Best AI tools for< Check Code Correctness >
20 - AI tool Sites
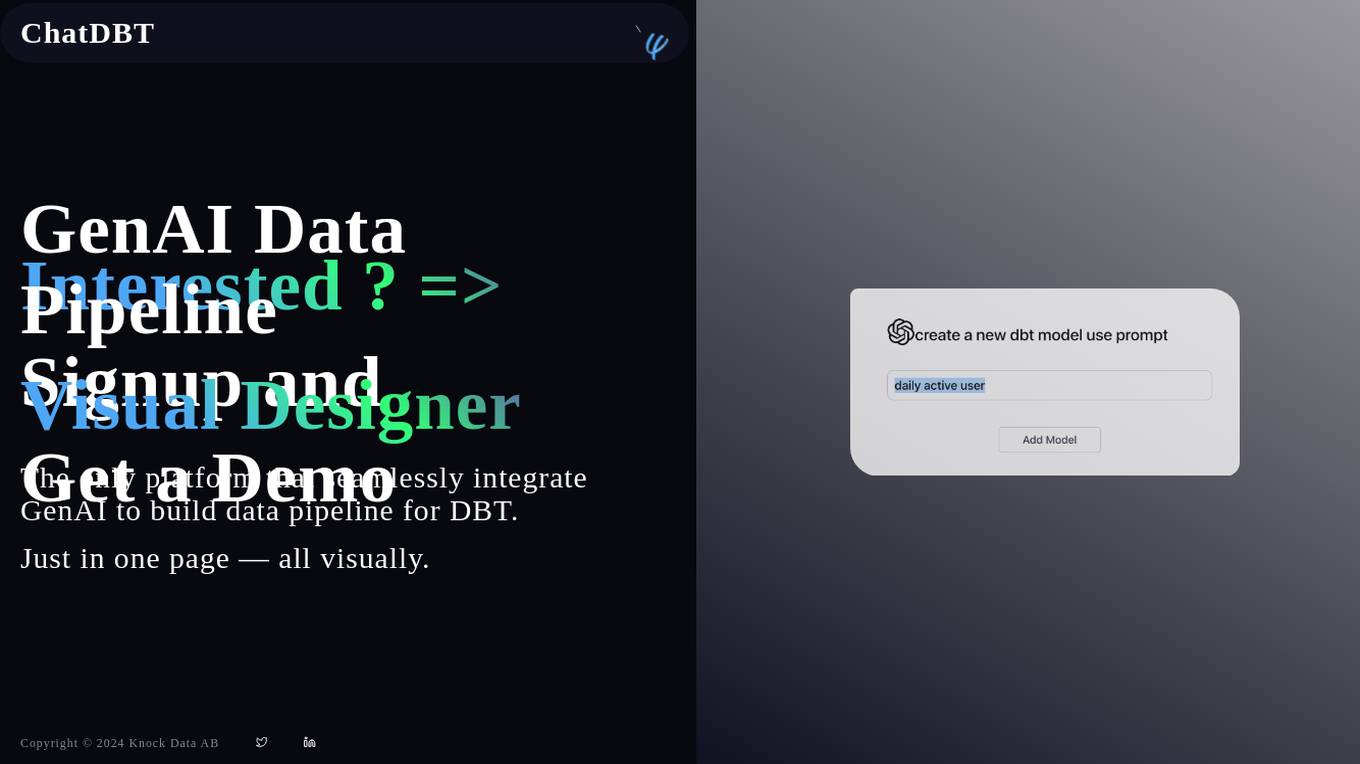
ChatDBT
ChatDBT is a DBT designer with prompting that helps you write better DBT code. It provides a user-friendly interface that makes it easy to create and edit DBT models, and it includes a number of features that can help you improve the quality of your code.
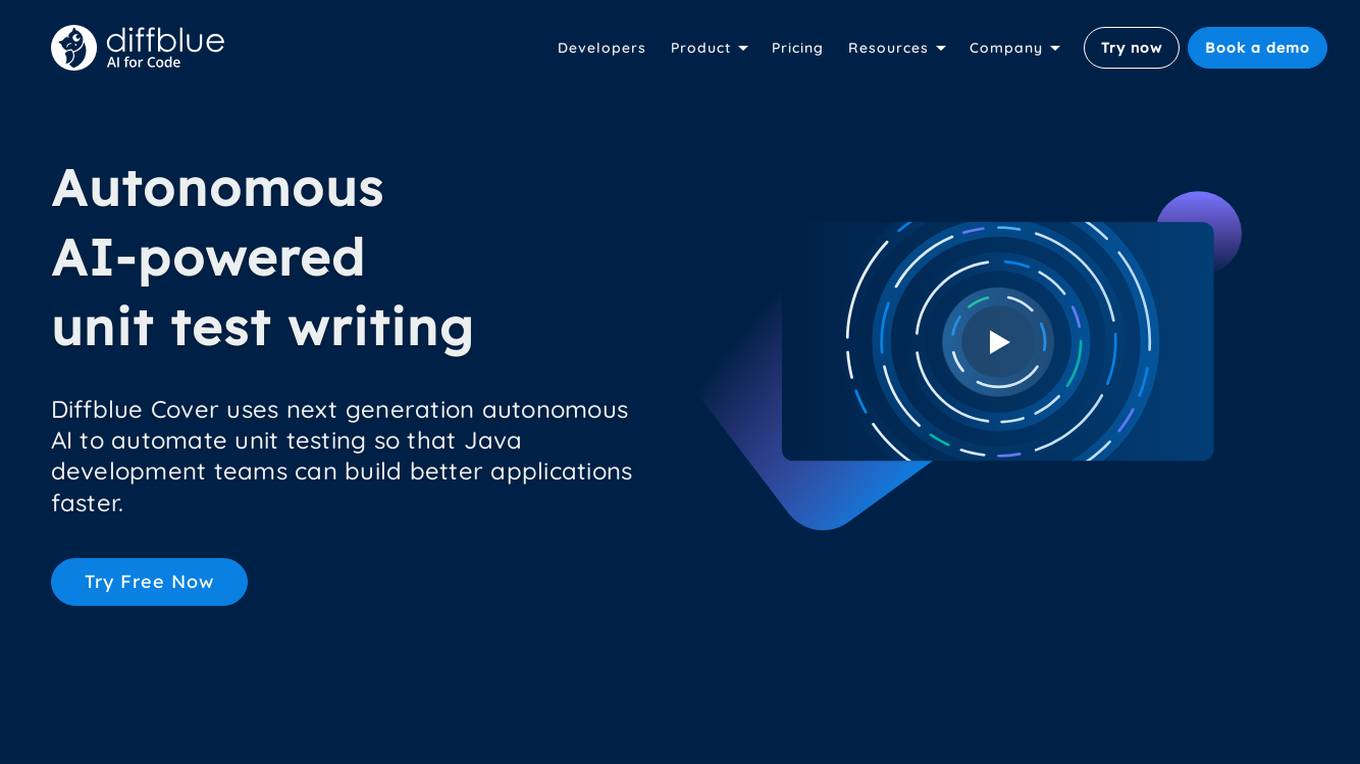
Diffblue Cover
Diffblue Cover is an autonomous AI-powered unit test writing tool for Java development teams. It uses next-generation autonomous AI to automate unit testing, freeing up developers to focus on more creative work. Diffblue Cover can write a complete and correct Java unit test every 2 seconds, and it is directly integrated into CI pipelines, unlike AI-powered code suggestions that require developers to check the code for bugs. Diffblue Cover is trusted by the world's leading organizations, including Goldman Sachs, and has been proven to improve quality, lower developer effort, help with code understanding, reduce risk, and increase deployment frequency.
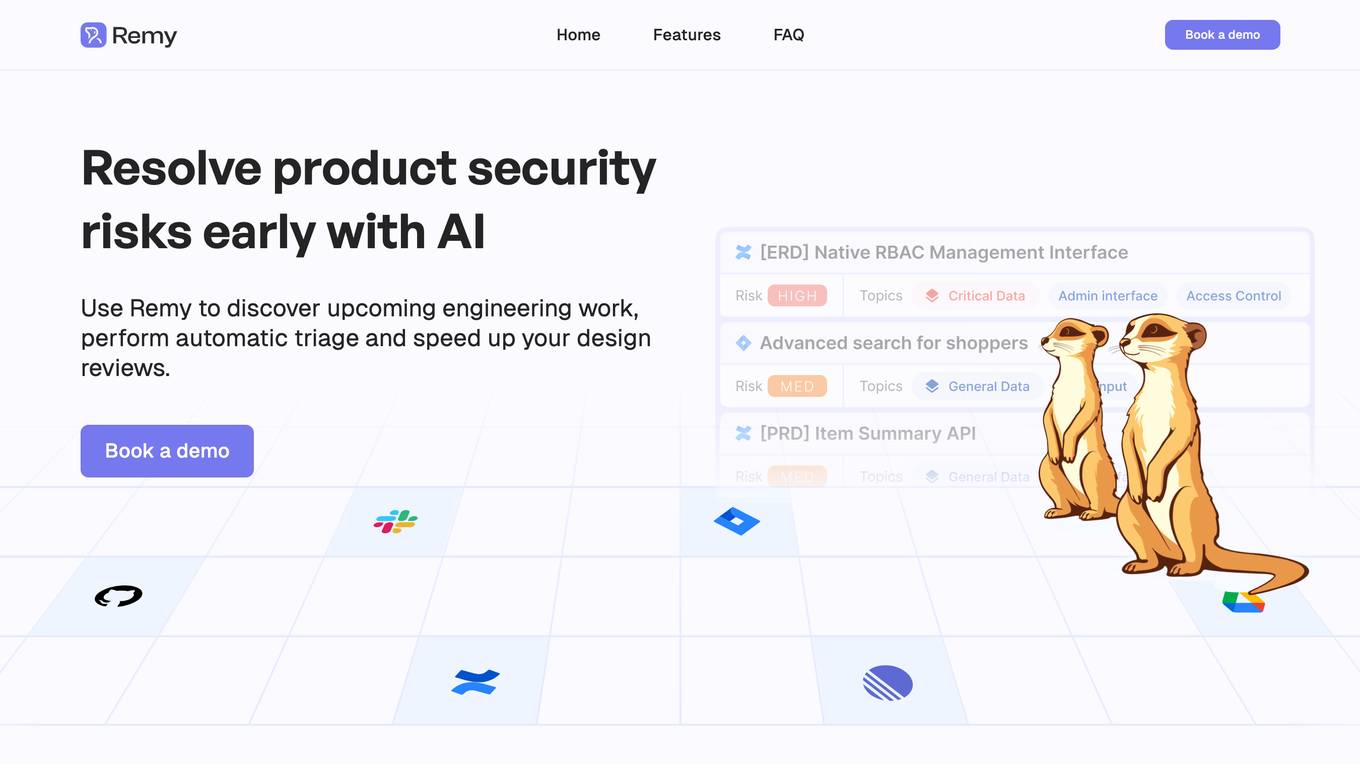
Error Code Analyzer
The website appears to be experiencing a 403 Forbidden error, which means the server is refusing to respond to the request. This could be due to various reasons such as insufficient permissions, server misconfiguration, or a client error. The error message '403 Forbidden' is a standard HTTP status code that indicates the server understood the request but refuses to authorize it. It is important to troubleshoot and resolve the issue to regain access to the website.
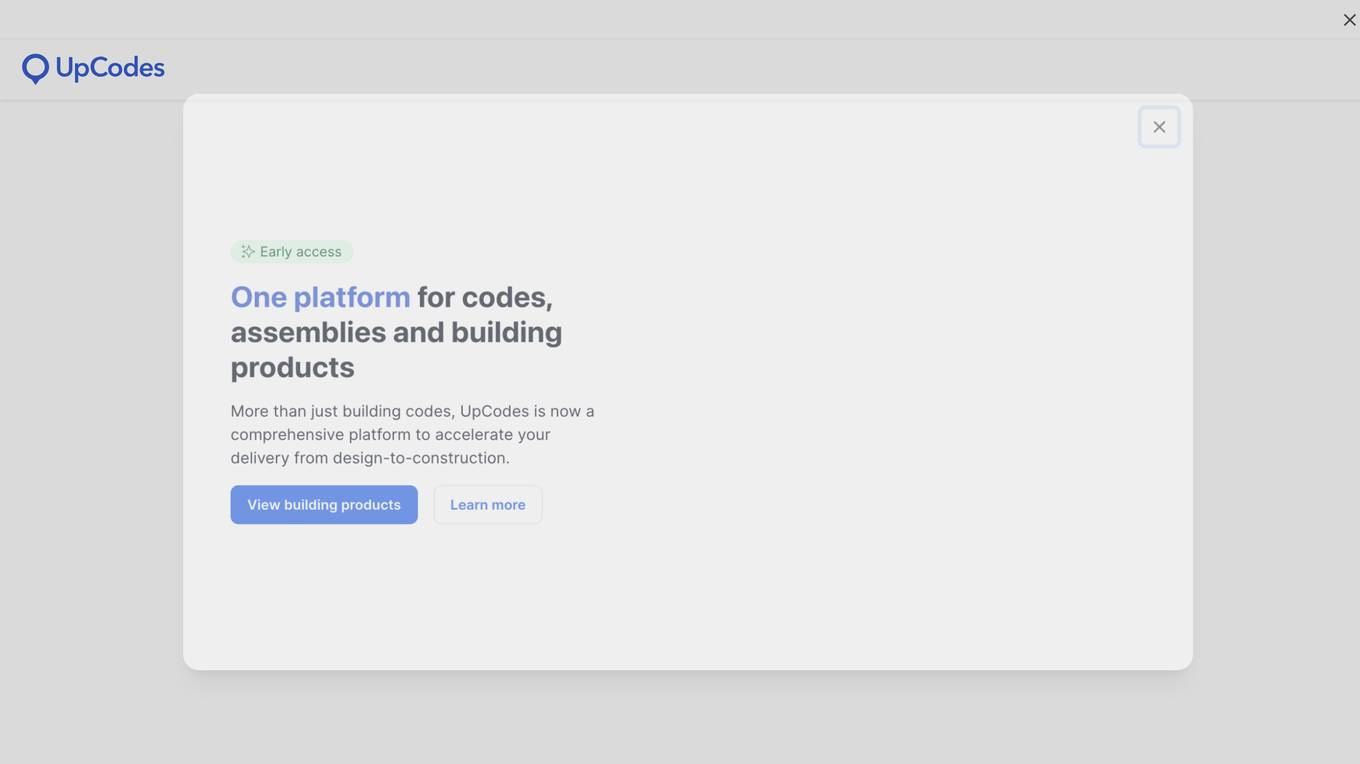
UpCodes
UpCodes is a searchable platform that provides access to building codes, assemblies, and building products libraries. It offers a comprehensive database of regulations and standards for construction projects, enabling users to easily search and reference relevant information. With UpCodes, architects, engineers, contractors, and other industry professionals can streamline their workflow, ensure compliance with codes, and enhance the quality of their designs. The platform is designed to simplify the process of accessing and interpreting building codes, saving time and reducing errors in construction projects.
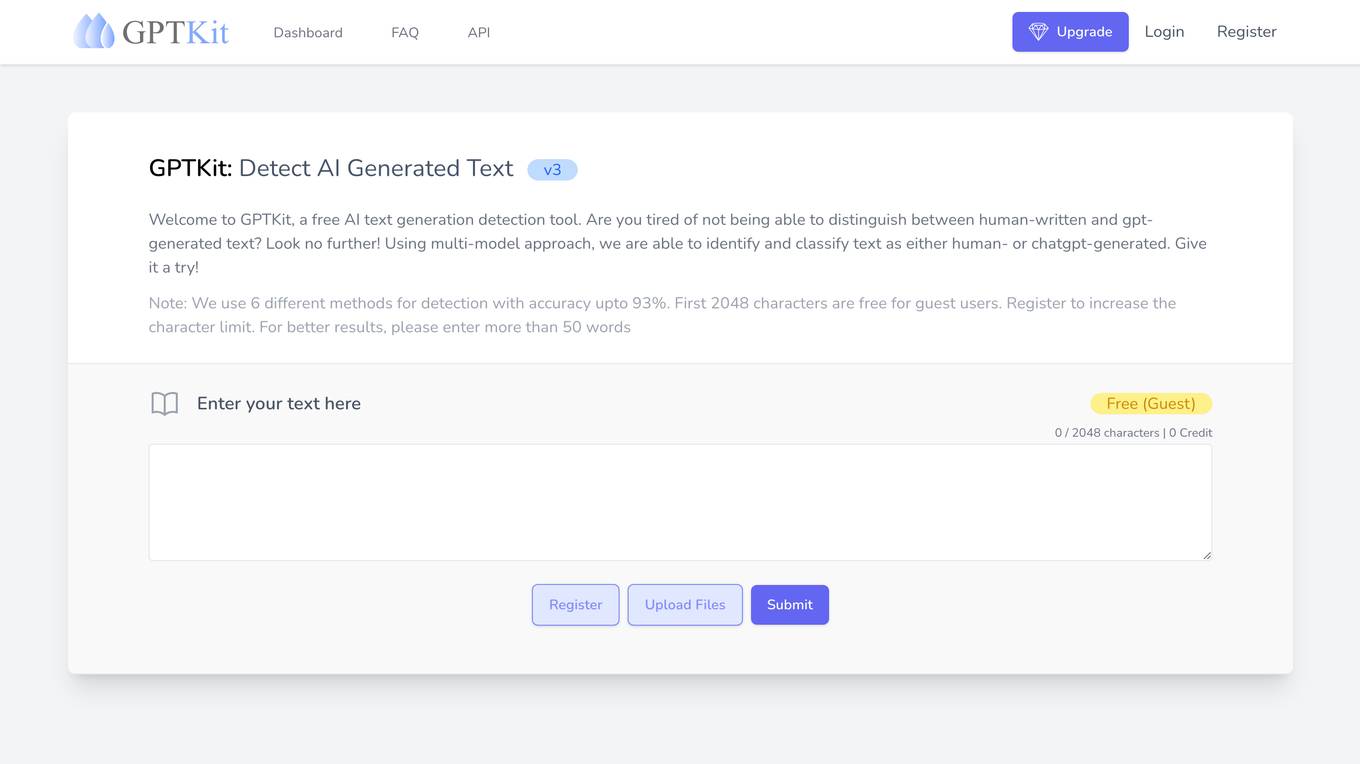
GPTKit
GPTKit is a free AI text generation detection tool that utilizes six different AI-based content detection techniques to identify and classify text as either human- or AI-generated. It provides reports on the authenticity and reality of the analyzed content, with an accuracy of approximately 93%. The first 2048 characters in every request are free, and users can register for free to get 2048 characters/request.
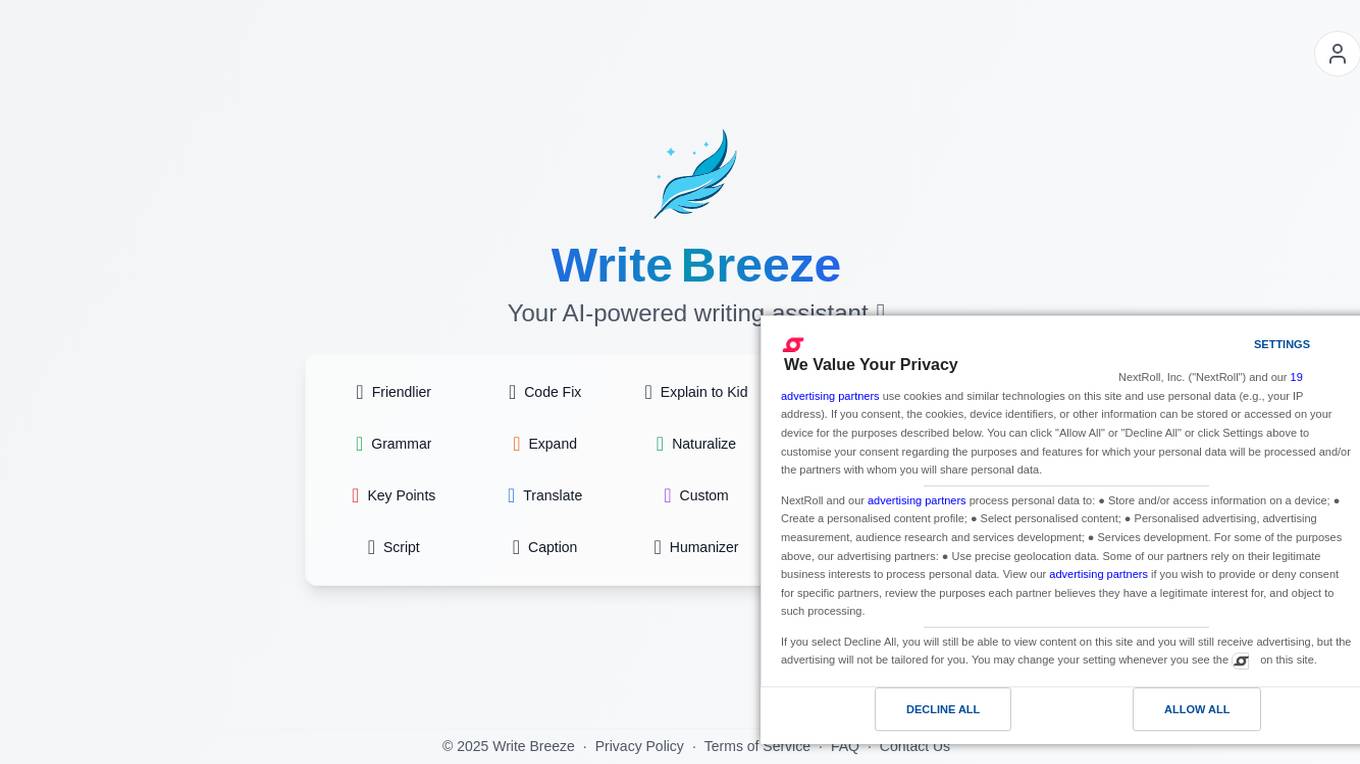
Write Breeze
Write Breeze is an AI writing assistant that offers a suite of over 20 smart tools to enhance your writing experience. From grammar and style suggestions to content optimization, Write Breeze helps users create polished and engaging content effortlessly. Whether you're a student, professional writer, or content creator, Write Breeze is designed to streamline your writing process and elevate the quality of your work.
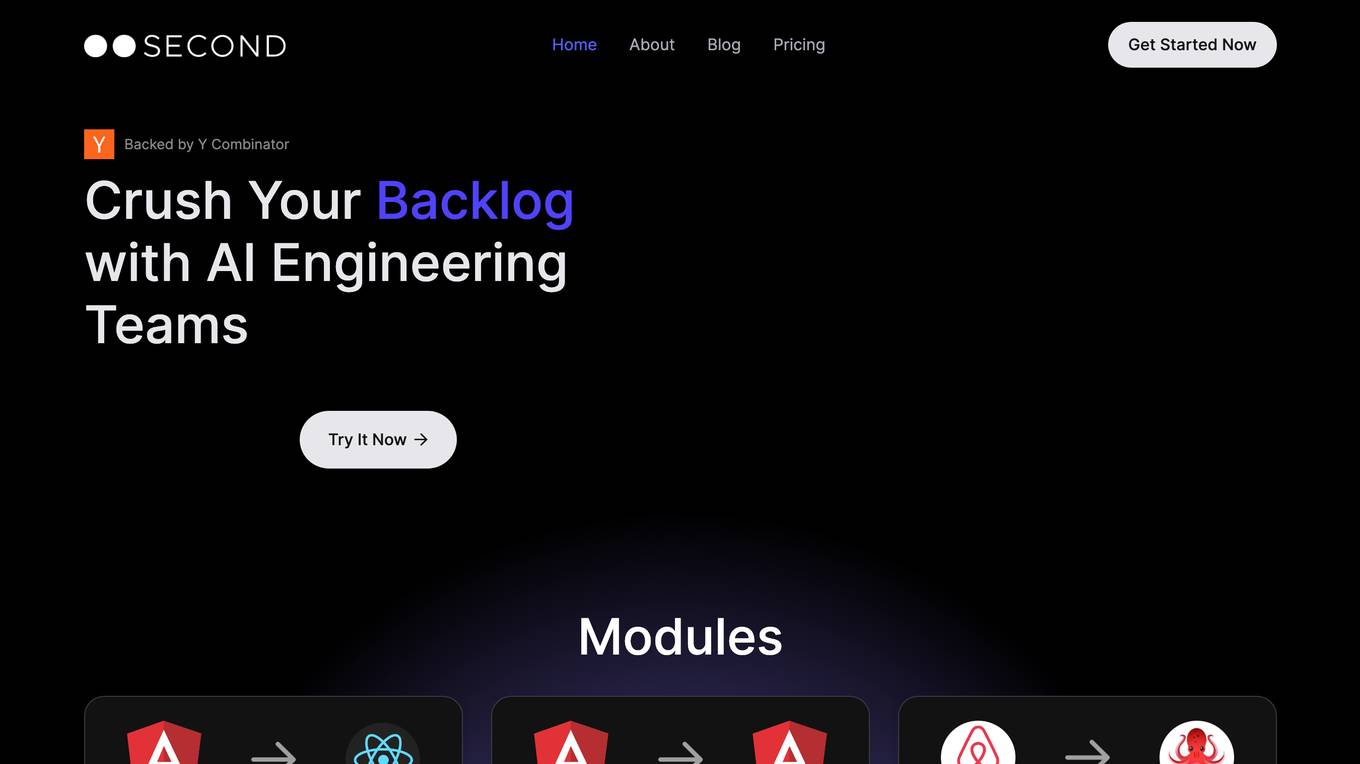
second.dev
second.dev is a domain that is currently parked for free, courtesy of GoDaddy.com. The website does not offer any specific services or products but rather serves as a placeholder for potential future use. It is important to note that any references to companies, products, or services on the site are not controlled by GoDaddy.com LLC and do not imply any association or endorsement by GoDaddy.
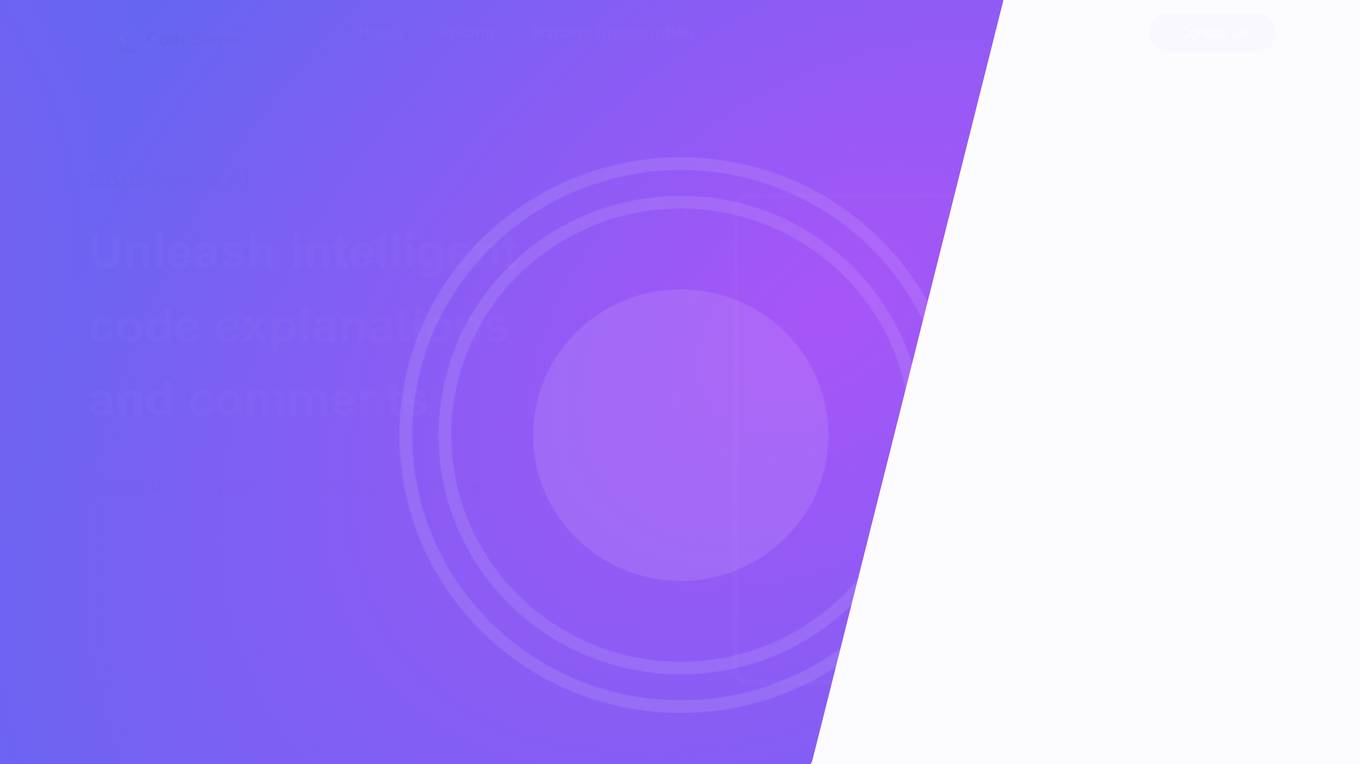
Site Not Found
The website page seems to be a placeholder or error page with the message 'Site Not Found'. It indicates that the user may not have deployed an app yet or may have an empty directory. The page suggests referring to hosting documentation to deploy the first app. The site appears to be under construction or experiencing technical issues.
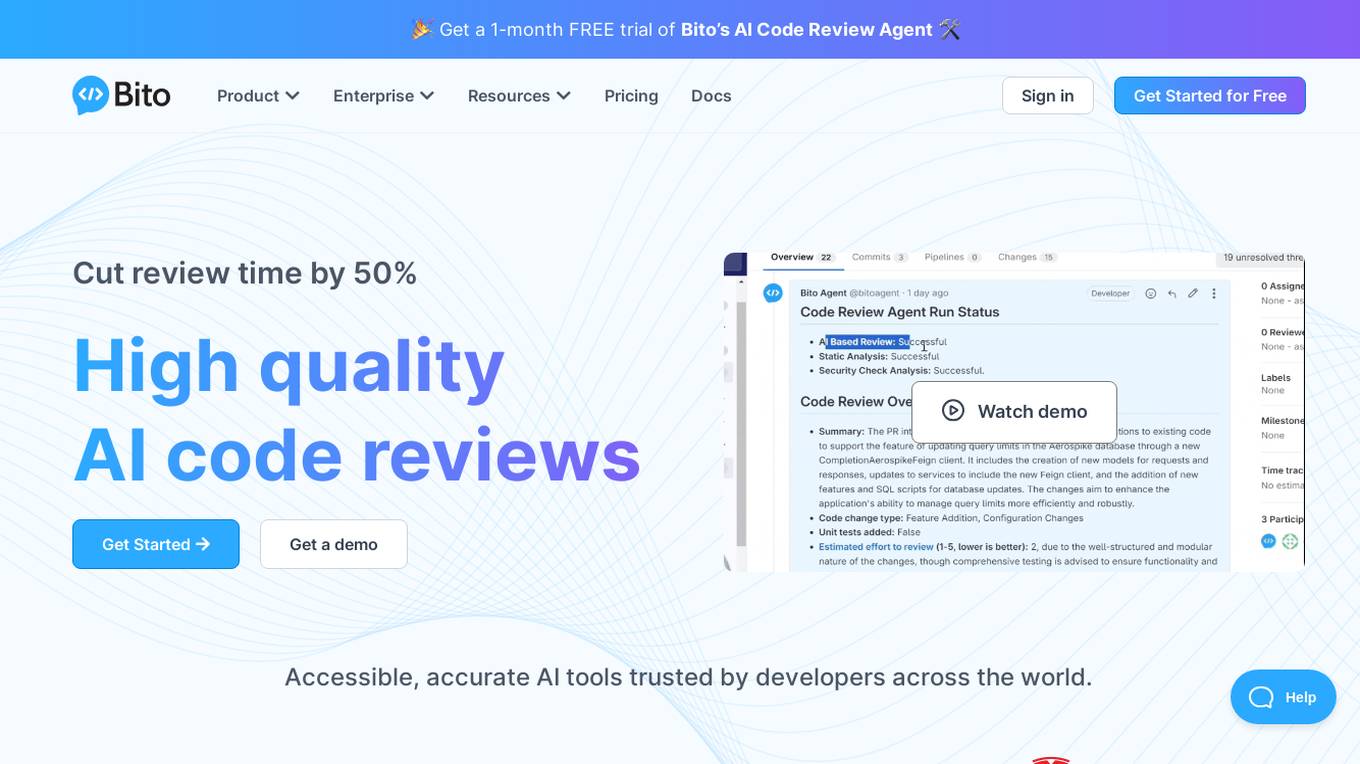
Bito AI
Bito AI is an AI-powered code review tool that helps developers write better code faster. It provides real-time feedback on code quality, security, and performance, and can also generate test cases and documentation. Bito AI is trusted by developers across the world, and has been shown to reduce review time by 50%.
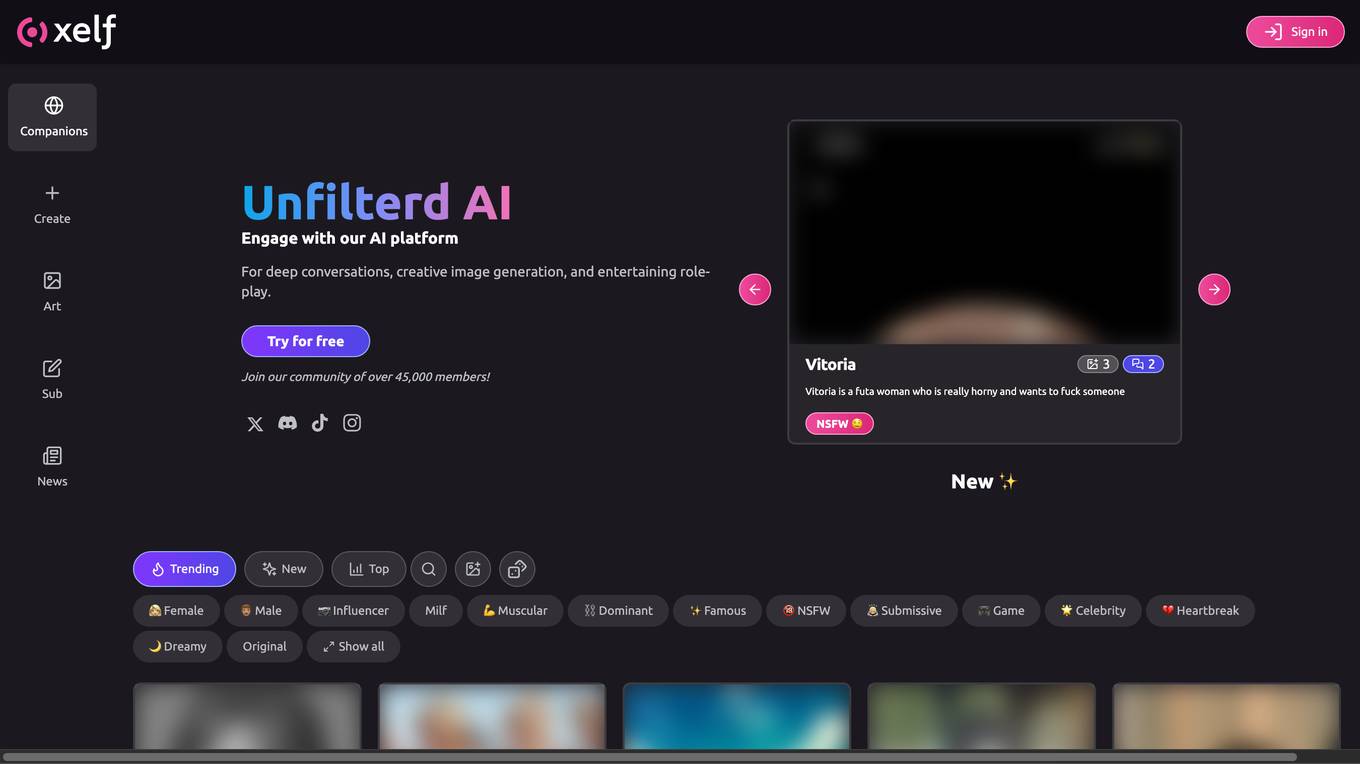
404 Error Page
The website displays a '404: NOT_FOUND' error message indicating that the deployment cannot be found. It provides a code (DEPLOYMENT_NOT_FOUND) and an ID (sin1::22md2-1720772812453-4893618e160a) for reference. Users are directed to check the documentation for further information and troubleshooting.
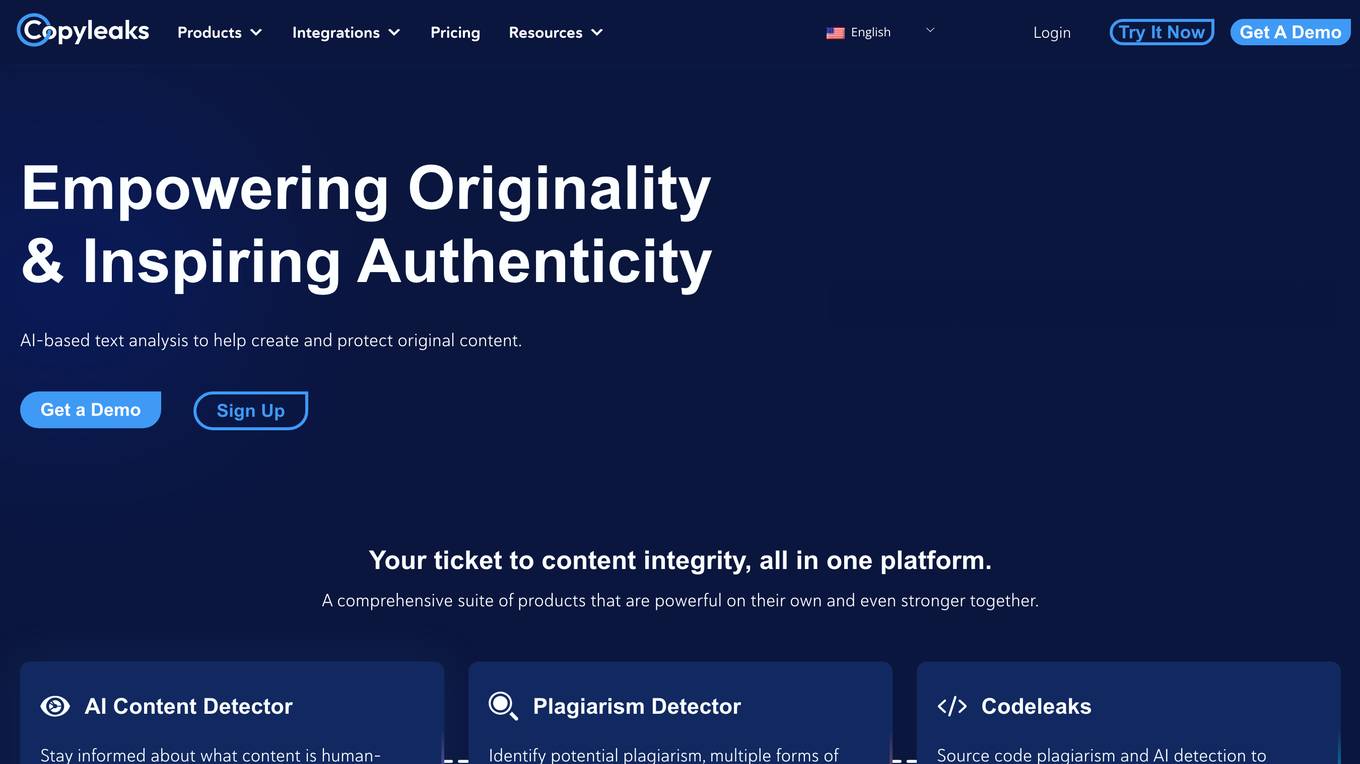
Copyleaks
Copyleaks is an AI-based plagiarism and AI content detection tool that helps users detect AI-generated code, plagiarized and modified source code, and provides essential licensing details. It offers solutions for academic integrity, governance and compliance, unauthorized large language model (LLM) usage, AI model training, and intellectual property protection. The tool includes products such as AI Detector, Plagiarism Checker, Writing Assistant, and API Integration, empowering users to ensure content integrity and transparency. Copyleaks also provides resources like news, AI testing methodologies, help center, success stories, and a blog to support users in protecting their content and adopting AI responsibly.
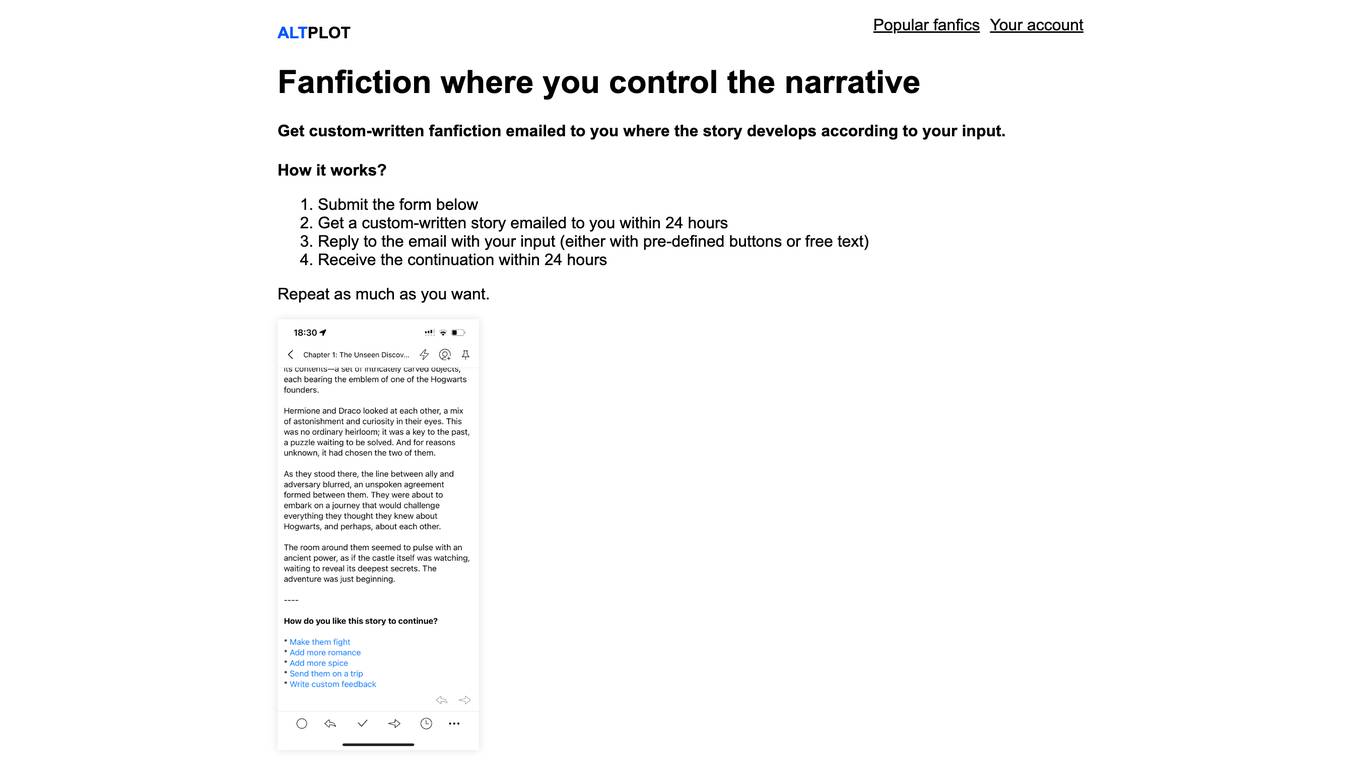
Altplot.com
Altplot.com is a website that currently appears to be experiencing technical difficulties, as indicated by the error code 521 displayed on the page. The error message suggests that the web server is down, resulting in the inability to establish a connection and display the web page content. Users encountering this issue are advised to wait a few minutes and try refreshing the page. Alternatively, website owners are instructed to contact their hosting provider to address the unresponsive web server. The error message also references Cloudflare, a service provider that offers performance and security solutions for websites.
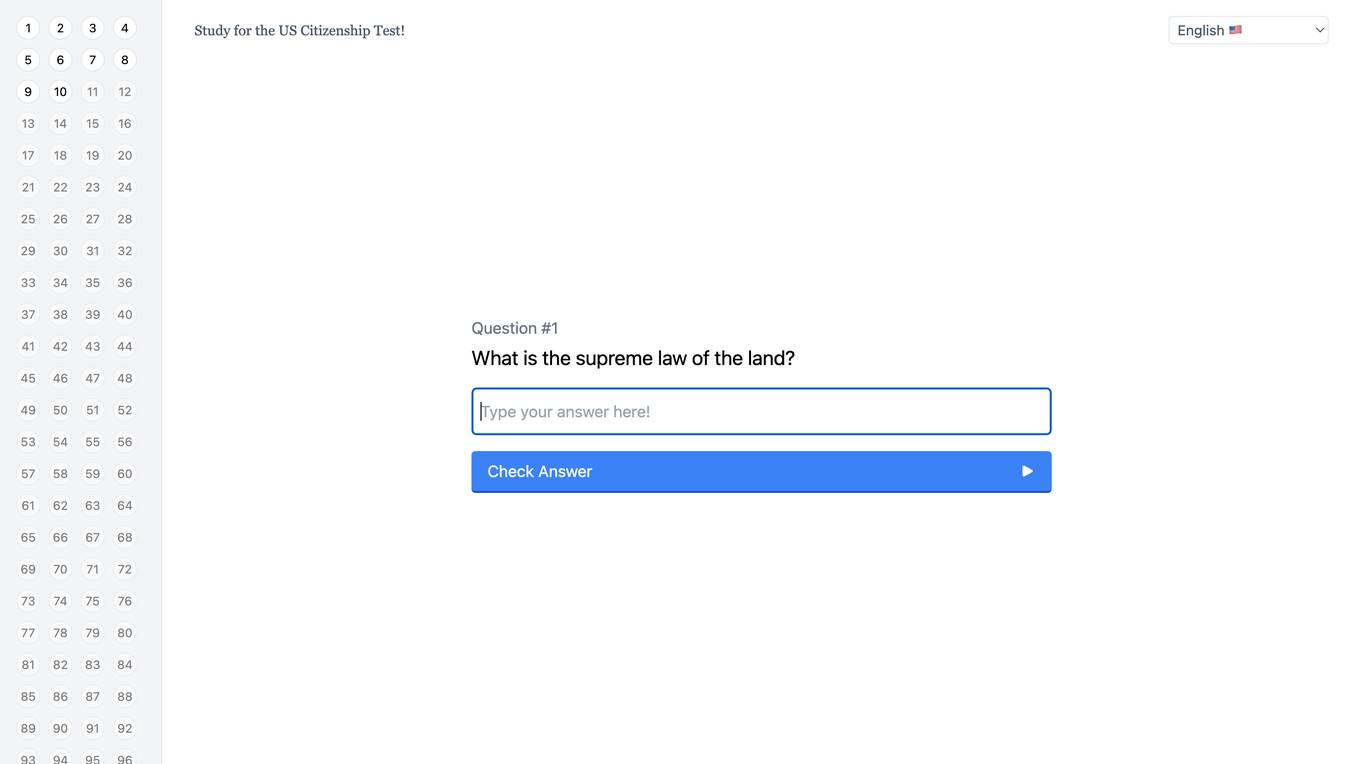
Client-side Exception Error Handler
The website appears to be encountering an application error, specifically a client-side exception. This error message indicates that there is an issue with the code running on the user's browser, prompting them to check the browser console for more detailed information. The website may be experiencing technical difficulties that need to be addressed by the developers to ensure proper functionality and user experience.
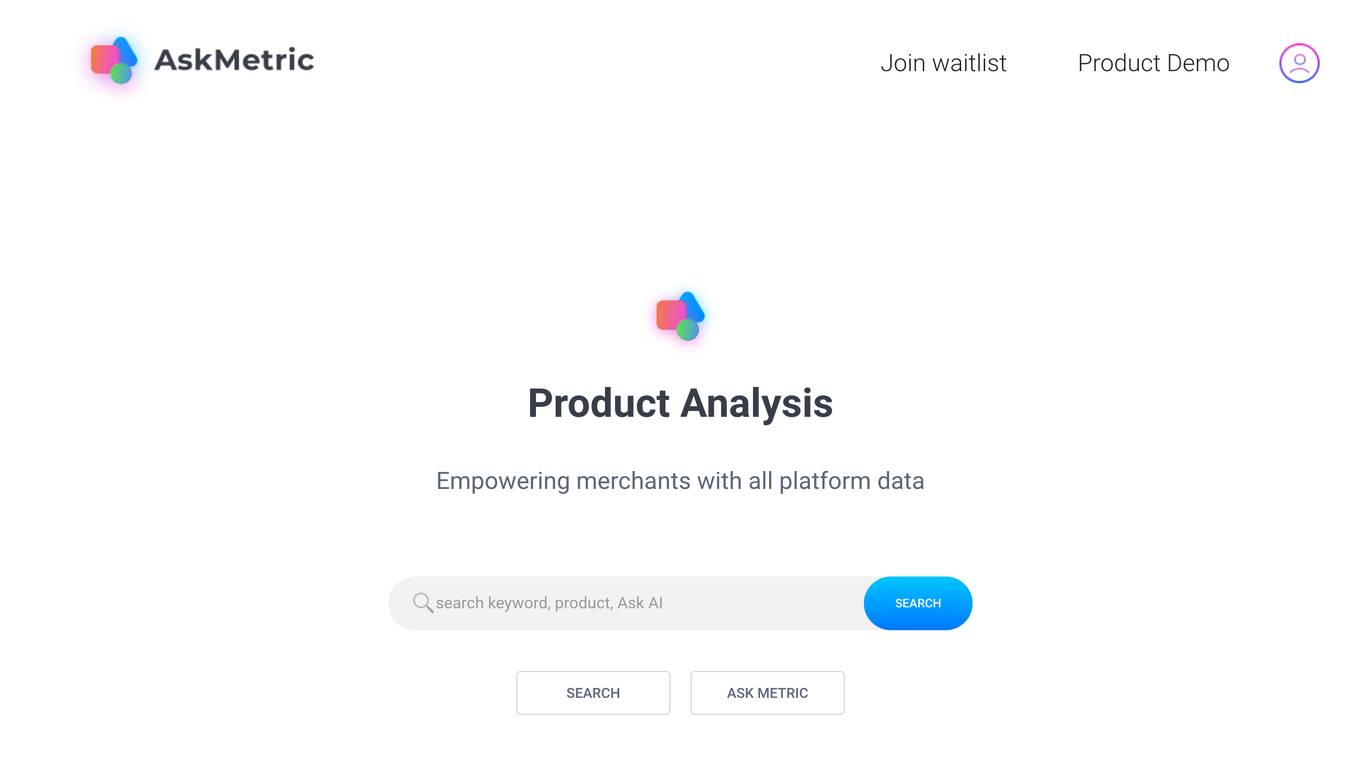
404 Error Not Found
The website displays a 404 error message indicating that the deployment cannot be found. It provides a code (DEPLOYMENT_NOT_FOUND) and an ID (sin1::k7xdt-1736614074909-2dc430118e75) for reference. Users are directed to check the documentation for further information and troubleshooting.
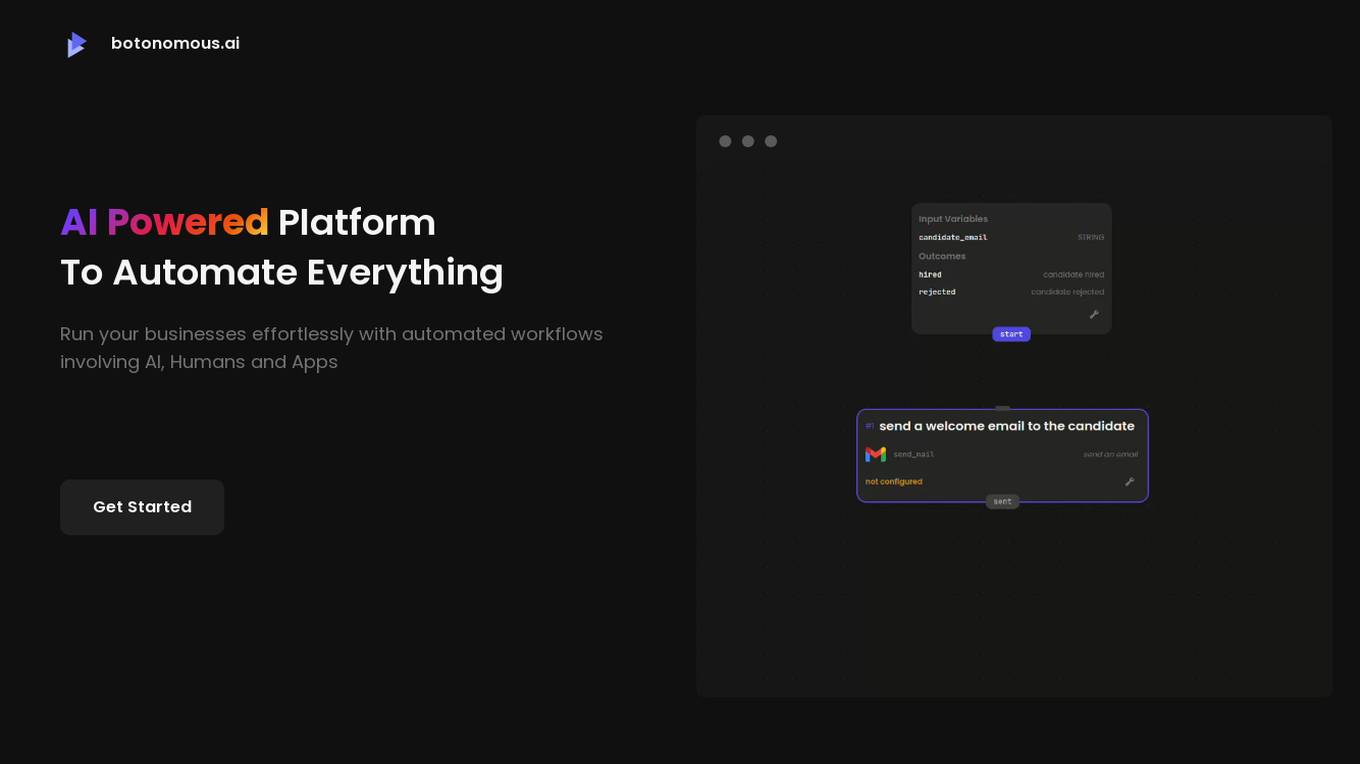
Botonomous
Botonomous is an AI-powered platform that helps businesses automate their workflows. With Botonomous, you can create advanced automations for any domain, check your flows for potential errors before running them, run multiple nodes concurrently without waiting for the completion of the previous step, create complex, non-linear flows with no-code, and design human interactions to participate in your automations. Botonomous also offers a variety of other features, such as webhooks, scheduled triggers, secure secret management, and a developer community.
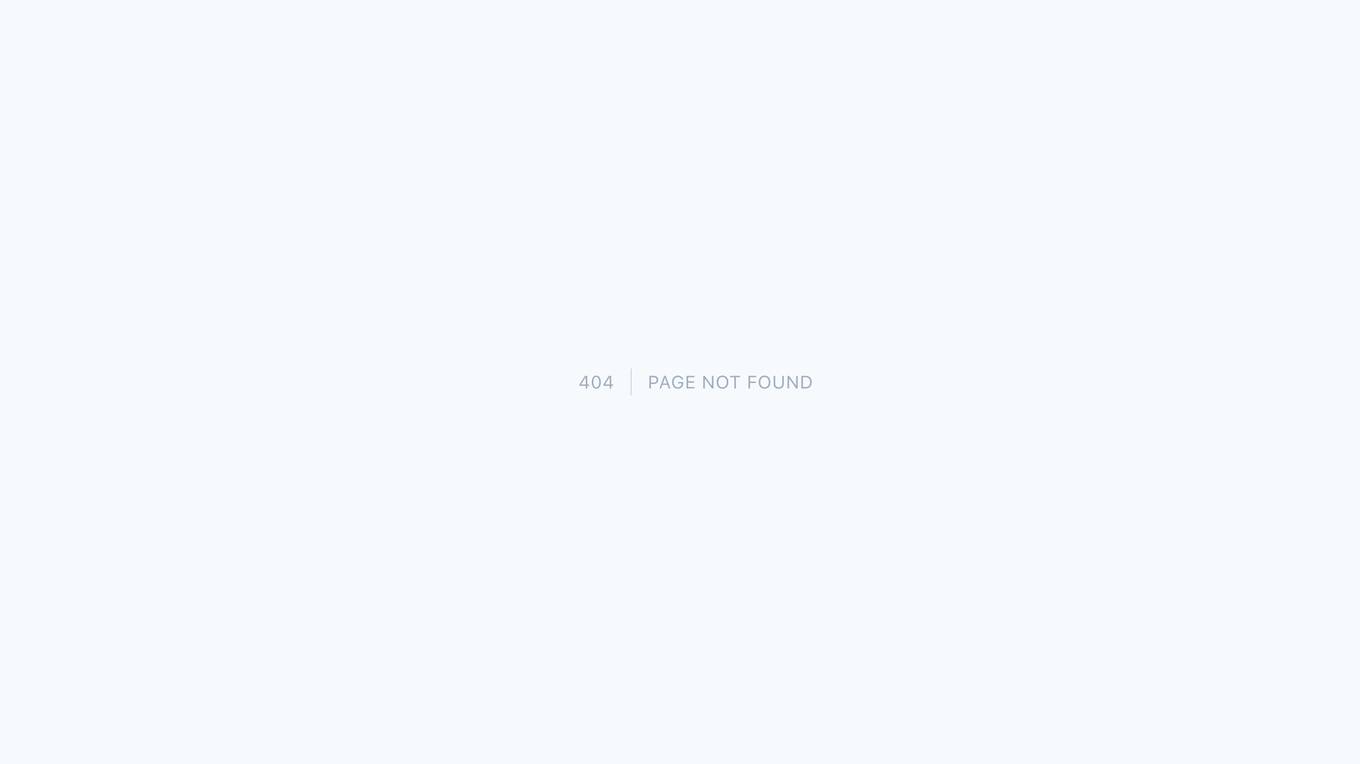
404 Page Not Found
The website is a simple web page that displays a '404 Page not found' error message. It seems that the page you are trying to access does not exist on the server. This error message is a standard response code in HTTP indicating that the client was able to communicate with the server, but the server could not find what was requested. The website does not offer any specific functionality or content beyond displaying this error message.
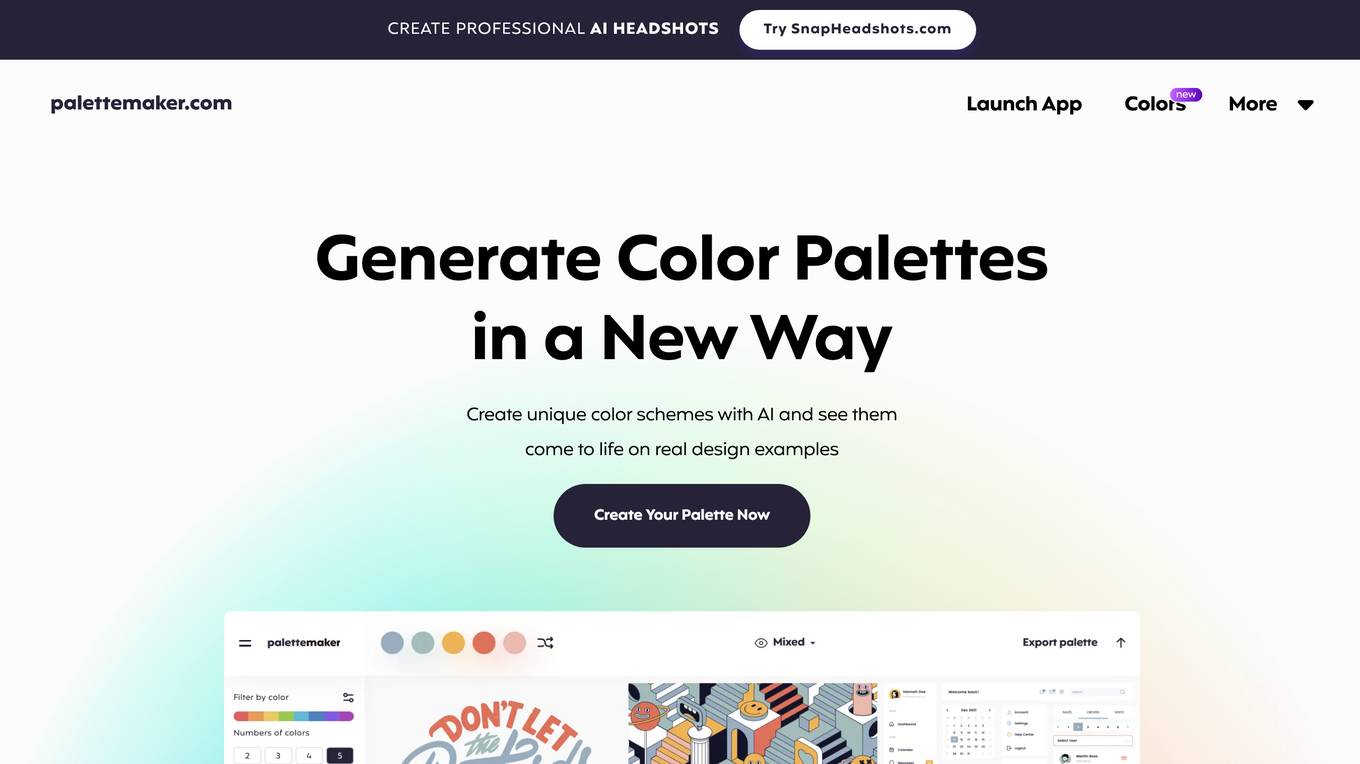
PaletteMaker
PaletteMaker is a unique tool for creative professionals and color lovers that allows you to create color palettes and test their behavior in pre-made design examples from the most common creative fields such as Logo design, UI/UX, Patterns, Posters and more. Check Color Behavior See how color works together in various of situations in graphic design. AI Color Palettes Filter palettes of different color tone and number of colors. Diverse Creative Fields Check your colors on logo, ui design, posters, illustrations and more. Create Palettes On-The-Go Instantly see the magic of creating color palettes. Totally Free PaletteMaker is created by professional designers, it’s completely free to use and forever will be. Powerful Export Export your palette in various formats, such as Procreate, Adobe ASE, Image, and even Code.
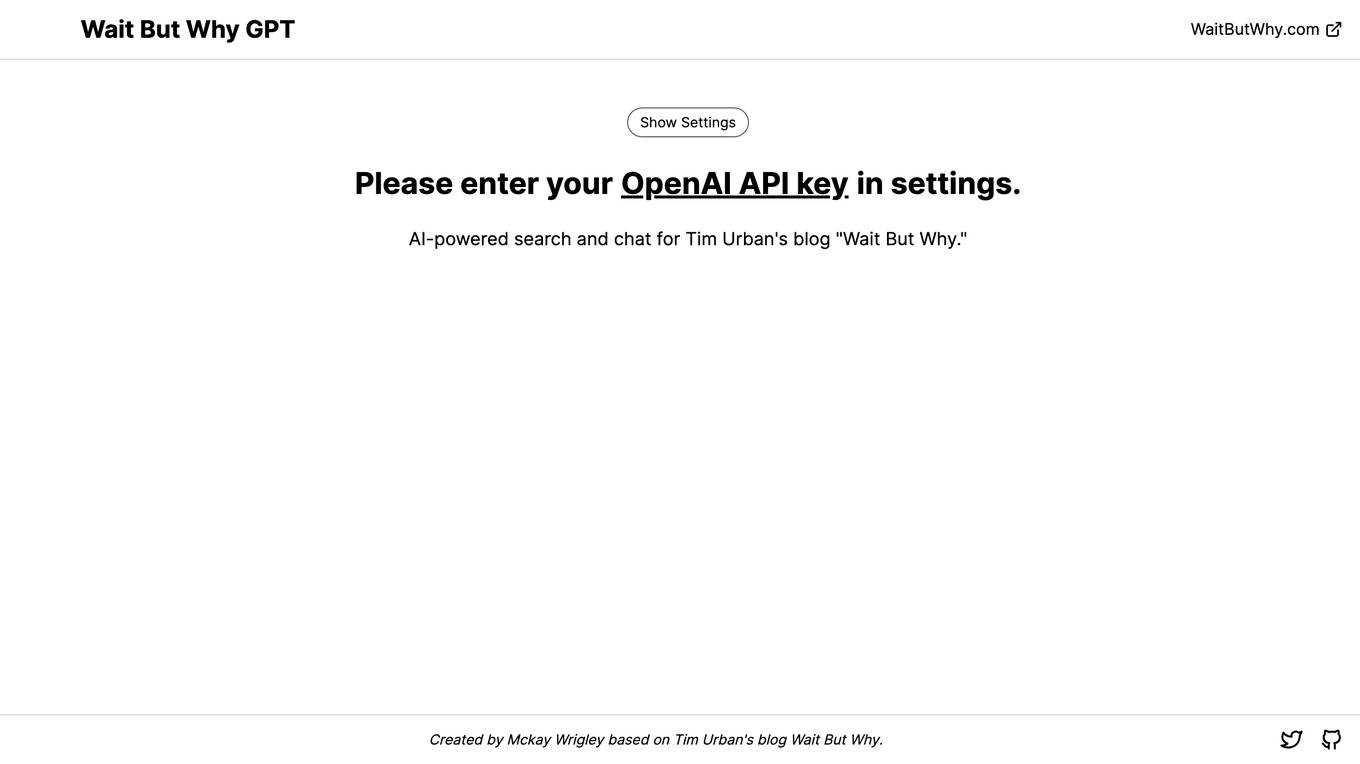
404 Error Page
The website displays a 404 error message indicating that the deployment cannot be found. It provides a code and an ID for reference, along with a suggestion to check the documentation for more information and troubleshooting.
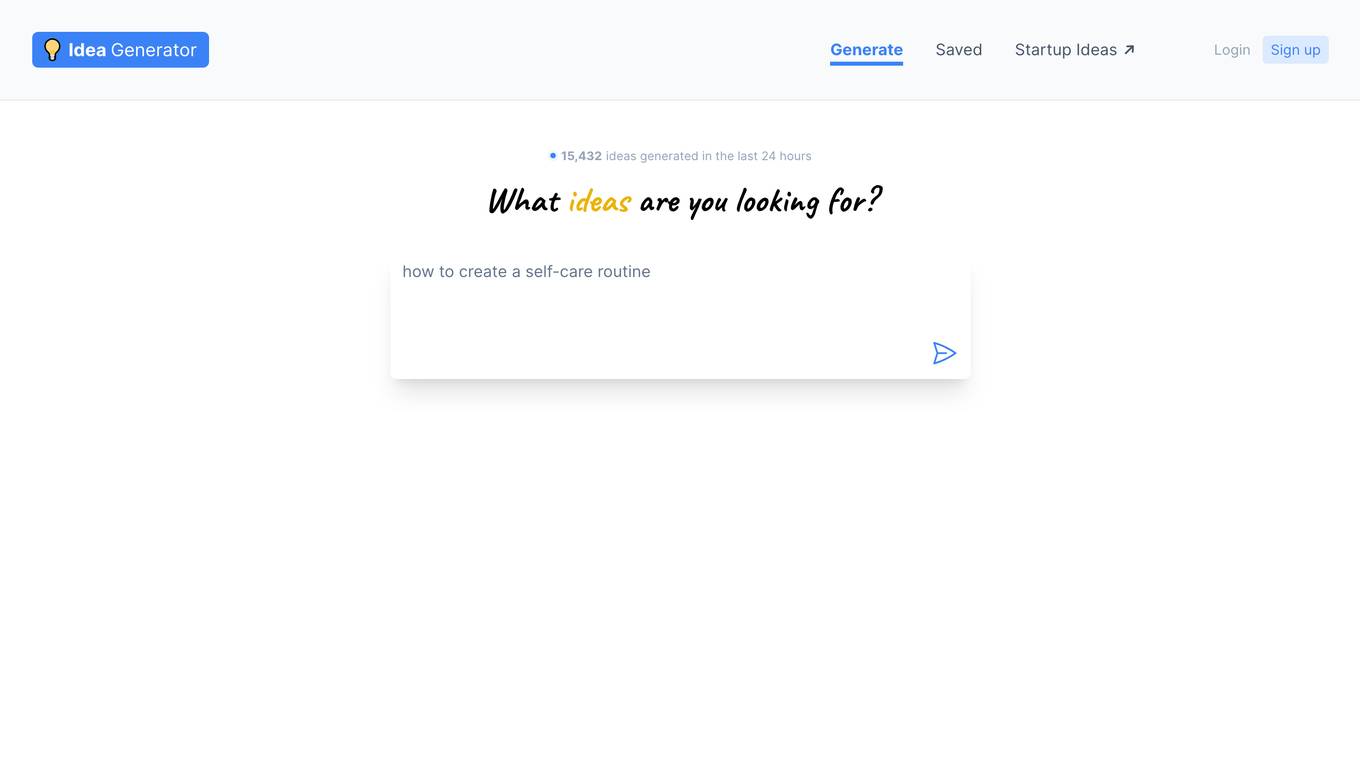
404 Error Notifier
The website displays a 404 error message indicating that the deployment cannot be found. It provides a code (DEPLOYMENT_NOT_FOUND) and an ID (sin1::spxmx-1740329113552-88538b58eccb) for reference. Users are directed to check the documentation for further information and troubleshooting.
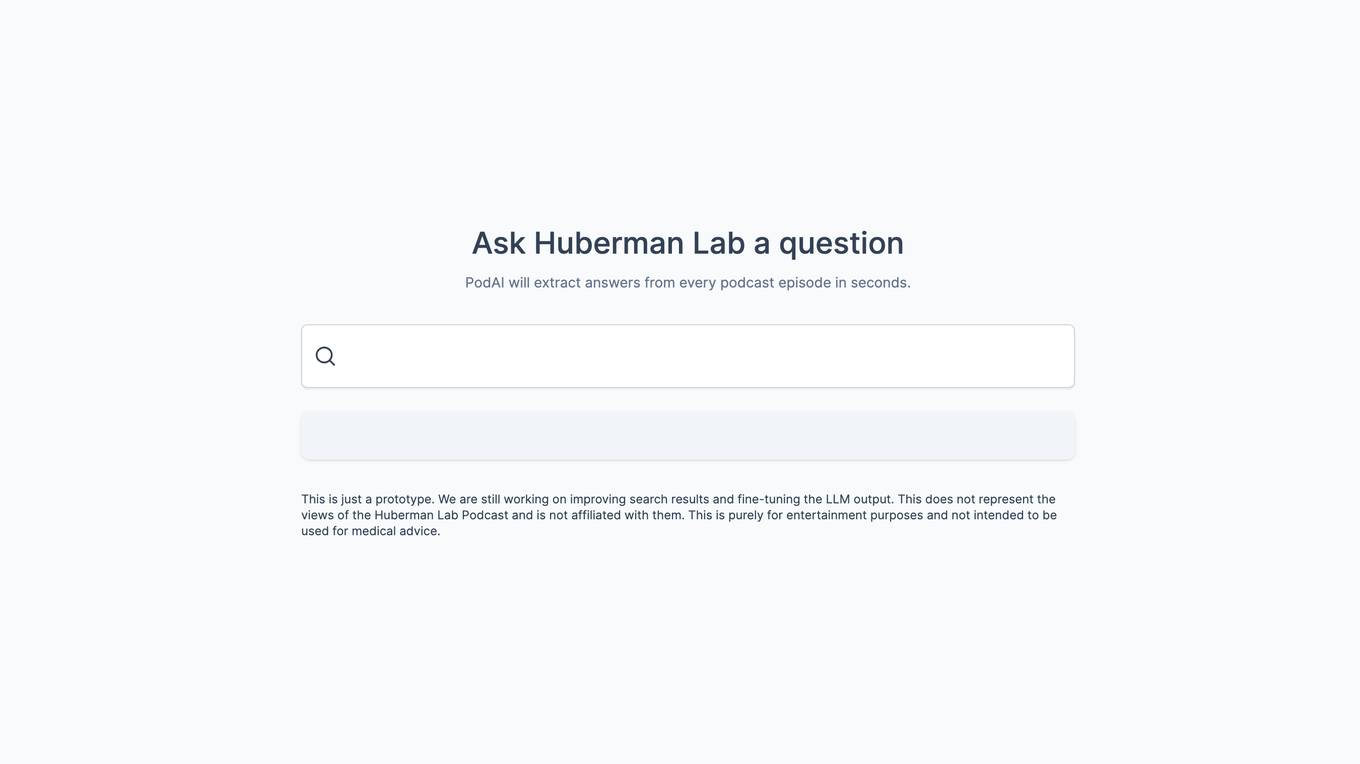
ErrorResolver
The website displays a 404 error message indicating that the deployment cannot be found. It provides a code (DEPLOYMENT_NOT_FOUND) and an ID (sin1::q5x5p-1740415919309-d80de0d14a35) for reference. Users are directed to check the documentation for further information and troubleshooting.
20 - Open Source AI Tools
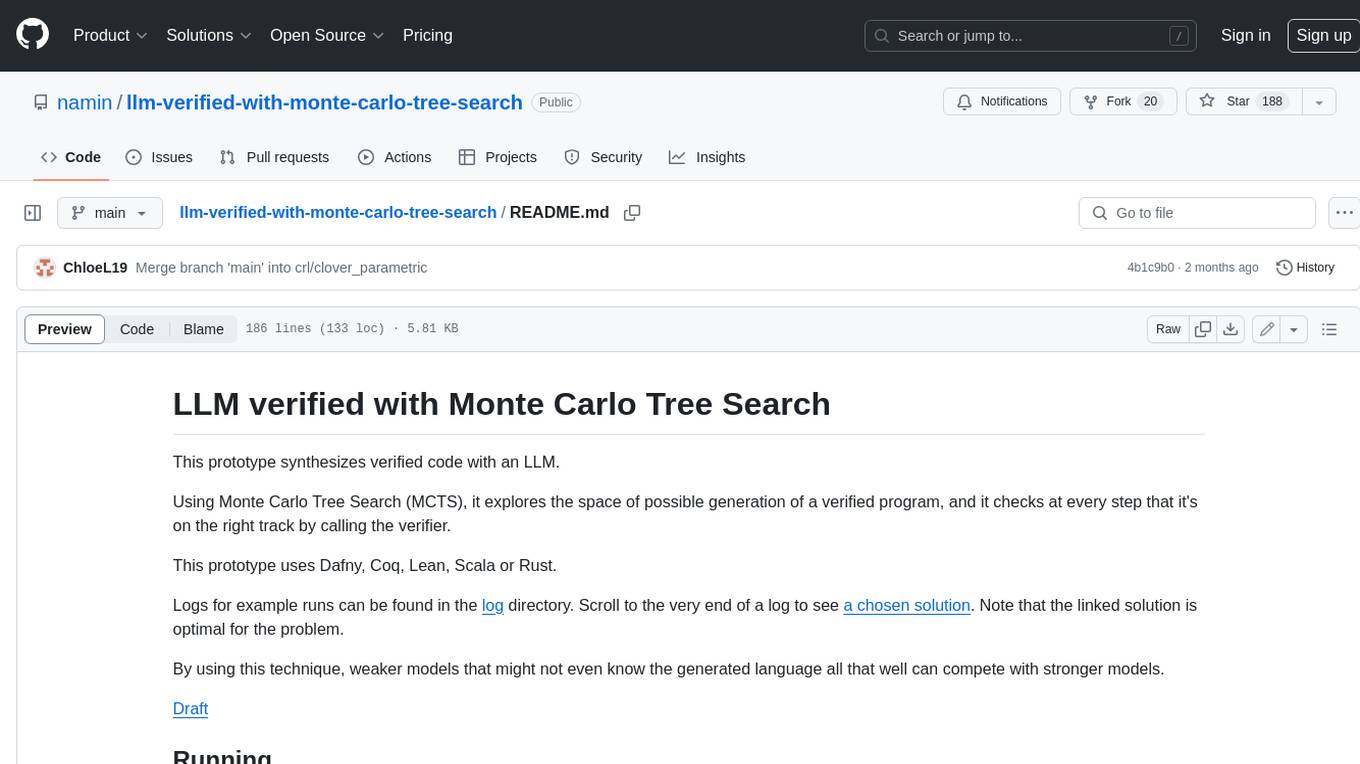
llm-verified-with-monte-carlo-tree-search
This prototype synthesizes verified code with an LLM using Monte Carlo Tree Search (MCTS). It explores the space of possible generation of a verified program and checks at every step that it's on the right track by calling the verifier. This prototype uses Dafny, Coq, Lean, Scala, or Rust. By using this technique, weaker models that might not even know the generated language all that well can compete with stronger models.
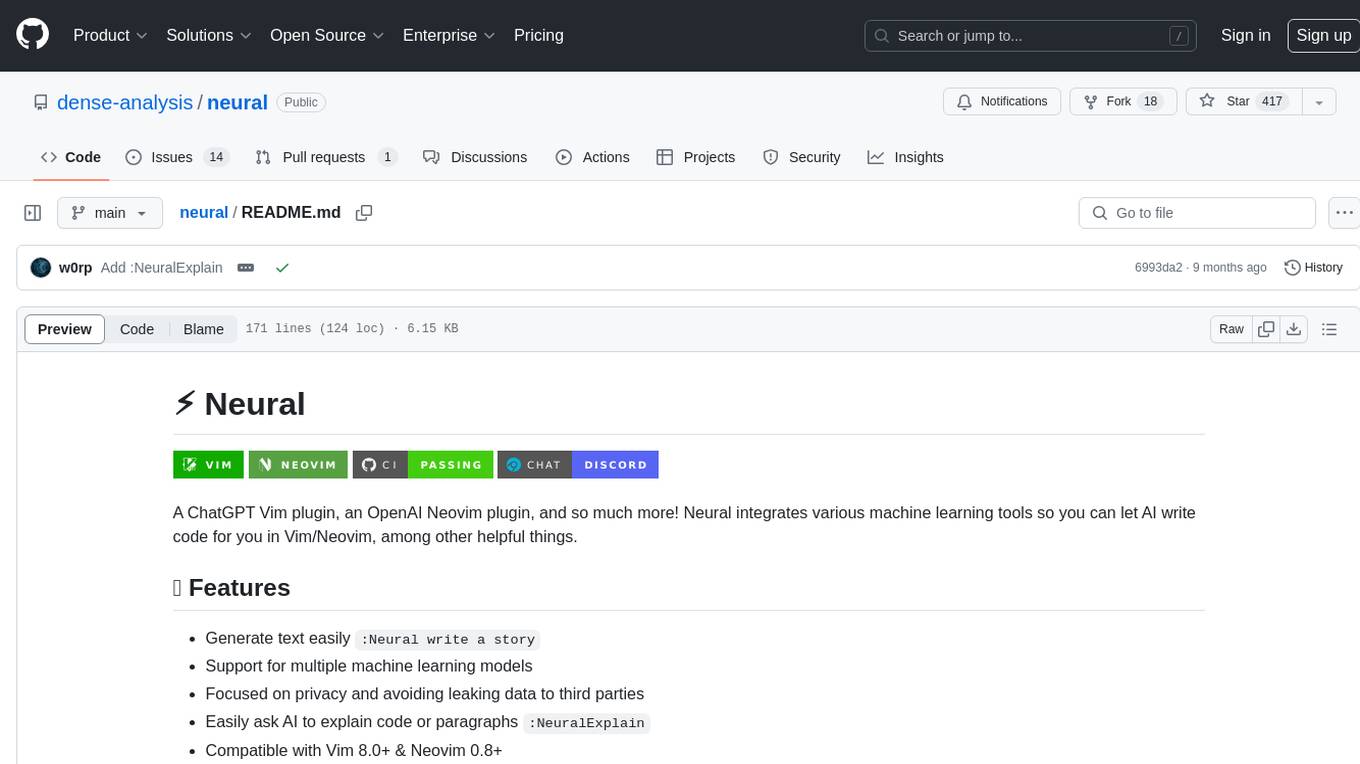
neural
Neural is a Vim and Neovim plugin that integrates various machine learning tools to assist users in writing code, generating text, and explaining code or paragraphs. It supports multiple machine learning models, focuses on privacy, and is compatible with Vim 8.0+ and Neovim 0.8+. Users can easily configure Neural to interact with third-party machine learning tools, such as OpenAI, to enhance code generation and completion. The plugin also provides commands like `:NeuralExplain` to explain code or text and `:NeuralStop` to stop Neural from working. Neural is maintained by the Dense Analysis team and comes with a disclaimer about sending input data to third-party servers for machine learning queries.
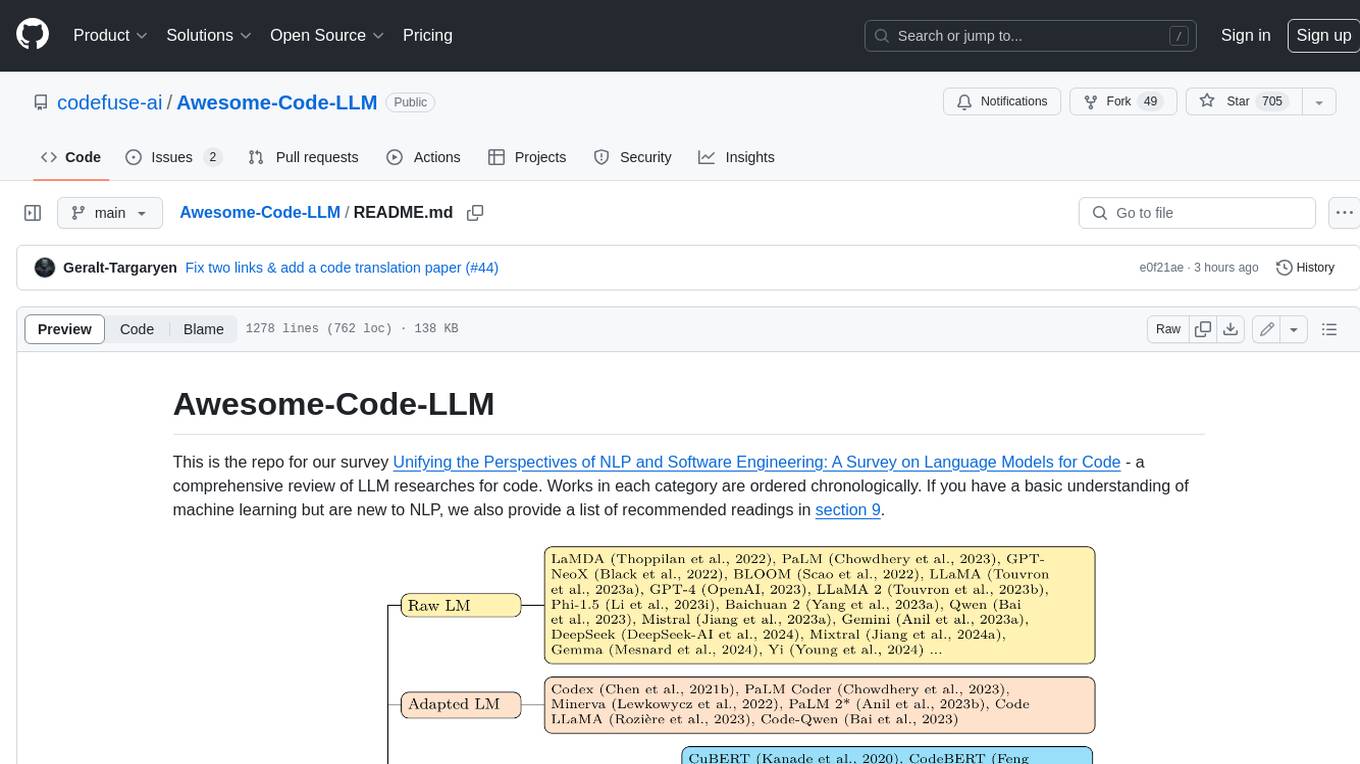
Awesome-Code-LLM
Analyze the following text from a github repository (name and readme text at end) . Then, generate a JSON object with the following keys and provide the corresponding information for each key, in lowercase letters: 'description' (detailed description of the repo, must be less than 400 words,Ensure that no line breaks and quotation marks.),'for_jobs' (List 5 jobs suitable for this tool,in lowercase letters), 'ai_keywords' (keywords of the tool,user may use those keyword to find the tool,in lowercase letters), 'for_tasks' (list of 5 specific tasks user can use this tool to do,in lowercase letters), 'answer' (in english languages)
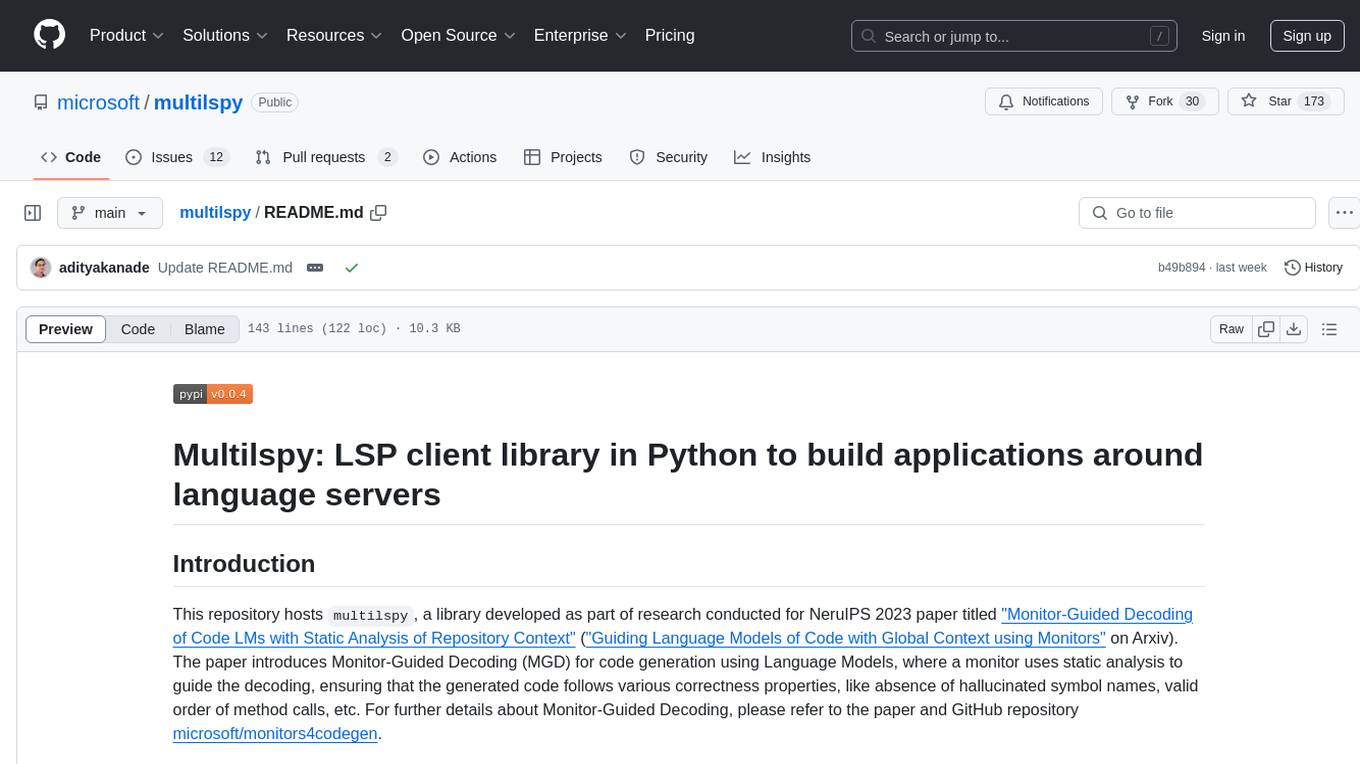
multilspy
Multilspy is a Python library developed for research purposes to facilitate the creation of language server clients for querying and obtaining results of static analyses from various language servers. It simplifies the process by handling server setup, communication, and configuration parameters, providing a common interface for different languages. The library supports features like finding function/class definitions, callers, completions, hover information, and document symbols. It is designed to work with AI systems like Large Language Models (LLMs) for tasks such as Monitor-Guided Decoding to ensure code generation correctness and boost compilability.
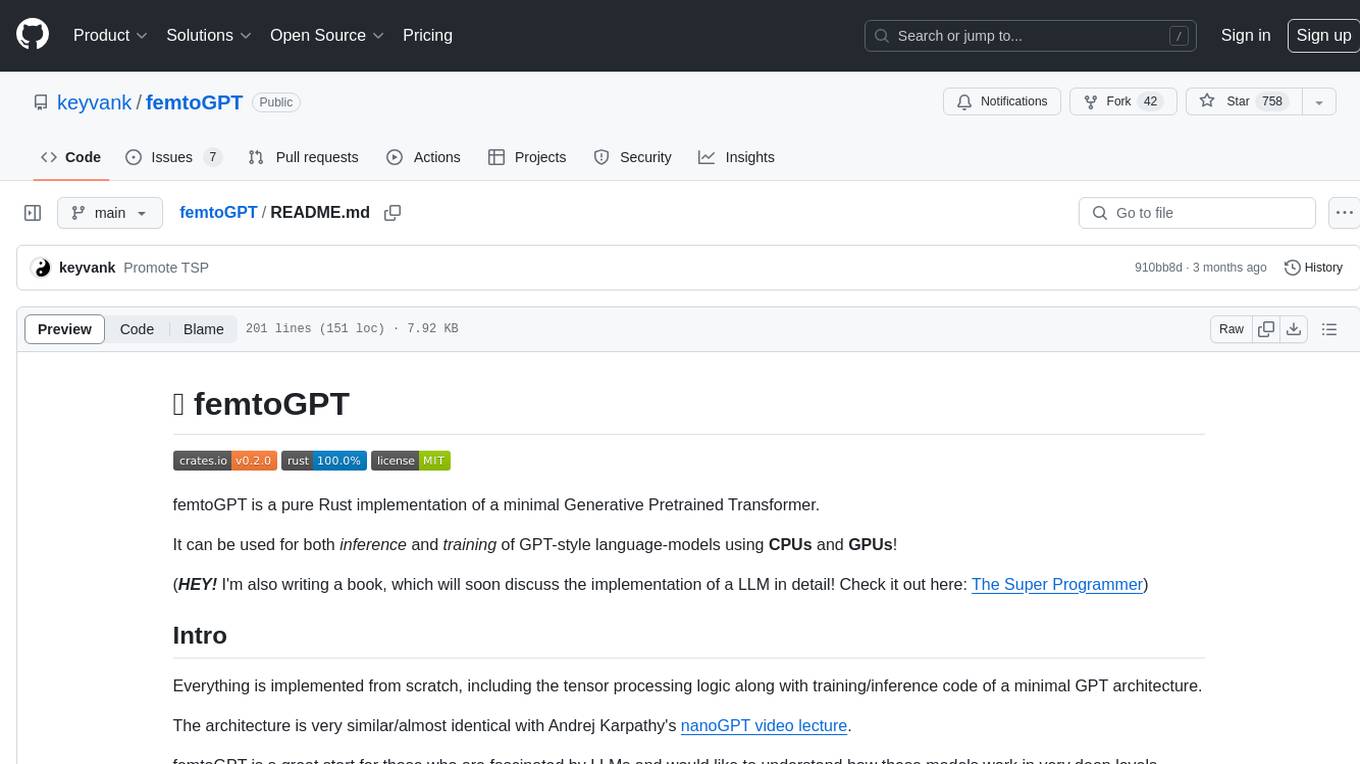
femtoGPT
femtoGPT is a pure Rust implementation of a minimal Generative Pretrained Transformer. It can be used for both inference and training of GPT-style language models using CPUs and GPUs. The tool is implemented from scratch, including tensor processing logic and training/inference code of a minimal GPT architecture. It is a great start for those fascinated by LLMs and wanting to understand how these models work at deep levels. The tool uses random generation libraries, data-serialization libraries, and a parallel computing library. It is relatively fast on CPU and correctness of gradients is checked using the gradient-check method.
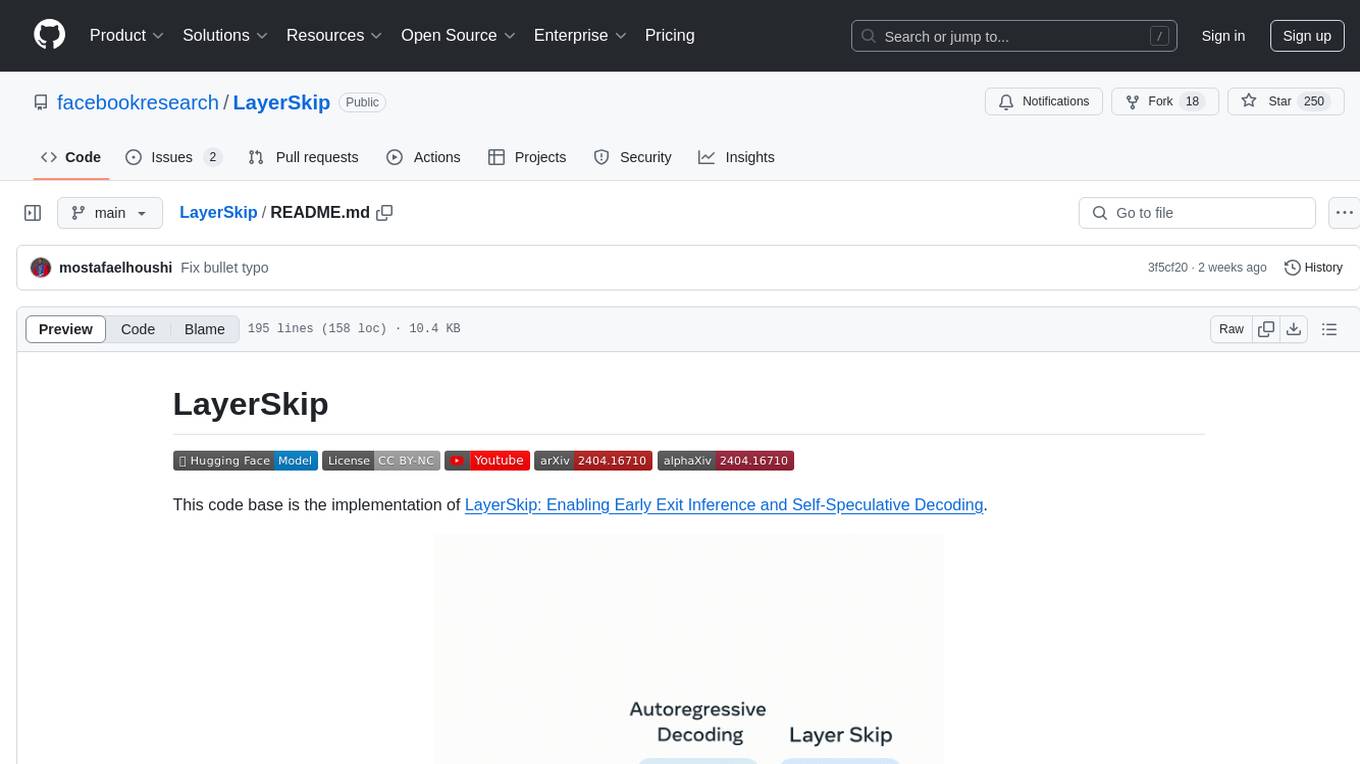
LayerSkip
LayerSkip is an implementation enabling early exit inference and self-speculative decoding. It provides a code base for running models trained using the LayerSkip recipe, offering speedup through self-speculative decoding. The tool integrates with Hugging Face transformers and provides checkpoints for various LLMs. Users can generate tokens, benchmark on datasets, evaluate tasks, and sweep over hyperparameters to optimize inference speed. The tool also includes correctness verification scripts and Docker setup instructions. Additionally, other implementations like gpt-fast and Native HuggingFace are available. Training implementation is a work-in-progress, and contributions are welcome under the CC BY-NC license.
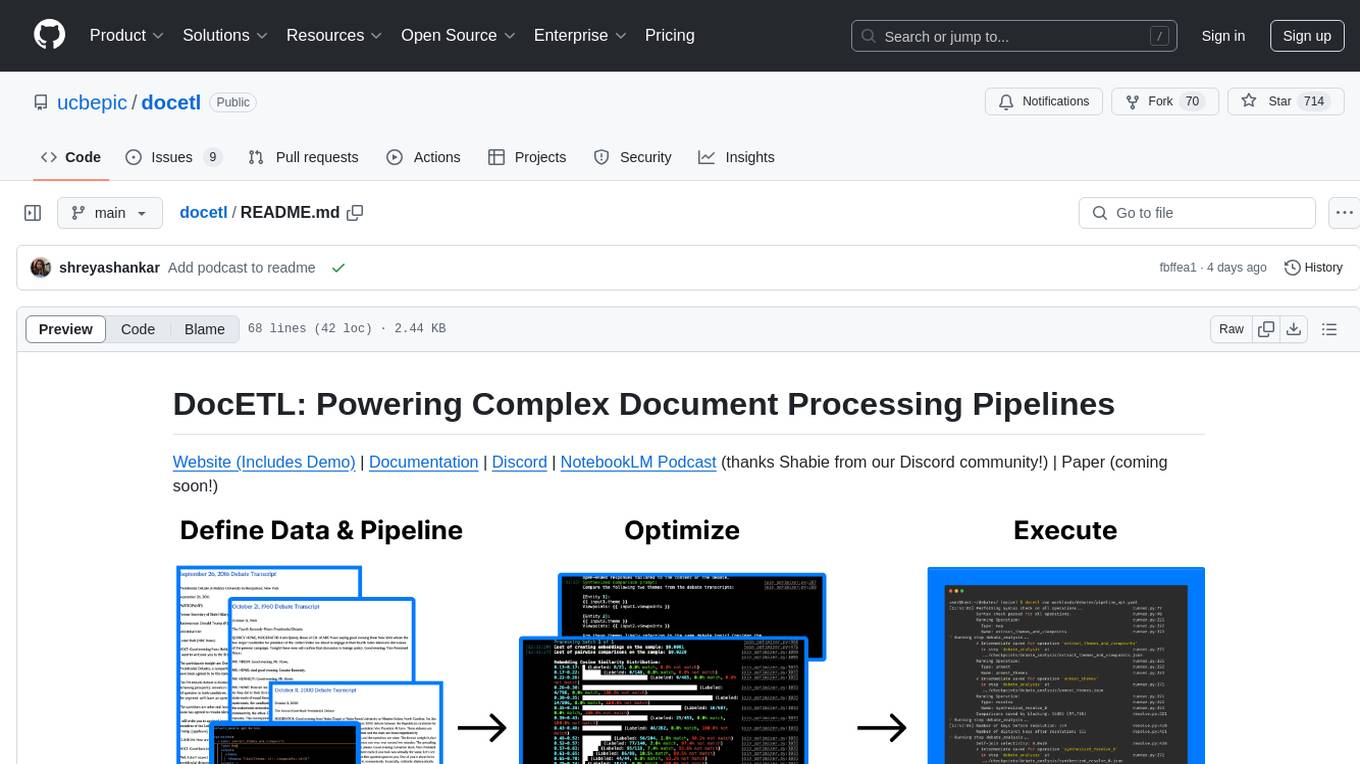
docetl
DocETL is a tool for creating and executing data processing pipelines, especially suited for complex document processing tasks. It offers a low-code, declarative YAML interface to define LLM-powered operations on complex data. Ideal for maximizing correctness and output quality for semantic processing on a collection of data, representing complex tasks via map-reduce, maximizing LLM accuracy, handling long documents, and automating task retries based on validation criteria.
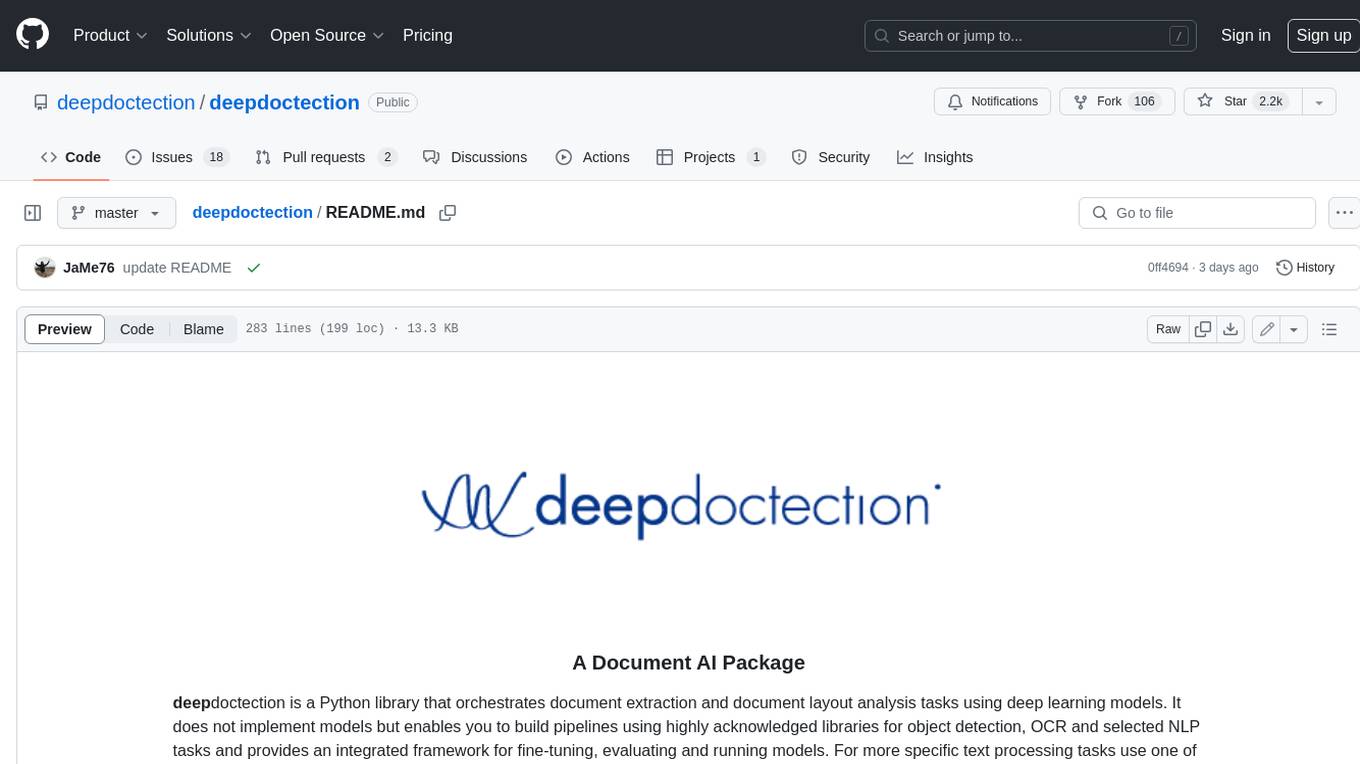
deepdoctection
**deep** doctection is a Python library that orchestrates document extraction and document layout analysis tasks using deep learning models. It does not implement models but enables you to build pipelines using highly acknowledged libraries for object detection, OCR and selected NLP tasks and provides an integrated framework for fine-tuning, evaluating and running models. For more specific text processing tasks use one of the many other great NLP libraries. **deep** doctection focuses on applications and is made for those who want to solve real world problems related to document extraction from PDFs or scans in various image formats. **deep** doctection provides model wrappers of supported libraries for various tasks to be integrated into pipelines. Its core function does not depend on any specific deep learning library. Selected models for the following tasks are currently supported: * Document layout analysis including table recognition in Tensorflow with **Tensorpack**, or PyTorch with **Detectron2**, * OCR with support of **Tesseract**, **DocTr** (Tensorflow and PyTorch implementations available) and a wrapper to an API for a commercial solution, * Text mining for native PDFs with **pdfplumber**, * Language detection with **fastText**, * Deskewing and rotating images with **jdeskew**. * Document and token classification with all LayoutLM models provided by the **Transformer library**. (Yes, you can use any LayoutLM-model with any of the provided OCR-or pdfplumber tools straight away!). * Table detection and table structure recognition with **table-transformer**. * There is a small dataset for token classification available and a lot of new tutorials to show, how to train and evaluate this dataset using LayoutLMv1, LayoutLMv2, LayoutXLM and LayoutLMv3. * Comprehensive configuration of **analyzer** like choosing different models, output parsing, OCR selection. Check this notebook or the docs for more infos. * Document layout analysis and table recognition now runs with **Torchscript** (CPU) as well and **Detectron2** is not required anymore for basic inference. * [**new**] More angle predictors for determining the rotation of a document based on **Tesseract** and **DocTr** (not contained in the built-in Analyzer). * [**new**] Token classification with **LiLT** via **transformers**. We have added a model wrapper for token classification with LiLT and added a some LiLT models to the model catalog that seem to look promising, especially if you want to train a model on non-english data. The training script for LayoutLM can be used for LiLT as well and we will be providing a notebook on how to train a model on a custom dataset soon. **deep** doctection provides on top of that methods for pre-processing inputs to models like cropping or resizing and to post-process results, like validating duplicate outputs, relating words to detected layout segments or ordering words into contiguous text. You will get an output in JSON format that you can customize even further by yourself. Have a look at the **introduction notebook** in the notebook repo for an easy start. Check the **release notes** for recent updates. **deep** doctection or its support libraries provide pre-trained models that are in most of the cases available at the **Hugging Face Model Hub** or that will be automatically downloaded once requested. For instance, you can find pre-trained object detection models from the Tensorpack or Detectron2 framework for coarse layout analysis, table cell detection and table recognition. Training is a substantial part to get pipelines ready on some specific domain, let it be document layout analysis, document classification or NER. **deep** doctection provides training scripts for models that are based on trainers developed from the library that hosts the model code. Moreover, **deep** doctection hosts code to some well established datasets like **Publaynet** that makes it easy to experiment. It also contains mappings from widely used data formats like COCO and it has a dataset framework (akin to **datasets** so that setting up training on a custom dataset becomes very easy. **This notebook** shows you how to do this. **deep** doctection comes equipped with a framework that allows you to evaluate predictions of a single or multiple models in a pipeline against some ground truth. Check again **here** how it is done. Having set up a pipeline it takes you a few lines of code to instantiate the pipeline and after a for loop all pages will be processed through the pipeline.
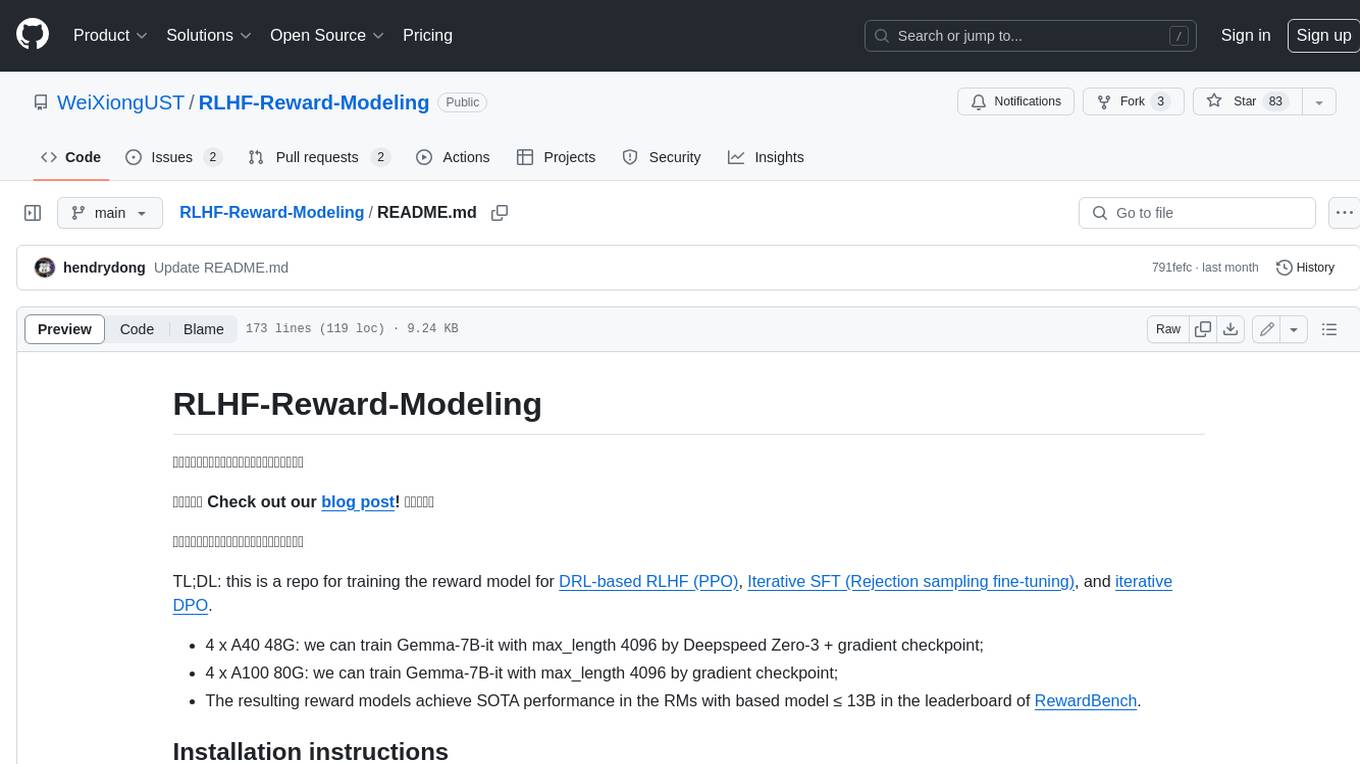
RLHF-Reward-Modeling
This repository contains code for training reward models for Deep Reinforcement Learning-based Reward-modulated Hierarchical Fine-tuning (DRL-based RLHF), Iterative Selection Fine-tuning (Rejection sampling fine-tuning), and iterative Decision Policy Optimization (DPO). The reward models are trained using a Bradley-Terry model based on the Gemma and Mistral language models. The resulting reward models achieve state-of-the-art performance on the RewardBench leaderboard for reward models with base models of up to 13B parameters.
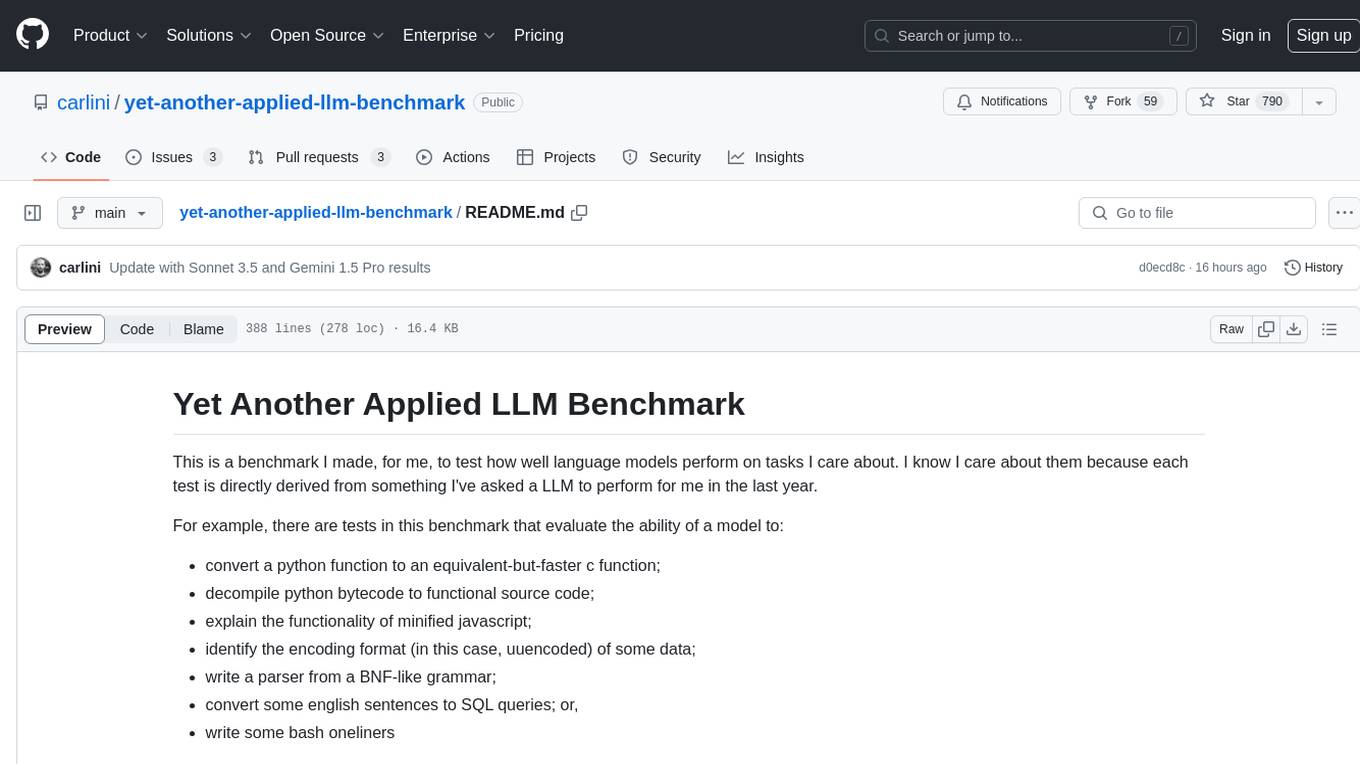
yet-another-applied-llm-benchmark
Yet Another Applied LLM Benchmark is a collection of diverse tests designed to evaluate the capabilities of language models in performing real-world tasks. The benchmark includes tests such as converting code, decompiling bytecode, explaining minified JavaScript, identifying encoding formats, writing parsers, and generating SQL queries. It features a dataflow domain-specific language for easily adding new tests and has nearly 100 tests based on actual scenarios encountered when working with language models. The benchmark aims to assess whether models can effectively handle tasks that users genuinely care about.
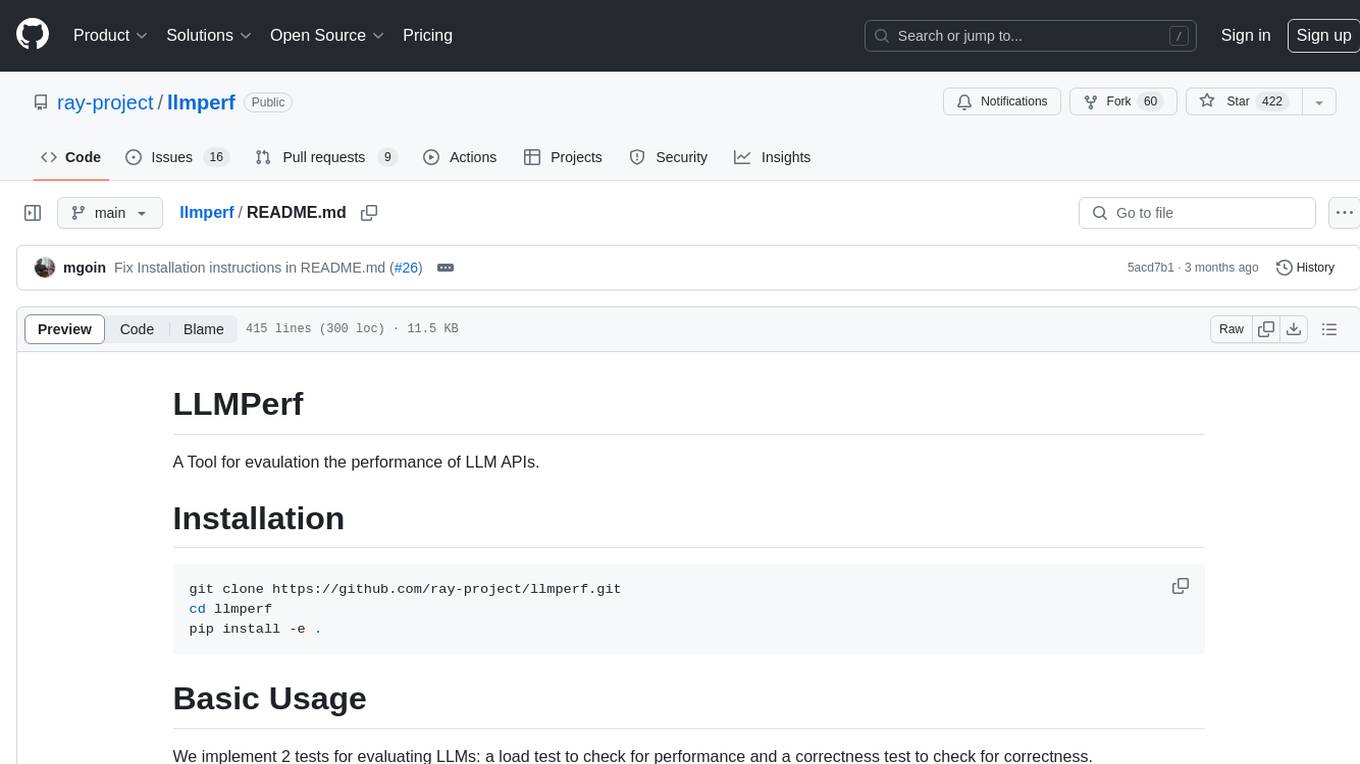
llmperf
LLMPerf is a tool designed for evaluating the performance of Language Model APIs. It provides functionalities for conducting load tests to measure inter-token latency and generation throughput, as well as correctness tests to verify the responses. The tool supports various LLM APIs including OpenAI, Anthropic, TogetherAI, Hugging Face, LiteLLM, Vertex AI, and SageMaker. Users can set different parameters for the tests and analyze the results to assess the performance of the LLM APIs. LLMPerf aims to standardize prompts across different APIs and provide consistent evaluation metrics for comparison.
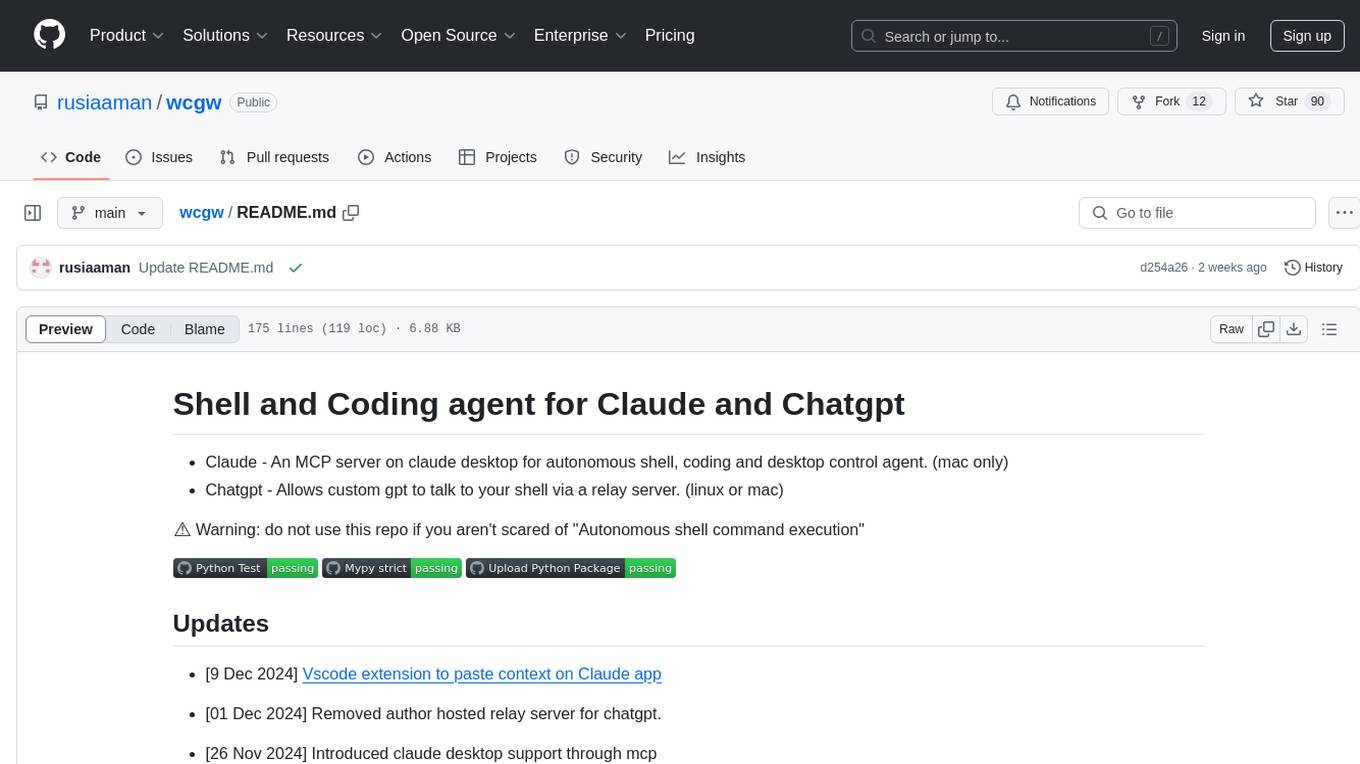
wcgw
wcgw is a shell and coding agent designed for Claude and Chatgpt. It provides full shell access with no restrictions, desktop control on Claude for screen capture and control, interactive command handling, large file editing, and REPL support. Users can use wcgw to create, execute, and iterate on tasks, such as solving problems with Python, finding code instances, setting up projects, creating web apps, editing large files, and running server commands. Additionally, wcgw supports computer use on Docker containers for desktop control. The tool can be extended with a VS Code extension for pasting context on Claude app and integrates with Chatgpt for custom GPT interactions.
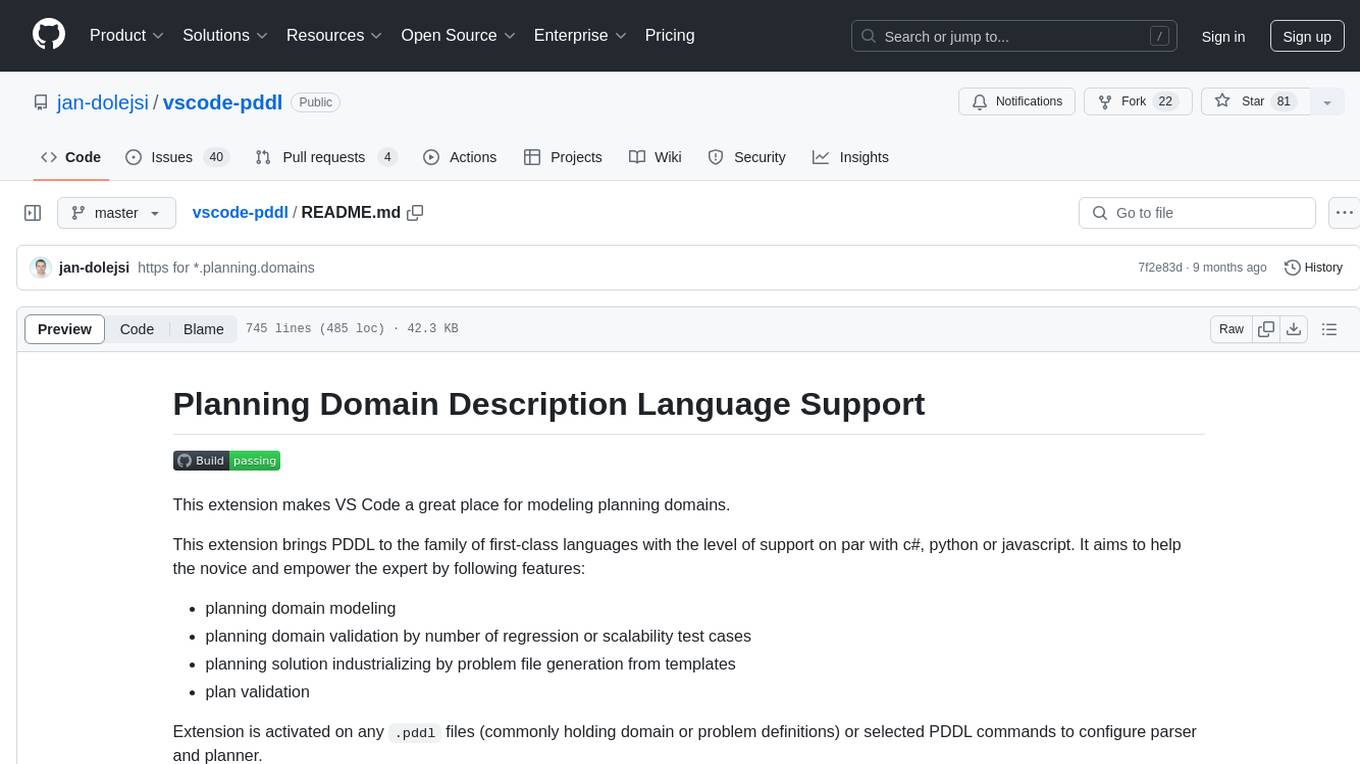
vscode-pddl
The vscode-pddl extension provides comprehensive support for Planning Domain Description Language (PDDL) in Visual Studio Code. It enables users to model planning domains, validate them, industrialize planning solutions, and run planners. The extension offers features like syntax highlighting, auto-completion, plan visualization, plan validation, plan happenings evaluation, search debugging, and integration with Planning.Domains. Users can create PDDL files, run planners, visualize plans, and debug search algorithms efficiently within VS Code.
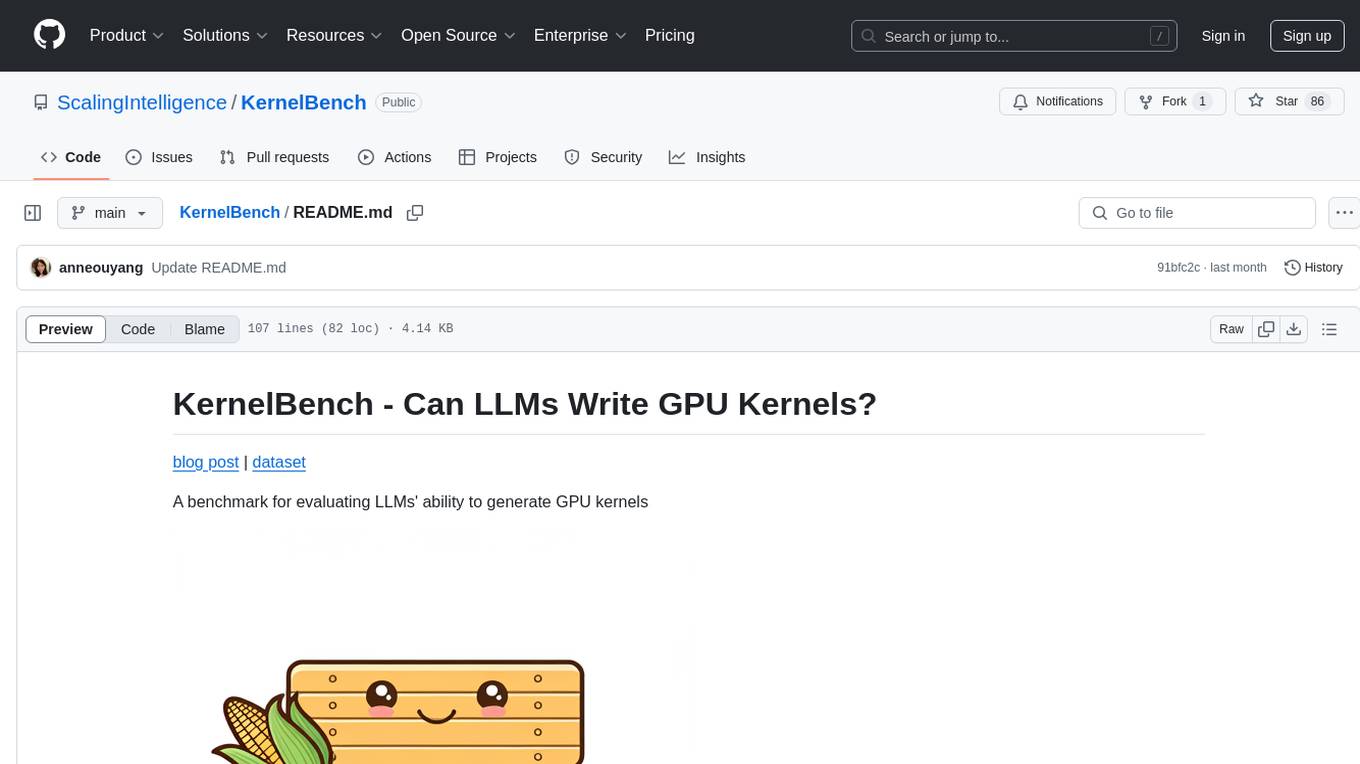
KernelBench
KernelBench is a benchmark tool designed to evaluate Large Language Models' (LLMs) ability to generate GPU kernels. It focuses on transpiling operators from PyTorch to CUDA kernels at different levels of granularity. The tool categorizes problems into four levels, ranging from single-kernel operators to full model architectures, and assesses solutions based on compilation, correctness, and speed. The repository provides a structured directory layout, setup instructions, usage examples for running single or multiple problems, and upcoming roadmap features like additional GPU platform support and integration with other frameworks.
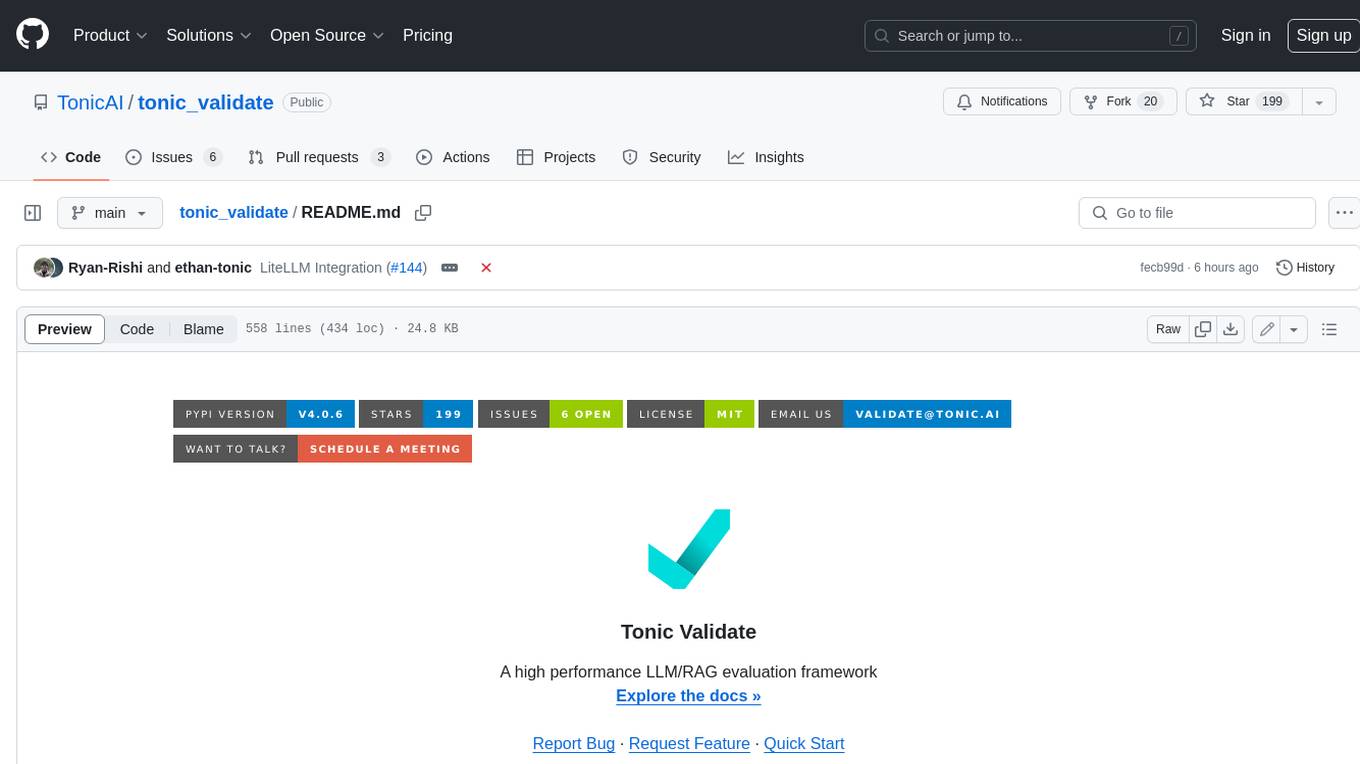
tonic_validate
Tonic Validate is a framework for the evaluation of LLM outputs, such as Retrieval Augmented Generation (RAG) pipelines. Validate makes it easy to evaluate, track, and monitor your LLM and RAG applications. Validate allows you to evaluate your LLM outputs through the use of our provided metrics which measure everything from answer correctness to LLM hallucination. Additionally, Validate has an optional UI to visualize your evaluation results for easy tracking and monitoring.
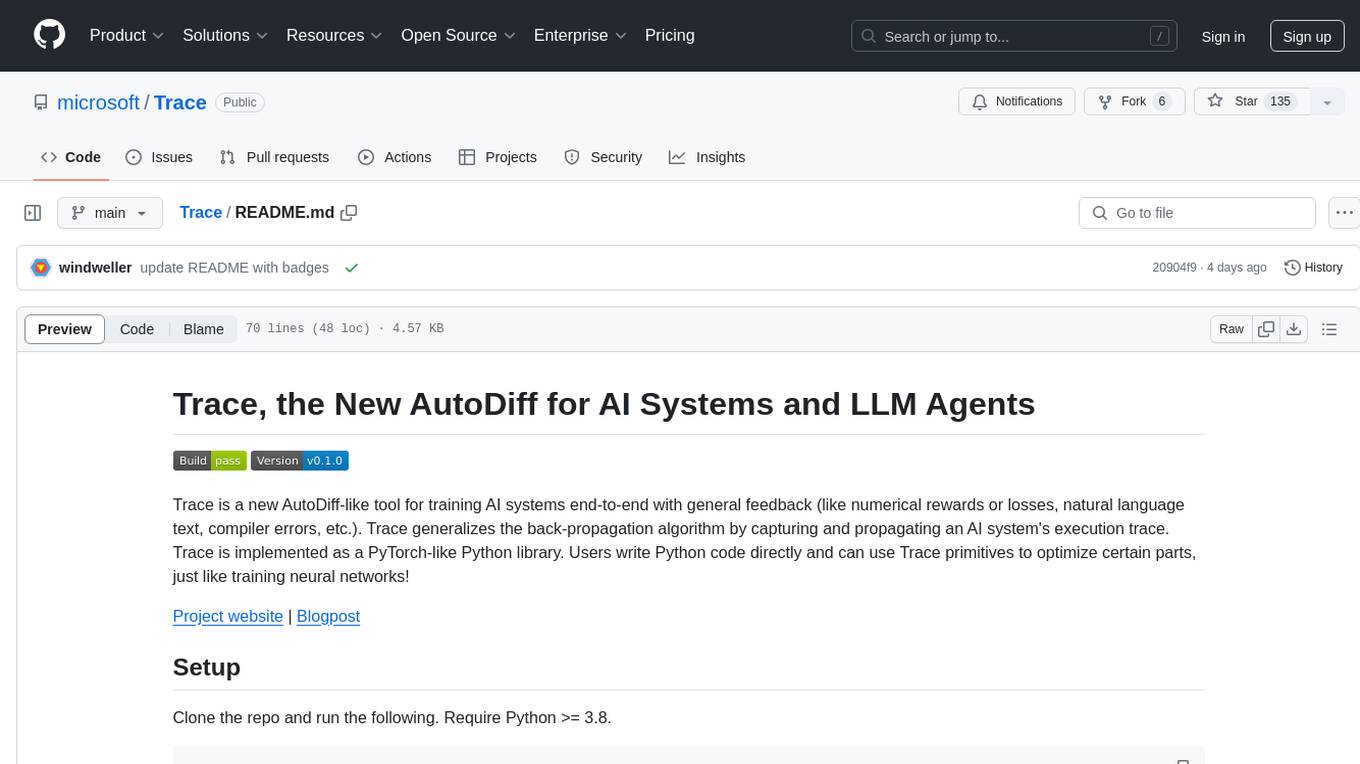
Trace
Trace is a new AutoDiff-like tool for training AI systems end-to-end with general feedback. It generalizes the back-propagation algorithm by capturing and propagating an AI system's execution trace. Implemented as a PyTorch-like Python library, users can write Python code directly and use Trace primitives to optimize certain parts, similar to training neural networks.
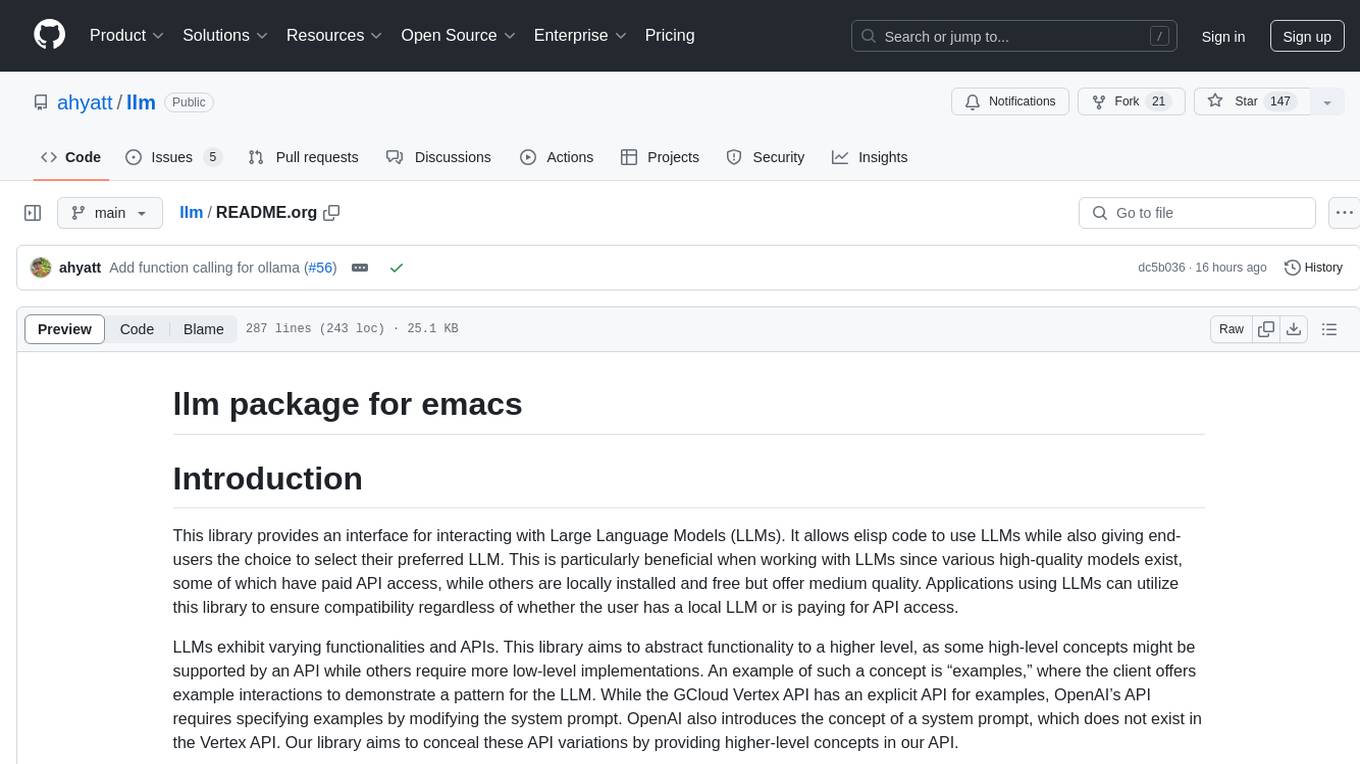
llm
The 'llm' package for Emacs provides an interface for interacting with Large Language Models (LLMs). It abstracts functionality to a higher level, concealing API variations and ensuring compatibility with various LLMs. Users can set up providers like OpenAI, Gemini, Vertex, Claude, Ollama, GPT4All, and a fake client for testing. The package allows for chat interactions, embeddings, token counting, and function calling. It also offers advanced prompt creation and logging capabilities. Users can handle conversations, create prompts with placeholders, and contribute by creating providers.
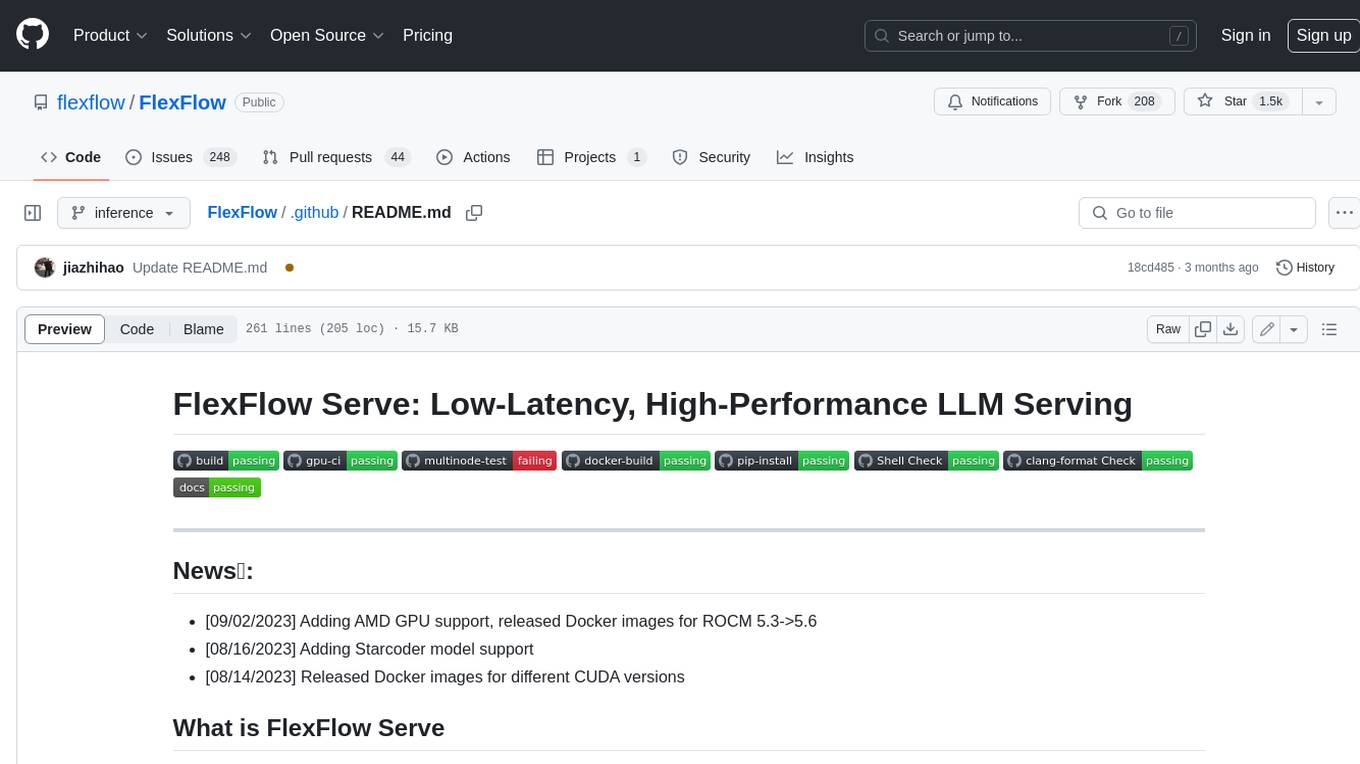
FlexFlow
FlexFlow Serve is an open-source compiler and distributed system for **low latency**, **high performance** LLM serving. FlexFlow Serve outperforms existing systems by 1.3-2.0x for single-node, multi-GPU inference and by 1.4-2.4x for multi-node, multi-GPU inference.
20 - OpenAI Gpts
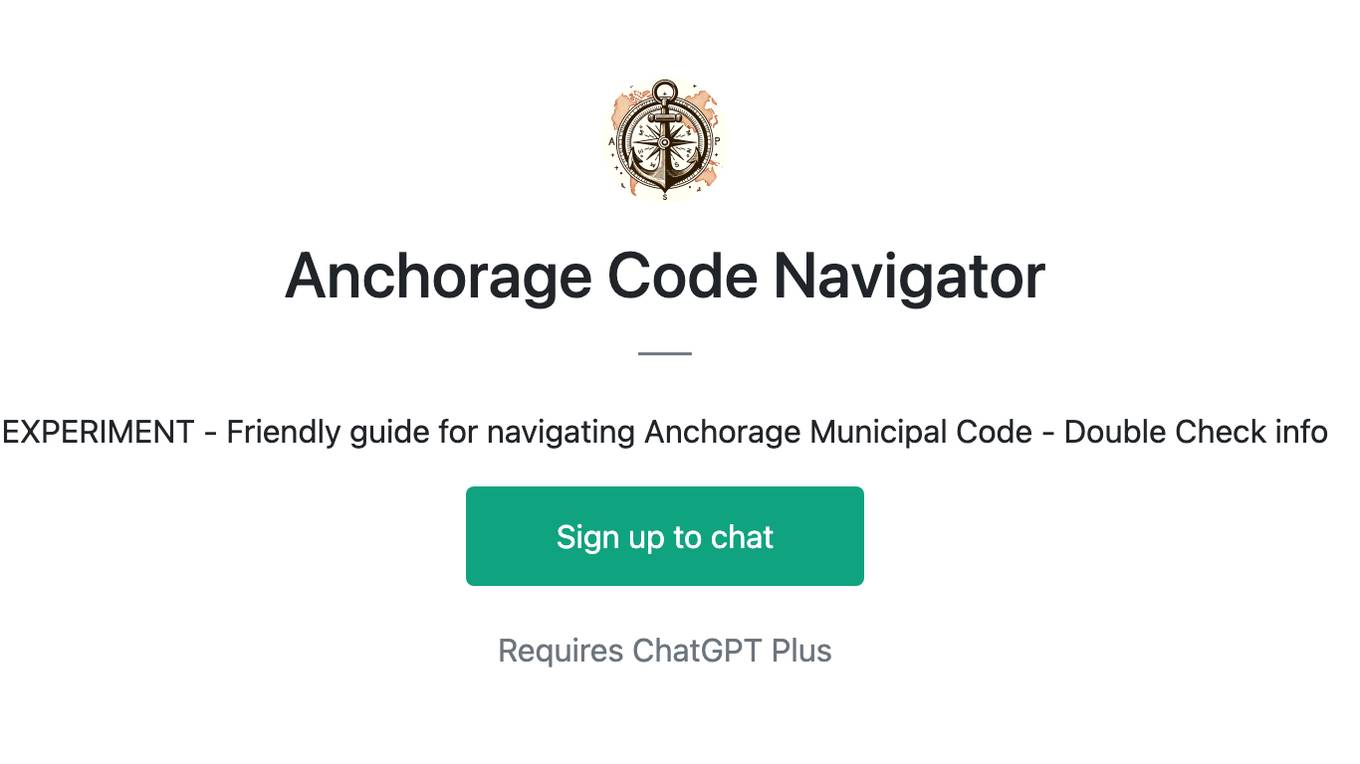
Anchorage Code Navigator
EXPERIMENT - Friendly guide for navigating Anchorage Municipal Code - Double Check info
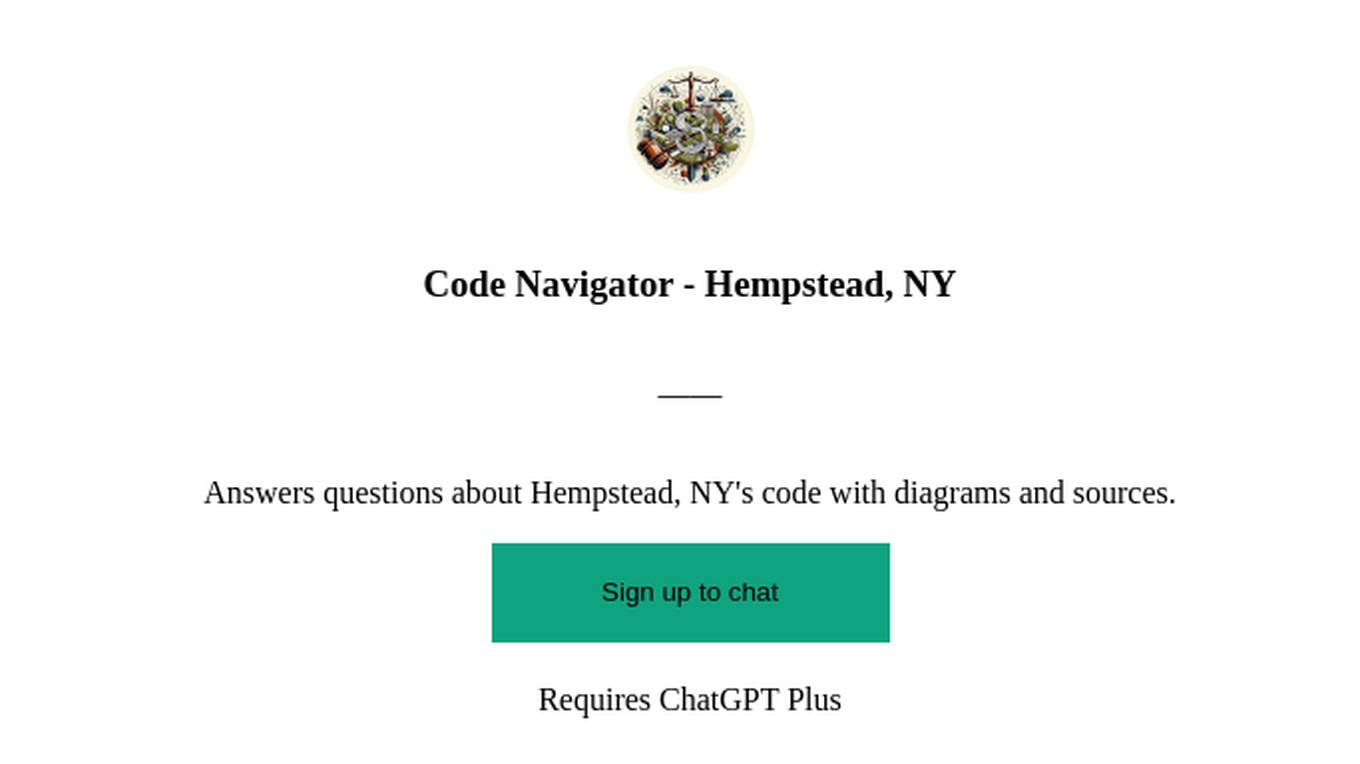
Code Navigator - Hempstead, NY
Answers questions about Hempstead, NY's code with diagrams and sources.
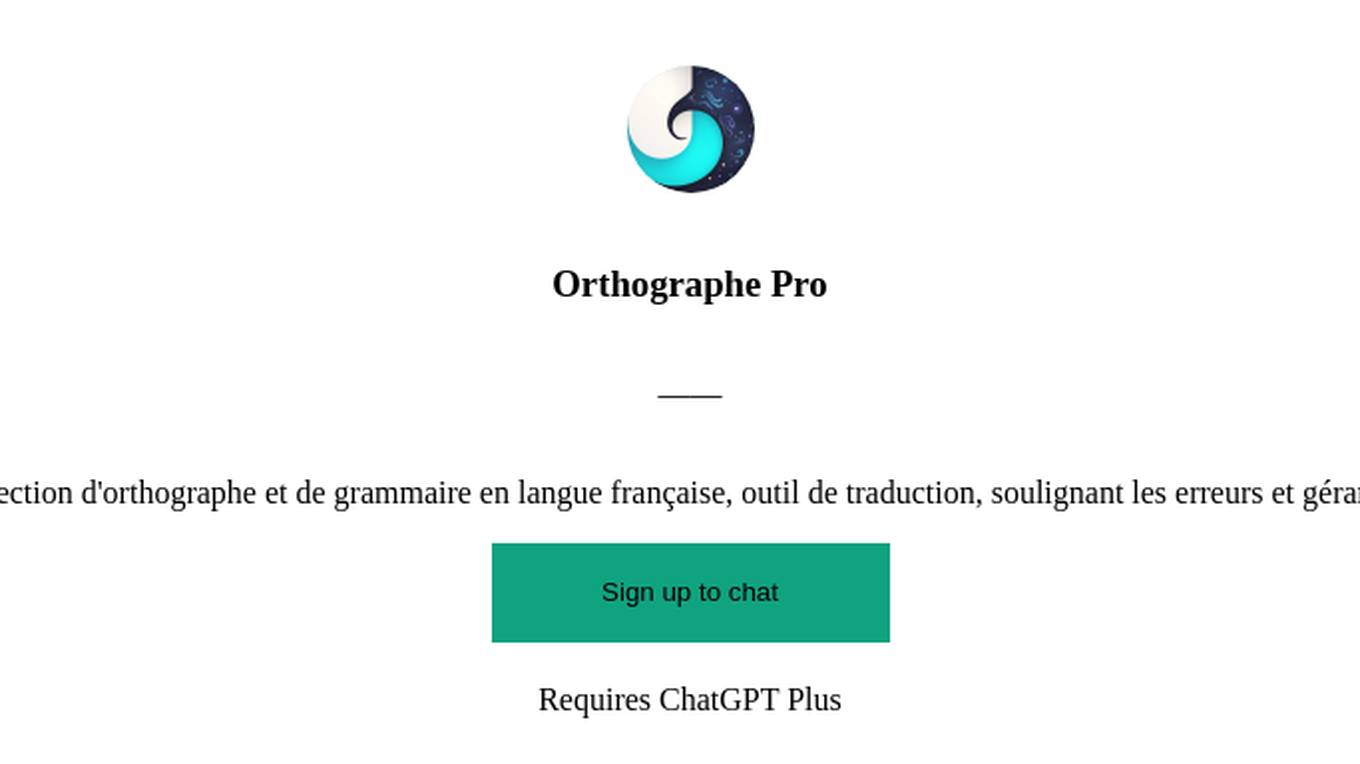
Orthographe Pro
Un outil de correction d'orthographe et de grammaire en langue française, outil de traduction, soulignant les erreurs et gérant le code HTML.
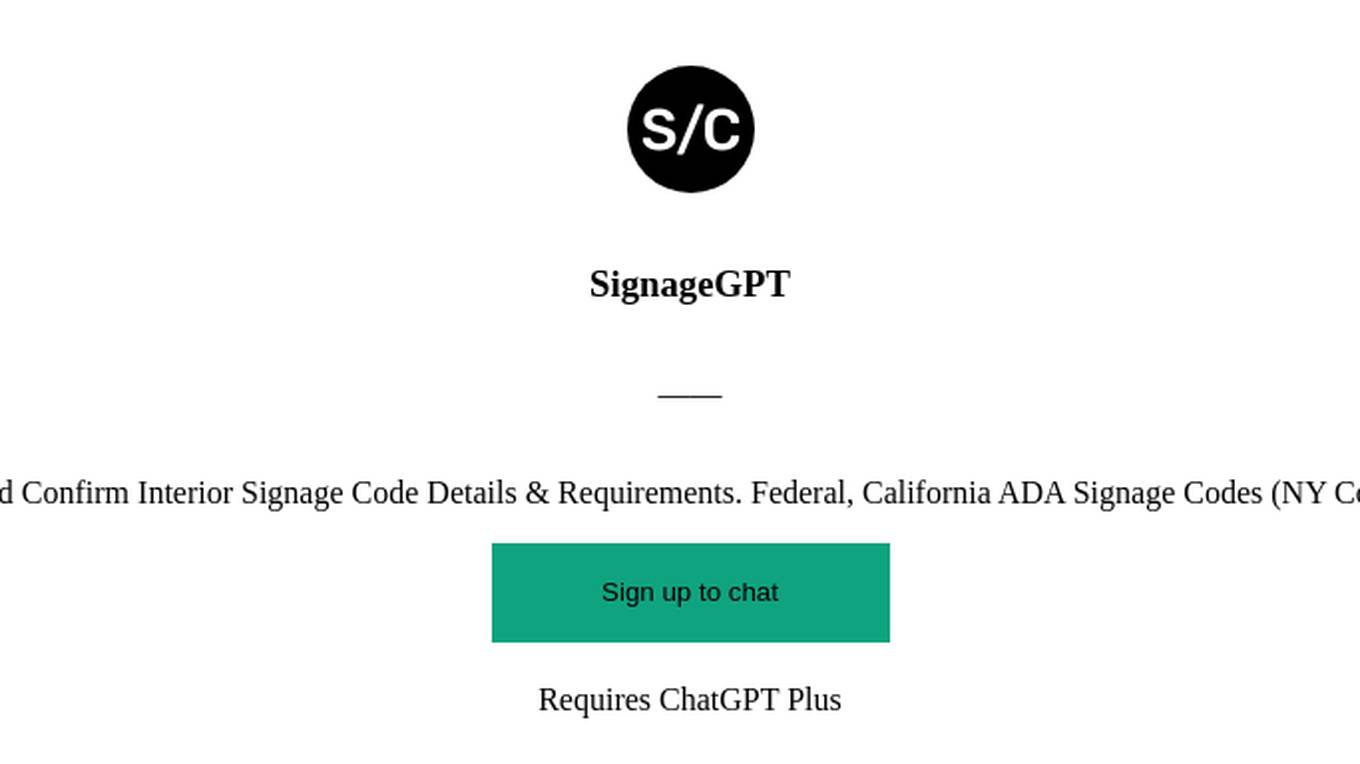
SignageGPT
Identify and Confirm Interior Signage Code Details & Requirements. Federal, California ADA Signage Codes (NY Coming Soon)
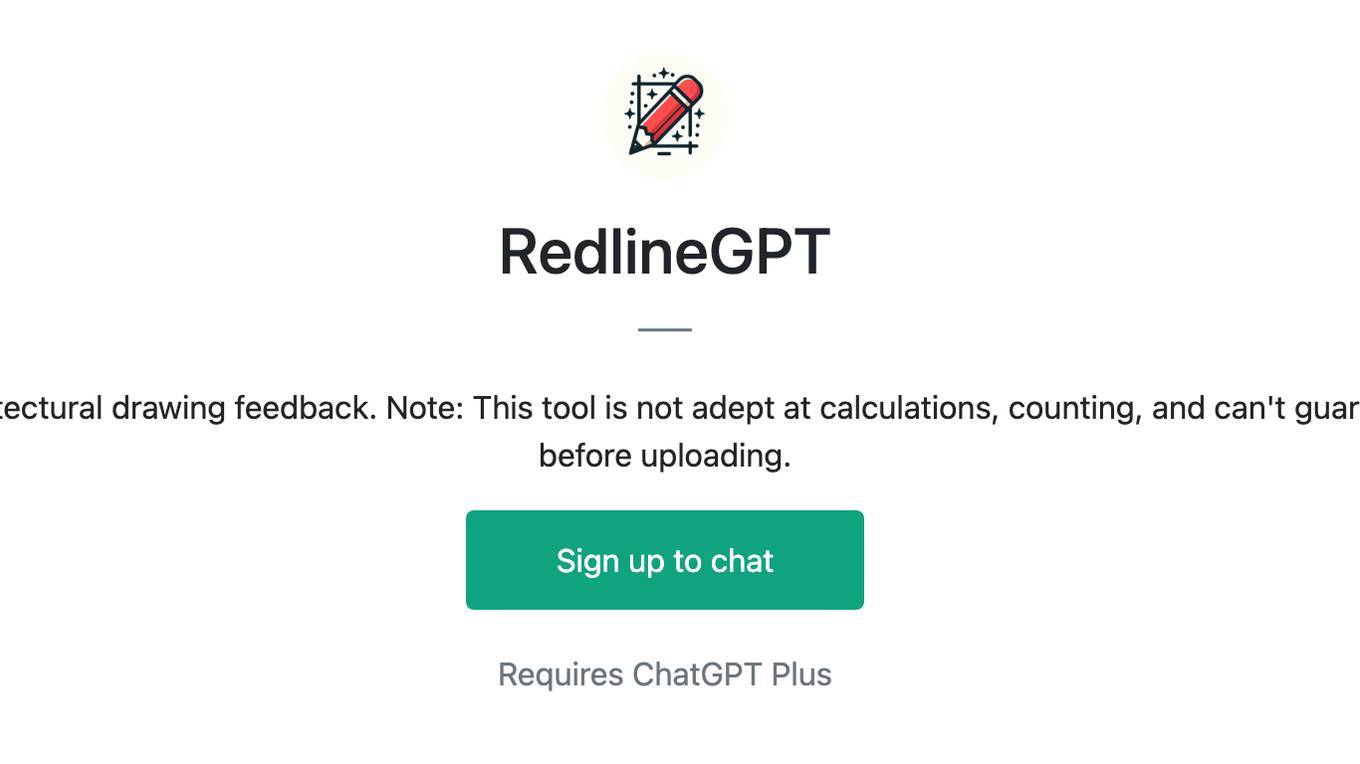
RedlineGPT
Upload a jpg/png (<5MB, <2000px) for architectural drawing feedback. Note: This tool is not adept at calculations, counting, and can't guarantee code compliance. Consider IP issues before uploading.