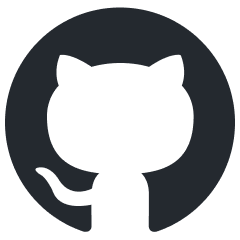
bilingual_book_maker
Make bilingual epub books Using AI translate
Stars: 7771
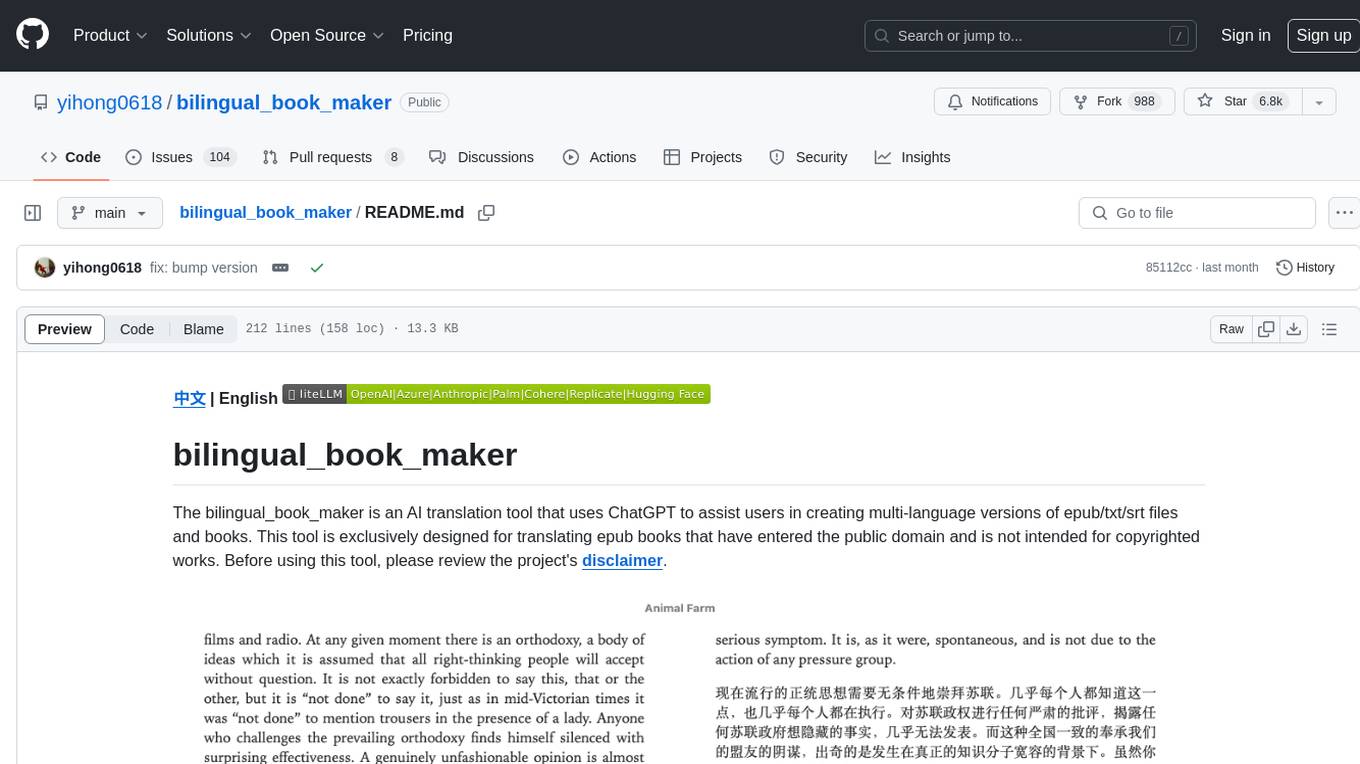
The bilingual_book_maker is an AI translation tool that uses ChatGPT to assist users in creating multi-language versions of epub/txt/srt files and books. It supports various models like gpt-4, gpt-3.5-turbo, claude-2, palm, llama-2, azure-openai, command-nightly, and gemini. Users need ChatGPT or OpenAI token, epub/txt books, internet access, and Python 3.8+. The tool provides options to specify OpenAI API key, model selection, target language, proxy server, context addition, translation style, and more. It generates bilingual books in epub format after translation. Users can test translations, set batch size, tweak prompts, and use different models like DeepL, Google Gemini, Tencent TranSmart, and more. The tool also supports retranslation, translating specific tags, and e-reader type specification. Docker usage is available for easy setup.
README:
中文 | English
The bilingual_book_maker is an AI translation tool that uses ChatGPT to assist users in creating multi-language versions of epub/txt/srt files and books. This tool is exclusively designed for translating epub books that have entered the public domain and is not intended for copyrighted works. Before using this tool, please review the project's disclaimer.
gpt-4, gpt-3.5-turbo, claude-2, palm, llama-2, azure-openai, command-nightly, gemini
For using Non-OpenAI models, use class liteLLM()
- liteLLM supports all models above.
Find more info here for using liteLLM: https://github.com/BerriAI/litellm/blob/main/setup.py
- ChatGPT or OpenAI token 1
- epub/txt books
- Environment with internet access or proxy
- Python 3.8+
A sample book, test_books/animal_farm.epub
, is provided for testing purposes.
pip install -r requirements.txt
python3 make_book.py --book_name test_books/animal_farm.epub --openai_key ${openai_key} --test
OR
pip install -U bbook_maker
bbook --book_name test_books/animal_farm.epub --openai_key ${openai_key} --test
- Use
--openai_key
option to specify OpenAI API key. If you have multiple keys, separate them by commas (xxx,xxx,xxx) to reduce errors caused by API call limits. Or, just set environment variableBBM_OPENAI_API_KEY
instead. - A sample book,
test_books/animal_farm.epub
, is provided for testing purposes. - The default underlying model is GPT-3.5-turbo, which is used by ChatGPT currently. Use
--model gpt4
to change the underlying model toGPT4
. You can also useGPT4omini
. - Important to note that
gpt-4
is significantly more expensive thangpt-4-turbo
, but to avoid bumping into rate limits, we automatically balance queries acrossgpt-4-1106-preview
,gpt-4
,gpt-4-32k
,gpt-4-0613
,gpt-4-32k-0613
. - If you want to use a specific model alias with OpenAI (eg
gpt-4-1106-preview
orgpt-3.5-turbo-0125
), you can use--model openai --model_list gpt-4-1106-preview,gpt-3.5-turbo-0125
.--model_list
takes a comma-separated list of model aliases. - If using chatgptapi, you can add
--use_context
to add a context paragraph to each passage sent to the model for translation (see below).
-
DeepL Support DeepL model DeepL Translator need pay to get the token
python3 make_book.py --book_name test_books/animal_farm.epub --model deepl --deepl_key ${deepl_key}
-
DeepL free
python3 make_book.py --book_name test_books/animal_farm.epub --model deeplfree
-
Use Claude model to translate
python3 make_book.py --book_name test_books/animal_farm.epub --model claude --claude_key ${claude_key}
-
Google Translate
python3 make_book.py --book_name test_books/animal_farm.epub --model google
-
Caiyun Translate
python3 make_book.py --book_name test_books/animal_farm.epub --model caiyun --caiyun_key ${caiyun_key}
-
Gemini
Support Google Gemini model, use
--model gemini
for Gemini Flash or--model geminipro
for Gemini Pro. If you want to use a specific model alias with Gemini (eggemini-1.5-flash-002
orgemini-1.5-flash-8b-exp-0924
), you can use--model gemini --model_list gemini-1.5-flash-002,gemini-1.5-flash-8b-exp-0924
.--model_list
takes a comma-separated list of model aliases.python3 make_book.py --book_name test_books/animal_farm.epub --model gemini --gemini_key ${gemini_key}
-
python3 make_book.py --book_name test_books/animal_farm.epub --model tencentransmart
-
python3 make_book.py --book_name test_books/animal_farm.epub --model xai --xai_key ${xai_key}
-
Support Ollama self-host models, If ollama server is not running on localhost, use
--api_base http://x.x.x.x:port/v1
to point to the ollama server addresspython3 make_book.py --book_name test_books/animal_farm.epub --ollama_model ${ollama_model_name}
-
GroqCloud currently supports models: you can find from Supported Models
python3 make_book.py --book_name test_books/animal_farm.epub --groq_key [your_key] --model groq --model_list llama3-8b-8192
- Once the translation is complete, a bilingual book named
${book_name}_bilingual.epub
would be generated. - If there are any errors or you wish to interrupt the translation by pressing
CTRL+C
. A book named{book_name}_bilingual_temp.epub
would be generated. You can simply rename it to any desired name.
-
--test
:Use
--test
option to preview the result if you haven't paid for the service. Note that there is a limit and it may take some time. -
--language
:Set the target language like
--language "Simplified Chinese"
. Default target language is"Simplified Chinese"
. Read available languages by helper message:python make_book.py --help
-
--proxy
:Use
--proxy
option to specify proxy server for internet access. Enter a string such ashttp://127.0.0.1:7890
. -
--resume
:Use
--resume
option to manually resume the process after an interruption.python3 make_book.py --book_name test_books/animal_farm.epub --model google --resume
-
--translate-tags
:epub is made of html files. By default, we only translate contents in
<p>
. Use--translate-tags
to specify tags need for translation. Use comma to separate multiple tags. For example:--translate-tags h1,h2,h3,p,div
-
--book_from
:Use
--book_from
option to specify e-reader type (Now onlykobo
is available), and use--device_path
to specify the mounting point. -
--api_base
:If you want to change api_base like using Cloudflare Workers, use
--api_base <URL>
to support it. Note: the api url should be 'https://xxxx/v1
'. Quotation marks are required. -
--allow_navigable_strings
:If you want to translate strings in an e-book that aren't labeled with any tags, you can use the
--allow_navigable_strings
parameter. This will add the strings to the translation queue. Note that it's best to look for e-books that are more standardized if possible. -
--prompt
:To tweak the prompt, use the
--prompt
parameter. Valid placeholders for theuser
role template include{text}
and{language}
. It supports a few ways to configure the prompt:-
If you don't need to set the
system
role content, you can simply set it up like this:--prompt "Translate {text} to {language}."
or--prompt prompt_template_sample.txt
(example of a text file can be found at ./prompt_template_sample.txt). -
If you need to set the
system
role content, you can use the following format:--prompt '{"user":"Translate {text} to {language}", "system": "You are a professional translator."}'
or--prompt prompt_template_sample.json
(example of a JSON file can be found at ./prompt_template_sample.json). -
You can also set the
user
andsystem
role prompt by setting environment variables:BBM_CHATGPTAPI_USER_MSG_TEMPLATE
andBBM_CHATGPTAPI_SYS_MSG
.
-
-
--batch_size
:Use the
--batch_size
parameter to specify the number of lines for batch translation (default is 10, currently only effective for txt files). -
--accumulated_num
:Wait for how many tokens have been accumulated before starting the translation. gpt3.5 limits the total_token to 4090. For example, if you use
--accumulated_num 1600
, maybe openai will output 2200 tokens and maybe 200 tokens for other messages in the system messages user messages, 1600+2200+200=4000, So you are close to reaching the limit. You have to choose your own value, there is no way to know if the limit is reached before sending -
--use_context
:prompts the model to create a three-paragraph summary. If it's the beginning of the translation, it will summarize the entire passage sent (the size depending on
--accumulated_num
). For subsequent passages, it will amend the summary to include details from the most recent passage, creating a running one-paragraph context payload of the important details of the entire translated work. This improves consistency of flow and tone throughout the translation. This option is available for all ChatGPT-compatible models and Gemini models. -
--context_paragraph_limit
:Use
--context_paragraph_limit
to set a limit on the number of context paragraphs when using the--use_context
option. -
--temperature
:Use
--temperature
to set the temperature parameter forchatgptapi
/gpt4
/claude
models. For example:--temperature 0.7
. -
--block_size
:Use
--block_size
to merge multiple paragraphs into one block. This may increase accuracy and speed up the process but can disturb the original format. Must be used with--single_translate
. For example:--block_size 5 --single_translate
. -
--single_translate
:Use
--single_translate
to output only the translated book without creating a bilingual version. -
--translation_style
:example:
--translation_style "color: #808080; font-style: italic;"
-
--retranslate "$translated_filepath" "file_name_in_epub" "start_str" "end_str"(optional)
:Retranslate from start_str to end_str's tag:
python3 "make_book.py" --book_name "test_books/animal_farm.epub" --retranslate 'test_books/animal_farm_bilingual.epub' 'index_split_002.html' 'in spite of the present book shortage which' 'This kind of thing is not a good symptom. Obviously'
Retranslate start_str's tag:
python3 "make_book.py" --book_name "test_books/animal_farm.epub" --retranslate 'test_books/animal_farm_bilingual.epub' 'index_split_002.html' 'in spite of the present book shortage which'
Note if use pip install bbook_maker
all commands can change to bbook_maker args
# Test quickly
python3 make_book.py --book_name test_books/animal_farm.epub --openai_key ${openai_key} --test --language zh-hans
# Test quickly for src
python3 make_book.py --book_name test_books/Lex_Fridman_episode_322.srt --openai_key ${openai_key} --test
# Or translate the whole book
python3 make_book.py --book_name test_books/animal_farm.epub --openai_key ${openai_key} --language zh-hans
# Or translate the whole book using Gemini flash
python3 make_book.py --book_name test_books/animal_farm.epub --gemini_key ${gemini_key} --model gemini
# Use a specific list of Gemini model aliases
python3 make_book.py --book_name test_books/animal_farm.epub --gemini_key ${gemini_key} --model gemini --model_list gemini-1.5-flash-002,gemini-1.5-flash-8b-exp-0924
# Set env OPENAI_API_KEY to ignore option --openai_key
export OPENAI_API_KEY=${your_api_key}
# Use the GPT-4 model with context to Japanese
python3 make_book.py --book_name test_books/animal_farm.epub --model gpt4 --use_context --language ja
# Use a specific OpenAI model alias
python3 make_book.py --book_name test_books/animal_farm.epub --model openai --model_list gpt-4-1106-preview --openai_key ${openai_key}
**Note** you can use other `openai like` model in this way
python3 make_book.py --book_name test_books/animal_farm.epub --model openai --model_list yi-34b-chat-0205 --openai_key ${openai_key} --api_base "https://api.lingyiwanwu.com/v1"
# Use a specific list of OpenAI model aliases
python3 make_book.py --book_name test_books/animal_farm.epub --model openai --model_list gpt-4-1106-preview,gpt-4-0125-preview,gpt-3.5-turbo-0125 --openai_key ${openai_key}
# Use the DeepL model with Japanese
python3 make_book.py --book_name test_books/animal_farm.epub --model deepl --deepl_key ${deepl_key} --language ja
# Use the Claude model with Japanese
python3 make_book.py --book_name test_books/animal_farm.epub --model claude --claude_key ${claude_key} --language ja
# Use the CustomAPI model with Japanese
python3 make_book.py --book_name test_books/animal_farm.epub --model customapi --custom_api ${custom_api} --language ja
# Translate contents in <div> and <p>
python3 make_book.py --book_name test_books/animal_farm.epub --translate-tags div,p
# Tweaking the prompt
python3 make_book.py --book_name test_books/animal_farm.epub --prompt prompt_template_sample.txt
# or
python3 make_book.py --book_name test_books/animal_farm.epub --prompt prompt_template_sample.json
# or
python3 make_book.py --book_name test_books/animal_farm.epub --prompt "Please translate \`{text}\` to {language}"
# Translate books download from Rakuten Kobo on kobo e-reader
python3 make_book.py --book_from kobo --device_path /tmp/kobo
# translate txt file
python3 make_book.py --book_name test_books/the_little_prince.txt --test --language zh-hans
# aggregated translation txt file
python3 make_book.py --book_name test_books/the_little_prince.txt --test --batch_size 20
# Using Caiyun model to translate
# (the api currently only support: simplified chinese <-> english, simplified chinese <-> japanese)
# the official Caiyun has provided a test token (3975l6lr5pcbvidl6jl2)
# you can apply your own token by following this tutorial(https://bobtranslate.com/service/translate/caiyun.html)
python3 make_book.py --model caiyun --caiyun_key 3975l6lr5pcbvidl6jl2 --book_name test_books/animal_farm.epub
# Set env BBM_CAIYUN_API_KEY to ignore option --openai_key
export BBM_CAIYUN_API_KEY=${your_api_key}
More understandable example
python3 make_book.py --book_name 'animal_farm.epub' --openai_key sk-XXXXX --api_base 'https://xxxxx/v1'
# Or python3 is not in your PATH
python make_book.py --book_name 'animal_farm.epub' --openai_key sk-XXXXX --api_base 'https://xxxxx/v1'
Microsoft Azure Endpoints
python3 make_book.py --book_name 'animal_farm.epub' --openai_key XXXXX --api_base 'https://example-endpoint.openai.azure.com' --deployment_id 'deployment-name'
# Or python3 is not in your PATH
python make_book.py --book_name 'animal_farm.epub' --openai_key XXXXX --api_base 'https://example-endpoint.openai.azure.com' --deployment_id 'deployment-name'
You can use Docker if you don't want to deal with setting up the environment.
# Build image
docker build --tag bilingual_book_maker .
# Run container
# "$folder_path" represents the folder where your book file locates. Also, it is where the processed file will be stored.
# Windows PowerShell
$folder_path=your_folder_path # $folder_path="C:\Users\user\mybook\"
$book_name=your_book_name # $book_name="animal_farm.epub"
$openai_key=your_api_key # $openai_key="sk-xxx"
$language=your_language # see utils.py
docker run --rm --name bilingual_book_maker --mount type=bind,source=$folder_path,target='/app/test_books' bilingual_book_maker --book_name "/app/test_books/$book_name" --openai_key $openai_key --language $language
# Linux
export folder_path=${your_folder_path}
export book_name=${your_book_name}
export openai_key=${your_api_key}
export language=${your_language}
docker run --rm --name bilingual_book_maker --mount type=bind,source=${folder_path},target='/app/test_books' bilingual_book_maker --book_name "/app/test_books/${book_name}" --openai_key ${openai_key} --language "${language}"
For example:
# Linux
docker run --rm --name bilingual_book_maker --mount type=bind,source=/home/user/my_books,target='/app/test_books' bilingual_book_maker --book_name /app/test_books/animal_farm.epub --openai_key sk-XXX --test --test_num 1 --language zh-hant
- API token from free trial has limit. If you want to speed up the process, consider paying for the service or use multiple OpenAI tokens
- PR is welcome
- Any issues or PRs are welcome.
- TODOs in the issue can also be selected.
- Please run
black make_book.py
2 before submitting the code.
- 书译 iOS -> AI 全书翻译工具
Thank you, that's enough.
For Tasks:
Click tags to check more tools for each tasksFor Jobs:
Alternative AI tools for bilingual_book_maker
Similar Open Source Tools
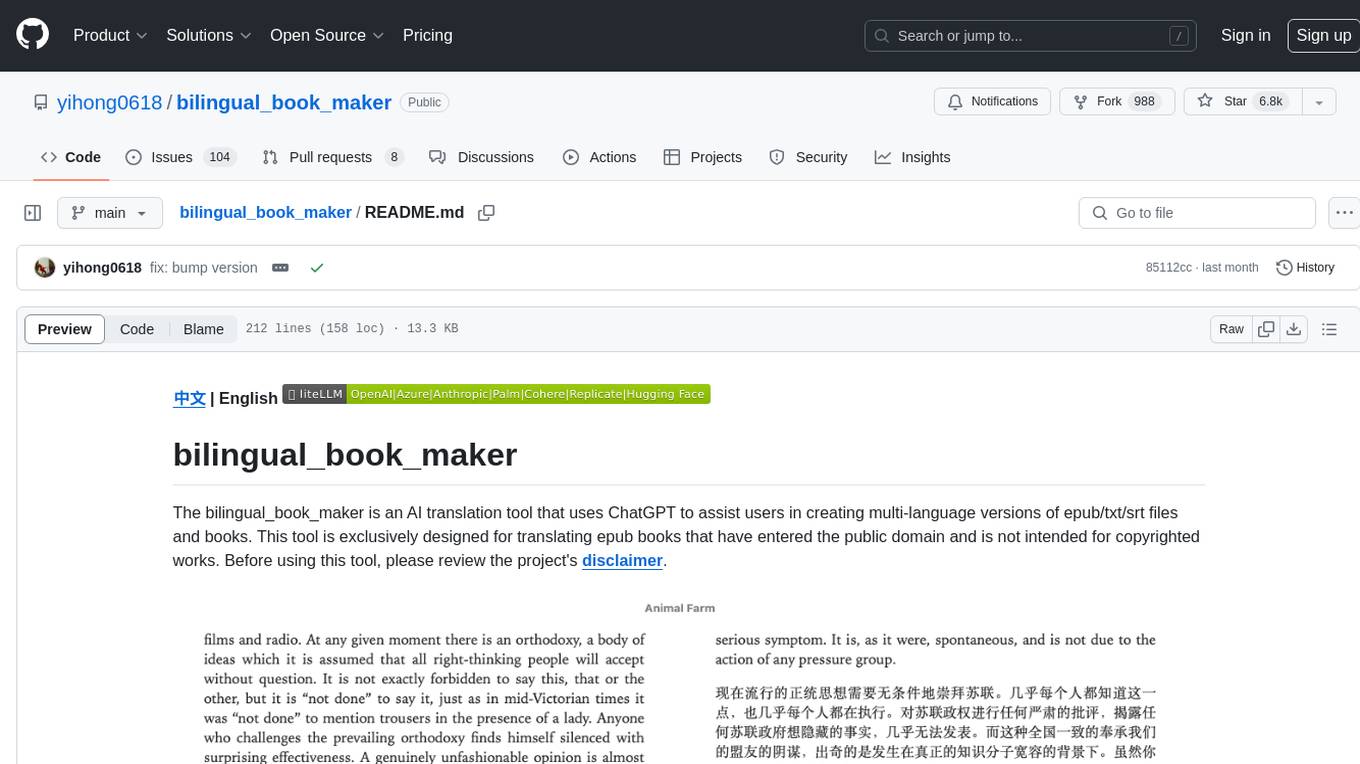
bilingual_book_maker
The bilingual_book_maker is an AI translation tool that uses ChatGPT to assist users in creating multi-language versions of epub/txt/srt files and books. It supports various models like gpt-4, gpt-3.5-turbo, claude-2, palm, llama-2, azure-openai, command-nightly, and gemini. Users need ChatGPT or OpenAI token, epub/txt books, internet access, and Python 3.8+. The tool provides options to specify OpenAI API key, model selection, target language, proxy server, context addition, translation style, and more. It generates bilingual books in epub format after translation. Users can test translations, set batch size, tweak prompts, and use different models like DeepL, Google Gemini, Tencent TranSmart, and more. The tool also supports retranslation, translating specific tags, and e-reader type specification. Docker usage is available for easy setup.
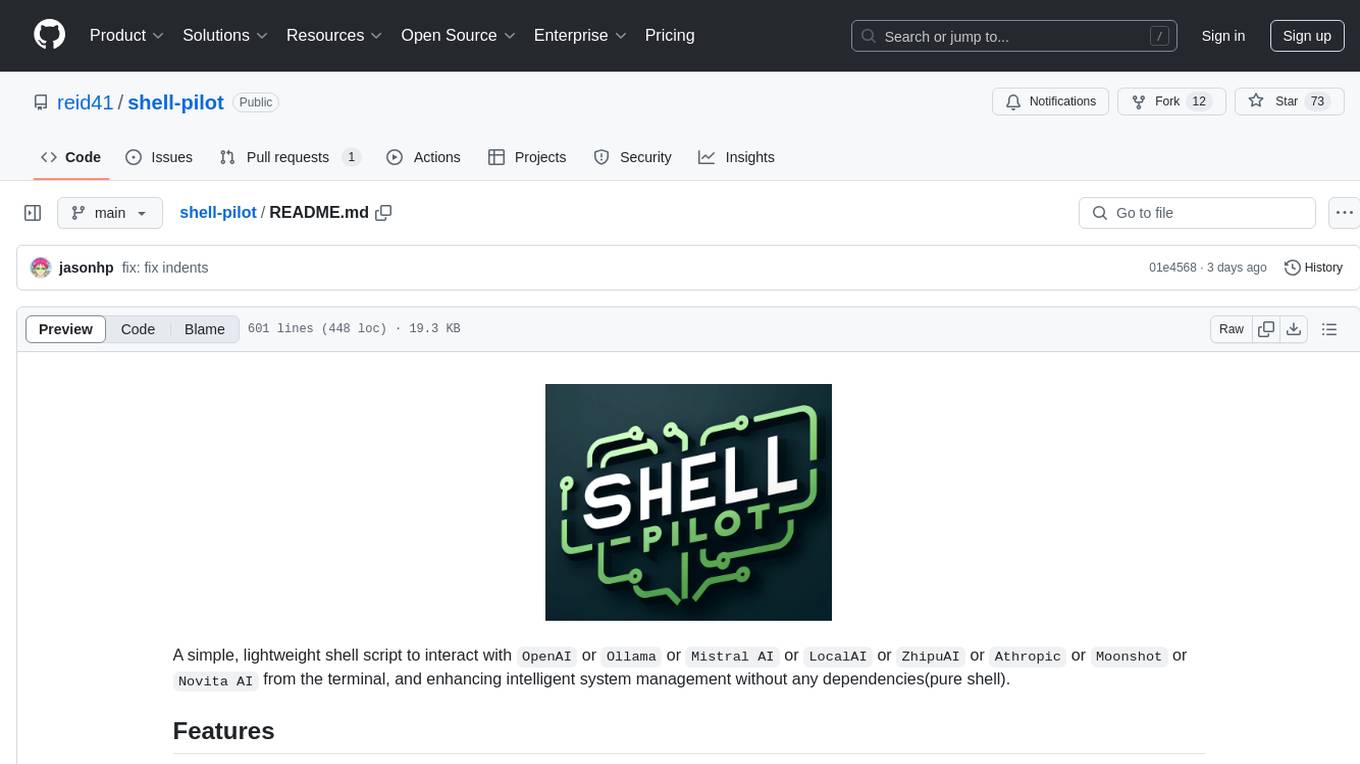
shell-pilot
Shell-pilot is a simple, lightweight shell script designed to interact with various AI models such as OpenAI, Ollama, Mistral AI, LocalAI, ZhipuAI, Anthropic, Moonshot, and Novita AI from the terminal. It enhances intelligent system management without any dependencies, offering features like setting up a local LLM repository, using official models and APIs, viewing history and session persistence, passing input prompts with pipe/redirector, listing available models, setting request parameters, generating and running commands in the terminal, easy configuration setup, system package version checking, and managing system aliases.
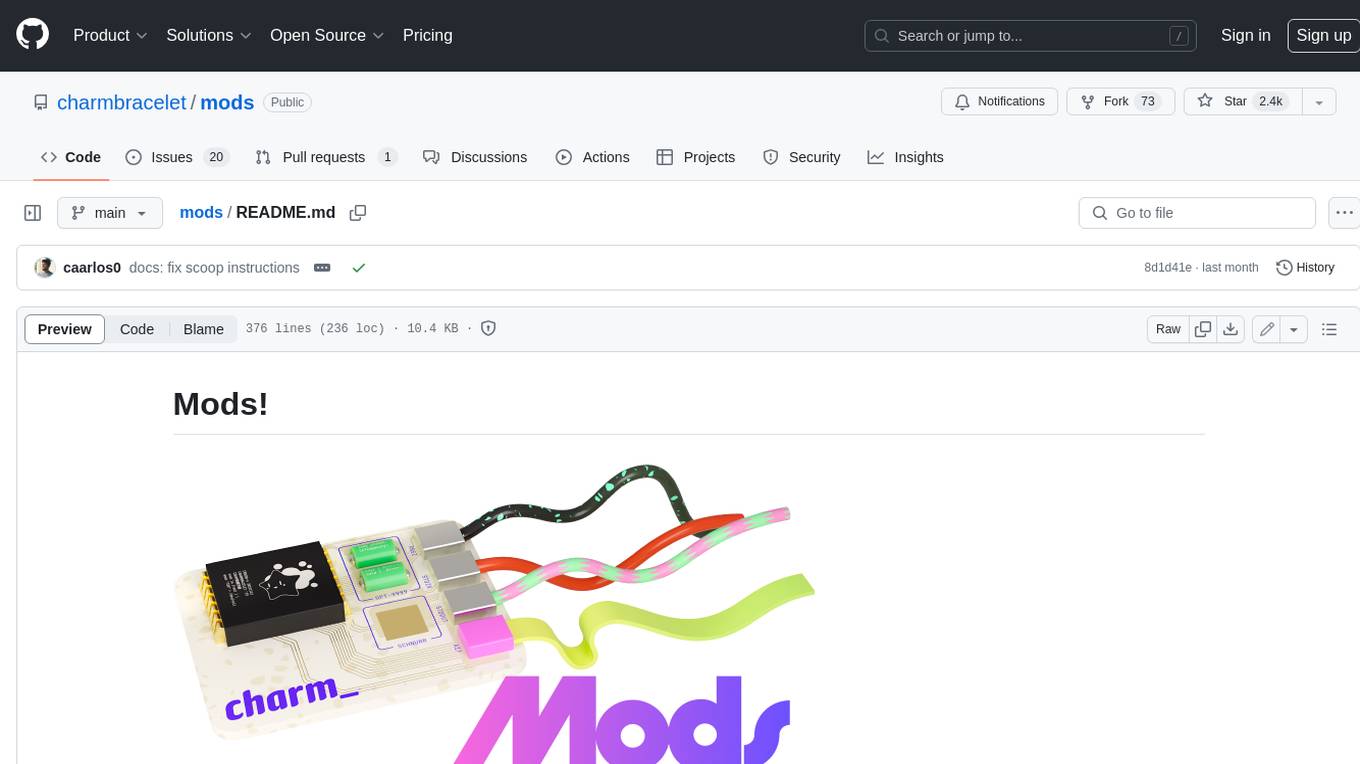
mods
AI for the command line, built for pipelines. LLM based AI is really good at interpreting the output of commands and returning the results in CLI friendly text formats like Markdown. Mods is a simple tool that makes it super easy to use AI on the command line and in your pipelines. Mods works with OpenAI, Groq, Azure OpenAI, and LocalAI To get started, install Mods and check out some of the examples below. Since Mods has built-in Markdown formatting, you may also want to grab Glow to give the output some _pizzazz_.
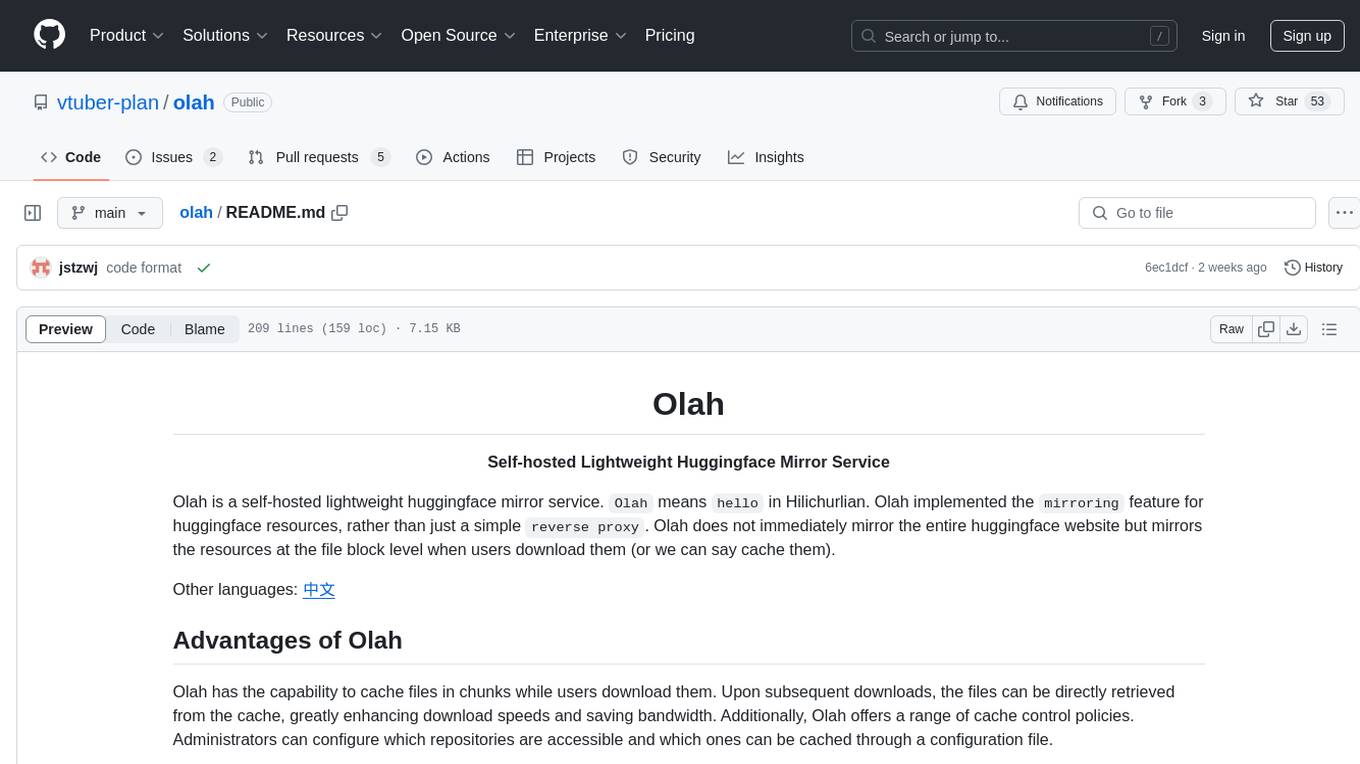
olah
Olah is a self-hosted lightweight Huggingface mirror service that implements mirroring feature for Huggingface resources at file block level, enhancing download speeds and saving bandwidth. It offers cache control policies and allows administrators to configure accessible repositories. Users can install Olah with pip or from source, set up the mirror site, and download models and datasets using huggingface-cli. Olah provides additional configurations through a configuration file for basic setup and accessibility restrictions. Future work includes implementing an administrator and user system, OOS backend support, and mirror update schedule task. Olah is released under the MIT License.
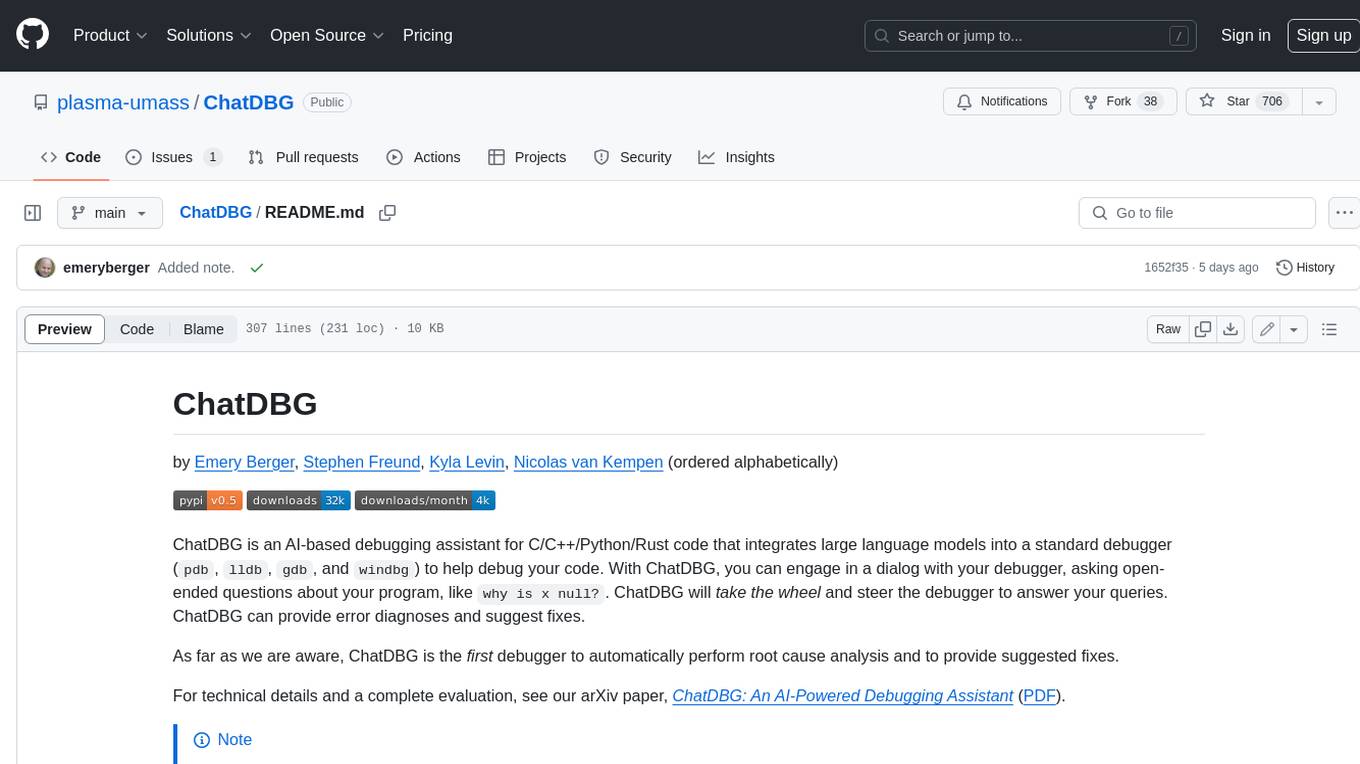
ChatDBG
ChatDBG is an AI-based debugging assistant for C/C++/Python/Rust code that integrates large language models into a standard debugger (`pdb`, `lldb`, `gdb`, and `windbg`) to help debug your code. With ChatDBG, you can engage in a dialog with your debugger, asking open-ended questions about your program, like `why is x null?`. ChatDBG will _take the wheel_ and steer the debugger to answer your queries. ChatDBG can provide error diagnoses and suggest fixes. As far as we are aware, ChatDBG is the _first_ debugger to automatically perform root cause analysis and to provide suggested fixes.
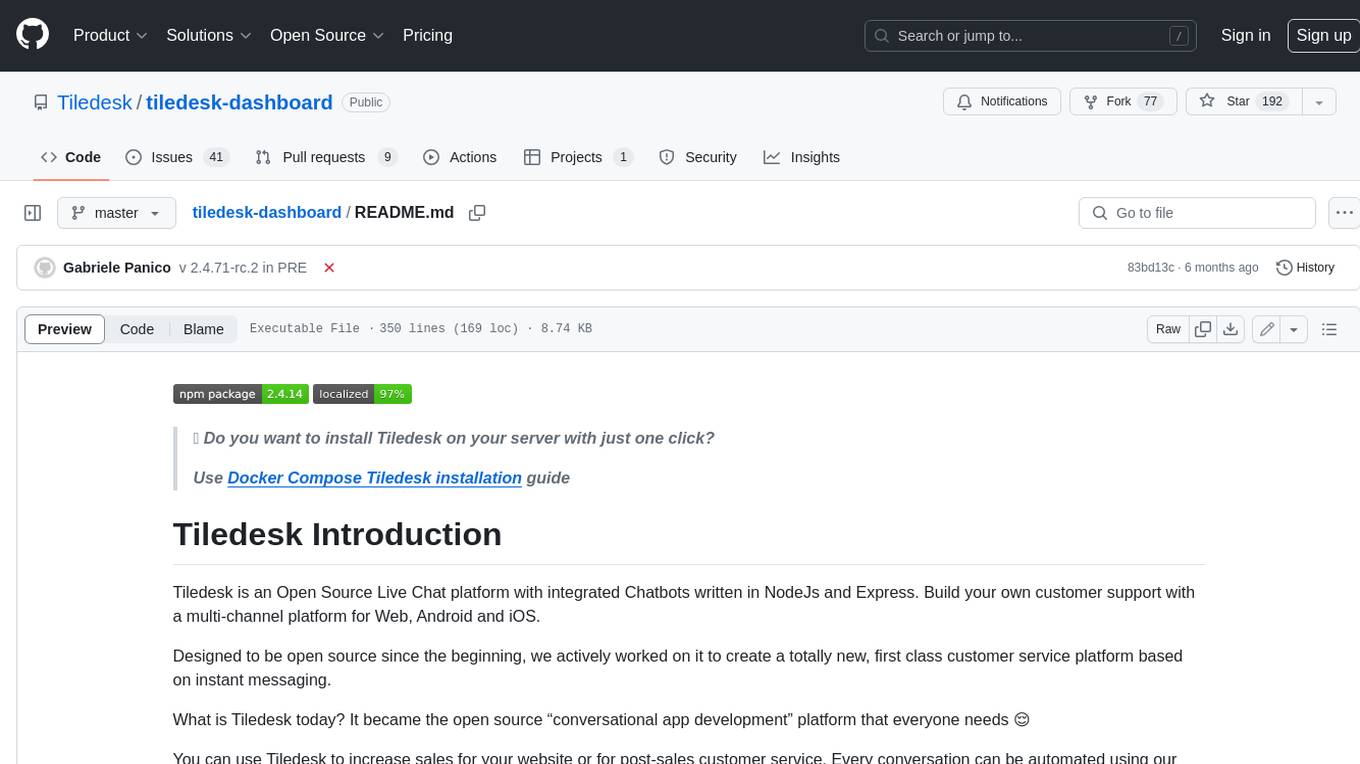
tiledesk-dashboard
Tiledesk is an open-source live chat platform with integrated chatbots written in Node.js and Express. It is designed to be a multi-channel platform for web, Android, and iOS, and it can be used to increase sales or provide post-sales customer service. Tiledesk's chatbot technology allows for automation of conversations, and it also provides APIs and webhooks for connecting external applications. Additionally, it offers a marketplace for apps and features such as CRM, ticketing, and data export.
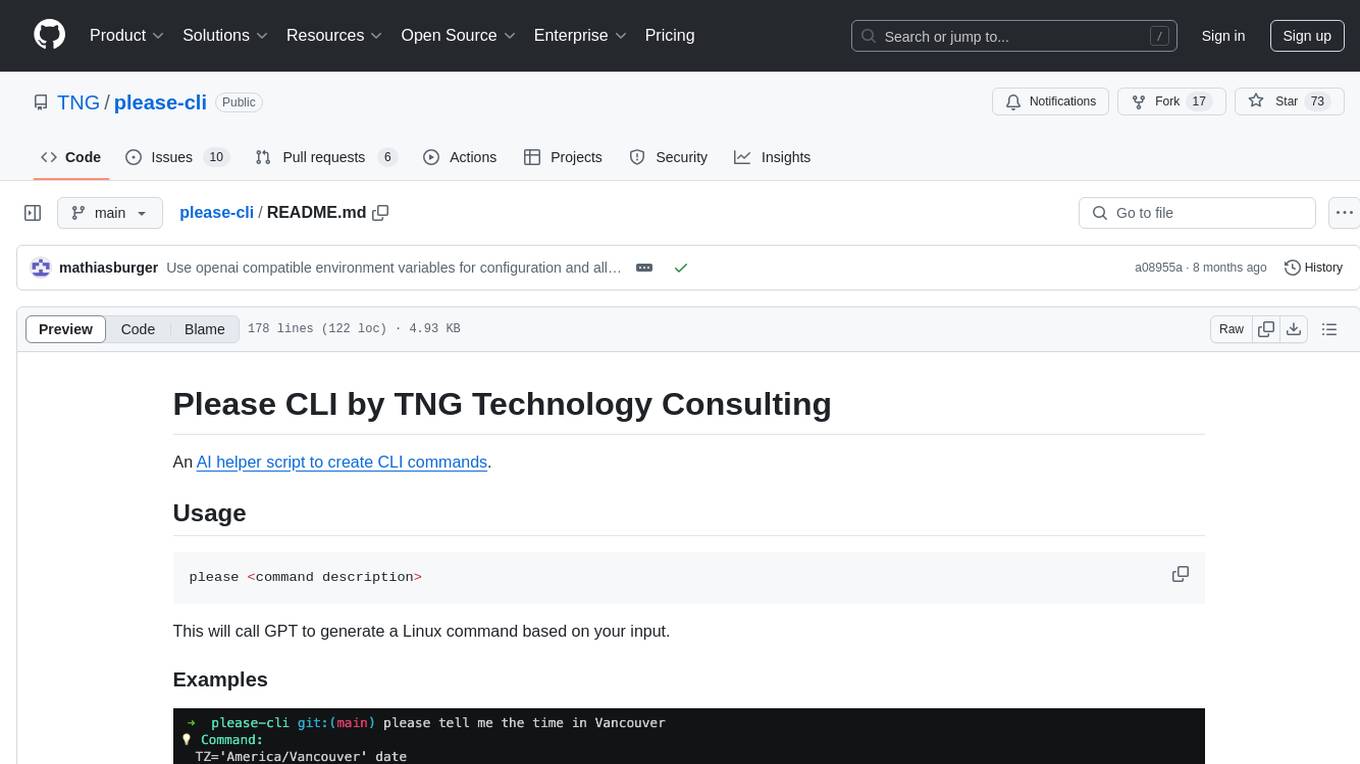
please-cli
Please CLI is an AI helper script designed to create CLI commands by leveraging the GPT model. Users can input a command description, and the script will generate a Linux command based on that input. The tool offers various functionalities such as invoking commands, copying commands to the clipboard, asking questions about commands, and more. It supports parameters for explanation, using different AI models, displaying additional output, storing API keys, querying ChatGPT with specific models, showing the current version, and providing help messages. Users can install Please CLI via Homebrew, apt, Nix, dpkg, AUR, or manually from source. The tool requires an OpenAI API key for operation and offers configuration options for setting API keys and OpenAI settings. Please CLI is licensed under the Apache License 2.0 by TNG Technology Consulting GmbH.
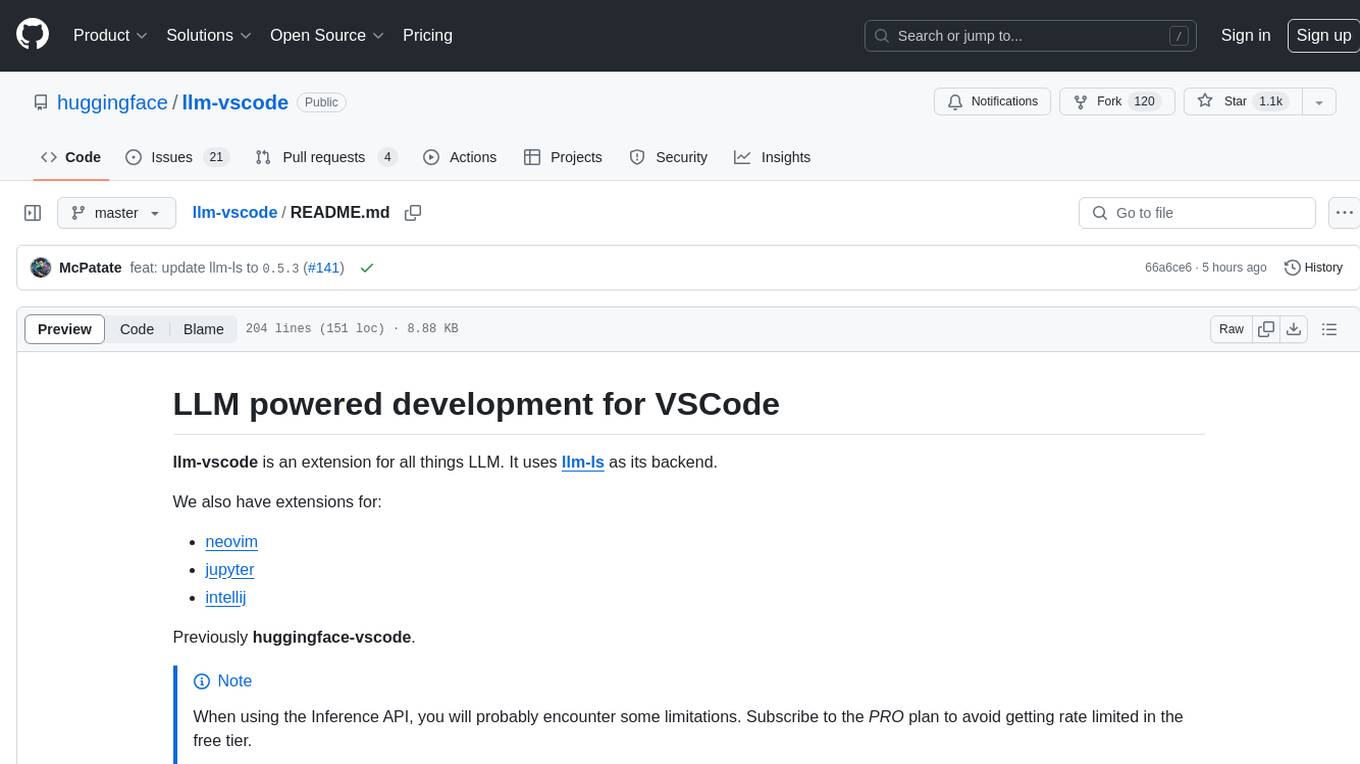
llm-vscode
llm-vscode is an extension designed for all things LLM, utilizing llm-ls as its backend. It offers features such as code completion with 'ghost-text' suggestions, the ability to choose models for code generation via HTTP requests, ensuring prompt size fits within the context window, and code attribution checks. Users can configure the backend, suggestion behavior, keybindings, llm-ls settings, and tokenization options. Additionally, the extension supports testing models like Code Llama 13B, Phind/Phind-CodeLlama-34B-v2, and WizardLM/WizardCoder-Python-34B-V1.0. Development involves cloning llm-ls, building it, and setting up the llm-vscode extension for use.
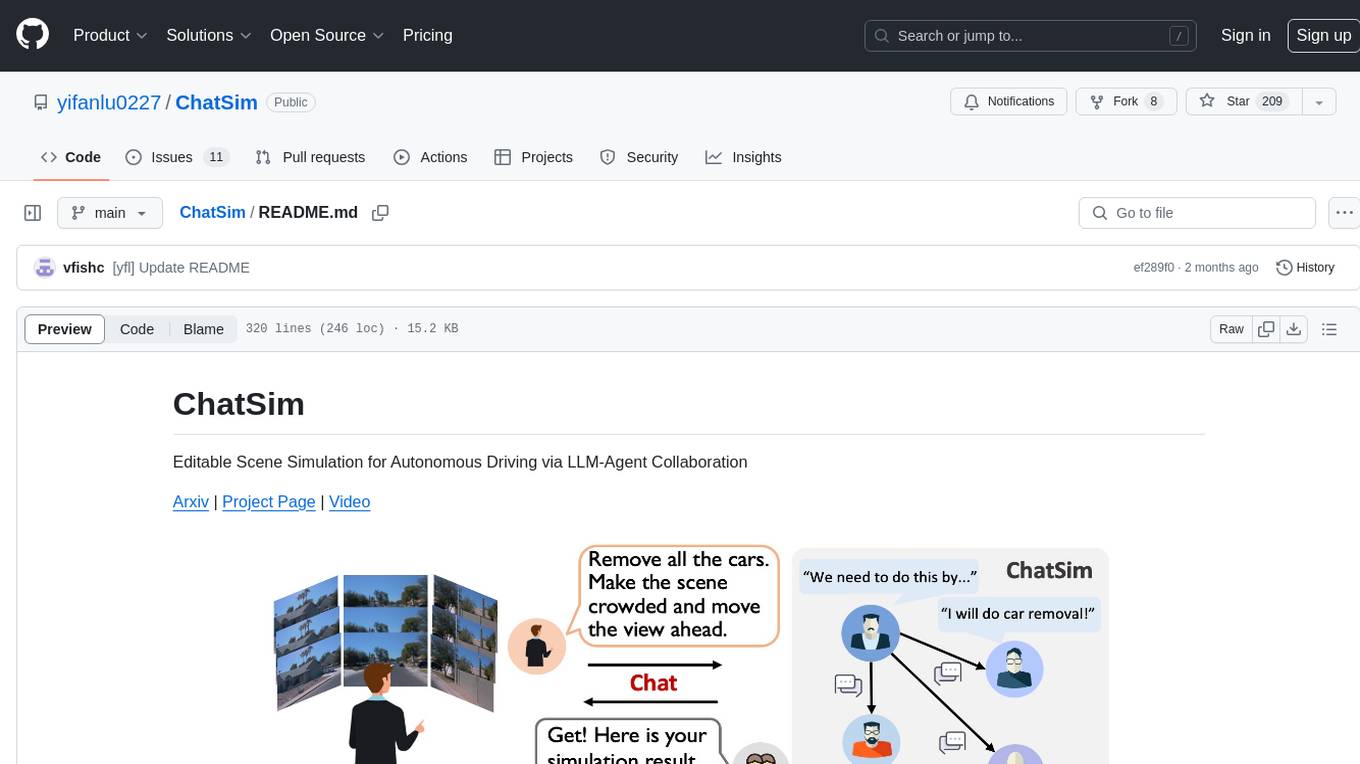
ChatSim
ChatSim is a tool designed for editable scene simulation for autonomous driving via LLM-Agent collaboration. It provides functionalities for setting up the environment, installing necessary dependencies like McNeRF and Inpainting tools, and preparing data for simulation. Users can train models, simulate scenes, and track trajectories for smoother and more realistic results. The tool integrates with Blender software and offers options for training McNeRF models and McLight's skydome estimation network. It also includes a trajectory tracking module for improved trajectory tracking. ChatSim aims to facilitate the simulation of autonomous driving scenarios with collaborative LLM-Agents.
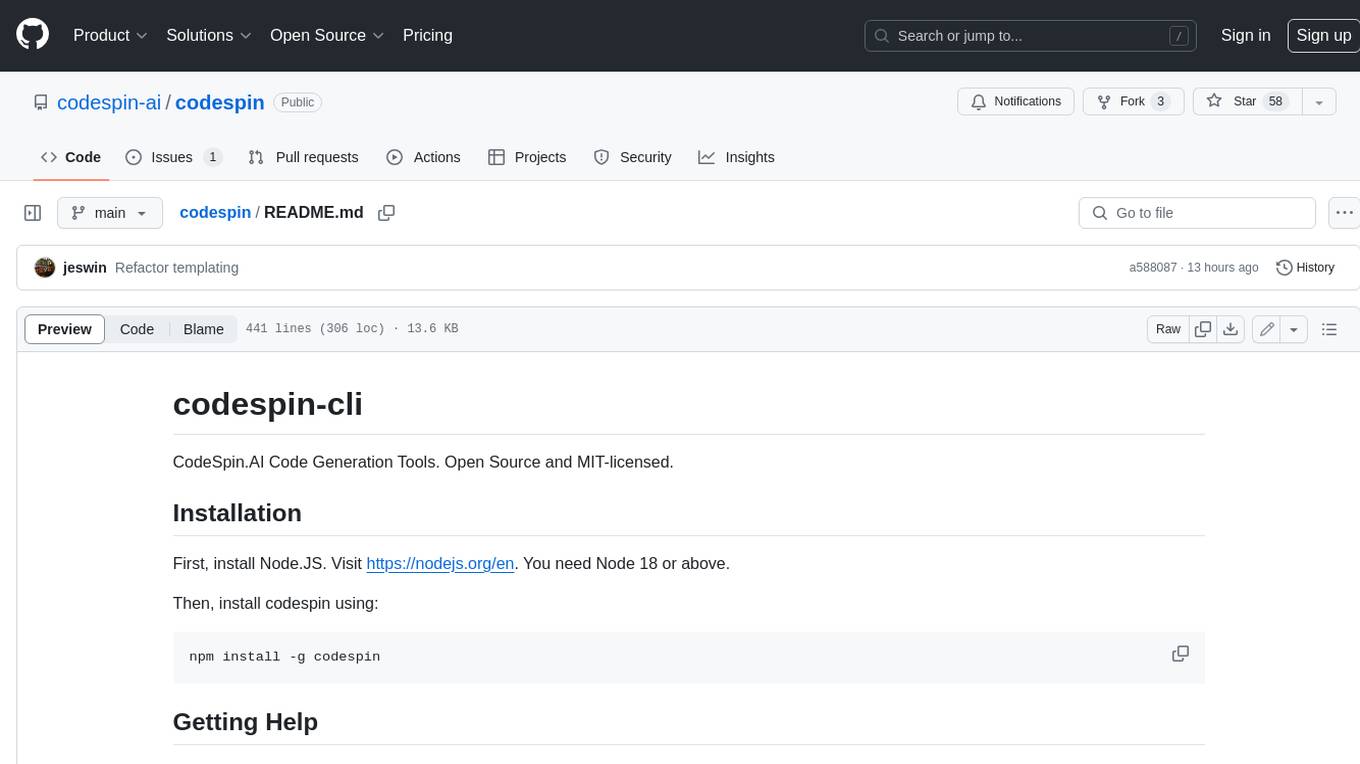
codespin
CodeSpin.AI is a set of open-source code generation tools that leverage large language models (LLMs) to automate coding tasks. With CodeSpin, you can generate code in various programming languages, including Python, JavaScript, Java, and C++, by providing natural language prompts. CodeSpin offers a range of features to enhance code generation, such as custom templates, inline prompting, and the ability to use ChatGPT as an alternative to API keys. Additionally, CodeSpin provides options for regenerating code, executing code in prompt files, and piping data into the LLM for processing. By utilizing CodeSpin, developers can save time and effort in coding tasks, improve code quality, and explore new possibilities in code generation.
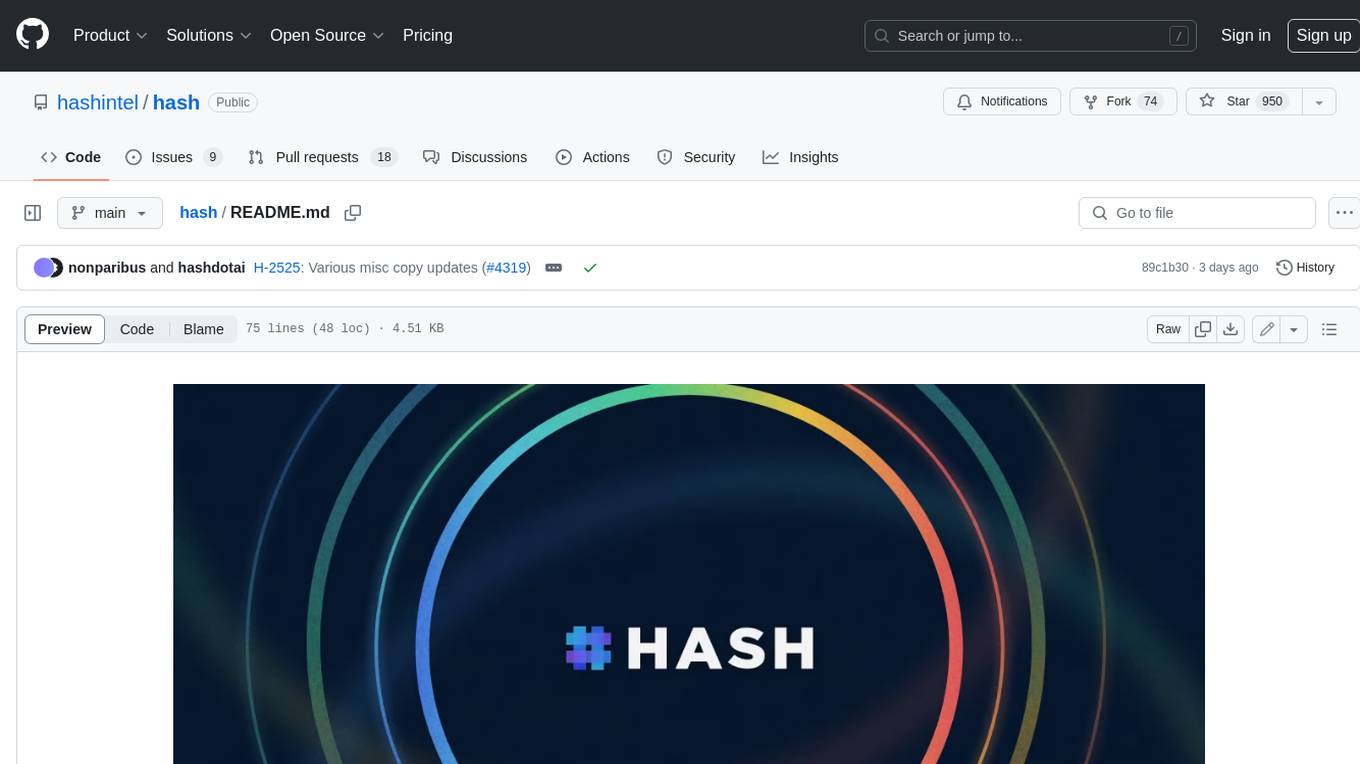
hash
HASH is a self-building, open-source database which grows, structures and checks itself. With it, we're creating a platform for decision-making, which helps you integrate, understand and use data in a variety of different ways.
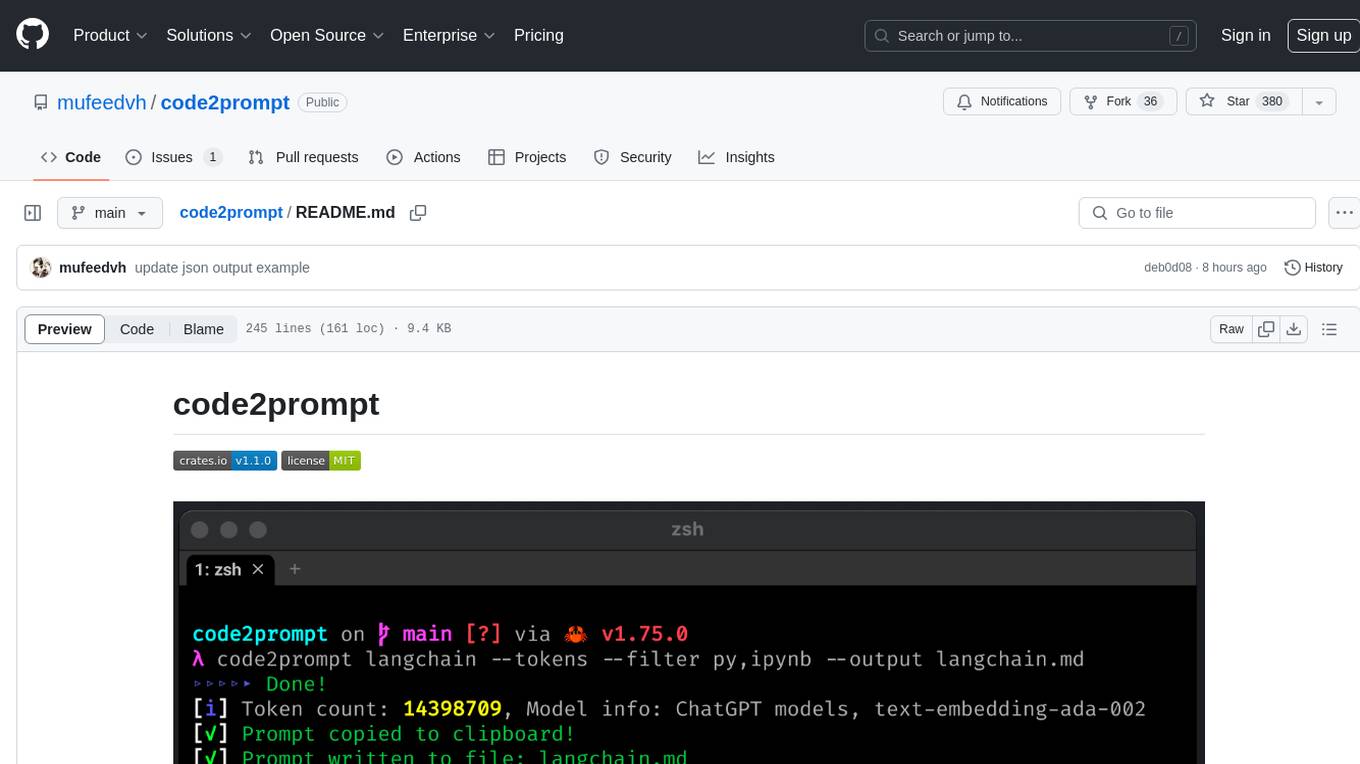
code2prompt
code2prompt is a command-line tool that converts your codebase into a single LLM prompt with a source tree, prompt templating, and token counting. It automates generating LLM prompts from codebases of any size, customizing prompt generation with Handlebars templates, respecting .gitignore, filtering and excluding files using glob patterns, displaying token count, including Git diff output, copying prompt to clipboard, saving prompt to an output file, excluding files and folders, adding line numbers to source code blocks, and more. It helps streamline the process of creating LLM prompts for code analysis, generation, and other tasks.
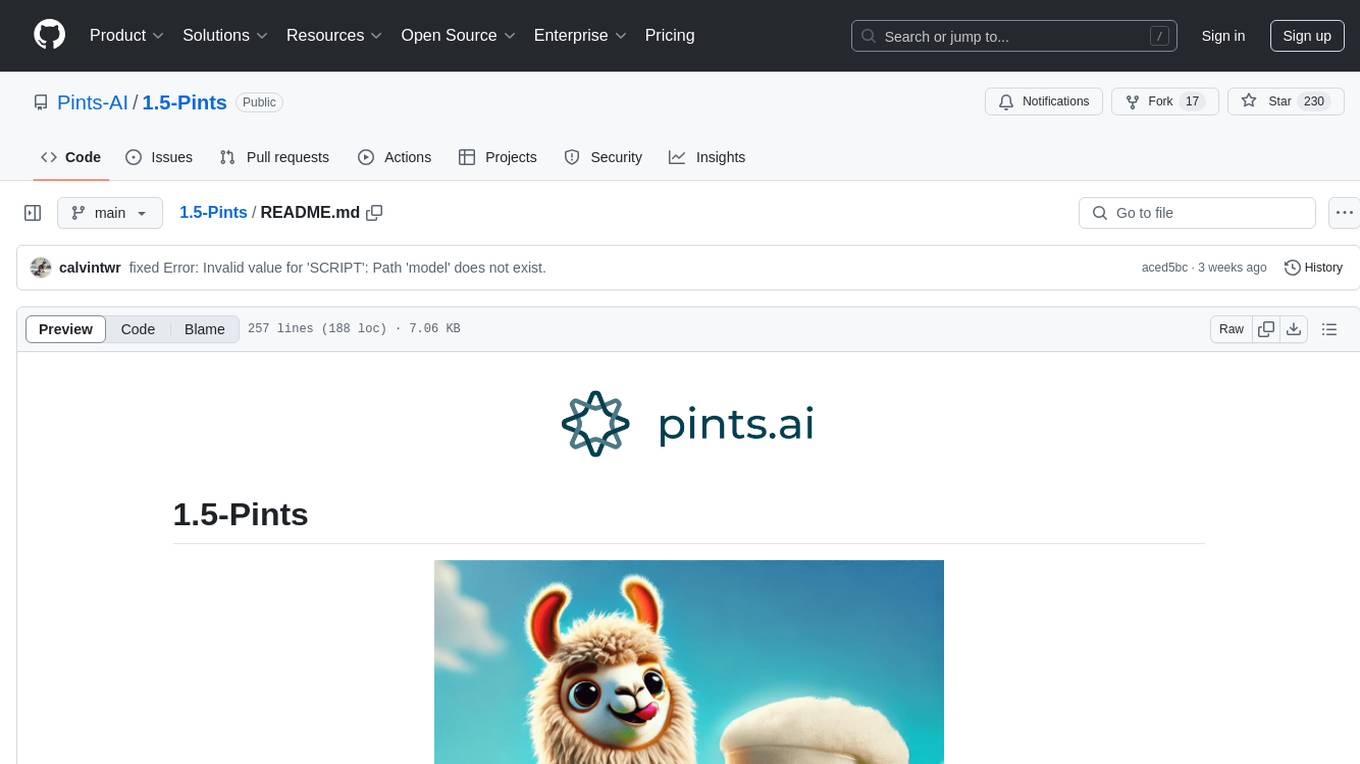
1.5-Pints
1.5-Pints is a repository that provides a recipe to pre-train models in 9 days, aiming to create AI assistants comparable to Apple OpenELM and Microsoft Phi. It includes model architecture, training scripts, and utilities for 1.5-Pints and 0.12-Pint developed by Pints.AI. The initiative encourages replication, experimentation, and open-source development of Pint by sharing the model's codebase and architecture. The repository offers installation instructions, dataset preparation scripts, model training guidelines, and tools for model evaluation and usage. Users can also find information on finetuning models, converting lit models to HuggingFace models, and running Direct Preference Optimization (DPO) post-finetuning. Additionally, the repository includes tests to ensure code modifications do not disrupt the existing functionality.
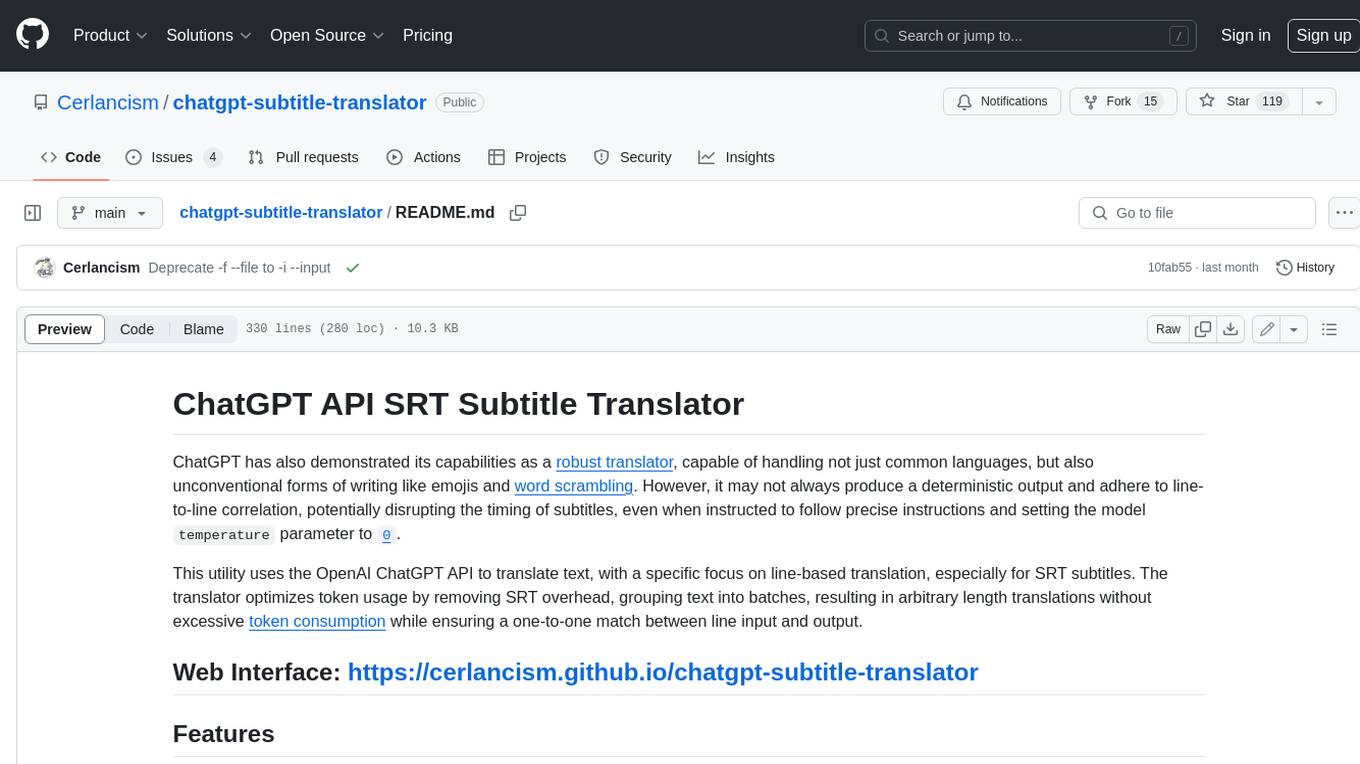
chatgpt-subtitle-translator
This tool utilizes the OpenAI ChatGPT API to translate text, with a focus on line-based translation, particularly for SRT subtitles. It optimizes token usage by removing SRT overhead and grouping text into batches, allowing for arbitrary length translations without excessive token consumption while maintaining a one-to-one match between line input and output.
For similar tasks
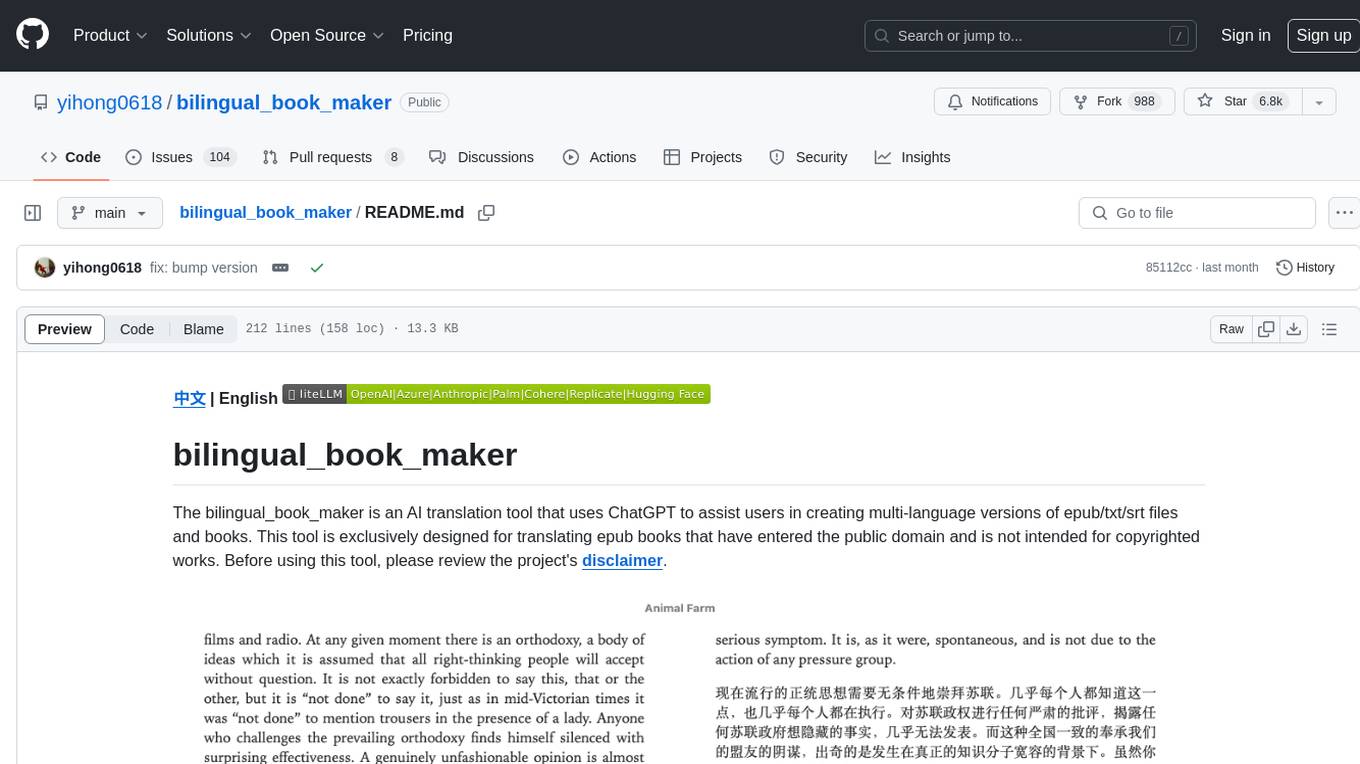
bilingual_book_maker
The bilingual_book_maker is an AI translation tool that uses ChatGPT to assist users in creating multi-language versions of epub/txt/srt files and books. It supports various models like gpt-4, gpt-3.5-turbo, claude-2, palm, llama-2, azure-openai, command-nightly, and gemini. Users need ChatGPT or OpenAI token, epub/txt books, internet access, and Python 3.8+. The tool provides options to specify OpenAI API key, model selection, target language, proxy server, context addition, translation style, and more. It generates bilingual books in epub format after translation. Users can test translations, set batch size, tweak prompts, and use different models like DeepL, Google Gemini, Tencent TranSmart, and more. The tool also supports retranslation, translating specific tags, and e-reader type specification. Docker usage is available for easy setup.
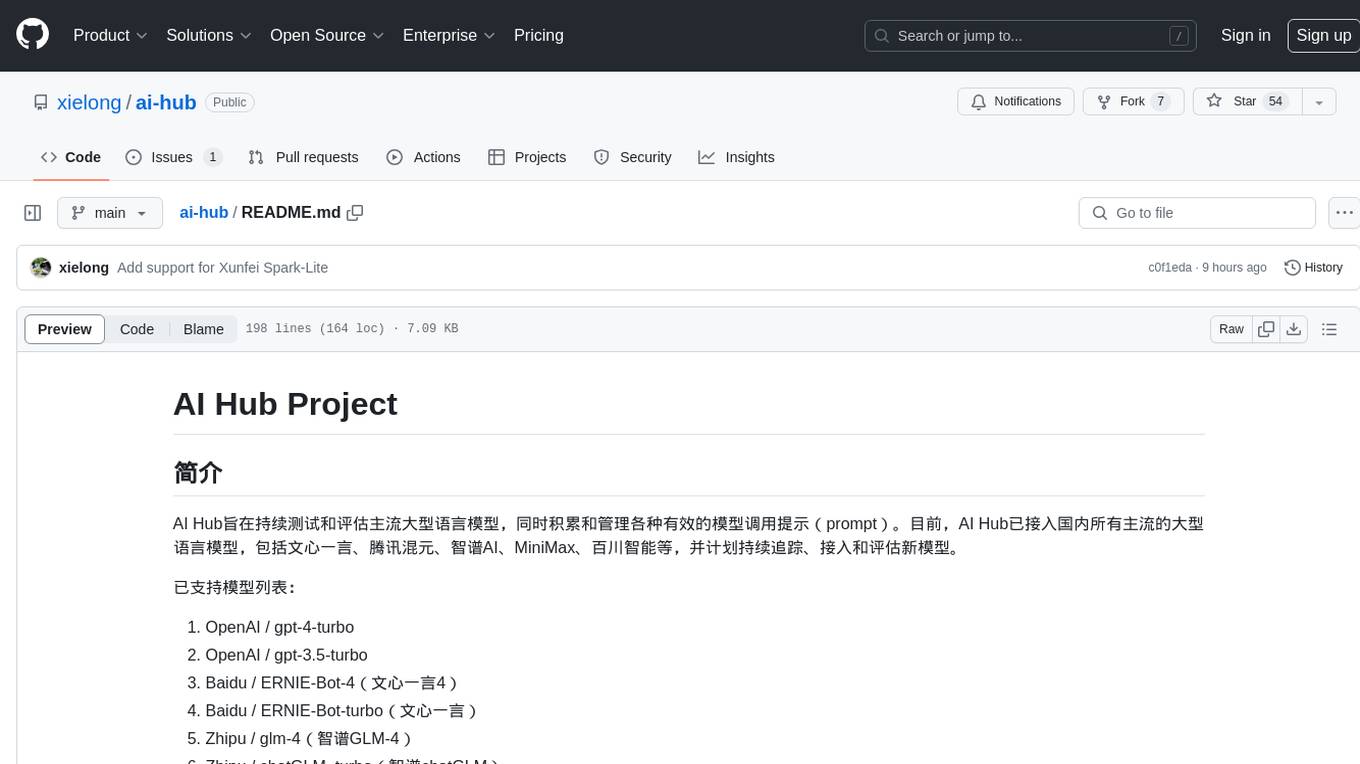
ai-hub
AI Hub Project aims to continuously test and evaluate mainstream large language models, while accumulating and managing various effective model invocation prompts. It has integrated all mainstream large language models in China, including OpenAI GPT-4 Turbo, Baidu ERNIE-Bot-4, Tencent ChatPro, MiniMax abab5.5-chat, and more. The project plans to continuously track, integrate, and evaluate new models. Users can access the models through REST services or Java code integration. The project also provides a testing suite for translation, coding, and benchmark testing.
For similar jobs
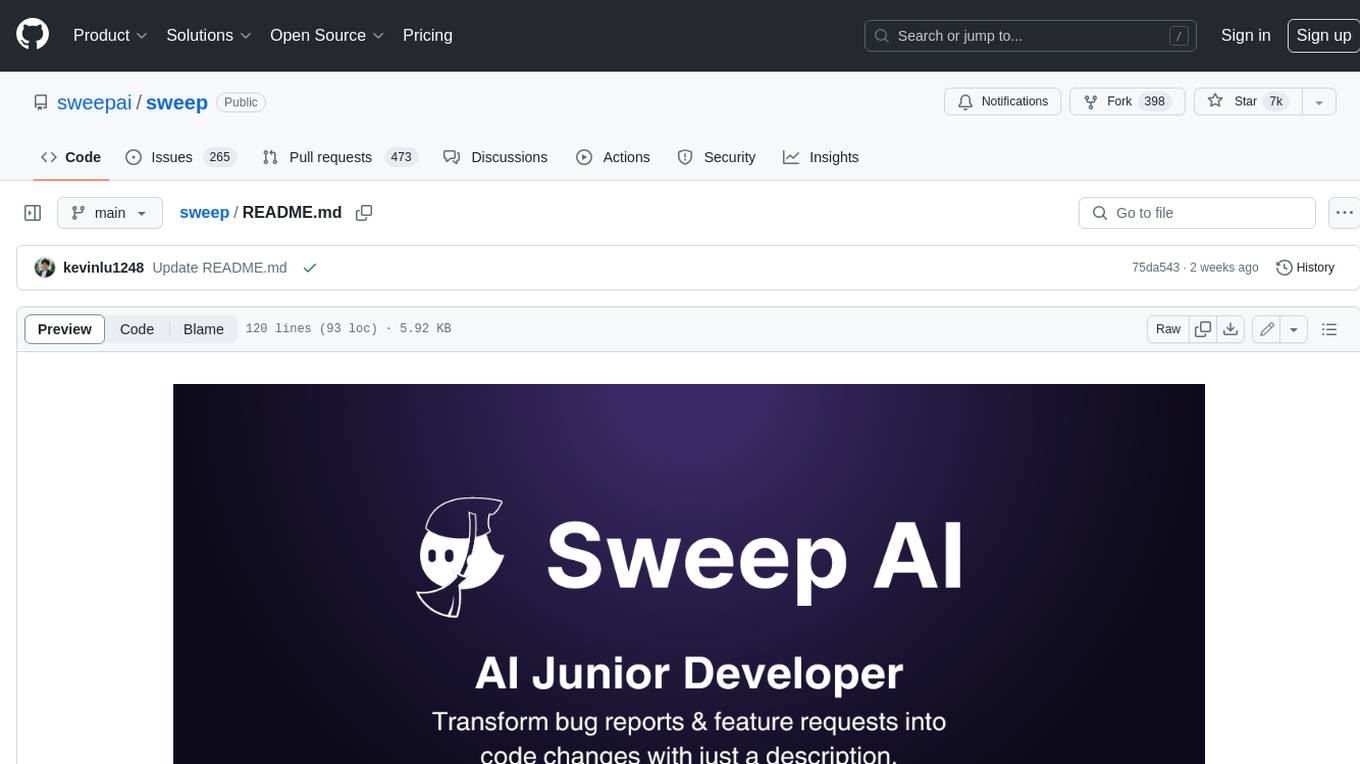
sweep
Sweep is an AI junior developer that turns bugs and feature requests into code changes. It automatically handles developer experience improvements like adding type hints and improving test coverage.
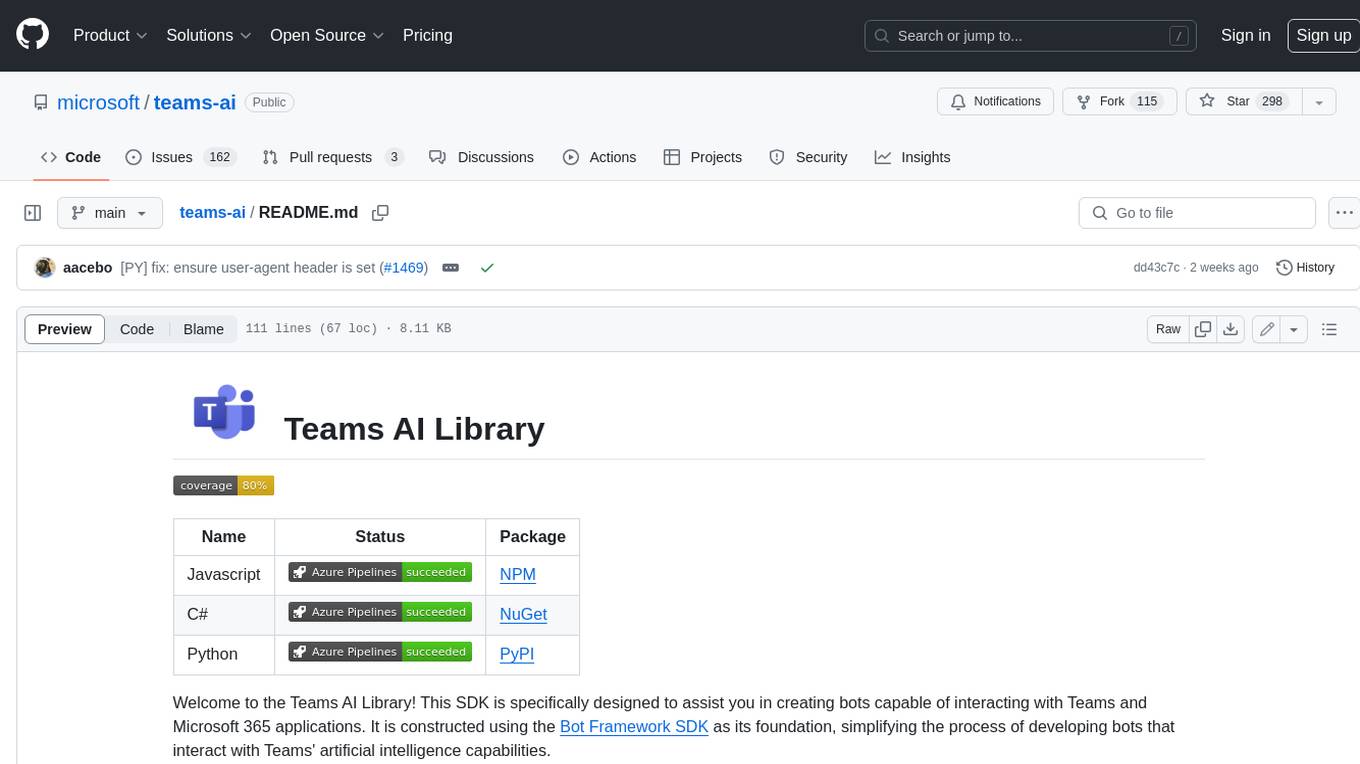
teams-ai
The Teams AI Library is a software development kit (SDK) that helps developers create bots that can interact with Teams and Microsoft 365 applications. It is built on top of the Bot Framework SDK and simplifies the process of developing bots that interact with Teams' artificial intelligence capabilities. The SDK is available for JavaScript/TypeScript, .NET, and Python.
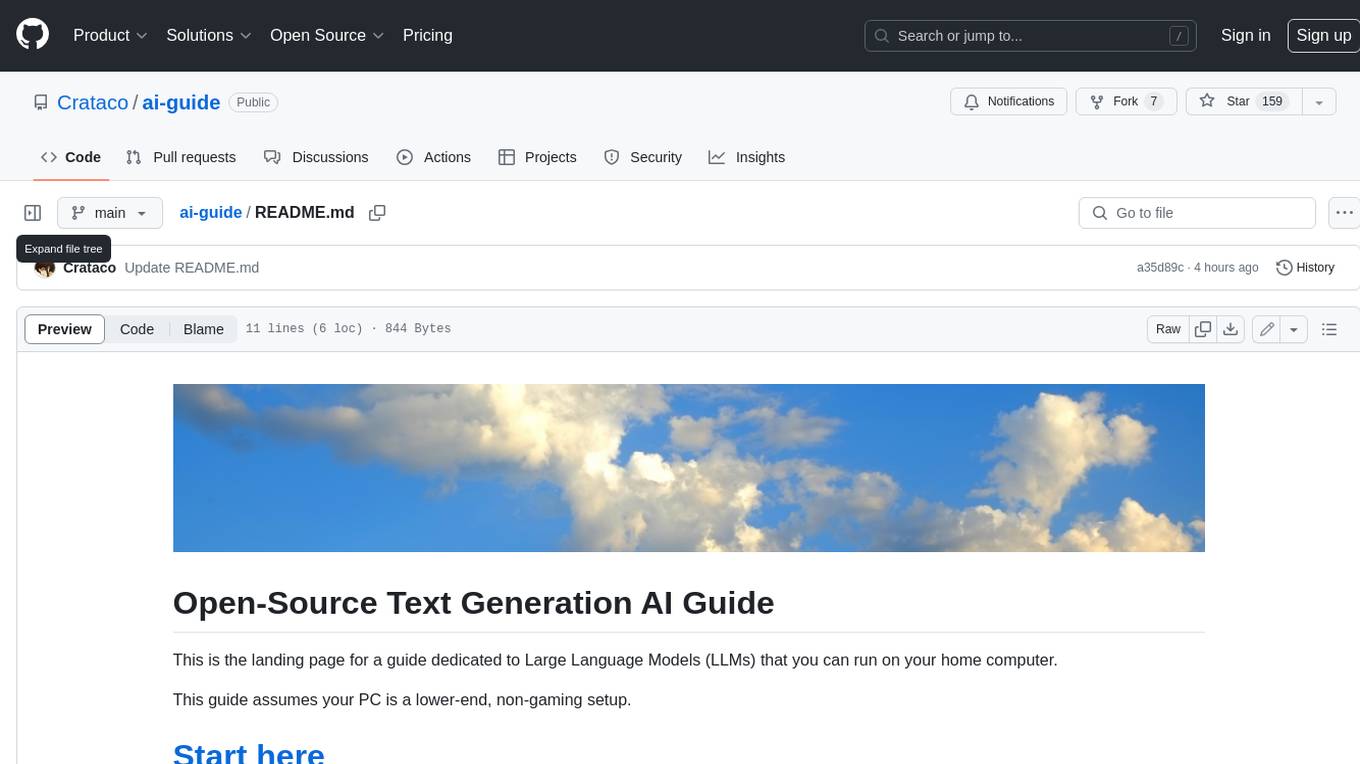
ai-guide
This guide is dedicated to Large Language Models (LLMs) that you can run on your home computer. It assumes your PC is a lower-end, non-gaming setup.
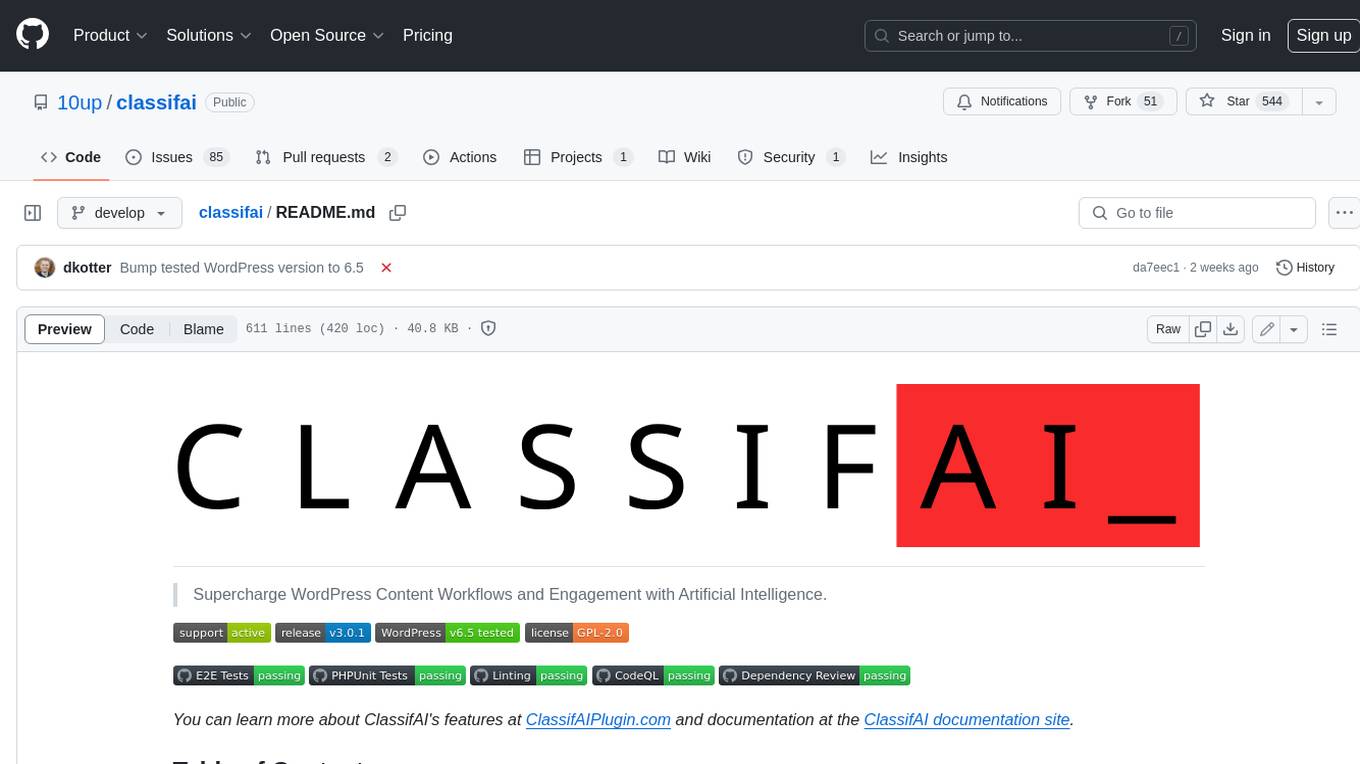
classifai
Supercharge WordPress Content Workflows and Engagement with Artificial Intelligence. Tap into leading cloud-based services like OpenAI, Microsoft Azure AI, Google Gemini and IBM Watson to augment your WordPress-powered websites. Publish content faster while improving SEO performance and increasing audience engagement. ClassifAI integrates Artificial Intelligence and Machine Learning technologies to lighten your workload and eliminate tedious tasks, giving you more time to create original content that matters.
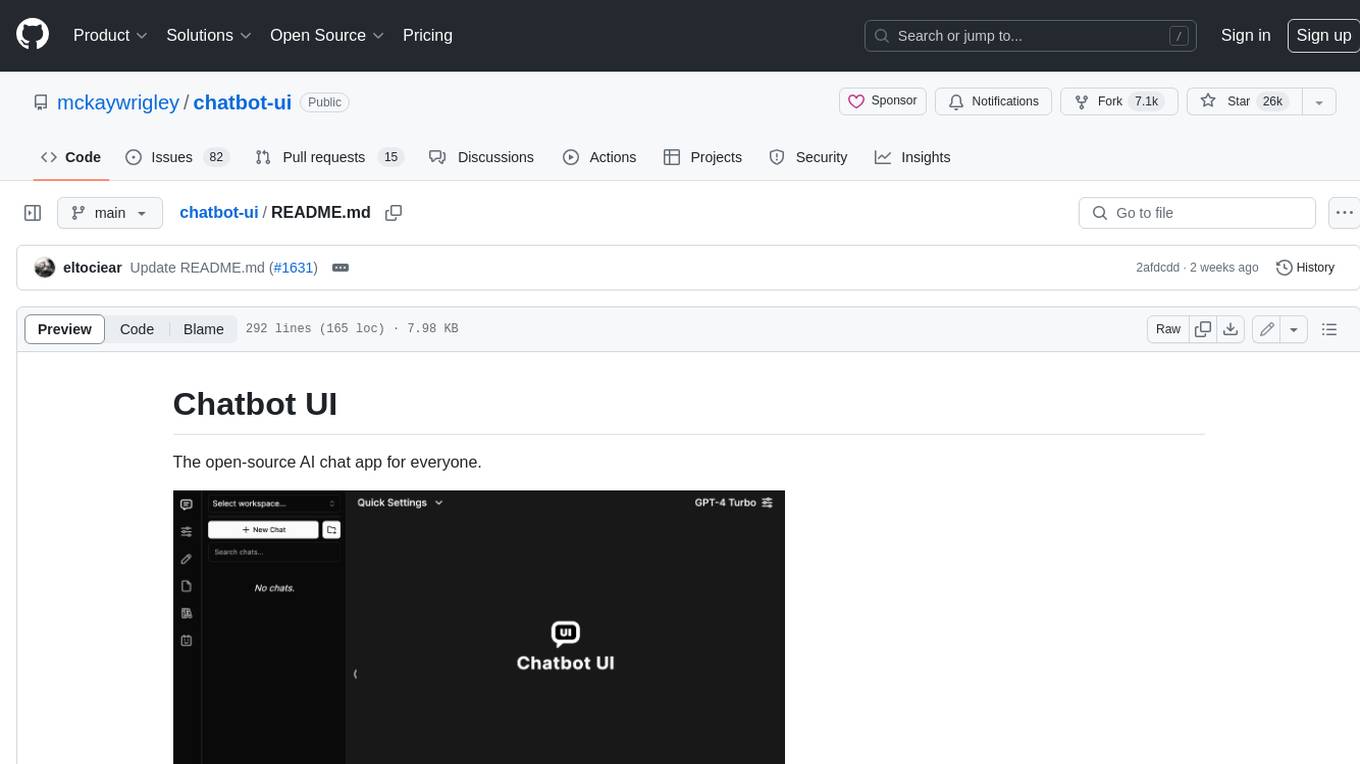
chatbot-ui
Chatbot UI is an open-source AI chat app that allows users to create and deploy their own AI chatbots. It is easy to use and can be customized to fit any need. Chatbot UI is perfect for businesses, developers, and anyone who wants to create a chatbot.
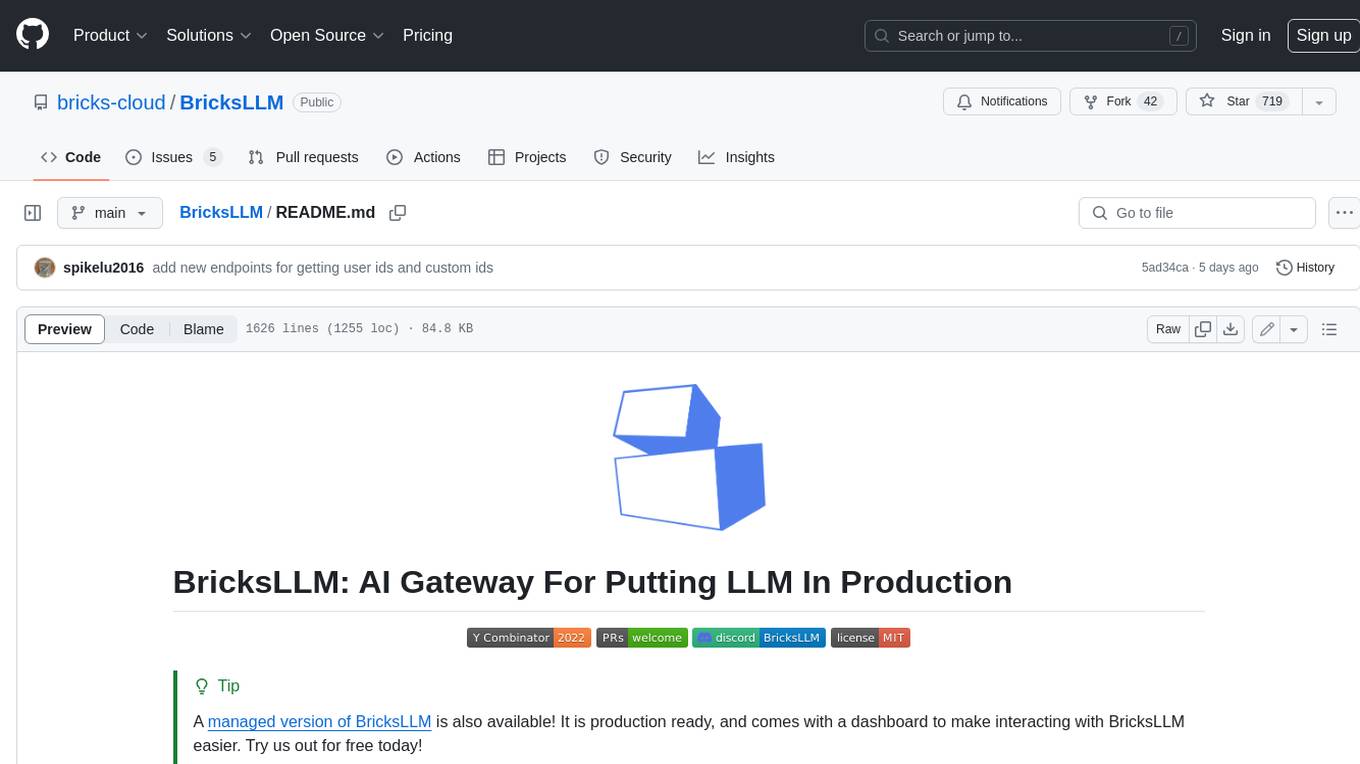
BricksLLM
BricksLLM is a cloud native AI gateway written in Go. Currently, it provides native support for OpenAI, Anthropic, Azure OpenAI and vLLM. BricksLLM aims to provide enterprise level infrastructure that can power any LLM production use cases. Here are some use cases for BricksLLM: * Set LLM usage limits for users on different pricing tiers * Track LLM usage on a per user and per organization basis * Block or redact requests containing PIIs * Improve LLM reliability with failovers, retries and caching * Distribute API keys with rate limits and cost limits for internal development/production use cases * Distribute API keys with rate limits and cost limits for students
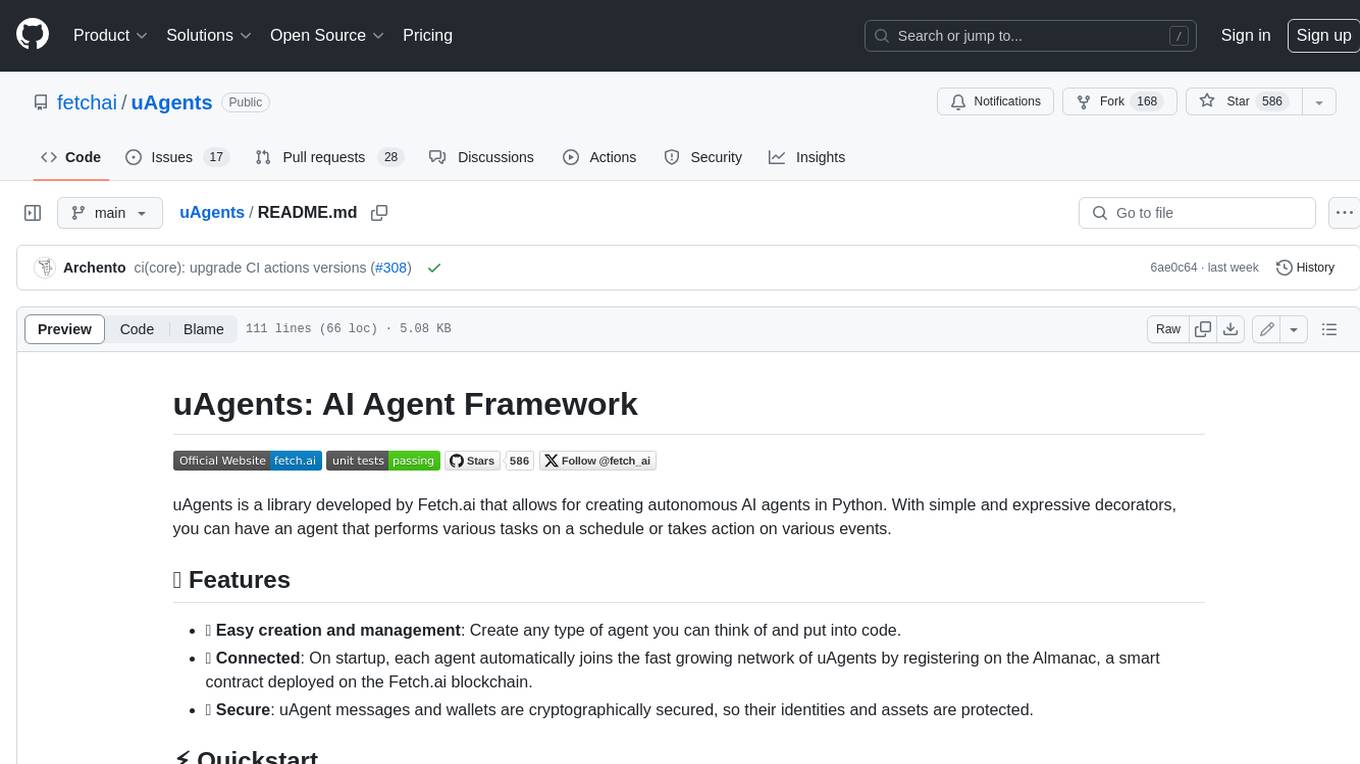
uAgents
uAgents is a Python library developed by Fetch.ai that allows for the creation of autonomous AI agents. These agents can perform various tasks on a schedule or take action on various events. uAgents are easy to create and manage, and they are connected to a fast-growing network of other uAgents. They are also secure, with cryptographically secured messages and wallets.
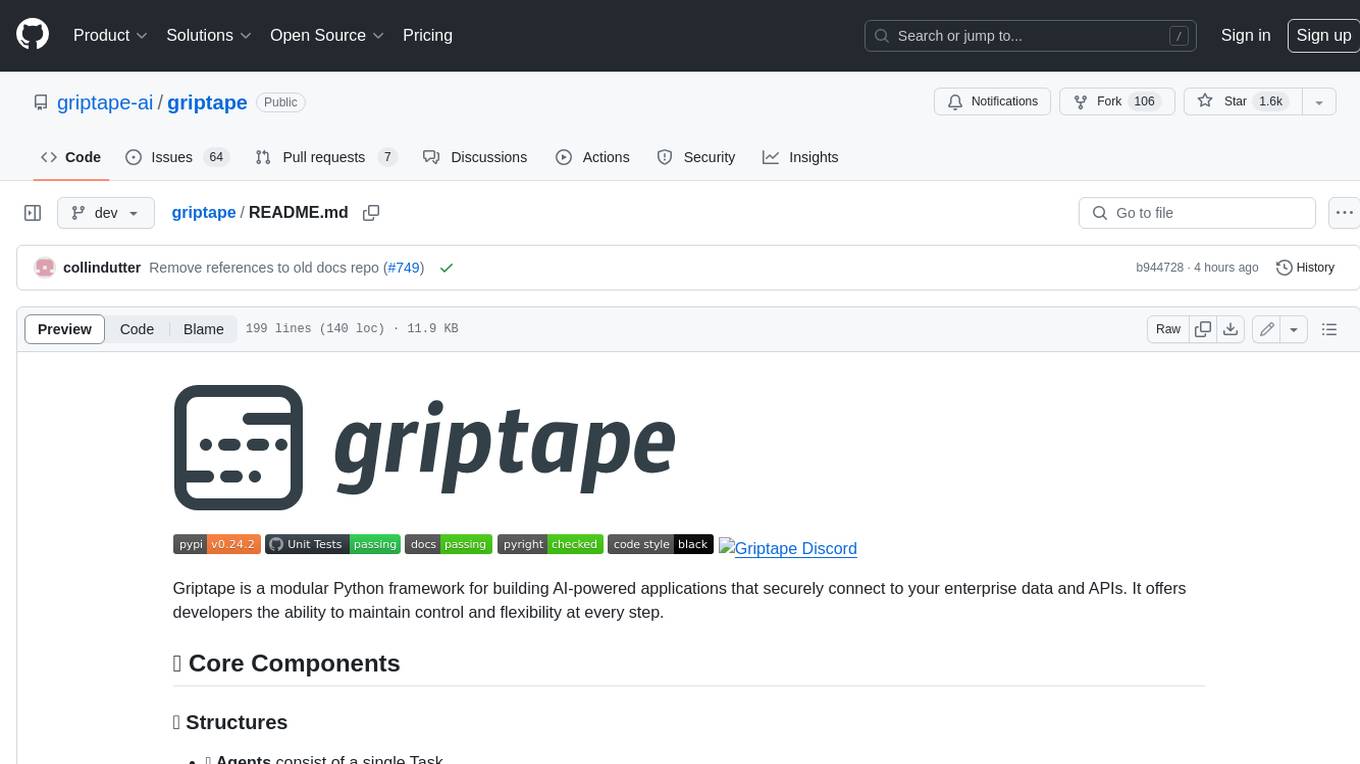
griptape
Griptape is a modular Python framework for building AI-powered applications that securely connect to your enterprise data and APIs. It offers developers the ability to maintain control and flexibility at every step. Griptape's core components include Structures (Agents, Pipelines, and Workflows), Tasks, Tools, Memory (Conversation Memory, Task Memory, and Meta Memory), Drivers (Prompt and Embedding Drivers, Vector Store Drivers, Image Generation Drivers, Image Query Drivers, SQL Drivers, Web Scraper Drivers, and Conversation Memory Drivers), Engines (Query Engines, Extraction Engines, Summary Engines, Image Generation Engines, and Image Query Engines), and additional components (Rulesets, Loaders, Artifacts, Chunkers, and Tokenizers). Griptape enables developers to create AI-powered applications with ease and efficiency.