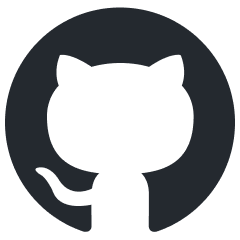
Odyssey
Odyssey: Empowering Minecraft Agents with Open-World Skills
Stars: 302
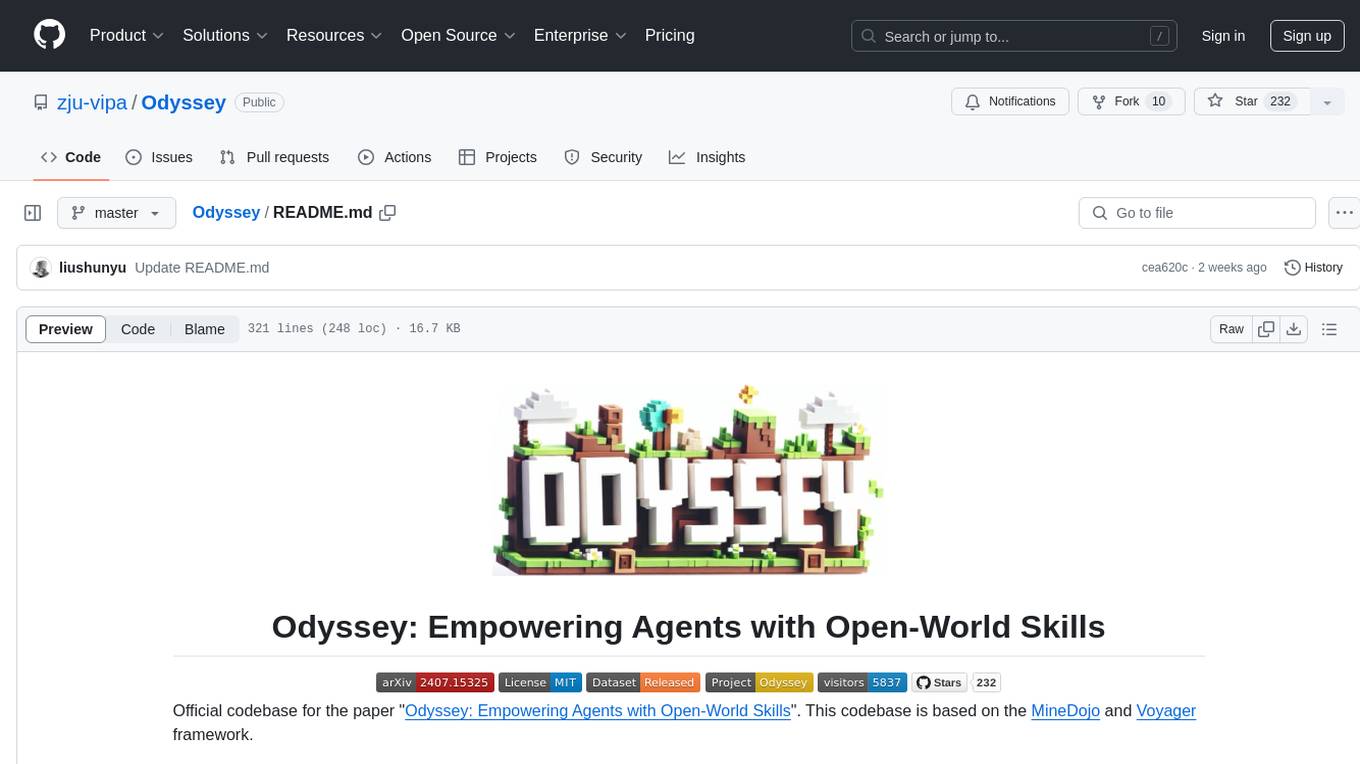
Odyssey is a framework designed to empower agents with open-world skills in Minecraft. It provides an interactive agent with a skill library, a fine-tuned LLaMA-3 model, and an open-world benchmark for evaluating agent capabilities. The framework enables agents to explore diverse gameplay opportunities in the vast Minecraft world by offering primitive and compositional skills, extensive training data, and various long-term planning tasks. Odyssey aims to advance research on autonomous agent solutions by providing datasets, model weights, and code for public use.
README:
Official codebase for the paper "Odyssey: Empowering Minecraft Agents with Open-World Skills". This codebase is based on the Voyager framework.
Abstract: Recent studies have delved into constructing generalist agents for open-world environments like Minecraft. Despite the encouraging results, existing efforts mainly focus on solving basic programmatic tasks, e.g., material collection and tool-crafting following the Minecraft tech-tree, treating the ObtainDiamond task as the ultimate goal. This limitation stems from the narrowly defined set of actions available to agents, requiring them to learn effective long-horizon strategies from scratch. Consequently, discovering diverse gameplay opportunities in the open world becomes challenging. In this work, we introduce Odyssey, a new framework that empowers Large Language Model (LLM)-based agents with open-world skills to explore the vast Minecraft world. Odyssey comprises three key parts:
- (1) An interactive agent with an open-world skill library that consists of 40 primitive skills and 183 compositional skills.
- (2) A fine-tuned LLaMA-3 model trained on a large question-answering dataset with 390k+ instruction entries derived from the Minecraft Wiki.
- (3) A new agent capability benchmark includes the long-term planning task, the dynamic-immediate planning task, and the autonomous exploration task.
Extensive experiments demonstrate that the proposed Odyssey framework can effectively evaluate different capabilities of LLM-based agents. All datasets, model weights, and code are publicly available to motivate future research on more advanced autonomous agent solutions.
-
[Mar 5, 2025]
🔥 We have uploaded our new paper titled "Parallelized Planning-Acting for Efficient LLM-based Multi-Agent Systems" to arXiv. -
[Feb 23, 2025]
🔥 We have open-sourced the Multi-Agent Framework to align with our latest paper. -
[Oct 1, 2024]
🔥 We have additionally compared more baselines (with different open-sourced LLMs and agents) and designed more test scenarios (for the long-term planning task and the dynamic-immediate planning task) in the updated version of the paper. -
[Sep 1, 2024]
🔥 We have additionally open-sourced the Web Crawler Program, which was used to collect data from Minecraft Wikis. Researchers can modify this program to crawl data relevant to their needs. -
[Aug 14, 2024]
🔥 We have additionally open-sourced the Comprehensive Skill Library, aiming to provide an automated tool to collect all collectible and craftable items in Minecraft. -
[Jul 23, 2024]
🔥 The paper for ODYSSEY has been uploaded to arXiv! -
[Jun 13, 2024]
🔥 The GitHub repository for ODYSSEY has been open-sourced!
All demonstration videos were captured using the spectator mode within Minecraft. To comply with GitHub's file size restrictions, some videos have been accelerated.
Mining Diamonds from Scratch:
Craft Sword and Combat Zombie:
Shear a Sheep and Milk a Cow:
Autonomous Exploration: (Only First Few Rounds)
-
LLM-Backend
Code to deploy LLM backend.
-
MC-Crawler
Crawling Minecraft game information from Minecraft Wiki and storing data in markdown format.
-
MineMA-Model-Fine-Tuning
Code to fine-tune the LLaMa model and generate training and test datasets.
-
Odyssey
Code for Minecraft agents based on a large language model and skill library.
We use Python ≥ 3.9 and Node.js ≥ 16.13.0. We have tested on Ubuntu 20.04, Windows 10, and macOS.
cd Odyssey
pip install -e .
pip install -r requirements.txt
npm install -g yarn
cd Odyssey/odyssey/env/mineflayer
yarn install
cd Odyssey/odyssey/env/mineflayer/mineflayer-collectblock
npx tsc
cd Odyssey/odyssey/env/mineflayer
yarn install
cd Odyssey/odyssey/env/mineflayer/node_modules/mineflayer-collectblock
npx tsc
You can deploy a Minecraft server using docker. See here.
-
Need to install git-lfs first.
-
Download the embedding model repository
git lfs install git clone https://huggingface.co/sentence-transformers/paraphrase-multilingual-MiniLM-L12-v2.git
-
The directory where you clone the repository is then used to set
embedding_dir
.
You need to create config.json
according to the format of conf/config.json.keep.this
in conf
directory.
-
server_host
: LLaMa backend server ip. -
server_port
: LLaMa backend server port. -
NODE_SERVER_PORT
: Node service port. -
SENTENT_EMBEDDING_DIR
: Path to your embedding model. -
MC_SERVER_HOST
: Minecraft server ip. -
MC_SERVER_PORT
: Minecraft server port.
After completing the above installation and configuration, you can start the agent by simply running python main.py
. To operate the agent under different task scenarios, manually modify the function you wish to execute. Below are the task scenarios.
def test_subgoal():
odyssey_l3_8b = Odyssey(
mc_port=mc_port,
mc_host=mc_host,
env_wait_ticks=env_wait_ticks,
skill_library_dir="./skill_library",
reload=True, # set to True if the skill_json updated
embedding_dir=embedding_dir, # your model path
environment='subgoal',
resume=False,
server_port=node_port,
critic_agent_model_name = ModelType.LLAMA3_8B_V3,
comment_agent_model_name = ModelType.LLAMA3_8B_V3,
curriculum_agent_qa_model_name = ModelType.LLAMA3_8B_V3,
curriculum_agent_model_name = ModelType.LLAMA3_8B_V3,
action_agent_model_name = ModelType.LLAMA3_8B_V3,
)
# 5 classic MC tasks
test_sub_goals = ["craft crafting table", "craft wooden pickaxe", "craft stone pickaxe", "craft iron pickaxe", "mine diamond"]
try:
odyssey_l3_8b.inference_sub_goal(task="subgoal_llama3_8b_v3", sub_goals=test_sub_goals)
except Exception as e:
print(e)
Model | For what |
---|---|
action_agent_model_name | Choose one of the k retrieved skills to execute |
curriculum_agent_model_name | Propose tasks for farming and explore |
curriculum_agent_qa_model_name | Schedule subtasks for combat, generate QA context, and rank the order to kill monsters |
critic_agent_model_name | Action critic |
comment_agent_model_name | Give the critic about the last combat result, in order to reschedule subtasks for combat |
def test_combat():
odyssey_l3_70b = Odyssey(
mc_port=mc_port,
mc_host=mc_host,
env_wait_ticks=env_wait_ticks,
skill_library_dir="./skill_library",
reload=True, # set to True if the skill_json updated
embedding_dir=embedding_dir, # your model path
environment='combat',
resume=False,
server_port=node_port,
critic_agent_model_name = ModelType.LLAMA3_70B_V1,
comment_agent_model_name = ModelType.LLAMA3_70B_V1,
curriculum_agent_qa_model_name = ModelType.LLAMA3_70B_V1,
curriculum_agent_model_name = ModelType.LLAMA3_70B_V1,
action_agent_model_name = ModelType.LLAMA3_70B_V1,
)
multi_rounds_tasks = ["1 enderman", "3 zombie"]
l70_v1_combat_benchmark = [
# Single-mob tasks
"1 skeleton", "1 spider", "1 zombified_piglin", "1 zombie",
# Multi-mob tasks
"1 zombie, 1 skeleton", "1 zombie, 1 spider", "1 zombie, 1 skeleton, 1 spider"
]
for task in l70_v1_combat_benchmark:
odyssey_l3_70b.inference(task=task, reset_env=False, feedback_rounds=1)
for task in multi_rounds_tasks:
odyssey_l3_70b.inference(task=task, reset_env=False, feedback_rounds=3)
def test_farming():
odyssey_l3_8b = Odyssey(
mc_port=mc_port,
mc_host=mc_host,
env_wait_ticks=env_wait_ticks,
skill_library_dir="./skill_library",
reload=True, # set to True if the skill_json updated
embedding_dir=embedding_dir, # your model path
environment='farming',
resume=False,
server_port=node_port,
critic_agent_model_name = ModelType.LLAMA3_8B_V3,
comment_agent_model_name = ModelType.LLAMA3_8B_V3,
curriculum_agent_qa_model_name = ModelType.LLAMA3_8B_V3,
curriculum_agent_model_name = ModelType.LLAMA3_8B_V3,
action_agent_model_name = ModelType.LLAMA3_8B_V3,
)
farming_benchmark = [
# Single-goal tasks
"collect 1 wool by shearing 1 sheep",
"collect 1 bucket of milk",
"cook 1 meat (beef or mutton or pork or chicken)",
# Multi-goal tasks
"collect and plant 1 seed (wheat or melon or pumpkin)"
]
for goal in farming_benchmark:
odyssey_l3_8b.learn(goals=goal, reset_env=False)
def explore():
odyssey_l3_8b = Odyssey(
mc_port=mc_port,
mc_host=mc_host,
env_wait_ticks=env_wait_ticks,
skill_library_dir="./skill_library",
reload=True, # set to True if the skill_json updated
embedding_dir=embedding_dir, # your model path
environment='explore',
resume=False,
server_port=node_port,
critic_agent_model_name = ModelType.LLAMA3_8B,
comment_agent_model_name = ModelType.LLAMA3_8B,
curriculum_agent_qa_model_name = ModelType.LLAMA3_8B,
curriculum_agent_model_name = ModelType.LLAMA3_8B,
action_agent_model_name = ModelType.LLAMA3_8B,
username='bot1_8b'
)
odyssey_l3_8b.learn()
ID | Paper | Authors | Venue |
---|---|---|---|
1 | MineRL: A Large-Scale Dataset of Minecraft Demonstrations | William H. Guss, Brandon Houghton, Nicholay Topin, Phillip Wang, Cayden Codel, Manuela Veloso, Ruslan Salakhutdinov | IJCAI 2019 |
2 | Video PreTraining (VPT): Learning to Act by Watching Unlabeled Online Videos | Bowen Baker, Ilge Akkaya, Peter Zhokhov, Joost Huizinga, Jie Tang, Adrien Ecoffet, Brandon Houghton, Raul Sampedro, Jeff Clune | arXiv 2022 |
3 | MineDojo: Building Open-Ended Embodied Agents with Internet-Scale Knowledge | Linxi Fan, Guanzhi Wang, Yunfan Jiang, Ajay Mandlekar, Yuncong Yang, Haoyi Zhu, Andrew Tang, De-An Huang, Yuke Zhu, Anima Anandkumar | NeurIPS 2022 |
4 | Open-World Multi-Task Control Through Goal-Aware Representation Learning and Adaptive Horizon Prediction | Shaofei Cai, Zihao Wang, Xiaojian Ma, Anji Liu, Yitao Liang | CVPR 2023 |
5 | Describe, Explain, Plan and Select: Interactive Planning with Large Language Models Enables Open-World Multi-Task Agents | Zihao Wang, Shaofei Cai, Guanzhou Chen, Anji Liu, Xiaojian Ma, Yitao Liang | NeurIPS 2023 |
6 | Skill Reinforcement Learning and Planning for Open-World Long-Horizon Tasks | Haoqi Yuan, Chi Zhang, Hongcheng Wang, Feiyang Xie, Penglin Cai, Hao Dong, Zongqing Lu | NeurIPS Workshop 2023 |
7 | Voyager: An Open-Ended Embodied Agent with Large Language Models | Guanzhi Wang, Yuqi Xie, Yunfan Jiang, Ajay Mandlekar, Chaowei Xiao, Yuke Zhu, Linxi Fan, Anima Anandkumar | arXiv 2023 |
8 | Ghost in the Minecraft: Generally Capable Agents for Open-World Environments via Large Language Models with Text-based Knowledge and Memory | Xizhou Zhu, Yuntao Chen, Hao Tian, Chenxin Tao, Weijie Su, Chenyu Yang, Gao Huang, Bin Li, Lewei Lu, Xiaogang Wang, Yu Qiao, Zhaoxiang Zhang, Jifeng Dai | arXiv 2023 |
9 | STEVE-1: A Generative Model for Text-to-Behavior in Minecraft | Shalev Lifshitz, Keiran Paster, Harris Chan, Jimmy Ba, Sheila McIlraith | NeurIPS 2023 |
10 | GROOT: Learning to Follow Instructions by Watching Gameplay Videos | Shaofei Cai, Bowei Zhang, Zihao Wang, Xiaojian Ma, Anji Liu, Yitao Liang | arXiv 2023 |
11 | MCU: A Task-centric Framework for Open-ended Agent Evaluation in Minecraft | Haowei Lin, Zihao Wang, Jianzhu Ma, Yitao Liang | arXiv 2023 |
12 | LLaMA Rider: Spurring Large Language Models to Explore the Open World | Yicheng Feng, Yuxuan Wang, Jiazheng Liu, Sipeng Zheng, Zongqing Lu | arXiv 2023 |
13 | JARVIS-1: Open-World Multi-task Agents with Memory-Augmented Multimodal Language Models | Zihao Wang, Shaofei Cai, Anji Liu, Yonggang Jin, Jinbing Hou, Bowei Zhang, Haowei Lin, Zhaofeng He, Zilong Zheng, Yaodong Yang, Xiaojian Ma, Yitao Liang | arXiv 2023 |
14 | See and Think: Embodied Agent in Virtual Environment | Zhonghan Zhao, Wenhao Chai, Xuan Wang, Li Boyi, Shengyu Hao, Shidong Cao, Tian Ye, Jenq-Neng Hwang, Gaoang Wang | arXiv 2023 |
15 | Creative Agents: Empowering Agents with Imagination for Creative Tasks | Chi Zhang, Penglin Cai, Yuhui Fu, Haoqi Yuan, Zongqing Lu | arXiv 2023 |
16 | MP5: A Multi-modal Open-ended Embodied System in Minecraft via Active Perception | Yiran Qin, Enshen Zhou, Qichang Liu, Zhenfei Yin, Lu Sheng, Ruimao Zhang, Yu Qiao, Jing Shao | arXiv 2024 |
17 | Auto MC-Reward: Automated Dense Reward Design with Large Language Models for Minecraft | Hao Li, Xue Yang, Zhaokai Wang, Xizhou Zhu, Jie Zhou, Yu Qiao, Xiaogang Wang, Hongsheng Li, Lewei Lu, Jifeng Dai | arXiv 2024 |
18 | MrSteve: Instruction-Following Agents in Minecraft with What-Where-When Memory | Junyeong Park, Junmo Cho, Sungjin Ahn | arXiv 2024 |
19 | Project Sid: Many-agent simulations toward AI civilization | Altera.AL, Andrew Ahn, Nic Becker, Stephanie Carroll, Nico Christie, Manuel Cortes, Arda Demirci, Melissa Du, Frankie Li, Shuying Luo, Peter Y Wang, Mathew Willows, Feitong Yang, Guangyu Robert Yang | arXiv 2024 |
20 | ADAM: An Embodied Causal Agent in Open-World Environments | Shu Yu, Chaochao Lu | arXiv 2024 |
21 | WALL-E: World Alignment by Rule Learning Improves World Model-based LLM Agents | Siyu Zhou, Tianyi Zhou, Yijun Yang, Guodong Long, Deheng Ye, Jing Jiang, Chengqi Zhang | arXiv 2024 |
22 | Optimus-1: Hybrid Multimodal Memory Empowered Agents Excel in Long-Horizon Tasks | Zaijing Li, Yuquan Xie, Rui Shao, Gongwei Chen, Dongmei Jiang, Liqiang Nie | NeurIPS 2024 |
23 | OmniJARVIS: Unified Vision-Language-Action Tokenization Enables Open-World Instruction Following Agents | Zihao Wang, Shaofei Cai, Zhancun Mu, Haowei Lin, Ceyao Zhang, Xuejie Liu, Qing Li, Anji Liu, Xiaojian Ma, Yitao Liang | NeurIPS 2024 |
24 | VillagerAgent: A Graph-Based Multi-Agent Framework for Coordinating Complex Task Dependencies in Minecraft | Yubo Dong, Xukun Zhu, Zhengzhe Pan, Linchao Zhu, Yi Yang | ACL 2024 |
25 | GROOT-2: Weakly Supervised Multi-Modal Instruction Following Agents | Shaofei Cai, Bowei Zhang, Zihao Wang, Haowei Lin, Xiaojian Ma, Anji Liu, Yitao Liang | arXiv 2024 |
If you find this work useful for your research, please cite our paper:
@article{Odyssey2024,
title={Odyssey: Empowering Agents with Open-World Skills},
author={Shunyu Liu and Yaoru Li and Kongcheng Zhang and Zhenyu Cui and Wenkai Fang and Yuxuan Zheng and Tongya Zheng and Mingli Song},
journal={arXiv preprint arXiv:2407.15325},
year={2024}
}
Component | License |
---|---|
Codebase | MIT License |
Minecraft Q&A Dataset | Creative Commons Attribution Non Commercial Share Alike 3.0 Unported (CC BY-NC-SA 3.0) |
This project is developed by VIPA Lab from Zhejiang University. Please feel free to contact me via email ([email protected]) if you are interested in our research :)
For Tasks:
Click tags to check more tools for each tasksFor Jobs:
Alternative AI tools for Odyssey
Similar Open Source Tools
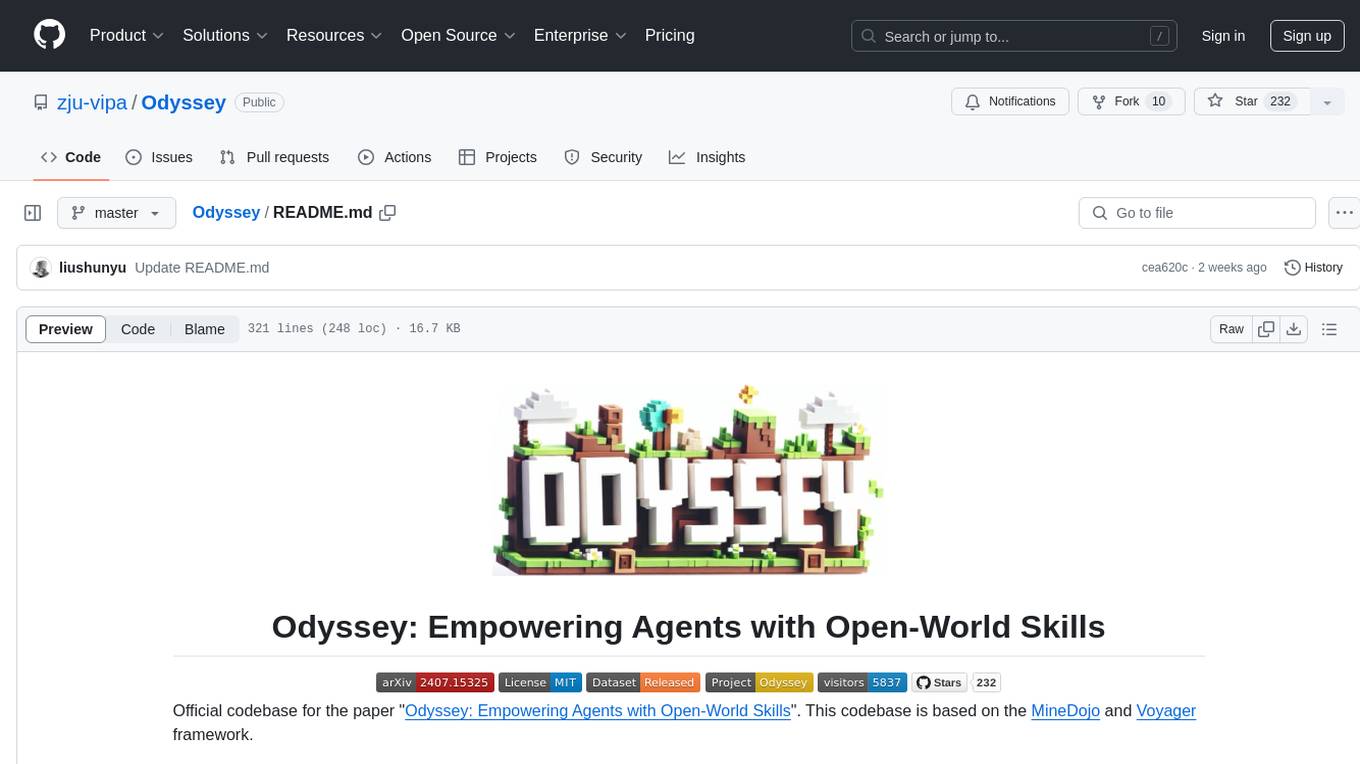
Odyssey
Odyssey is a framework designed to empower agents with open-world skills in Minecraft. It provides an interactive agent with a skill library, a fine-tuned LLaMA-3 model, and an open-world benchmark for evaluating agent capabilities. The framework enables agents to explore diverse gameplay opportunities in the vast Minecraft world by offering primitive and compositional skills, extensive training data, and various long-term planning tasks. Odyssey aims to advance research on autonomous agent solutions by providing datasets, model weights, and code for public use.
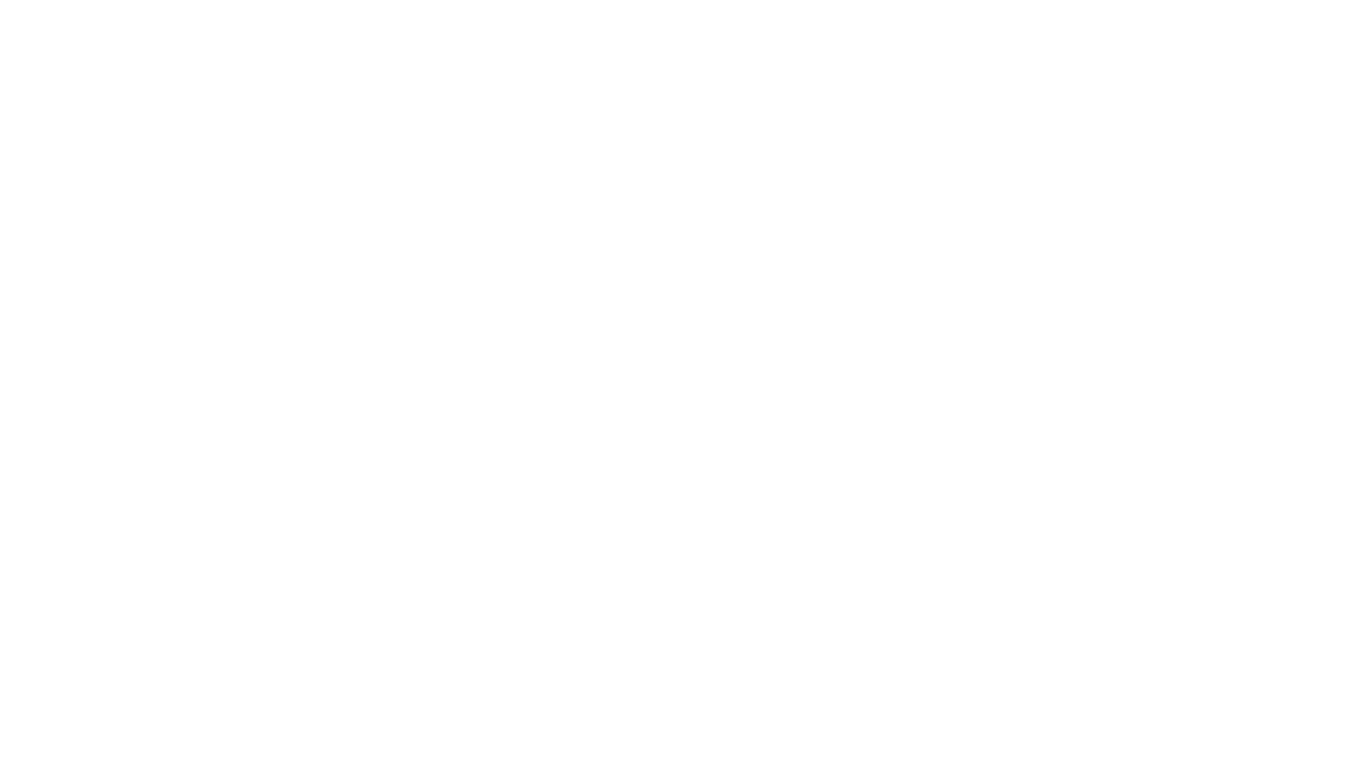
PIXIU
PIXIU is a project designed to support the development, fine-tuning, and evaluation of Large Language Models (LLMs) in the financial domain. It includes components like FinBen, a Financial Language Understanding and Prediction Evaluation Benchmark, FIT, a Financial Instruction Dataset, and FinMA, a Financial Large Language Model. The project provides open resources, multi-task and multi-modal financial data, and diverse financial tasks for training and evaluation. It aims to encourage open research and transparency in the financial NLP field.
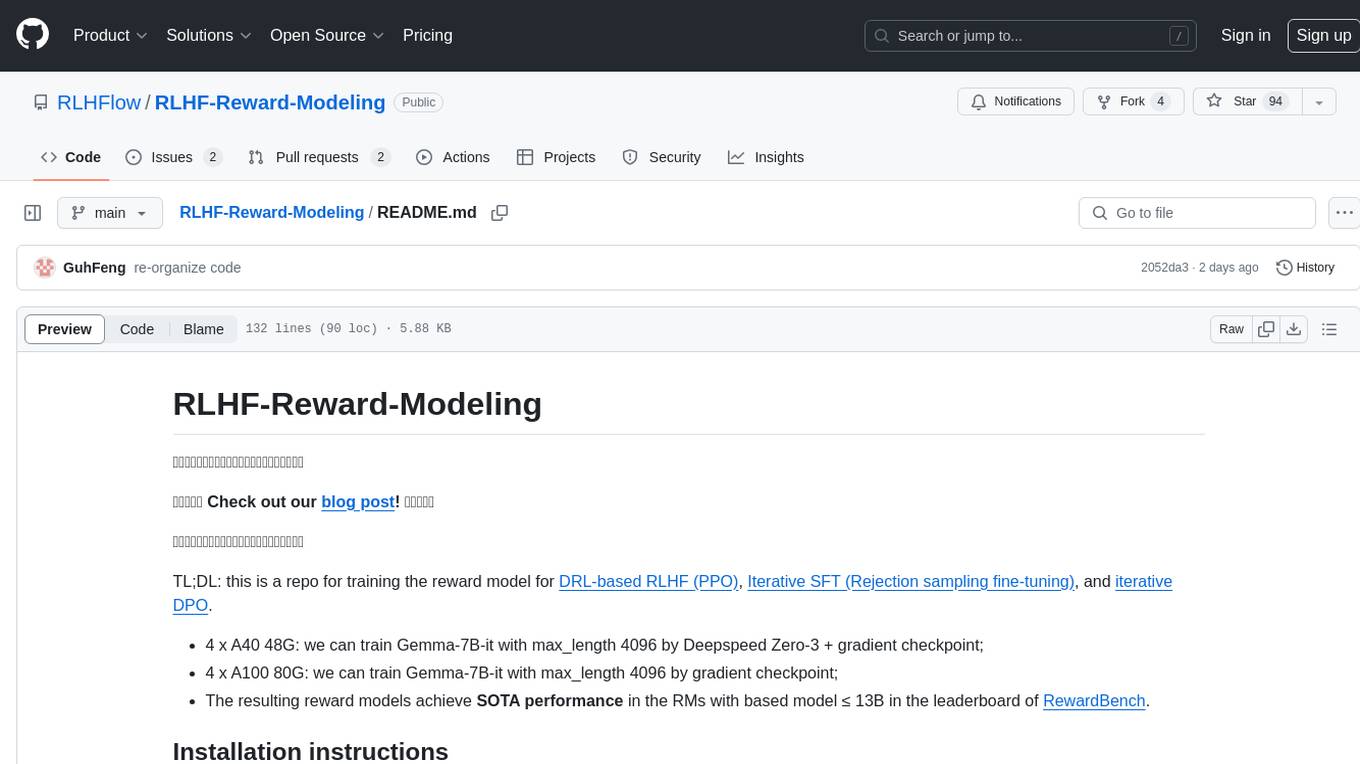
RLHF-Reward-Modeling
This repository, RLHF-Reward-Modeling, is dedicated to training reward models for DRL-based RLHF (PPO), Iterative SFT, and iterative DPO. It provides state-of-the-art performance in reward models with a base model size of up to 13B. The installation instructions involve setting up the environment and aligning the handbook. Dataset preparation requires preprocessing conversations into a standard format. The code can be run with Gemma-2b-it, and evaluation results can be obtained using provided datasets. The to-do list includes various reward models like Bradley-Terry, preference model, regression-based reward model, and multi-objective reward model. The repository is part of iterative rejection sampling fine-tuning and iterative DPO.
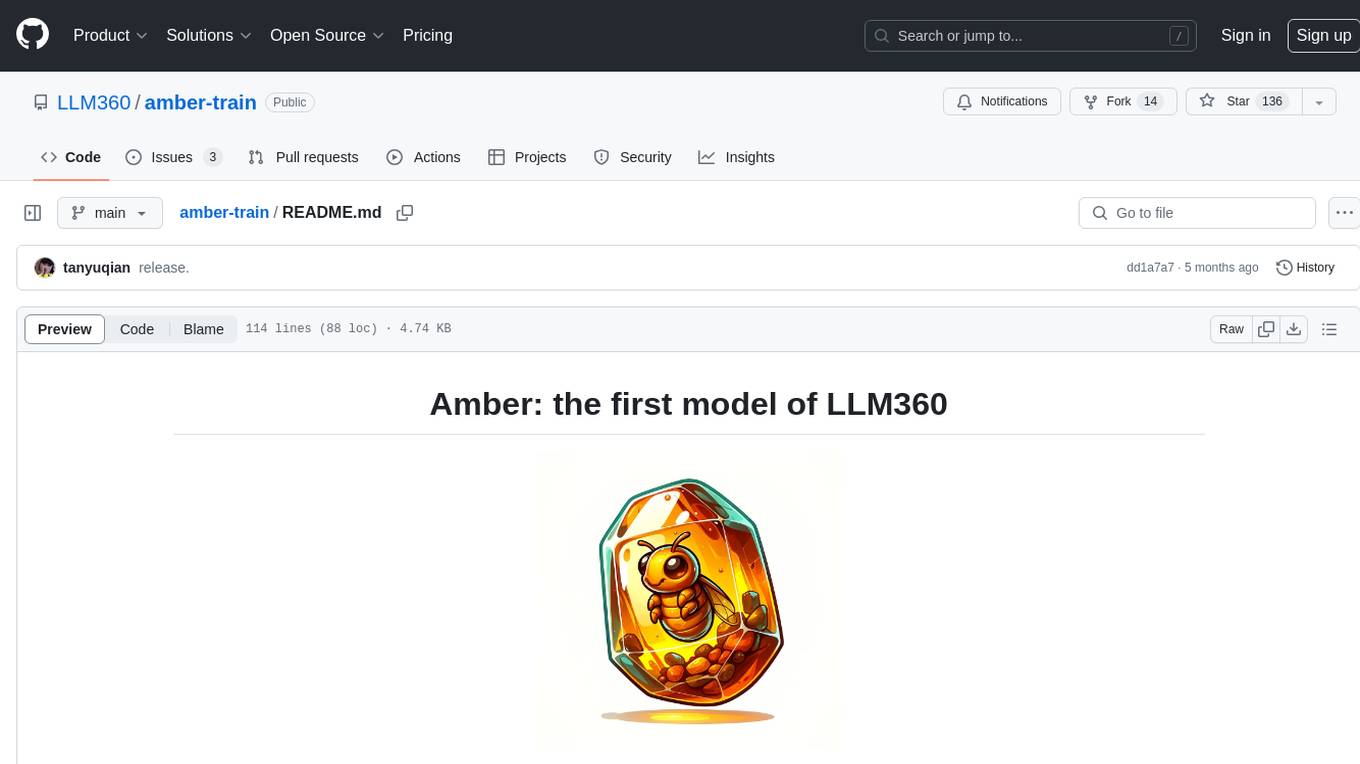
amber-train
Amber is the first model in the LLM360 family, an initiative for comprehensive and fully open-sourced LLMs. It is a 7B English language model with the LLaMA architecture. The model type is a language model with the same architecture as LLaMA-7B. It is licensed under Apache 2.0. The resources available include training code, data preparation, metrics, and fully processed Amber pretraining data. The model has been trained on various datasets like Arxiv, Book, C4, Refined-Web, StarCoder, StackExchange, and Wikipedia. The hyperparameters include a total of 6.7B parameters, hidden size of 4096, intermediate size of 11008, 32 attention heads, 32 hidden layers, RMSNorm ε of 1e^-6, max sequence length of 2048, and a vocabulary size of 32000.
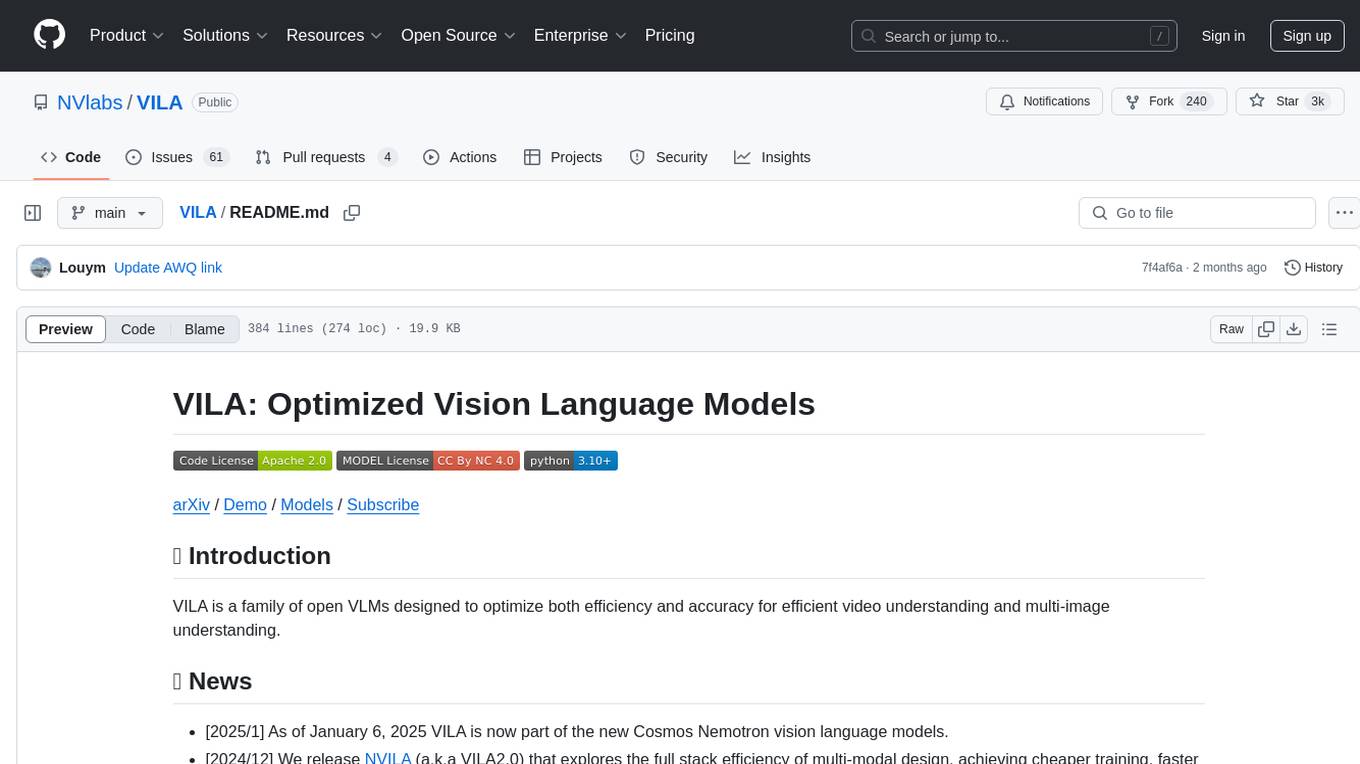
VILA
VILA is a family of open Vision Language Models optimized for efficient video understanding and multi-image understanding. It includes models like NVILA, LongVILA, VILA-M3, VILA-U, and VILA-1.5, each offering specific features and capabilities. The project focuses on efficiency, accuracy, and performance in various tasks related to video, image, and language understanding and generation. VILA models are designed to be deployable on diverse NVIDIA GPUs and support long-context video understanding, medical applications, and multi-modal design.
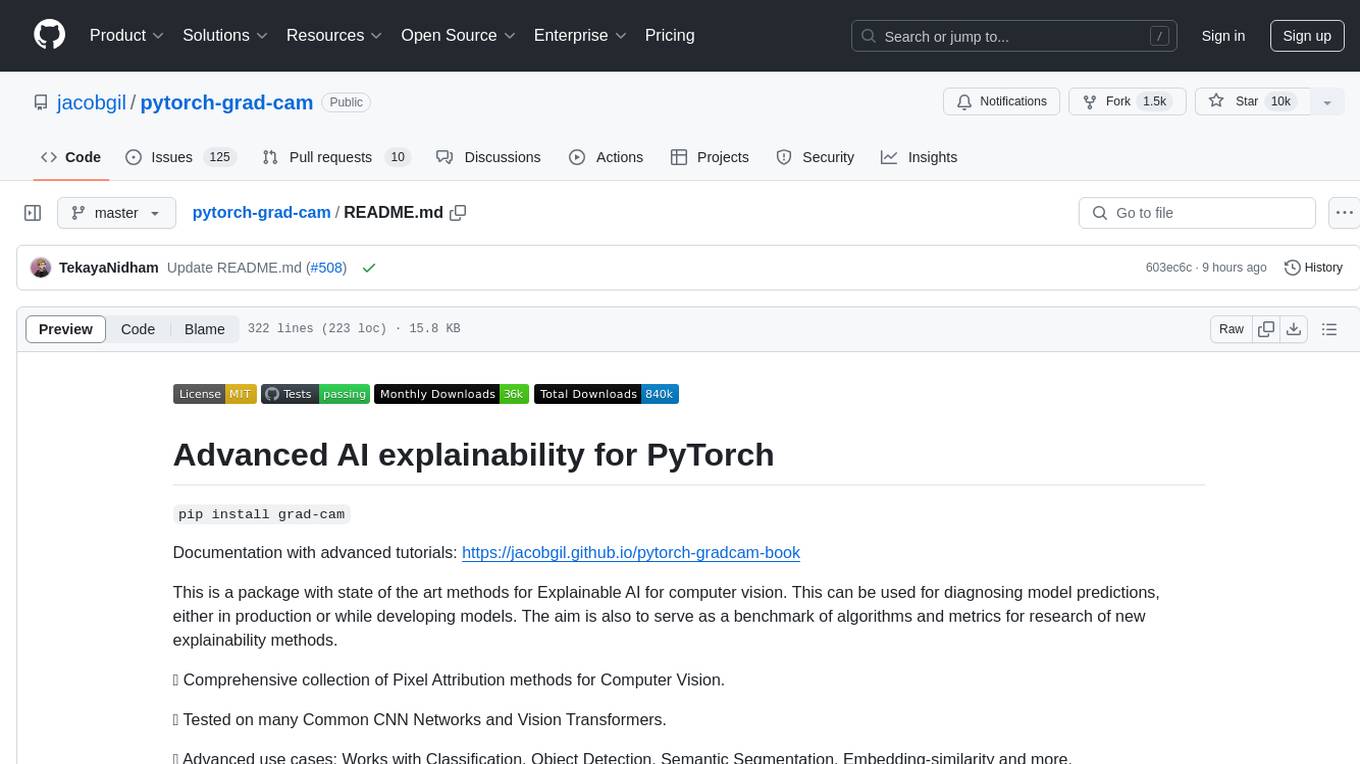
pytorch-grad-cam
This repository provides advanced AI explainability for PyTorch, offering state-of-the-art methods for Explainable AI in computer vision. It includes a comprehensive collection of Pixel Attribution methods for various tasks like Classification, Object Detection, Semantic Segmentation, and more. The package supports high performance with full batch image support and includes metrics for evaluating and tuning explanations. Users can visualize and interpret model predictions, making it suitable for both production and model development scenarios.
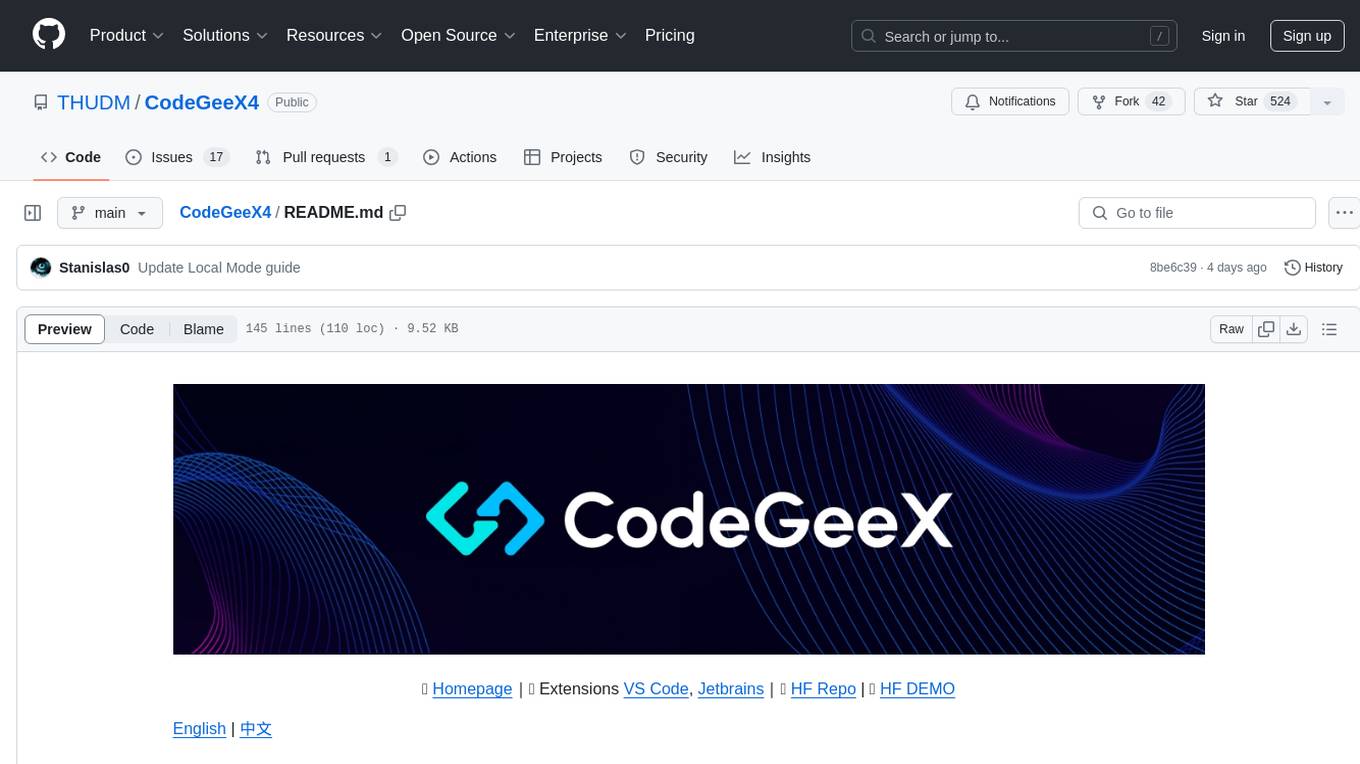
CodeGeeX4
CodeGeeX4-ALL-9B is an open-source multilingual code generation model based on GLM-4-9B, offering enhanced code generation capabilities. It supports functions like code completion, code interpreter, web search, function call, and repository-level code Q&A. The model has competitive performance on benchmarks like BigCodeBench and NaturalCodeBench, outperforming larger models in terms of speed and performance.
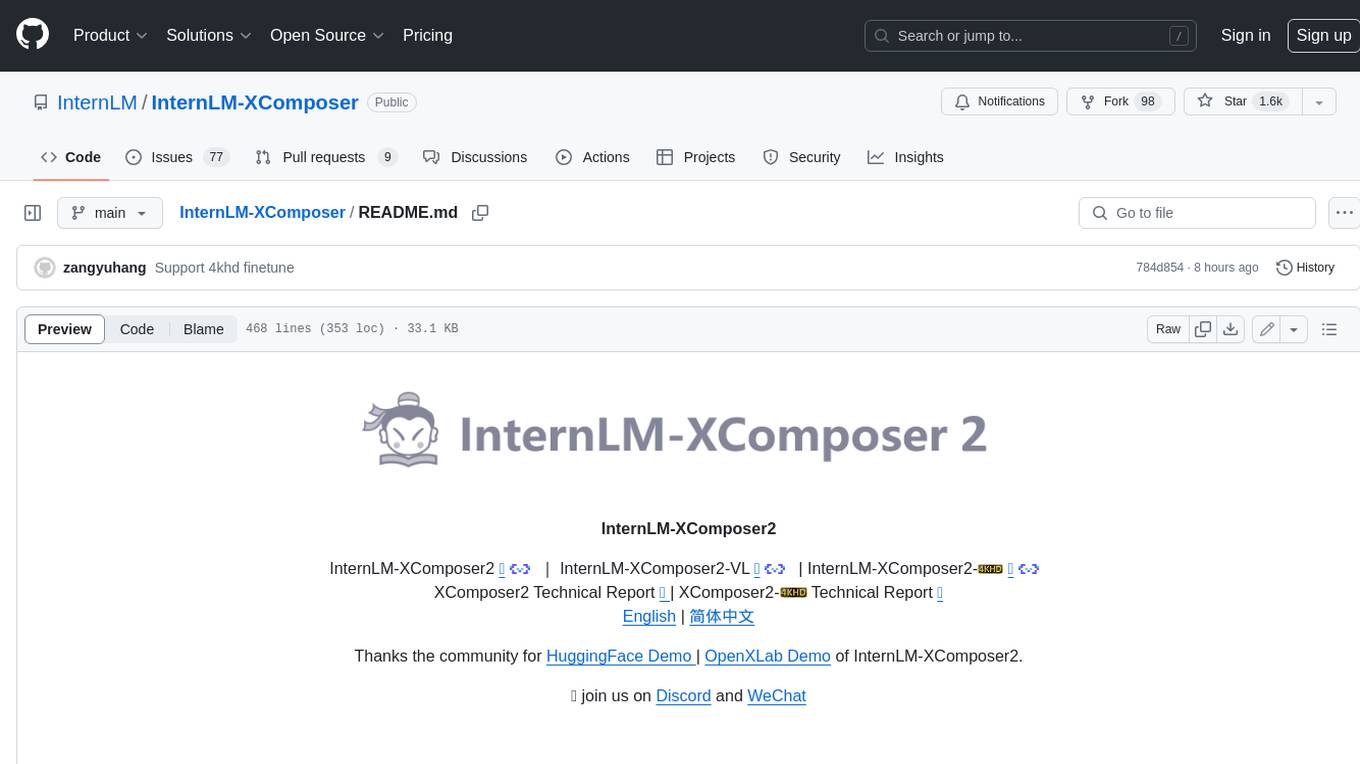
InternLM-XComposer
InternLM-XComposer2 is a groundbreaking vision-language large model (VLLM) based on InternLM2-7B excelling in free-form text-image composition and comprehension. It boasts several amazing capabilities and applications: * **Free-form Interleaved Text-Image Composition** : InternLM-XComposer2 can effortlessly generate coherent and contextual articles with interleaved images following diverse inputs like outlines, detailed text requirements and reference images, enabling highly customizable content creation. * **Accurate Vision-language Problem-solving** : InternLM-XComposer2 accurately handles diverse and challenging vision-language Q&A tasks based on free-form instructions, excelling in recognition, perception, detailed captioning, visual reasoning, and more. * **Awesome performance** : InternLM-XComposer2 based on InternLM2-7B not only significantly outperforms existing open-source multimodal models in 13 benchmarks but also **matches or even surpasses GPT-4V and Gemini Pro in 6 benchmarks** We release InternLM-XComposer2 series in three versions: * **InternLM-XComposer2-4KHD-7B** 🤗: The high-resolution multi-task trained VLLM model with InternLM-7B as the initialization of the LLM for _High-resolution understanding_ , _VL benchmarks_ and _AI assistant_. * **InternLM-XComposer2-VL-7B** 🤗 : The multi-task trained VLLM model with InternLM-7B as the initialization of the LLM for _VL benchmarks_ and _AI assistant_. **It ranks as the most powerful vision-language model based on 7B-parameter level LLMs, leading across 13 benchmarks.** * **InternLM-XComposer2-VL-1.8B** 🤗 : A lightweight version of InternLM-XComposer2-VL based on InternLM-1.8B. * **InternLM-XComposer2-7B** 🤗: The further instruction tuned VLLM for _Interleaved Text-Image Composition_ with free-form inputs. Please refer to Technical Report and 4KHD Technical Reportfor more details.
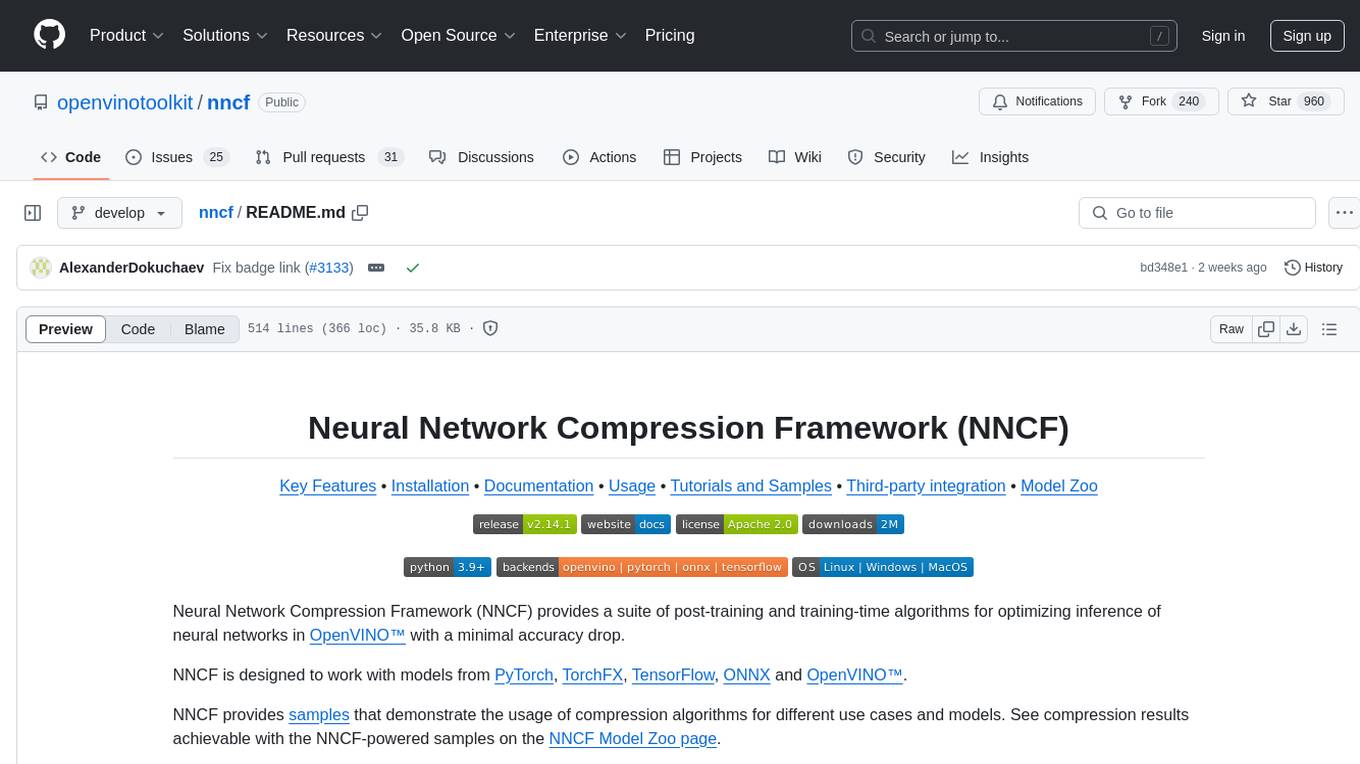
nncf
Neural Network Compression Framework (NNCF) provides a suite of post-training and training-time algorithms for optimizing inference of neural networks in OpenVINOâ„¢ with a minimal accuracy drop. It is designed to work with models from PyTorch, TorchFX, TensorFlow, ONNX, and OpenVINOâ„¢. NNCF offers samples demonstrating compression algorithms for various use cases and models, with the ability to add different compression algorithms easily. It supports GPU-accelerated layers, distributed training, and seamless combination of pruning, sparsity, and quantization algorithms. NNCF allows exporting compressed models to ONNX or TensorFlow formats for use with OpenVINOâ„¢ toolkit, and supports Accuracy-Aware model training pipelines via Adaptive Compression Level Training and Early Exit Training.
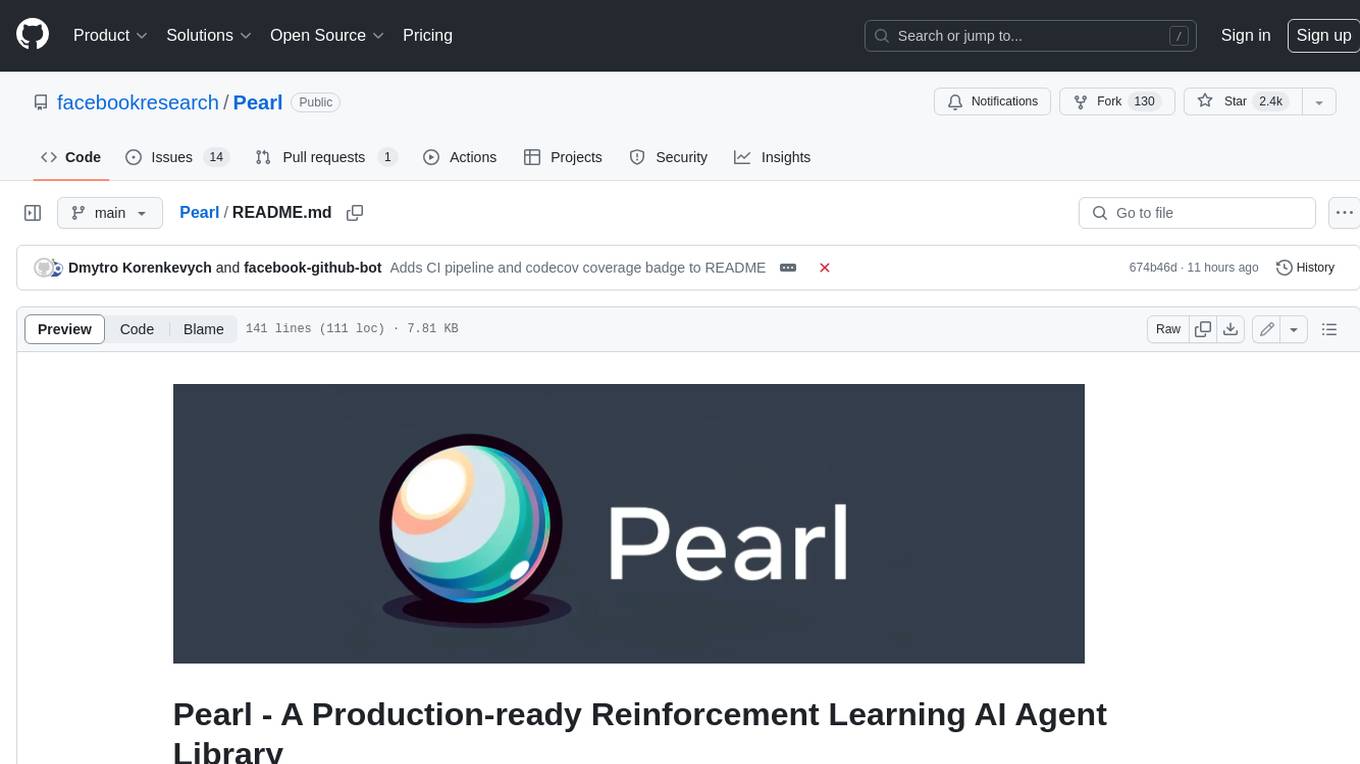
Pearl
Pearl is a production-ready Reinforcement Learning AI agent library open-sourced by the Applied Reinforcement Learning team at Meta. It enables researchers and practitioners to develop Reinforcement Learning AI agents that prioritize cumulative long-term feedback over immediate feedback and can adapt to environments with limited observability, sparse feedback, and high stochasticity. Pearl offers a diverse set of unique features for production environments, including dynamic action spaces, offline learning, intelligent neural exploration, safe decision making, history summarization, and data augmentation.
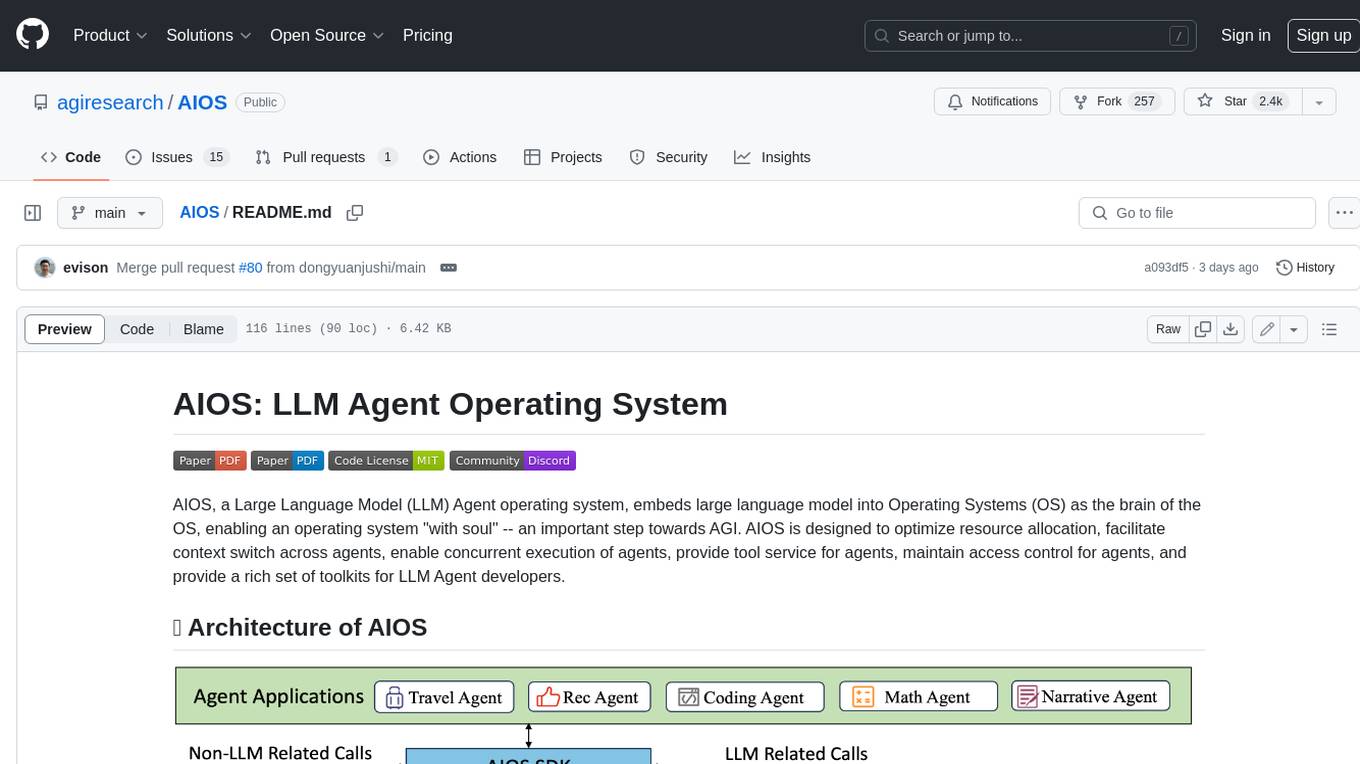
AIOS
AIOS, a Large Language Model (LLM) Agent operating system, embeds large language model into Operating Systems (OS) as the brain of the OS, enabling an operating system "with soul" -- an important step towards AGI. AIOS is designed to optimize resource allocation, facilitate context switch across agents, enable concurrent execution of agents, provide tool service for agents, maintain access control for agents, and provide a rich set of toolkits for LLM Agent developers.
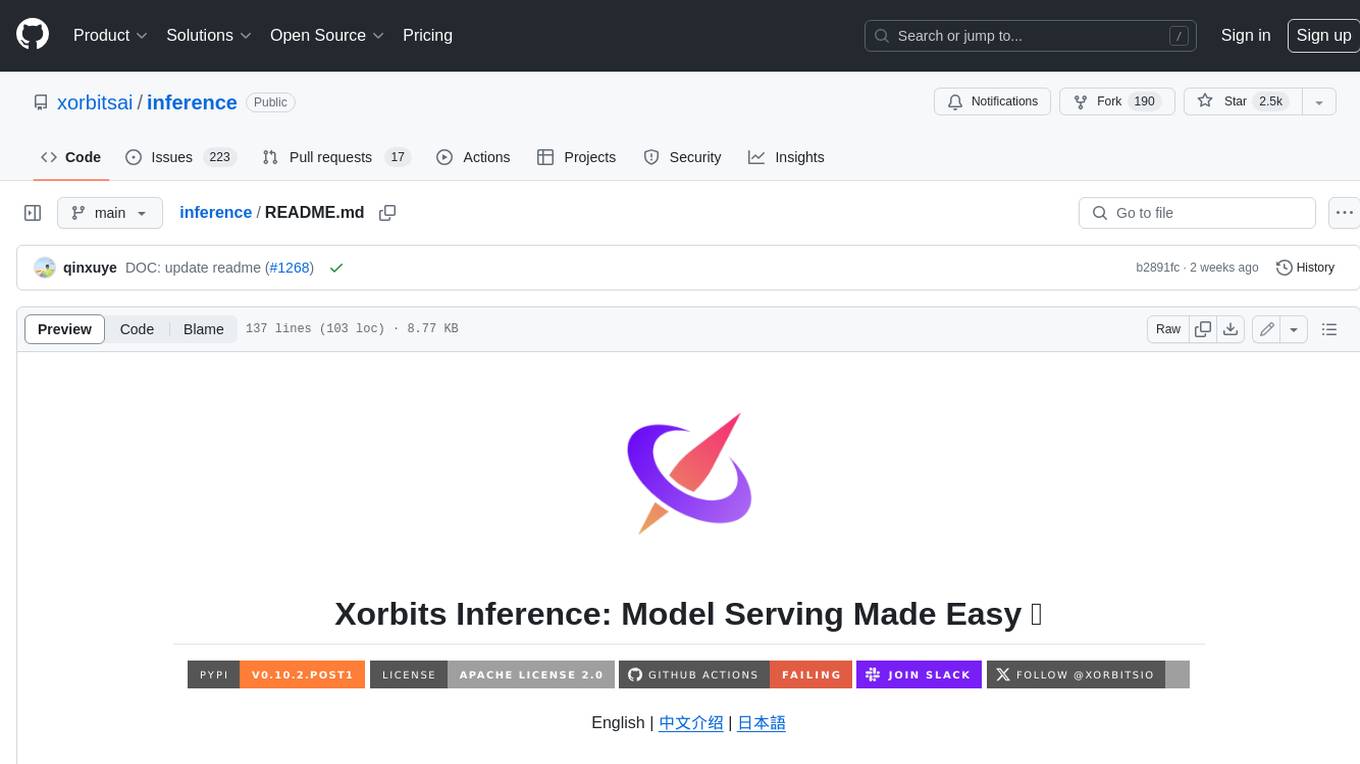
inference
Xorbits Inference (Xinference) is a powerful and versatile library designed to serve language, speech recognition, and multimodal models. With Xorbits Inference, you can effortlessly deploy and serve your or state-of-the-art built-in models using just a single command. Whether you are a researcher, developer, or data scientist, Xorbits Inference empowers you to unleash the full potential of cutting-edge AI models.
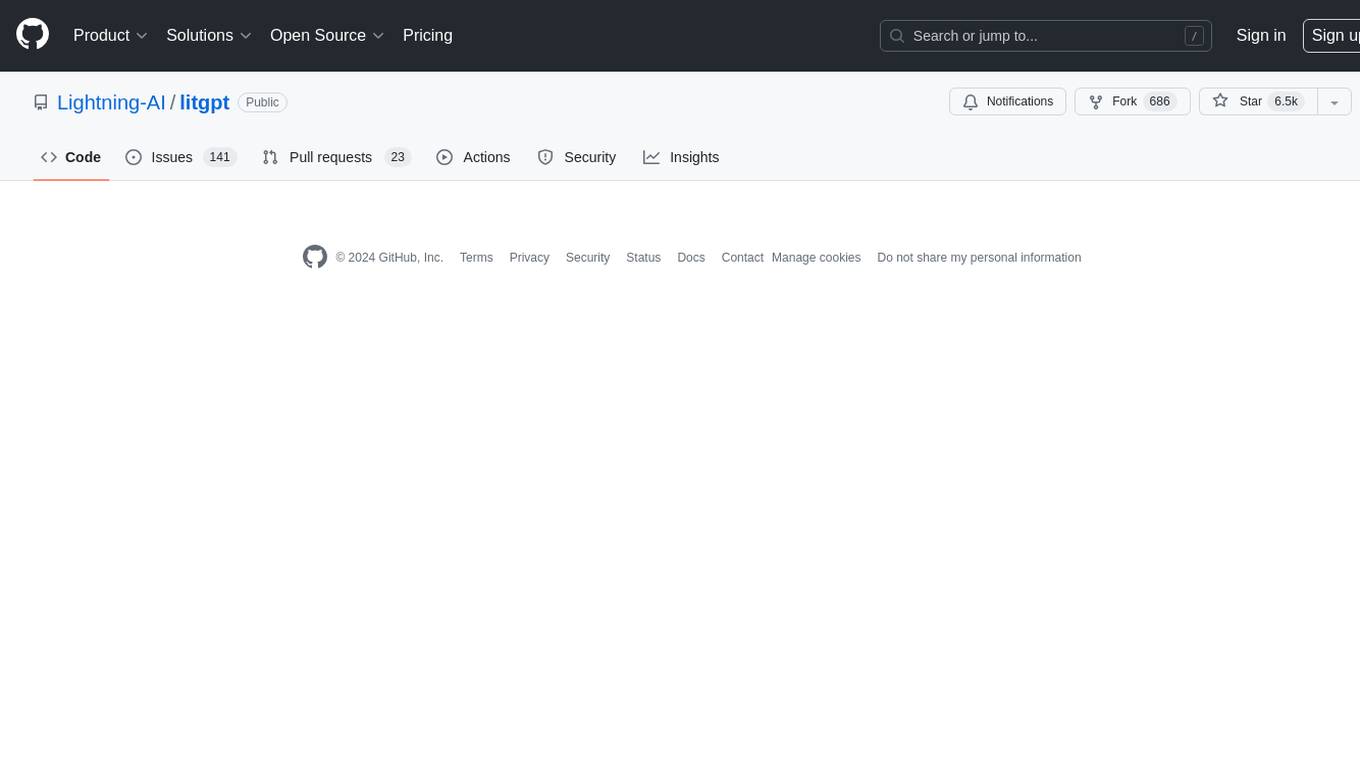
litgpt
LitGPT is a command-line tool designed to easily finetune, pretrain, evaluate, and deploy 20+ LLMs **on your own data**. It features highly-optimized training recipes for the world's most powerful open-source large-language-models (LLMs).
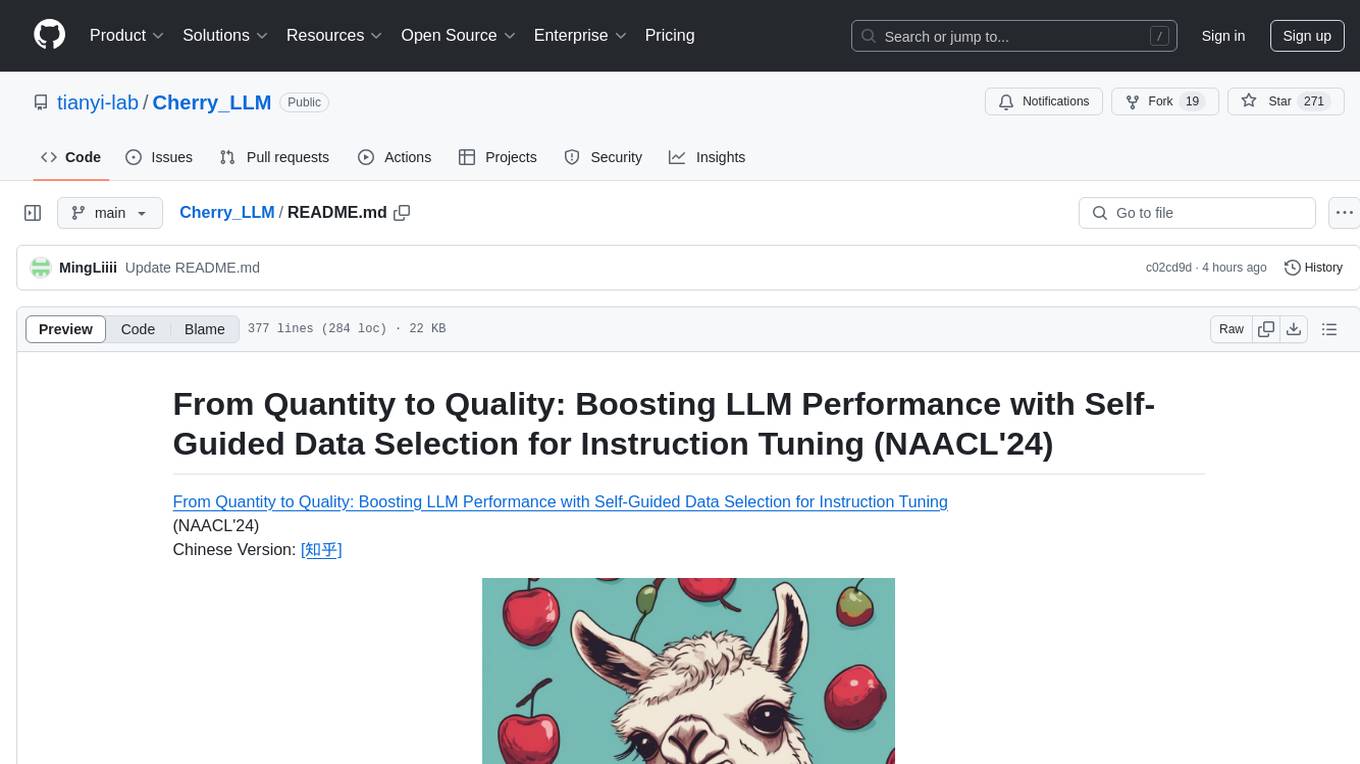
Cherry_LLM
Cherry Data Selection project introduces a self-guided methodology for LLMs to autonomously discern and select cherry samples from open-source datasets, minimizing manual curation and cost for instruction tuning. The project focuses on selecting impactful training samples ('cherry data') to enhance LLM instruction tuning by estimating instruction-following difficulty. The method involves phases like 'Learning from Brief Experience', 'Evaluating Based on Experience', and 'Retraining from Self-Guided Experience' to improve LLM performance.
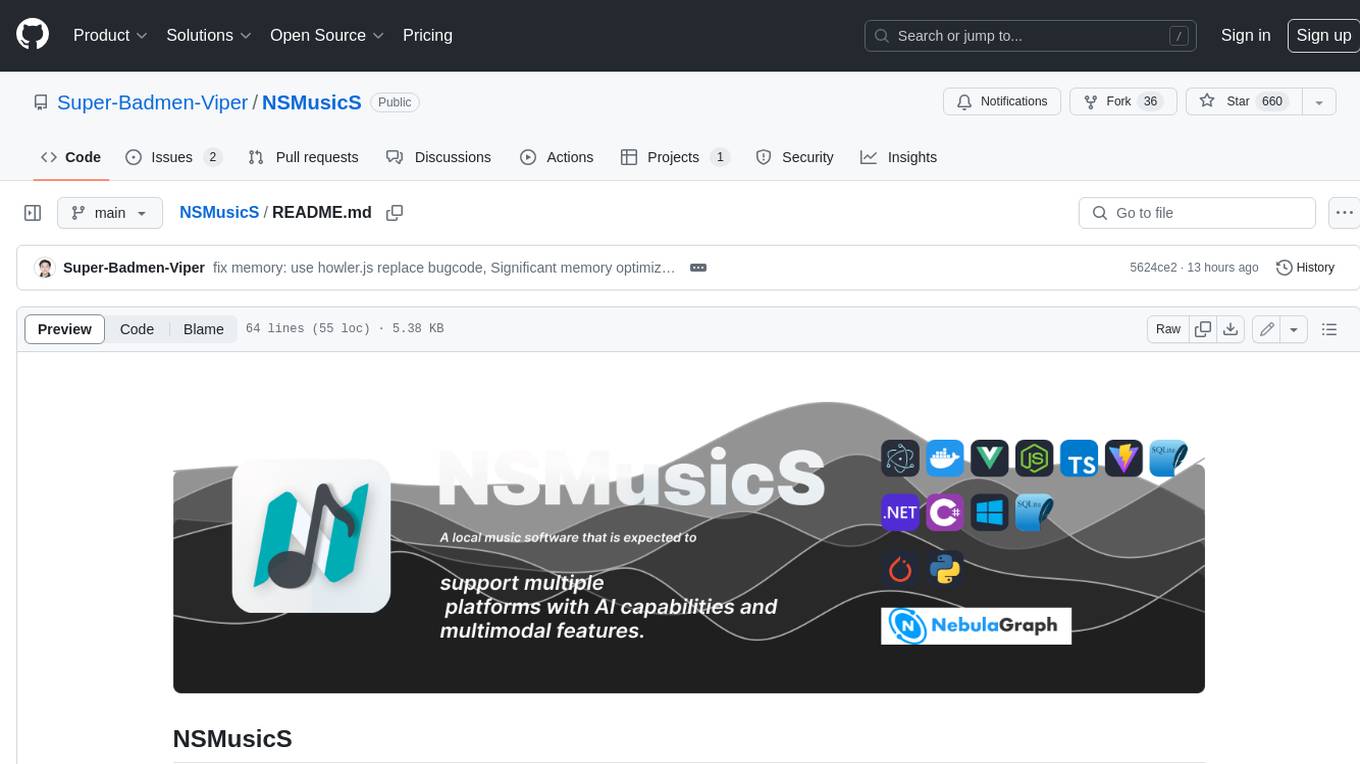
NSMusicS
NSMusicS is a local music software that is expected to support multiple platforms with AI capabilities and multimodal features. The goal of NSMusicS is to integrate various functions (such as artificial intelligence, streaming, music library management, cross platform, etc.), which can be understood as similar to Navidrome but with more features than Navidrome. It wants to become a plugin integrated application that can almost have all music functions.
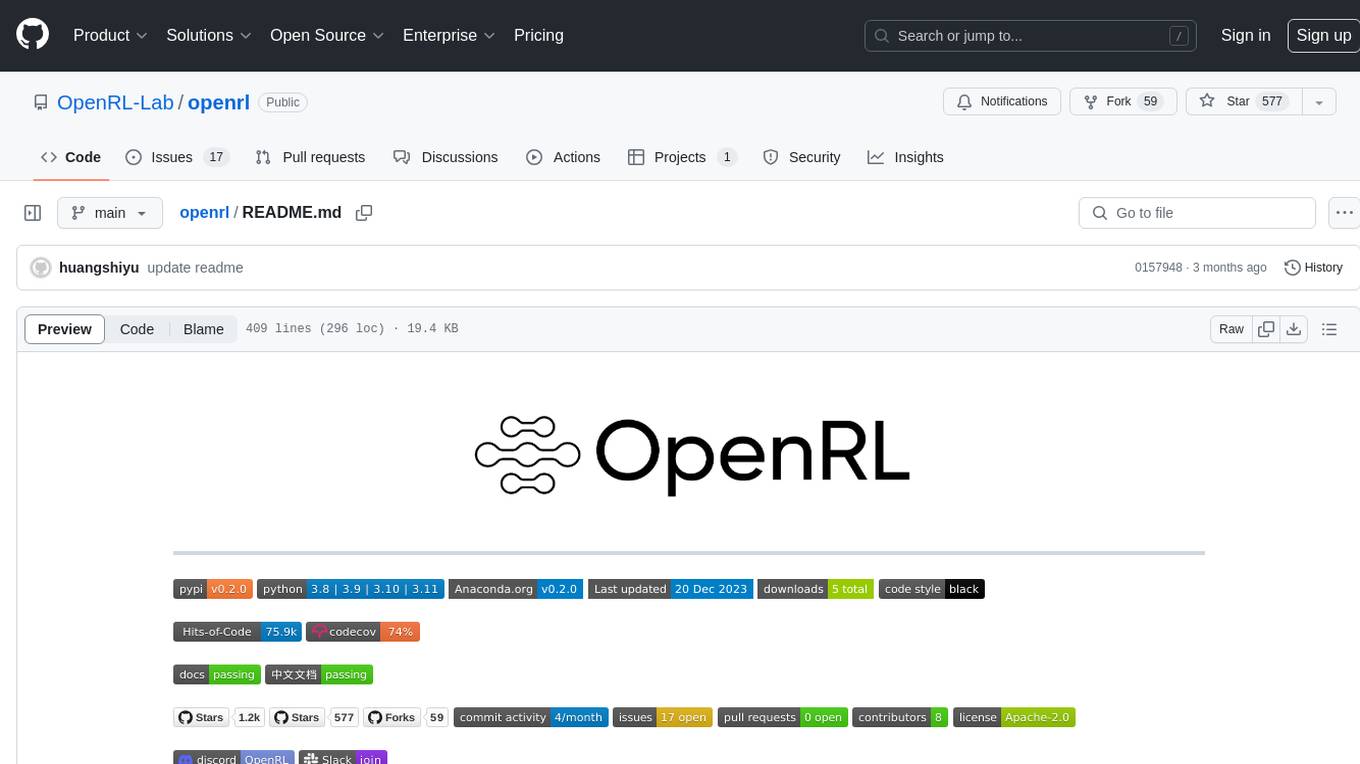
openrl
OpenRL is an open-source general reinforcement learning research framework that supports training for various tasks such as single-agent, multi-agent, offline RL, self-play, and natural language. Developed based on PyTorch, the goal of OpenRL is to provide a simple-to-use, flexible, efficient and sustainable platform for the reinforcement learning research community. It supports a universal interface for all tasks/environments, single-agent and multi-agent tasks, offline RL training with expert dataset, self-play training, reinforcement learning training for natural language tasks, DeepSpeed, Arena for evaluation, importing models and datasets from Hugging Face, user-defined environments, models, and datasets, gymnasium environments, callbacks, visualization tools, unit testing, and code coverage testing. It also supports various algorithms like PPO, DQN, SAC, and environments like Gymnasium, MuJoCo, Atari, and more.
For similar tasks
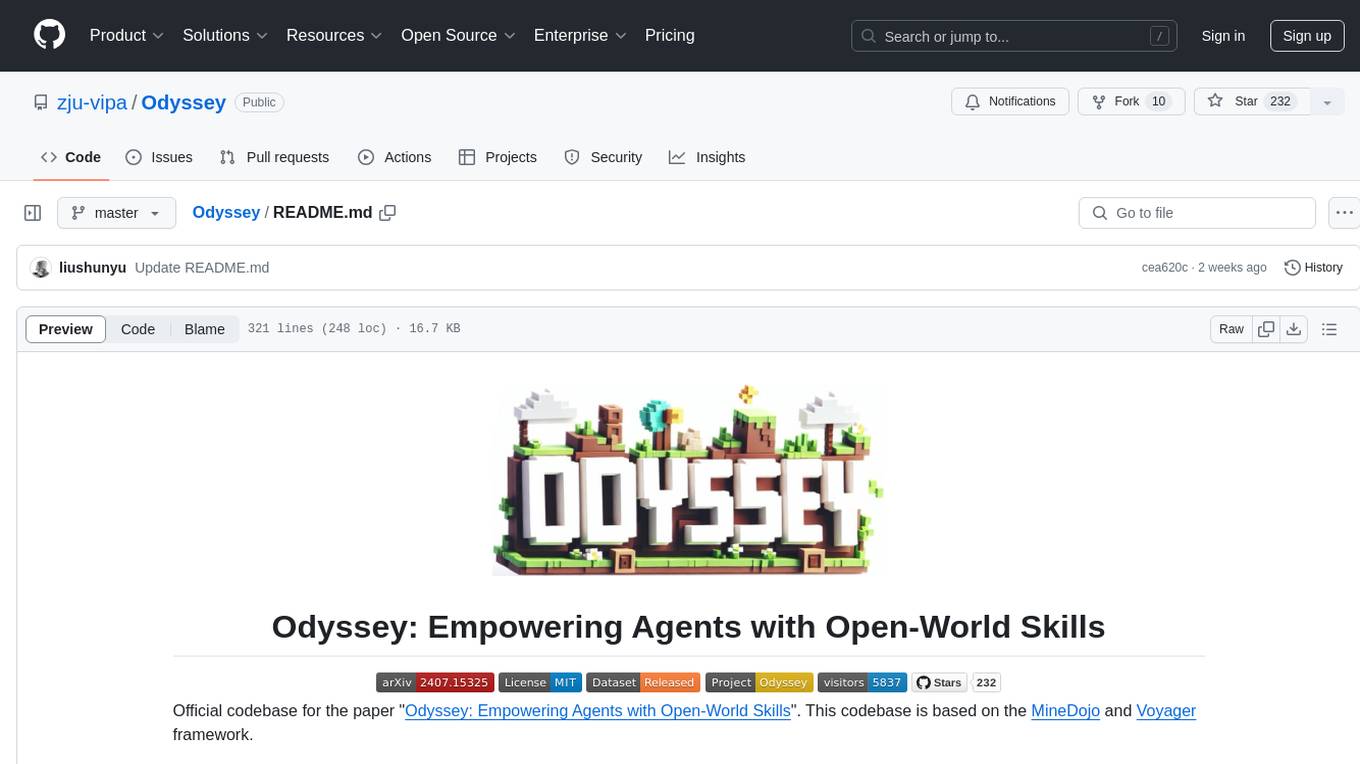
Odyssey
Odyssey is a framework designed to empower agents with open-world skills in Minecraft. It provides an interactive agent with a skill library, a fine-tuned LLaMA-3 model, and an open-world benchmark for evaluating agent capabilities. The framework enables agents to explore diverse gameplay opportunities in the vast Minecraft world by offering primitive and compositional skills, extensive training data, and various long-term planning tasks. Odyssey aims to advance research on autonomous agent solutions by providing datasets, model weights, and code for public use.
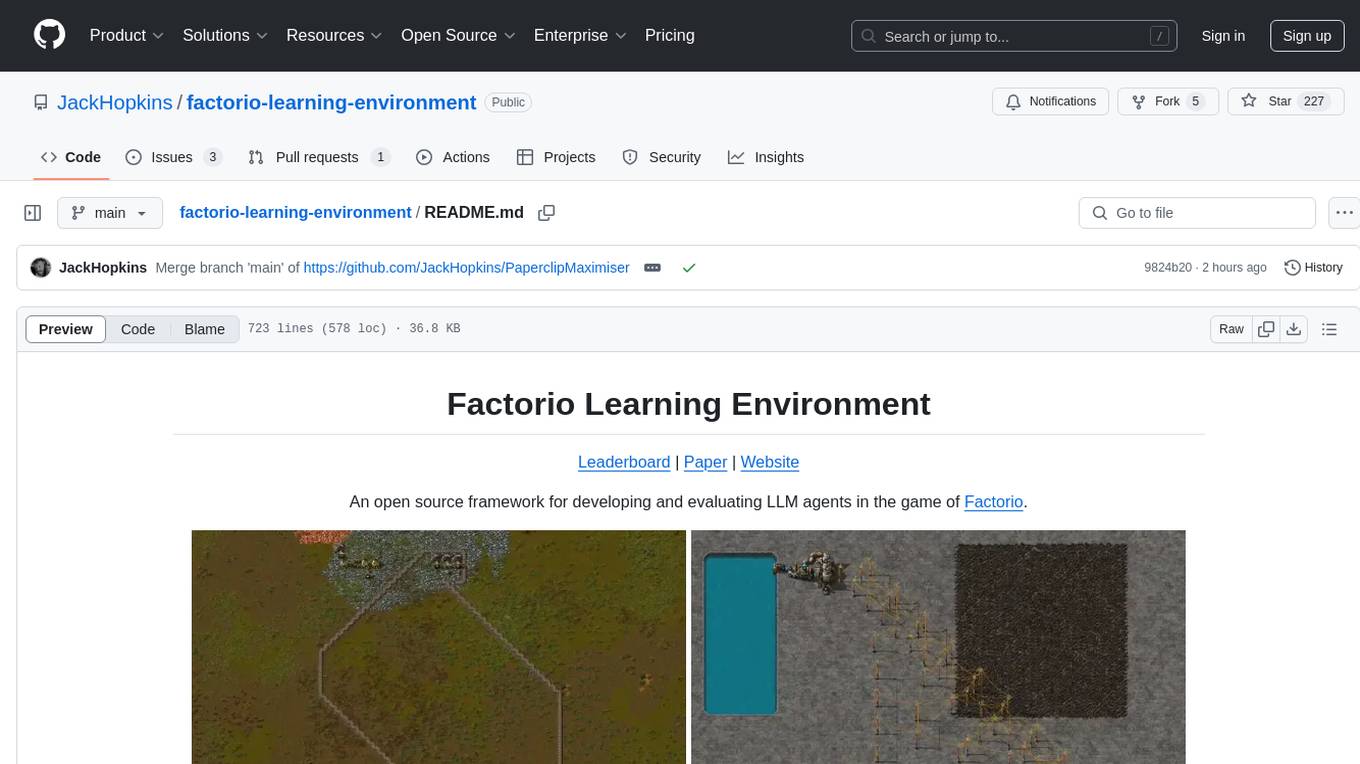
factorio-learning-environment
Factorio Learning Environment is an open source framework designed for developing and evaluating LLM agents in the game of Factorio. It provides two settings: Lab-play with structured tasks and Open-play for building large factories. Results show limitations in spatial reasoning and automation strategies. Agents interact with the environment through code synthesis, observation, action, and feedback. Tools are provided for game actions and state representation. Agents operate in episodes with observation, planning, and action execution. Tasks specify agent goals and are implemented in JSON files. The project structure includes directories for agents, environment, cluster, data, docs, eval, and more. A database is used for checkpointing agent steps. Benchmarks show performance metrics for different configurations.
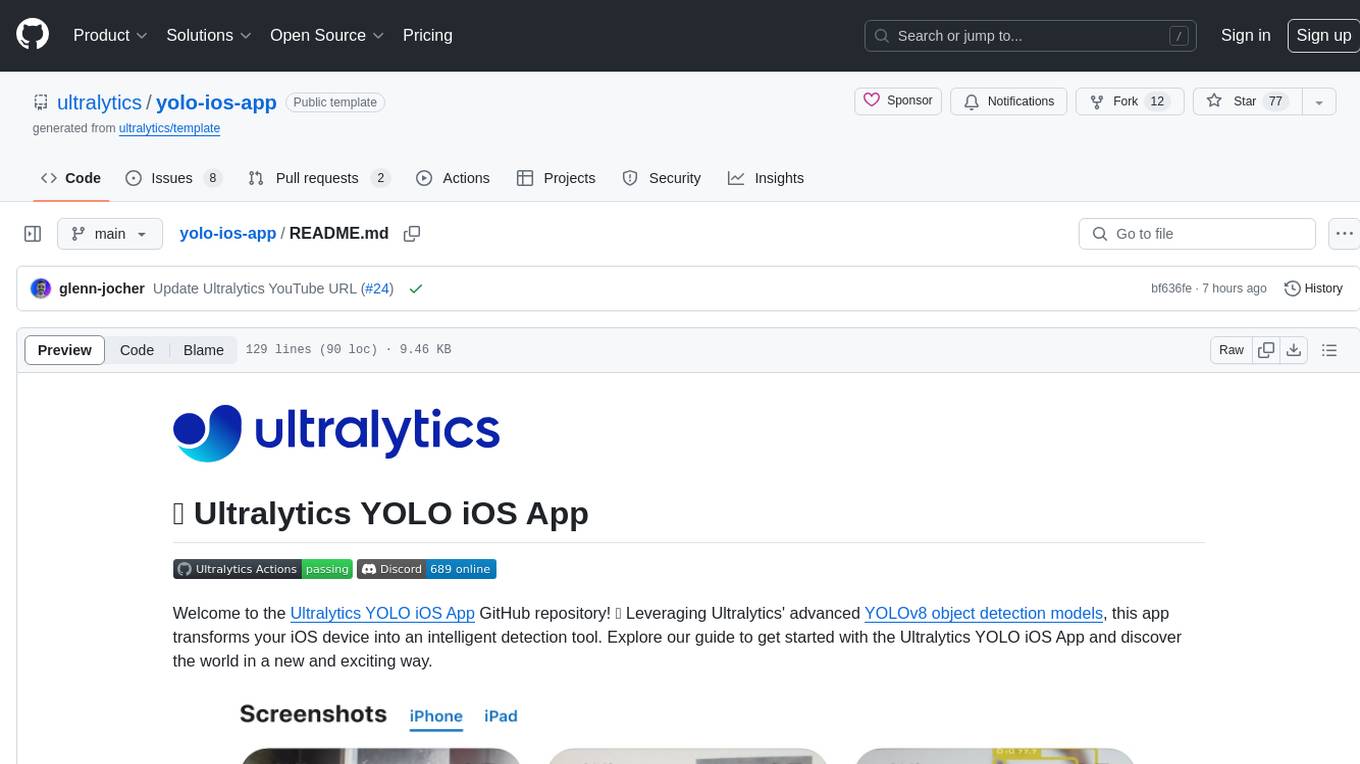
yolo-ios-app
The Ultralytics YOLO iOS App GitHub repository offers an advanced object detection tool leveraging YOLOv8 models for iOS devices. Users can transform their devices into intelligent detection tools to explore the world in a new and exciting way. The app provides real-time detection capabilities with multiple AI models to choose from, ranging from 'nano' to 'x-large'. Contributors are welcome to participate in this open-source project, and licensing options include AGPL-3.0 for open-source use and an Enterprise License for commercial integration. Users can easily set up the app by following the provided steps, including cloning the repository, adding YOLOv8 models, and running the app on their iOS devices.
For similar jobs
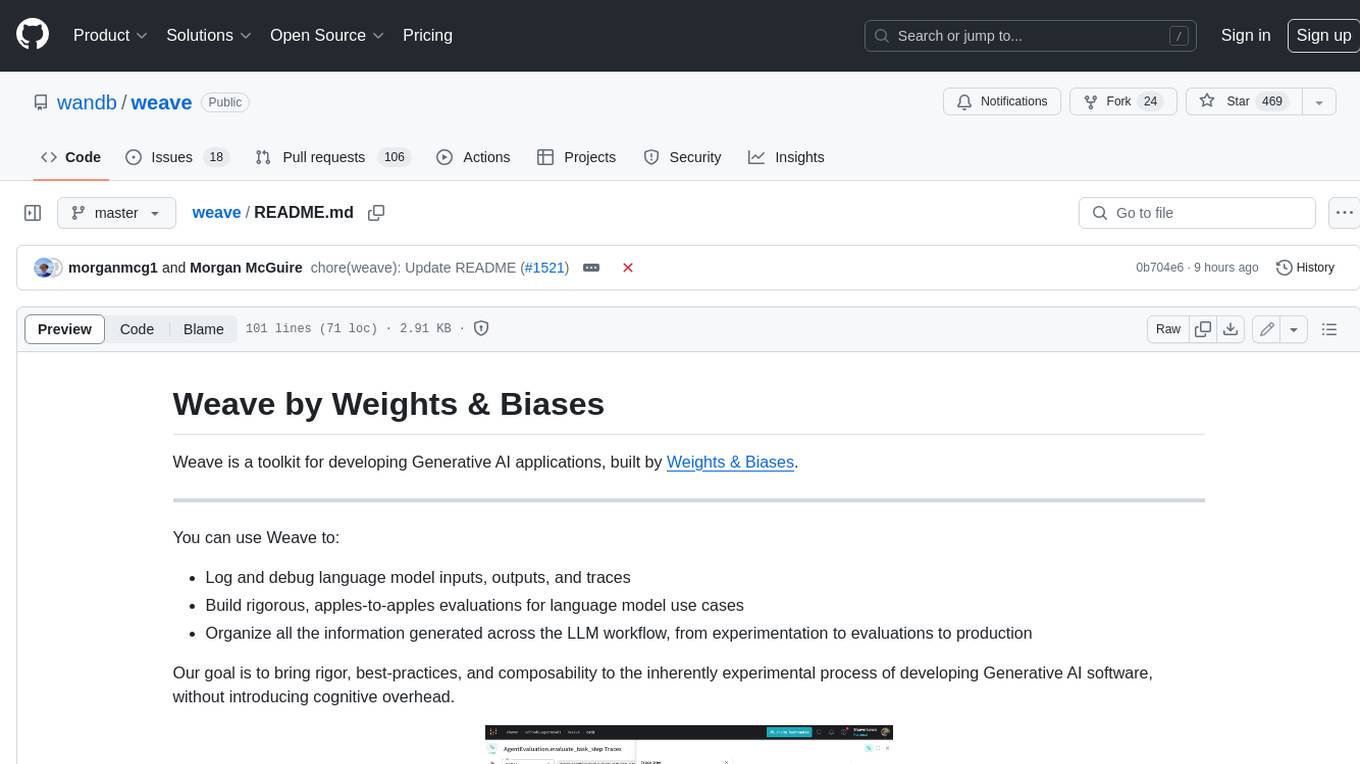
weave
Weave is a toolkit for developing Generative AI applications, built by Weights & Biases. With Weave, you can log and debug language model inputs, outputs, and traces; build rigorous, apples-to-apples evaluations for language model use cases; and organize all the information generated across the LLM workflow, from experimentation to evaluations to production. Weave aims to bring rigor, best-practices, and composability to the inherently experimental process of developing Generative AI software, without introducing cognitive overhead.
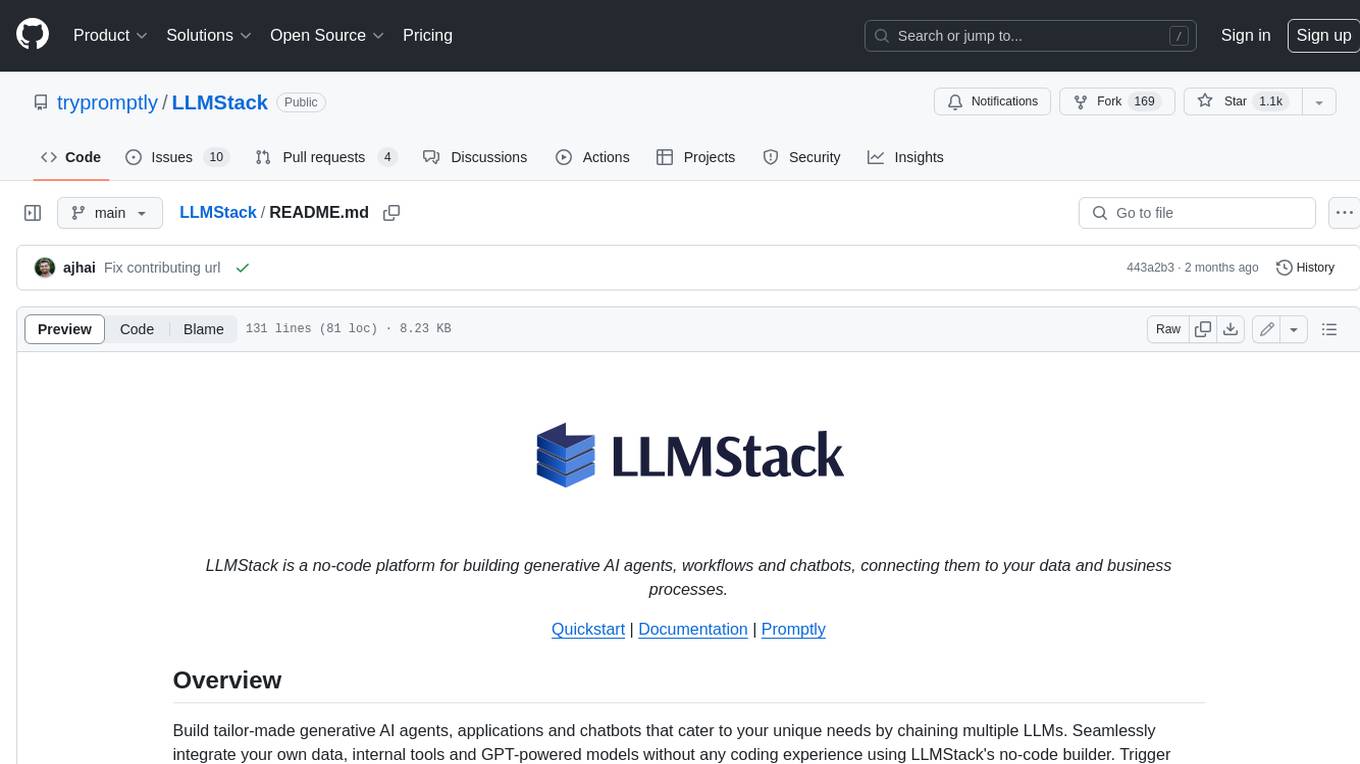
LLMStack
LLMStack is a no-code platform for building generative AI agents, workflows, and chatbots. It allows users to connect their own data, internal tools, and GPT-powered models without any coding experience. LLMStack can be deployed to the cloud or on-premise and can be accessed via HTTP API or triggered from Slack or Discord.
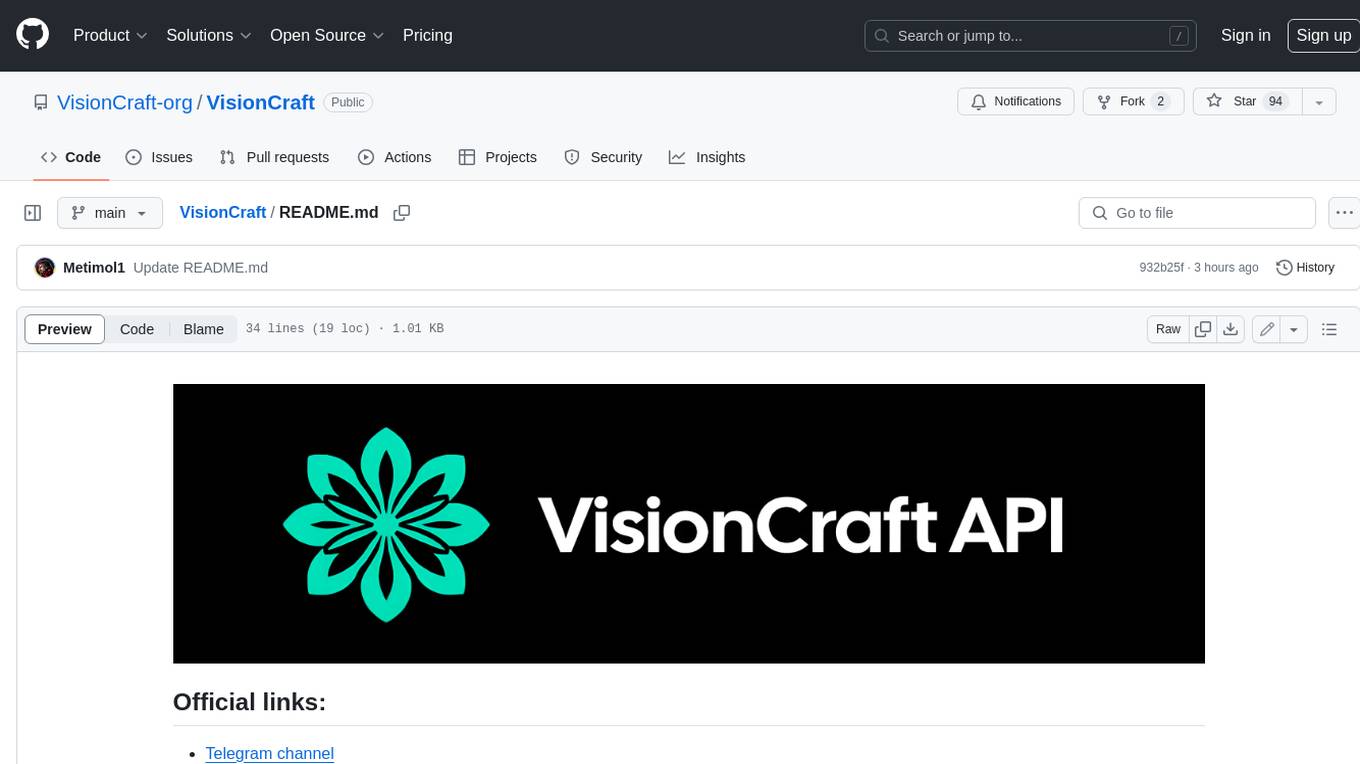
VisionCraft
The VisionCraft API is a free API for using over 100 different AI models. From images to sound.
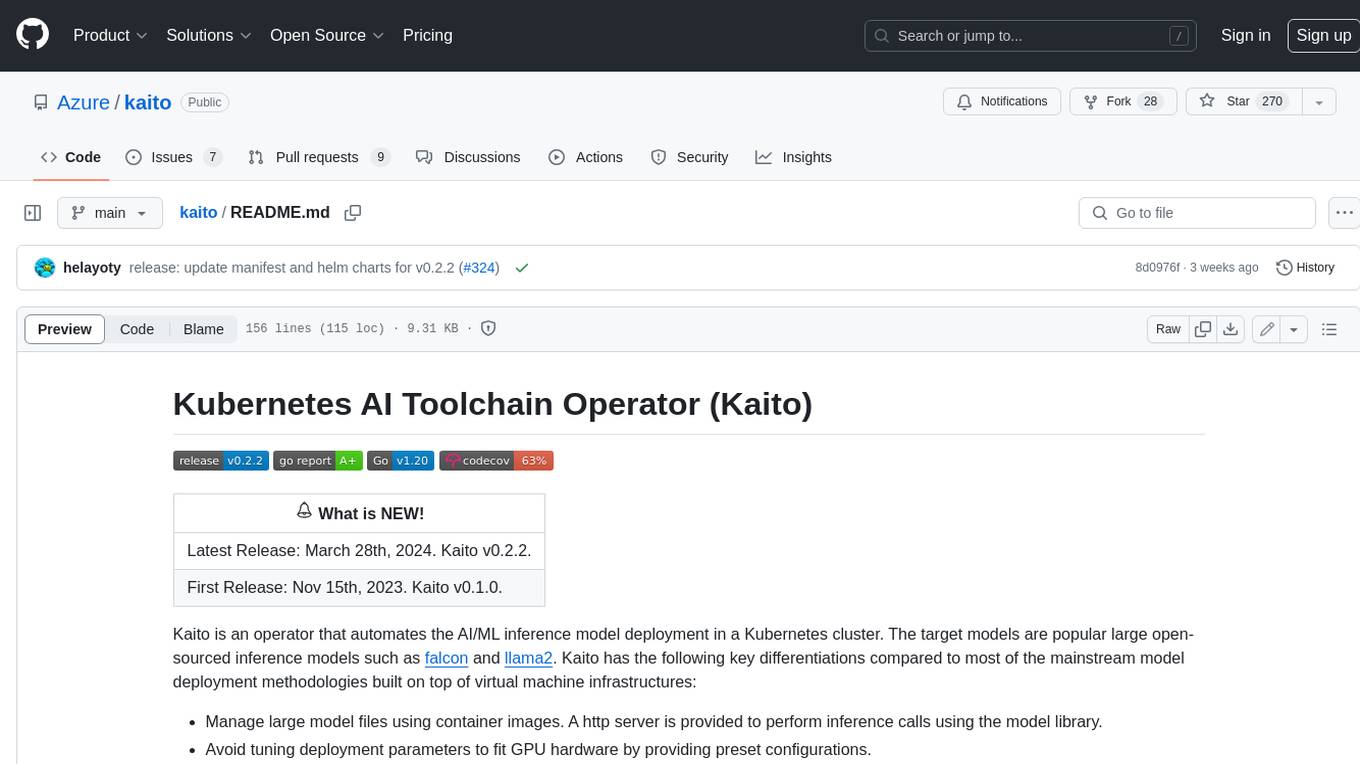
kaito
Kaito is an operator that automates the AI/ML inference model deployment in a Kubernetes cluster. It manages large model files using container images, avoids tuning deployment parameters to fit GPU hardware by providing preset configurations, auto-provisions GPU nodes based on model requirements, and hosts large model images in the public Microsoft Container Registry (MCR) if the license allows. Using Kaito, the workflow of onboarding large AI inference models in Kubernetes is largely simplified.
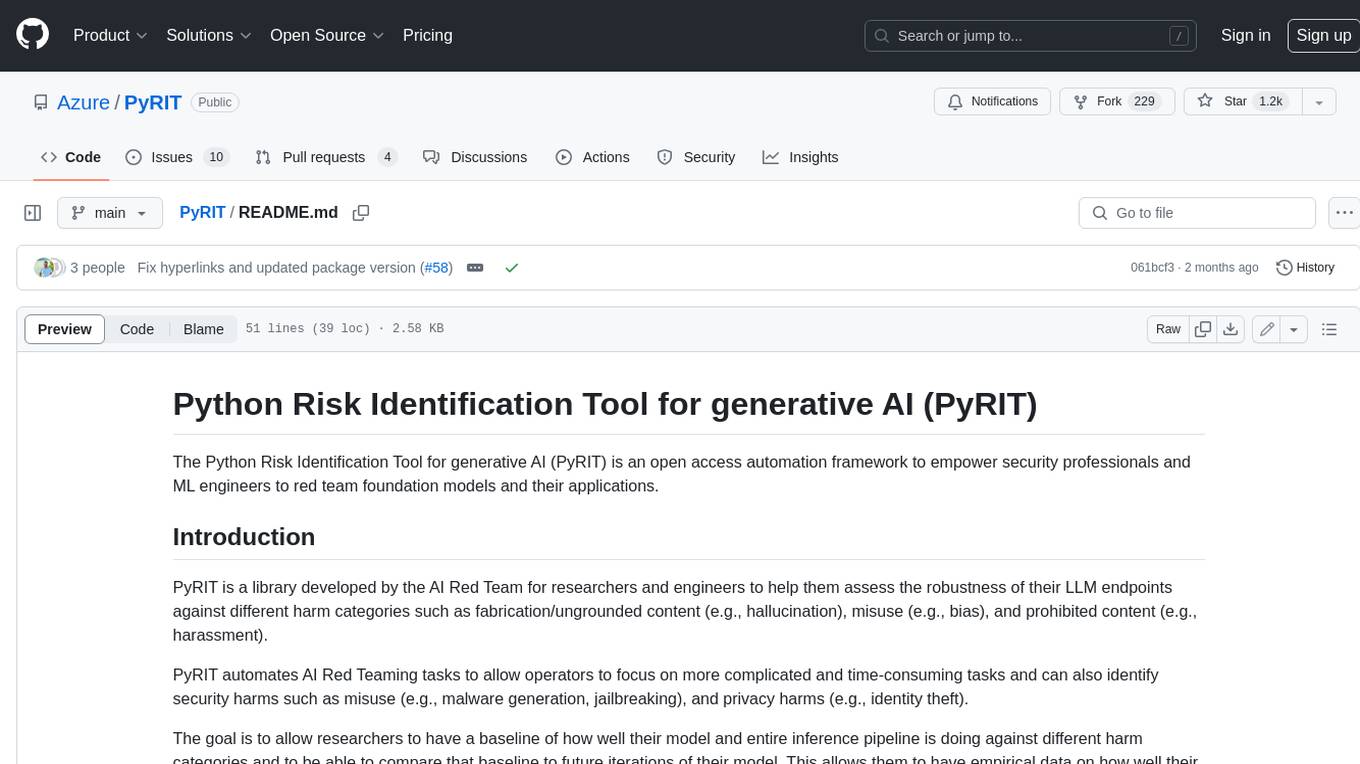
PyRIT
PyRIT is an open access automation framework designed to empower security professionals and ML engineers to red team foundation models and their applications. It automates AI Red Teaming tasks to allow operators to focus on more complicated and time-consuming tasks and can also identify security harms such as misuse (e.g., malware generation, jailbreaking), and privacy harms (e.g., identity theft). The goal is to allow researchers to have a baseline of how well their model and entire inference pipeline is doing against different harm categories and to be able to compare that baseline to future iterations of their model. This allows them to have empirical data on how well their model is doing today, and detect any degradation of performance based on future improvements.
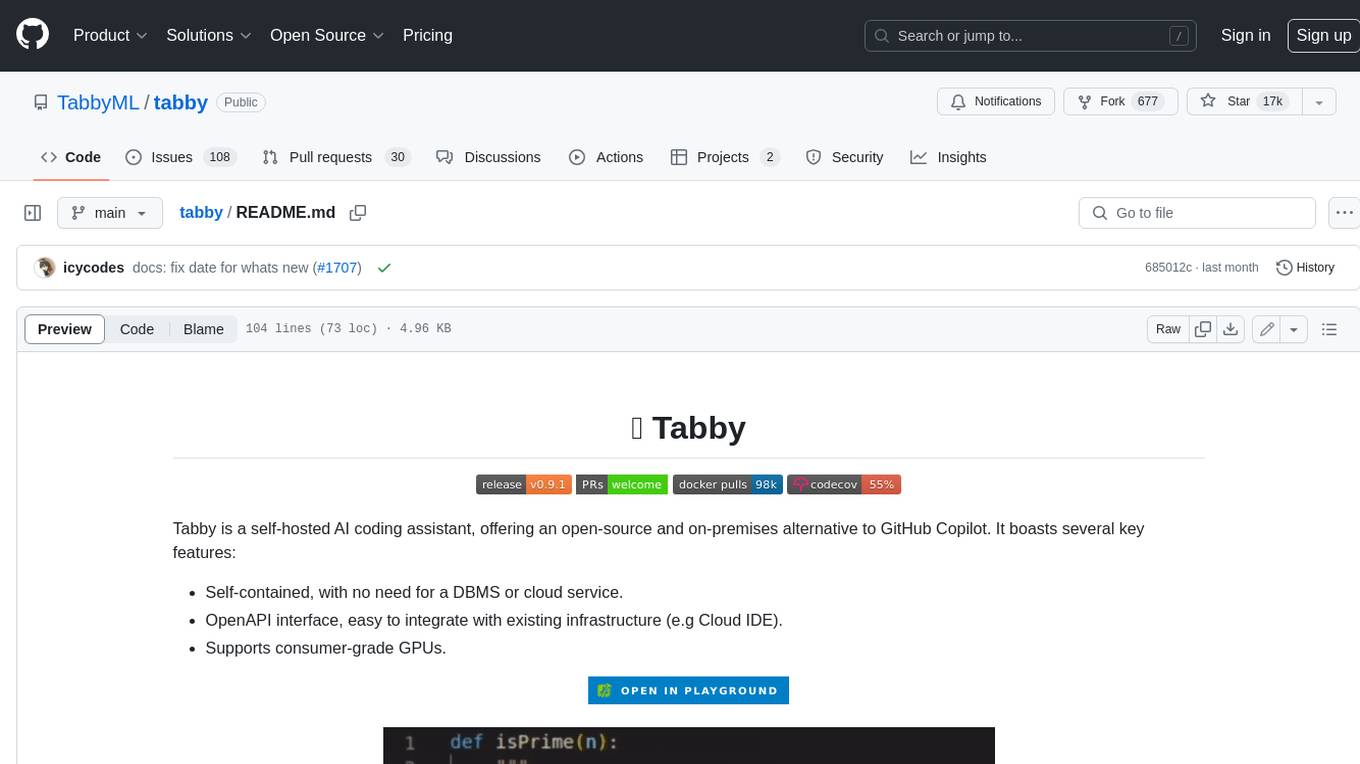
tabby
Tabby is a self-hosted AI coding assistant, offering an open-source and on-premises alternative to GitHub Copilot. It boasts several key features: * Self-contained, with no need for a DBMS or cloud service. * OpenAPI interface, easy to integrate with existing infrastructure (e.g Cloud IDE). * Supports consumer-grade GPUs.
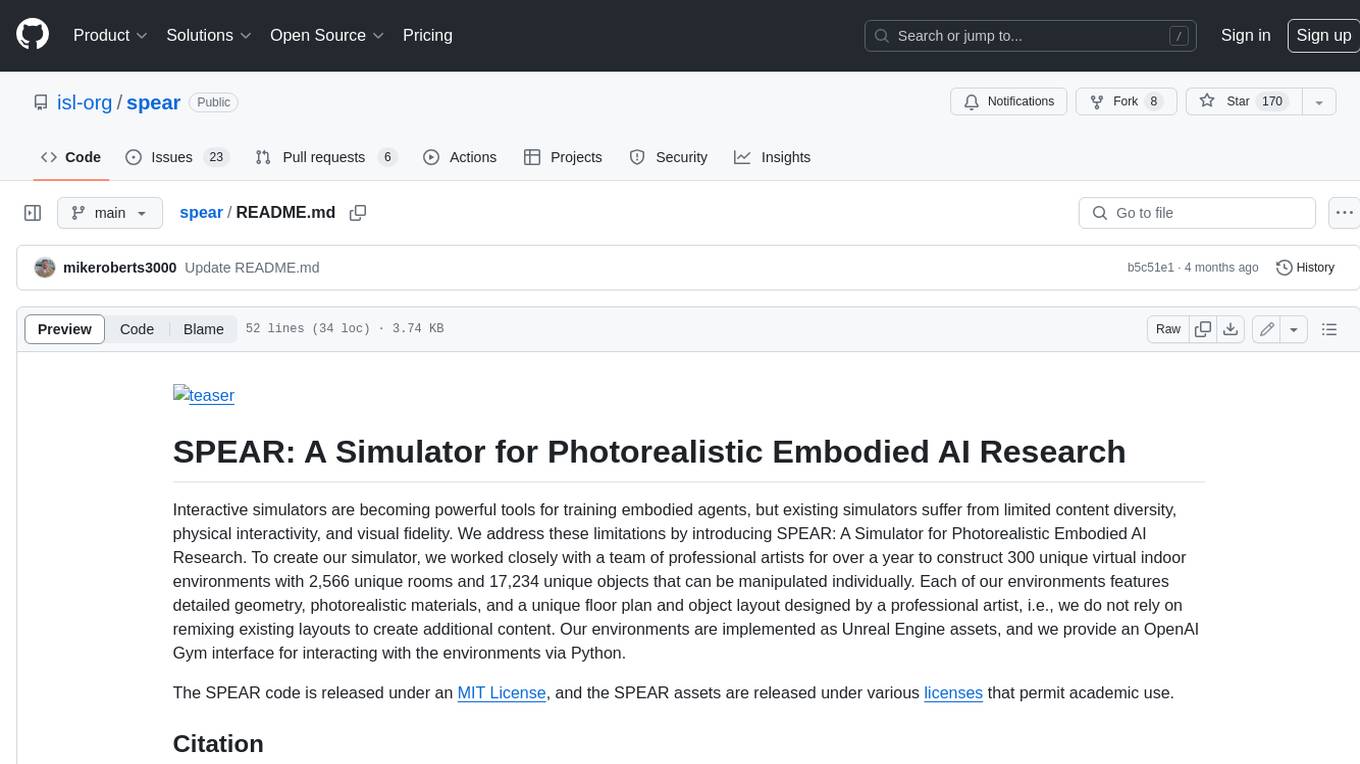
spear
SPEAR (Simulator for Photorealistic Embodied AI Research) is a powerful tool for training embodied agents. It features 300 unique virtual indoor environments with 2,566 unique rooms and 17,234 unique objects that can be manipulated individually. Each environment is designed by a professional artist and features detailed geometry, photorealistic materials, and a unique floor plan and object layout. SPEAR is implemented as Unreal Engine assets and provides an OpenAI Gym interface for interacting with the environments via Python.
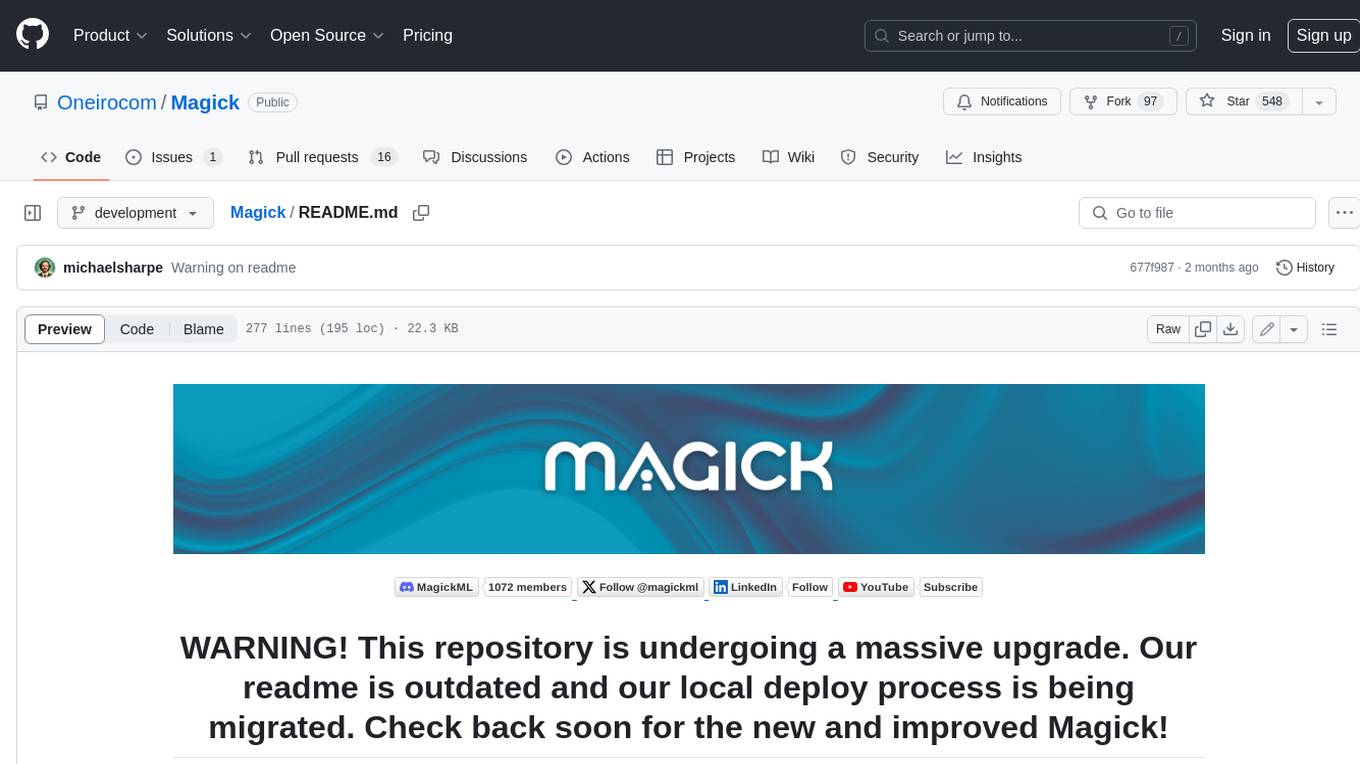
Magick
Magick is a groundbreaking visual AIDE (Artificial Intelligence Development Environment) for no-code data pipelines and multimodal agents. Magick can connect to other services and comes with nodes and templates well-suited for intelligent agents, chatbots, complex reasoning systems and realistic characters.