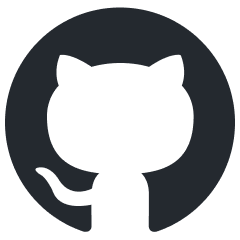
tappas
High-performance, optimized pre-trained template AI application pipelines for systems using Hailo devices
Stars: 122
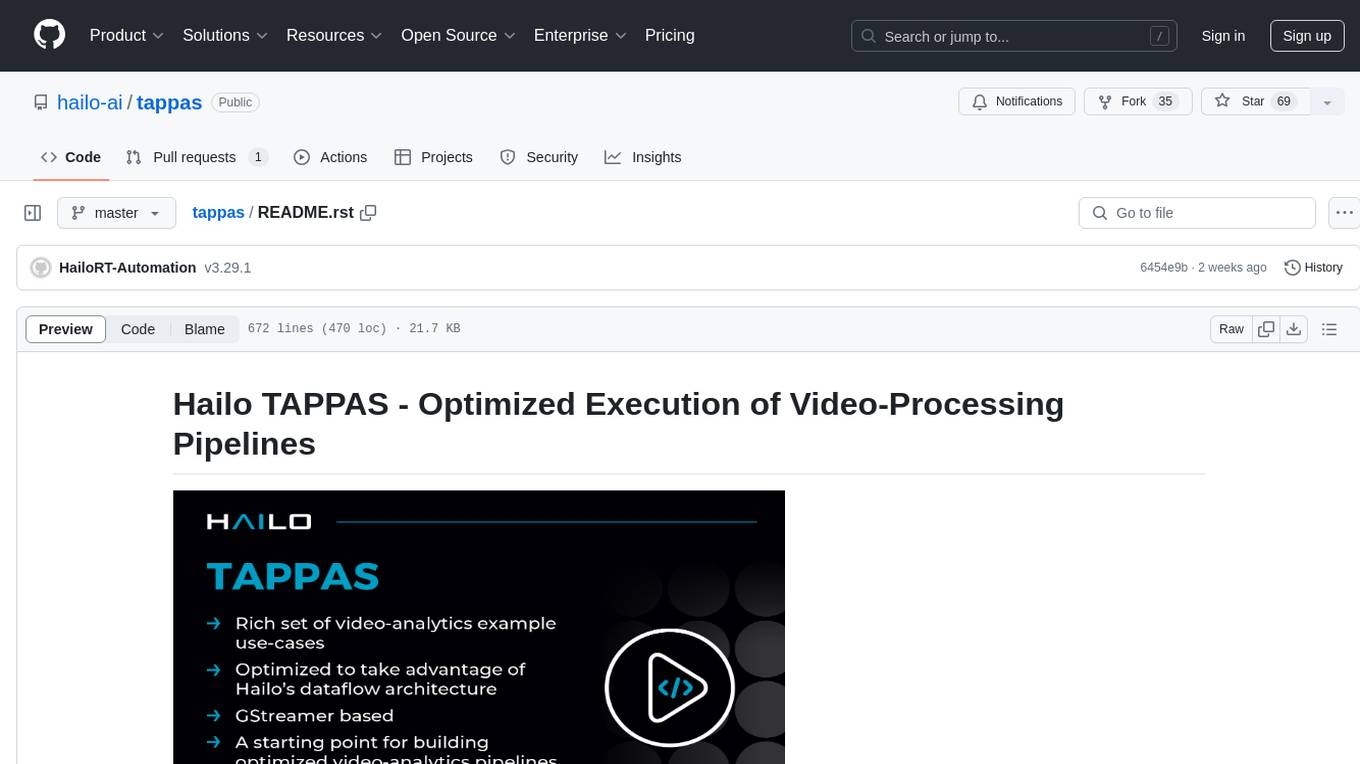
Hailo TAPPAS is a set of full application examples that implement pipeline elements and pre-trained AI tasks. It demonstrates Hailo's system integration scenarios on predefined systems, aiming to accelerate time to market, simplify integration with Hailo's runtime SW stack, and provide a starting point for customers to fine-tune their applications. The tool supports both Hailo-15 and Hailo-8, offering various example applications optimized for different common hosts. TAPPAS includes pipelines for single network, two network, and multi-stream processing, as well as high-resolution processing via tiling. It also provides example use case pipelines like License Plate Recognition and Multi-Person Multi-Camera Tracking. The tool is regularly updated with new features, bug fixes, and platform support.
README:
.. |gstreamer| image:: https://img.shields.io/badge/gstreamer-1.16%20%7C%201.18%20%7C%201.20-blue :target: https://gstreamer.freedesktop.org/ :alt: Gstreamer 1.16 | 1.18 | 1.20 :width: 150 :height: 20
.. |hailort| image:: https://img.shields.io/badge/HailoRT-4.19.0-green :target: https://github.com/hailo-ai/hailort :alt: HailoRT :height: 20
.. |license| image:: https://img.shields.io/badge/License-LGPLv2.1-green :target: https://github.com/hailo-ai/tappas/blob/master/LICENSE :alt: License: LGPL v2.1 :height: 20
.. |check_mark| image:: ./resources/check_mark.png :width: 20 :align: middle
.. image:: ./resources/github_Tappas_Mar24.jpg :height: 300 :width: 600 :align: center
.. raw:: html
|gstreamer| |hailort| |license|
TAPPAS is Hailo's set of full application examples, implementing pipeline elements and pre-trained AI tasks.
Demonstrating Hailo's system integration scenario of specific use cases on predefined systems (software and Hardware platforms). It can be used for evaluations, reference code and demos:
- Accelerating time to market by reducing development time and deployment effort
- Simplifying integration with Hailo’s runtime SW stack
- Providing a starting point for customers to fine-tune their applications
.. image:: ./resources/HAILO_TAPPAS_SW_STACK.svg
Prerequisites ^^^^^^^^^^^^^
- Hailo-8 device
- HailoRT PCIe driver installed
- At least 6GB's of free disk space
.. note:: This version is compatible with HailoRT v4.20.
Installation ^^^^^^^^^^^^
.. list-table:: :header-rows: 1
-
- Option
- Instructions
- Supported OS
-
- Hailo SW Suite*
-
SW Suite Install guide <docs/installation/sw-suite-install.rst>
_ - Ubuntu x86 20.04, Ubuntu x86 22.04
-
- Pre-built Docker image
-
Docker install guide <docs/installation/docker-install.rst>
_ - Ubuntu x86 20.04, Ubuntu x86 22.04, Ubuntu aarch64 20.04 (64-bit)
-
- Manual install
-
Manual install guide <docs/installation/manual-install.rst>
_ - Ubuntu x86 20.04, Ubuntu x86 22.04, Ubuntu aarch64 20.04
-
- Yocto installation
-
Read more about Yocto installation <docs/installation/yocto.rst>
_ - Yocto supported BSP's
-
- Raspberry Pi 4 installation
-
Read more about Raspberry Pi 4 installation <docs/installation/raspberry-pi-install.rst>
_ - Raspberry Pi OS
-
- Raspberry Pi 5 installation
-
Read more about Raspberry Pi 5 installation <https://github.com/hailo-ai/hailo-rpi5-examples/blob/main/doc/install-raspberry-pi5.md>
_ - Raspberry Pi OS
* It is recommended to start your development journey by first installing the Hailo SW Suite
Documentation ^^^^^^^^^^^^^
-
Framework architecture and elements documentation <docs/TAPPAS_architecture.rst>
_ -
Guide to writing your own C++ postprocess element <docs/write_your_own_application/write-your-own-postprocess.rst>
_ -
Guide to writing your own Python postprocess element <docs/write_your_own_application/write-your-own-python-postprocess.rst>
_ -
Debugging and profiling performance <docs/write_your_own_application/debugging.rst>
_ -
Cross compile <tools/cross_compiler/README.rst>
_ - A guide for cross-compiling
TAPPAS is now released separately for Hailo-8, for Hailo-15 please refer to https://github.com/hailo-ai/tappas/tree/master-vpu.
For a quick start with Hailo-15, please refer to the Vision Processor Software Package documentation section
in Hailo's Developer Zone <https://hailo.ai/developer-zone/documentation/>
_.
.. note:: For the Raspberry Pi 5 applications, go to
Hailo Raspberry Pi 5 Examples <https://github.com/hailo-ai/hailo-rpi5-examples>
_.
.. note:: These example applications are part of the Hailo AI Software Suite.
Hailo offers an additional set of
Application Code Examples <https://github.com/hailo-ai/Hailo-Application-Code-Examples>
_.
TAPPAS comes with a rich set of pre-configured pipelines optimized for different common hosts.
.. important:: * All example applications utilize both the host (for non-neural tasks) and the Neural-Network Core (for neural-networks inference), therefore performance results are affected by the host. * General application examples do not include any architecture-specific accelerator usage, and therefore will provide the easiest way to run an application, but with sub-optimal performance. * Architecture-specific application examples (i.MX, Raspberry PI, etc.) use platform-specific hardware accelerators and are not compatible with different architectures.
.. note:: All i.MX example application are validated on i.MX8 and i.MX6 platforms and are compatible with the architectures.
.. note:: Running application examples requires a direct connection to a monitor.
Basic Single Network Pipelines ^^^^^^^^^^^^^^^^^^^^^^^^^^^^^^
Pipelines that run a single network. The diagram below shows the pipeline data-flow.
.. image:: resources/single_net_pipeline.jpg
The following table details the currently available examples.
.. list-table:: :header-rows: 1 :stub-columns: 1 :widths: 40 12 12 12 12 12 :align: center
-
-
General <apps/h8/gstreamer/general/README.rst>
_ -
i.MX8 <apps/h8/gstreamer/imx8/README.rst>
_ -
RPi4 <apps/h8/gstreamer/raspberrypi/README.rst>
_ -
x86 Hardware Accelerated <apps/h8/gstreamer/x86_hw_accelerated/README.rst>
_ -
Rockchip <apps/h8/gstreamer/rockchip/README.rst>
_
-
- Object Detection
- |check_mark|
- |check_mark|
- |check_mark|
- |check_mark|
-
- Depth Estimation
- |check_mark|
- |check_mark|
- |check_mark|
-
- Instance segmentation
- |check_mark|
-
- Classification with Python Postprocessing
- |check_mark|
-
- Object Detection Multiple Devices (Century)
- |check_mark|
- |check_mark|
-
- Face Recognition
- |check_mark|
Two Network Pipelines ^^^^^^^^^^^^^^^^^^^^^
Examples of basic pipelines running two networks. The cascaded (serial) flow shows two networks running in series. This example pipeline is of the popular configuration where the first network is a detector which finds some Region-of-Interest (ROI) in the input image and the second network processes the cropped ROI (a face-detection-and-landmarking use case of this pipeline is shown at the top of this guide). The pipeline is shown in the following diagram:
.. image:: resources/cascaded_nets_pipeline.png
.. list-table:: :header-rows: 1 :stub-columns: 1 :widths: 40 12 12 12 12 12 :align: center
-
-
General <apps/h8/gstreamer/general/README.rst>
_ -
i.MX8 <apps/h8/gstreamer/imx8/README.rst>
_ -
RPi4 <apps/h8/gstreamer/raspberrypi/README.rst>
_ -
x86 Hardware Accelerated <apps/h8/gstreamer/x86_hw_accelerated/README.rst>
_ -
Rockchip <apps/h8/gstreamer/rockchip/README.rst>
_
-
- Cascaded - Face Detection & Landmarks
- |check_mark|
- |check_mark|
-
- Cascaded - Person Det & Single Person Pose Estimation
- |check_mark|
- |check_mark|
-
- Cascaded - Face Detection & Recognition
- |check_mark|
Multi-Stream Pipelines ^^^^^^^^^^^^^^^^^^^^^^
.. image:: docs/resources/one_network_multi_stream.png
.. list-table:: :header-rows: 1 :stub-columns: 1 :widths: 40 12 12 12 12 12 :align: center
-
-
General <apps/h8/gstreamer/general/README.rst>
_ -
i.MX8 <apps/h8/gstreamer/imx8/README.rst>
_ -
RPi4 <apps/h8/gstreamer/raspberrypi/README.rst>
_ -
x86 Hardware Accelerated <apps/h8/gstreamer/x86_hw_accelerated/README.rst>
_ -
Rockchip <apps/h8/gstreamer/rockchip/README.rst>
_
-
- Multi-stream Object Detection
- |check_mark|
- |check_mark|
- |check_mark|
-
- Multi-stream Multi-Device Object Detection
- |check_mark|
Pipelines for High-Resolution Processing Via Tiling ^^^^^^^^^^^^^^^^^^^^^^^^^^^^^^^^^^^^^^^^^^^^^^^^^^^
.. image:: docs/resources/tiling-example.png
.. list-table:: :header-rows: 1 :stub-columns: 1 :widths: 40 12 12 12 12 12 :align: center
-
-
General <apps/h8/gstreamer/general/README.rst>
_ -
i.MX8 <apps/h8/gstreamer/imx8/README.rst>
_ -
RPi4 <apps/h8/gstreamer/raspberrypi/README.rst>
_ -
x86 Hardware Accelerated <apps/h8/gstreamer/x86_hw_accelerated/README.rst>
_ -
Rockchip <apps/h8/gstreamer/rockchip/README.rst>
_
-
- HD Object Detection
- |check_mark|
- |check_mark|
Example Use Case Pipelines ^^^^^^^^^^^^^^^^^^^^^^^^^^
Our LPR reference application demonstrates the use of 3 networks, with a database. The pipeline demonstrates inference based decision making (Vehicle detection) for secondary inference tasks (License plate data extraction). This allows multiple networks to cooperate in the pipeline for reactive behavior.
.. image:: resources/lpr_pipeline.png
Our Multi-Person Multi-Camera Tracking reference application demonstrates person tracking across multiple streams using RE-ID tracking. The pipeline demonstrates another method for inference based decision making that also connects between different video streams.
.. image:: resources/re_id_pipeline.png
.. list-table:: :header-rows: 1 :stub-columns: 1 :widths: 40 12 12 12 12 12 :align: center
-
-
General <apps/h8/gstreamer/general/README.rst>
_ -
i.MX8 <apps/h8/gstreamer/imx8/README.rst>
_ -
RPi4 <apps/h8/gstreamer/raspberrypi/README.rst>
_ -
x86 Hardware Accelerated <apps/h8/gstreamer/x86_hw_accelerated/README.rst>
_ -
Rockchip <apps/h8/gstreamer/rockchip/README.rst>
_
-
- LPR
- |check_mark|
- |check_mark|
- |check_mark|
-
- RE-ID
- |check_mark|
If you need support, please post your question on our Hailo community Forum <https://community.hailo.ai/>
_ for assistance.
Contact information is available at hailo.ai <https://hailo.ai/contact-us/>
_.
v3.31.0 (December 2024)
- Known issue: detection.sh application in imx8m has some fps degradation.
v3.30.0 (October 2024)
- TAPPAS is now released separately for Hailo-8, for Hailo-15 please refer to https://github.com/hailo-ai/tappas/tree/master-vpu
- Various bug fixes and stability Improvements
v3.29.1 (August 2024)
-
Hailo-8:
- Updated infrastructure to better support Raspberry Pi 5
- Added an option to control Yolo (Detection) Hailort post-process parameters via a JSON configuration
- Semantic segmentation post-process now extracts the argmax tensor using Regular Expressions
v3.29.0 (July 2024)
-
Hailo-15:
- Updated all Hailo-15 example applications to use the latest API
- Added a new C++ based example application that demonstrates the use of the
Hailo-15 API
Link <core/hailo/apps/hailo15/ai_example_app/README.rst>
_ - This release is aligned with the Hailo-15 Vision Processor Software Package 2024-07
-
Hailo-8:
- Added a new example application which demonstrates x86 hardware-accelerated
multi-stream detection <apps/h8/gstreamer/x86_hw_accelerated/multistream_detection/README.rst>
_ - Various bug fixes and stability improvements for
Raspberry Pi 5 <https://github.com/hailo-ai/hailo-rpi5-examples>
_
- Added a new example application which demonstrates x86 hardware-accelerated
-
Fixed various stability issues across apps and platforms
v3.28.1 (May 2024)
- Added a new property to
hailomuxer
which allows the sub-frame to be leaky -
hailooverlay
now properly supports Hailo-15 with a new DMABuf sync mechanism -
hailovideoscale
(Hailo-15 Element) now supports theletterbox
property
v3.28.0 (April 2024)
- Tappas was updated in this version, with a revised list of supported platform and apps
- Added
yolov8
(as default) to Detection application examples - Fixed various stability issues across apps and platforms
- This release is aligned to Hailo-15 Vision Processor Software Package 2024-04
v3.27.2 (March 2024)
- Aligned to Hailo-15 Vision Processor Software Package 2024-01.2
- Added a new example application - Frontend Update
- Updated the hailo OSD API
- Detection application now works with an updated
hailonet
element - Various bugs fixes
v3.27.1 (February 2024)
- Aligned to Hailo-15 Vision Processor Software Package 2024-01.1
- Added a new Hailo-15 external host application that saves udp stream to file
v3.27.0 (January 2024)
-
Updated the Hailo-15 applications to use the updated Media Library implementation:
- Basic Security Camera (streaming)
- Detection
- Single Stream OSD (On-Screen Display)
-
Added a folder for external host scripts and added the UDP Stream Display script
.. note:: TAPPAS supports both Hailo-15 and Hailo-8. Temporarily, in this version, only the following Hailo-8 based example applications are supported:
* Detection
* yolov5
* mobilenet_ssd
* Multi-Stream Detection
* Multi-Stream Detection
* MultiStream Detection with Stream Multiplexer
* License Plate Recognition
These applications are supported under the general folder (x86-based platforms).
v3.26.2 (December 2023)
- Aligned to Hailo-15 Vision Processor Software Package 2023-10.2
- Add grayscale support for Media Library Front-end
- Various bug fixes for Hailo-15 pipelines
v3.26.1 (November 2023)
- Aligned to Hailo-15 Vision Processor Software Package 2023-10.1
- Updated OSD configuration to support new dynamic features and adjust to the updated Media Library implementation
- Added a script for displaying UDP streams
- Basic security camera (Media Library implementation) now support 5 outputs
v3.26.0 (October 2023)
-
Added Hailo-15 supported application examples:
- Detection
- License Plate Recognition
- A new Hailo-15 specific example application - Basic Security Camera (streaming)
-
Removed Yolact models support from Instance Segmentation
-
Various bug fixes:
- Fixed the Detection application on i.MX6 platforms
- Fixed an issue with Face Recognition which prevented faces to be recognized ins some scenarios
- Fixed an issue which caused a warning when running some networks
v3.25.0 (July 2023)
- Improved Yolov5seg post-process performance
- Updated Yolo networks to use the HailoRT native post-process (selected models)
- Added "non-blocking mode" and "wait-time" properties to hailoroundrobin element
v3.24.0 (March 2023)
-
Added support for
Rockchip RK3588 <apps/h8/gstreamer/rockchip/README.rst>
_ (validated on Firefly ITX-3588J platform) -
Video Management System now supports multi-device (Ubuntu 22.04 only)
-
Video Management System (single device) now works on Ubuntu 20.04
-
Added a new model to
Instance Segmentation Pipeline <apps/h8/gstreamer/general/instance_segmentation/README.rst>
_:-
yolov5seg
- which has improved performance compared toyolact
-
-
New applications for
i.MX8 <apps/h8/gstreamer/imx8/README.rst>
_:- Object Detection and Pose Estimation (cascaded)
- Multi-Stream Detection
-
Added a TAPPAS Graphic User Interface to easily run selected general example applications (preview) on the TAPPAS Docker - to activate it, run
tappas-gui
-
Added back
yolox_l_leaky
to theCentury general application <apps/h8/gstreamer/general/century/README.rst>
_ -
Reduced docker size
v3.23.1 (February 2023)
- Updated to HailoRT 4.12.1
- Fixed a documentation mistake in
Writing your own Python postprocess <docs/write_your_own_application/write-your-own-python-postprocess.rst>
_
v3.23.0 (December 2022)
-
New Apps:
-
Added
x86_hw_accelerated <apps/h8/gstreamer/x86_hw_accelerated/README.rst>
_ example pipelines that use Video Acceleration API (VA-API) over Intel processors that supportQuick Sync <https://en.wikipedia.org/wiki/Intel_Quick_Sync_Video>
_:- Video Management System - a pipeline that demonstrates a VMS application which runs several streams and different tasks - Face Recognition, Face Attributes and Person Attributes. Currently this example pipeline is supported on Ubuntu 22.04 only
-
Multi-stream detection <apps/h8/gstreamer/x86_hw_accelerated/multistream_detection/README.rst>
_ -
Century <apps/h8/gstreamer/x86_hw_accelerated/century/README.rst>
_
-
Pose Estimation pipeline with two cascading networks -
Person detection and single person pose estimation <apps/h8/gstreamer/general/cascading_networks/README.rst>
_ -
Face recognition <apps/h8/gstreamer/general/face_recognition/README.rst>
_ -
Updated i.MX6 Object Detection App - New network, updated the pipeline to include i.MX6 hardware acceleration
-
-
Added new models to
Instance Segmentation Pipeline <apps/h8/gstreamer/general/instance_segmentation/README.rst>
_:- yolact_regnetx_1.6gf
- yolact_regnetx_800mf (80 classes)
-
Century app <apps/h8/gstreamer/general/century/README.rst>
_ now uses a new network (yolov5m) -
Multi-Camera Multi-Person Tracking (RE-ID) <apps/h8/gstreamer/general/multi_person_multi_camera_tracking/README.rst>
_ - Improved pipeline performance and accuracy -
Added support for Ubuntu 22.04 (release-grade)
v3.22.0 (November 2022)
- New element
hailoimportzmq
- provides an entry point for importing metadata exported byhailoexportzmq
(HailoObjects) into the pipeline - Added Depth Estimation, Object Detection and Classification pipelines for i.MX6 Pipelines
- Changed the debugging tracers to use an internal tracing mechanism
v3.21.0 (October 2022)
-
New Apps:
-
Multi-stream detection that uses HailoRT Stream Multiplexer <apps/h8/gstreamer/general/multistream_detection/README.rst>
_ - Demonstrates the usage of HailoRT stream multiplexer (preview)
-
-
New elements -
hailoexportfile
andhailoexportmq
which provide an access point in the pipeline to export metadata (HailoObjects) -
Improved pipeline profiling by adding new tracers and replacing the GUI of
gst-shark <docs/write_your_own_application/debugging.rst>
_ -
Ubuntu 22 is now supported (GStreamer 1.20, preview)
-
Yocto Kirkstone is now supported (GStreamer 1.20)
v3.20.0 (August 2022)
-
New Apps:
-
Detection every X frames pipeline <apps/h8/gstreamer/general/detection/README.rst>
_ - Demonstrates the ability of skipping frames using a tracker
-
-
Improvements to Multi-Camera Multi-Person Tracking (RE-ID) pipeline (released)
v3.19.1 (July 2022)
-
New Apps:
- Multi-Camera Multi-Person Tracking (RE-ID) pipeline
multi_person_multi_camera_tracking.sh <apps/h8/gstreamer/general/multi_person_multi_camera_tracking/README.rst>
_ (preview)
- Multi-Camera Multi-Person Tracking (RE-ID) pipeline
v3.19.0 (June 2022)
-
New Apps:
- Added Cascading networks, Depth Estimation, Pose Estimation and Semantic Segmentation pipelines for
i.MX Pipelines <apps/h8/gstreamer/imx8/README.rst>
_
- Added Cascading networks, Depth Estimation, Pose Estimation and Semantic Segmentation pipelines for
-
Added an option to control post-process parameters via a JSON configuration for the detection application
-
Added support for Raspberry Pi Raspbian OS
-
Native Application now uses TAPPAS post-process
-
LPR (License Plate Recognition) pipeline is simplified to bash only
-
New detection post-process - Nanodet
.. note:: Ubuntu 18.04 will be deprecated in TAPPAS future version
.. note:: Python 3.6 will be deprecated in TAPPAS future version
v3.18.0 (April 2022)
-
New Apps:
- LPR (License Plate Recognition) pipeline and facial landmark pipeline for
i.MX Pipelines <apps/h8/gstreamer/imx8/README.rst>
_
- LPR (License Plate Recognition) pipeline and facial landmark pipeline for
-
Added the ability of compiling a specific TAPPAS target (post-processes, elements)
-
Improved the performance of Raspberry Pi example applications
v3.17.0 (March 2022)
-
New Apps:
- LPR (License Plate Recognition) pipeline for
General Pipelines <apps/h8/gstreamer/general/README.rst>
_ (preview) - Detection & pose estimation app
- Detection (MobilenetSSD) - Multi scale tiling app
- LPR (License Plate Recognition) pipeline for
-
Update infrastructure to use new HailoRT installation packages
-
Code is now publicly available on
Github <https://github.com/hailo-ai/tappas>
_
v3.16.0 (March 2022)
-
New Apps:
- Hailo
Century <https://hailo.ai/product-hailo/hailo-8-century-evaluation-platform/>
_ app - Demonstrates detection on one video file source over 6 different Hailo-8 devices - Python app - A classification app using a post-process written in Python
- Hailo
-
New Elements:
- Tracking element "HailoTracker" - Add tracking capabilities
- Python element "HailoPyFilter" - Enables to write post-processes using Python
-
Yocto Hardknott is now supported
-
Raspberry Pi 4 Ubuntu dedicated apps
-
HailoCropper cropping bug fixes
-
HailoCropper now accepts cropping method as a shared object (.so)
v3.14.1 (March 2022)
- Fix Yocto Gatesgarth compilation issue
- Added support for hosts without X-Video adapter
v3.15.0 (February 2022)
-
New Apps:
- Detection and depth estimation - Networks switch app
- Detection (MobilenetSSD) - Single scale tilling app
v3.14.0 (January 2022)
-
New Apps:
- Cascading apps - Face detection and then facial landmarking
-
New Yocto layer - Meta-hailo-tappas
-
Window enlargement is now supported
-
Added the ability to run on multiple devices
-
Improved latency on Multi-device RTSP app
v3.13.0 (November 2021)
-
Context switch networks in multi-stream apps are now supported
-
New Apps:
- Yolact - Instance segmentation
- FastDepth - Depth estimation
- Two networks in parallel on the same device - FastDepth + Mobilenet SSD
- Retinaface
-
Control Element Integration - Displaying device stats inside a GStreamer pipeline (Power, Temperature)
-
New Yocto recipes - Compiling our GStreamer plugins is now available as a Yocto recipe
-
Added a C++ detection example (native C++ example for writing an app, without GStreamer)
v3.12.0 (October 2021)
- Detection app - MobilenetSSD added
- NVR multi-stream multi device app (detection and pose estimation)
- Facial Landmarks app
- Segmentation app
- Classification app
- Face detection app
- Hailomuxer gstreamer element
- Postprocess implementations for various networks
- GStreamer infrastructure improvements
- Added ARM architecture support and documentation
v3.11.0 (September 2021)
- GStreamer based initial release
- NVR multi-stream detection app
- Detection app
- Hailofilter gstreamer element
- Pose Estimation app
For Tasks:
Click tags to check more tools for each tasksFor Jobs:
Alternative AI tools for tappas
Similar Open Source Tools
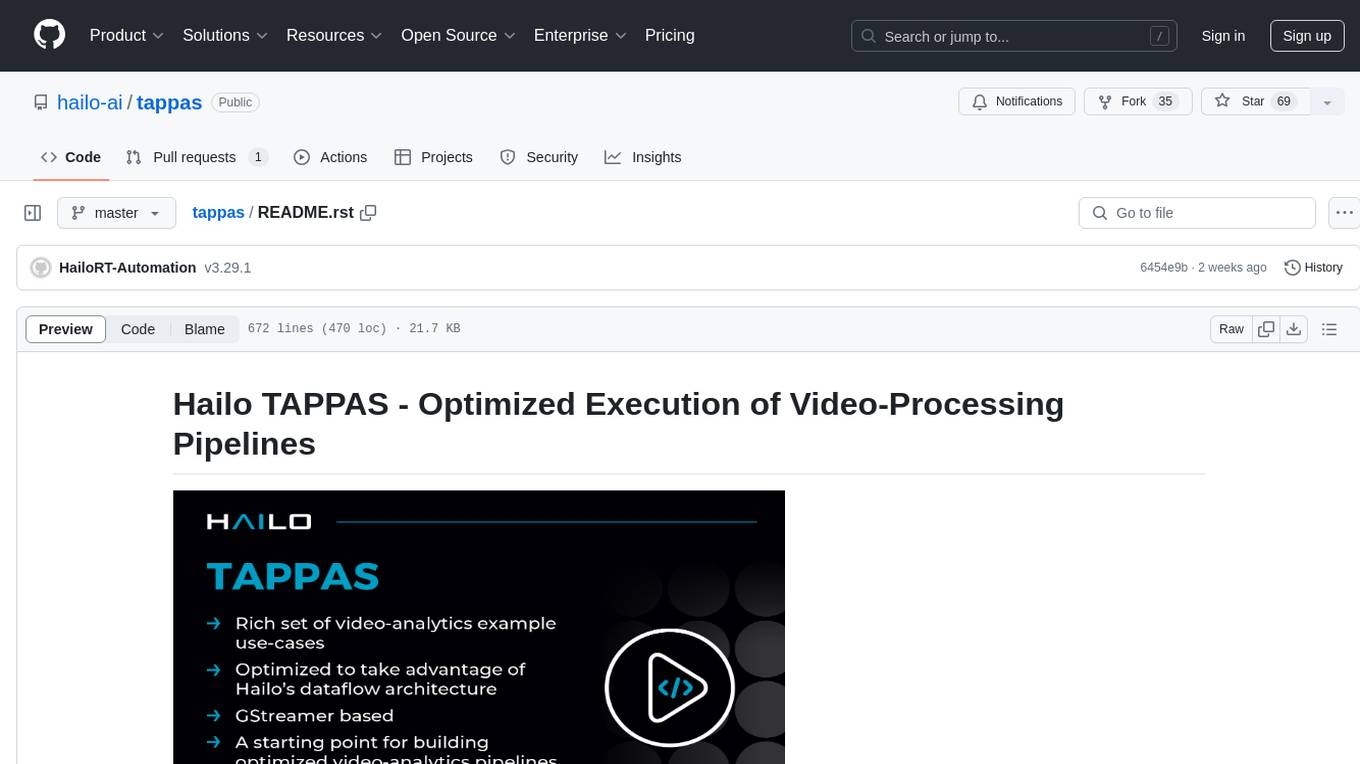
tappas
Hailo TAPPAS is a set of full application examples that implement pipeline elements and pre-trained AI tasks. It demonstrates Hailo's system integration scenarios on predefined systems, aiming to accelerate time to market, simplify integration with Hailo's runtime SW stack, and provide a starting point for customers to fine-tune their applications. The tool supports both Hailo-15 and Hailo-8, offering various example applications optimized for different common hosts. TAPPAS includes pipelines for single network, two network, and multi-stream processing, as well as high-resolution processing via tiling. It also provides example use case pipelines like License Plate Recognition and Multi-Person Multi-Camera Tracking. The tool is regularly updated with new features, bug fixes, and platform support.
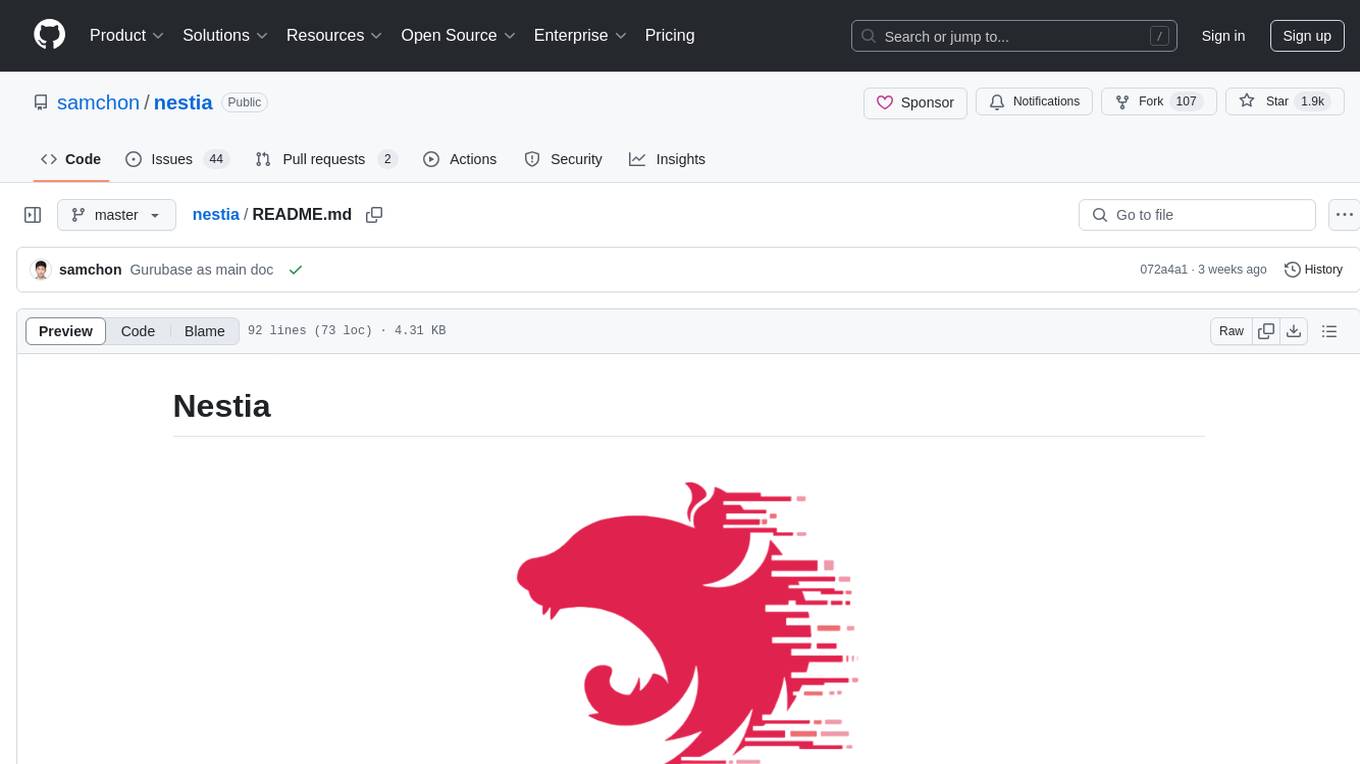
nestia
Nestia is a set of helper libraries for NestJS, providing super-fast/easy decorators, advanced WebSocket routes, Swagger generator, SDK library generator for clients, mockup simulator for client applications, automatic E2E test functions generator, test program utilizing e2e test functions, benchmark program using e2e test functions, super A.I. chatbot by Swagger document, Swagger-UI with online TypeScript editor, and a CLI tool. It enhances performance significantly and offers a collection of typed fetch functions with DTO structures like tRPC, along with a mockup simulator that is fully automated.
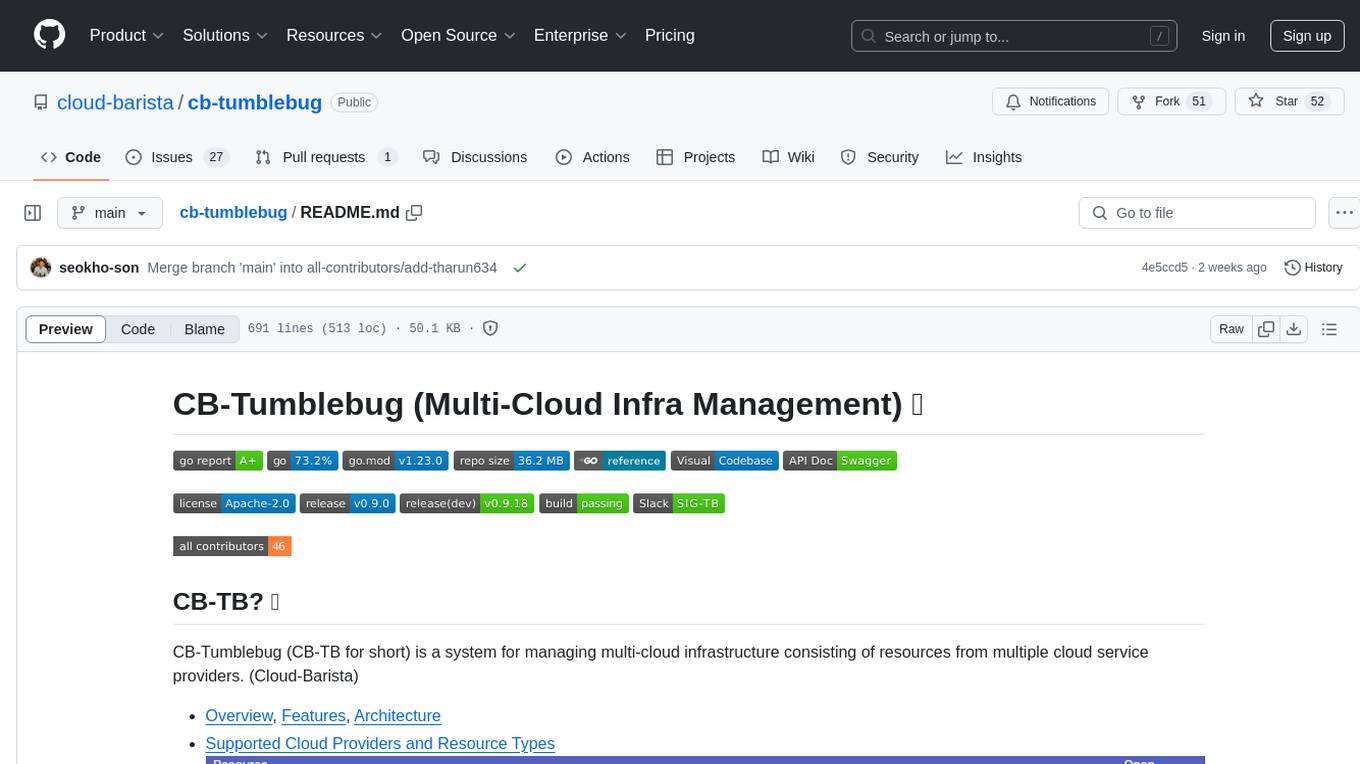
cb-tumblebug
CB-Tumblebug (CB-TB) is a system for managing multi-cloud infrastructure consisting of resources from multiple cloud service providers. It provides an overview, features, and architecture. The tool supports various cloud providers and resource types, with ongoing development and localization efforts. Users can deploy a multi-cloud infra with GPUs, enjoy multiple LLMs in parallel, and utilize LLM-related scripts. The tool requires Linux, Docker, Docker Compose, and Golang for building the source. Users can run CB-TB with Docker Compose or from the Makefile, set up prerequisites, contribute to the project, and view a list of contributors. The tool is licensed under an open-source license.
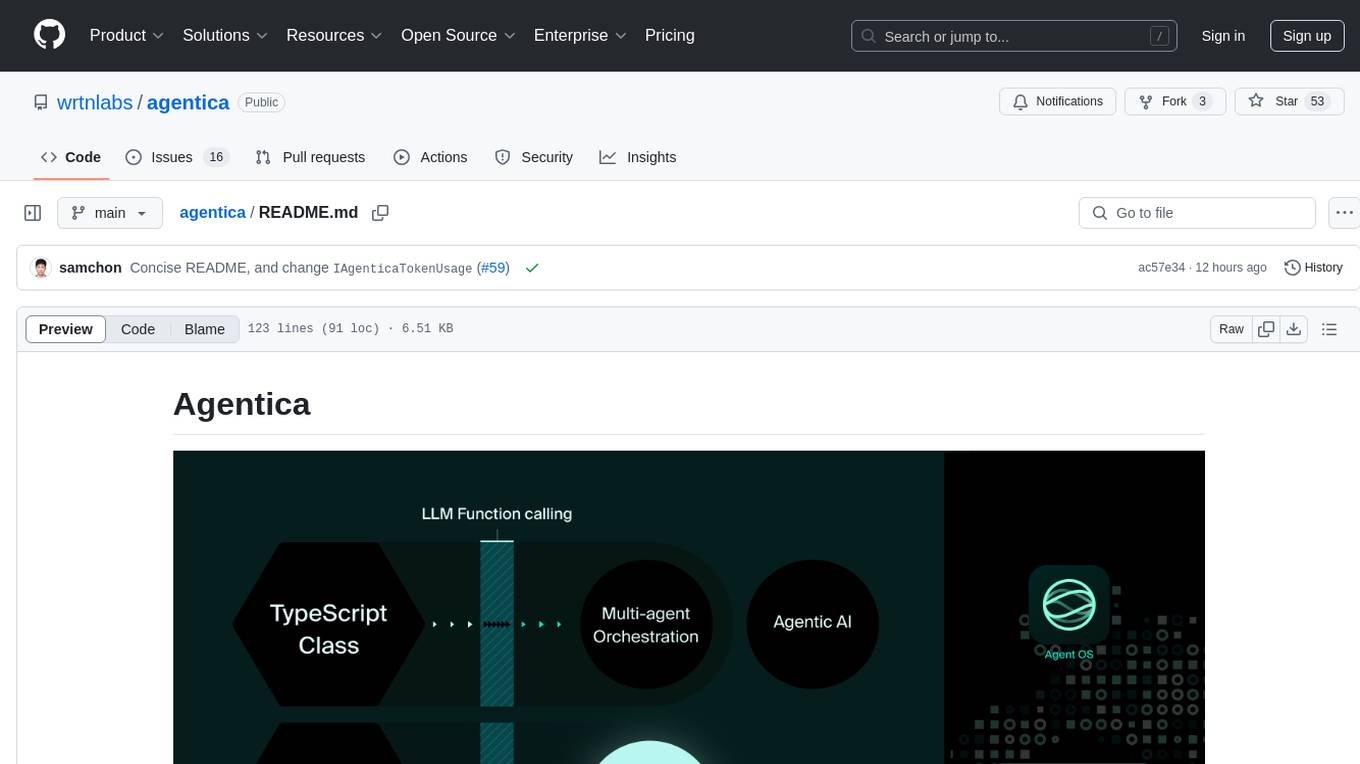
agentica
Agentica is a specialized Agentic AI library focused on LLM Function Calling. Users can provide Swagger/OpenAPI documents or TypeScript class types to Agentica for seamless functionality. The library simplifies AI development by handling various tasks effortlessly.
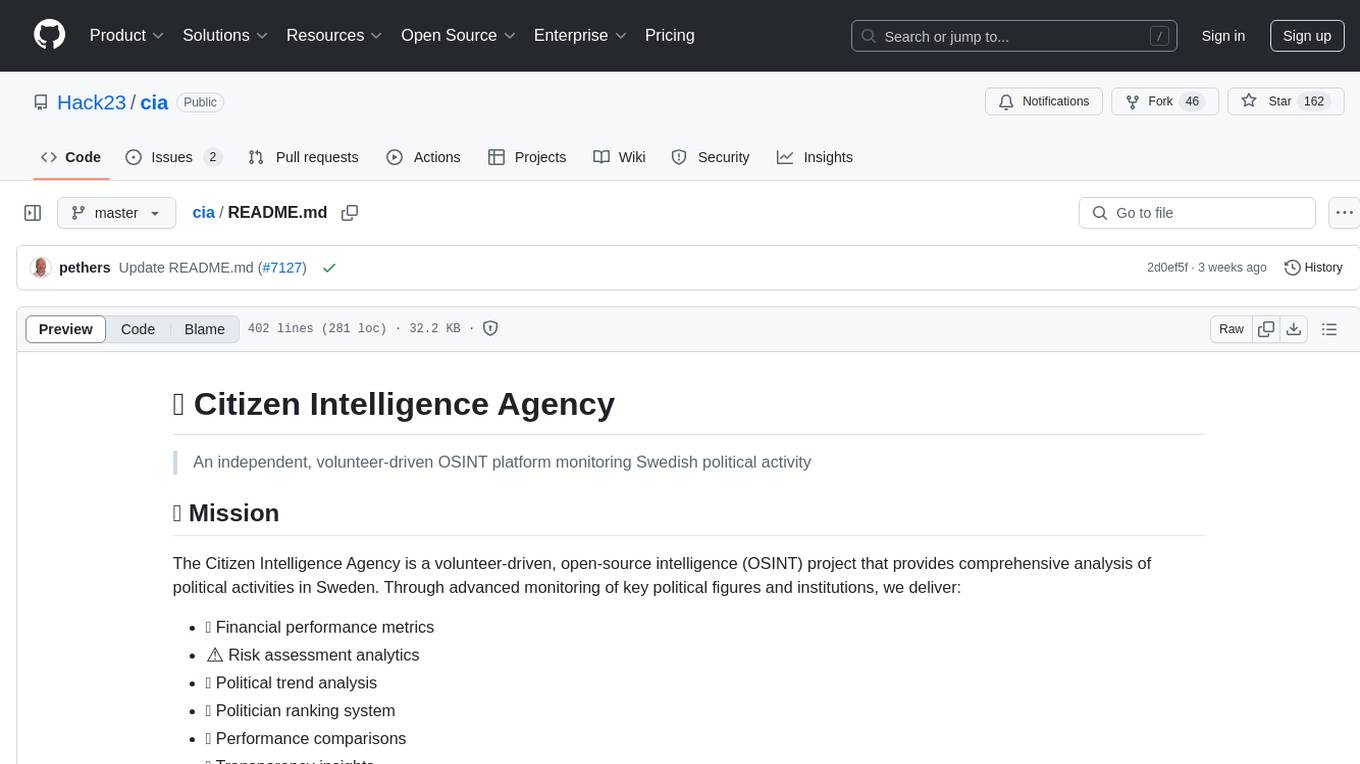
cia
CIA is a powerful open-source tool designed for data analysis and visualization. It provides a user-friendly interface for processing large datasets and generating insightful reports. With CIA, users can easily explore data, perform statistical analysis, and create interactive visualizations to communicate findings effectively. Whether you are a data scientist, analyst, or researcher, CIA offers a comprehensive set of features to streamline your data analysis workflow and uncover valuable insights.
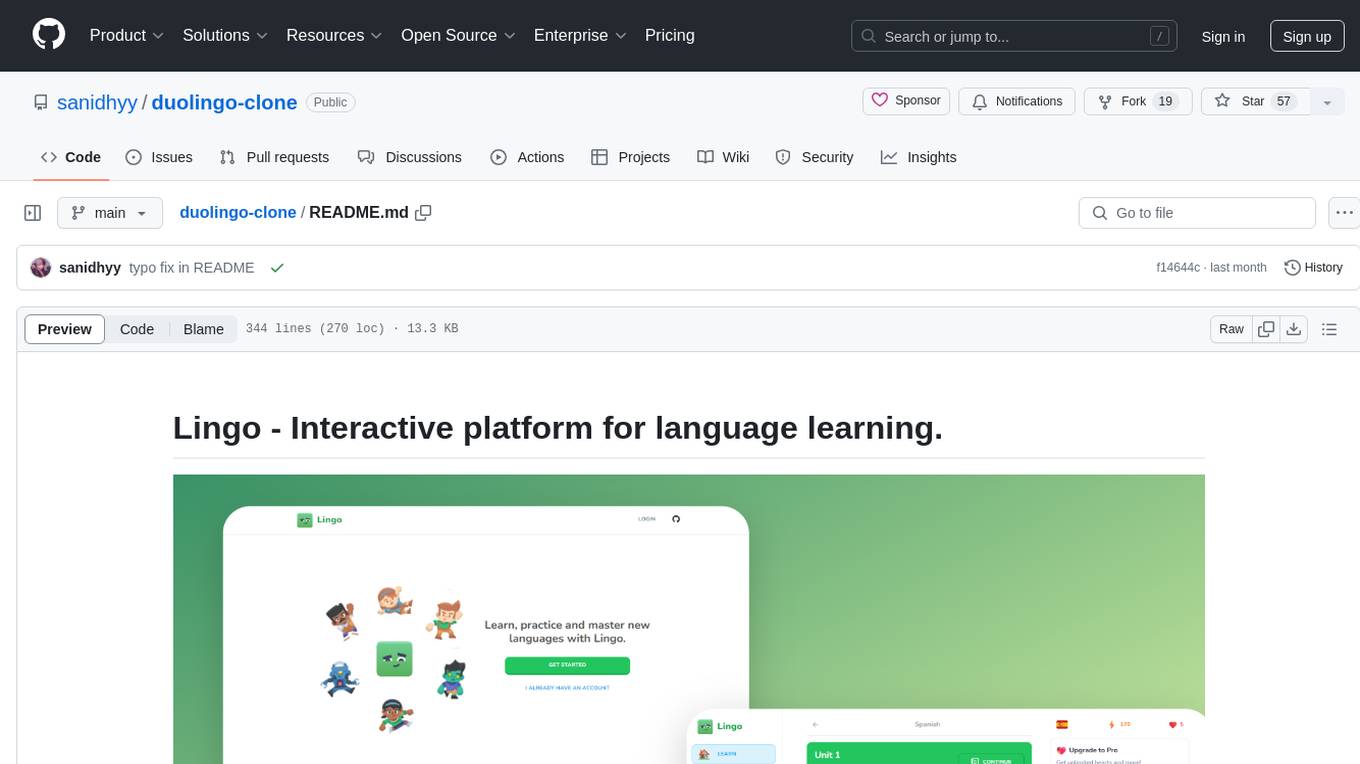
duolingo-clone
Lingo is an interactive platform for language learning that provides a modern UI/UX experience. It offers features like courses, quests, and a shop for users to engage with. The tech stack includes React JS, Next JS, Typescript, Tailwind CSS, Vercel, and Postgresql. Users can contribute to the project by submitting changes via pull requests. The platform utilizes resources from CodeWithAntonio, Kenney Assets, Freesound, Elevenlabs AI, and Flagpack. Key dependencies include @clerk/nextjs, @neondatabase/serverless, @radix-ui/react-avatar, and more. Users can follow the project creator on GitHub and Twitter, as well as subscribe to their YouTube channel for updates. To learn more about Next.js, users can refer to the Next.js documentation and interactive tutorial.
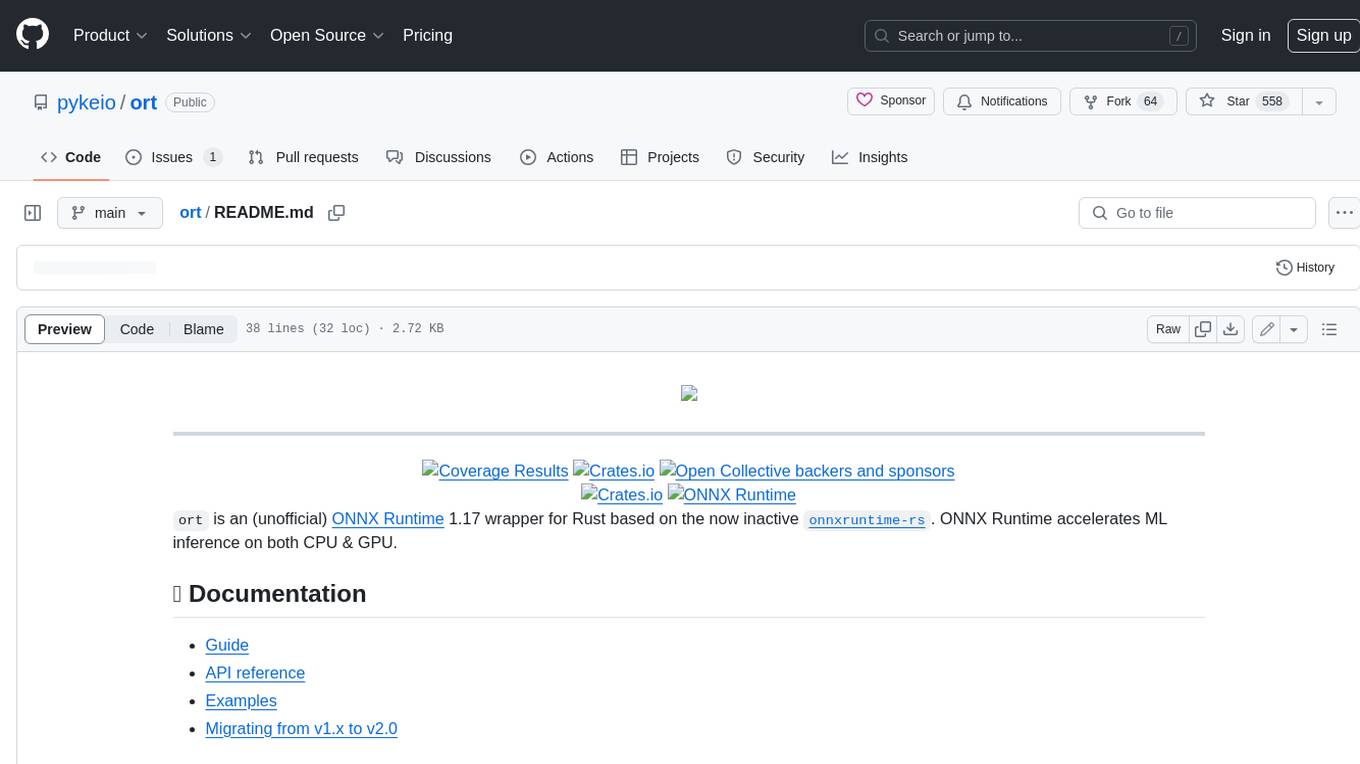
ort
Ort is an unofficial ONNX Runtime 1.17 wrapper for Rust based on the now inactive onnxruntime-rs. ONNX Runtime accelerates ML inference on both CPU and GPU.
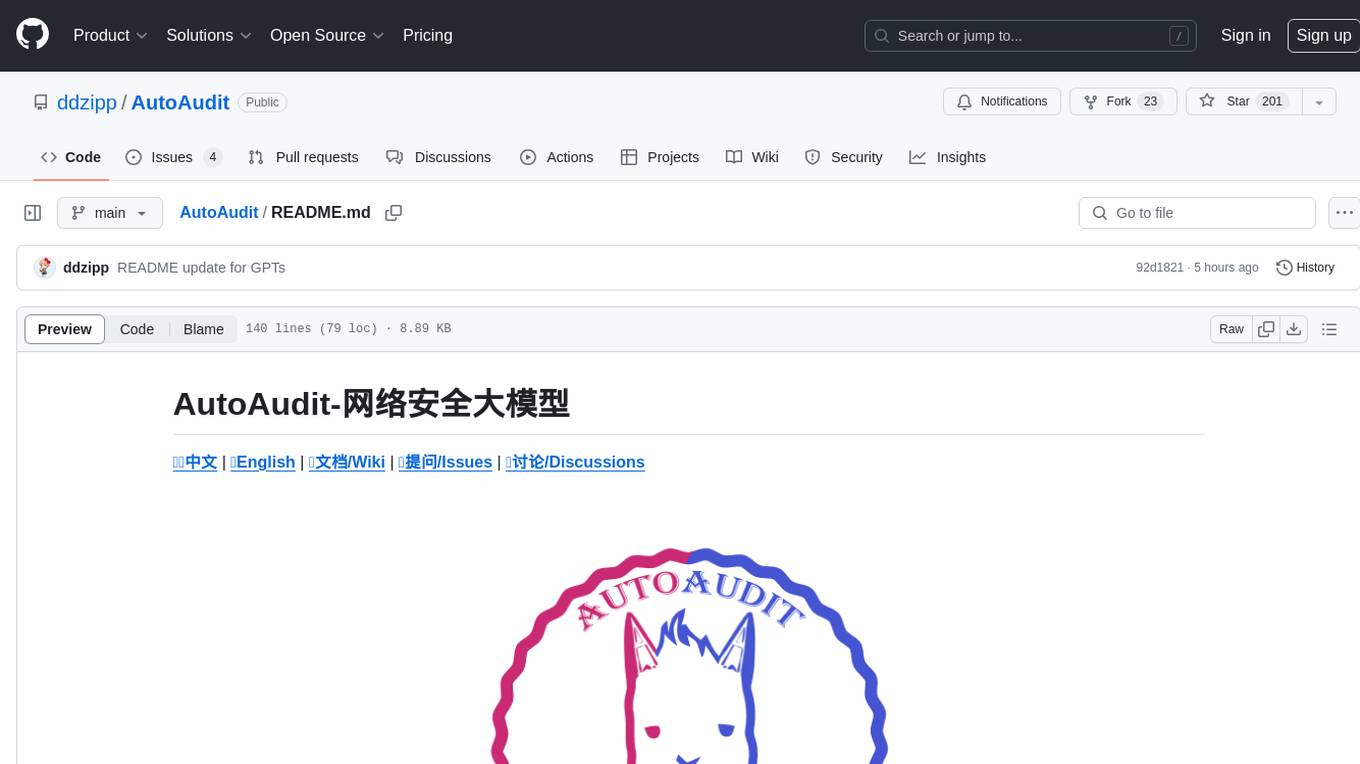
AutoAudit
AutoAudit is an open-source large language model specifically designed for the field of network security. It aims to provide powerful natural language processing capabilities for security auditing and network defense, including analyzing malicious code, detecting network attacks, and predicting security vulnerabilities. By coupling AutoAudit with ClamAV, a security scanning platform has been created for practical security audit applications. The tool is intended to assist security professionals with accurate and fast analysis and predictions to combat evolving network threats.
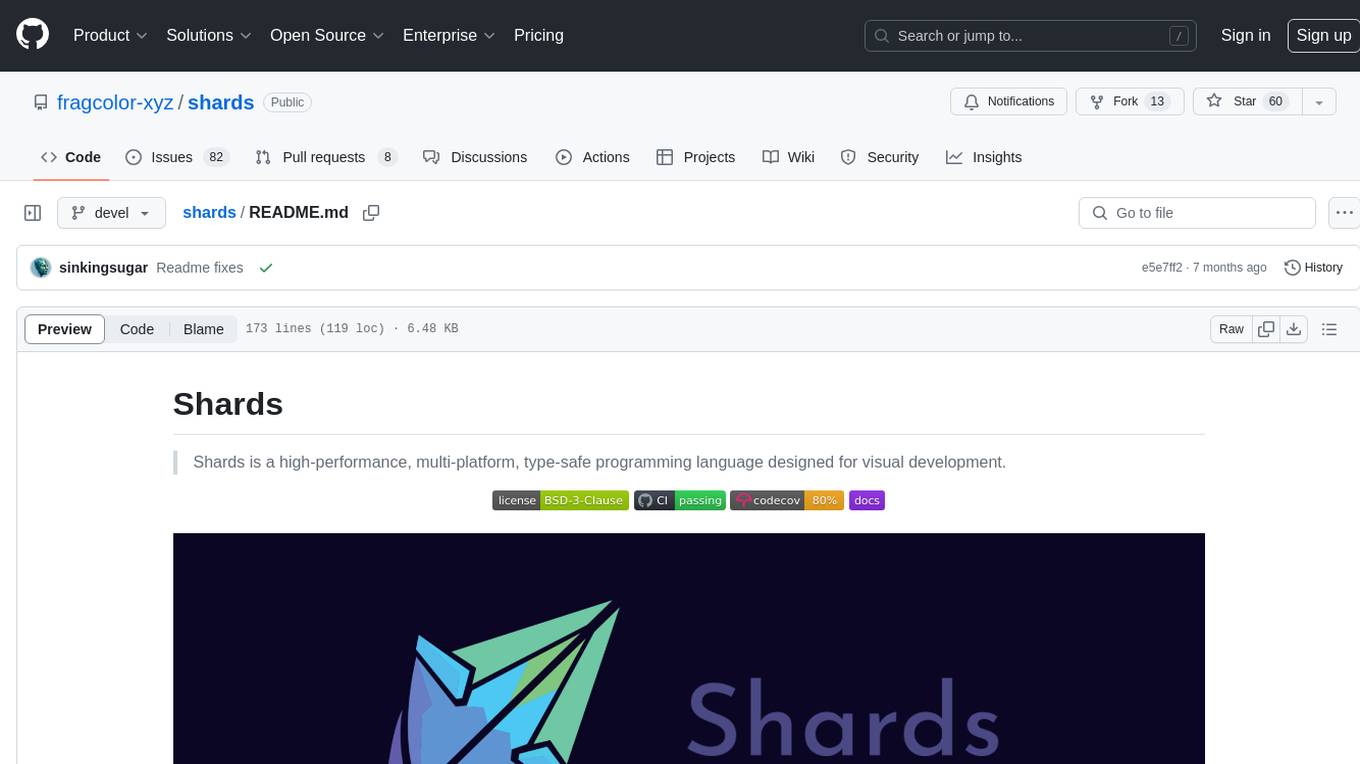
shards
Shards is a high-performance, multi-platform, type-safe programming language designed for visual development. It is a dataflow visual programming language that enables building full-fledged apps and games without traditional coding. Shards features automatic type checking, optimized shard implementations for high performance, and an intuitive visual workflow for beginners. The language allows seamless round-trip engineering between code and visual models, empowering users to create multi-platform apps easily. Shards also powers an upcoming AI-powered game creation system, enabling real-time collaboration and game development in a low to no-code environment.
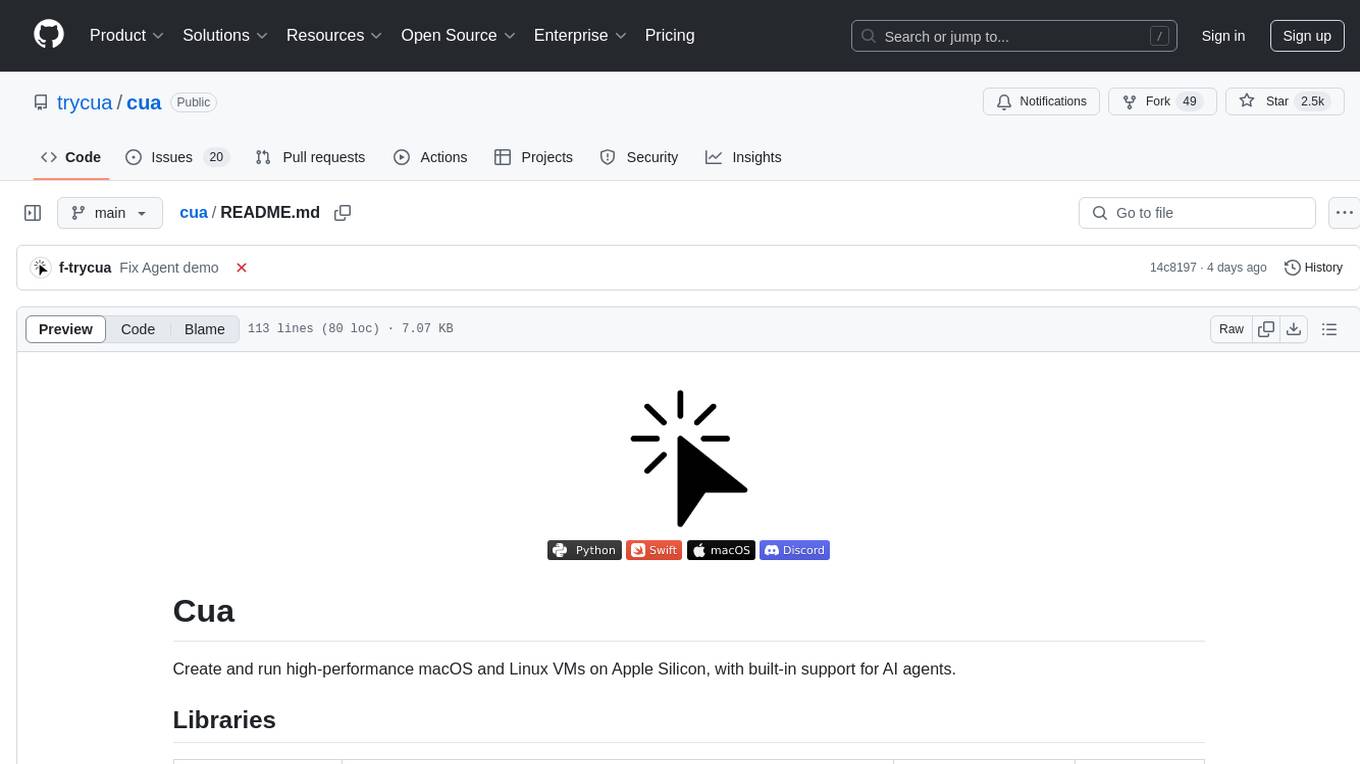
cua
Cua is a tool for creating and running high-performance macOS and Linux virtual machines on Apple Silicon, with built-in support for AI agents. It provides libraries like Lume for running VMs with near-native performance, Computer for interacting with sandboxes, and Agent for running agentic workflows. Users can refer to the documentation for onboarding, explore demos showcasing AI-Gradio and GitHub issue fixing, and utilize accessory libraries like Core, PyLume, Computer Server, and SOM. Contributions are welcome, and the tool is open-sourced under the MIT License.
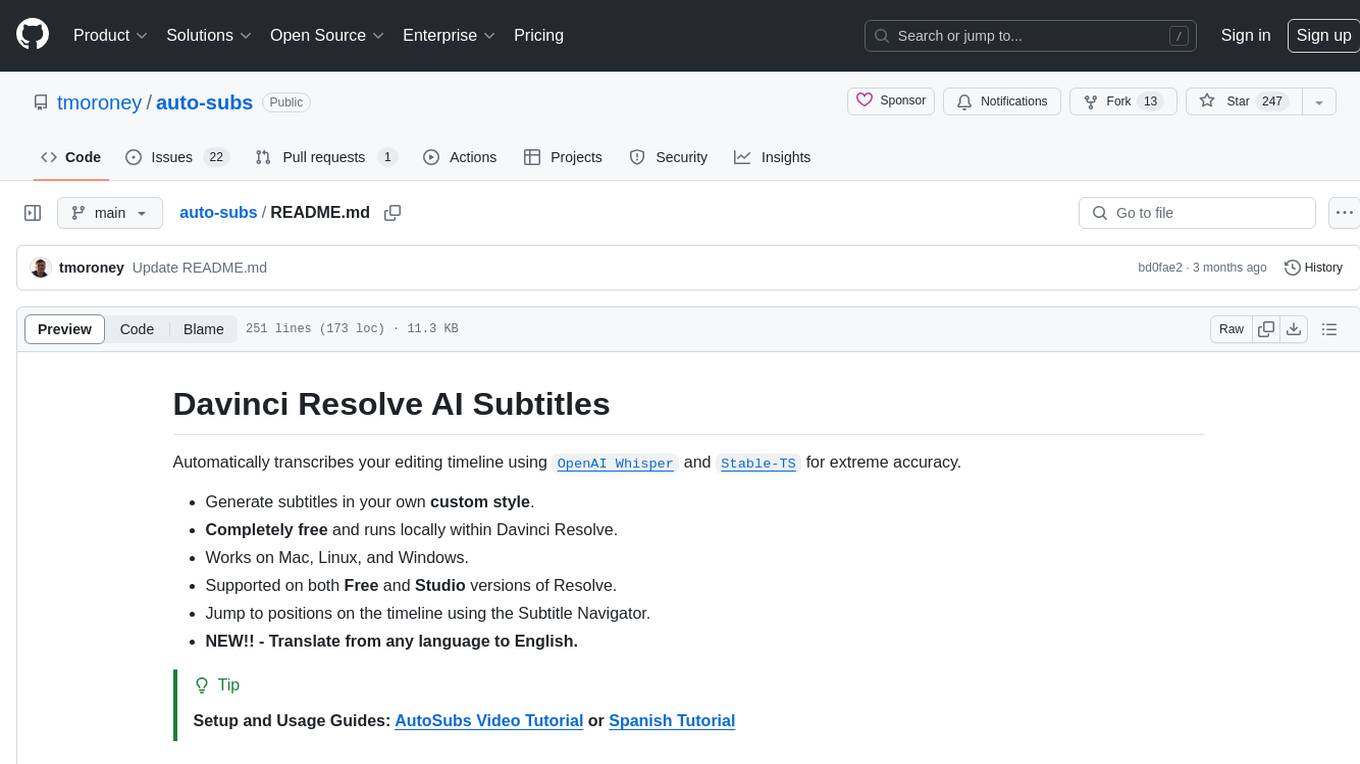
auto-subs
Auto-subs is a tool designed to automatically transcribe editing timelines using OpenAI Whisper and Stable-TS for extreme accuracy. It generates subtitles in a custom style, is completely free, and runs locally within Davinci Resolve. It works on Mac, Linux, and Windows, supporting both Free and Studio versions of Resolve. Users can jump to positions on the timeline using the Subtitle Navigator and translate from any language to English. The tool provides a user-friendly interface for creating and customizing subtitles for video content.
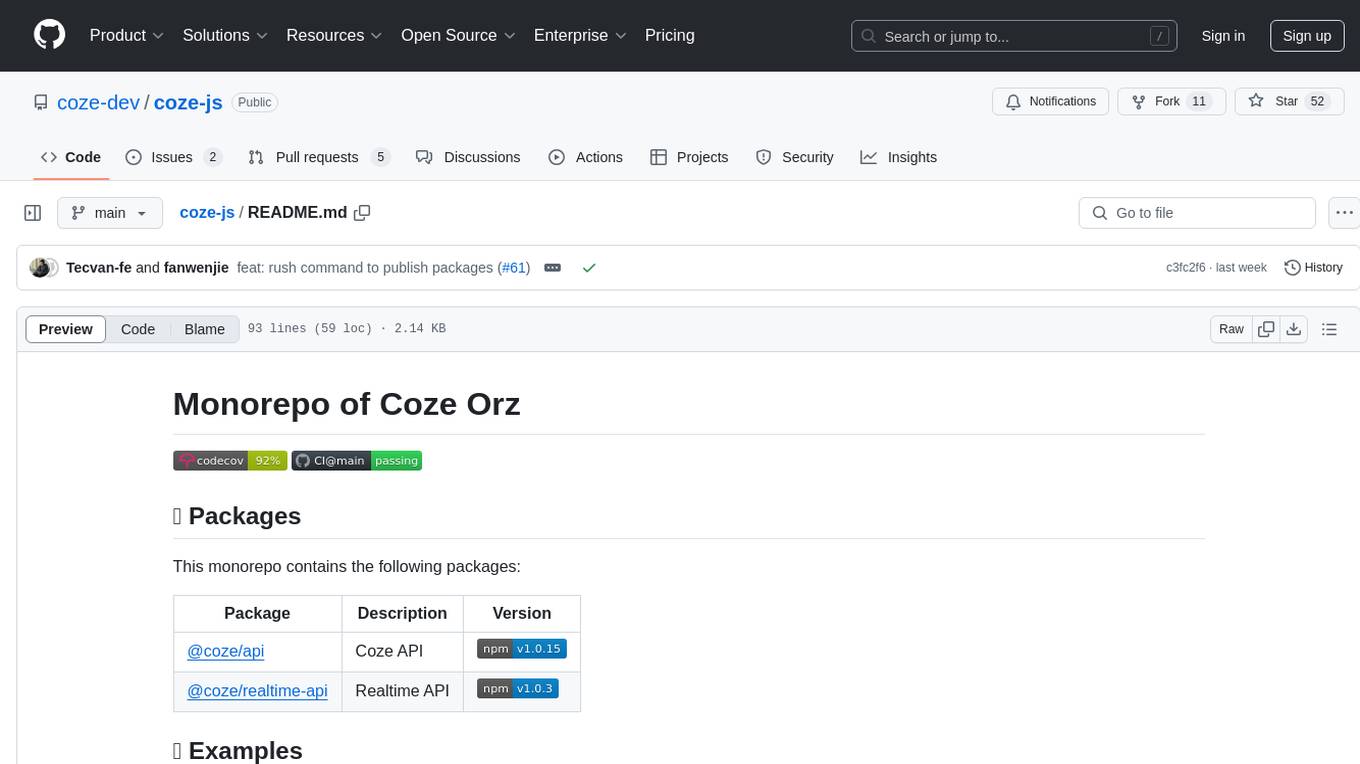
coze-js
Coze-js is a monorepo containing packages for Coze API and Realtime API. It provides usage examples for Node.js and React Web, as well as full console and sample call up demos. The tool requires Node.js 18+, pnpm 9.12.0, and Rush 5.140.0 for installation. Developers can start developing projects within the repository by following the provided steps. Each package in the monorepo can be developed and published independently, with documentation on contributing guidelines and publishing. The tool is licensed under MIT.
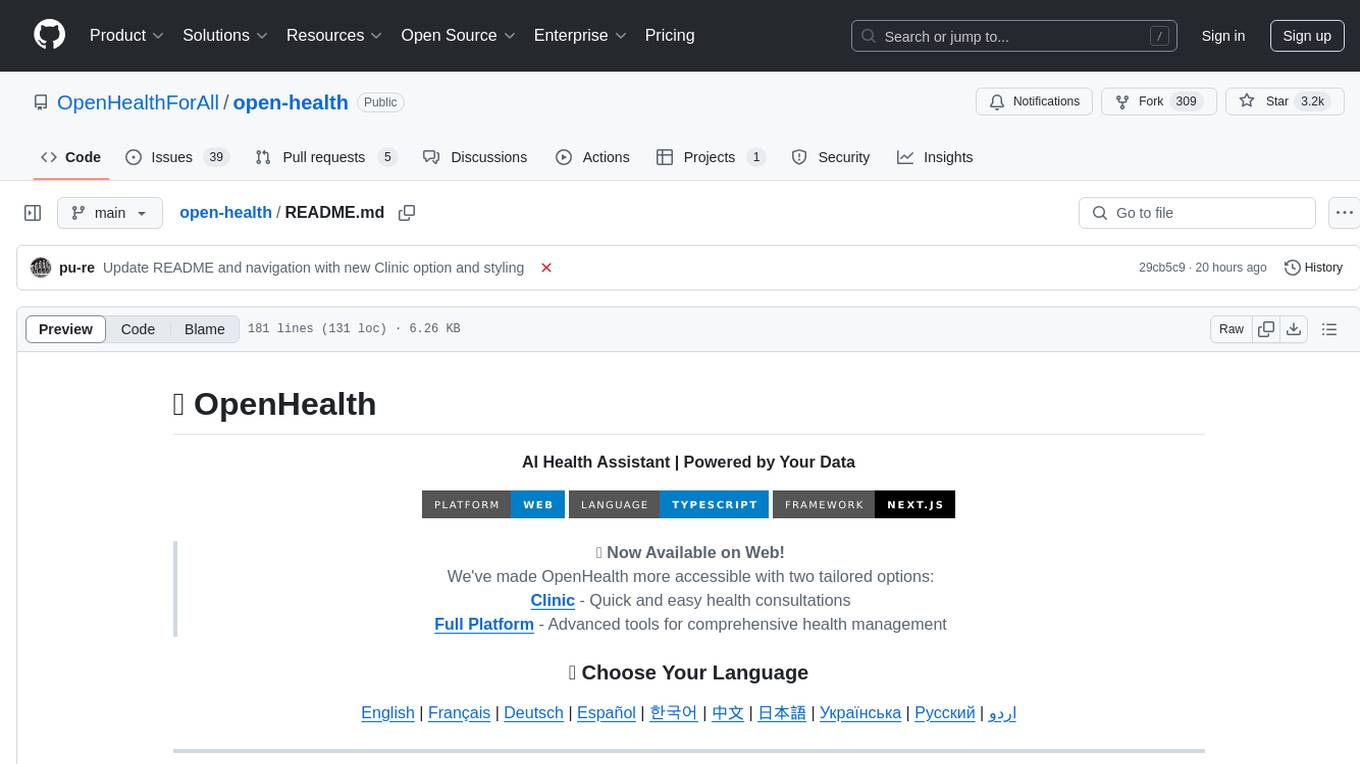
open-health
OpenHealth is an AI health assistant that helps users manage their health data by leveraging AI and personal health information. It allows users to consolidate health data, parse it smartly, and engage in contextual conversations with GPT-powered AI. The tool supports various data sources like blood test results, health checkup data, personal physical information, family history, and symptoms. OpenHealth aims to empower users to take control of their health by combining data and intelligence for actionable health management.
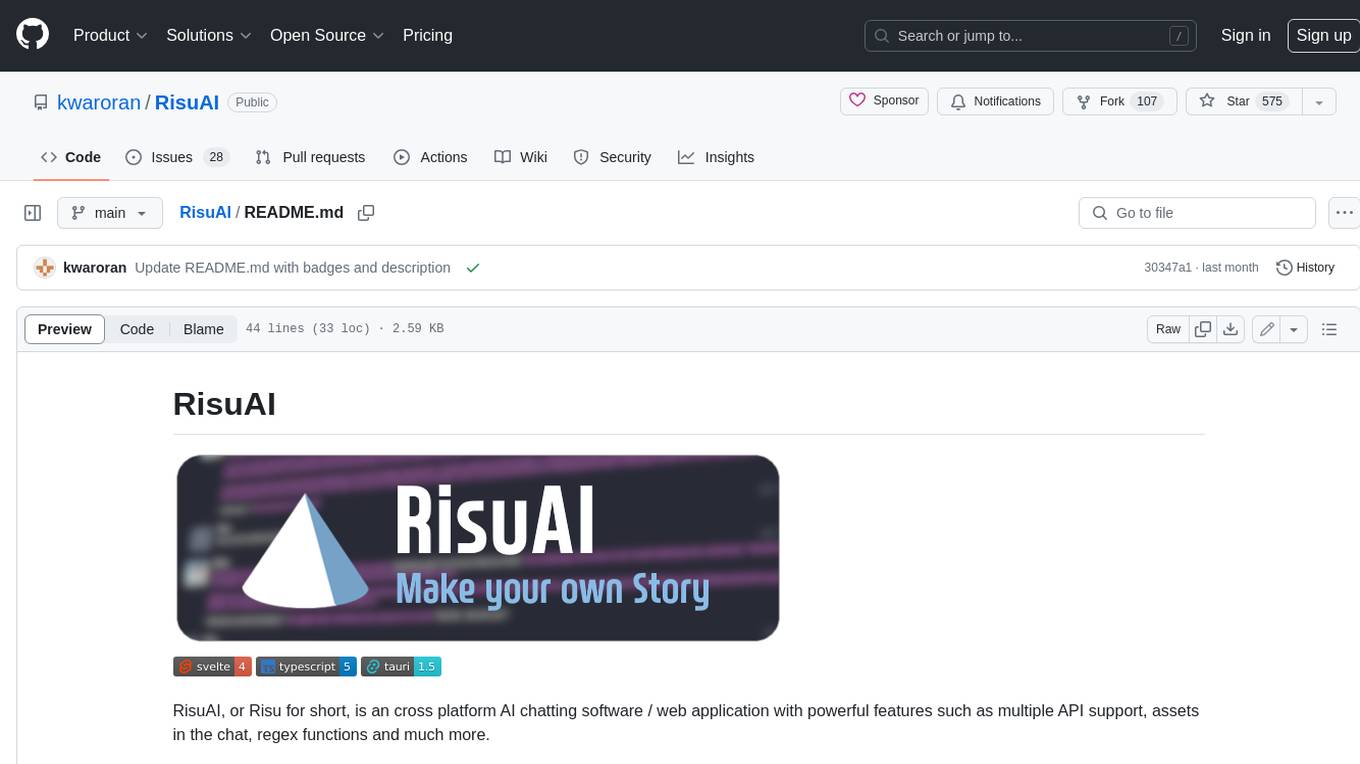
RisuAI
RisuAI, or Risu for short, is a cross-platform AI chatting software/web application with powerful features such as multiple API support, assets in the chat, regex functions, and much more.
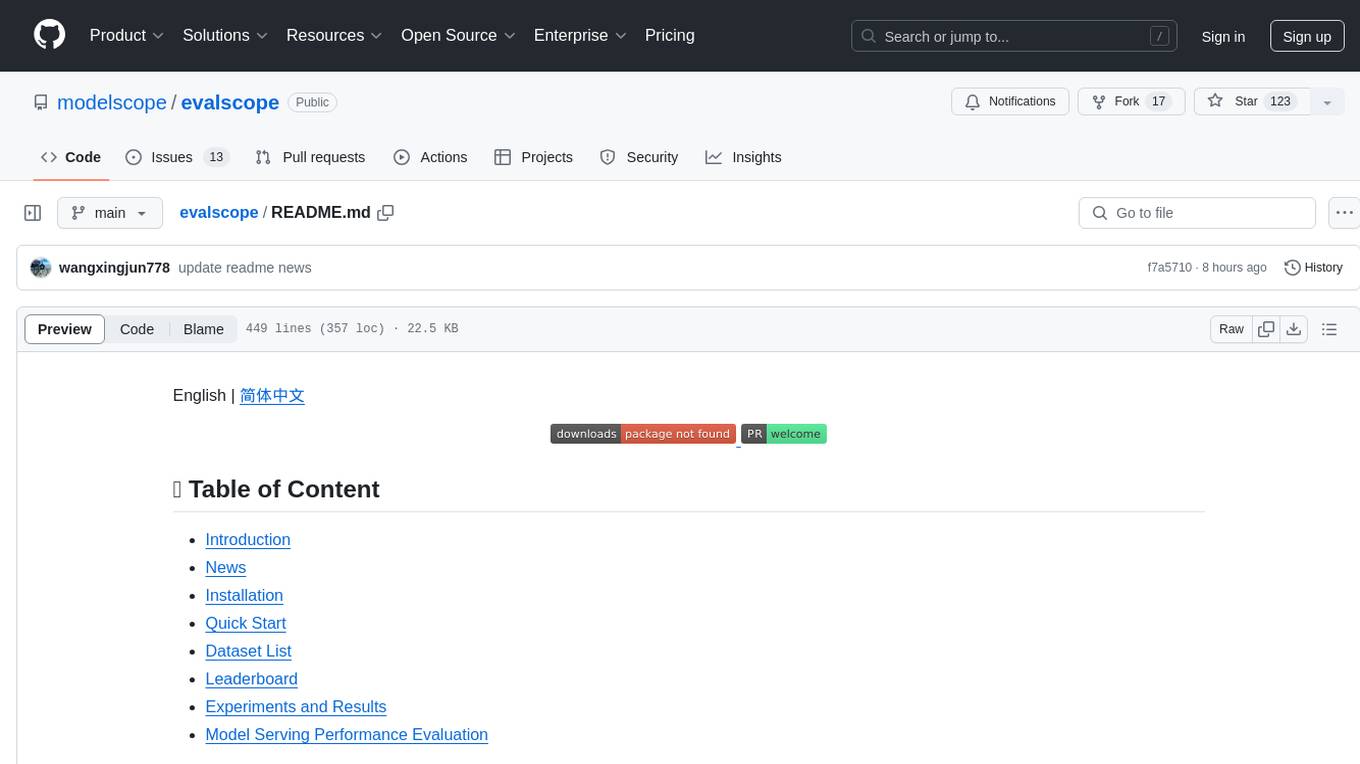
evalscope
Eval-Scope is a framework designed to support the evaluation of large language models (LLMs) by providing pre-configured benchmark datasets, common evaluation metrics, model integration, automatic evaluation for objective questions, complex task evaluation using expert models, reports generation, visualization tools, and model inference performance evaluation. It is lightweight, easy to customize, supports new dataset integration, model hosting on ModelScope, deployment of locally hosted models, and rich evaluation metrics. Eval-Scope also supports various evaluation modes like single mode, pairwise-baseline mode, and pairwise (all) mode, making it suitable for assessing and improving LLMs.
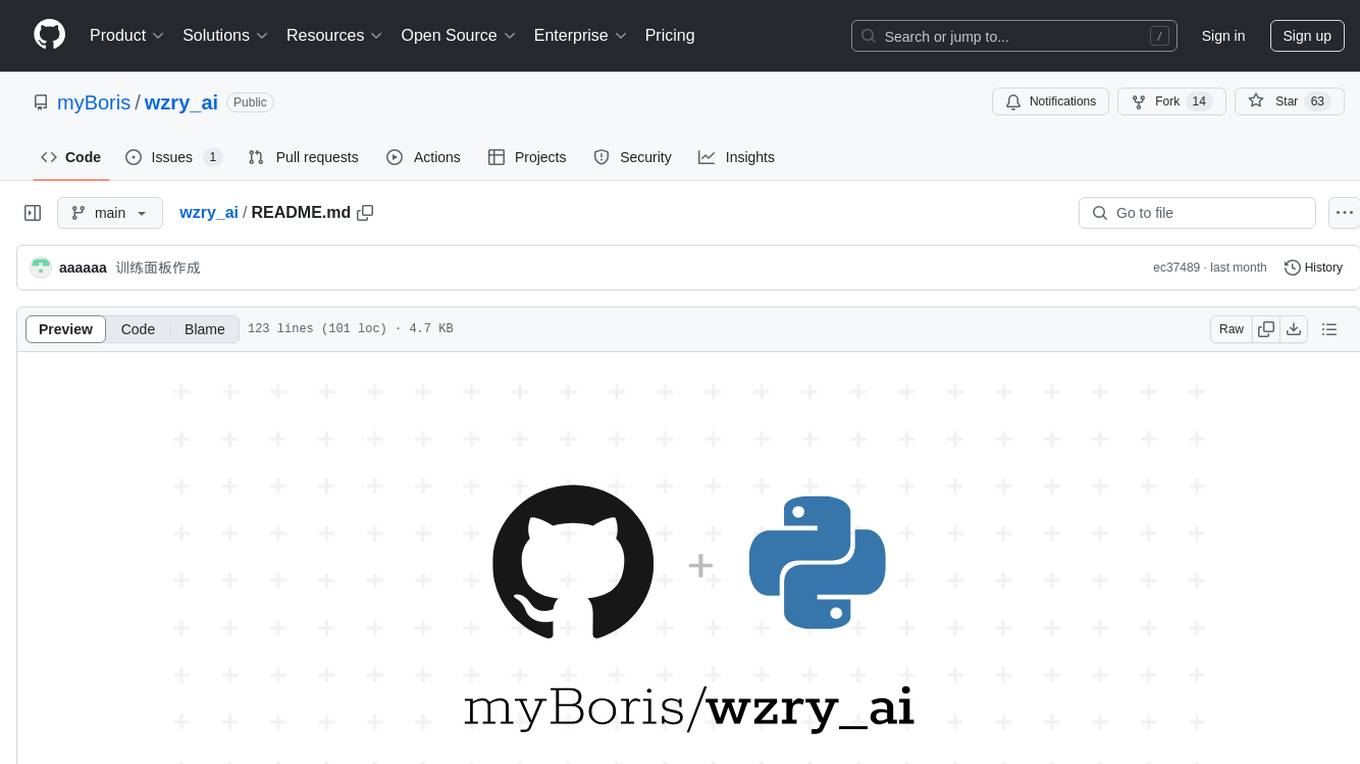
wzry_ai
This is an open-source project for playing the game King of Glory with an artificial intelligence model. The first phase of the project has been completed, and future upgrades will be built upon this foundation. The second phase of the project has started, and progress is expected to proceed according to plan. For any questions, feel free to join the QQ exchange group: 687853827. The project aims to learn artificial intelligence and strictly prohibits cheating. Detailed installation instructions are available in the doc/README.md file. Environment installation video: (bilibili) Welcome to follow, like, tip, comment, and provide your suggestions.
For similar tasks
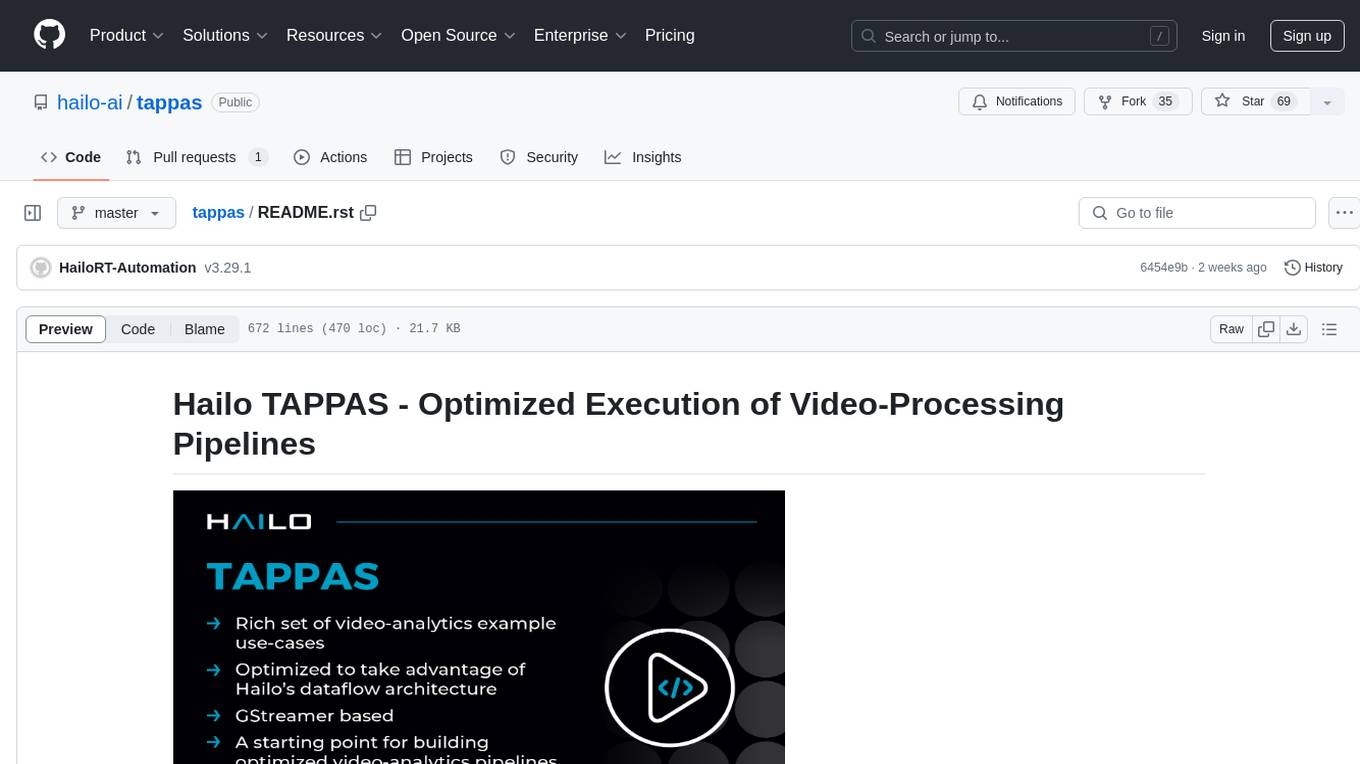
tappas
Hailo TAPPAS is a set of full application examples that implement pipeline elements and pre-trained AI tasks. It demonstrates Hailo's system integration scenarios on predefined systems, aiming to accelerate time to market, simplify integration with Hailo's runtime SW stack, and provide a starting point for customers to fine-tune their applications. The tool supports both Hailo-15 and Hailo-8, offering various example applications optimized for different common hosts. TAPPAS includes pipelines for single network, two network, and multi-stream processing, as well as high-resolution processing via tiling. It also provides example use case pipelines like License Plate Recognition and Multi-Person Multi-Camera Tracking. The tool is regularly updated with new features, bug fixes, and platform support.
For similar jobs
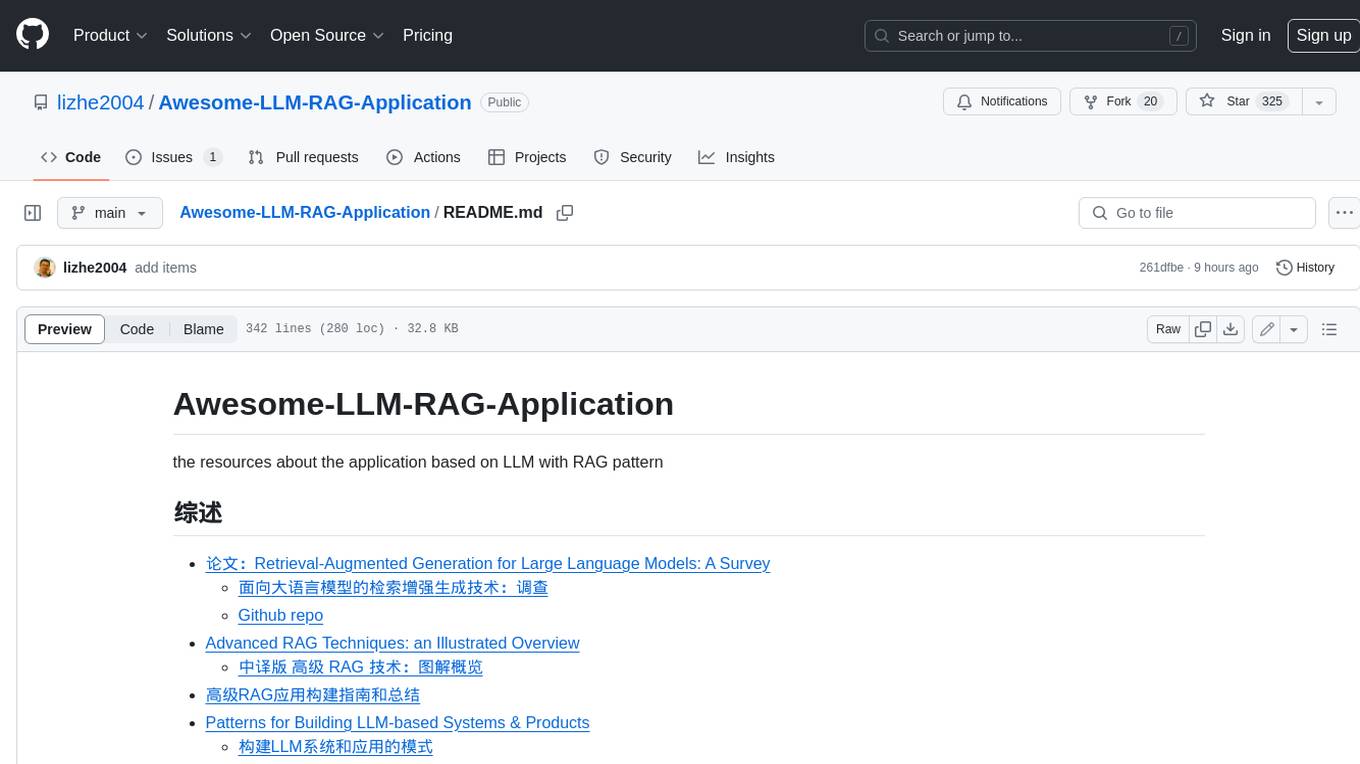
Awesome-LLM-RAG-Application
Awesome-LLM-RAG-Application is a repository that provides resources and information about applications based on Large Language Models (LLM) with Retrieval-Augmented Generation (RAG) pattern. It includes a survey paper, GitHub repo, and guides on advanced RAG techniques. The repository covers various aspects of RAG, including academic papers, evaluation benchmarks, downstream tasks, tools, and technologies. It also explores different frameworks, preprocessing tools, routing mechanisms, evaluation frameworks, embeddings, security guardrails, prompting tools, SQL enhancements, LLM deployment, observability tools, and more. The repository aims to offer comprehensive knowledge on RAG for readers interested in exploring and implementing LLM-based systems and products.
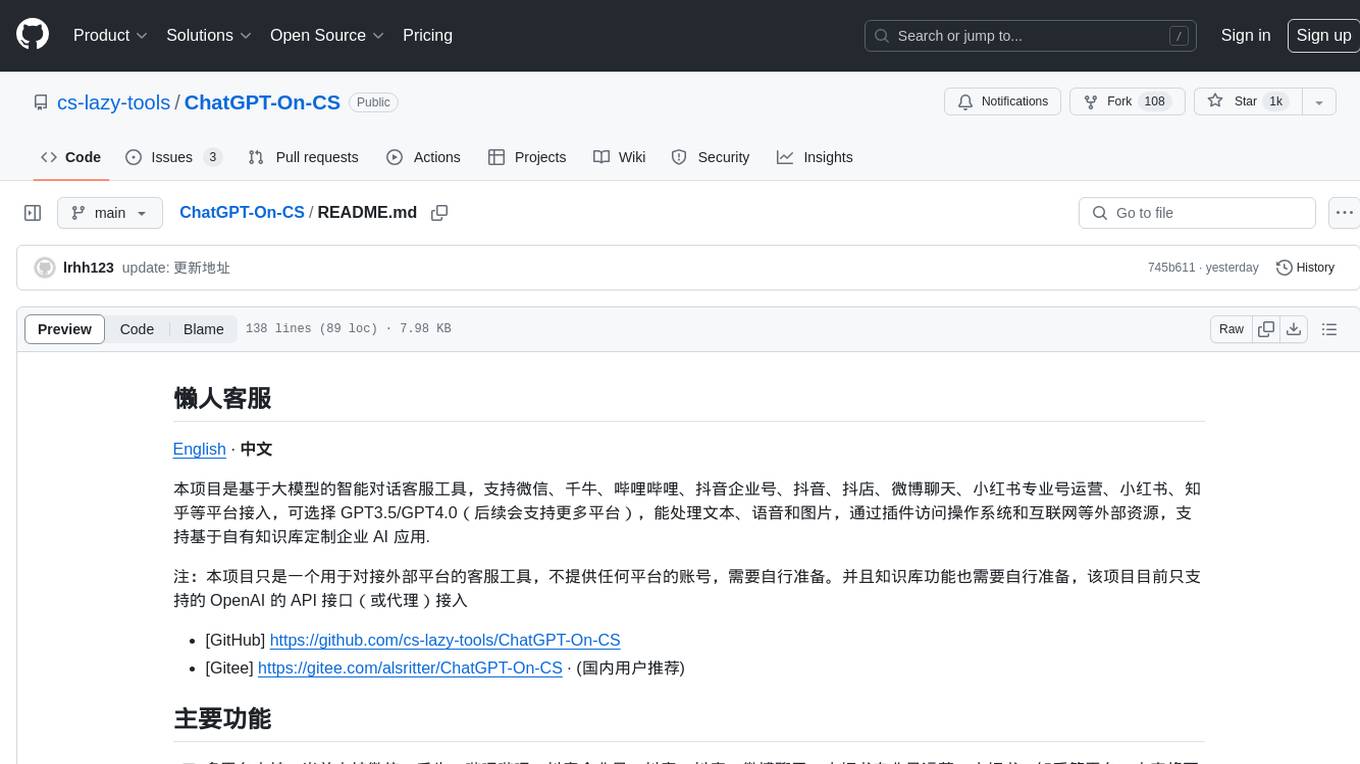
ChatGPT-On-CS
ChatGPT-On-CS is an intelligent chatbot tool based on large models, supporting various platforms like WeChat, Taobao, Bilibili, Douyin, Weibo, and more. It can handle text, voice, and image inputs, access external resources through plugins, and customize enterprise AI applications based on proprietary knowledge bases. Users can set custom replies, utilize ChatGPT interface for intelligent responses, send images and binary files, and create personalized chatbots using knowledge base files. The tool also features platform-specific plugin systems for accessing external resources and supports enterprise AI applications customization.
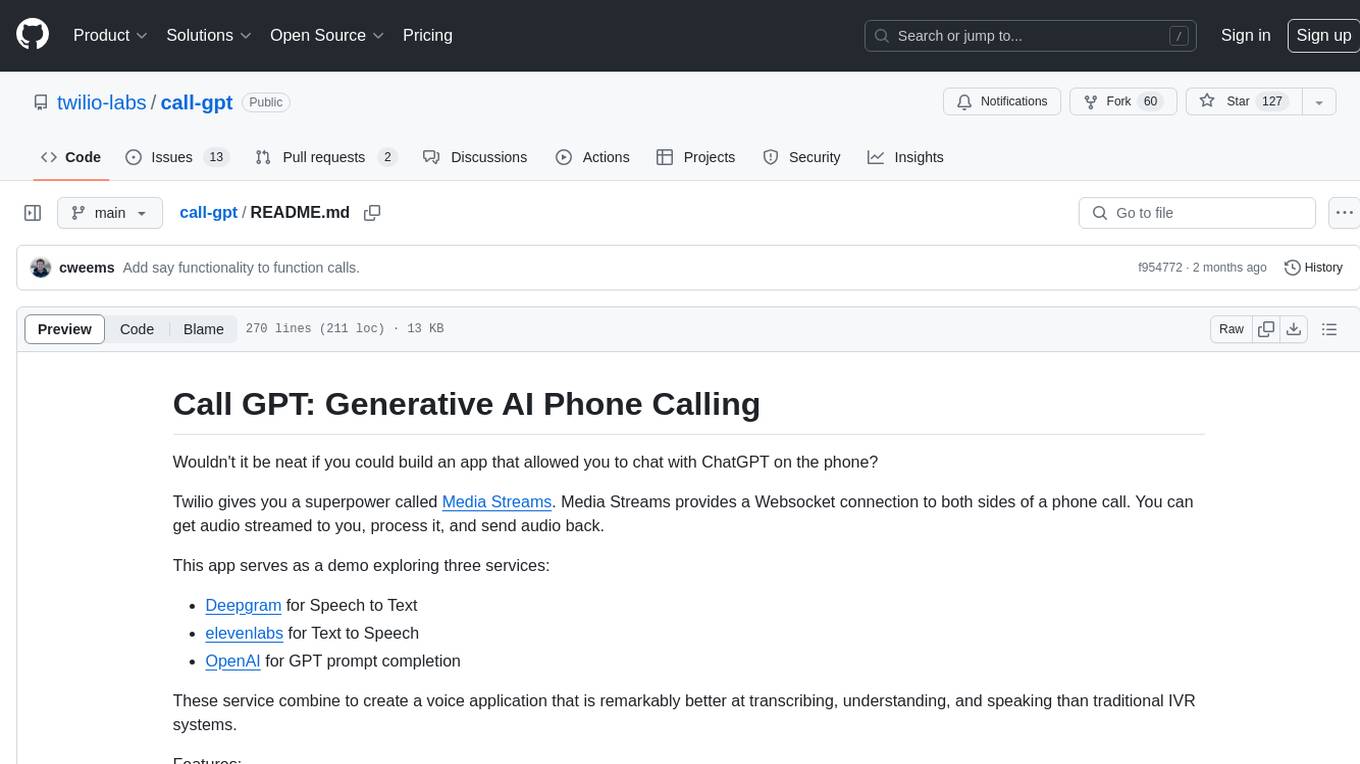
call-gpt
Call GPT is a voice application that utilizes Deepgram for Speech to Text, elevenlabs for Text to Speech, and OpenAI for GPT prompt completion. It allows users to chat with ChatGPT on the phone, providing better transcription, understanding, and speaking capabilities than traditional IVR systems. The app returns responses with low latency, allows user interruptions, maintains chat history, and enables GPT to call external tools. It coordinates data flow between Deepgram, OpenAI, ElevenLabs, and Twilio Media Streams, enhancing voice interactions.
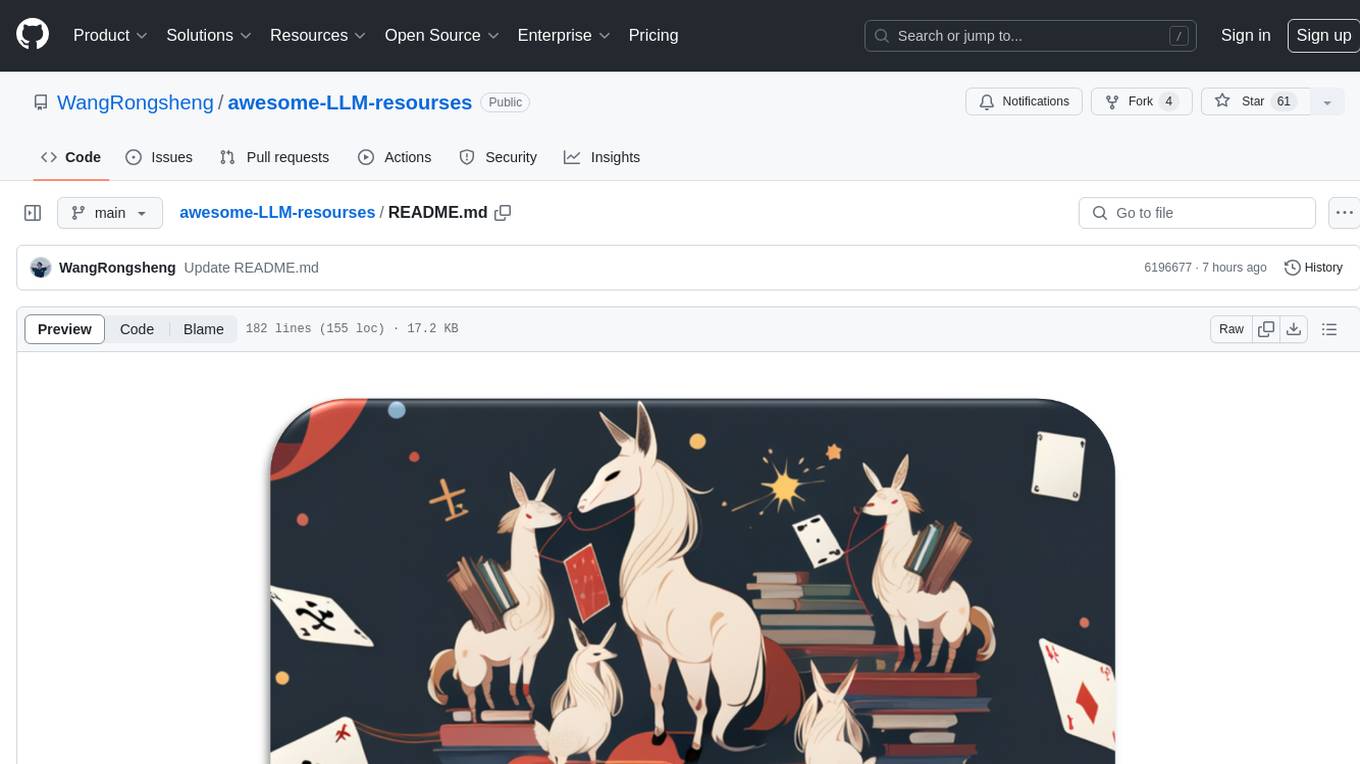
awesome-LLM-resourses
A comprehensive repository of resources for Chinese large language models (LLMs), including data processing tools, fine-tuning frameworks, inference libraries, evaluation platforms, RAG engines, agent frameworks, books, courses, tutorials, and tips. The repository covers a wide range of tools and resources for working with LLMs, from data labeling and processing to model fine-tuning, inference, evaluation, and application development. It also includes resources for learning about LLMs through books, courses, and tutorials, as well as insights and strategies from building with LLMs.
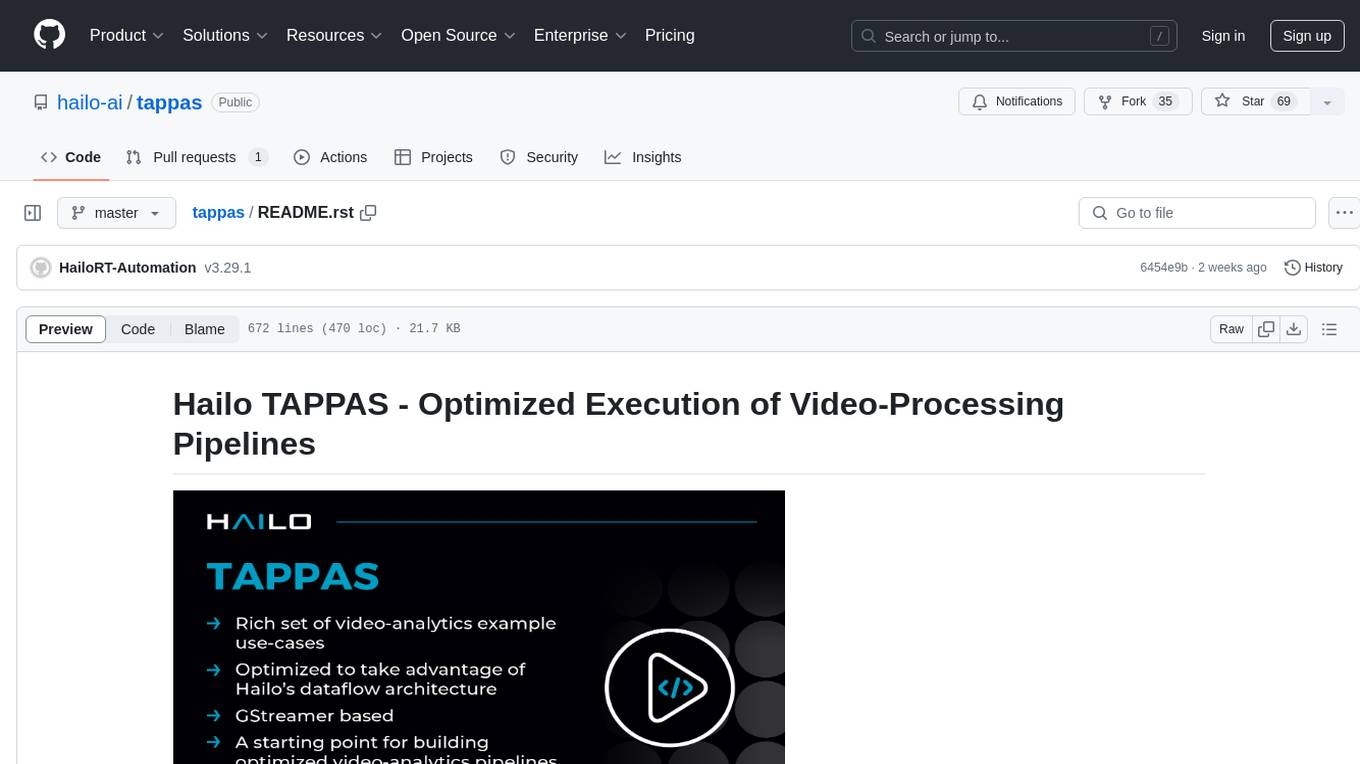
tappas
Hailo TAPPAS is a set of full application examples that implement pipeline elements and pre-trained AI tasks. It demonstrates Hailo's system integration scenarios on predefined systems, aiming to accelerate time to market, simplify integration with Hailo's runtime SW stack, and provide a starting point for customers to fine-tune their applications. The tool supports both Hailo-15 and Hailo-8, offering various example applications optimized for different common hosts. TAPPAS includes pipelines for single network, two network, and multi-stream processing, as well as high-resolution processing via tiling. It also provides example use case pipelines like License Plate Recognition and Multi-Person Multi-Camera Tracking. The tool is regularly updated with new features, bug fixes, and platform support.
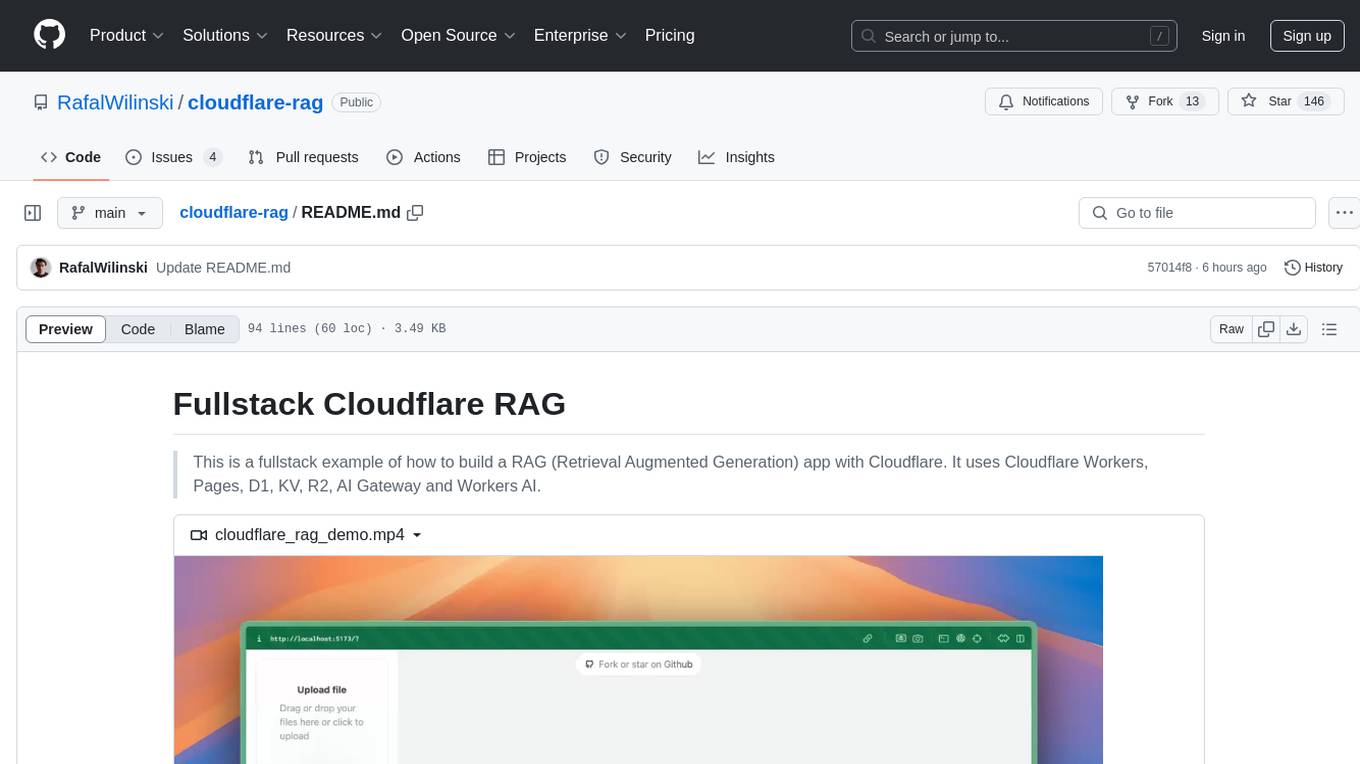
cloudflare-rag
This repository provides a fullstack example of building a Retrieval Augmented Generation (RAG) app with Cloudflare. It utilizes Cloudflare Workers, Pages, D1, KV, R2, AI Gateway, and Workers AI. The app features streaming interactions to the UI, hybrid RAG with Full-Text Search and Vector Search, switchable providers using AI Gateway, per-IP rate limiting with Cloudflare's KV, OCR within Cloudflare Worker, and Smart Placement for workload optimization. The development setup requires Node, pnpm, and wrangler CLI, along with setting up necessary primitives and API keys. Deployment involves setting up secrets and deploying the app to Cloudflare Pages. The project implements a Hybrid Search RAG approach combining Full Text Search against D1 and Hybrid Search with embeddings against Vectorize to enhance context for the LLM.
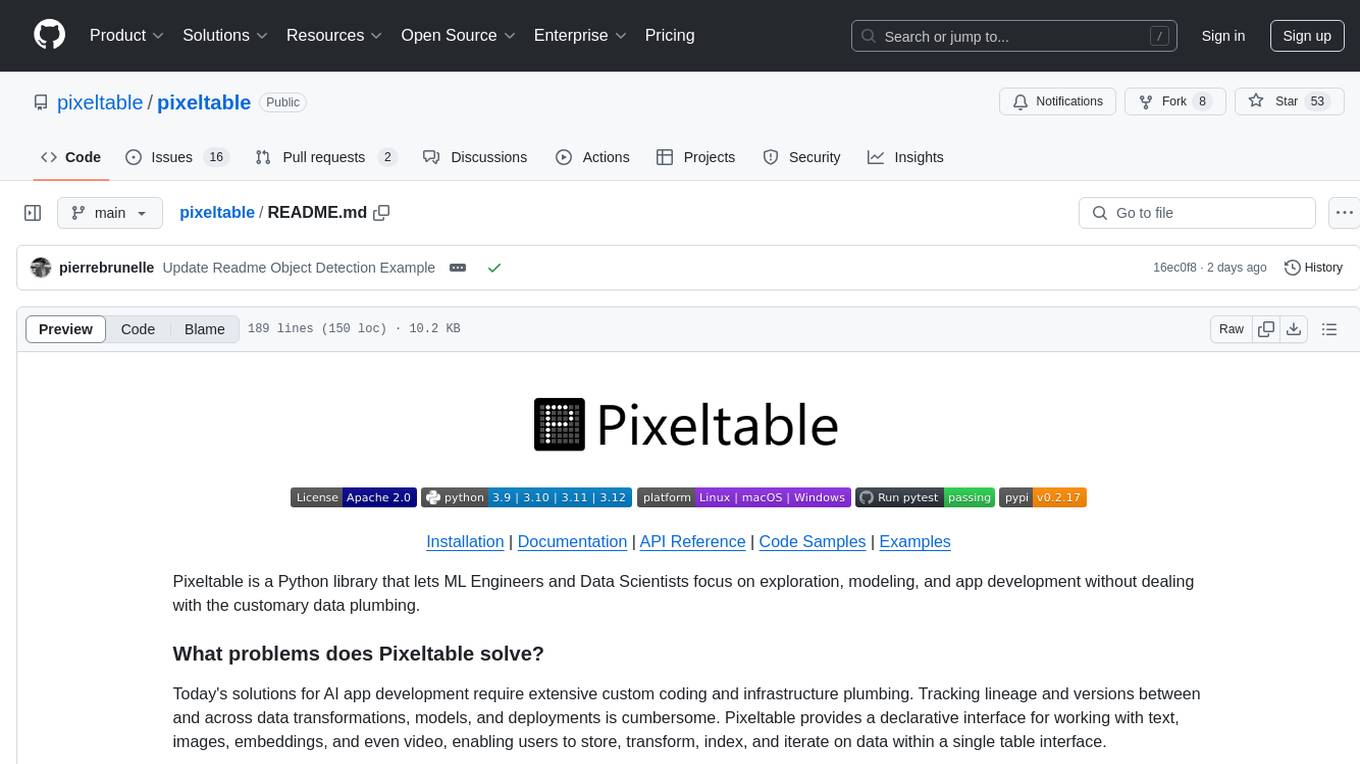
pixeltable
Pixeltable is a Python library designed for ML Engineers and Data Scientists to focus on exploration, modeling, and app development without the need to handle data plumbing. It provides a declarative interface for working with text, images, embeddings, and video, enabling users to store, transform, index, and iterate on data within a single table interface. Pixeltable is persistent, acting as a database unlike in-memory Python libraries such as Pandas. It offers features like data storage and versioning, combined data and model lineage, indexing, orchestration of multimodal workloads, incremental updates, and automatic production-ready code generation. The tool emphasizes transparency, reproducibility, cost-saving through incremental data changes, and seamless integration with existing Python code and libraries.
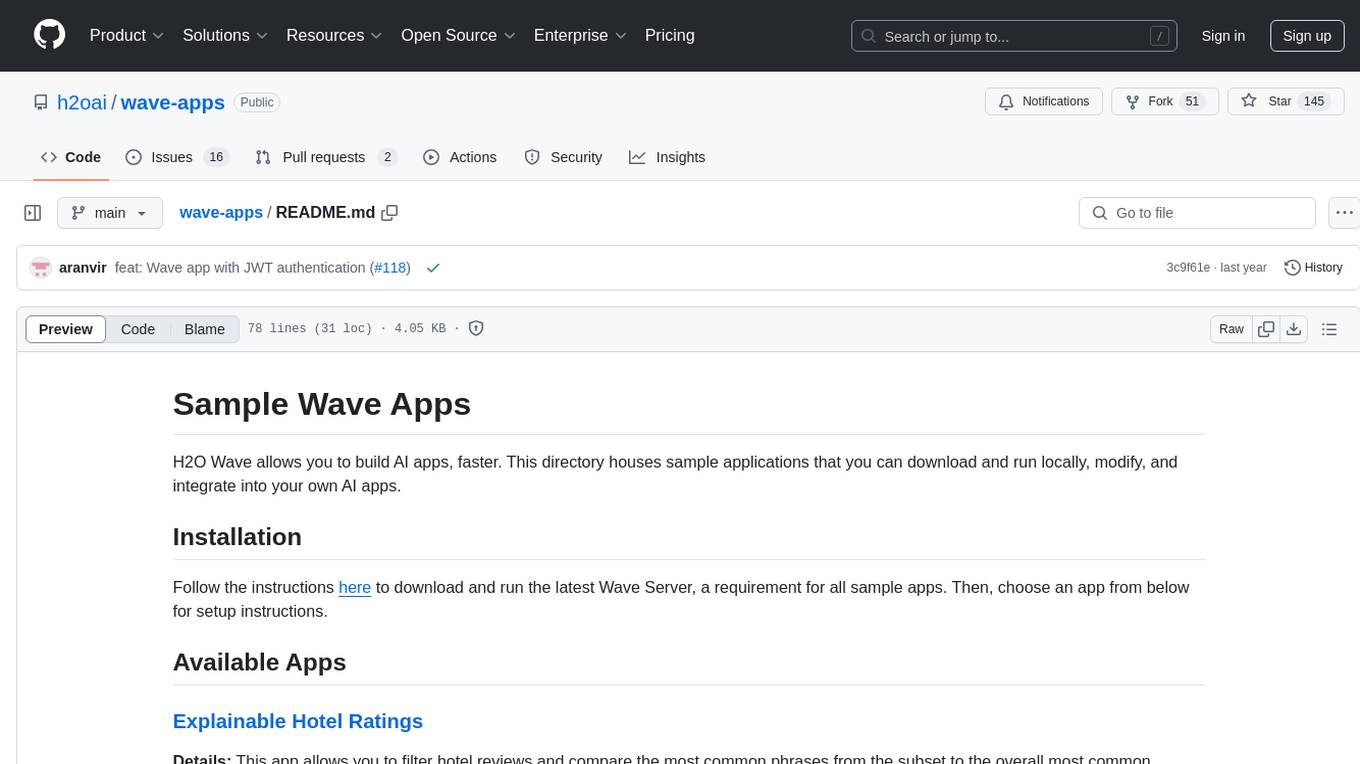
wave-apps
Wave Apps is a directory of sample applications built on H2O Wave, allowing users to build AI apps faster. The apps cover various use cases such as explainable hotel ratings, human-in-the-loop credit risk assessment, mitigating churn risk, online shopping recommendations, and sales forecasting EDA. Users can download, modify, and integrate these sample apps into their own projects to learn about app development and AI model deployment.