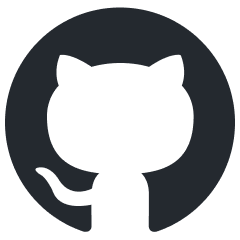
WeClone
欢迎star⭐。使用微信聊天记录微调大语言模型,使用微信语音消息大模➕0.5B大模型实现高质量声音克隆,并绑定到微信机器人,实现自己的数字分身。 数字克隆/数字分身/声音克隆/LLM/大语言模型/微信聊天机器人/LoRA
Stars: 368
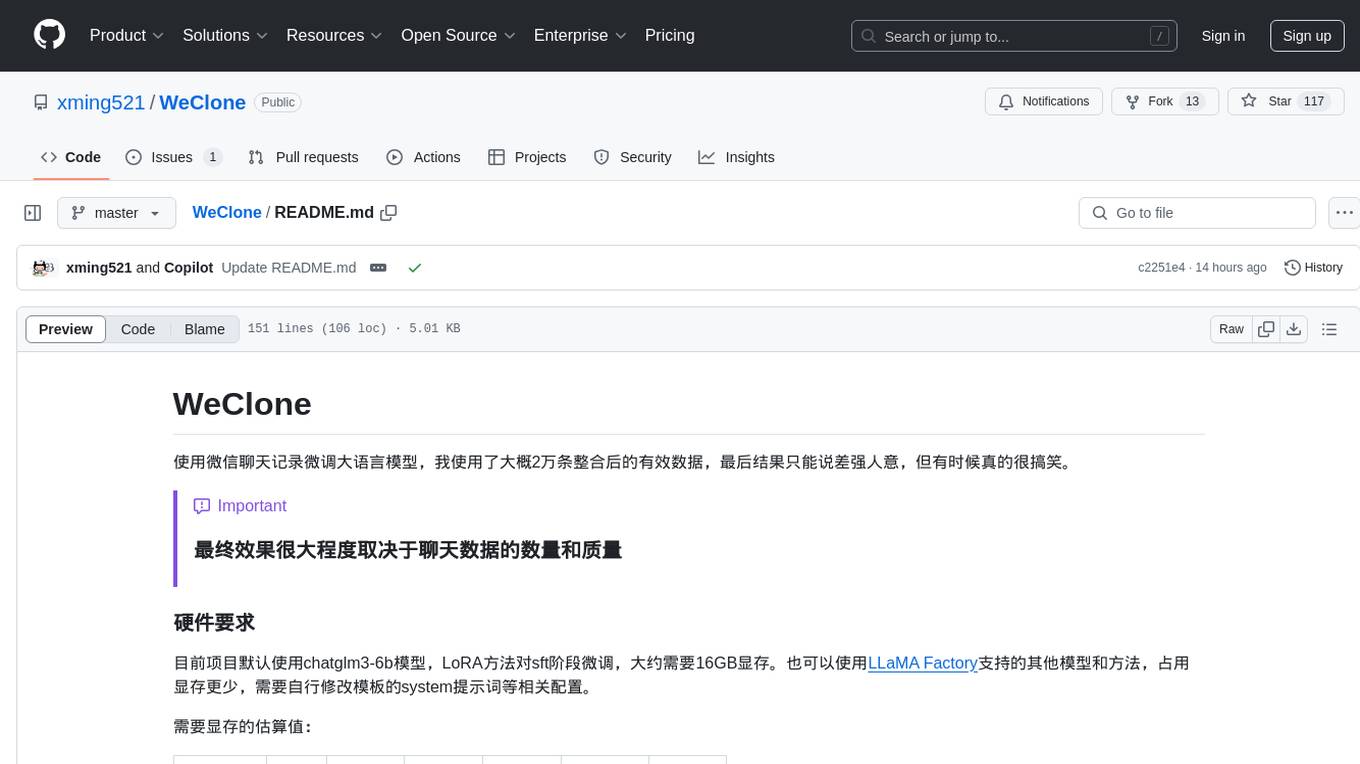
WeClone is a tool that fine-tunes large language models using WeChat chat records. It utilizes approximately 20,000 integrated and effective data points, resulting in somewhat satisfactory outcomes that are occasionally humorous. The tool's effectiveness largely depends on the quantity and quality of the chat data provided. It requires a minimum of 16GB of GPU memory for training using the default chatglm3-6b model with LoRA method. Users can also opt for other models and methods supported by LLAMA Factory, which consume less memory. The tool has specific hardware and software requirements, including Python, Torch, Transformers, Datasets, Accelerate, and other optional packages like CUDA and Deepspeed. The tool facilitates environment setup, data preparation, data preprocessing, model downloading, parameter configuration, model fine-tuning, and inference through a browser demo or API service. Additionally, it offers the ability to deploy a WeChat chatbot, although users should be cautious due to the risk of account suspension by WeChat.
README:
- 💬 使用微信聊天记录微调LLM
- 🎙️ 使用微信语音消息➕0.5B大模型实现高质量声音克隆 👉WeClone-audio
- 🔗 绑定到微信机器人,实现自己的数字分身
[!TIP] 新特性:WeClone-audio 模块,支持对微信语音进行克隆。
[!NOTE] 聊天机器人后续使用 AstrBot 实现
[!IMPORTANT] 微调LLM最终效果很大程度取决于聊天数据的数量和质量
目前项目默认使用chatglm3-6b模型,LoRA方法对sft阶段微调,大约需要16GB显存。也可以使用LLaMA Factory支持的其他模型和方法,占用显存更少,需要自行修改模板的system提示词等相关配置。
需要显存的估算值:
方法 | 精度 | 7B | 14B | 30B | 70B |
x B |
---|---|---|---|---|---|---|
Full (bf16 or fp16 ) |
32 | 120GB | 240GB | 600GB | 1200GB |
18x GB |
Full (pure_bf16 ) |
16 | 60GB | 120GB | 300GB | 600GB |
8x GB |
Freeze/LoRA/GaLore/APOLLO/BAdam | 16 | 16GB | 32GB | 64GB | 160GB |
2x GB |
QLoRA | 8 | 10GB | 20GB | 40GB | 80GB |
x GB |
QLoRA | 4 | 6GB | 12GB | 24GB | 48GB |
x/2 GB |
QLoRA | 2 | 4GB | 8GB | 16GB | 24GB |
x/4 GB |
建议使用 uv,这是一个非常快速的 Python 环境管理器。安装uv后,您可以使用以下命令创建一个新的Python环境并安装依赖项,注意这不包含xcodec(音频克隆)功能的依赖:
git clone https://github.com/xming521/WeClone.git
cd WeClone
uv venv .venv --python=3.9
source .venv/bin/activate
uv pip install --group main -e .
[!NOTE] 训练以及推理相关配置统一在文件settings.json
请使用PyWxDump提取微信聊天记录。下载软件并解密数据库后,点击聊天备份,导出类型为CSV,可以导出多个联系人或群聊,然后将导出的位于wxdump_tmp/export
的 csv
文件夹放在./data
目录即可,也就是不同人聊天记录的文件夹一起放在 ./data/csv
。 示例数据位于data/example_chat.csv。
项目默认去除了数据中的手机号、身份证号、邮箱、网址。还提供了一个禁用词词库blocked_words,可以自行添加需要过滤的词句(会默认去掉包括禁用词的整句)。
执行 ./make_dataset/csv_to_json.py
脚本对数据进行处理。
在同一人连续回答多句的情况下,有三种处理方式:
文件 | 处理方式 |
---|---|
csv_to_json.py | 用逗号连接 |
csv_to_json-单句回答.py(已废弃) | 只选择最长的回答作为最终数据 |
csv_to_json-单句多轮.py | 放在了提示词的'history'中 |
首选在Hugging Face下载ChatGLM3 模型。如果您在 Hugging Face 模型的下载中遇到了问题,可以通过下述方法使用魔搭社区,后续训练推理都需要先执行export USE_MODELSCOPE_HUB=1
来使用魔搭社区的模型。
由于模型较大,下载过程比较漫长请耐心等待。
export USE_MODELSCOPE_HUB=1 # Windows 使用 `set USE_MODELSCOPE_HUB=1`
git lfs install
git clone https://www.modelscope.cn/ZhipuAI/chatglm3-6b.git
魔搭社区的modeling_chatglm.py
文件需要更换为Hugging Face的
-
(可选)修改 settings.json选择本地下载好的其他模型。
-
修改
per_device_train_batch_size
以及gradient_accumulation_steps
来调整显存占用。 -
可以根据自己数据集的数量和质量修改
num_train_epochs
、lora_rank
、lora_dropout
等参数。
运行 src/train_sft.py
进行sft阶段微调,本人loss只降到了3.5左右,降低过多可能会过拟合,我使用了大概2万条整合后的有效数据。
python src/train_sft.py
pip install deepspeed
deepspeed --num_gpus=使用显卡数量 src/train_sft.py
python ./src/web_demo.py
python ./src/api_service.py
python ./src/api_service.py
python ./src/test_model.py
[!IMPORTANT]
微信有封号风险,建议使用小号,并且必须绑定银行卡才能使用
python ./src/api_service.py # 先启动api服务
python ./src/wechat_bot/main.py
默认在终端显示二维码,扫码登录即可。可以私聊或者在群聊中@机器人使用。
Todo
Todo
[!CAUTION] 请勿用于非法用途,否则后果自负。
- 本项目仅供学习交流使用,请勿用于非法用途,请勿用于非法用途,请勿用于非法用途,否则后果自负。
- 用户理解并同意,任何违反法律法规、侵犯他人合法权益的行为,均与本项目及其开发者无关,后果由用户自行承担。
- 您应该在下载保存使用本项目的24小时内,删除本项目的源代码和程序;超出此期限的任何使用行为,一概与本项目及其开发者无关。
- 本项目仅允许在授权情况下使用数据训练,严禁用于非法目的,否则自行承担所有相关责任;用户如因违反此规定而引发的任何法律责任,将由用户自行承担,与本项目及其开发者无关。
- 严禁用于窃取他人隐私,严禁用于窃取他人隐私,严禁用于窃取他人隐私,否则自行承担所有相关责任。
- 下载、保存、进一步浏览源代码或者下载安装、编译使用本程序,表示你同意本警告,并承诺遵守它;
- 禁止利用本项目的相关技术从事非法测试或渗透,禁止利用本项目的相关代码或相关技术从事任何非法工作,如因此产生的一切不良后果与本项目及其开发者无关。
- 任何因此产生的不良后果,包括但不限于数据泄露、系统瘫痪、侵犯隐私等,均与本项目及其开发者无关,责任由用户自行承担。
- 本免责声明可能根据项目运行情况和法律法规的变化进行修改和调整。用户应定期查阅本页面以获取最新版本的免责声明,使用本项目时应遵守最新版本的免责声明。
-
除本免责声明规定外,用户在使用本项目过程中应遵守相关的法律法规和道德规范。对于因用户违反相关规定而引发的任何纠纷或损失,本项目及其开发者不承担任何责任。
-
请用户慎重阅读并理解本免责声明的所有内容,确保在使用本项目时严格遵守相关规定。
请用户慎重阅读并理解本免责声明的所有内容,确保在使用本项目时严格遵守相关规定。
For Tasks:
Click tags to check more tools for each tasksFor Jobs:
Alternative AI tools for WeClone
Similar Open Source Tools
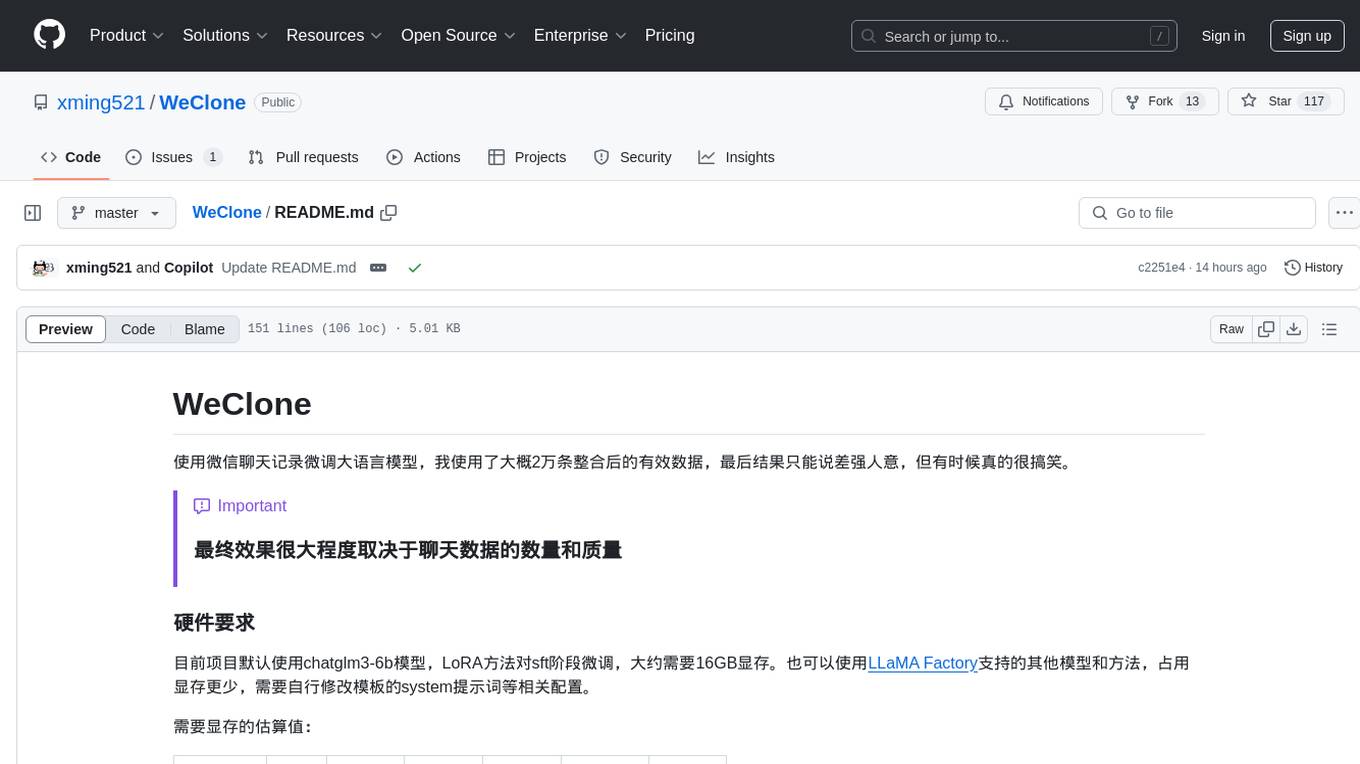
WeClone
WeClone is a tool that fine-tunes large language models using WeChat chat records. It utilizes approximately 20,000 integrated and effective data points, resulting in somewhat satisfactory outcomes that are occasionally humorous. The tool's effectiveness largely depends on the quantity and quality of the chat data provided. It requires a minimum of 16GB of GPU memory for training using the default chatglm3-6b model with LoRA method. Users can also opt for other models and methods supported by LLAMA Factory, which consume less memory. The tool has specific hardware and software requirements, including Python, Torch, Transformers, Datasets, Accelerate, and other optional packages like CUDA and Deepspeed. The tool facilitates environment setup, data preparation, data preprocessing, model downloading, parameter configuration, model fine-tuning, and inference through a browser demo or API service. Additionally, it offers the ability to deploy a WeChat chatbot, although users should be cautious due to the risk of account suspension by WeChat.
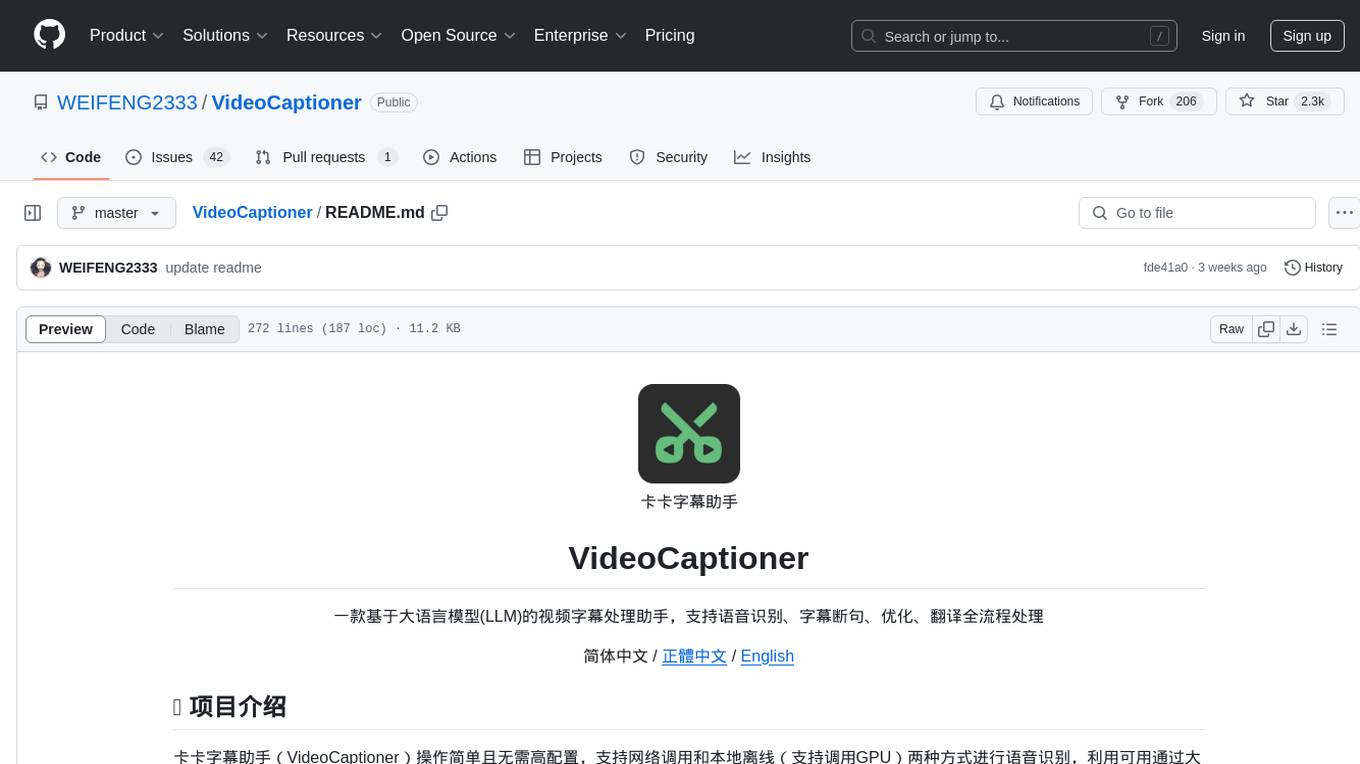
VideoCaptioner
VideoCaptioner is a video subtitle processing assistant based on a large language model (LLM), supporting speech recognition, subtitle segmentation, optimization, translation, and full-process handling. It is user-friendly and does not require high configuration, supporting both network calls and local offline (GPU-enabled) speech recognition. It utilizes a large language model for intelligent subtitle segmentation, correction, and translation, providing stunning subtitles for videos. The tool offers features such as accurate subtitle generation without GPU, intelligent segmentation and sentence splitting based on LLM, AI subtitle optimization and translation, batch video subtitle synthesis, intuitive subtitle editing interface with real-time preview and quick editing, and low model token consumption with built-in basic LLM model for easy use.
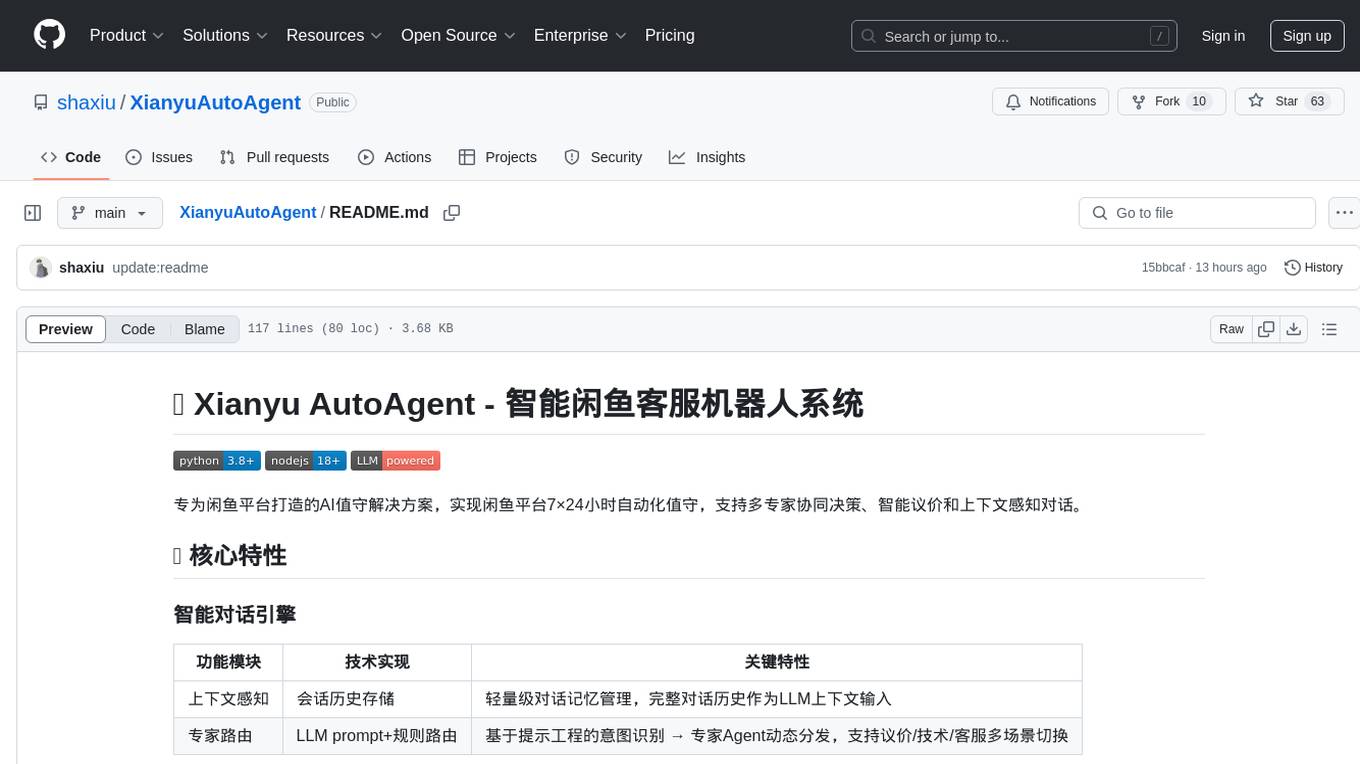
XianyuAutoAgent
Xianyu AutoAgent is an AI customer service robot system specifically designed for the Xianyu platform, providing 24/7 automated customer service, supporting multi-expert collaborative decision-making, intelligent bargaining, and context-aware conversations. The system includes intelligent conversation engine with features like context awareness and expert routing, business function matrix with modules like core engine, bargaining system, technical support, and operation monitoring. It requires Python 3.8+ and NodeJS 18+ for installation and operation. Users can customize prompts for different experts and contribute to the project through issues or pull requests.
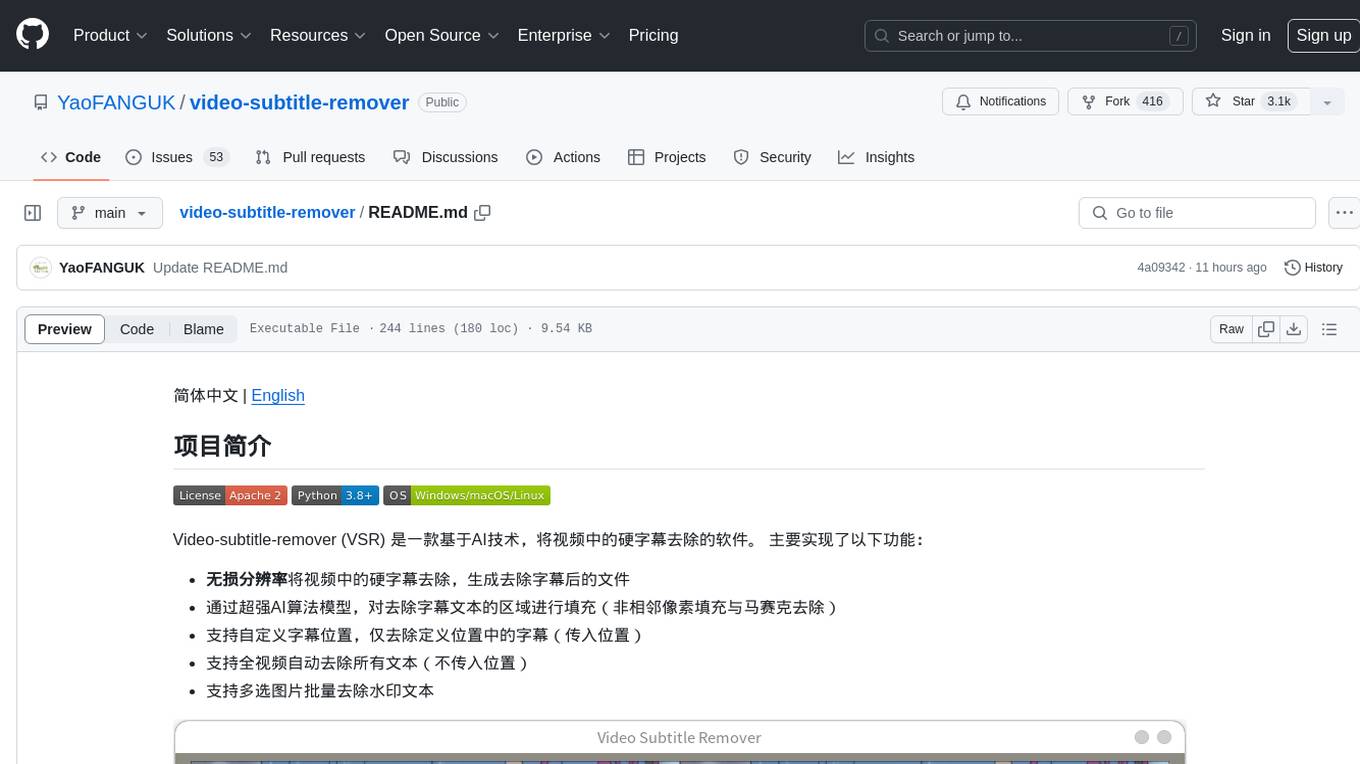
video-subtitle-remover
Video-subtitle-remover (VSR) is a software based on AI technology that removes hard subtitles from videos. It achieves the following functions: - Lossless resolution: Remove hard subtitles from videos, generate files with subtitles removed - Fill the region of removed subtitles using a powerful AI algorithm model (non-adjacent pixel filling and mosaic removal) - Support custom subtitle positions, only remove subtitles in defined positions (input position) - Support automatic removal of all text in the entire video (no input position required) - Support batch removal of watermark text from multiple images.
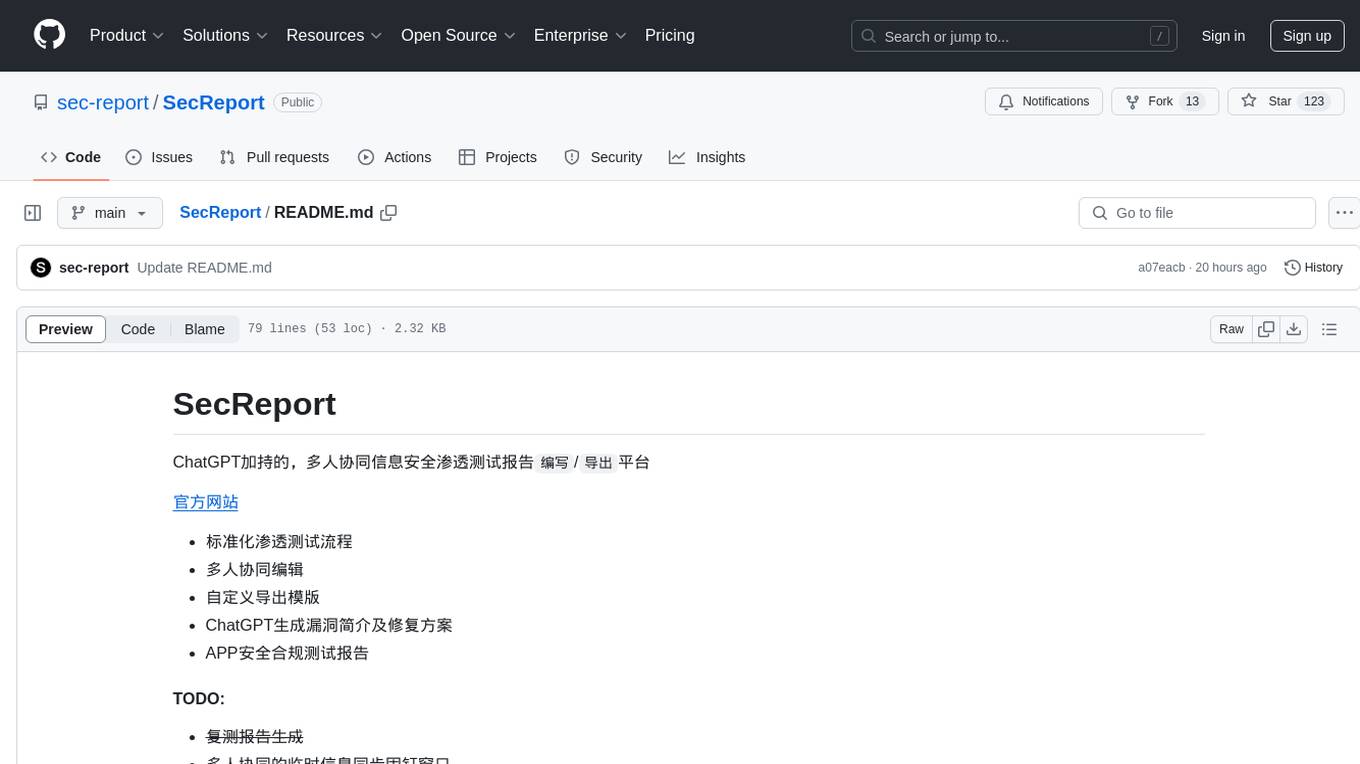
SecReport
SecReport is a platform for collaborative information security penetration testing report writing and exporting, powered by ChatGPT. It standardizes penetration testing processes, allows multiple users to edit reports, offers custom export templates, generates vulnerability summaries and fix suggestions using ChatGPT, and provides APP security compliance testing reports. The tool aims to streamline the process of creating and managing security reports for penetration testing and compliance purposes.
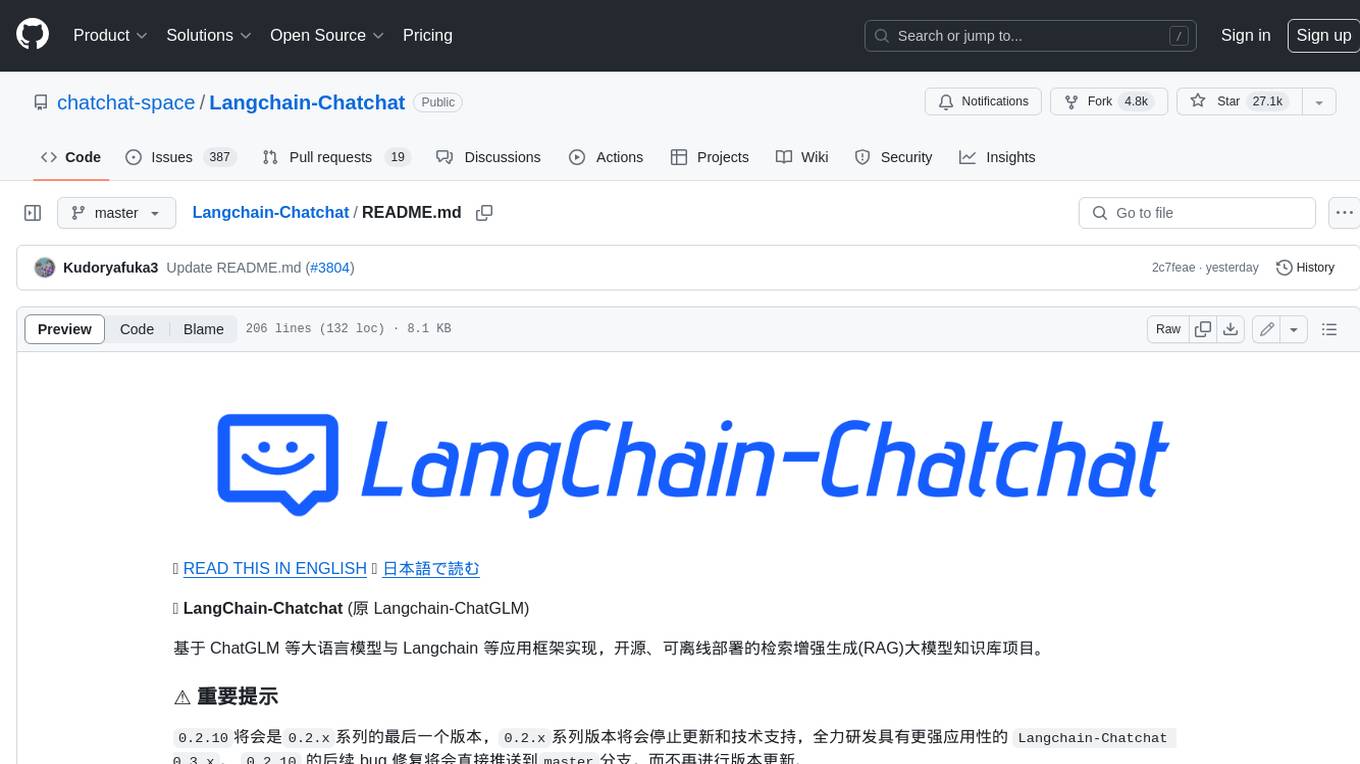
Langchain-Chatchat
LangChain-Chatchat is an open-source, offline-deployable retrieval-enhanced generation (RAG) large model knowledge base project based on large language models such as ChatGLM and application frameworks such as Langchain. It aims to establish a knowledge base Q&A solution that is friendly to Chinese scenarios, supports open-source models, and can run offline.
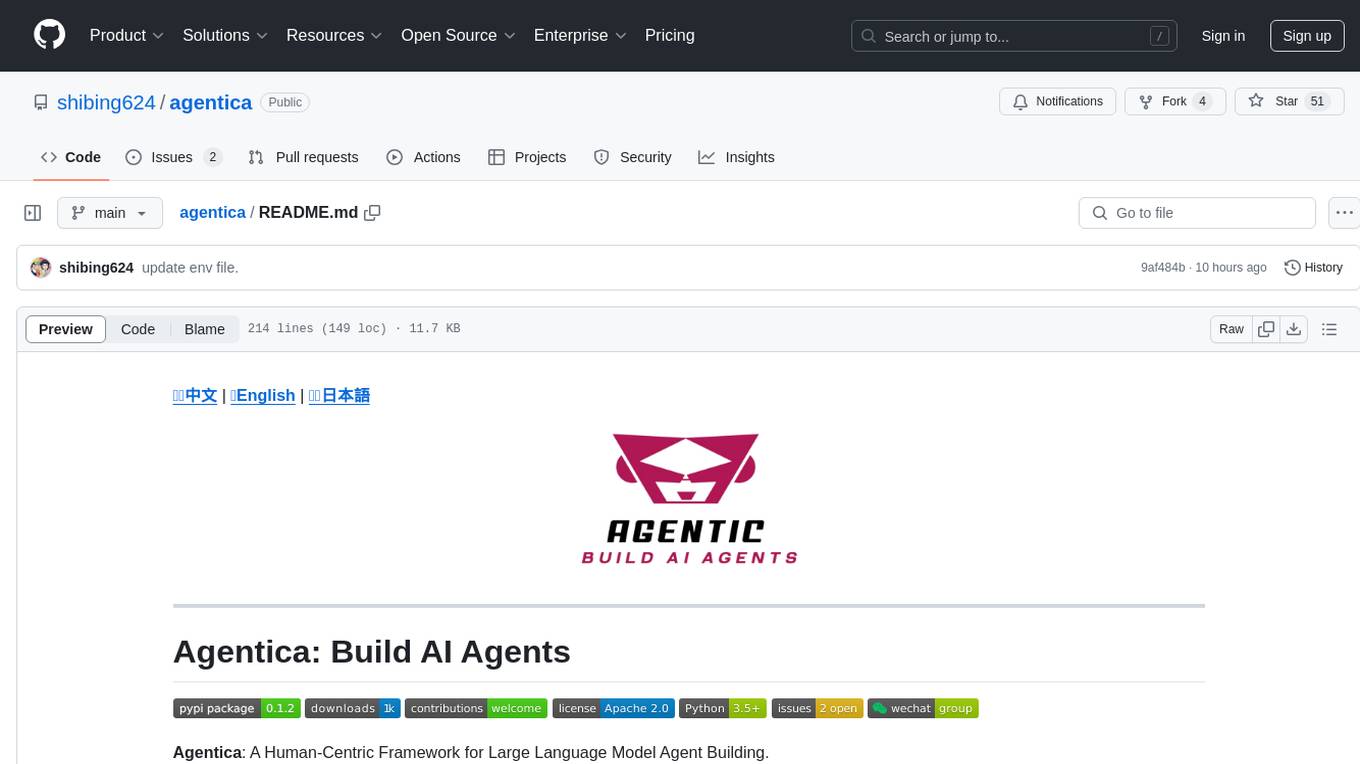
agentica
Agentica is a human-centric framework for building large language model agents. It provides functionalities for planning, memory management, tool usage, and supports features like reflection, planning and execution, RAG, multi-agent, multi-role, and workflow. The tool allows users to quickly code and orchestrate agents, customize prompts, and make API calls to various services. It supports API calls to OpenAI, Azure, Deepseek, Moonshot, Claude, Ollama, and Together. Agentica aims to simplify the process of building AI agents by providing a user-friendly interface and a range of functionalities for agent development.
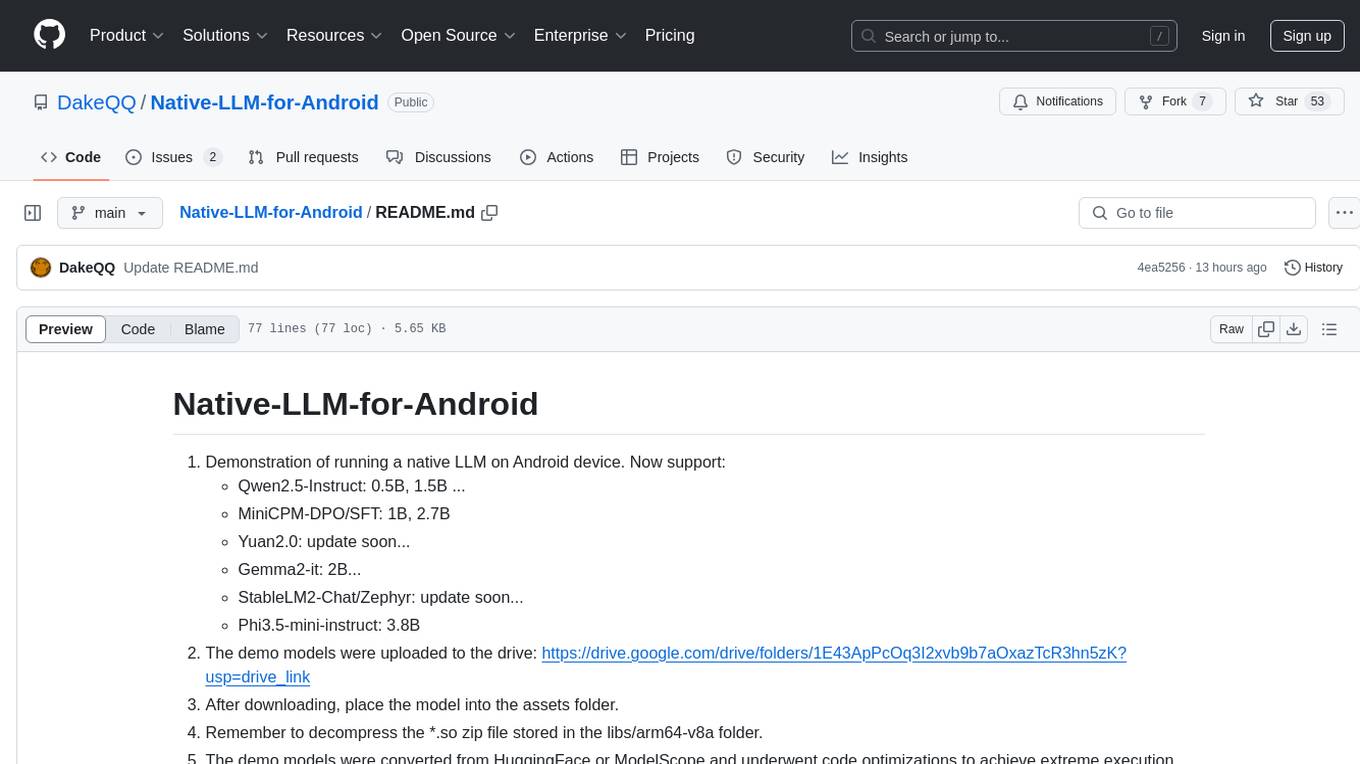
Native-LLM-for-Android
This repository provides a demonstration of running a native Large Language Model (LLM) on Android devices. It supports various models such as Qwen2.5-Instruct, MiniCPM-DPO/SFT, Yuan2.0, Gemma2-it, StableLM2-Chat/Zephyr, and Phi3.5-mini-instruct. The demo models are optimized for extreme execution speed after being converted from HuggingFace or ModelScope. Users can download the demo models from the provided drive link, place them in the assets folder, and follow specific instructions for decompression and model export. The repository also includes information on quantization methods and performance benchmarks for different models on various devices.
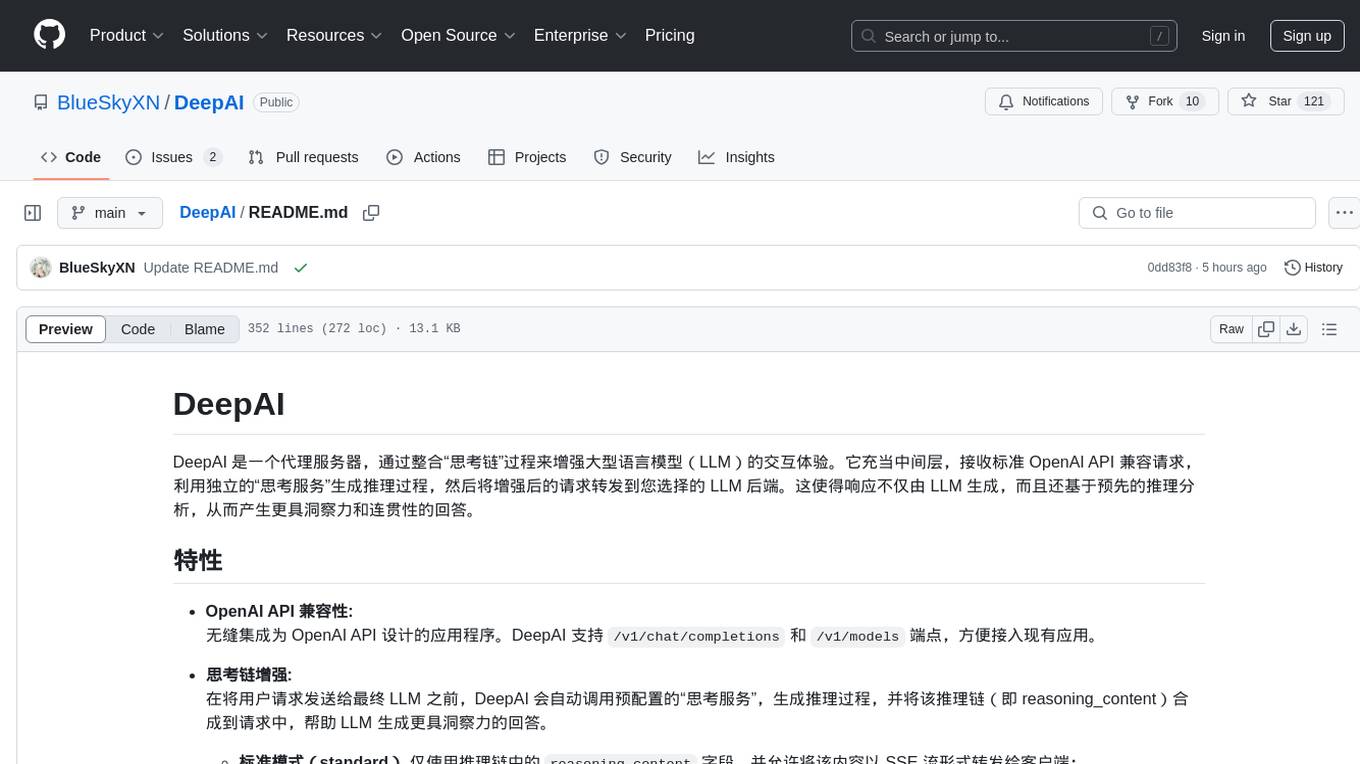
DeepAI
DeepAI is a proxy server that enhances the interaction experience of large language models (LLMs) by integrating the 'thinking chain' process. It acts as an intermediary layer, receiving standard OpenAI API compatible requests, using independent 'thinking services' to generate reasoning processes, and then forwarding the enhanced requests to the LLM backend of your choice. This ensures that responses are not only generated by the LLM but also based on pre-inference analysis, resulting in more insightful and coherent answers. DeepAI supports seamless integration with applications designed for the OpenAI API, providing endpoints for '/v1/chat/completions' and '/v1/models', making it easy to integrate into existing applications. It offers features such as reasoning chain enhancement, flexible backend support, API key routing, weighted random selection, proxy support, comprehensive logging, and graceful shutdown.
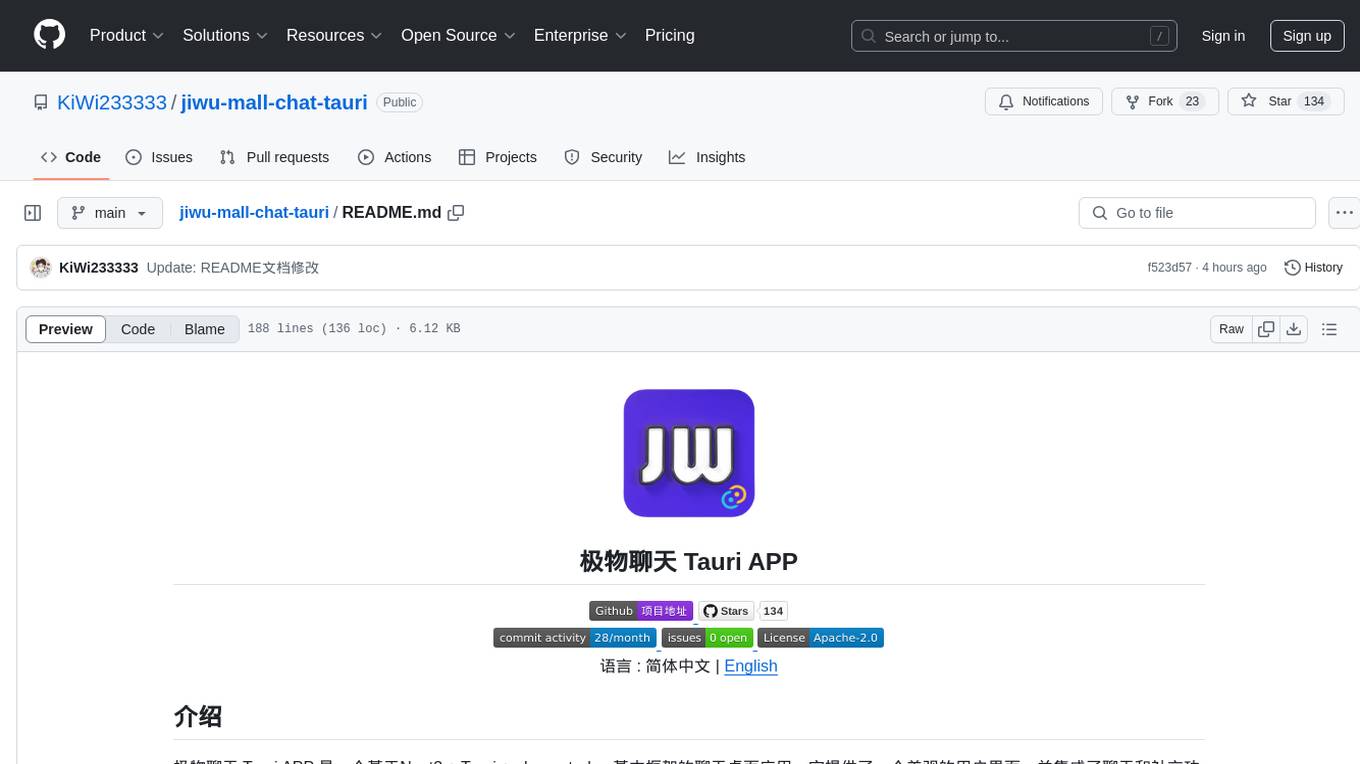
jiwu-mall-chat-tauri
Jiwu Chat Tauri APP is a desktop chat application based on Nuxt3 + Tauri + Element Plus framework. It provides a beautiful user interface with integrated chat and social functions. It also supports AI shopping chat and global dark mode. Users can engage in real-time chat, share updates, and interact with AI customer service through this application.
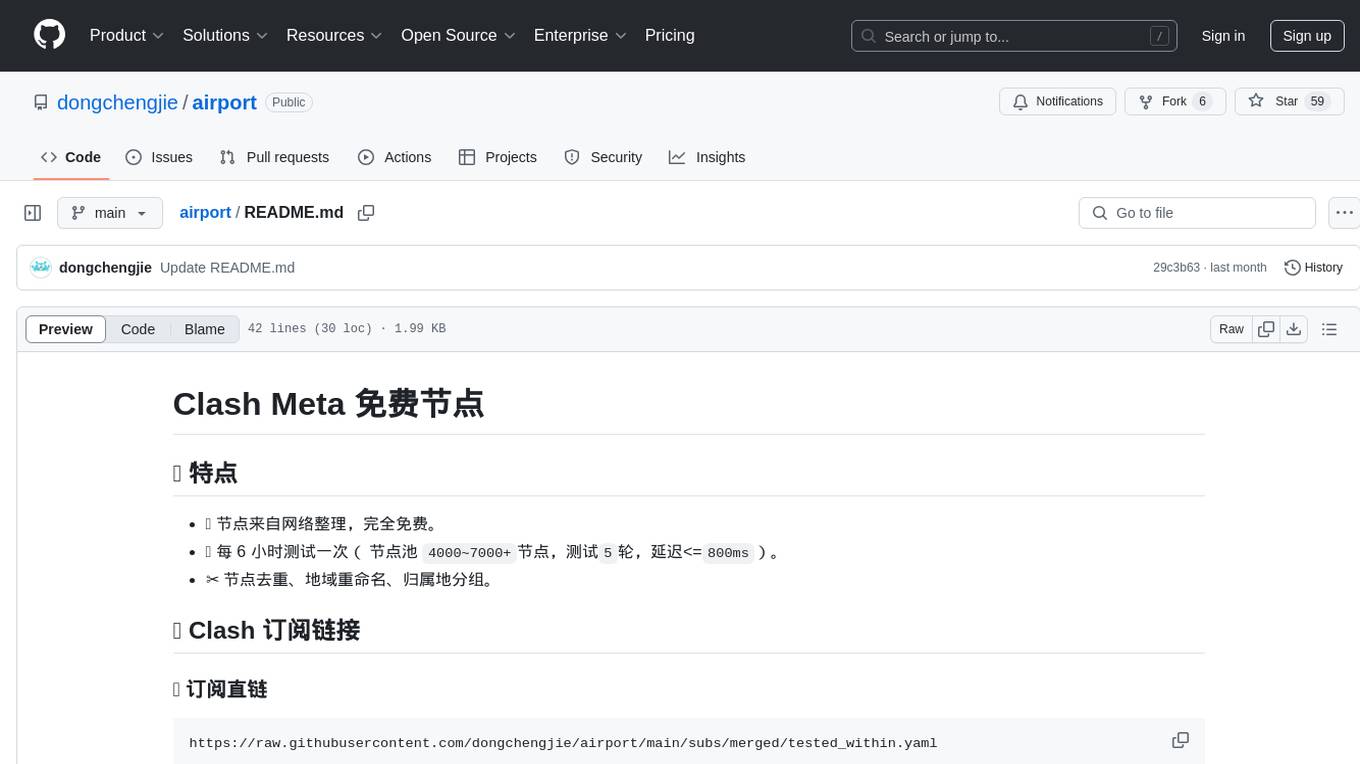
airport
The 'airport' repository provides free Clash Meta nodes sourced from the internet, with testing every 6 hours to ensure quality and low latency. It includes features such as node deduplication, regional renaming, and geographical grouping.
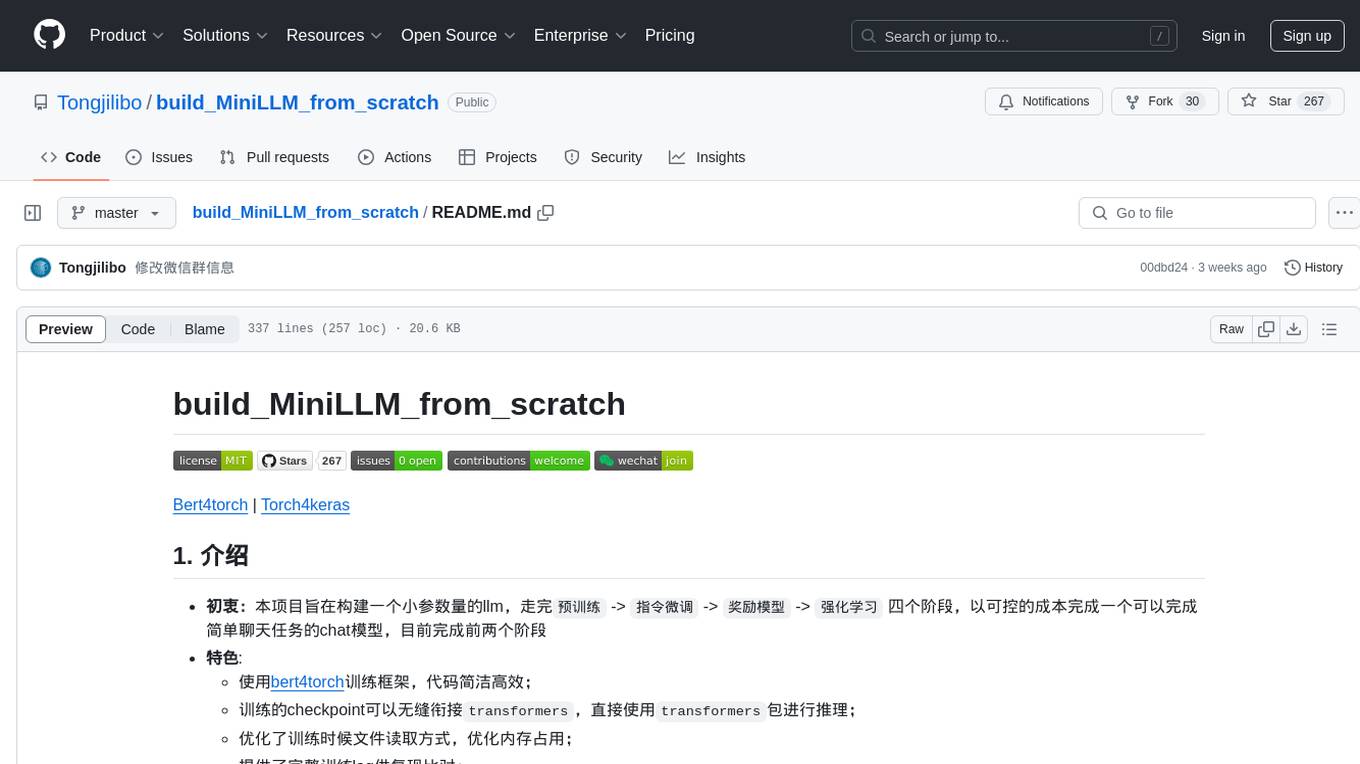
build_MiniLLM_from_scratch
This repository aims to build a low-parameter LLM model through pretraining, fine-tuning, model rewarding, and reinforcement learning stages to create a chat model capable of simple conversation tasks. It features using the bert4torch training framework, seamless integration with transformers package for inference, optimized file reading during training to reduce memory usage, providing complete training logs for reproducibility, and the ability to customize robot attributes. The chat model supports multi-turn conversations. The trained model currently only supports basic chat functionality due to limitations in corpus size, model scale, SFT corpus size, and quality.
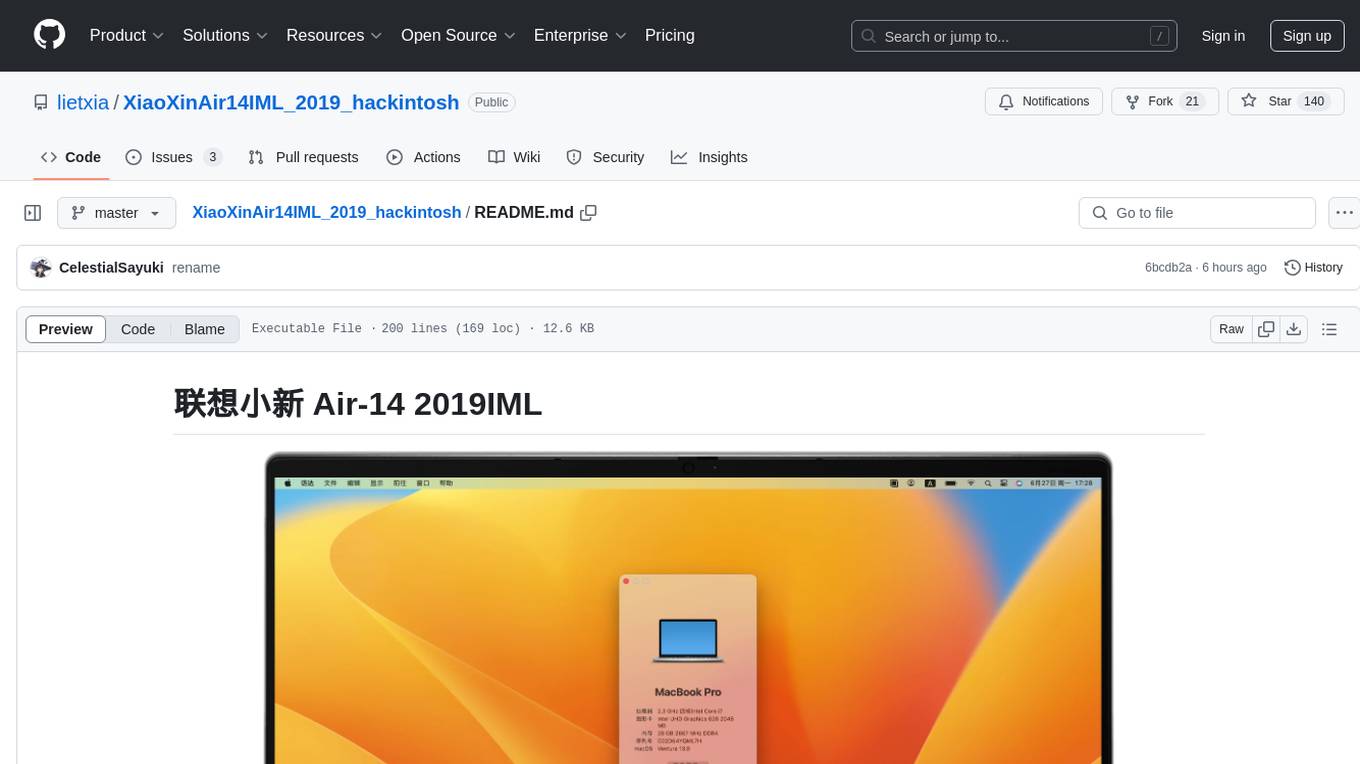
XiaoXinAir14IML_2019_hackintosh
XiaoXinAir14IML_2019_hackintosh is a repository dedicated to enabling macOS installation on Lenovo XiaoXin Air-14 IML 2019 laptops. The repository provides detailed information on the hardware specifications, supported systems, BIOS versions, related models, installation methods, updates, patches, and recommended settings. It also includes tools and guides for BIOS modifications, enabling high-resolution display settings, Bluetooth synchronization between macOS and Windows 10, voltage adjustments for efficiency, and experimental support for YogaSMC. The repository offers solutions for various issues like sleep support, sound card emulation, and battery information. It acknowledges the contributions of developers and tools like OpenCore, itlwm, VoodooI2C, and ALCPlugFix.
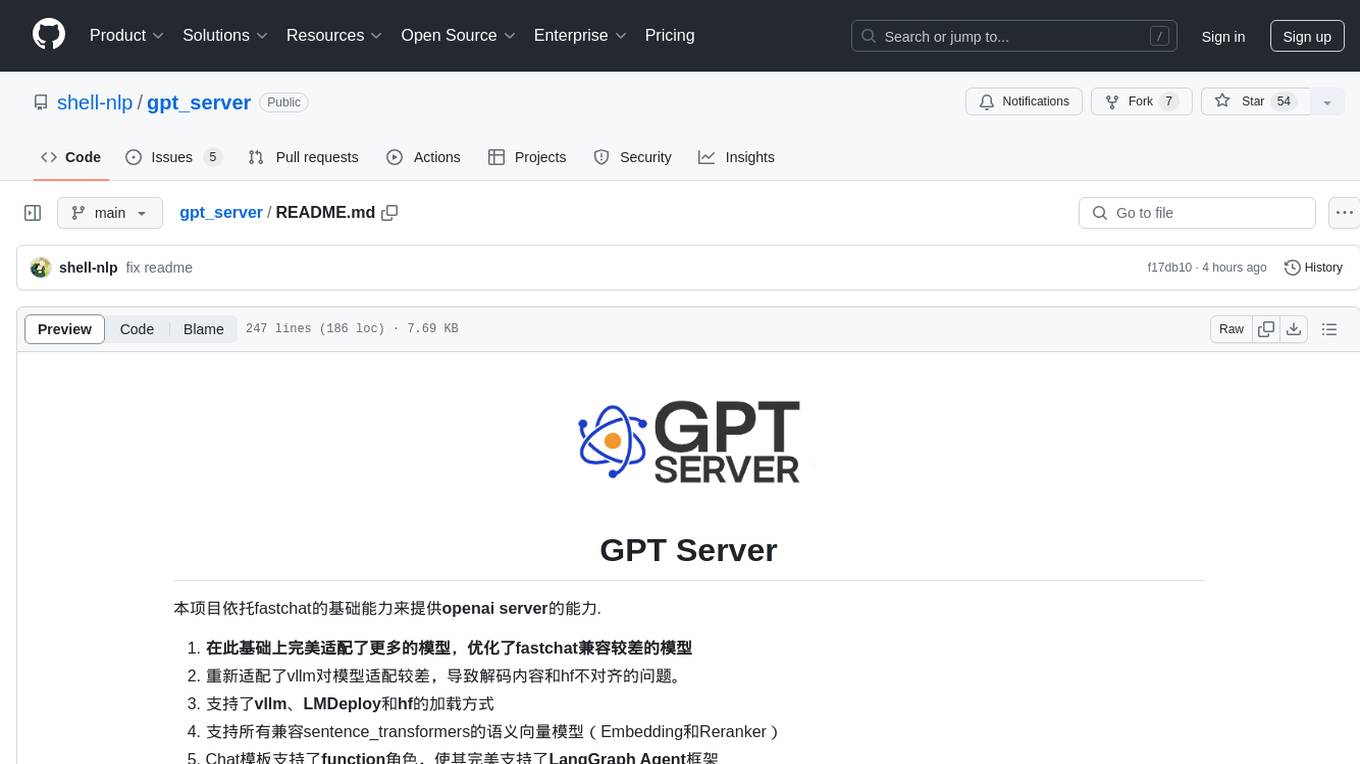
gpt_server
The GPT Server project leverages the basic capabilities of FastChat to provide the capabilities of an openai server. It perfectly adapts more models, optimizes models with poor compatibility in FastChat, and supports loading vllm, LMDeploy, and hf in various ways. It also supports all sentence_transformers compatible semantic vector models, including Chat templates with function roles, Function Calling (Tools) capability, and multi-modal large models. The project aims to reduce the difficulty of model adaptation and project usage, making it easier to deploy the latest models with minimal code changes.
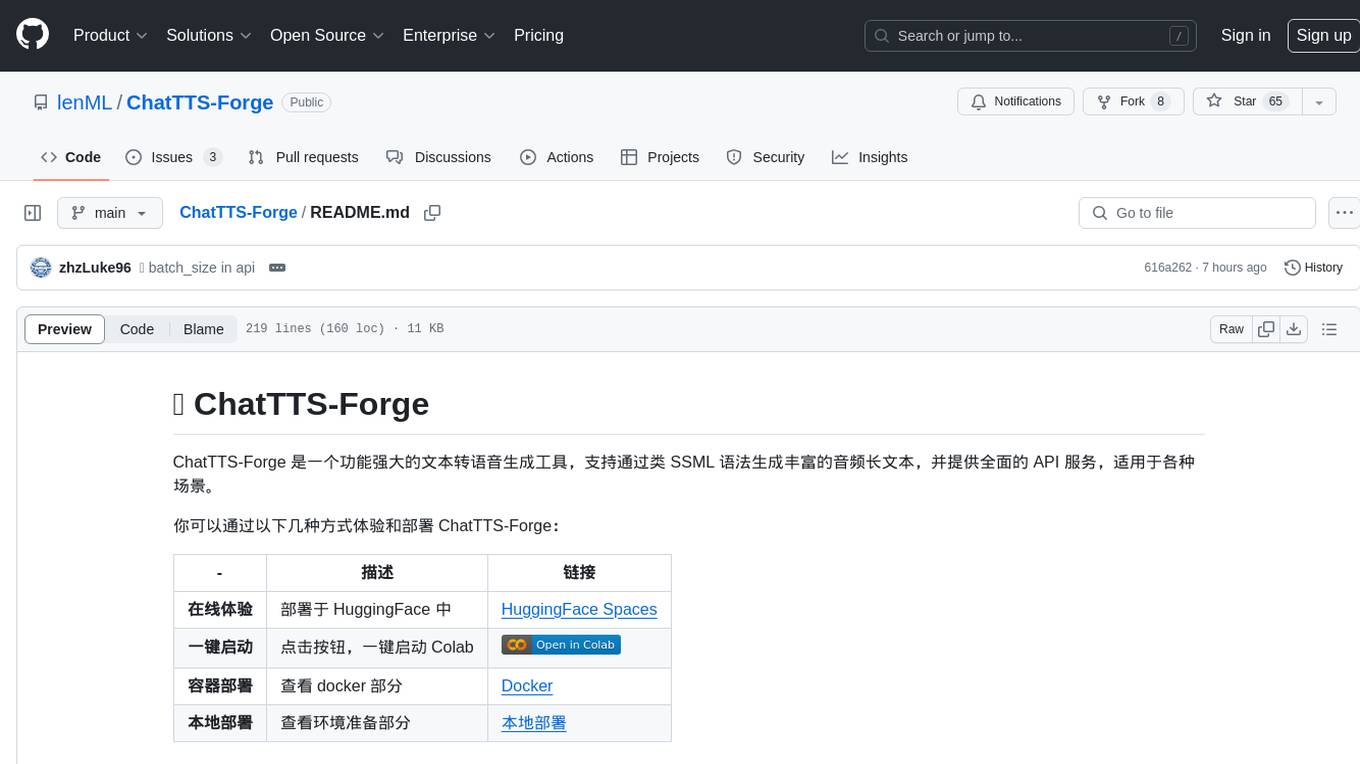
ChatTTS-Forge
ChatTTS-Forge is a powerful text-to-speech generation tool that supports generating rich audio long texts using a SSML-like syntax and provides comprehensive API services, suitable for various scenarios. It offers features such as batch generation, support for generating super long texts, style prompt injection, full API services, user-friendly debugging GUI, OpenAI-style API, Google-style API, support for SSML-like syntax, speaker management, style management, independent refine API, text normalization optimized for ChatTTS, and automatic detection and processing of markdown format text. The tool can be experienced and deployed online through HuggingFace Spaces, launched with one click on Colab, deployed using containers, or locally deployed after cloning the project, preparing models, and installing necessary dependencies.
For similar tasks
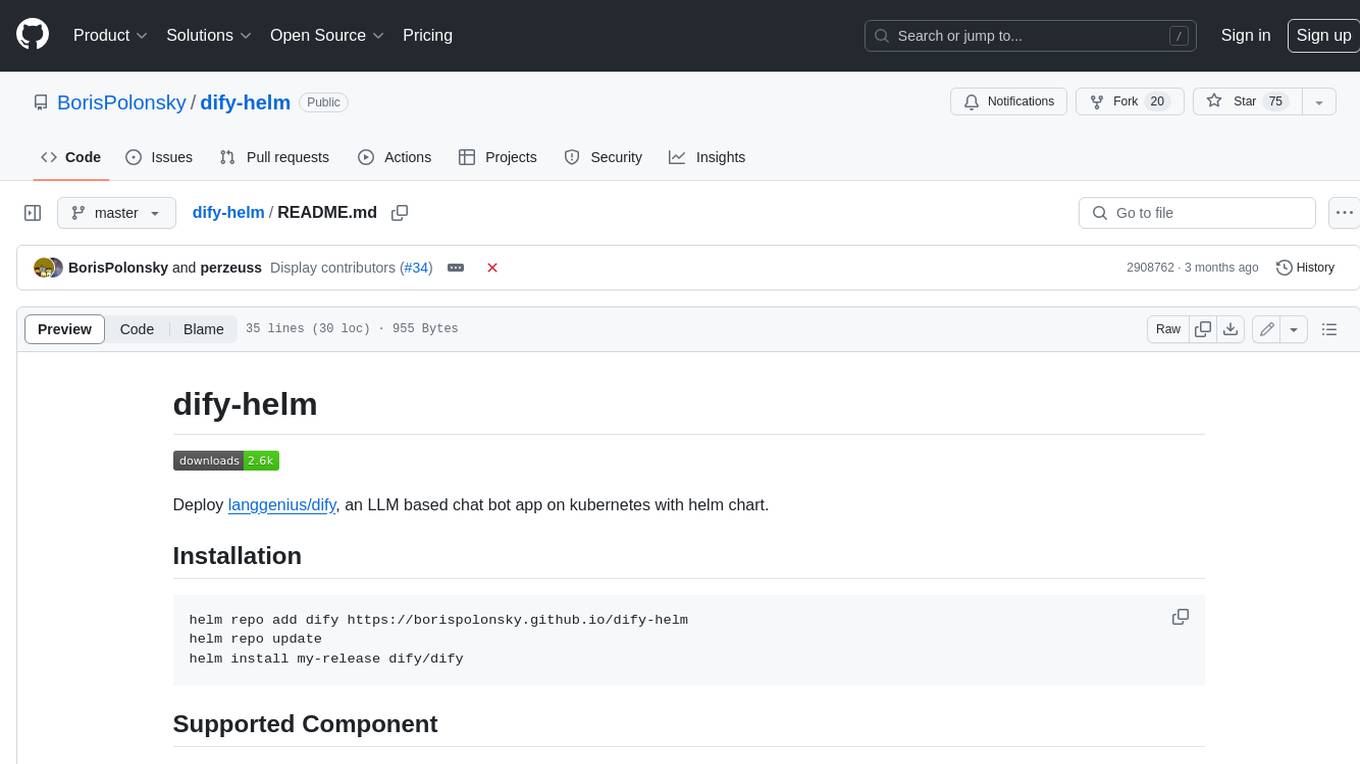
dify-helm
Deploy langgenius/dify, an LLM based chat bot app on kubernetes with helm chart.
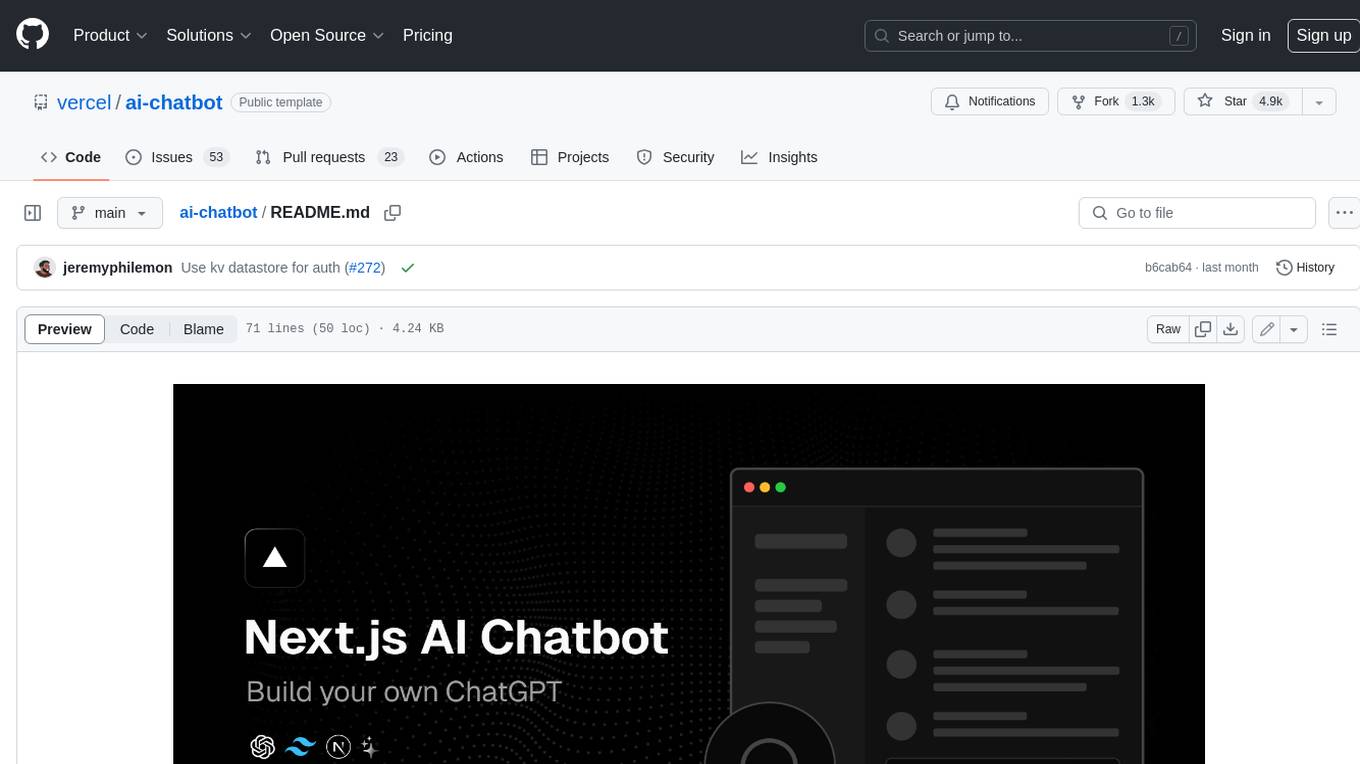
ai-chatbot
Next.js AI Chatbot is an open-source app template for building AI chatbots using Next.js, Vercel AI SDK, OpenAI, and Vercel KV. It includes features like Next.js App Router, React Server Components, Vercel AI SDK for streaming chat UI, support for various AI models, Tailwind CSS styling, Radix UI for headless components, chat history management, rate limiting, session storage with Vercel KV, and authentication with NextAuth.js. The template allows easy deployment to Vercel and customization of AI model providers.
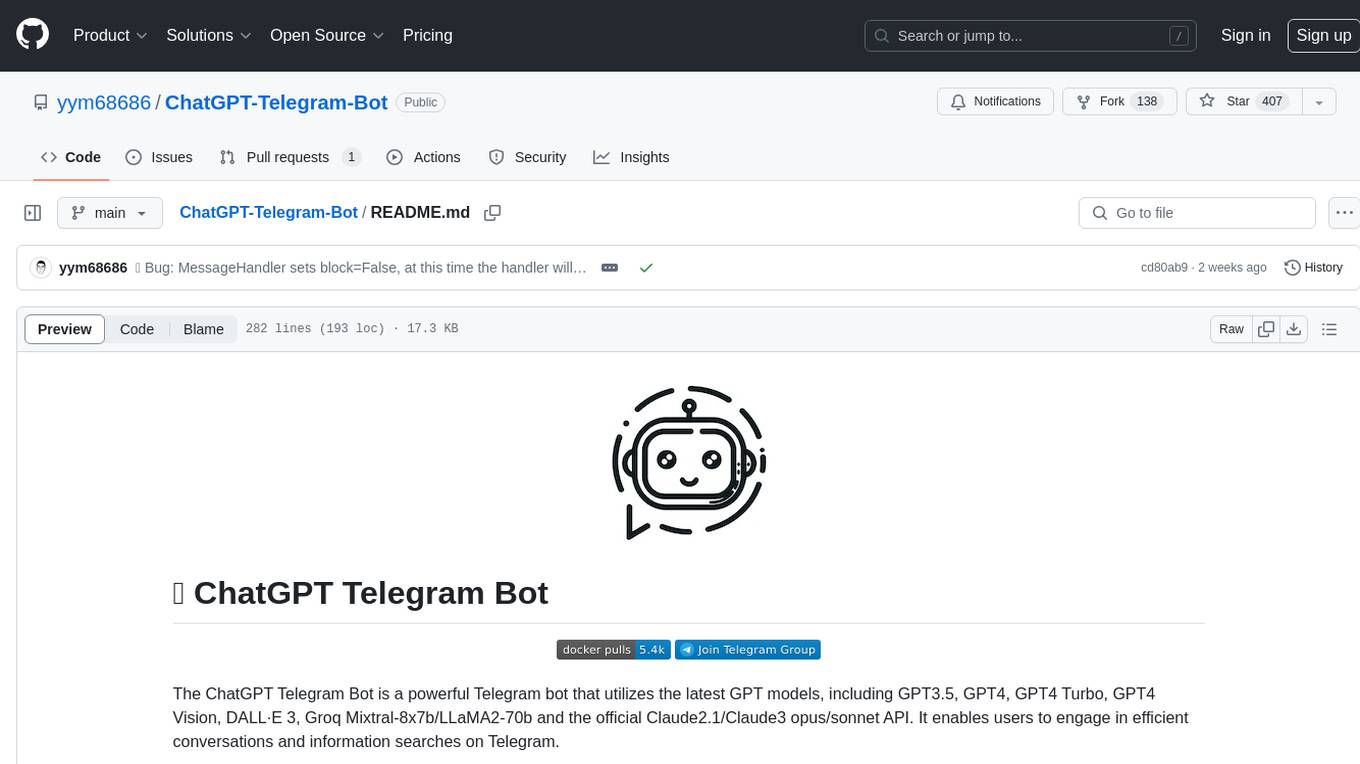
ChatGPT-Telegram-Bot
The ChatGPT Telegram Bot is a powerful Telegram bot that utilizes various GPT models, including GPT3.5, GPT4, GPT4 Turbo, GPT4 Vision, DALL·E 3, Groq Mixtral-8x7b/LLaMA2-70b, and Claude2.1/Claude3 opus/sonnet API. It enables users to engage in efficient conversations and information searches on Telegram. The bot supports multiple AI models, online search with DuckDuckGo and Google, user-friendly interface, efficient message processing, document interaction, Markdown rendering, and convenient deployment options like Zeabur, Replit, and Docker. Users can set environment variables for configuration and deployment. The bot also provides Q&A functionality, supports model switching, and can be deployed in group chats with whitelisting. The project is open source under GPLv3 license.
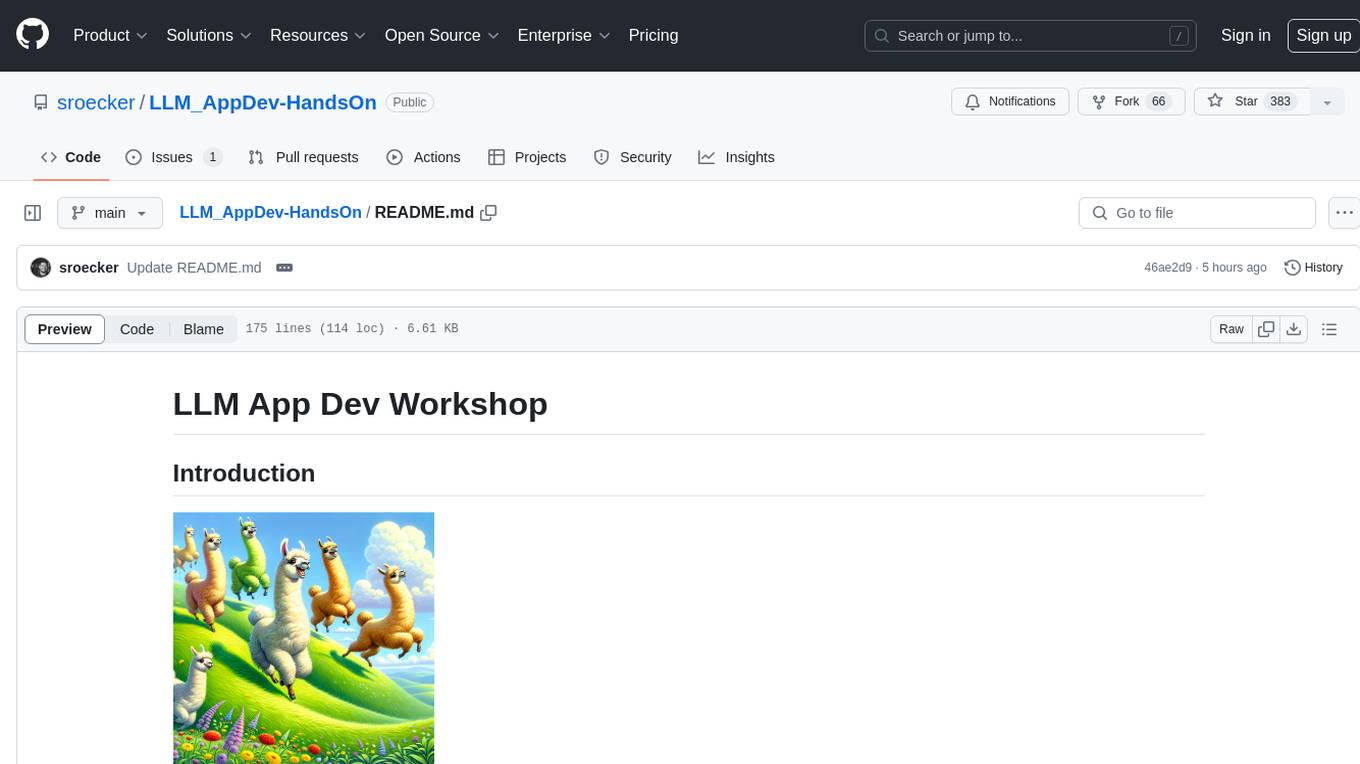
LLM_AppDev-HandsOn
This repository showcases how to build a simple LLM-based chatbot for answering questions based on documents using retrieval augmented generation (RAG) technique. It also provides guidance on deploying the chatbot using Podman or on the OpenShift Container Platform. The workshop associated with this repository introduces participants to LLMs & RAG concepts and demonstrates how to customize the chatbot for specific purposes. The software stack relies on open-source tools like streamlit, LlamaIndex, and local open LLMs via Ollama, making it accessible for GPU-constrained environments.
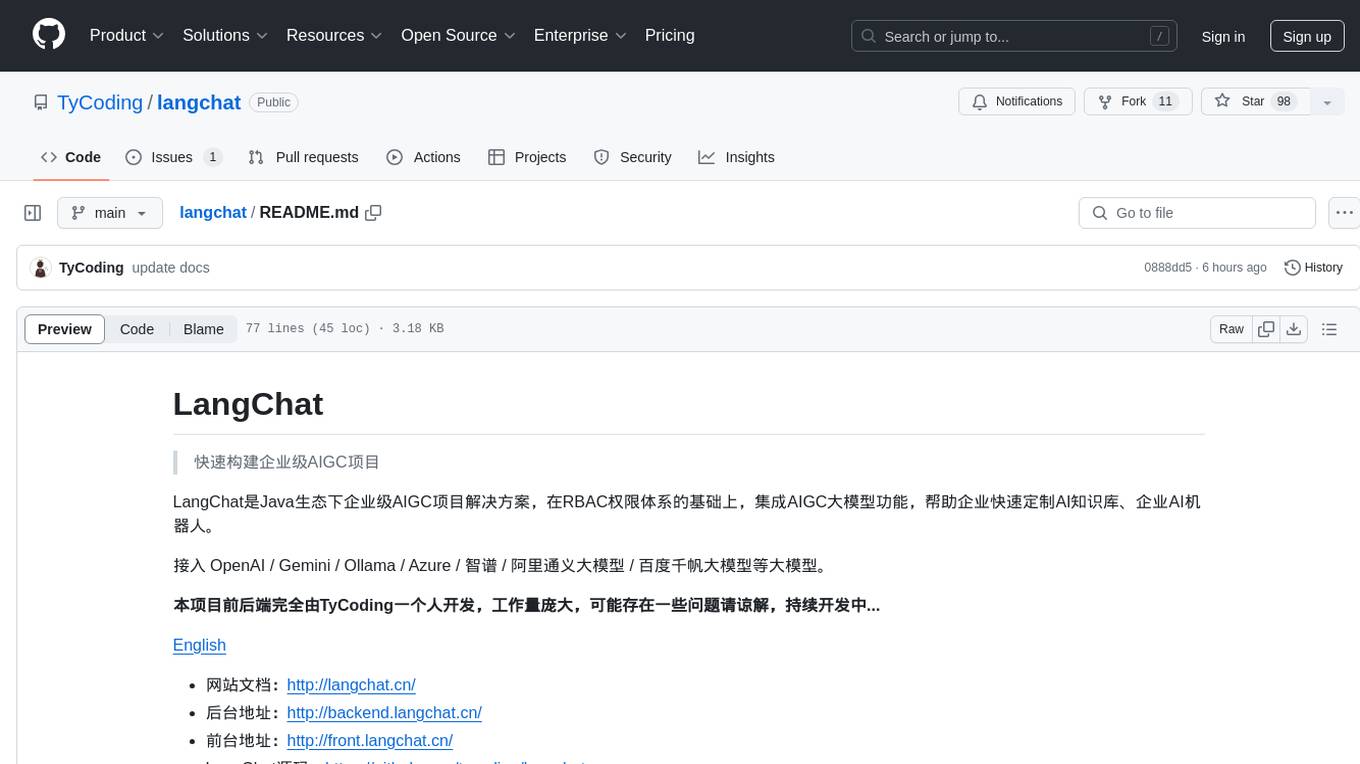
langchat
LangChat is an enterprise AIGC project solution in the Java ecosystem. It integrates AIGC large model functionality on top of the RBAC permission system to help enterprises quickly customize AI knowledge bases and enterprise AI robots. It supports integration with various large models such as OpenAI, Gemini, Ollama, Azure, Zhifu, Alibaba Tongyi, Baidu Qianfan, etc. The project is developed solely by TyCoding and is continuously evolving. It features multi-modality, dynamic configuration, knowledge base support, advanced RAG capabilities, function call customization, multi-channel deployment, workflows visualization, AIGC client application, and more.
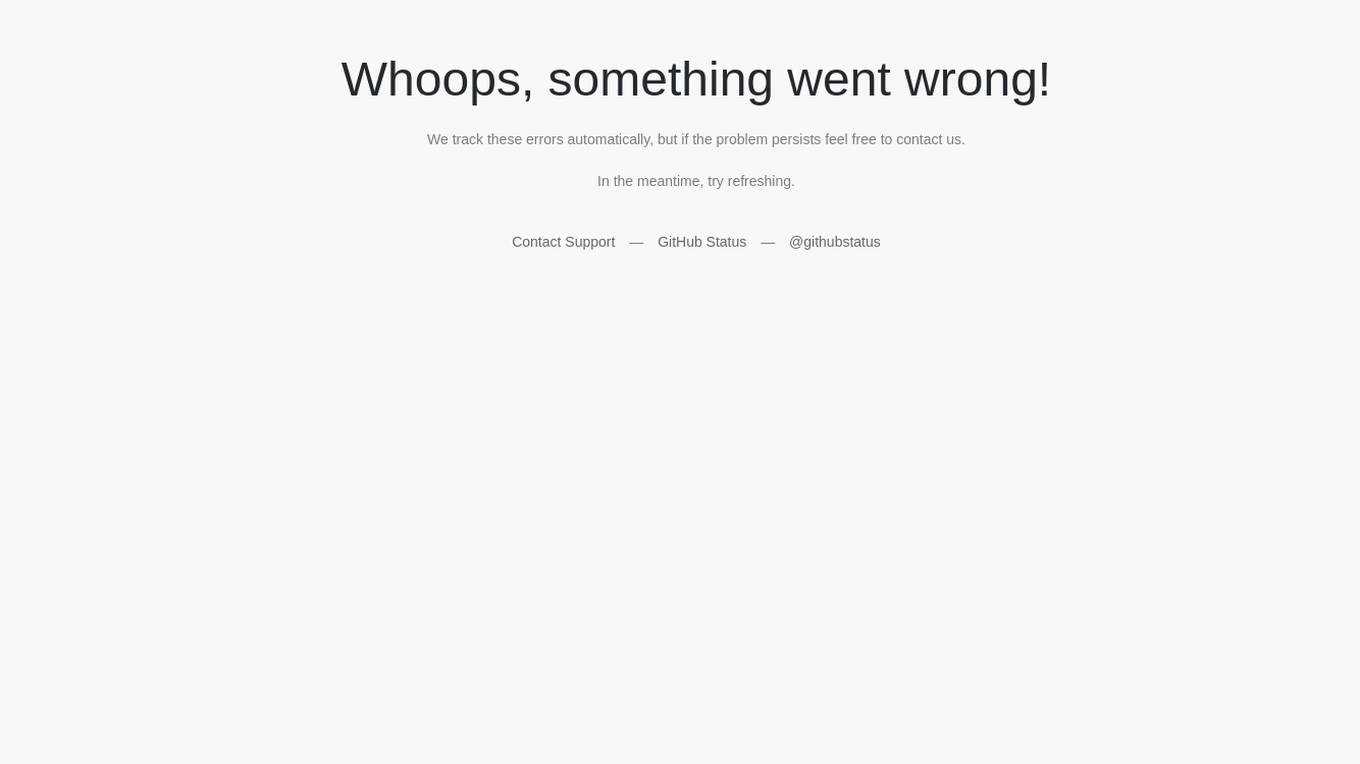
ai-sdk-chrome-ai
The ai-sdk-chrome-ai repository is an open-source chatbot built with Next.js, the Vercel AI SDK, and the Chrome AI provider. It features Next.js App Router, Vercel AI SDK for interacting with the Gemini Nano model, shadcn/ui, Tailwind CSS styling, and Radix UI for headless component primitives. Users can deploy their own version of the chatbot to Vercel with one click and run it locally by installing dependencies and running the dev server. The repository provides a template for creating and customizing a chatbot powered by AI technology.
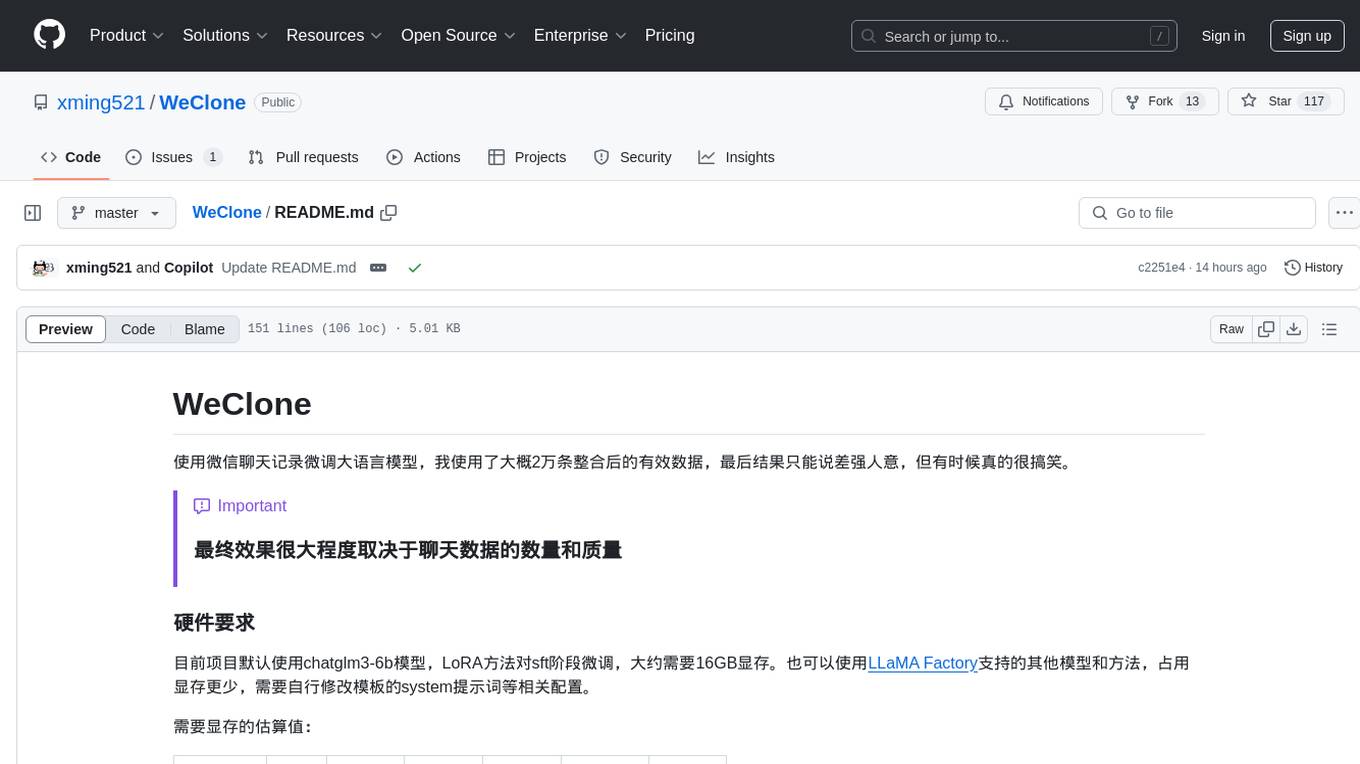
WeClone
WeClone is a tool that fine-tunes large language models using WeChat chat records. It utilizes approximately 20,000 integrated and effective data points, resulting in somewhat satisfactory outcomes that are occasionally humorous. The tool's effectiveness largely depends on the quantity and quality of the chat data provided. It requires a minimum of 16GB of GPU memory for training using the default chatglm3-6b model with LoRA method. Users can also opt for other models and methods supported by LLAMA Factory, which consume less memory. The tool has specific hardware and software requirements, including Python, Torch, Transformers, Datasets, Accelerate, and other optional packages like CUDA and Deepspeed. The tool facilitates environment setup, data preparation, data preprocessing, model downloading, parameter configuration, model fine-tuning, and inference through a browser demo or API service. Additionally, it offers the ability to deploy a WeChat chatbot, although users should be cautious due to the risk of account suspension by WeChat.
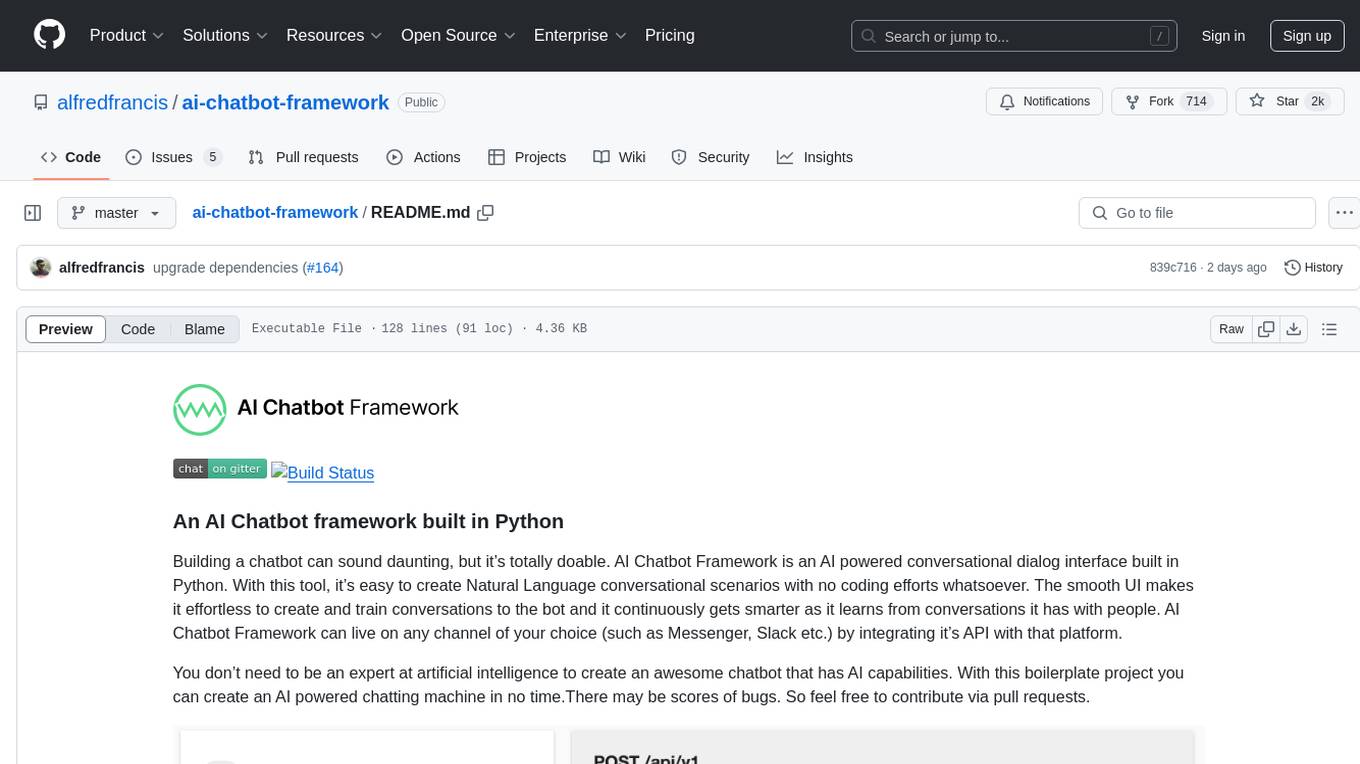
ai-chatbot-framework
An AI Chatbot framework built in Python. It allows users to easily create Natural Language conversational scenarios with no coding efforts. The tool continuously learns from conversations to improve its capabilities. It can be integrated with various channels like Messenger and Slack. Users can create AI-powered chatbots without expertise in artificial intelligence.
For similar jobs
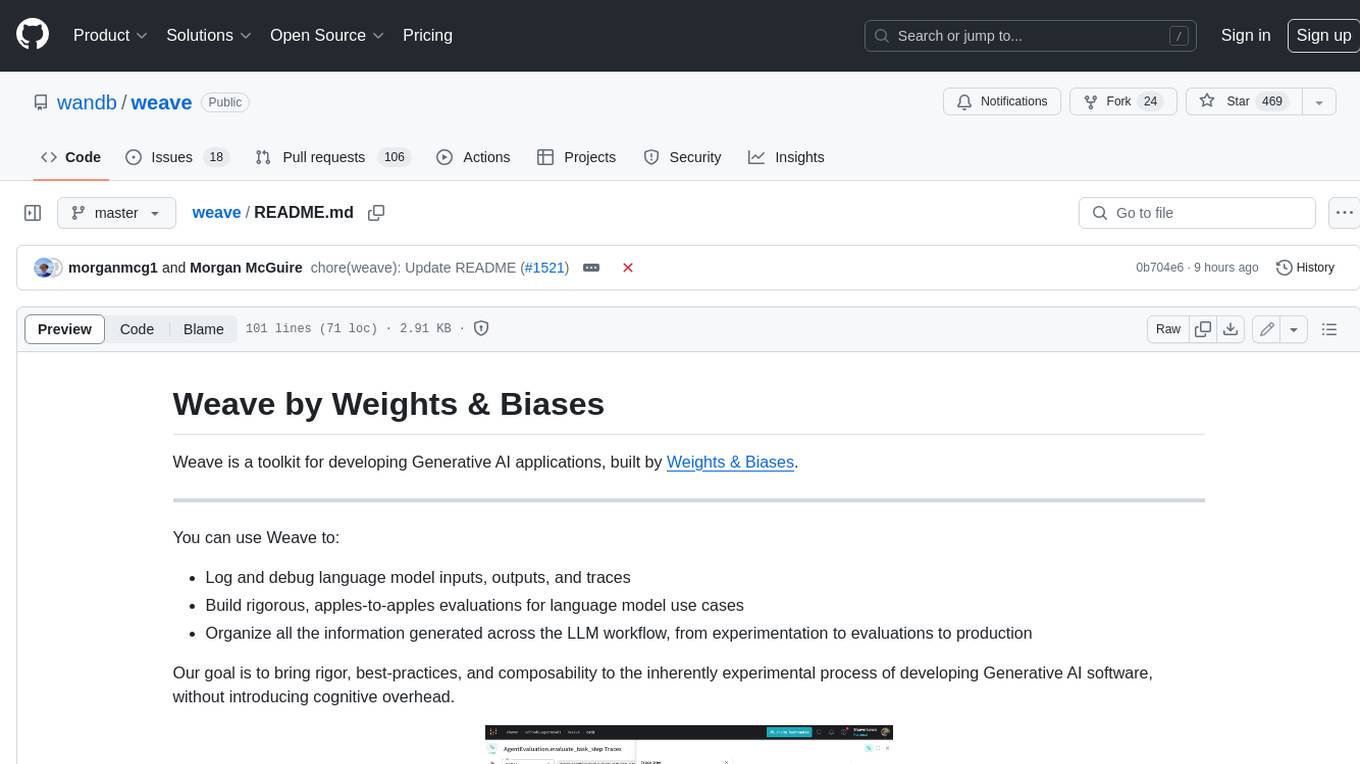
weave
Weave is a toolkit for developing Generative AI applications, built by Weights & Biases. With Weave, you can log and debug language model inputs, outputs, and traces; build rigorous, apples-to-apples evaluations for language model use cases; and organize all the information generated across the LLM workflow, from experimentation to evaluations to production. Weave aims to bring rigor, best-practices, and composability to the inherently experimental process of developing Generative AI software, without introducing cognitive overhead.
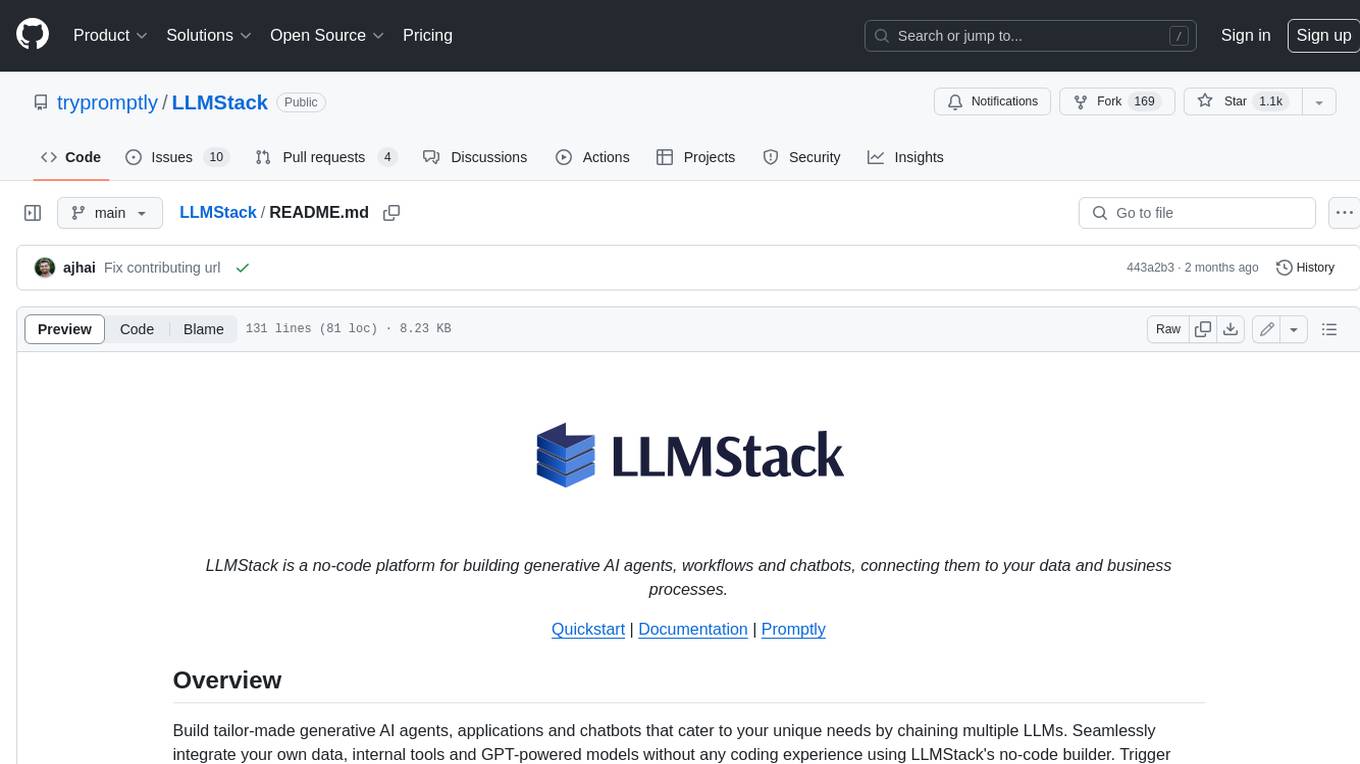
LLMStack
LLMStack is a no-code platform for building generative AI agents, workflows, and chatbots. It allows users to connect their own data, internal tools, and GPT-powered models without any coding experience. LLMStack can be deployed to the cloud or on-premise and can be accessed via HTTP API or triggered from Slack or Discord.
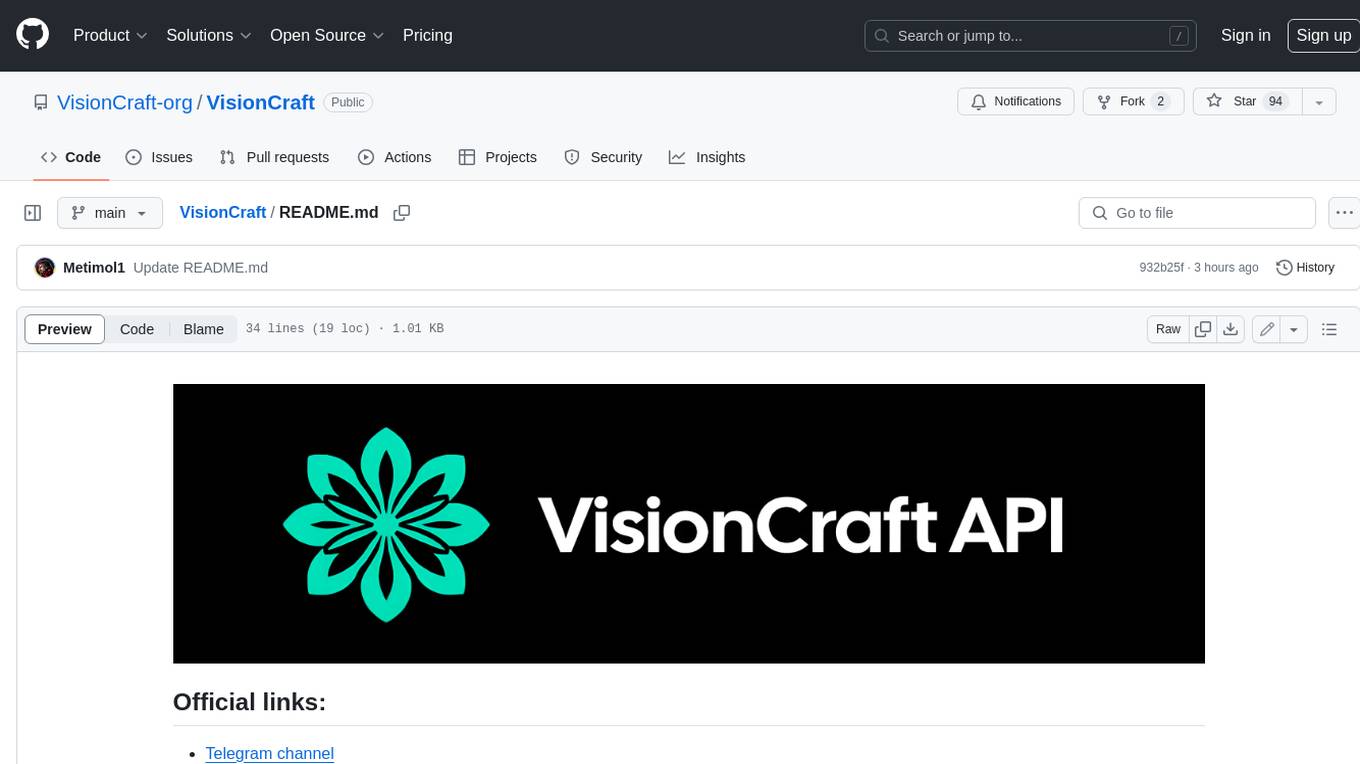
VisionCraft
The VisionCraft API is a free API for using over 100 different AI models. From images to sound.
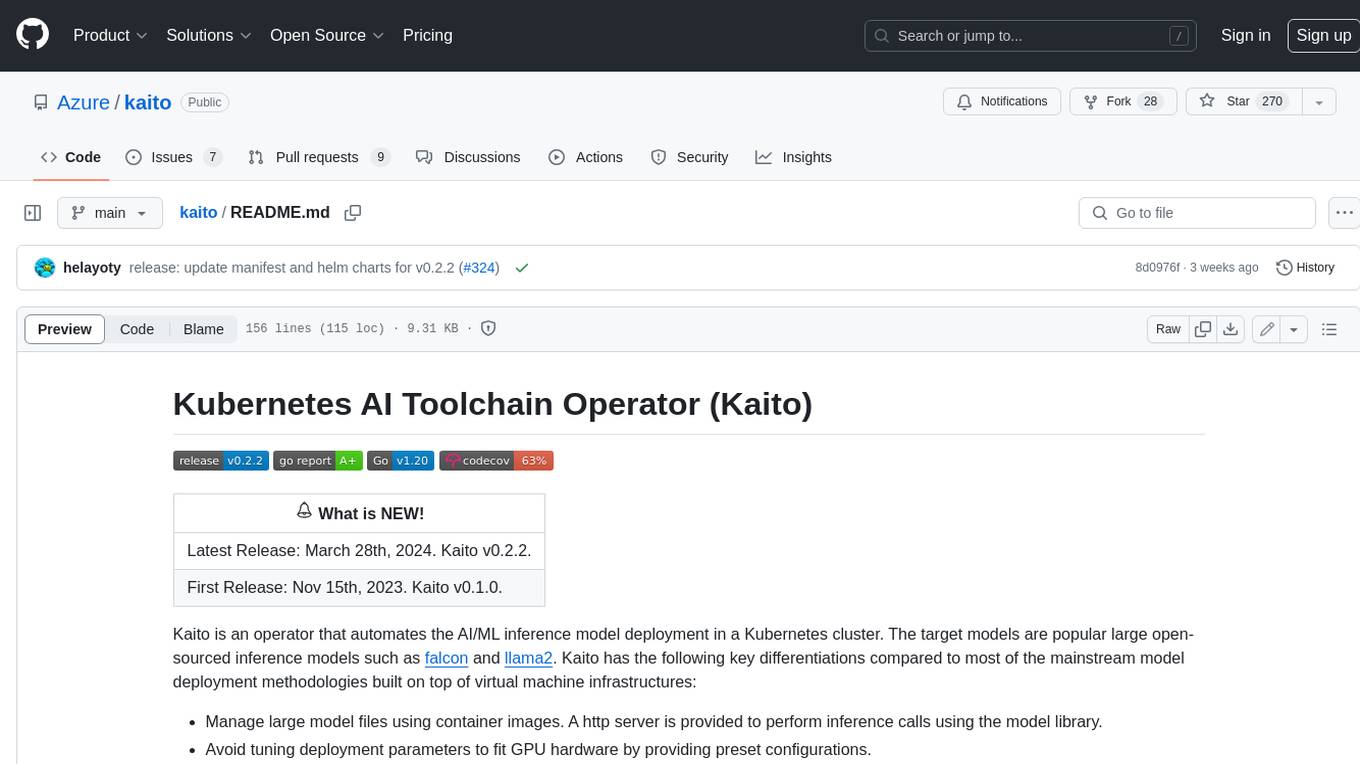
kaito
Kaito is an operator that automates the AI/ML inference model deployment in a Kubernetes cluster. It manages large model files using container images, avoids tuning deployment parameters to fit GPU hardware by providing preset configurations, auto-provisions GPU nodes based on model requirements, and hosts large model images in the public Microsoft Container Registry (MCR) if the license allows. Using Kaito, the workflow of onboarding large AI inference models in Kubernetes is largely simplified.
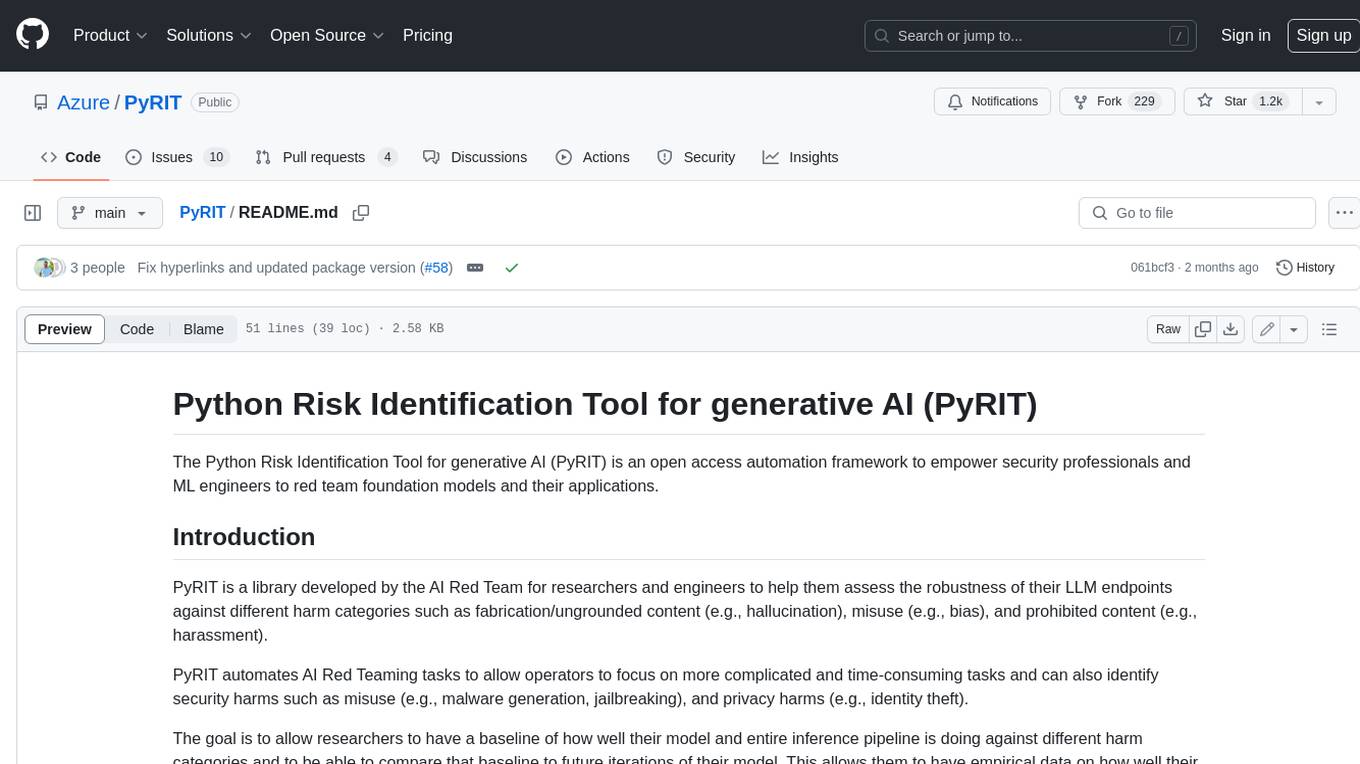
PyRIT
PyRIT is an open access automation framework designed to empower security professionals and ML engineers to red team foundation models and their applications. It automates AI Red Teaming tasks to allow operators to focus on more complicated and time-consuming tasks and can also identify security harms such as misuse (e.g., malware generation, jailbreaking), and privacy harms (e.g., identity theft). The goal is to allow researchers to have a baseline of how well their model and entire inference pipeline is doing against different harm categories and to be able to compare that baseline to future iterations of their model. This allows them to have empirical data on how well their model is doing today, and detect any degradation of performance based on future improvements.
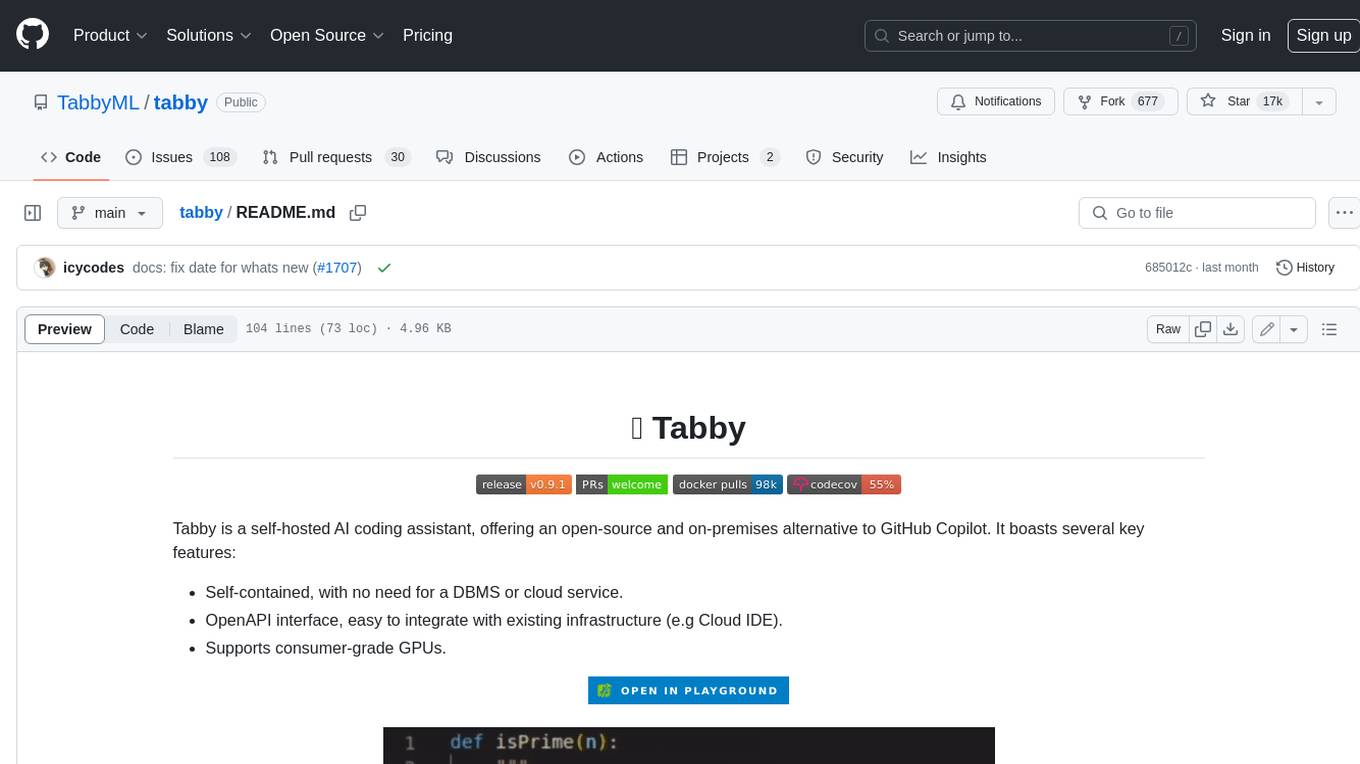
tabby
Tabby is a self-hosted AI coding assistant, offering an open-source and on-premises alternative to GitHub Copilot. It boasts several key features: * Self-contained, with no need for a DBMS or cloud service. * OpenAPI interface, easy to integrate with existing infrastructure (e.g Cloud IDE). * Supports consumer-grade GPUs.
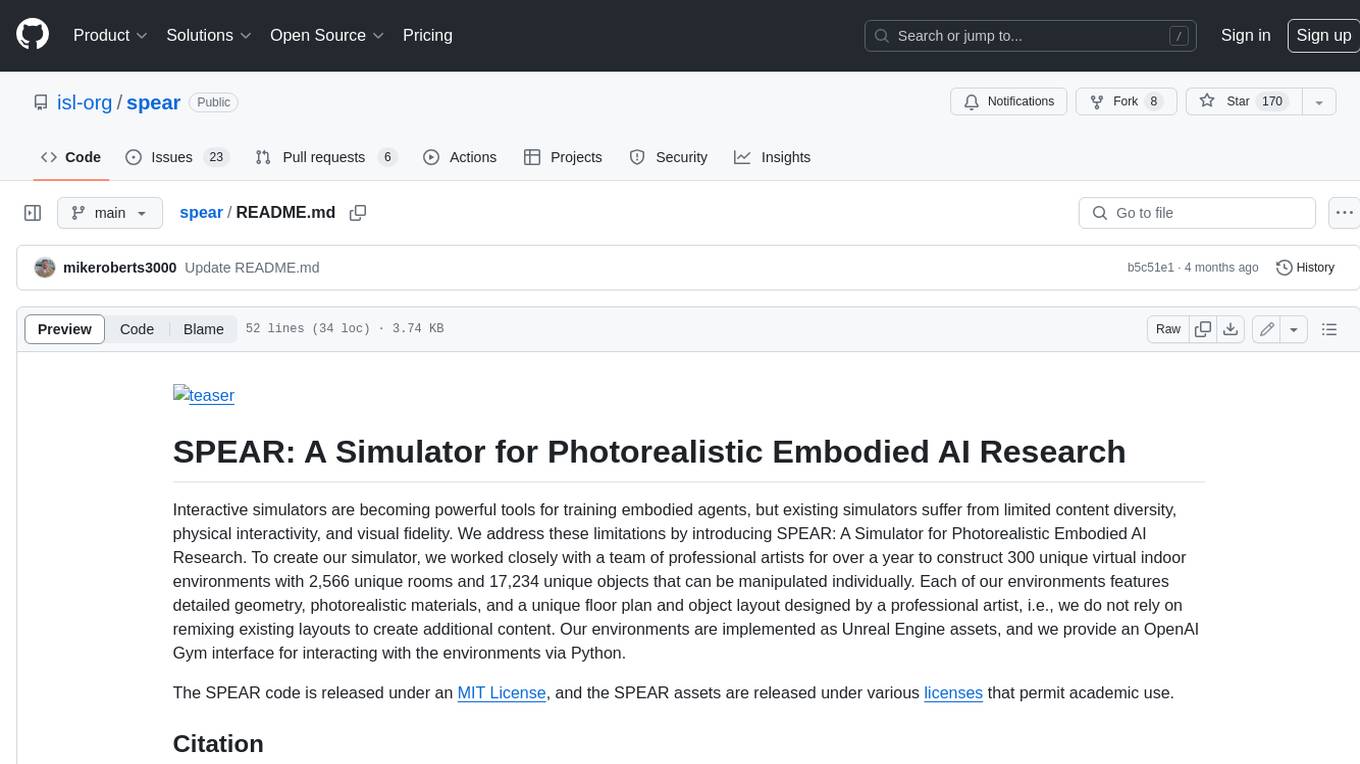
spear
SPEAR (Simulator for Photorealistic Embodied AI Research) is a powerful tool for training embodied agents. It features 300 unique virtual indoor environments with 2,566 unique rooms and 17,234 unique objects that can be manipulated individually. Each environment is designed by a professional artist and features detailed geometry, photorealistic materials, and a unique floor plan and object layout. SPEAR is implemented as Unreal Engine assets and provides an OpenAI Gym interface for interacting with the environments via Python.
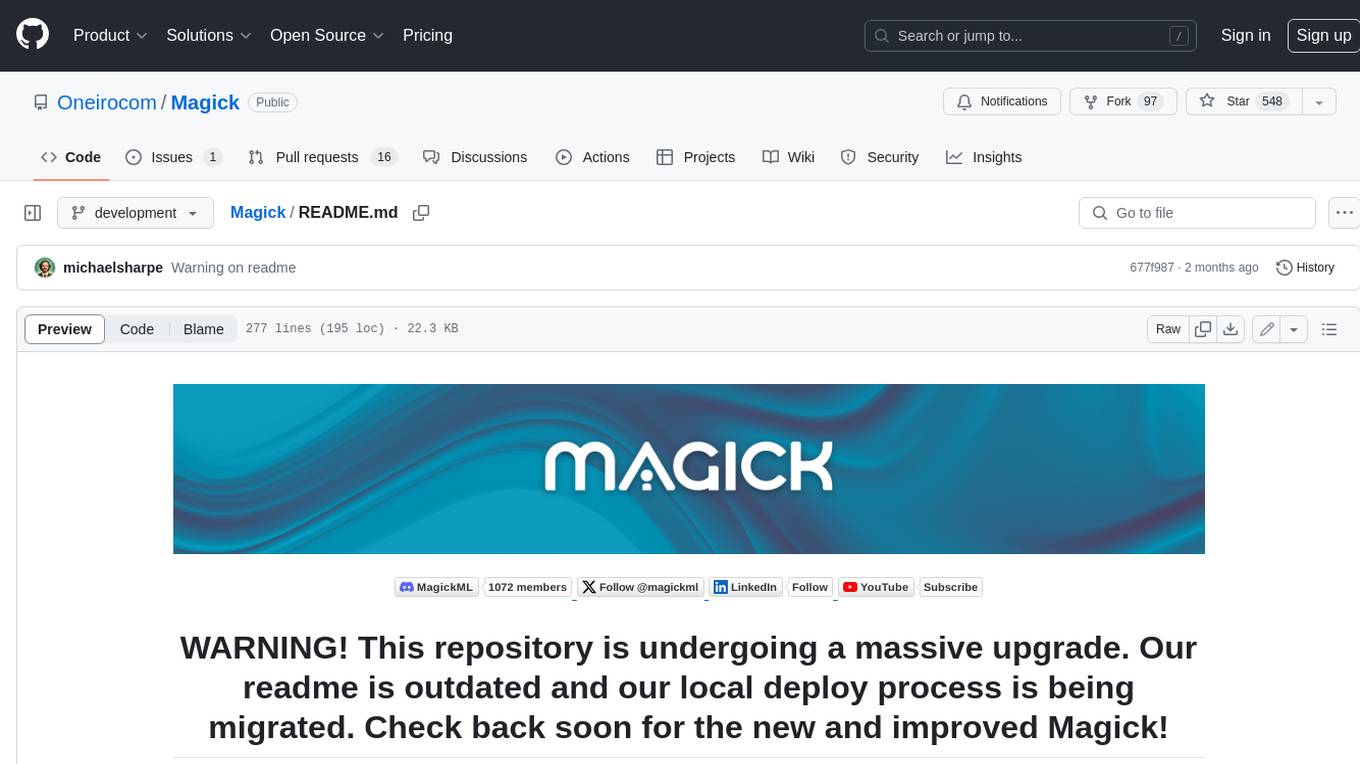
Magick
Magick is a groundbreaking visual AIDE (Artificial Intelligence Development Environment) for no-code data pipelines and multimodal agents. Magick can connect to other services and comes with nodes and templates well-suited for intelligent agents, chatbots, complex reasoning systems and realistic characters.