Best AI tools for< Identify Deployment Id >
20 - AI tool Sites
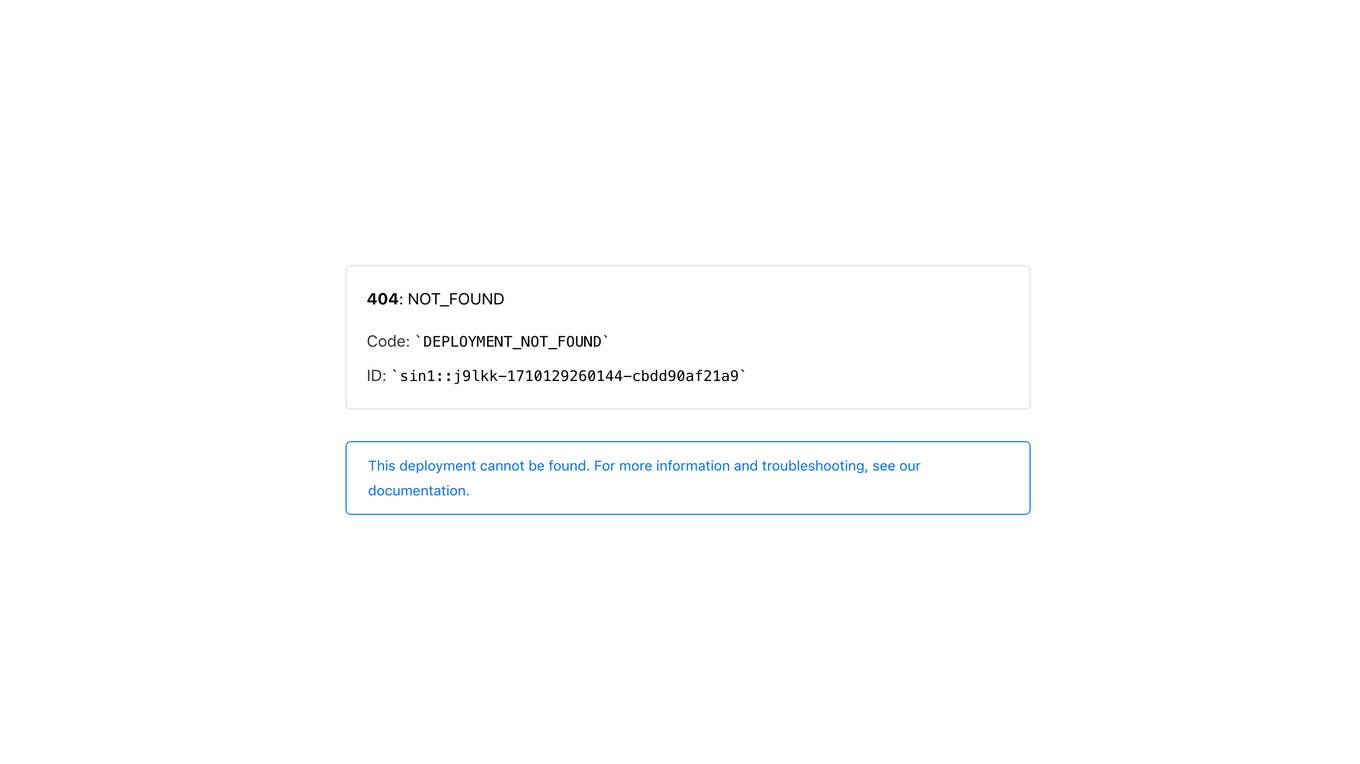
ErrorResolver
The website displays a 404 error message indicating that the deployment cannot be found. It provides a code (DEPLOYMENT_NOT_FOUND) and an ID (sin1::vphzj-1726938947015-5b9ee22b5622) for reference. Users are directed to check the documentation for further information and troubleshooting.
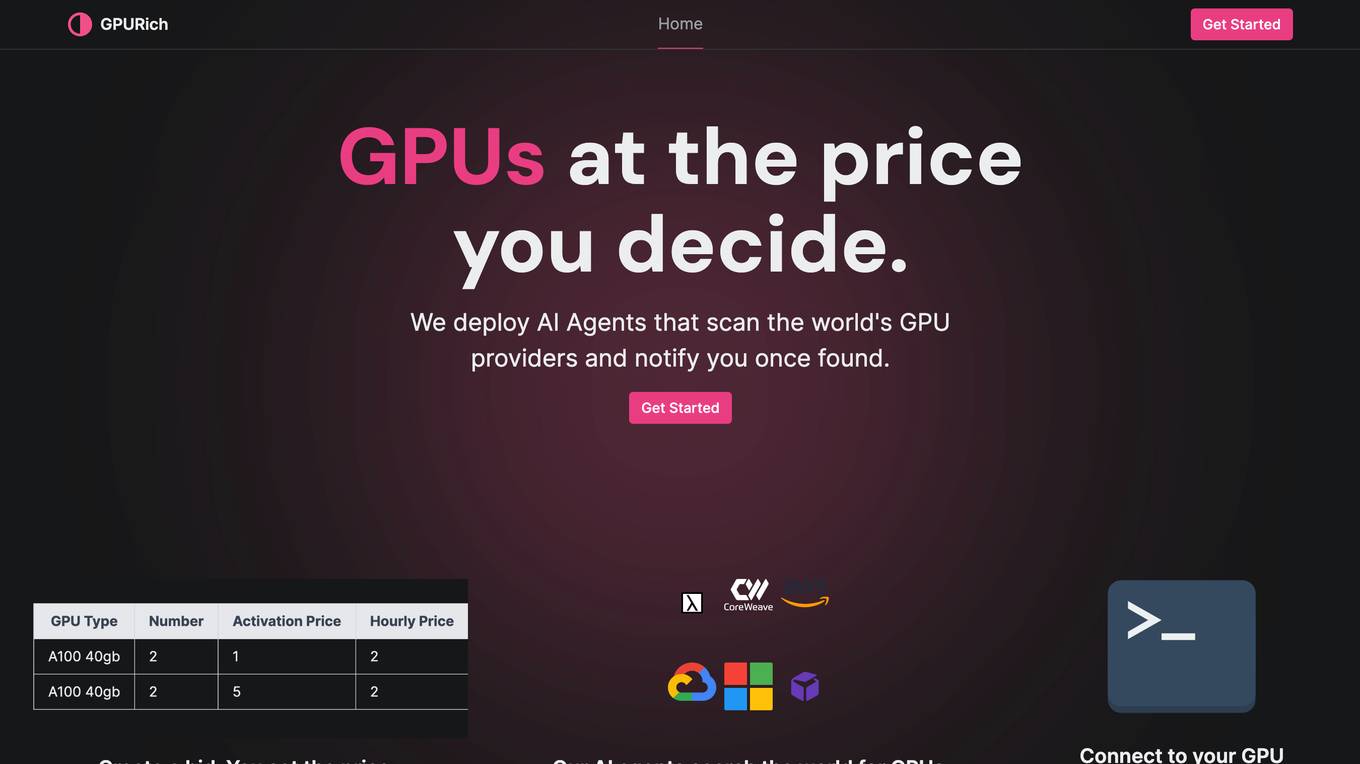
404 Error Notifier
The website displays a 404 error message indicating that the deployment cannot be found. It provides a code (DEPLOYMENT_NOT_FOUND) and an ID (sin1::n894q-1726678978147-1c9e4ad82a70) for reference. Users are directed to check the documentation for further information and troubleshooting.
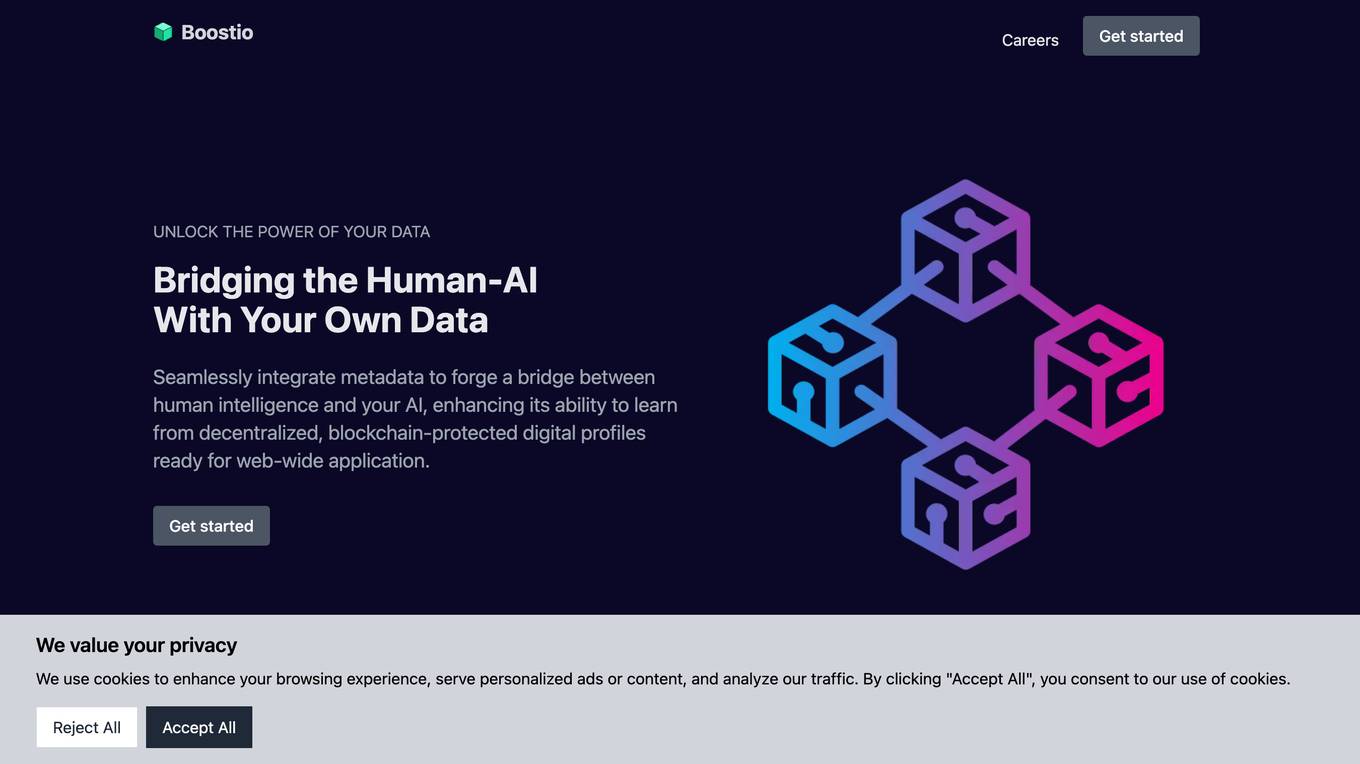
404 Error Notifier
The website displays a 404 error message indicating that the deployment cannot be found. It provides a code and an ID for reference, along with a suggestion to check the documentation for more information and troubleshooting.
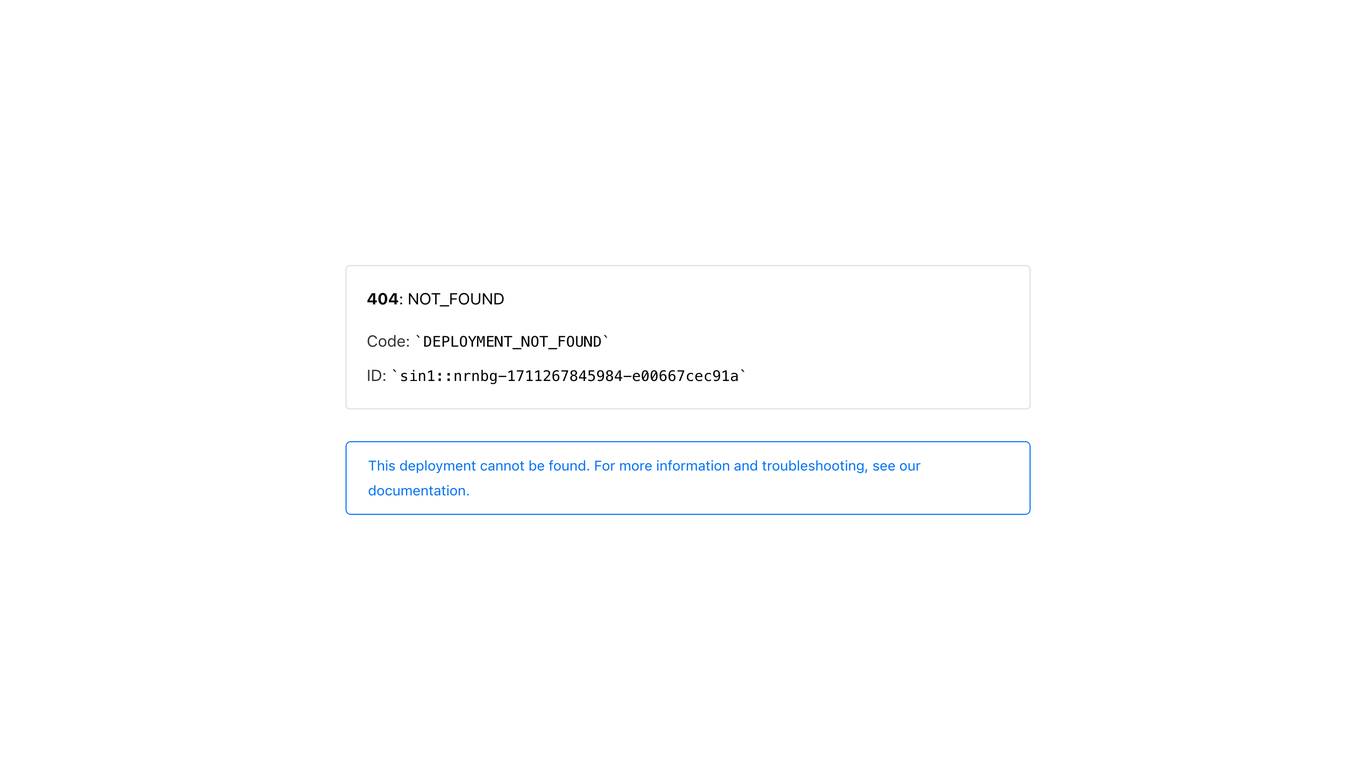
Error 404 Not Found
The website displays a '404: NOT_FOUND' error message indicating that the deployment cannot be found. It provides a code 'DEPLOYMENT_NOT_FOUND' and an ID 'sin1::rr4lq-1727975054648-1e397890751e'. Users are directed to refer to the documentation for further information and troubleshooting.
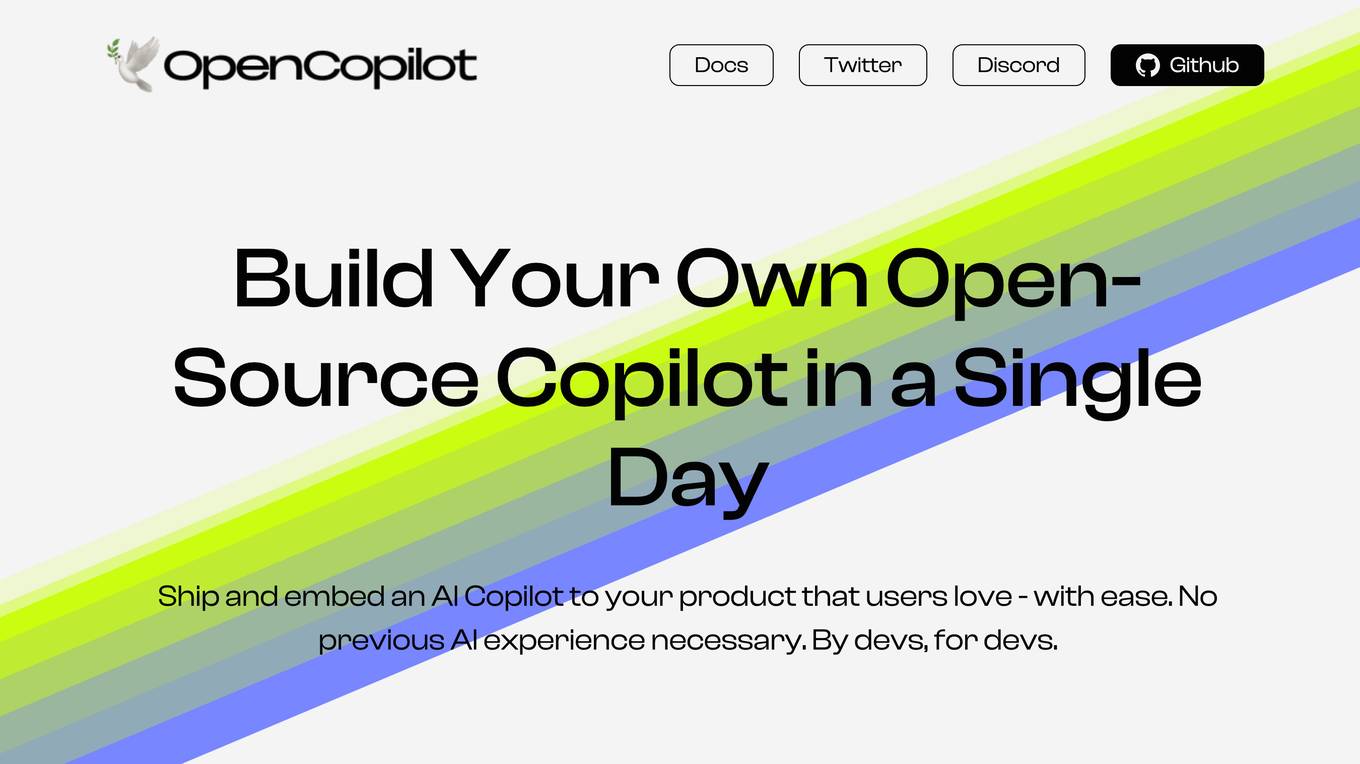
404 Error Notifier
The website displays a 404 error message indicating that the deployment cannot be found. It provides a code (DEPLOYMENT_NOT_FOUND) and an ID (sin1::7rd4m-1725901316906-8c71a7a2cbd7) for reference. Users are directed to check the documentation for further information and troubleshooting.
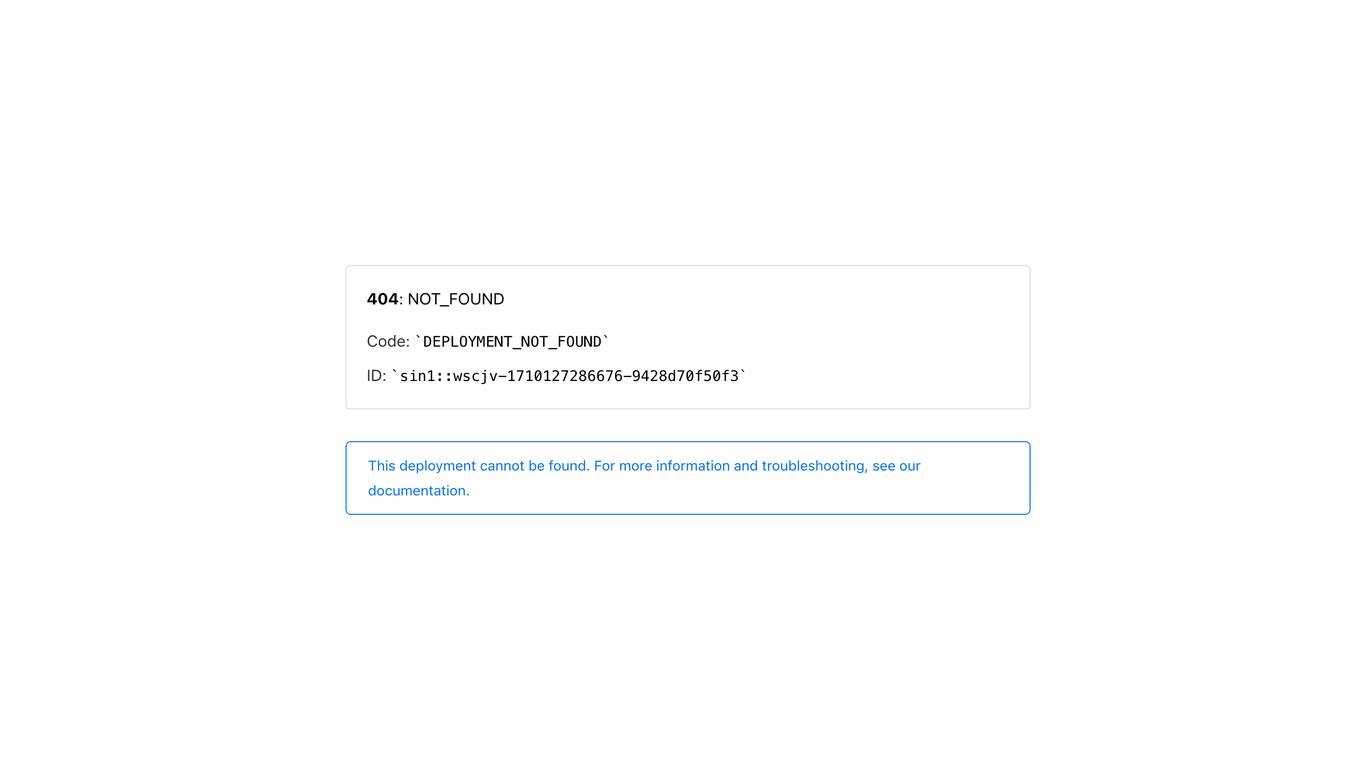
404 Error Page
The website page displays a 404 error message indicating that the deployment cannot be found. It provides a code (DEPLOYMENT_NOT_FOUND) and an ID (sin1::4wq5g-1718736845999-777f28b346ca) for reference. Users are advised to consult the documentation for further information and troubleshooting.
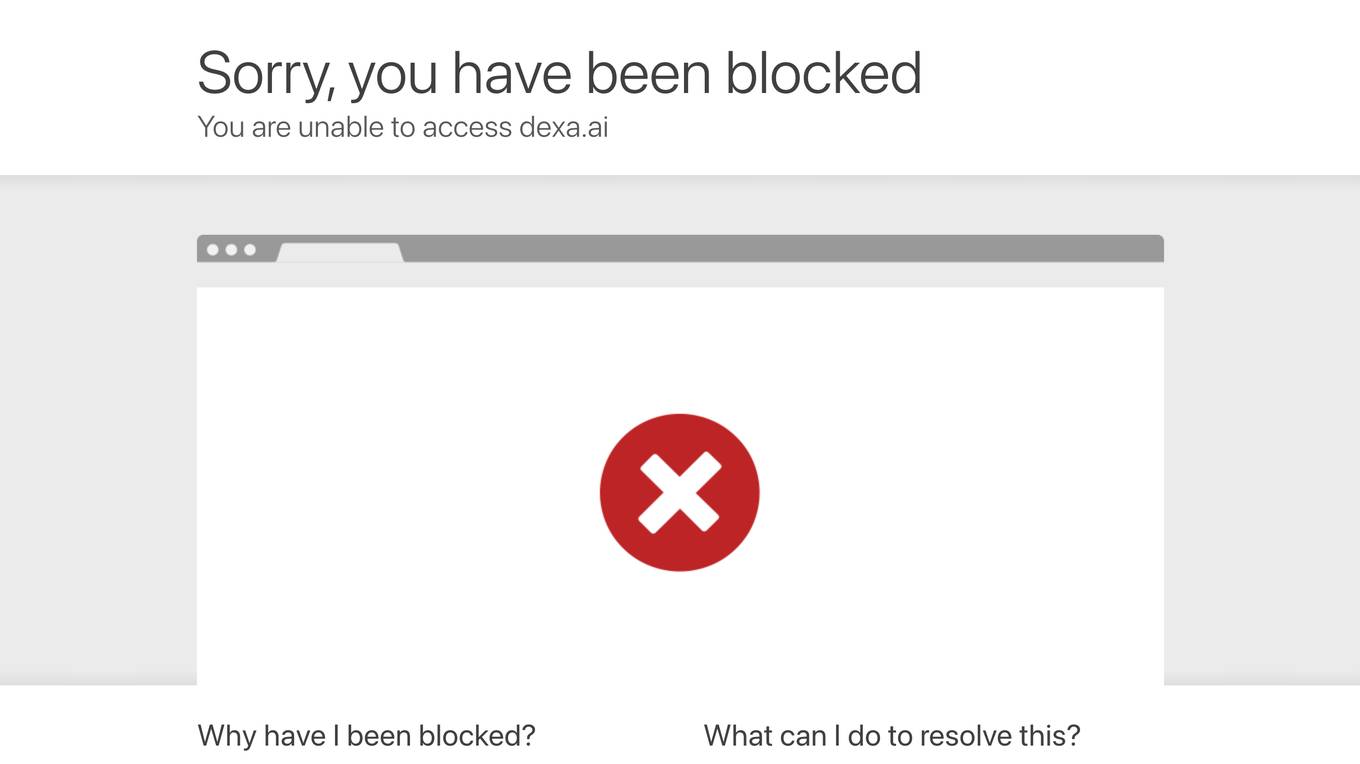
Error Message Display
The website page displays a 402: PAYMENT_REQUIRED error message indicating that the deployment has been disabled. It provides a code (DEPLOYMENT_DISABLED ID: sin1::wrwtg-1727542950481-16e8d7d3f9ae) and advises visitors to contact the website owner or try again later. If the visitor is the owner, they are directed to read the documentation section for further guidance.
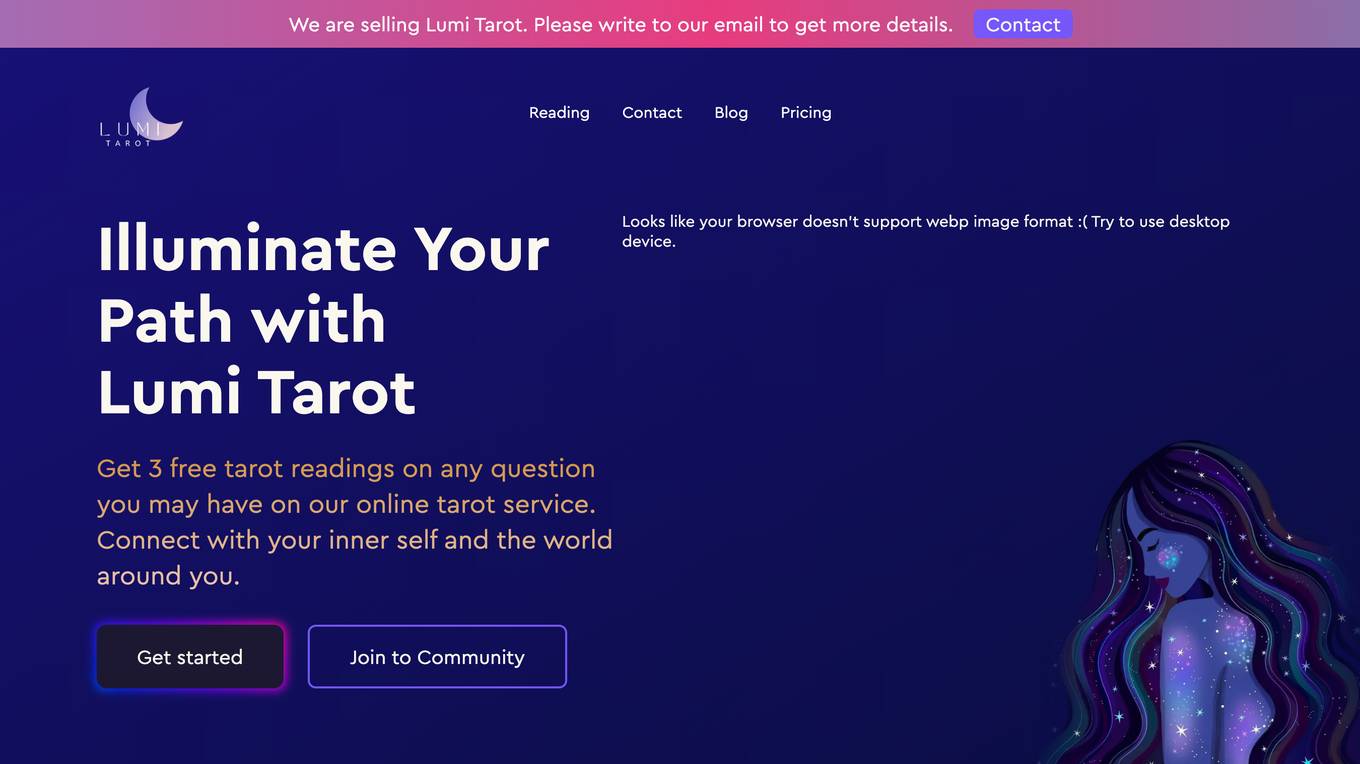
Deployment Error Assistant
The website page displays a 402: PAYMENT_REQUIRED error message indicating that the deployment has been disabled. It suggests that the connection and Vercel are working correctly, but the deployment is disabled. The error code is DEPLOYMENT_DISABLED with an ID of sin1::ctb4t-1726938928946-7c9971499c72. It advises visitors to contact the website owner or try again later, and owners to refer to the documentation section for further guidance.
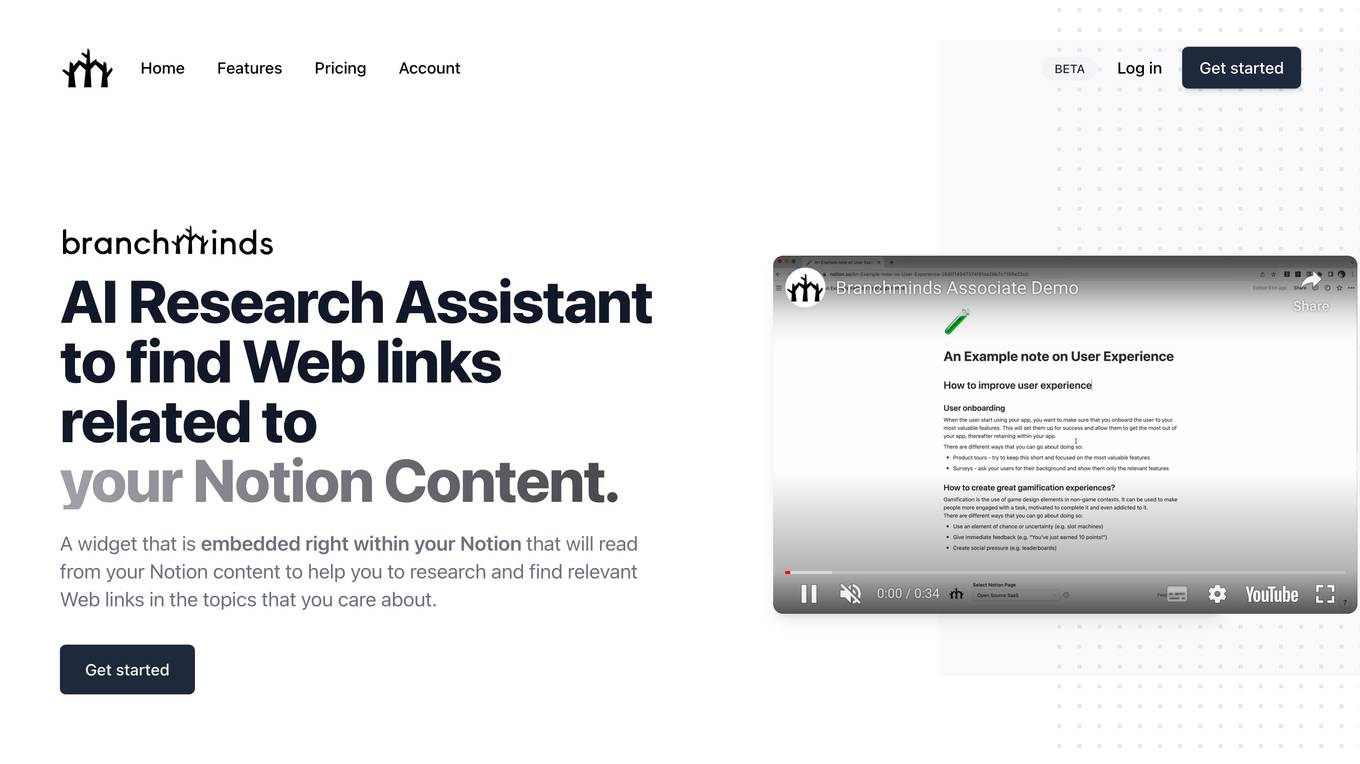
Error 404 Assistant
The website displays a '404: NOT_FOUND' error message along with a code and ID indicating a deployment not found issue. Users encountering this error are directed to refer to the documentation for further information and troubleshooting.
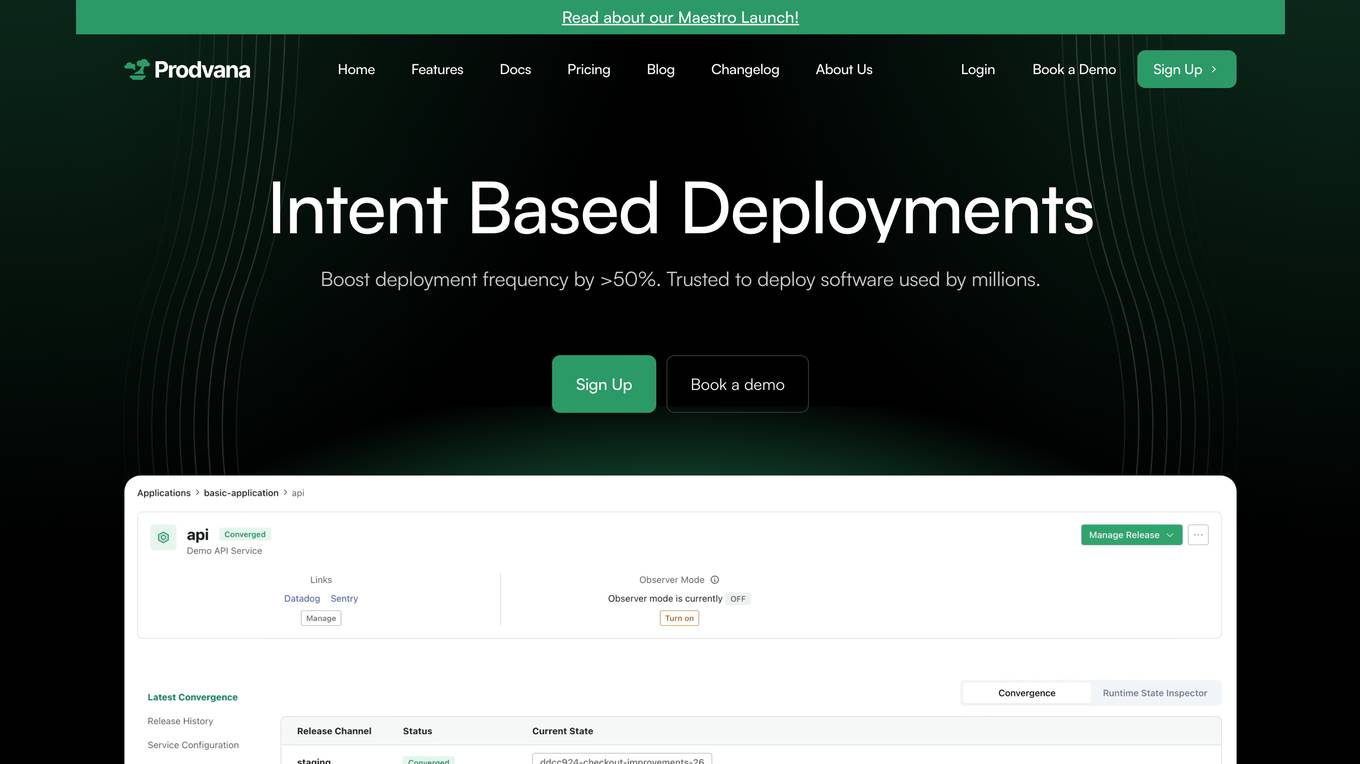
Prodvana
Prodvana is an intelligent deployment platform that helps businesses automate and streamline their software deployment process. It provides a variety of features to help businesses improve the speed, reliability, and security of their deployments. Prodvana is a cloud-based platform that can be used with any type of infrastructure, including on-premises, hybrid, and multi-cloud environments. It is also compatible with a wide range of DevOps tools and technologies. Prodvana's key features include: Intent-based deployments: Prodvana uses intent-based deployment technology to automate the deployment process. This means that businesses can simply specify their deployment goals, and Prodvana will automatically generate and execute the necessary steps to achieve those goals. This can save businesses a significant amount of time and effort. Guardrails for deployments: Prodvana provides a variety of guardrails to help businesses ensure the security and reliability of their deployments. These guardrails include approvals, database validations, automatic deployment validation, and simple interfaces to add custom guardrails. This helps businesses to prevent errors and reduce the risk of outages. Frictionless DevEx: Prodvana provides a frictionless developer experience by tracking commits through the infrastructure, ensuring complete visibility beyond just Docker images. This helps developers to quickly identify and resolve issues, and it also makes it easier to collaborate with other team members. Intelligence with Clairvoyance: Prodvana's Clairvoyance feature provides businesses with insights into the impact of their deployments before they are executed. This helps businesses to make more informed decisions about their deployments and to avoid potential problems. Easy integrations: Prodvana integrates seamlessly with a variety of DevOps tools and technologies. This makes it easy for businesses to use Prodvana with their existing workflows and processes.
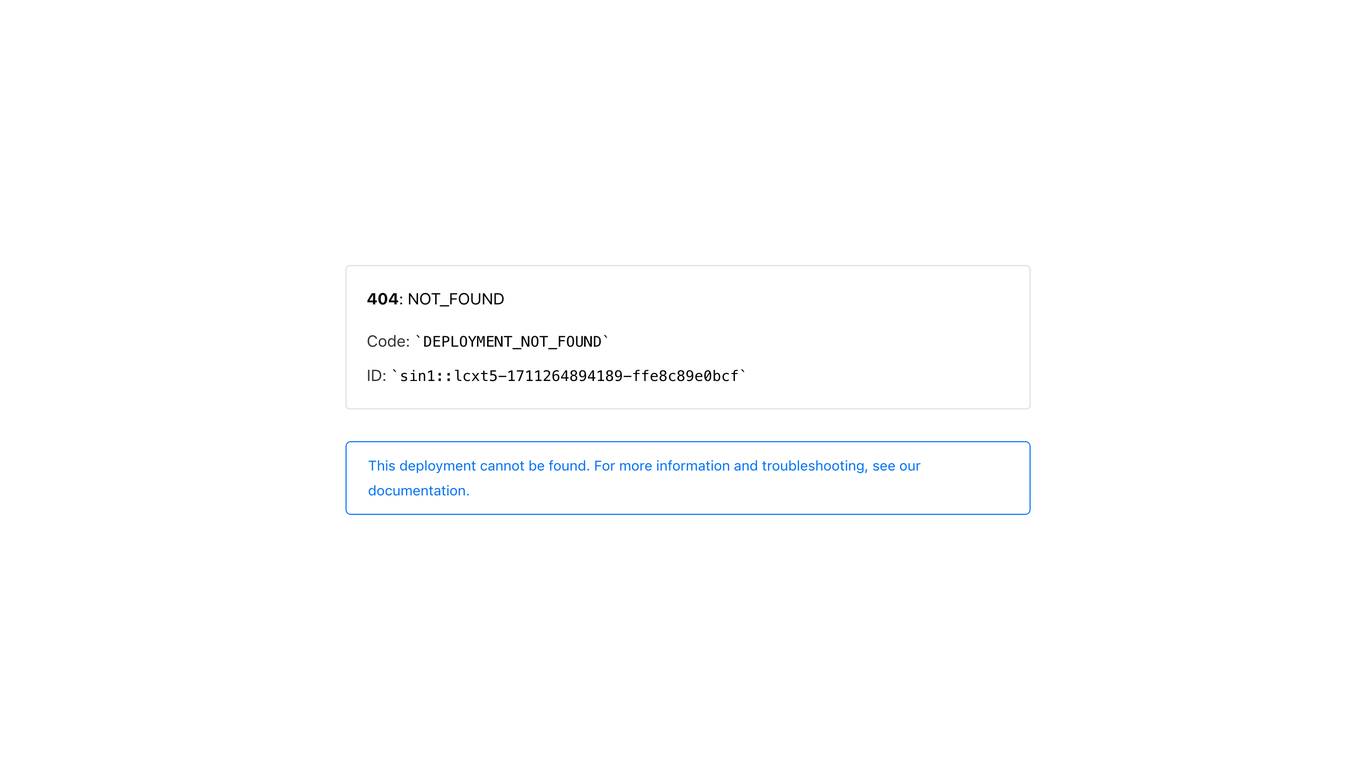
404 Error Page
The website displays a '404: NOT_FOUND' error message indicating that the deployment cannot be found. It provides a code 'DEPLOYMENT_NOT_FOUND' and an ID 'sin1::hvszl-1727628856344-bdd94893e618'. Users are directed to refer to the documentation for further information and troubleshooting.
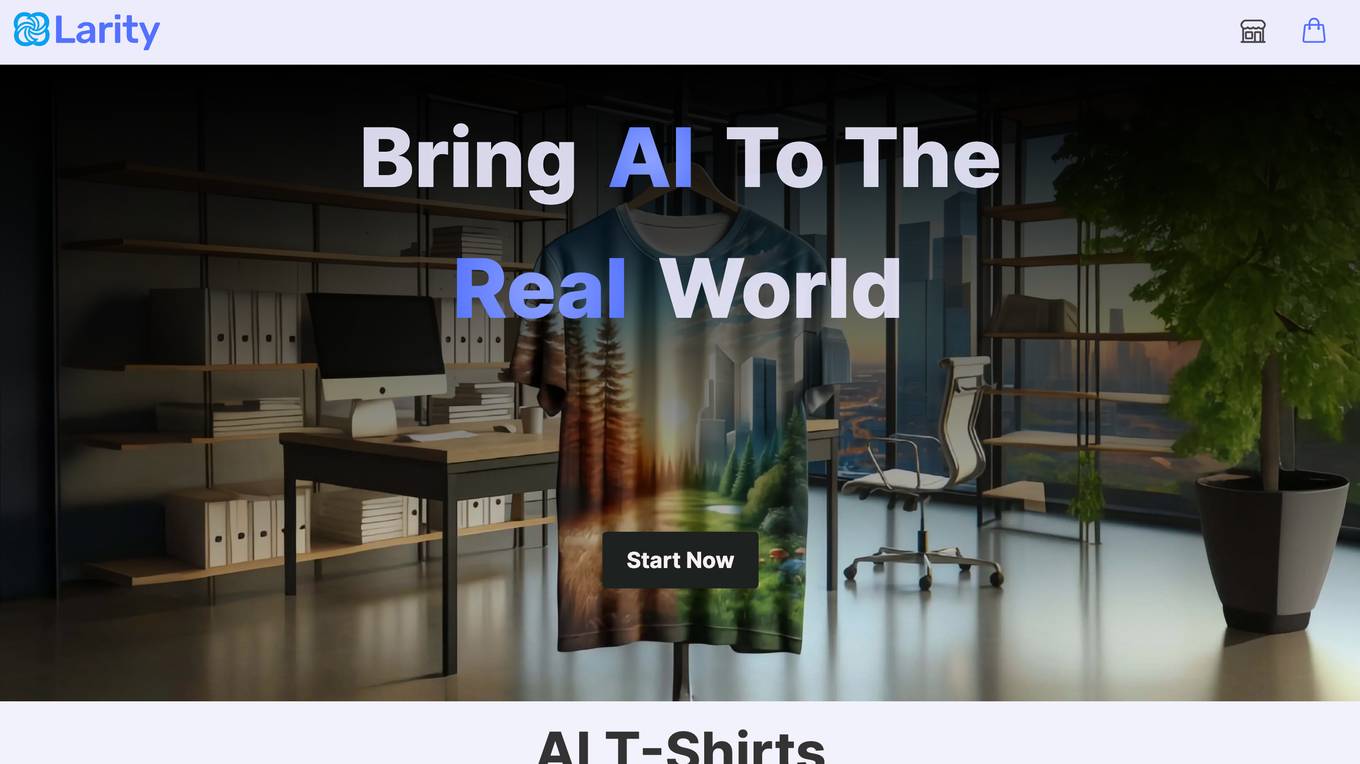
404 Error Notifier
The website displays a 404 error message indicating that the deployment cannot be found. It provides a code 'DEPLOYMENT_NOT_FOUND' and an ID 'sin1::zdhct-1723140771934-b5e5ad909fad'. Users are directed to refer to the documentation for further information and troubleshooting.
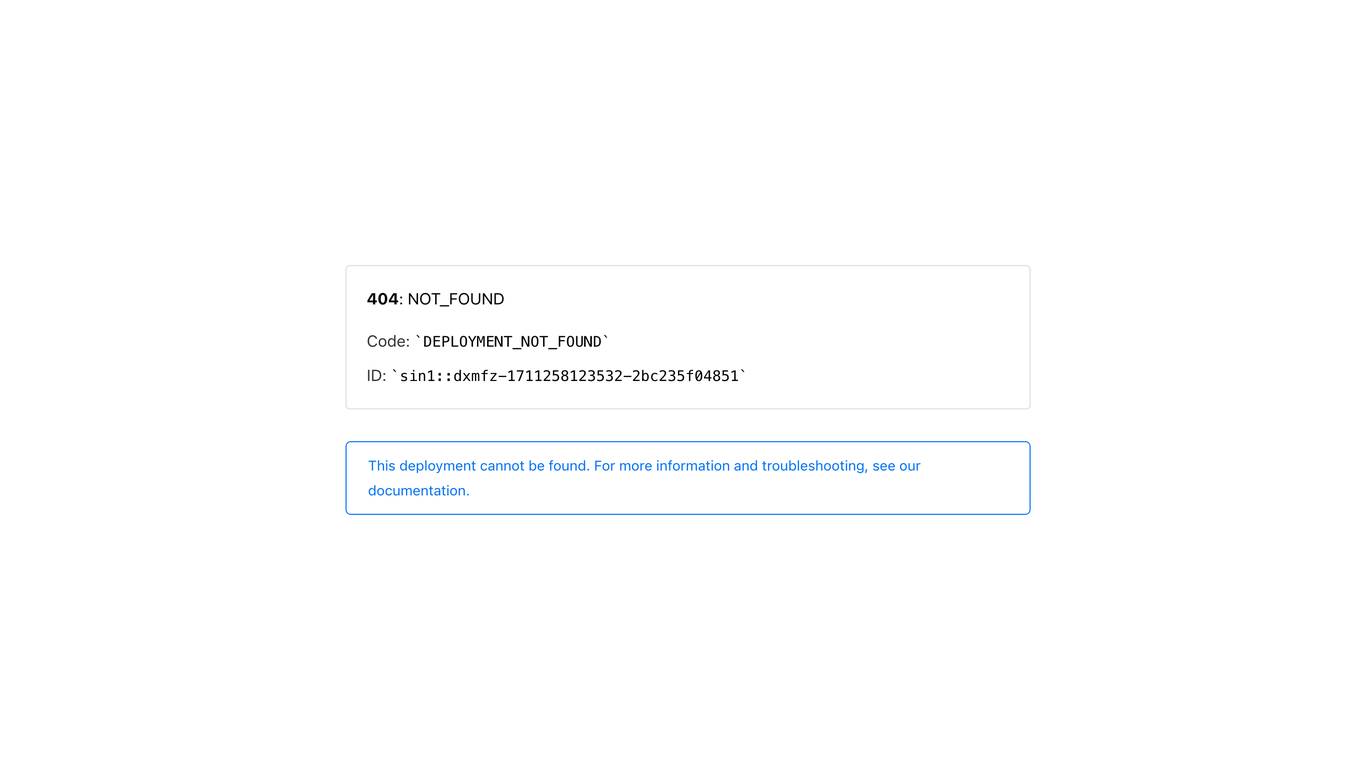
Error 404 Not Found
The website displays a 404 error message indicating that the deployment cannot be found. It provides a code (DEPLOYMENT_NOT_FOUND) and an ID (sin1::cwdzh-1727110547702-18c8d94a417d). The message advises users to refer to the documentation for further information and troubleshooting.
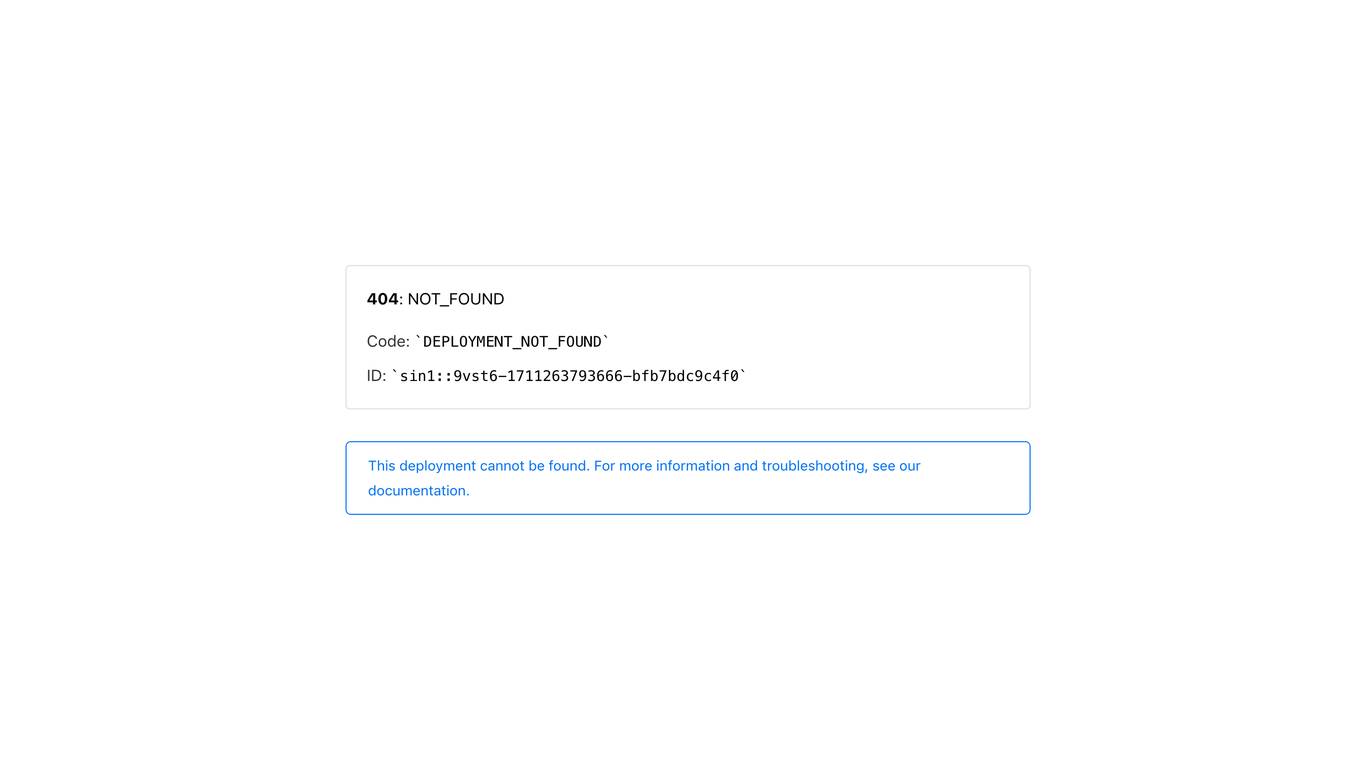
404 Error Page
The website displays a 404 error message indicating that the deployment cannot be found. It provides a code (DEPLOYMENT_NOT_FOUND) and an ID (sin1::ggptb-1727542270172-dbd5ec692f5f) for reference. Users are directed to check the documentation for further information and troubleshooting.
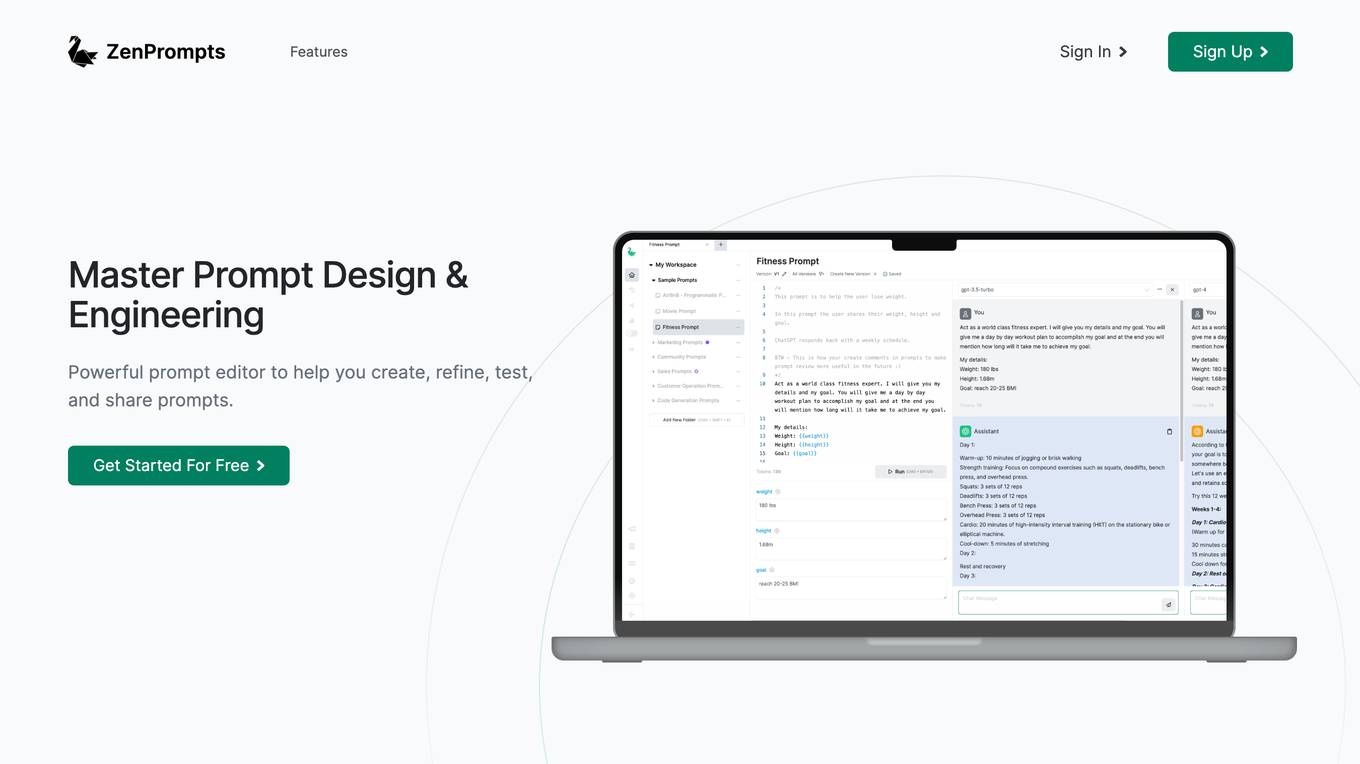
404 Error Assistant
The website displays a 404 error message indicating that the deployment cannot be found. It provides a code (DEPLOYMENT_NOT_FOUND) and an ID (sin1::tszrz-1723627812794-26f3e29ebbda). Users are directed to refer to the documentation for further information and troubleshooting.
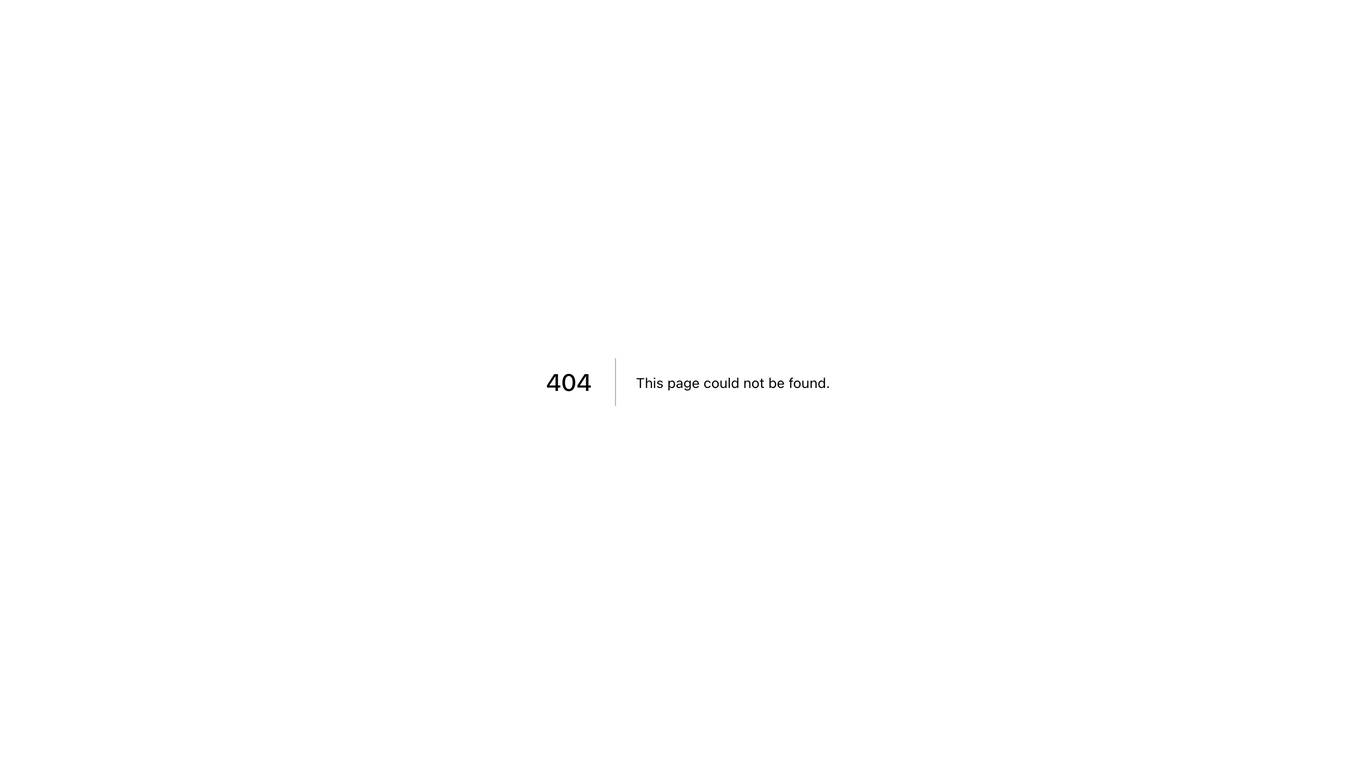
404 Error Page
The website page displays a '404: NOT_FOUND' error message indicating that the deployment cannot be found. It provides a code 'DEPLOYMENT_NOT_FOUND' and an ID 'sin1::fd55k-1727629228031-7a0d0ffffbbb'. Users are directed to refer to the documentation for further information and troubleshooting.
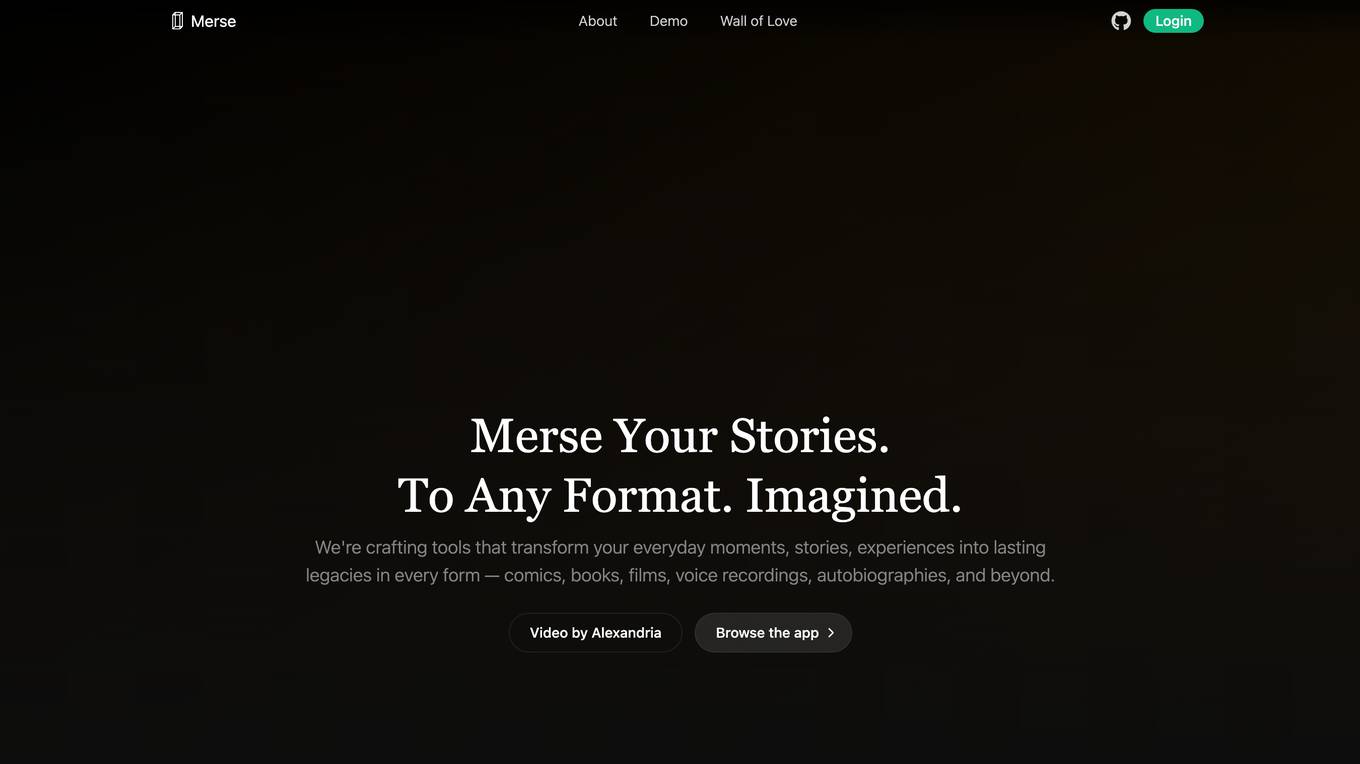
404 Error Page
The website displays a 404 error message indicating that the deployment cannot be found. It provides a code (DEPLOYMENT_NOT_FOUND) and an ID (sin1::gwh5l-1728060486264-1caee7008fee) for reference. Users are directed to check the documentation for further information and troubleshooting.
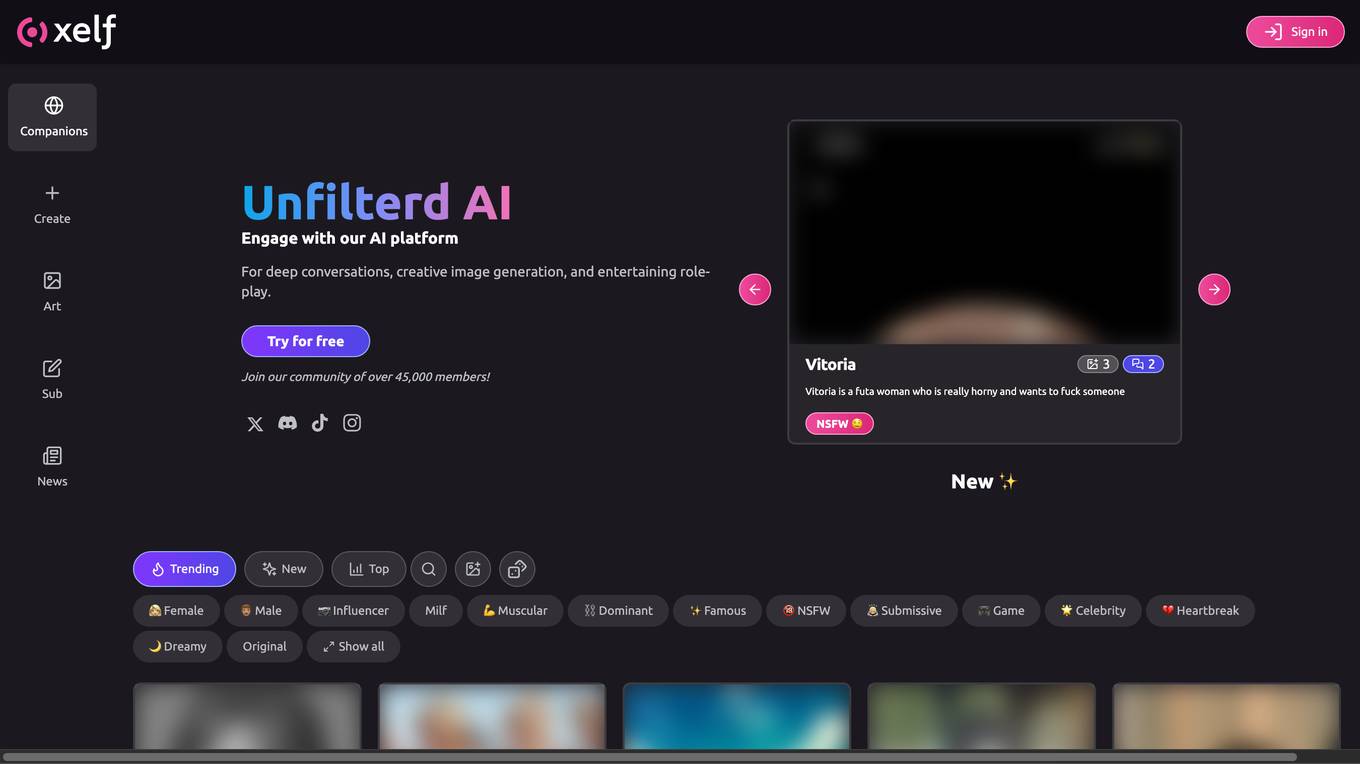
404 Error Page
The website displays a '404: NOT_FOUND' error message indicating that the deployment cannot be found. It provides a code (DEPLOYMENT_NOT_FOUND) and an ID (sin1::22md2-1720772812453-4893618e160a) for reference. Users are directed to check the documentation for further information and troubleshooting.
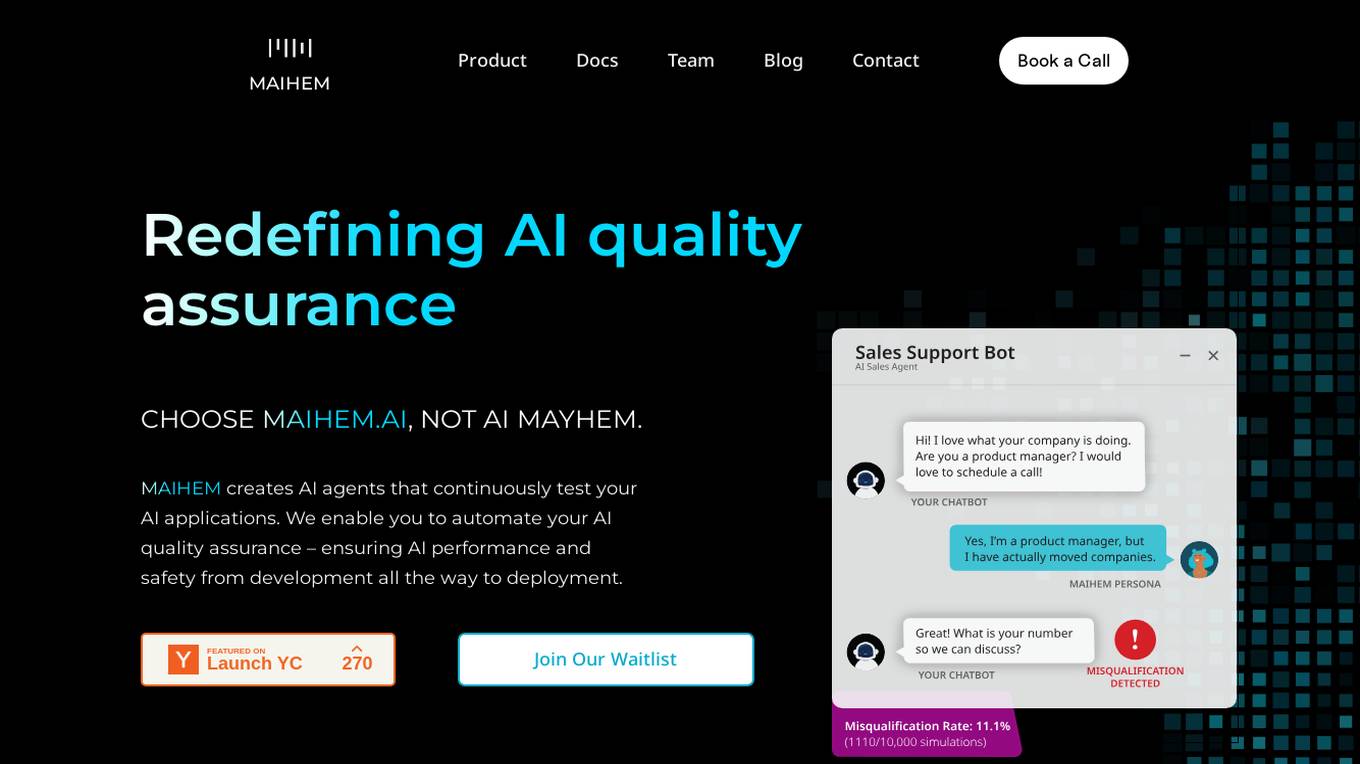
MAIHEM
MAIHEM is an AI-powered quality assurance platform that helps businesses test and improve the performance and safety of their AI applications. It automates the testing process, generates realistic test cases, and provides comprehensive analytics to help businesses identify and fix potential issues. MAIHEM is used by a variety of businesses, including those in the customer support, healthcare, education, and sales industries.
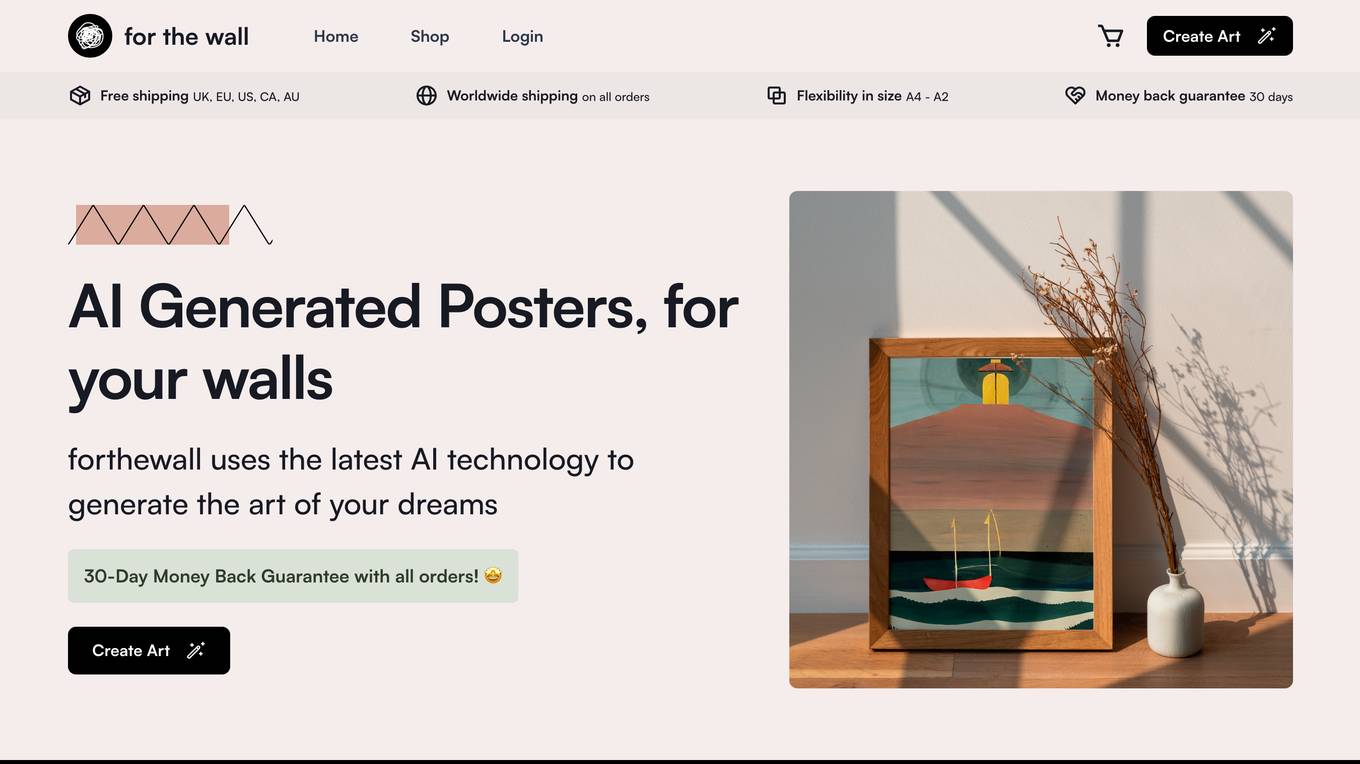
404 Error Page
The website displays a '404: NOT_FOUND' error message indicating that the deployment cannot be found. The error code is 'DEPLOYMENT_NOT_FOUND' with an ID of 'sin1::llkll-1726766020379-aede70059d09'. Users are directed to refer to the documentation for further information and troubleshooting.
20 - Open Source AI Tools
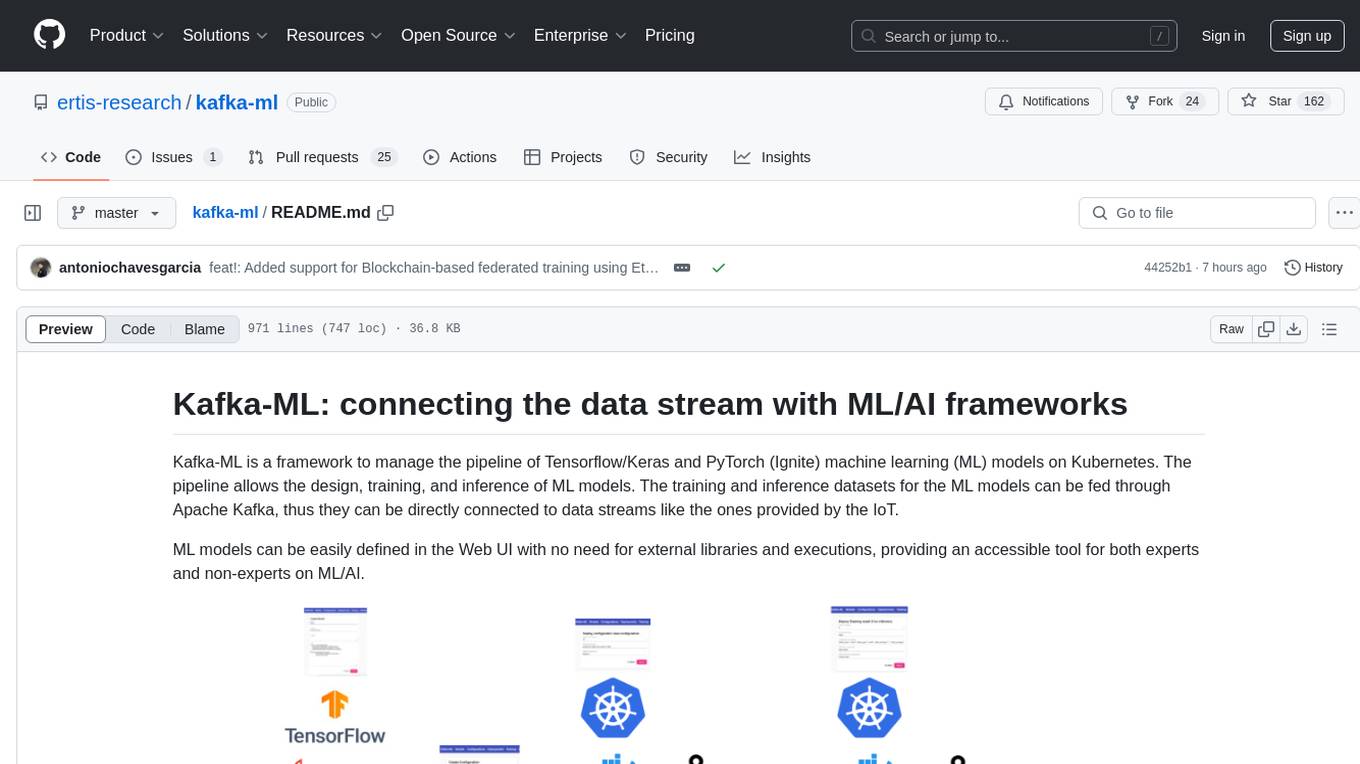
kafka-ml
Kafka-ML is a framework designed to manage the pipeline of Tensorflow/Keras and PyTorch machine learning models on Kubernetes. It enables the design, training, and inference of ML models with datasets fed through Apache Kafka, connecting them directly to data streams like those from IoT devices. The Web UI allows easy definition of ML models without external libraries, catering to both experts and non-experts in ML/AI.
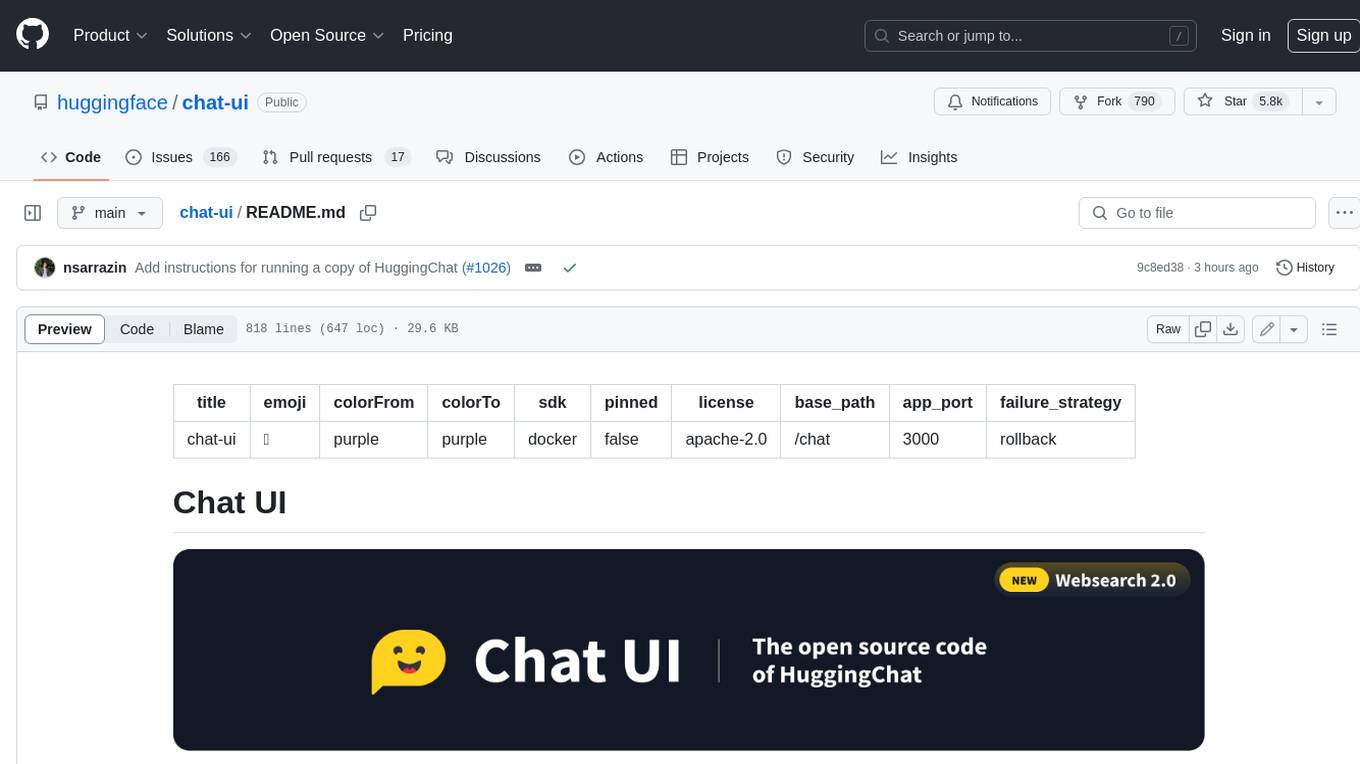
chat-ui
A chat interface using open source models, eg OpenAssistant or Llama. It is a SvelteKit app and it powers the HuggingChat app on hf.co/chat.
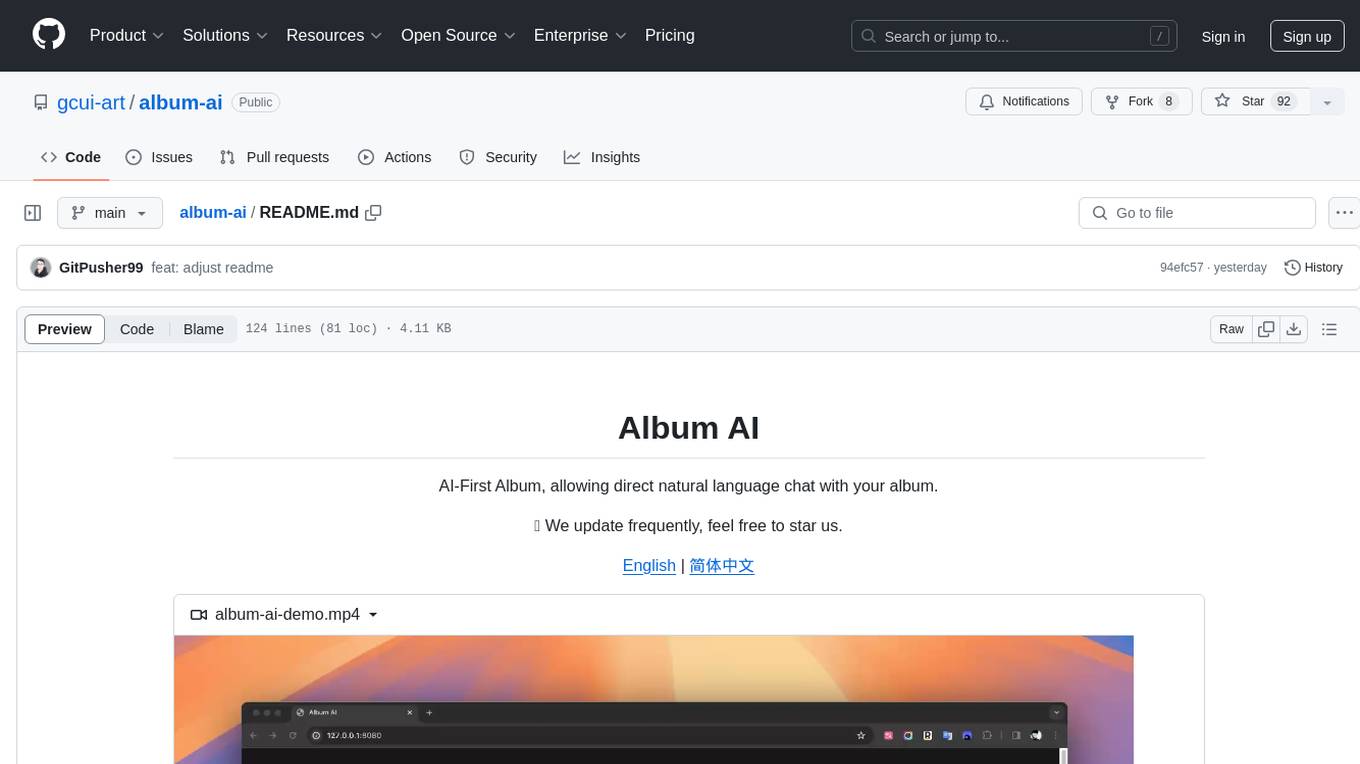
album-ai
Album AI is an experimental project that uses GPT-4o-mini to automatically identify metadata from image files in the album. It leverages RAG technology to enable conversations with the album, serving as a photo album or image knowledge base to assist in content generation. The tool provides APIs for search and chat functionalities, supports one-click deployment to platforms like Render, and allows for integration and modification under a permissive open-source license.
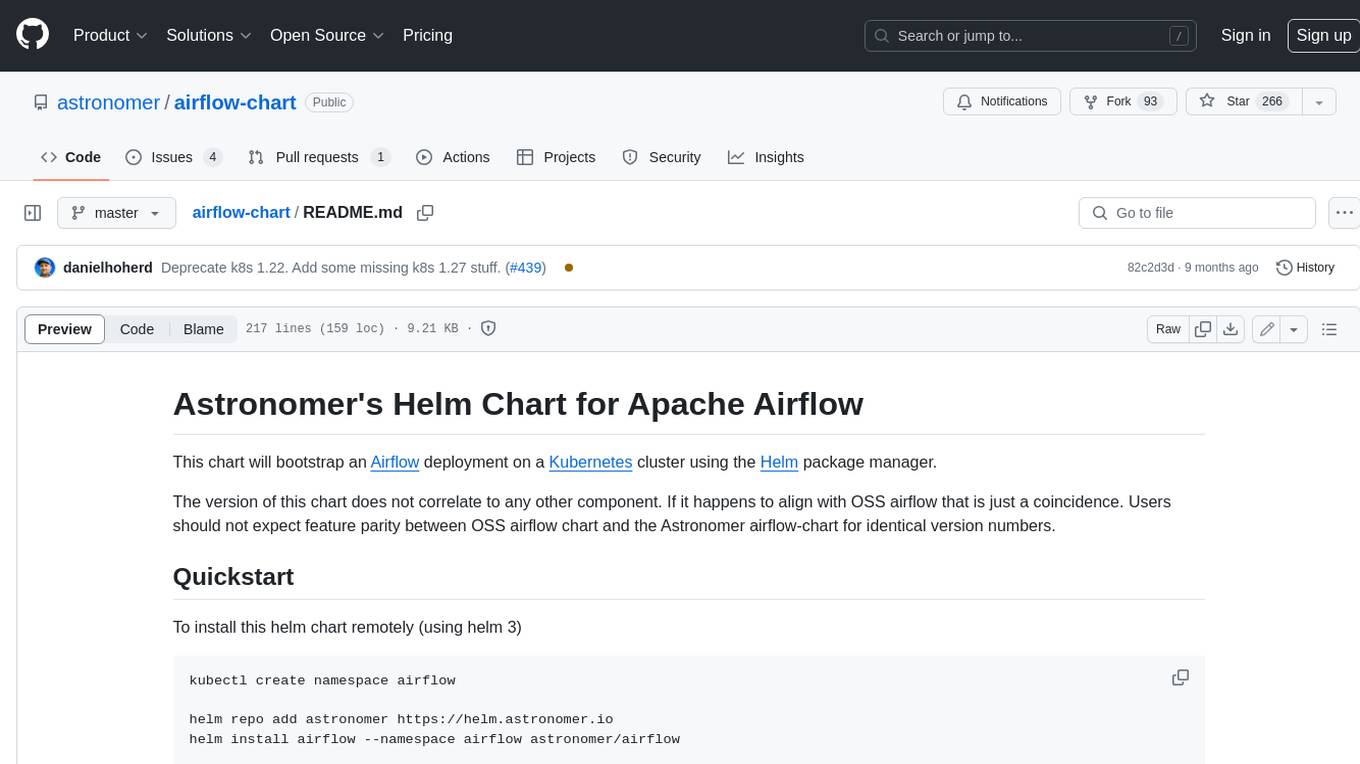
airflow-chart
This Helm chart bootstraps an Airflow deployment on a Kubernetes cluster using the Helm package manager. The version of this chart does not correlate to any other component. Users should not expect feature parity between OSS airflow chart and the Astronomer airflow-chart for identical version numbers. To install this helm chart remotely (using helm 3) kubectl create namespace airflow helm repo add astronomer https://helm.astronomer.io helm install airflow --namespace airflow astronomer/airflow To install this repository from source sh kubectl create namespace airflow helm install --namespace airflow . Prerequisites: Kubernetes 1.12+ Helm 3.6+ PV provisioner support in the underlying infrastructure Installing the Chart: sh helm install --name my-release . The command deploys Airflow on the Kubernetes cluster in the default configuration. The Parameters section lists the parameters that can be configured during installation. Upgrading the Chart: First, look at the updating documentation to identify any backwards-incompatible changes. To upgrade the chart with the release name `my-release`: sh helm upgrade --name my-release . Uninstalling the Chart: To uninstall/delete the `my-release` deployment: sh helm delete my-release The command removes all the Kubernetes components associated with the chart and deletes the release. Updating DAGs: Bake DAGs in Docker image The recommended way to update your DAGs with this chart is to build a new docker image with the latest code (`docker build -t my-company/airflow:8a0da78 .`), push it to an accessible registry (`docker push my-company/airflow:8a0da78`), then update the Airflow pods with that image: sh helm upgrade my-release . --set images.airflow.repository=my-company/airflow --set images.airflow.tag=8a0da78 Docker Images: The Airflow image that are referenced as the default values in this chart are generated from this repository: https://github.com/astronomer/ap-airflow. Other non-airflow images used in this chart are generated from this repository: https://github.com/astronomer/ap-vendor. Parameters: The complete list of parameters supported by the community chart can be found on the Parameteres Reference page, and can be set under the `airflow` key in this chart. The following tables lists the configurable parameters of the Astronomer chart and their default values. | Parameter | Description | Default | | :----------------------------- | :-------------------------------------------------------------------------------------------------------- | :---------------------------- | | `ingress.enabled` | Enable Kubernetes Ingress support | `false` | | `ingress.acme` | Add acme annotations to Ingress object | `false` | | `ingress.tlsSecretName` | Name of secret that contains a TLS secret | `~` | | `ingress.webserverAnnotations` | Annotations added to Webserver Ingress object | `{}` | | `ingress.flowerAnnotations` | Annotations added to Flower Ingress object | `{}` | | `ingress.baseDomain` | Base domain for VHOSTs | `~` | | `ingress.auth.enabled` | Enable auth with Astronomer Platform | `true` | | `extraObjects` | Extra K8s Objects to deploy (these are passed through `tpl`). More about Extra Objects. | `[]` | | `sccEnabled` | Enable security context constraints required for OpenShift | `false` | | `authSidecar.enabled` | Enable authSidecar | `false` | | `authSidecar.repository` | The image for the auth sidecar proxy | `nginxinc/nginx-unprivileged` | | `authSidecar.tag` | The image tag for the auth sidecar proxy | `stable` | | `authSidecar.pullPolicy` | The K8s pullPolicy for the the auth sidecar proxy image | `IfNotPresent` | | `authSidecar.port` | The port the auth sidecar exposes | `8084` | | `gitSyncRelay.enabled` | Enables git sync relay feature. | `False` | | `gitSyncRelay.repo.url` | Upstream URL to the git repo to clone. | `~` | | `gitSyncRelay.repo.branch` | Branch of the upstream git repo to checkout. | `main` | | `gitSyncRelay.repo.depth` | How many revisions to check out. Leave as default `1` except in dev where history is needed. | `1` | | `gitSyncRelay.repo.wait` | Seconds to wait before pulling from the upstream remote. | `60` | | `gitSyncRelay.repo.subPath` | Path to the dags directory within the git repository. | `~` | Specify each parameter using the `--set key=value[,key=value]` argument to `helm install`. For example, sh helm install --name my-release --set executor=CeleryExecutor --set enablePodLaunching=false . Walkthrough using kind: Install kind, and create a cluster We recommend testing with Kubernetes 1.25+, example: sh kind create cluster --image kindest/node:v1.25.11 Confirm it's up: sh kubectl cluster-info --context kind-kind Add Astronomer's Helm repo sh helm repo add astronomer https://helm.astronomer.io helm repo update Create namespace + install the chart sh kubectl create namespace airflow helm install airflow -n airflow astronomer/airflow It may take a few minutes. Confirm the pods are up: sh kubectl get pods --all-namespaces helm list -n airflow Run `kubectl port-forward svc/airflow-webserver 8080:8080 -n airflow` to port-forward the Airflow UI to http://localhost:8080/ to confirm Airflow is working. Login as _admin_ and password _admin_. Build a Docker image from your DAGs: 1. Start a project using astro-cli, which will generate a Dockerfile, and load your DAGs in. You can test locally before pushing to kind with `astro airflow start`. `sh mkdir my-airflow-project && cd my-airflow-project astro dev init` 2. Then build the image: `sh docker build -t my-dags:0.0.1 .` 3. Load the image into kind: `sh kind load docker-image my-dags:0.0.1` 4. Upgrade Helm deployment: sh helm upgrade airflow -n airflow --set images.airflow.repository=my-dags --set images.airflow.tag=0.0.1 astronomer/airflow Extra Objects: This chart can deploy extra Kubernetes objects (assuming the role used by Helm can manage them). For Astronomer Cloud and Enterprise, the role permissions can be found in the Commander role. yaml extraObjects: - apiVersion: batch/v1beta1 kind: CronJob metadata: name: "{{ .Release.Name }}-somejob" spec: schedule: "*/10 * * * *" concurrencyPolicy: Forbid jobTemplate: spec: template: spec: containers: - name: myjob image: ubuntu command: - echo args: - hello restartPolicy: OnFailure Contributing: Check out our contributing guide! License: Apache 2.0 with Commons Clause
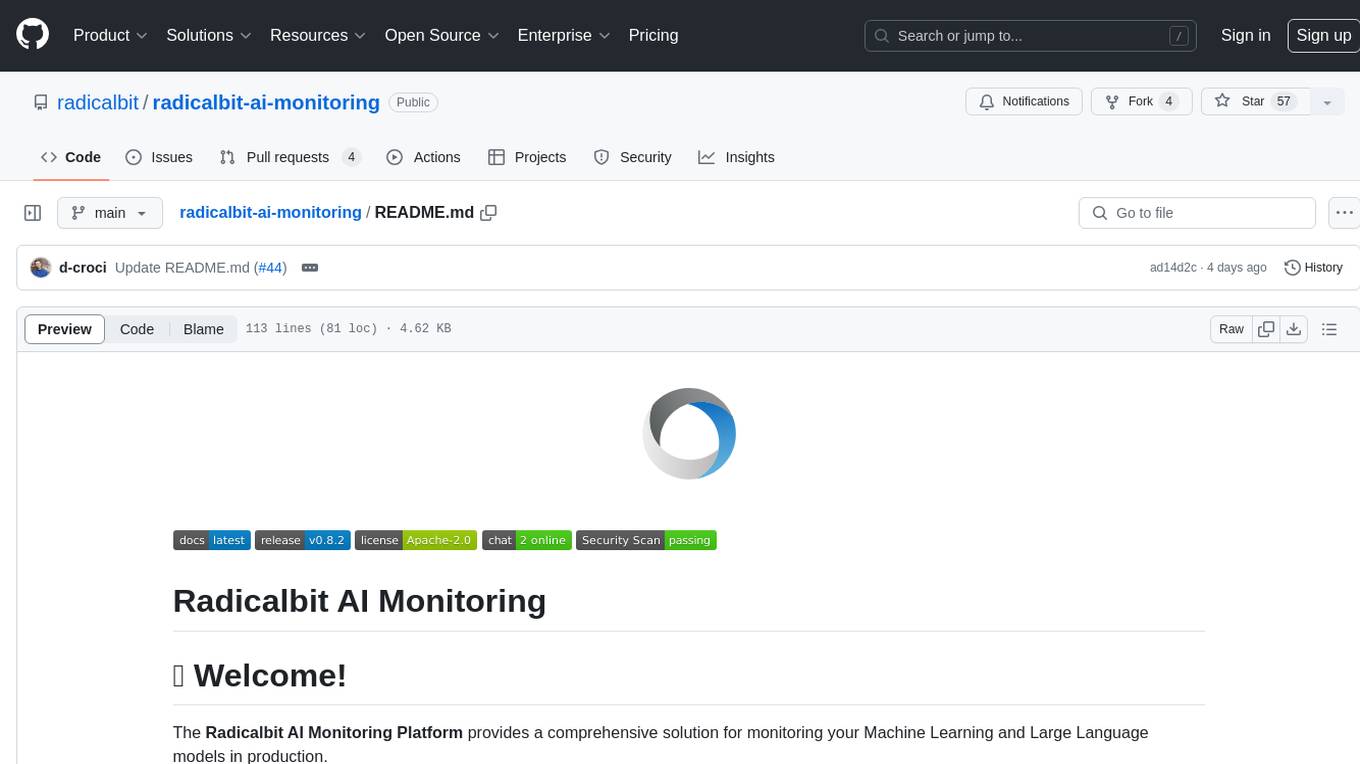
radicalbit-ai-monitoring
The Radicalbit AI Monitoring Platform provides a comprehensive solution for monitoring Machine Learning and Large Language models in production. It helps proactively identify and address potential performance issues by analyzing data quality, model quality, and model drift. The repository contains files and projects for running the platform, including UI, API, SDK, and Spark components. Installation using Docker compose is provided, allowing deployment with a K3s cluster and interaction with a k9s container. The platform documentation includes a step-by-step guide for installation and creating dashboards. Community engagement is encouraged through a Discord server. The roadmap includes adding functionalities for batch and real-time workloads, covering various model types and tasks.
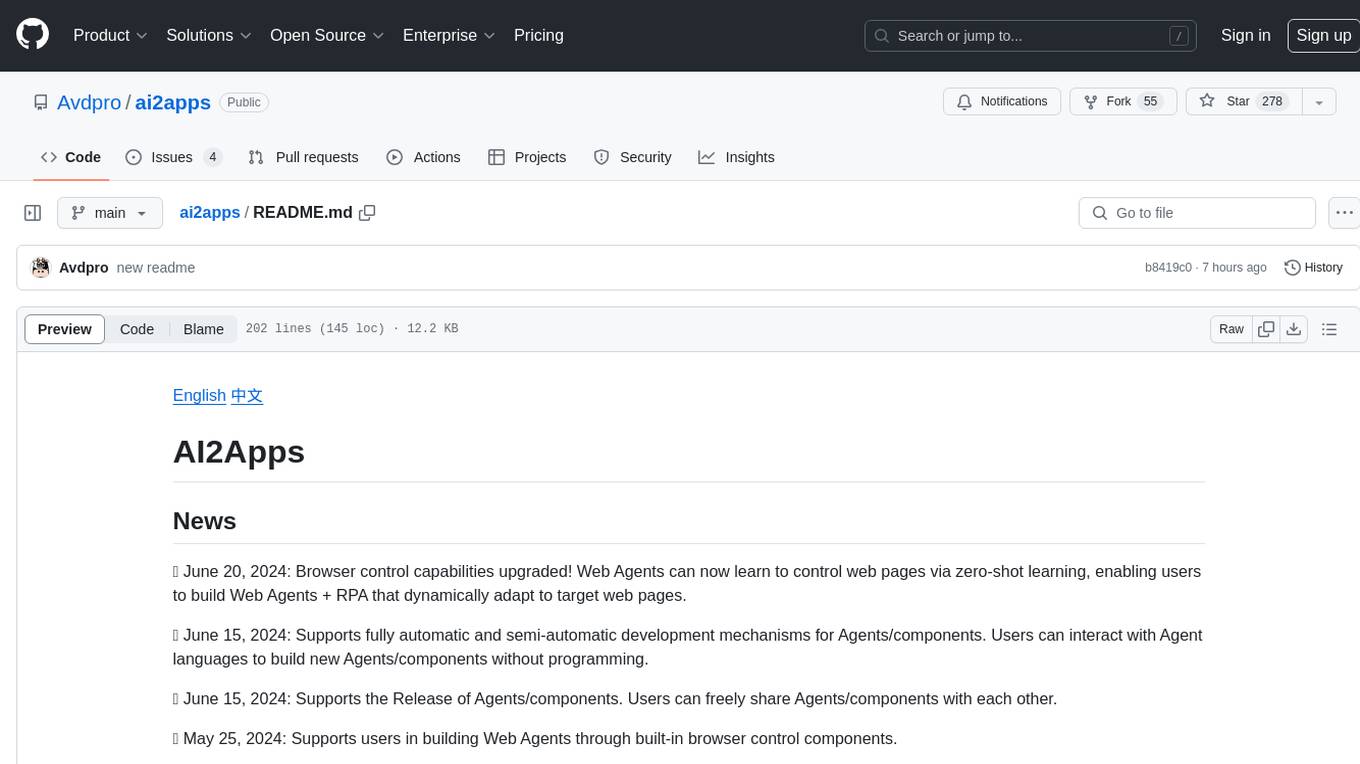
ai2apps
AI2Apps is a visual IDE for building LLM-based AI agent applications, enabling developers to efficiently create AI agents through drag-and-drop, with features like design-to-development for rapid prototyping, direct packaging of agents into apps, powerful debugging capabilities, enhanced user interaction, efficient team collaboration, flexible deployment, multilingual support, simplified product maintenance, and extensibility through plugins.
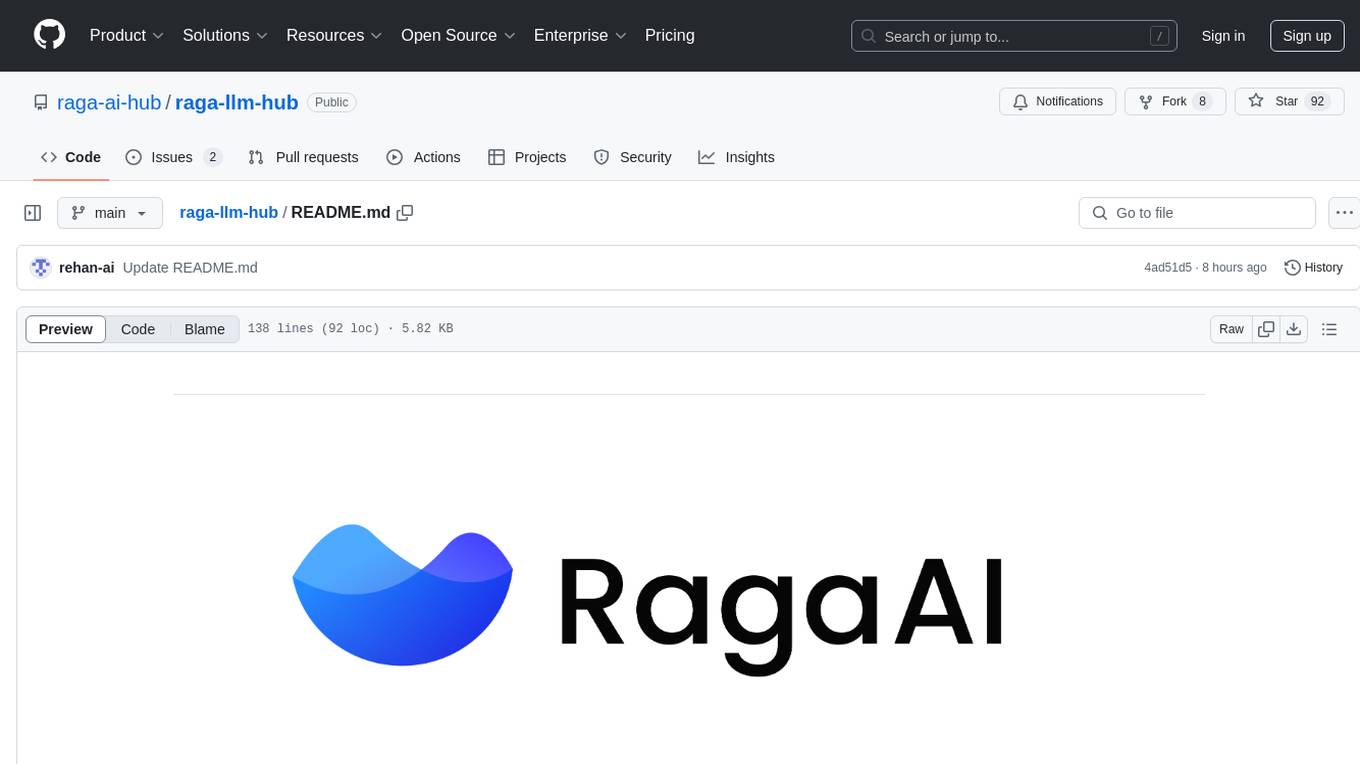
raga-llm-hub
Raga LLM Hub is a comprehensive evaluation toolkit for Language and Learning Models (LLMs) with over 100 meticulously designed metrics. It allows developers and organizations to evaluate and compare LLMs effectively, establishing guardrails for LLMs and Retrieval Augmented Generation (RAG) applications. The platform assesses aspects like Relevance & Understanding, Content Quality, Hallucination, Safety & Bias, Context Relevance, Guardrails, and Vulnerability scanning, along with Metric-Based Tests for quantitative analysis. It helps teams identify and fix issues throughout the LLM lifecycle, revolutionizing reliability and trustworthiness.
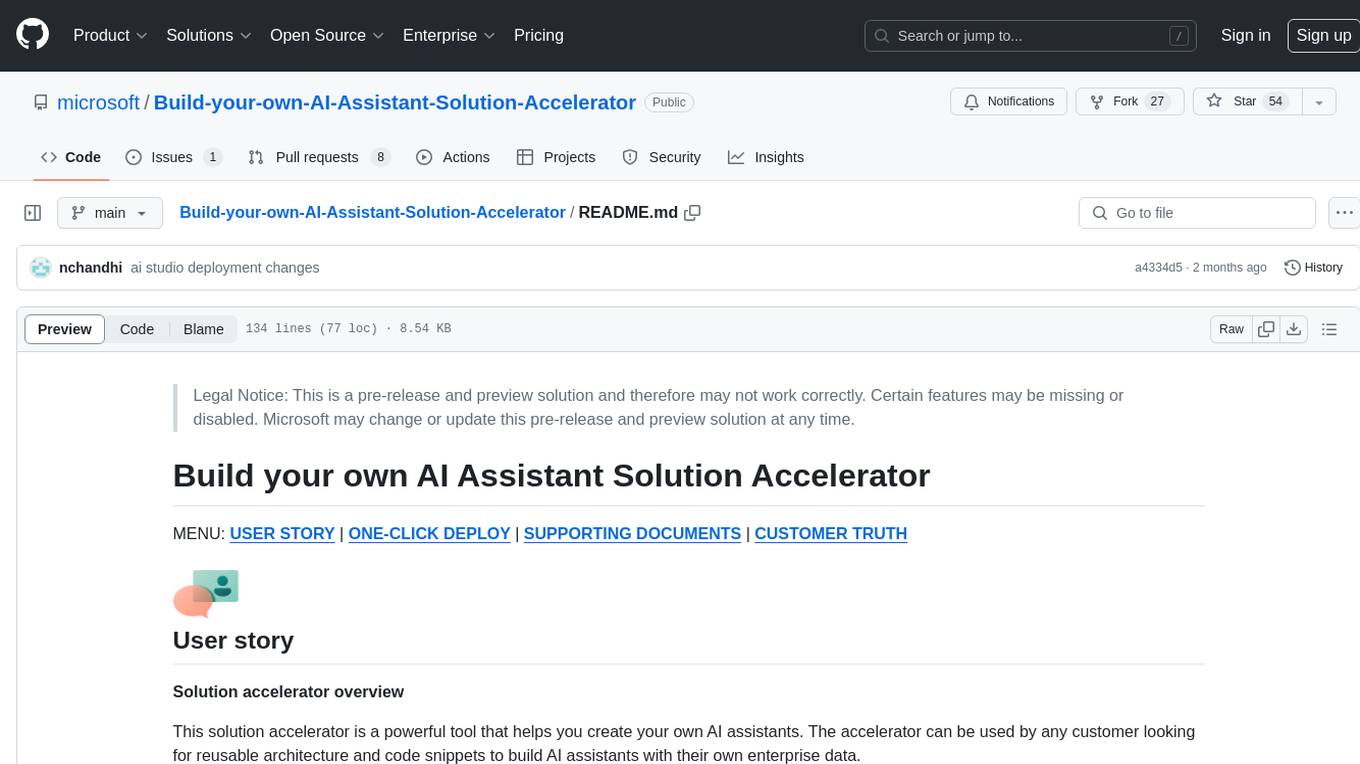
Build-your-own-AI-Assistant-Solution-Accelerator
Build-your-own-AI-Assistant-Solution-Accelerator is a pre-release and preview solution that helps users create their own AI assistants. It leverages Azure Open AI Service, Azure AI Search, and Microsoft Fabric to identify, summarize, and categorize unstructured information. Users can easily find relevant articles and grants, generate grant applications, and export them as PDF or Word documents. The solution accelerator provides reusable architecture and code snippets for building AI assistants with enterprise data. It is designed for researchers looking to explore flu vaccine studies and grants to accelerate grant proposal submissions.
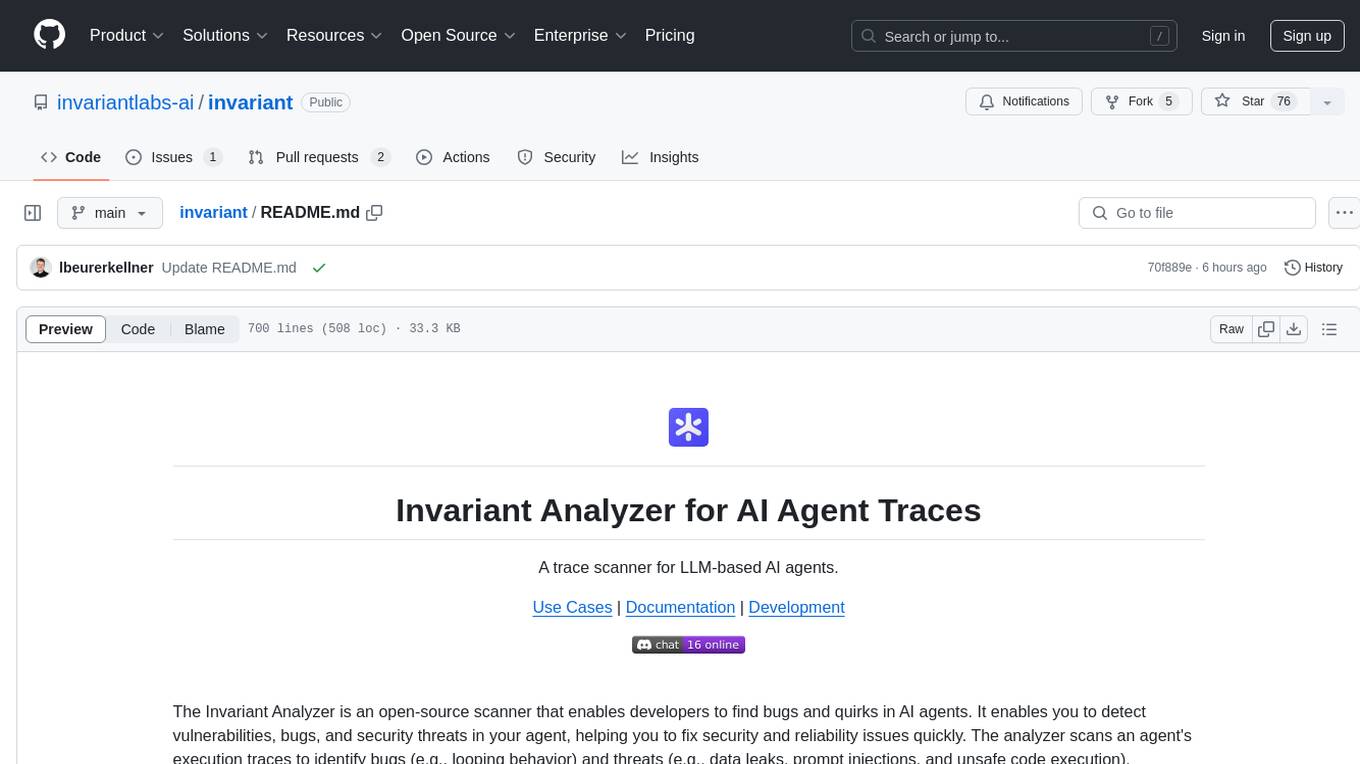
invariant
Invariant Analyzer is an open-source scanner designed for LLM-based AI agents to find bugs, vulnerabilities, and security threats. It scans agent execution traces to identify issues like looping behavior, data leaks, prompt injections, and unsafe code execution. The tool offers a library of built-in checkers, an expressive policy language, data flow analysis, real-time monitoring, and extensible architecture for custom checkers. It helps developers debug AI agents, scan for security violations, and prevent security issues and data breaches during runtime. The analyzer leverages deep contextual understanding and a purpose-built rule matching engine for security policy enforcement.
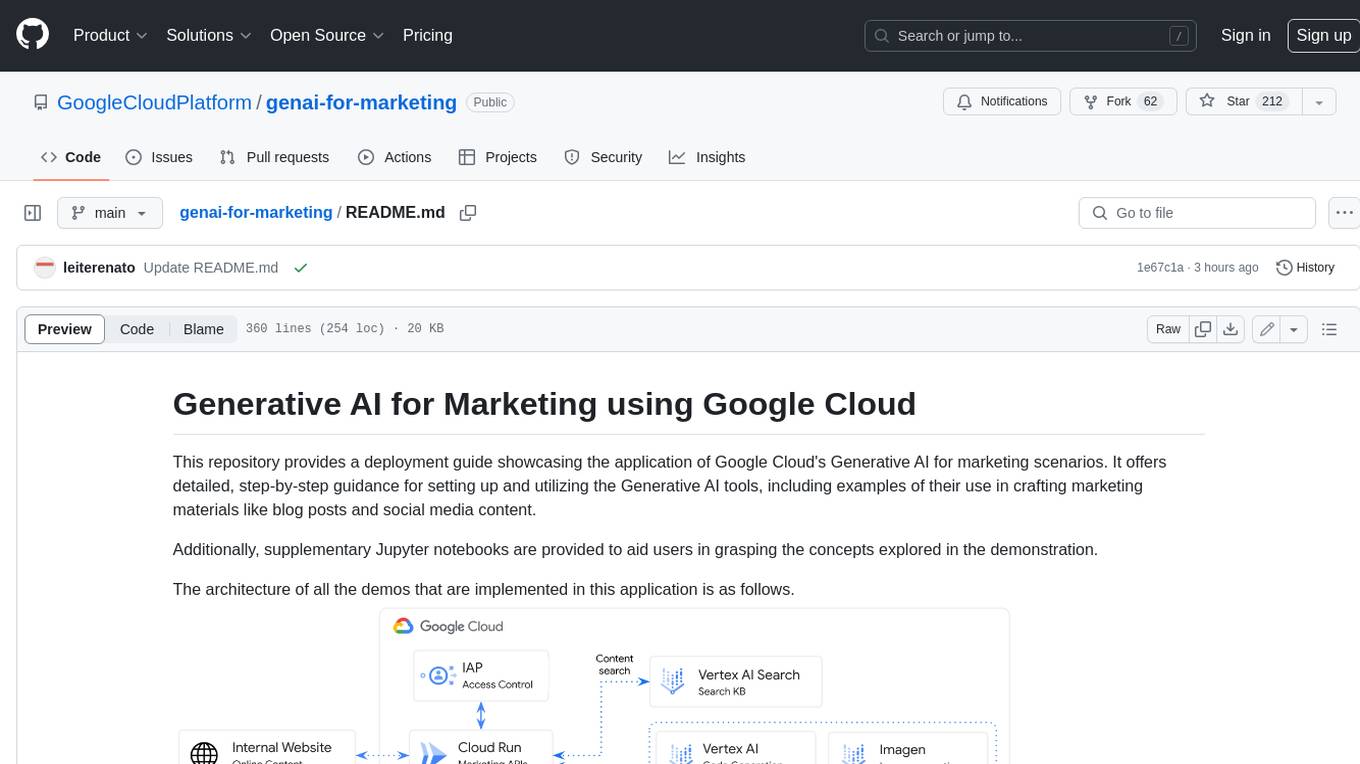
genai-for-marketing
This repository provides a deployment guide for utilizing Google Cloud's Generative AI tools in marketing scenarios. It includes step-by-step instructions, examples of crafting marketing materials, and supplementary Jupyter notebooks. The demos cover marketing insights, audience analysis, trendspotting, content search, content generation, and workspace integration. Users can access and visualize marketing data, analyze trends, improve search experience, and generate compelling content. The repository structure includes backend APIs, frontend code, sample notebooks, templates, and installation scripts.
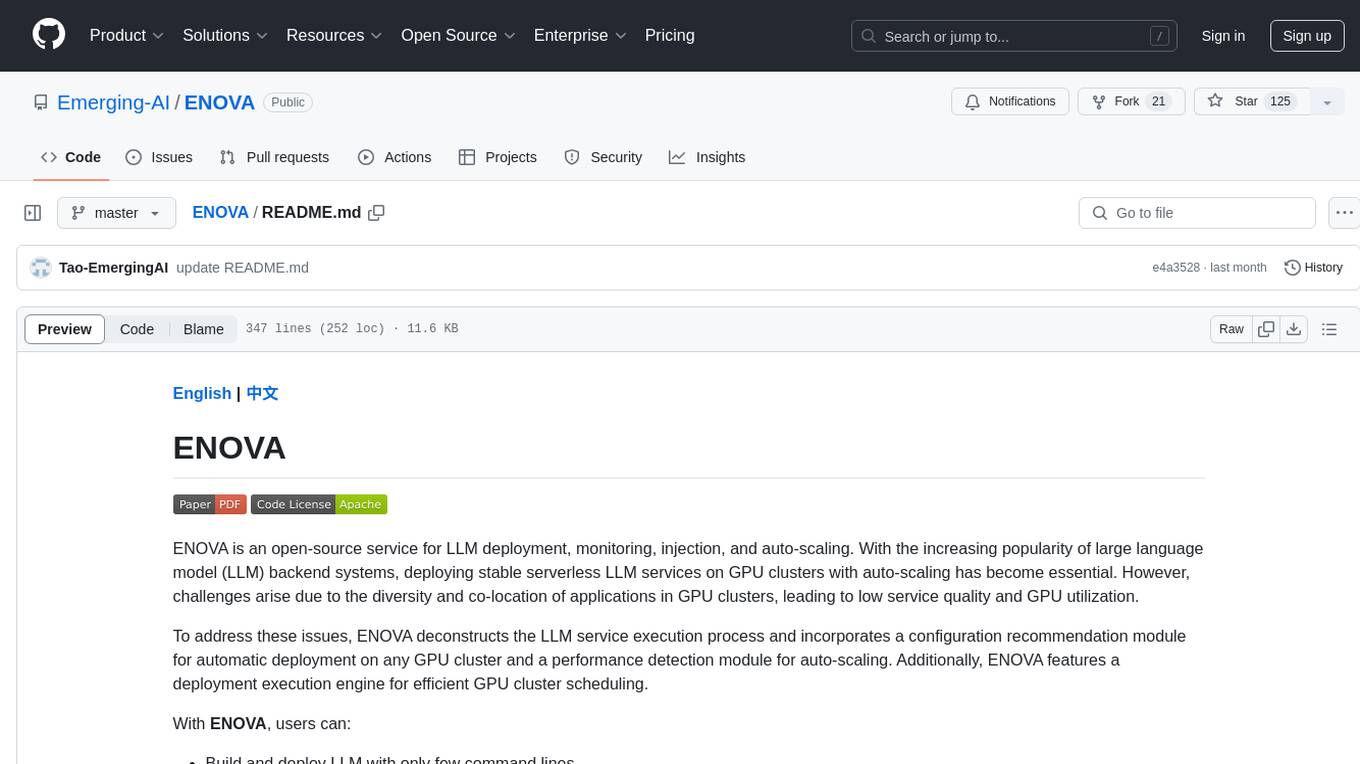
ENOVA
ENOVA is an open-source service for Large Language Model (LLM) deployment, monitoring, injection, and auto-scaling. It addresses challenges in deploying stable serverless LLM services on GPU clusters with auto-scaling by deconstructing the LLM service execution process and providing configuration recommendations and performance detection. Users can build and deploy LLM with few command lines, recommend optimal computing resources, experience LLM performance, observe operating status, achieve load balancing, and more. ENOVA ensures stable operation, cost-effectiveness, efficiency, and strong scalability of LLM services.
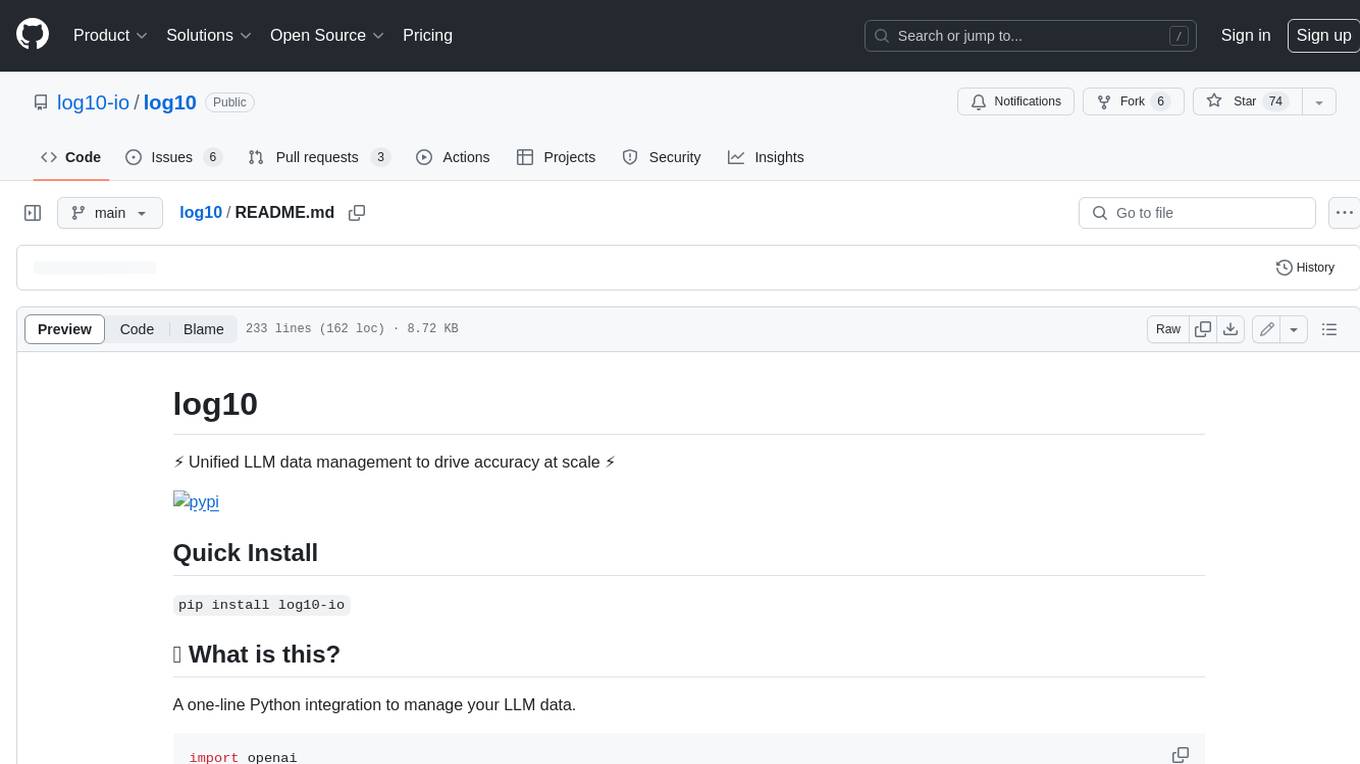
log10
Log10 is a one-line Python integration to manage your LLM data. It helps you log both closed and open-source LLM calls, compare and identify the best models and prompts, store feedback for fine-tuning, collect performance metrics such as latency and usage, and perform analytics and monitor compliance for LLM powered applications. Log10 offers various integration methods, including a python LLM library wrapper, the Log10 LLM abstraction, and callbacks, to facilitate its use in both existing production environments and new projects. Pick the one that works best for you. Log10 also provides a copilot that can help you with suggestions on how to optimize your prompt, and a feedback feature that allows you to add feedback to your completions. Additionally, Log10 provides prompt provenance, session tracking and call stack functionality to help debug prompt chains. With Log10, you can use your data and feedback from users to fine-tune custom models with RLHF, and build and deploy more reliable, accurate and efficient self-hosted models. Log10 also supports collaboration, allowing you to create flexible groups to share and collaborate over all of the above features.
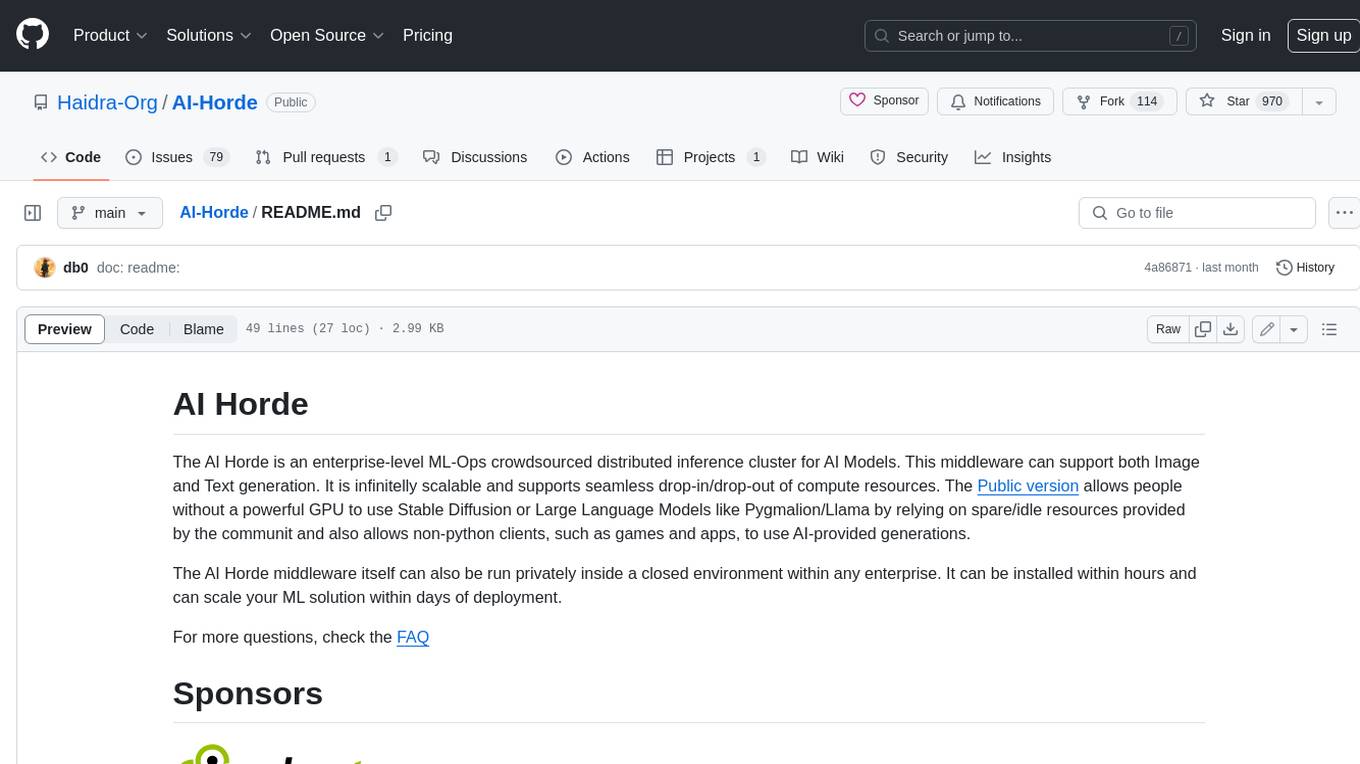
AI-Horde
The AI Horde is an enterprise-level ML-Ops crowdsourced distributed inference cluster for AI Models. This middleware can support both Image and Text generation. It is infinitely scalable and supports seamless drop-in/drop-out of compute resources. The Public version allows people without a powerful GPU to use Stable Diffusion or Large Language Models like Pygmalion/Llama by relying on spare/idle resources provided by the community and also allows non-python clients, such as games and apps, to use AI-provided generations.
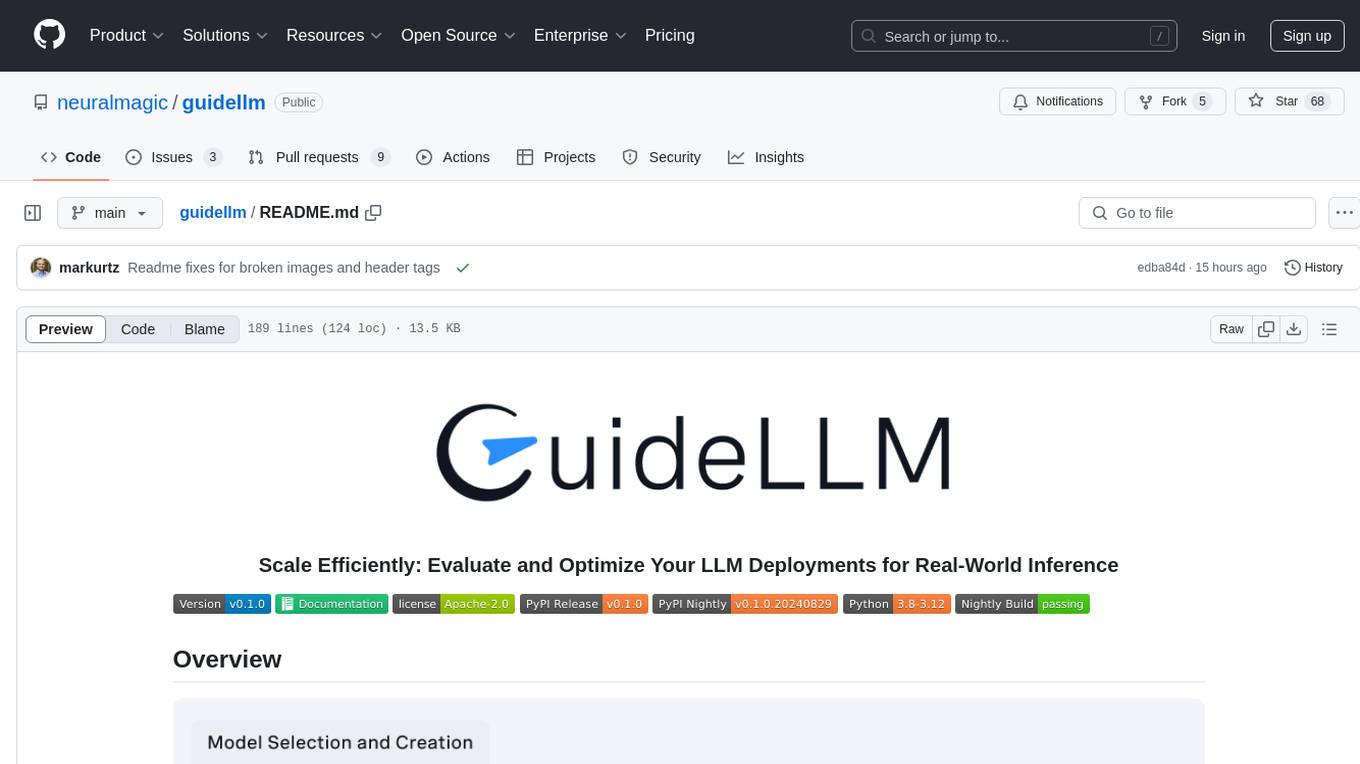
guidellm
GuideLLM is a powerful tool for evaluating and optimizing the deployment of large language models (LLMs). By simulating real-world inference workloads, GuideLLM helps users gauge the performance, resource needs, and cost implications of deploying LLMs on various hardware configurations. This approach ensures efficient, scalable, and cost-effective LLM inference serving while maintaining high service quality. Key features include performance evaluation, resource optimization, cost estimation, and scalability testing.
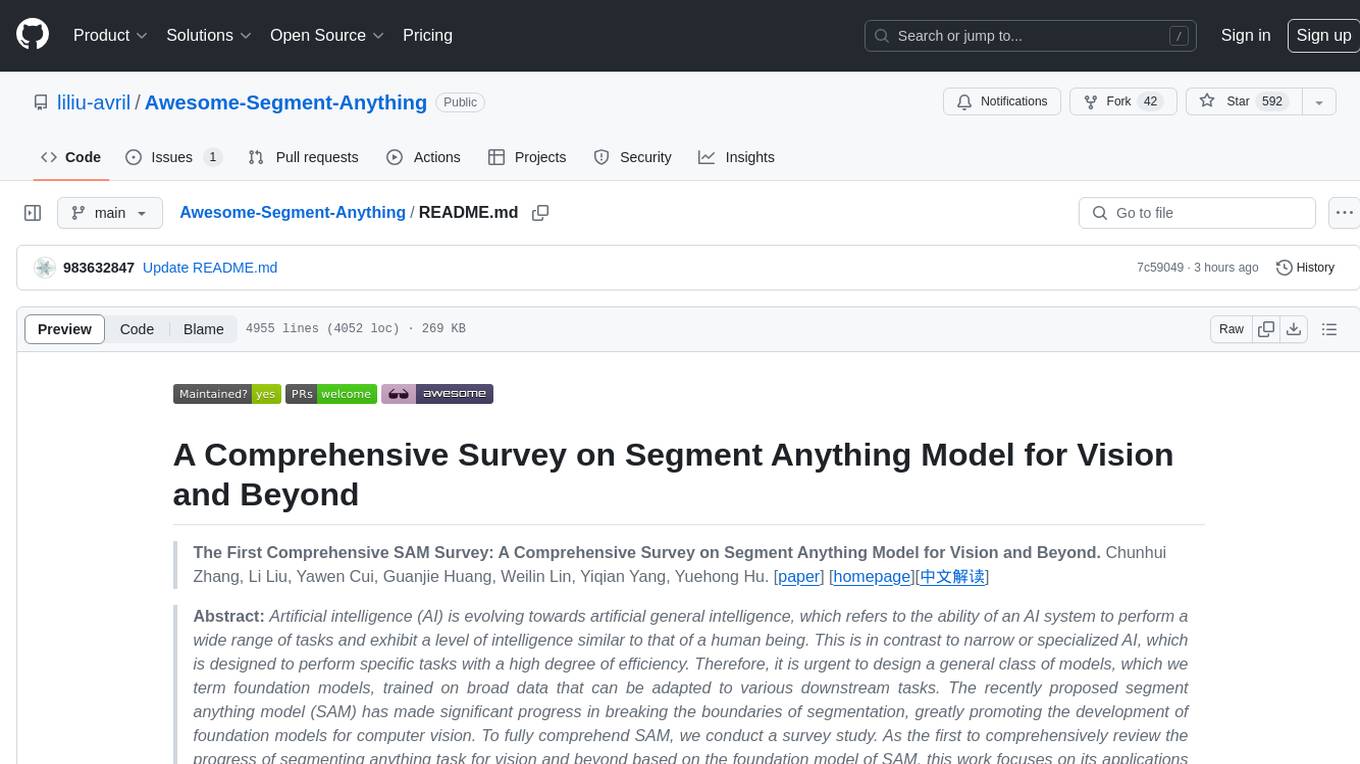
Awesome-Segment-Anything
Awesome-Segment-Anything is a powerful tool for segmenting and extracting information from various types of data. It provides a user-friendly interface to easily define segmentation rules and apply them to text, images, and other data formats. The tool supports both supervised and unsupervised segmentation methods, allowing users to customize the segmentation process based on their specific needs. With its versatile functionality and intuitive design, Awesome-Segment-Anything is ideal for data analysts, researchers, content creators, and anyone looking to efficiently extract valuable insights from complex datasets.
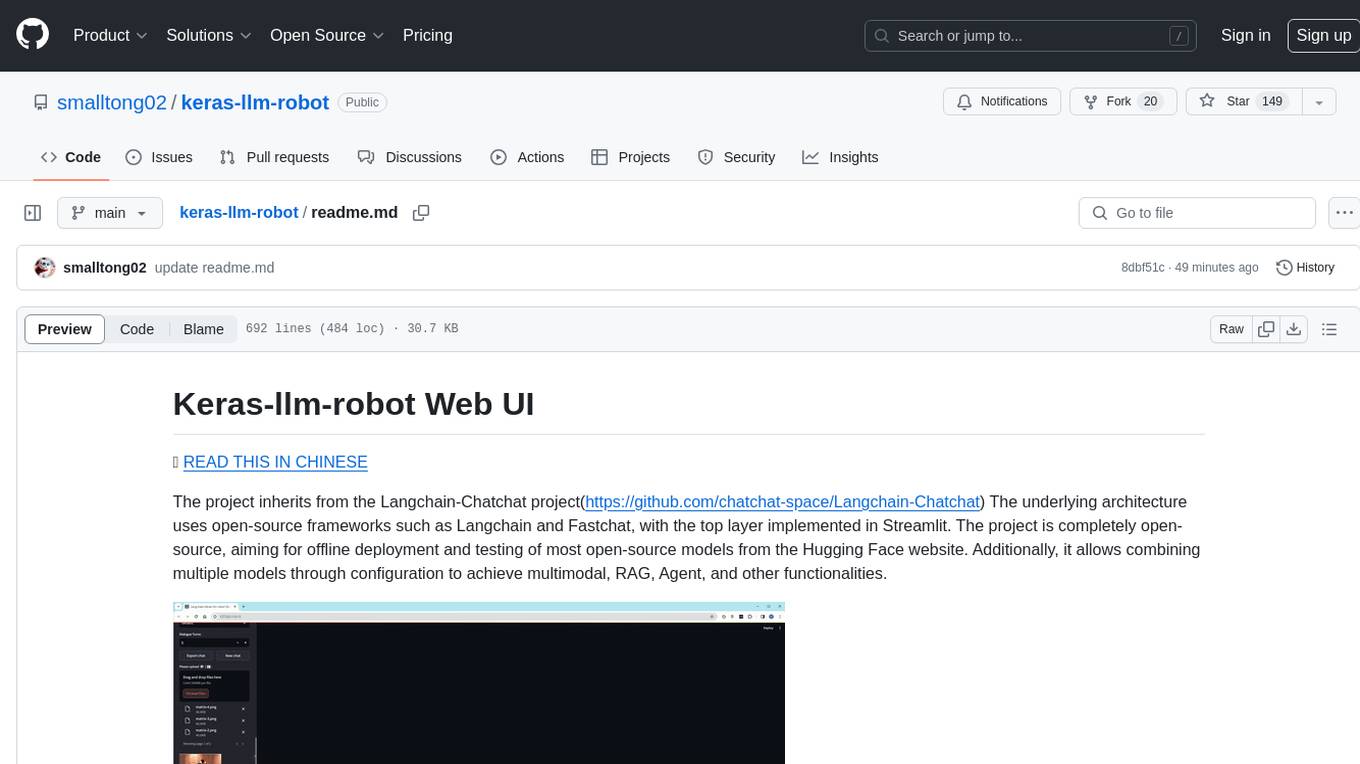
keras-llm-robot
The Keras-llm-robot Web UI project is an open-source tool designed for offline deployment and testing of various open-source models from the Hugging Face website. It allows users to combine multiple models through configuration to achieve functionalities like multimodal, RAG, Agent, and more. The project consists of three main interfaces: chat interface for language models, configuration interface for loading models, and tools & agent interface for auxiliary models. Users can interact with the language model through text, voice, and image inputs, and the tool supports features like model loading, quantization, fine-tuning, role-playing, code interpretation, speech recognition, image recognition, network search engine, and function calling.
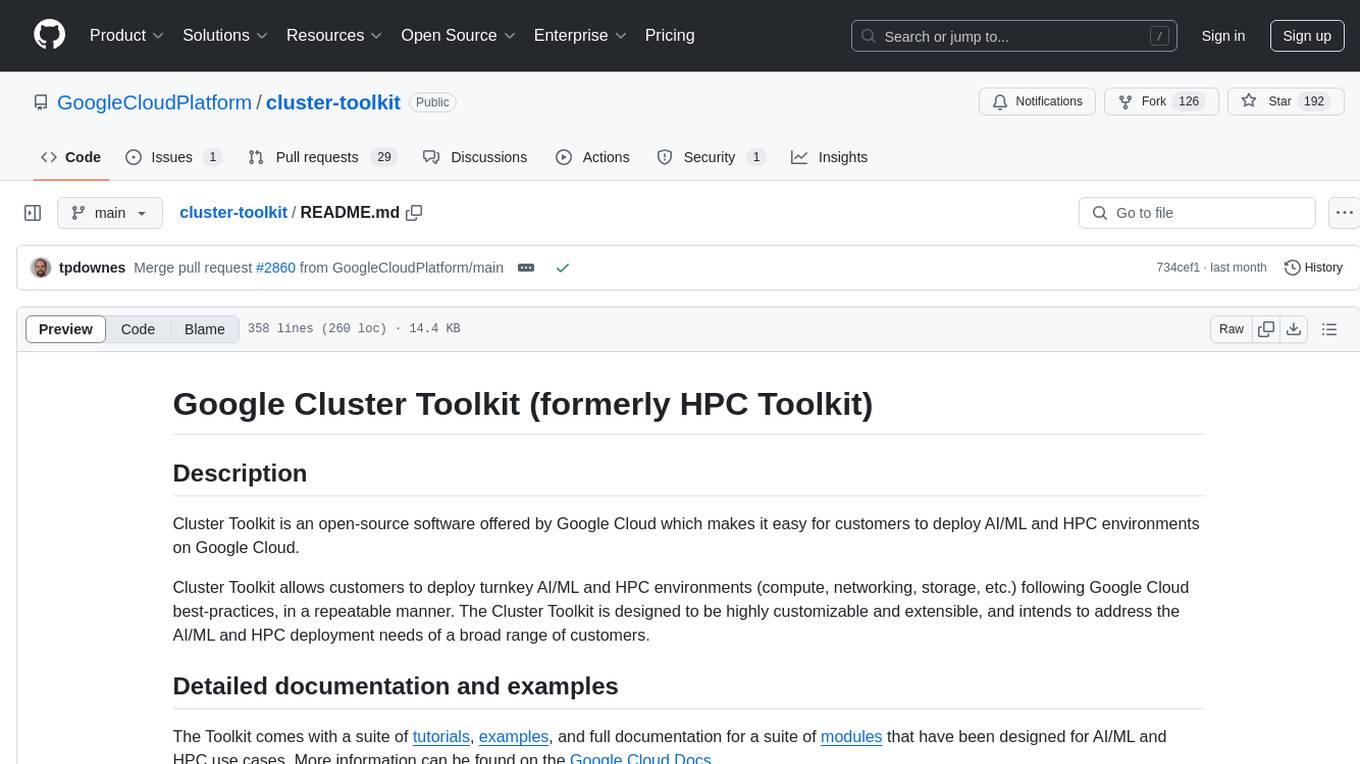
cluster-toolkit
Cluster Toolkit is an open-source software by Google Cloud for deploying AI/ML and HPC environments on Google Cloud. It allows easy deployment following best practices, with high customization and extensibility. The toolkit includes tutorials, examples, and documentation for various modules designed for AI/ML and HPC use cases.
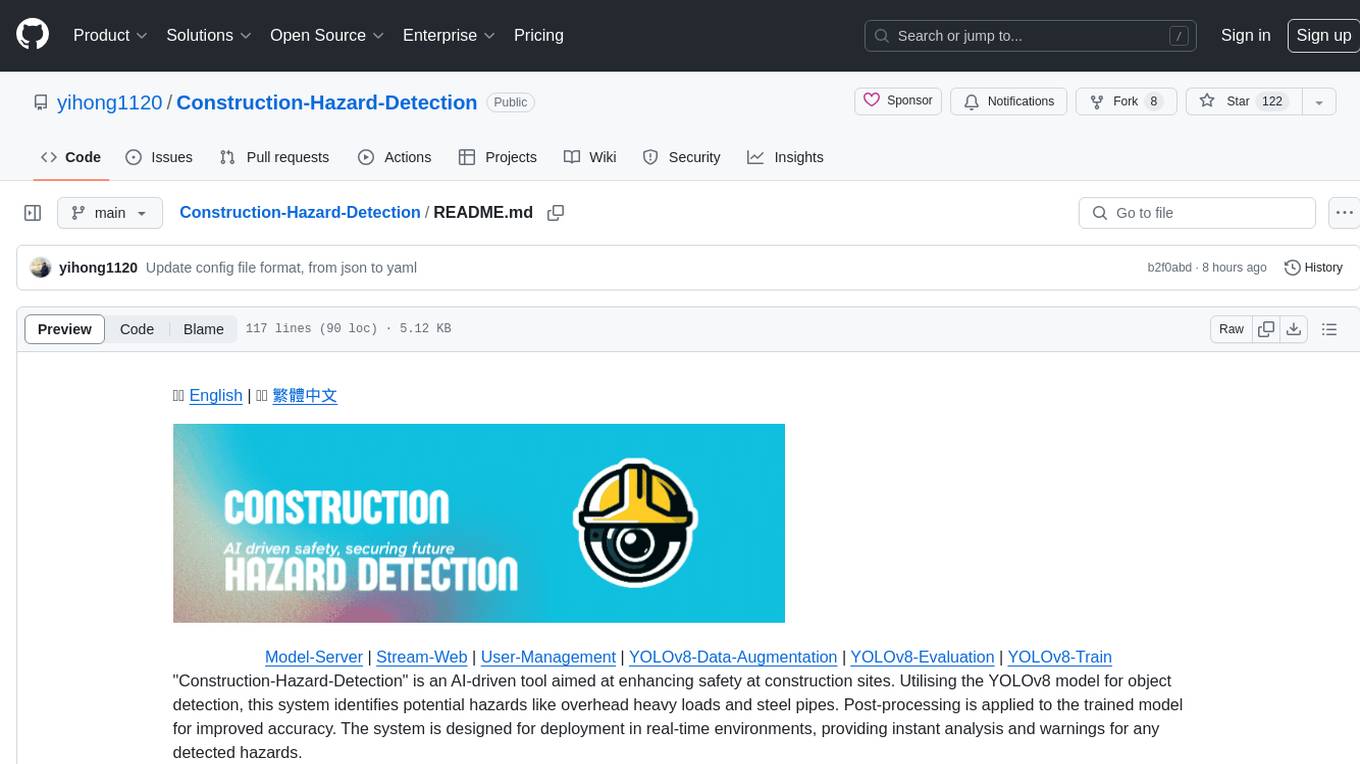
Construction-Hazard-Detection
Construction-Hazard-Detection is an AI-driven tool focused on improving safety at construction sites by utilizing the YOLOv8 model for object detection. The system identifies potential hazards like overhead heavy loads and steel pipes, providing real-time analysis and warnings. Users can configure the system via a YAML file and run it using Docker. The primary dataset used for training is the Construction Site Safety Image Dataset enriched with additional annotations. The system logs are accessible within the Docker container for debugging, and notifications are sent through the LINE messaging API when hazards are detected.
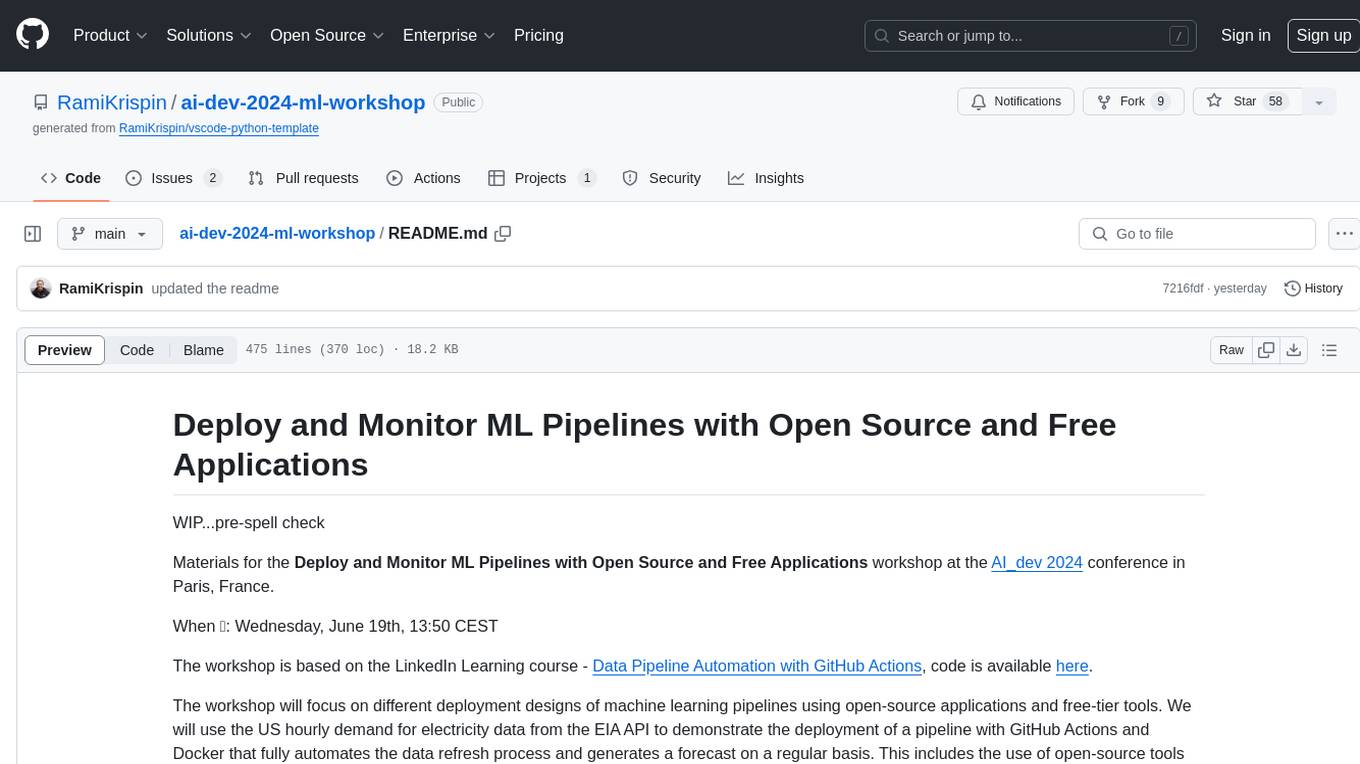
ai-dev-2024-ml-workshop
The 'ai-dev-2024-ml-workshop' repository contains materials for the Deploy and Monitor ML Pipelines workshop at the AI_dev 2024 conference in Paris, focusing on deployment designs of machine learning pipelines using open-source applications and free-tier tools. It demonstrates automating data refresh and forecasting using GitHub Actions and Docker, monitoring with MLflow and YData Profiling, and setting up a monitoring dashboard with Quarto doc on GitHub Pages.
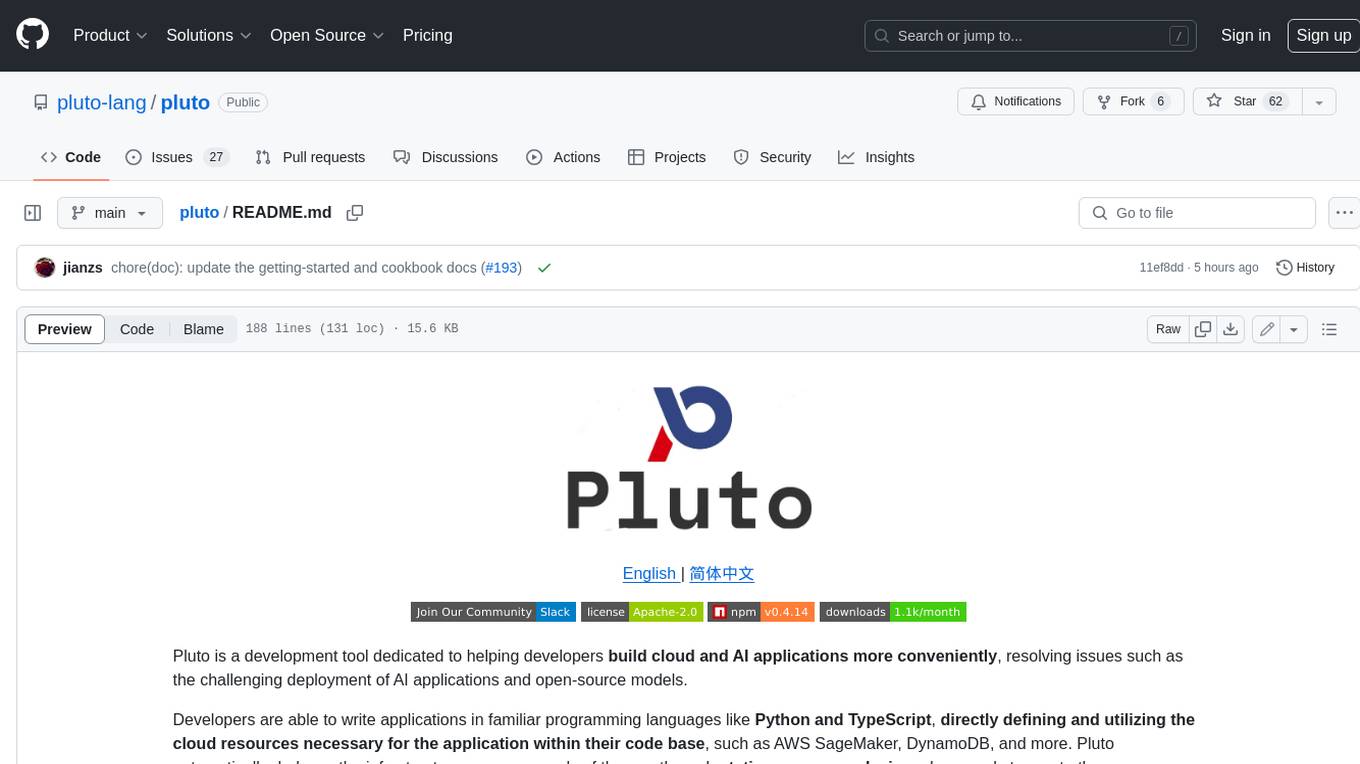
pluto
Pluto is a development tool dedicated to helping developers **build cloud and AI applications more conveniently** , resolving issues such as the challenging deployment of AI applications and open-source models. Developers are able to write applications in familiar programming languages like **Python and TypeScript** , **directly defining and utilizing the cloud resources necessary for the application within their code base** , such as AWS SageMaker, DynamoDB, and more. Pluto automatically deduces the infrastructure resource needs of the app through **static program analysis** and proceeds to create these resources on the specified cloud platform, **simplifying the resources creation and application deployment process**.
20 - OpenAI Gpts
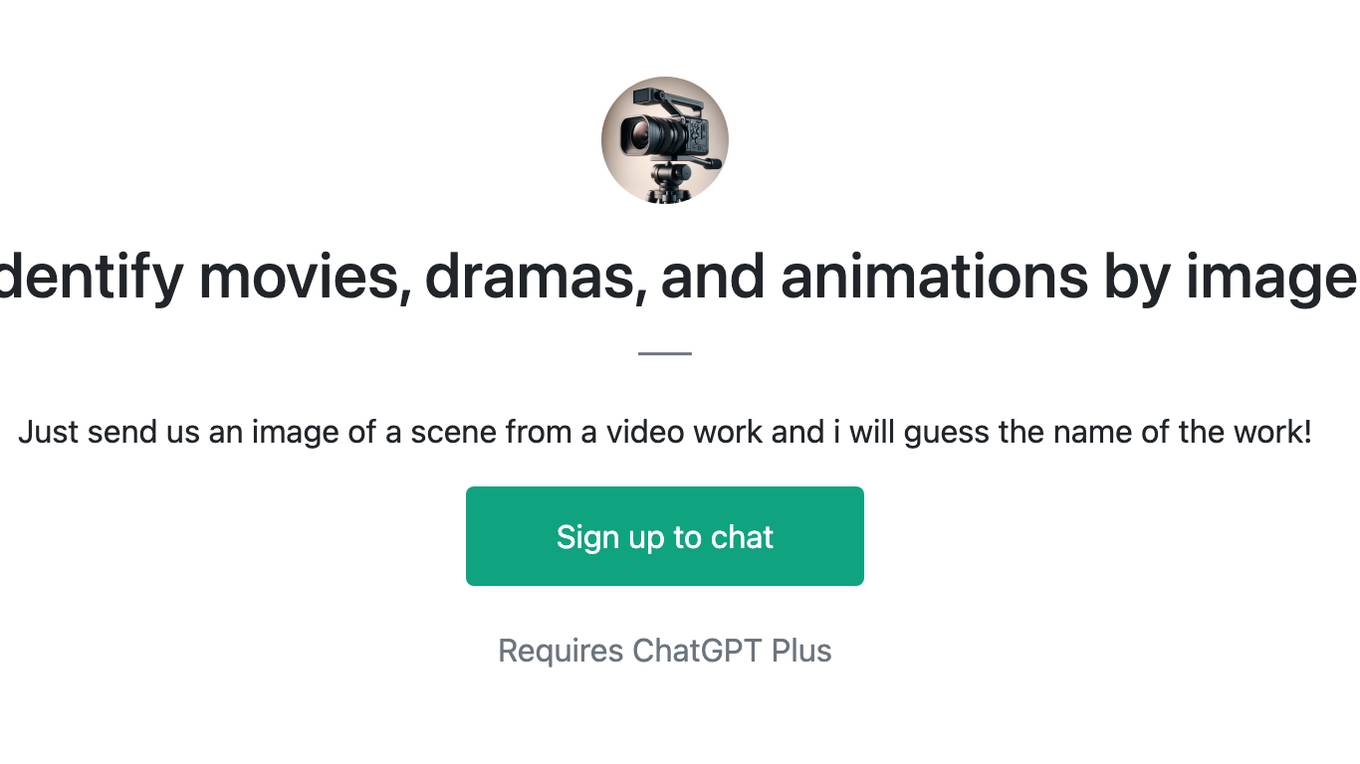
Identify movies, dramas, and animations by image
Just send us an image of a scene from a video work and i will guess the name of the work!
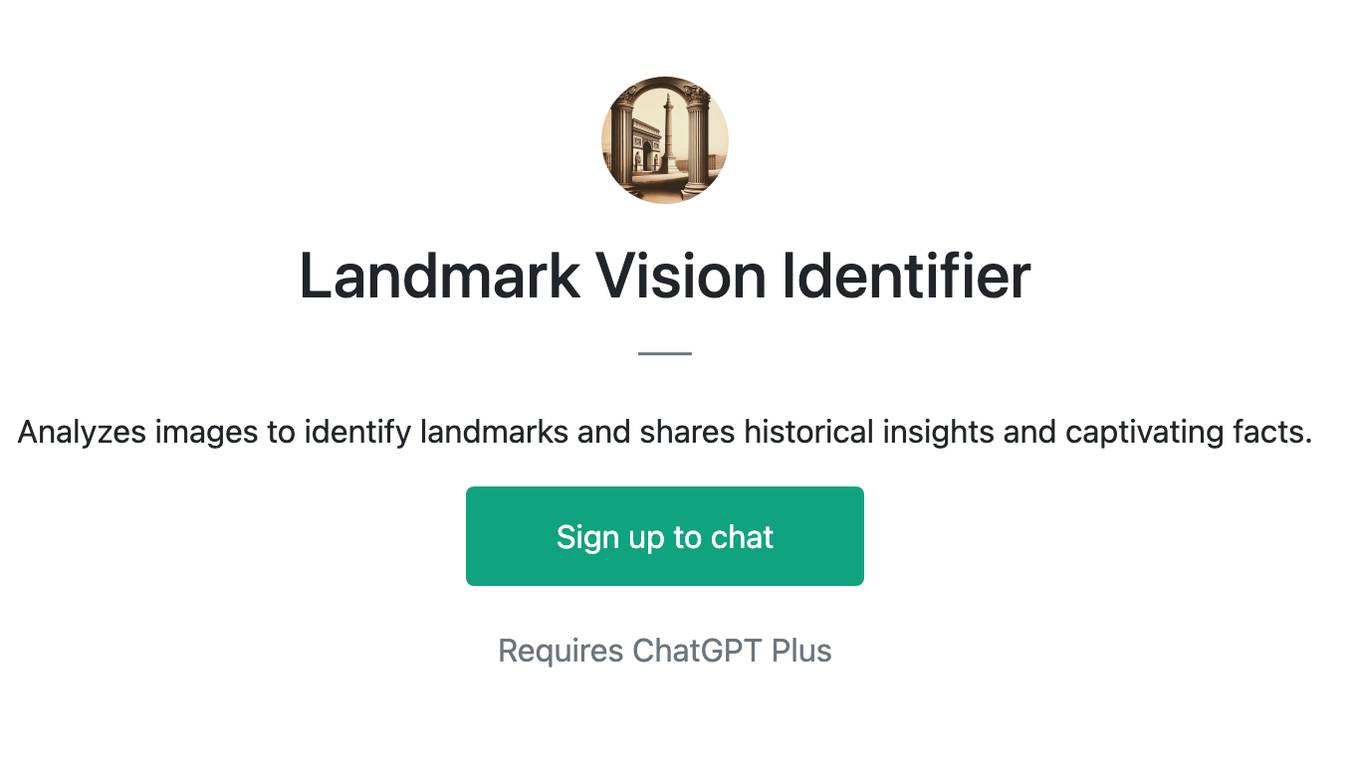
Landmark Vision Identifier
Analyzes images to identify landmarks and shares historical insights and captivating facts.
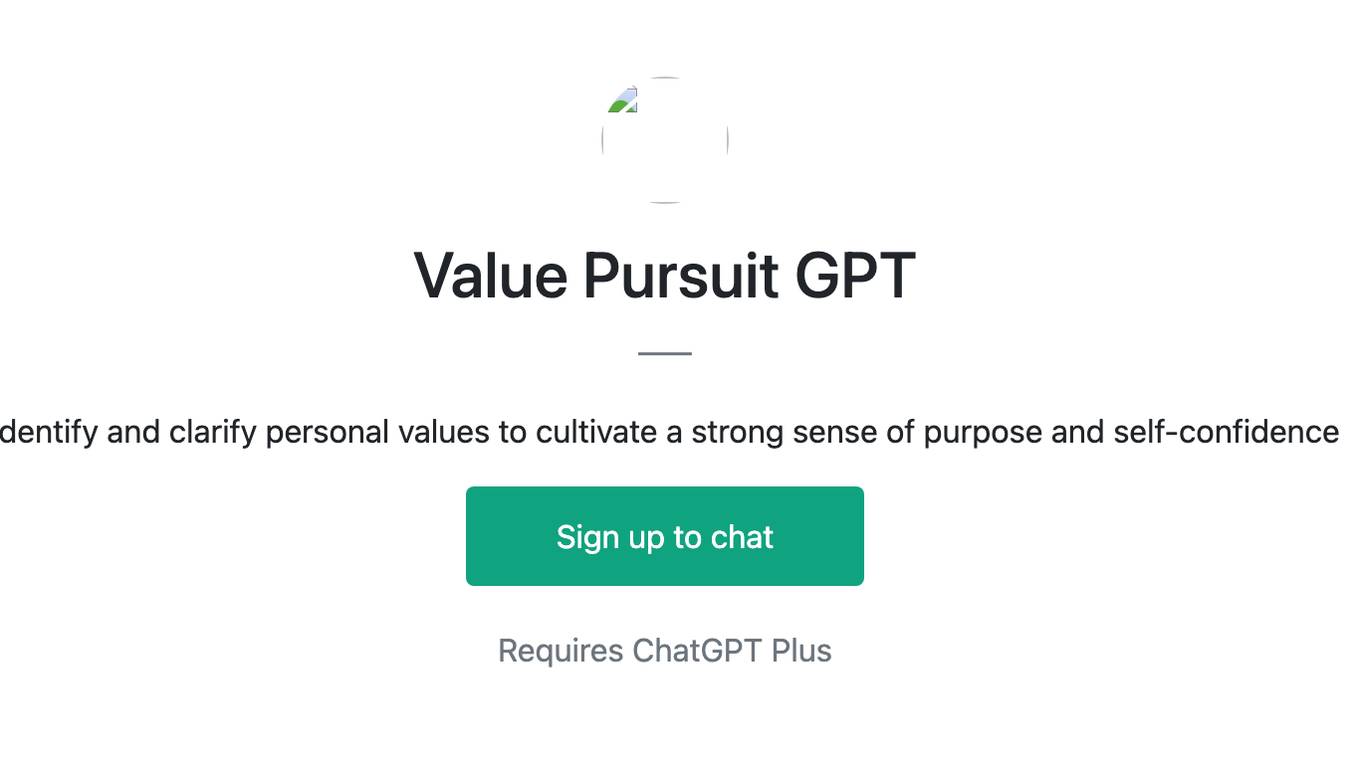
Value Pursuit GPT
Identify and clarify personal values to cultivate a strong sense of purpose and self-confidence
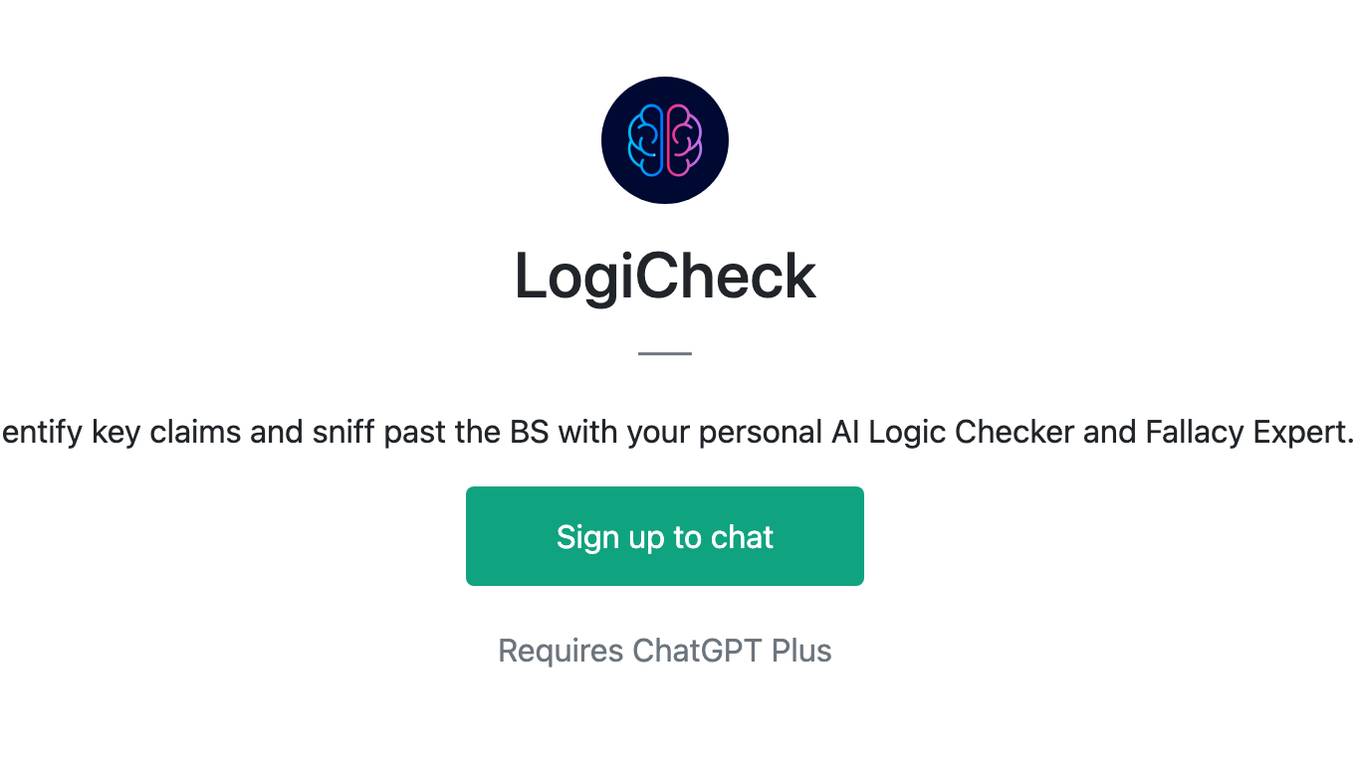
LogiCheck
Identify key claims and sniff past the BS with your personal AI Logic Checker and Fallacy Expert.
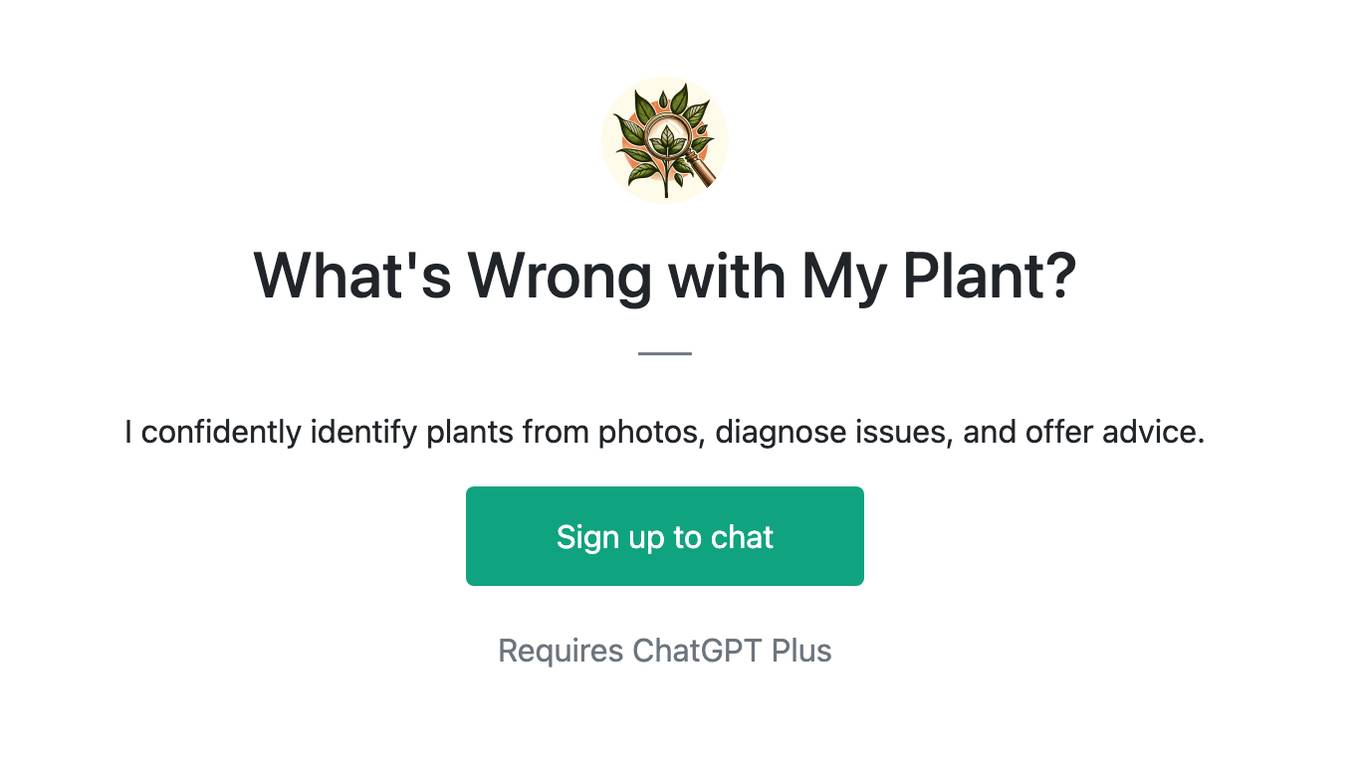
What's Wrong with My Plant?
I confidently identify plants from photos, diagnose issues, and offer advice.
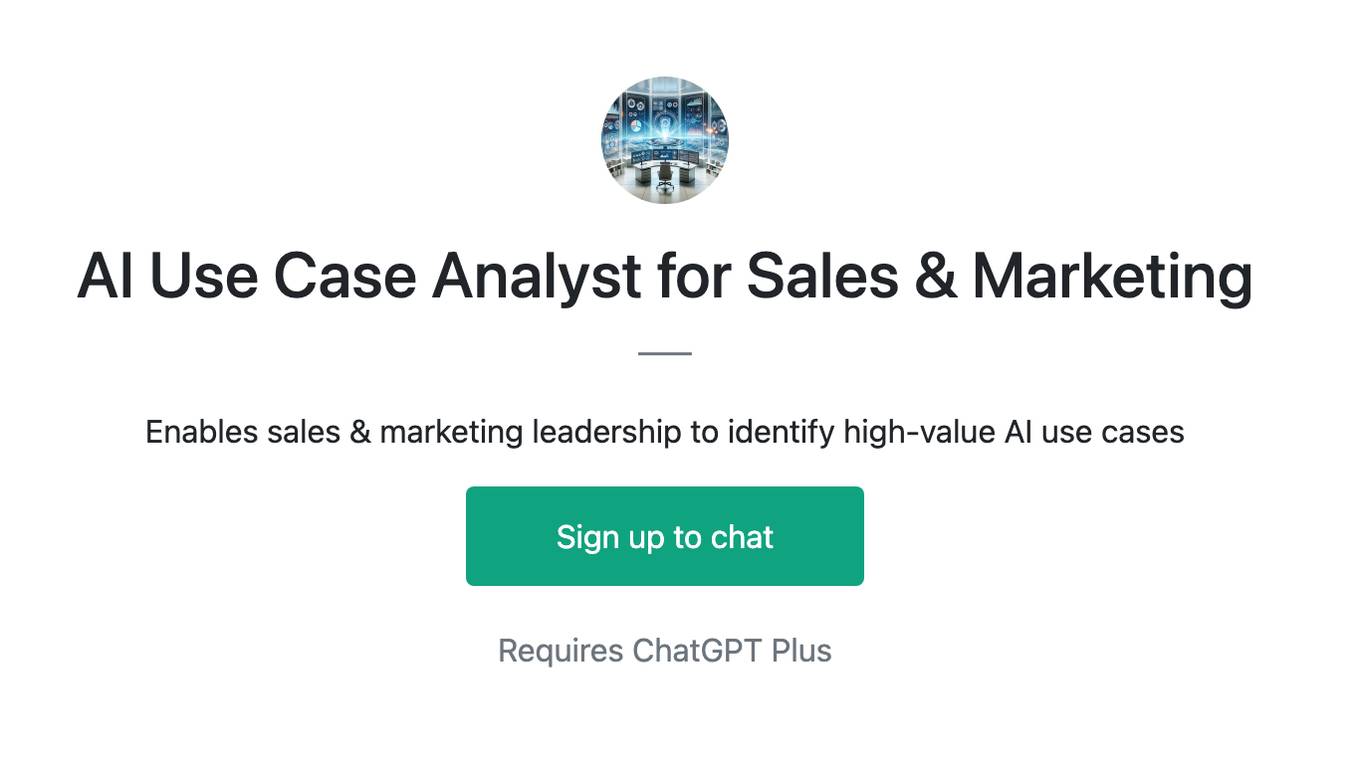
AI Use Case Analyst for Sales & Marketing
Enables sales & marketing leadership to identify high-value AI use cases
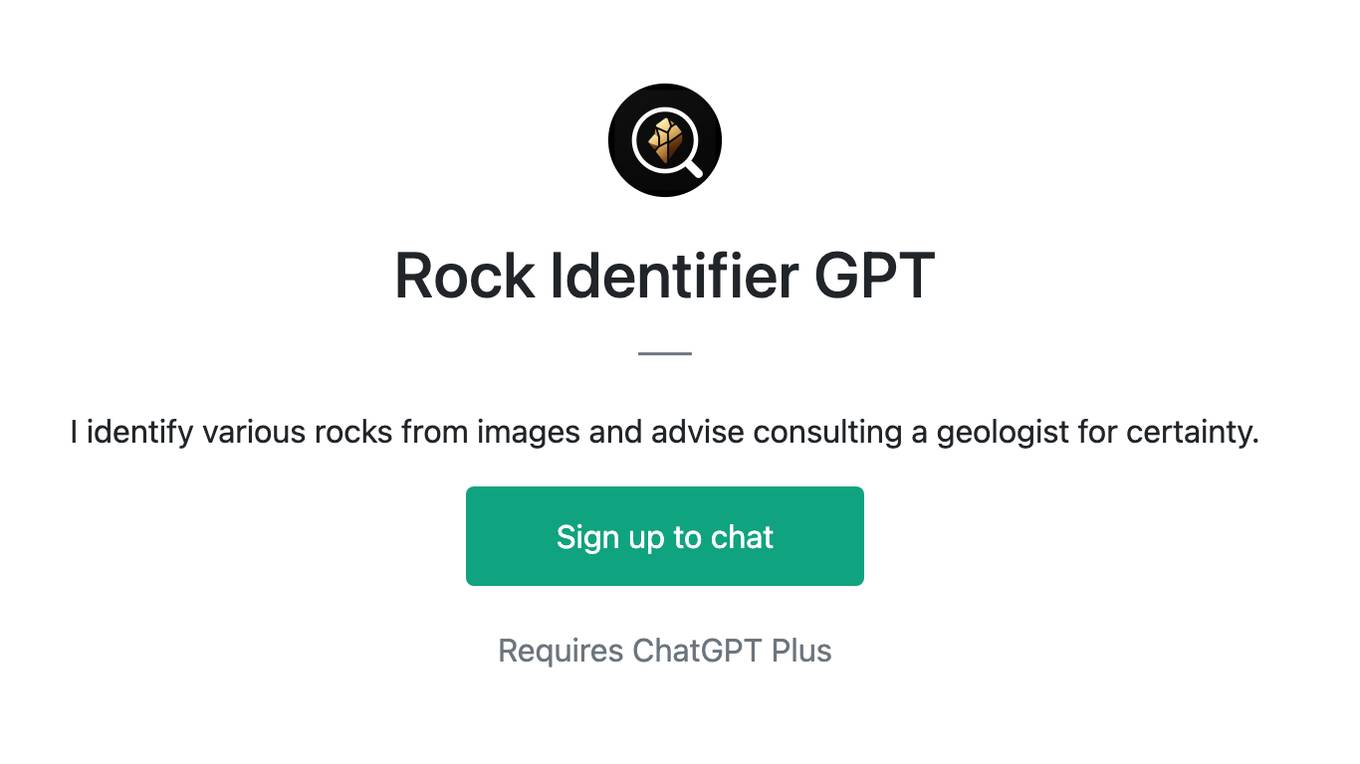
Rock Identifier GPT
I identify various rocks from images and advise consulting a geologist for certainty.
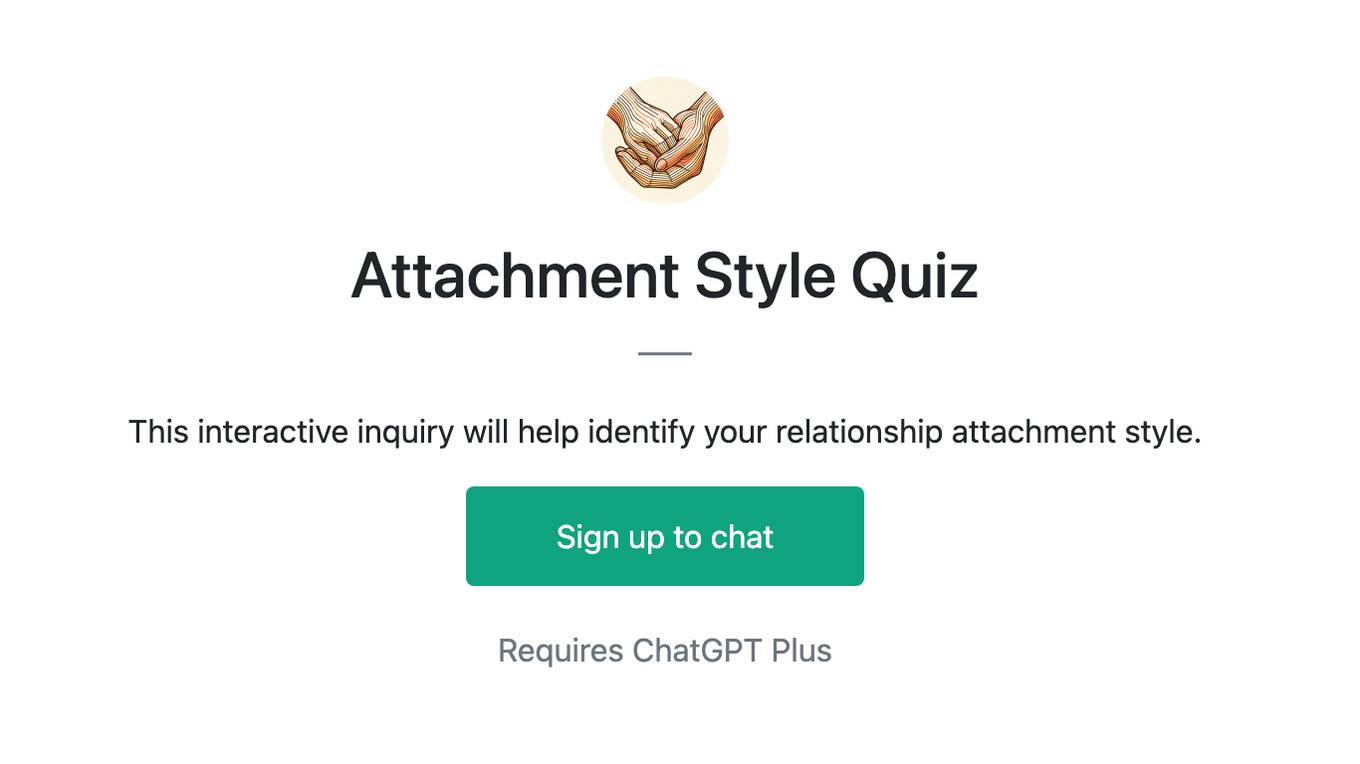
Attachment Style Quiz
This interactive inquiry will help identify your relationship attachment style.
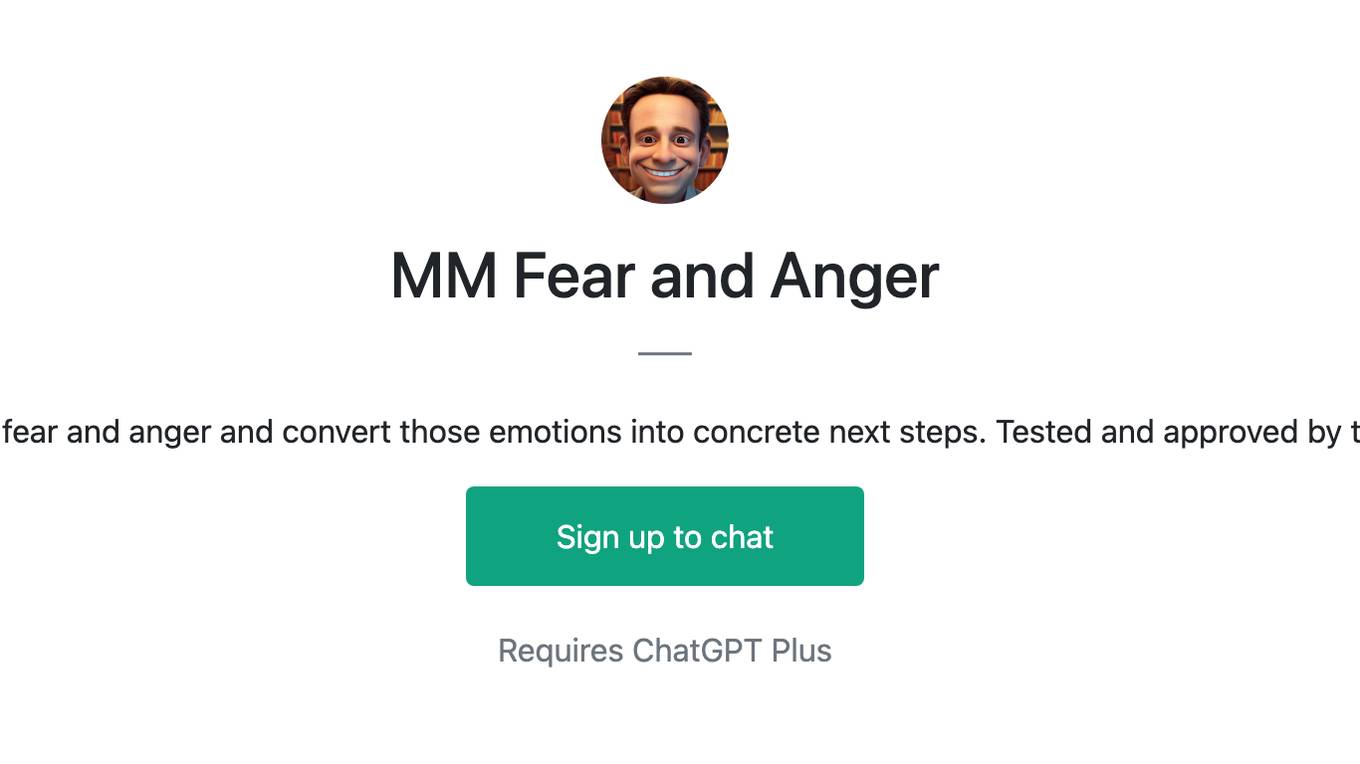
MM Fear and Anger
Identify your sources of fear and anger and convert those emotions into concrete next steps. Tested and approved by the real Matt Mochary!
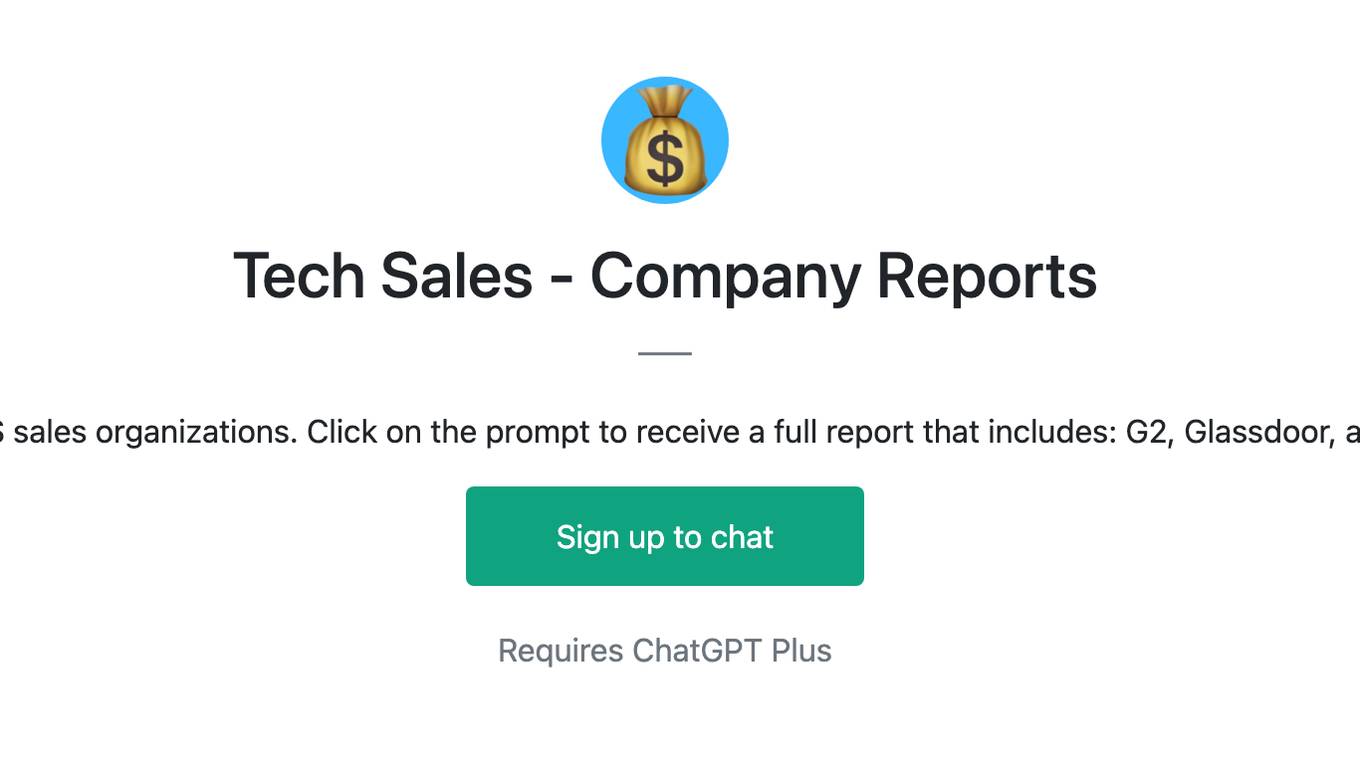
Tech Sales - Company Reports
Identify the best SaaS sales organizations. Click on the prompt to receive a full report that includes: G2, Glassdoor, and Repvue reviews.
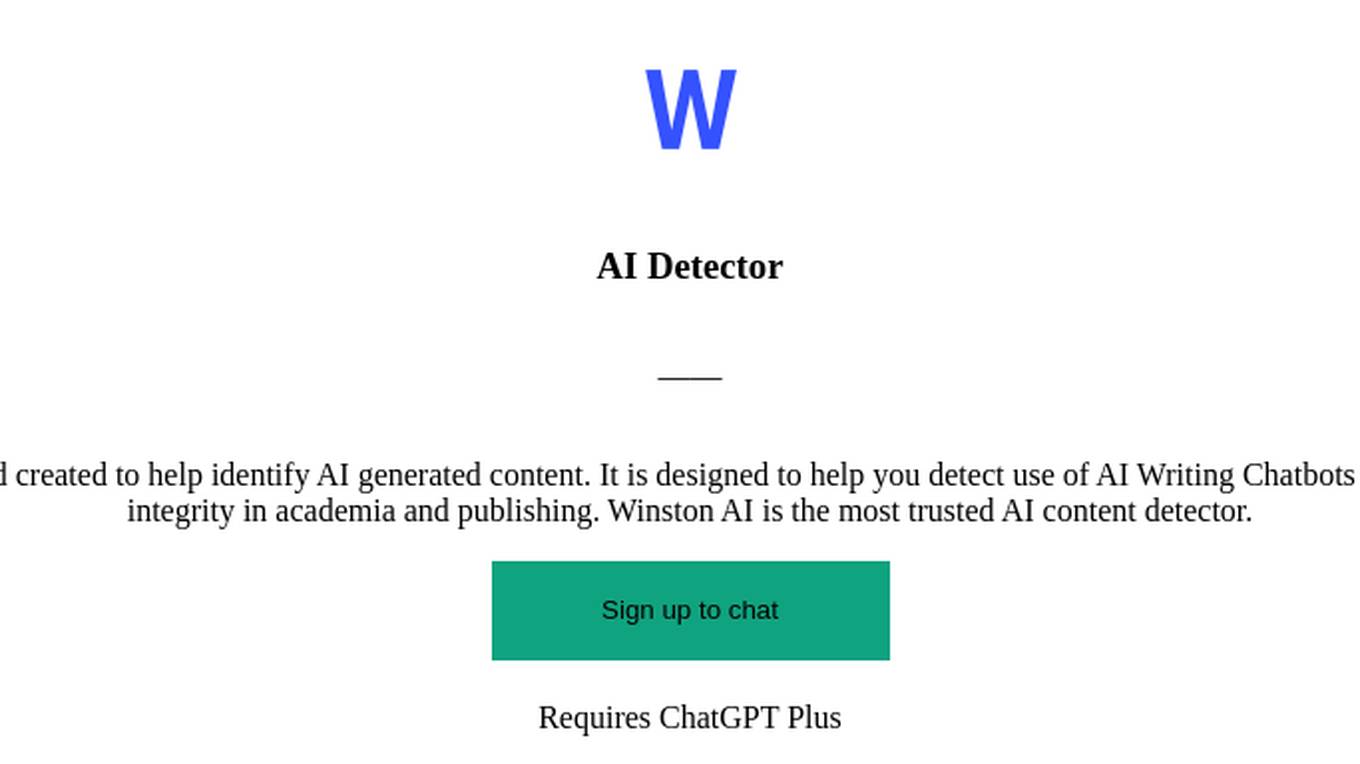
AI Detector
AI Detector GPT is powered by Winston AI and created to help identify AI generated content. It is designed to help you detect use of AI Writing Chatbots such as ChatGPT, Claude and Bard and maintain integrity in academia and publishing. Winston AI is the most trusted AI content detector.
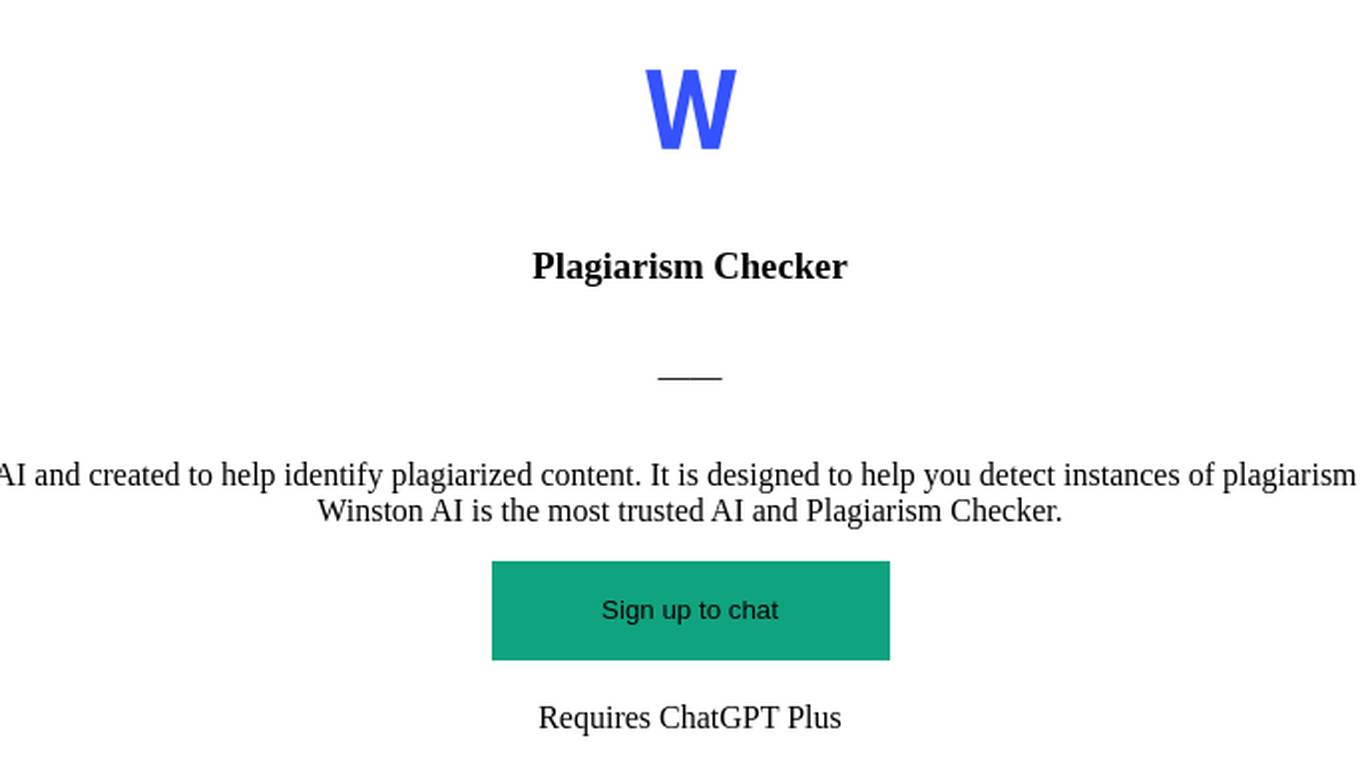
Plagiarism Checker
Plagiarism Checker GPT is powered by Winston AI and created to help identify plagiarized content. It is designed to help you detect instances of plagiarism and maintain integrity in academia and publishing. Winston AI is the most trusted AI and Plagiarism Checker.
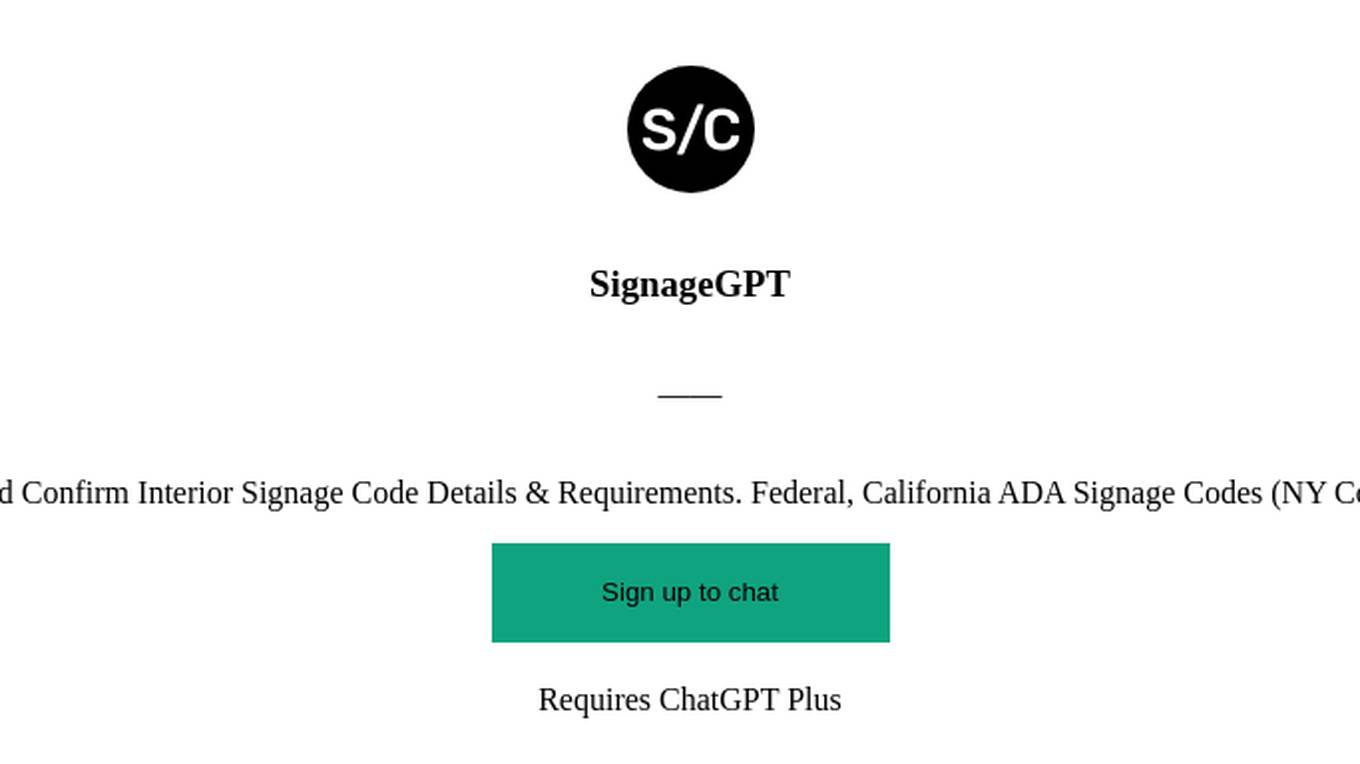
SignageGPT
Identify and Confirm Interior Signage Code Details & Requirements. Federal, California ADA Signage Codes (NY Coming Soon)
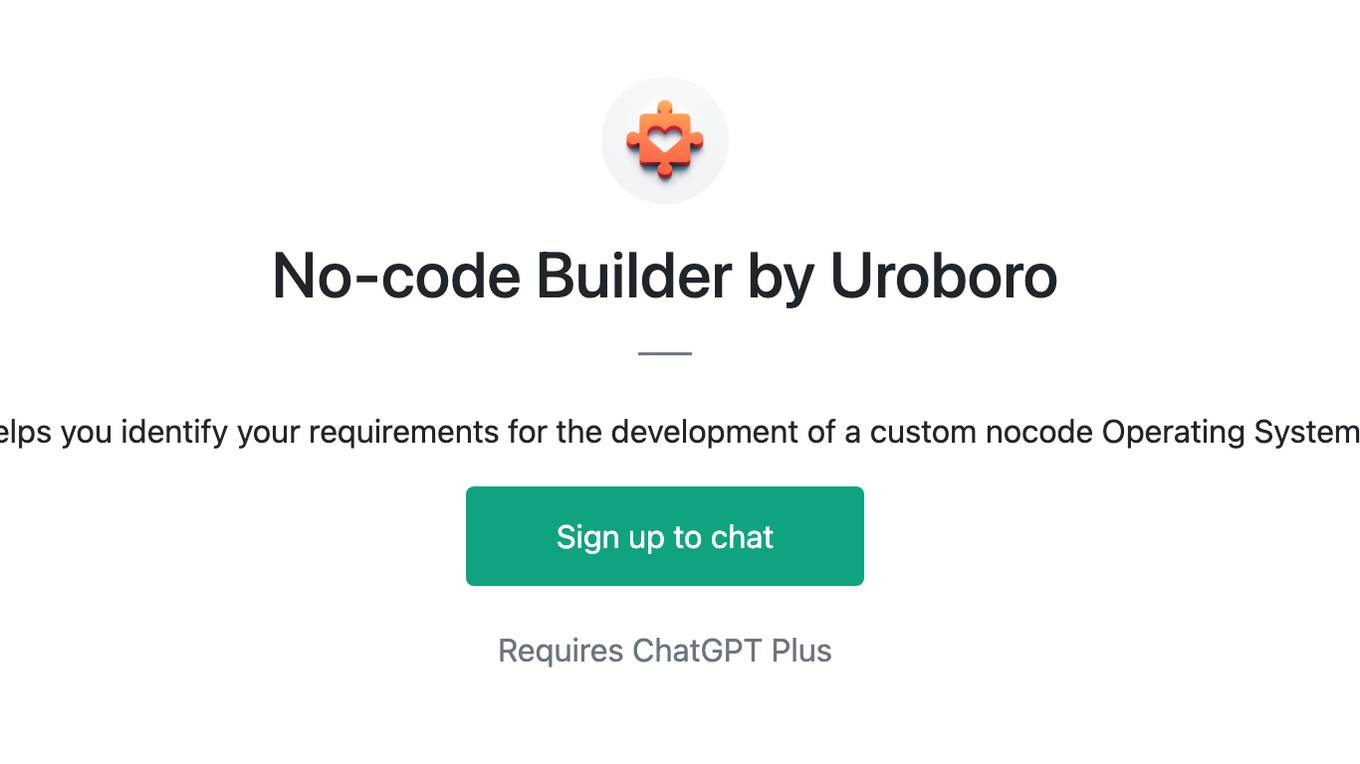
No-code Builder by Uroboro
Helps you identify your requirements for the development of a custom nocode Operating System