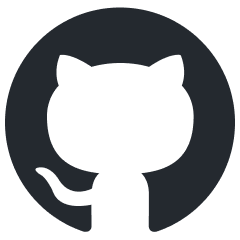
awesome-MLSecOps
A curated list of MLSecOps tools, articles and other resources on security applied to Machine Learning and MLOps systems.
Stars: 204
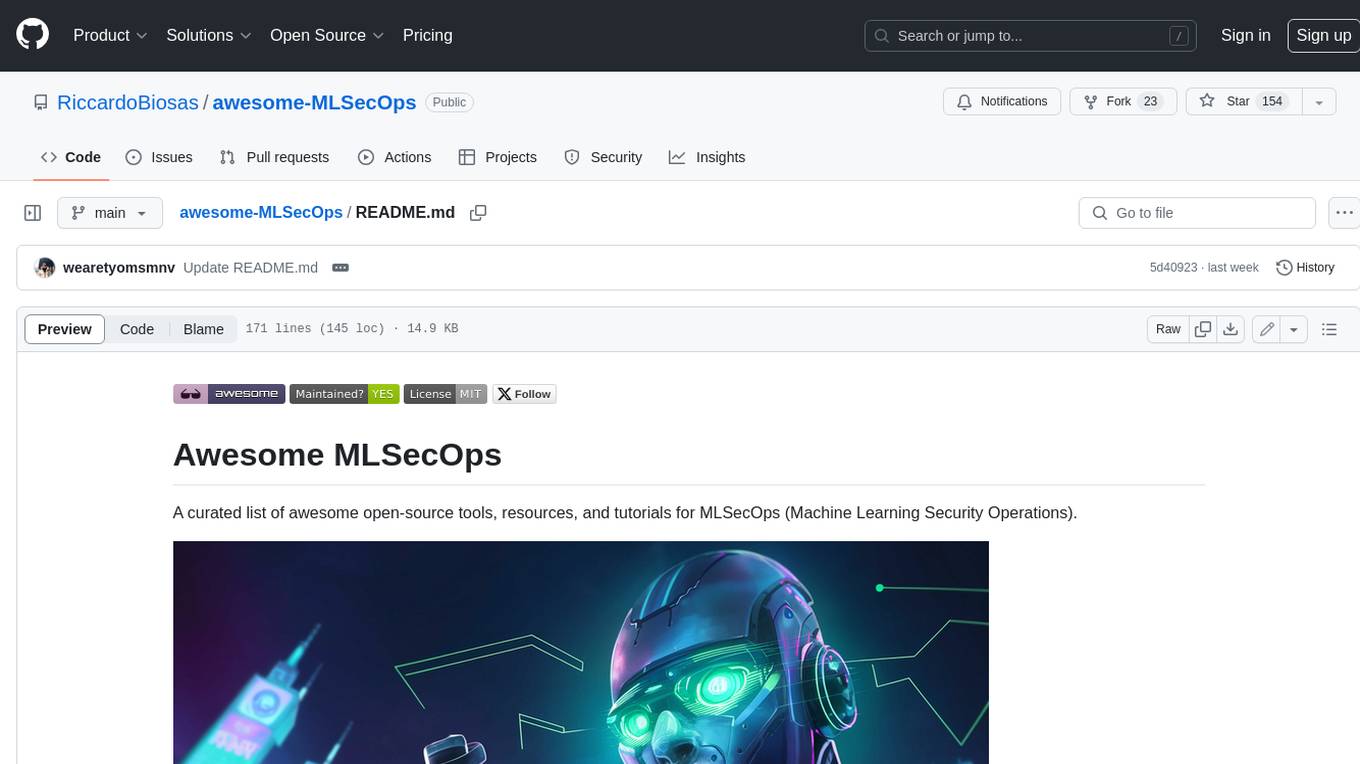
Awesome MLSecOps is a curated list of open-source tools, resources, and tutorials for MLSecOps (Machine Learning Security Operations). It includes a wide range of security tools and libraries for protecting machine learning models against adversarial attacks, as well as resources for AI security, data anonymization, model security, and more. The repository aims to provide a comprehensive collection of tools and information to help users secure their machine learning systems and infrastructure.
README:
A curated list of awesome open-source tools, resources, and tutorials for MLSecOps (Machine Learning Security Operations).
- Open Source Security Tools
- Commercial Tools
- DATA
- ML Code Security
- 101 Resources
- Attack Vectors
- Blogs and Publications
- MLOps Infrastructure Vulnerabilities
- Community Resources
- Infographics
- Contributions
- Contributors
In this section, you and I can take a look at what opensource solutions and PoCs, exist to accomplish the task of ML protection. Of course, some of them are unsupported or will have difficulties to run. However, not mentioning them is a big crime.
Tool | Description |
---|---|
ModelScan | Protection Against ML Model Serialization Attacks |
NB Defense | Secure Jupyter Notebooks |
Garak | LLM vulnerability scanner |
Adversarial Robustness Toolbox | Library of defense methods for ML models against adversarial attacks |
MLSploit | Cloud framework for interactive experimentation with adversarial machine learning research |
TensorFlow Privacy | Library of privacy-preserving machine learning algorithms and tools |
Foolbox | Python toolbox for creating and evaluating adversarial attacks and defenses |
Advertorch | Python toolbox for adversarial robustness research |
Artificial Intelligence Threat Matrix | Framework for identifying and mitigating threats to machine learning systems |
Adversarial ML Threat Matrix | Adversarial Threat Landscape for AI Systems |
CleverHans | A library of adversarial examples and defenses for machine learning models |
AdvBox | Advbox is a toolbox to generate adversarial examples that fool neural networks in PaddlePaddle、PyTorch、Caffe2、MxNet、Keras、TensorFlow |
Audit AI | Bias Testing for Generalized Machine Learning Applications |
Deep Pwning | Deep-pwning is a lightweight framework for experimenting with machine learning models with the goal of evaluating their robustness against a motivated adversary |
Privacy Meter | An open-source library to audit data privacy in statistical and machine learning algorithms |
TensorFlow Model Analysis | A library for analyzing, validating, and monitoring machine learning models in production |
PromptInject | A framework that assembles adversarial prompts |
TextAttack | TextAttack is a Python framework for adversarial attacks, data augmentation, and model training in NLP |
OpenAttack | An Open-Source Package for Textual Adversarial Attack |
TextFooler | A Model for Natural Language Attack on Text Classification and Inference |
Flawed Machine Learning Security | Practical examples of "Flawed Machine Learning Security" together with ML Security best practice across the end to end stages of the machine learning model lifecycle from training, to packaging, to deployment |
Adversarial Machine Learning CTF | This repository is a CTF challenge, showing a security flaw in most (all?) common artificial neural networks. They are vulnerable for adversarial images |
Damn Vulnerable LLM Project | A Large Language Model designed for getting hacked |
Gandalf Lakera | Prompt Injection CTF playground |
Vigil | LLM prompt injection and security scanner |
PALLMs (Payloads for Attacking Large Language Models) | list of various payloads for attacking LLMs collected in one place |
AI-exploits | exploits for MlOps systems. It's not just in the inputs given to LLMs such as ChatGPT |
Offensive ML Playbook | Offensive ML Playbook. Notes on machine learning attacks and pentesting |
AnonLLM | Anonymize Personally Identifiable Information (PII) for Large Language Model APIs |
AI Goat | vulnerable LLM CTF challenges |
Pyrit | The Python Risk Identification Tool for generative AI |
Raze to the Ground: Query-Efficient Adversarial HTML Attacks on Machine-Learning Phishing Webpage Detectors | Source code of the paper "Raze to the Ground: Query-Efficient Adversarial HTML Attacks on Machine-Learning Phishing Webpage Detectors" accepted at AISec '23 |
Giskard | Open-source testing tool for LLM applications |
Safetensors | Convert pickle to a safe serialization option |
Citadel Lens | Quality testing of models according to industry standards |
Model-Inversion-Attack-ToolBox | A framework for implementing Model Inversion attacks |
NeMo-Guardials | NeMo Guardrails allow developers building LLM-based applications to easily add programmable guardrails between the application code and the LLM |
AugLy | A tool for generating adversarial attacks |
Knockoffnets | PoC to implement BlackBox attacks to steal model data |
Robust Intelligence Continous Validation | Tool for continuous model validation for compliance with standards |
VGER | Jupyter Attack framework |
AIShield Watchtower | An open source tool from AIShield for studying AI models and scanning for vulnerabilities |
PS-fuzz | tool for scanning LLM vulnerabilities |
Mindgard-cli | Check security of you AI via CLI |
PurpleLLama3 | Check LLM security with Meta LLM Benchmark |
Model transparency | generate model signing |
ARTkit | Automated prompt-based testing and evaluation of Gen AI applications |
LangBiTe | A Bias Tester framework for LLMs |
OpenDP | The core library of differential privacy algorithms powering the OpenDP Project |
TF-encrypted | Encryption for tensorflow |
Tool | Description |
---|---|
Databricks Platform, Azure Databricks | Datalake data management and implementation tool |
Hidden Layer AI Detection Response | Tool for detecting and responding to incidents |
Guardian | Model protection in CI/CD |
Tool | Description |
---|---|
ARX - Data Anonymization Tool | Tool for anonymizing datasets |
Data-Veil | Data masking and anonymization tool |
Tool for IMG anonymization | Image anonymization |
Tool for DATA anonymization | Data anonymization |
BMW-Anonymization-Api | This repository allows you to anonymize sensitive information in images/videos. The solution is fully compatible with the DL-based training/inference solutions that we already published/will publish for Object Detection and Semantic Segmentation |
DeepPrivacy2 | A Toolbox for Realistic Image Anonymization |
PPAP | Latent-space-level Image Anonymization with Adversarial Protector Networks |
- lintML - Security linter for ML, by Nvidia
- HiddenLayer: Model as Code - Research about some vectors in ML libraries
- Copycat CNN - Proof-of-concept on how to generate a copy of a Convolutional Neural Network
- differential-privacy-library - Library designed for differential privacy and machine learning
You can find here a list of resources to help you get into the topic of AI security. Understand what attacks exist and how they can be used by an attacker.
- AI Security 101
- Web LLM attacks
- Microsoft AI Red Team
- AI Risk Assessment for ML Engineers
- Microsoft - Generative AI Security for beginners
Full size map in this repository
more in Adversarial AI Attacks, Mitigations, and Defense Strategies: A cybersecurity professional's guide to AI attacks, threat modeling, and securing AI with MLSecOps
🌱 The AI security community is growing. New blogs and many researchers are emerging. In this paragraph you can see examples of some blogs.
- 🛡️ Red-Teaming Large Language Models
- 🔍 Google's AI red-team
- 🔒 The MLSecOps Top 10 vulnerabilities
- 🏴☠️ Token Smuggling Jailbreak via Adversarial Prompt
- ☣️ Just How Toxic is Data Poisoning? A Unified Benchmark for Backdoor and Data Poisoning Attacks
- 📊 We need a new way to measure AI security
- 🕵️ PrivacyRaven: Implementing a proof of concept for model inversion
- 🧠 Adversarial Prompts Engineering
- 🔫 TextAttack: A Framework for Adversarial Attacks, Data Augmentation, and Adversarial Training in NLP
- 📋 Trail Of Bits' audit of Hugging Face's safetensors library
- 🔝 OWASP Top 10 for Large Language Model Applications
- 🔐 LLM Security
- 🔑 Is you MLOps infrastructure leaking secrets?
- 🚩 Embrace The Red, blog where show how u can hack LLM's.
- 🎙️ Audio-jacking: Using generative AI to distort live audio transactions
- 🌐 HADESS - Web LLM Attacks
- 🧰 WTF-blog - MlSecOps frameworks ... Which ones are available and what is the difference?
- 📚 DreadNode Paper Stack
Very interesting articles on MlOps infrastructure vulnerabilities. In some of them you can even find ready-made exploits.
- SILENT SABOTAGE - Study on bot compromise for converting Pickle to SafeTensors
- NOT SO CLEAR: HOW MLOPS SOLUTIONS CAN MUDDY THE WATERS OF YOUR SUPPLY CHAIN - Study on vulnerabilities for the ClearML platform
- Uncovering Azure's Silent Threats: A Journey into Cloud Vulnerabilities - Study on security issues of Azure MLAAS
- The MLOps Security Landscape
- Confused Learning: Supply Chain Attacks through Machine Learning Models
Official implementation of "AgentPoison: Red-teaming LLM Agents via Memory or Knowledge Base Backdoor Poisoning". This project explores methods of data poisoning and backdoor insertion in LLM agents to assess their resilience against such attacks.
Research on methods of embedding malicious payloads into deep neural networks.
Investigation of backdoor attacks on deep learning models, focusing on creating undetectable vulnerabilities within models.
Techniques for stealing deep learning models through various attack vectors, enabling adversaries to replicate or access models.
Model extraction without using data, allowing for the recovery of models without access to the original data.
Tool for mapping and analyzing large language models (LLMs), exploring the structure and behavior of various LLMs.
Federated learning pipeline using Google Cloud infrastructure, enabling model training on distributed data.
Attack using ensemble class activation maps to introduce errors in models by manipulating activation maps.
Methods for attacking deep models under various conditions and constraints, focusing on creating more resilient attacks.
Research on adaptive attacks on machine learning models, enabling the creation of attacks that can adapt to model defenses.
Knowledge transfer in zero-shot scenarios, exploring methods to transfer knowledge between models without prior training on target data.
Attack for generating informative labels, aimed at covertly extracting data from trained models.
Enhancing DMI (Data Mining and Integration) methods using additional knowledge to improve accuracy and efficiency.
Research on methods for visualizing and interpreting machine learning models, providing insights into model workings.
Attacks that can be "plugged and played" without needing model modifications, offering flexible and universal attack methods.
Tool for analyzing and processing snapshot data, enabling efficient handling of data snapshots.
Research on the trade-offs between privacy and robustness in models, aiming to balance these two aspects in machine learning.
Methods for data leakage from trained models, exploring ways to extract private information from machine learning models.
Research on blind information extraction attacks, enabling data retrieval without access to the model's internal structure.
Differential privacy methods for deep learning, ensuring data privacy during model training.
Defense methods using MMD-mixup, aimed at improving model robustness against attacks.
Tools for protecting memory from attacks, exploring ways to prevent data leaks from model memory.
Methods for merging and splitting data to improve training, optimizing the use of heterogeneous data in models.
Attacks on face recognition models using attributes, exploring ways to manipulate facial attributes to induce errors.
Attacks on face verification models, aimed at disrupting authentication systems based on face recognition.
Using GANs to create malware, exploring methods for generating malicious code with generative models.
Methods for generating adversarial perturbations using generative models, aimed at introducing errors in deep models.
Adversarial attacks using Relativistic AdvGAN, exploring methods for creating more realistic and effective attacks.
Attacks on large language models, exploring vulnerabilities and protection methods for LLMs.
Safe fine-tuning of large language models, aiming to prevent data leaks and ensure security during LLM tuning.
Methods for evaluating trust in models, exploring ways to determine the reliability and safety of machine learning models.
Benchmark for evaluating prompts, providing tools for testing and optimizing queries to large language models.
Tool for analyzing and evaluating models based on ROM codes, exploring various aspects of model performance and resilience.
Research on privacy in large language models, aiming to protect data and prevent leaks from LLMs.
- MLSecOps
- MLSecOps Podcast
- MITRE ATLAS™ and SLACK COMMUNITY
- MlSecOps communtiy and SLACK COMMUNITY
- MITRE ATLAS™ (Adversarial Threat Landscape for Artificial-Intelligence Systems)
- OWASP AI Exchange
- OWASP Machine Learning Security Top Ten
- OWASP Top 10 for Large Language Model Applications
- OWASP LLMSVS
- OWASP Periodic Table of AI Security
- OWASP SLACK
- Awesome LLM Security
- Hackstery
- PWNAI
- AiSec_X_Feed
- HUNTR Discord community
- AIRSK
- AI Vulnerability Database
- Incident AI Database
- Defcon AI Village CTF
- Awesome AI Security
- MLSecOps Reference Repository
- Awesome LVLM Attack
- Awesome MLLM Safety
- Adversarial AI Attacks, Mitigations, and Defense Strategies: A cybersecurity professional's guide to AI attacks, threat modeling, and securing AI with MLSecOps
- Privacy-Preserving Machine Learning
- Generative AI Security: Theories and Practices (Future of Business and Finance)
All contributions to this list are welcome! Please feel free to submit a pull request with any additions or improvements.
![]() @riccardobiosas |
![]() @badarahmed |
![]() @deadbits |
![]() @wearetyomsmnv |
![]() @anmorgan24 |
![]() @mik0w |
![]() @alexcombessie |
If you find this project useful, please consider giving it a star ⭐️
This project is licensed under the MIT License - see the LICENSE file for details.
Made with ❤️
For Tasks:
Click tags to check more tools for each tasksFor Jobs:
Alternative AI tools for awesome-MLSecOps
Similar Open Source Tools
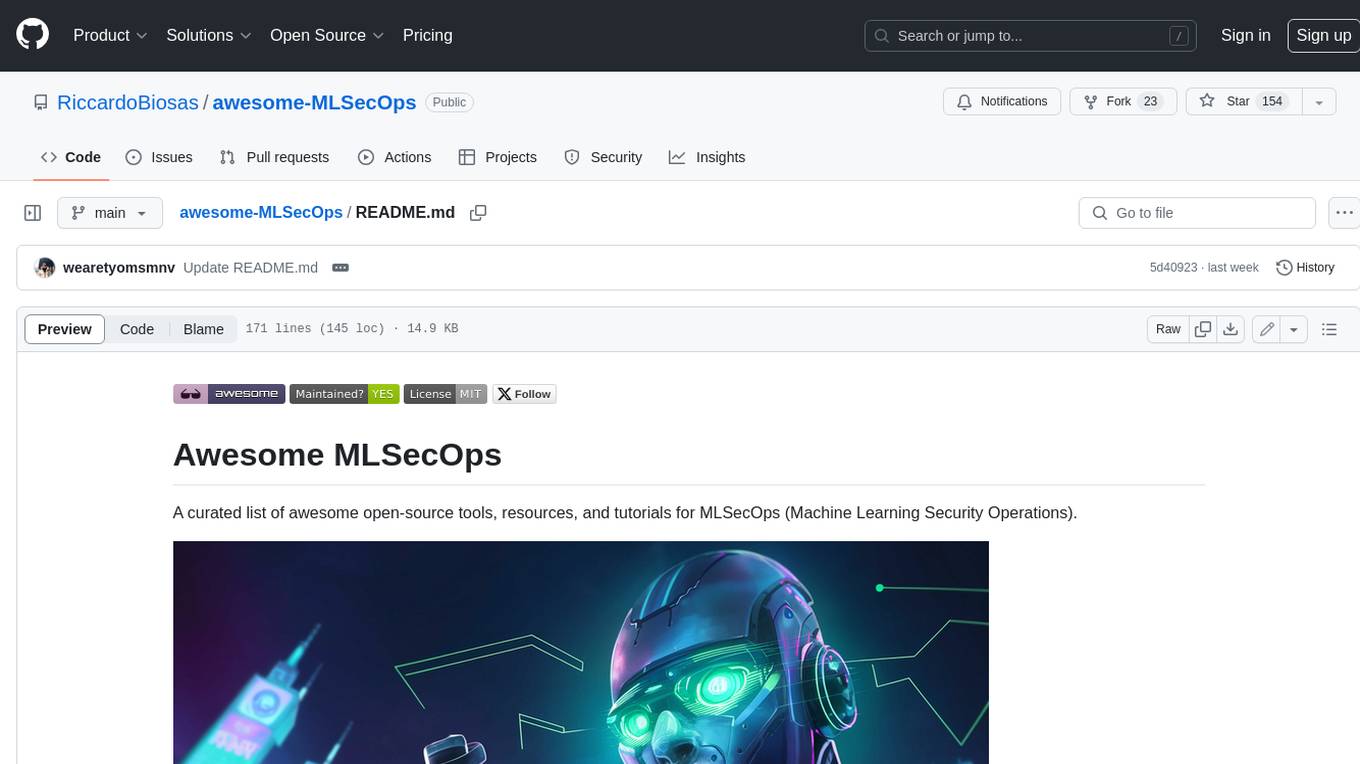
awesome-MLSecOps
Awesome MLSecOps is a curated list of open-source tools, resources, and tutorials for MLSecOps (Machine Learning Security Operations). It includes a wide range of security tools and libraries for protecting machine learning models against adversarial attacks, as well as resources for AI security, data anonymization, model security, and more. The repository aims to provide a comprehensive collection of tools and information to help users secure their machine learning systems and infrastructure.
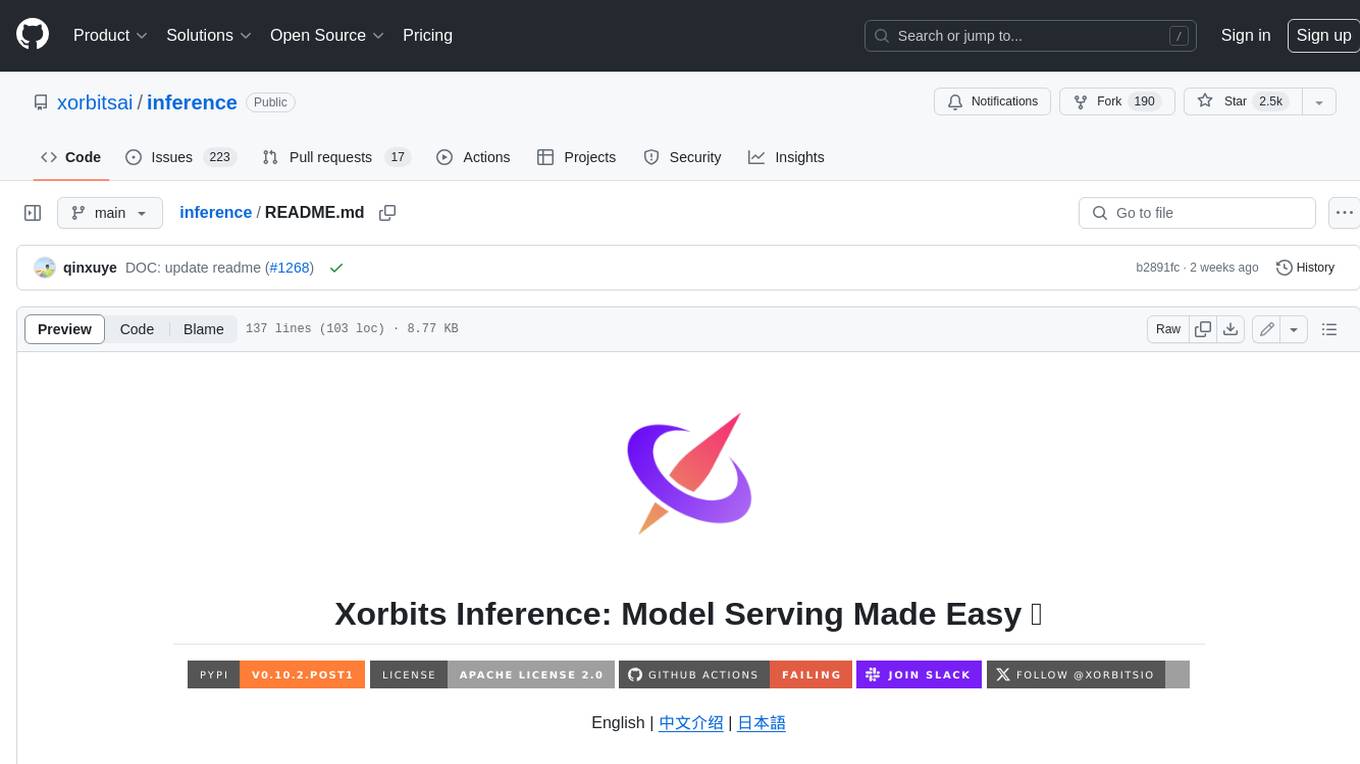
inference
Xorbits Inference (Xinference) is a powerful and versatile library designed to serve language, speech recognition, and multimodal models. With Xorbits Inference, you can effortlessly deploy and serve your or state-of-the-art built-in models using just a single command. Whether you are a researcher, developer, or data scientist, Xorbits Inference empowers you to unleash the full potential of cutting-edge AI models.
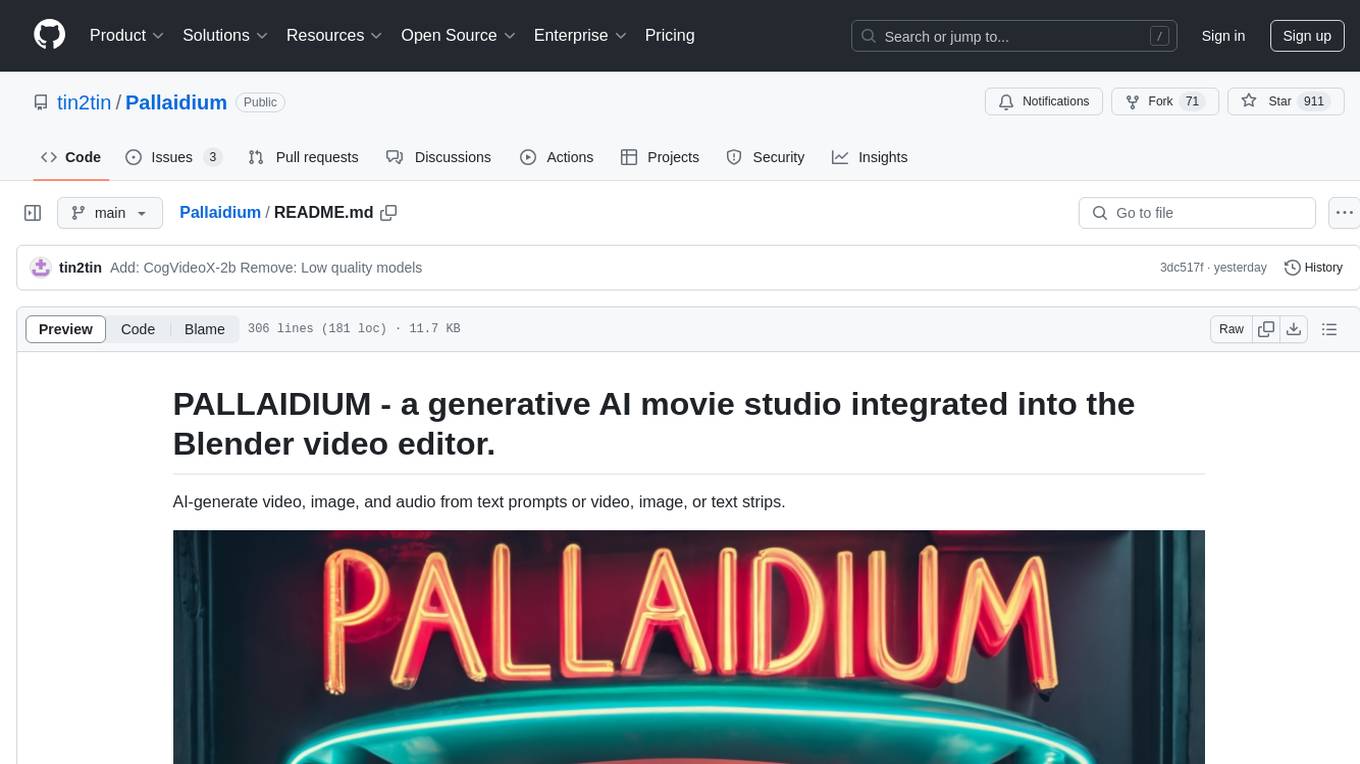
Pallaidium
Pallaidium is a generative AI movie studio integrated into the Blender video editor. It allows users to AI-generate video, image, and audio from text prompts or existing media files. The tool provides various features such as text to video, text to audio, text to speech, text to image, image to image, image to video, video to video, image to text, and more. It requires a Windows system with a CUDA-supported Nvidia card and at least 6 GB VRAM. Pallaidium offers batch processing capabilities, text to audio conversion using Bark, and various performance optimization tips. Users can install the tool by downloading the add-on and following the installation instructions provided. The tool comes with a set of restrictions on usage, prohibiting the generation of harmful, pornographic, violent, or false content.
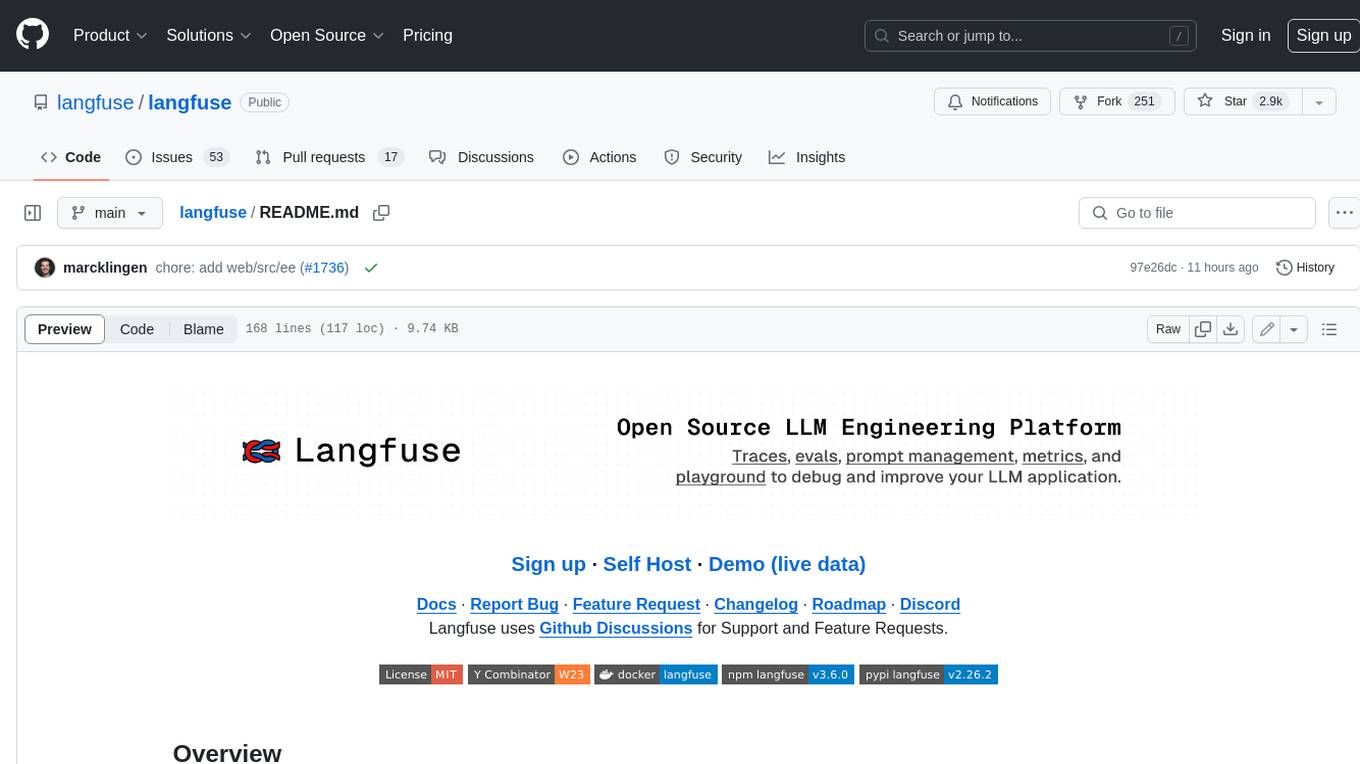
langfuse
Langfuse is a powerful tool that helps you develop, monitor, and test your LLM applications. With Langfuse, you can: * **Develop:** Instrument your app and start ingesting traces to Langfuse, inspect and debug complex logs, and manage, version, and deploy prompts from within Langfuse. * **Monitor:** Track metrics (cost, latency, quality) and gain insights from dashboards & data exports, collect and calculate scores for your LLM completions, run model-based evaluations, collect user feedback, and manually score observations in Langfuse. * **Test:** Track and test app behaviour before deploying a new version, test expected in and output pairs and benchmark performance before deploying, and track versions and releases in your application. Langfuse is easy to get started with and offers a generous free tier. You can sign up for Langfuse Cloud or deploy Langfuse locally or on your own infrastructure. Langfuse also offers a variety of integrations to make it easy to connect to your LLM applications.
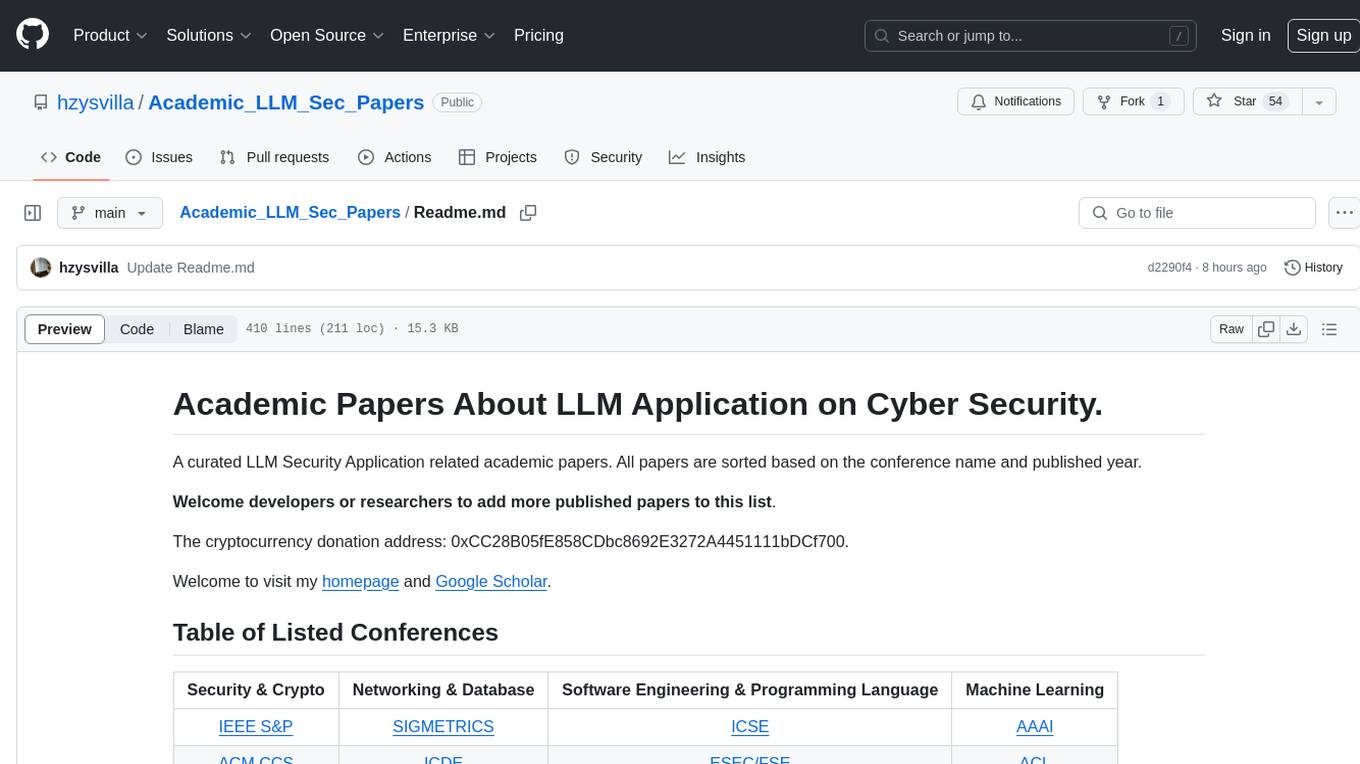
Academic_LLM_Sec_Papers
Academic_LLM_Sec_Papers is a curated collection of academic papers related to LLM Security Application. The repository includes papers sorted by conference name and published year, covering topics such as large language models for blockchain security, software engineering, machine learning, and more. Developers and researchers are welcome to contribute additional published papers to the list. The repository also provides information on listed conferences and journals related to security, networking, software engineering, and cryptography. The papers cover a wide range of topics including privacy risks, ethical concerns, vulnerabilities, threat modeling, code analysis, fuzzing, and more.
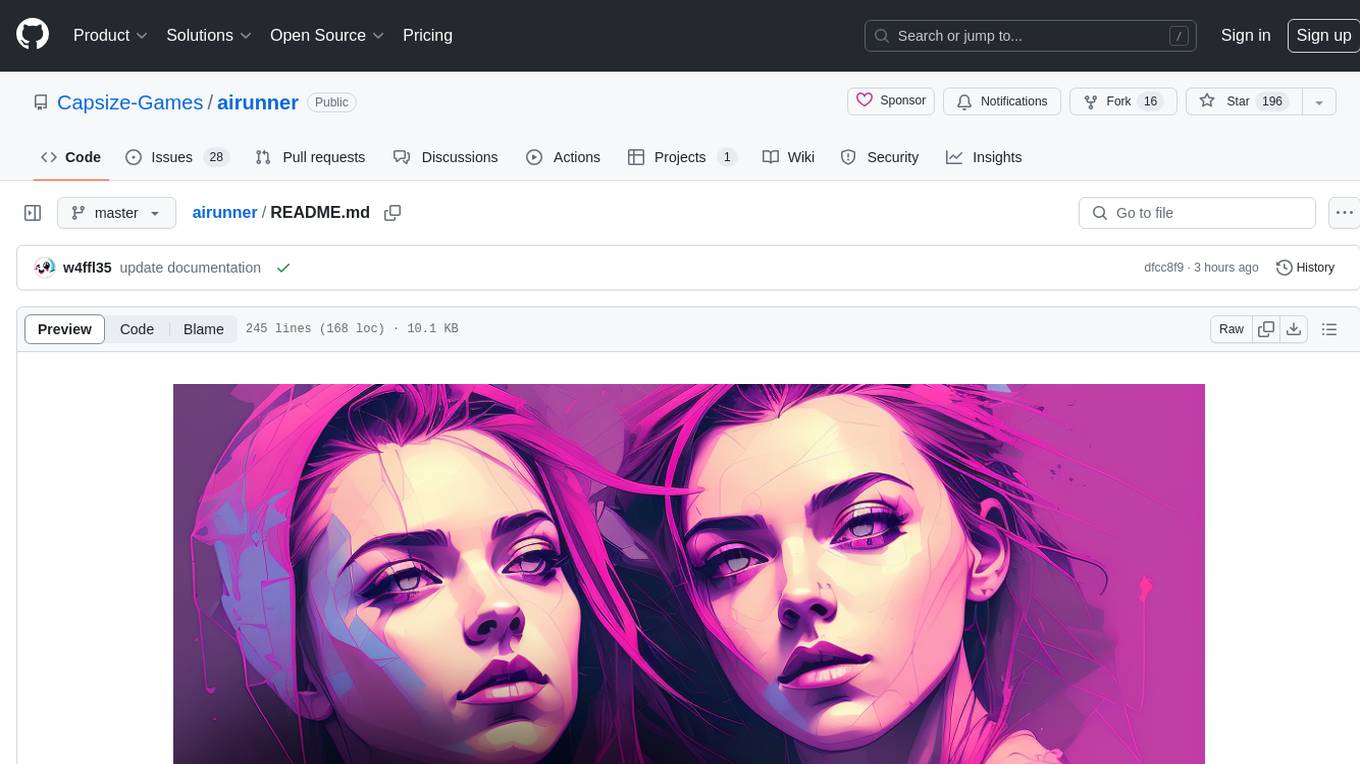
airunner
AI Runner is a multi-modal AI interface that allows users to run open-source large language models and AI image generators on their own hardware. The tool provides features such as voice-based chatbot conversations, text-to-speech, speech-to-text, vision-to-text, text generation with large language models, image generation capabilities, image manipulation tools, utility functions, and more. It aims to provide a stable and user-friendly experience with security updates, a new UI, and a streamlined installation process. The application is designed to run offline on users' hardware without relying on a web server, offering a smooth and responsive user experience.
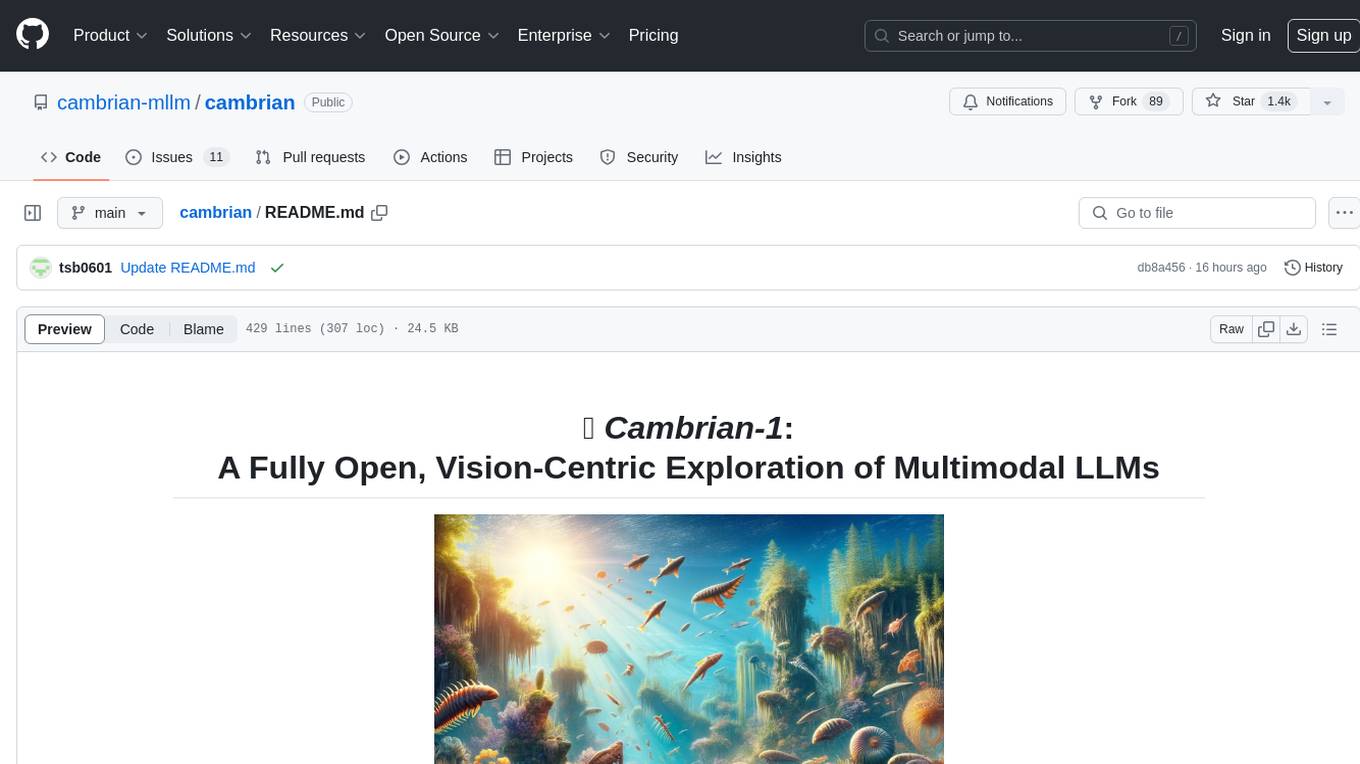
cambrian
Cambrian-1 is a fully open project focused on exploring multimodal Large Language Models (LLMs) with a vision-centric approach. It offers competitive performance across various benchmarks with models at different parameter levels. The project includes training configurations, model weights, instruction tuning data, and evaluation details. Users can interact with Cambrian-1 through a Gradio web interface for inference. The project is inspired by LLaVA and incorporates contributions from Vicuna, LLaMA, and Yi. Cambrian-1 is licensed under Apache 2.0 and utilizes datasets and checkpoints subject to their respective original licenses.
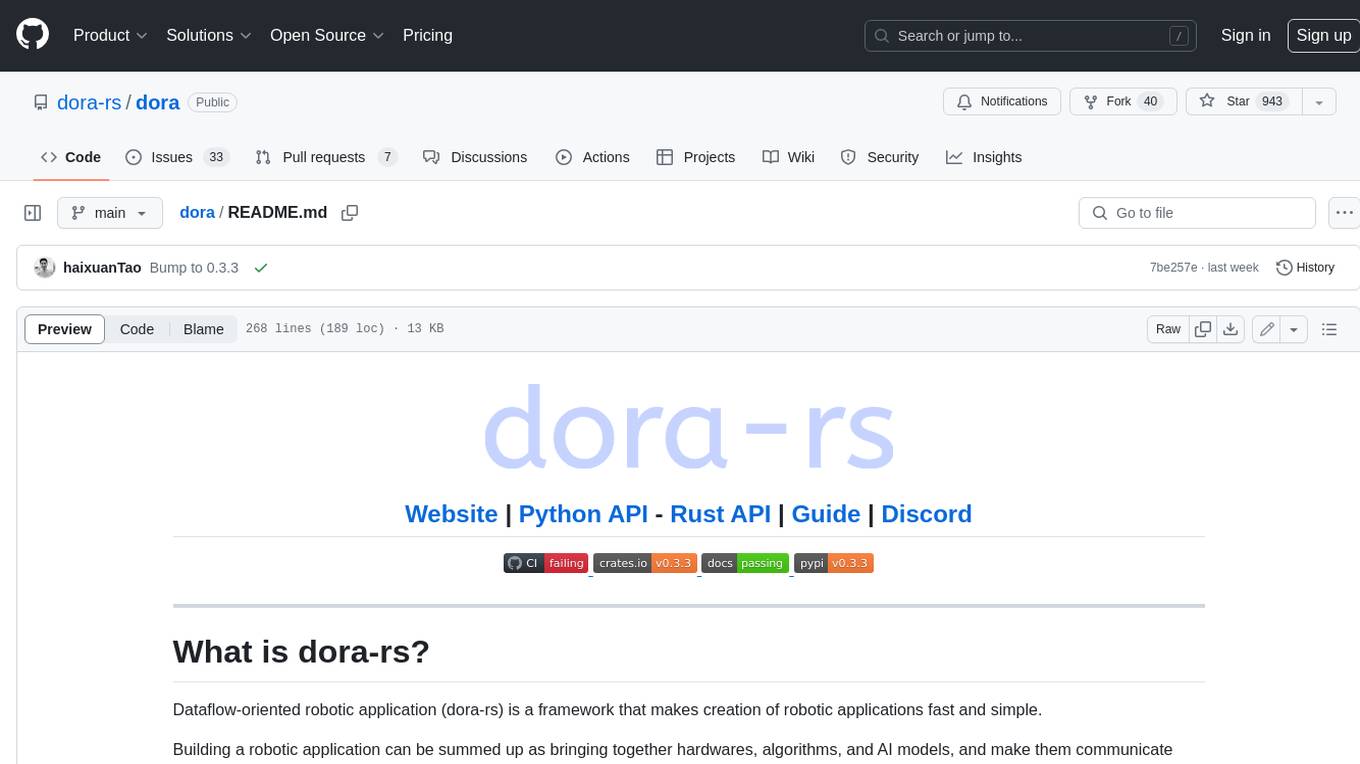
dora
Dataflow-oriented robotic application (dora-rs) is a framework that makes creation of robotic applications fast and simple. Building a robotic application can be summed up as bringing together hardwares, algorithms, and AI models, and make them communicate with each others. At dora-rs, we try to: make integration of hardware and software easy by supporting Python, C, C++, and also ROS2. make communication low latency by using zero-copy Arrow messages. dora-rs is still experimental and you might experience bugs, but we're working very hard to make it stable as possible.
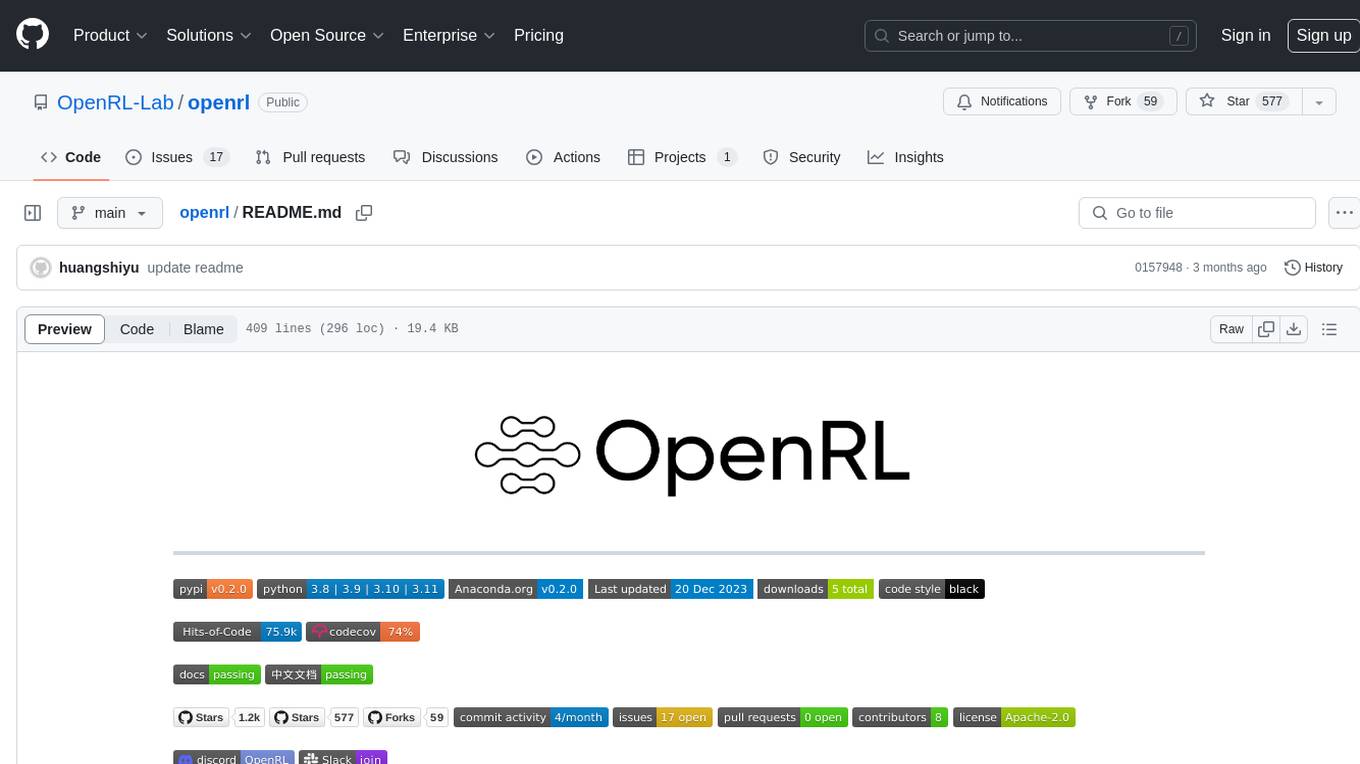
openrl
OpenRL is an open-source general reinforcement learning research framework that supports training for various tasks such as single-agent, multi-agent, offline RL, self-play, and natural language. Developed based on PyTorch, the goal of OpenRL is to provide a simple-to-use, flexible, efficient and sustainable platform for the reinforcement learning research community. It supports a universal interface for all tasks/environments, single-agent and multi-agent tasks, offline RL training with expert dataset, self-play training, reinforcement learning training for natural language tasks, DeepSpeed, Arena for evaluation, importing models and datasets from Hugging Face, user-defined environments, models, and datasets, gymnasium environments, callbacks, visualization tools, unit testing, and code coverage testing. It also supports various algorithms like PPO, DQN, SAC, and environments like Gymnasium, MuJoCo, Atari, and more.
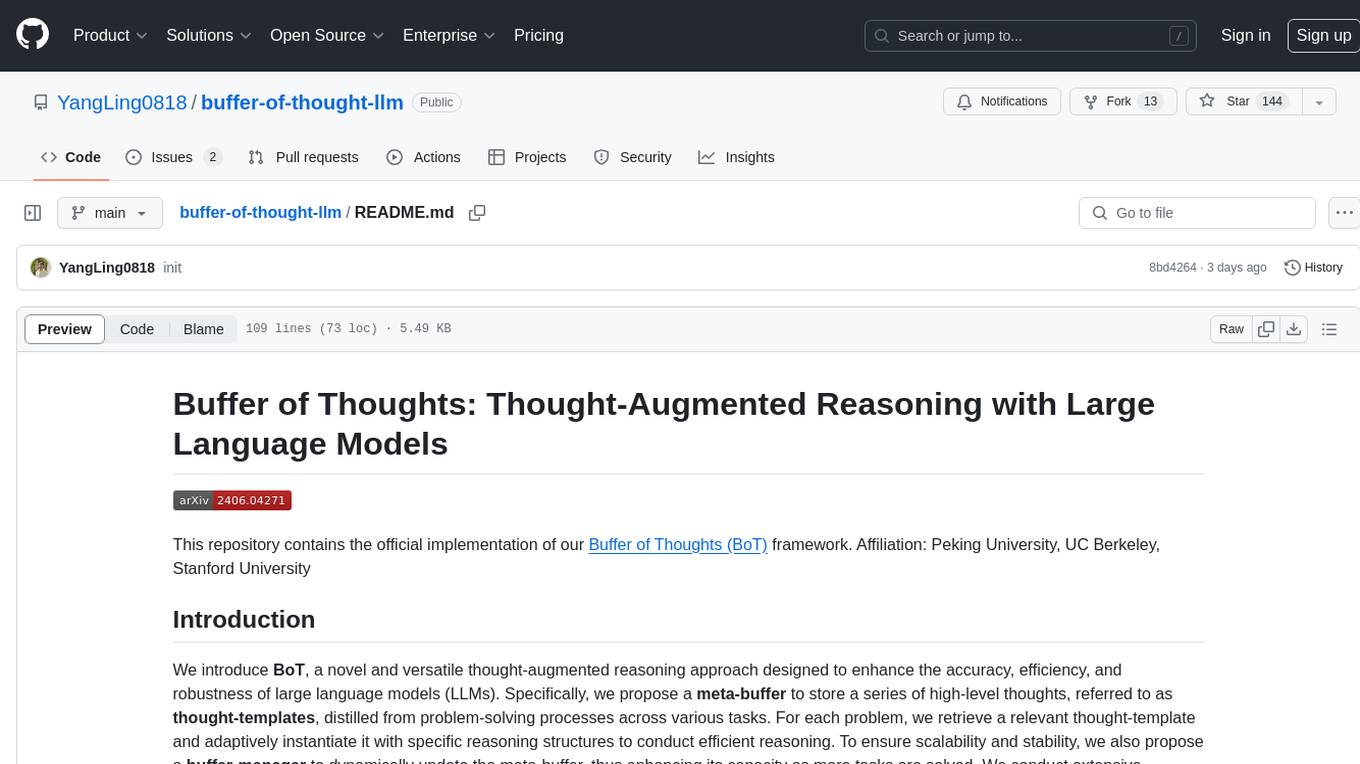
buffer-of-thought-llm
Buffer of Thoughts (BoT) is a thought-augmented reasoning framework designed to enhance the accuracy, efficiency, and robustness of large language models (LLMs). It introduces a meta-buffer to store high-level thought-templates distilled from problem-solving processes, enabling adaptive reasoning for efficient problem-solving. The framework includes a buffer-manager to dynamically update the meta-buffer, ensuring scalability and stability. BoT achieves significant performance improvements on reasoning-intensive tasks and demonstrates superior generalization ability and robustness while being cost-effective compared to other methods.
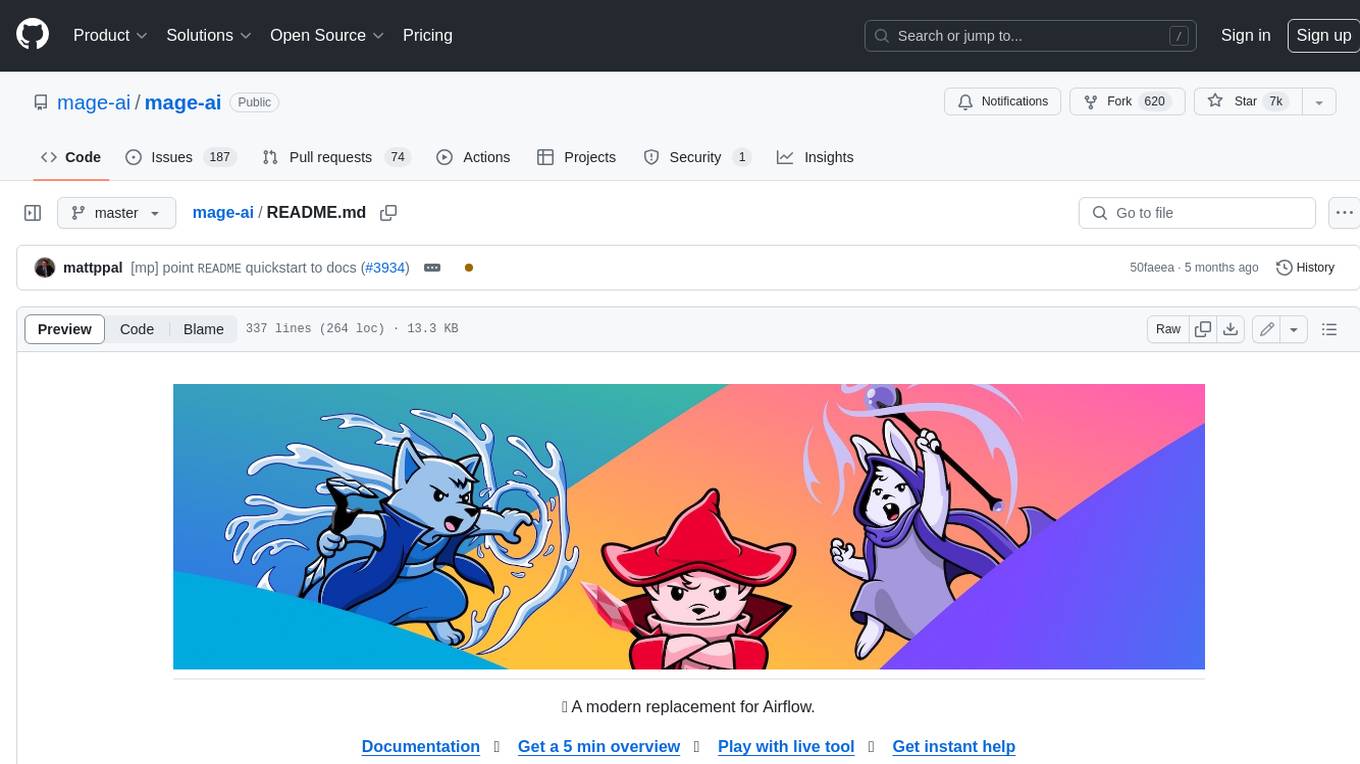
mage-ai
Mage is an open-source data pipeline tool for transforming and integrating data. It offers an easy developer experience, engineering best practices built-in, and data as a first-class citizen. Mage makes it easy to build, preview, and launch data pipelines, and provides observability and scaling capabilities. It supports data integrations, streaming pipelines, and dbt integration.
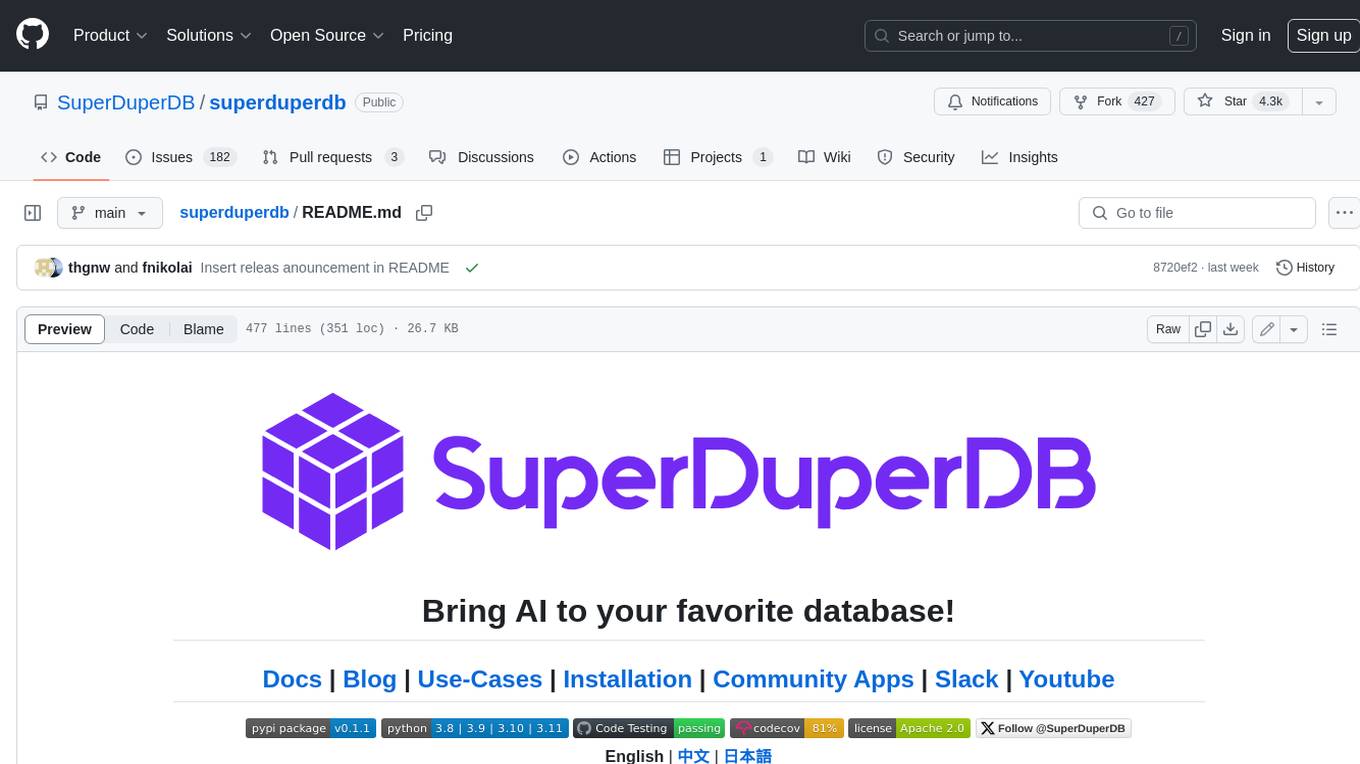
superduperdb
SuperDuperDB is a Python framework for integrating AI models, APIs, and vector search engines directly with your existing databases, including hosting of your own models, streaming inference and scalable model training/fine-tuning. Build, deploy and manage any AI application without the need for complex pipelines, infrastructure as well as specialized vector databases, and moving our data there, by integrating AI at your data's source: - Generative AI, LLMs, RAG, vector search - Standard machine learning use-cases (classification, segmentation, regression, forecasting recommendation etc.) - Custom AI use-cases involving specialized models - Even the most complex applications/workflows in which different models work together SuperDuperDB is **not** a database. Think `db = superduper(db)`: SuperDuperDB transforms your databases into an intelligent platform that allows you to leverage the full AI and Python ecosystem. A single development and deployment environment for all your AI applications in one place, fully scalable and easy to manage.
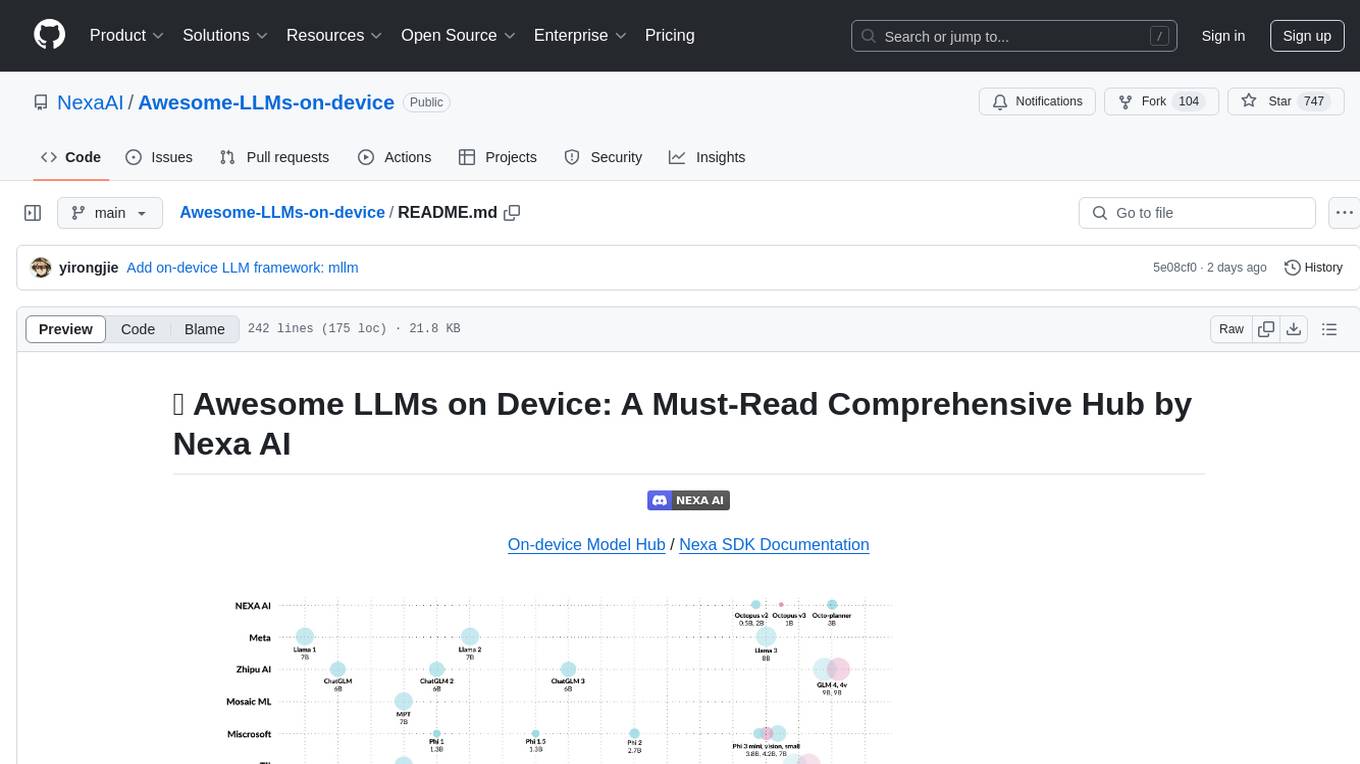
Awesome-LLMs-on-device
Welcome to the ultimate hub for on-device Large Language Models (LLMs)! This repository is your go-to resource for all things related to LLMs designed for on-device deployment. Whether you're a seasoned researcher, an innovative developer, or an enthusiastic learner, this comprehensive collection of cutting-edge knowledge is your gateway to understanding, leveraging, and contributing to the exciting world of on-device LLMs.
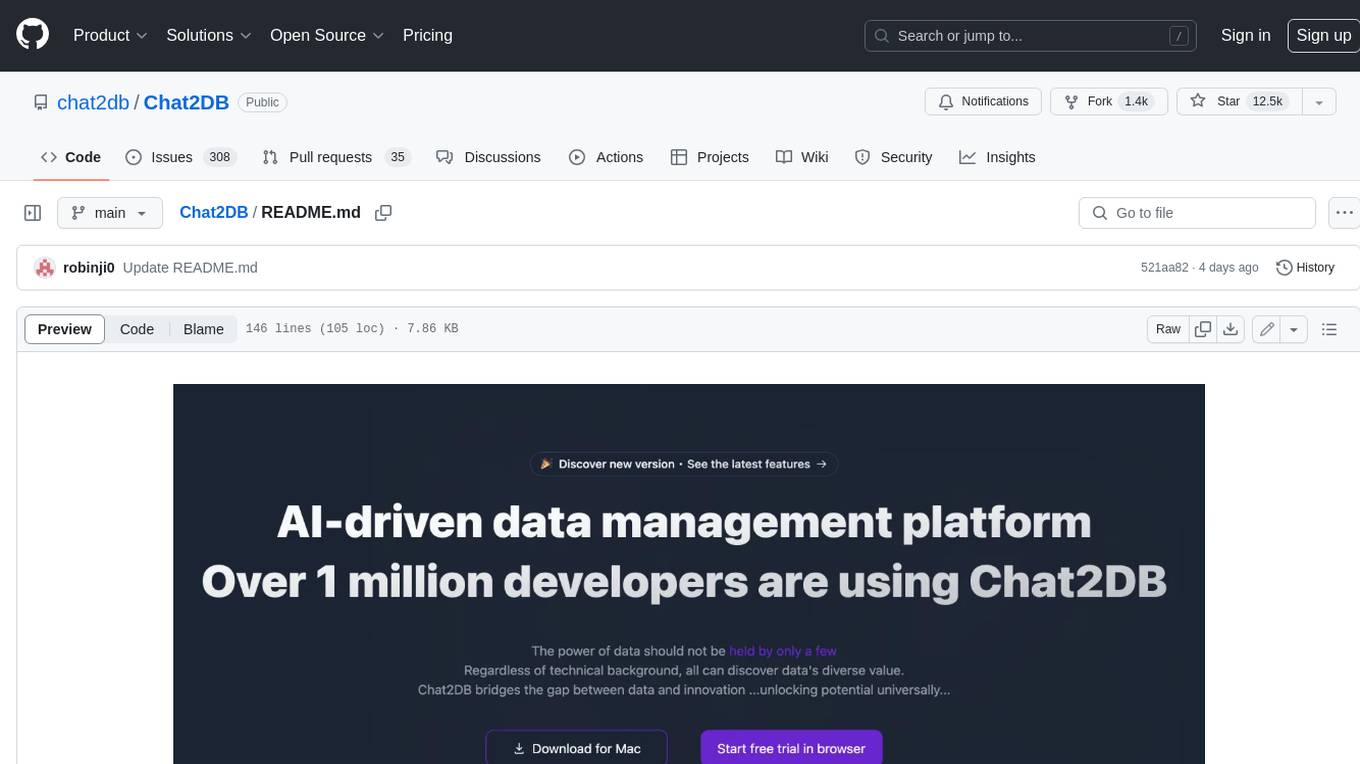
Chat2DB
Chat2DB is an AI-driven data development and analysis platform that enables users to communicate with databases using natural language. It supports a wide range of databases, including MySQL, PostgreSQL, Oracle, SQLServer, SQLite, MariaDB, ClickHouse, DM, Presto, DB2, OceanBase, Hive, KingBase, MongoDB, Redis, and Snowflake. Chat2DB provides a user-friendly interface that allows users to query databases, generate reports, and explore data using natural language commands. It also offers a variety of features to help users improve their productivity, such as auto-completion, syntax highlighting, and error checking.
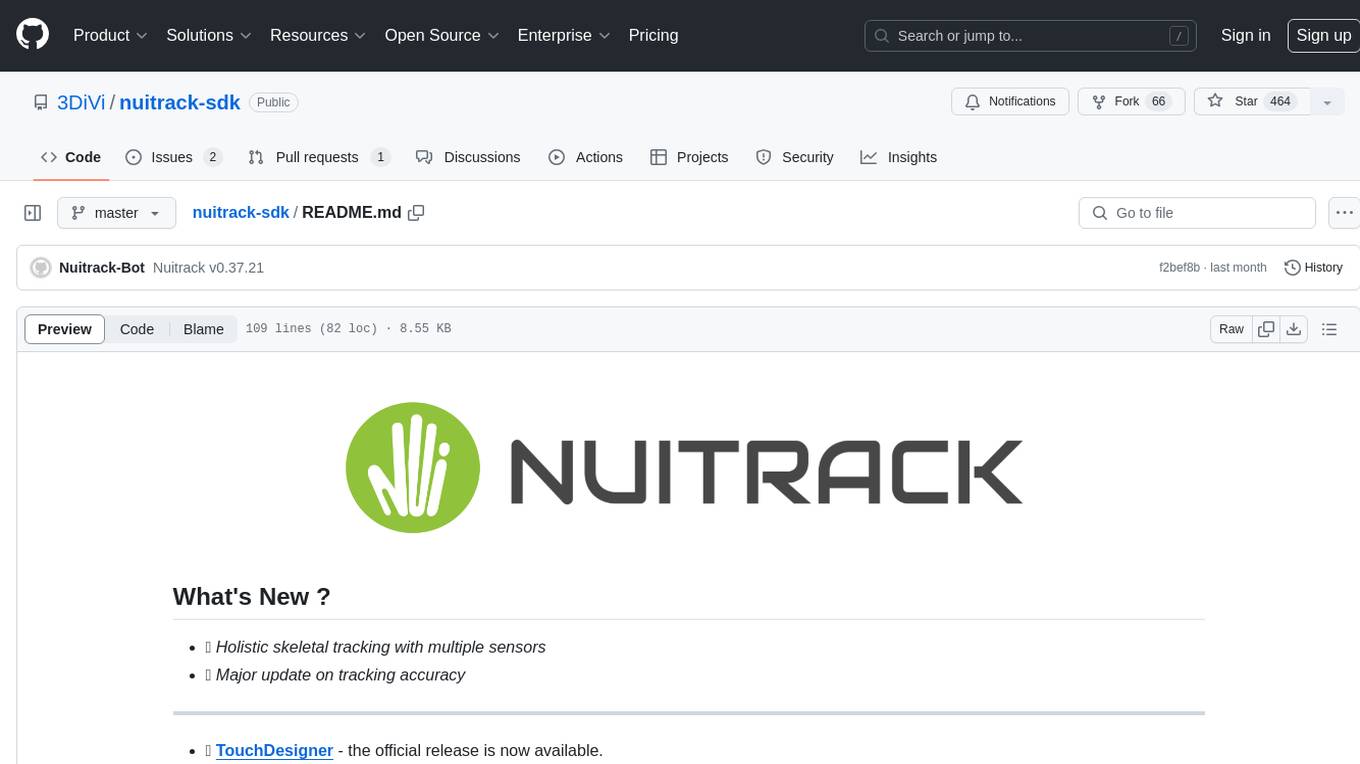
nuitrack-sdk
Nuitrack™ is an ultimate 3D body tracking solution developed by 3DiVi Inc. It enables body motion analytics applications for virtually any widespread depth sensors and hardware platforms, supporting a wide range of applications from real-time gesture recognition on embedded platforms to large-scale multisensor analytical systems. Nuitrack provides highly-sophisticated 3D skeletal tracking, basic facial analysis, hand tracking, and gesture recognition APIs for UI control. It offers two skeletal tracking engines: classical for embedded hardware and AI for complex poses, providing a human-centric spatial understanding tool for natural and intelligent user engagement.
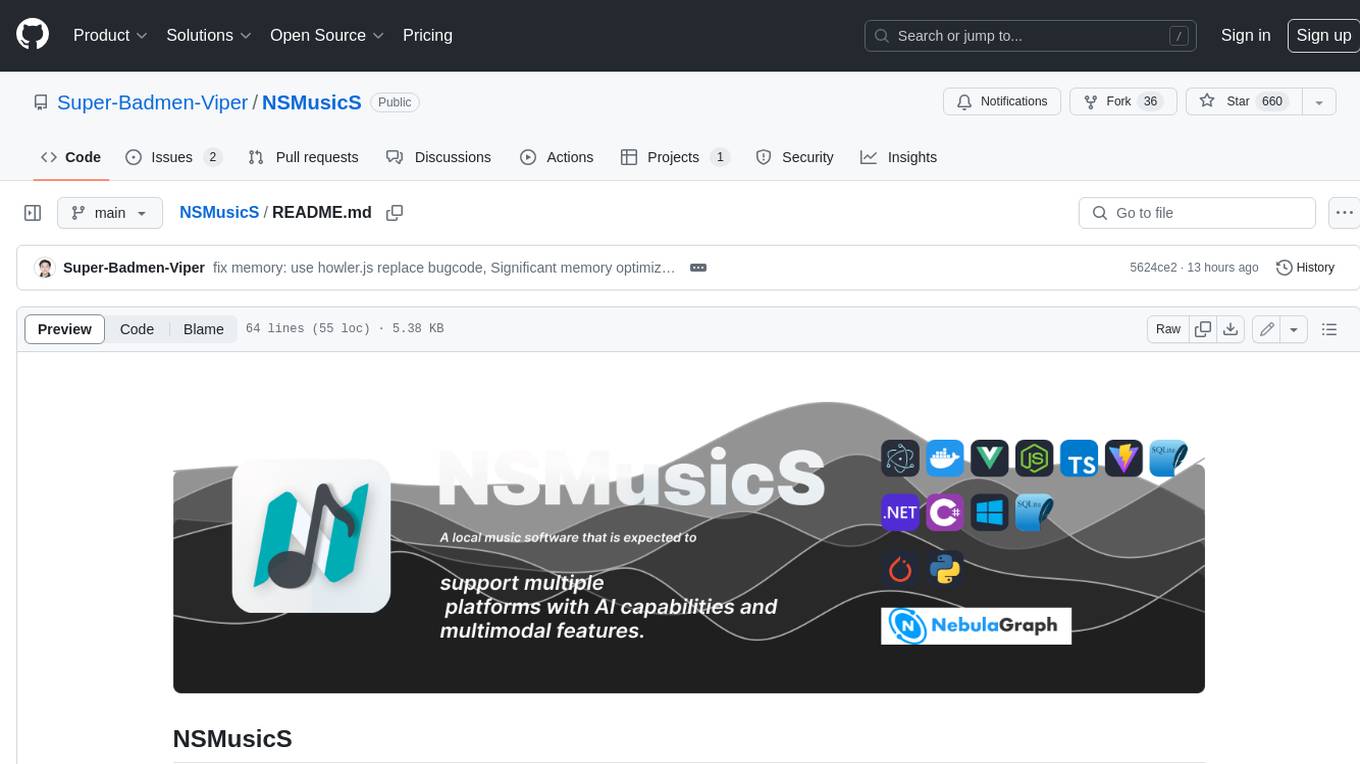
NSMusicS
NSMusicS is a local music software that is expected to support multiple platforms with AI capabilities and multimodal features. The goal of NSMusicS is to integrate various functions (such as artificial intelligence, streaming, music library management, cross platform, etc.), which can be understood as similar to Navidrome but with more features than Navidrome. It wants to become a plugin integrated application that can almost have all music functions.
For similar tasks
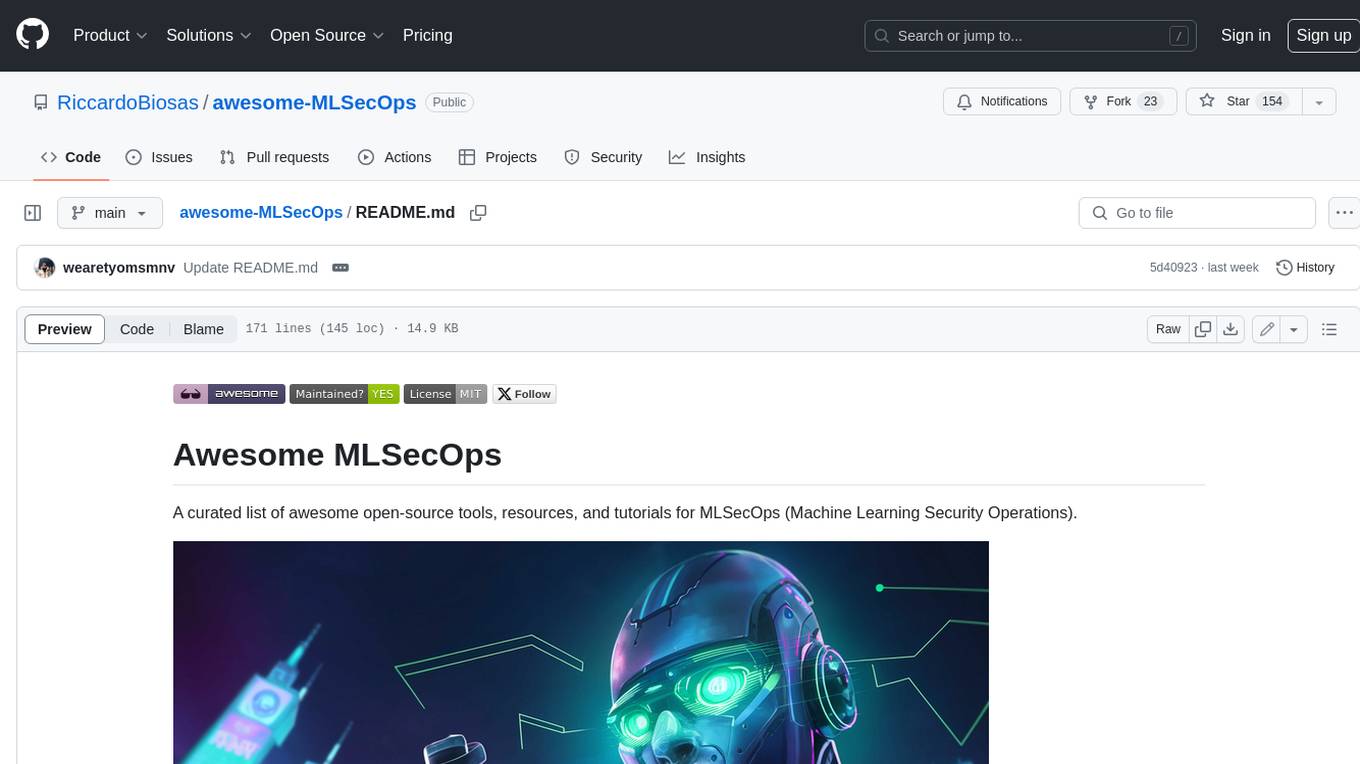
awesome-MLSecOps
Awesome MLSecOps is a curated list of open-source tools, resources, and tutorials for MLSecOps (Machine Learning Security Operations). It includes a wide range of security tools and libraries for protecting machine learning models against adversarial attacks, as well as resources for AI security, data anonymization, model security, and more. The repository aims to provide a comprehensive collection of tools and information to help users secure their machine learning systems and infrastructure.
For similar jobs
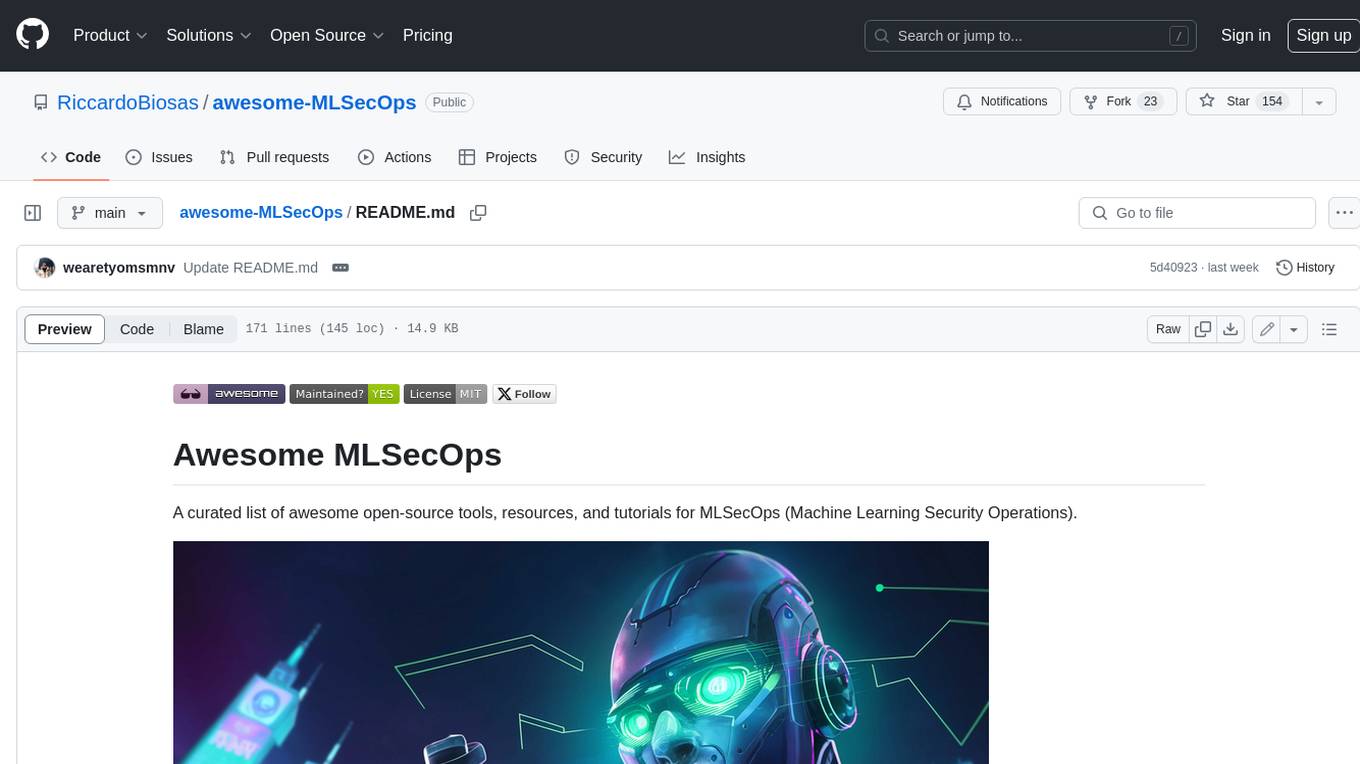
awesome-MLSecOps
Awesome MLSecOps is a curated list of open-source tools, resources, and tutorials for MLSecOps (Machine Learning Security Operations). It includes a wide range of security tools and libraries for protecting machine learning models against adversarial attacks, as well as resources for AI security, data anonymization, model security, and more. The repository aims to provide a comprehensive collection of tools and information to help users secure their machine learning systems and infrastructure.
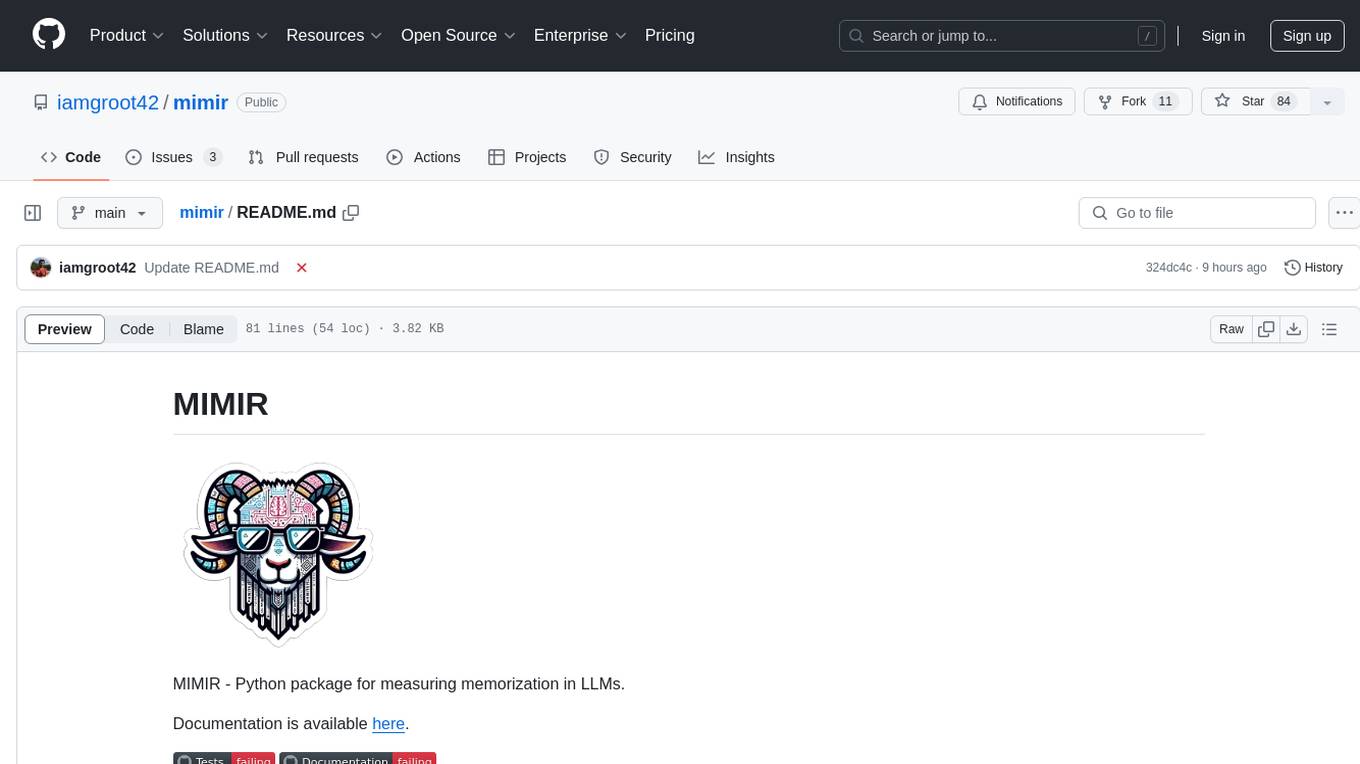
mimir
MIMIR is a Python package designed for measuring memorization in Large Language Models (LLMs). It provides functionalities for conducting experiments related to membership inference attacks on LLMs. The package includes implementations of various attacks such as Likelihood, Reference-based, Zlib Entropy, Neighborhood, Min-K% Prob, Min-K%++, Gradient Norm, and allows users to extend it by adding their own datasets and attacks.
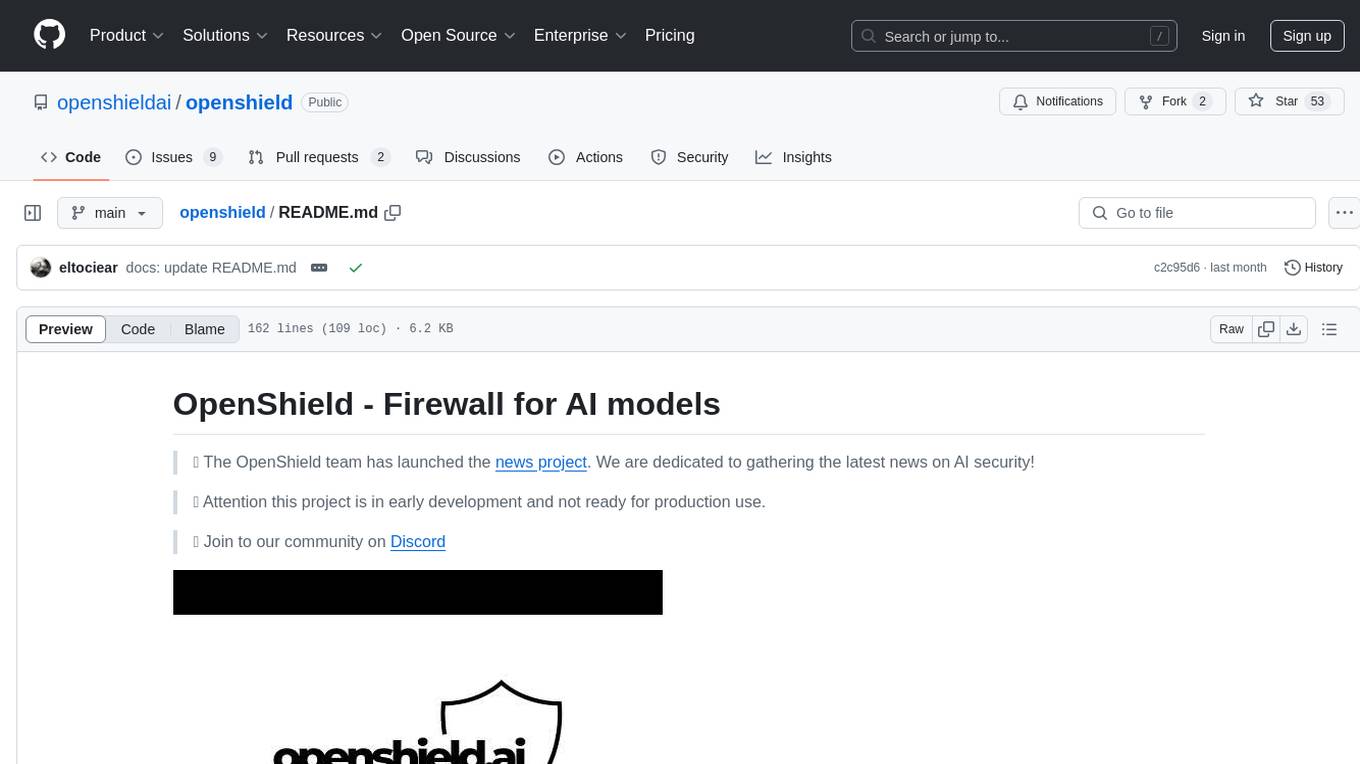
openshield
OpenShield is a firewall designed for AI models to protect against various attacks such as prompt injection, insecure output handling, training data poisoning, model denial of service, supply chain vulnerabilities, sensitive information disclosure, insecure plugin design, excessive agency granting, overreliance, and model theft. It provides rate limiting, content filtering, and keyword filtering for AI models. The tool acts as a transparent proxy between AI models and clients, allowing users to set custom rate limits for OpenAI endpoints and perform tokenizer calculations for OpenAI models. OpenShield also supports Python and LLM based rules, with upcoming features including rate limiting per user and model, prompts manager, content filtering, keyword filtering based on LLM/Vector models, OpenMeter integration, and VectorDB integration. The tool requires an OpenAI API key, Postgres, and Redis for operation.