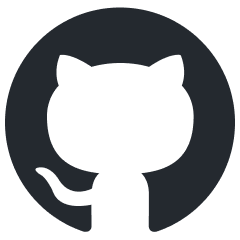
refact
AI Agent that handles engineering tasks end-to-end: integrates with developersβ tools, plans, executes, and iterates until it achieves a successful result.
Stars: 1846
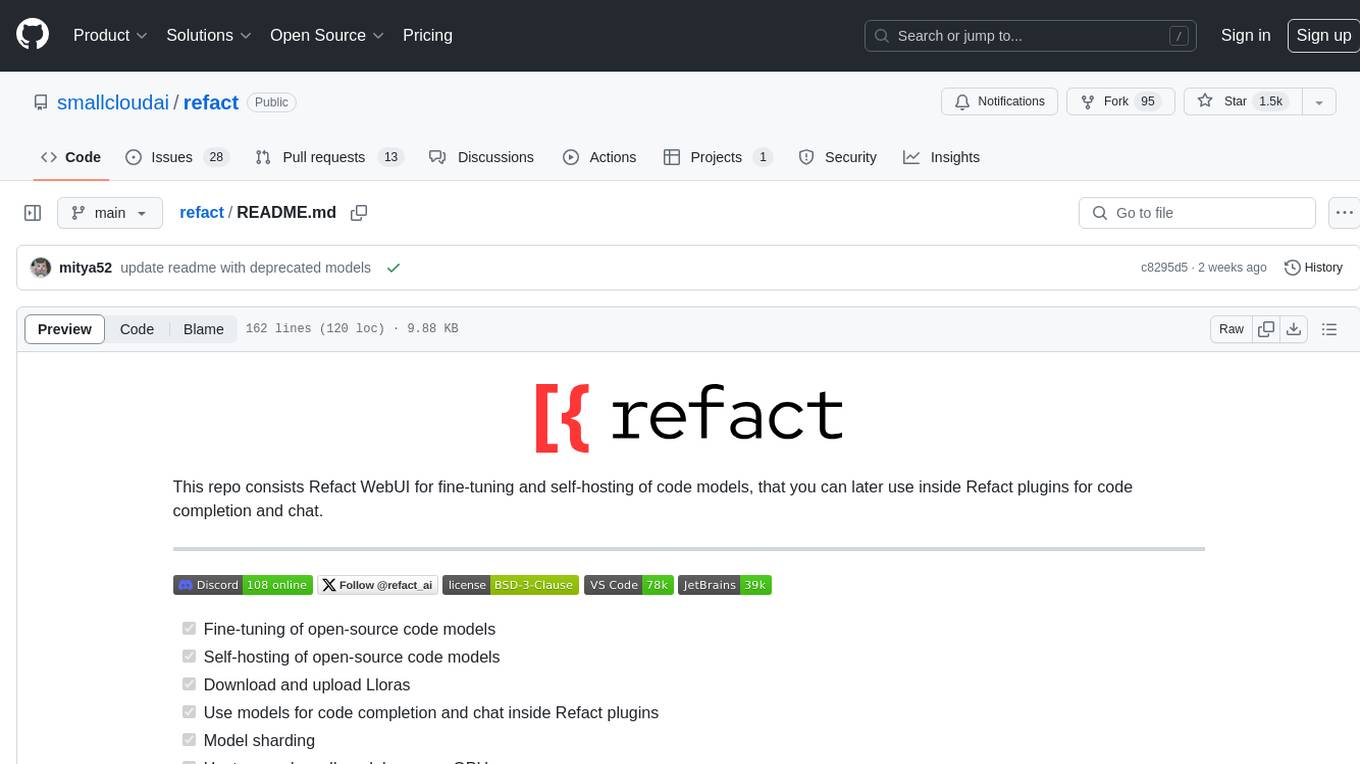
This repository contains Refact WebUI for fine-tuning and self-hosting of code models, which can be used inside Refact plugins for code completion and chat. Users can fine-tune open-source code models, self-host them, download and upload Lloras, use models for code completion and chat inside Refact plugins, shard models, host multiple small models on one GPU, and connect GPT-models for chat using OpenAI and Anthropic keys. The repository provides a Docker container for running the self-hosted server and supports various models for completion, chat, and fine-tuning. Refact is free for individuals and small teams under the BSD-3-Clause license, with custom installation options available for GPU support. The community and support include contributing guidelines, GitHub issues for bugs, a community forum, Discord for chatting, and Twitter for product news and updates.
README:
Refact Agent is a free, open-source AI Agent that handles engineering tasks end-to-end. It deeply understands your codebases and integrates with your tools, databases, and browsers to automate complex, multi-step tasks.
Refact Agent works effortlessly with the tools and databases you already use:
- π Version Control: GitHub, GitLab
- ποΈ Databases: PostgreSQL, MySQL
- π οΈ Debugging: Pdb
- π³ Containerization: Docker
- β Deploy On-Premise: Maintain 100% control over your codebase.
- π§ Access State-of-the-Art Models: Supports Claude 3.7 Sonnet, GPT-4o, o3-mini, and more.
- π Bring Your Own Key (BYOK): Use your own API keys for external LLMs.
- π¬ Integrated IDE Chat: Stay in your workflow, no need to switch between tools!
- β‘ Free, Unlimited, Context-Aware Auto-Completion: Code faster with smart AI suggestions.
- π οΈ Supports 25+ Programming Languages: Python, JavaScript, Java, Rust, TypeScript, PHP, C++, C#, Go, and many more!
π View Full List of Supported Models
π’ Using AI for work? Letβs bring it to your company!
Fill out this form β Our AI Agent will be tailored to your companyβs data, learning from feedback, and helping organize knowledge for better collaboration with your team.
- π Core Features and Functionality
- π€ Which Tasks Can Refact Help You With?
- βοΈ QuickStart
- π³ Running Refact Self-Hosted in a Docker Container
- π Getting Started with Plugins
- π Documentation
- π₯ Contribution
- π Join the Community
β Unlimited accurate auto-completion with context awareness β Powered by Qwen2.5-Coder-1.5B, utilizing Retrieval-Augmented Generation (RAG).
β Integrated in-IDE Chat β AI deeply understands your code and provides relevant, intelligent answers.
β Integrated with Tools β Works with GitHub, GitLab, PostgreSQL, MySQL, Pdb, Docker, and shell commands.
β State-of-the-Art Models β Supports Claude 3.7 Sonnet, GPT-4o, o3-mini, and more.
β Bring Your Own Key (BYOK) β Use your own API keys for external LLMs.
-
π Generate code from natural language prompts (even with typos).
-
π Refactor code for better quality and readability.
-
π Explain code to quickly understand unfamiliar code.
-
π Debug code to detect and fix errors faster.
-
π§ͺ Generate unit tests for reliable code.
-
π Code Review with AI-assisted suggestions.
-
π Create Documentation to keep knowledge up to date.
-
π· Generate Docstrings for structured documentation.
You can install the Refact repository without Docker:
pip install .
For GPU with CUDA capability >= 8.0 and flash-attention v2 support:
FLASH_ATTENTION_FORCE_BUILD=TRUE MAX_JOBS=4 INSTALL_OPTIONAL=TRUE pip install .
The easiest way to run the self-hosted server is using a pre-built Docker image.
See CONTRIBUTING.md
for installation without a Docker container.
- Download Refact for VS Code or JetBrains.
-
Set up a custom inference URL:
http://127.0.0.1:8008
-
Configure the plugin settings:
- JetBrains: Settings > Tools > Refact.ai > Advanced > Inference URL
- VSCode: Extensions > Refact.ai Assistant > Settings > Address URL
For detailed guidance and best practices, check out our documentation.
Want to contribute to our project? We're always open to new ideas and features!
- Check out GitHub Issues β See what we're working on or suggest your own ideas.
-
Read our Contributing Guide β Check out
Contributing.md
to get started.
Your contributions help shape the future of Refact Agent! π
We're all about open-source and empowering developers with AI tools. Our vision is to build the future of programming. Join us and be part of the journey!
π’ Join our Discord server β A community-run space for discussion, questions, and feedback.
Made with β€οΈ by developers who automate the boring, so you can focus on building the future.
For Tasks:
Click tags to check more tools for each tasksFor Jobs:
Alternative AI tools for refact
Similar Open Source Tools
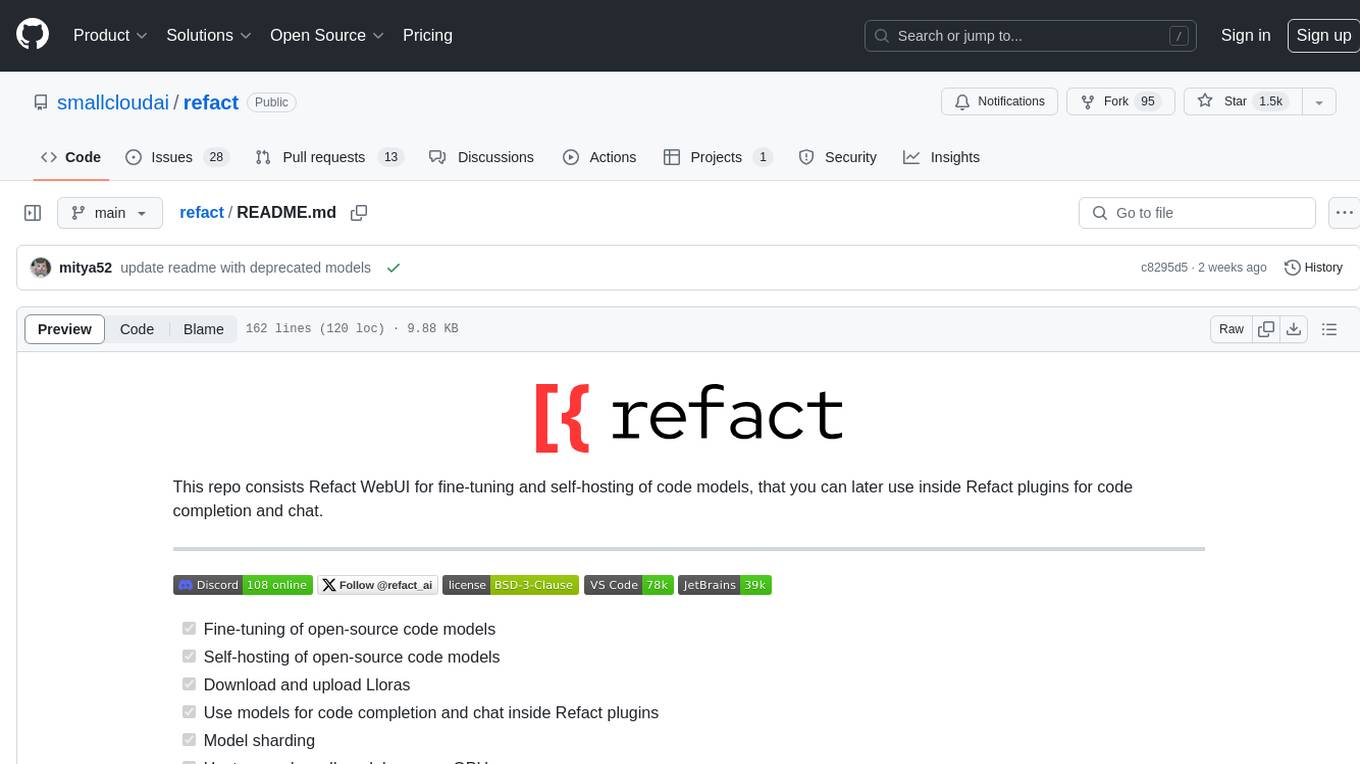
refact
This repository contains Refact WebUI for fine-tuning and self-hosting of code models, which can be used inside Refact plugins for code completion and chat. Users can fine-tune open-source code models, self-host them, download and upload Lloras, use models for code completion and chat inside Refact plugins, shard models, host multiple small models on one GPU, and connect GPT-models for chat using OpenAI and Anthropic keys. The repository provides a Docker container for running the self-hosted server and supports various models for completion, chat, and fine-tuning. Refact is free for individuals and small teams under the BSD-3-Clause license, with custom installation options available for GPU support. The community and support include contributing guidelines, GitHub issues for bugs, a community forum, Discord for chatting, and Twitter for product news and updates.
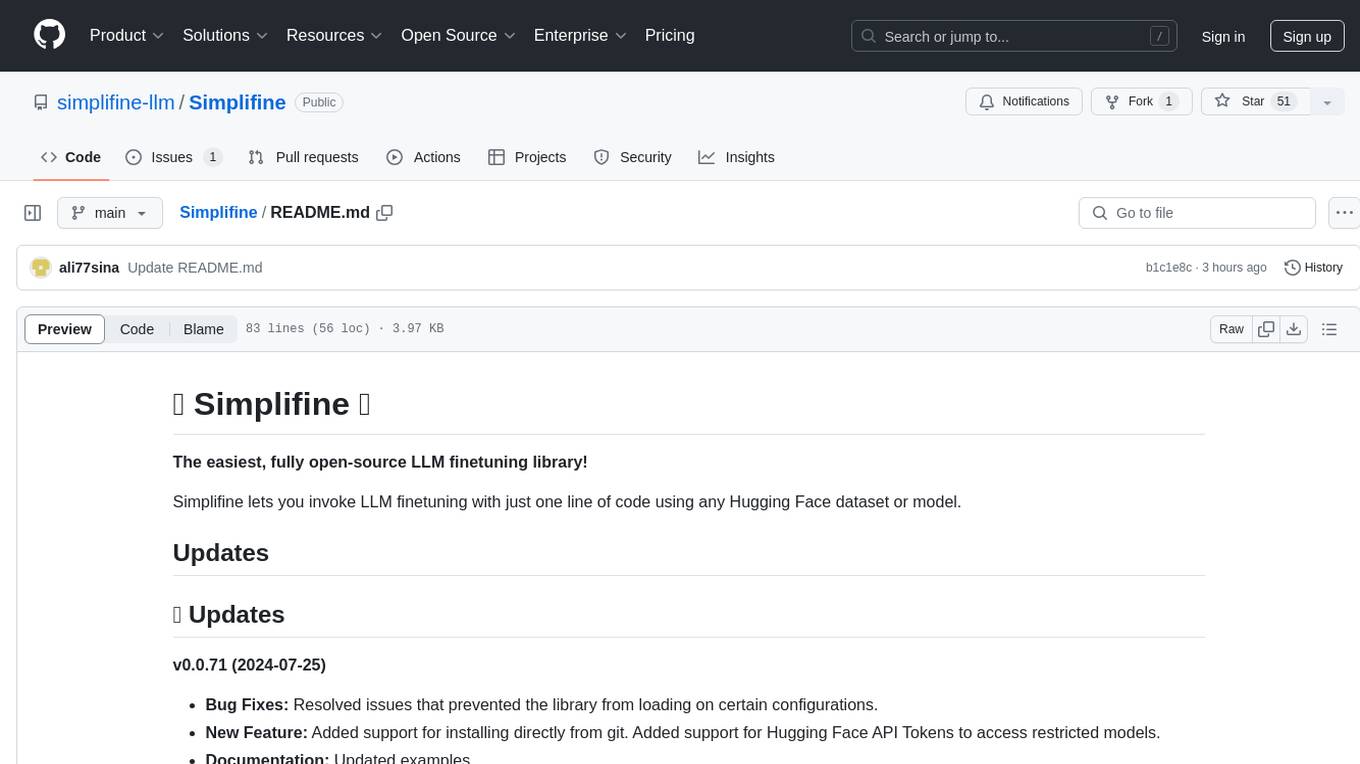
Simplifine
Simplifine is an open-source library designed for easy LLM finetuning, enabling users to perform tasks such as supervised fine tuning, question-answer finetuning, contrastive loss for embedding tasks, multi-label classification finetuning, and more. It provides features like WandB logging, in-built evaluation tools, automated finetuning parameters, and state-of-the-art optimization techniques. The library offers bug fixes, new features, and documentation updates in its latest version. Users can install Simplifine via pip or directly from GitHub. The project welcomes contributors and provides comprehensive documentation and support for users.
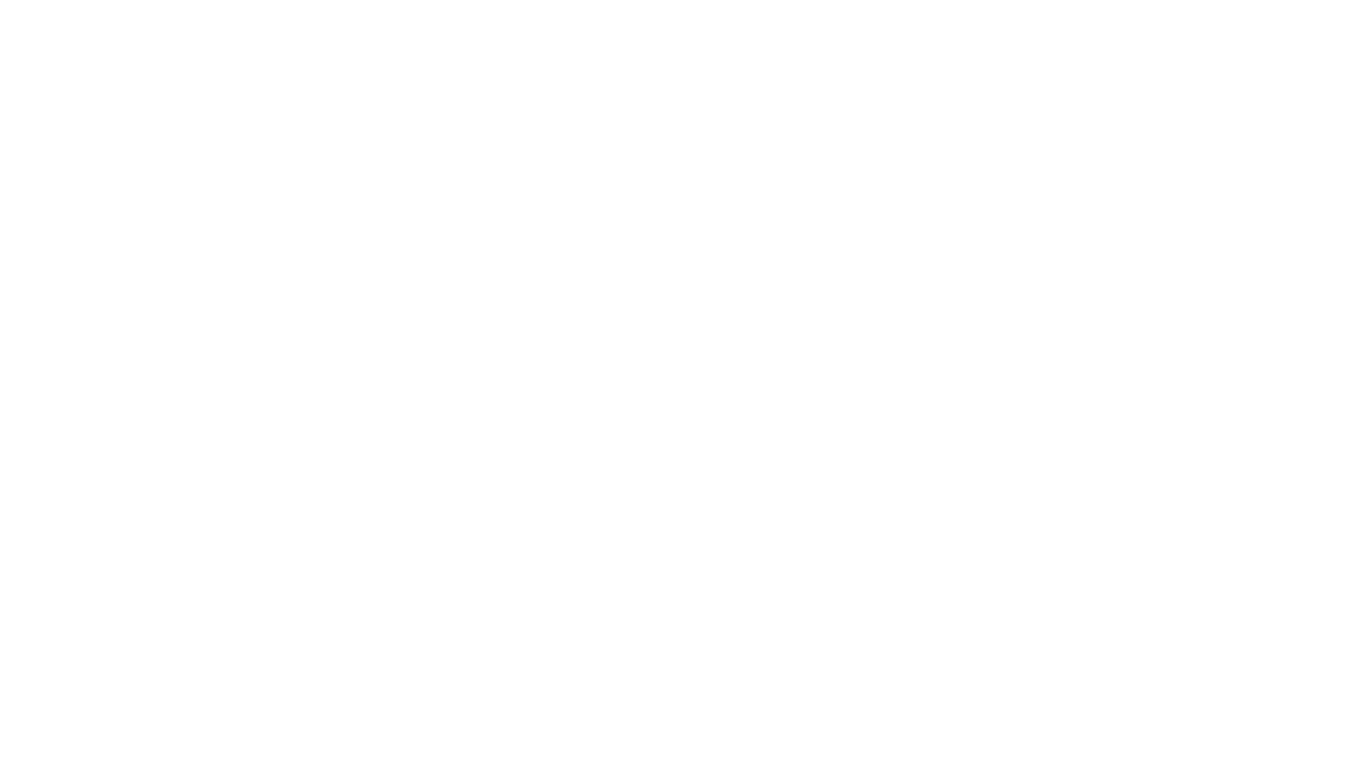
CushyStudio
CushyStudio is a generative AI platform designed for creatives of any level to effortlessly create stunning images, videos, and 3D models. It offers CushyApps, a collection of visual tools tailored for different artistic tasks, and CushyKit, an extensive toolkit for custom apps development and task automation. Users can dive into the AI revolution, unleash their creativity, share projects, and connect with a vibrant community. The platform aims to simplify the AI art creation process and provide a user-friendly environment for designing interfaces, adding custom logic, and accessing various tools.
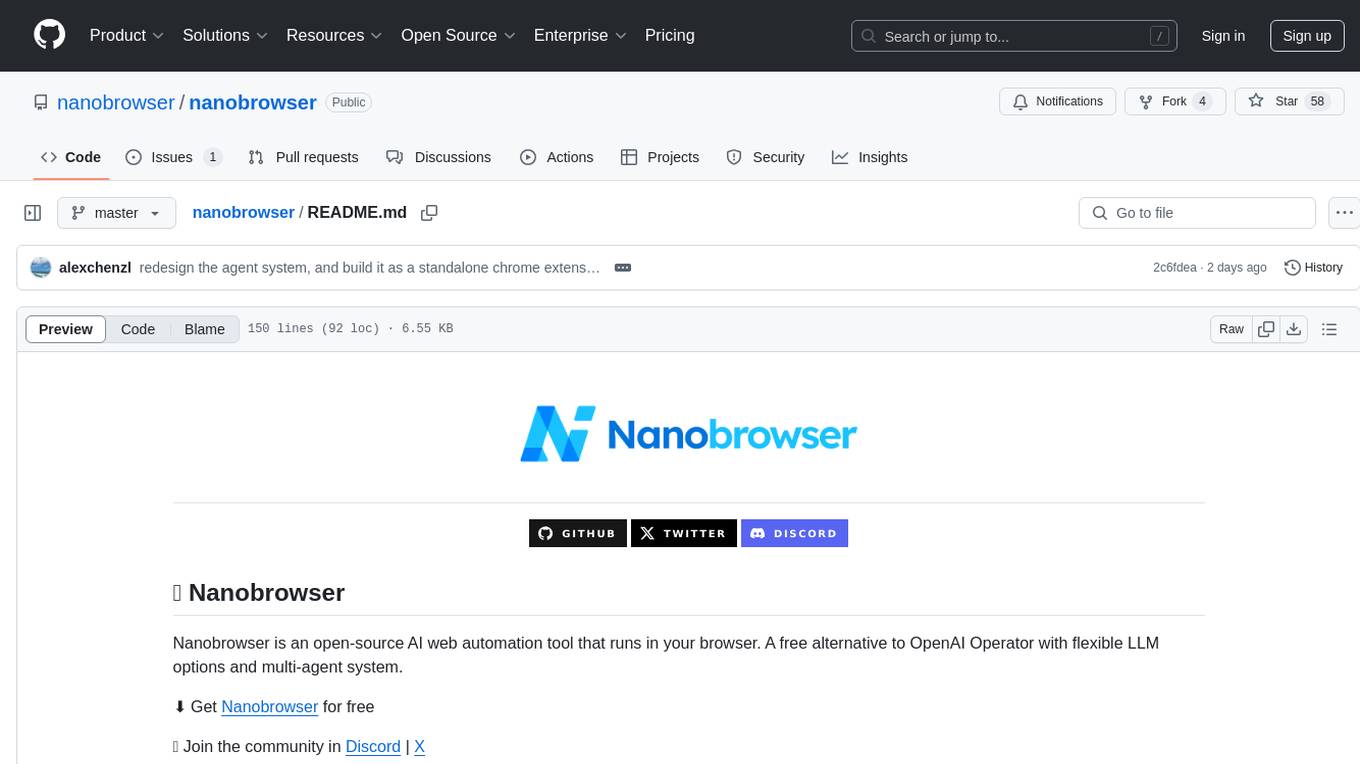
nanobrowser
Nanobrowser is an open-source AI web automation tool that runs in your browser. It is a free alternative to OpenAI Operator with flexible LLM options and a multi-agent system. Nanobrowser offers premium web automation capabilities while keeping users in complete control, with features like a multi-agent system, interactive side panel, task automation, follow-up questions, and multiple LLM support. Users can easily download and install Nanobrowser as a Chrome extension, configure agent models, and accomplish tasks such as news summary, GitHub research, and shopping research with just a sentence. The tool uses a specialized multi-agent system powered by large language models to understand and execute complex web tasks. Nanobrowser is actively developed with plans to expand LLM support, implement security measures, optimize memory usage, enable session replay, and develop specialized agents for domain-specific tasks. Contributions from the community are welcome to improve Nanobrowser and build the future of web automation.
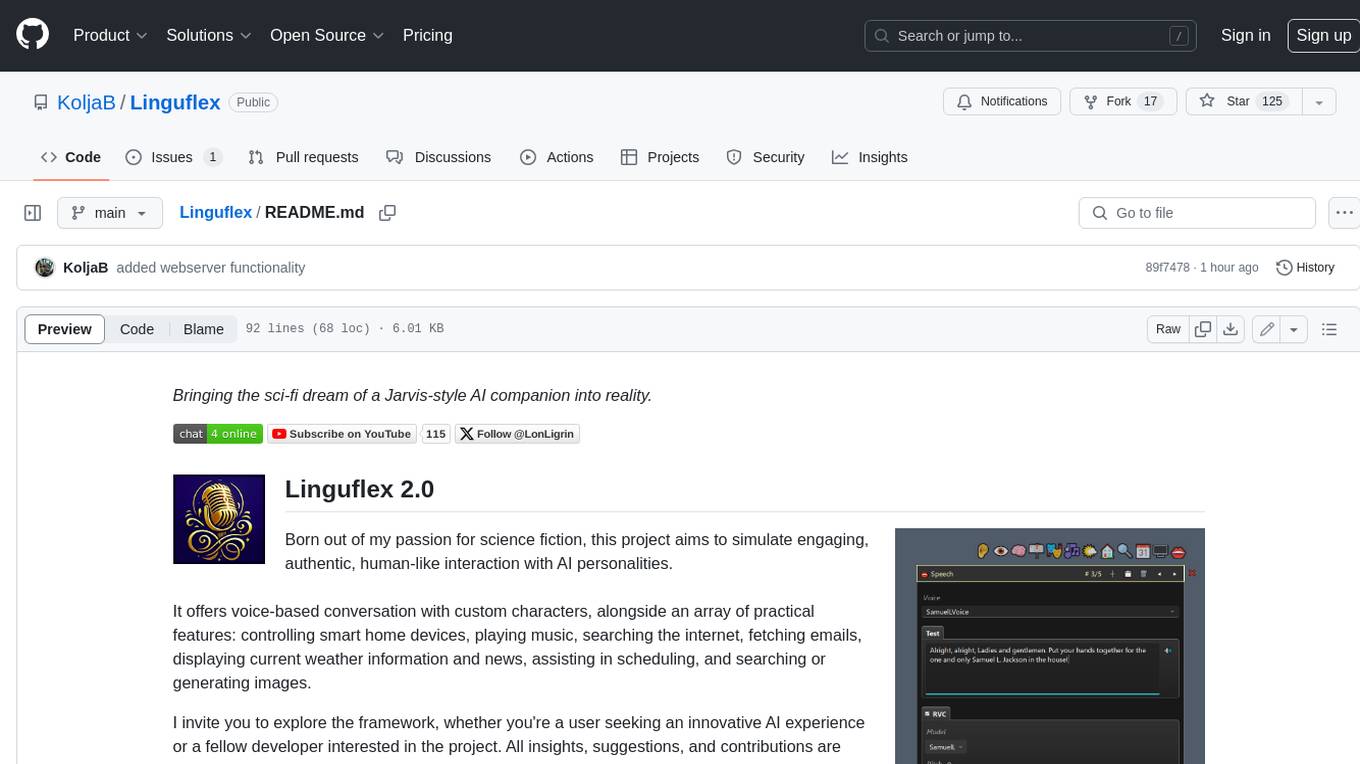
Linguflex
Linguflex is a project that aims to simulate engaging, authentic, human-like interaction with AI personalities. It offers voice-based conversation with custom characters, alongside an array of practical features such as controlling smart home devices, playing music, searching the internet, fetching emails, displaying current weather information and news, assisting in scheduling, and searching or generating images.
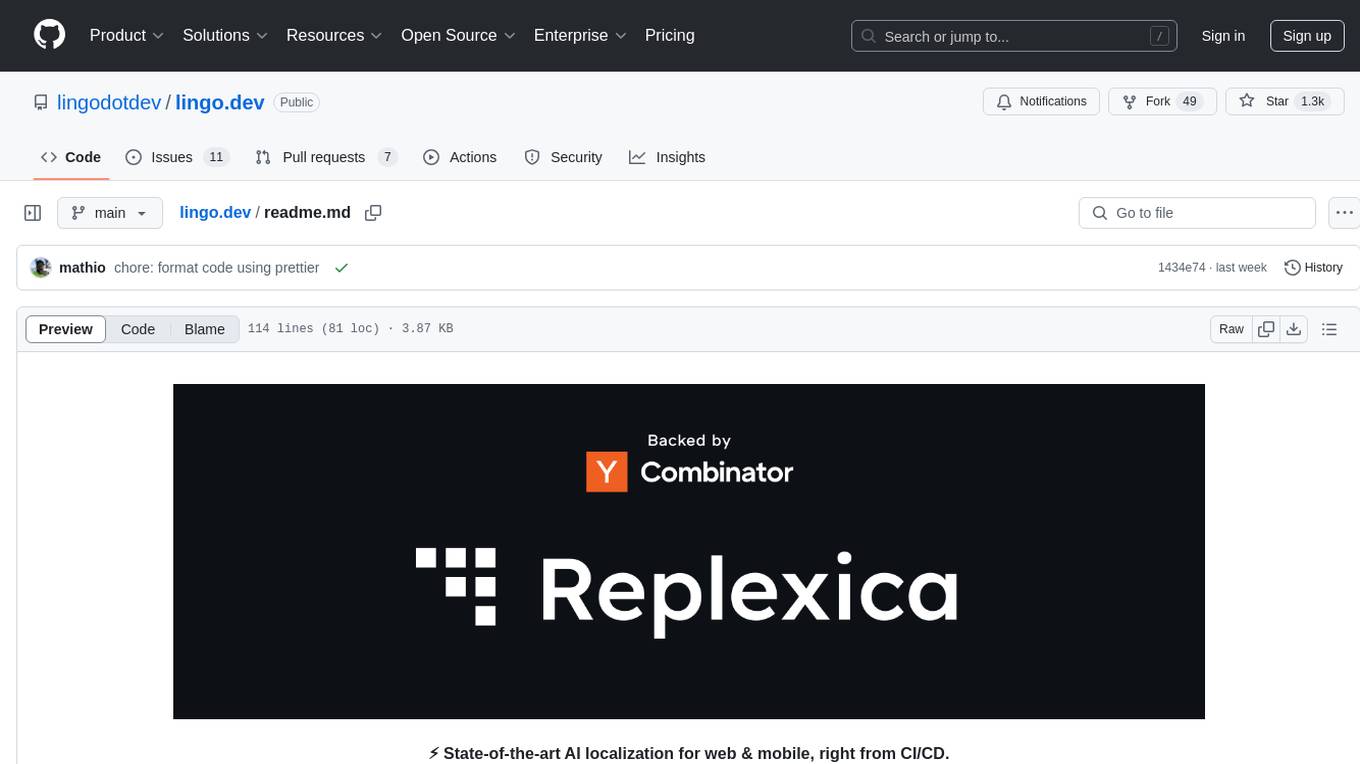
lingo.dev
Replexica AI automates software localization end-to-end, producing authentic translations instantly across 60+ languages. Teams can do localization 100x faster with state-of-the-art quality, reaching more paying customers worldwide. The tool offers a GitHub Action for CI/CD automation and supports various formats like JSON, YAML, CSV, and Markdown. With lightning-fast AI localization, auto-updates, native quality translations, developer-friendly CLI, and scalability for startups and enterprise teams, Replexica is a top choice for efficient and effective software localization.
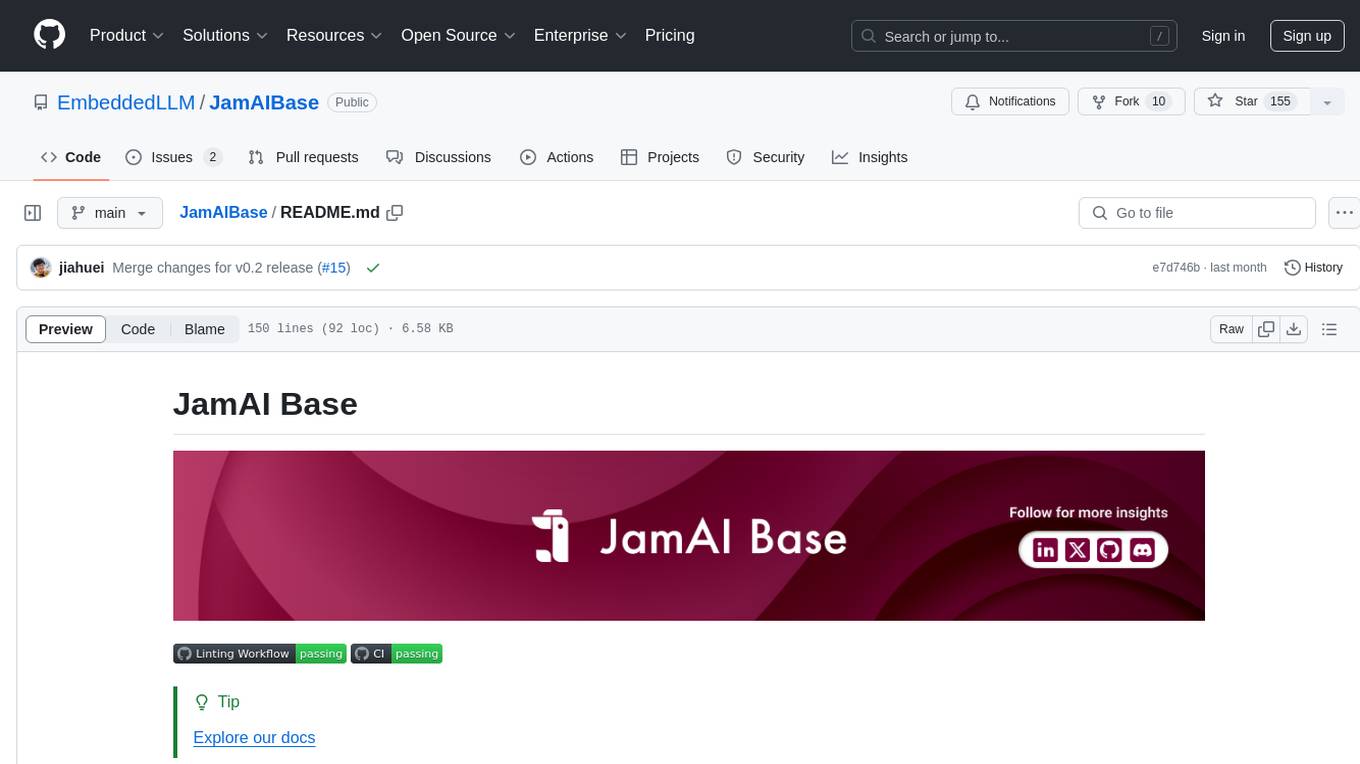
JamAIBase
JamAI Base is an open-source platform integrating SQLite and LanceDB databases with managed memory and RAG capabilities. It offers built-in LLM, vector embeddings, and reranker orchestration accessible through a spreadsheet-like UI and REST API. Users can transform static tables into dynamic entities, facilitate real-time interactions, manage structured data, and simplify chatbot development. The tool focuses on ease of use, scalability, flexibility, declarative paradigm, and innovative RAG techniques, making complex data operations accessible to users with varying technical expertise.
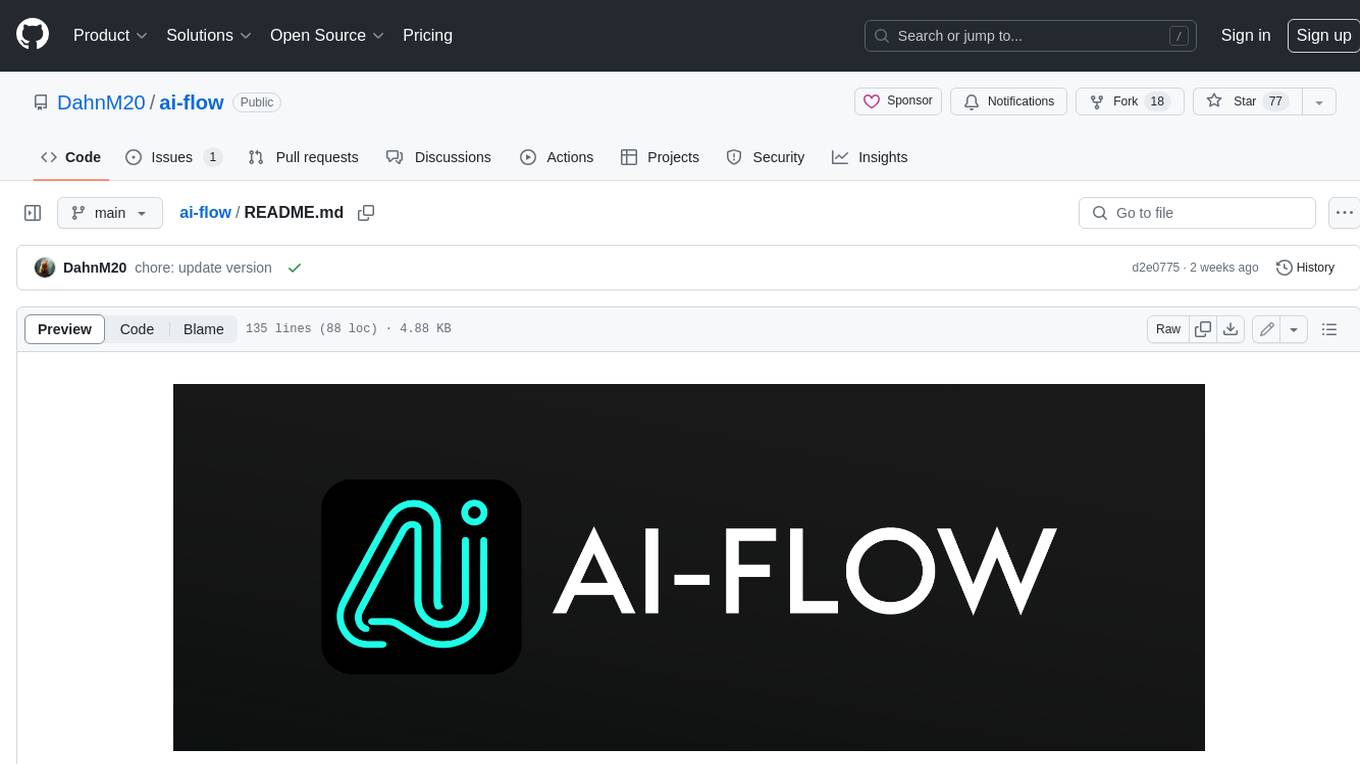
ai-flow
AI Flow is an open-source, user-friendly UI application that empowers you to seamlessly connect multiple AI models together, specifically leveraging the capabilities of multiples AI APIs such as OpenAI, StabilityAI and Replicate. In a nutshell, AI Flow provides a visual platform for crafting and managing AI-driven workflows, thereby facilitating diverse and dynamic AI interactions.
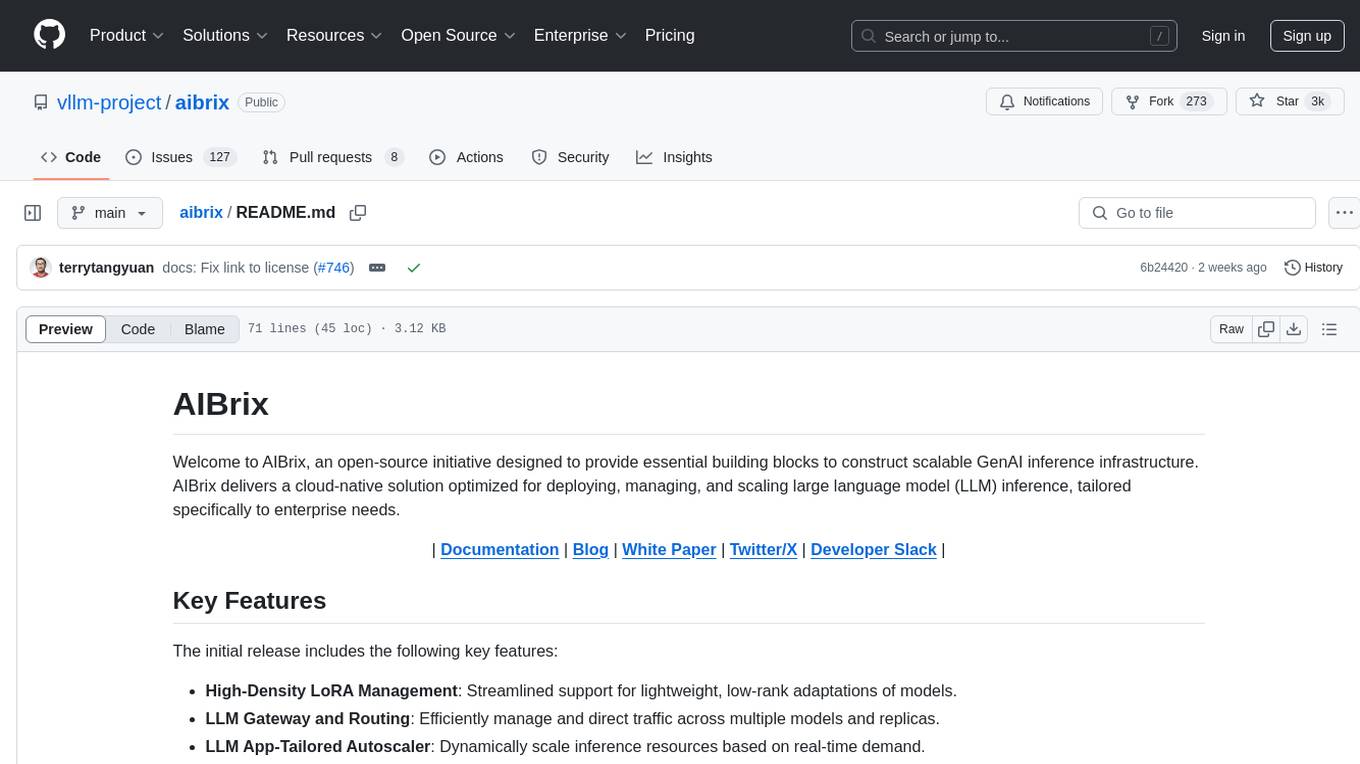
aibrix
AIBrix is an open-source initiative providing essential building blocks for scalable GenAI inference infrastructure. It delivers a cloud-native solution optimized for deploying, managing, and scaling large language model (LLM) inference, tailored to enterprise needs. Key features include High-Density LoRA Management, LLM Gateway and Routing, LLM App-Tailored Autoscaler, Unified AI Runtime, Distributed Inference, Distributed KV Cache, Cost-efficient Heterogeneous Serving, and GPU Hardware Failure Detection.
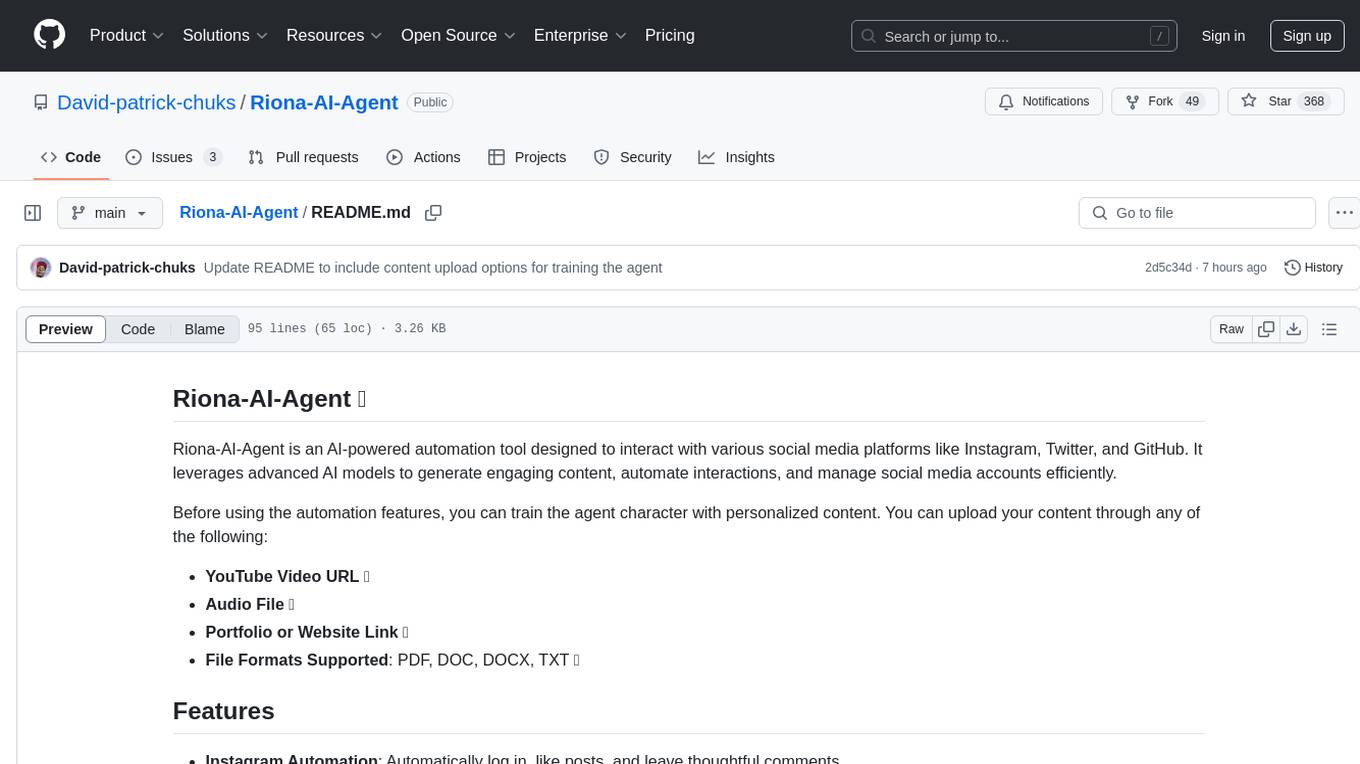
Riona-AI-Agent
Riona-AI-Agent is a versatile AI chatbot designed to assist users in various tasks. It utilizes natural language processing and machine learning algorithms to understand user queries and provide accurate responses. The chatbot can be integrated into websites, applications, and messaging platforms to enhance user experience and streamline communication. With its customizable features and easy deployment, Riona-AI-Agent is suitable for businesses, developers, and individuals looking to automate customer support, provide information, and engage with users in a conversational manner.
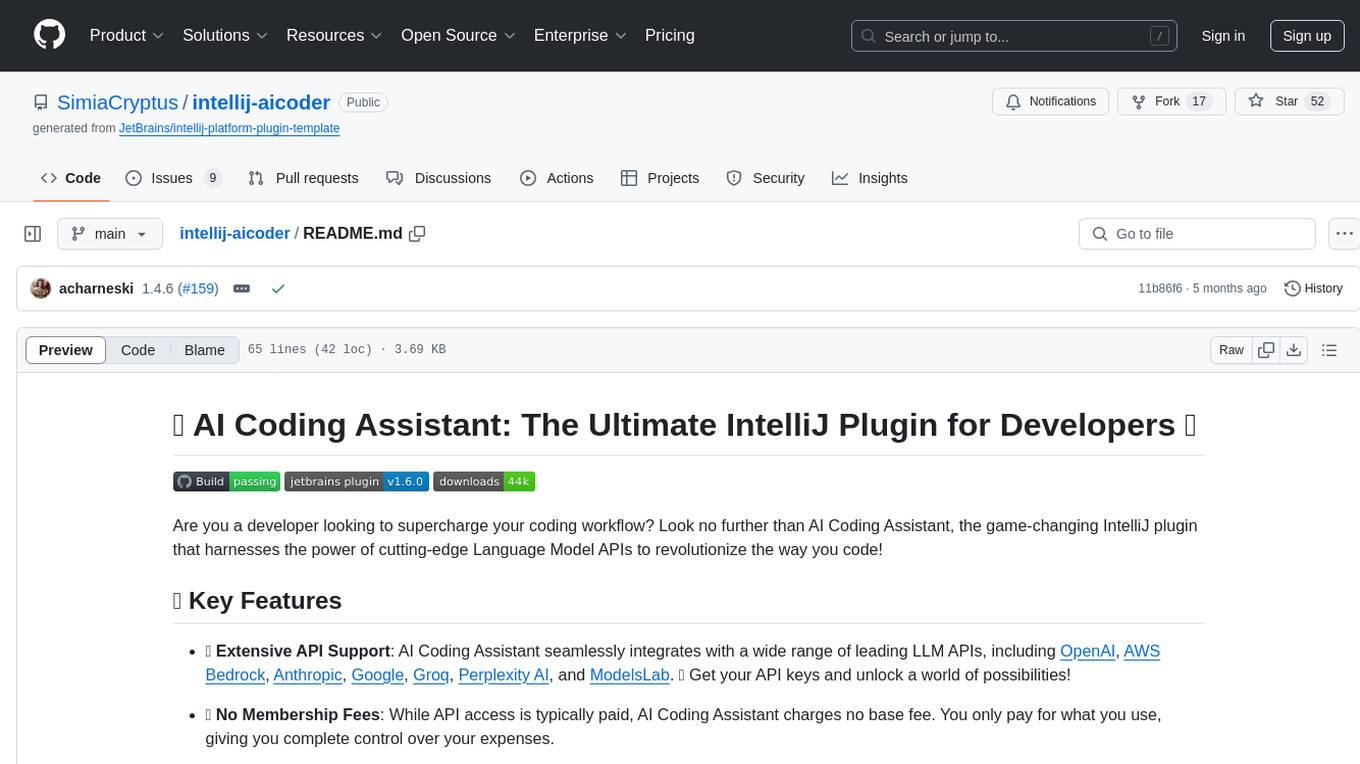
intellij-aicoder
AI Coding Assistant is a free and open-source IntelliJ plugin that leverages cutting-edge Language Model APIs to enhance developers' coding experience. It seamlessly integrates with various leading LLM APIs, offers an intuitive toolbar UI, and allows granular control over API requests. With features like Code & Patch Chat, Planning with AI Agents, Markdown visualization, and versatile text processing capabilities, this tool aims to streamline coding workflows and boost productivity.
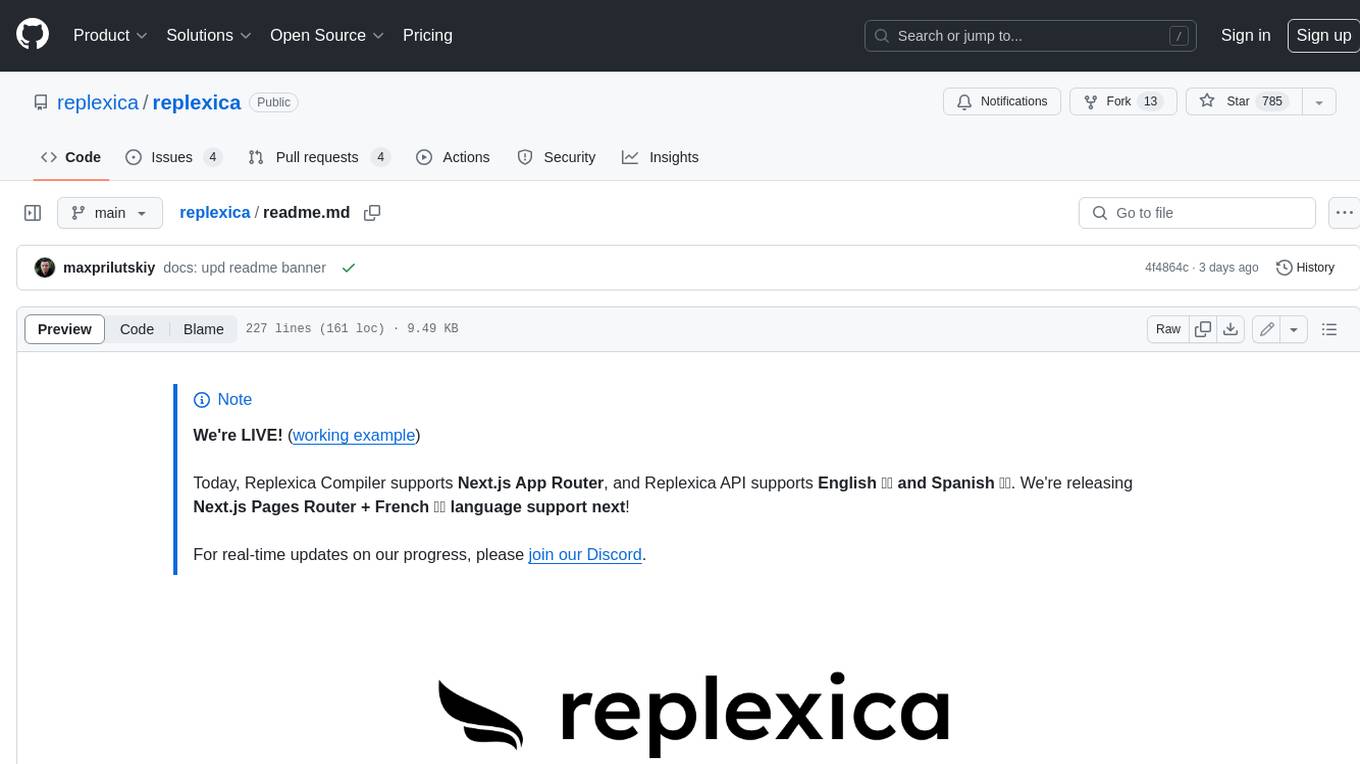
replexica
Replexica is an i18n toolkit for React, to ship multi-language apps fast. It doesn't require extracting text into JSON files, and uses AI-powered API for content processing. It comes in two parts: 1. Replexica Compiler - an open-source compiler plugin for React; 2. Replexica API - an i18n API in the cloud that performs translations using LLMs. (Usage based, has a free tier.) Replexica supports several i18n formats: 1. JSON-free Replexica compiler format; 2. .md files for Markdown content; 3. Legacy JSON and YAML-based formats.
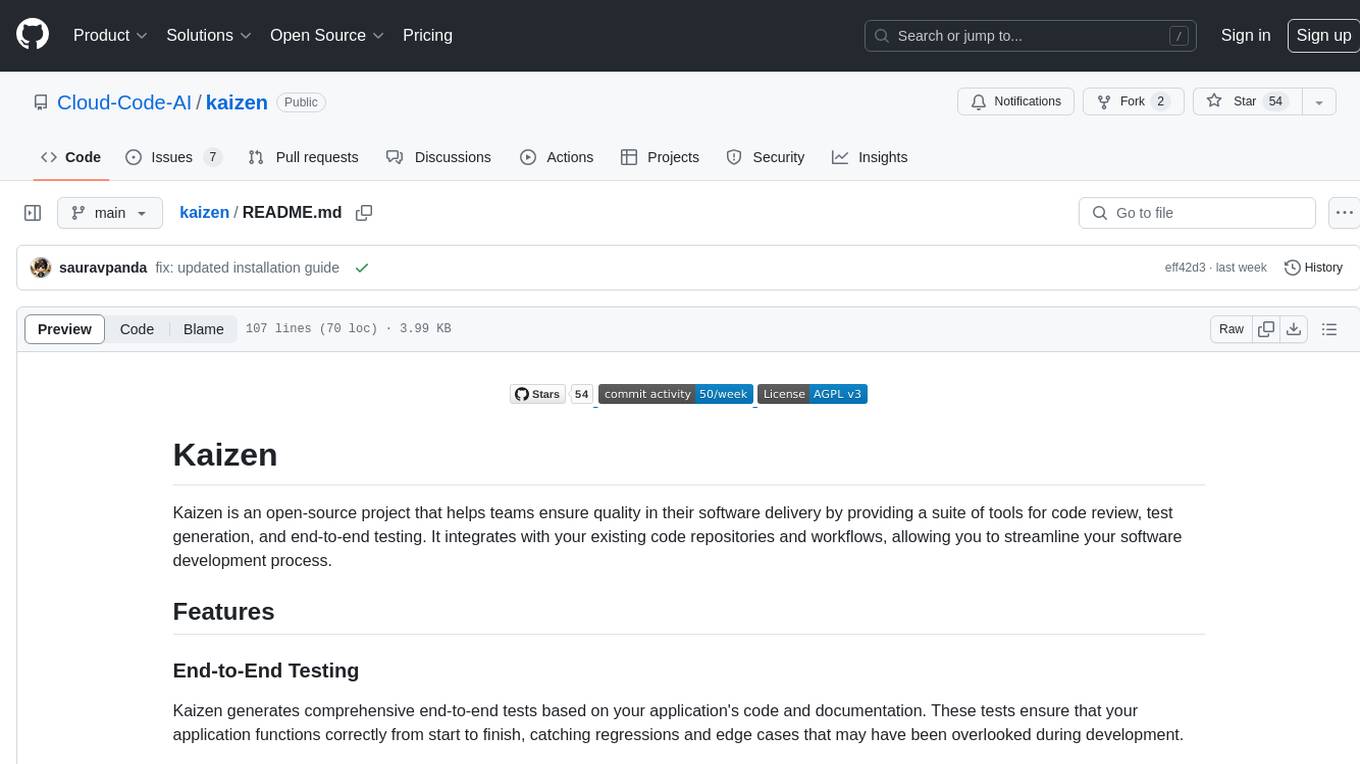
kaizen
Kaizen is an open-source project that helps teams ensure quality in their software delivery by providing a suite of tools for code review, test generation, and end-to-end testing. It integrates with your existing code repositories and workflows, allowing you to streamline your software development process. Kaizen generates comprehensive end-to-end tests, provides UI testing and review, and automates code review with insightful feedback. The file structure includes components for API server, logic, actors, generators, LLM integrations, documentation, and sample code. Getting started involves installing the Kaizen package, generating tests for websites, and executing tests. The tool also runs an API server for GitHub App actions. Contributions are welcome under the AGPL License.
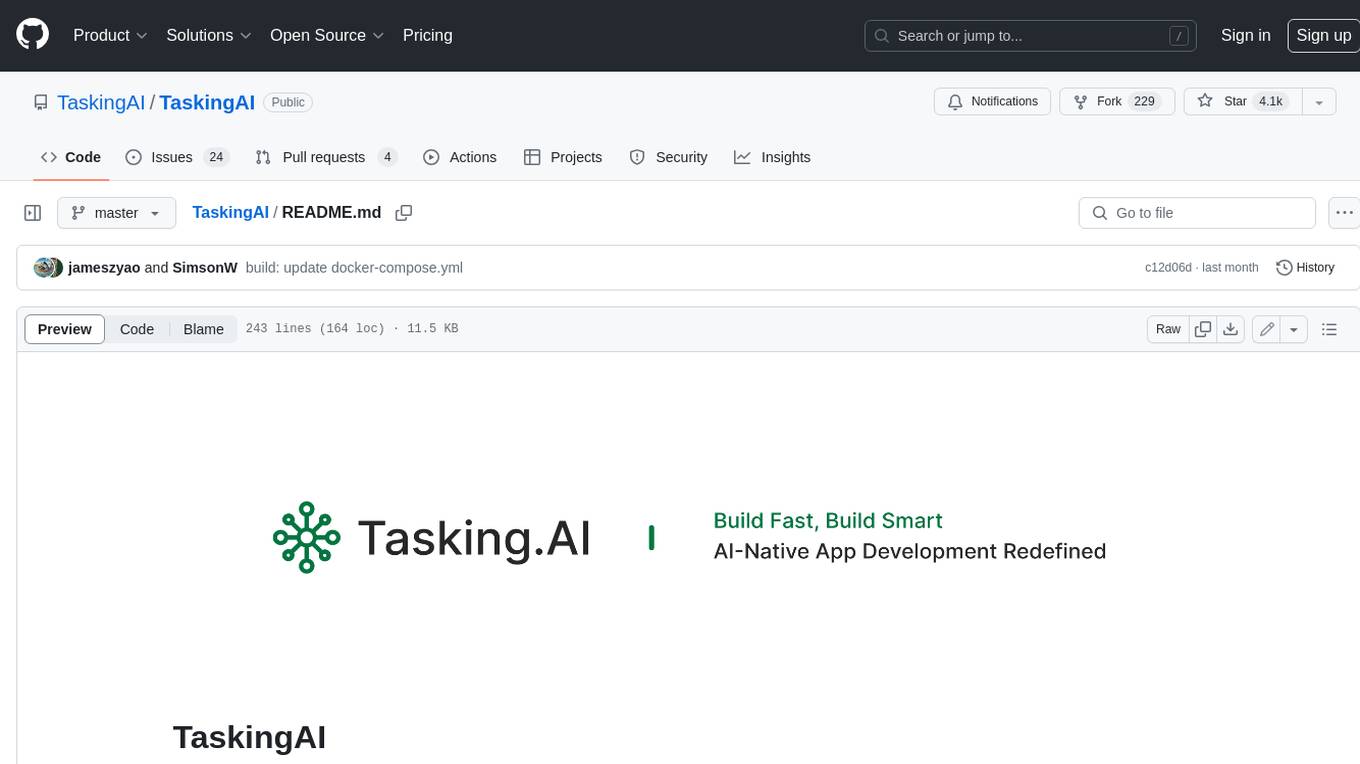
TaskingAI
TaskingAI brings Firebase's simplicity to **AI-native app development**. The platform enables the creation of GPTs-like multi-tenant applications using a wide range of LLMs from various providers. It features distinct, modular functions such as Inference, Retrieval, Assistant, and Tool, seamlessly integrated to enhance the development process. TaskingAIβs cohesive design ensures an efficient, intelligent, and user-friendly experience in AI application development.
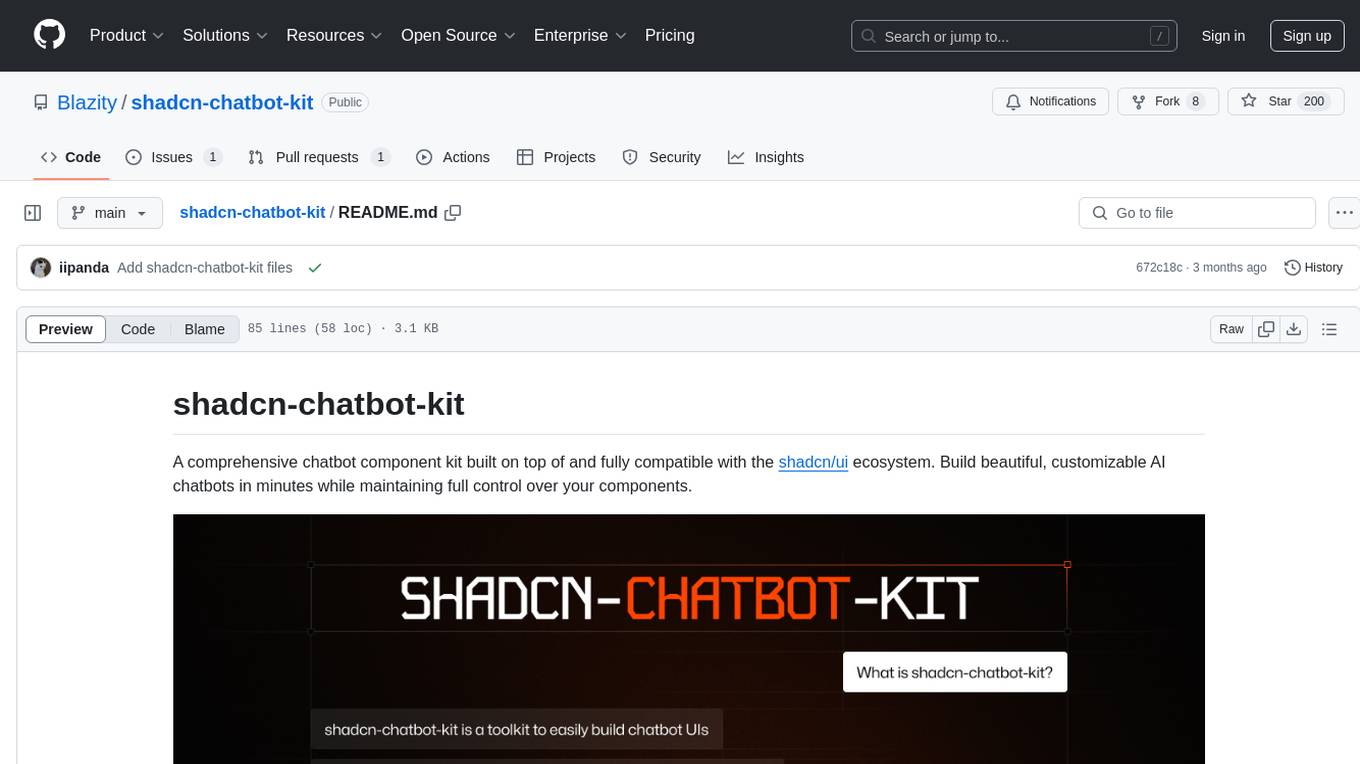
shadcn-chatbot-kit
A comprehensive chatbot component kit built on top of and fully compatible with the shadcn/ui ecosystem. Build beautiful, customizable AI chatbots in minutes while maintaining full control over your components. The kit includes pre-built chat components, auto-scroll message area, message input with auto-resize textarea and file upload support, prompt suggestions, message actions, loading states, and more. Fully themeable, highly customizable, and responsive design. Built with modern web standards and best practices. Installation instructions available with detailed documentation. Customizable using CSS variables.
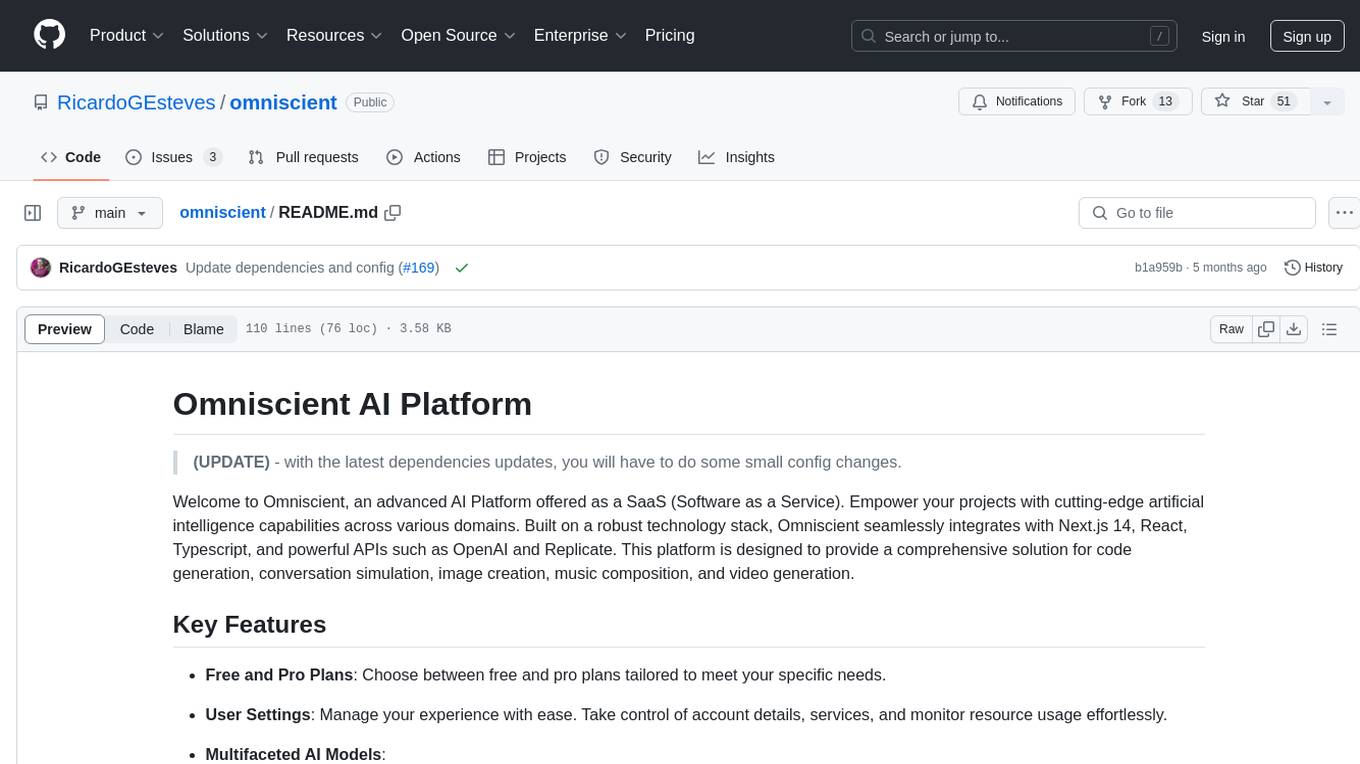
omniscient
Omniscient is an advanced AI Platform offered as a SaaS, empowering projects with cutting-edge artificial intelligence capabilities. Seamlessly integrating with Next.js 14, React, Typescript, and APIs like OpenAI and Replicate, it provides solutions for code generation, conversation simulation, image creation, music composition, and video generation.
For similar tasks
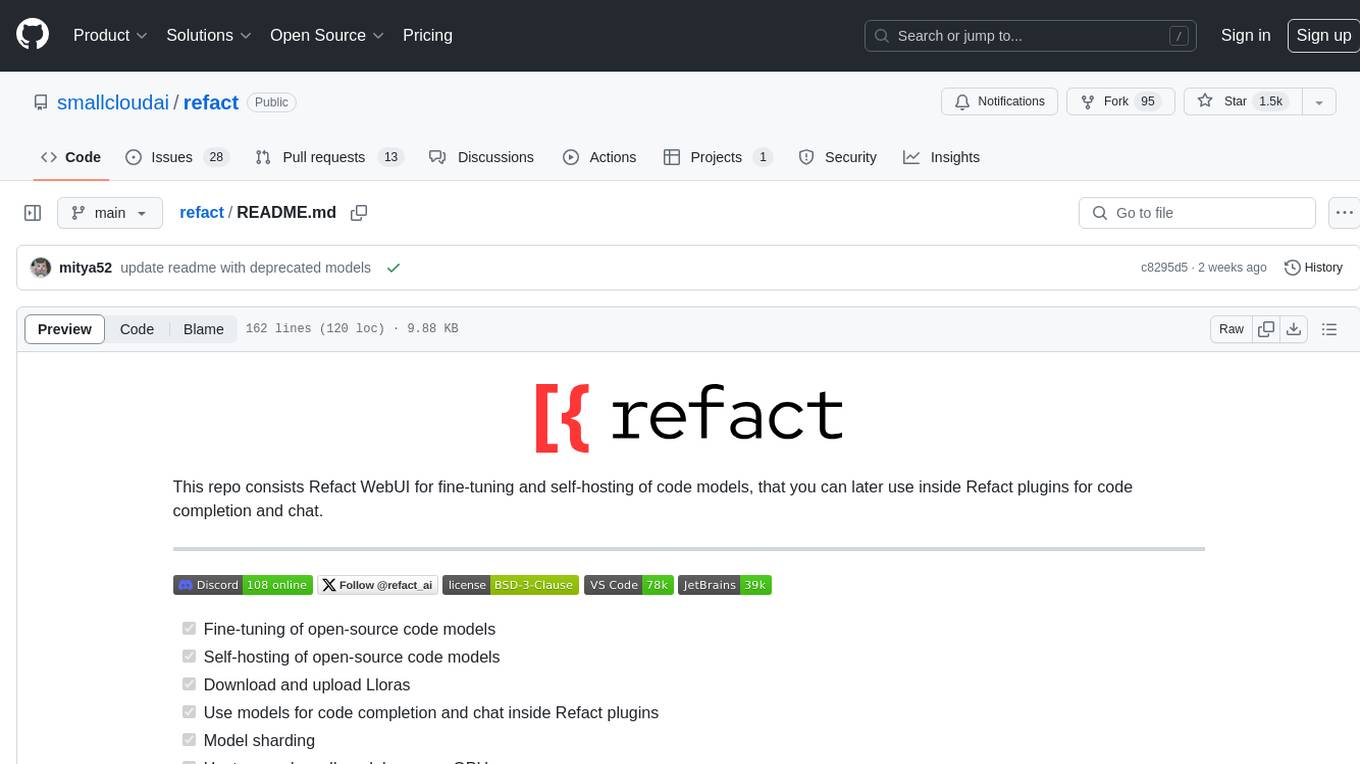
refact
This repository contains Refact WebUI for fine-tuning and self-hosting of code models, which can be used inside Refact plugins for code completion and chat. Users can fine-tune open-source code models, self-host them, download and upload Lloras, use models for code completion and chat inside Refact plugins, shard models, host multiple small models on one GPU, and connect GPT-models for chat using OpenAI and Anthropic keys. The repository provides a Docker container for running the self-hosted server and supports various models for completion, chat, and fine-tuning. Refact is free for individuals and small teams under the BSD-3-Clause license, with custom installation options available for GPU support. The community and support include contributing guidelines, GitHub issues for bugs, a community forum, Discord for chatting, and Twitter for product news and updates.
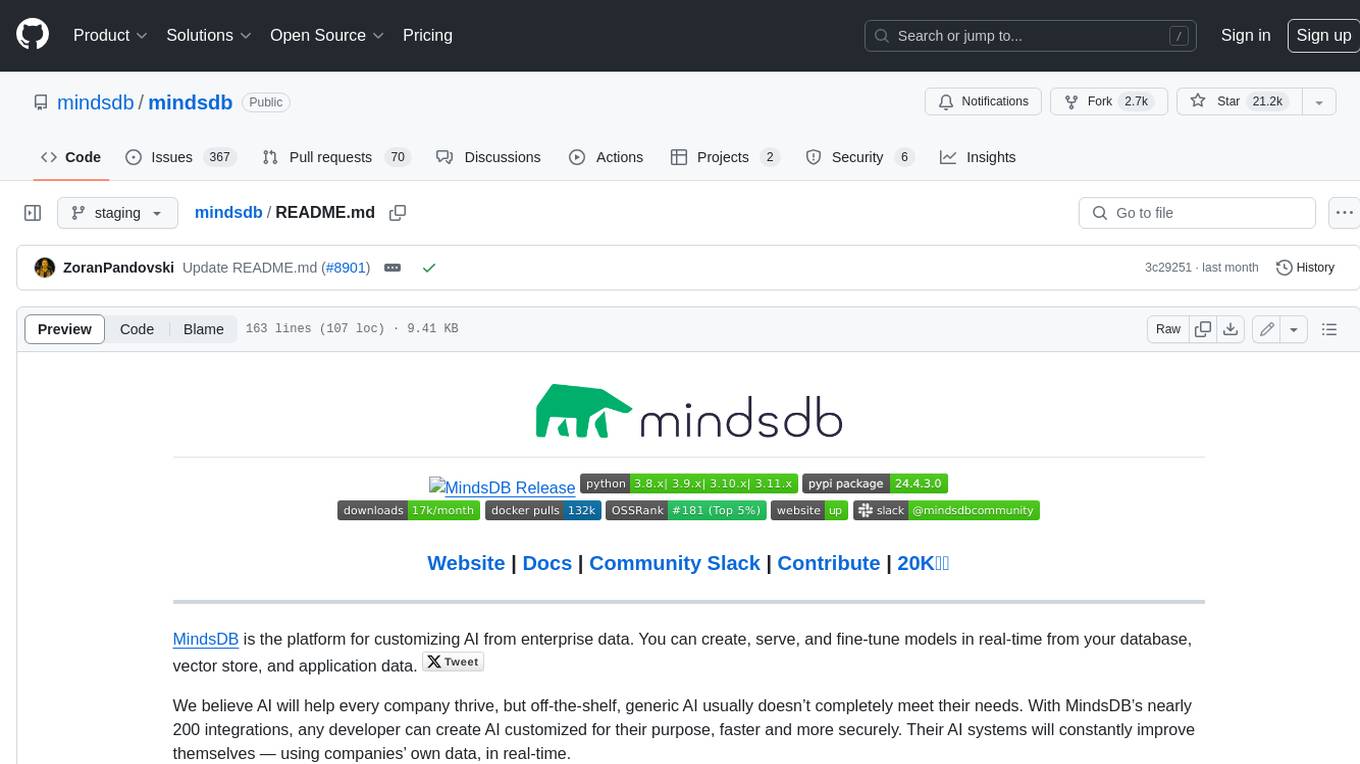
mindsdb
MindsDB is a platform for customizing AI from enterprise data. You can create, serve, and fine-tune models in real-time from your database, vector store, and application data. MindsDB "enhances" SQL syntax with AI capabilities to make it accessible for developers worldwide. With MindsDBβs nearly 200 integrations, any developer can create AI customized for their purpose, faster and more securely. Their AI systems will constantly improve themselves β using companiesβ own data, in real-time.
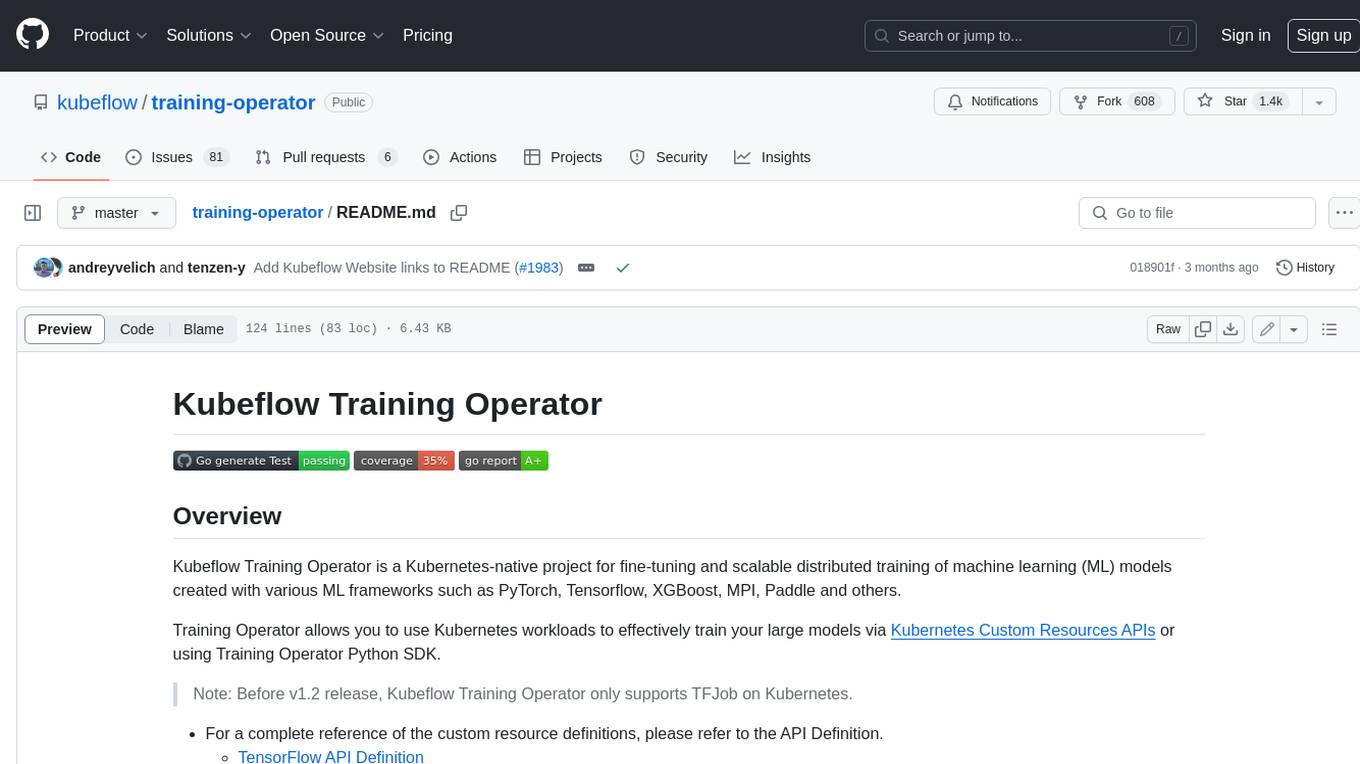
training-operator
Kubeflow Training Operator is a Kubernetes-native project for fine-tuning and scalable distributed training of machine learning (ML) models created with various ML frameworks such as PyTorch, Tensorflow, XGBoost, MPI, Paddle and others. Training Operator allows you to use Kubernetes workloads to effectively train your large models via Kubernetes Custom Resources APIs or using Training Operator Python SDK. > Note: Before v1.2 release, Kubeflow Training Operator only supports TFJob on Kubernetes. * For a complete reference of the custom resource definitions, please refer to the API Definition. * TensorFlow API Definition * PyTorch API Definition * Apache MXNet API Definition * XGBoost API Definition * MPI API Definition * PaddlePaddle API Definition * For details of all-in-one operator design, please refer to the All-in-one Kubeflow Training Operator * For details on its observability, please refer to the monitoring design doc.
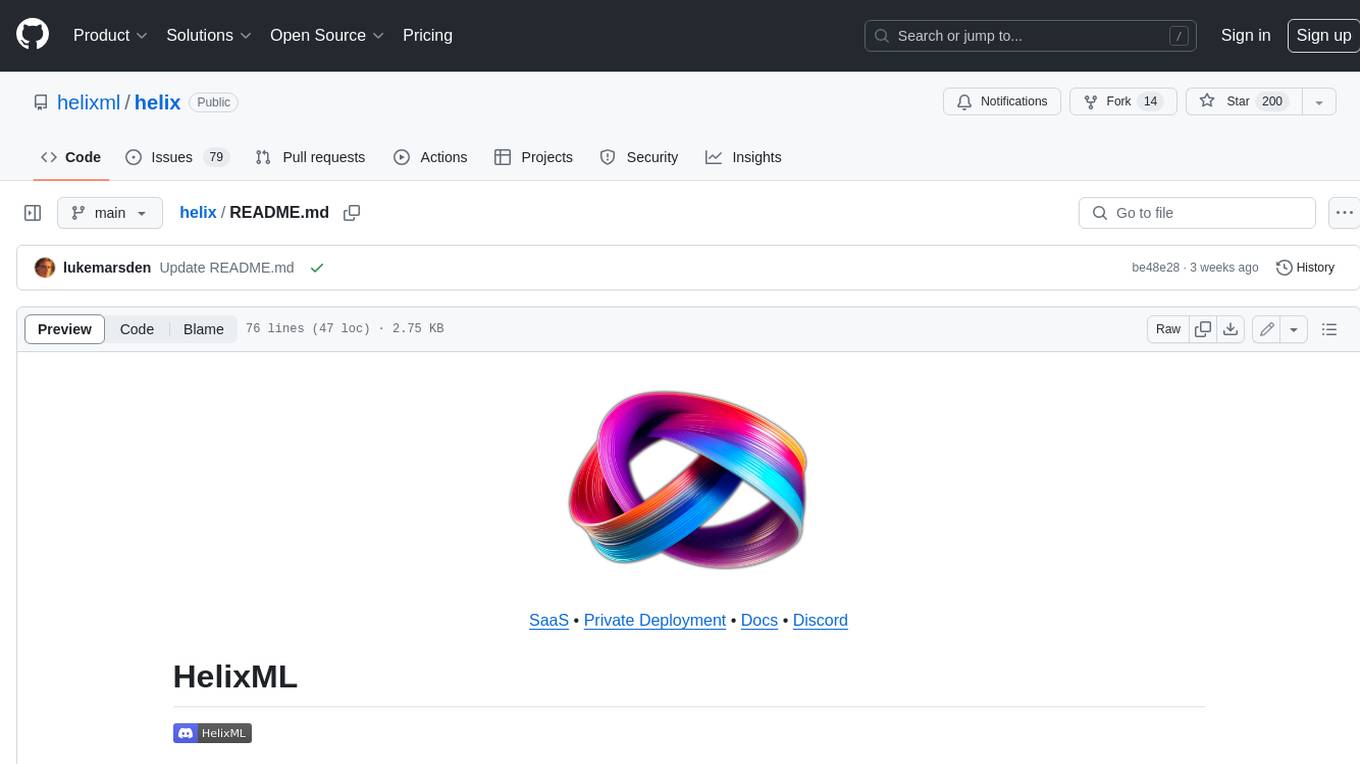
helix
HelixML is a private GenAI platform that allows users to deploy the best of open AI in their own data center or VPC while retaining complete data security and control. It includes support for fine-tuning models with drag-and-drop functionality. HelixML brings the best of open source AI to businesses in an ergonomic and scalable way, optimizing the tradeoff between GPU memory and latency.
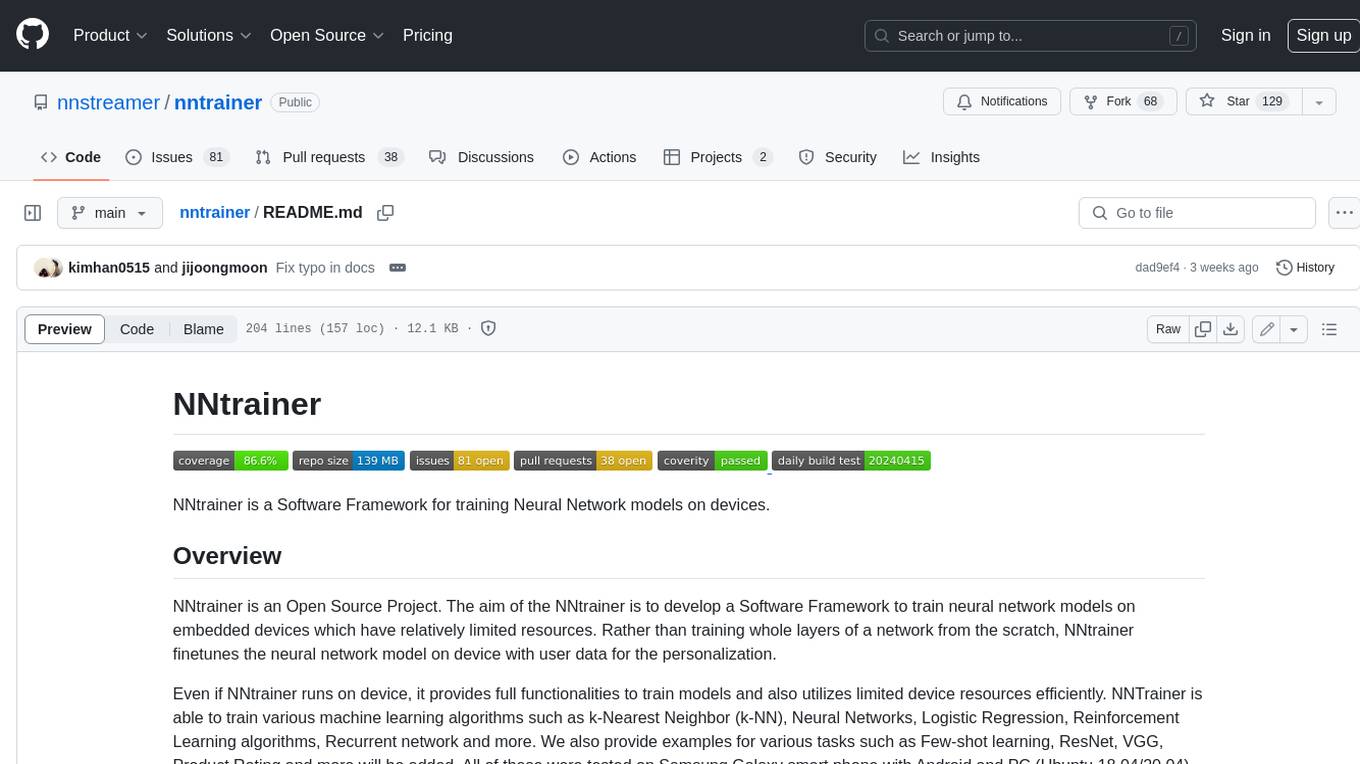
nntrainer
NNtrainer is a software framework for training neural network models on devices with limited resources. It enables on-device fine-tuning of neural networks using user data for personalization. NNtrainer supports various machine learning algorithms and provides examples for tasks such as few-shot learning, ResNet, VGG, and product rating. It is optimized for embedded devices and utilizes CBLAS and CUBLAS for accelerated calculations. NNtrainer is open source and released under the Apache License version 2.0.
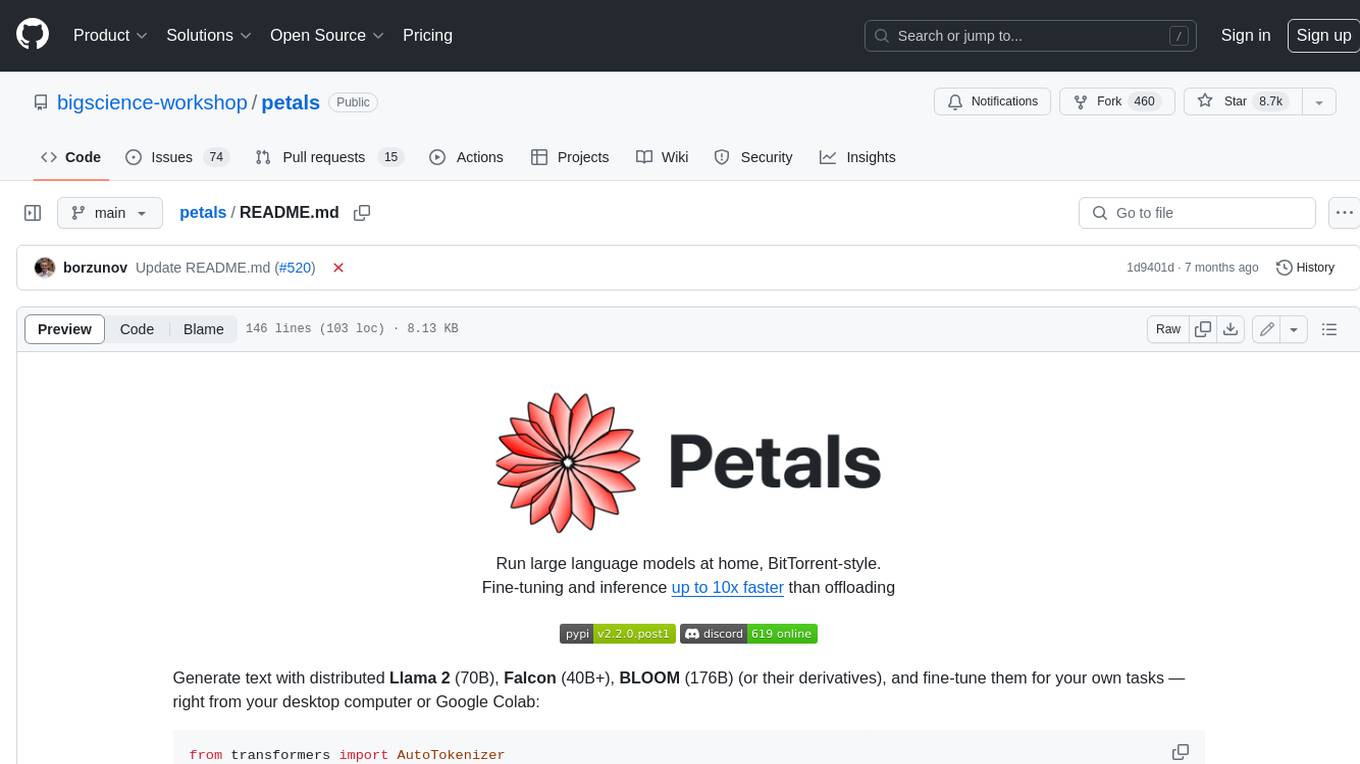
petals
Petals is a tool that allows users to run large language models at home in a BitTorrent-style manner. It enables fine-tuning and inference up to 10x faster than offloading. Users can generate text with distributed models like Llama 2, Falcon, and BLOOM, and fine-tune them for specific tasks directly from their desktop computer or Google Colab. Petals is a community-run system that relies on people sharing their GPUs to increase its capacity and offer a distributed network for hosting model layers.
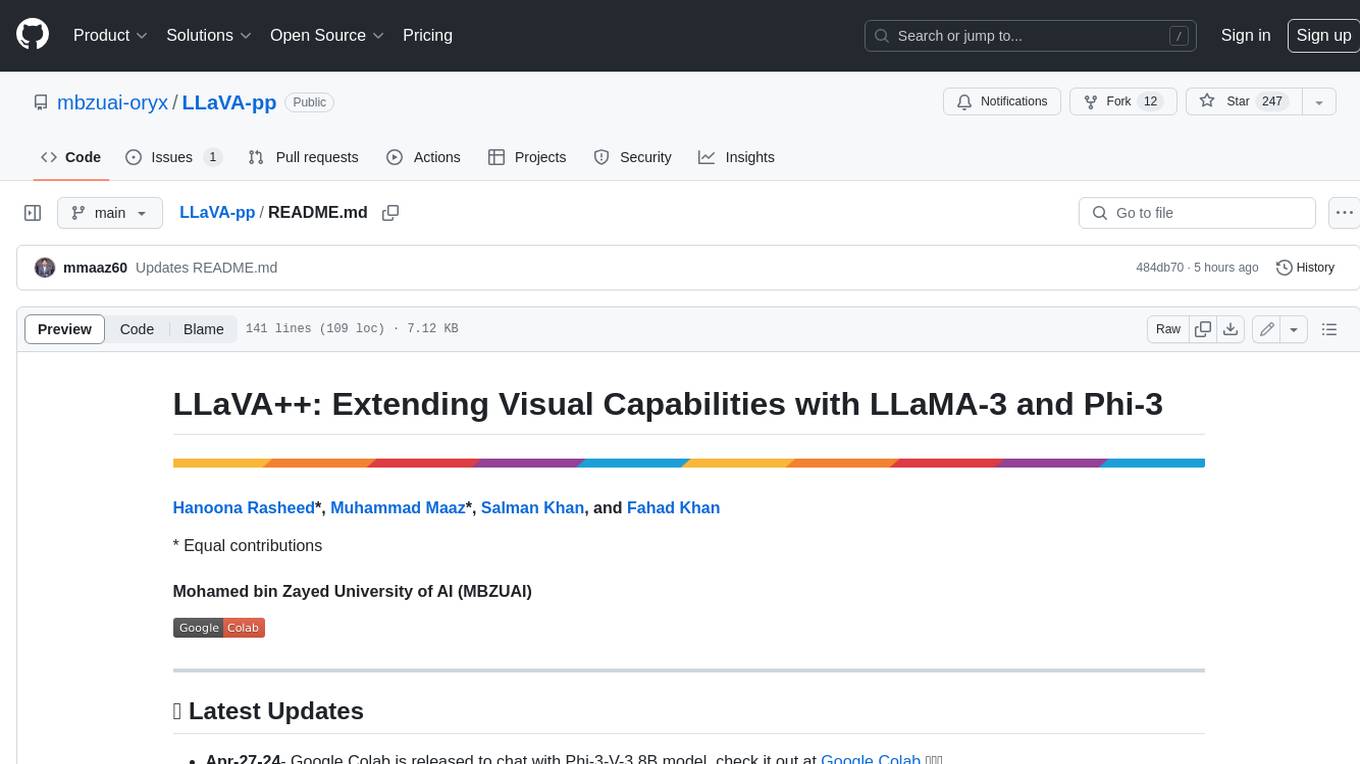
LLaVA-pp
This repository, LLaVA++, extends the visual capabilities of the LLaVA 1.5 model by incorporating the latest LLMs, Phi-3 Mini Instruct 3.8B, and LLaMA-3 Instruct 8B. It provides various models for instruction-following LMMS and academic-task-oriented datasets, along with training scripts for Phi-3-V and LLaMA-3-V. The repository also includes installation instructions and acknowledgments to related open-source contributions.
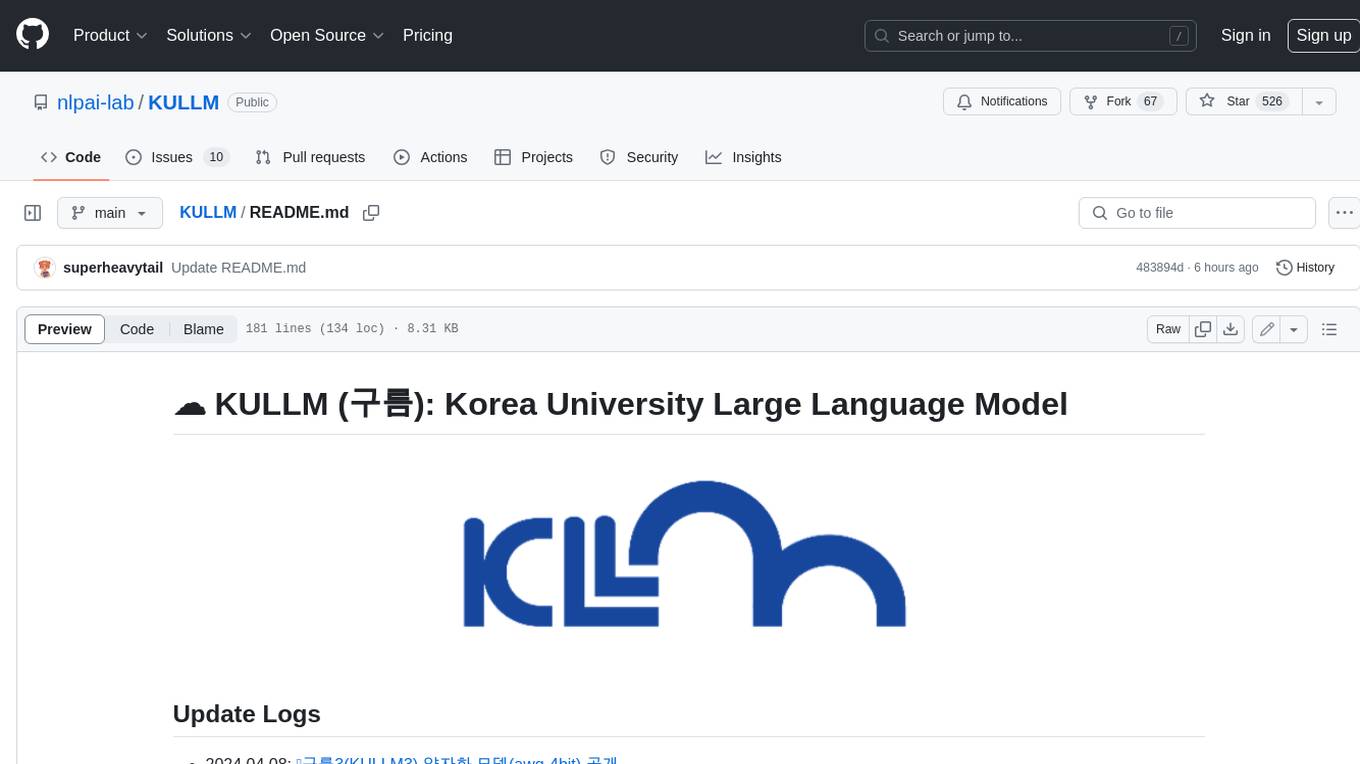
KULLM
KULLM (ꡬλ¦) is a Korean Large Language Model developed by Korea University NLP & AI Lab and HIAI Research Institute. It is based on the upstage/SOLAR-10.7B-v1.0 model and has been fine-tuned for instruction. The model has been trained on 8ΓA100 GPUs and is capable of generating responses in Korean language. KULLM exhibits hallucination and repetition phenomena due to its decoding strategy. Users should be cautious as the model may produce inaccurate or harmful results. Performance may vary in benchmarks without a fixed system prompt.
For similar jobs
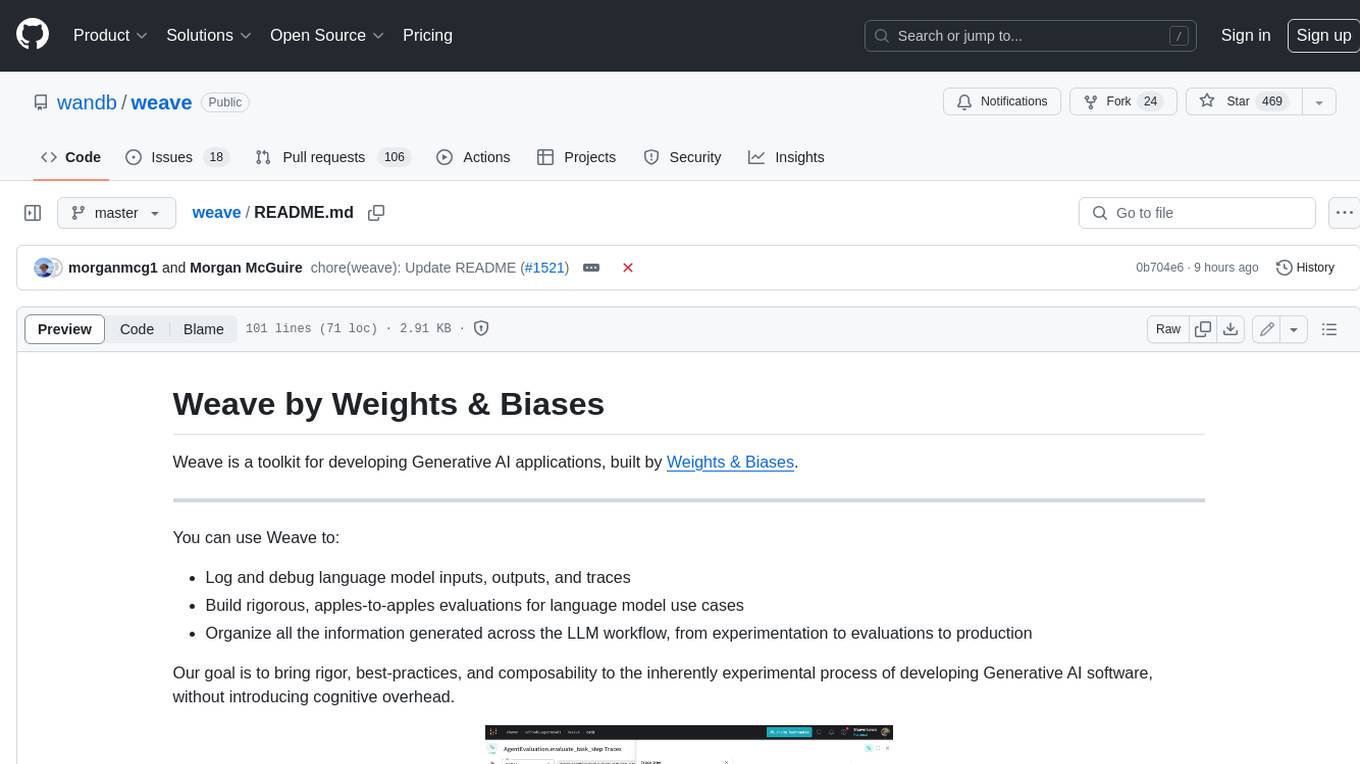
weave
Weave is a toolkit for developing Generative AI applications, built by Weights & Biases. With Weave, you can log and debug language model inputs, outputs, and traces; build rigorous, apples-to-apples evaluations for language model use cases; and organize all the information generated across the LLM workflow, from experimentation to evaluations to production. Weave aims to bring rigor, best-practices, and composability to the inherently experimental process of developing Generative AI software, without introducing cognitive overhead.
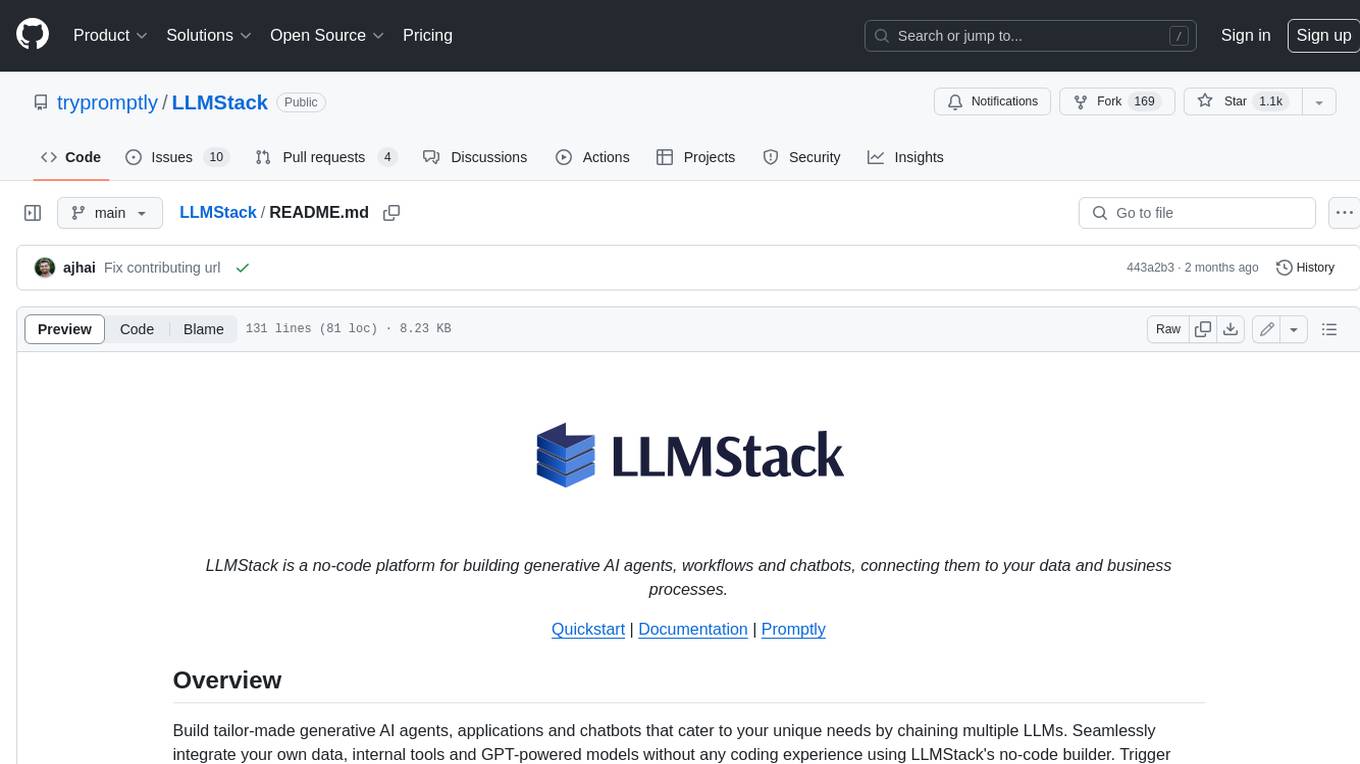
LLMStack
LLMStack is a no-code platform for building generative AI agents, workflows, and chatbots. It allows users to connect their own data, internal tools, and GPT-powered models without any coding experience. LLMStack can be deployed to the cloud or on-premise and can be accessed via HTTP API or triggered from Slack or Discord.
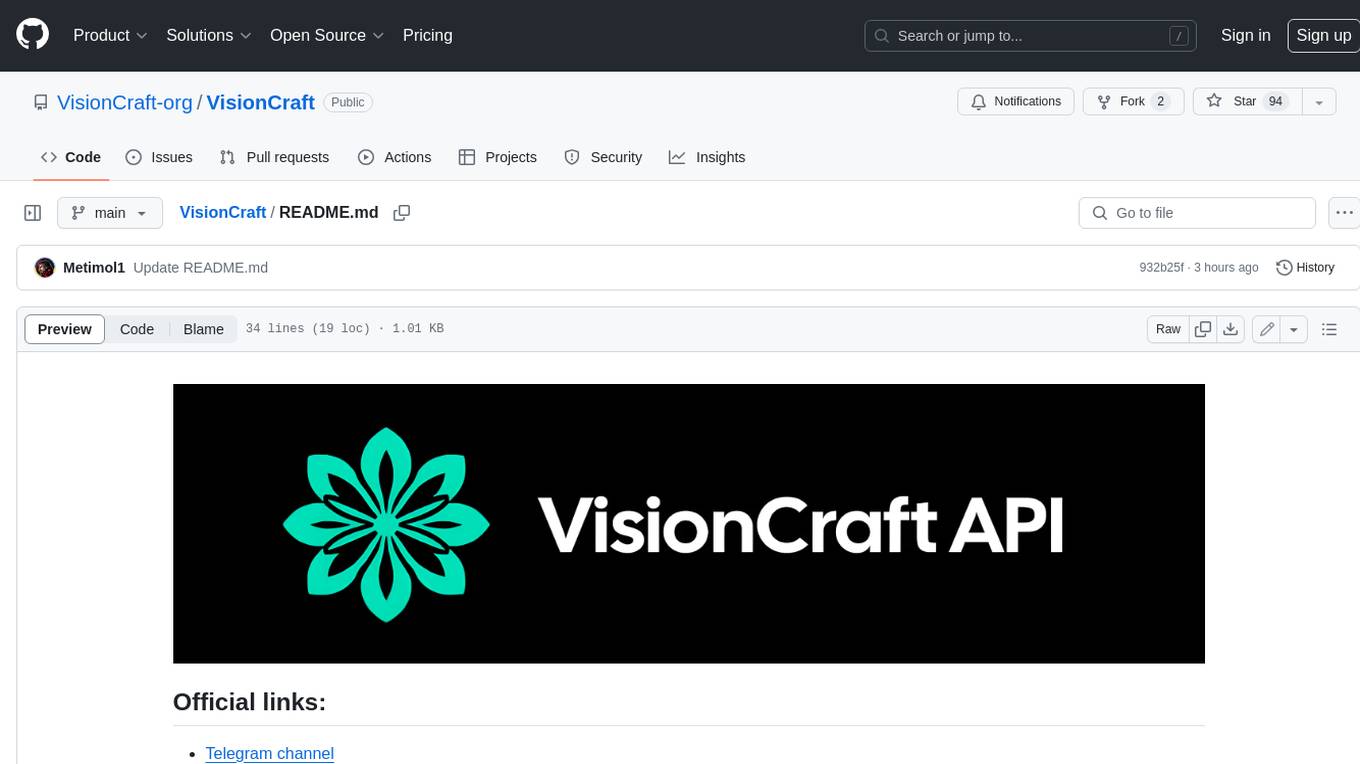
VisionCraft
The VisionCraft API is a free API for using over 100 different AI models. From images to sound.
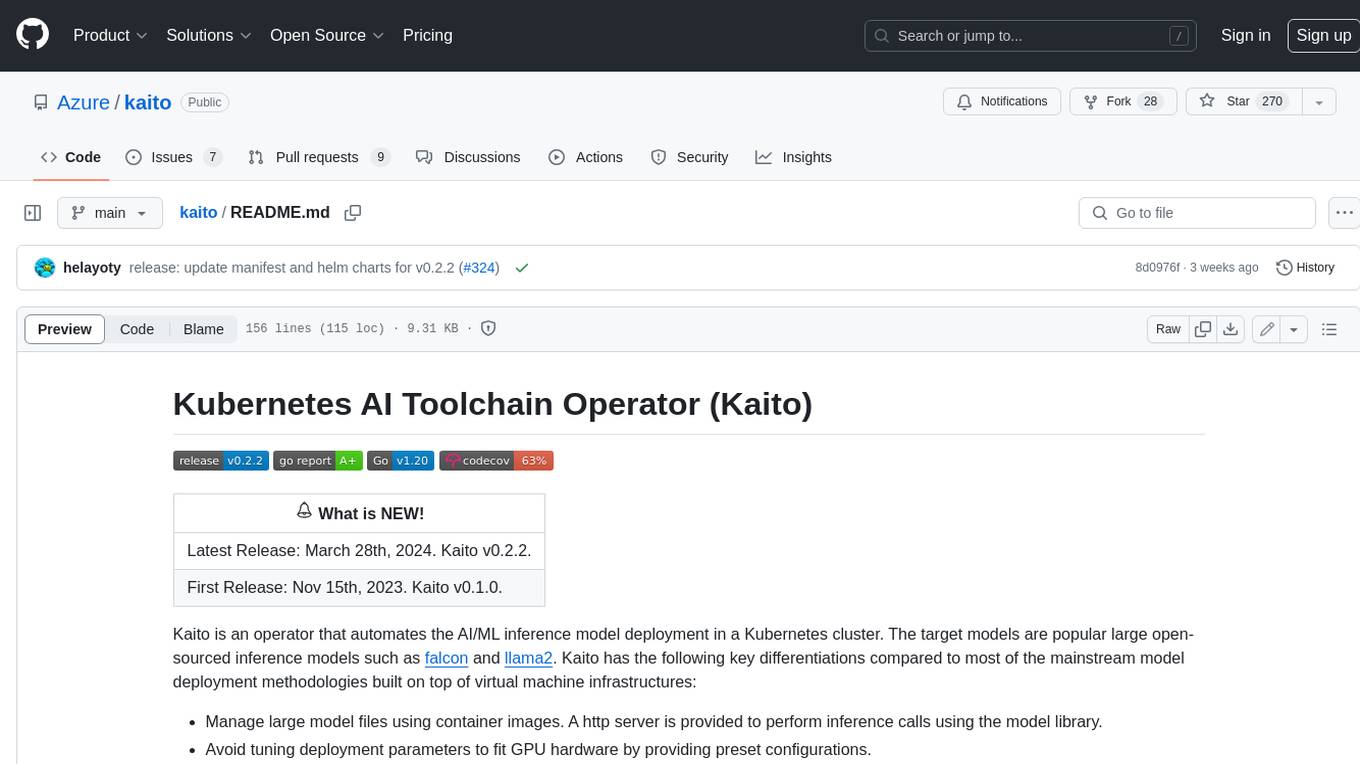
kaito
Kaito is an operator that automates the AI/ML inference model deployment in a Kubernetes cluster. It manages large model files using container images, avoids tuning deployment parameters to fit GPU hardware by providing preset configurations, auto-provisions GPU nodes based on model requirements, and hosts large model images in the public Microsoft Container Registry (MCR) if the license allows. Using Kaito, the workflow of onboarding large AI inference models in Kubernetes is largely simplified.
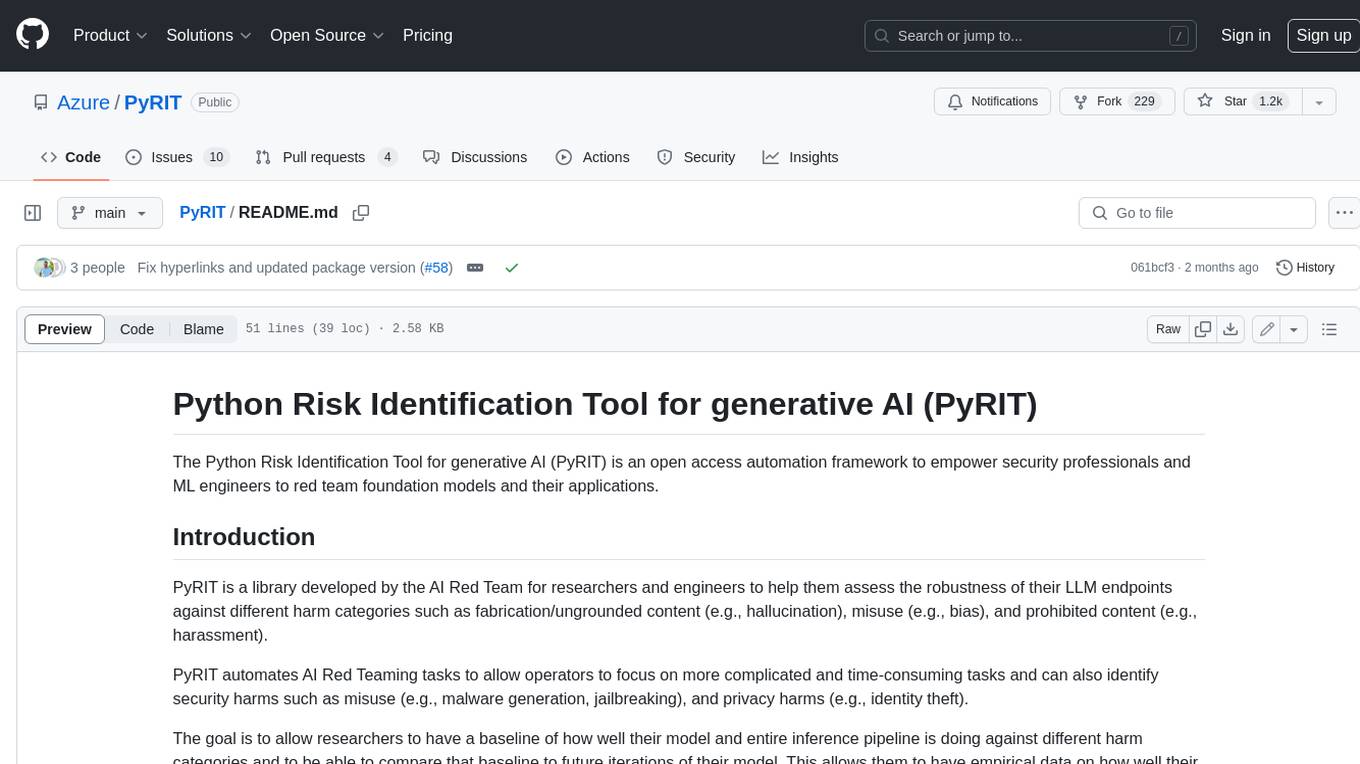
PyRIT
PyRIT is an open access automation framework designed to empower security professionals and ML engineers to red team foundation models and their applications. It automates AI Red Teaming tasks to allow operators to focus on more complicated and time-consuming tasks and can also identify security harms such as misuse (e.g., malware generation, jailbreaking), and privacy harms (e.g., identity theft). The goal is to allow researchers to have a baseline of how well their model and entire inference pipeline is doing against different harm categories and to be able to compare that baseline to future iterations of their model. This allows them to have empirical data on how well their model is doing today, and detect any degradation of performance based on future improvements.
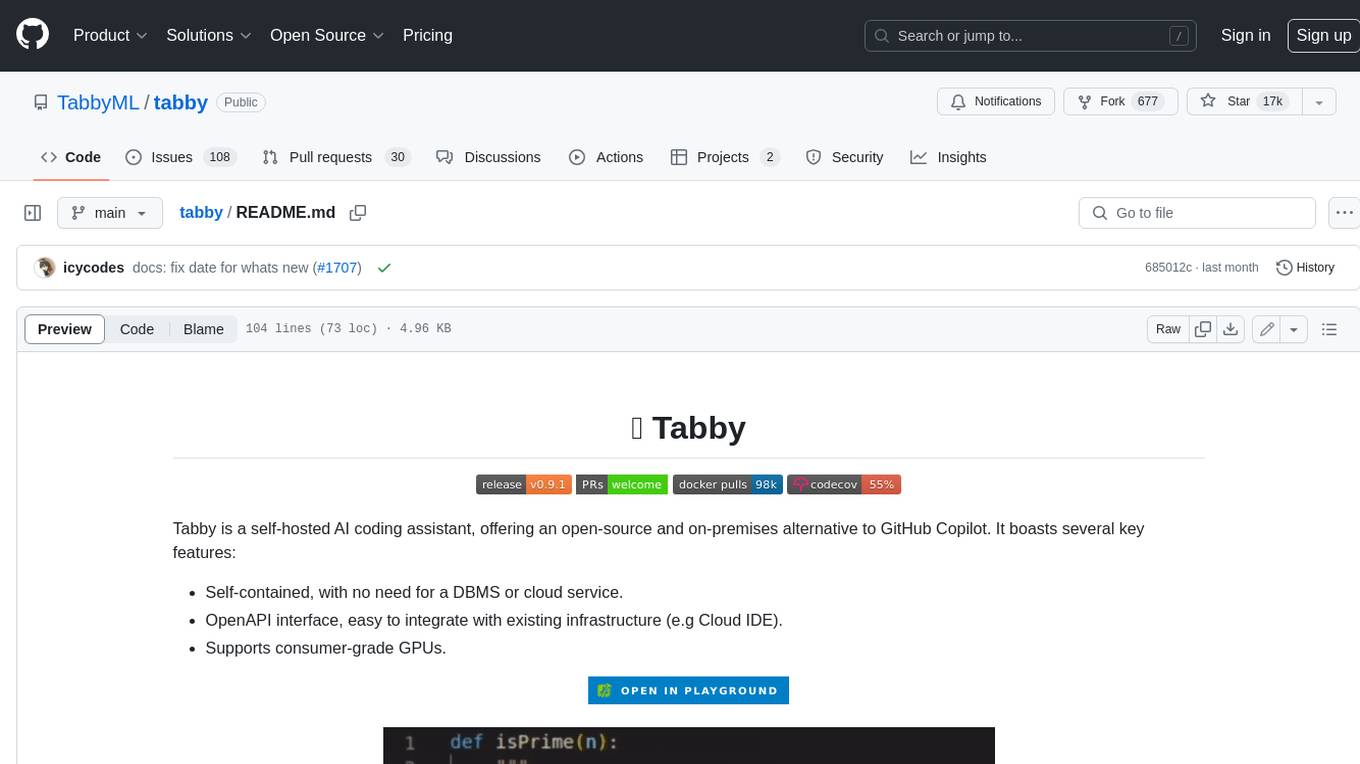
tabby
Tabby is a self-hosted AI coding assistant, offering an open-source and on-premises alternative to GitHub Copilot. It boasts several key features: * Self-contained, with no need for a DBMS or cloud service. * OpenAPI interface, easy to integrate with existing infrastructure (e.g Cloud IDE). * Supports consumer-grade GPUs.
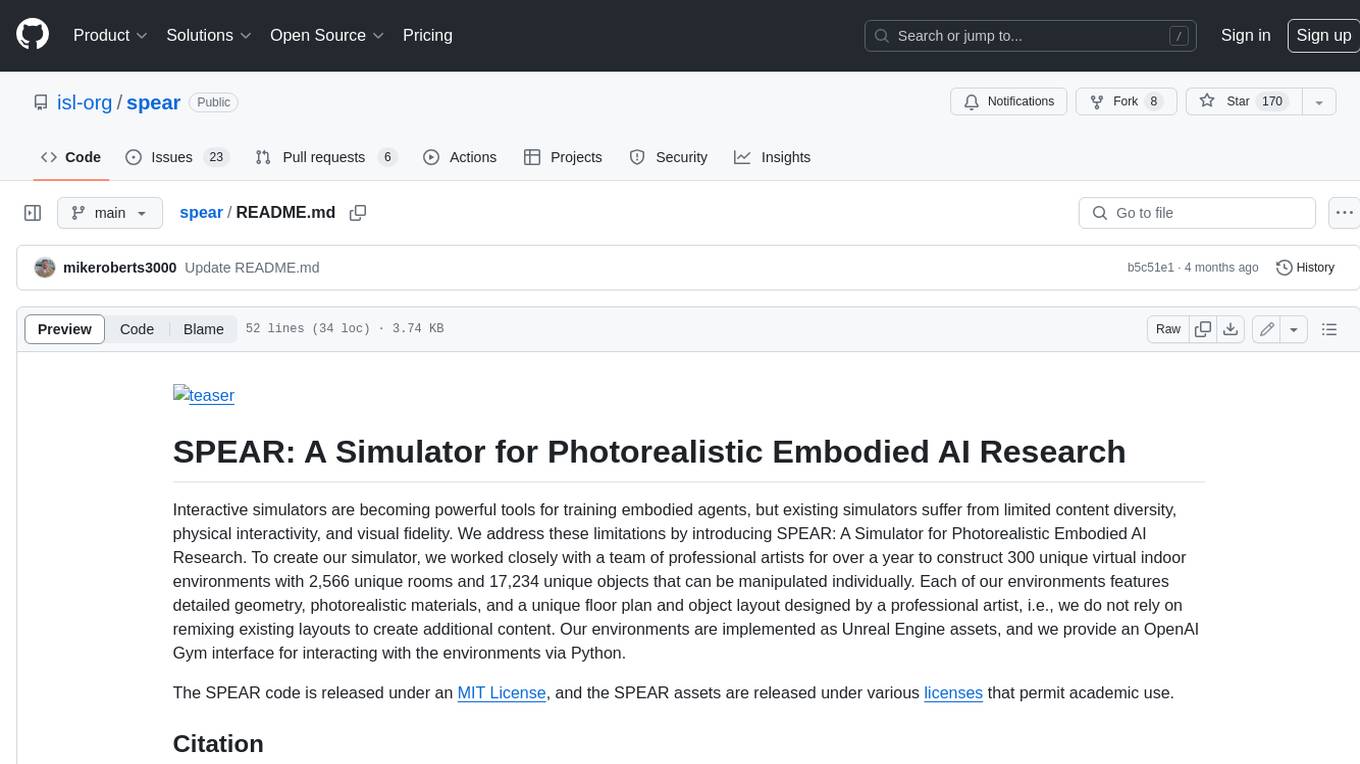
spear
SPEAR (Simulator for Photorealistic Embodied AI Research) is a powerful tool for training embodied agents. It features 300 unique virtual indoor environments with 2,566 unique rooms and 17,234 unique objects that can be manipulated individually. Each environment is designed by a professional artist and features detailed geometry, photorealistic materials, and a unique floor plan and object layout. SPEAR is implemented as Unreal Engine assets and provides an OpenAI Gym interface for interacting with the environments via Python.
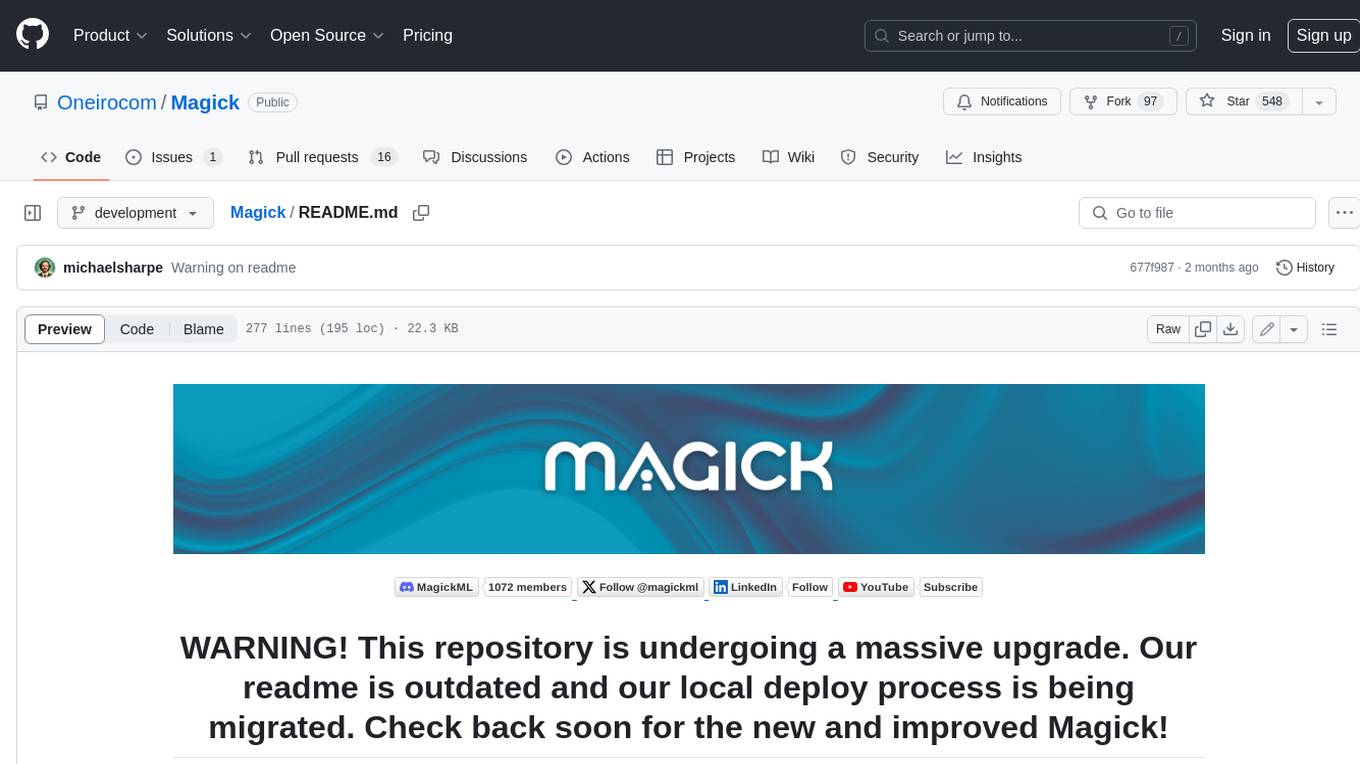
Magick
Magick is a groundbreaking visual AIDE (Artificial Intelligence Development Environment) for no-code data pipelines and multimodal agents. Magick can connect to other services and comes with nodes and templates well-suited for intelligent agents, chatbots, complex reasoning systems and realistic characters.