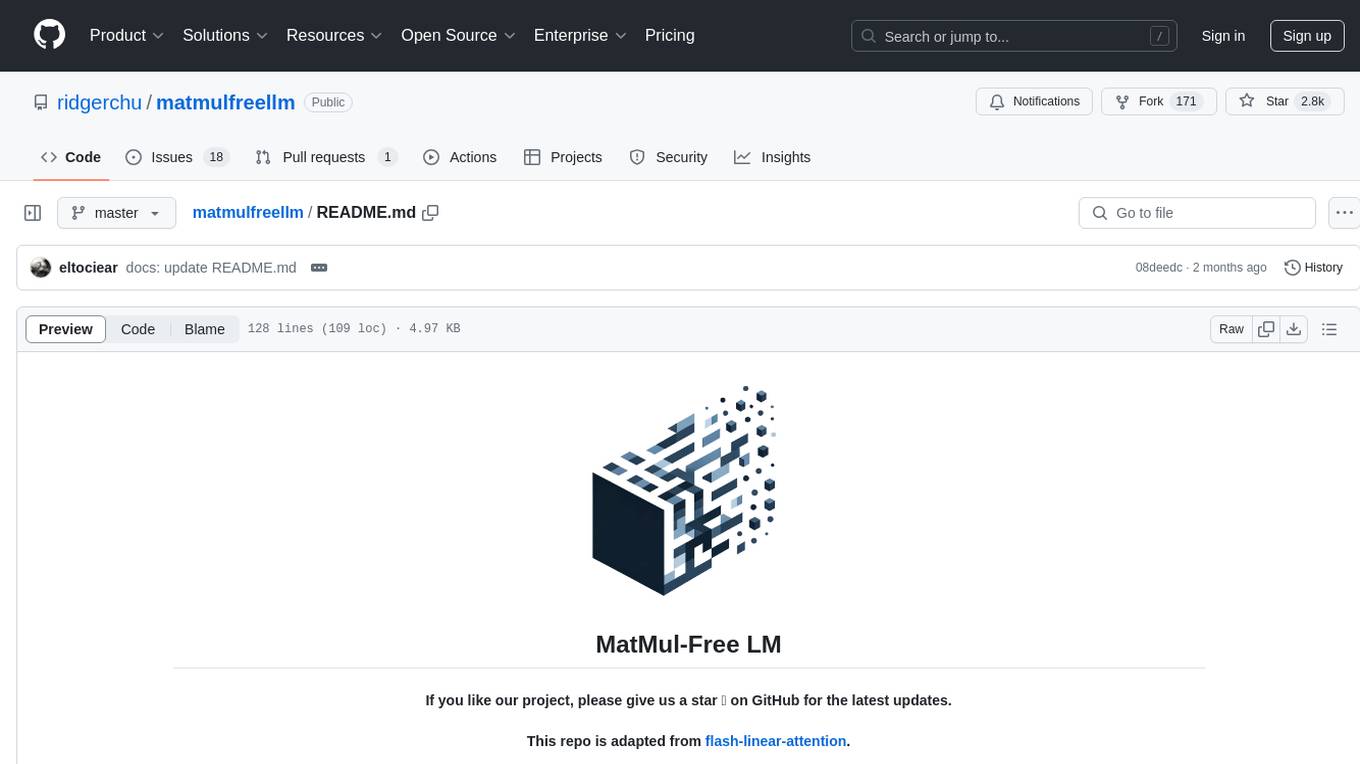
matmulfreellm
MatMul-Free LM is a language model architecture that eliminates the need for Matrix Multiplication (MatMul) operations. This repository provides an implementation of MatMul-Free LM that is compatible with the 🤗 Transformers library. It evaluates how the scaling law fits to different parameter models and compares the efficiency of the architecture in leveraging additional compute to improve performance. The repo includes pre-trained models, model implementations compatible with 🤗 Transformers library, and generation examples for text using the 🤗 text generation APIs.
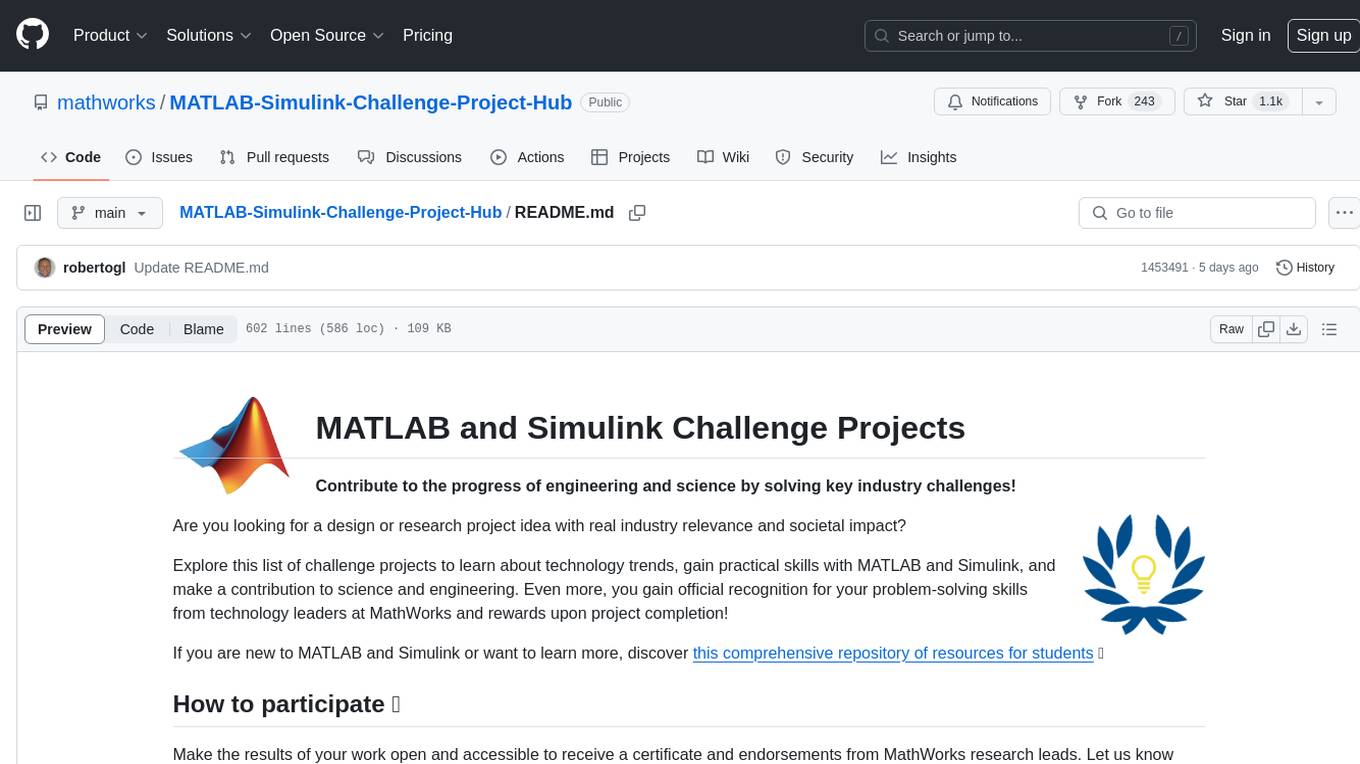
MATLAB-Simulink-Challenge-Project-Hub
MATLAB-Simulink-Challenge-Project-Hub is a repository aimed at contributing to the progress of engineering and science by providing challenge projects with real industry relevance and societal impact. The repository offers a wide range of projects covering various technology trends such as Artificial Intelligence, Autonomous Vehicles, Big Data, Computer Vision, and Sustainability. Participants can gain practical skills with MATLAB and Simulink while making a significant contribution to science and engineering. The projects are designed to enhance expertise in areas like Sustainability and Renewable Energy, Control, Modeling and Simulation, Machine Learning, and Robotics. By participating in these projects, individuals can receive official recognition for their problem-solving skills from technology leaders at MathWorks and earn rewards upon project completion.
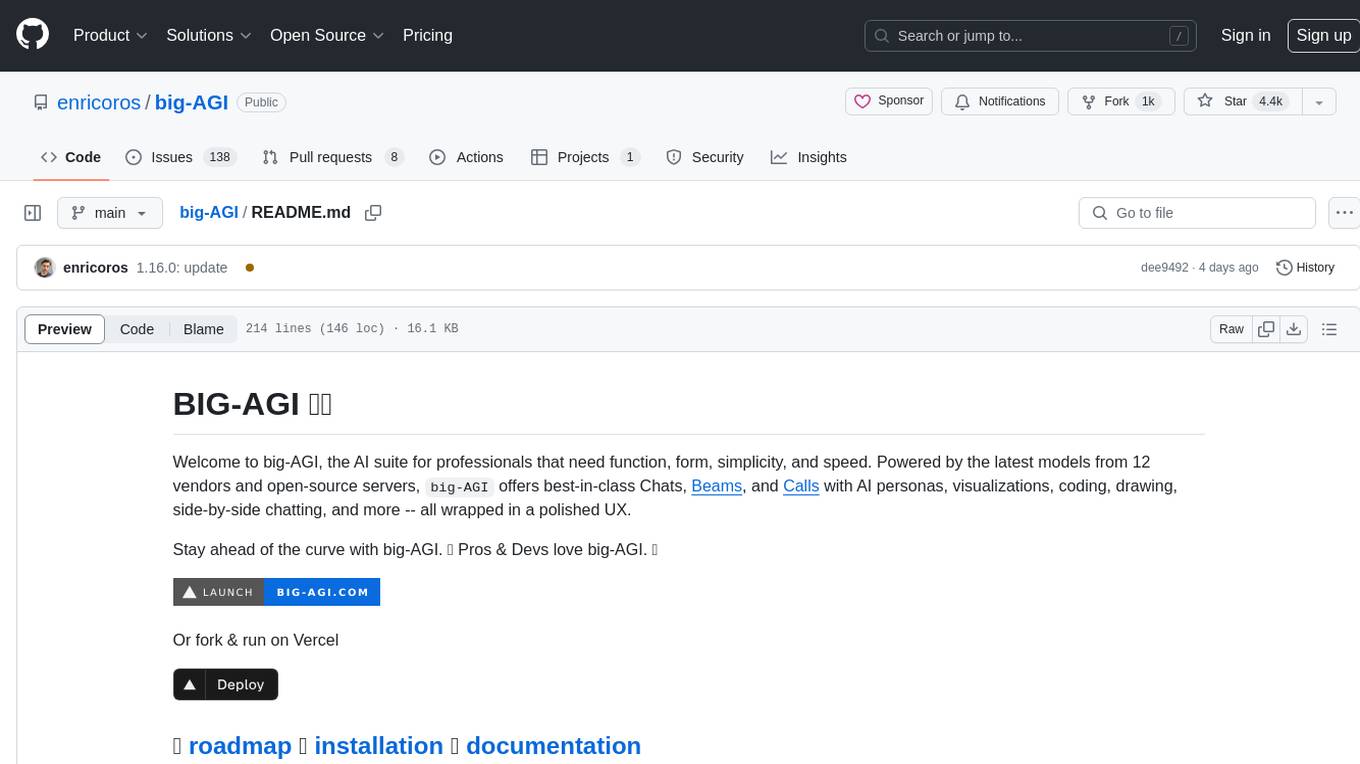
big-AGI
big-AGI is an AI suite designed for professionals seeking function, form, simplicity, and speed. It offers best-in-class Chats, Beams, and Calls with AI personas, visualizations, coding, drawing, side-by-side chatting, and more, all wrapped in a polished UX. The tool is powered by the latest models from 12 vendors and open-source servers, providing users with advanced AI capabilities and a seamless user experience. With continuous updates and enhancements, big-AGI aims to stay ahead of the curve in the AI landscape, catering to the needs of both developers and AI enthusiasts.
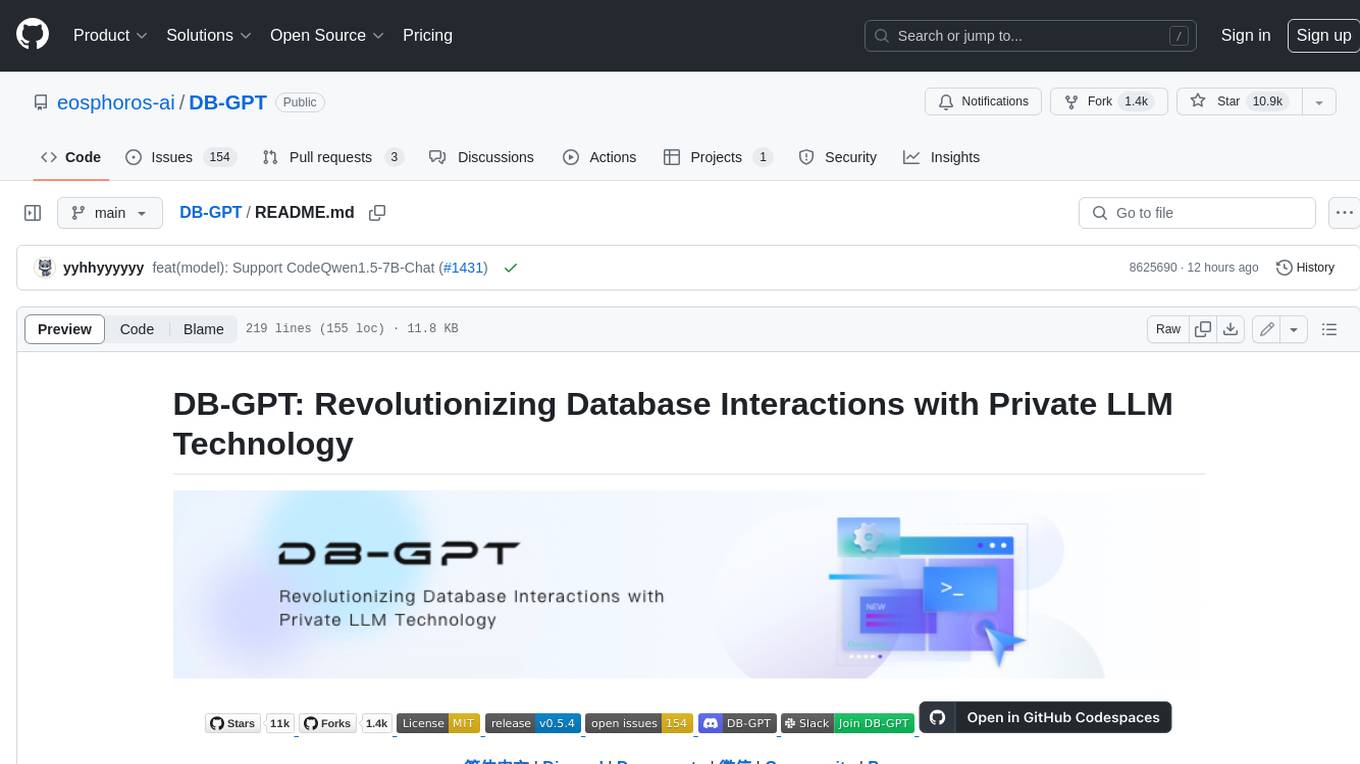
DB-GPT
DB-GPT is an open source AI native data app development framework with AWEL(Agentic Workflow Expression Language) and agents. It aims to build infrastructure in the field of large models, through the development of multiple technical capabilities such as multi-model management (SMMF), Text2SQL effect optimization, RAG framework and optimization, Multi-Agents framework collaboration, AWEL (agent workflow orchestration), etc. Which makes large model applications with data simpler and more convenient.
For similar tasks
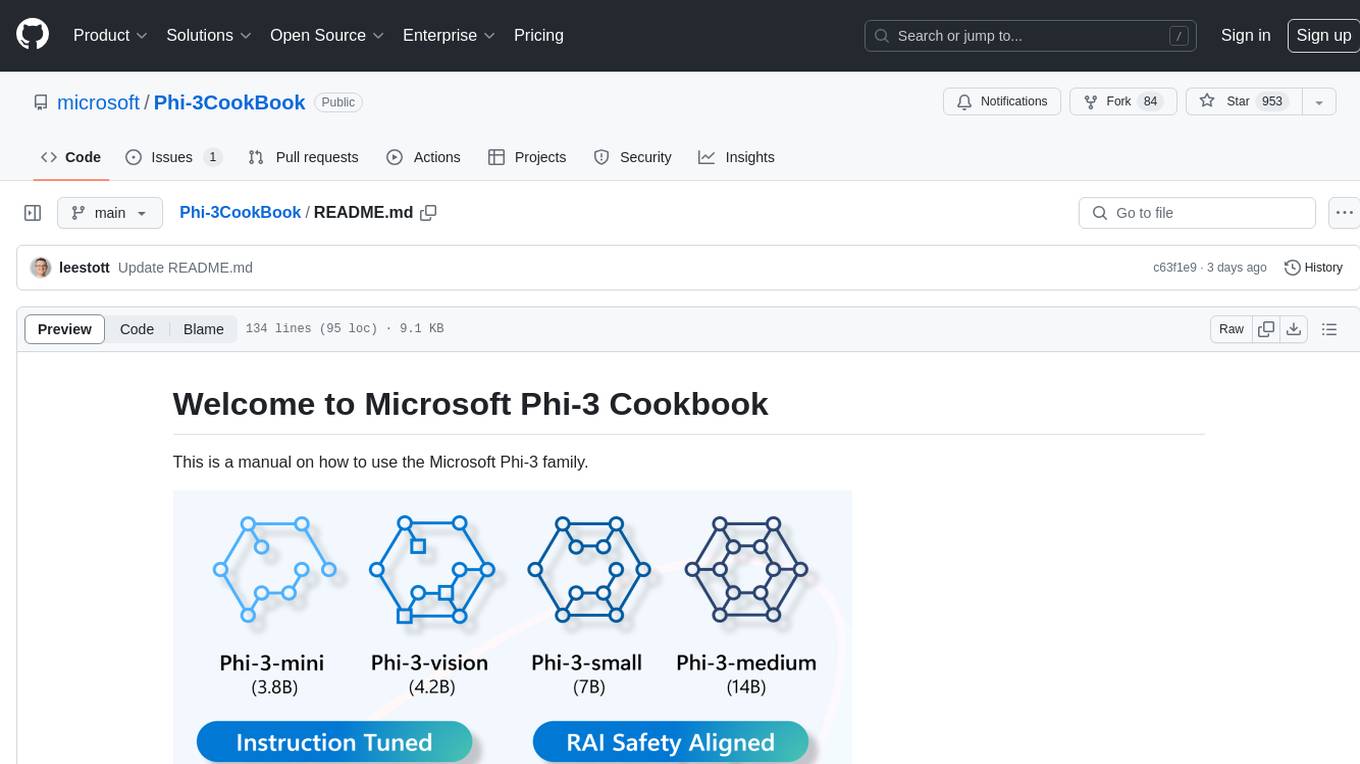
Phi-3CookBook
Phi-3CookBook is a manual on how to use the Microsoft Phi-3 family, which consists of open AI models developed by Microsoft. The Phi-3 models are highly capable and cost-effective small language models, outperforming models of similar and larger sizes across various language, reasoning, coding, and math benchmarks. The repository provides detailed information on different Phi-3 models, their performance, availability, and usage scenarios across different platforms like Azure AI Studio, Hugging Face, and Ollama. It also covers topics such as fine-tuning, evaluation, and end-to-end samples for Phi-3-mini and Phi-3-vision models, along with labs, workshops, and contributing guidelines.
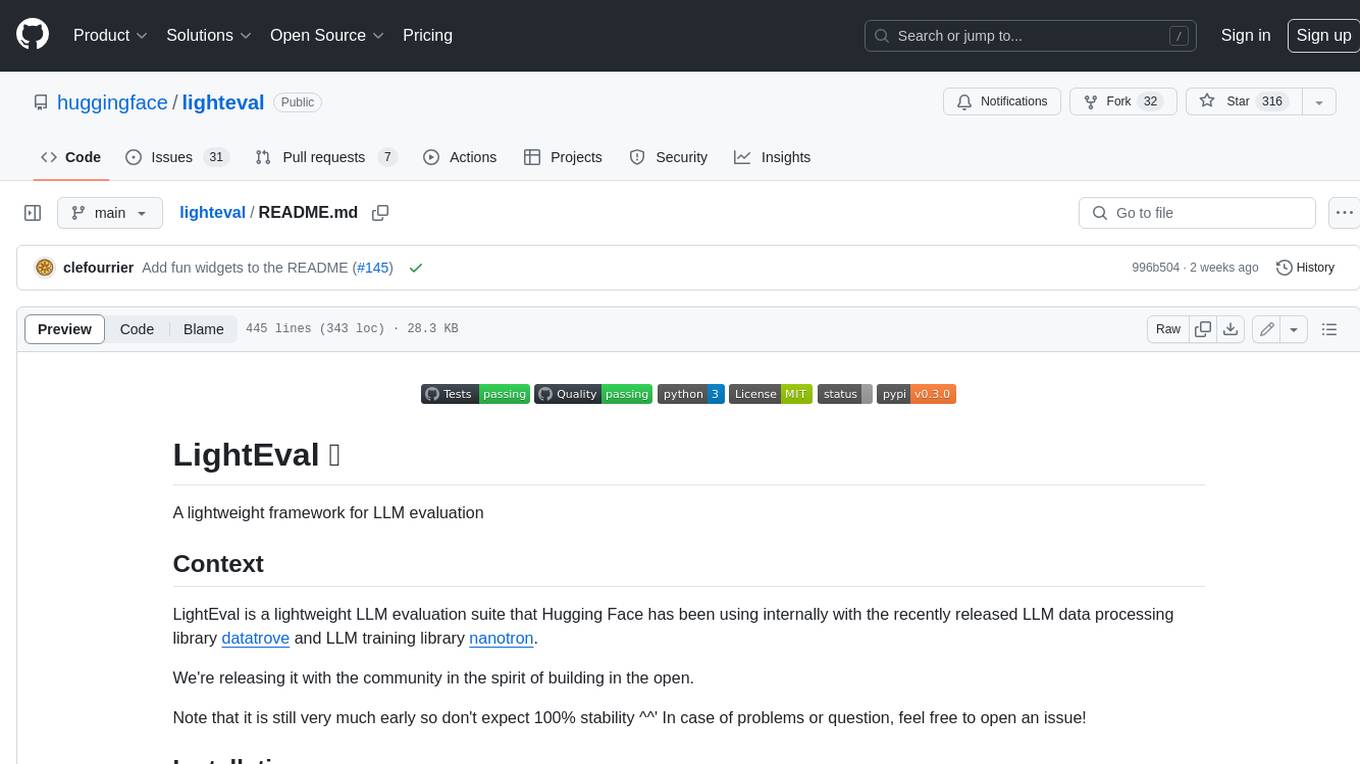
lighteval
LightEval is a lightweight LLM evaluation suite that Hugging Face has been using internally with the recently released LLM data processing library datatrove and LLM training library nanotron. We're releasing it with the community in the spirit of building in the open. Note that it is still very much early so don't expect 100% stability ^^' In case of problems or question, feel free to open an issue!
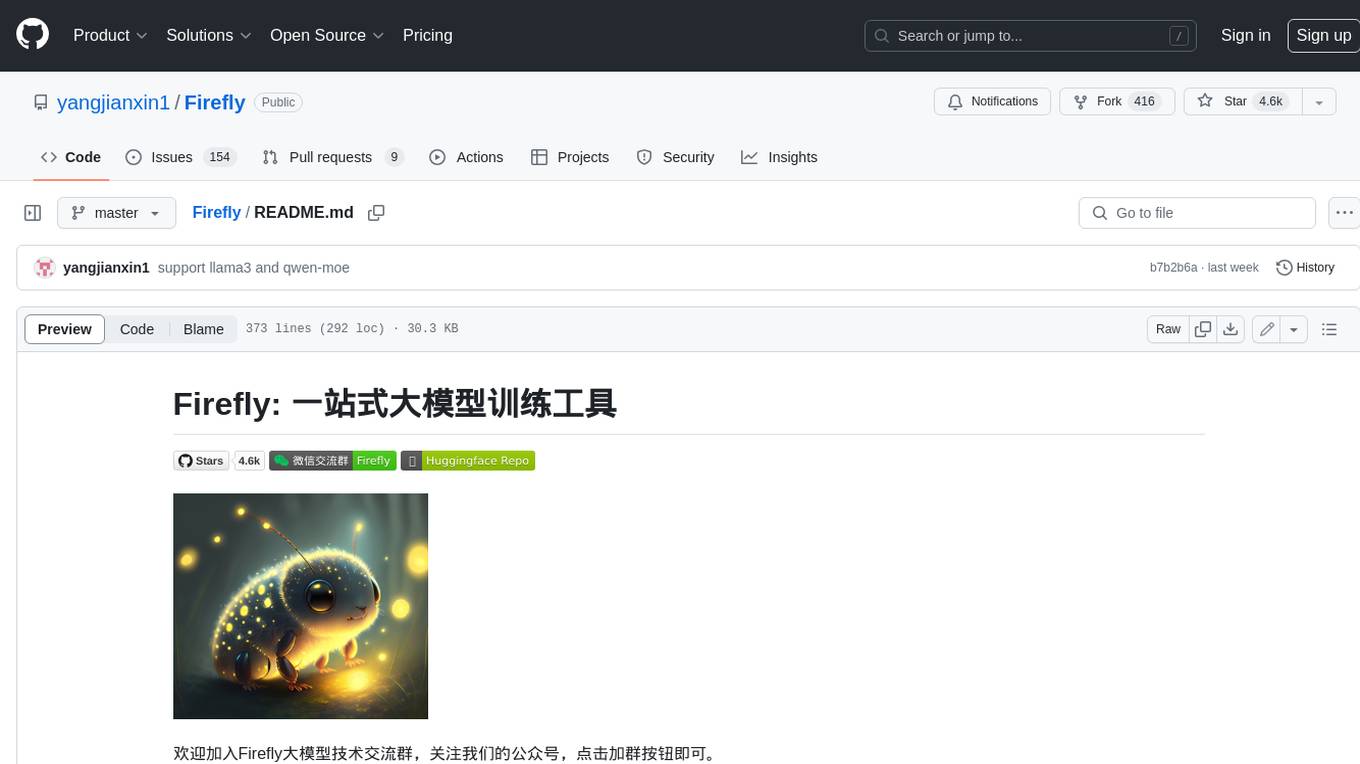
Firefly
Firefly is an open-source large model training project that supports pre-training, fine-tuning, and DPO of mainstream large models. It includes models like Llama3, Gemma, Qwen1.5, MiniCPM, Llama, InternLM, Baichuan, ChatGLM, Yi, Deepseek, Qwen, Orion, Ziya, Xverse, Mistral, Mixtral-8x7B, Zephyr, Vicuna, Bloom, etc. The project supports full-parameter training, LoRA, QLoRA efficient training, and various tasks such as pre-training, SFT, and DPO. Suitable for users with limited training resources, QLoRA is recommended for fine-tuning instructions. The project has achieved good results on the Open LLM Leaderboard with QLoRA training process validation. The latest version has significant updates and adaptations for different chat model templates.
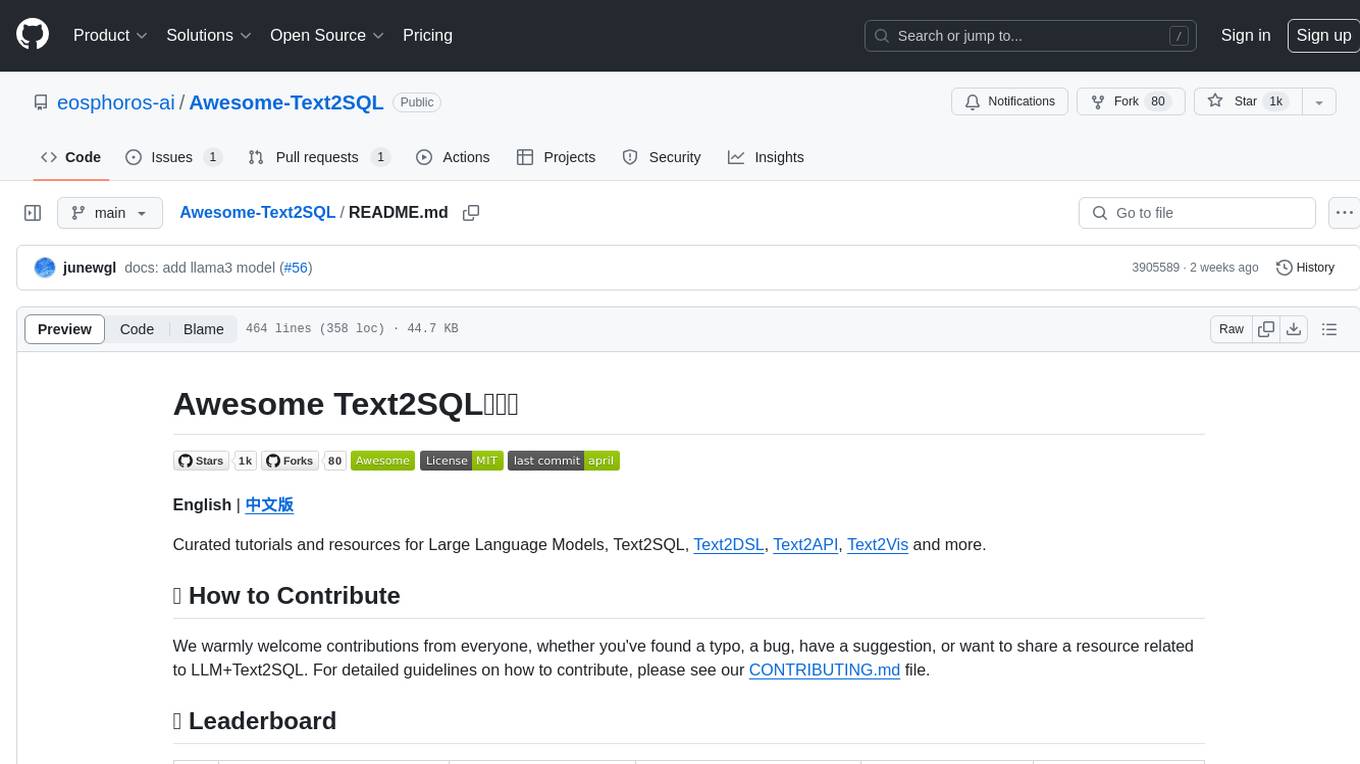
Awesome-Text2SQL
Awesome Text2SQL is a curated repository containing tutorials and resources for Large Language Models, Text2SQL, Text2DSL, Text2API, Text2Vis, and more. It provides guidelines on converting natural language questions into structured SQL queries, with a focus on NL2SQL. The repository includes information on various models, datasets, evaluation metrics, fine-tuning methods, libraries, and practice projects related to Text2SQL. It serves as a comprehensive resource for individuals interested in working with Text2SQL and related technologies.
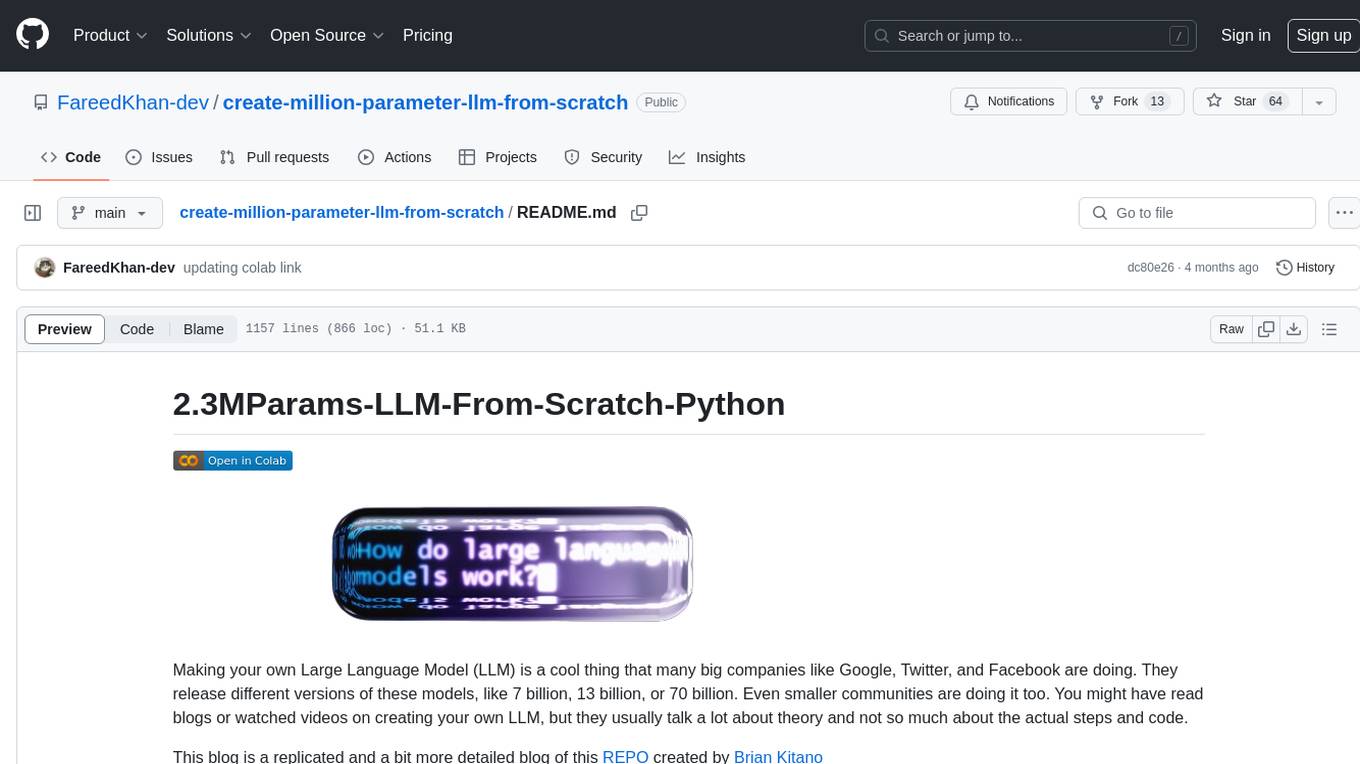
create-million-parameter-llm-from-scratch
The 'create-million-parameter-llm-from-scratch' repository provides a detailed guide on creating a Large Language Model (LLM) with 2.3 million parameters from scratch. The blog replicates the LLaMA approach, incorporating concepts like RMSNorm for pre-normalization, SwiGLU activation function, and Rotary Embeddings. The model is trained on a basic dataset to demonstrate the ease of creating a million-parameter LLM without the need for a high-end GPU.
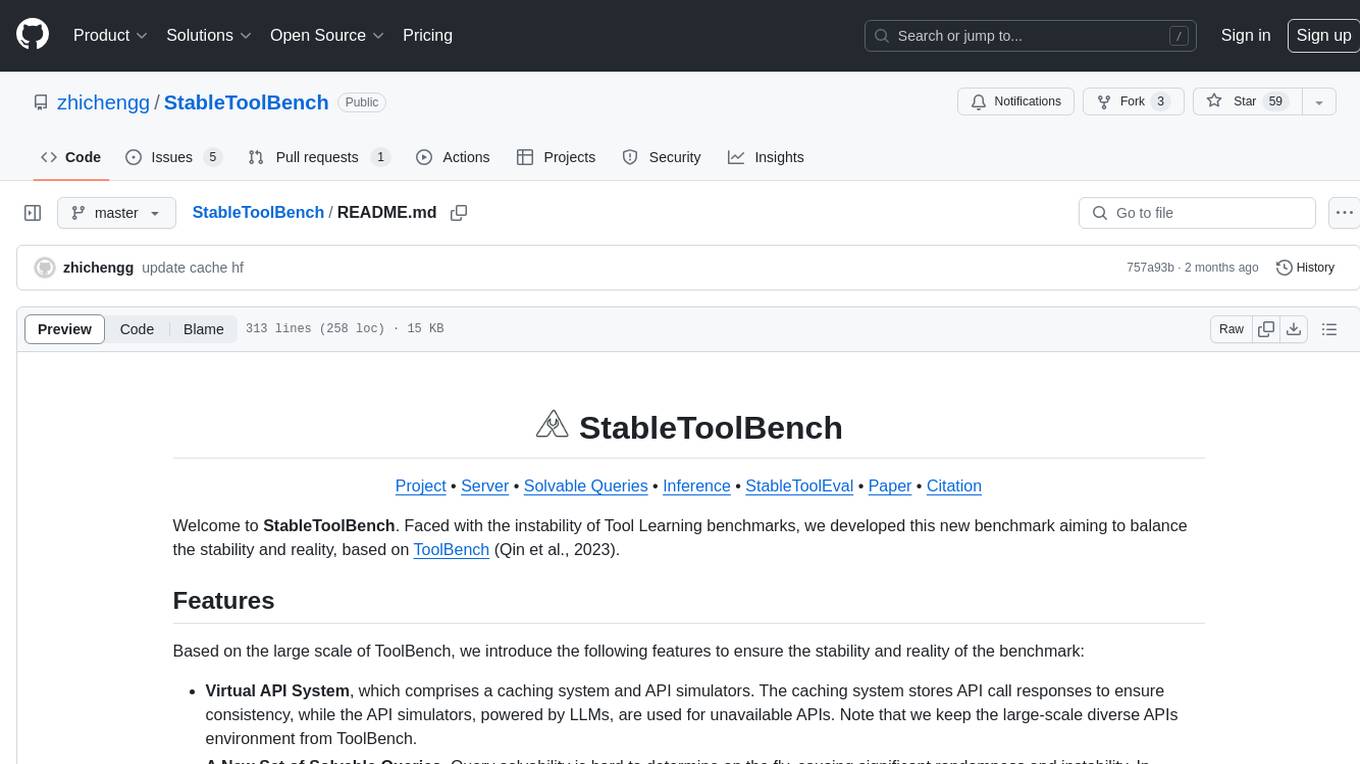
StableToolBench
StableToolBench is a new benchmark developed to address the instability of Tool Learning benchmarks. It aims to balance stability and reality by introducing features such as a Virtual API System with caching and API simulators, a new set of solvable queries determined by LLMs, and a Stable Evaluation System using GPT-4. The Virtual API Server can be set up either by building from source or using a prebuilt Docker image. Users can test the server using provided scripts and evaluate models with Solvable Pass Rate and Solvable Win Rate metrics. The tool also includes model experiments results comparing different models' performance.
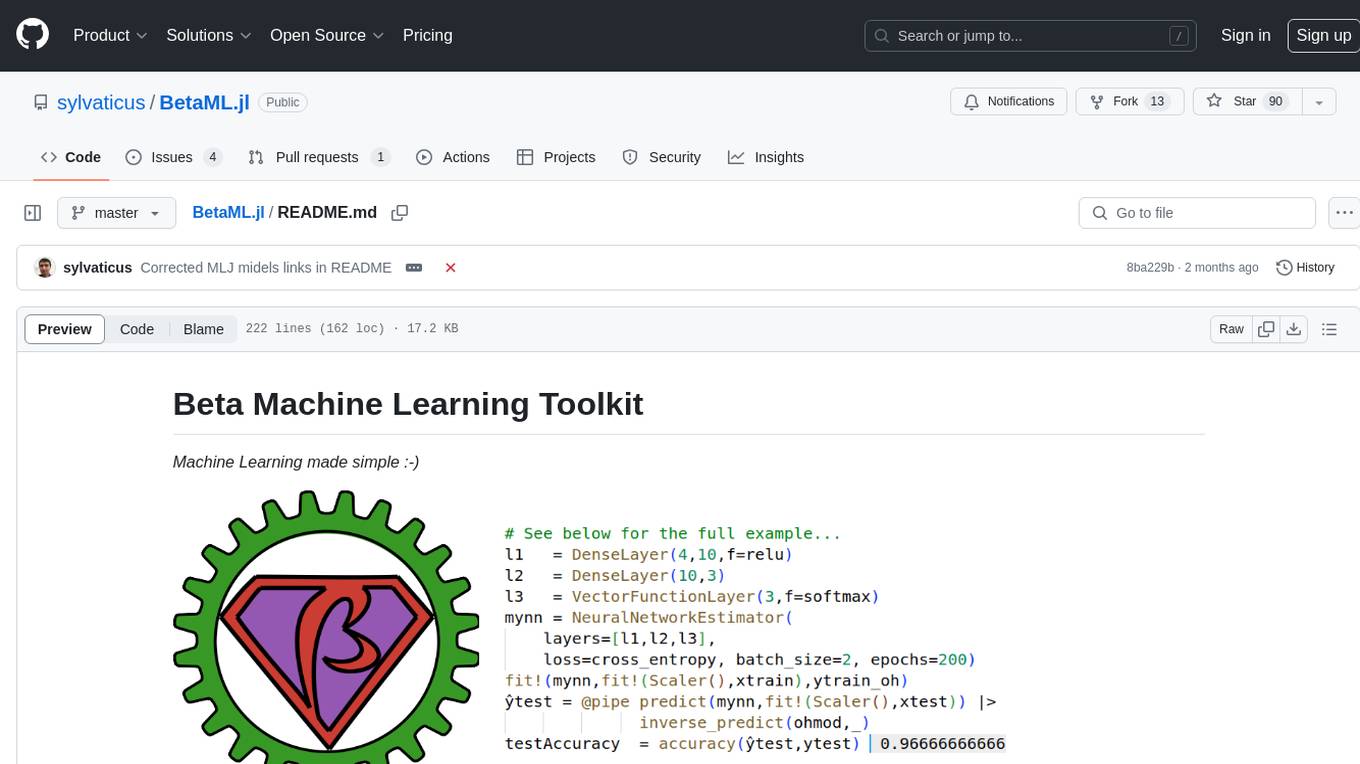
BetaML.jl
The Beta Machine Learning Toolkit is a package containing various algorithms and utilities for implementing machine learning workflows in multiple languages, including Julia, Python, and R. It offers a range of supervised and unsupervised models, data transformers, and assessment tools. The models are implemented entirely in Julia and are not wrappers for third-party models. Users can easily contribute new models or request implementations. The focus is on user-friendliness rather than computational efficiency, making it suitable for educational and research purposes.
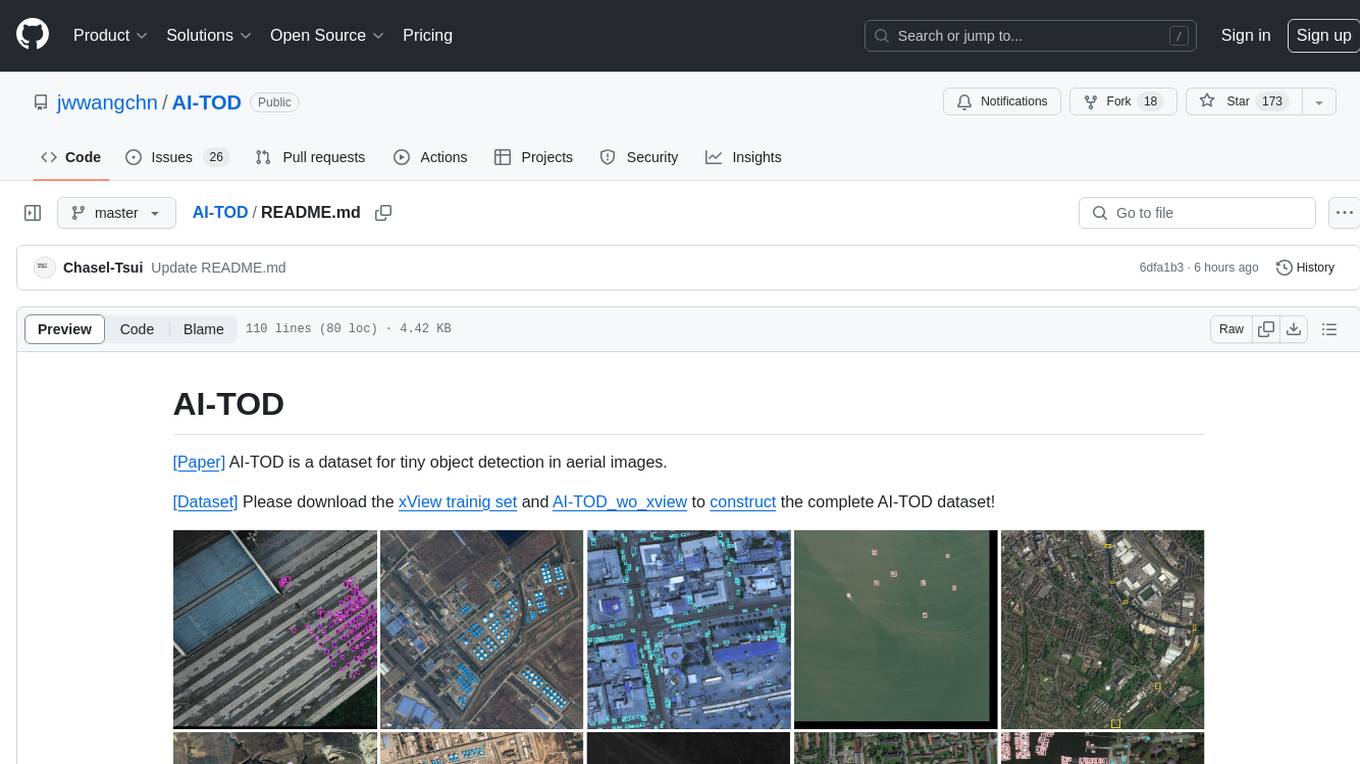
AI-TOD
AI-TOD is a dataset for tiny object detection in aerial images, containing 700,621 object instances across 28,036 images. Objects in AI-TOD are smaller with a mean size of 12.8 pixels compared to other aerial image datasets. To use AI-TOD, download xView training set and AI-TOD_wo_xview, then generate the complete dataset using the provided synthesis tool. The dataset is publicly available for academic and research purposes under CC BY-NC-SA 4.0 license.
For similar jobs
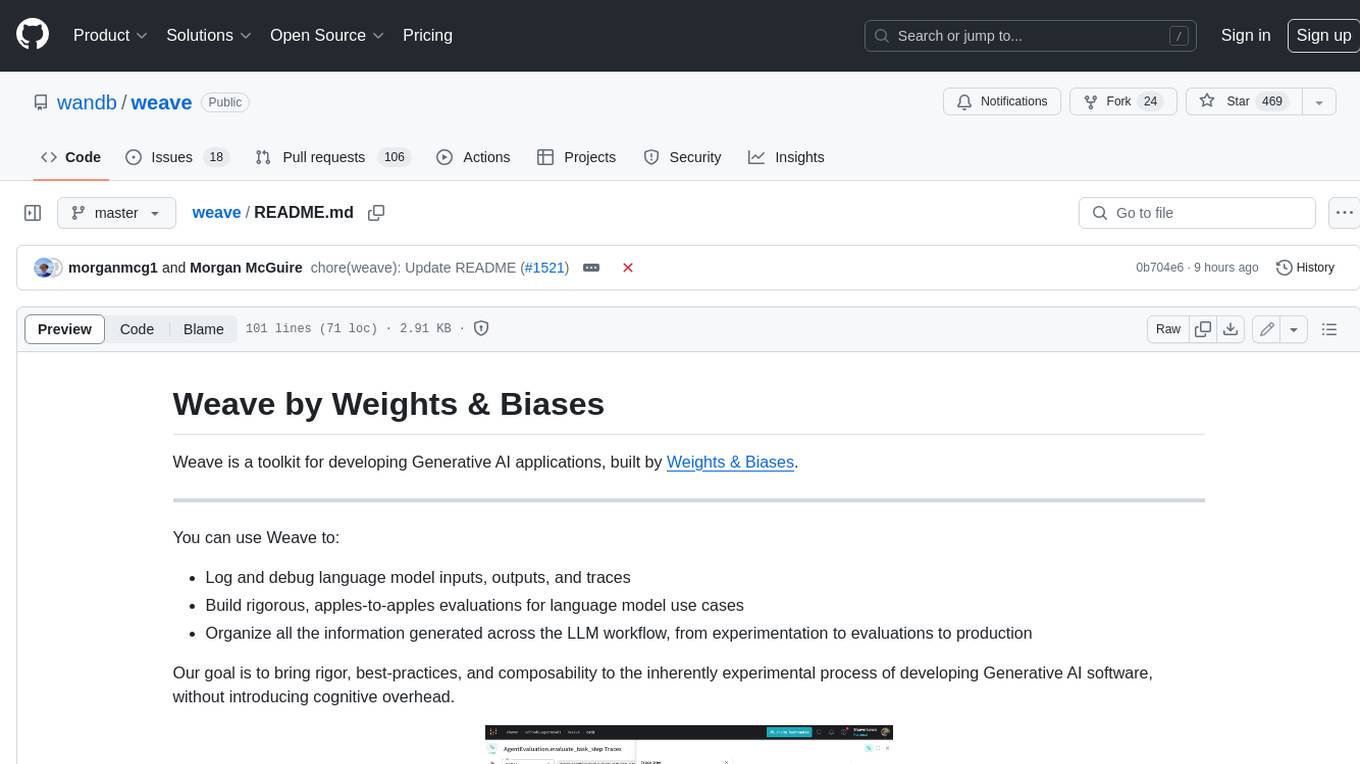
weave
Weave is a toolkit for developing Generative AI applications, built by Weights & Biases. With Weave, you can log and debug language model inputs, outputs, and traces; build rigorous, apples-to-apples evaluations for language model use cases; and organize all the information generated across the LLM workflow, from experimentation to evaluations to production. Weave aims to bring rigor, best-practices, and composability to the inherently experimental process of developing Generative AI software, without introducing cognitive overhead.
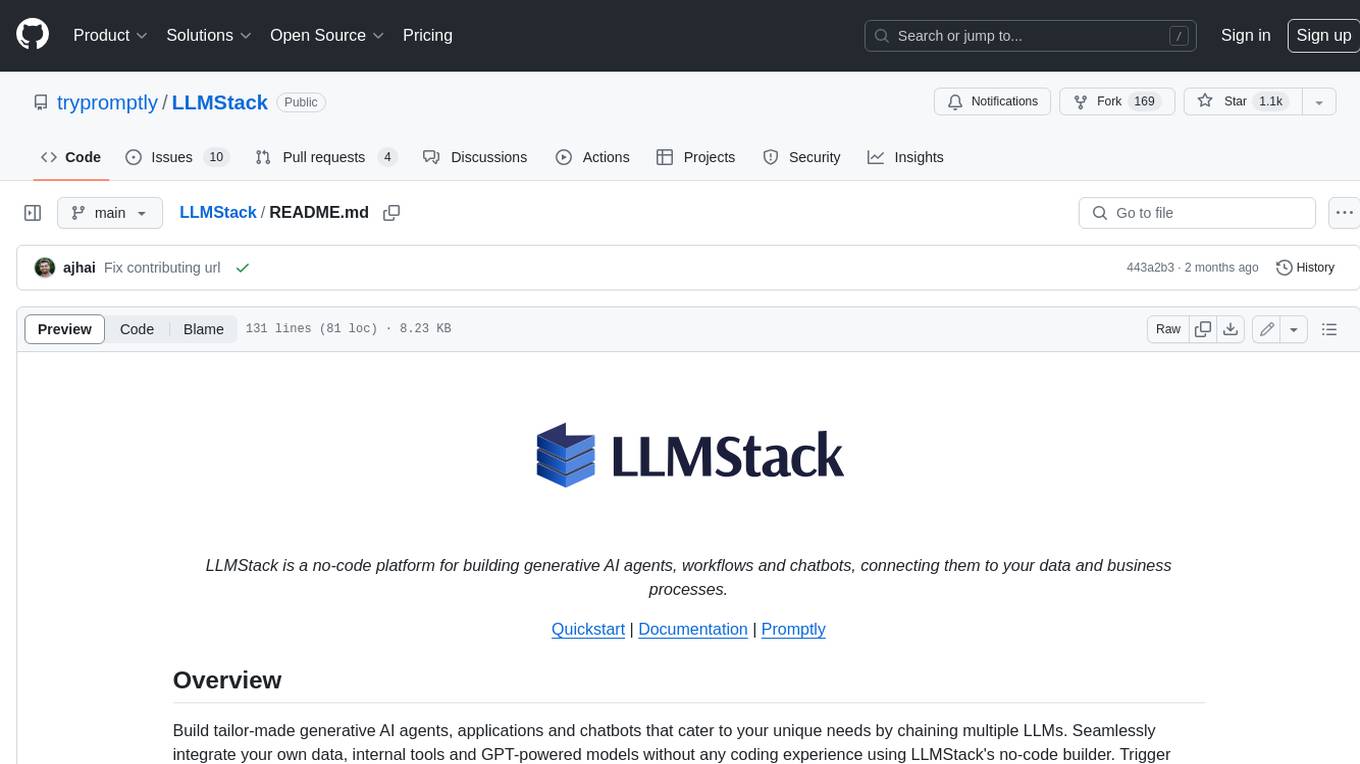
LLMStack
LLMStack is a no-code platform for building generative AI agents, workflows, and chatbots. It allows users to connect their own data, internal tools, and GPT-powered models without any coding experience. LLMStack can be deployed to the cloud or on-premise and can be accessed via HTTP API or triggered from Slack or Discord.
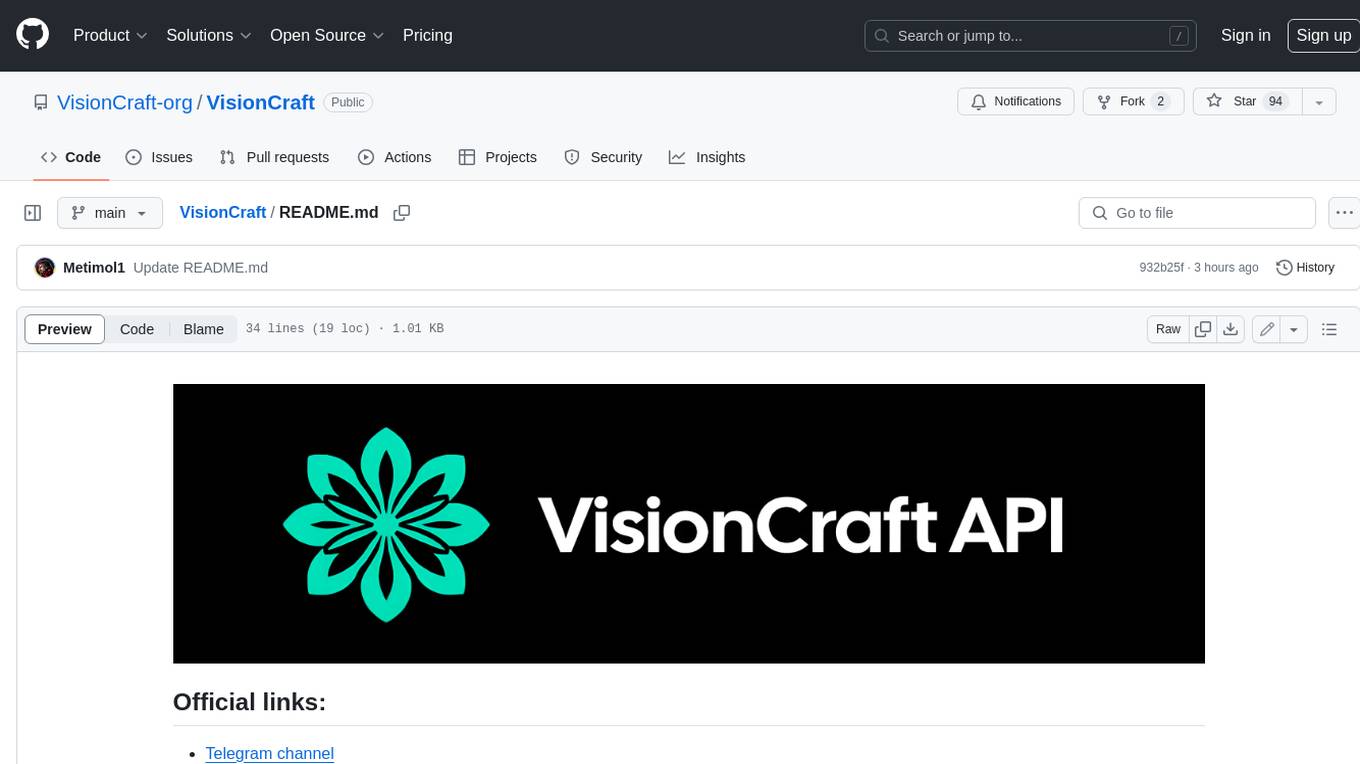
VisionCraft
The VisionCraft API is a free API for using over 100 different AI models. From images to sound.
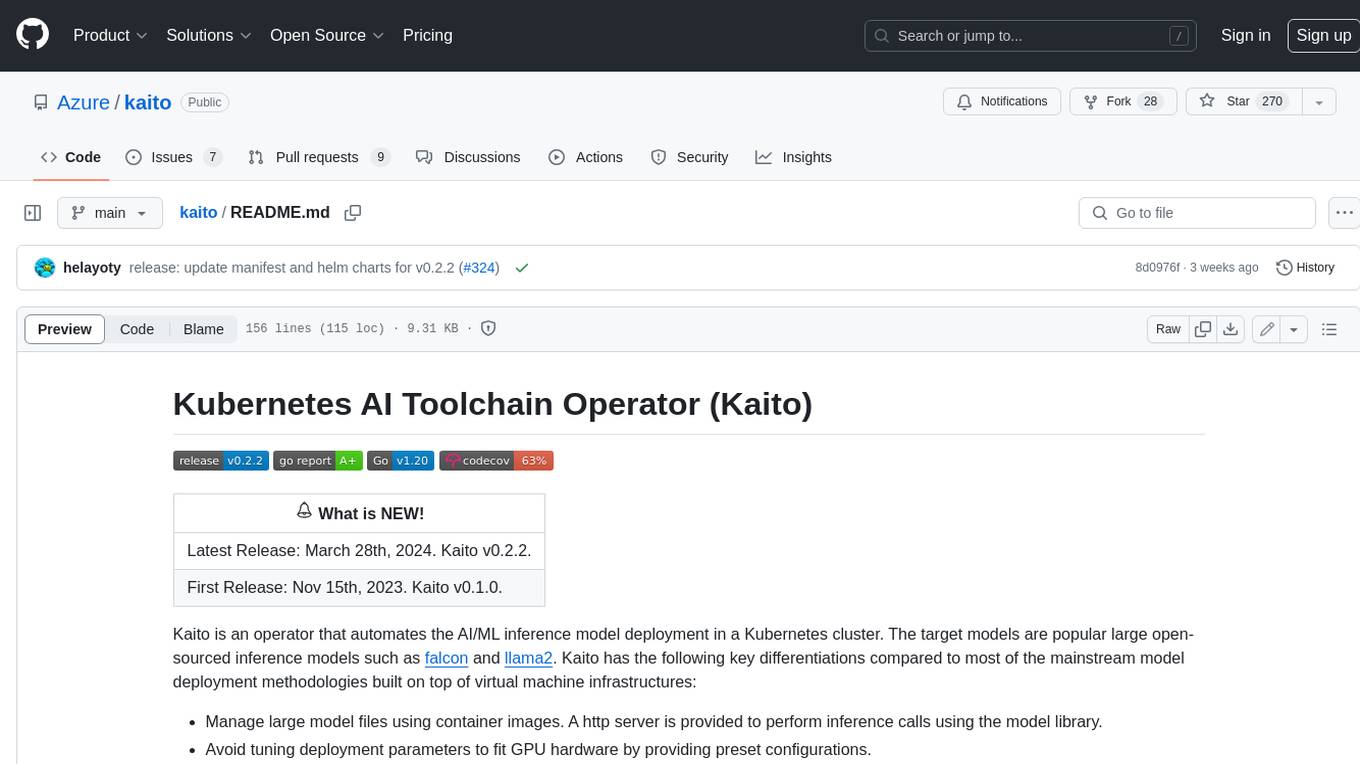
kaito
Kaito is an operator that automates the AI/ML inference model deployment in a Kubernetes cluster. It manages large model files using container images, avoids tuning deployment parameters to fit GPU hardware by providing preset configurations, auto-provisions GPU nodes based on model requirements, and hosts large model images in the public Microsoft Container Registry (MCR) if the license allows. Using Kaito, the workflow of onboarding large AI inference models in Kubernetes is largely simplified.
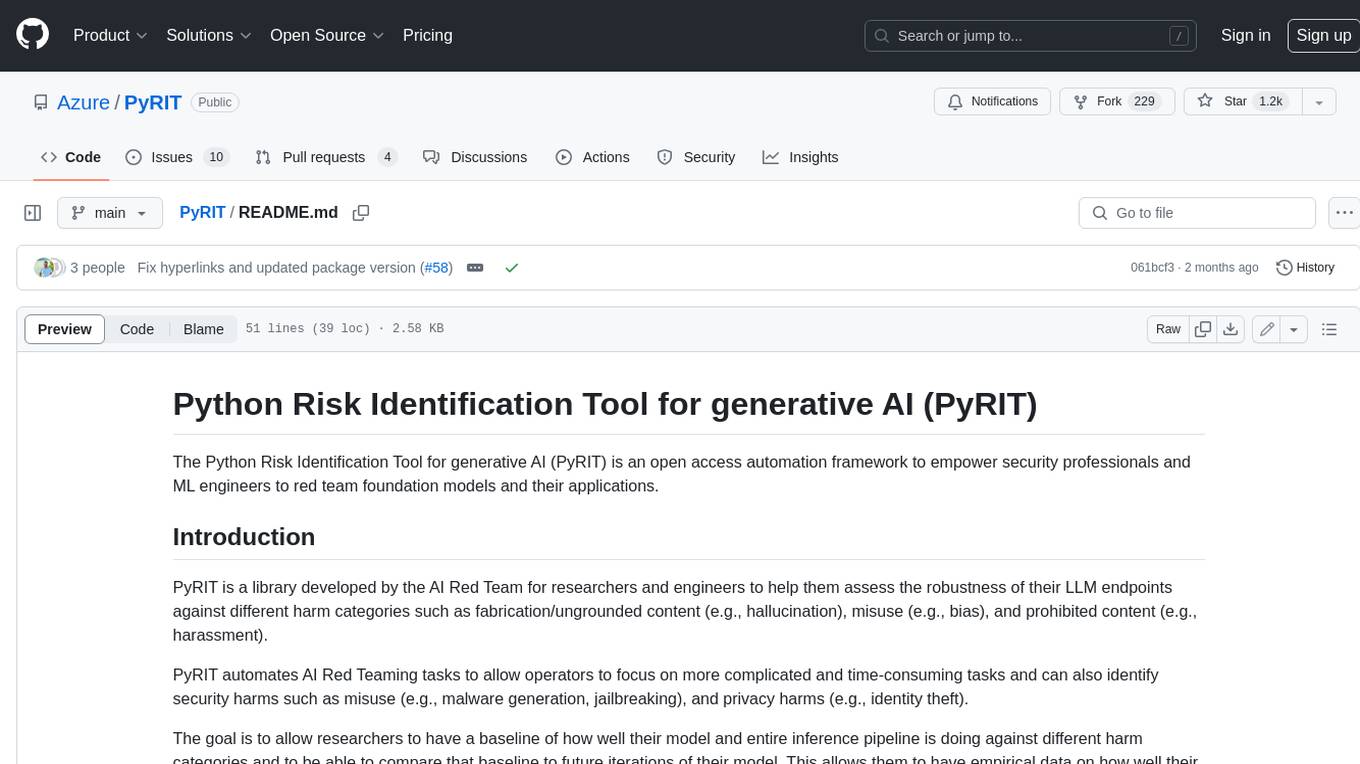
PyRIT
PyRIT is an open access automation framework designed to empower security professionals and ML engineers to red team foundation models and their applications. It automates AI Red Teaming tasks to allow operators to focus on more complicated and time-consuming tasks and can also identify security harms such as misuse (e.g., malware generation, jailbreaking), and privacy harms (e.g., identity theft). The goal is to allow researchers to have a baseline of how well their model and entire inference pipeline is doing against different harm categories and to be able to compare that baseline to future iterations of their model. This allows them to have empirical data on how well their model is doing today, and detect any degradation of performance based on future improvements.
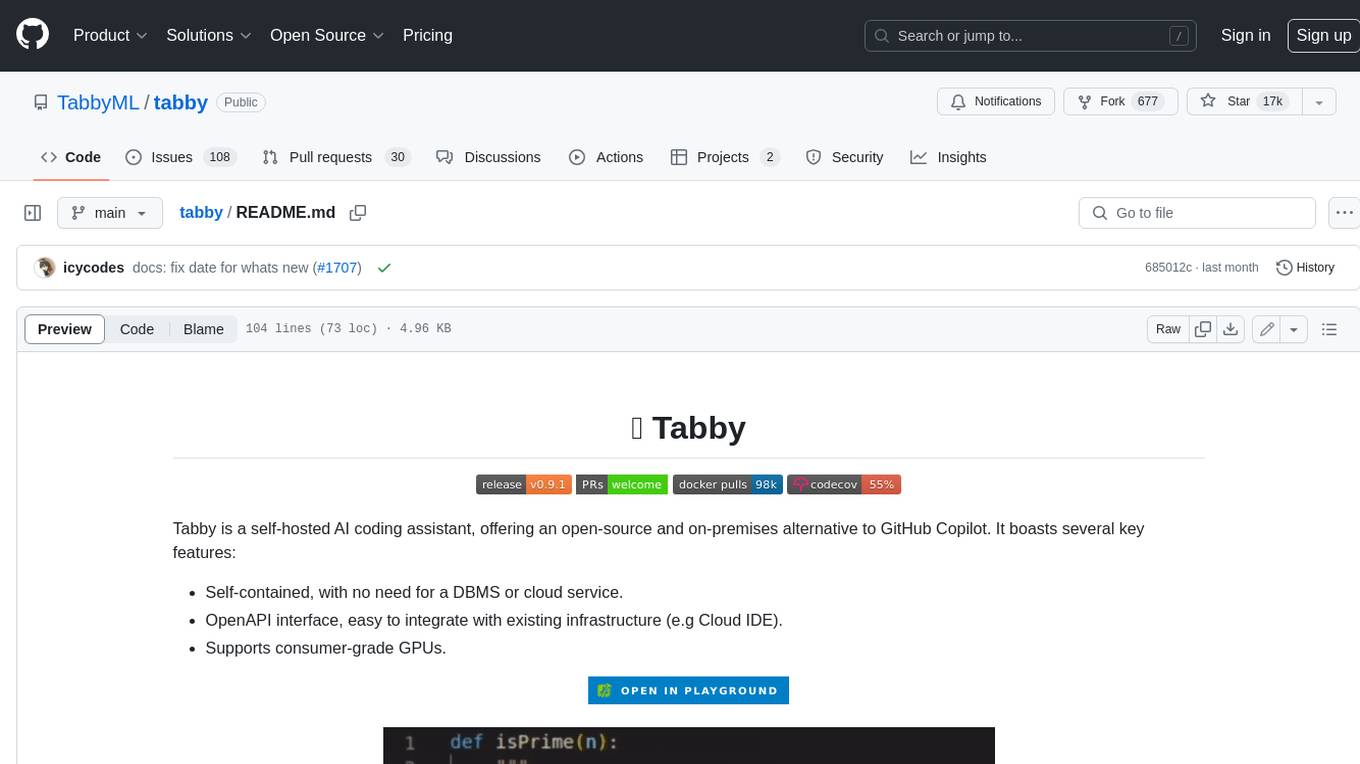
tabby
Tabby is a self-hosted AI coding assistant, offering an open-source and on-premises alternative to GitHub Copilot. It boasts several key features: * Self-contained, with no need for a DBMS or cloud service. * OpenAPI interface, easy to integrate with existing infrastructure (e.g Cloud IDE). * Supports consumer-grade GPUs.
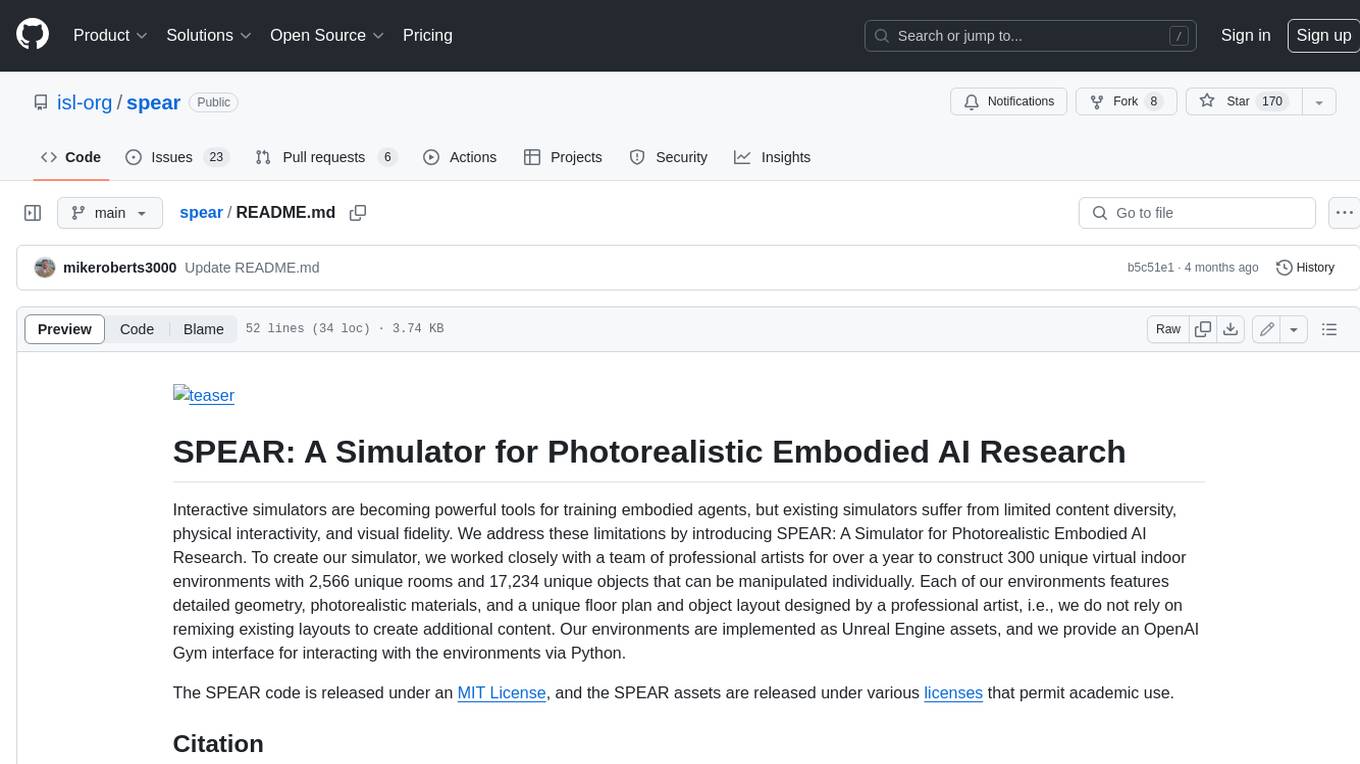
spear
SPEAR (Simulator for Photorealistic Embodied AI Research) is a powerful tool for training embodied agents. It features 300 unique virtual indoor environments with 2,566 unique rooms and 17,234 unique objects that can be manipulated individually. Each environment is designed by a professional artist and features detailed geometry, photorealistic materials, and a unique floor plan and object layout. SPEAR is implemented as Unreal Engine assets and provides an OpenAI Gym interface for interacting with the environments via Python.
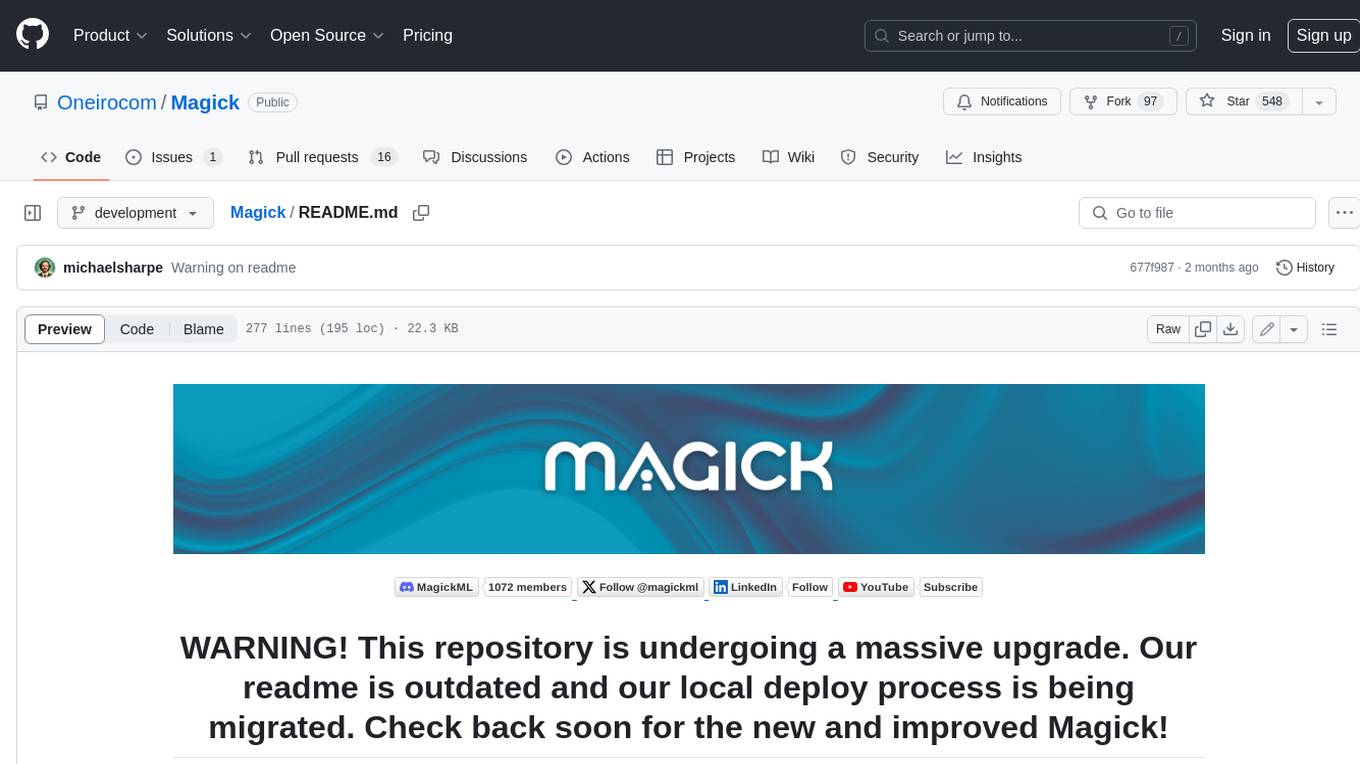
Magick
Magick is a groundbreaking visual AIDE (Artificial Intelligence Development Environment) for no-code data pipelines and multimodal agents. Magick can connect to other services and comes with nodes and templates well-suited for intelligent agents, chatbots, complex reasoning systems and realistic characters.