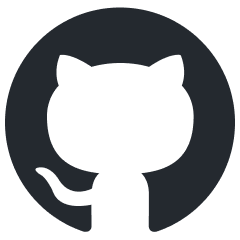
Next-Gen-Dialogue
AI powered dialogue visual designer for Unity
Stars: 89
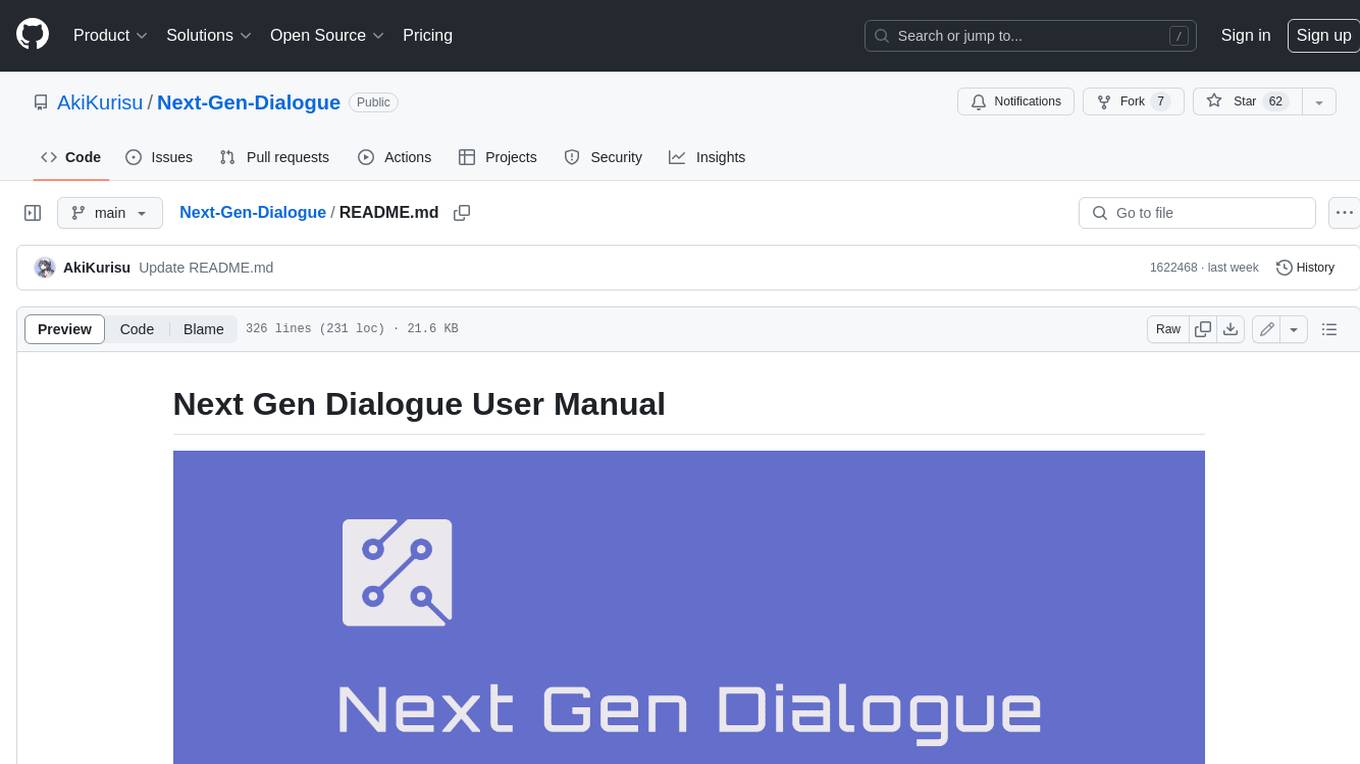
Next Gen Dialogue is a Unity dialogue plugin that combines traditional dialogue design with AI techniques. It features a visual dialogue editor, modular dialogue functions, AIGC support for generating dialogue at runtime, AIGC baking dialogue in Editor, and runtime debugging. The plugin aims to provide an experimental approach to dialogue design using large language models. Users can create dialogue trees, generate dialogue content using AI, and bake dialogue content in advance. The tool also supports localization, VITS speech synthesis, and one-click translation. Users can create dialogue by code using the DialogueSystem and DialogueTree components.
README:
Next Gen Dialogue is a Unity dialogue plugin combined with large language model design, won the 2023 Unity AI Plugin Excellence Award from Unity China.
It combines the traditional dialogue design pattern with AIGC to simlify your workflow. Hopes you enjoy it.

Demo video https://www.bilibili.com/video/BV1hg4y1U7FG
- Features
- Supported version
- Install
- Quick Start
- Nodes
- Modules
- Extensions
- Resolvers
- Create Dialogue in Script
- Visual dialogue editor
- Modular dialogue function
- AIGC dialogue
- Custom actions support
- Unity 2022.3 or Later
Using git URL to download package by Unity PackageManager https://github.com/AkiKurisu/Next-Gen-Dialogue.git
"dependencies": {
"com.kurisu.chris": "1.2.4",
"com.kurisu.chris-modules": "1.2.4",
"com.kurisu.ceres": "0.3.2",
"com.unity.nuget.newtonsoft-json": "3.2.1"
}
The experimental features of Next Gen Dialogue are placed in the Modules folder and will not be enabled without installing the corresponding dependencies.
You can view the dependencies in the README.md
document under its folder.
If you are using this plugin for the first time, it is recommended to play the following example scenes first:
1.Normal Usage.unity
this scene contains the use of NextGenDialogueComponent and NextGenDialogueGraphAsset;
2.Editor Bake Dialogue.unity
this scene contains the sample of baking conversation conversation in the use of AI dialogue Baker in Editor;
3.Build Dialogue by Code.unity
this scene contains the use of Code to generate dialogue.
4.Bake Novel.unity
An example of using ChatGPT to infinitely generate dialogue trees.
NextGenDialogueComponent
and NextGenDialogueGraphAsset
are used to store dialogue data. In order to facilitate understanding, it is collectively referred to as dialogue tree.
The following process is to create a dialogue tree that contains only a single dialogue and a single option:
-
Mount
NextGenDialogueComponent
on any gameObject -
Click
Open Dialogue Graph
to enter the editor -
Create the Container/dialogue node, the node is the dialogue container used in the game
-
Connect the Parent port of the dialogue Node to the root node. You can have multiple dialogue in one dialogueTree, but only those connected to the root node will be used.
-
Create the Container/Piece node and create our first dialogue fragment
-
Right -click Piece node
Add Module
addContent Module
, you can fill in the contents of the conversation inContent
-
Create a Container/Option node and create a dialogue option corresponding to the PIECE node
-
Right-click Piece node
Add Option
, connect Piece with Option -
Very important: At least one Piece node needs to be added to the Dialogue as the first piece of the dialogue. You can click
Collect All Pieces
in context menu to collect all pieces in the Graph to the dialogue and adjust priority of them.- For priority, please refer to General Module-Condition Module
-
Click on the upper left of the editor's
Save
to save dialogue -
Click Play to enter PlayMode
-
Click on NextGenDialogueComponent
Play dialogue
to play conversation -
Click
Open Dialogue Graph
to enter the debug mode
The playing dialogue piece will be displayed in green
From V2, Next Gen Dialogue now use Ceres.Flow
to implement custom action feature.
You can now add ExecuteFlowModule
to fire a flow execution event at runtime.
For more details about Ceres.Flow
, please refer to AkiKurisu/Ceres.
You can use AI dialogue Baker to bake the dialogue content generated by AI in advance when designing the dialogue tree, so as to improve the workflow efficiency without affecting your design framework.
- The basic dialogue graph design is consistent with the process of Create a Dialogue Graph
- Add
AI Bake Module
for the fragments or options that need to be baked, and remove the module for nodes that do not need to be baked - Select the type of LLM you are baking with
- Select in turn the nodes that AI dialogue Baker needs to recognize, the order of recognition is based on the order selected by the mouse, and finally select the nodes that need to be baked
- If the selection is successful, you can see the preview input content at the bottom of the editor
- Click the
Bake Dialogue
button on theAI Bake Module
and wait for the AI response - After the language model responds, a
Content Module
will be automatically added to the node to store the baked dialogue content - You can continuously generate conversations based on your needs
Different from talking directly to AI in baking dialogue, novel mode allows AI to play the role of copywriter and planner to write dialogue, so it can control options and fragments more precisely. Please refer to the example: 4.Bake Novel.unity
NGD use node based visual editor framework, most of the features are presented through nodes. The construction dialogue are divided into the following parts in NGD:
Name | Description |
---|---|
Dialogue | Used to define dialogues, such as the first piece of the dialogue and other attributes |
Piece | dialogue piece, usually store the core dialogue content |
Option | dialogue options, usually used for interaction and bridging dialogues |
In addition to the above nodes, a more flexible concept is used in NGD, that is, Module. You can use Module to change the output form of the dialogue, such as Google translation, localization, add callbacks, or be executed as a markup.
The following are built-in general modules:
Name | Description |
---|---|
Content | Provide text content for Option or Piece |
TargetID | Add jumping target dialogue fragments for Option |
PreUpdate | Add pre-update behavior for Container, it will update when jumping to the Container |
Callback | Add callback behavior for Option, they will be updated after selection |
Condition | Add judgment behavior for Option or Piece, it will be updated when jumping to the Container, if the return value is Status.Failure , the Container will be discarded. If it is the first Piece of the dialogue, the system will try to jump to the next Piece according to the order in which the Pieces are placed in the dialogue |
Next Piece | Add the next dialogue segment after the end of the Piece. If there is no option, it will jump to the specified dialogue segment after playing the content of the Piece |
Google Translate | Use Google Translate to translate the content of current Option or Piece |
The following are the built-in AIGC modules:
Name | Description |
---|---|
Prompt | Prompt words that provide the basis for subsequent dialogue generation |
Editor modules are used to provide some useful tools for the editor, such as translation.
Add Editor/EditorTranslateModule in the Dialogue node, set the source language (sourceLanguageCode
) and target language (targetLanguageCode
) of the translation, right-click and select Translate All Contents
to perform all Piece and Option with ContentModule
translate.
For nodes other than ContentModule
, if the TranslateEntryAttribute
is added to the field, you can right-click a single node to translate it.
public class ExampleAction : Action
{
//Notify field can be translated
//* Only work for SharedString and string
[SerializeField, Multiline, TranslateEntry]
private SharedString value;
}
The following are extensions, you need to install the corresponding Package or configure the corresponding environment before use:
Based on the UnityEngine.Localization plugin to support the localization of dialogue
Name | Description |
---|---|
Localized Content | Provide content for Option or Piece after getting text from localization |
For VITS local deployment, please refer to this repository: VITS Simple API
If you want to use the VITS module, please use it with VITSAIReResolver. For the use of the Resolver, please refer to the following Resolver
Name | Description |
---|---|
VITS Voice | Use VITS speech synthesis model to generate language for Piece or Option in real time |
Before use, you need to install the corresponding dependencies of Modules/VITS
and open the local VITS server (refer to Modules/VITS/README.md
). Add AIGC/VITSModule
to the node where speech needs to be generated, right-click and select Bake Audio
If you are satisfied with the generated audio, click Download
to save it locally to complete the baking, otherwise the audio file will not be retained after exiting the editor.
It is no longer necessary to start the VITS server at runtime after baking is complete.
- If the AudioClip field is empty, the run generation mode is enabled by default. If there is no connection, the conversation may not proceed. If you only need to use the baking function, please keep the AudioClip field not empty at all times.
Resolver is used to detect the Module in the Container at runtime and execute a series of preset logic such as injecting dependencies and executing behaviors, the difference between NGD's built-in Resolver is as follows:
Name | Description |
---|---|
Default Resolver | The most basic resolver, supporting all built-in common modules |
VITS Resolver | Additionally detect VITS modules to generate voice in real time |
-
In-scene Global Resolver You can mount the
VITSSetup
script on any GameObject to enable AIResolver in the scene -
Dialogue specified Resolver
You can add
VITSResolverModule
to the dialogue node to specify the resolver used by the dialogue, and you can also click the Setting button in the upper right corner of the module and select which Resolvers to be replaced inAdvanced Settings
NGD is divided into two parts, DialogueSystem and DialogueGraph. The former defines the data structure of the dialogue, which is interpreted by resolver after receiving the data. The latter provides a visual scripting solution and inherits the interface from the former. So you can also use scripts to write dialogues, examples are as follows:
using UnityEngine;
public class CodeDialogueBuilder : MonoBehaviour
{
private RuntimeDialogueBuilder _builder;
private void Start()
{
PlayDialogue();
}
private void PlayDialogue()
{
var dialogueSystem = DialogueSystem.Get();
_builder = new RuntimeDialogueBuilder();
// First Piece
_builder.AddPiece(GetFirstPiece());
// Second Piece
_builder.AddPiece(GetSecondPiece());
dialogueSystem.StartDialogue(_builder);
}
private static Piece GetFirstPiece()
{
var piece = Piece.GetPooled();
piece.AddContent("This is the first dialogue piece");
piece.ID = "01";
piece.AddOption(new Option
{
Content = "Jump to Next",
TargetID = "02"
});
return piece;
}
private static Piece GetSecondPiece()
{
var piece = Piece.GetPooled();
piece.AddContent("This is the second dialogue piece");
piece.ID = "02";
piece.AddOption(GetFirstOption());
piece.AddOption(GetSecondOption());
return piece;
}
private static Option GetFirstOption()
{
var callBackOption = Option.GetPooled();
// Add CallBack Module
callBackOption.AddModule(new CallBackModule(() => Debug.Log("Hello World!")));
callBackOption.Content = "Log";
return callBackOption;
}
private static Option GetSecondOption()
{
var option = Option.GetPooled();
option.Content = "Back To First";
option.TargetID = "01";
return option;
}
}
For Tasks:
Click tags to check more tools for each tasksFor Jobs:
Alternative AI tools for Next-Gen-Dialogue
Similar Open Source Tools
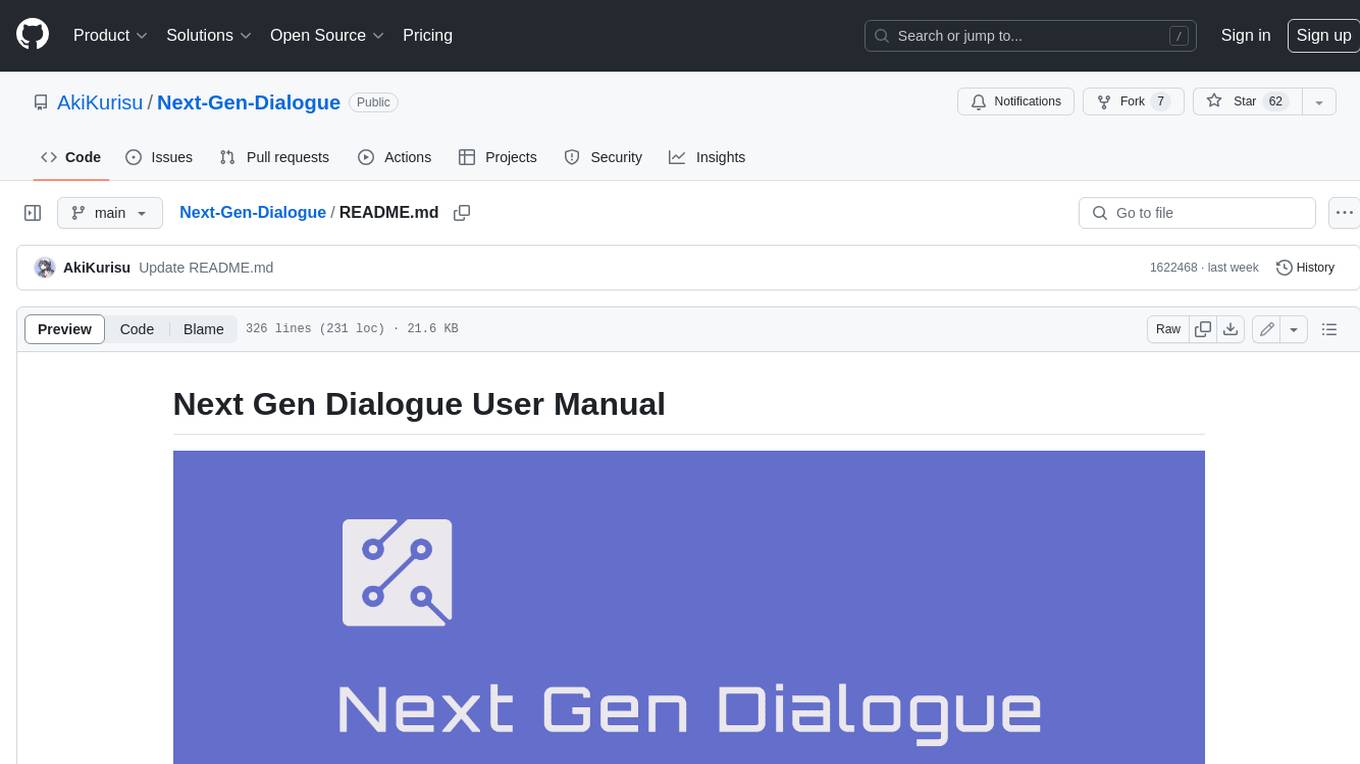
Next-Gen-Dialogue
Next Gen Dialogue is a Unity dialogue plugin that combines traditional dialogue design with AI techniques. It features a visual dialogue editor, modular dialogue functions, AIGC support for generating dialogue at runtime, AIGC baking dialogue in Editor, and runtime debugging. The plugin aims to provide an experimental approach to dialogue design using large language models. Users can create dialogue trees, generate dialogue content using AI, and bake dialogue content in advance. The tool also supports localization, VITS speech synthesis, and one-click translation. Users can create dialogue by code using the DialogueSystem and DialogueTree components.
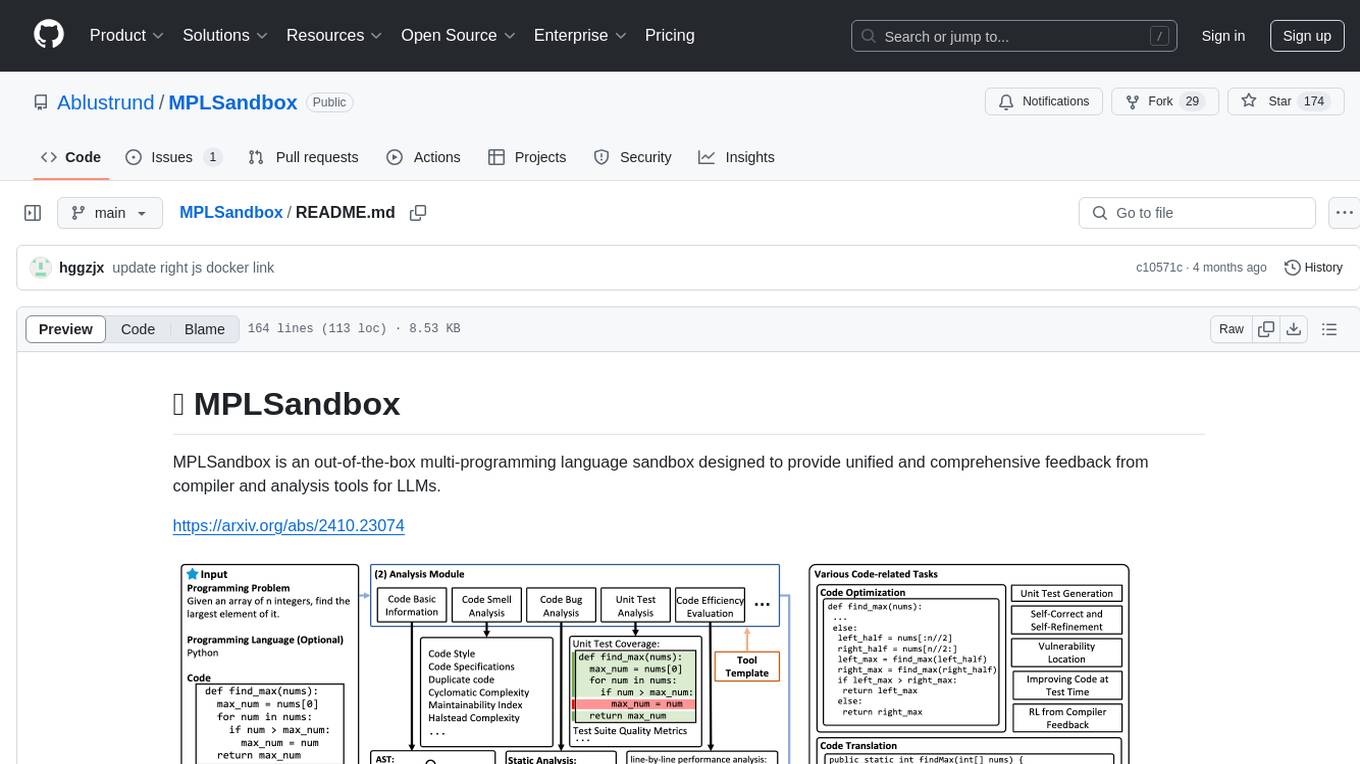
MPLSandbox
MPLSandbox is an out-of-the-box multi-programming language sandbox designed to provide unified and comprehensive feedback from compiler and analysis tools for LLMs. It simplifies code analysis for researchers and can be seamlessly integrated into LLM training and application processes to enhance performance in a range of code-related tasks. The sandbox environment ensures safe code execution, the code analysis module offers comprehensive analysis reports, and the information integration module combines compilation feedback and analysis results for complex code-related tasks.
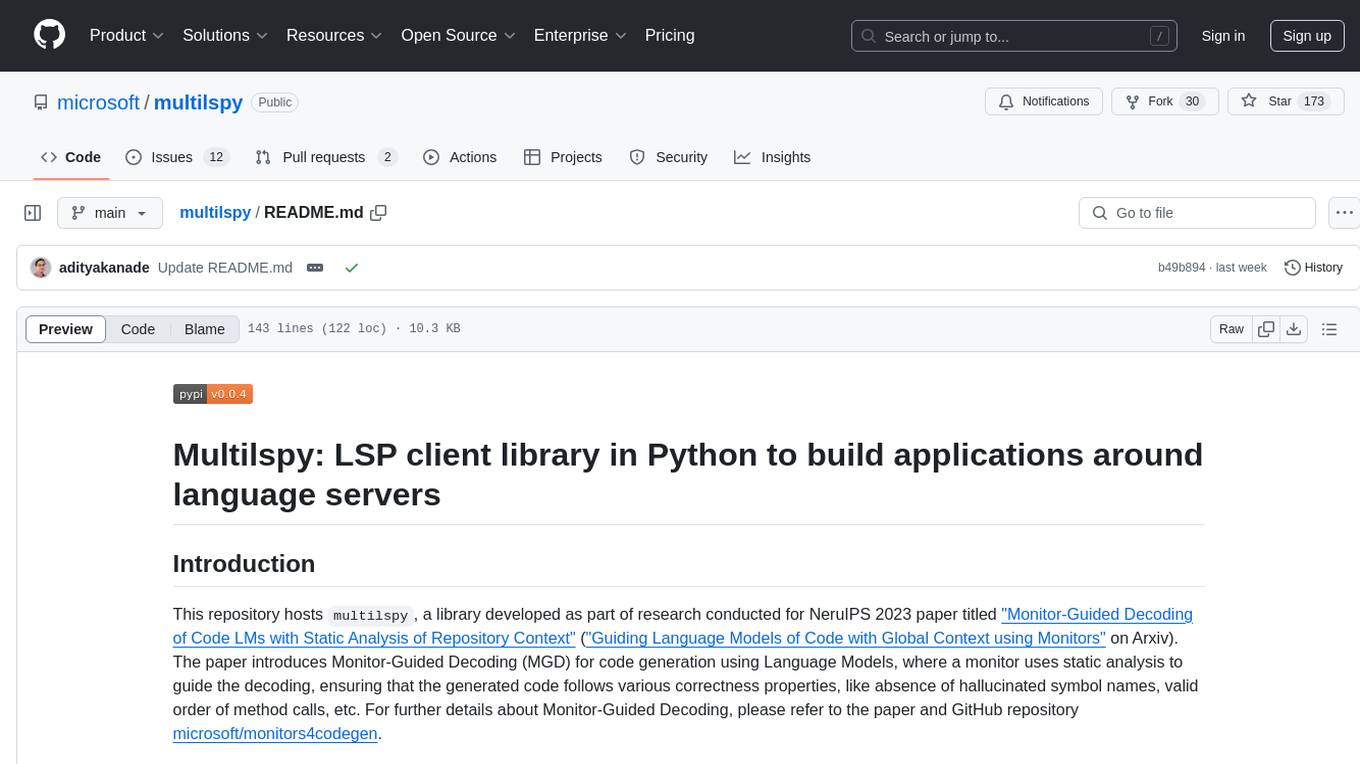
multilspy
Multilspy is a Python library developed for research purposes to facilitate the creation of language server clients for querying and obtaining results of static analyses from various language servers. It simplifies the process by handling server setup, communication, and configuration parameters, providing a common interface for different languages. The library supports features like finding function/class definitions, callers, completions, hover information, and document symbols. It is designed to work with AI systems like Large Language Models (LLMs) for tasks such as Monitor-Guided Decoding to ensure code generation correctness and boost compilability.
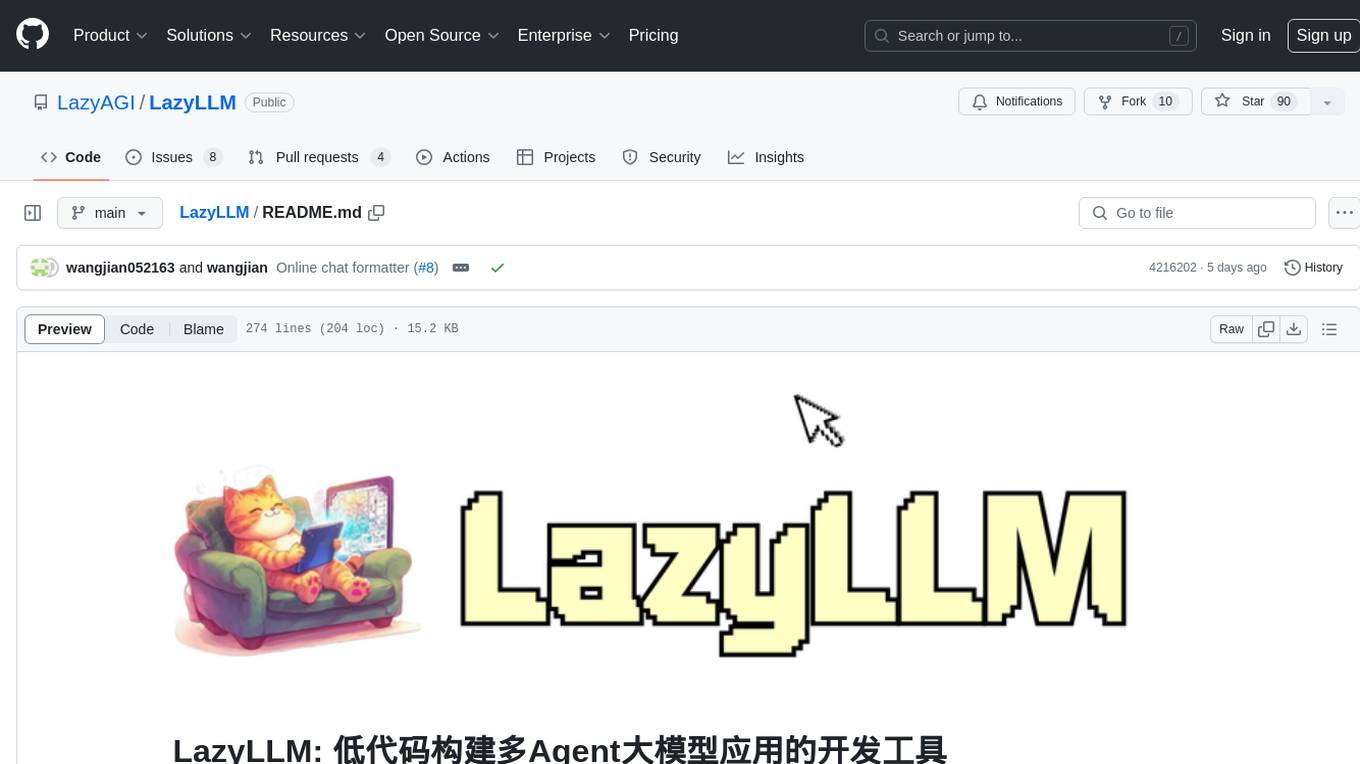
LazyLLM
LazyLLM is a low-code development tool for building complex AI applications with multiple agents. It assists developers in building AI applications at a low cost and continuously optimizing their performance. The tool provides a convenient workflow for application development and offers standard processes and tools for various stages of application development. Users can quickly prototype applications with LazyLLM, analyze bad cases with scenario task data, and iteratively optimize key components to enhance the overall application performance. LazyLLM aims to simplify the AI application development process and provide flexibility for both beginners and experts to create high-quality applications.
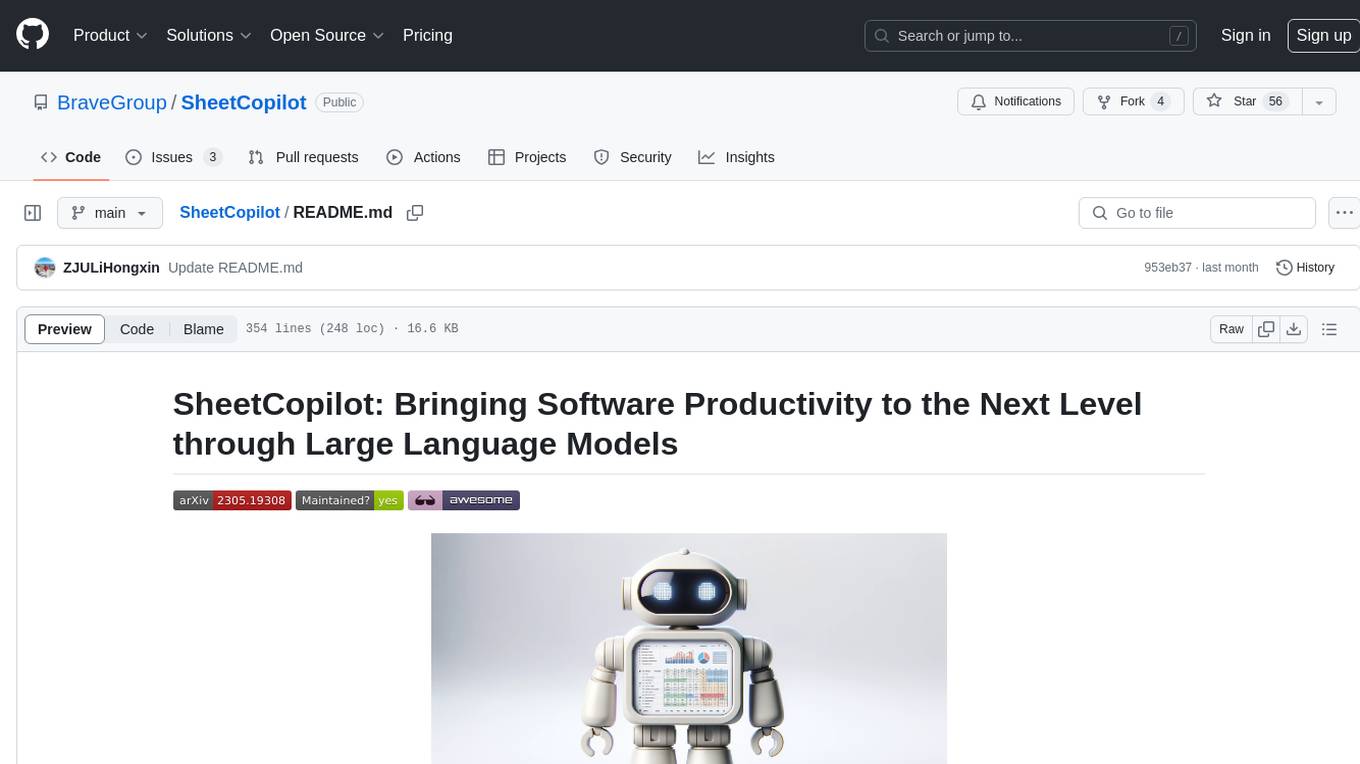
SheetCopilot
SheetCopilot is an assistant agent that manipulates spreadsheets by following user commands. It leverages Large Language Models (LLMs) to interact with spreadsheets like a human expert, enabling non-expert users to complete tasks on complex software such as Google Sheets and Excel via a language interface. The tool observes spreadsheet states, polishes generated solutions based on external action documents and error feedback, and aims to improve success rate and efficiency. SheetCopilot offers a dataset with diverse task categories and operations, supporting operations like entry & manipulation, management, formatting, charts, and pivot tables. Users can interact with SheetCopilot in Excel or Google Sheets, executing tasks like calculating revenue, creating pivot tables, and plotting charts. The tool's evaluation includes performance comparisons with leading LLMs and VBA-based methods on specific datasets, showcasing its capabilities in controlling various aspects of a spreadsheet.
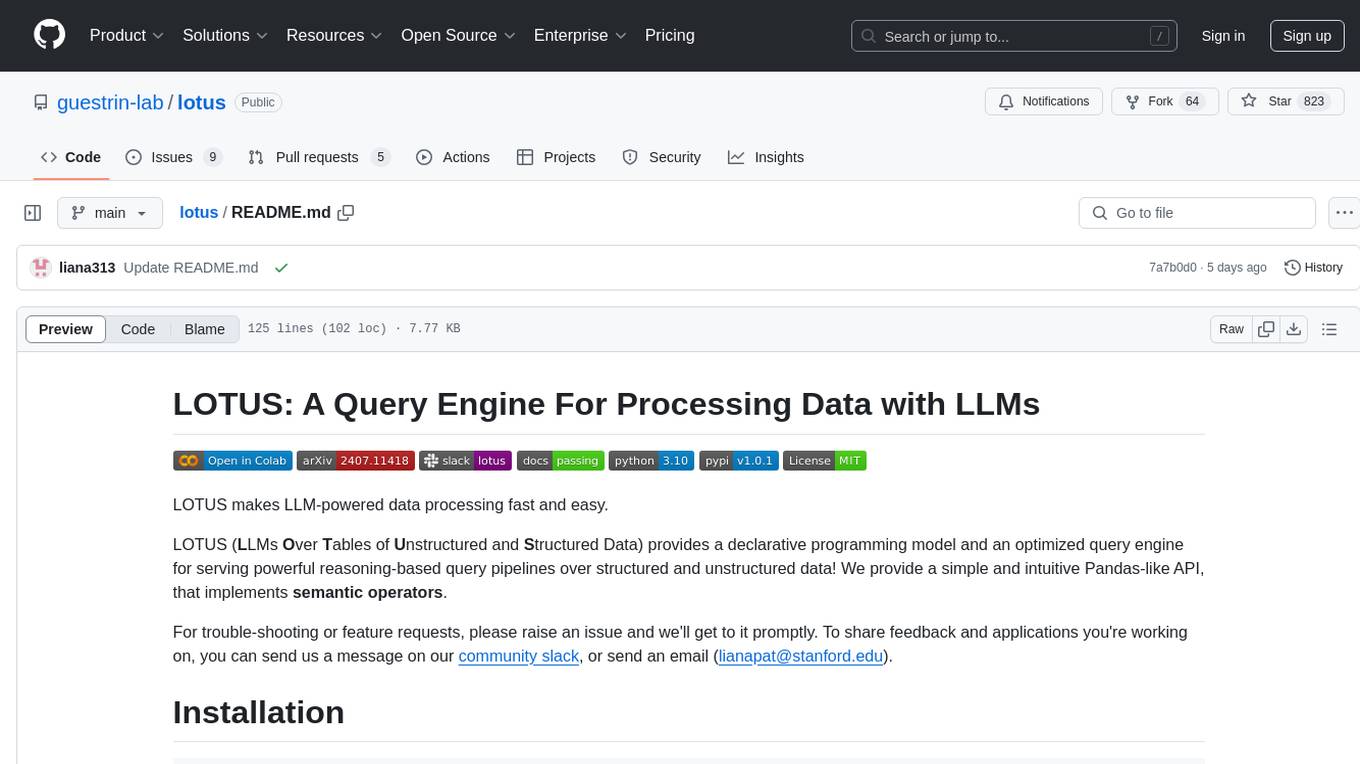
lotus
LOTUS (LLMs Over Tables of Unstructured and Structured Data) is a query engine that provides a declarative programming model and an optimized query engine for reasoning-based query pipelines over structured and unstructured data. It offers a simple and intuitive Pandas-like API with semantic operators for fast and easy LLM-powered data processing. The tool implements a semantic operator programming model, allowing users to write AI-based pipelines with high-level logic and leaving the rest of the work to the query engine. LOTUS supports various semantic operators like sem_map, sem_filter, sem_extract, sem_agg, sem_topk, sem_join, sem_sim_join, and sem_search, enabling users to perform tasks like mapping records, filtering data, aggregating records, and more. The tool also supports different model classes such as LM, RM, and Reranker for language modeling, retrieval, and reranking tasks respectively.
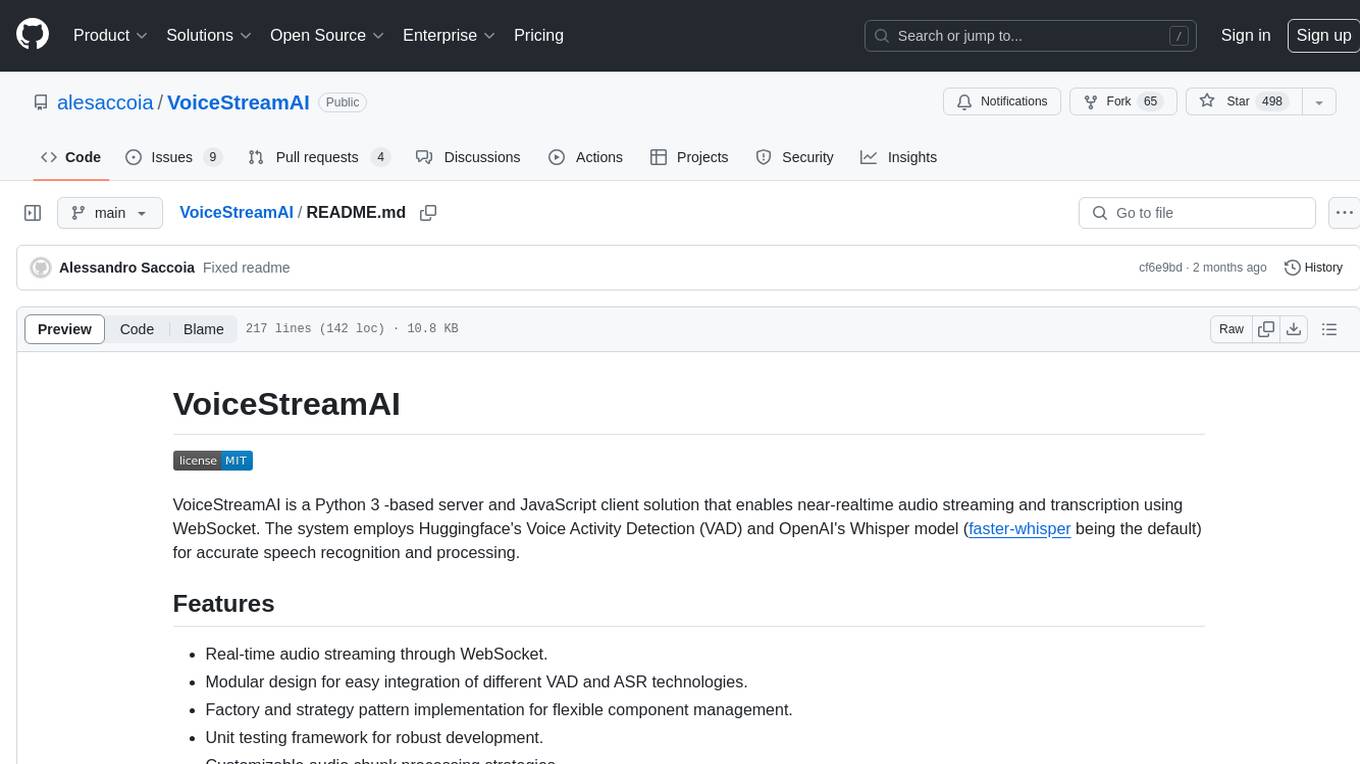
VoiceStreamAI
VoiceStreamAI is a Python 3-based server and JavaScript client solution for near-realtime audio streaming and transcription using WebSocket. It employs Huggingface's Voice Activity Detection (VAD) and OpenAI's Whisper model for accurate speech recognition. The system features real-time audio streaming, modular design for easy integration of VAD and ASR technologies, customizable audio chunk processing strategies, support for multilingual transcription, and secure sockets support. It uses a factory and strategy pattern implementation for flexible component management and provides a unit testing framework for robust development.
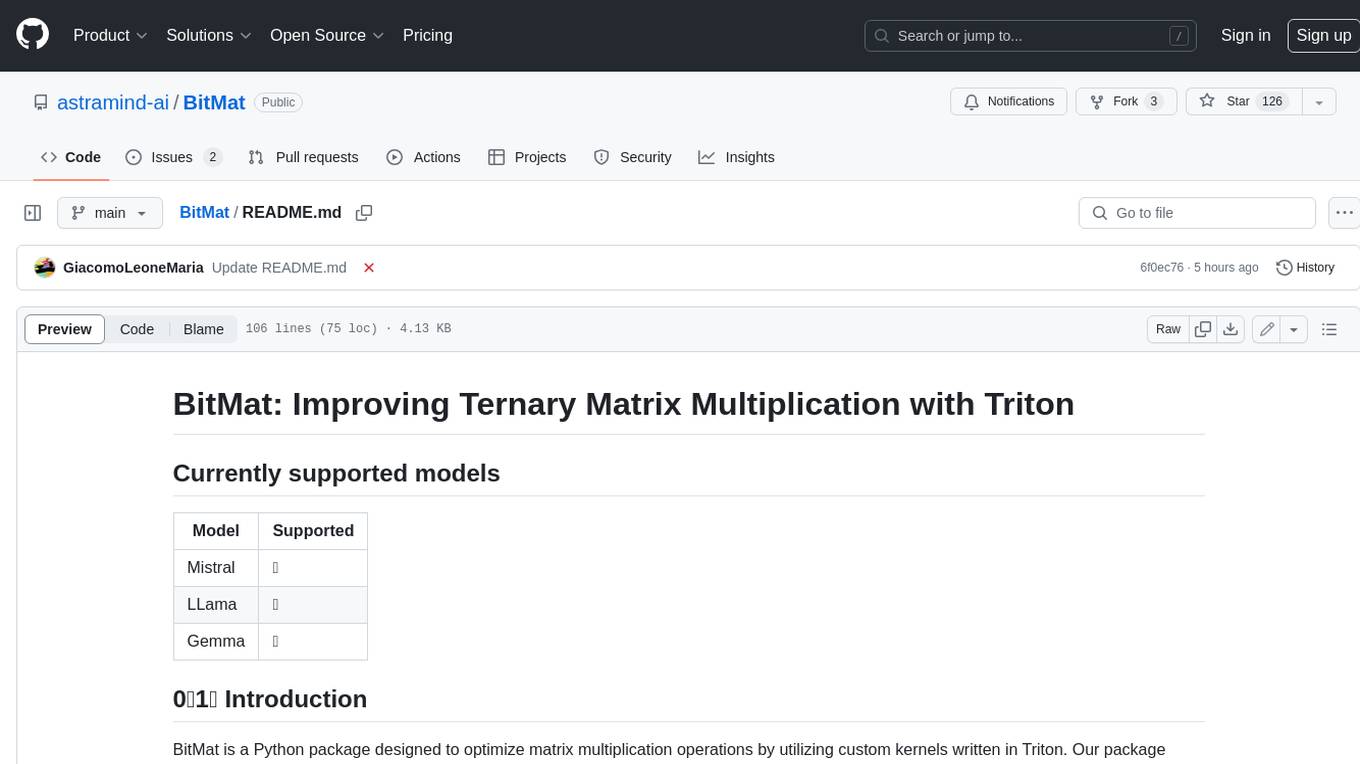
BitMat
BitMat is a Python package designed to optimize matrix multiplication operations by utilizing custom kernels written in Triton. It leverages the principles outlined in the "1bit-LLM Era" paper, specifically utilizing packed int8 data to enhance computational efficiency and performance in deep learning and numerical computing tasks.
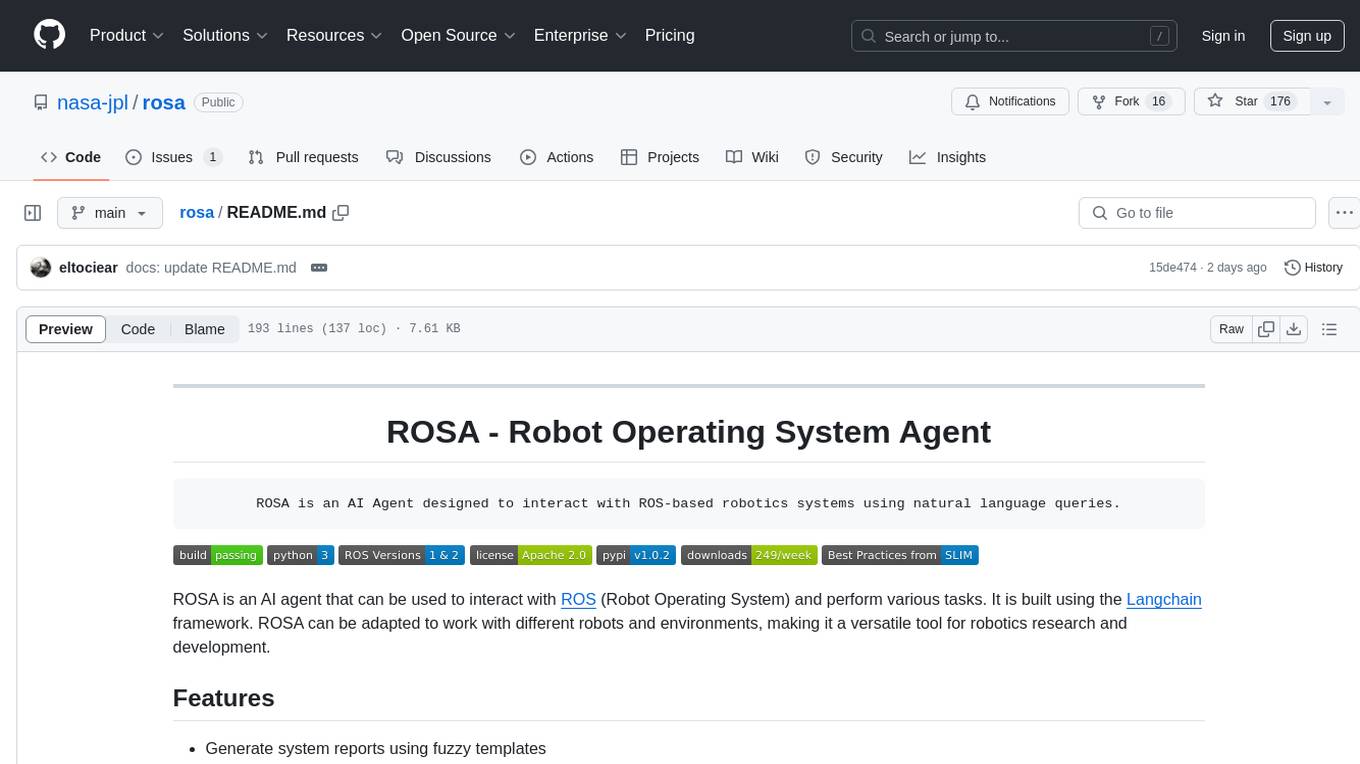
rosa
ROSA is an AI Agent designed to interact with ROS-based robotics systems using natural language queries. It can generate system reports, read and parse ROS log files, adapt to new robots, and run various ROS commands using natural language. The tool is versatile for robotics research and development, providing an easy way to interact with robots and the ROS environment.
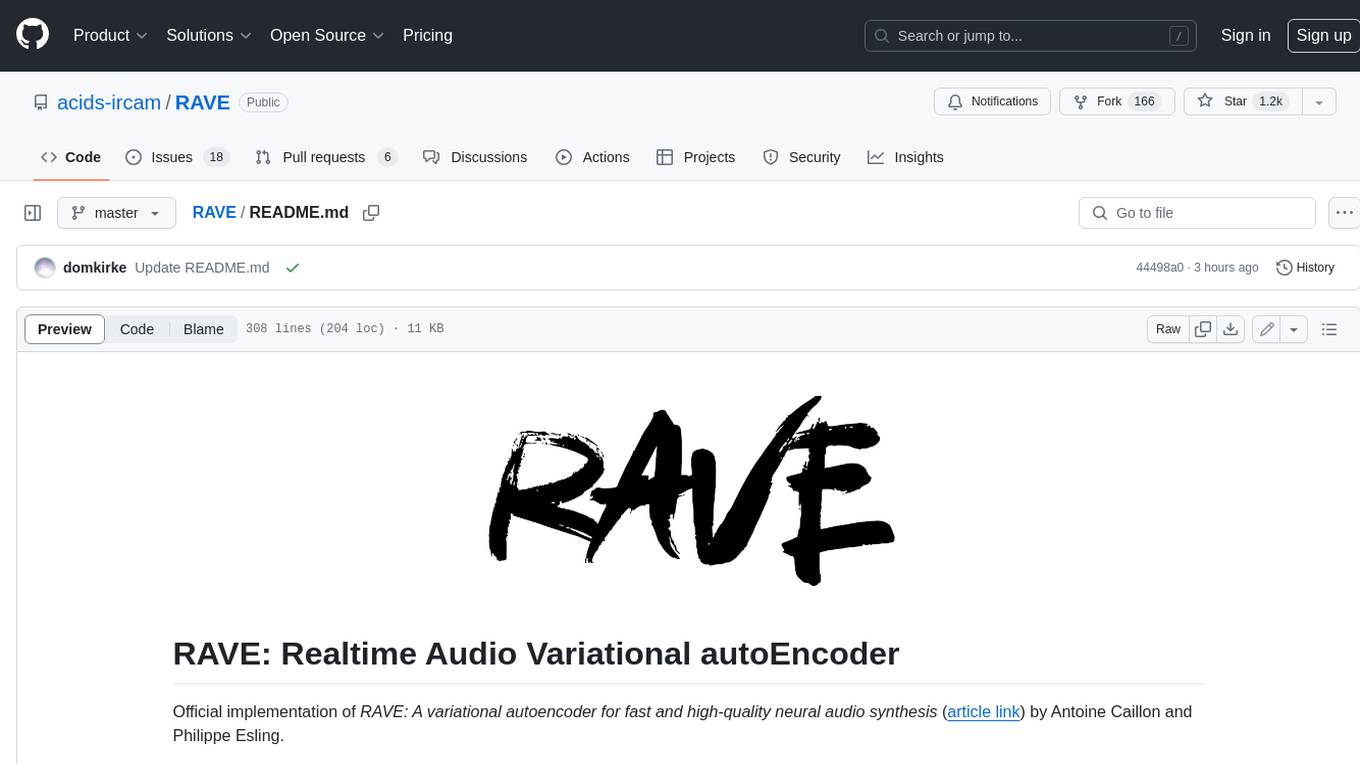
RAVE
RAVE is a variational autoencoder for fast and high-quality neural audio synthesis. It can be used to generate new audio samples from a given dataset, or to modify the style of existing audio samples. RAVE is easy to use and can be trained on a variety of audio datasets. It is also computationally efficient, making it suitable for real-time applications.
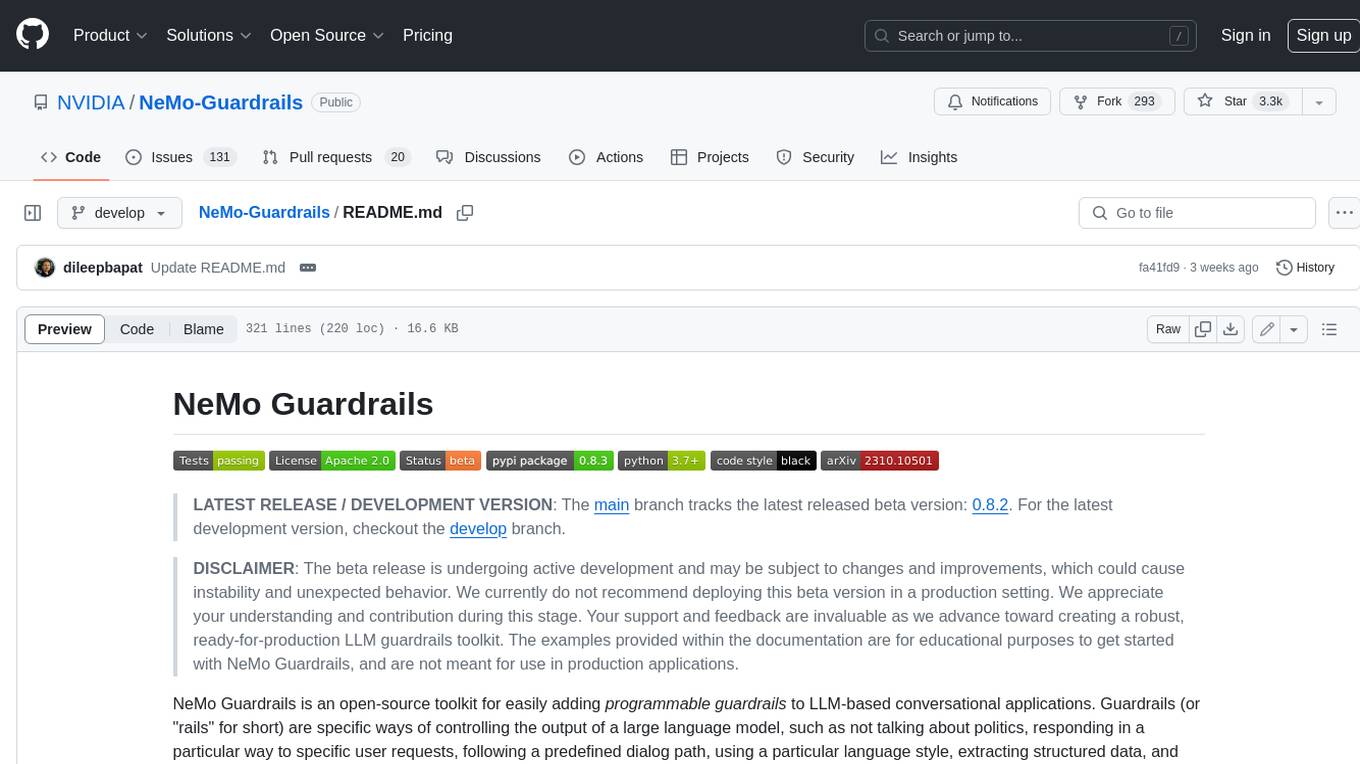
NeMo-Guardrails
NeMo Guardrails is an open-source toolkit for easily adding _programmable guardrails_ to LLM-based conversational applications. Guardrails (or "rails" for short) are specific ways of controlling the output of a large language model, such as not talking about politics, responding in a particular way to specific user requests, following a predefined dialog path, using a particular language style, extracting structured data, and more.
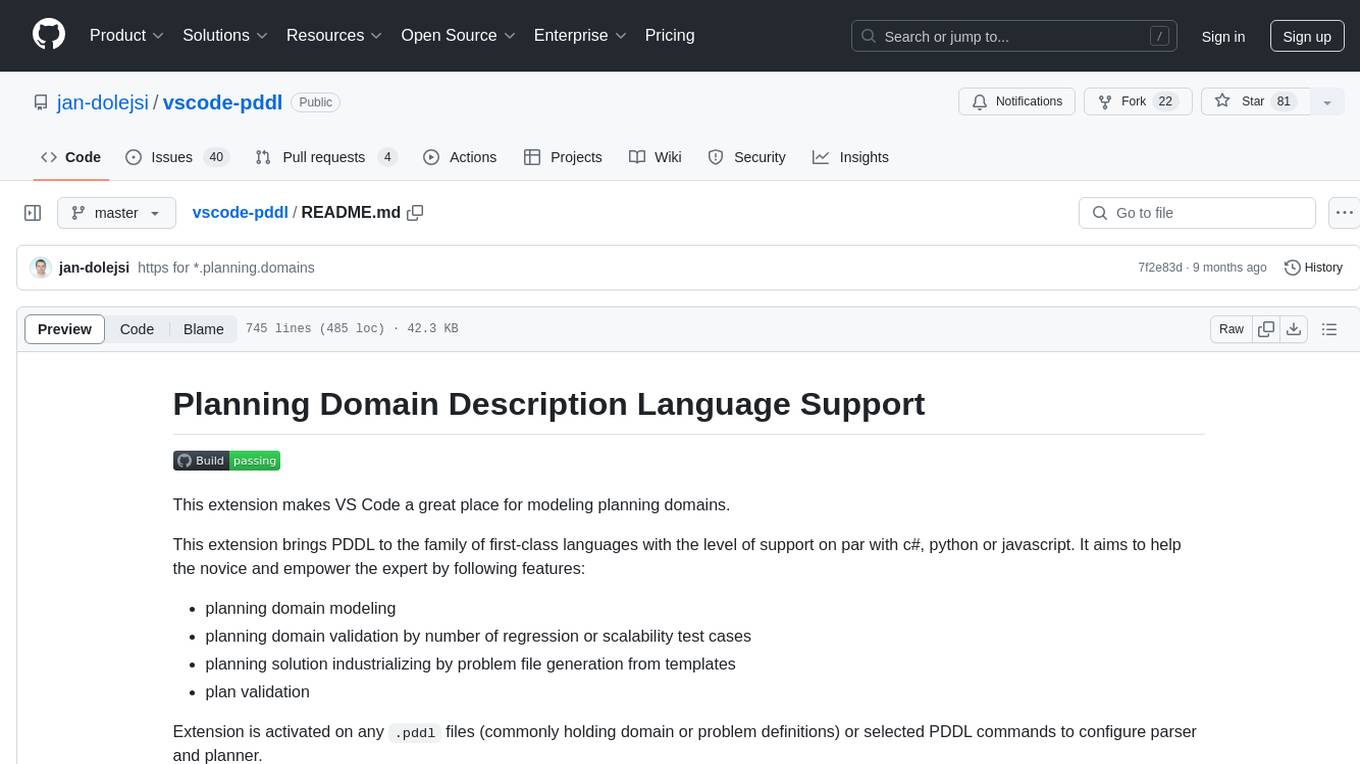
vscode-pddl
The vscode-pddl extension provides comprehensive support for Planning Domain Description Language (PDDL) in Visual Studio Code. It enables users to model planning domains, validate them, industrialize planning solutions, and run planners. The extension offers features like syntax highlighting, auto-completion, plan visualization, plan validation, plan happenings evaluation, search debugging, and integration with Planning.Domains. Users can create PDDL files, run planners, visualize plans, and debug search algorithms efficiently within VS Code.
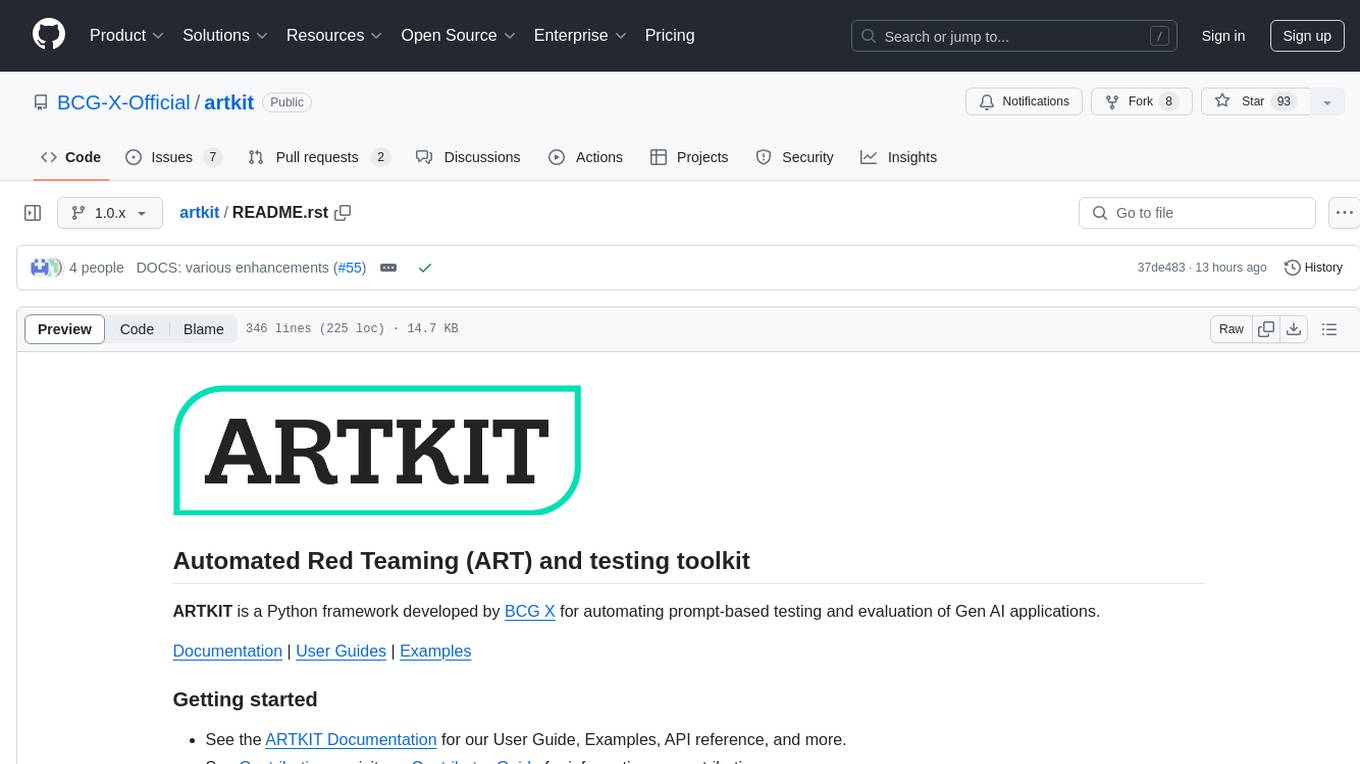
artkit
ARTKIT is a Python framework developed by BCG X for automating prompt-based testing and evaluation of Gen AI applications. It allows users to develop automated end-to-end testing and evaluation pipelines for Gen AI systems, supporting multi-turn conversations and various testing scenarios like Q&A accuracy, brand values, equitability, safety, and security. The framework provides a simple API, asynchronous processing, caching, model agnostic support, end-to-end pipelines, multi-turn conversations, robust data flows, and visualizations. ARTKIT is designed for customization by data scientists and engineers to enhance human-in-the-loop testing and evaluation, emphasizing the importance of tailored testing for each Gen AI use case.
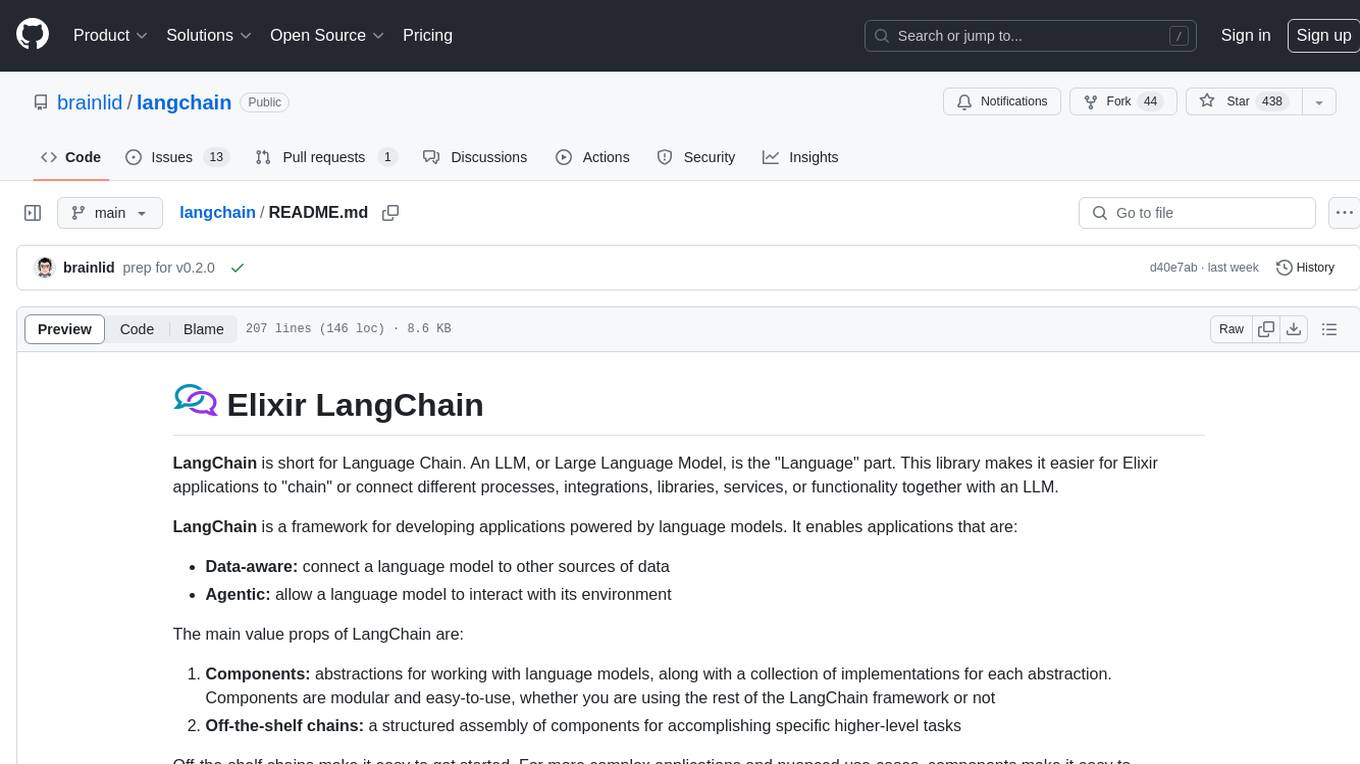
langchain
LangChain is a framework for developing Elixir applications powered by language models. It enables applications to connect language models to other data sources and interact with the environment. The library provides components for working with language models and off-the-shelf chains for specific tasks. It aims to assist in building applications that combine large language models with other sources of computation or knowledge. LangChain is written in Elixir and is not aimed for parity with the JavaScript and Python versions due to differences in programming paradigms and design choices. The library is designed to make it easy to integrate language models into applications and expose features, data, and functionality to the models.
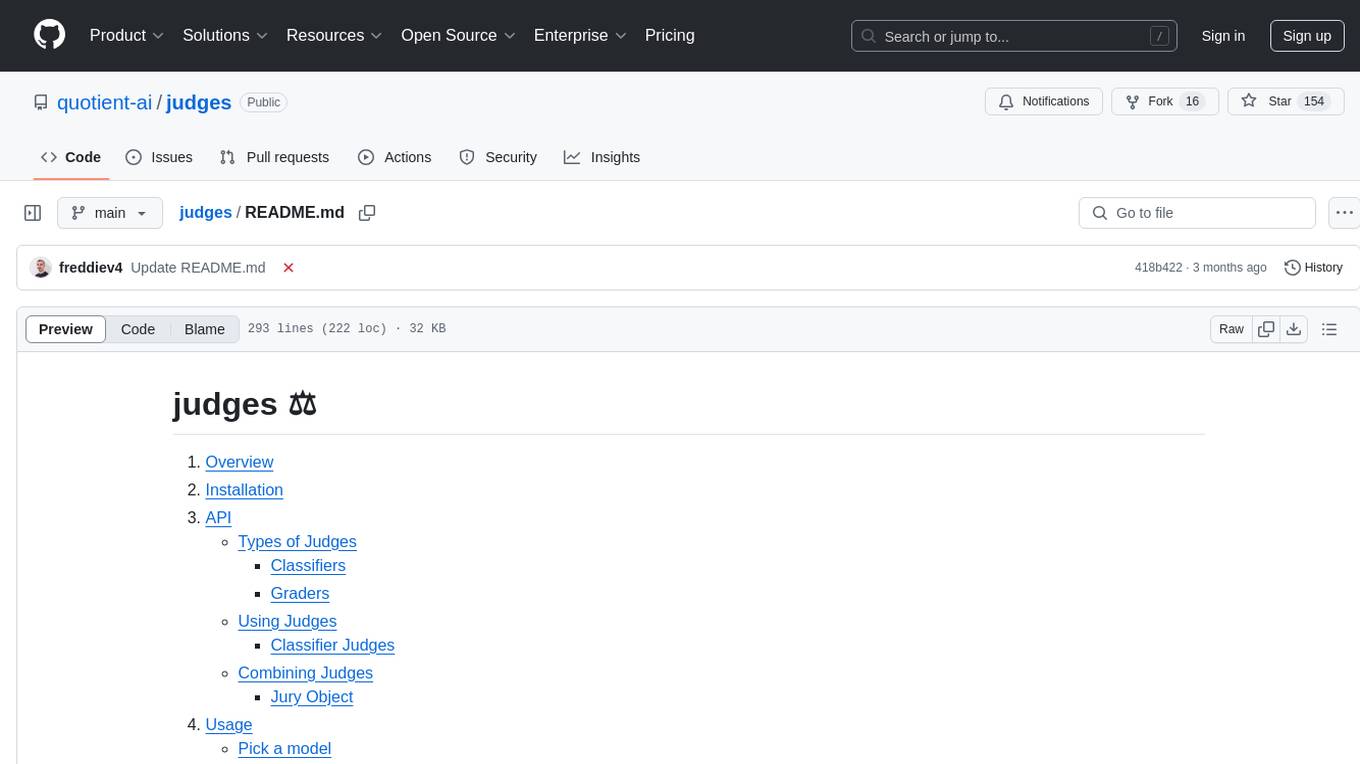
judges
The 'judges' repository is a small library designed for using and creating LLM-as-a-Judge evaluators. It offers a curated set of LLM evaluators in a low-friction format for various use cases, backed by research. Users can use these evaluators off-the-shelf or as inspiration for building custom LLM evaluators. The library provides two types of judges: Classifiers that return boolean values and Graders that return scores on a numerical or Likert scale. Users can combine multiple judges using the 'Jury' object and evaluate input-output pairs with the '.judge()' method. Additionally, the repository includes detailed instructions on picking a model, sending data to an LLM, using classifiers, combining judges, and creating custom LLM judges with 'AutoJudge'.
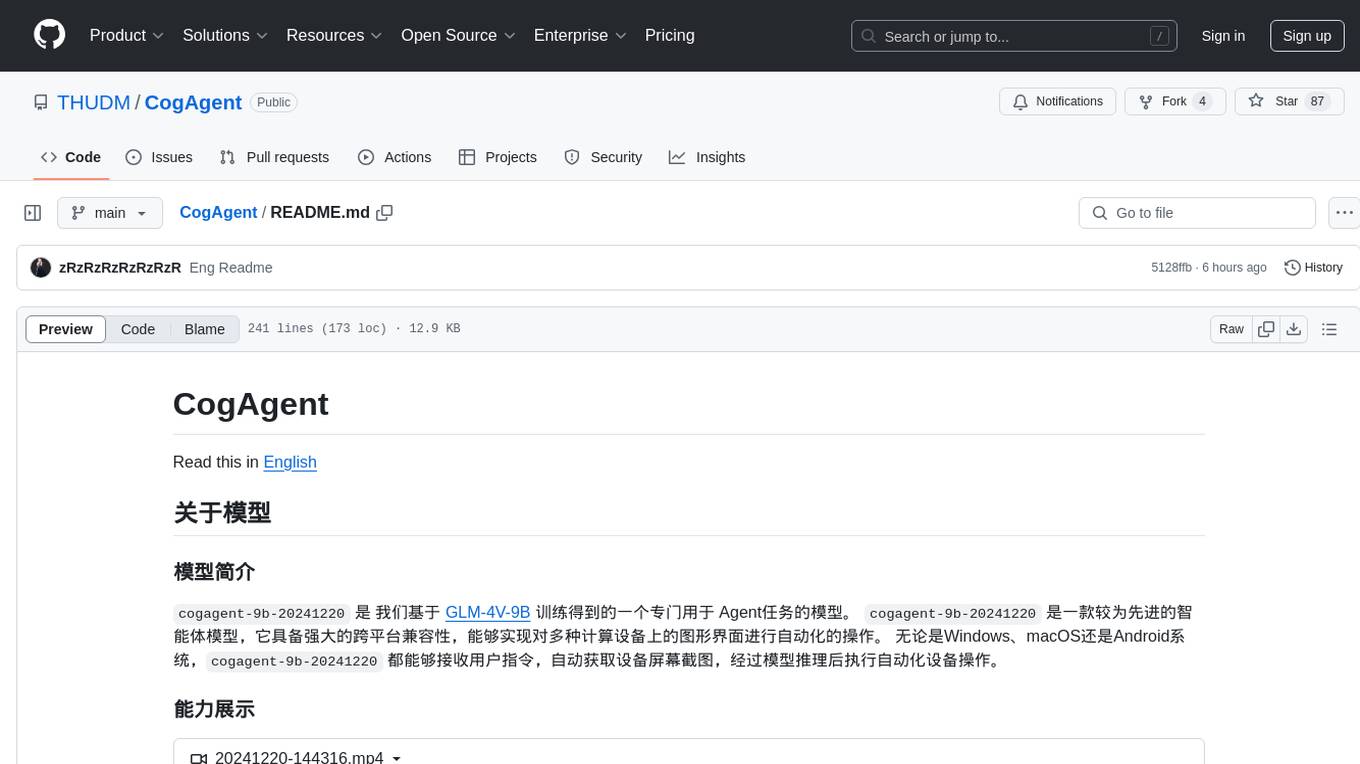
CogAgent
CogAgent is an advanced intelligent agent model designed for automating operations on graphical interfaces across various computing devices. It supports platforms like Windows, macOS, and Android, enabling users to issue commands, capture device screenshots, and perform automated operations. The model requires a minimum of 29GB of GPU memory for inference at BF16 precision and offers capabilities for executing tasks like sending Christmas greetings and sending emails. Users can interact with the model by providing task descriptions, platform specifications, and desired output formats.
For similar tasks
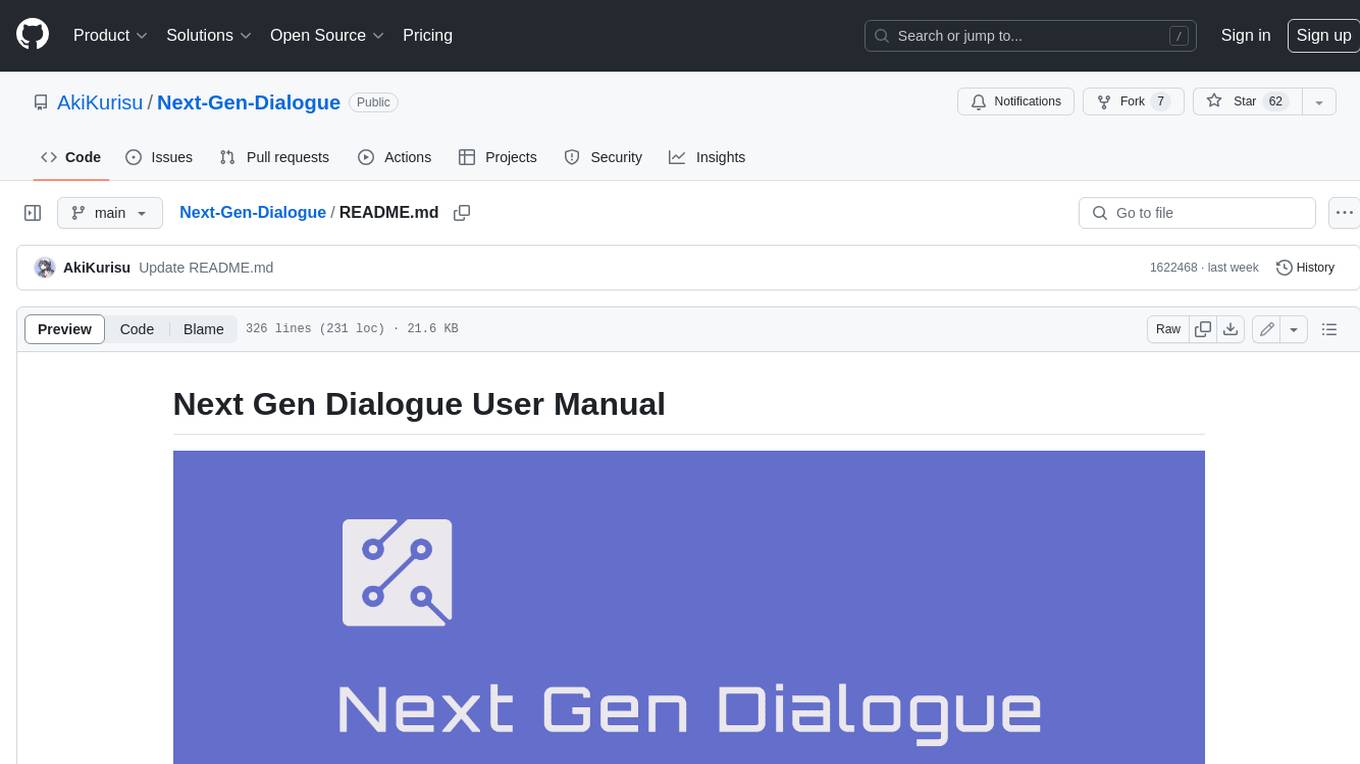
Next-Gen-Dialogue
Next Gen Dialogue is a Unity dialogue plugin that combines traditional dialogue design with AI techniques. It features a visual dialogue editor, modular dialogue functions, AIGC support for generating dialogue at runtime, AIGC baking dialogue in Editor, and runtime debugging. The plugin aims to provide an experimental approach to dialogue design using large language models. Users can create dialogue trees, generate dialogue content using AI, and bake dialogue content in advance. The tool also supports localization, VITS speech synthesis, and one-click translation. Users can create dialogue by code using the DialogueSystem and DialogueTree components.
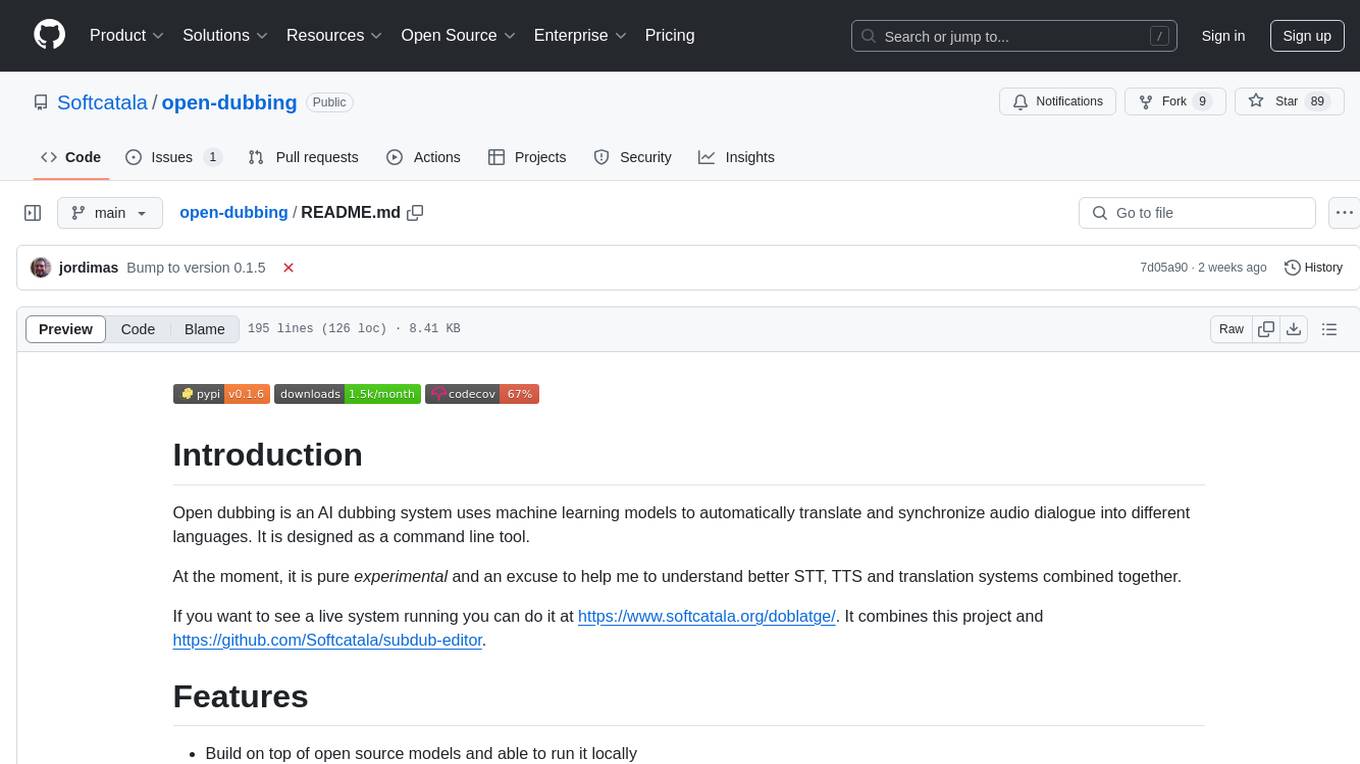
open-dubbing
Open dubbing is an AI dubbing system that uses machine learning models to automatically translate and synchronize audio dialogue into different languages. It is designed as a command line tool. The project is experimental and aims to explore speech-to-text, text-to-speech, and translation systems combined. It supports multiple text-to-speech engines, translation engines, and gender voice detection. The tool can automatically dub videos, detect source language, and is built on open-source models. The roadmap includes better voice control, optimization for long videos, and support for multiple video input formats. Users can post-edit dubbed files by manually adjusting text, voice, and timings. Supported languages vary based on the combination of systems used.
For similar jobs
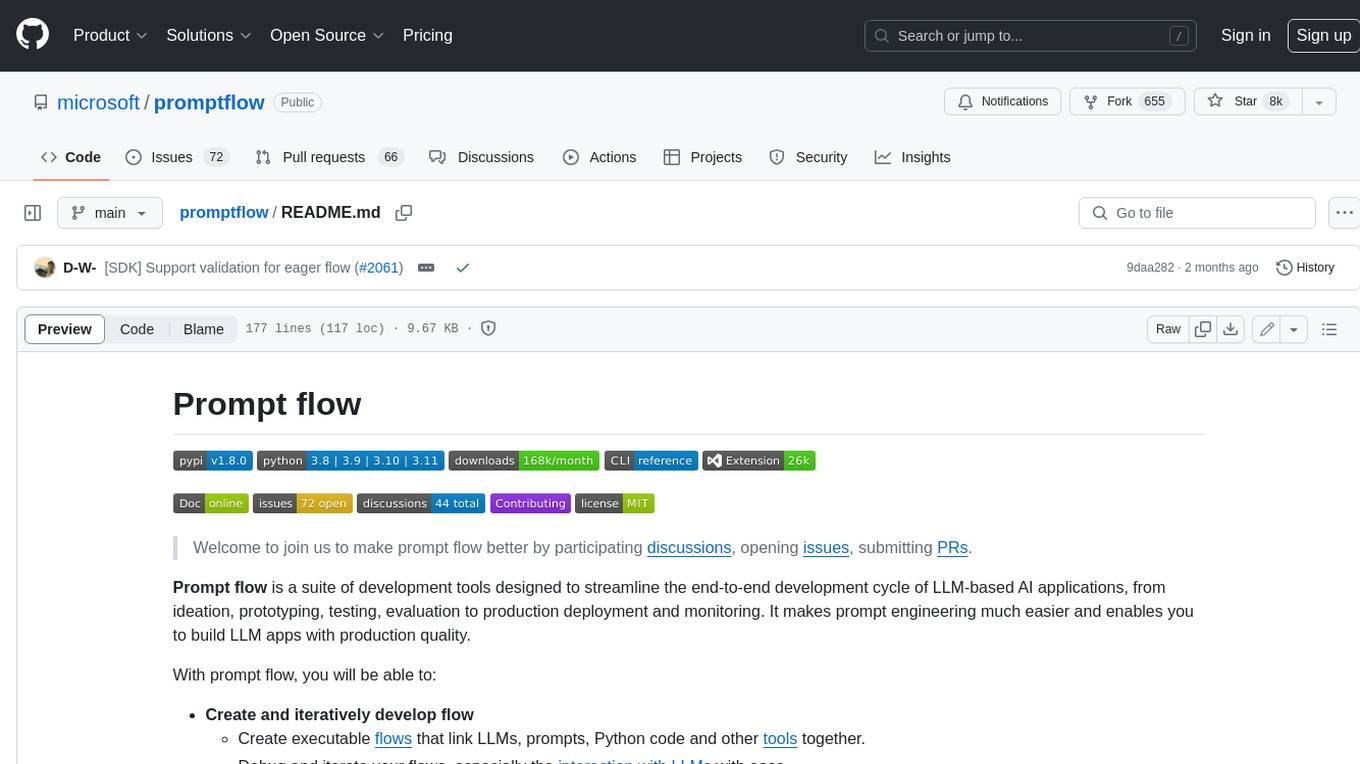
promptflow
**Prompt flow** is a suite of development tools designed to streamline the end-to-end development cycle of LLM-based AI applications, from ideation, prototyping, testing, evaluation to production deployment and monitoring. It makes prompt engineering much easier and enables you to build LLM apps with production quality.
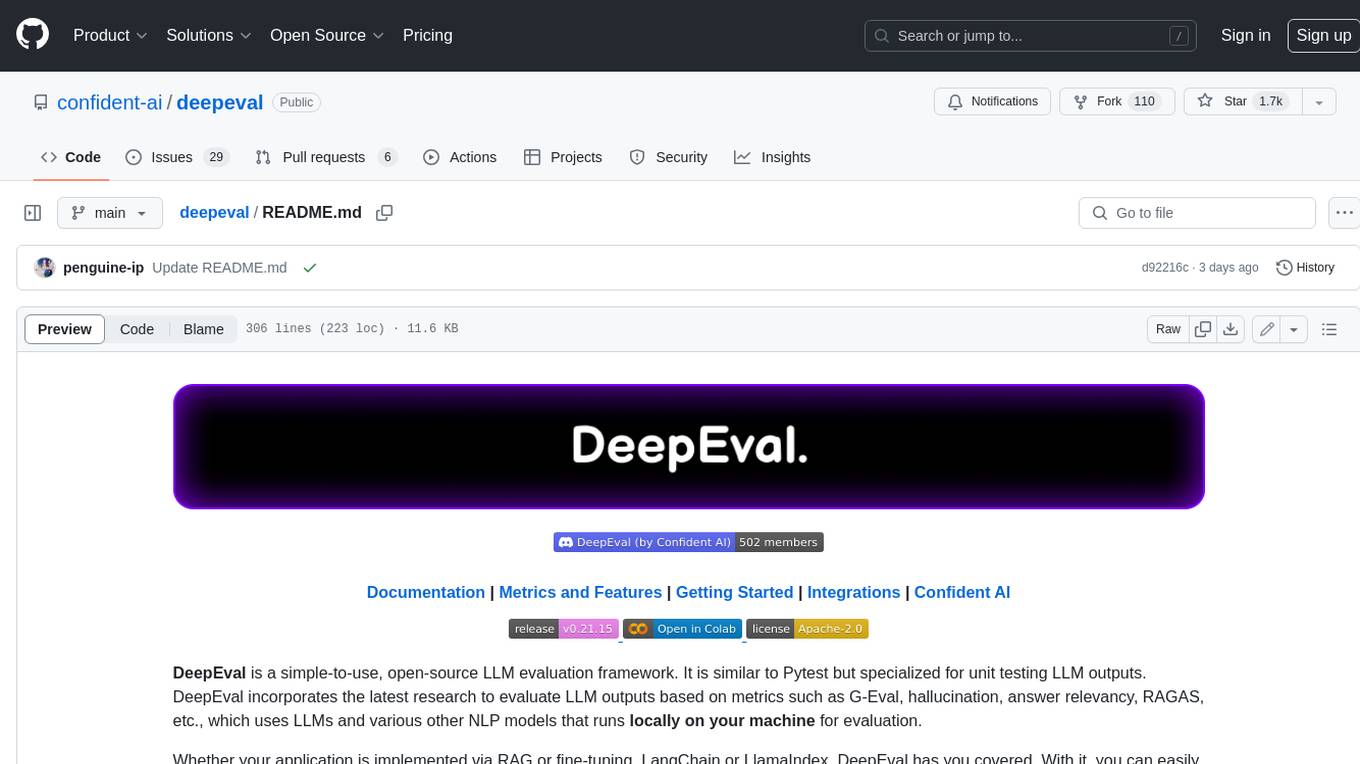
deepeval
DeepEval is a simple-to-use, open-source LLM evaluation framework specialized for unit testing LLM outputs. It incorporates various metrics such as G-Eval, hallucination, answer relevancy, RAGAS, etc., and runs locally on your machine for evaluation. It provides a wide range of ready-to-use evaluation metrics, allows for creating custom metrics, integrates with any CI/CD environment, and enables benchmarking LLMs on popular benchmarks. DeepEval is designed for evaluating RAG and fine-tuning applications, helping users optimize hyperparameters, prevent prompt drifting, and transition from OpenAI to hosting their own Llama2 with confidence.
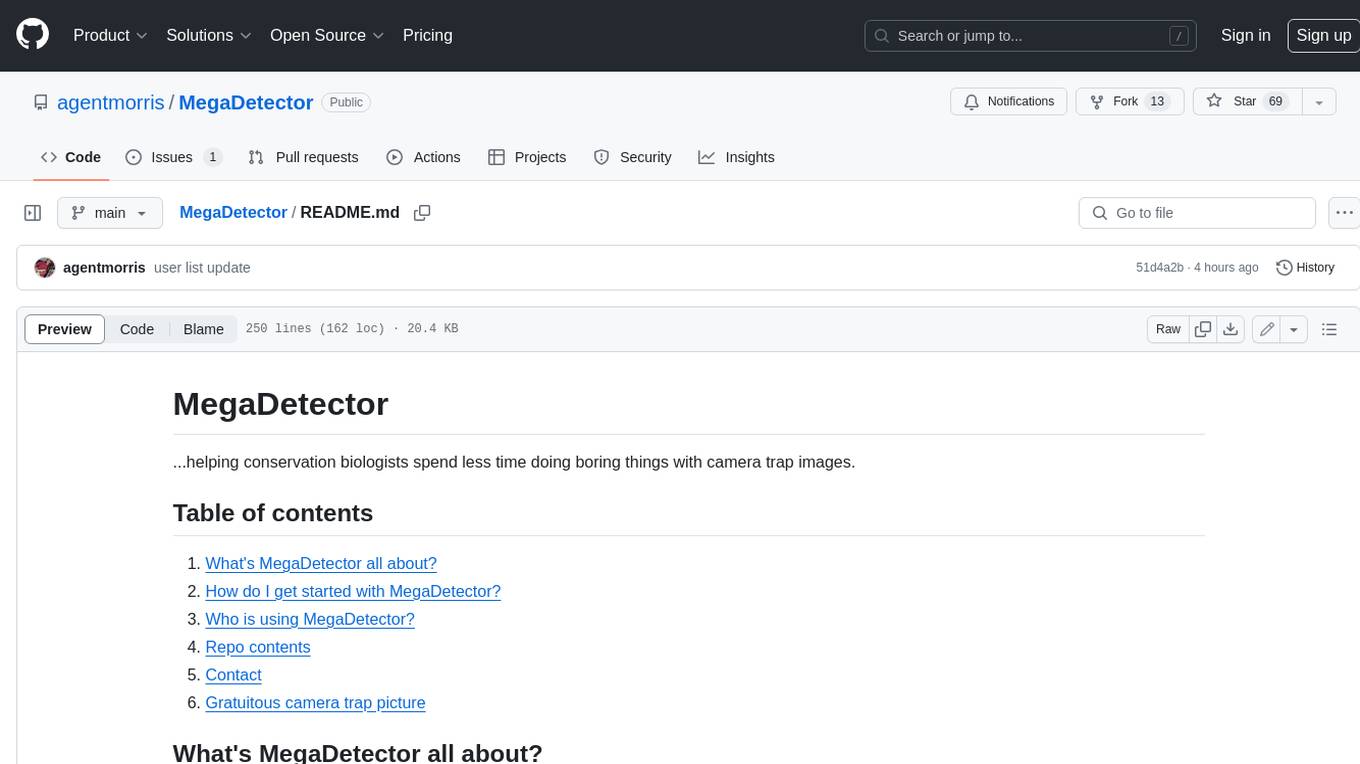
MegaDetector
MegaDetector is an AI model that identifies animals, people, and vehicles in camera trap images (which also makes it useful for eliminating blank images). This model is trained on several million images from a variety of ecosystems. MegaDetector is just one of many tools that aims to make conservation biologists more efficient with AI. If you want to learn about other ways to use AI to accelerate camera trap workflows, check out our of the field, affectionately titled "Everything I know about machine learning and camera traps".
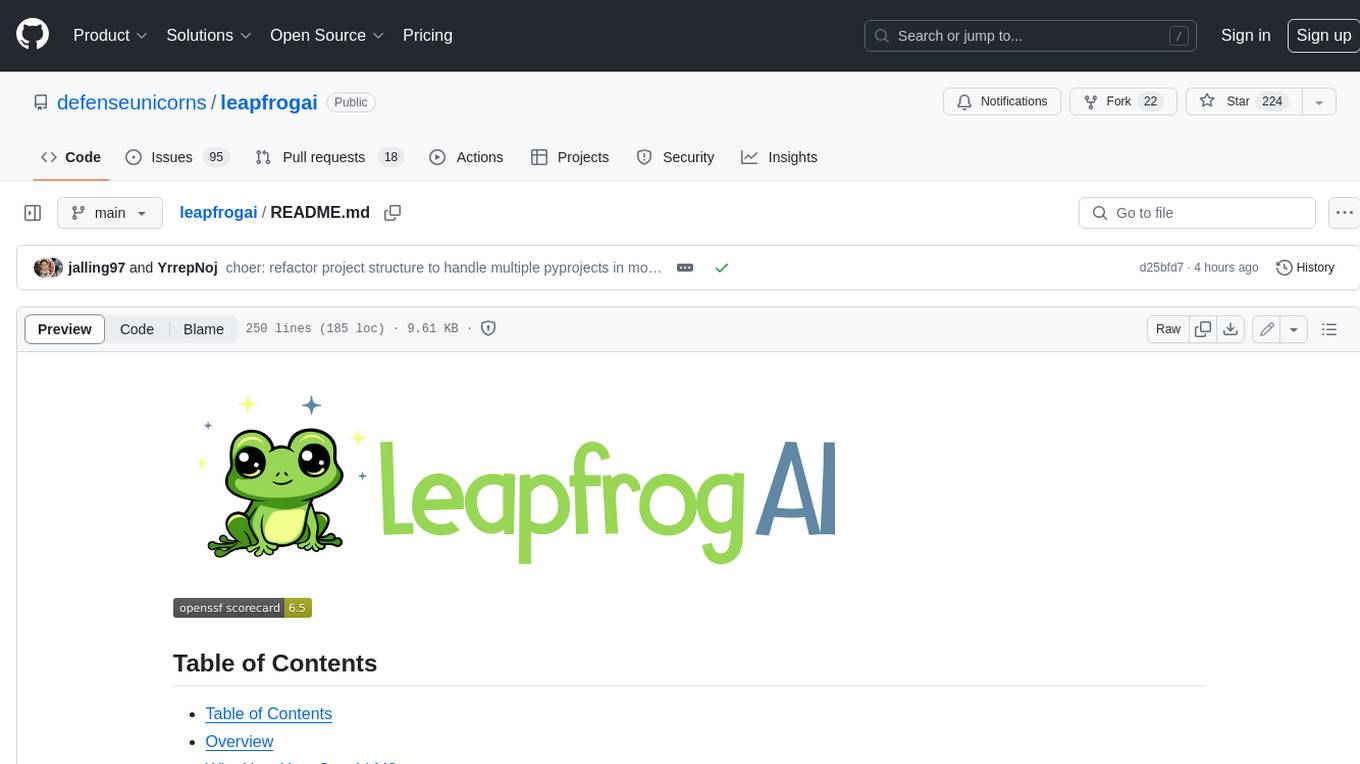
leapfrogai
LeapfrogAI is a self-hosted AI platform designed to be deployed in air-gapped resource-constrained environments. It brings sophisticated AI solutions to these environments by hosting all the necessary components of an AI stack, including vector databases, model backends, API, and UI. LeapfrogAI's API closely matches that of OpenAI, allowing tools built for OpenAI/ChatGPT to function seamlessly with a LeapfrogAI backend. It provides several backends for various use cases, including llama-cpp-python, whisper, text-embeddings, and vllm. LeapfrogAI leverages Chainguard's apko to harden base python images, ensuring the latest supported Python versions are used by the other components of the stack. The LeapfrogAI SDK provides a standard set of protobuffs and python utilities for implementing backends and gRPC. LeapfrogAI offers UI options for common use-cases like chat, summarization, and transcription. It can be deployed and run locally via UDS and Kubernetes, built out using Zarf packages. LeapfrogAI is supported by a community of users and contributors, including Defense Unicorns, Beast Code, Chainguard, Exovera, Hypergiant, Pulze, SOSi, United States Navy, United States Air Force, and United States Space Force.
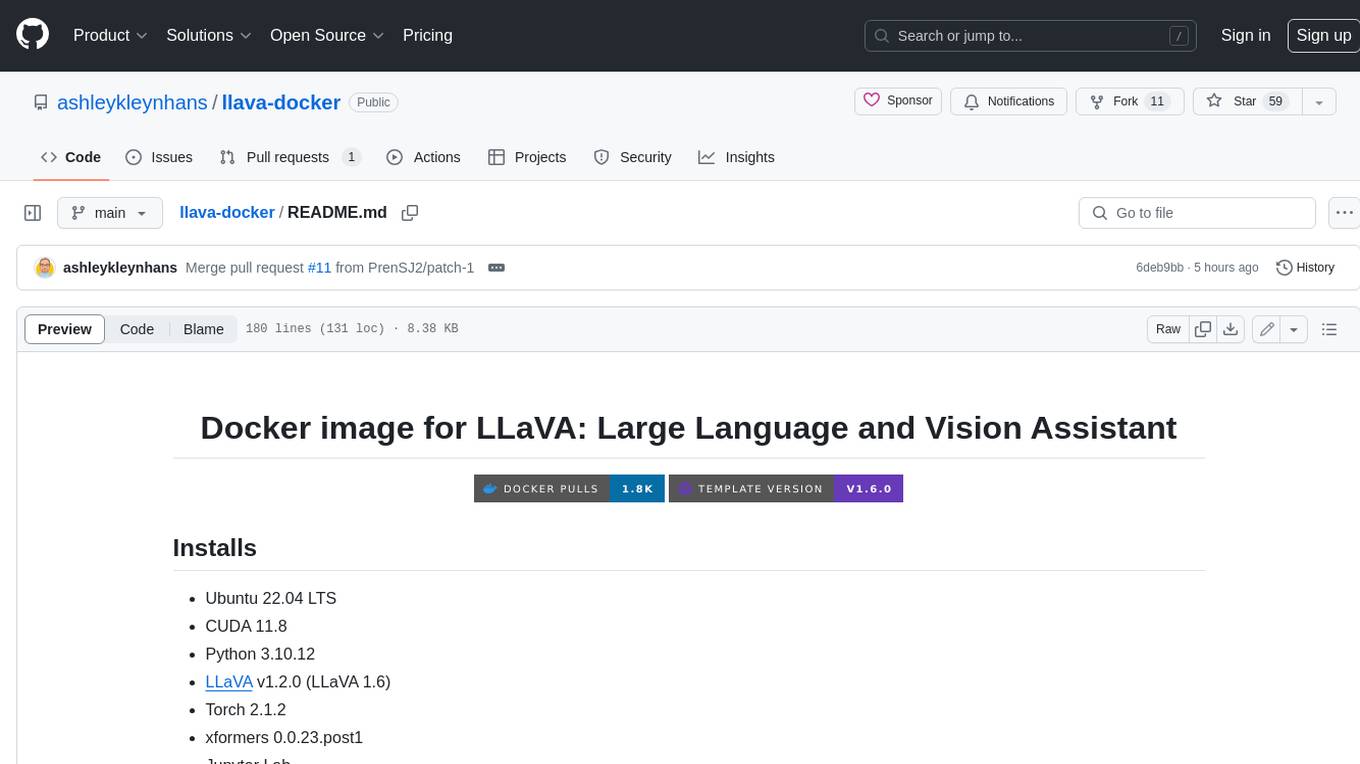
llava-docker
This Docker image for LLaVA (Large Language and Vision Assistant) provides a convenient way to run LLaVA locally or on RunPod. LLaVA is a powerful AI tool that combines natural language processing and computer vision capabilities. With this Docker image, you can easily access LLaVA's functionalities for various tasks, including image captioning, visual question answering, text summarization, and more. The image comes pre-installed with LLaVA v1.2.0, Torch 2.1.2, xformers 0.0.23.post1, and other necessary dependencies. You can customize the model used by setting the MODEL environment variable. The image also includes a Jupyter Lab environment for interactive development and exploration. Overall, this Docker image offers a comprehensive and user-friendly platform for leveraging LLaVA's capabilities.
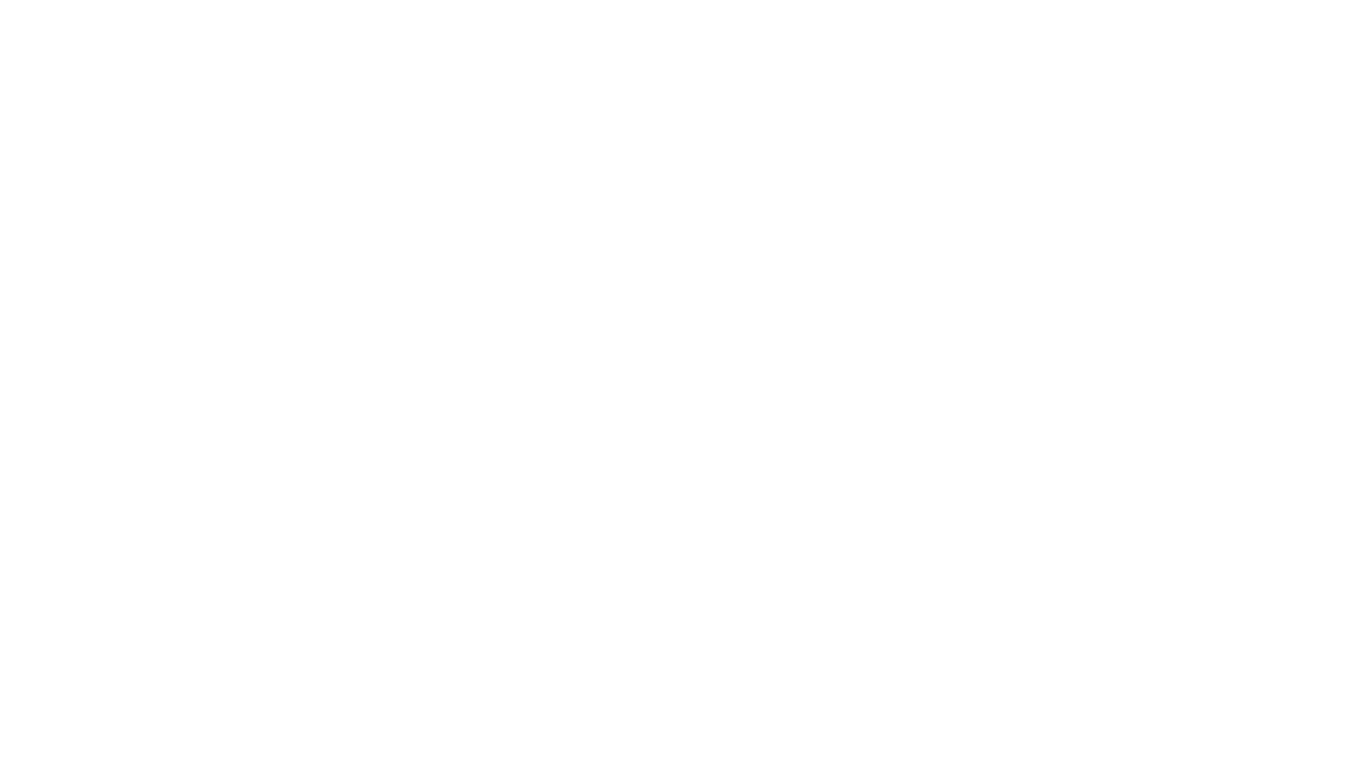
carrot
The 'carrot' repository on GitHub provides a list of free and user-friendly ChatGPT mirror sites for easy access. The repository includes sponsored sites offering various GPT models and services. Users can find and share sites, report errors, and access stable and recommended sites for ChatGPT usage. The repository also includes a detailed list of ChatGPT sites, their features, and accessibility options, making it a valuable resource for ChatGPT users seeking free and unlimited GPT services.
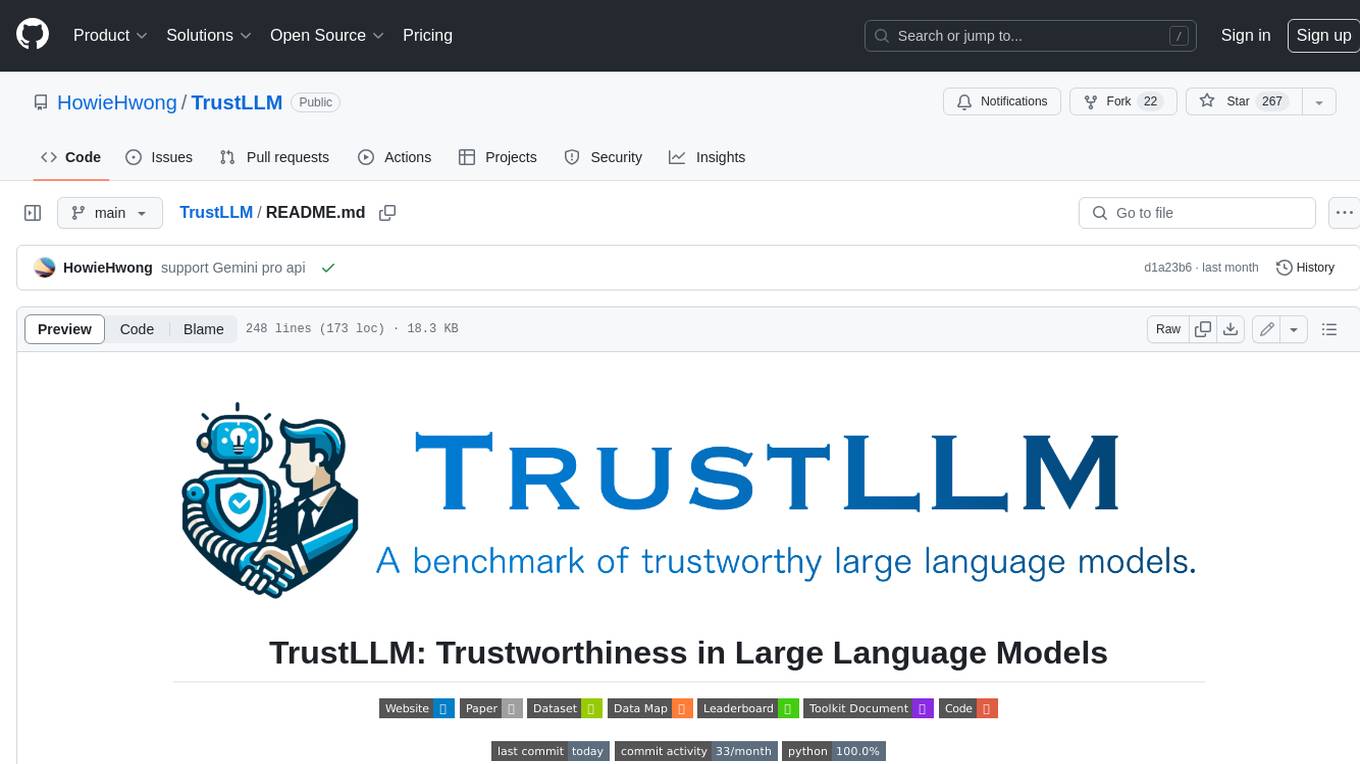
TrustLLM
TrustLLM is a comprehensive study of trustworthiness in LLMs, including principles for different dimensions of trustworthiness, established benchmark, evaluation, and analysis of trustworthiness for mainstream LLMs, and discussion of open challenges and future directions. Specifically, we first propose a set of principles for trustworthy LLMs that span eight different dimensions. Based on these principles, we further establish a benchmark across six dimensions including truthfulness, safety, fairness, robustness, privacy, and machine ethics. We then present a study evaluating 16 mainstream LLMs in TrustLLM, consisting of over 30 datasets. The document explains how to use the trustllm python package to help you assess the performance of your LLM in trustworthiness more quickly. For more details about TrustLLM, please refer to project website.
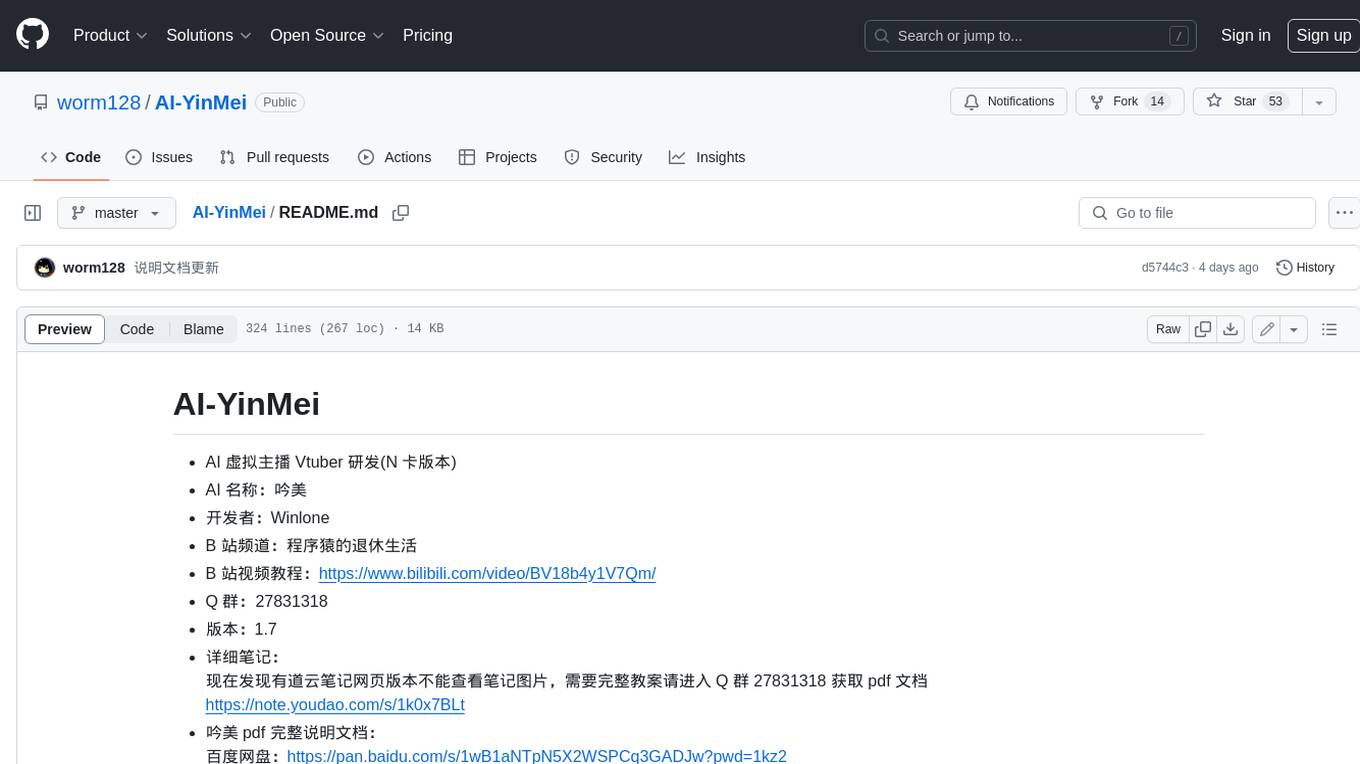
AI-YinMei
AI-YinMei is an AI virtual anchor Vtuber development tool (N card version). It supports fastgpt knowledge base chat dialogue, a complete set of solutions for LLM large language models: [fastgpt] + [one-api] + [Xinference], supports docking bilibili live broadcast barrage reply and entering live broadcast welcome speech, supports Microsoft edge-tts speech synthesis, supports Bert-VITS2 speech synthesis, supports GPT-SoVITS speech synthesis, supports expression control Vtuber Studio, supports painting stable-diffusion-webui output OBS live broadcast room, supports painting picture pornography public-NSFW-y-distinguish, supports search and image search service duckduckgo (requires magic Internet access), supports image search service Baidu image search (no magic Internet access), supports AI reply chat box [html plug-in], supports AI singing Auto-Convert-Music, supports playlist [html plug-in], supports dancing function, supports expression video playback, supports head touching action, supports gift smashing action, supports singing automatic start dancing function, chat and singing automatic cycle swing action, supports multi scene switching, background music switching, day and night automatic switching scene, supports open singing and painting, let AI automatically judge the content.