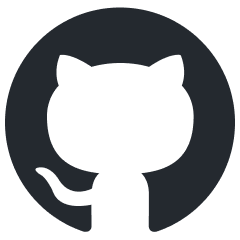
Co-LLM-Agents
[ICLR 2024] Source codes for the paper "Building Cooperative Embodied Agents Modularly with Large Language Models"
Stars: 245
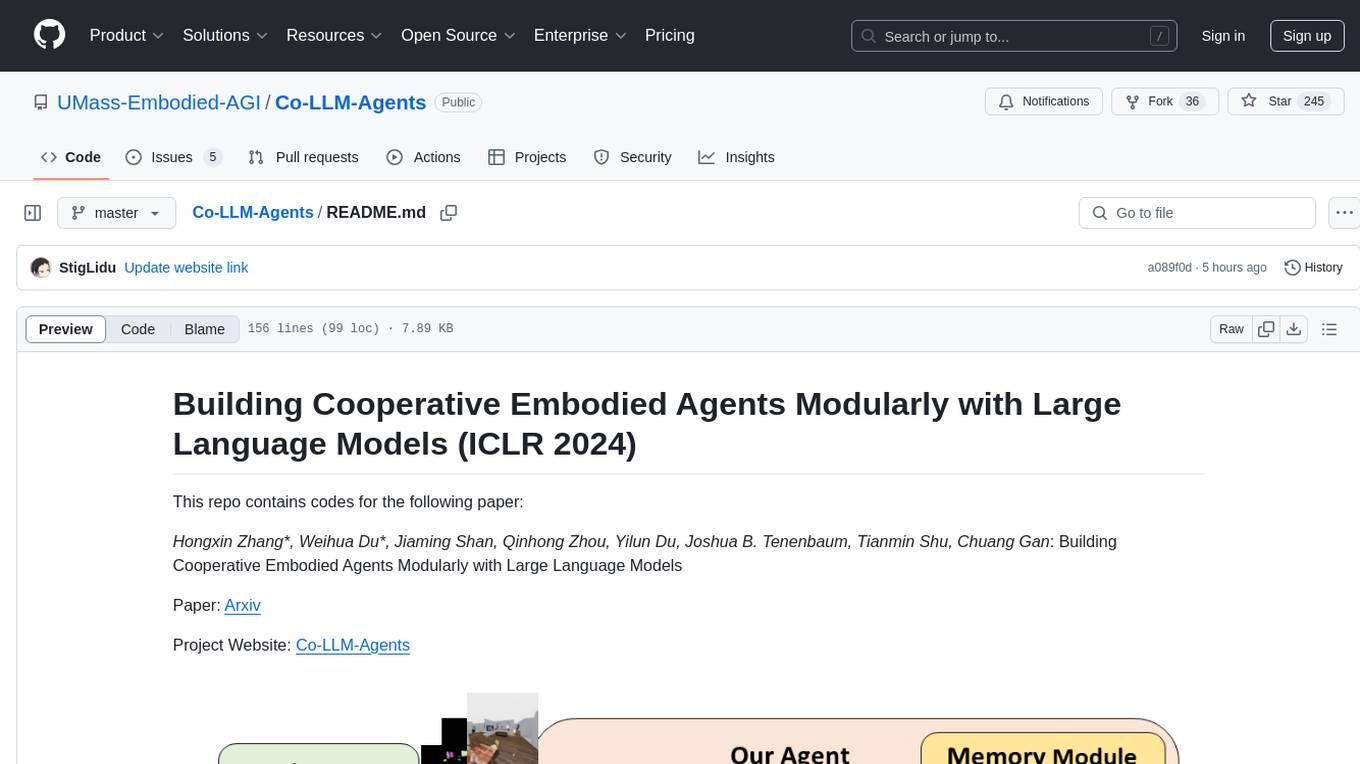
Co-LLM-Agents is a repository containing codes for the paper 'Building Cooperative Embodied Agents Modularly with Large Language Models'. The project focuses on developing cooperative embodied agents using large language models, with a specific emphasis on the ThreeDWorld Multi-Agent Transport environment. The repository provides implementations, installation instructions, and example scripts for running experiments with the CoELA model. It extends the ThreeDWorld Transport Challenge into a multi-agent setting, enabling agents to transport target objects using containers and communicate with each other. Additionally, it includes the Communicative Watch-And-Help challenge, where agents can send messages to each other while performing tasks such as preparing meals, washing dishes, and setting up dinner tables.
README:
This repo contains codes for the following paper:
Hongxin Zhang*, Weihua Du*, Jiaming Shan, Qinhong Zhou, Yilun Du, Joshua B. Tenenbaum, Tianmin Shu, Chuang Gan: Building Cooperative Embodied Agents Modularly with Large Language Models
Paper: Arxiv
Project Website: Co-LLM-Agents
[8/25/2024]: Updates on the navigation module of agents on the ThreeDWorld Multi-Agent Transport
environment to fix the navigation issues.
[9/4/2023]: ThreeDWorld Multi-Agent Transport
no longer provides ground truth segmentation mask in default. We implement a vision detection module with a fine-tuned Mask-RCNN model. For more details, please read README in tdw_mat.
[8/1/2023]: We provide the VirtualHome Simulator executable we used here. If you met XDG_RUNTIME_DIR not set in the environment
error previously, please check if you are using the new version we provided.
For detailed instructions on the installation of the two embodied multi-agent environments Communicative Watch-And-Help
and ThreeDWorld Multi-Agent Transport
, please refer to the Setup sections in cwah/README.md
and tdw_mat/README.md
respectively.
Run the following commands step by step to set up the environments:
cd tdw_mat
conda create -n tdw_mat python=3.9
conda activate tdw_mat
pip install -e .
If you're running TDW on a remote Linux server, follow the TDW Installation Document to configure the X server.
After that, you can run the demo scene to verify your setup:
python demo/demo_scene.py
Step 1: Get the VirtualHome Simulator and API and put it at the same level as the cwah
folder.
Clone the VirtualHome API repository:
git clone --branch wah https://github.com/xavierpuigf/virtualhome.git
Download the Simulator (Linux x86-64 version), and unzip it.
gdown https://drive.google.com/uc?id=1L79SxE07Jt-8-_uCvNnkwz5Kf6AjtaGp
unzip executable.zip
chmod +x executable/linux_exec.v2.3.0.x86_64
The files should be organized as follows:
|--cwah/
|--virtualhome/
|--executable/
Step 2: Install Requirements
cd cwah
conda create --name cwah python=3.8
conda activate cwah
pip install -r requirements.txt
The main implementation code of our CoELA is in tdw_mat/LLM
and tdw_mat/tdw_gym/lm_agent.py
.
We also prepare example scripts to run experiments with HP baseline and our CoELA under the folder tdw_mat/scripts
.
For example, to run experiments with two CoELA on ThreeDWorld Multi-Agent Transport
, run the following command in folder tdw_mat
.
./scripts/test_LMs-gpt-4.sh
We extend the ThreeDWorld Transport Challenge into a multi-agent setting with more types of objects and containers, more realistic object placements, and support communication between agents, named ThreeDWorld Multi-Agent Transport (TDW-MAT), built on top of the TDW platform.
The agents are tasked to transport as many target objects as possible to the goal position with the help of containers as tools. One container can carry most three objects, and without containers, the agent can transport only two objects at a time. The agents have the ego-centric visual observation and action space as before with a new communication action added.
We selected $6$ scenes from the TDW-House dataset and sampled $2$ types of tasks and $2$ settings in each of the scenes, making a test set of $24$ episodes. Every scene has $6$ to $8$ rooms, $10$ objects, and a few containers. An episode is terminated if all the target objects have been transported to the goal position or the maximum number of frames ($3000$) is reached.
The tasks are named food task
and stuff task
. Containers for the food task
can be found in both the kitchen and living room, while containers for the stuff task
can be found in the living room and office.
The configuration and distribution of containers vary based on two distinct settings: the Enough Container Setting
and the Rare Container Setting
. In the Enough Container Setting
, the ratio of containers to objects stands at $1:2$, and containers associated with a specific task are located in no more than two rooms. On the other hand, in the Rare Container Setting
, the container-to-object ratio decreases to $1:5$. This distribution differs from the "Enough Container Setting" as containers in the Rare Container Setting
are strictly localized to a single room.
One example of scenes, target objects, and containers is shown in the following image:
- Transport Rate (TR): The fraction of the target objects successfully transported to the goal position.
- Efficiency Improvements (EI): The efficiency improvements of cooperating with base agents.
Communicative Watch-And-Help(C-WAH) is an extension of the Watch-And-Help challenge, which enables agents to send messages to each other. Sending messages, alongside other actions, takes one timestep and has an upper limit on message length.
Five types of tasks are available in C-WAH, named Prepare afternoon tea
, Wash dishes
, Prepare a meal
, Put groceries
, and Set up a dinner table
. These tasks include a range of housework, and each task contains a few subgoals, which are described by predicates. A predicate is in ON/IN(x, y)
format, that is, Put x ON/IN y
. The detailed descriptions of tasks are listed in the following table:
Task Name | Predicate Set |
---|---|
Prepare afternoon tea | ON(cupcake,coffeetable), ON(pudding,coffeetable), ON(apple,coffeetable), ON(juice,coffeetable), ON(wine,coffeetable) |
Wash dishes | IN(plate,dishwasher), IN(fork,dishwasher) |
Prepare a meal | ON(coffeepot,dinnertable),ON(cupcake,dinnertable), ON(pancake,dinnertable), ON(poundcake,dinnertable), ON(pudding,dinnertable), ON(apple,dinnertable), ON(juice,dinnertable), ON(wine,dinnertable) |
Put groceries | IN(cupcake,fridge), IN(pancake,fridge), IN(poundcake,fridge), IN(pudding,fridge), IN(apple,fridge), IN(juice,fridge), IN(wine,fridge) |
Set up a dinner table | ON(plate,dinnertable), ON(fork,dinnertable) |
The task goal is to satisfy all the given subgoals within $250$ time steps, and the number of subgoals in each task ranges from $3$ to $5$.
- Average Steps (L): Number of steps to finish the task;
- Efficiency Improvement (EI): The efficiency improvements of cooperating with base agents.
We noticed many interesting agents' behaviors exhibited in our experiments and identified several cooperative behaviors.
There are more interesting cases and demos on our website!
If you find our work useful, please consider citing:
@article{zhang2024building,
title={Building Cooperative Embodied Agents Modularly with Large Language Models},
author={Zhang, Hongxin and Du, Weihua and Shan, Jiaming and Zhou, Qinhong and Du, Yilun and Tenenbaum, Joshua B and Shu, Tianmin and Gan, Chuang},
journal={ICLR},
year={2024}
}
For Tasks:
Click tags to check more tools for each tasksFor Jobs:
Alternative AI tools for Co-LLM-Agents
Similar Open Source Tools
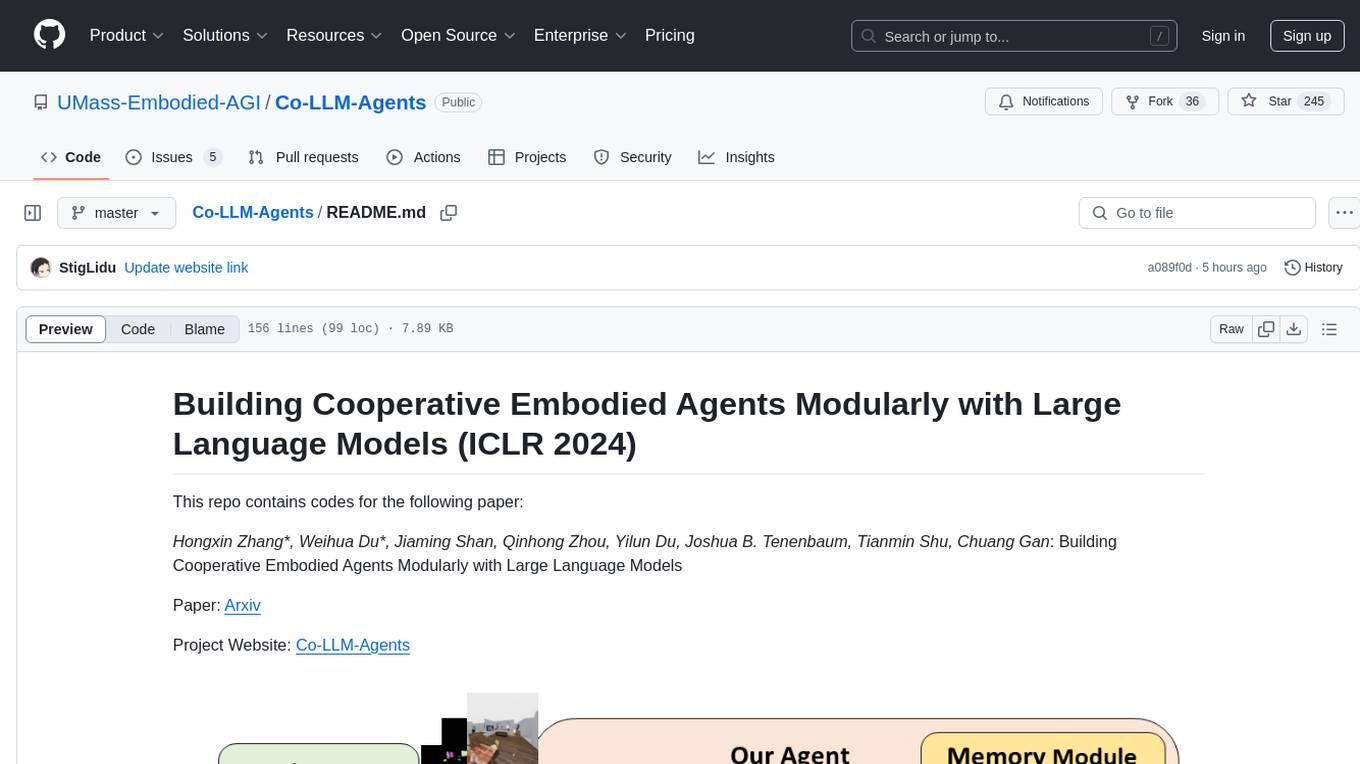
Co-LLM-Agents
Co-LLM-Agents is a repository containing codes for the paper 'Building Cooperative Embodied Agents Modularly with Large Language Models'. The project focuses on developing cooperative embodied agents using large language models, with a specific emphasis on the ThreeDWorld Multi-Agent Transport environment. The repository provides implementations, installation instructions, and example scripts for running experiments with the CoELA model. It extends the ThreeDWorld Transport Challenge into a multi-agent setting, enabling agents to transport target objects using containers and communicate with each other. Additionally, it includes the Communicative Watch-And-Help challenge, where agents can send messages to each other while performing tasks such as preparing meals, washing dishes, and setting up dinner tables.
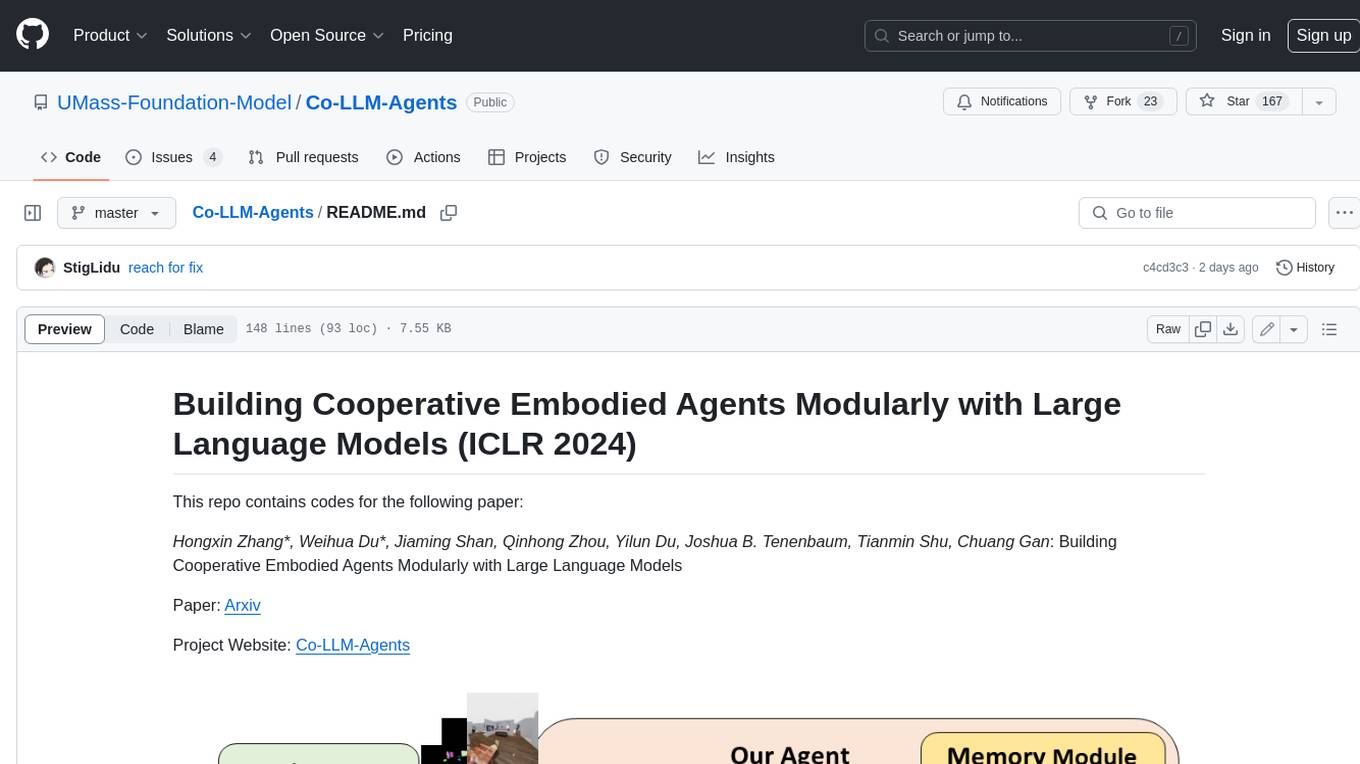
Co-LLM-Agents
This repository contains code for building cooperative embodied agents modularly with large language models. The agents are trained to perform tasks in two different environments: ThreeDWorld Multi-Agent Transport (TDW-MAT) and Communicative Watch-And-Help (C-WAH). TDW-MAT is a multi-agent environment where agents must transport objects to a goal position using containers. C-WAH is an extension of the Watch-And-Help challenge, which enables agents to send messages to each other. The code in this repository can be used to train agents to perform tasks in both of these environments.
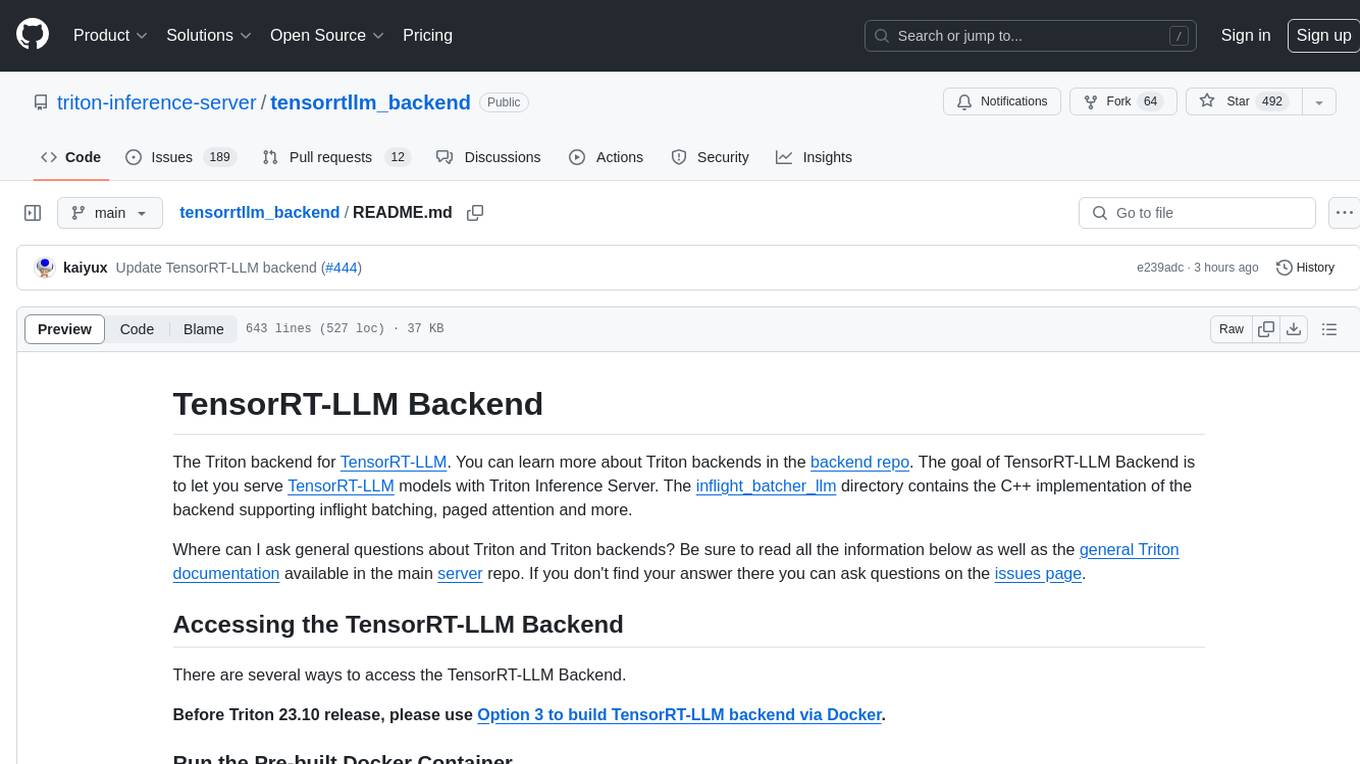
tensorrtllm_backend
The TensorRT-LLM Backend is a Triton backend designed to serve TensorRT-LLM models with Triton Inference Server. It supports features like inflight batching, paged attention, and more. Users can access the backend through pre-built Docker containers or build it using scripts provided in the repository. The backend can be used to create models for tasks like tokenizing, inferencing, de-tokenizing, ensemble modeling, and more. Users can interact with the backend using provided client scripts and query the server for metrics related to request handling, memory usage, KV cache blocks, and more. Testing for the backend can be done following the instructions in the 'ci/README.md' file.
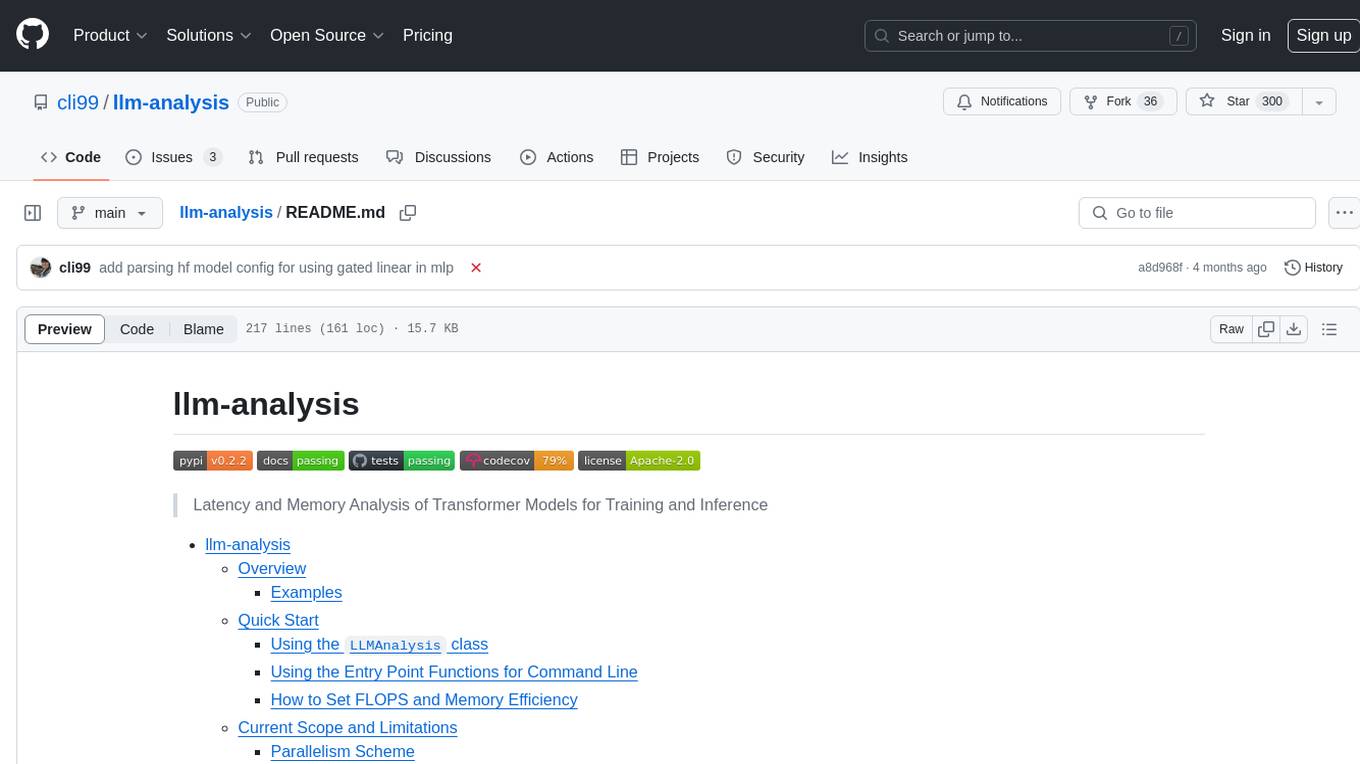
llm-analysis
llm-analysis is a tool designed for Latency and Memory Analysis of Transformer Models for Training and Inference. It automates the calculation of training or inference latency and memory usage for Large Language Models (LLMs) or Transformers based on specified model, GPU, data type, and parallelism configurations. The tool helps users to experiment with different setups theoretically, understand system performance, and optimize training/inference scenarios. It supports various parallelism schemes, communication methods, activation recomputation options, data types, and fine-tuning strategies. Users can integrate llm-analysis in their code using the `LLMAnalysis` class or use the provided entry point functions for command line interface. The tool provides lower-bound estimations of memory usage and latency, and aims to assist in achieving feasible and optimal setups for training or inference.
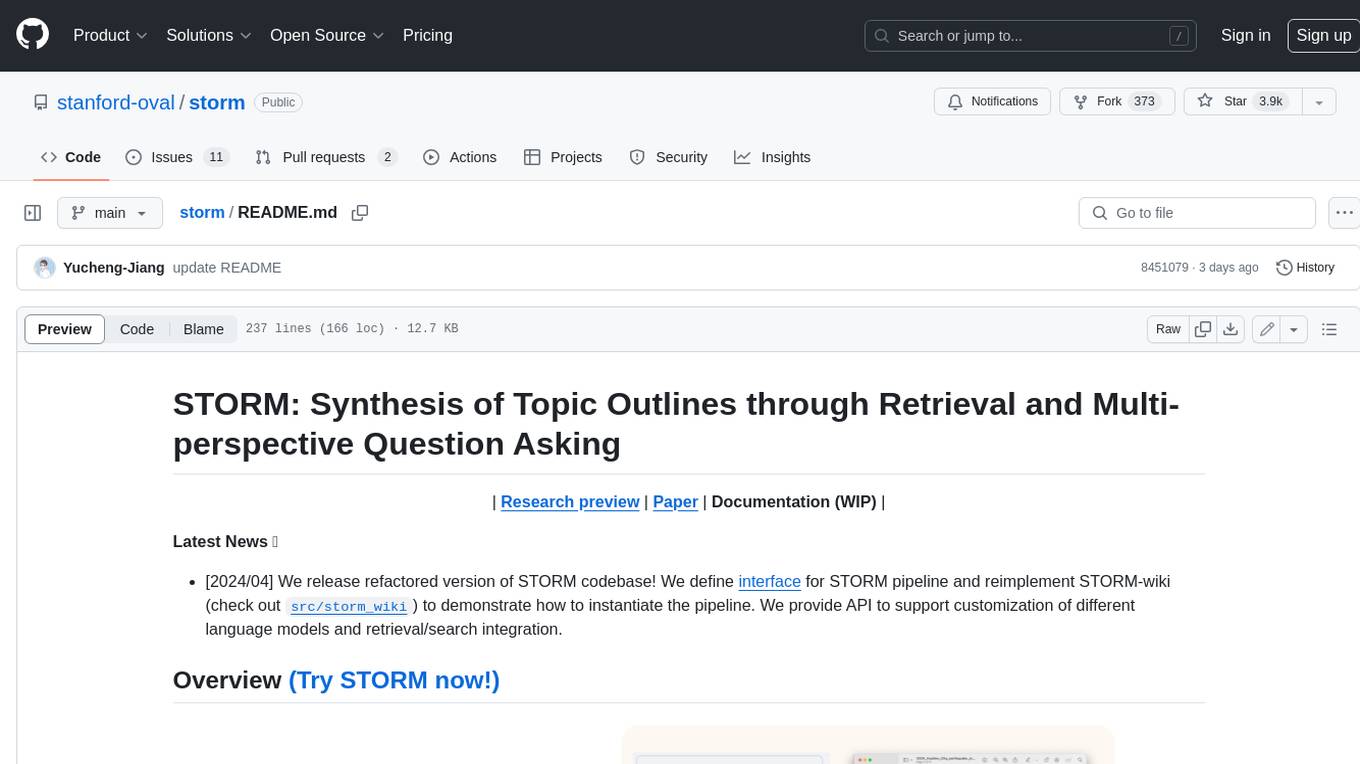
storm
STORM is a LLM system that writes Wikipedia-like articles from scratch based on Internet search. While the system cannot produce publication-ready articles that often require a significant number of edits, experienced Wikipedia editors have found it helpful in their pre-writing stage. **Try out our [live research preview](https://storm.genie.stanford.edu/) to see how STORM can help your knowledge exploration journey and please provide feedback to help us improve the system 🙏!**
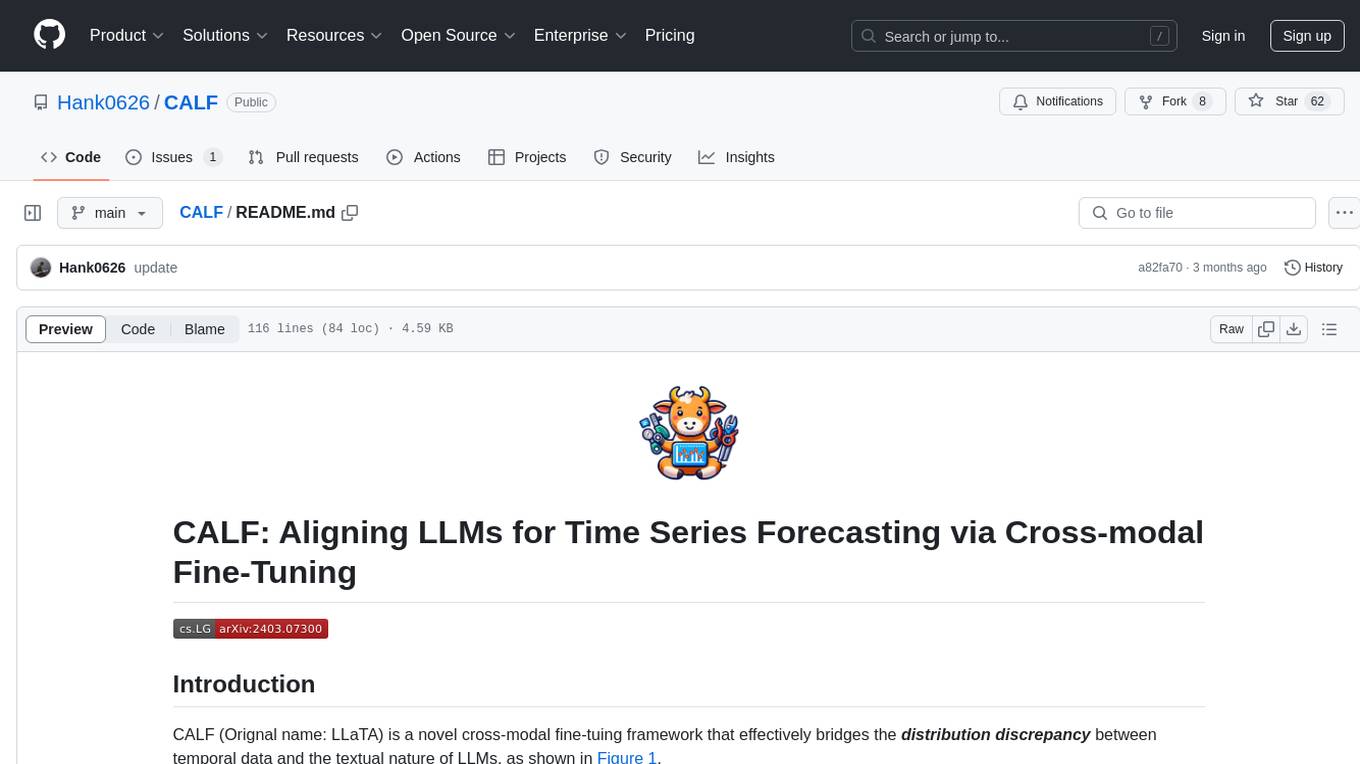
CALF
CALF (LLaTA) is a cross-modal fine-tuning framework that bridges the distribution discrepancy between temporal data and the textual nature of LLMs. It introduces three cross-modal fine-tuning techniques: Cross-Modal Match Module, Feature Regularization Loss, and Output Consistency Loss. The framework aligns time series and textual inputs, ensures effective weight updates, and maintains consistent semantic context for time series data. CALF provides scripts for long-term and short-term forecasting, requires Python 3.9, and utilizes word token embeddings for model training.
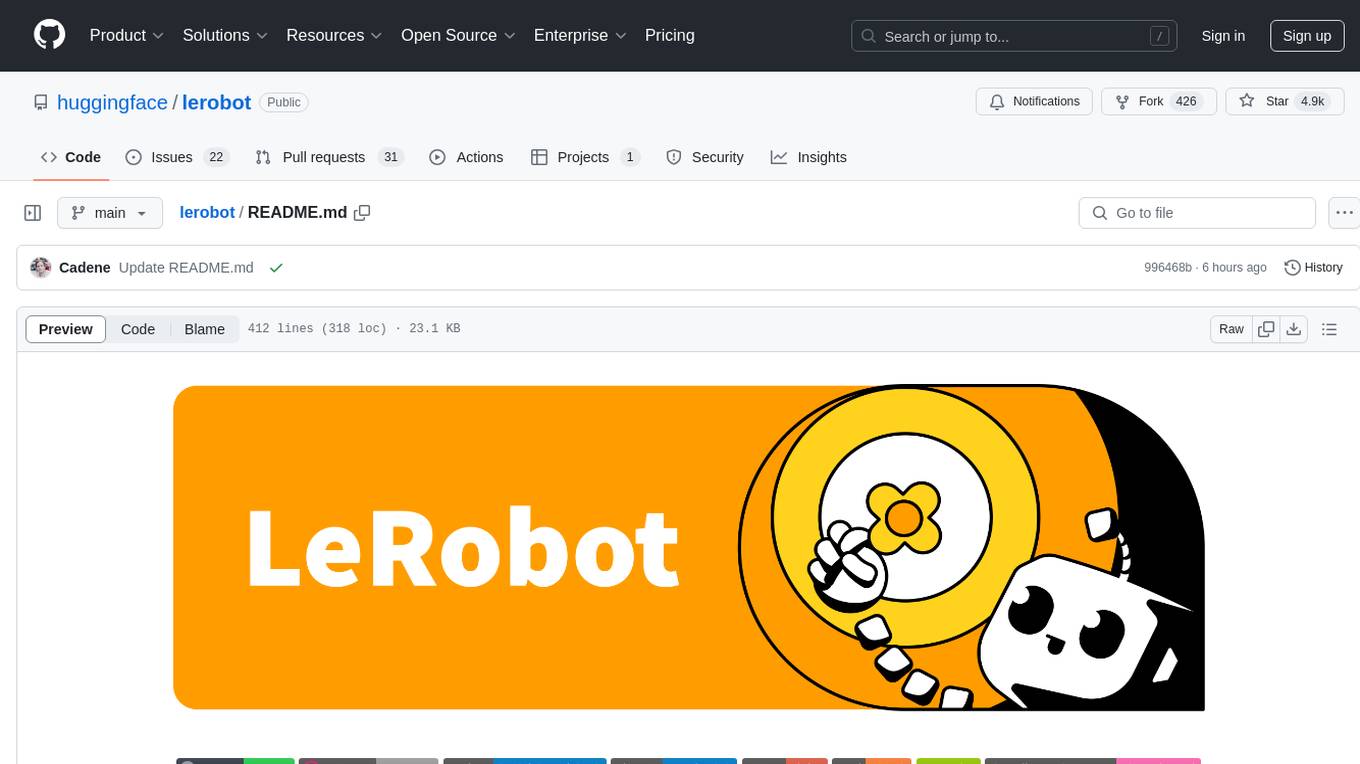
lerobot
LeRobot is a state-of-the-art AI library for real-world robotics in PyTorch. It aims to provide models, datasets, and tools to lower the barrier to entry to robotics, focusing on imitation learning and reinforcement learning. LeRobot offers pretrained models, datasets with human-collected demonstrations, and simulation environments. It plans to support real-world robotics on affordable and capable robots. The library hosts pretrained models and datasets on the Hugging Face community page.
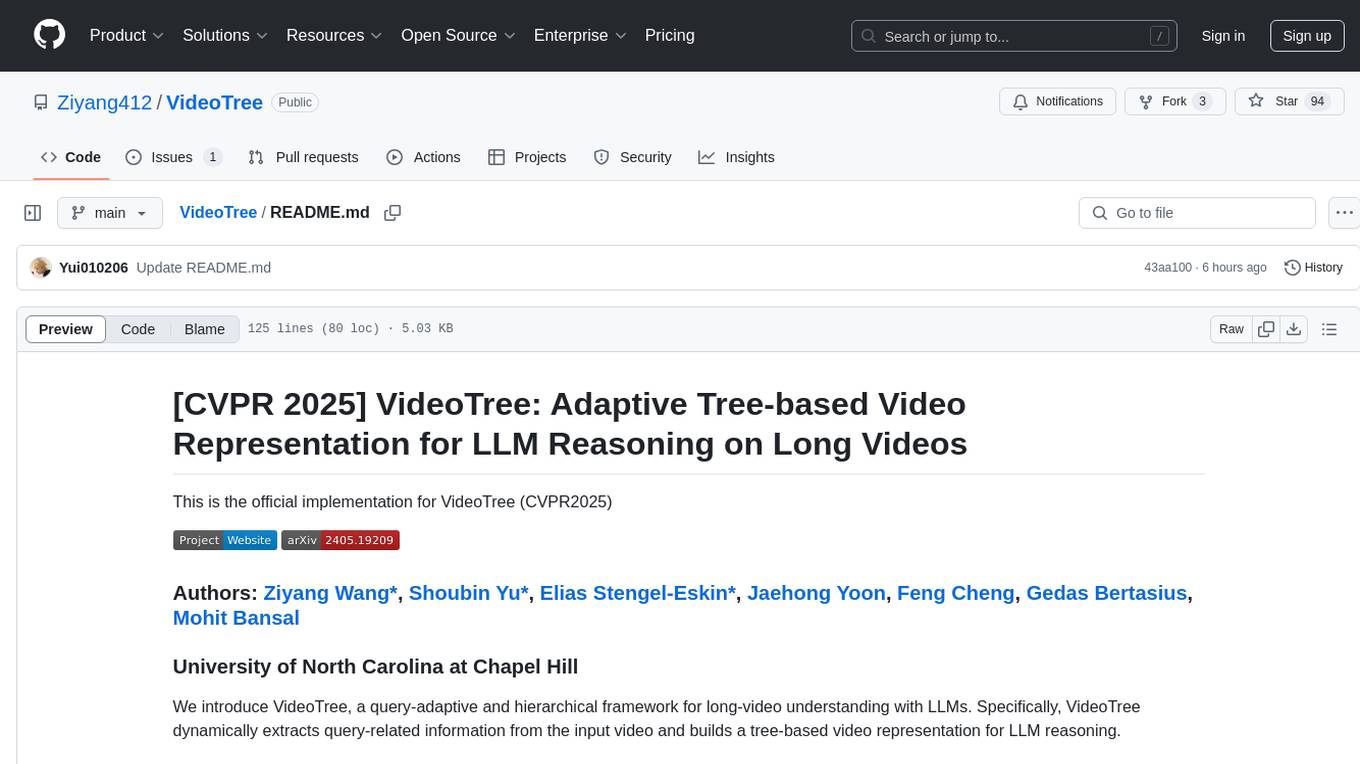
VideoTree
VideoTree is an official implementation for a query-adaptive and hierarchical framework for understanding long videos with LLMs. It dynamically extracts query-related information from input videos and builds a tree-based video representation for LLM reasoning. The tool requires Python 3.8 or above and leverages models like LaViLa and EVA-CLIP-8B for feature extraction. It also provides scripts for tasks like Adaptive Breath Expansion, Relevance-based Depth Expansion, and LLM Reasoning. The codebase is being updated to incorporate scripts/captions for NeXT-QA and IntentQA in the future.
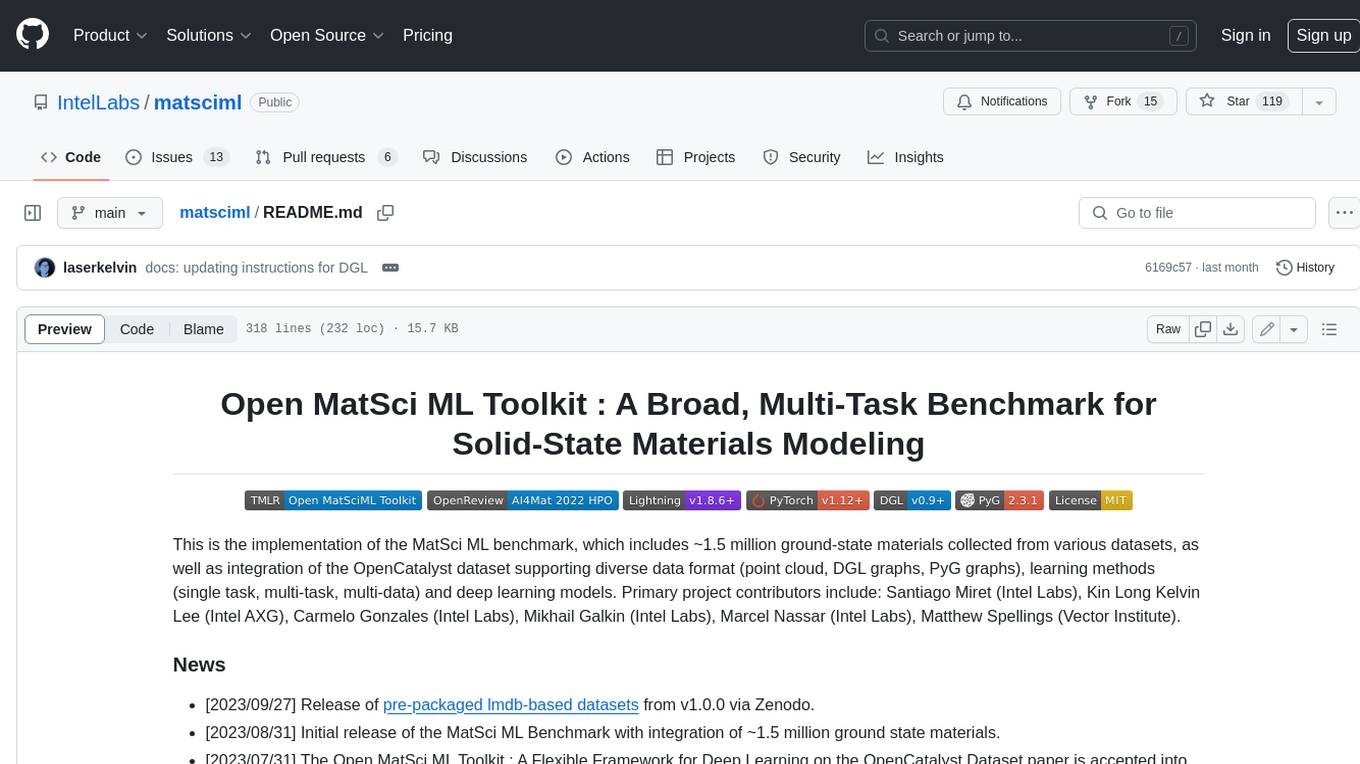
matsciml
The Open MatSci ML Toolkit is a flexible framework for machine learning in materials science. It provides a unified interface to a variety of materials science datasets, as well as a set of tools for data preprocessing, model training, and evaluation. The toolkit is designed to be easy to use for both beginners and experienced researchers, and it can be used to train models for a wide range of tasks, including property prediction, materials discovery, and materials design.
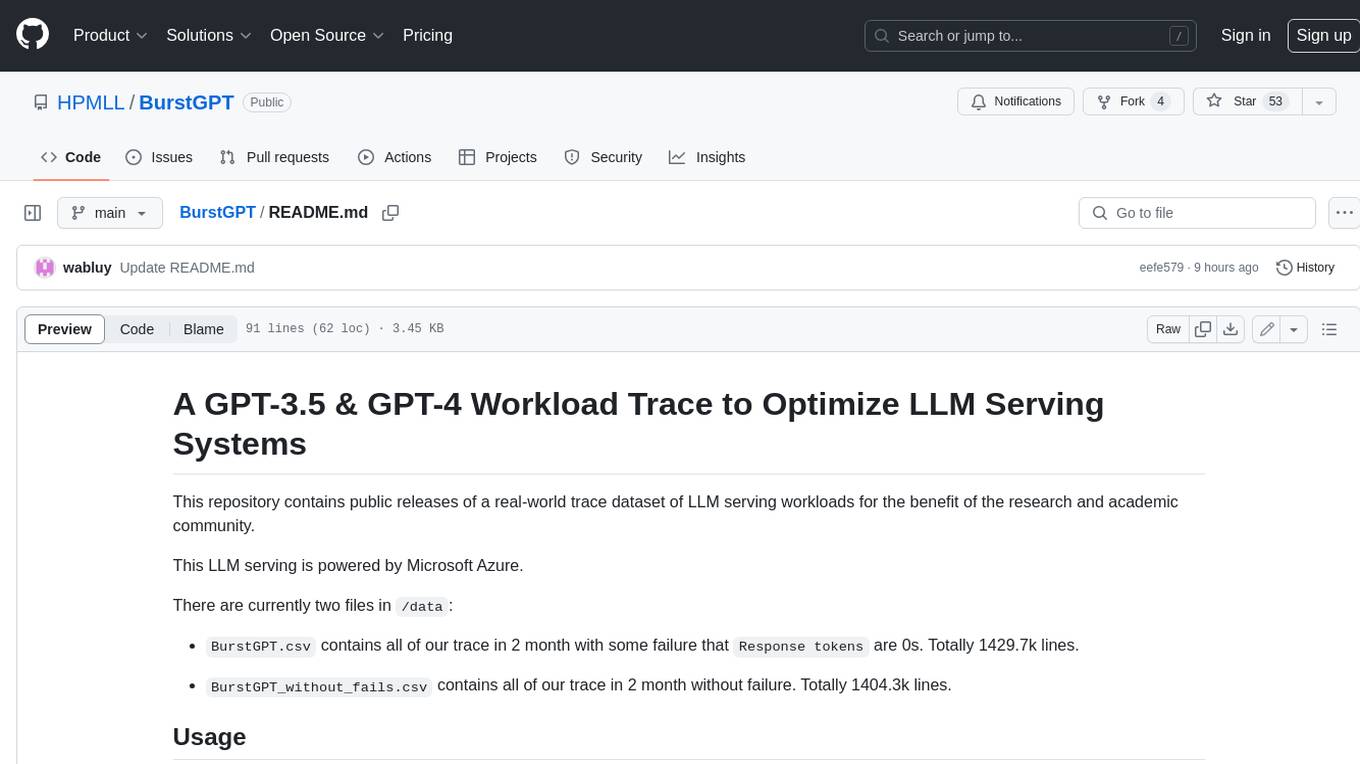
BurstGPT
This repository provides a real-world trace dataset of LLM serving workloads for research and academic purposes. The dataset includes two files, BurstGPT.csv with trace data for 2 months including some failures, and BurstGPT_without_fails.csv without any failures. Users can scale the RPS in the trace, model patterns, and leverage the trace for various evaluations. Future plans include updating the time range of the trace, adding request end times, updating conversation logs, and open-sourcing a benchmark suite for LLM inference. The dataset covers 61 consecutive days, contains 1.4 million lines, and is approximately 50MB in size.
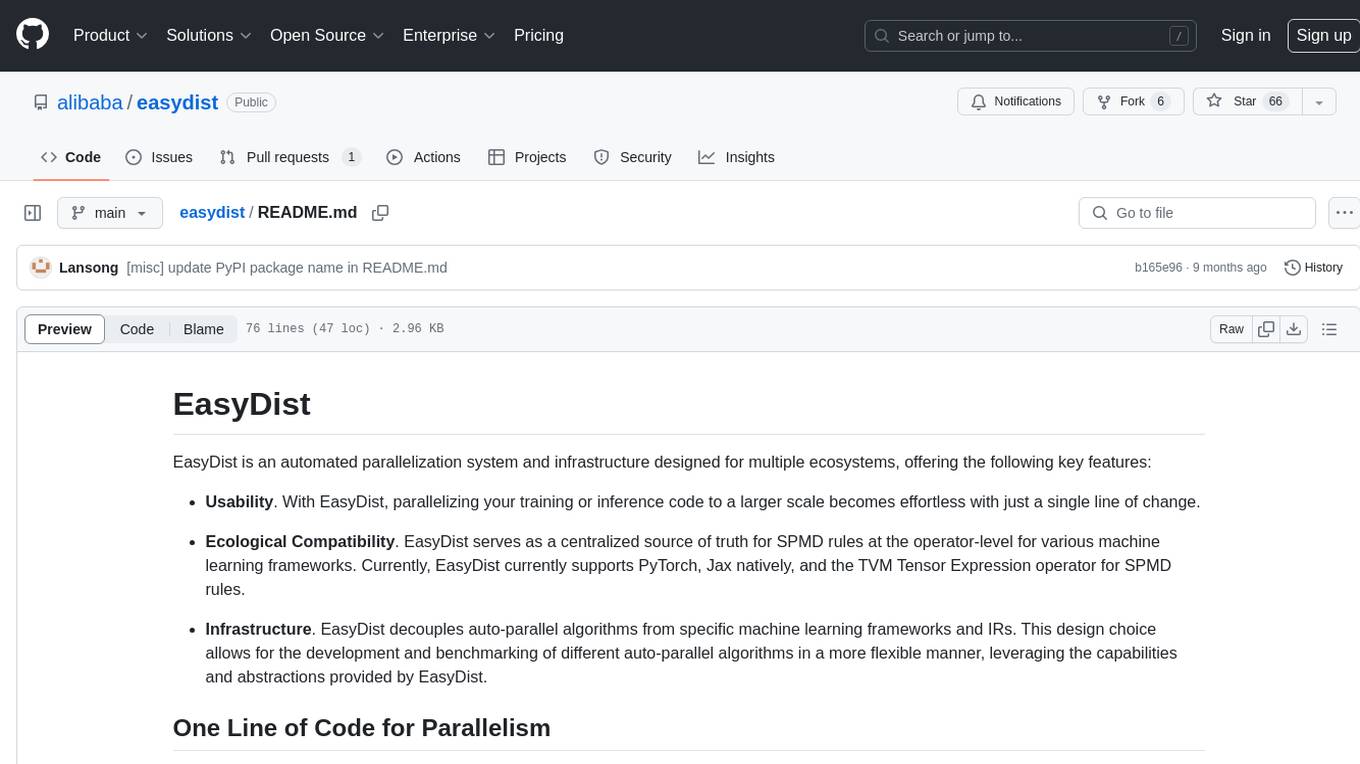
easydist
EasyDist is an automated parallelization system and infrastructure designed for multiple ecosystems. It offers usability by making parallelizing training or inference code effortless with just a single line of change. It ensures ecological compatibility by serving as a centralized source of truth for SPMD rules at the operator-level for various machine learning frameworks. EasyDist decouples auto-parallel algorithms from specific frameworks and IRs, allowing for the development and benchmarking of different auto-parallel algorithms in a flexible manner. The architecture includes MetaOp, MetaIR, and the ShardCombine Algorithm for SPMD sharding rules without manual annotations.
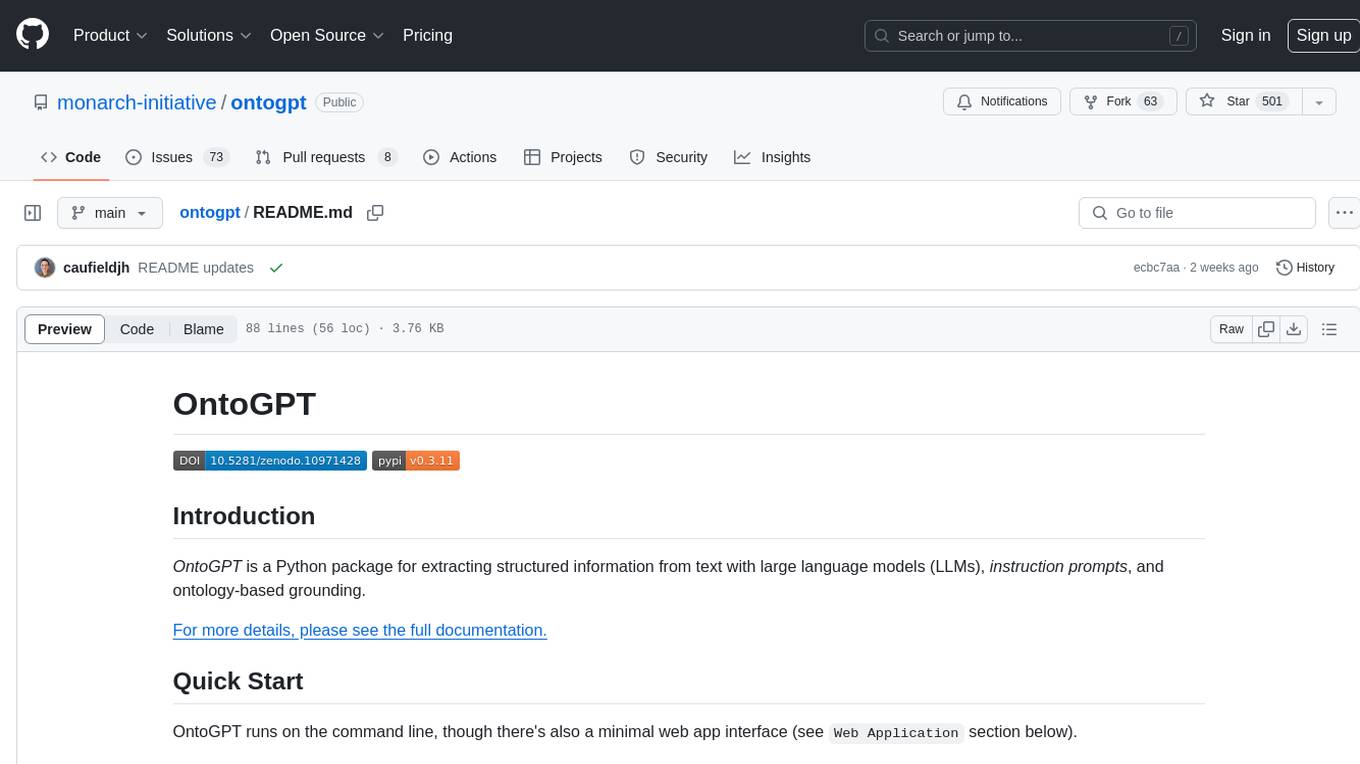
ontogpt
OntoGPT is a Python package for extracting structured information from text using large language models, instruction prompts, and ontology-based grounding. It provides a command line interface and a minimal web app for easy usage. The tool has been evaluated on test data and is used in related projects like TALISMAN for gene set analysis. OntoGPT enables users to extract information from text by specifying relevant terms and provides the extracted objects as output.
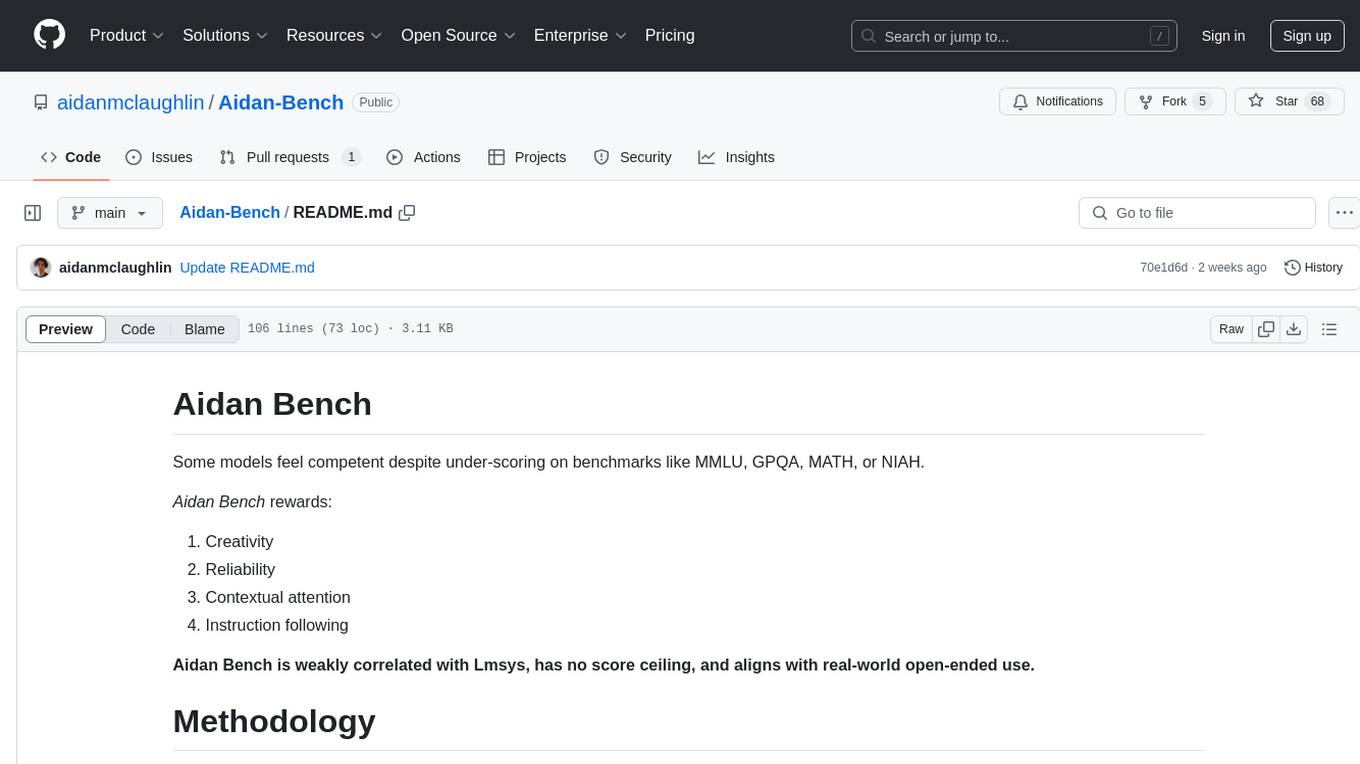
Aidan-Bench
Aidan Bench is a tool that rewards creativity, reliability, contextual attention, and instruction following. It is weakly correlated with Lmsys, has no score ceiling, and aligns with real-world open-ended use. The tool involves giving LLMs open-ended questions and evaluating their answers based on novelty scores. Users can set up the tool by installing required libraries and setting up API keys. The project allows users to run benchmarks for different models and provides flexibility in threading options.
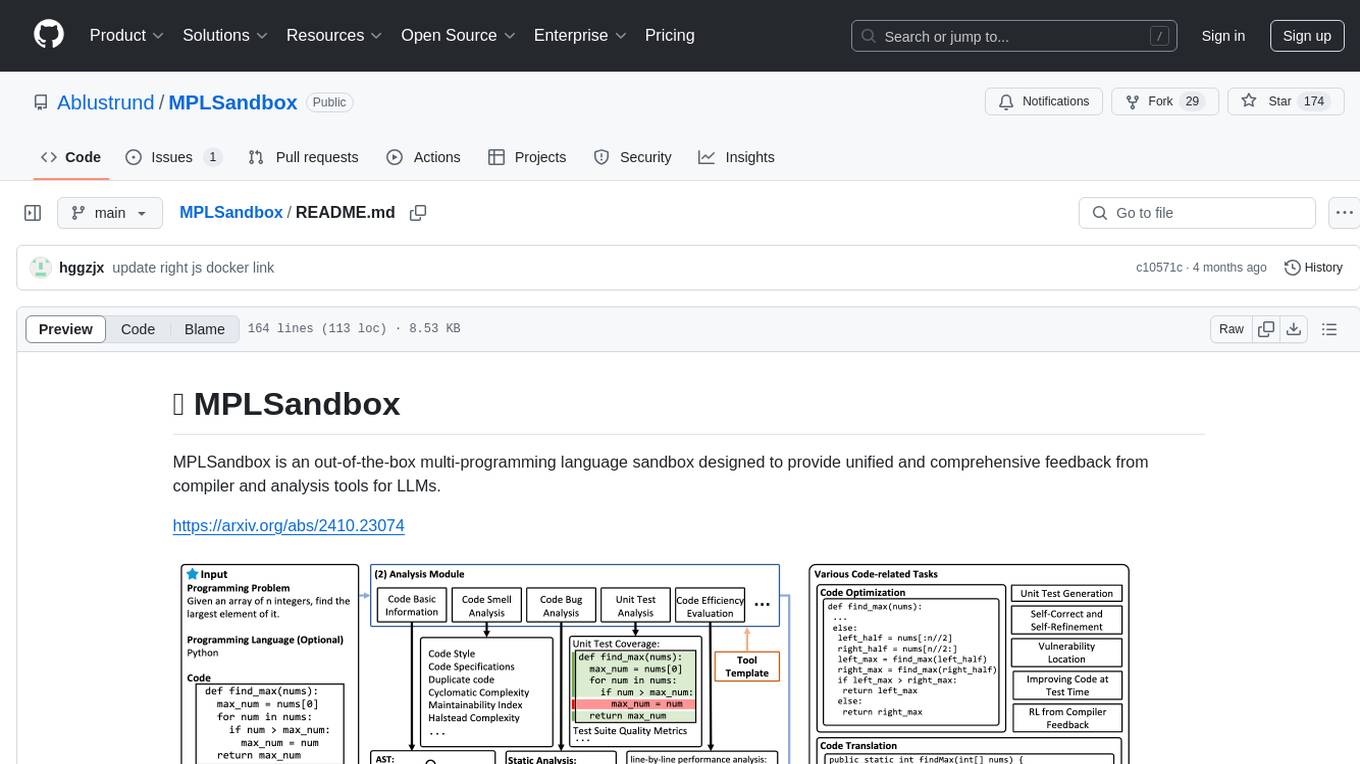
MPLSandbox
MPLSandbox is an out-of-the-box multi-programming language sandbox designed to provide unified and comprehensive feedback from compiler and analysis tools for LLMs. It simplifies code analysis for researchers and can be seamlessly integrated into LLM training and application processes to enhance performance in a range of code-related tasks. The sandbox environment ensures safe code execution, the code analysis module offers comprehensive analysis reports, and the information integration module combines compilation feedback and analysis results for complex code-related tasks.
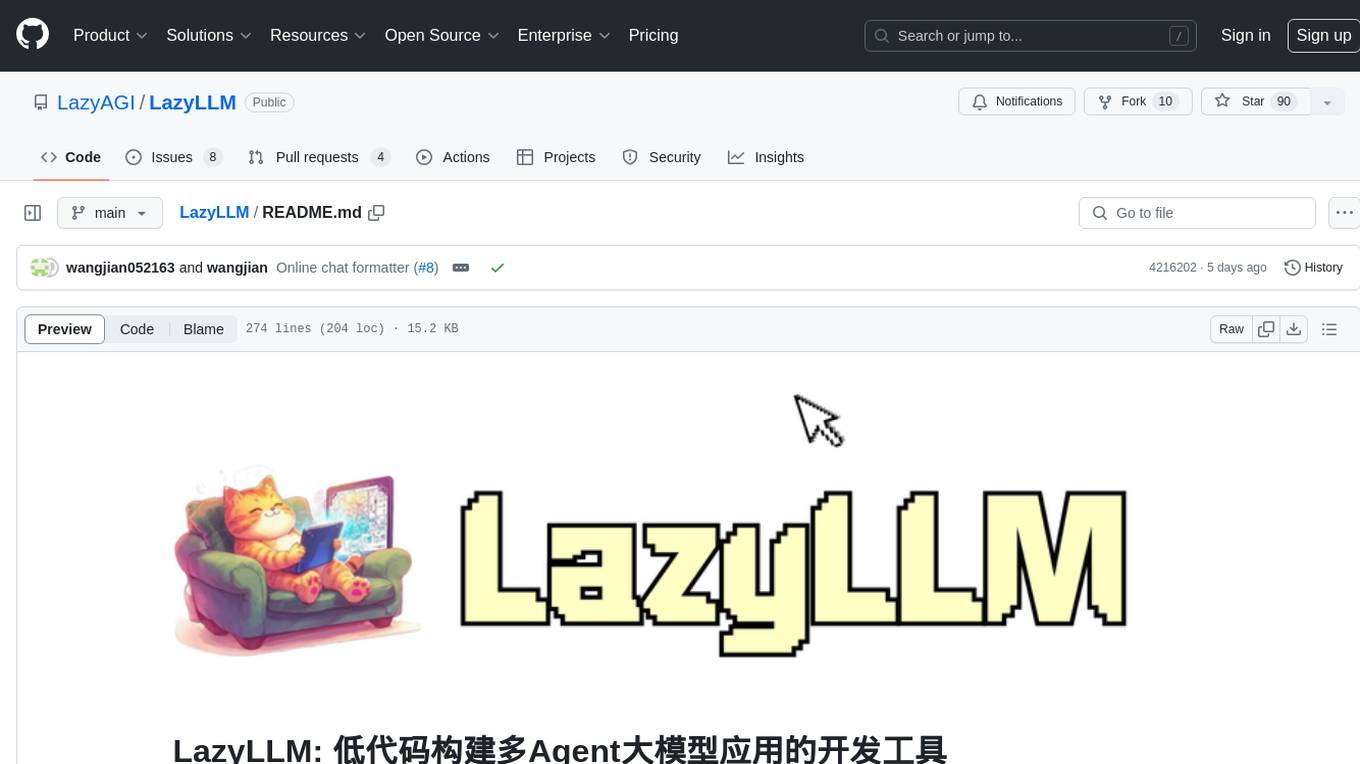
LazyLLM
LazyLLM is a low-code development tool for building complex AI applications with multiple agents. It assists developers in building AI applications at a low cost and continuously optimizing their performance. The tool provides a convenient workflow for application development and offers standard processes and tools for various stages of application development. Users can quickly prototype applications with LazyLLM, analyze bad cases with scenario task data, and iteratively optimize key components to enhance the overall application performance. LazyLLM aims to simplify the AI application development process and provide flexibility for both beginners and experts to create high-quality applications.
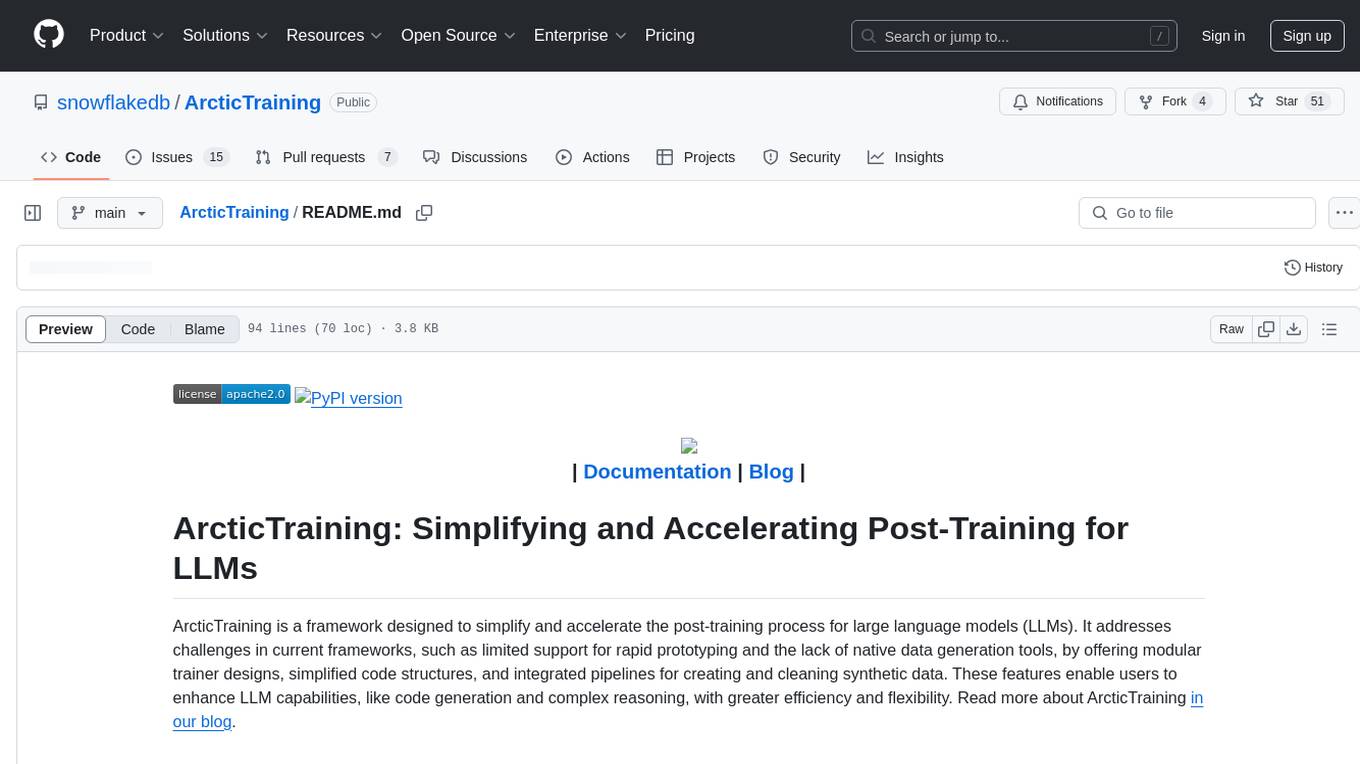
ArcticTraining
ArcticTraining is a framework designed to simplify and accelerate the post-training process for large language models (LLMs). It offers modular trainer designs, simplified code structures, and integrated pipelines for creating and cleaning synthetic data, enabling users to enhance LLM capabilities like code generation and complex reasoning with greater efficiency and flexibility.
For similar tasks
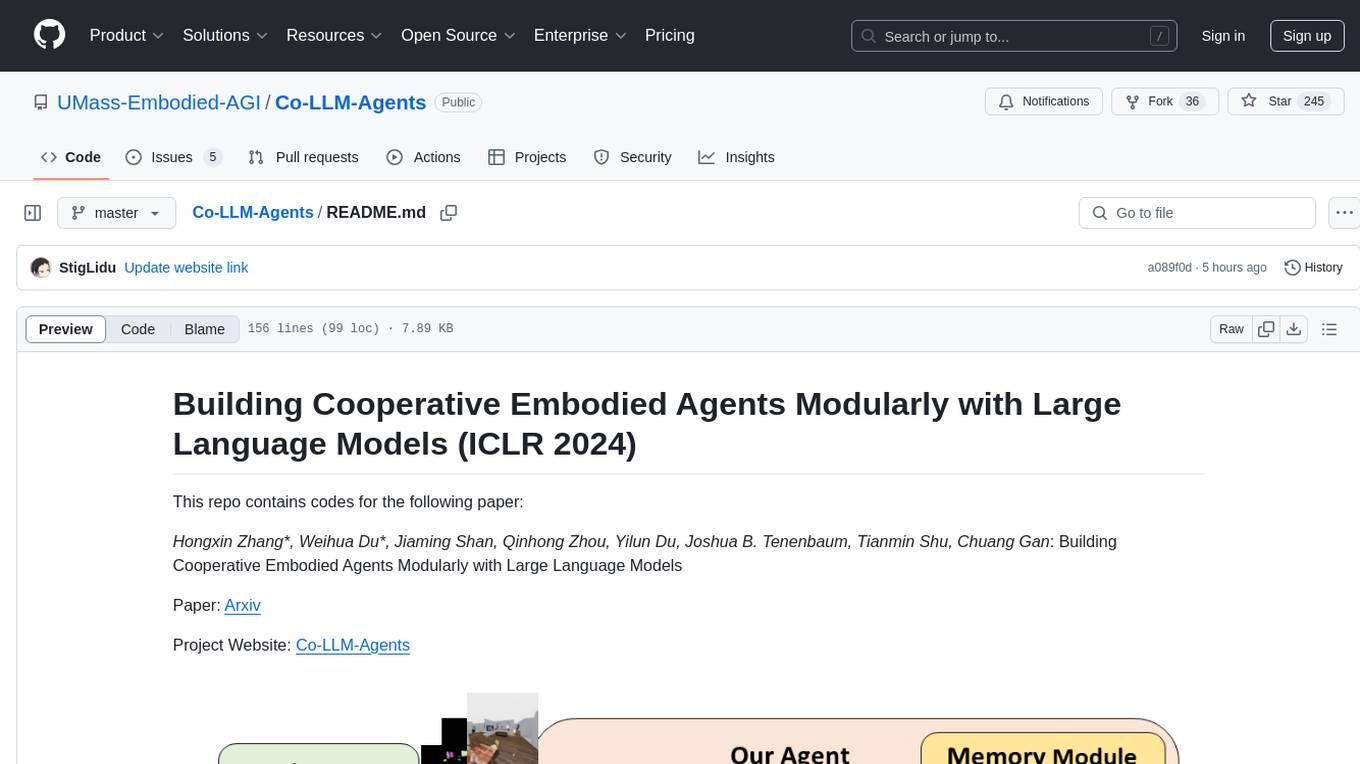
Co-LLM-Agents
Co-LLM-Agents is a repository containing codes for the paper 'Building Cooperative Embodied Agents Modularly with Large Language Models'. The project focuses on developing cooperative embodied agents using large language models, with a specific emphasis on the ThreeDWorld Multi-Agent Transport environment. The repository provides implementations, installation instructions, and example scripts for running experiments with the CoELA model. It extends the ThreeDWorld Transport Challenge into a multi-agent setting, enabling agents to transport target objects using containers and communicate with each other. Additionally, it includes the Communicative Watch-And-Help challenge, where agents can send messages to each other while performing tasks such as preparing meals, washing dishes, and setting up dinner tables.
For similar jobs
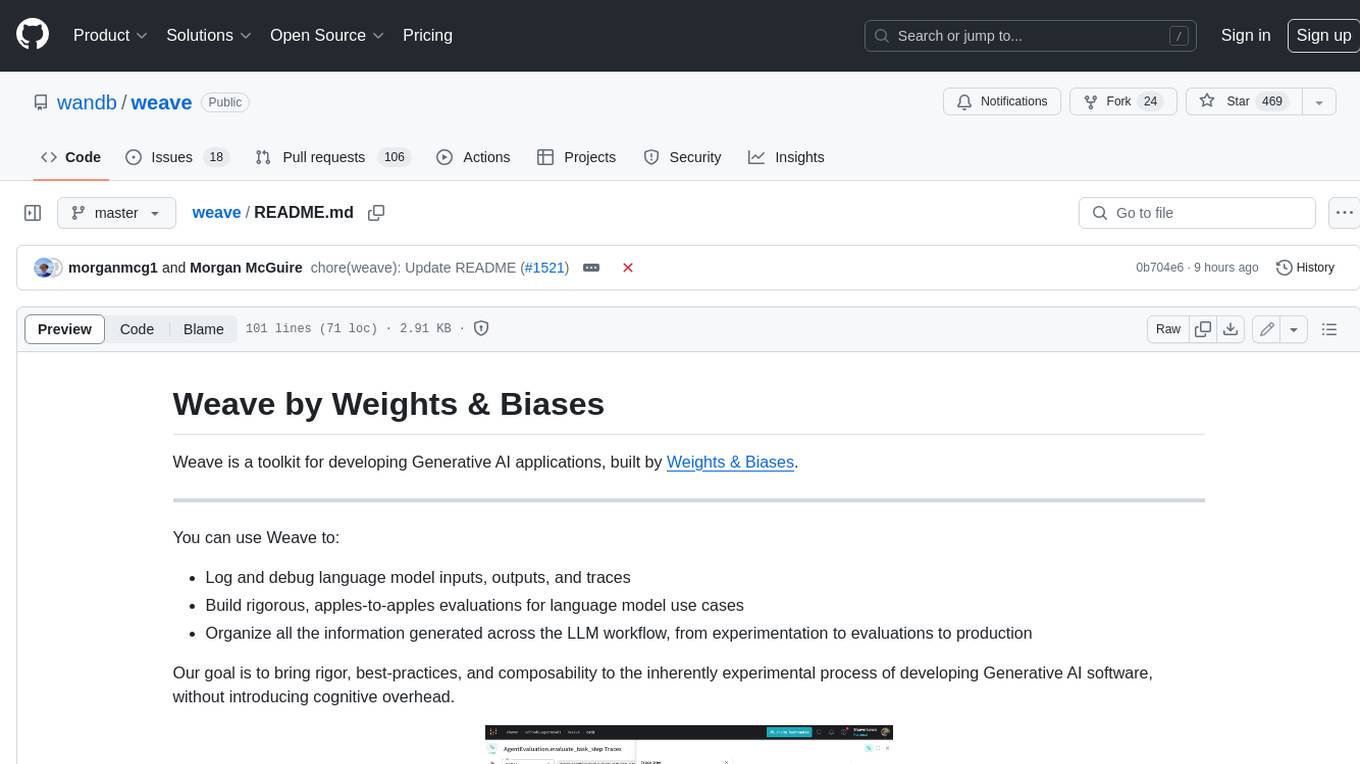
weave
Weave is a toolkit for developing Generative AI applications, built by Weights & Biases. With Weave, you can log and debug language model inputs, outputs, and traces; build rigorous, apples-to-apples evaluations for language model use cases; and organize all the information generated across the LLM workflow, from experimentation to evaluations to production. Weave aims to bring rigor, best-practices, and composability to the inherently experimental process of developing Generative AI software, without introducing cognitive overhead.
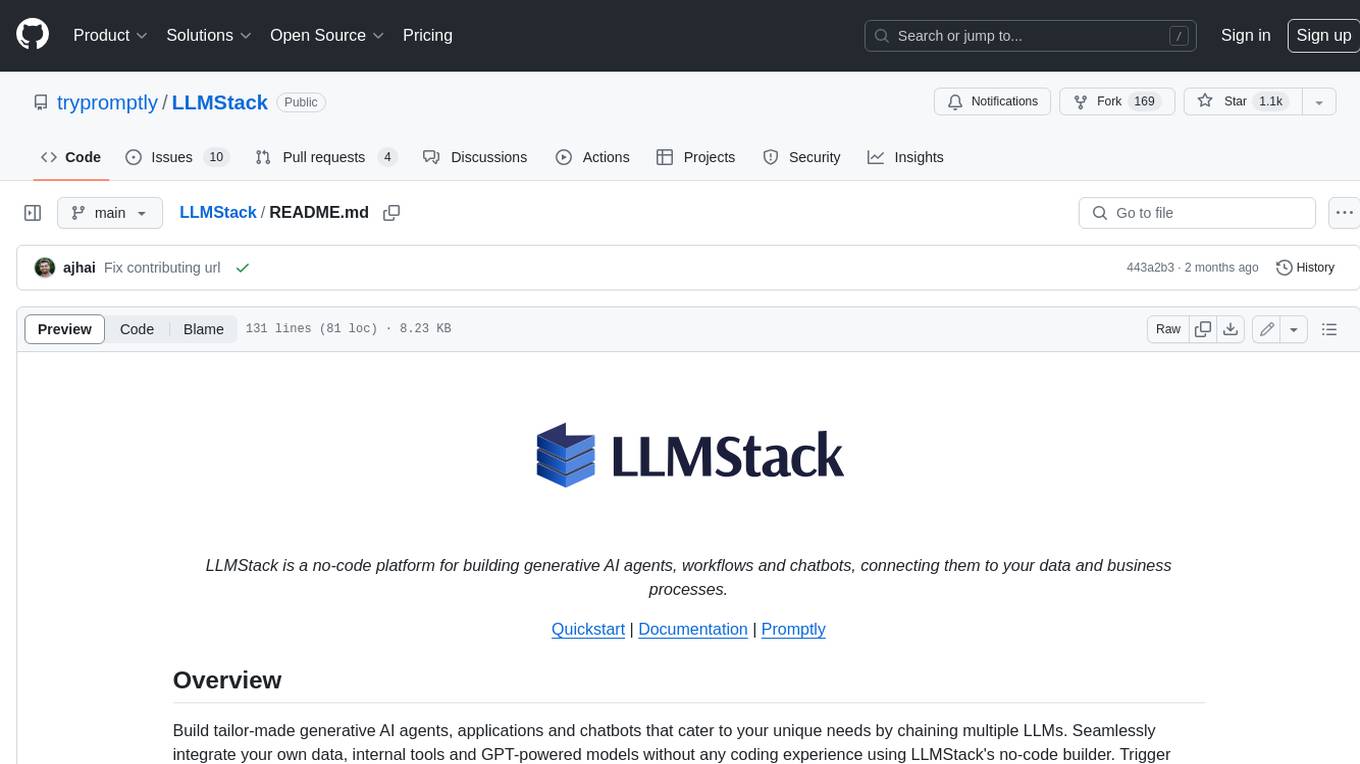
LLMStack
LLMStack is a no-code platform for building generative AI agents, workflows, and chatbots. It allows users to connect their own data, internal tools, and GPT-powered models without any coding experience. LLMStack can be deployed to the cloud or on-premise and can be accessed via HTTP API or triggered from Slack or Discord.
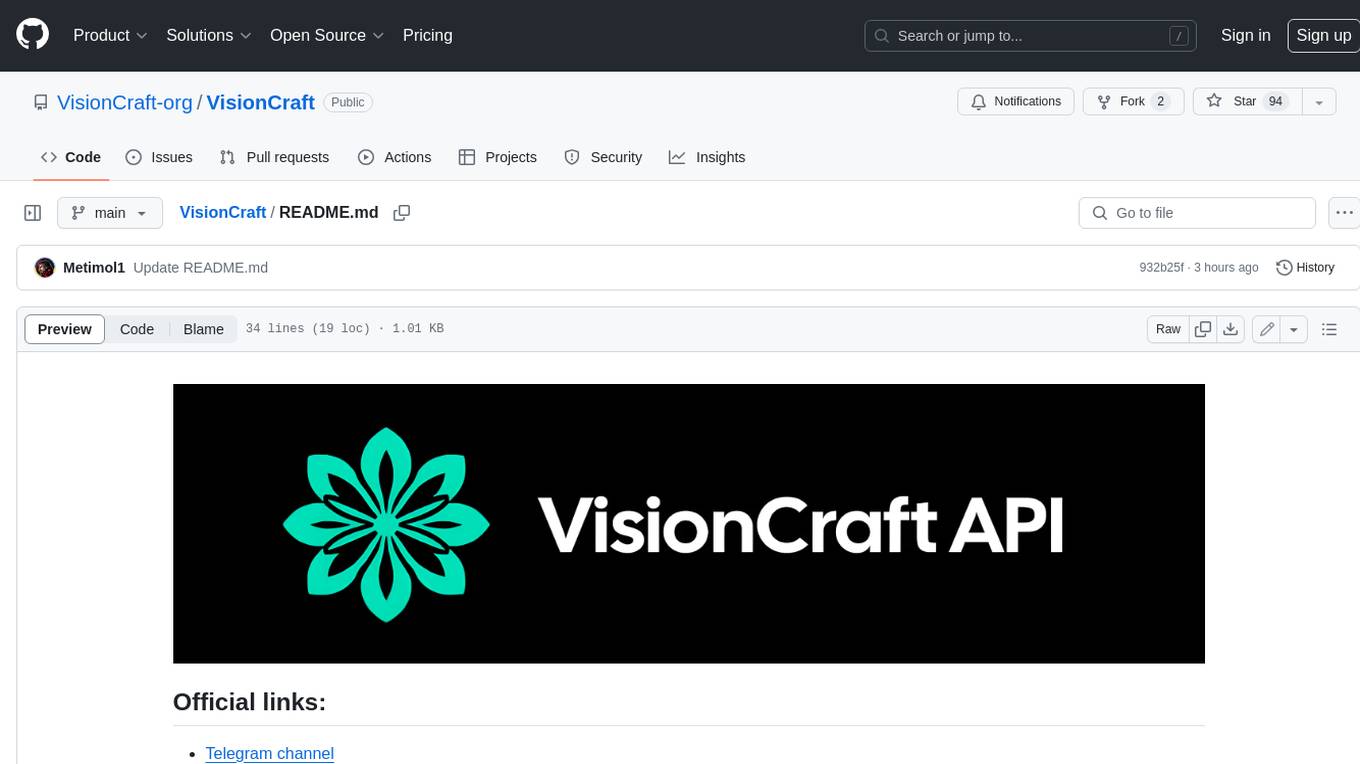
VisionCraft
The VisionCraft API is a free API for using over 100 different AI models. From images to sound.
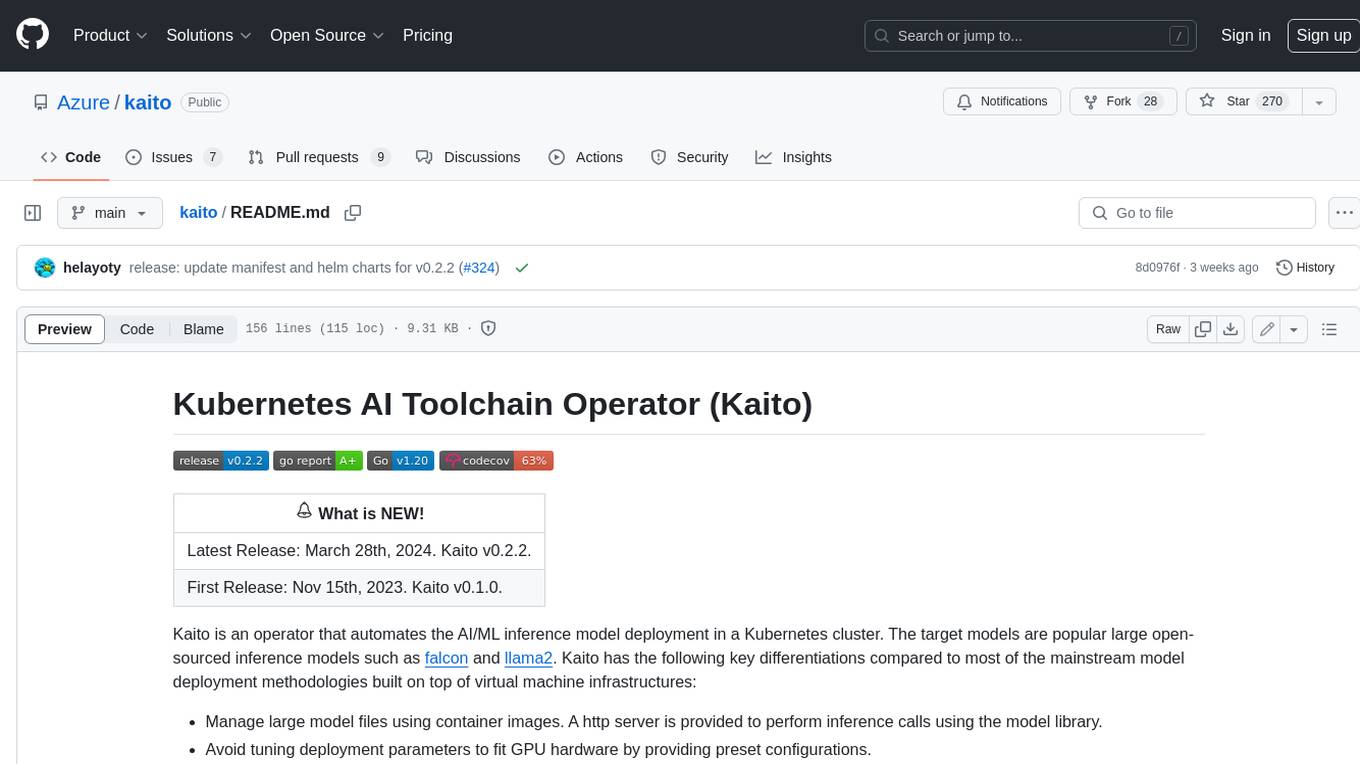
kaito
Kaito is an operator that automates the AI/ML inference model deployment in a Kubernetes cluster. It manages large model files using container images, avoids tuning deployment parameters to fit GPU hardware by providing preset configurations, auto-provisions GPU nodes based on model requirements, and hosts large model images in the public Microsoft Container Registry (MCR) if the license allows. Using Kaito, the workflow of onboarding large AI inference models in Kubernetes is largely simplified.
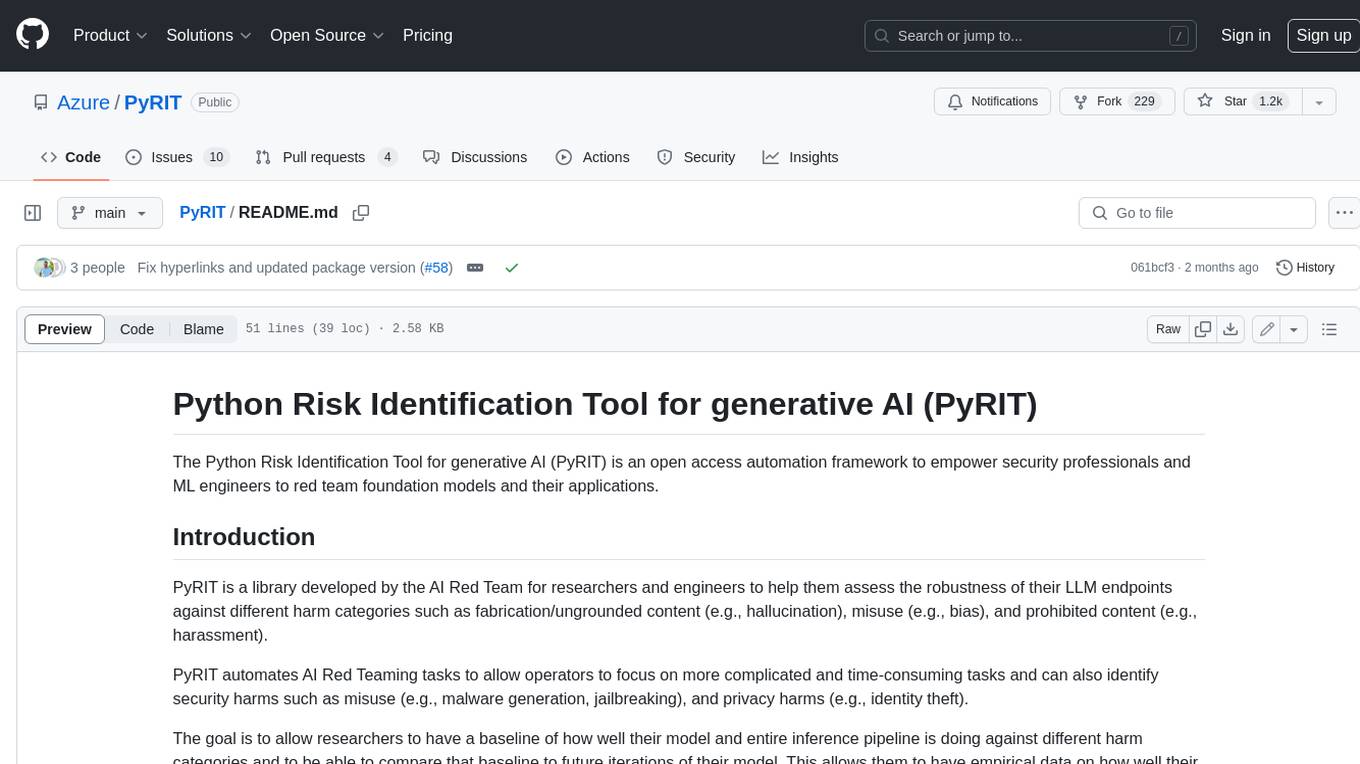
PyRIT
PyRIT is an open access automation framework designed to empower security professionals and ML engineers to red team foundation models and their applications. It automates AI Red Teaming tasks to allow operators to focus on more complicated and time-consuming tasks and can also identify security harms such as misuse (e.g., malware generation, jailbreaking), and privacy harms (e.g., identity theft). The goal is to allow researchers to have a baseline of how well their model and entire inference pipeline is doing against different harm categories and to be able to compare that baseline to future iterations of their model. This allows them to have empirical data on how well their model is doing today, and detect any degradation of performance based on future improvements.
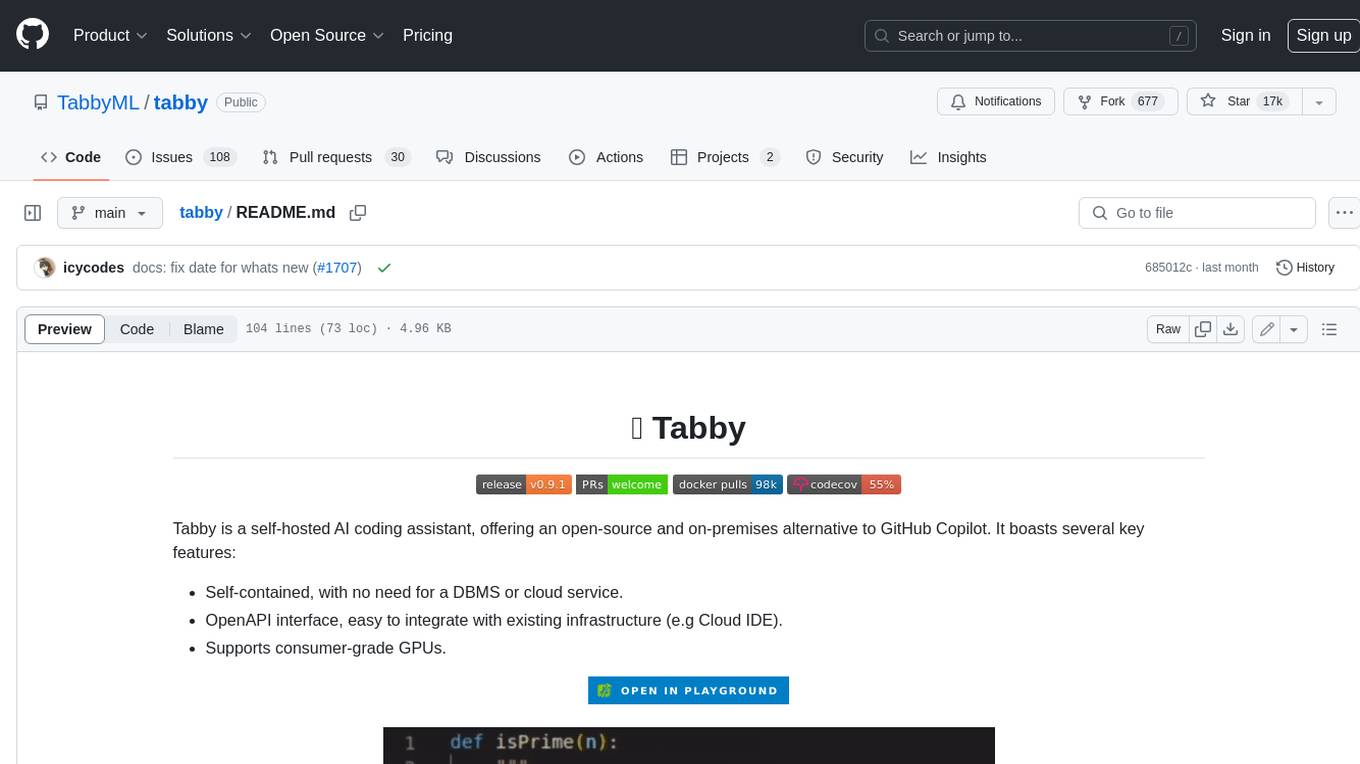
tabby
Tabby is a self-hosted AI coding assistant, offering an open-source and on-premises alternative to GitHub Copilot. It boasts several key features: * Self-contained, with no need for a DBMS or cloud service. * OpenAPI interface, easy to integrate with existing infrastructure (e.g Cloud IDE). * Supports consumer-grade GPUs.
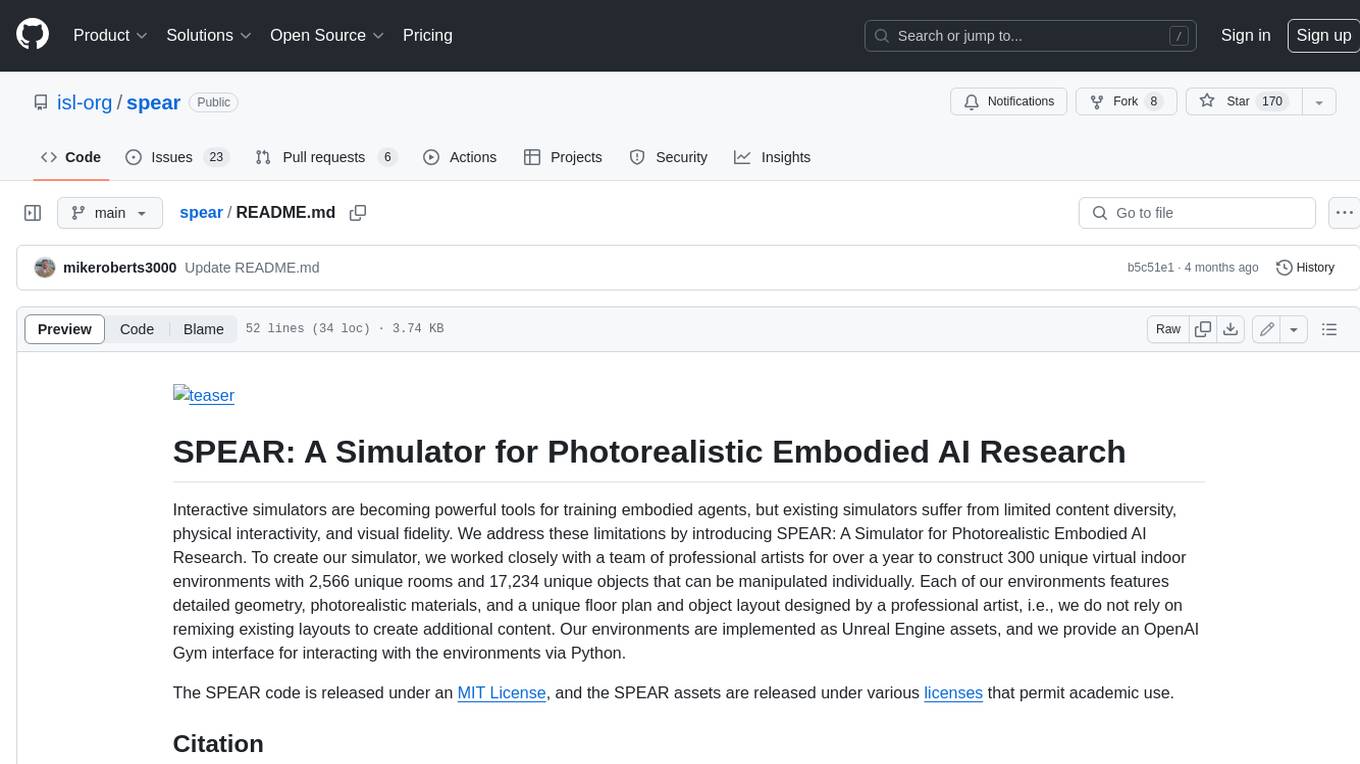
spear
SPEAR (Simulator for Photorealistic Embodied AI Research) is a powerful tool for training embodied agents. It features 300 unique virtual indoor environments with 2,566 unique rooms and 17,234 unique objects that can be manipulated individually. Each environment is designed by a professional artist and features detailed geometry, photorealistic materials, and a unique floor plan and object layout. SPEAR is implemented as Unreal Engine assets and provides an OpenAI Gym interface for interacting with the environments via Python.
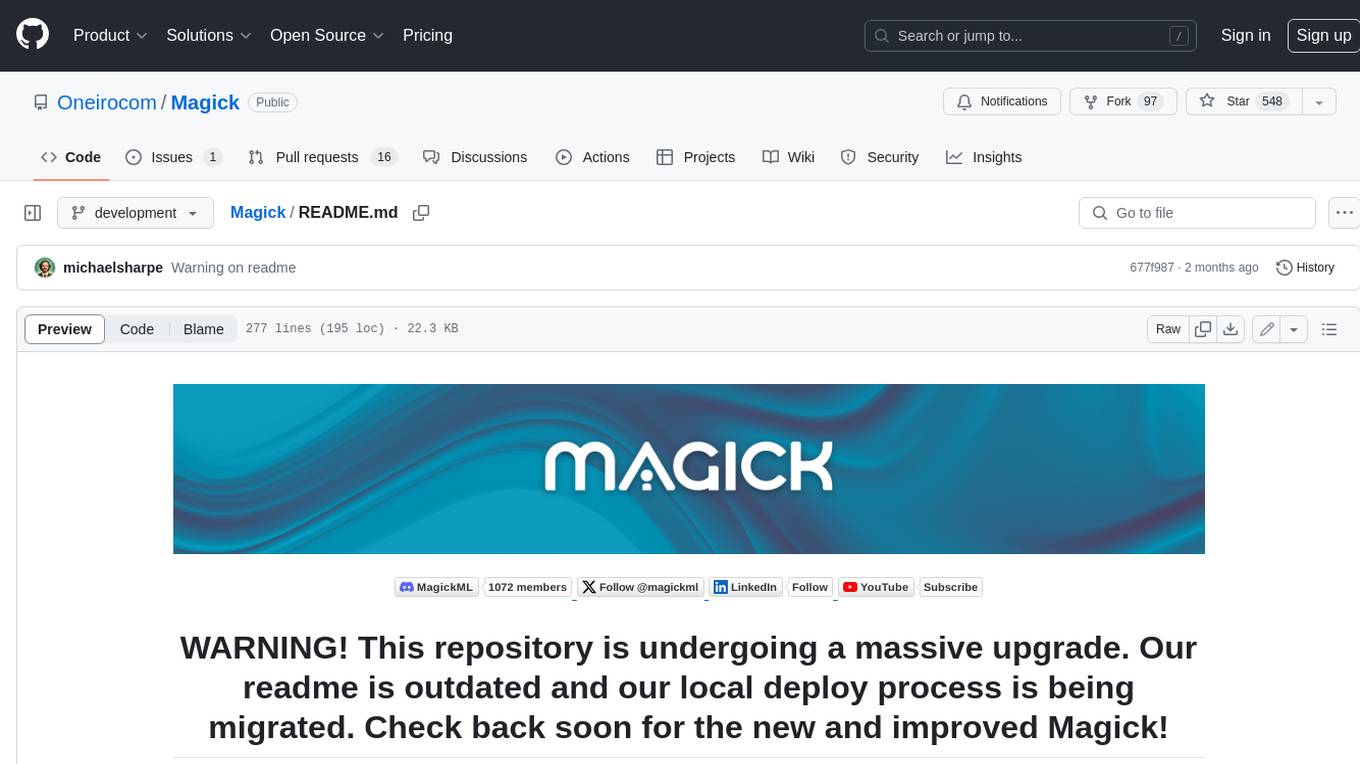
Magick
Magick is a groundbreaking visual AIDE (Artificial Intelligence Development Environment) for no-code data pipelines and multimodal agents. Magick can connect to other services and comes with nodes and templates well-suited for intelligent agents, chatbots, complex reasoning systems and realistic characters.