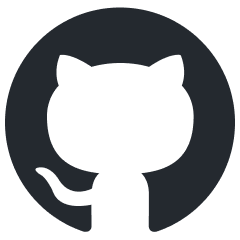
langgraph-studio
Desktop app for prototyping and debugging LangGraph applications locally.
Stars: 1491
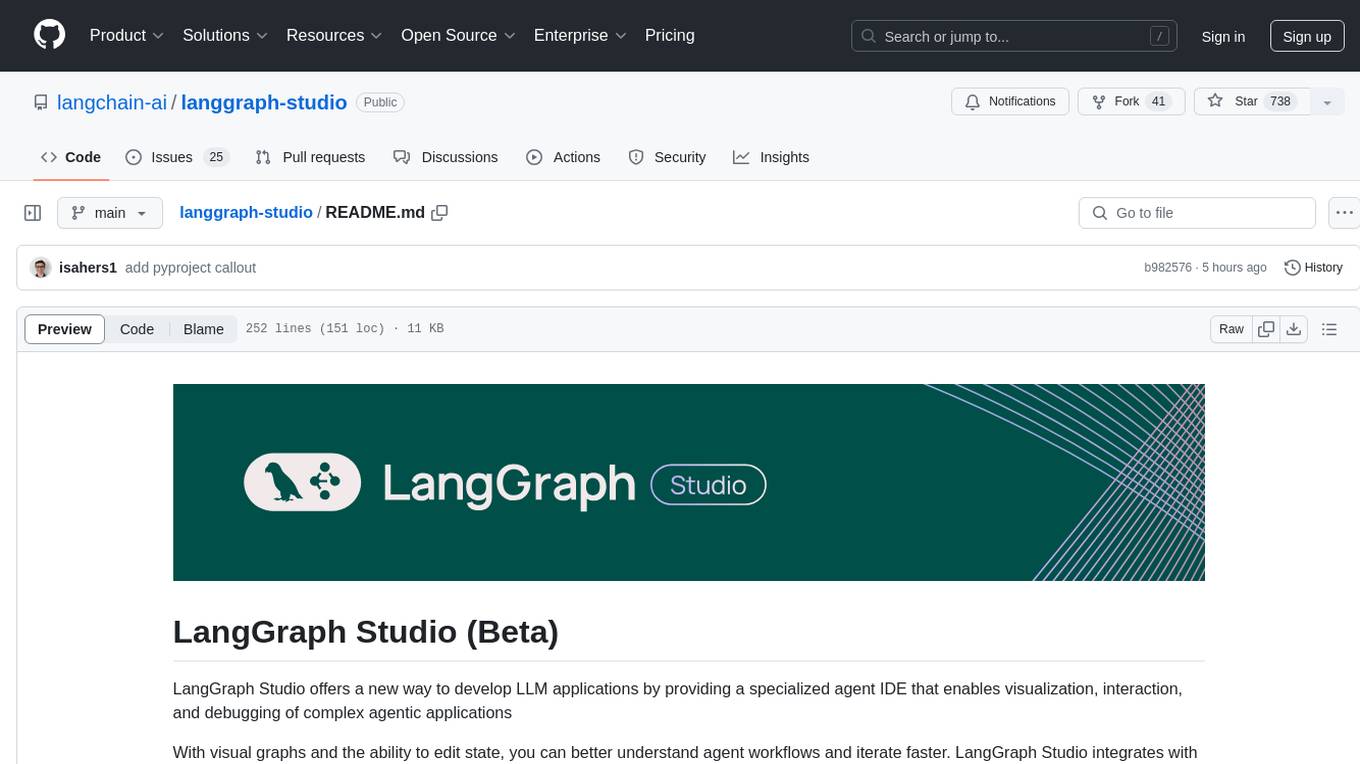
LangGraph Studio is a specialized agent IDE that enables visualization, interaction, and debugging of complex agentic applications. It offers visual graphs and state editing to better understand agent workflows and iterate faster. Users can collaborate with teammates using LangSmith to debug failure modes. The tool integrates with LangSmith and requires Docker installed. Users can create and edit threads, configure graph runs, add interrupts, and support human-in-the-loop workflows. LangGraph Studio allows interactive modification of project config and graph code, with live sync to the interactive graph for easier iteration on long-running agents.
README:
LangGraph Studio offers a new way to develop LLM applications by providing a specialized agent IDE that enables visualization, interaction, and debugging of complex agentic applications
With visual graphs and the ability to edit state, you can better understand agent workflows and iterate faster. LangGraph Studio integrates with LangSmith so you can collaborate with teammates to debug failure modes.
While in Beta, LangGraph Studio is available for free to all LangSmith users on any plan tier. Sign up for LangSmith here.
Download the latest .dmg
file of LangGraph Studio by clicking here or by visiting the releases page.
Currently, only macOS is supported. Windows and Linux support is coming soon. We also depend on Docker Engine to be running, currently we only support the following runtimes:
LangGraph Studio requires docker-compose version 2.22.0+ or higher. Please make sure you have Docker Desktop or Orbstack installed and running before continuing.
To use LangGraph Studio, make sure you have a project with a LangGraph app set up.
For this example, we will use this example repository here which uses a requirements.txt
file for dependencies:
git clone https://github.com/langchain-ai/langgraph-example.git
If you would like to use a pyproject.toml
file instead for managing dependencies, you can use this example repository.
git clone https://github.com/langchain-ai/langgraph-example-pyproject.git
You will then want to create a .env
file with the relevant environment variables:
cp .env.example .env
You should then open up the .env
file and fill in with relevant OpenAI, Anthropic, and Tavily API keys.
If you already have them set in your environment, you can save them to this .env file with the following commands:
echo "OPENAI_API_KEY=\"$OPENAI_API_KEY\"" > .env
echo "ANTHROPIC_API_KEY=\"$ANTHROPIC_API_KEY\"" >> .env
echo "TAVILY_API_KEY=\"$TAVILY_API_KEY\"" >> .env
Note: do NOT add a LANGSMITH_API_KEY to the .env file. We will do this automatically for you when you authenticate, and manually setting this may cause errors.
Once you've set up the project, you can use it in LangGraph Studio. Let's dive in!
When you open LangGraph Studio desktop app for the first time, you need to login via LangSmith.
Once you have successfully authenticated, you can choose the LangGraph application folder to use — you can either drag and drop or manually select it in the file picker. If you are using the example project, the folder would be langgraph-example
.
[!IMPORTANT] The application directory you select needs to contain correctly configured
langgraph.json
file. See more information on how to configure it here and how to set up a LangGraph app here.
Once you select a valid project, LangGraph Studio will start a LangGraph API server and you should see a UI with your graph rendered.
Now we can run the graph! LangGraph Studio lets you run your graph with different inputs and configurations.
To start a new run:
- In the dropdown menu (top-left corner of the left-hand pane), select a graph. In our example the graph is called
agent
. The list of graphs corresponds to thegraphs
keys in yourlanggraph.json
configuration. - In the bottom of the left-hand pane, edit the
Input
section. - Click
Submit
to invoke the selected graph. - View output of the invocation in the right-hand pane.
The following video shows how to start a new run:
https://github.com/user-attachments/assets/e0e7487e-17e2-4194-a4ad-85b346c2f1c4
To change configuration for a given graph run, press Configurable
button in the Input
section. Then click Submit
to invoke the graph.
[!IMPORTANT] In order for the
Configurable
menu to be visible, make sure to specify config schema when creatingStateGraph
. You can read more about how to add config schema to your graph here.
The following video shows how to edit configuration and start a new run:
https://github.com/user-attachments/assets/8495b476-7e33-42d4-85cb-2f9269bea20c
When you open LangGraph Studio, you will automatically be in a new thread window. If you have an existing thread open, follow these steps to create a new thread:
- In the top-right corner of the right-hand pane, press
+
to open a new thread menu.
The following video shows how to create a thread:
https://github.com/user-attachments/assets/78d4a692-2042-48e2-a7e2-5a7ca3d5a611
To select a thread:
- Click on
New Thread
/Thread <thread-id>
label at the top of the right-hand pane to open a thread list dropdown. - Select a thread that you wish to view / edit.
The following video shows how to select a thread:
https://github.com/user-attachments/assets/5f0dbd63-fa59-4496-8d8e-4fb8d0eab893
LangGraph Studio allows you to edit the thread state and fork the threads to create alternative graph execution with the updated state. To do it:
- Select a thread you wish to edit.
- In the right-hand pane hover over the step you wish to edit and click on "pencil" icon to edit.
- Make your edits.
- Click
Fork
to update the state and create a new graph execution with the updated state.
The following video shows how to edit a thread in the studio:
https://github.com/user-attachments/assets/47f887e7-2e3f-46ce-977c-f474c3cd797e
You might want to execute your graph step by step, or stop graph execution before/after a specific node executes. You can do so by adding interrupts. Interrupts can be set for all nodes (i.e. walk through the agent execution step by step) or for specific nodes. An interrupt in LangGraph Studio means that the graph execution will be interrupted both before and after a given node runs.
To walk through the agent execution step by step, you can add interrupts to a all or a subset of nodes in the graph:
- In the dropdown menu (top-right corner of the left-hand pane), click
Interrupt
. - Select a subset of nodes to interrupt on, or click
Interrupt on all
.
The following video shows how to add interrupts to all nodes:
https://github.com/user-attachments/assets/db44ebda-4d6e-482d-9ac8-ea8f5f0148ea
- Navigate to the left-hand pane with the graph visualization.
- Hover over a node you want to add an interrupt to. You should see a
+
button show up on the left side of the node. - Click
+
to invoke the selected graph. - Run the graph by adding
Input
/ configuration and clickingSubmit
The following video shows how to add interrupts to a specific node:
https://github.com/user-attachments/assets/13429609-18fc-4f21-9cb9-4e0daeea62c4
To remove the interrupt, simply follow the same step and press x
button on the left side of the node.
In addition to interrupting on a node and editing the graph state, you might want to support human-in-the-loop workflows with the ability to manually update state. Here is a modified version of agent.py
with agent
and human
nodes, where the graph execution will be interrupted on human
node. This will let you send input as part of the human
node. This can be useful when you want the agent to get user input. This essentially replaces how you might use input()
if you were running this from the command line.
from typing import TypedDict, Annotated, Sequence, Literal
from langchain_core.messages import BaseMessage, HumanMessage
from langchain_anthropic import ChatAnthropic
from langgraph.graph import StateGraph, END, add_messages
class AgentState(TypedDict):
messages: Annotated[Sequence[BaseMessage], add_messages]
model = ChatAnthropic(temperature=0, model_name="claude-3-sonnet-20240229")
def call_model(state: AgentState) -> AgentState:
messages = state["messages"]
response = model.invoke(messages)
return {"messages": [response]}
# no-op node that should be interrupted on
def human_feedback(state: AgentState) -> AgentState:
pass
def should_continue(state: AgentState) -> Literal["agent", "end"]:
messages = state['messages']
last_message = messages[-1]
if isinstance(last_message, HumanMessage):
return "agent"
return "end"
workflow = StateGraph(AgentState)
workflow.set_entry_point("agent")
workflow.add_node("agent", call_model)
workflow.add_node("human", human_feedback)
workflow.add_edge("agent", "human")
workflow.add_conditional_edges(
"human",
should_continue,
{
"agent": "agent",
"end": END,
},
)
graph = workflow.compile(interrupt_before=["human"])
The following video shows how to manually send state updates (i.e. messages in our example) when interrupted:
https://github.com/user-attachments/assets/f6d4fd18-df4d-45b7-8b1b-ad8506d08abd
LangGraph Studio allows you to modify your project config (langgraph.json
) interactively.
To modify the config from the studio, follow these steps:
- Click
Configure
on the bottom right. This will open an interactive config menu with the values that correspond to the existinglanggraph.json
. - Make your edits.
- Click
Save and Restart
to reload the LangGraph API server with the updated config.
The following video shows how to edit project config from the studio:
https://github.com/user-attachments/assets/86d7d1f7-800c-4739-80bc-8122b4728817
With LangGraph Studio you can modify your graph code and sync the changes live to the interactive graph.
To modify your graph from the studio, follow these steps:
- Click
Open in VS Code
on the bottom right. This will open the project that is currently opened in LangGraph studio. - Make changes to the
.py
files where the compiled graph is defined or associated dependencies. - LangGraph studio will automatically reload once the changes are saved in the project directory.
The following video shows how to open code editor from the studio:
https://github.com/user-attachments/assets/8ac0443d-460b-438e-a379-182ec9f68ff5
After you modify the underlying code you can also replay a node in the graph. For example, if an agent responds poorly, you can update the agent node implementation in your code editor and rerun it. This can make it much easier to iterate on long-running agents.
https://github.com/user-attachments/assets/9ec1b8ed-c6f8-433d-8bef-0dbda58a1075
For Tasks:
Click tags to check more tools for each tasksFor Jobs:
Alternative AI tools for langgraph-studio
Similar Open Source Tools
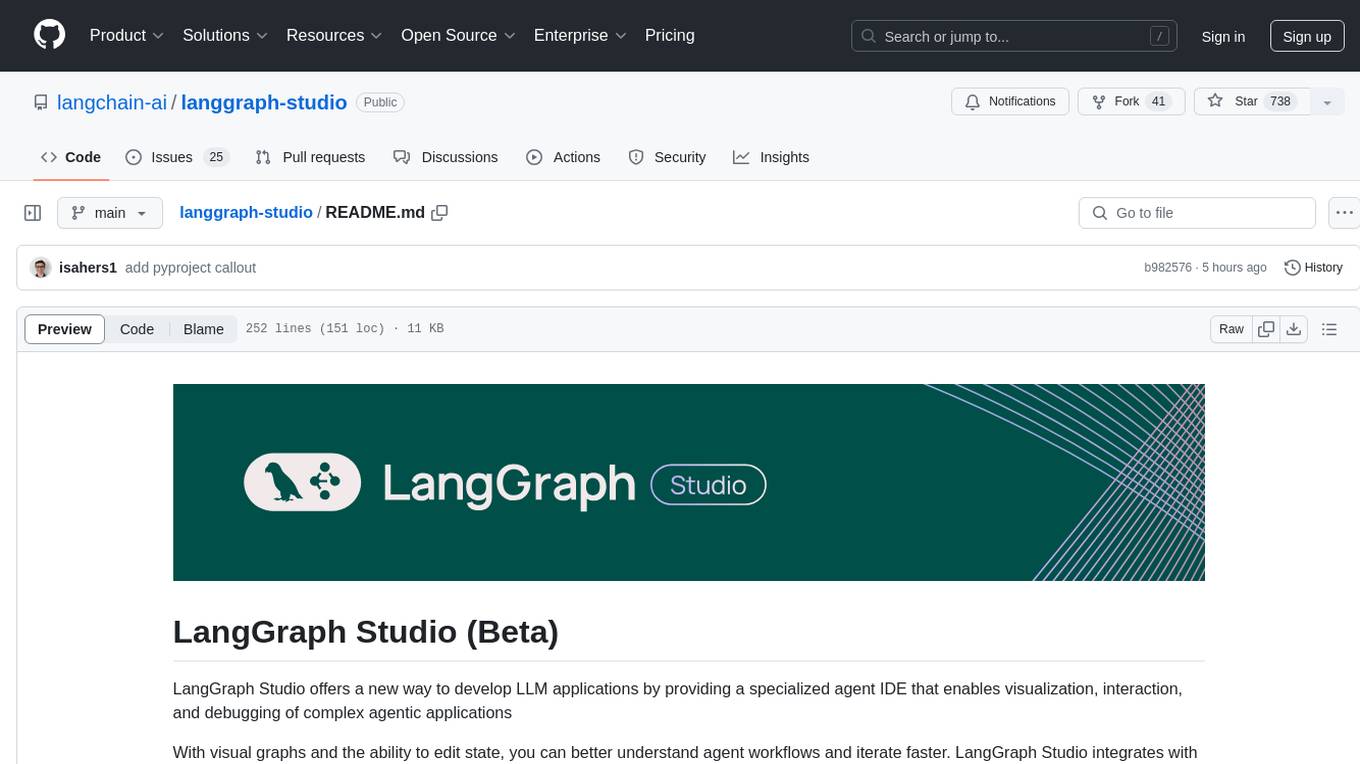
langgraph-studio
LangGraph Studio is a specialized agent IDE that enables visualization, interaction, and debugging of complex agentic applications. It offers visual graphs and state editing to better understand agent workflows and iterate faster. Users can collaborate with teammates using LangSmith to debug failure modes. The tool integrates with LangSmith and requires Docker installed. Users can create and edit threads, configure graph runs, add interrupts, and support human-in-the-loop workflows. LangGraph Studio allows interactive modification of project config and graph code, with live sync to the interactive graph for easier iteration on long-running agents.
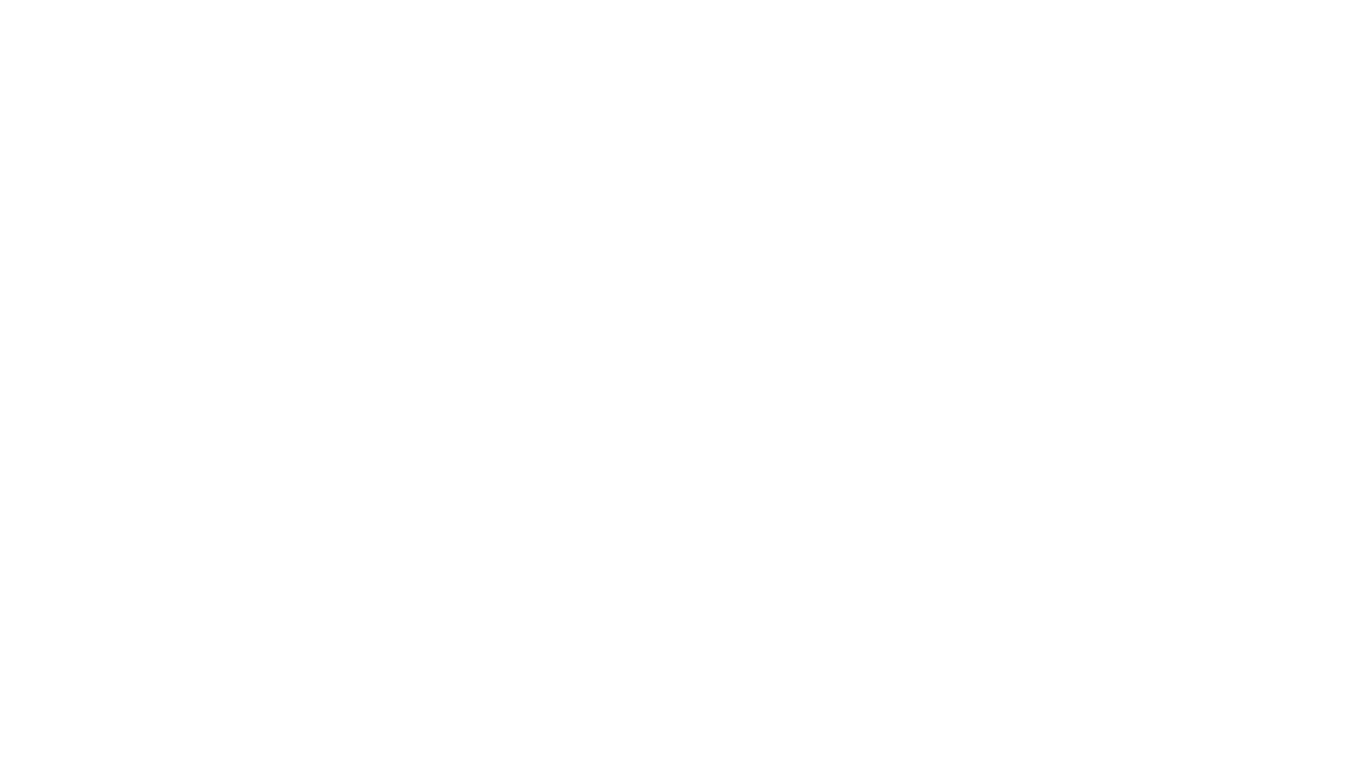
brokk
Brokk is a code assistant designed to understand code semantically, allowing LLMs to work effectively on large codebases. It offers features like agentic search, summarizing related classes, parsing stack traces, adding source for usages, and autonomously fixing errors. Users can interact with Brokk through different panels and commands, enabling them to manipulate context, ask questions, search codebase, run shell commands, and more. Brokk helps with tasks like debugging regressions, exploring codebase, AI-powered refactoring, and working with dependencies. It is particularly useful for making complex, multi-file edits with o1pro.
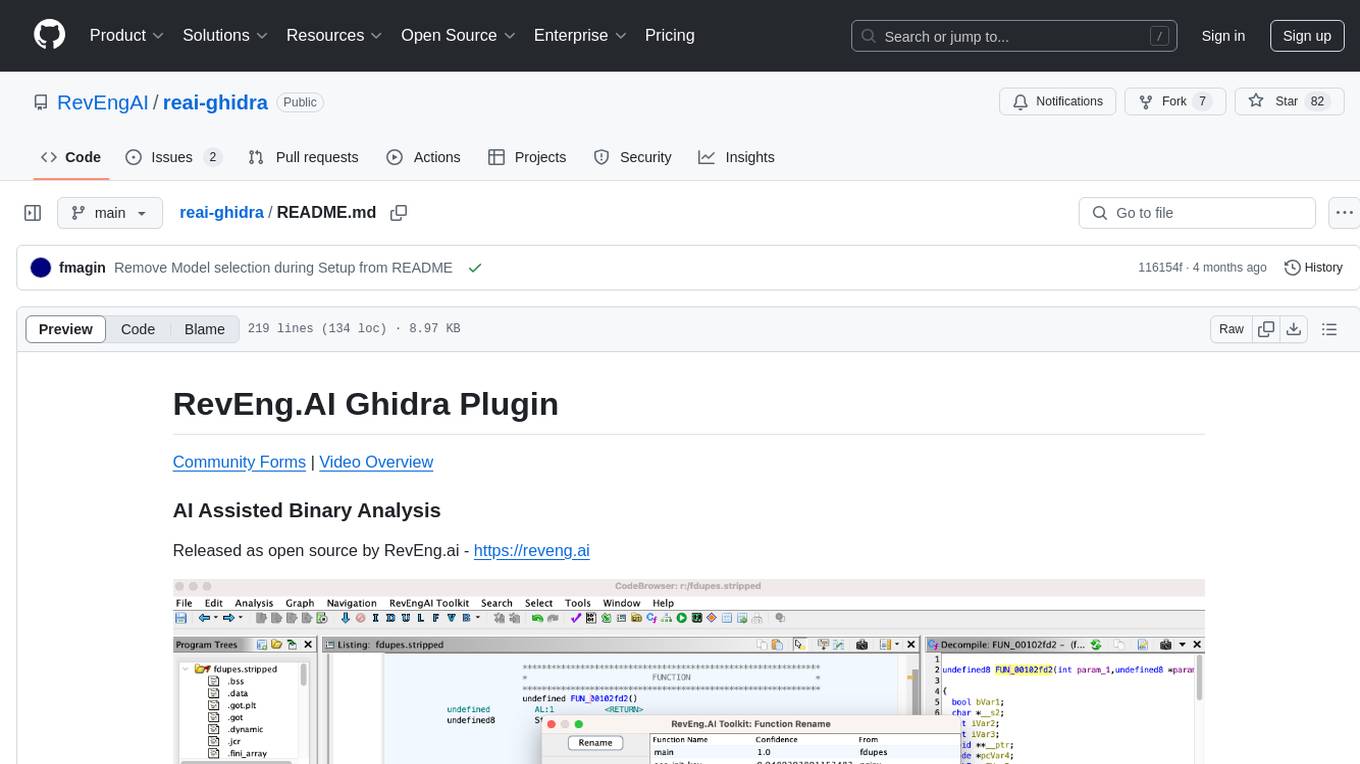
reai-ghidra
The RevEng.AI Ghidra Plugin by RevEng.ai allows users to interact with their API within Ghidra for Binary Code Similarity analysis to aid in Reverse Engineering stripped binaries. Users can upload binaries, rename functions above a confidence threshold, and view similar functions for a selected function.
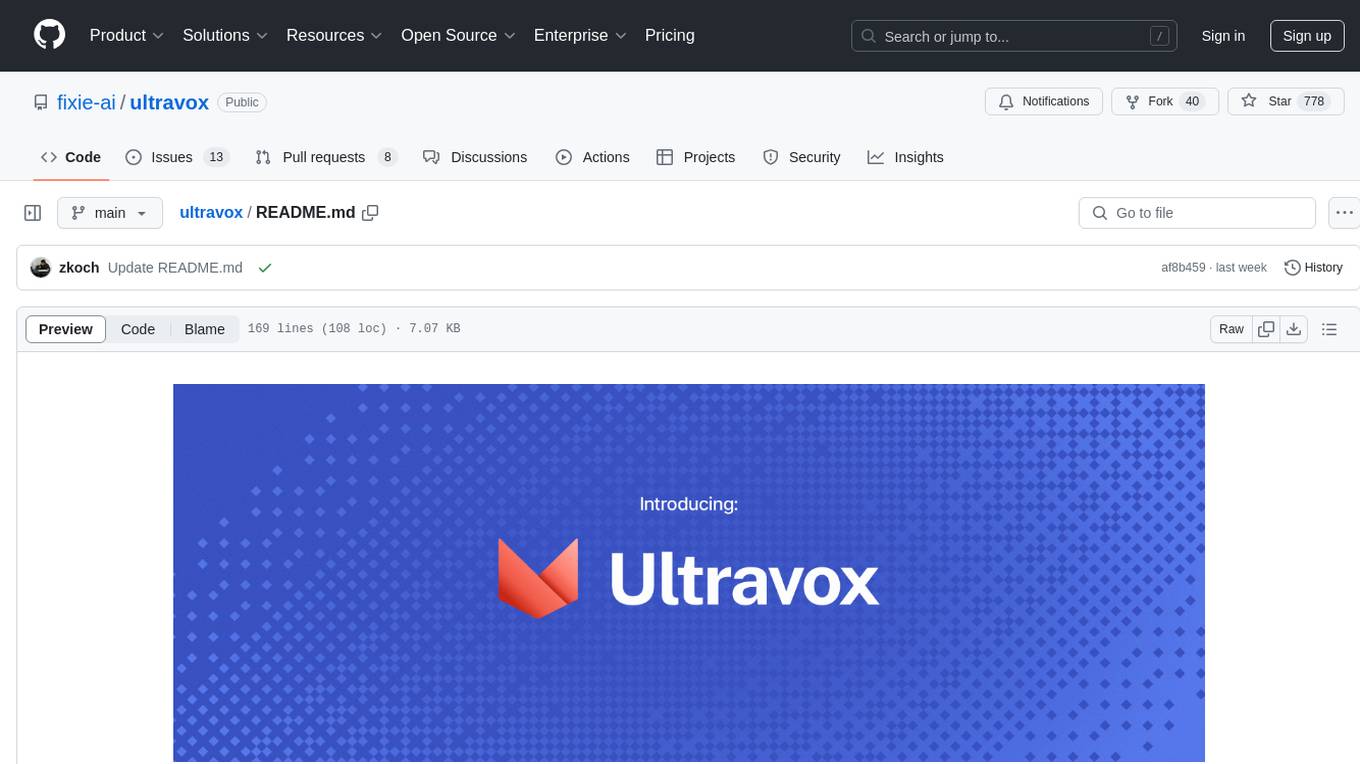
ultravox
Ultravox is a fast multimodal Language Model (LLM) that can understand both text and human speech in real-time without the need for a separate Audio Speech Recognition (ASR) stage. By extending Meta's Llama 3 model with a multimodal projector, Ultravox converts audio directly into a high-dimensional space used by Llama 3, enabling quick responses and potential understanding of paralinguistic cues like timing and emotion in human speech. The current version (v0.3) has impressive speed metrics and aims for further enhancements. Ultravox currently converts audio to streaming text and plans to emit speech tokens for direct audio conversion. The tool is open for collaboration to enhance this functionality.
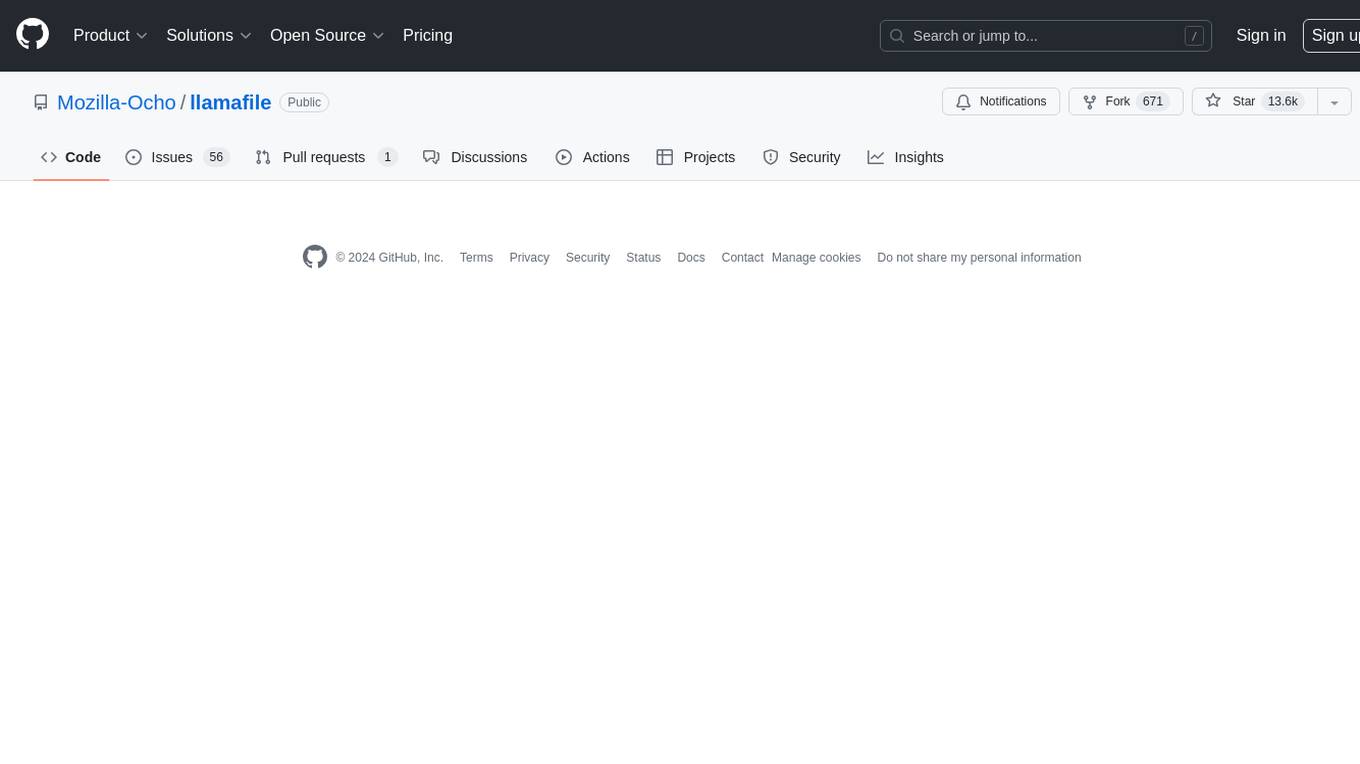
llamafile
llamafile is a tool that enables users to distribute and run Large Language Models (LLMs) with a single file. It combines llama.cpp with Cosmopolitan Libc to create a framework that simplifies the complexity of LLMs into a single-file executable called a 'llamafile'. Users can run these executable files locally on most computers without the need for installation, making open LLMs more accessible to developers and end users. llamafile also provides example llamafiles for various LLM models, allowing users to try out different LLMs locally. The tool supports multiple CPU microarchitectures, CPU architectures, and operating systems, making it versatile and easy to use.
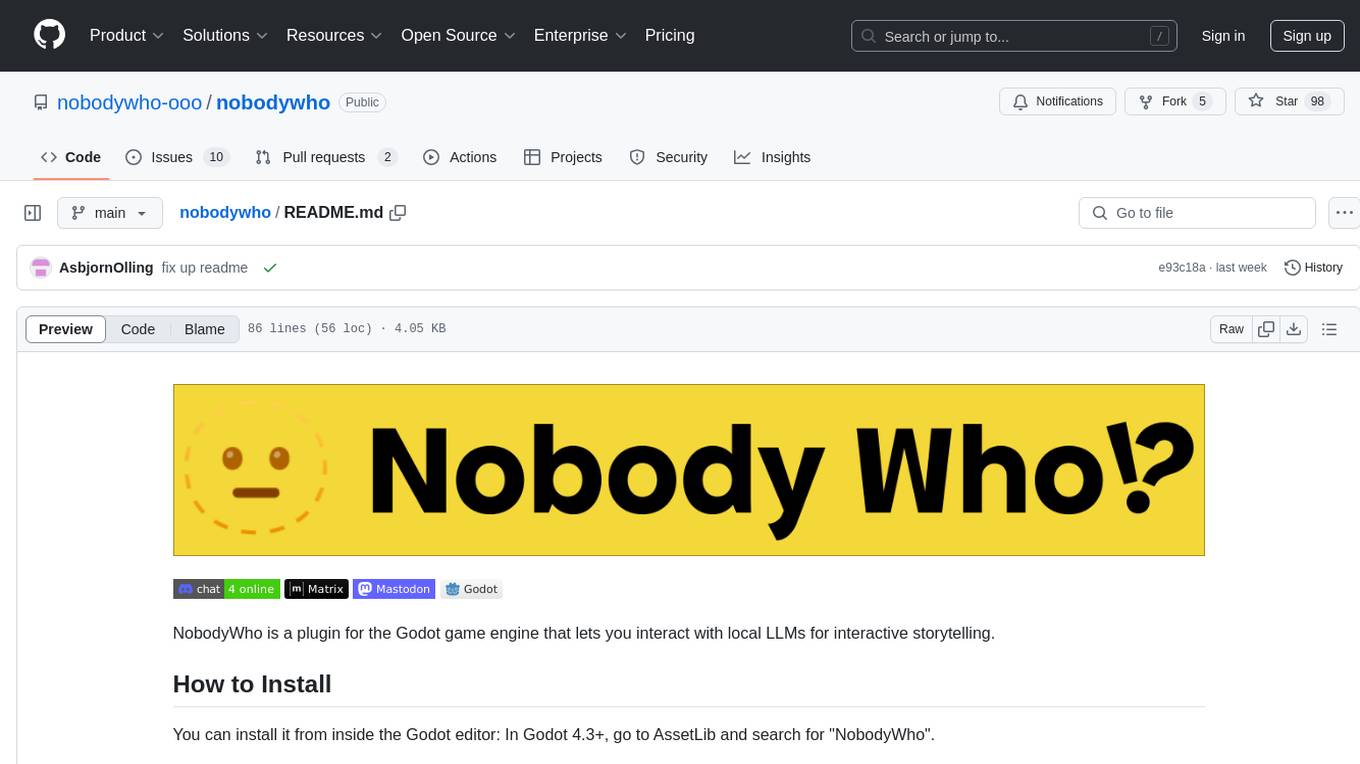
nobodywho
NobodyWho is a plugin for the Godot game engine that enables interaction with local LLMs for interactive storytelling. Users can install it from Godot editor or GitHub releases page, providing their own LLM in GGUF format. The plugin consists of `NobodyWhoModel` node for model file, `NobodyWhoChat` node for chat interaction, and `NobodyWhoEmbedding` node for generating embeddings. It offers a programming interface for sending text to LLM, receiving responses, and starting the LLM worker.
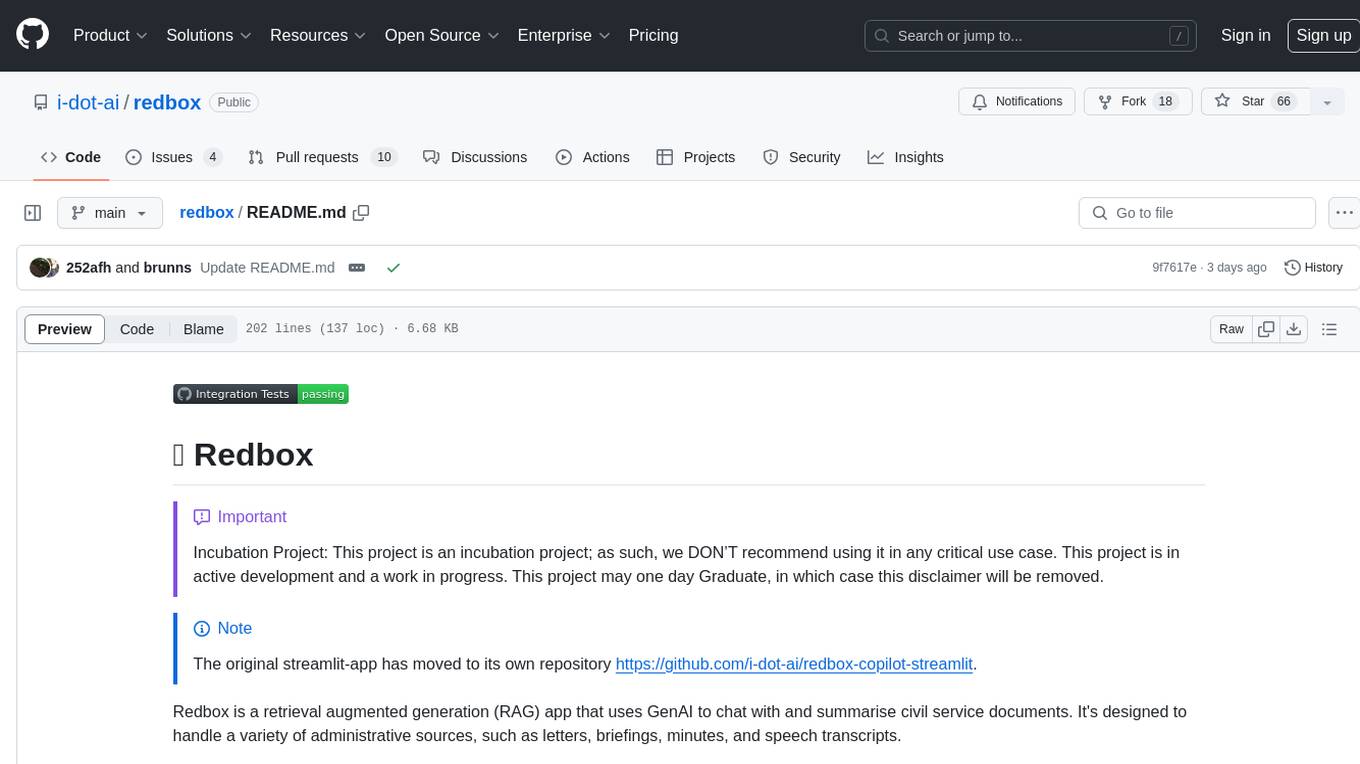
redbox
Redbox is a retrieval augmented generation (RAG) app that uses GenAI to chat with and summarise civil service documents. It increases organisational memory by indexing documents and can summarise reports read months ago, supplement them with current work, and produce a first draft that lets civil servants focus on what they do best. The project uses a microservice architecture with each microservice running in its own container defined by a Dockerfile. Dependencies are managed using Python Poetry. Contributions are welcome, and the project is licensed under the MIT License. Security measures are in place to ensure user data privacy and considerations are being made to make the core-api secure.
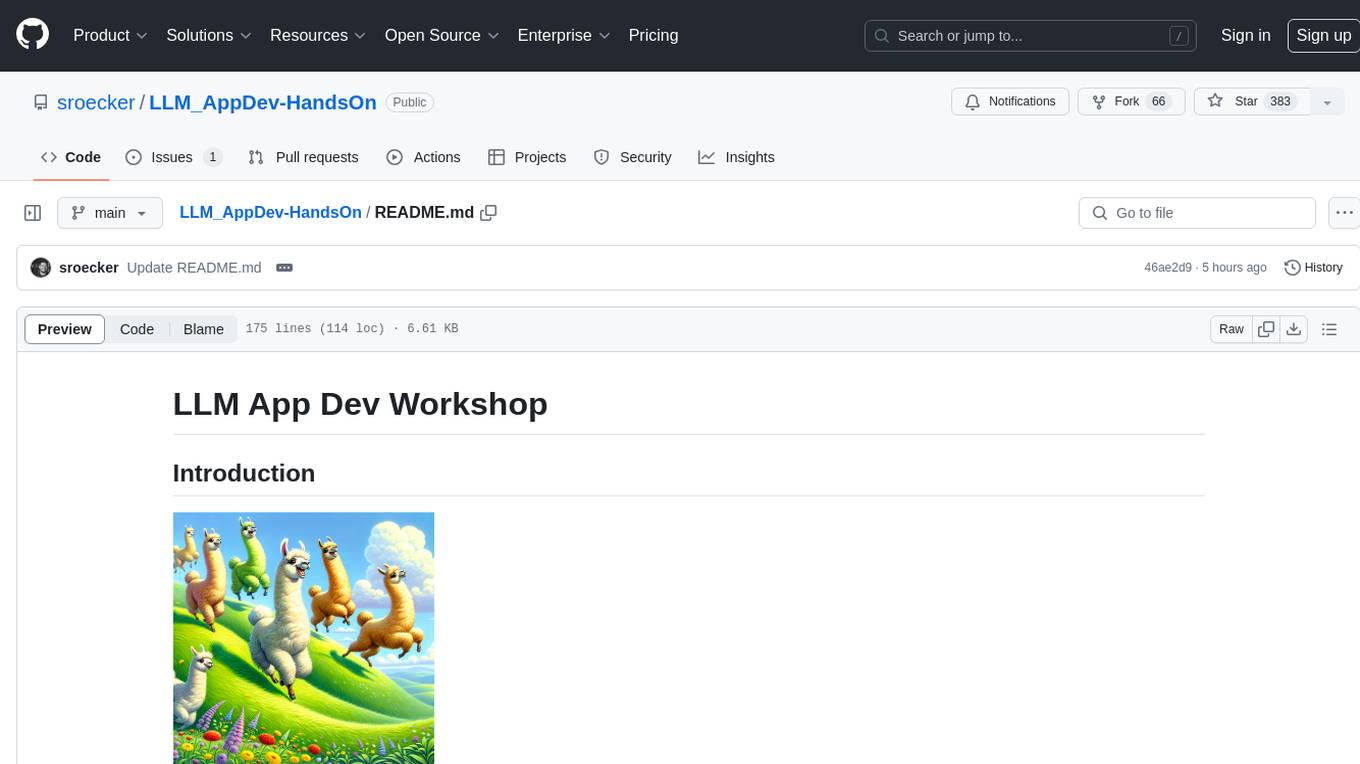
LLM_AppDev-HandsOn
This repository showcases how to build a simple LLM-based chatbot for answering questions based on documents using retrieval augmented generation (RAG) technique. It also provides guidance on deploying the chatbot using Podman or on the OpenShift Container Platform. The workshop associated with this repository introduces participants to LLMs & RAG concepts and demonstrates how to customize the chatbot for specific purposes. The software stack relies on open-source tools like streamlit, LlamaIndex, and local open LLMs via Ollama, making it accessible for GPU-constrained environments.
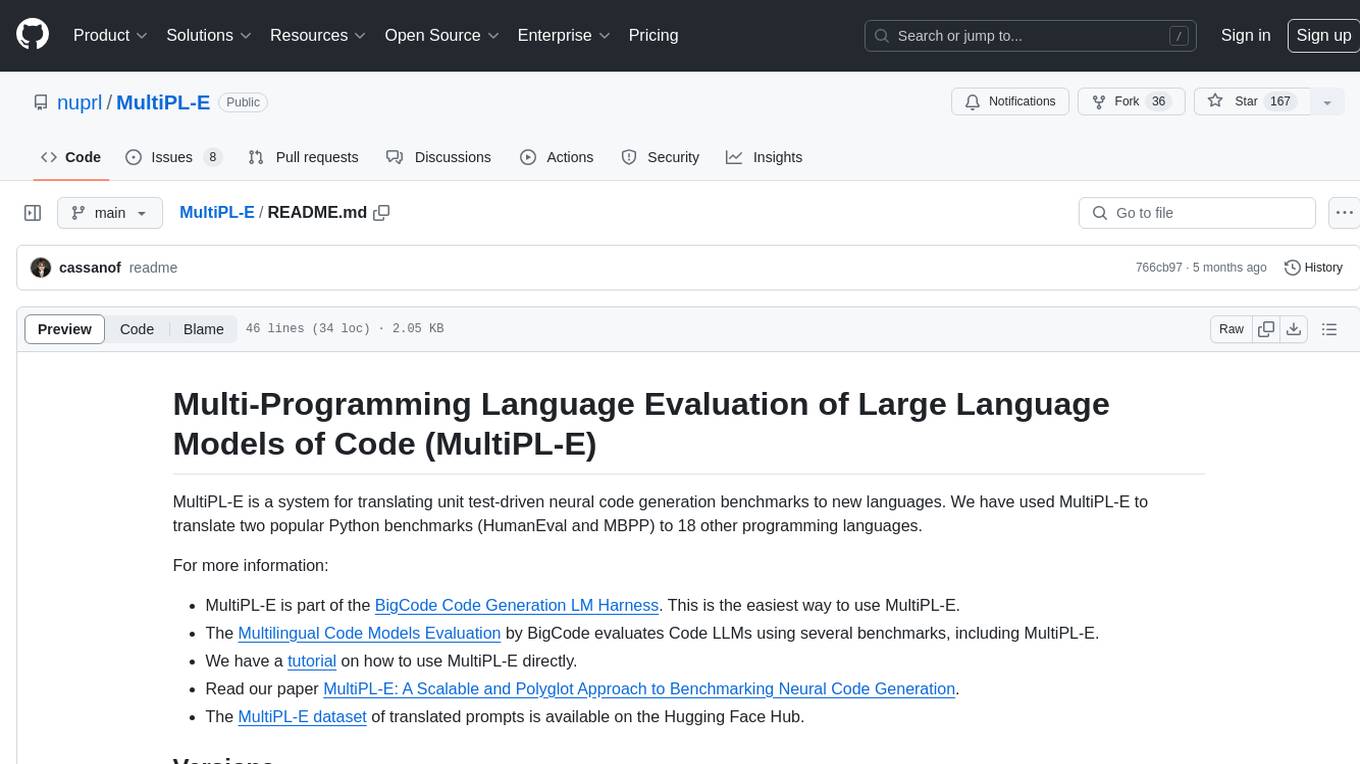
MultiPL-E
MultiPL-E is a system for translating unit test-driven neural code generation benchmarks to new languages. It is part of the BigCode Code Generation LM Harness and allows for evaluating Code LLMs using various benchmarks. The tool supports multiple versions with improvements and new language additions, providing a scalable and polyglot approach to benchmarking neural code generation. Users can access a tutorial for direct usage and explore the dataset of translated prompts on the Hugging Face Hub.
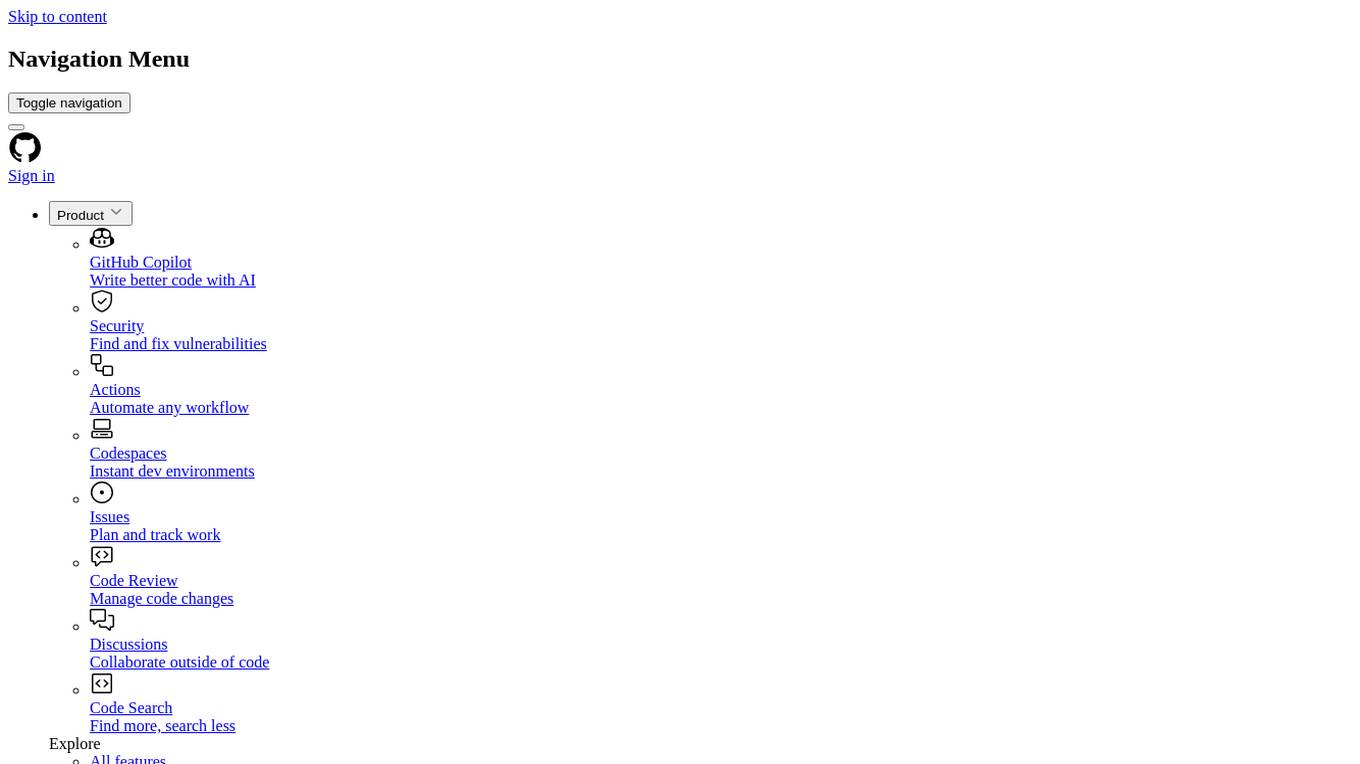
cog-comfyui
Cog-ComfyUI is a tool designed to run ComfyUI workflows on Replicate. It allows users to easily integrate their own workflows into their app or website using the Replicate API. The tool includes popular model weights and custom nodes, with the option to request more custom nodes or models. Users can get their API JSON, gather input files, and use custom LoRAs from CivitAI or HuggingFace. Additionally, users can run their workflows and set up their own dedicated instances for better performance and control. The tool provides options for private deployments, forking using Cog, or creating new models from the train tab on Replicate. It also offers guidance on developing locally and running the Web UI from a Cog container.
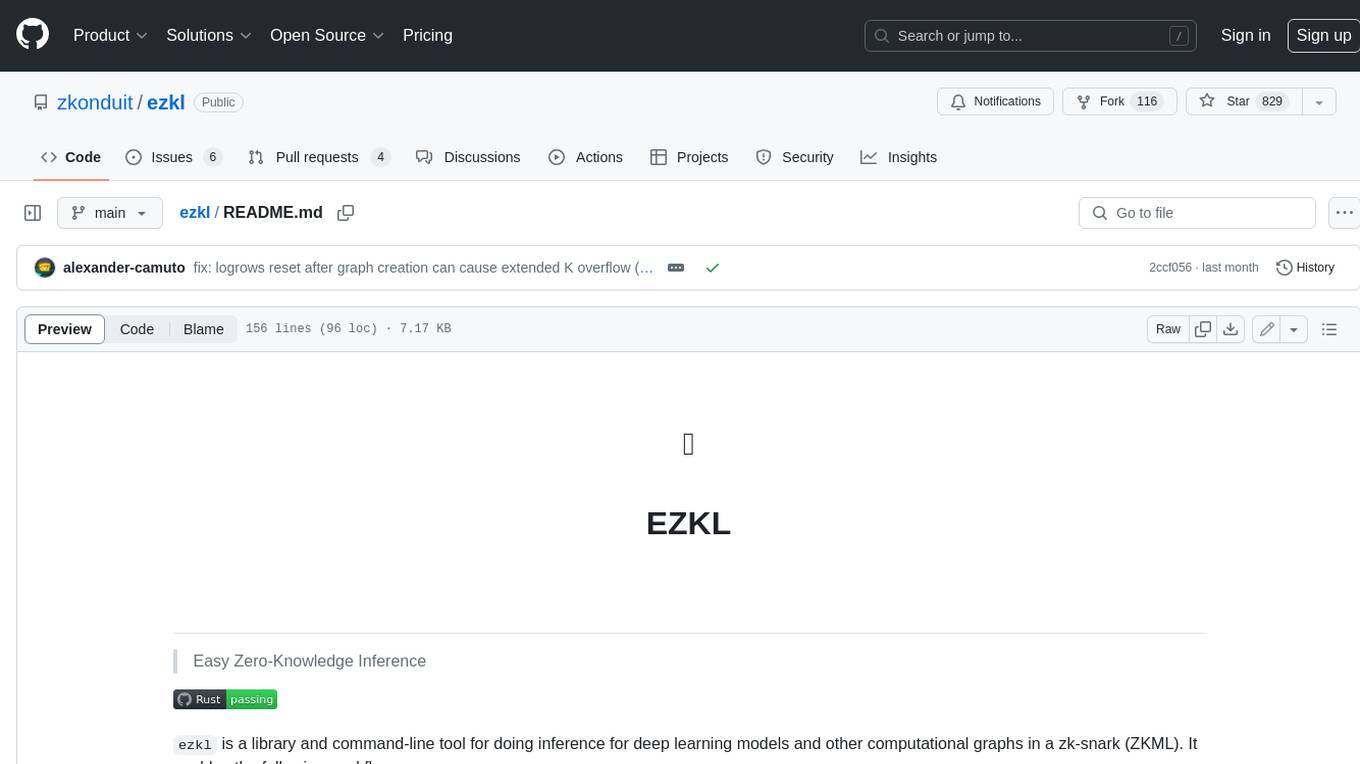
ezkl
EZKL is a library and command-line tool for doing inference for deep learning models and other computational graphs in a zk-snark (ZKML). It enables the following workflow: 1. Define a computational graph, for instance a neural network (but really any arbitrary set of operations), as you would normally in pytorch or tensorflow. 2. Export the final graph of operations as an .onnx file and some sample inputs to a .json file. 3. Point ezkl to the .onnx and .json files to generate a ZK-SNARK circuit with which you can prove statements such as: > "I ran this publicly available neural network on some private data and it produced this output" > "I ran my private neural network on some public data and it produced this output" > "I correctly ran this publicly available neural network on some public data and it produced this output" In the backend we use the collaboratively-developed Halo2 as a proof system. The generated proofs can then be verified with much less computational resources, including on-chain (with the Ethereum Virtual Machine), in a browser, or on a device.
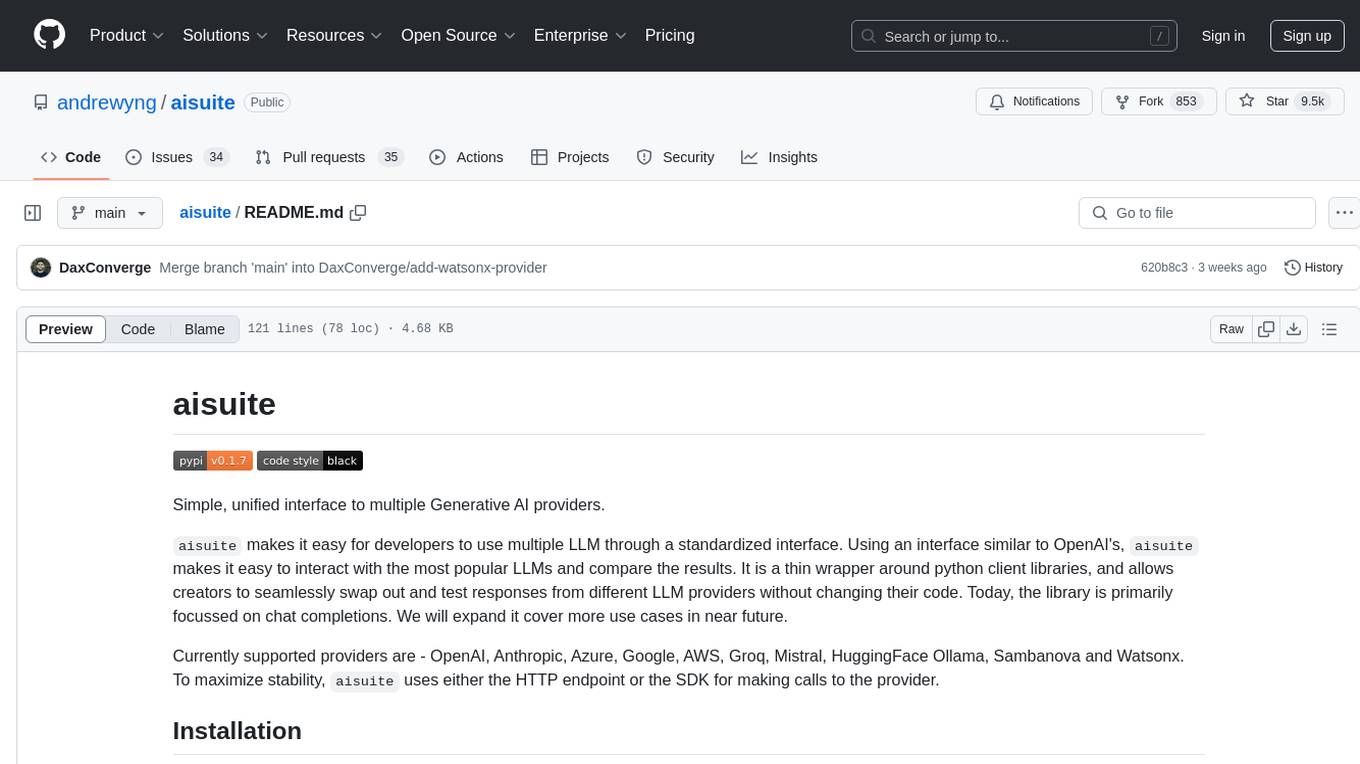
aisuite
Aisuite is a simple, unified interface to multiple Generative AI providers. It allows developers to easily interact with various Language Model (LLM) providers like OpenAI, Anthropic, Azure, Google, AWS, and more through a standardized interface. The library focuses on chat completions and provides a thin wrapper around python client libraries, enabling creators to test responses from different LLM providers without changing their code. Aisuite maximizes stability by using HTTP endpoints or SDKs for making calls to the providers. Users can install the base package or specific provider packages, set up API keys, and utilize the library to generate chat completion responses from different models.
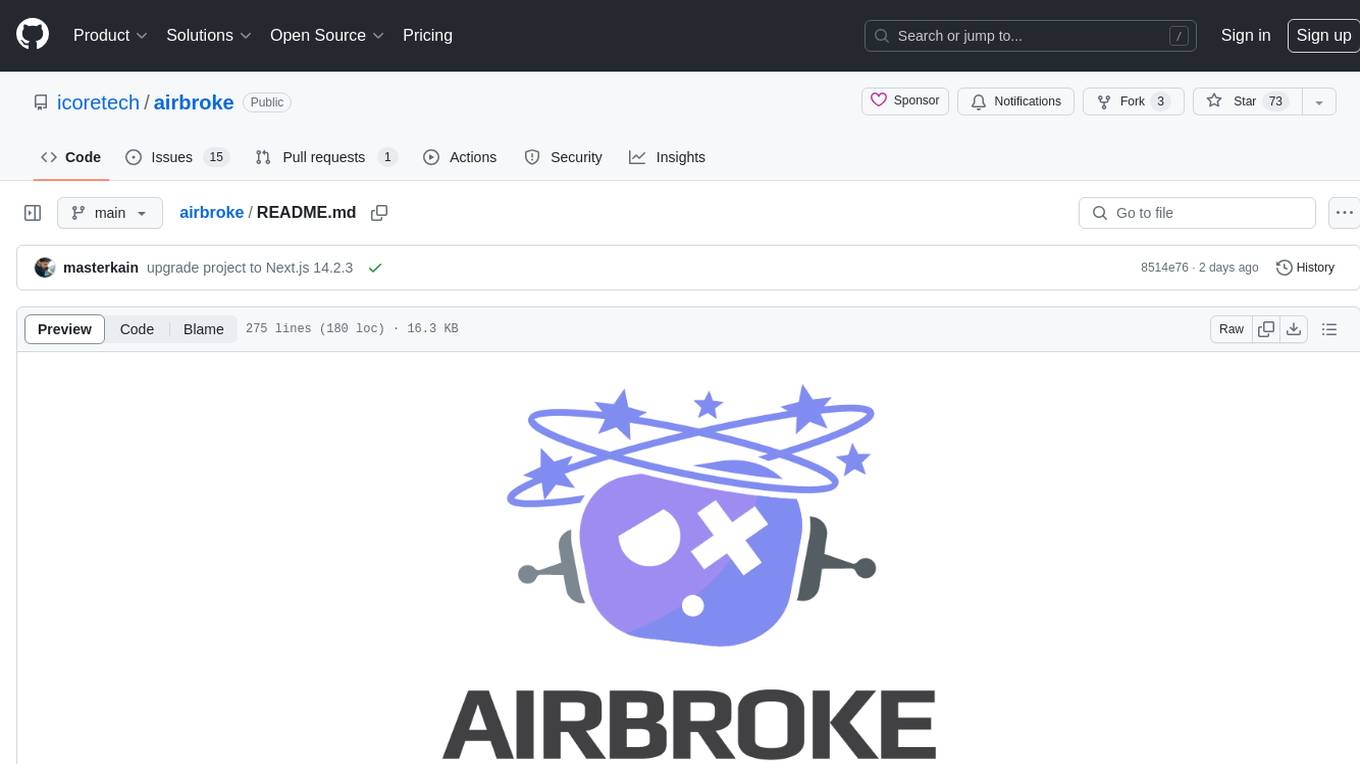
airbroke
Airbroke is an open-source error catcher tool designed for modern web applications. It provides a PostgreSQL-based backend with an Airbrake-compatible HTTP collector endpoint and a React-based frontend for error management. The tool focuses on simplicity, maintaining a small database footprint even under heavy data ingestion. Users can ask AI about issues, replay HTTP exceptions, and save/manage bookmarks for important occurrences. Airbroke supports multiple OAuth providers for secure user authentication and offers occurrence charts for better insights into error occurrences. The tool can be deployed in various ways, including building from source, using Docker images, deploying on Vercel, Render.com, Kubernetes with Helm, or Docker Compose. It requires Node.js, PostgreSQL, and specific system resources for deployment.
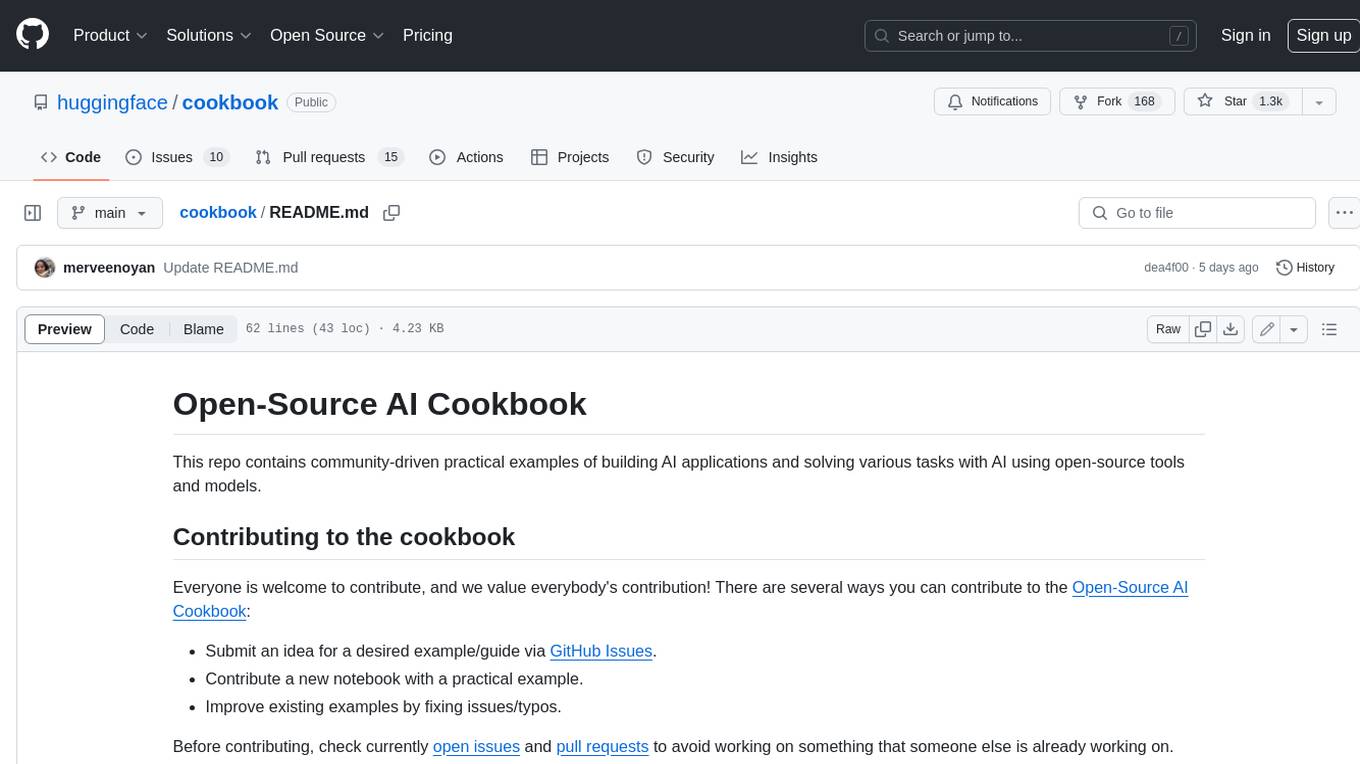
cookbook
This repository contains community-driven practical examples of building AI applications and solving various tasks with AI using open-source tools and models. Everyone is welcome to contribute, and we value everybody's contribution! There are several ways you can contribute to the Open-Source AI Cookbook: Submit an idea for a desired example/guide via GitHub Issues. Contribute a new notebook with a practical example. Improve existing examples by fixing issues/typos. Before contributing, check currently open issues and pull requests to avoid working on something that someone else is already working on.
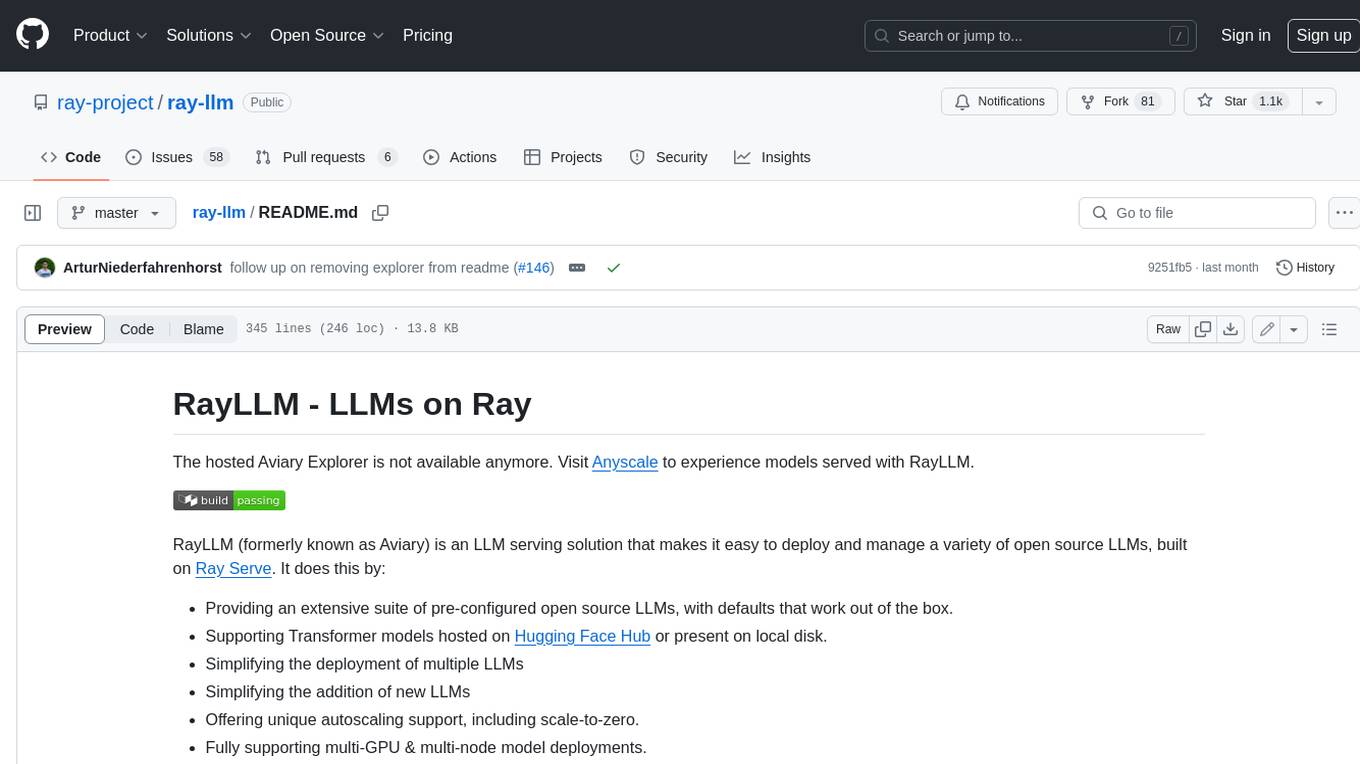
ray-llm
RayLLM (formerly known as Aviary) is an LLM serving solution that makes it easy to deploy and manage a variety of open source LLMs, built on Ray Serve. It provides an extensive suite of pre-configured open source LLMs, with defaults that work out of the box. RayLLM supports Transformer models hosted on Hugging Face Hub or present on local disk. It simplifies the deployment of multiple LLMs, the addition of new LLMs, and offers unique autoscaling support, including scale-to-zero. RayLLM fully supports multi-GPU & multi-node model deployments and offers high performance features like continuous batching, quantization and streaming. It provides a REST API that is similar to OpenAI's to make it easy to migrate and cross test them. RayLLM supports multiple LLM backends out of the box, including vLLM and TensorRT-LLM.
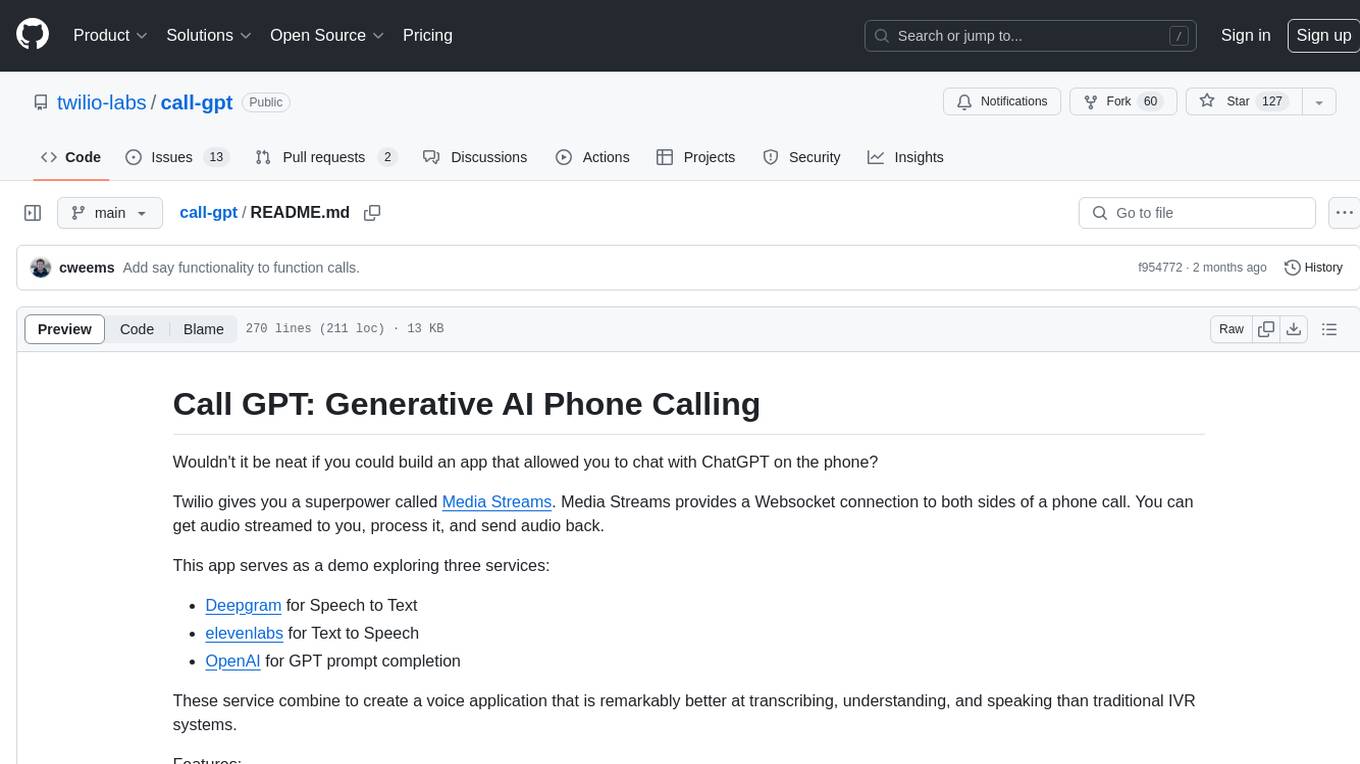
call-gpt
Call GPT is a voice application that utilizes Deepgram for Speech to Text, elevenlabs for Text to Speech, and OpenAI for GPT prompt completion. It allows users to chat with ChatGPT on the phone, providing better transcription, understanding, and speaking capabilities than traditional IVR systems. The app returns responses with low latency, allows user interruptions, maintains chat history, and enables GPT to call external tools. It coordinates data flow between Deepgram, OpenAI, ElevenLabs, and Twilio Media Streams, enhancing voice interactions.
For similar tasks
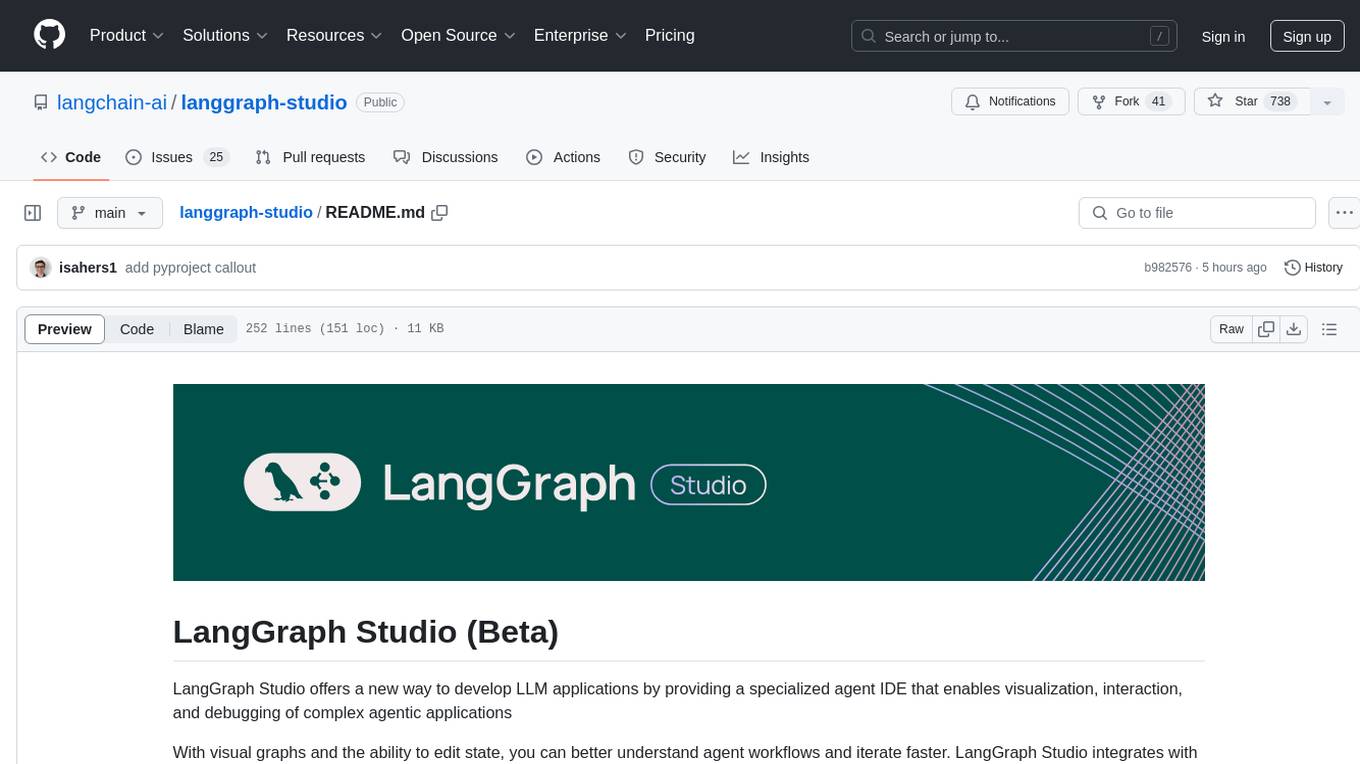
langgraph-studio
LangGraph Studio is a specialized agent IDE that enables visualization, interaction, and debugging of complex agentic applications. It offers visual graphs and state editing to better understand agent workflows and iterate faster. Users can collaborate with teammates using LangSmith to debug failure modes. The tool integrates with LangSmith and requires Docker installed. Users can create and edit threads, configure graph runs, add interrupts, and support human-in-the-loop workflows. LangGraph Studio allows interactive modification of project config and graph code, with live sync to the interactive graph for easier iteration on long-running agents.
For similar jobs
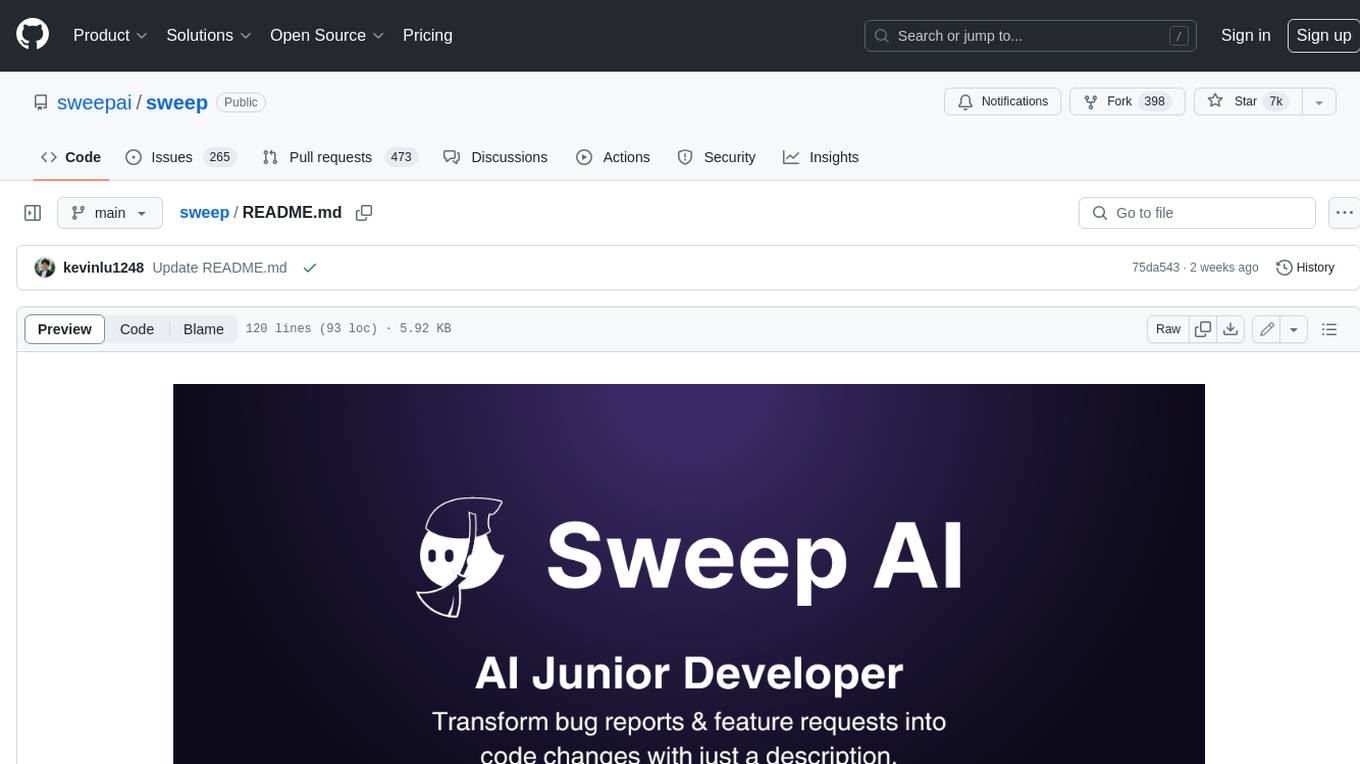
sweep
Sweep is an AI junior developer that turns bugs and feature requests into code changes. It automatically handles developer experience improvements like adding type hints and improving test coverage.
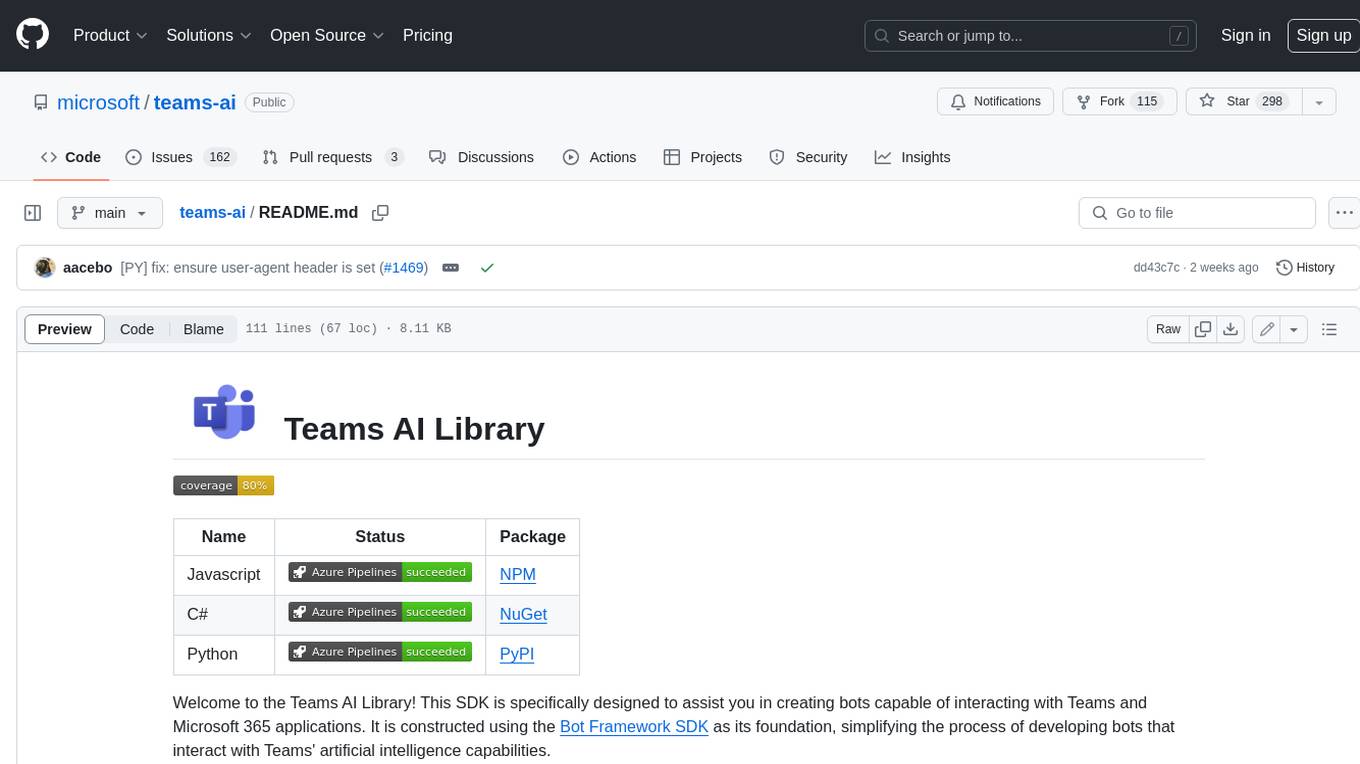
teams-ai
The Teams AI Library is a software development kit (SDK) that helps developers create bots that can interact with Teams and Microsoft 365 applications. It is built on top of the Bot Framework SDK and simplifies the process of developing bots that interact with Teams' artificial intelligence capabilities. The SDK is available for JavaScript/TypeScript, .NET, and Python.
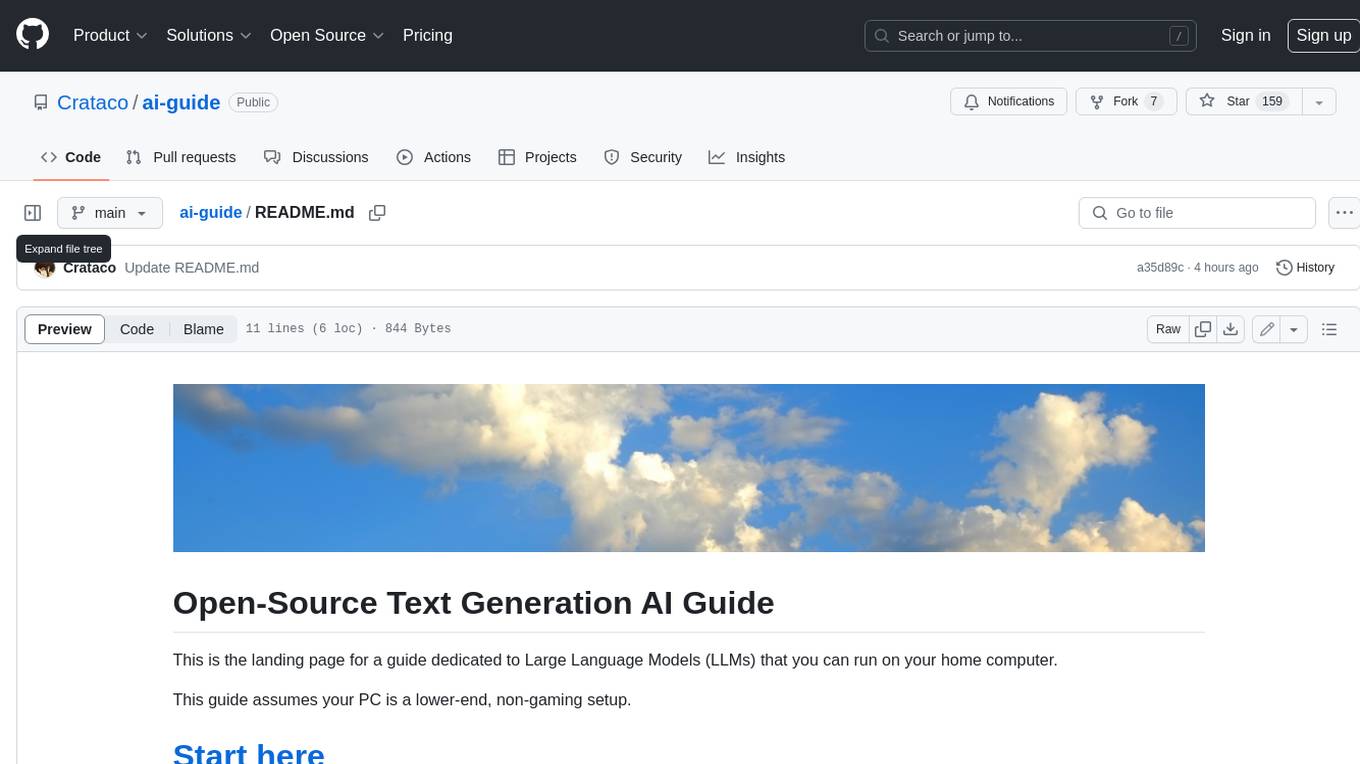
ai-guide
This guide is dedicated to Large Language Models (LLMs) that you can run on your home computer. It assumes your PC is a lower-end, non-gaming setup.
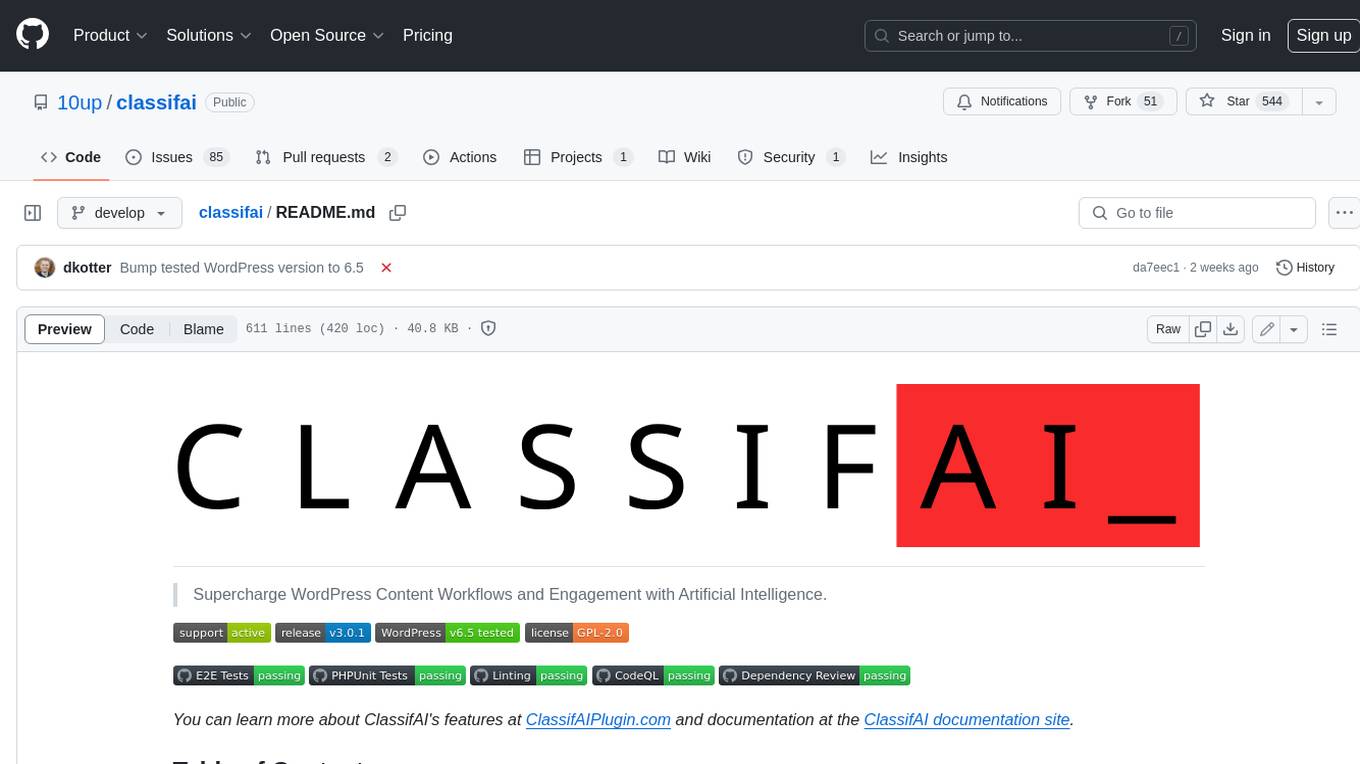
classifai
Supercharge WordPress Content Workflows and Engagement with Artificial Intelligence. Tap into leading cloud-based services like OpenAI, Microsoft Azure AI, Google Gemini and IBM Watson to augment your WordPress-powered websites. Publish content faster while improving SEO performance and increasing audience engagement. ClassifAI integrates Artificial Intelligence and Machine Learning technologies to lighten your workload and eliminate tedious tasks, giving you more time to create original content that matters.
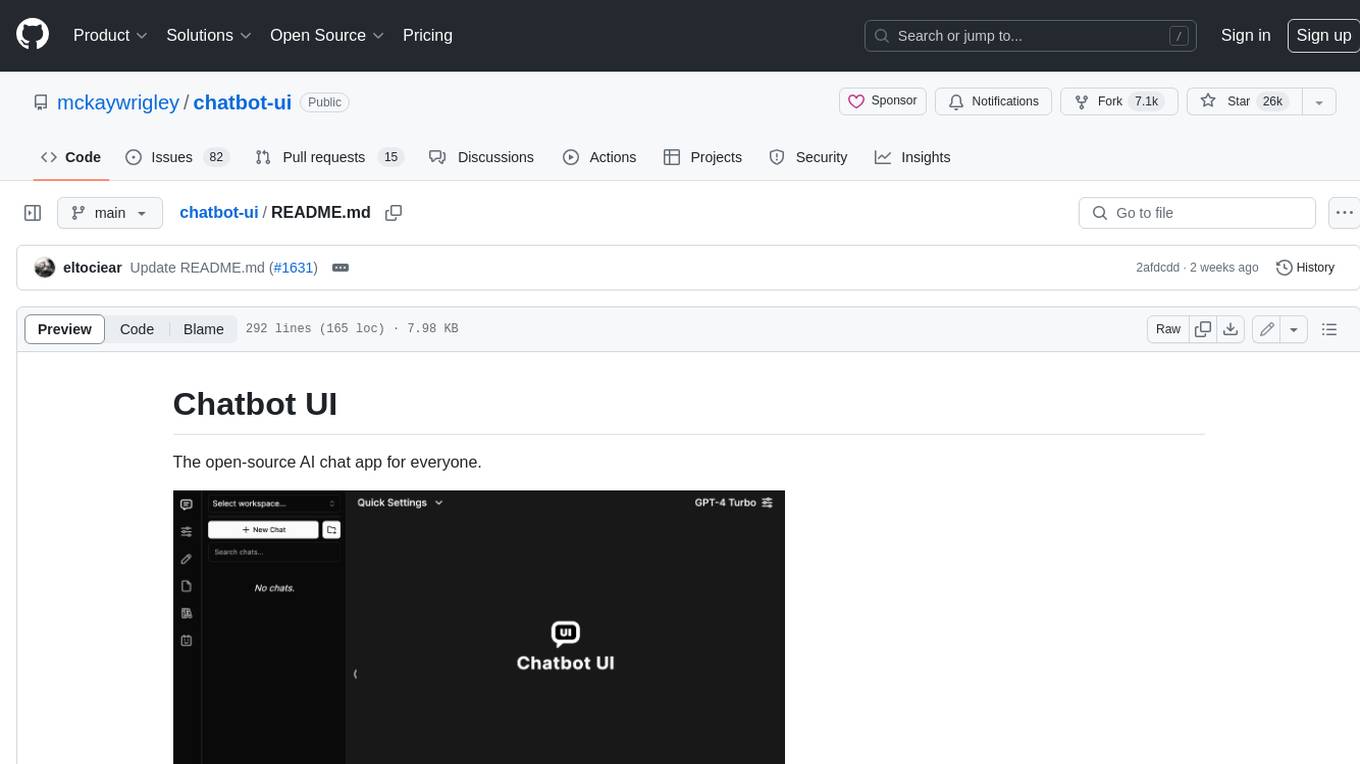
chatbot-ui
Chatbot UI is an open-source AI chat app that allows users to create and deploy their own AI chatbots. It is easy to use and can be customized to fit any need. Chatbot UI is perfect for businesses, developers, and anyone who wants to create a chatbot.
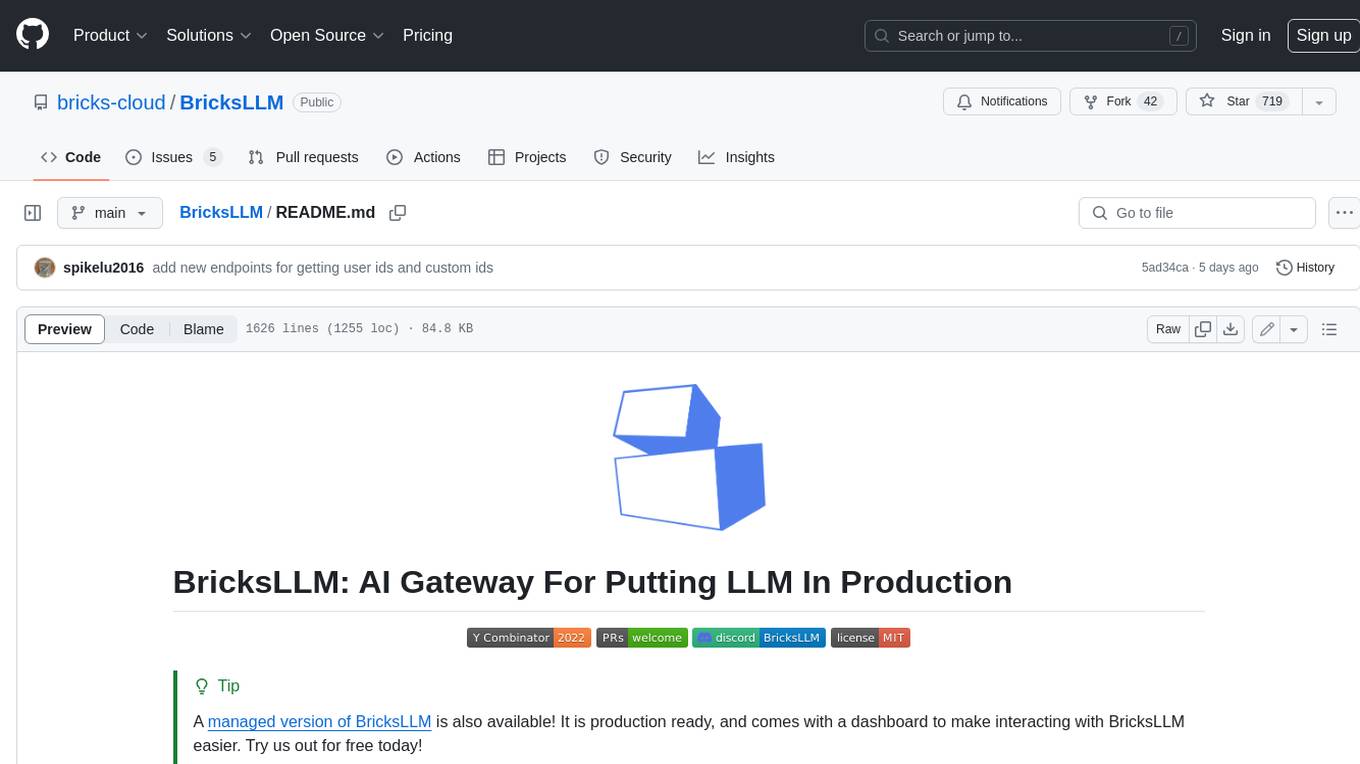
BricksLLM
BricksLLM is a cloud native AI gateway written in Go. Currently, it provides native support for OpenAI, Anthropic, Azure OpenAI and vLLM. BricksLLM aims to provide enterprise level infrastructure that can power any LLM production use cases. Here are some use cases for BricksLLM: * Set LLM usage limits for users on different pricing tiers * Track LLM usage on a per user and per organization basis * Block or redact requests containing PIIs * Improve LLM reliability with failovers, retries and caching * Distribute API keys with rate limits and cost limits for internal development/production use cases * Distribute API keys with rate limits and cost limits for students
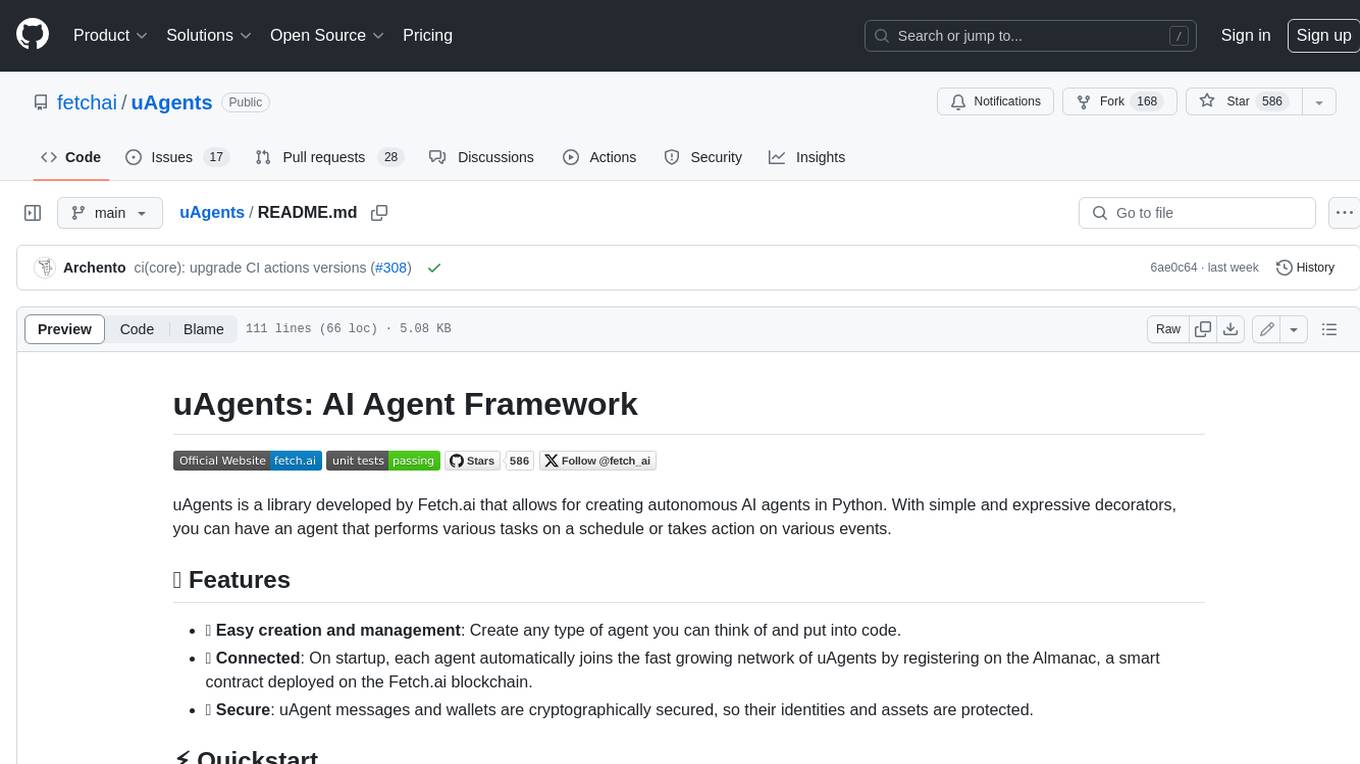
uAgents
uAgents is a Python library developed by Fetch.ai that allows for the creation of autonomous AI agents. These agents can perform various tasks on a schedule or take action on various events. uAgents are easy to create and manage, and they are connected to a fast-growing network of other uAgents. They are also secure, with cryptographically secured messages and wallets.
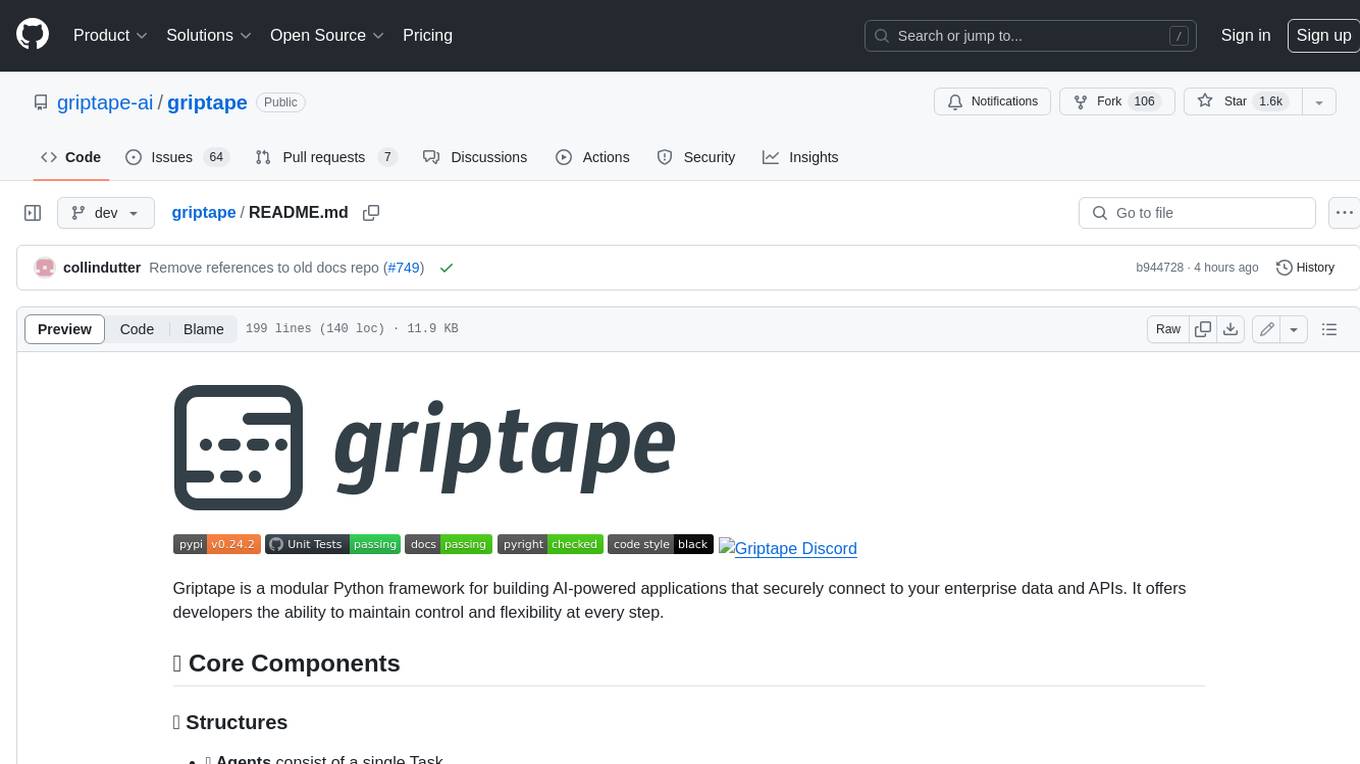
griptape
Griptape is a modular Python framework for building AI-powered applications that securely connect to your enterprise data and APIs. It offers developers the ability to maintain control and flexibility at every step. Griptape's core components include Structures (Agents, Pipelines, and Workflows), Tasks, Tools, Memory (Conversation Memory, Task Memory, and Meta Memory), Drivers (Prompt and Embedding Drivers, Vector Store Drivers, Image Generation Drivers, Image Query Drivers, SQL Drivers, Web Scraper Drivers, and Conversation Memory Drivers), Engines (Query Engines, Extraction Engines, Summary Engines, Image Generation Engines, and Image Query Engines), and additional components (Rulesets, Loaders, Artifacts, Chunkers, and Tokenizers). Griptape enables developers to create AI-powered applications with ease and efficiency.