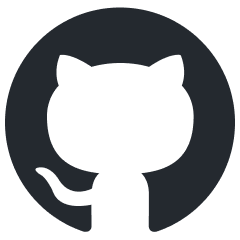
inspectus
LLM Analytics
Stars: 600
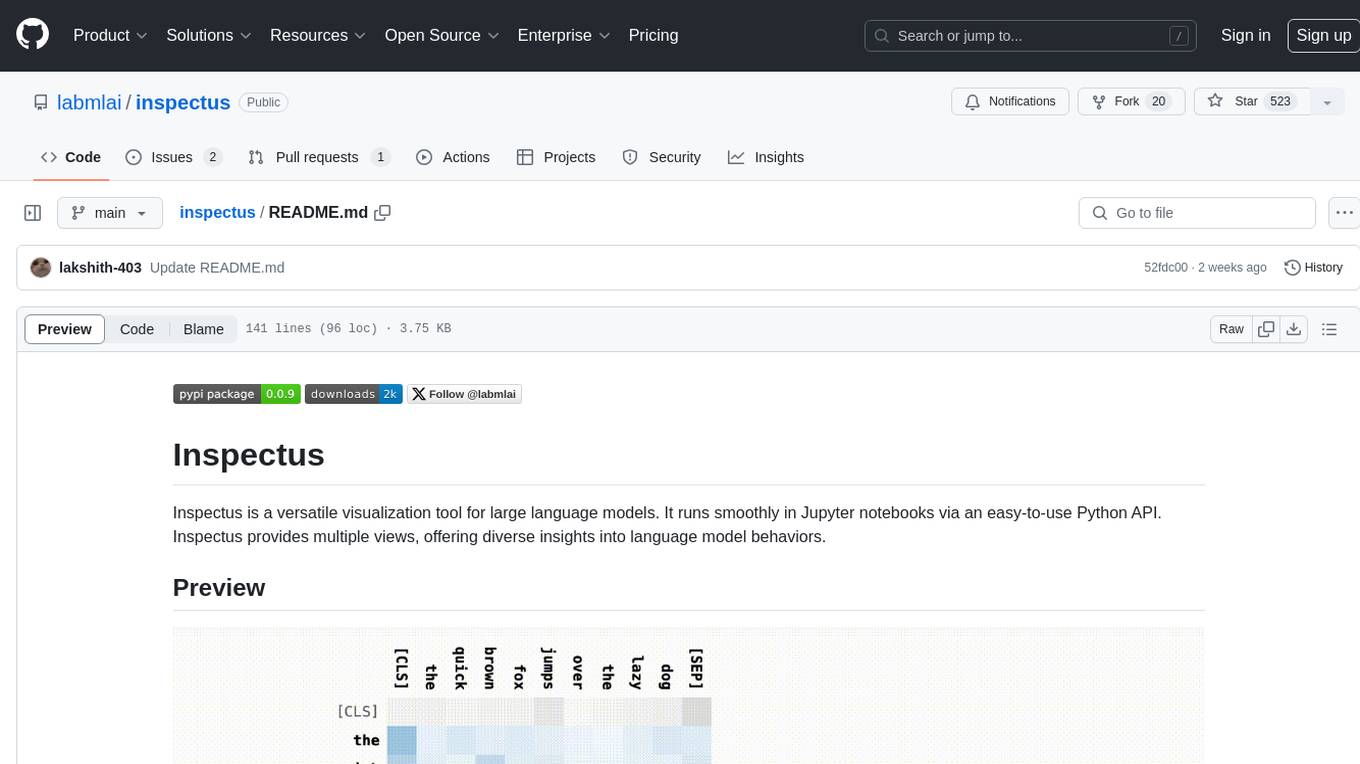
Inspectus is a versatile visualization tool for large language models. It provides multiple views, including Attention Matrix, Query Token Heatmap, Key Token Heatmap, and Dimension Heatmap, to offer insights into language model behaviors. Users can interact with the tool in Jupyter notebooks through an easy-to-use Python API. Inspectus allows users to visualize attention scores between tokens, analyze how tokens focus on each other during processing, and explore the relationships between query and key tokens. The tool supports the visualization of attention maps from Huggingface transformers and custom attention maps, making it a valuable resource for researchers and developers working with language models.
README:
Inspectus is a versatile visualization tool for machine learning. It runs smoothly in Jupyter notebooks via an easy-to-use Python API.
- Installation
- Attention Visualization
- Token Visualization
- Distribution Plot
- Setting up for Development
- Citing
pip install inspectus
Inspectus provides visualization tools for attention mechanisms in deep learning models. It provides a set of comprehensive views, making it easier to understand how these models work.
Click a token to select it and deselect others. Clicking again will select all again. To change the state of only one token, do shift+click
Attention Matrix: Visualizes the attention scores between tokens, highlighting how each token focuses on others during processing.
Query Token Heatmap: Shows the sum of attention scores between each query and selected key tokens
Key Token Heatmap: Shows the sum of attention scores between each key and selected query tokens
Dimension Heatmap: Shows the sum of attention scores for each item in dimensions (Layers and Heads) normalized over the dimension.
Import the library
import inspectus
Simple usage
# attn: Attention map; a 2-4D tensor or attention maps from Huggingface transformers
inspectus.attention(attn, tokens)
For different query and key tokens
inspectus.attention(attns, query_tokens, key_tokens)
For detailed API documentation, please refer to the official documentation.
from transformers import AutoTokenizer, GPT2LMHeadModel, AutoConfig
import torch
import inspectus
# Initialize the tokenizer and model
context_length = 128
tokenizer = AutoTokenizer.from_pretrained("huggingface-course/code-search-net-tokenizer")
config = AutoConfig.from_pretrained(
"gpt2",
vocab_size=len(tokenizer),
n_ctx=context_length,
bos_token_id=tokenizer.bos_token_id,
eos_token_id=tokenizer.eos_token_id,
)
model = GPT2LMHeadModel(config)
# Tokenize the input text
text= 'The quick brown fox jumps over the lazy dog'
tokenized = tokenizer(
text,
return_tensors='pt',
return_offsets_mapping=True
)
input_ids = tokenized['input_ids']
tokens = [text[s: e] for s, e in tokenized['offset_mapping'][0]]
with torch.no_grad():
res = model(input_ids=input_ids.to(model.device), output_attentions=True)
# Visualize the attention maps using the Inspectus library
inspectus.attention(res['attentions'], tokens)
Check out the notebook here: Huggingface Tutorial
import numpy as np
import inspectus
# 2D attention representing attention values between Query and Key tokens
attn = np.random.rand(3, 3)
# Visualize the attention values using the Inspectus library
# The first argument is the attention matrix
# The second argument is the list of query tokens
# The third argument is the list of key tokens
inspectus.attention(arr, ['a', 'b', 'c'], ['d', 'e', 'f'])
Check out the notebook here: Custom attention map tutorial
This tool is used to visualize some metric related to tokens. This supports multiple metrics and the metric used for the visualization can be selected using the dropdown. Along with metrics, any aditional information can be added to tokens.
import inspectus
inspectus.tokens(['hello', 'world'], np.random.rand(2))
The distribution plot is a plot that shows the distribution of a series of data. At each step, the distribution of the data is calculated and maximum of 5 bands are drawn from 9 basis points. (0, 6.68, 15.87, 30.85, 50.00, 69.15, 84.13, 93.32, 100.00)
import inspectus
inspectus.distribution({'x': [x for x in range(0, 100)]})
To focus on parts of the plot and zoom in, the minimap can be used. To select a single plot, use the legend on the top right.
For comprehensive usage guide please check the notebook here: Distribution Plot Tutorial
This plot can be used to identify the existence of outliers in the data. Following notebooks demonstrate how to use the distribution plot to identify outliers in the MNIST training loss.
If you use Inspectus for academic research, please cite the library using the following BibTeX entry.
@misc{inspectus,
author = {Varuna Jayasiri, Lakshith Nishshanke},
title = {inspectus: A visualization and analytics tool for large language models},
year = {2024},
url = {https://github.com/labmlai/inspectus},
}
For Tasks:
Click tags to check more tools for each tasksFor Jobs:
Alternative AI tools for inspectus
Similar Open Source Tools
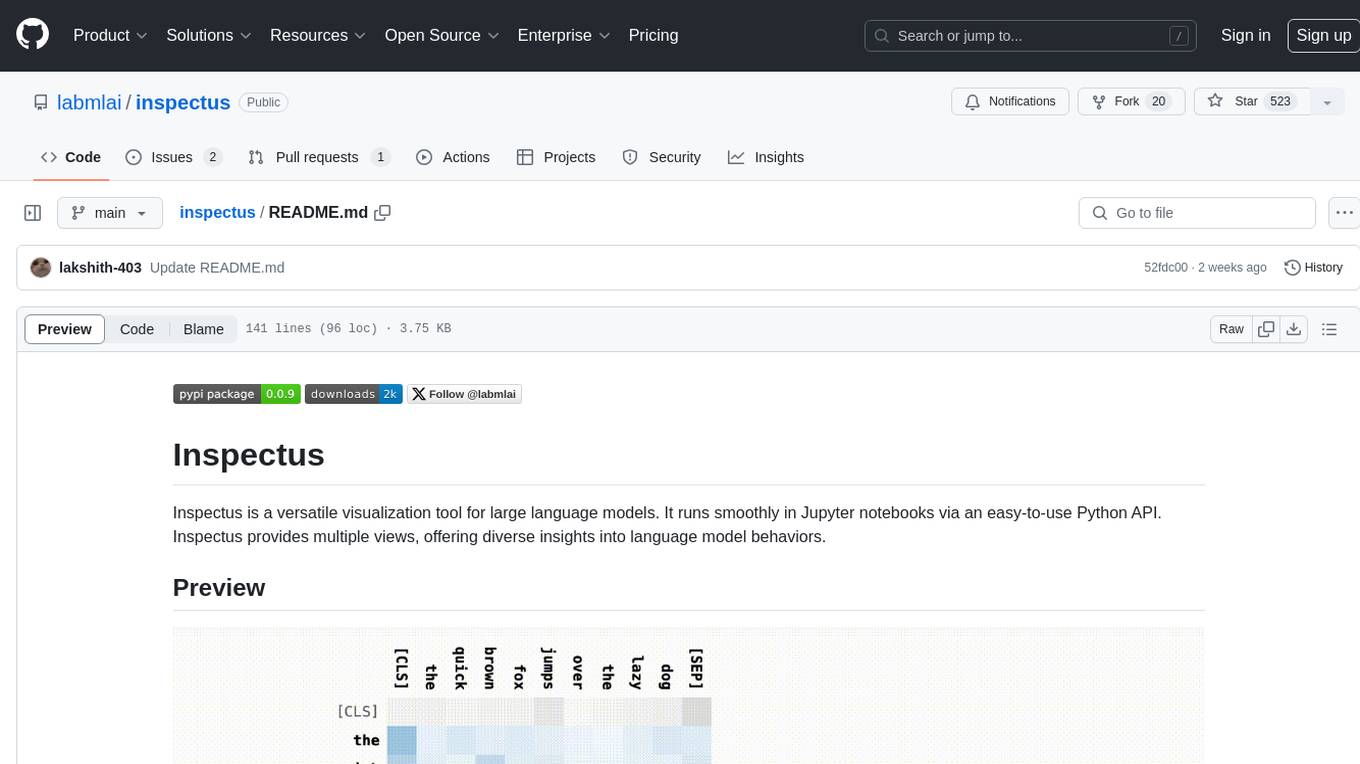
inspectus
Inspectus is a versatile visualization tool for large language models. It provides multiple views, including Attention Matrix, Query Token Heatmap, Key Token Heatmap, and Dimension Heatmap, to offer insights into language model behaviors. Users can interact with the tool in Jupyter notebooks through an easy-to-use Python API. Inspectus allows users to visualize attention scores between tokens, analyze how tokens focus on each other during processing, and explore the relationships between query and key tokens. The tool supports the visualization of attention maps from Huggingface transformers and custom attention maps, making it a valuable resource for researchers and developers working with language models.
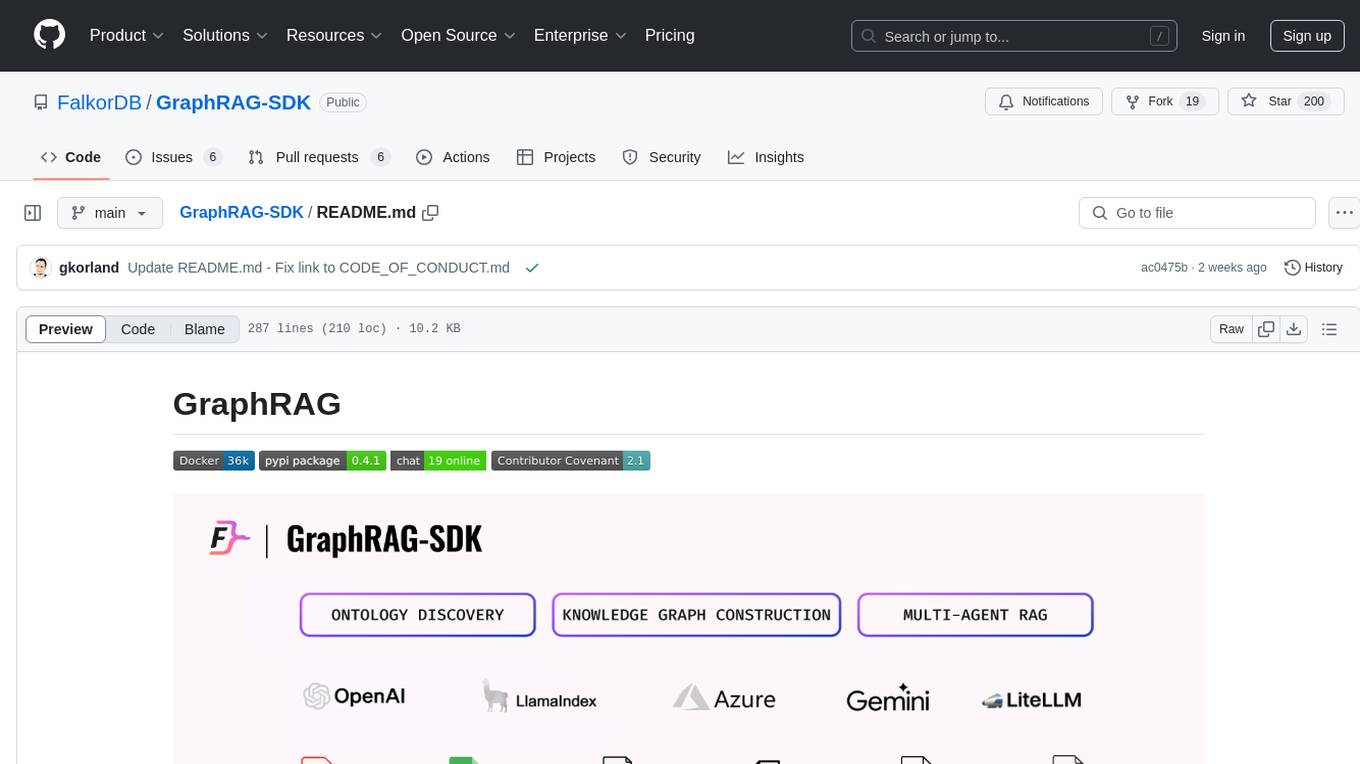
GraphRAG-SDK
Build fast and accurate GenAI applications with GraphRAG SDK, a specialized toolkit for building Graph Retrieval-Augmented Generation (GraphRAG) systems. It integrates knowledge graphs, ontology management, and state-of-the-art LLMs to deliver accurate, efficient, and customizable RAG workflows. The SDK simplifies the development process by automating ontology creation, knowledge graph agent creation, and query handling, enabling users to interact and query their knowledge graphs effectively. It supports multi-agent systems and orchestrates agents specialized in different domains. The SDK is optimized for FalkorDB, ensuring high performance and scalability for large-scale applications. By leveraging knowledge graphs, it enables semantic relationships and ontology-driven queries that go beyond standard vector similarity, enhancing retrieval-augmented generation capabilities.
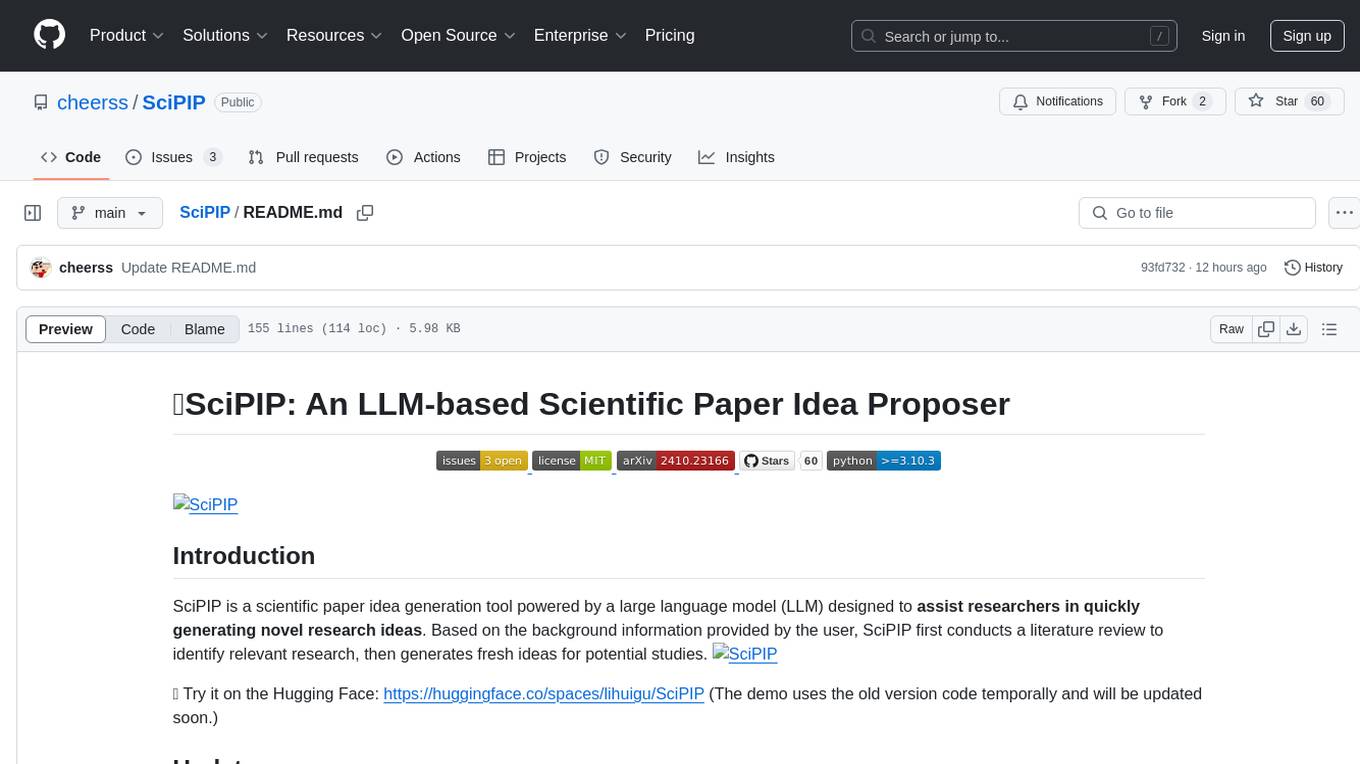
SciPIP
SciPIP is a scientific paper idea generation tool powered by a large language model (LLM) designed to assist researchers in quickly generating novel research ideas. It conducts a literature review based on user-provided background information and generates fresh ideas for potential studies. The tool is designed to help researchers in various fields by providing a GUI environment for idea generation, supporting NLP, multimodal, and CV fields, and allowing users to interact with the tool through a web app or terminal. SciPIP uses Neo4j as its database and provides functionalities for generating new ideas, fetching papers, and constructing the database.
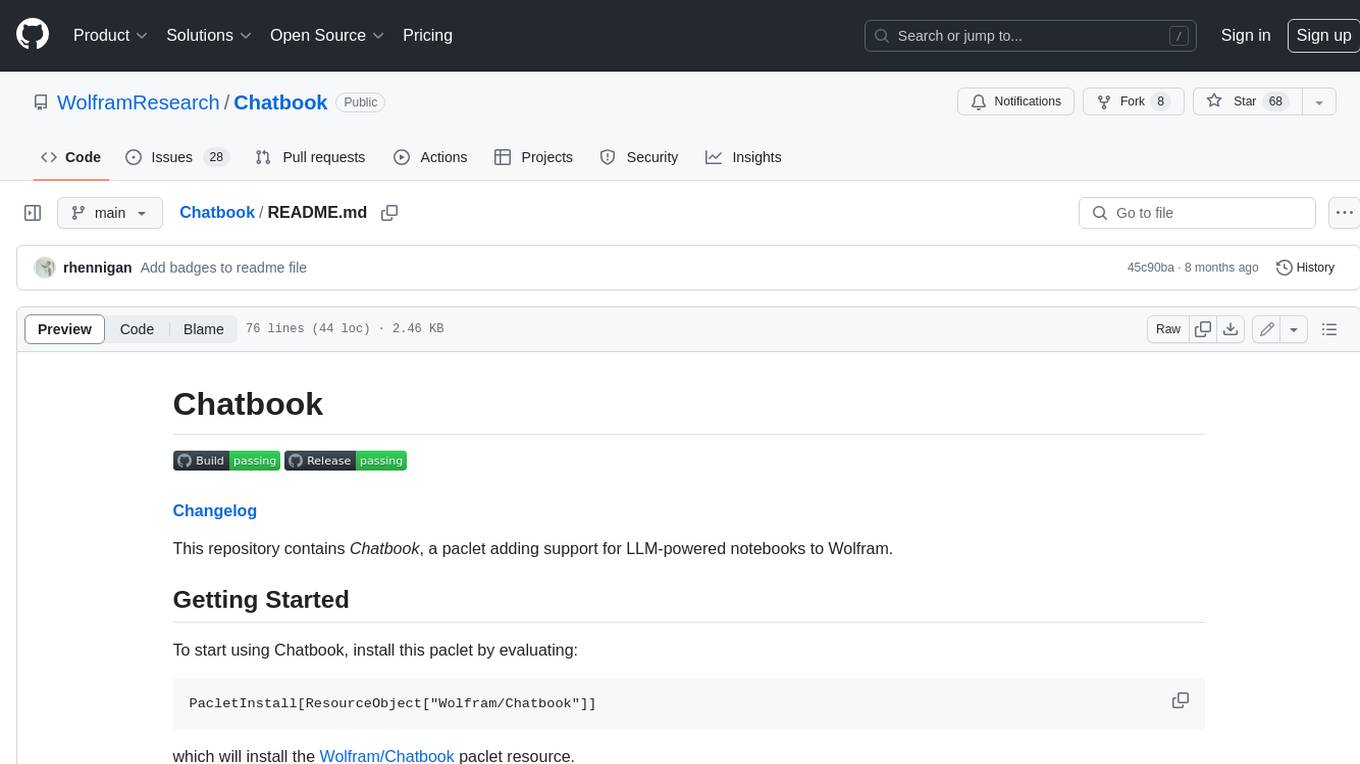
Chatbook
Chatbook is a paclet that adds support for LLM-powered notebooks to Wolfram. It allows users to interact with ChatGPT and generate immediately evaluatable Wolfram code. The code can be evaluated in place immediately, making it easy to explore and experiment with ideas.
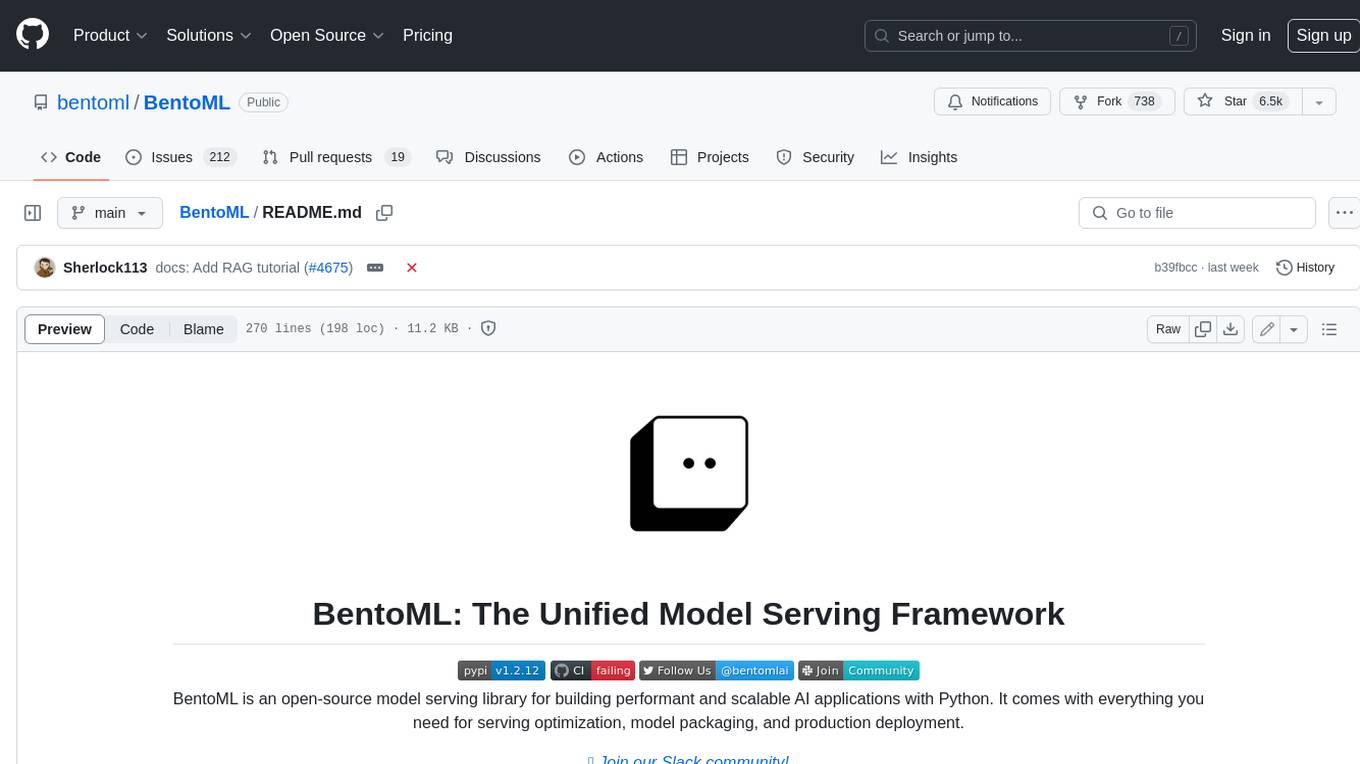
BentoML
BentoML is an open-source model serving library for building performant and scalable AI applications with Python. It comes with everything you need for serving optimization, model packaging, and production deployment.
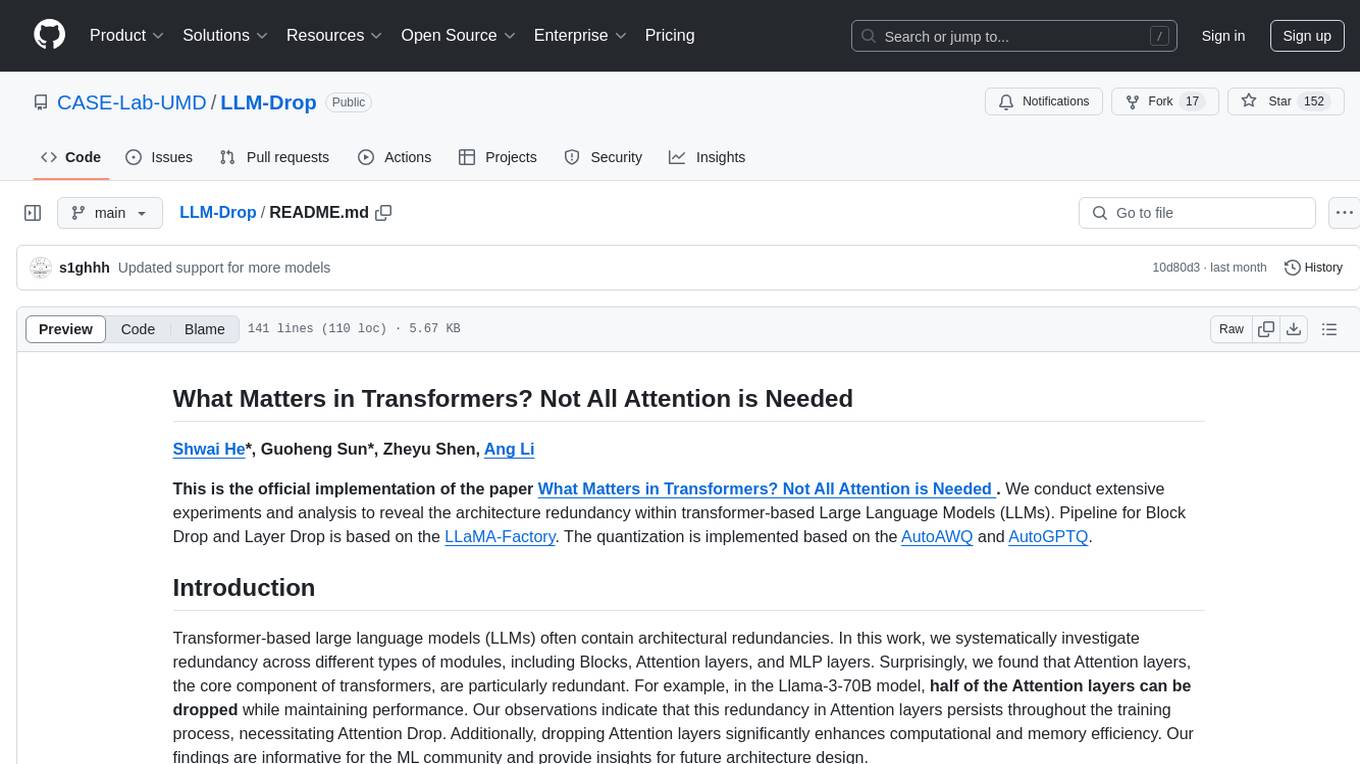
LLM-Drop
LLM-Drop is an official implementation of the paper 'What Matters in Transformers? Not All Attention is Needed'. The tool investigates redundancy in transformer-based Large Language Models (LLMs) by analyzing the architecture of Blocks, Attention layers, and MLP layers. It reveals that dropping certain Attention layers can enhance computational and memory efficiency without compromising performance. The tool provides a pipeline for Block Drop and Layer Drop based on LLaMA-Factory, and implements quantization using AutoAWQ and AutoGPTQ.
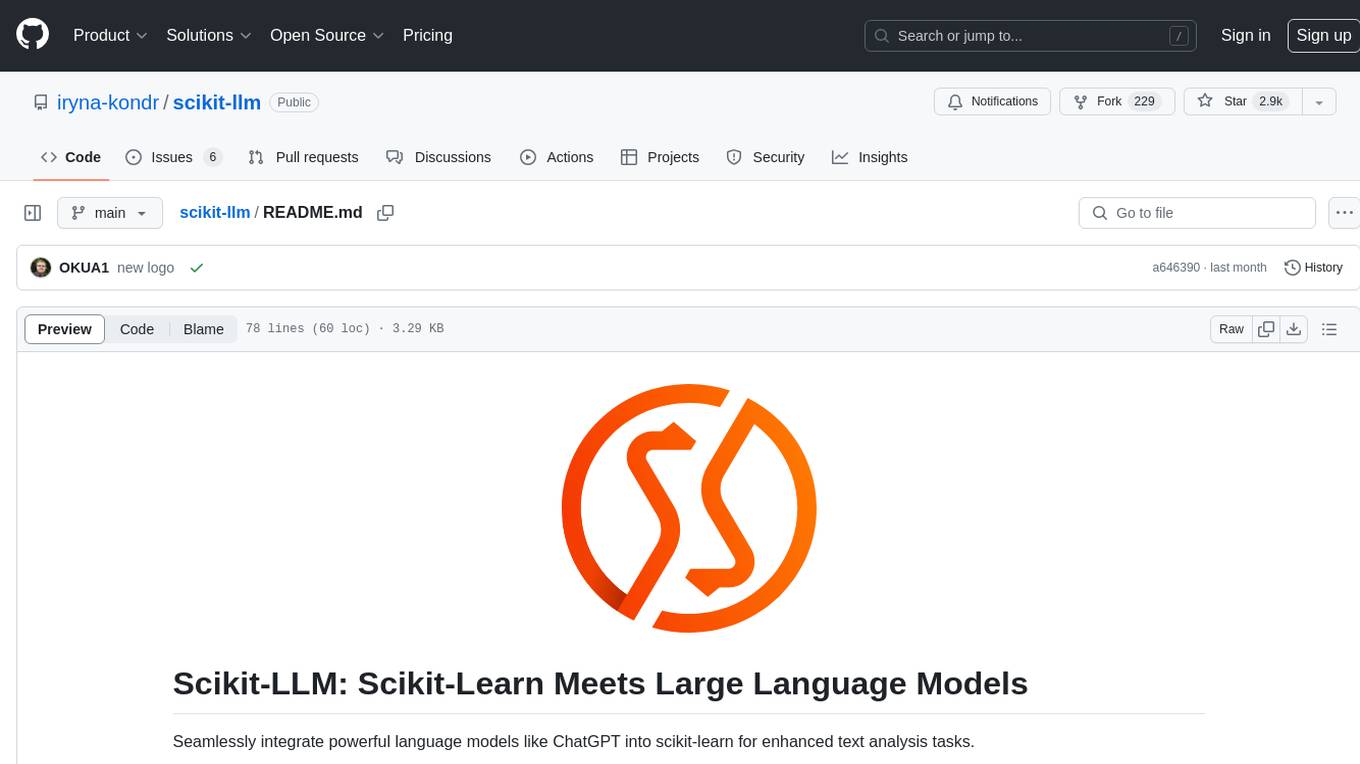
scikit-llm
Scikit-LLM is a tool that seamlessly integrates powerful language models like ChatGPT into scikit-learn for enhanced text analysis tasks. It allows users to leverage large language models for various text analysis applications within the familiar scikit-learn framework. The tool simplifies the process of incorporating advanced language processing capabilities into machine learning pipelines, enabling users to benefit from the latest advancements in natural language processing.
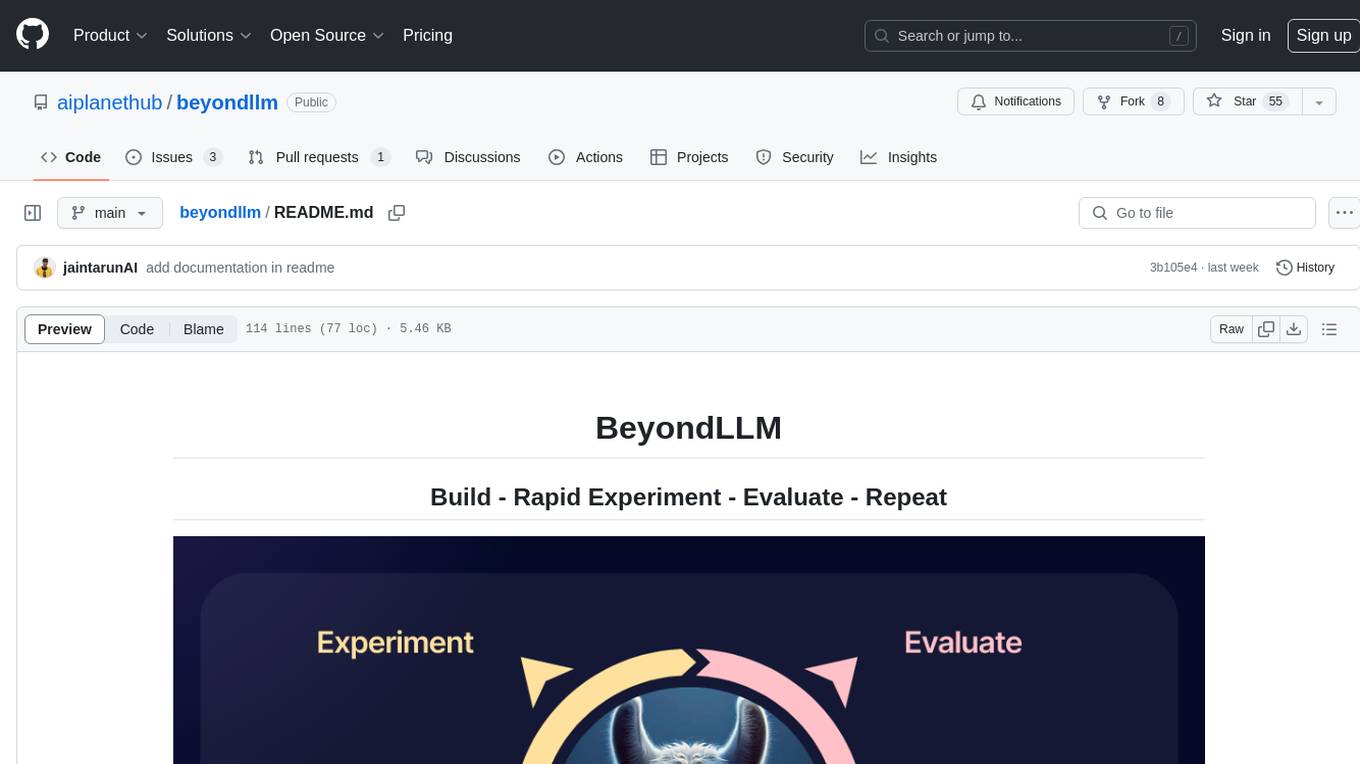
beyondllm
Beyond LLM offers an all-in-one toolkit for experimentation, evaluation, and deployment of Retrieval-Augmented Generation (RAG) systems. It simplifies the process with automated integration, customizable evaluation metrics, and support for various Large Language Models (LLMs) tailored to specific needs. The aim is to reduce LLM hallucination risks and enhance reliability.
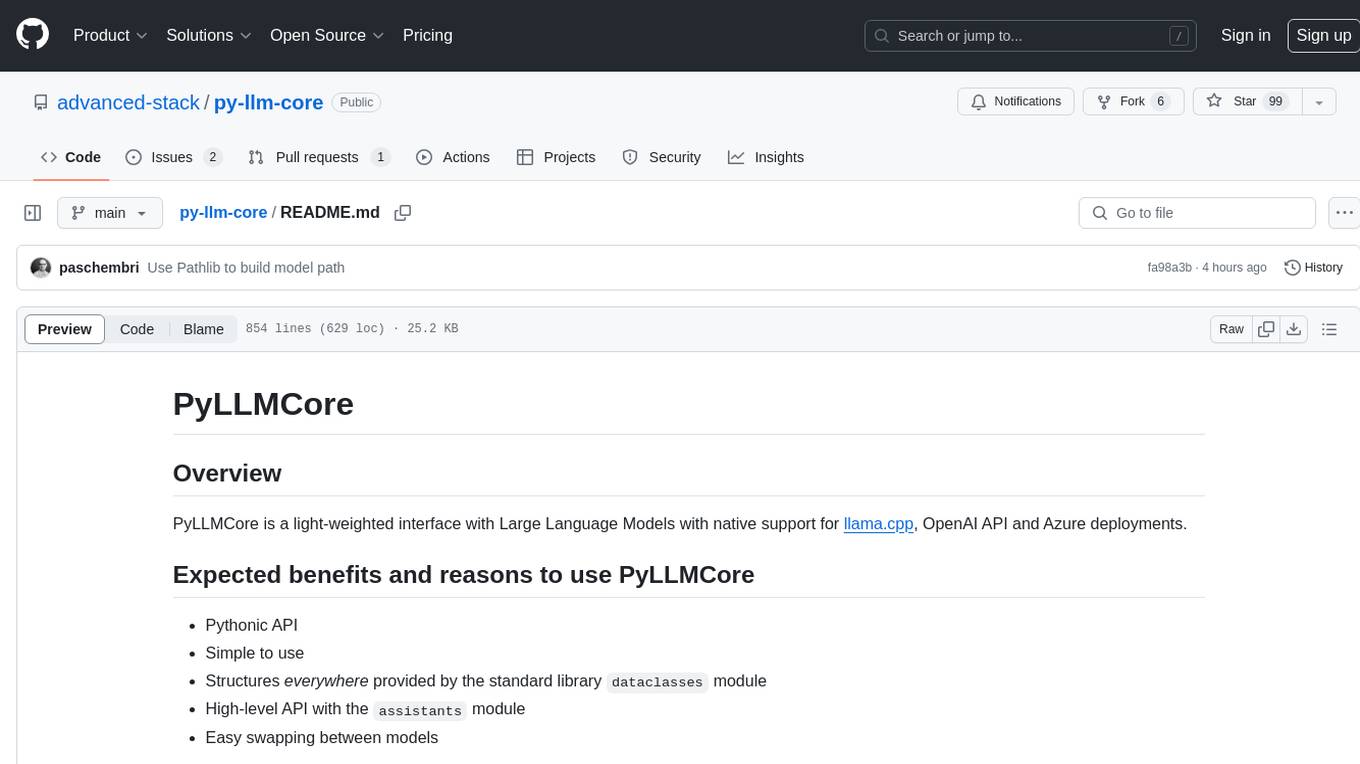
py-llm-core
PyLLMCore is a light-weighted interface with Large Language Models with native support for llama.cpp, OpenAI API, and Azure deployments. It offers a Pythonic API that is simple to use, with structures provided by the standard library dataclasses module. The high-level API includes the assistants module for easy swapping between models. PyLLMCore supports various models including those compatible with llama.cpp, OpenAI, and Azure APIs. It covers use cases such as parsing, summarizing, question answering, hallucinations reduction, context size management, and tokenizing. The tool allows users to interact with language models for tasks like parsing text, summarizing content, answering questions, reducing hallucinations, managing context size, and tokenizing text.
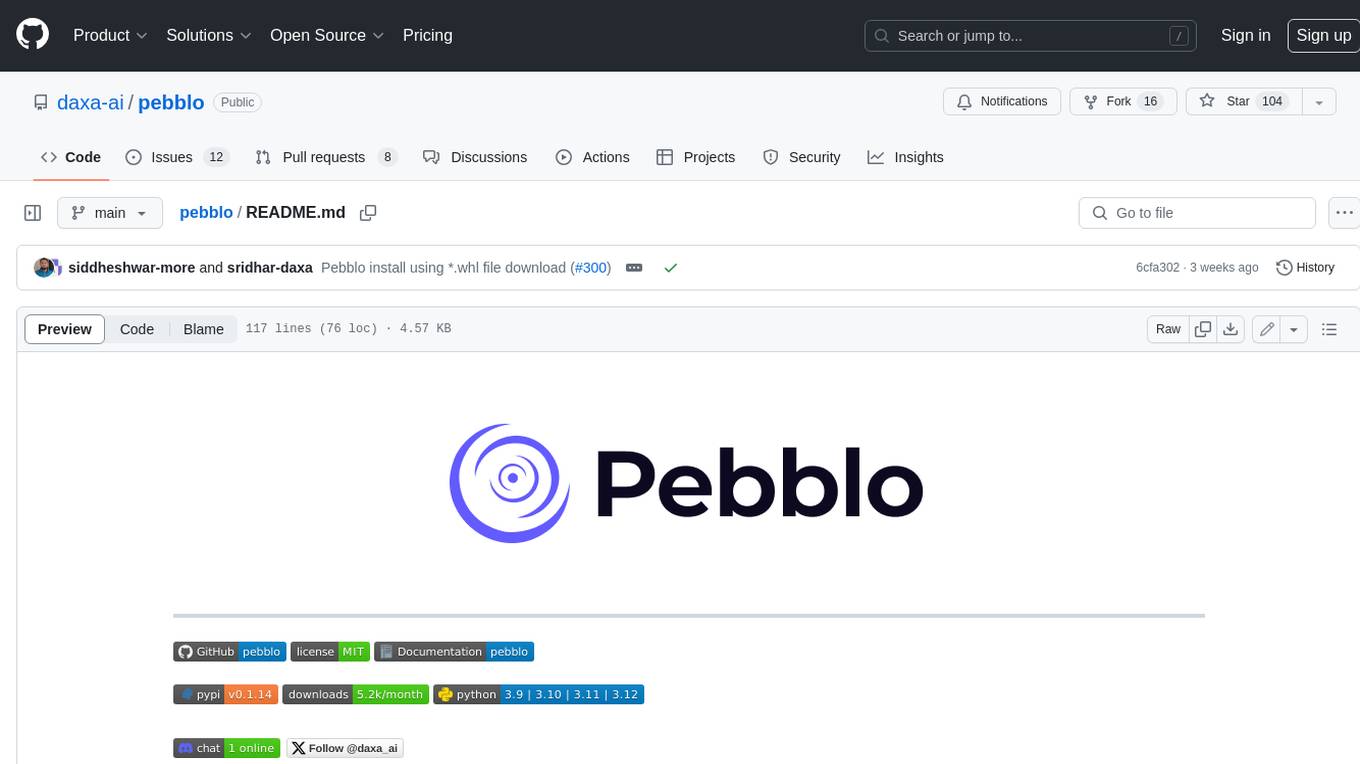
pebblo
Pebblo enables developers to safely load data and promote their Gen AI app to deployment without worrying about the organization’s compliance and security requirements. The project identifies semantic topics and entities found in the loaded data and summarizes them on the UI or a PDF report.
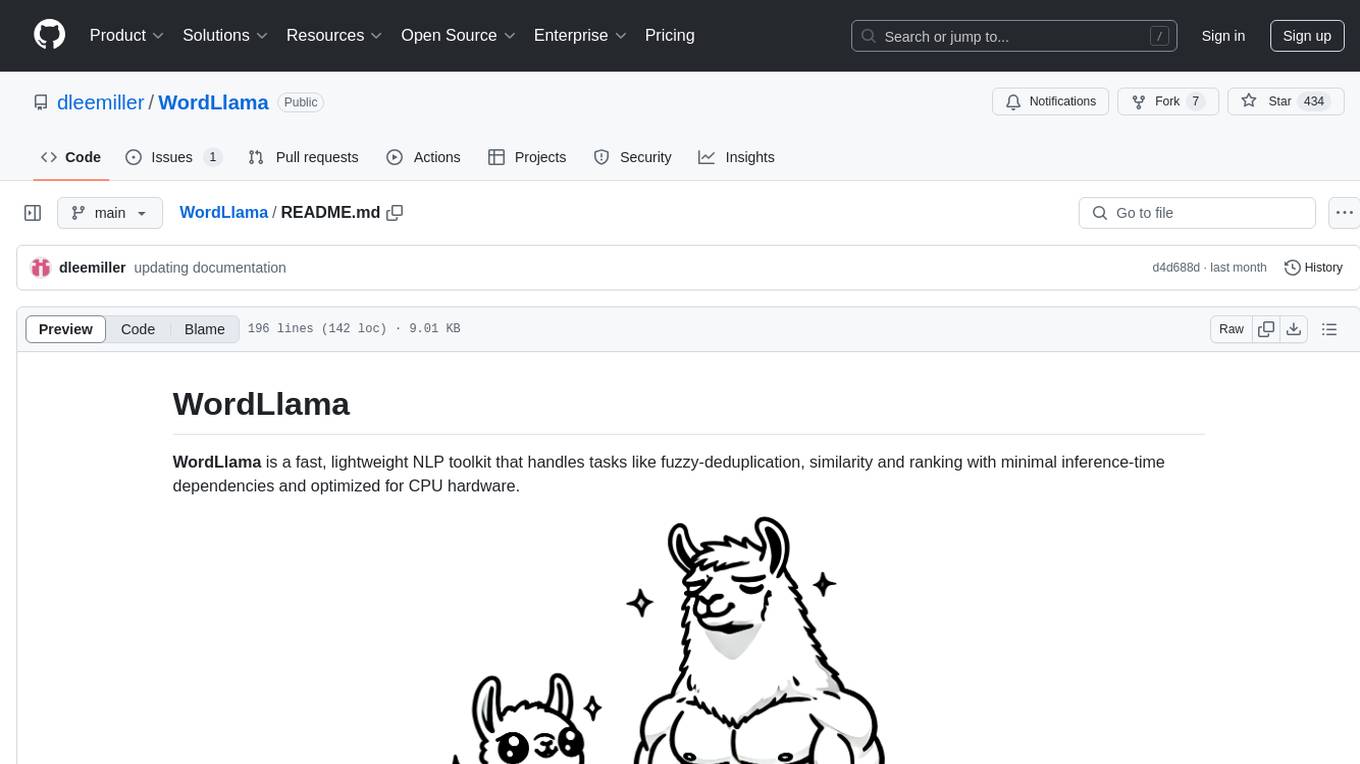
WordLlama
WordLlama is a fast, lightweight NLP toolkit optimized for CPU hardware. It recycles components from large language models to create efficient word representations. It offers features like Matryoshka Representations, low resource requirements, binarization, and numpy-only inference. The tool is suitable for tasks like semantic matching, fuzzy deduplication, ranking, and clustering, making it a good option for NLP-lite tasks and exploratory analysis.
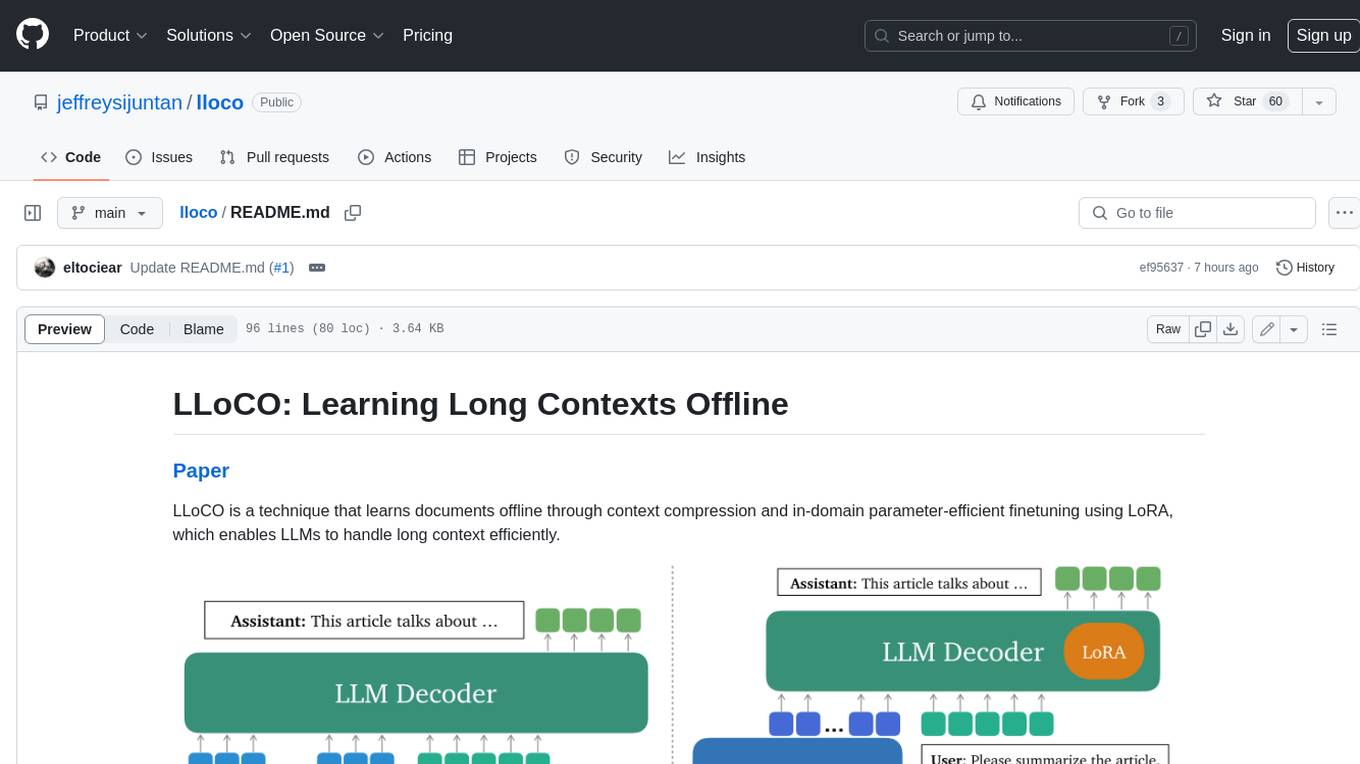
lloco
LLoCO is a technique that learns documents offline through context compression and in-domain parameter-efficient finetuning using LoRA, which enables LLMs to handle long context efficiently.
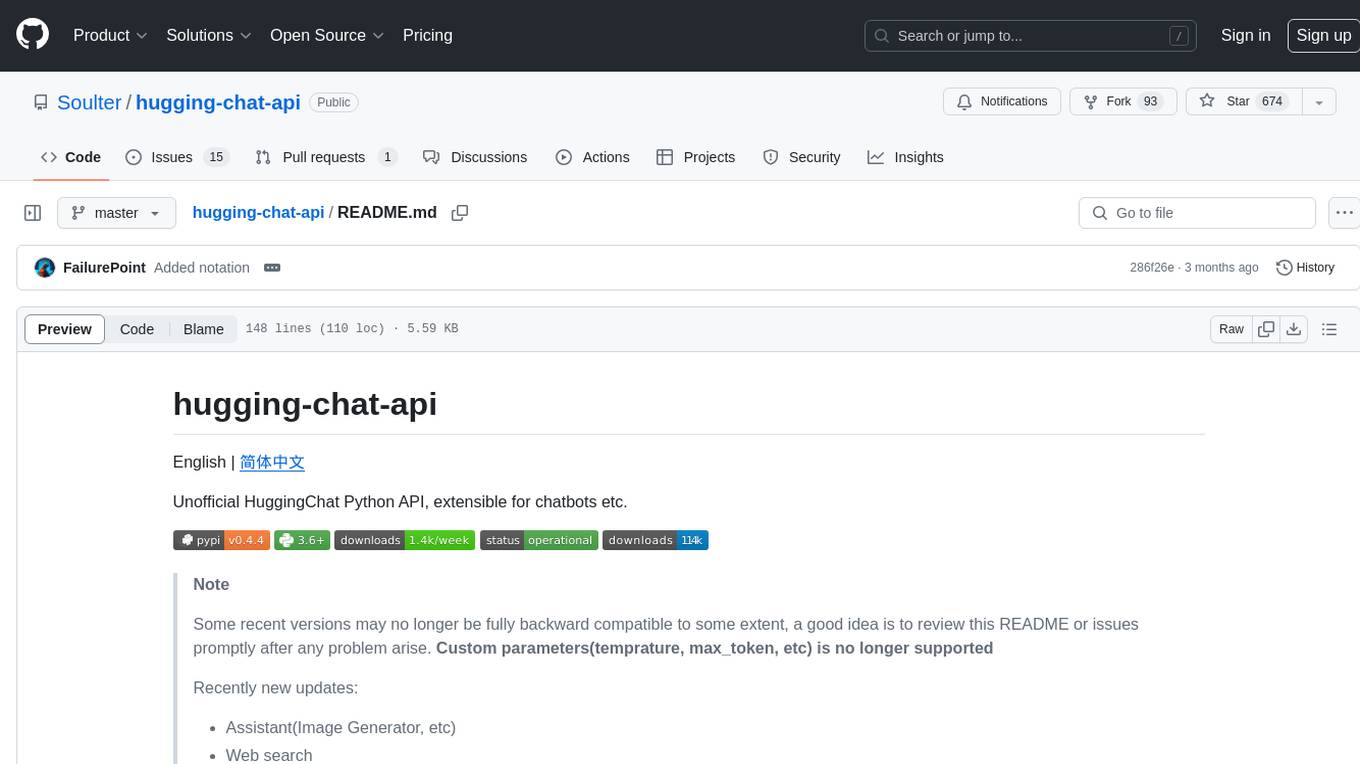
hugging-chat-api
Unofficial HuggingChat Python API for creating chatbots, supporting features like image generation, web search, memorizing context, and changing LLMs. Users can log in, chat with the ChatBot, perform web searches, create new conversations, manage conversations, switch models, get conversation info, use assistants, and delete conversations. The API also includes a CLI mode with various commands for interacting with the tool. Users are advised not to use the application for high-stakes decisions or advice and to avoid high-frequency requests to preserve server resources.
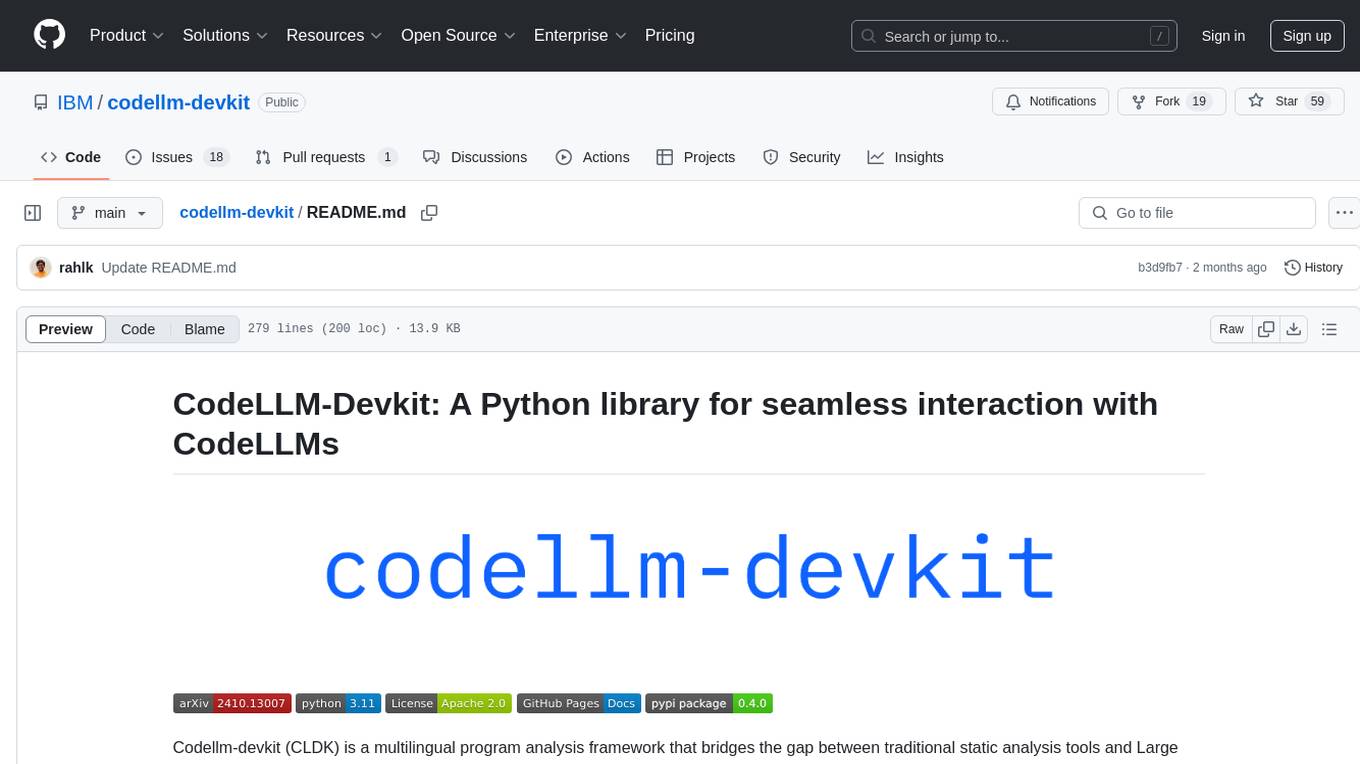
codellm-devkit
Codellm-devkit (CLDK) is a Python library that serves as a multilingual program analysis framework bridging traditional static analysis tools and Large Language Models (LLMs) specialized for code (CodeLLMs). It simplifies the process of analyzing codebases across multiple programming languages, enabling the extraction of meaningful insights and facilitating LLM-based code analysis. The library provides a unified interface for integrating outputs from various analysis tools and preparing them for effective use by CodeLLMs. Codellm-devkit aims to enable the development and experimentation of robust analysis pipelines that combine traditional program analysis tools and CodeLLMs, reducing friction in multi-language code analysis and ensuring compatibility across different tools and LLM platforms. It is designed to seamlessly integrate with popular analysis tools like WALA, Tree-sitter, LLVM, and CodeQL, acting as a crucial intermediary layer for efficient communication between these tools and CodeLLMs. The project is continuously evolving to include new tools and frameworks, maintaining its versatility for code analysis and LLM integration.
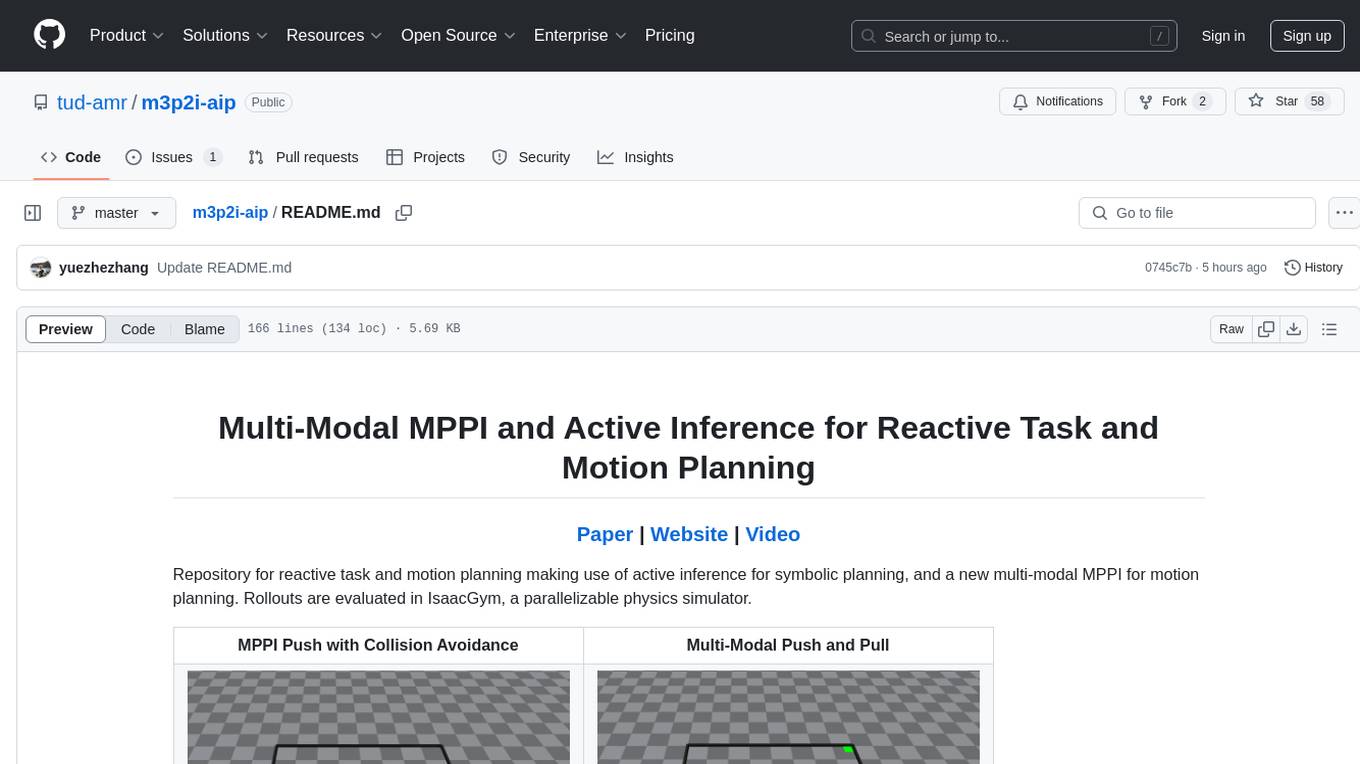
m3p2i-aip
Repository for reactive task and motion planning using active inference for symbolic planning and multi-modal MPPI for motion planning. Rollouts are evaluated in IsaacGym, a parallelizable physics simulator. The tool provides functionalities for push, pull, pick, and multi-modal push-pull tasks with collision avoidance.
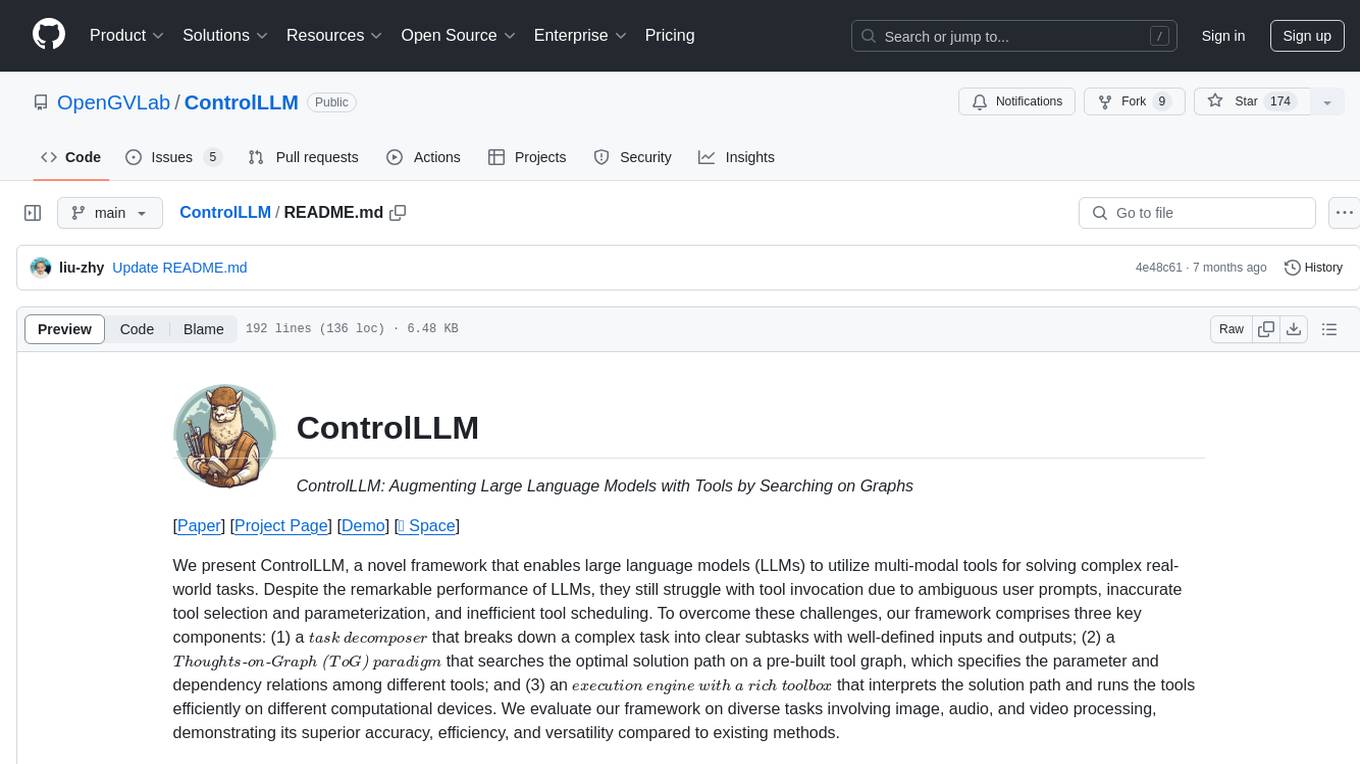
ControlLLM
ControlLLM is a framework that empowers large language models to leverage multi-modal tools for solving complex real-world tasks. It addresses challenges like ambiguous user prompts, inaccurate tool selection, and inefficient tool scheduling by utilizing a task decomposer, a Thoughts-on-Graph paradigm, and an execution engine with a rich toolbox. The framework excels in tasks involving image, audio, and video processing, showcasing superior accuracy, efficiency, and versatility compared to existing methods.
For similar tasks
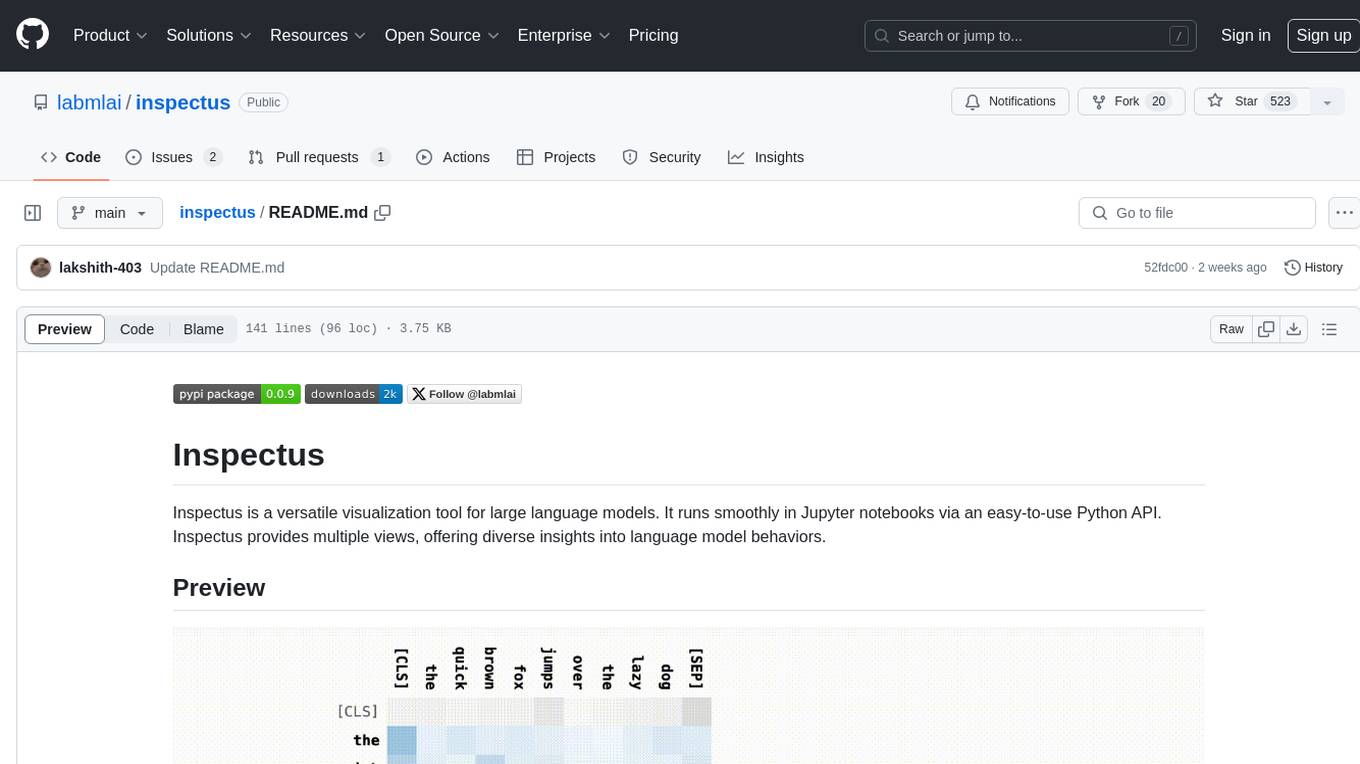
inspectus
Inspectus is a versatile visualization tool for large language models. It provides multiple views, including Attention Matrix, Query Token Heatmap, Key Token Heatmap, and Dimension Heatmap, to offer insights into language model behaviors. Users can interact with the tool in Jupyter notebooks through an easy-to-use Python API. Inspectus allows users to visualize attention scores between tokens, analyze how tokens focus on each other during processing, and explore the relationships between query and key tokens. The tool supports the visualization of attention maps from Huggingface transformers and custom attention maps, making it a valuable resource for researchers and developers working with language models.
For similar jobs
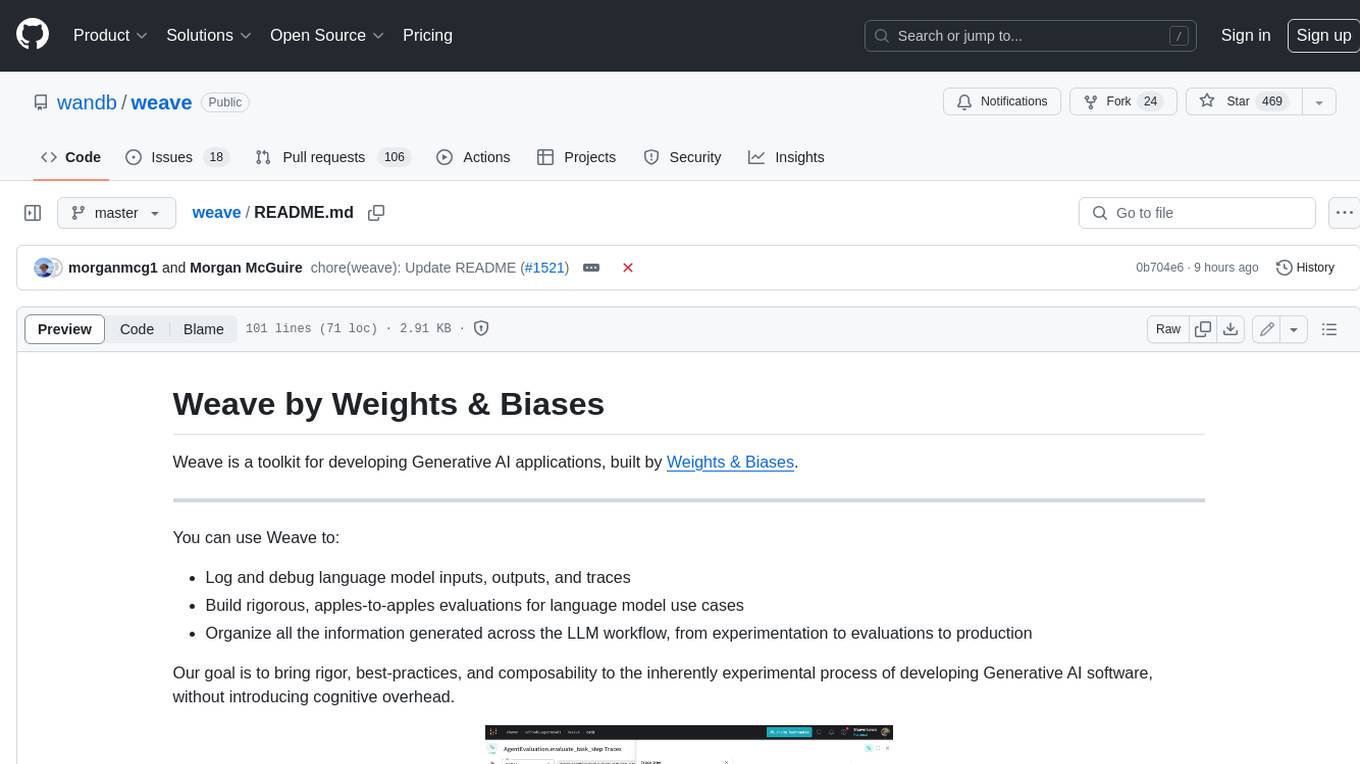
weave
Weave is a toolkit for developing Generative AI applications, built by Weights & Biases. With Weave, you can log and debug language model inputs, outputs, and traces; build rigorous, apples-to-apples evaluations for language model use cases; and organize all the information generated across the LLM workflow, from experimentation to evaluations to production. Weave aims to bring rigor, best-practices, and composability to the inherently experimental process of developing Generative AI software, without introducing cognitive overhead.
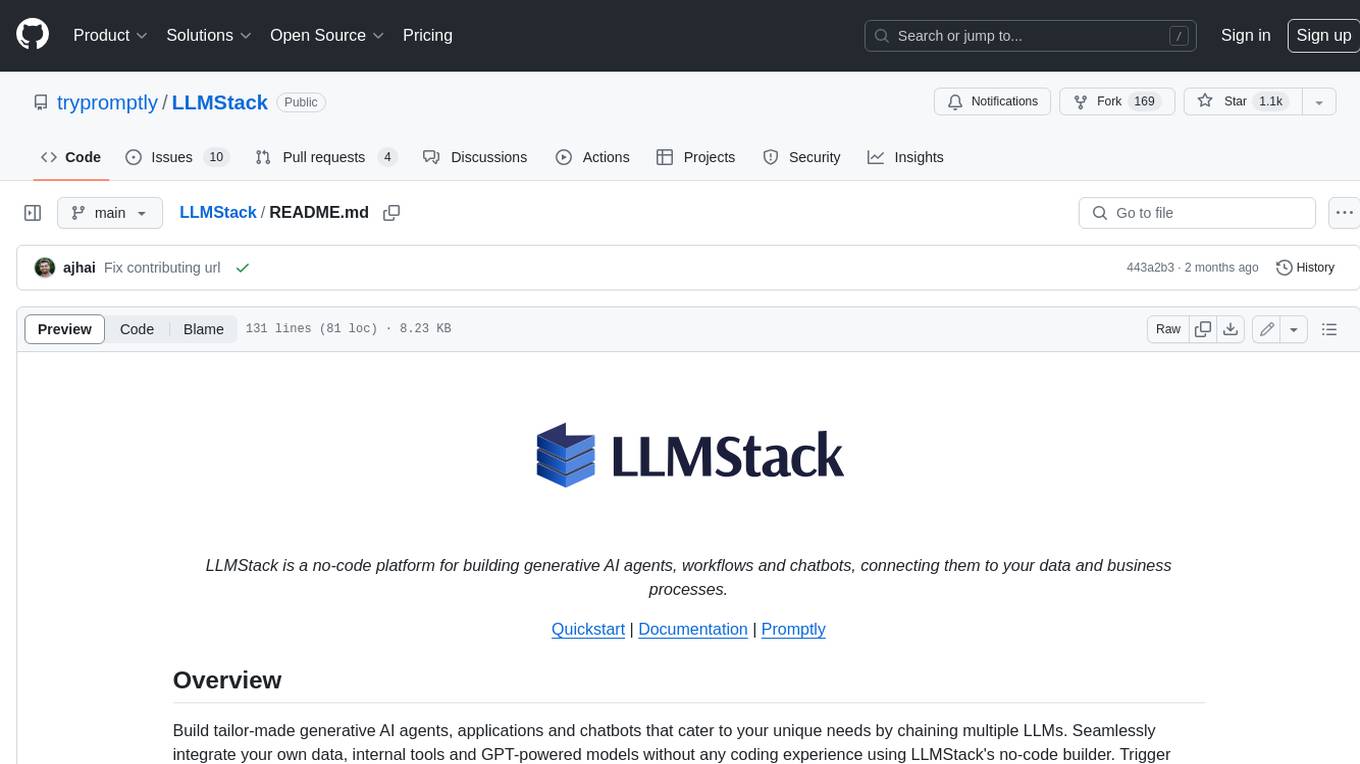
LLMStack
LLMStack is a no-code platform for building generative AI agents, workflows, and chatbots. It allows users to connect their own data, internal tools, and GPT-powered models without any coding experience. LLMStack can be deployed to the cloud or on-premise and can be accessed via HTTP API or triggered from Slack or Discord.
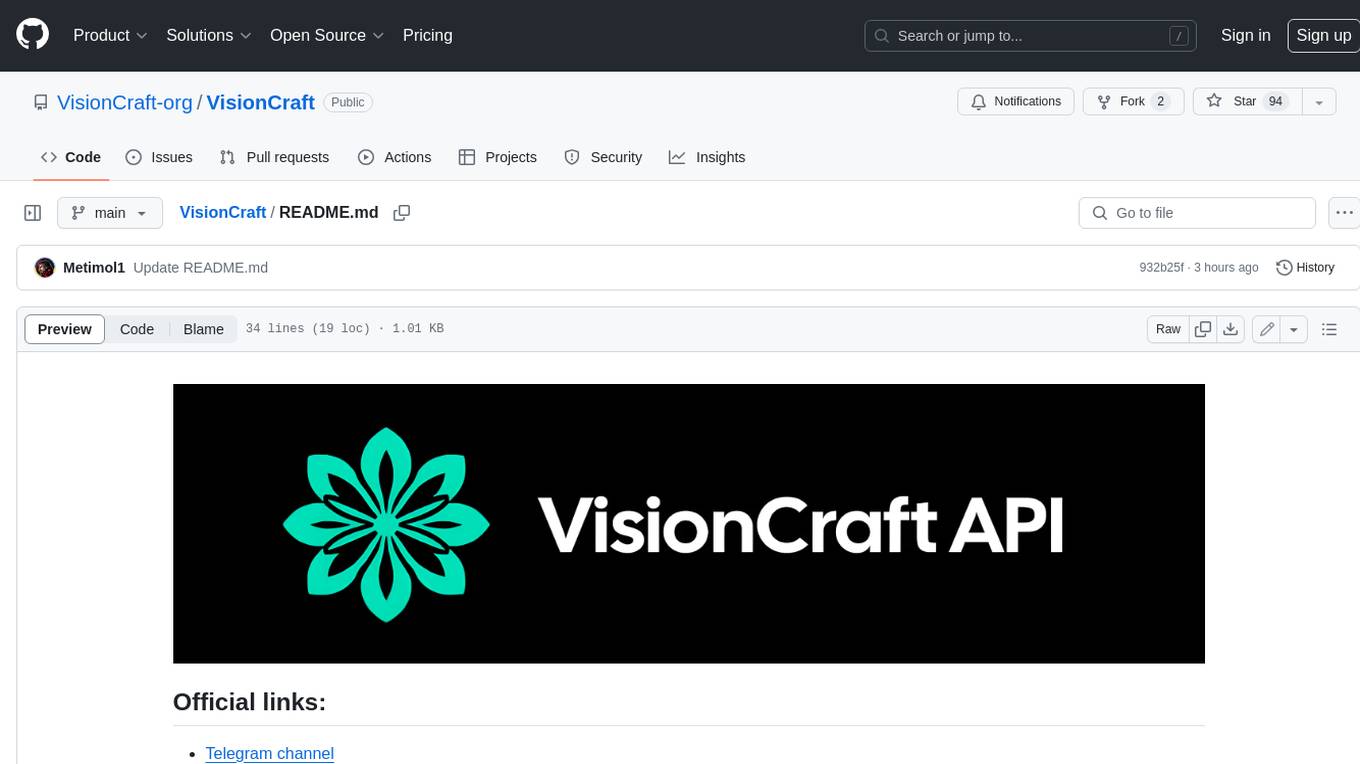
VisionCraft
The VisionCraft API is a free API for using over 100 different AI models. From images to sound.
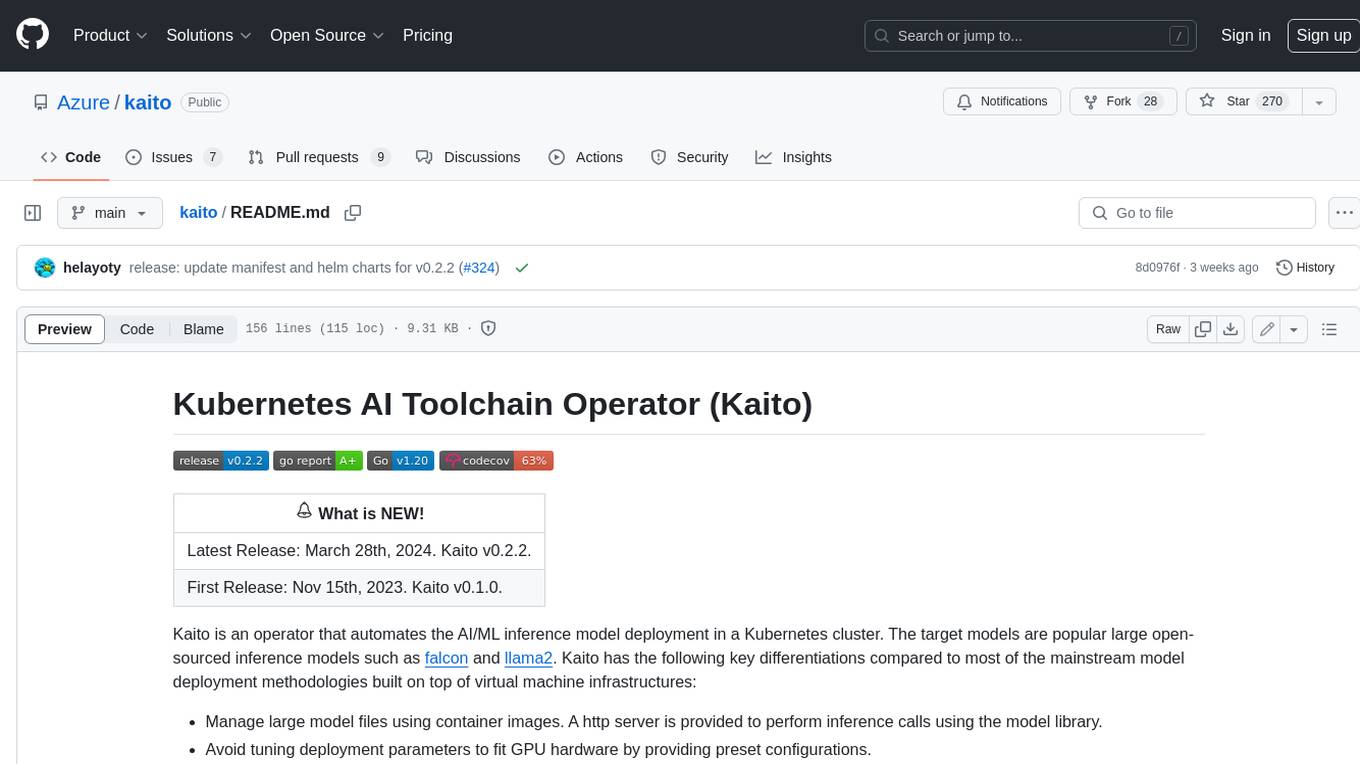
kaito
Kaito is an operator that automates the AI/ML inference model deployment in a Kubernetes cluster. It manages large model files using container images, avoids tuning deployment parameters to fit GPU hardware by providing preset configurations, auto-provisions GPU nodes based on model requirements, and hosts large model images in the public Microsoft Container Registry (MCR) if the license allows. Using Kaito, the workflow of onboarding large AI inference models in Kubernetes is largely simplified.
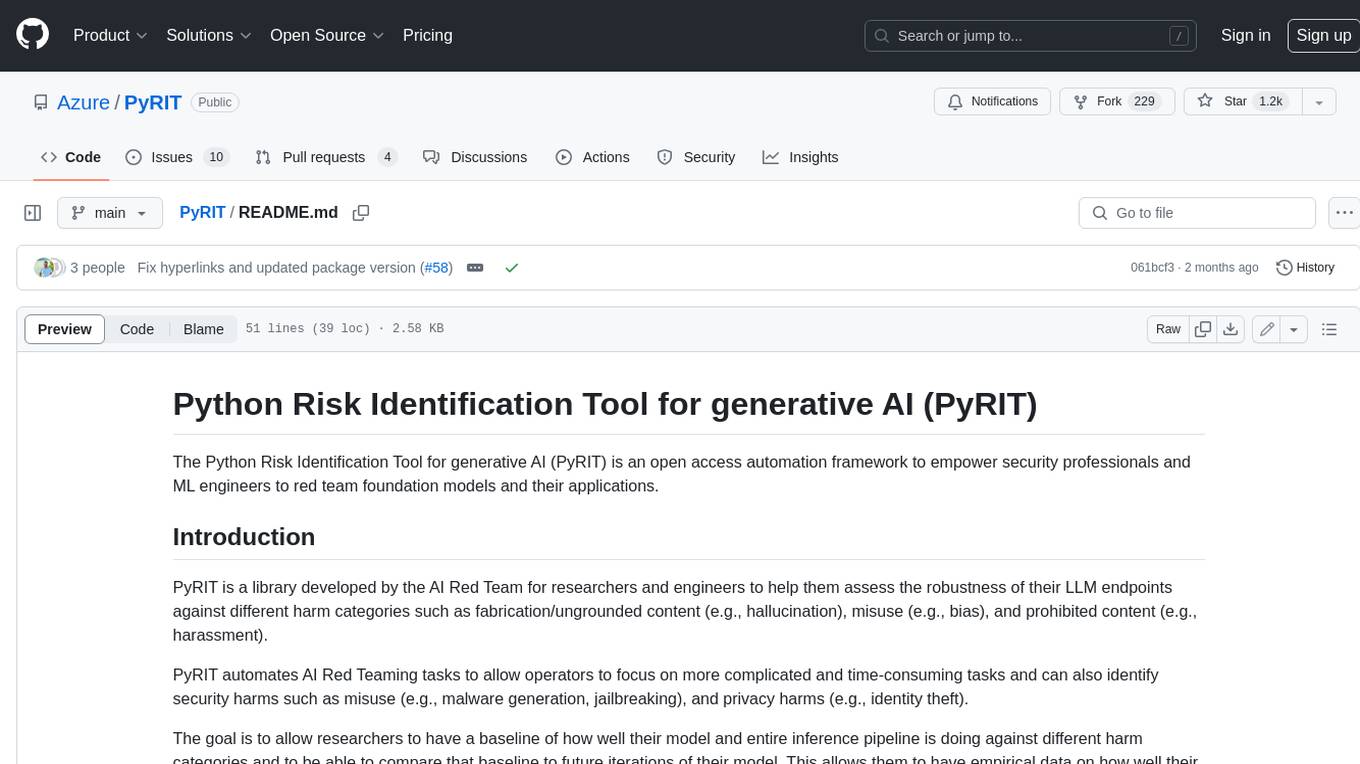
PyRIT
PyRIT is an open access automation framework designed to empower security professionals and ML engineers to red team foundation models and their applications. It automates AI Red Teaming tasks to allow operators to focus on more complicated and time-consuming tasks and can also identify security harms such as misuse (e.g., malware generation, jailbreaking), and privacy harms (e.g., identity theft). The goal is to allow researchers to have a baseline of how well their model and entire inference pipeline is doing against different harm categories and to be able to compare that baseline to future iterations of their model. This allows them to have empirical data on how well their model is doing today, and detect any degradation of performance based on future improvements.
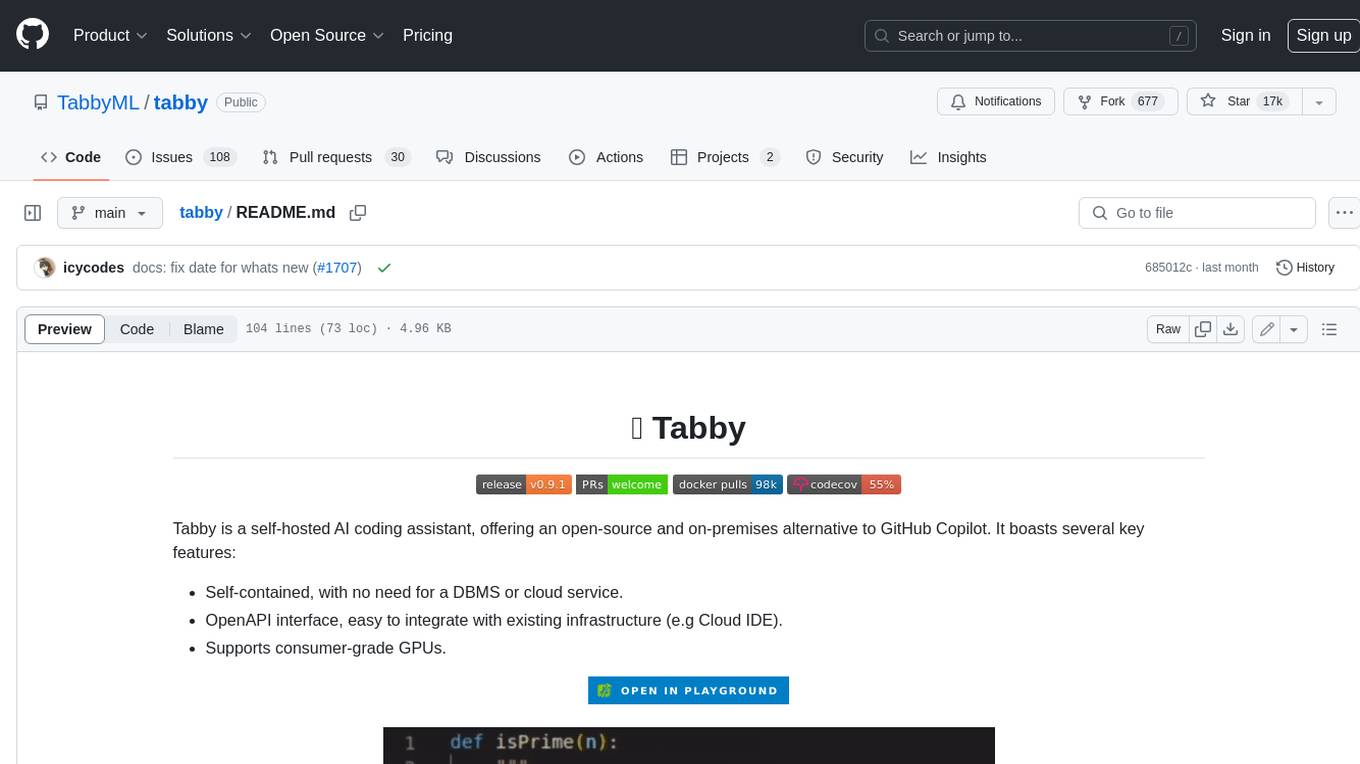
tabby
Tabby is a self-hosted AI coding assistant, offering an open-source and on-premises alternative to GitHub Copilot. It boasts several key features: * Self-contained, with no need for a DBMS or cloud service. * OpenAPI interface, easy to integrate with existing infrastructure (e.g Cloud IDE). * Supports consumer-grade GPUs.
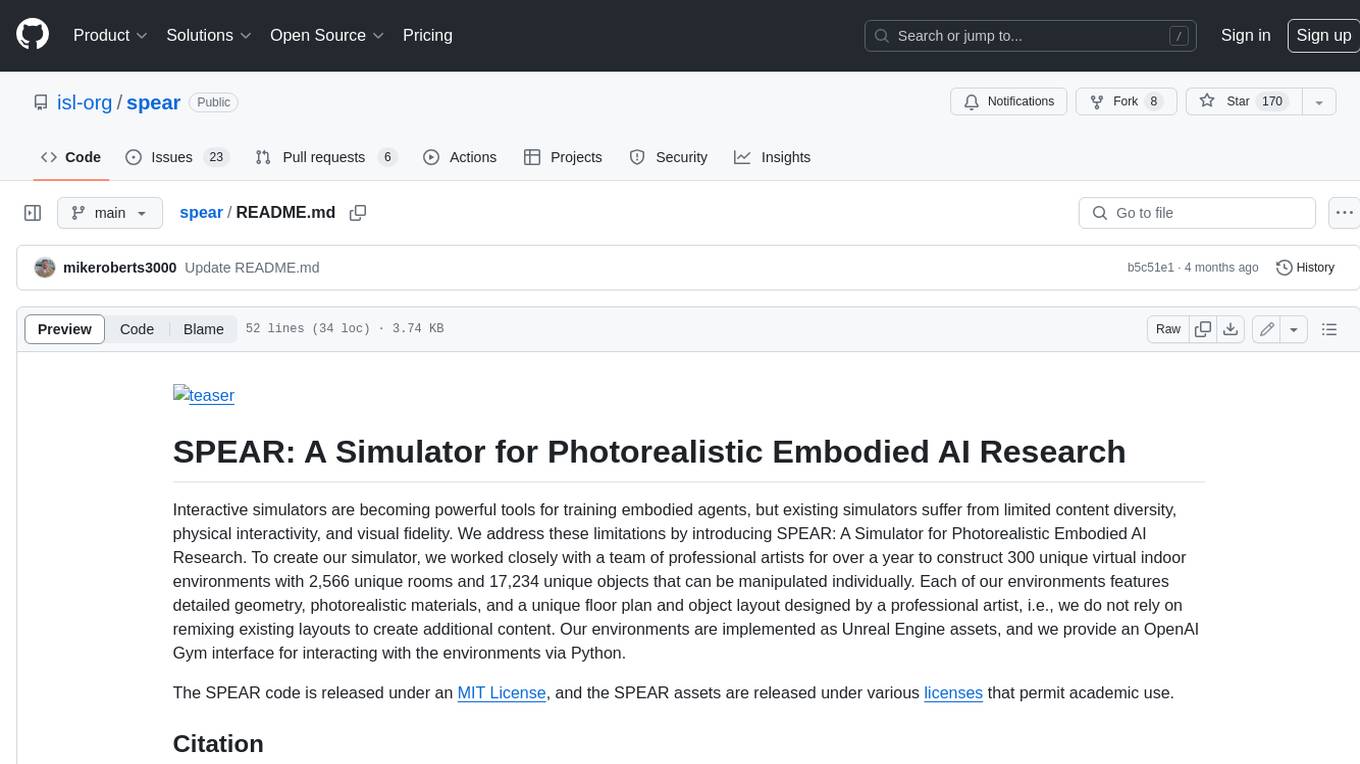
spear
SPEAR (Simulator for Photorealistic Embodied AI Research) is a powerful tool for training embodied agents. It features 300 unique virtual indoor environments with 2,566 unique rooms and 17,234 unique objects that can be manipulated individually. Each environment is designed by a professional artist and features detailed geometry, photorealistic materials, and a unique floor plan and object layout. SPEAR is implemented as Unreal Engine assets and provides an OpenAI Gym interface for interacting with the environments via Python.
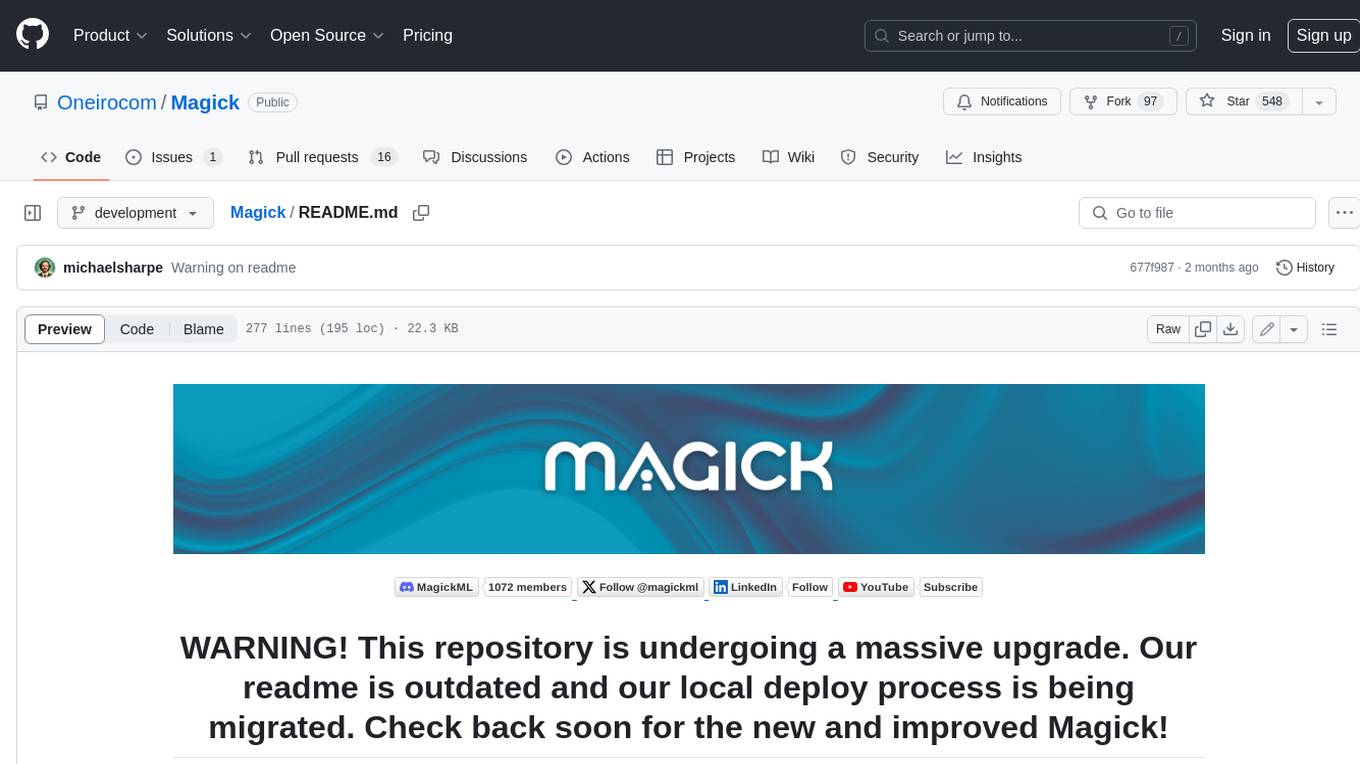
Magick
Magick is a groundbreaking visual AIDE (Artificial Intelligence Development Environment) for no-code data pipelines and multimodal agents. Magick can connect to other services and comes with nodes and templates well-suited for intelligent agents, chatbots, complex reasoning systems and realistic characters.