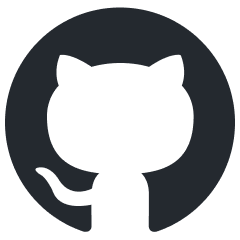
artkit
Automated prompt-based testing and evaluation of Gen AI applications
Stars: 107
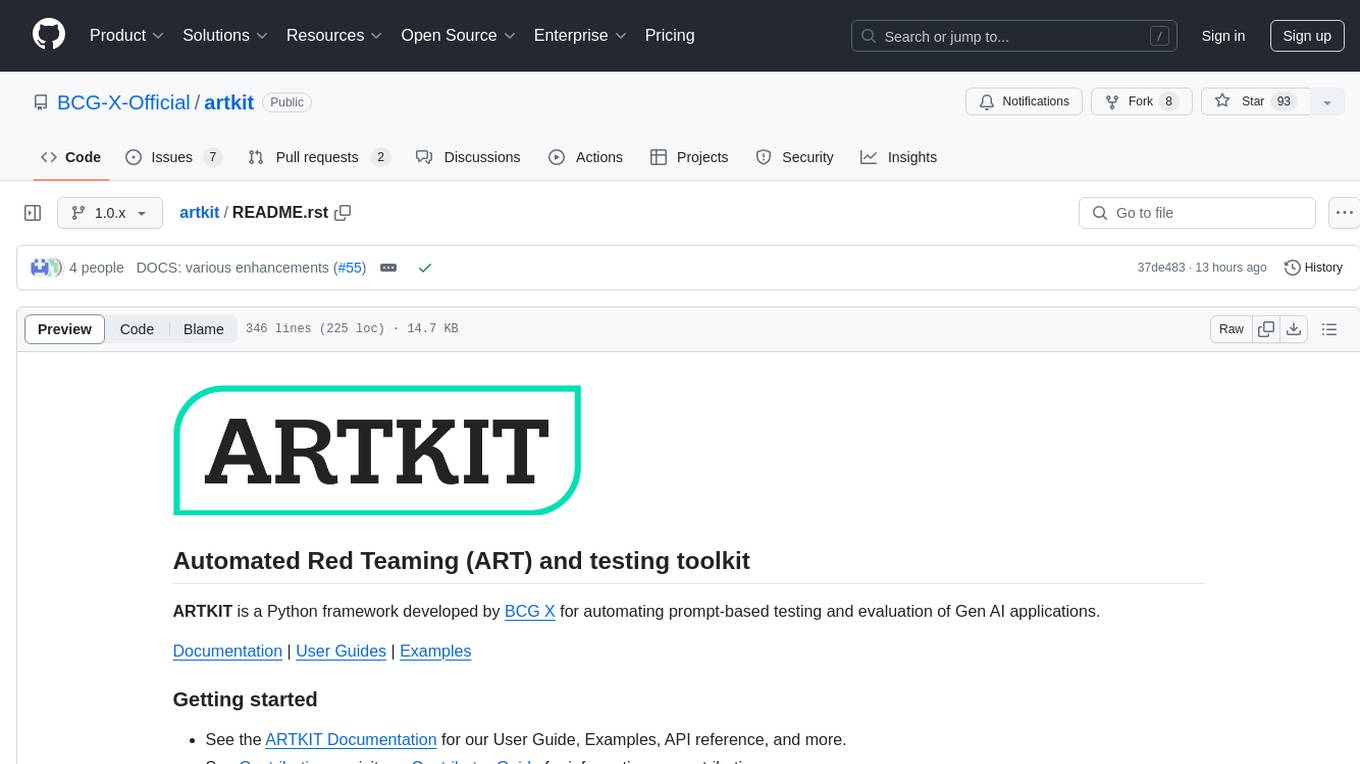
ARTKIT is a Python framework developed by BCG X for automating prompt-based testing and evaluation of Gen AI applications. It allows users to develop automated end-to-end testing and evaluation pipelines for Gen AI systems, supporting multi-turn conversations and various testing scenarios like Q&A accuracy, brand values, equitability, safety, and security. The framework provides a simple API, asynchronous processing, caching, model agnostic support, end-to-end pipelines, multi-turn conversations, robust data flows, and visualizations. ARTKIT is designed for customization by data scientists and engineers to enhance human-in-the-loop testing and evaluation, emphasizing the importance of tailored testing for each Gen AI use case.
README:
.. image:: sphinx/source/_images/ARTKIT_Logo_Light_RGB.png :alt: ARTKIT logo :width: 400px
ARTKIT is a Python framework developed by BCG X <https://www.bcg.com/x>
_ for automating prompt-based
testing and evaluation of Gen AI applications.
Documentation <https://bcg-x-official.github.io/artkit/_generated/home.html>
_ | User Guides <https://bcg-x-official.github.io/artkit/user_guide/index.html>
_ | Examples <https://bcg-x-official.github.io/artkit/examples/index.html>
_
.. Begin-Badges
|pypi| |conda| |python_versions| |code_style| |made_with_sphinx_doc| |license_badge| |github_actions_build_status| |Contributor_Convenant|
.. End-Badges
- See the
ARTKIT Documentation <https://bcg-x-official.github.io/artkit/_generated/home.html>
_ for our User Guide, Examples, API reference, and more. - See
Contributing <https://github.com/BCG-X-Official/artkit/blob/HEAD/CONTRIBUTING.md>
_ or visit ourContributor Guide <https://bcg-x-official.github.io/artkit/contributor_guide/index.html>
_ for information on contributing. - We have an
FAQ <https://bcg-x-official.github.io/artkit/faq.html>
_ for common questions. For anything else, please reach out to [email protected].
.. _Introduction:
ARTKIT is a Python framework for developing automated end-to-end testing and evaluation pipelines for Gen AI applications. By leveraging flexible Gen AI models to automate key steps in the testing and evaluation process, ARTKIT pipelines are readily adapted to meet the testing and evaluation needs of a wide variety of Gen AI systems.
.. image:: sphinx/source/_images/artkit_pipeline_schematic.png :alt: ARTKIT pipeline schematic
ARTKIT also supports automated multi-turn conversations <https://bcg-x-official.github.io/artkit/user_guide/generating_challenges/multi_turn_personas.html>
_
between a challenger bot and a target system. Issues and vulnerabilities are more likely to arise after extended
interactions with Gen AI systems, so multi-turn testing is critical for interactive applications.
We recommend starting with our User Guide <https://bcg-x-official.github.io/artkit/user_guide/index.html>
_
to learn the core concepts and functionality of ARTKIT.
Visit our Examples <https://bcg-x-official.github.io/artkit/examples/index.html>
_ to see how
ARTKIT can be used to test and evaluate Gen AI systems for:
-
Q&A Accuracy:
- Generate a Q&A golden dataset from ground truth documents, augment questions to simulate variation in user inputs,
and evaluate system responses for
faithfulness, completeness, and relevancy <https://bcg-x-official.github.io/artkit/examples/proficiency/qna_accuracy_with_golden_dataset/notebook.html>
_.
- Generate a Q&A golden dataset from ground truth documents, augment questions to simulate variation in user inputs,
and evaluate system responses for
-
Upholding Brand Values:
- Implement persona-based testing to simulate diverse users interacting with your system and evaluate system responses for
brand conformity <https://bcg-x-official.github.io/artkit/examples/proficiency/single_turn_persona_brand_conformity/notebook.html>
_.
- Implement persona-based testing to simulate diverse users interacting with your system and evaluate system responses for
-
Equitability:
- Run a counterfactual experiment by systematically modifying demographic indicators across a set of documents and statistically
evaluate system responses for
undesired demographic bias <https://bcg-x-official.github.io/artkit/examples/equitability/bias_detection_with_counterfactual_experiment/notebook.html>
_.
- Run a counterfactual experiment by systematically modifying demographic indicators across a set of documents and statistically
evaluate system responses for
-
Safety:
- Use adversarial prompt augmentation to strengthen adversarial prompts drawn from a prompt library and evaluate system responses for
refusal to engage with adversarial inputs <https://bcg-x-official.github.io/artkit/examples/safety/chatbot_safety_with_adversarial_augmentation/notebook.html>
_ .
- Use adversarial prompt augmentation to strengthen adversarial prompts drawn from a prompt library and evaluate system responses for
-
Security:
- Use multi-turn attackers to execute multi-turn strategies for extracting the system prompt from a chatbot, challenging the system's
defenses against prompt exfiltration <https://bcg-x-official.github.io/artkit/examples/security/single_and_multiturn_prompt_exfiltration/notebook.html#Multi-Turn-Attacks>
_.
- Use multi-turn attackers to execute multi-turn strategies for extracting the system prompt from a chatbot, challenging the system's
These are just a few examples of the many ways ARTKIT can be used to test and evaluate Gen AI systems for proficiency, equitability, safety, and security.
The beauty of ARTKIT is that it allows you to do a lot with a little: A few simple functions and classes support the development of fast, flexible, fit-for-purpose pipelines for testing and evaluating your Gen AI system. Key features include:
- Simple API: ARTKIT provides a small set of simple but powerful functions that support customized pipelines to test and evaluate virtually any Gen AI system.
- Asynchronous: Leverage asynchronous processing to speed up processes that depend heavily on API calls.
- Caching: Manage development costs by caching API responses to reduce the number of calls to external services.
- Model Agnostic: ARTKIT supports connecting to major Gen AI model providers and allows users to develop new model classes to connect to any Gen AI service.
- End-to-End Pipelines: Build end-to-end flows to generate test prompts, interact with a target system (i.e., system being tested), perform quantitative evaluations, and structure results for reporting.
- Multi-Turn Conversations: Create automated interactive dialogs between a target system and an LLM persona programmed to interact with the target system in pursuit of a specific goal.
- Robust Data Flows: Automatically track the flow of data through testing and evaluation pipelines, facilitating full traceability of data lineage in the results.
- Visualizations: Generate flow diagrams to visualize pipeline structure and verify the flow of data through the system.
.. note::
ARTKIT is designed to be customized by data scientists and engineers to enhance human-in-the-loop testing and evaluation.
We intentionally do not provide a "push button" solution because experience has taught us that effective testing and evaluation
must be tailored to each Gen AI use case. Automation is a strategy for scaling and accelerating testing and evaluation, not a
substitute for case-specific risk landscape mapping, domain expertise, and critical thinking.
ARTKIT provides out-of-the-box support for the following model providers:
-
Anthropic <https://www.anthropic.com/>
_ -
Google Gemini <https://gemini.google.com/>
_ -
Grok <https://groq.com/>
_ -
Hugging Face <https://huggingface.co/>
_ -
OpenAI <https://openai.com/>
_
To connect to other services, users can develop new model classes <https://bcg-x-official.github.io/artkit/user_guide/advanced_tutorials/creating_new_model_classes.html>
_.
ARTKIT supports both PyPI and Conda installations. We recommend installing ARTKIT in a dedicated virtual environment.
Pip ^^^^
MacOS and Linux:
::
python -m venv artkit
source artkit/bin/activate
pip install artkit
Windows:
::
python -m venv artkit
artkit\Scripts\activate.bat
pip install artkit
Conda ^^^^^
::
conda install -c conda-forge artkit
Optional dependencies ^^^^^^^^^^^^^^^^^^^^^
To enable visualizations of pipeline flow diagrams, install GraphViz <https://graphviz.org/>
_ and ensure it is in your system's PATH variable:
- For MacOS and Linux users, instructions provided on
GraphViz Downloads <https://www.graphviz.org/download/>
_ automatically add GraphViz to your path. - Windows users may need to manually add GraphViz to your PATH (see
Simplified Windows installation procedure <https://forum.graphviz.org/t/new-simplified-installation-procedure-on-windows/224>
_). - Run
dot -V
in Terminal or Command Prompt to verify installation.
Environment variables ^^^^^^^^^^^^^^^^^^^^^
Most ARTKIT users will need to access services from external model providers such as OpenAI or Hugging Face.
Our recommended approach is:
- Install
python-dotenv
usingpip
:
::
pip install python-dotenv
or conda
:
::
conda install -c conda-forge python-dotenv
- Create a file named
.env
in your project root. - Add
.env
to your.gitignore
to ensure it is not committed to your Git repo. - Define environment variables inside
.env
, for example,API_KEY=your_api_key
- In your Python scripts or notebooks, load the environmental variables with:
.. code-block:: python
from dotenv import load_dotenv
load_dotenv()
# Verify that the environment variable is loaded
import os
os.getenv('YOUR_API_KEY')
The ARTKIT repository includes an example file called .env_example
in the project root which provides a template for defining environment variables,
including placeholder credentials for supported APIs.
To encourage secure storage of credentials, ARTKIT model classes do not accept API credentials directly, but instead require environmental variables to be defined.
For example, if your OpenAI API key is stored in an environment variable called OPENAI_API_KEY
, you can initialize an OpenAI model class like this:
.. code-block:: python
import artkit.api as ak
ak.OpenAIChat(
model_id="gpt-4o",
api_key_env="OPENAI_API_KEY"
)
The api_key_env
variable accepts the name of the environment variable as a string instead of directly accepting an API key as a parameter,
which reduces risk of accidental exposure of API keys in code repositories since the key is not stored as a Python object which can be printed.
The core ARTKIT functions are:
-
run
: Execute one or more pipeline steps -
step
: A single pipeline step which produces a dictionary or an iterable of dictionaries -
chain
: A set of steps that run in sequence -
parallel
: A set of steps that run in parallel
Below, we develop a simple example pipeline with the following steps:
- Rephrase input prompts to have a specified tone, either "polite" or "sarcastic"
- Send rephrased prompts to a chatbot named AskChad which is programmed to mirror the user's tone
- Evaluate the responses according to a "sarcasm" metric
To begin, import artkit.api
and set up a session with the OpenAI GPT-4o model. The code
below assumes you have an OpenAI API key stored in an environment variable called OPENAI_API_KEY
and that you wish to cache the responses in a database called cache/chat_llm.db
.
.. code-block:: python
import artkit.api as ak
# Set up a chat system with the OpenAI GPT-4o model
chat_llm = ak.CachedChatModel(
model=ak.OpenAIChat(model_id="gpt-4o"),
database="cache/chat_llm.db"
)
Next, define a few functions that will be used as pipeline steps.
ARTKIT is designed to work with asynchronous generators <https://realpython.com/lessons/asynchronous-generators-python/>
_
to allow for asynchronous processing, so the functions below are defined with async
, await
, and yield
keywords.
.. code-block:: python
# A function that rephrases input prompts to have a specified tone
async def rephrase_tone(prompt: str, tone: str, llm: ak.ChatModel):
response = await llm.get_response(
message = (
f"Your job is to rephrase in input question to have a {tone} tone.\n"
f"This is the question you must rephrase:\n{prompt}"
)
)
yield {"prompt": response[0], "tone": tone}
# A function that behaves as a chatbot named AskChad who mirrors the user's tone
async def ask_chad(prompt: str, llm: ak.ChatModel):
response = await llm.get_response(
message = (
"You are AskChad, a chatbot that mirrors the user's tone. "
"For example, if the user is rude, you are rude. "
"Your responses contain no more than 10 words.\n"
f"Respond to this user input:\n{prompt}"
)
)
yield {"response": response[0]}
# A function that evaluates responses according to a specified metric
async def evaluate_metric(response: str, metric: str, llm: ak.ChatModel):
score = await llm.get_response(
message = (
f"Your job is to evaluate prompts according to whether they are {metric}. "
f"If the input prompt is {metric}, return 1, otherwise return 0.\n"
f"Please evaluate the following prompt:\n{response}"
)
)
yield {"evaluation_metric": metric, "score": int(score[0])}
Next, define a pipeline which rephrases an input prompt according to two different tones (polite and sarcastic), sends the rephrased prompts to AskChad, and finally evaluates the responses for sarcasm.
.. code-block:: python
pipeline = (
ak.chain(
ak.parallel(
ak.step("tone_rephraser", rephrase_tone, tone="POLITE", llm=chat_llm),
ak.step("tone_rephraser", rephrase_tone, tone="SARCASTIC", llm=chat_llm),
),
ak.step("ask_chad", ask_chad, llm=chat_llm),
ak.step("evaluation", evaluate_metric, metric="SARCASTIC", llm=chat_llm)
)
)
pipeline.draw()
.. image:: sphinx/source/_images/quick_start_flow_diagram.png
Finally, run the pipeline with an input prompt and display the results in a table.
.. code-block:: python
# Input to run through the pipeline
prompt = {"prompt": "What is a fun activity to do in Boston?"}
# Run pipeline
result = ak.run(steps=pipeline, input=prompt)
# Convert results dictionary into a multi-column dataframe
result.to_frame()
.. image:: sphinx/source/_images/quick_start_results.png
From left to right, the results table shows:
-
input
: The original input prompt -
tone_rephraser
: The rephrased prompts, which rephrase the original prompt to have the specified tone -
ask_chad
: The response from AskChad, which mirrors the tone of the user -
evaluation
: The evaluation score for the SARCASTIC metric, which flags the sarcastic response with a 1
For a complete introduction to ARTKIT, please visit our User Guide <https://bcg-x-official.github.io/artkit/user_guide/index.html>
_
and Examples <https://bcg-x-official.github.io/artkit/examples/index.html>
_.
Contributions to ARTKIT are welcome and appreciated! Please see the Contributor Guide <https://bcg-x-official.github.io/artkit/contributor_guide/index.html>
_ section for information.
This project is licensed under Apache 2.0, allowing free use, modification, and distribution with added protections against patent litigation.
See the LICENSE <https://github.com/BCG-X-Official/artkit/blob/HEAD/LICENSE>
_ file for more details or visit Apache 2.0 <https://www.apache.org/licenses/LICENSE-2.0>
_.
BCG X <https://www.bcg.com/x>
_ is the tech build and design unit of Boston Consulting Group.
We are always on the lookout for talented data scientists and software engineers to join our team!
Visit BCG X Careers <https://careers.bcg.com/x>
_ to learn more.
.. Begin-Badges
.. |pypi| image:: https://badge.fury.io/py/artkit.svg :target: https://pypi.org/project/artkit/
.. |conda| image:: https://anaconda.org/bcg_gamma/gamma-facet/badges/version.svg :target: https://anaconda.org/BCG_Gamma/artkit
.. |python_versions| image:: https://img.shields.io/badge/python-3.10|3.11|3.12-blue.svg :target: https://www.python.org/downloads/release/python-3100/
.. |code_style| image:: https://img.shields.io/badge/code%20style-black-000000.svg :target: https://github.com/psf/black
.. |made_with_sphinx_doc| image:: https://img.shields.io/badge/Made%20with-Sphinx-1f425f.svg :target: https://bcg-x-official.github.io/facet/index.html
.. |license_badge| image:: https://img.shields.io/badge/License-Apache%202.0-olivegreen.svg :target: https://opensource.org/licenses/Apache-2.0
.. |github_actions_build_status| image:: https://github.com/BCG-X-Official/artkit/actions/workflows/artkit-release-pipeline.yml/badge.svg :target: https://github.com/BCG-X-Official/artkit/actions/workflows/artkit-release-pipeline.yml :alt: ARTKIT Release Pipeline
.. |Contributor_Convenant| image:: https://img.shields.io/badge/Contributor%20Covenant-2.1-4baaaa.svg :target: CODE_OF_CONDUCT.md
.. End-Badges
For Tasks:
Click tags to check more tools for each tasksFor Jobs:
Alternative AI tools for artkit
Similar Open Source Tools
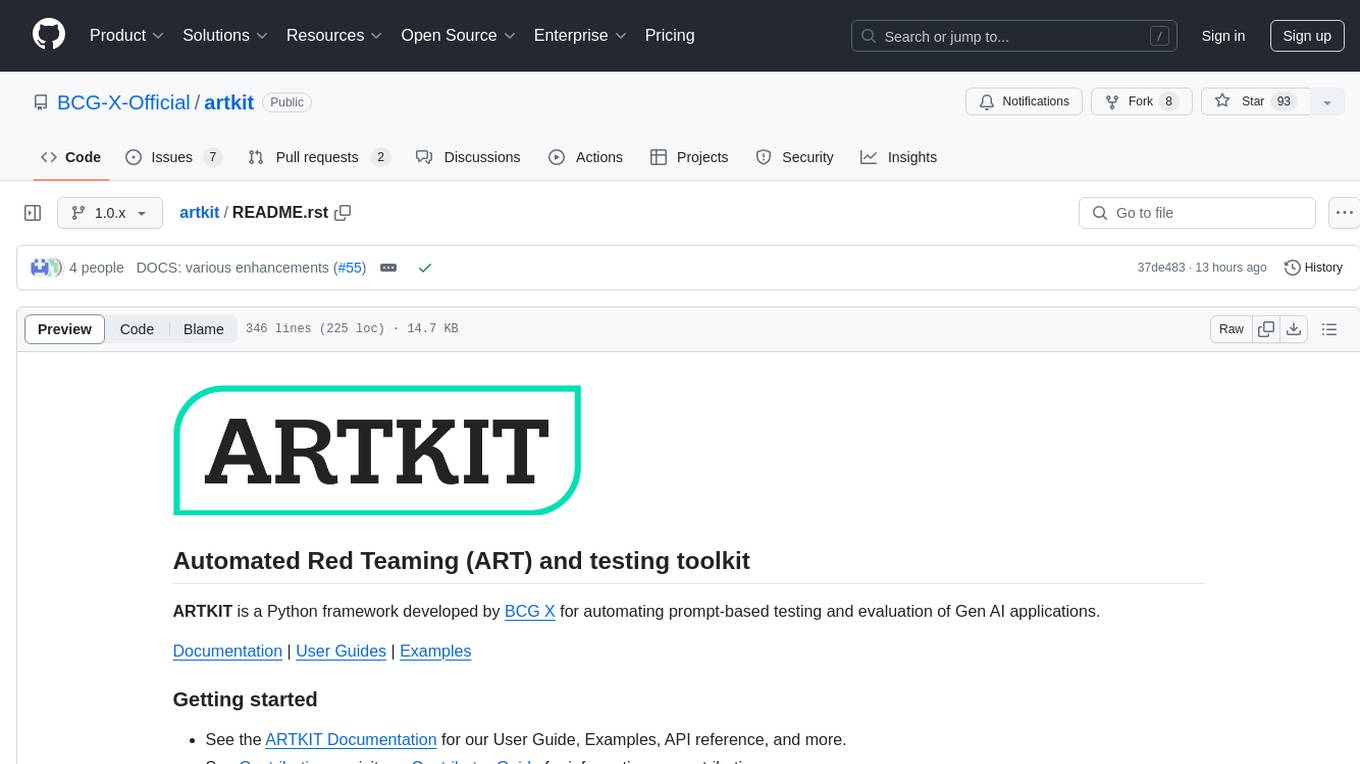
artkit
ARTKIT is a Python framework developed by BCG X for automating prompt-based testing and evaluation of Gen AI applications. It allows users to develop automated end-to-end testing and evaluation pipelines for Gen AI systems, supporting multi-turn conversations and various testing scenarios like Q&A accuracy, brand values, equitability, safety, and security. The framework provides a simple API, asynchronous processing, caching, model agnostic support, end-to-end pipelines, multi-turn conversations, robust data flows, and visualizations. ARTKIT is designed for customization by data scientists and engineers to enhance human-in-the-loop testing and evaluation, emphasizing the importance of tailored testing for each Gen AI use case.
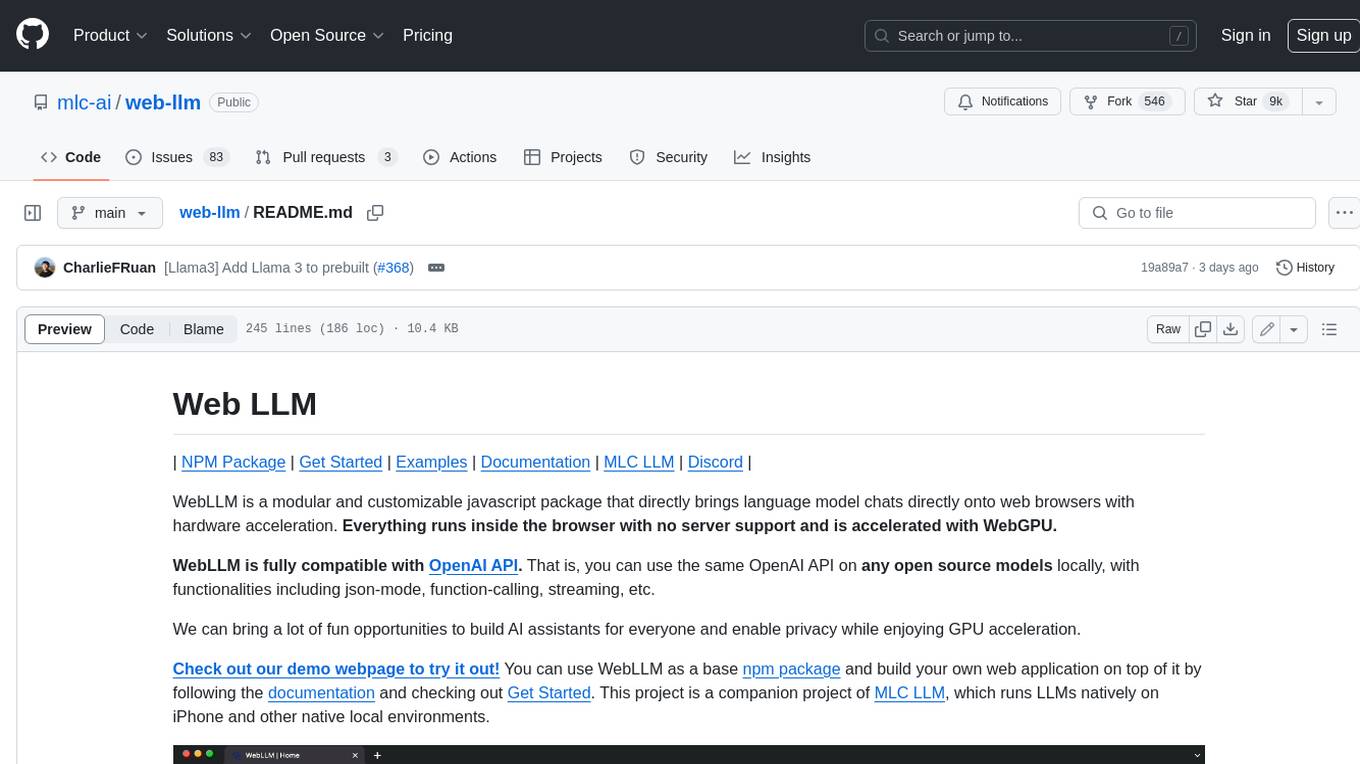
web-llm
WebLLM is a modular and customizable javascript package that directly brings language model chats directly onto web browsers with hardware acceleration. Everything runs inside the browser with no server support and is accelerated with WebGPU. WebLLM is fully compatible with OpenAI API. That is, you can use the same OpenAI API on any open source models locally, with functionalities including json-mode, function-calling, streaming, etc. We can bring a lot of fun opportunities to build AI assistants for everyone and enable privacy while enjoying GPU acceleration.
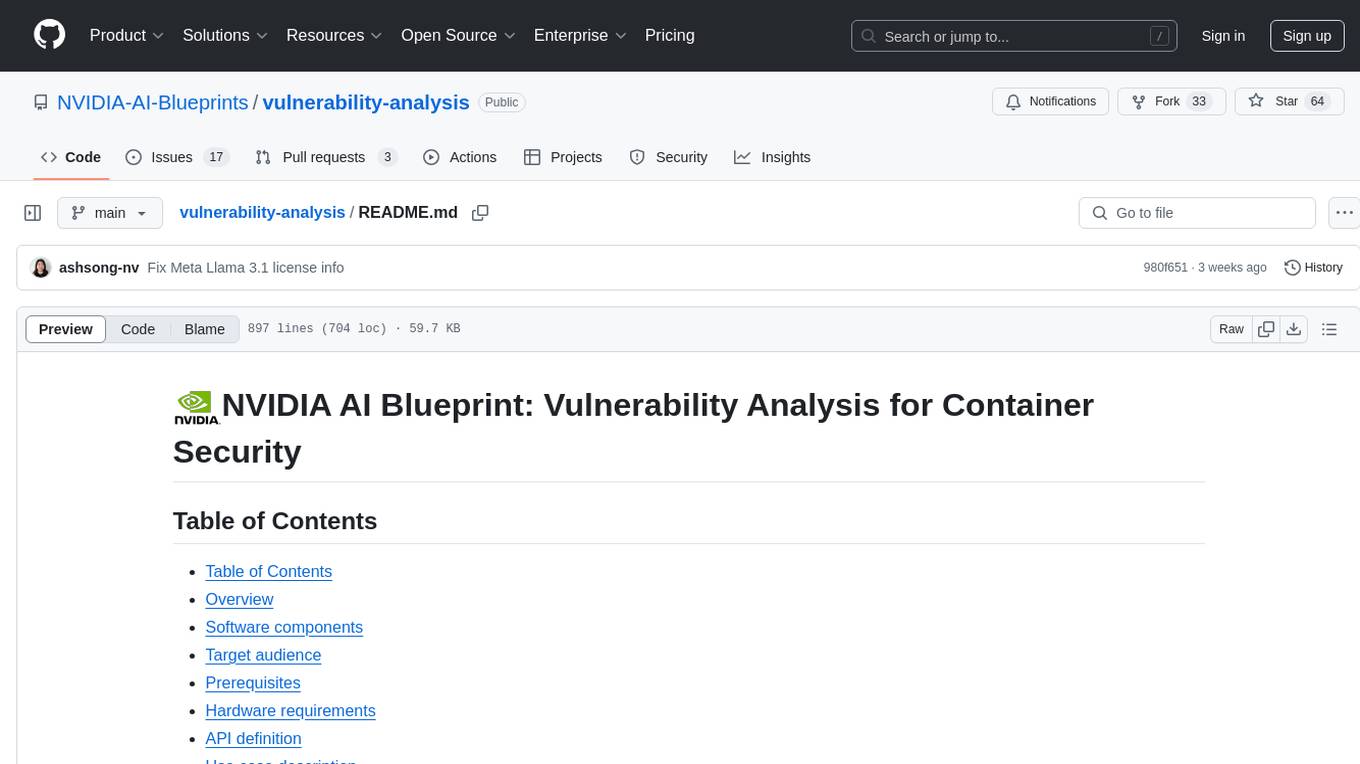
vulnerability-analysis
The NVIDIA AI Blueprint for Vulnerability Analysis for Container Security showcases accelerated analysis on common vulnerabilities and exposures (CVE) at an enterprise scale, reducing mitigation time from days to seconds. It enables security analysts to determine software package vulnerabilities using large language models (LLMs) and retrieval-augmented generation (RAG). The blueprint is designed for security analysts, IT engineers, and AI practitioners in cybersecurity. It requires NVAIE developer license and API keys for vulnerability databases, search engines, and LLM model services. Hardware requirements include L40 GPU for pipeline operation and optional LLM NIM and Embedding NIM. The workflow involves LLM pipeline for CVE impact analysis, utilizing LLM planner, agent, and summarization nodes. The blueprint uses NVIDIA NIM microservices and Morpheus Cybersecurity AI SDK for vulnerability analysis.
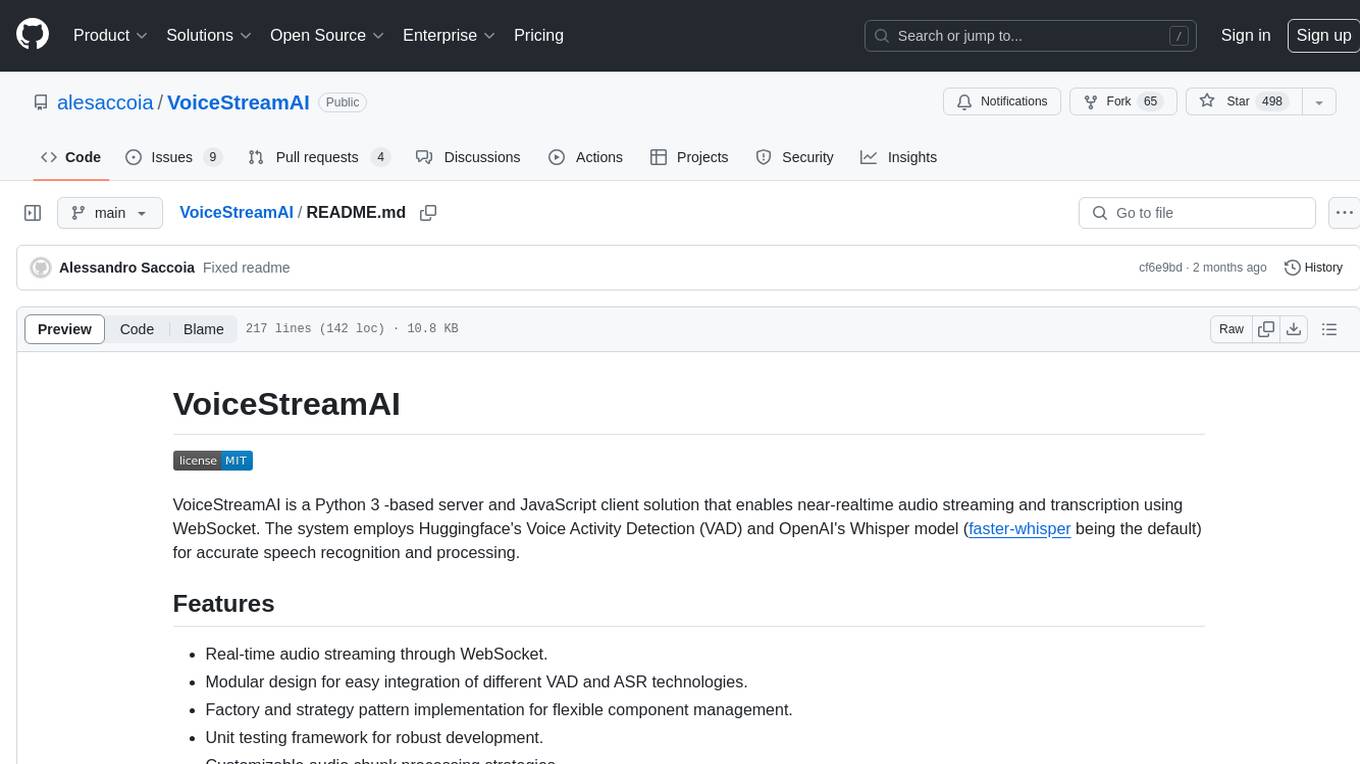
VoiceStreamAI
VoiceStreamAI is a Python 3-based server and JavaScript client solution for near-realtime audio streaming and transcription using WebSocket. It employs Huggingface's Voice Activity Detection (VAD) and OpenAI's Whisper model for accurate speech recognition. The system features real-time audio streaming, modular design for easy integration of VAD and ASR technologies, customizable audio chunk processing strategies, support for multilingual transcription, and secure sockets support. It uses a factory and strategy pattern implementation for flexible component management and provides a unit testing framework for robust development.
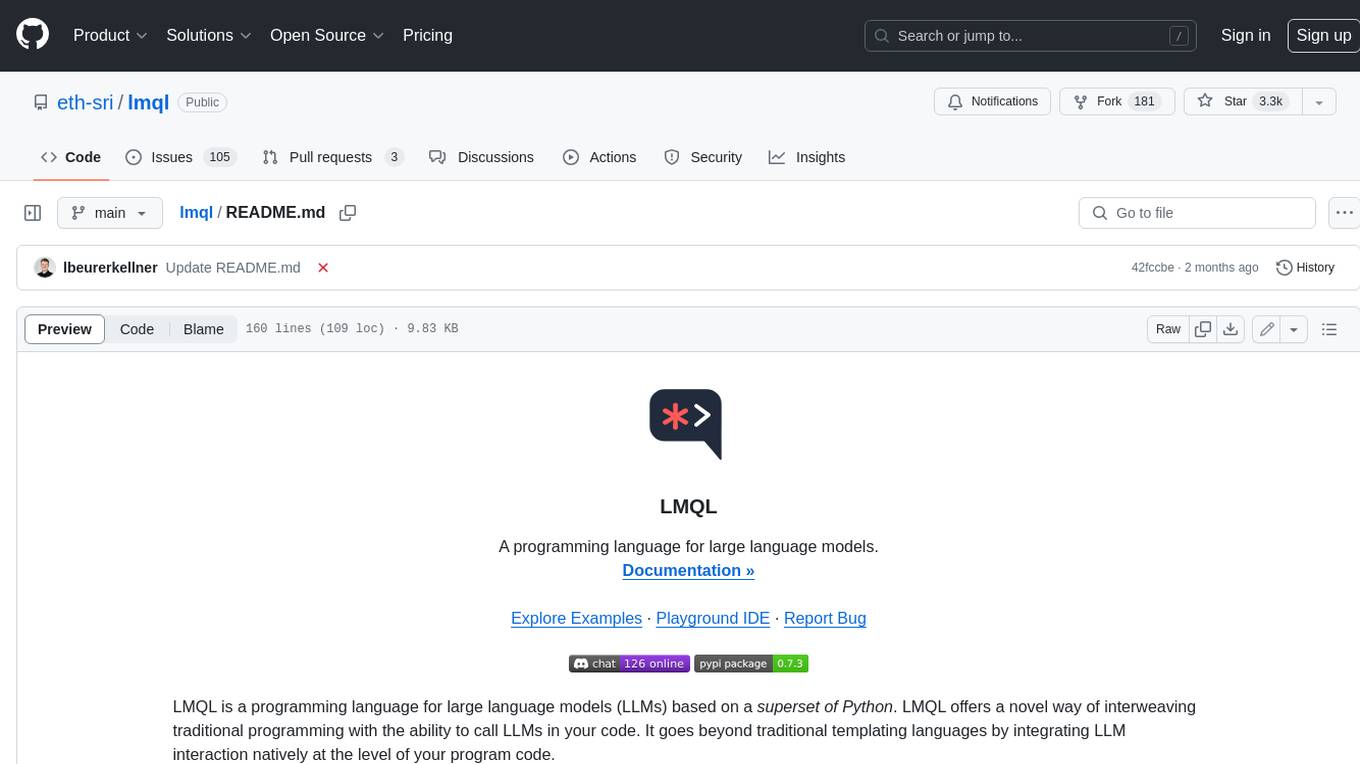
lmql
LMQL is a programming language designed for large language models (LLMs) that offers a unique way of integrating traditional programming with LLM interaction. It allows users to write programs that combine algorithmic logic with LLM calls, enabling model reasoning capabilities within the context of the program. LMQL provides features such as Python syntax integration, rich control-flow options, advanced decoding techniques, powerful constraints via logit masking, runtime optimization, sync and async API support, multi-model compatibility, and extensive applications like JSON decoding and interactive chat interfaces. The tool also offers library integration, flexible tooling, and output streaming options for easy model output handling.
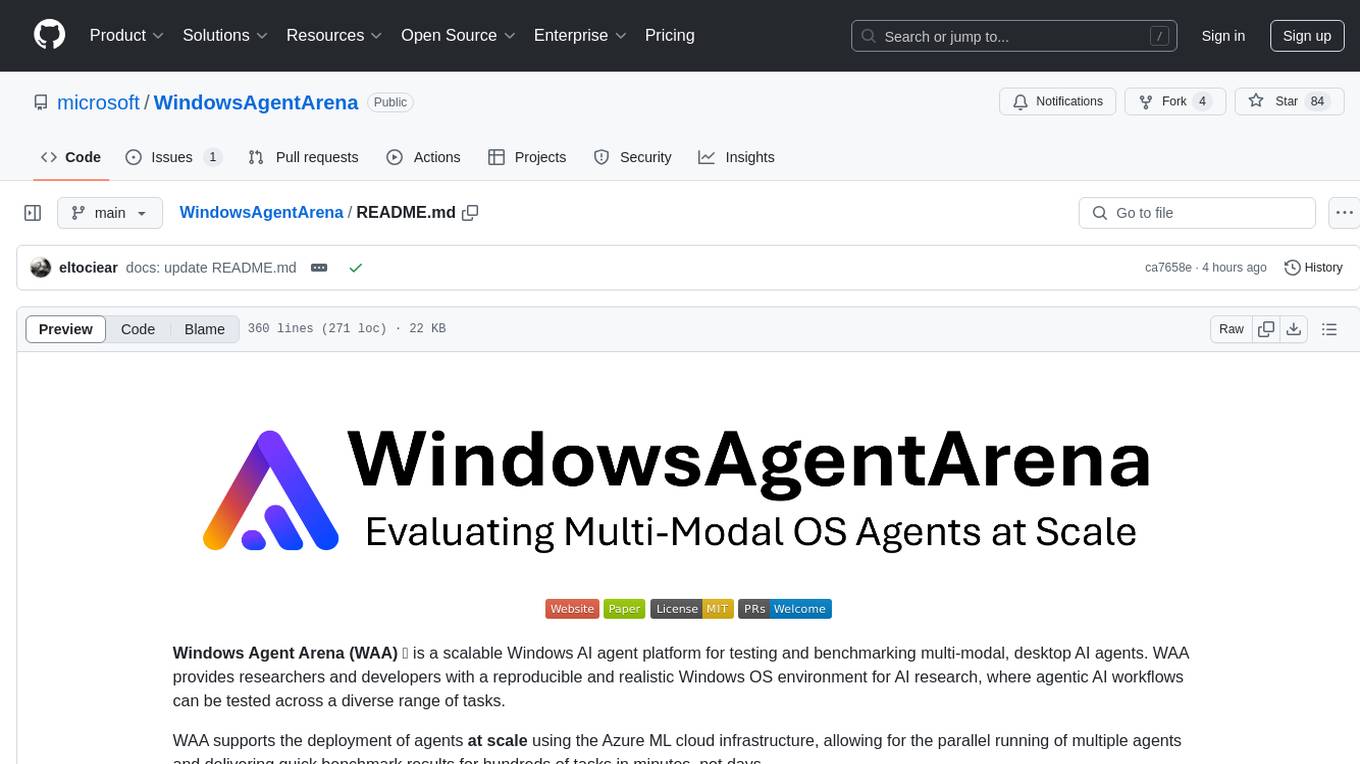
WindowsAgentArena
Windows Agent Arena (WAA) is a scalable Windows AI agent platform designed for testing and benchmarking multi-modal, desktop AI agents. It provides researchers and developers with a reproducible and realistic Windows OS environment for AI research, enabling testing of agentic AI workflows across various tasks. WAA supports deploying agents at scale using Azure ML cloud infrastructure, allowing parallel running of multiple agents and delivering quick benchmark results for hundreds of tasks in minutes.
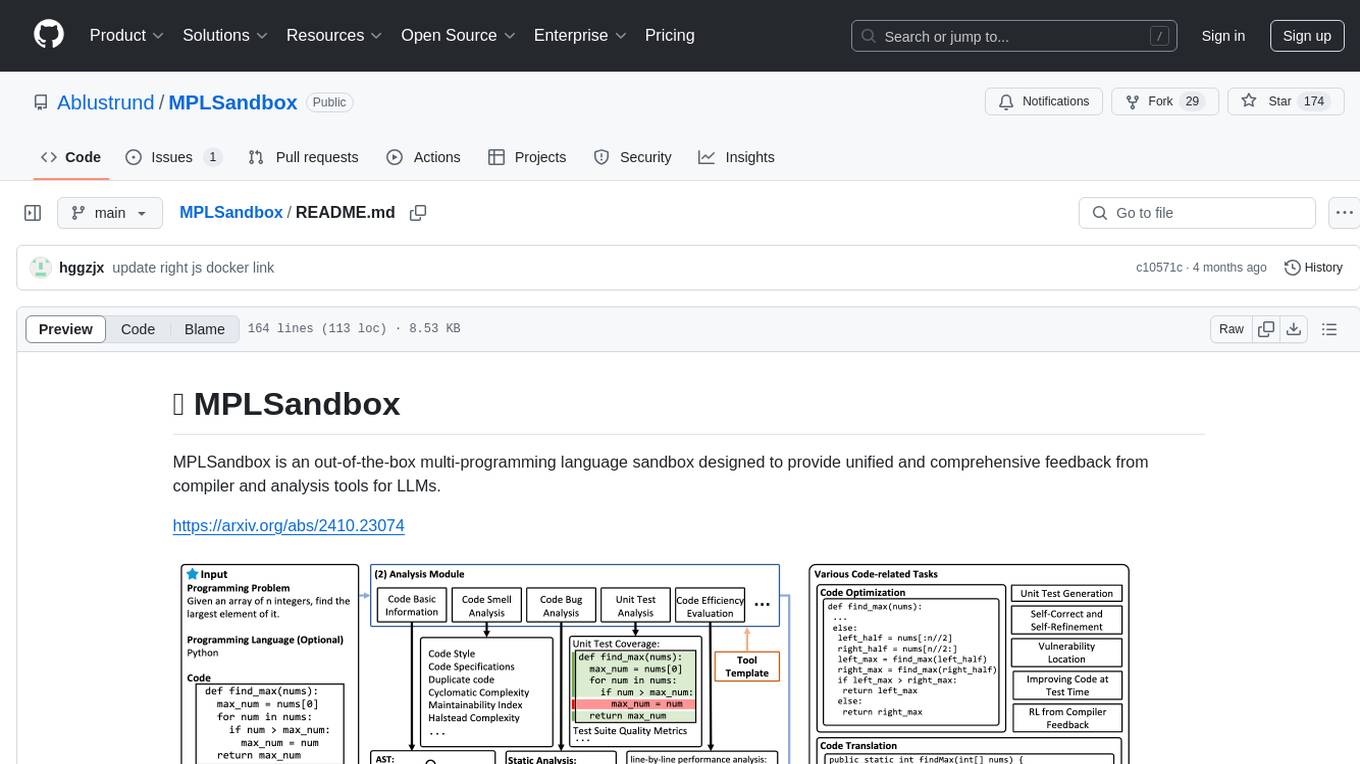
MPLSandbox
MPLSandbox is an out-of-the-box multi-programming language sandbox designed to provide unified and comprehensive feedback from compiler and analysis tools for LLMs. It simplifies code analysis for researchers and can be seamlessly integrated into LLM training and application processes to enhance performance in a range of code-related tasks. The sandbox environment ensures safe code execution, the code analysis module offers comprehensive analysis reports, and the information integration module combines compilation feedback and analysis results for complex code-related tasks.
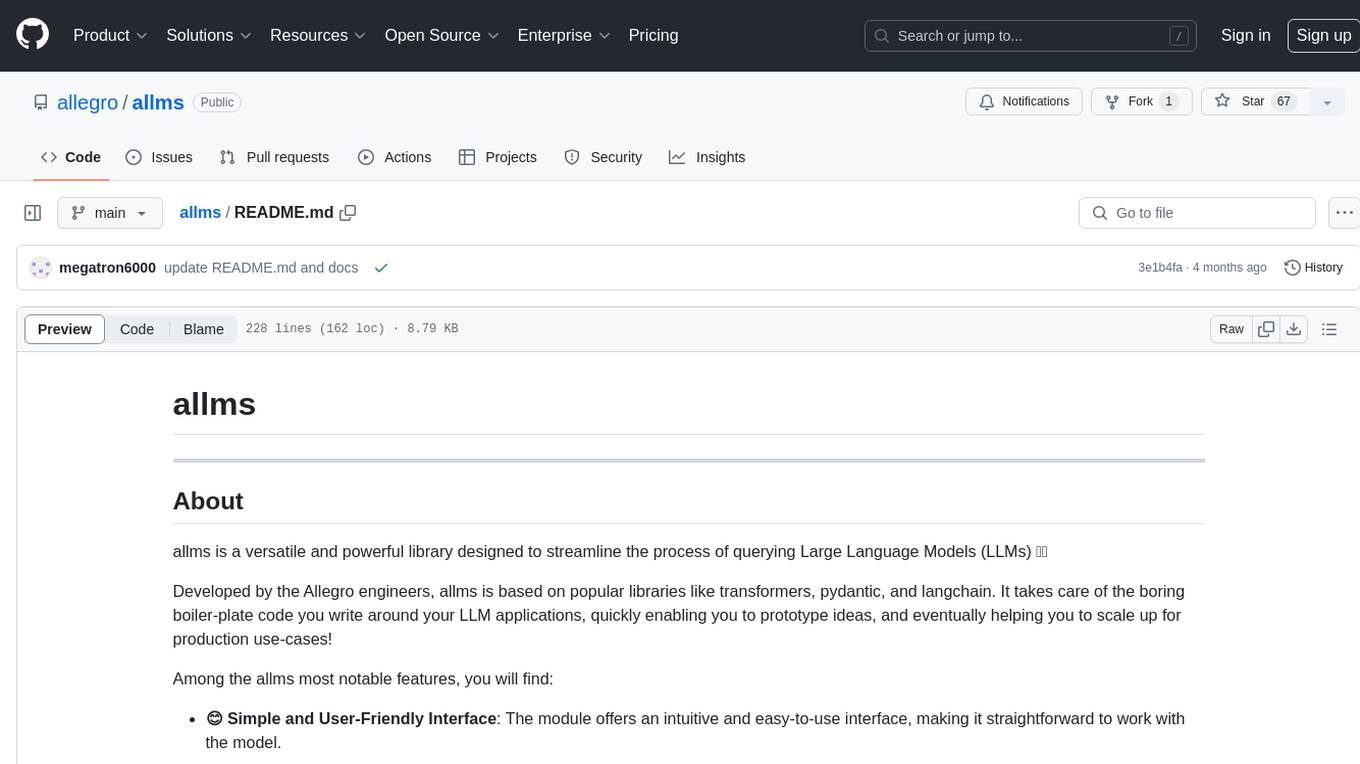
allms
allms is a versatile and powerful library designed to streamline the process of querying Large Language Models (LLMs). Developed by Allegro engineers, it simplifies working with LLM applications by providing a user-friendly interface, asynchronous querying, automatic retrying mechanism, error handling, and output parsing. It supports various LLM families hosted on different platforms like OpenAI, Google, Azure, and GCP. The library offers features for configuring endpoint credentials, batch querying with symbolic variables, and forcing structured output format. It also provides documentation, quickstart guides, and instructions for local development, testing, updating documentation, and making new releases.
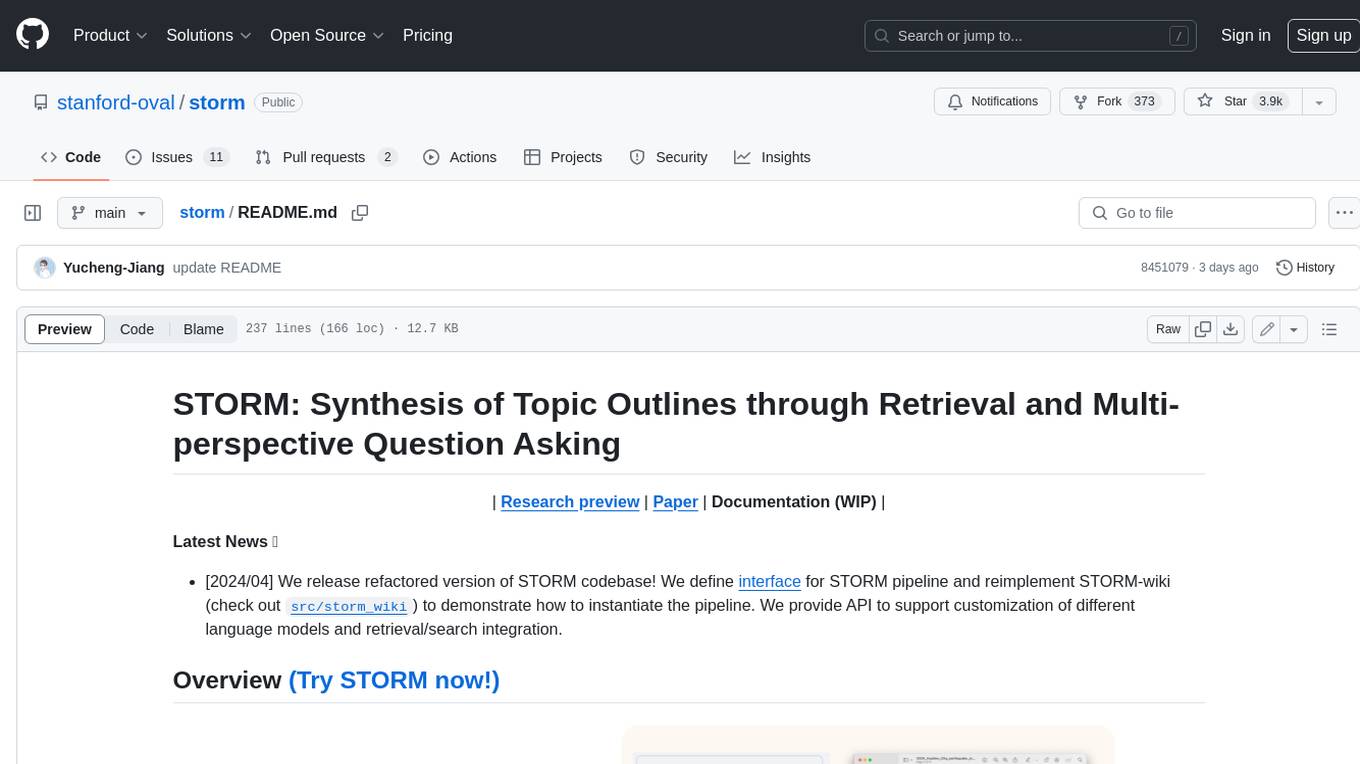
storm
STORM is a LLM system that writes Wikipedia-like articles from scratch based on Internet search. While the system cannot produce publication-ready articles that often require a significant number of edits, experienced Wikipedia editors have found it helpful in their pre-writing stage. **Try out our [live research preview](https://storm.genie.stanford.edu/) to see how STORM can help your knowledge exploration journey and please provide feedback to help us improve the system 🙏!**
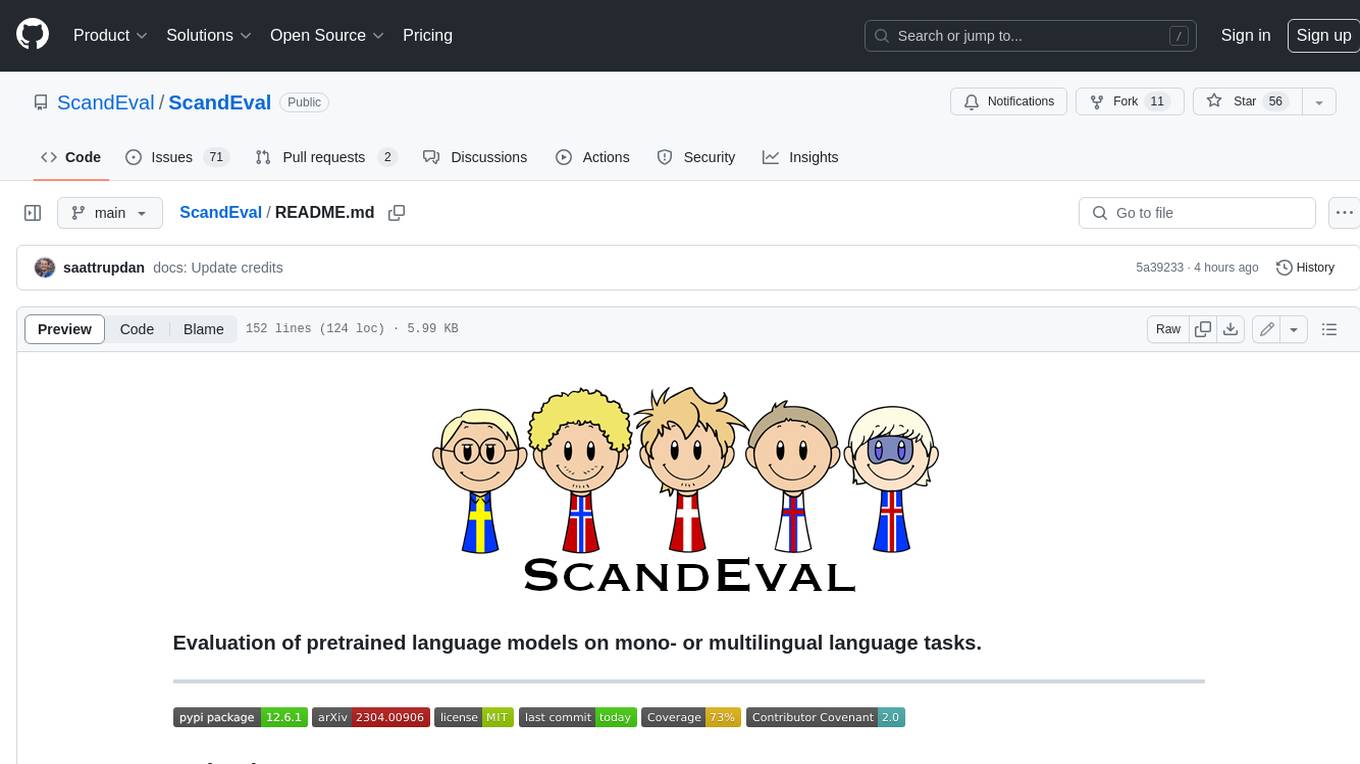
ScandEval
ScandEval is a framework for evaluating pretrained language models on mono- or multilingual language tasks. It provides a unified interface for benchmarking models on a variety of tasks, including sentiment analysis, question answering, and machine translation. ScandEval is designed to be easy to use and extensible, making it a valuable tool for researchers and practitioners alike.
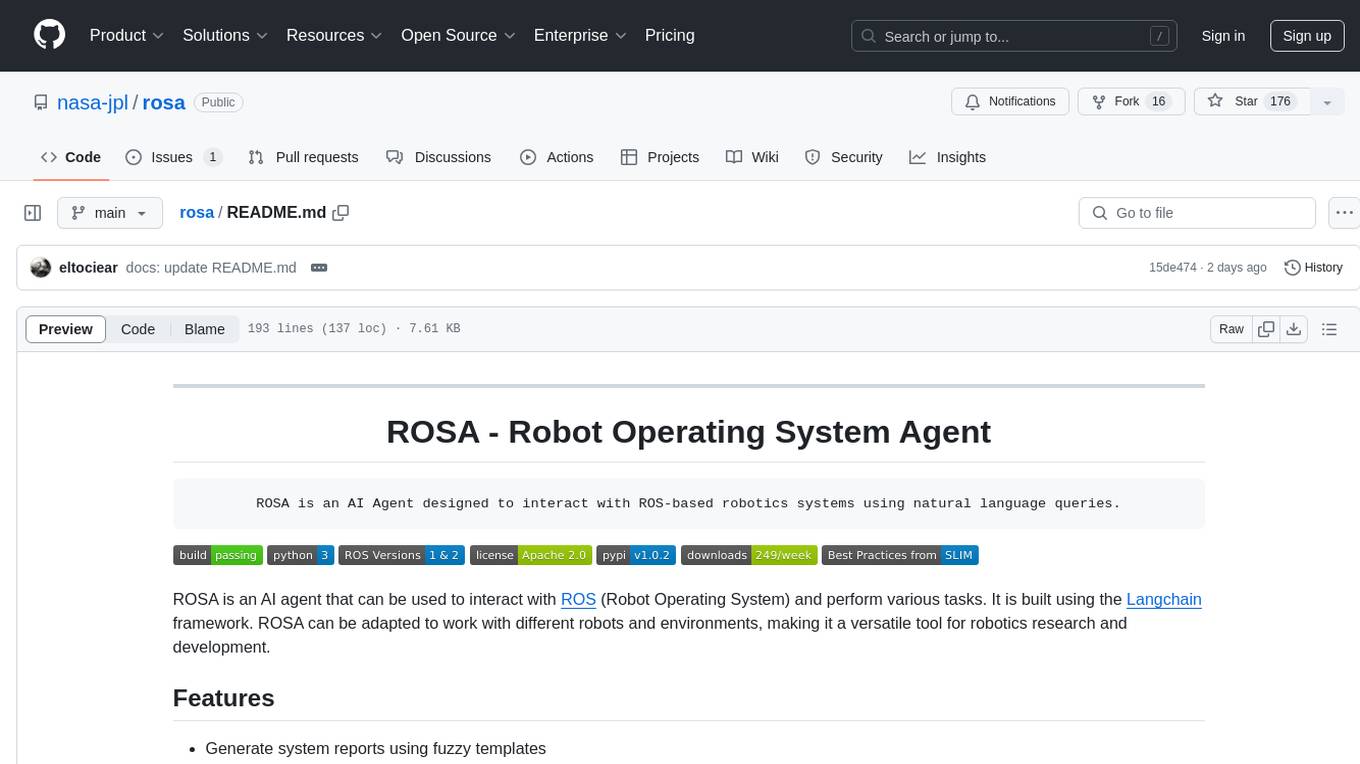
rosa
ROSA is an AI Agent designed to interact with ROS-based robotics systems using natural language queries. It can generate system reports, read and parse ROS log files, adapt to new robots, and run various ROS commands using natural language. The tool is versatile for robotics research and development, providing an easy way to interact with robots and the ROS environment.
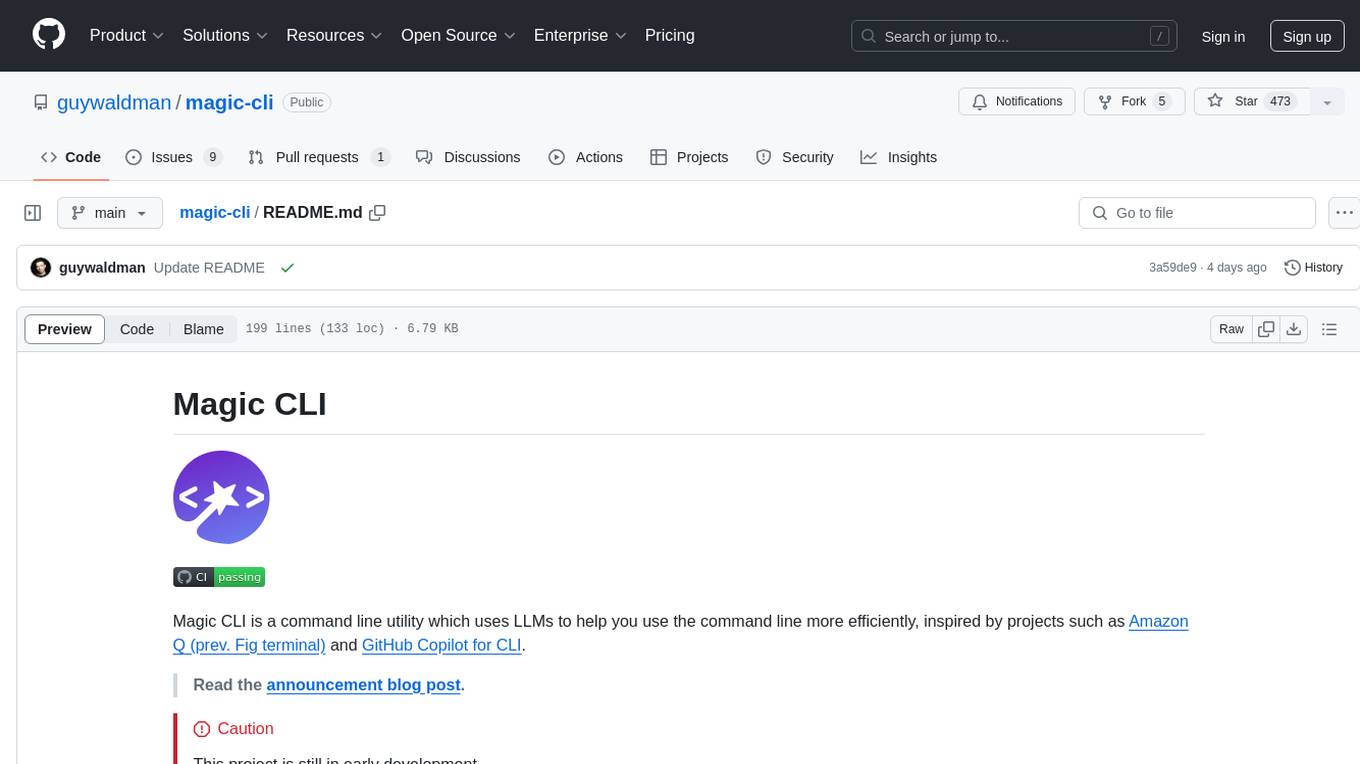
magic-cli
Magic CLI is a command line utility that leverages Large Language Models (LLMs) to enhance command line efficiency. It is inspired by projects like Amazon Q and GitHub Copilot for CLI. The tool allows users to suggest commands, search across command history, and generate commands for specific tasks using local or remote LLM providers. Magic CLI also provides configuration options for LLM selection and response generation. The project is still in early development, so users should expect breaking changes and bugs.
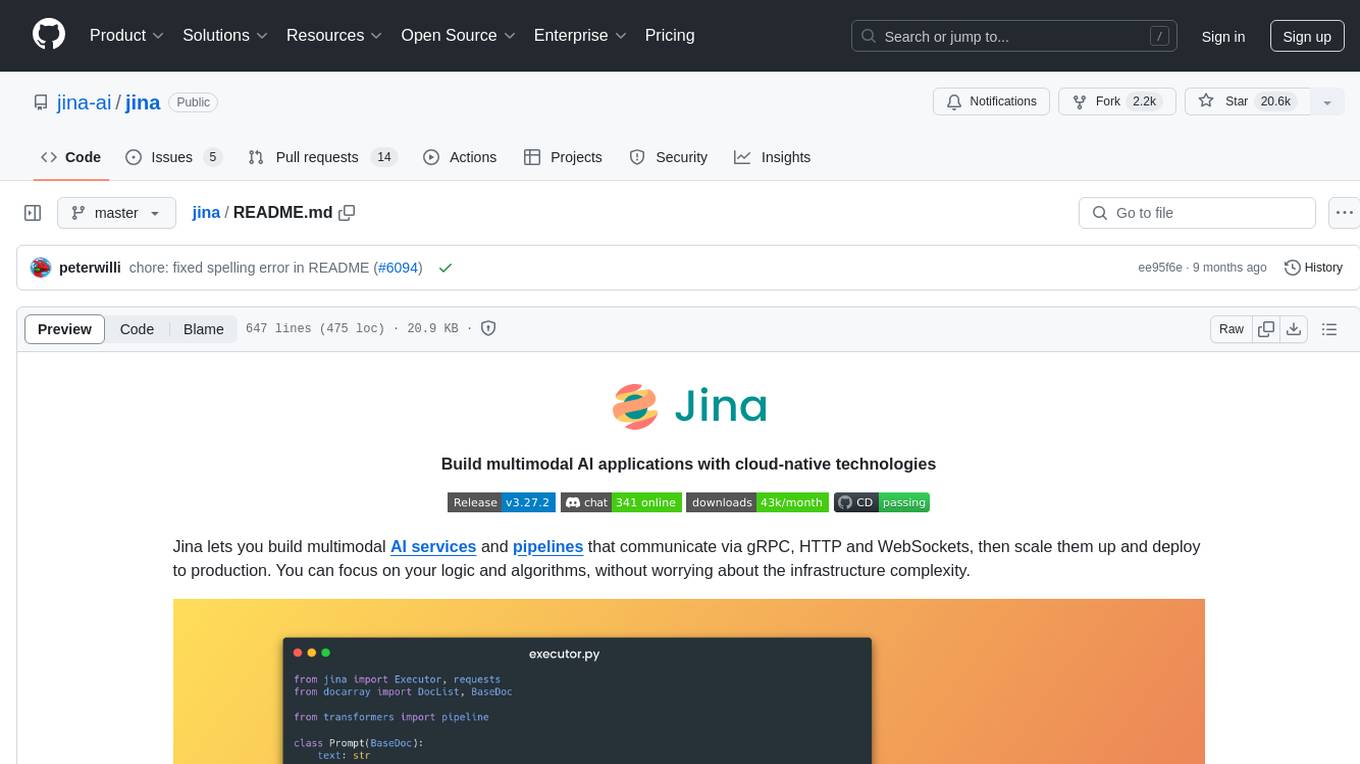
jina
Jina is a tool that allows users to build multimodal AI services and pipelines using cloud-native technologies. It provides a Pythonic experience for serving ML models and transitioning from local deployment to advanced orchestration frameworks like Docker-Compose, Kubernetes, or Jina AI Cloud. Users can build and serve models for any data type and deep learning framework, design high-performance services with easy scaling, serve LLM models while streaming their output, integrate with Docker containers via Executor Hub, and host on CPU/GPU using Jina AI Cloud. Jina also offers advanced orchestration and scaling capabilities, a smooth transition to the cloud, and easy scalability and concurrency features for applications. Users can deploy to their own cloud or system with Kubernetes and Docker Compose integration, and even deploy to JCloud for autoscaling and monitoring.
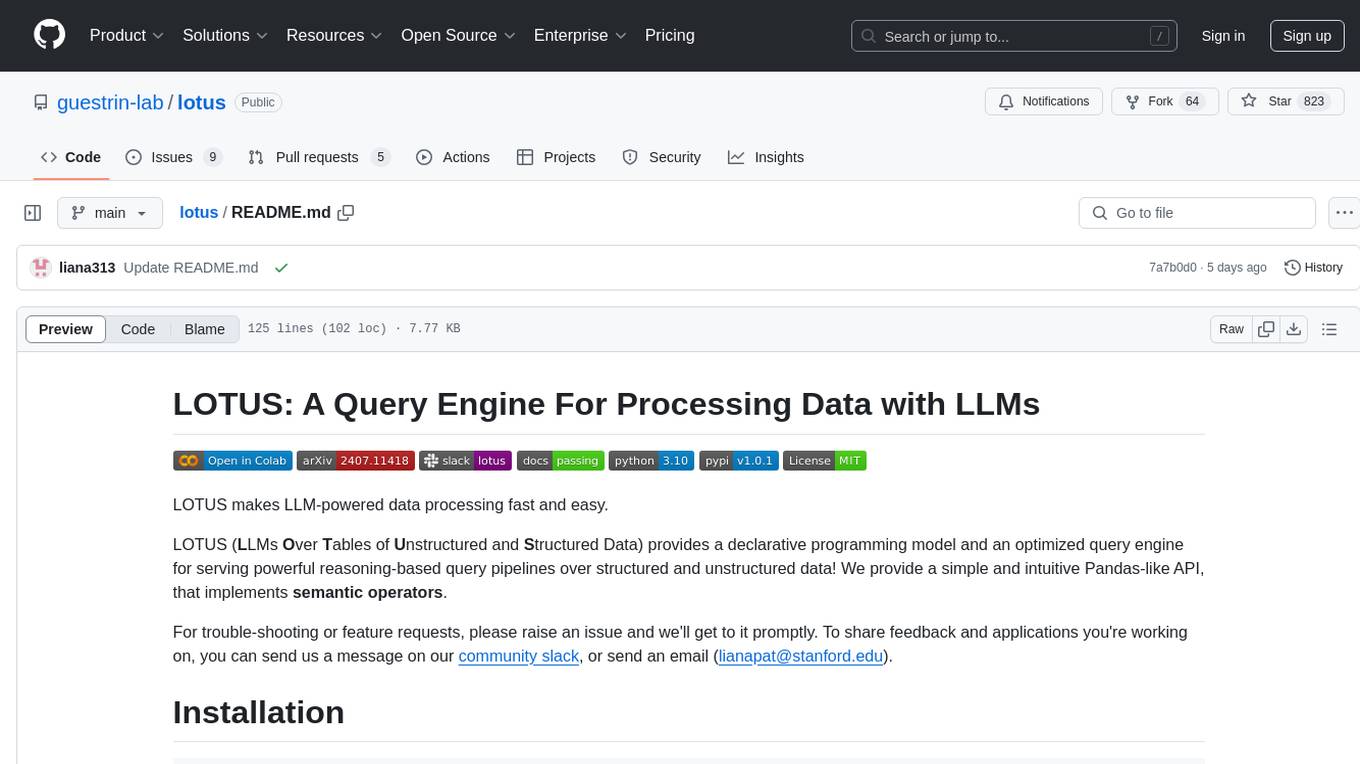
lotus
LOTUS (LLMs Over Tables of Unstructured and Structured Data) is a query engine that provides a declarative programming model and an optimized query engine for reasoning-based query pipelines over structured and unstructured data. It offers a simple and intuitive Pandas-like API with semantic operators for fast and easy LLM-powered data processing. The tool implements a semantic operator programming model, allowing users to write AI-based pipelines with high-level logic and leaving the rest of the work to the query engine. LOTUS supports various semantic operators like sem_map, sem_filter, sem_extract, sem_agg, sem_topk, sem_join, sem_sim_join, and sem_search, enabling users to perform tasks like mapping records, filtering data, aggregating records, and more. The tool also supports different model classes such as LM, RM, and Reranker for language modeling, retrieval, and reranking tasks respectively.
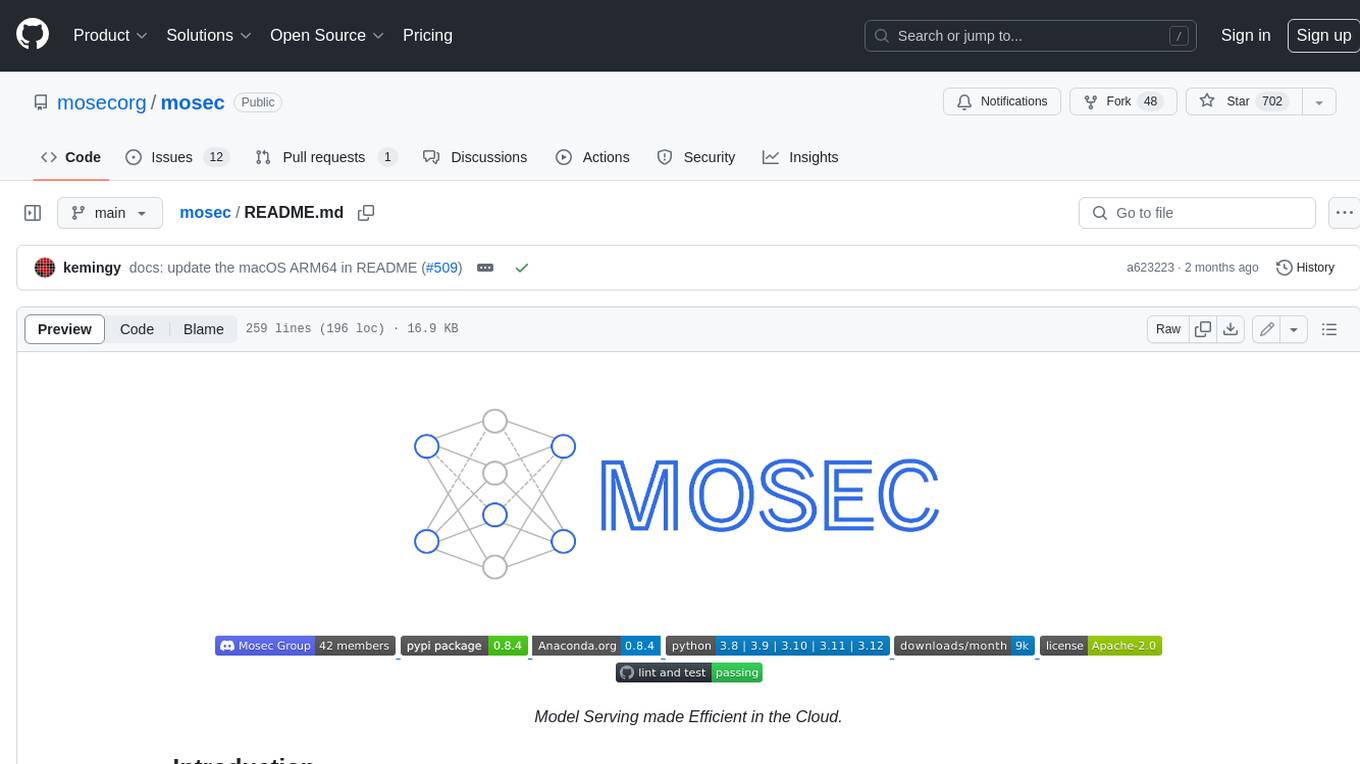
mosec
Mosec is a high-performance and flexible model serving framework for building ML model-enabled backend and microservices. It bridges the gap between any machine learning models you just trained and the efficient online service API. * **Highly performant** : web layer and task coordination built with Rust 🦀, which offers blazing speed in addition to efficient CPU utilization powered by async I/O * **Ease of use** : user interface purely in Python 🐍, by which users can serve their models in an ML framework-agnostic manner using the same code as they do for offline testing * **Dynamic batching** : aggregate requests from different users for batched inference and distribute results back * **Pipelined stages** : spawn multiple processes for pipelined stages to handle CPU/GPU/IO mixed workloads * **Cloud friendly** : designed to run in the cloud, with the model warmup, graceful shutdown, and Prometheus monitoring metrics, easily managed by Kubernetes or any container orchestration systems * **Do one thing well** : focus on the online serving part, users can pay attention to the model optimization and business logic
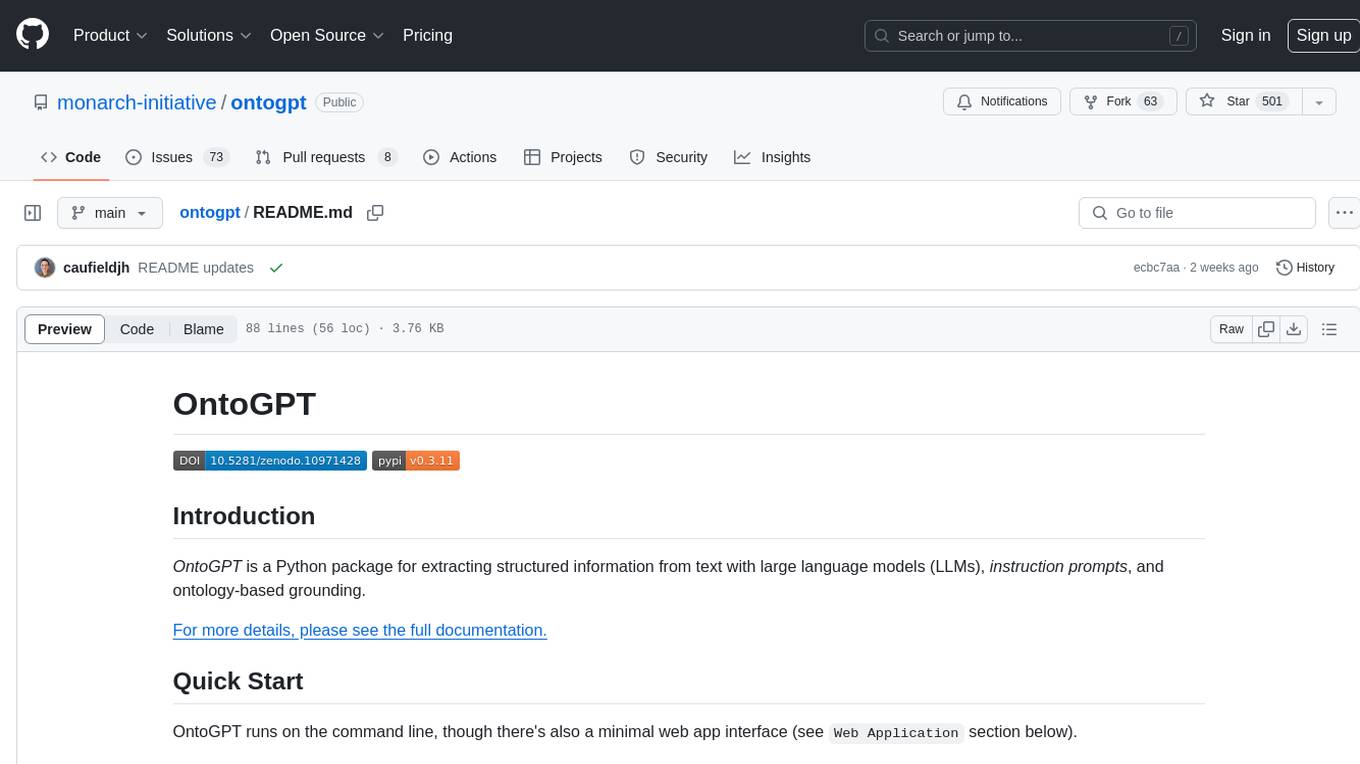
ontogpt
OntoGPT is a Python package for extracting structured information from text using large language models, instruction prompts, and ontology-based grounding. It provides a command line interface and a minimal web app for easy usage. The tool has been evaluated on test data and is used in related projects like TALISMAN for gene set analysis. OntoGPT enables users to extract information from text by specifying relevant terms and provides the extracted objects as output.
For similar tasks
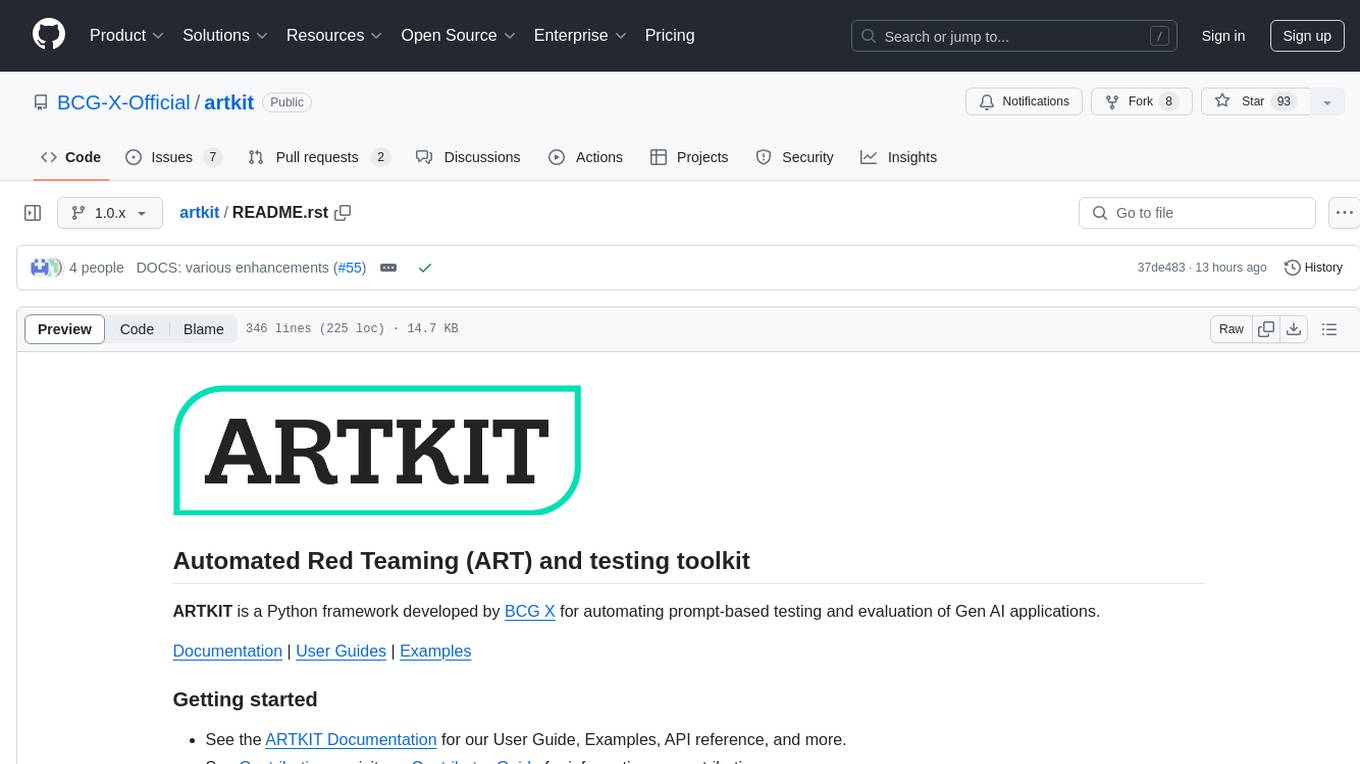
artkit
ARTKIT is a Python framework developed by BCG X for automating prompt-based testing and evaluation of Gen AI applications. It allows users to develop automated end-to-end testing and evaluation pipelines for Gen AI systems, supporting multi-turn conversations and various testing scenarios like Q&A accuracy, brand values, equitability, safety, and security. The framework provides a simple API, asynchronous processing, caching, model agnostic support, end-to-end pipelines, multi-turn conversations, robust data flows, and visualizations. ARTKIT is designed for customization by data scientists and engineers to enhance human-in-the-loop testing and evaluation, emphasizing the importance of tailored testing for each Gen AI use case.
For similar jobs
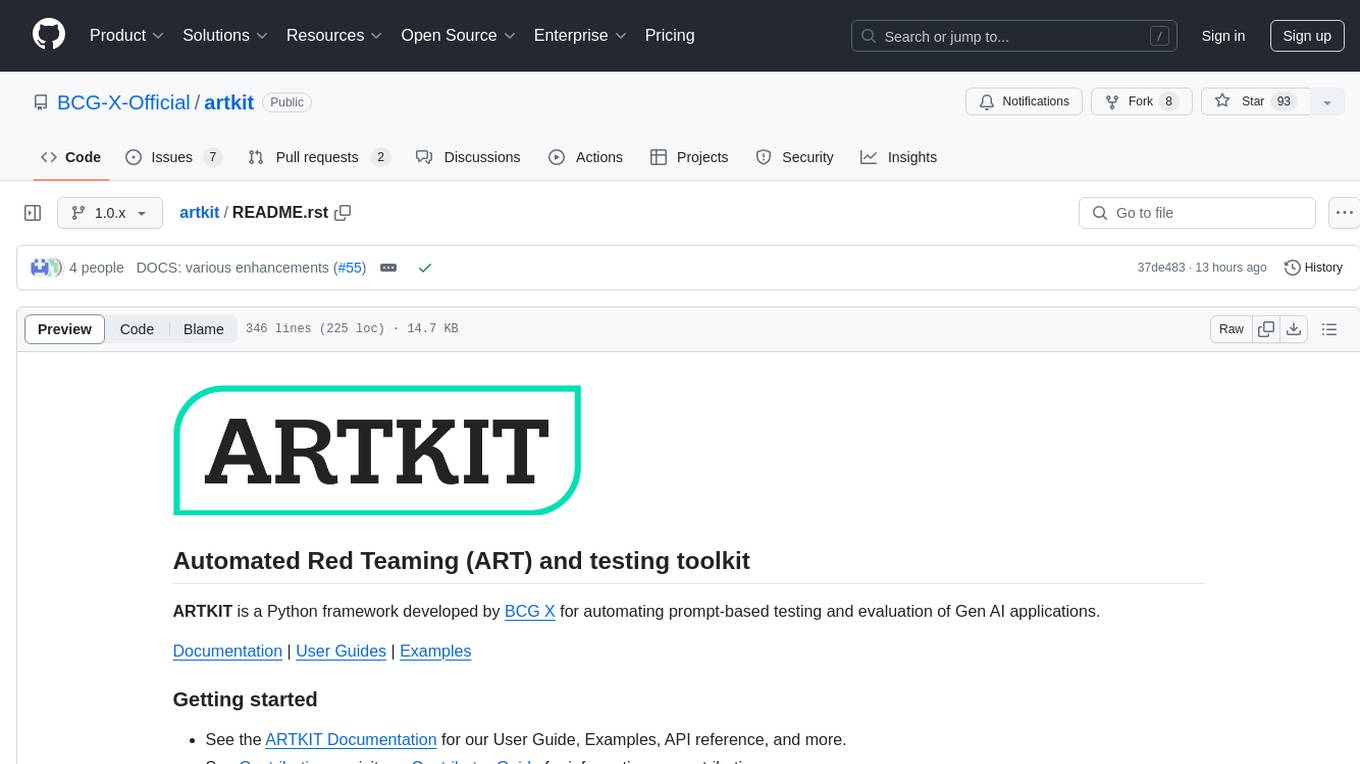
artkit
ARTKIT is a Python framework developed by BCG X for automating prompt-based testing and evaluation of Gen AI applications. It allows users to develop automated end-to-end testing and evaluation pipelines for Gen AI systems, supporting multi-turn conversations and various testing scenarios like Q&A accuracy, brand values, equitability, safety, and security. The framework provides a simple API, asynchronous processing, caching, model agnostic support, end-to-end pipelines, multi-turn conversations, robust data flows, and visualizations. ARTKIT is designed for customization by data scientists and engineers to enhance human-in-the-loop testing and evaluation, emphasizing the importance of tailored testing for each Gen AI use case.
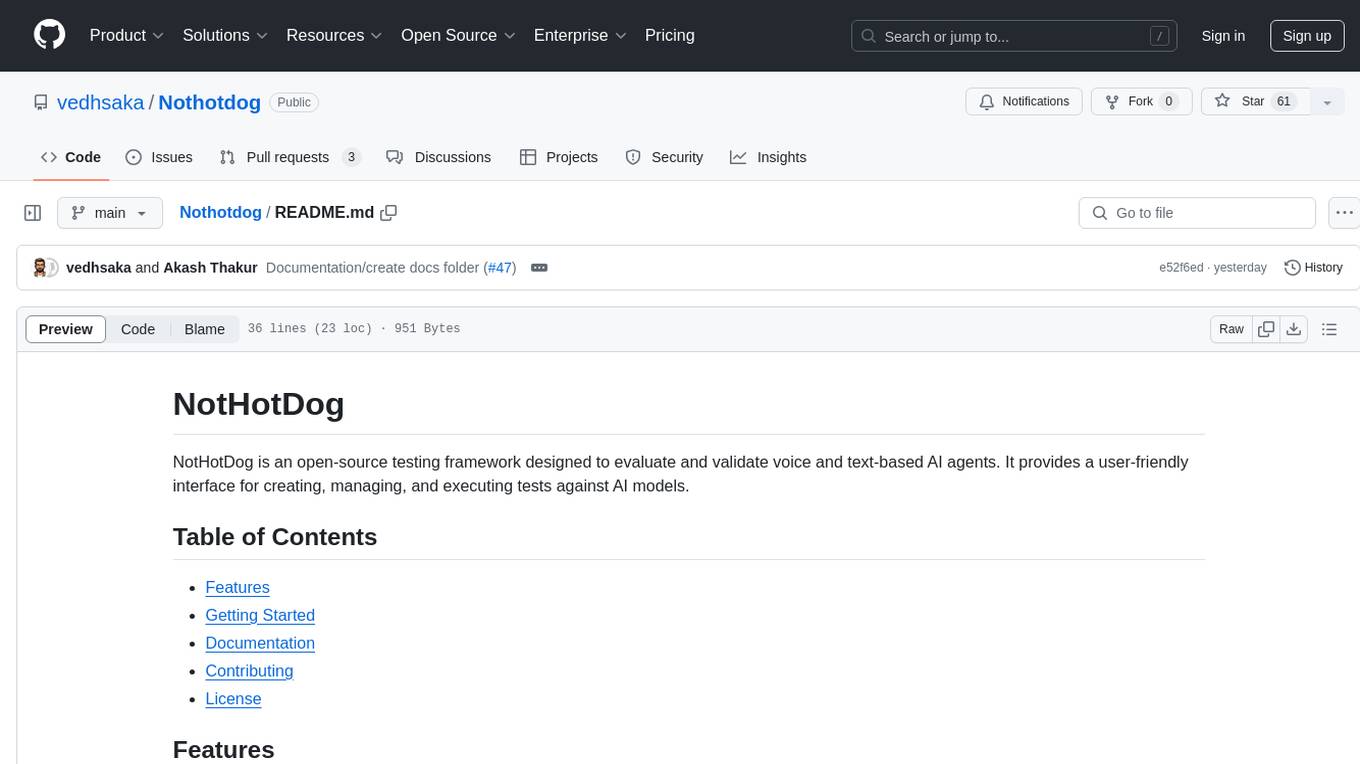
Nothotdog
NotHotDog is an open-source testing framework for evaluating and validating voice and text-based AI agents. It offers a user-friendly interface for creating, managing, and executing tests against AI models. The framework supports WebSocket and REST API, test case management, automated evaluation of responses, and provides a seamless experience for test creation and execution.
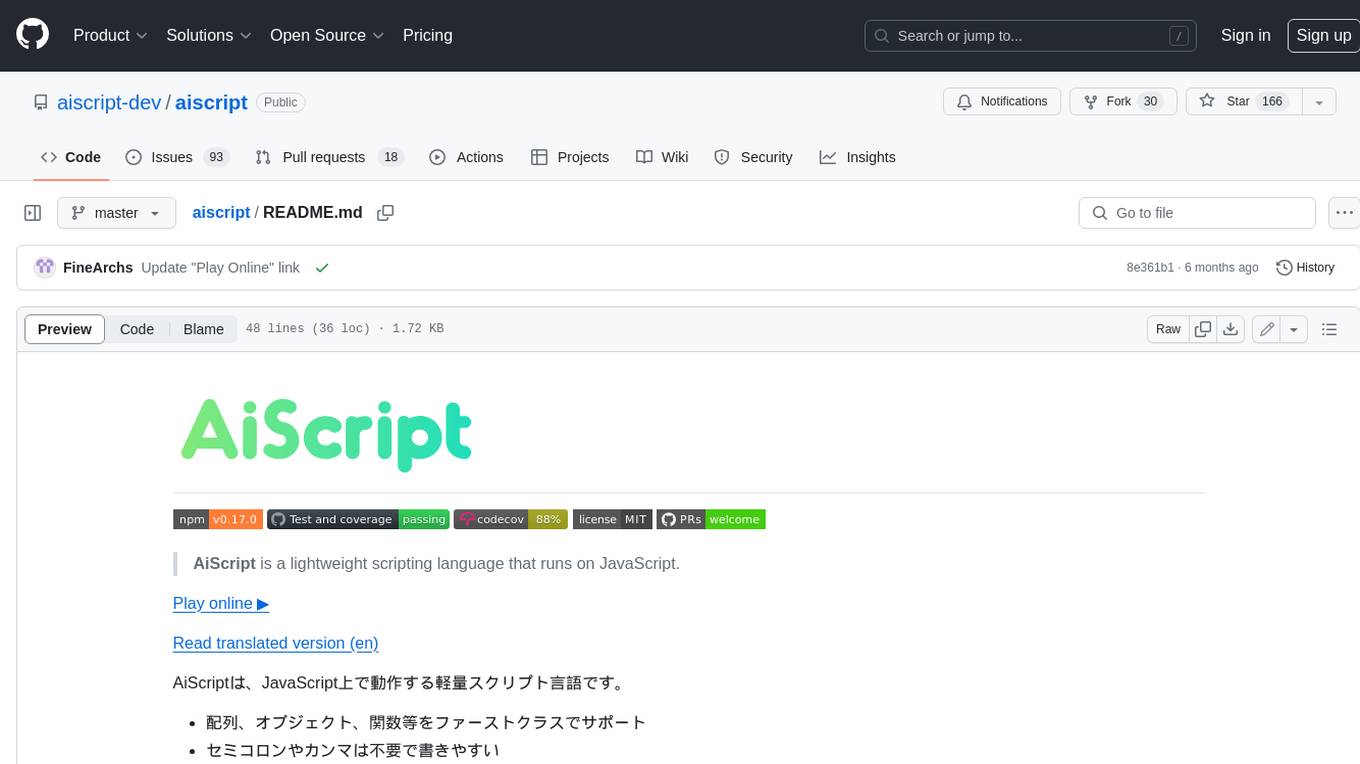
aiscript
AiScript is a lightweight scripting language that runs on JavaScript. It supports arrays, objects, and functions as first-class citizens, and is easy to write without the need for semicolons or commas. AiScript runs in a secure sandbox environment, preventing infinite loops from freezing the host. It also allows for easy provision of variables and functions from the host.
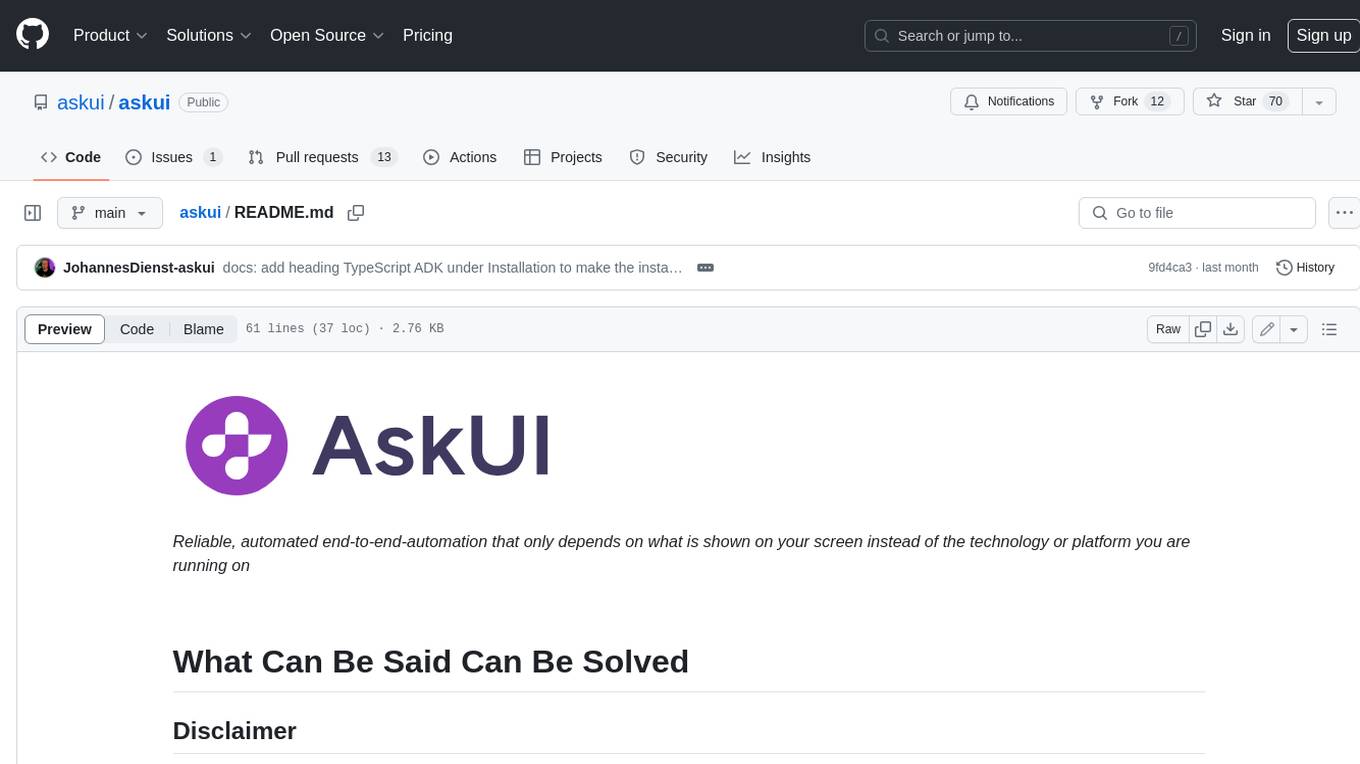
askui
AskUI is a reliable, automated end-to-end automation tool that only depends on what is shown on your screen instead of the technology or platform you are running on.
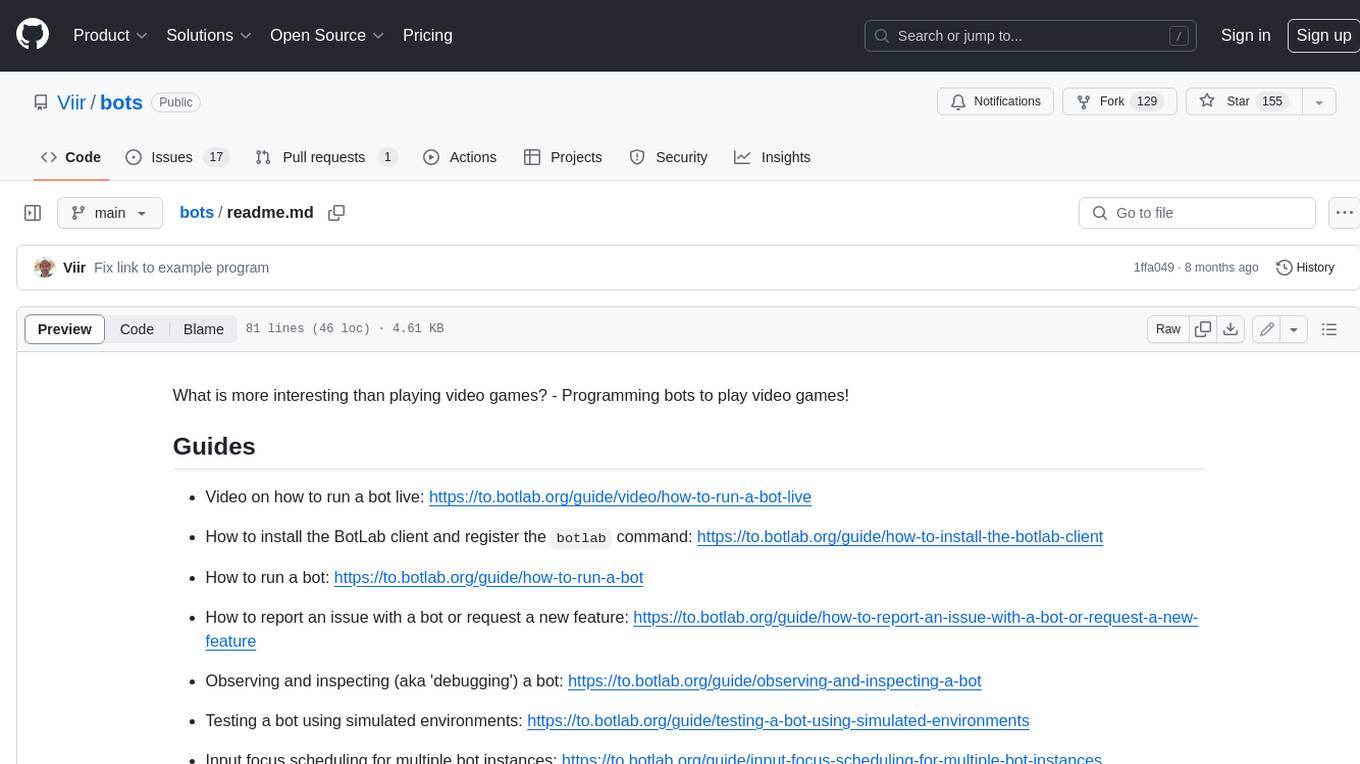
bots
The 'bots' repository is a collection of guides, tools, and example bots for programming bots to play video games. It provides resources on running bots live, installing the BotLab client, debugging bots, testing bots in simulated environments, and more. The repository also includes example bots for games like EVE Online, Tribal Wars 2, and Elvenar. Users can learn about developing bots for specific games, syntax of the Elm programming language, and tools for memory reading development. Additionally, there are guides on bot programming, contributing to BotLab, and exploring Elm syntax and core library.
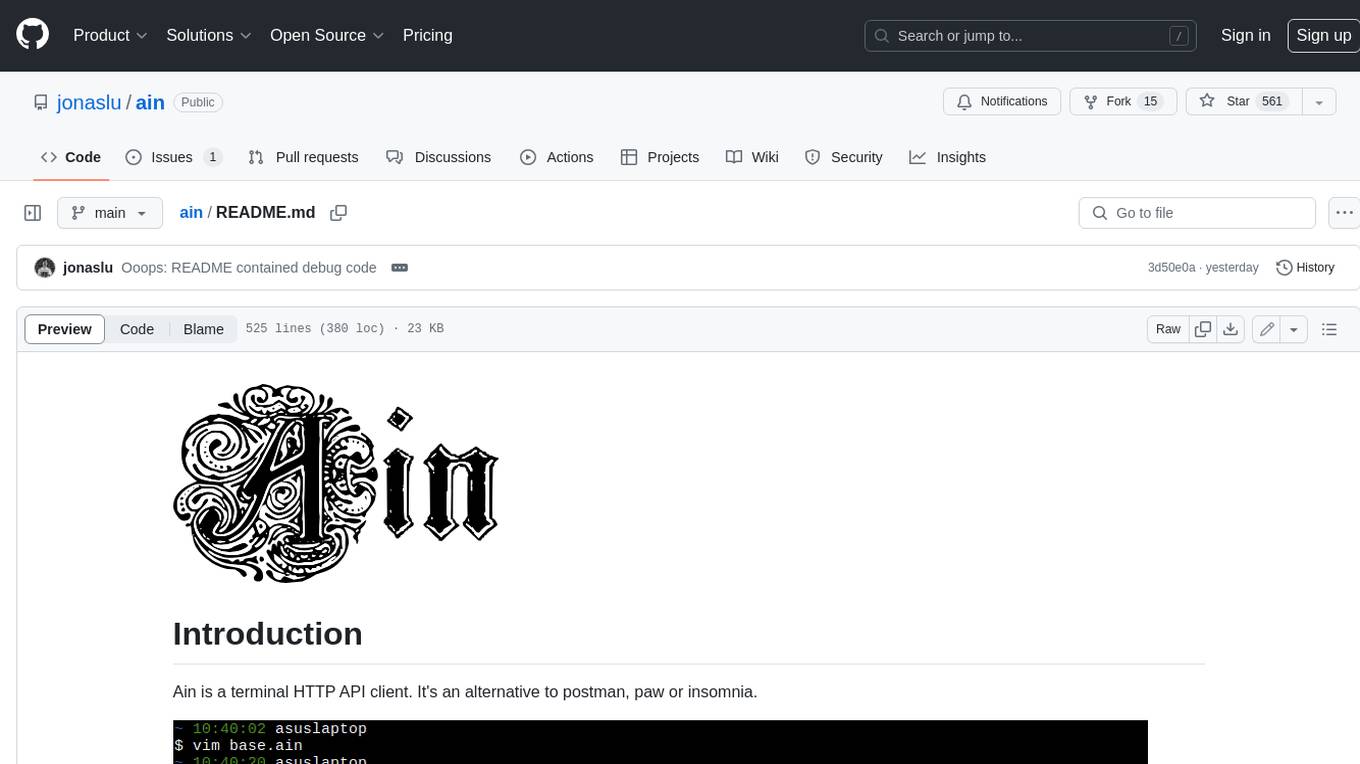
ain
Ain is a terminal HTTP API client designed for scripting input and processing output via pipes. It allows flexible organization of APIs using files and folders, supports shell-scripts and executables for common tasks, handles url-encoding, and enables sharing the resulting curl, wget, or httpie command-line. Users can put things that change in environment variables or .env-files, and pipe the API output for further processing. Ain targets users who work with many APIs using a simple file format and uses curl, wget, or httpie to make the actual calls.
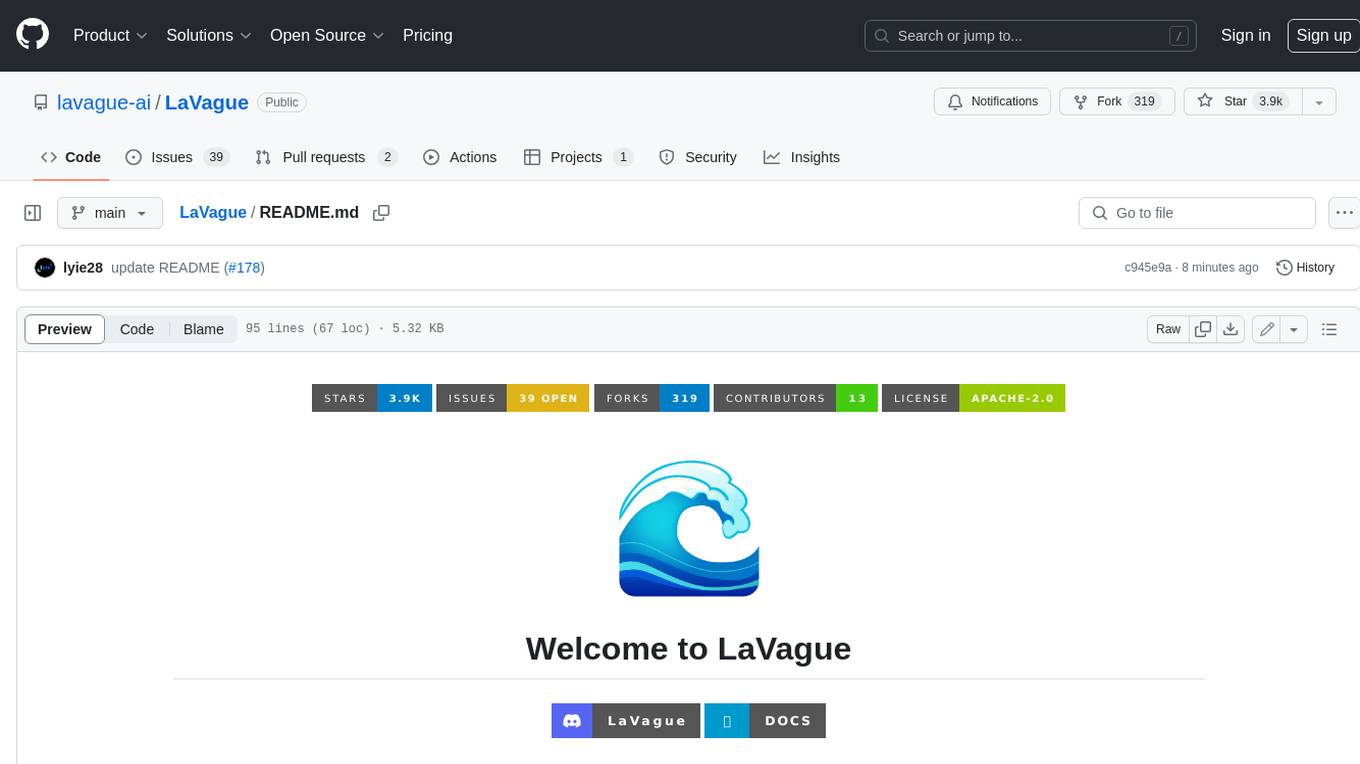
LaVague
LaVague is an open-source Large Action Model framework that uses advanced AI techniques to compile natural language instructions into browser automation code. It leverages Selenium or Playwright for browser actions. Users can interact with LaVague through an interactive Gradio interface to automate web interactions. The tool requires an OpenAI API key for default examples and offers a Playwright integration guide. Contributors can help by working on outlined tasks, submitting PRs, and engaging with the community on Discord. The project roadmap is available to track progress, but users should exercise caution when executing LLM-generated code using 'exec'.
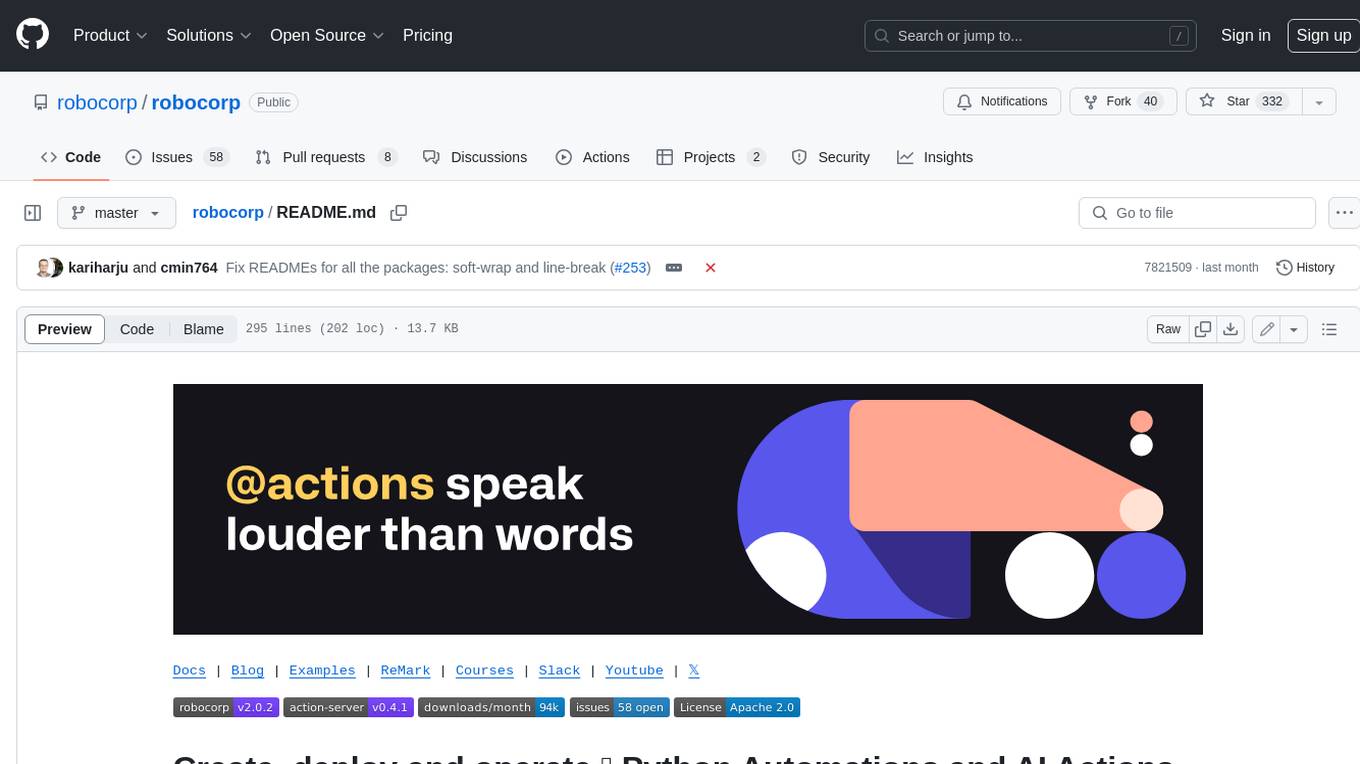
robocorp
Robocorp is a platform that allows users to create, deploy, and operate Python automations and AI actions. It provides an easy way to extend the capabilities of AI agents, assistants, and copilots with custom actions written in Python. Users can create and deploy tools, skills, loaders, and plugins that securely connect any AI Assistant platform to their data and applications. The Robocorp Action Server makes Python scripts compatible with ChatGPT and LangChain by automatically creating and exposing an API based on function declaration, type hints, and docstrings. It simplifies the process of developing and deploying AI actions, enabling users to interact with AI frameworks effortlessly.