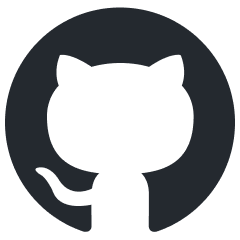
redis-ai-resources
✨ A curated list of awesome community resources, integrations, and examples of Redis in the AI ecosystem.
Stars: 170
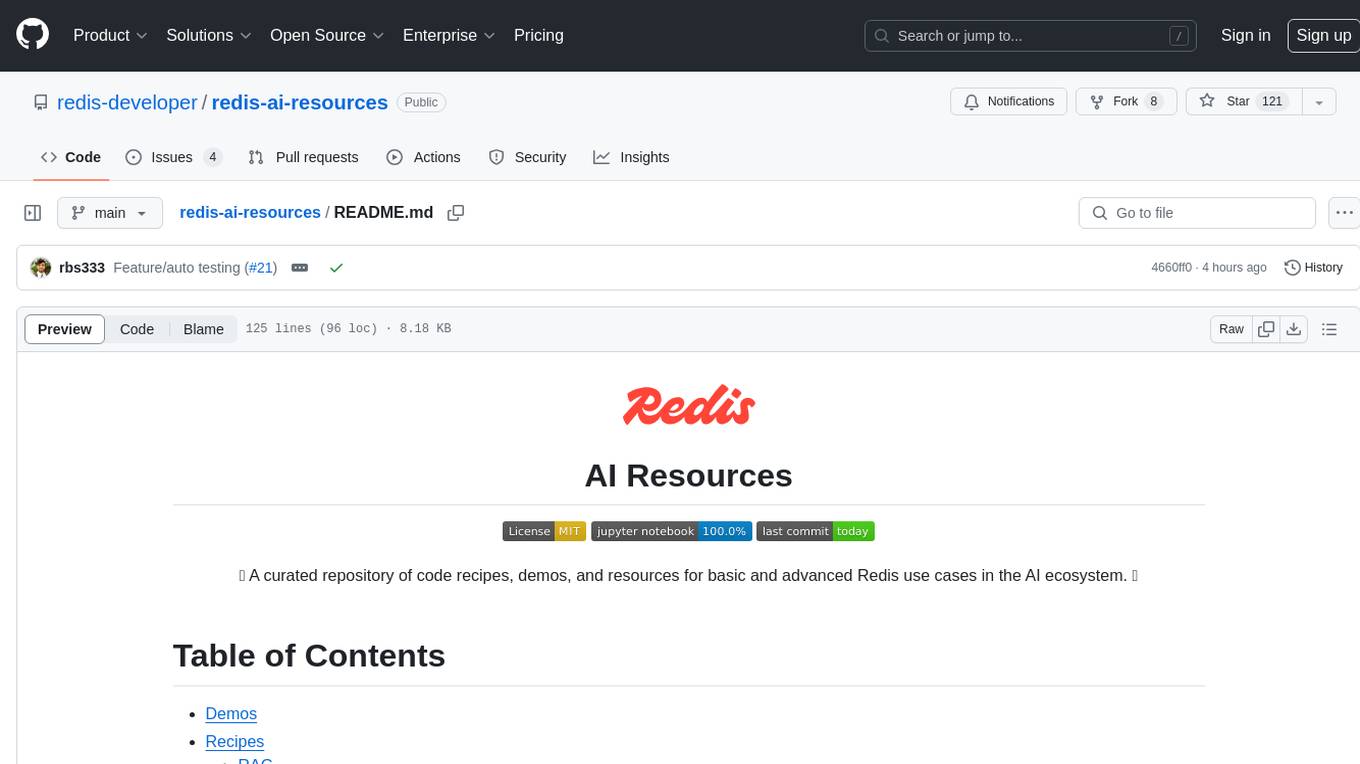
A curated repository of code recipes, demos, and resources for basic and advanced Redis use cases in the AI ecosystem. It includes demos for ArxivChatGuru, Redis VSS, Vertex AI & Redis, Agentic RAG, ArXiv Search, and Product Search. Recipes cover topics like Getting started with RAG, Semantic Cache, Advanced RAG, and Recommendation systems. The repository also provides integrations/tools like RedisVL, AWS Bedrock, LangChain Python, LangChain JS, LlamaIndex, Semantic Kernel, RelevanceAI, and DocArray. Additional content includes blog posts, talks, reviews, and documentation related to Vector Similarity Search, AI-Powered Document Search, Vector Databases, Real-Time Product Recommendations, and more. Benchmarks compare Redis against other Vector Databases and ANN benchmarks. Documentation includes QuickStart guides, official literature for Vector Similarity Search, Redis-py client library docs, Redis Stack documentation, and Redis client list.
README:
Demos | Recipes | Tutorials | Integrations | Content | Benchmarks | Docs
No faster way to get started than by diving in and playing around with a demo.
Demo | Description |
---|---|
Redis RAG Workbench | Interactive demo to build a RAG-based chatbot over a user-uploaded PDF. Toggle different settings and configurations to improve chatbot performance and quality. Utilizes RedisVL, LangChain, RAGAs, and more. |
Redis VSS - Simple Streamlit Demo | Streamlit demo of Redis Vector Search |
ArXiv Search | Full stack implementation of Redis with React FE |
Product Search | Vector search with Redis Stack and Redis Enterprise |
ArxivChatGuru | Streamlit demo of RAG over Arxiv documents with Redis & OpenAI |
Need quickstarts to begin your Redis AI journey? Start here.
Recipe | Description |
---|---|
/redis-intro/00_redis_intro.ipynb | The place to start if brand new to Redis |
/vector-search/00_redispy.ipynb | Vector search with Redis python client |
/vector-search/01_redisvl.ipynb | Vector search with Redis Vector Library |
/vector-search/02_hybrid_search.ipynb | Hybrid search techniques with Redis (BM25 + Vector) |
/vector-search/03_float16_support.ipynb | Shows how to convert a float32 index to use float16 |
Retrieval Augmented Generation (aka RAG) is a technique to enhance the ability of an LLM to respond to user queries. The retrieval part of RAG is supported by a vector database, which can return semantically relevant results to a user’s query, serving as contextual information to augment the generative capabilities of an LLM.
To get started with RAG, either from scratch or using a popular framework like Llamaindex or LangChain, go with these recipes:
Recipe | Description |
---|---|
/RAG/01_redisvl.ipynb | RAG from scratch with the Redis Vector Library |
/RAG/02_langchain.ipynb | RAG using Redis and LangChain |
/RAG/03_llamaindex.ipynb | RAG using Redis and LlamaIndex |
/RAG/04_advanced_redisvl.ipynb | Advanced RAG techniques |
/RAG/05_nvidia_ai_rag_redis.ipynb | RAG using Redis and Nvidia NIMs |
/RAG/06_ragas_evaluation.ipynb | Utilize the RAGAS framework to evaluate RAG performance |
/RAG/07_user_role_based_rag.ipynb | Implement a simple RBAC policy with vector search using Redis |
LLMs are stateless. To maintain context within a conversation chat sessions must be stored and resent to the LLM. Redis manages the storage and retrieval of chat sessions to maintain context and conversational relevance.
Recipe | Description |
---|---|
/llm-session-manager/00_session_manager.ipynb | LLM session manager with semantic similarity |
/llm-session-manager/01_multiple_sessions.ipynb | Handle multiple simultaneous chats with one instance |
An estimated 31% of LLM queries are potentially redundant (source). Redis enables semantic caching to help cut down on LLM costs quickly.
Recipe | Description |
---|---|
/semantic-cache/doc2cache_llama3_1.ipynb | Build a semantic cache using the Doc2Cache framework and Llama3.1 |
/semantic-cache/semantic_caching_gemini.ipynb | Build a semantic cache with Redis and Google Gemini |
Routing is a simple and effective way of preventing misuses with your AI application or for creating branching logic between data sources etc.
Recipe | Description |
---|---|
/semantic-router/00_semantic_routing.ipynb | Simple examples of how to build an allow/block list router in addition to a multi-topic router |
Recipe | Description |
---|---|
/agents/00_langgraph_redis_agentic_rag.ipynb | Notebook to get started with lang-graph and agents |
/agents/01_crewai_langgraph_redis.ipynb | Notebook to get started with lang-graph and agents |
/agents/02_full_featured_agent.ipynb | Notebook builds full tool calling agent with semantic cache and router |
Recipe | Description |
---|---|
/computer-vision/00_facial_recognition_facenet.ipynb | Build a facial recognition system using the Facenet embedding model and RedisVL. |
Recipe | Description |
---|---|
/recommendation-systems/00_content_filtering.ipynb | Intro content filtering example with redisvl |
/recommendation-systems/01_collaborative_filtering.ipynb | Intro collaborative filtering example with redisvl |
Recipe | Description |
---|---|
/feature-store/00_feast_credit_score.ipynb | Credit scoring system using Feast with Redis as the online store. |
Need a deeper-dive through different use cases and topics?
Tutorial | Description |
---|---|
Agentic RAG | A tutorial focused on agentic RAG with LlamaIndex and Cohere |
RAG on VertexAI | A RAG tutorial featuring Redis with Vertex AI |
Recommendation Systems w/ NVIDIA Merlin & Redis | Three examples, each escalating in complexity, showcasing the process of building a realtime recsys with NVIDIA and Redis |
Redis integrates with many different players in the AI ecosystem. Here's a curated list below:
Integration | Description |
---|---|
RedisVL | A dedicated Python client lib for Redis as a Vector DB |
AWS Bedrock | Streamlines GenAI deployment by offering foundational models as a unified API |
LangChain Python | Popular Python client lib for building LLM applications powered by Redis |
LangChain JS | Popular JS client lib for building LLM applications powered by Redis |
LlamaIndex | LlamaIndex Integration for Redis as a vector Database (formerly GPT-index) |
LiteLLM | Popular LLM proxy layer to help manage and streamline usage of multiple foundation models |
Semantic Kernel | Popular lib by MSFT to integrate LLMs with plugins |
RelevanceAI | Platform to tag, search and analyze unstructured data faster, built on Redis |
DocArray | DocArray Integration of Redis as a VectorDB by Jina AI |
- Vector Databases and Large Language Models - Talk given at LLMs in Production Part 1 by Sam Partee.
- Level-up RAG with RedisVL
- Improving RAG quality with RAGAs
- Vector Databases and AI-powered Search Talk - Video "Vector Databases and AI-powered Search" given by Sam Partee at SDSC 2023.
- NVIDIA RecSys with Redis
- Benchmarking results for vector databases - Benchmarking results for vector databases, including Redis and 7 other Vector Database players.
- Redis Vector Library Docs
- Redis Vector Search API Docs - Official Redis literature for Vector Similarity Search.
For Tasks:
Click tags to check more tools for each tasksFor Jobs:
Alternative AI tools for redis-ai-resources
Similar Open Source Tools
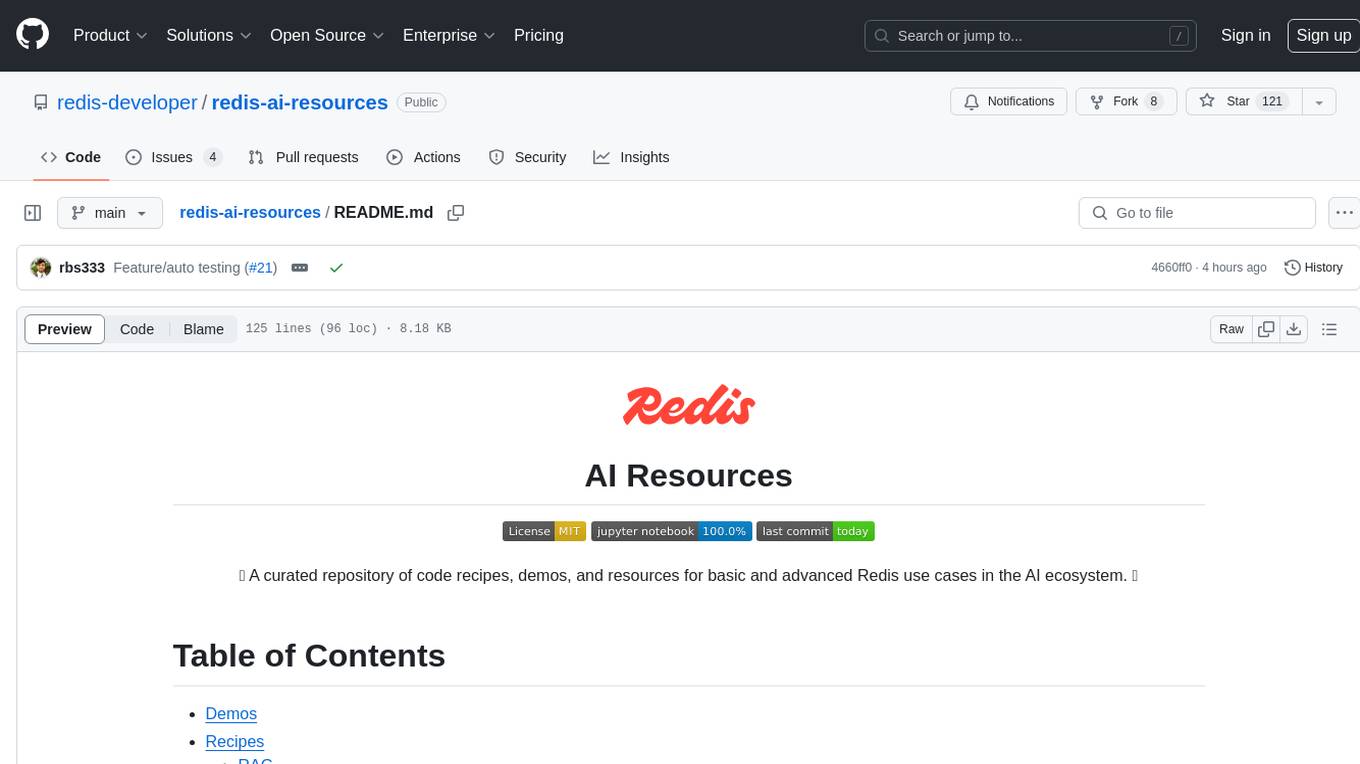
redis-ai-resources
A curated repository of code recipes, demos, and resources for basic and advanced Redis use cases in the AI ecosystem. It includes demos for ArxivChatGuru, Redis VSS, Vertex AI & Redis, Agentic RAG, ArXiv Search, and Product Search. Recipes cover topics like Getting started with RAG, Semantic Cache, Advanced RAG, and Recommendation systems. The repository also provides integrations/tools like RedisVL, AWS Bedrock, LangChain Python, LangChain JS, LlamaIndex, Semantic Kernel, RelevanceAI, and DocArray. Additional content includes blog posts, talks, reviews, and documentation related to Vector Similarity Search, AI-Powered Document Search, Vector Databases, Real-Time Product Recommendations, and more. Benchmarks compare Redis against other Vector Databases and ANN benchmarks. Documentation includes QuickStart guides, official literature for Vector Similarity Search, Redis-py client library docs, Redis Stack documentation, and Redis client list.
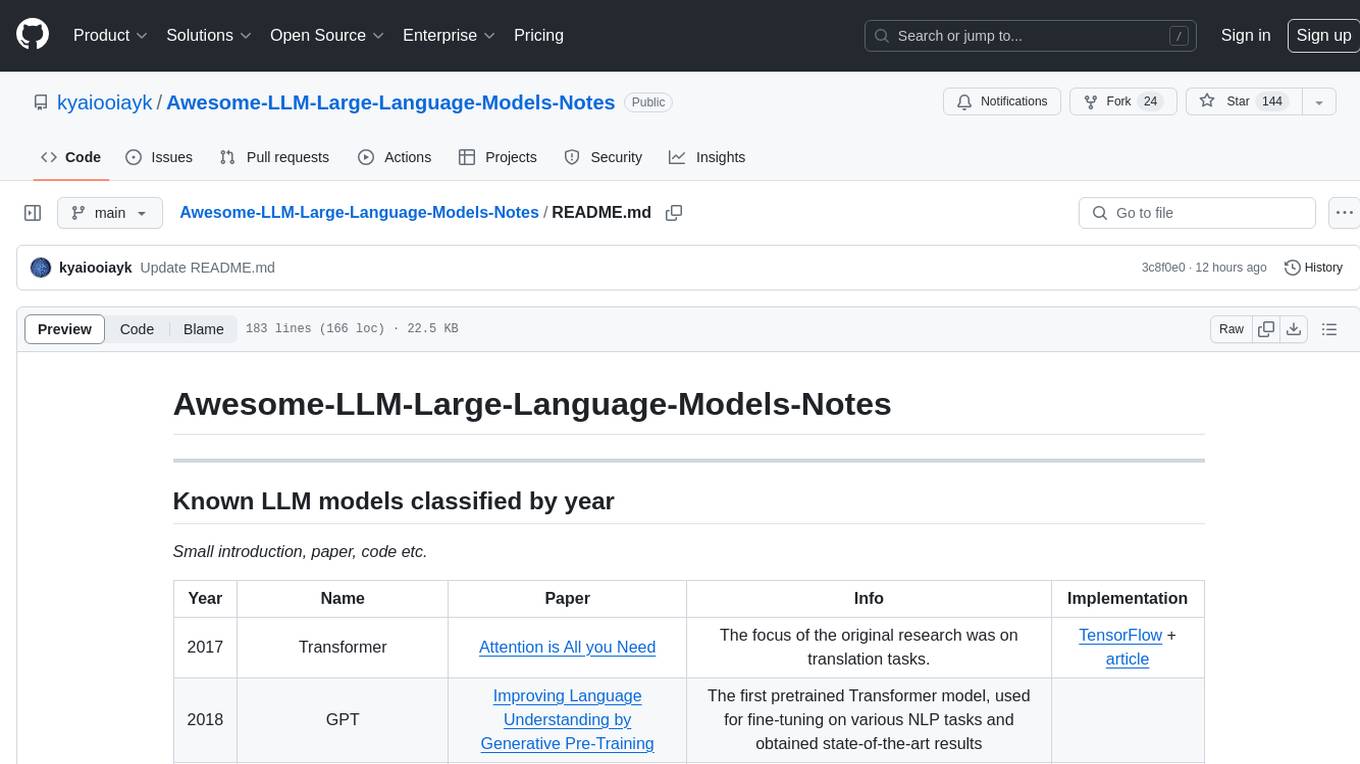
Awesome-LLM-Large-Language-Models-Notes
Awesome-LLM-Large-Language-Models-Notes is a repository that provides a comprehensive collection of information on various Large Language Models (LLMs) classified by year, size, and name. It includes details on known LLM models, their papers, implementations, and specific characteristics. The repository also covers LLM models classified by architecture, must-read papers, blog articles, tutorials, and implementations from scratch. It serves as a valuable resource for individuals interested in understanding and working with LLMs in the field of Natural Language Processing (NLP).
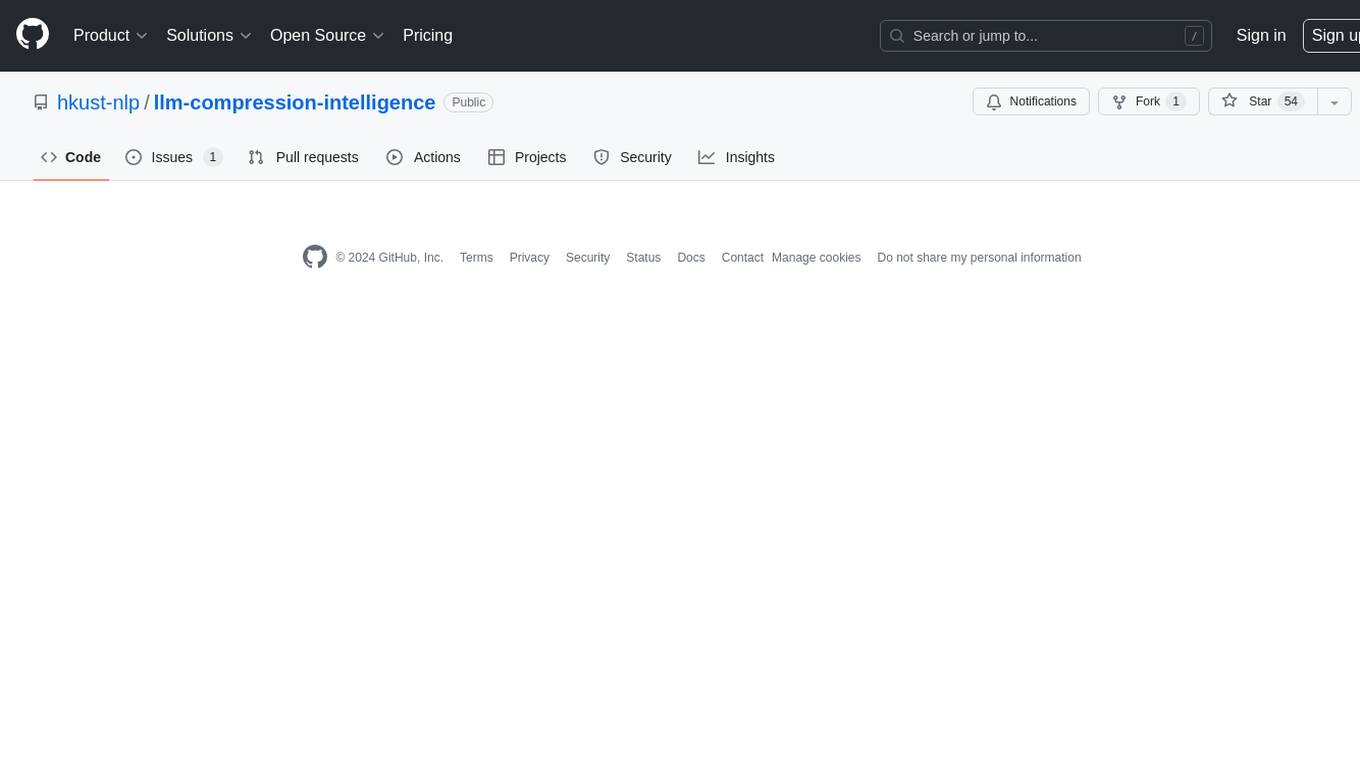
llm-compression-intelligence
This repository presents the findings of the paper "Compression Represents Intelligence Linearly". The study reveals a strong linear correlation between the intelligence of LLMs, as measured by benchmark scores, and their ability to compress external text corpora. Compression efficiency, derived from raw text corpora, serves as a reliable evaluation metric that is linearly associated with model capabilities. The repository includes the compression corpora used in the paper, code for computing compression efficiency, and data collection and processing pipelines.
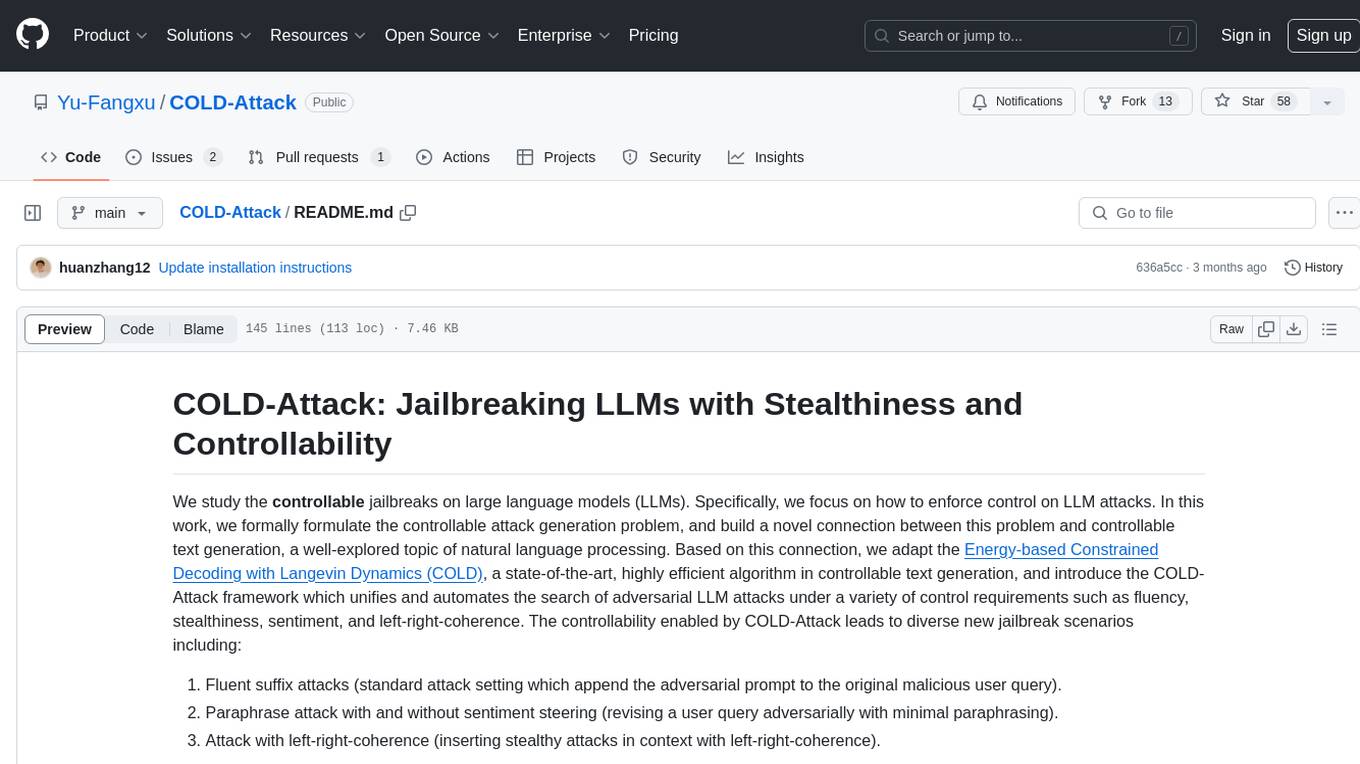
COLD-Attack
COLD-Attack is a framework designed for controllable jailbreaks on large language models (LLMs). It formulates the controllable attack generation problem and utilizes the Energy-based Constrained Decoding with Langevin Dynamics (COLD) algorithm to automate the search of adversarial LLM attacks with control over fluency, stealthiness, sentiment, and left-right-coherence. The framework includes steps for energy function formulation, Langevin dynamics sampling, and decoding process to generate discrete text attacks. It offers diverse jailbreak scenarios such as fluent suffix attacks, paraphrase attacks, and attacks with left-right-coherence.
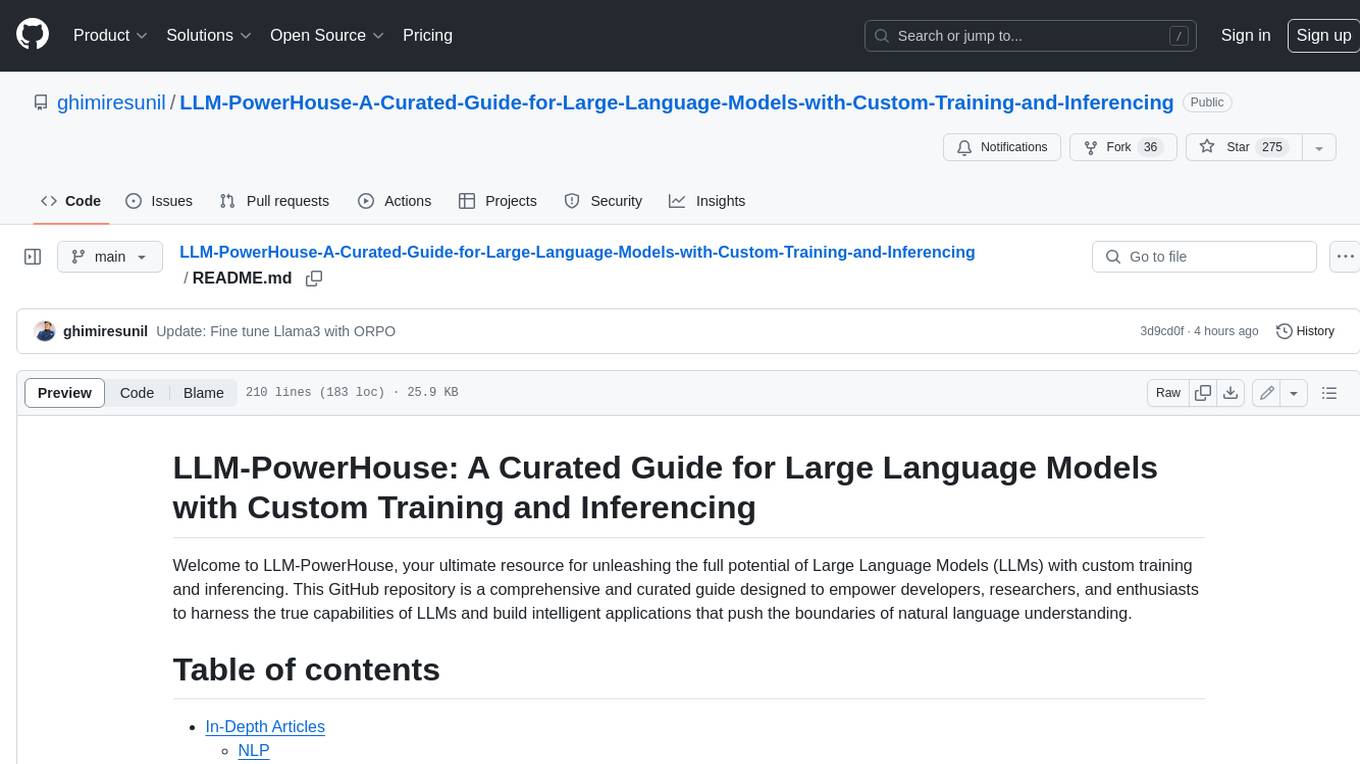
LLM-PowerHouse-A-Curated-Guide-for-Large-Language-Models-with-Custom-Training-and-Inferencing
LLM-PowerHouse is a comprehensive and curated guide designed to empower developers, researchers, and enthusiasts to harness the true capabilities of Large Language Models (LLMs) and build intelligent applications that push the boundaries of natural language understanding. This GitHub repository provides in-depth articles, codebase mastery, LLM PlayLab, and resources for cost analysis and network visualization. It covers various aspects of LLMs, including NLP, models, training, evaluation metrics, open LLMs, and more. The repository also includes a collection of code examples and tutorials to help users build and deploy LLM-based applications.
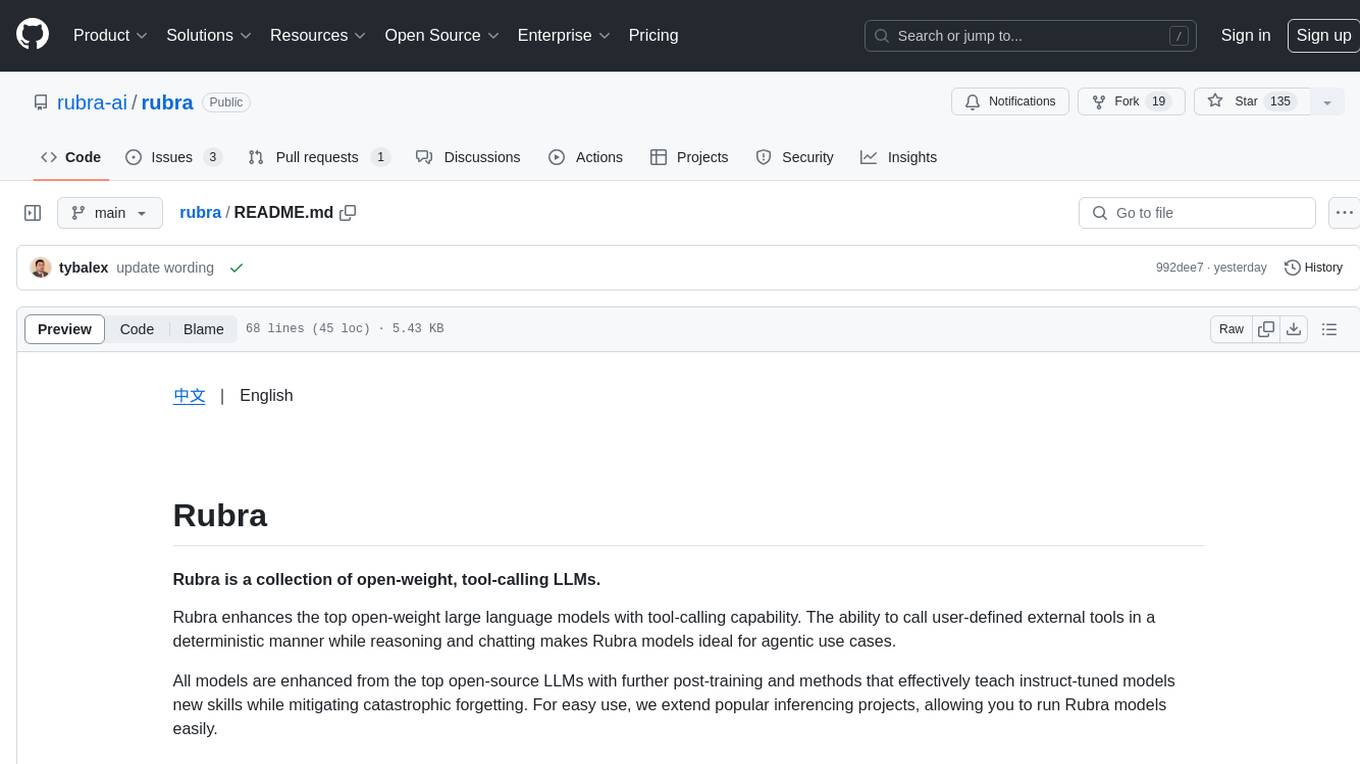
rubra
Rubra is a collection of open-weight large language models enhanced with tool-calling capability. It allows users to call user-defined external tools in a deterministic manner while reasoning and chatting, making it ideal for agentic use cases. The models are further post-trained to teach instruct-tuned models new skills and mitigate catastrophic forgetting. Rubra extends popular inferencing projects for easy use, enabling users to run the models easily.
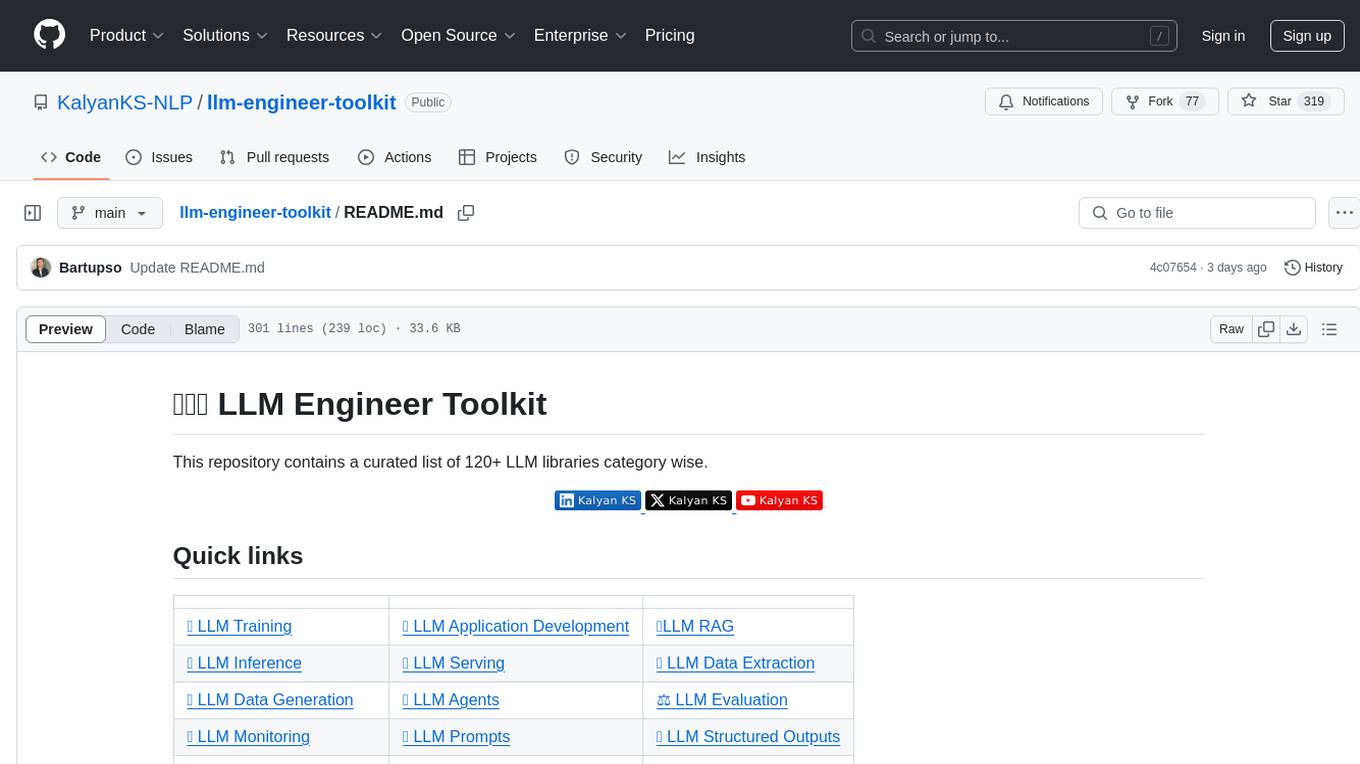
llm-engineer-toolkit
The LLM Engineer Toolkit is a curated repository containing over 120 LLM libraries categorized for various tasks such as training, application development, inference, serving, data extraction, data generation, agents, evaluation, monitoring, prompts, structured outputs, safety, security, embedding models, and other miscellaneous tools. It includes libraries for fine-tuning LLMs, building applications powered by LLMs, serving LLM models, extracting data, generating synthetic data, creating AI agents, evaluating LLM applications, monitoring LLM performance, optimizing prompts, handling structured outputs, ensuring safety and security, embedding models, and more. The toolkit covers a wide range of tools and frameworks to streamline the development, deployment, and optimization of large language models.
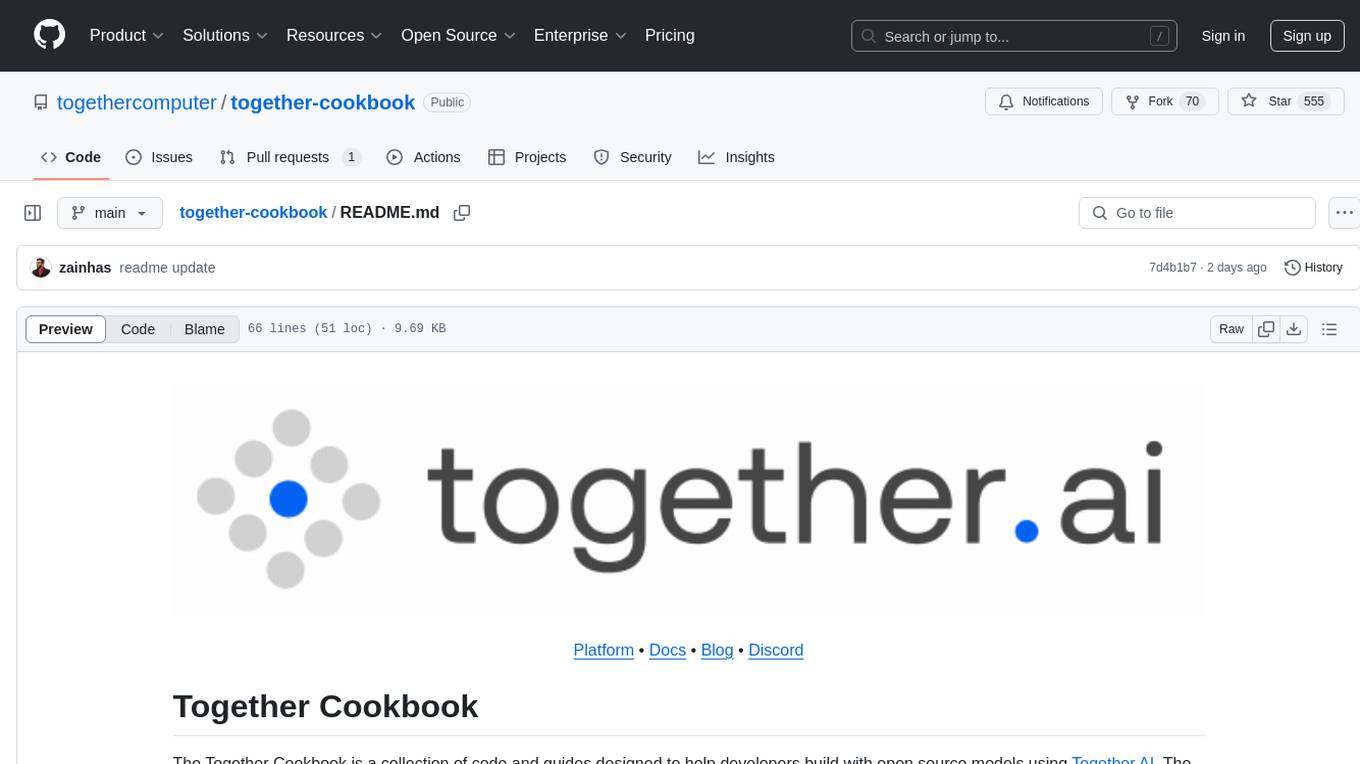
together-cookbook
The Together Cookbook is a collection of code and guides designed to help developers build with open source models using Together AI. The recipes provide examples on how to chain multiple LLM calls, create agents that route tasks to specialized models, run multiple LLMs in parallel, break down tasks into parallel subtasks, build agents that iteratively improve responses, perform LoRA fine-tuning and inference, fine-tune LLMs for repetition, improve summarization capabilities, fine-tune LLMs on multi-step conversations, implement retrieval-augmented generation, conduct multimodal search and conditional image generation, visualize vector embeddings, improve search results with rerankers, implement vector search with embedding models, extract structured text from images, summarize and evaluate outputs with LLMs, generate podcasts from PDF content, and get LLMs to generate knowledge graphs.
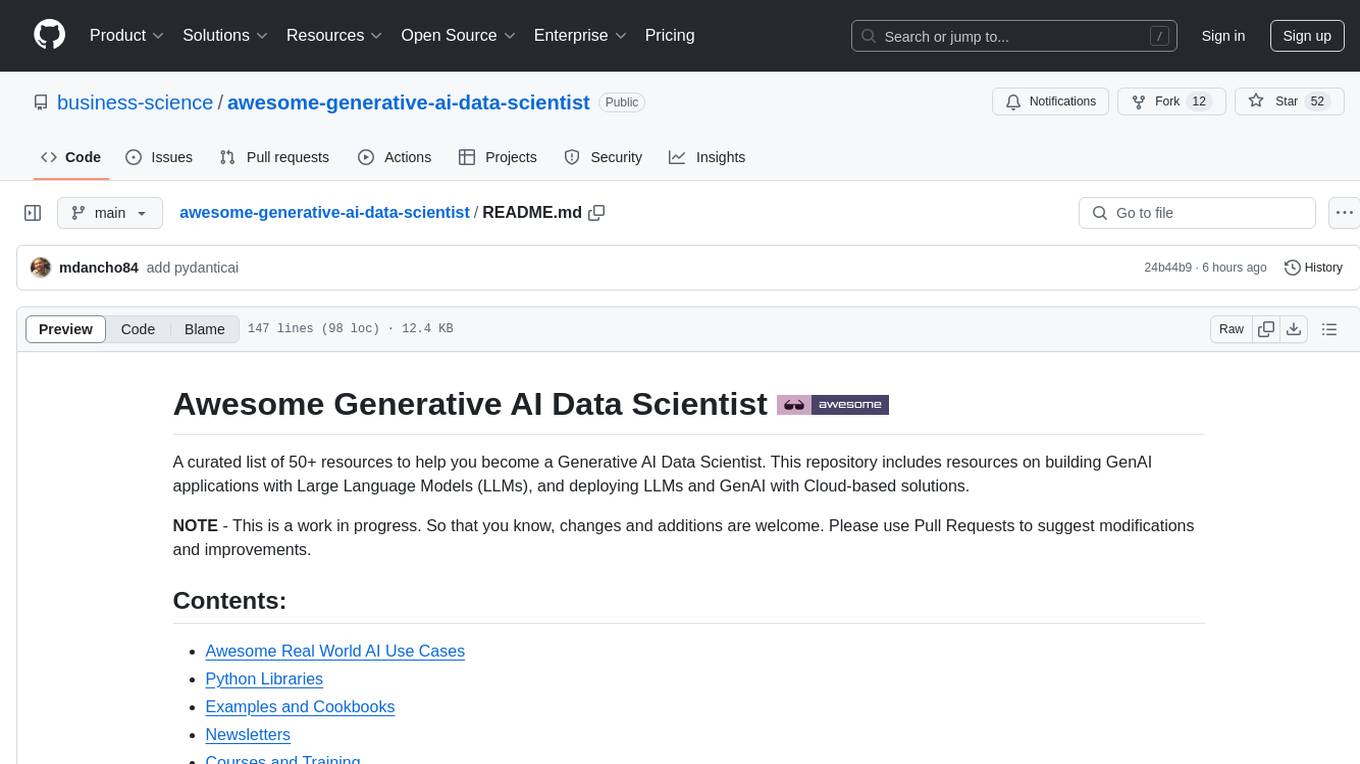
awesome-generative-ai-data-scientist
A curated list of 50+ resources to help you become a Generative AI Data Scientist. This repository includes resources on building GenAI applications with Large Language Models (LLMs), and deploying LLMs and GenAI with Cloud-based solutions.
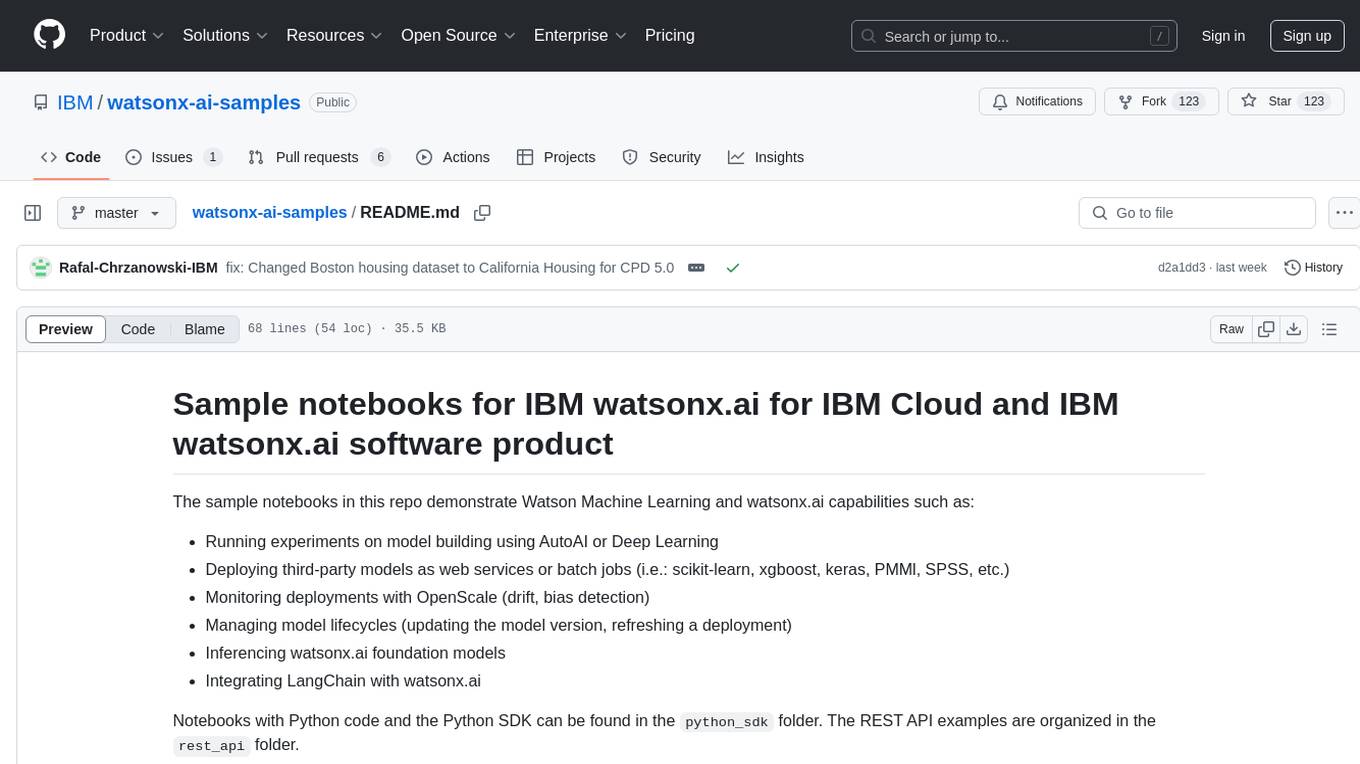
watsonx-ai-samples
Sample notebooks for IBM Watsonx.ai for IBM Cloud and IBM Watsonx.ai software product. The notebooks demonstrate capabilities such as running experiments on model building using AutoAI or Deep Learning, deploying third-party models as web services or batch jobs, monitoring deployments with OpenScale, managing model lifecycles, inferencing Watsonx.ai foundation models, and integrating LangChain with Watsonx.ai. Notebooks with Python code and the Python SDK can be found in the `python_sdk` folder. The REST API examples are organized in the `rest_api` folder.
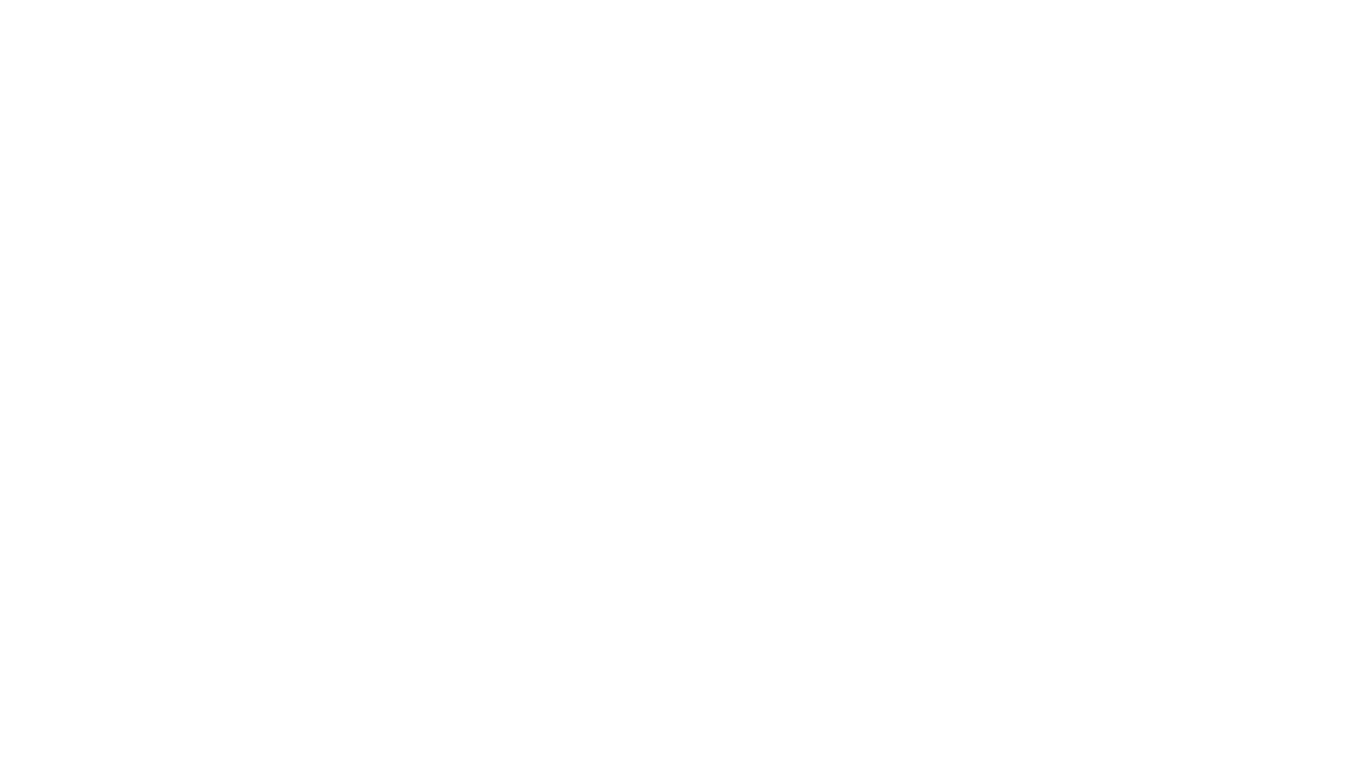
e2b-cookbook
E2B Cookbook provides example code and guides for building with E2B. E2B is a platform that allows developers to build custom code interpreters in their AI apps. It provides a dedicated SDK for building custom code interpreters, as well as a core SDK that can be used to build on top of E2B. E2B also provides documentation at e2b.dev/docs.
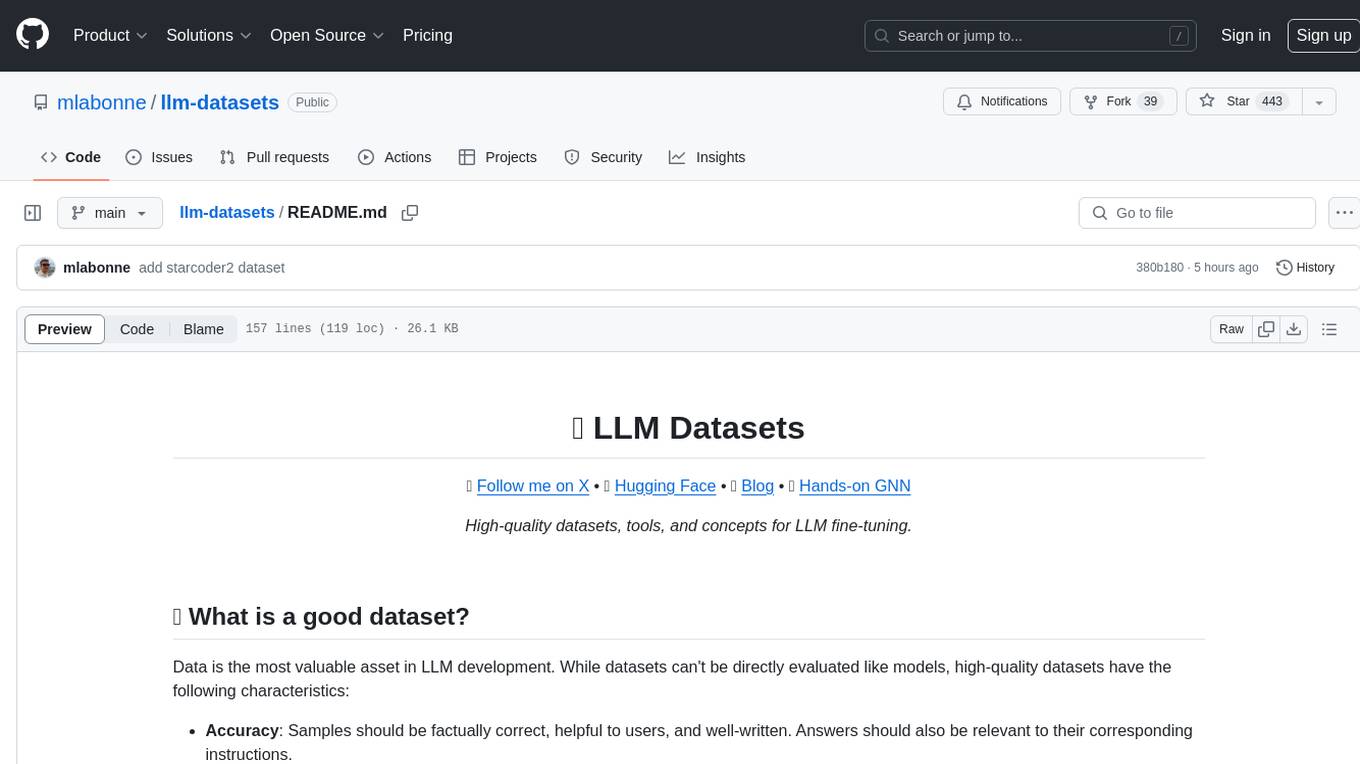
llm-datasets
LLM Datasets is a repository containing high-quality datasets, tools, and concepts for LLM fine-tuning. It provides datasets with characteristics like accuracy, diversity, and complexity to train large language models for various tasks. The repository includes datasets for general-purpose, math & logic, code, conversation & role-play, and agent & function calling domains. It also offers guidance on creating high-quality datasets through data deduplication, data quality assessment, data exploration, and data generation techniques.
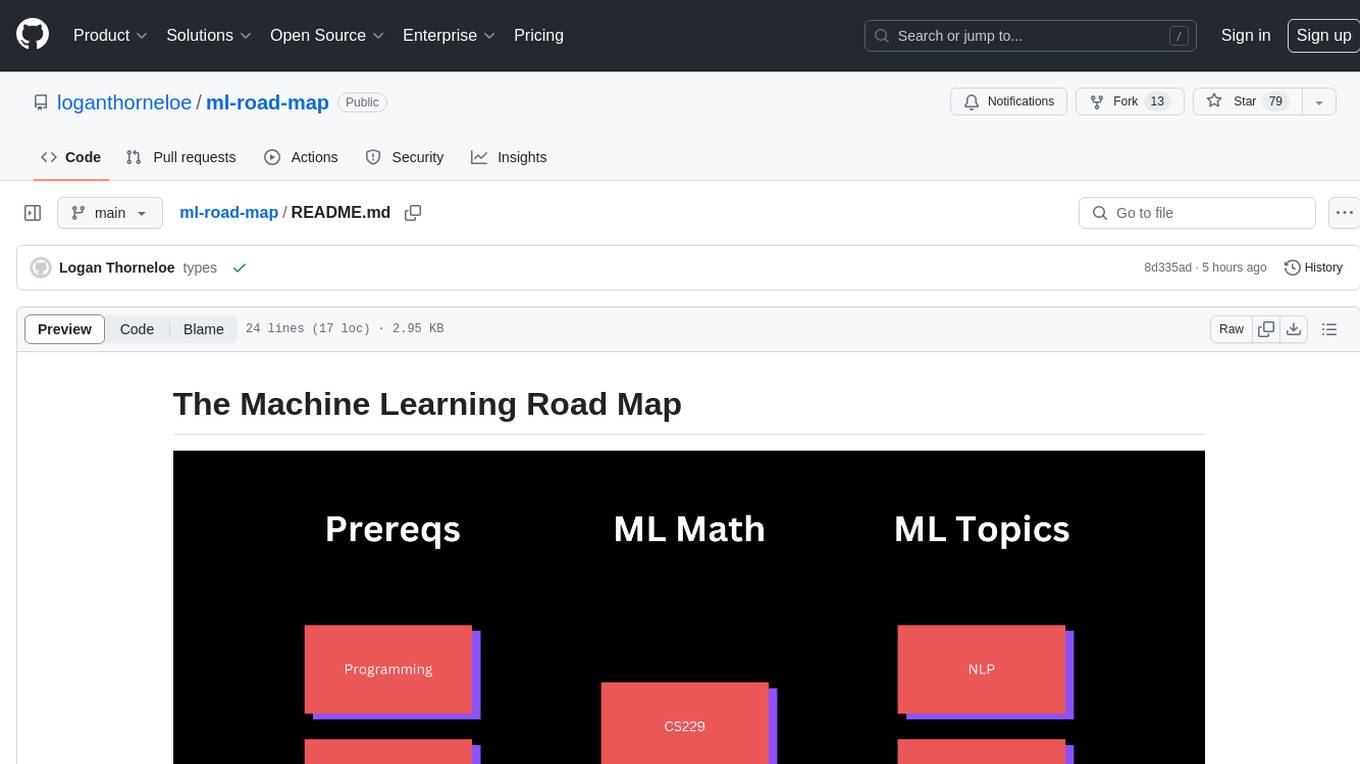
ml-road-map
The Machine Learning Road Map is a comprehensive guide designed to take individuals from various levels of machine learning knowledge to a basic understanding of machine learning principles using high-quality, free resources. It aims to simplify the complex and rapidly growing field of machine learning by providing a structured roadmap for learning. The guide emphasizes the importance of understanding AI for everyone, the need for patience in learning machine learning due to its complexity, and the value of learning from experts in the field. It covers five different paths to learning about machine learning, catering to consumers, aspiring AI researchers, ML engineers, developers interested in building ML applications, and companies looking to implement AI solutions.
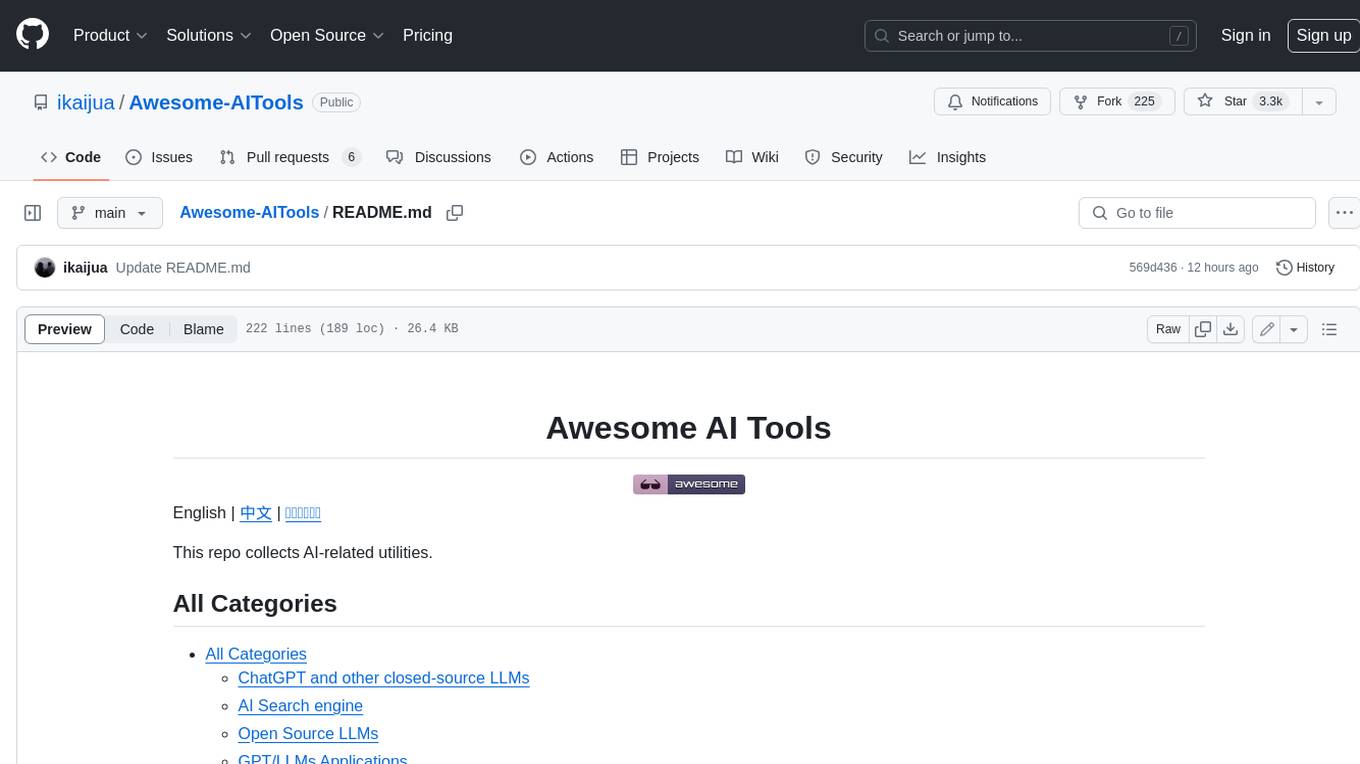
Awesome-AITools
This repo collects AI-related utilities. ## All Categories * All Categories * ChatGPT and other closed-source LLMs * AI Search engine * Open Source LLMs * GPT/LLMs Applications * LLM training platform * Applications that integrate multiple LLMs * AI Agent * Writing * Programming Development * Translation * AI Conversation or AI Voice Conversation * Image Creation * Speech Recognition * Text To Speech * Voice Processing * AI generated music or sound effects * Speech translation * Video Creation * Video Content Summary * OCR(Optical Character Recognition)
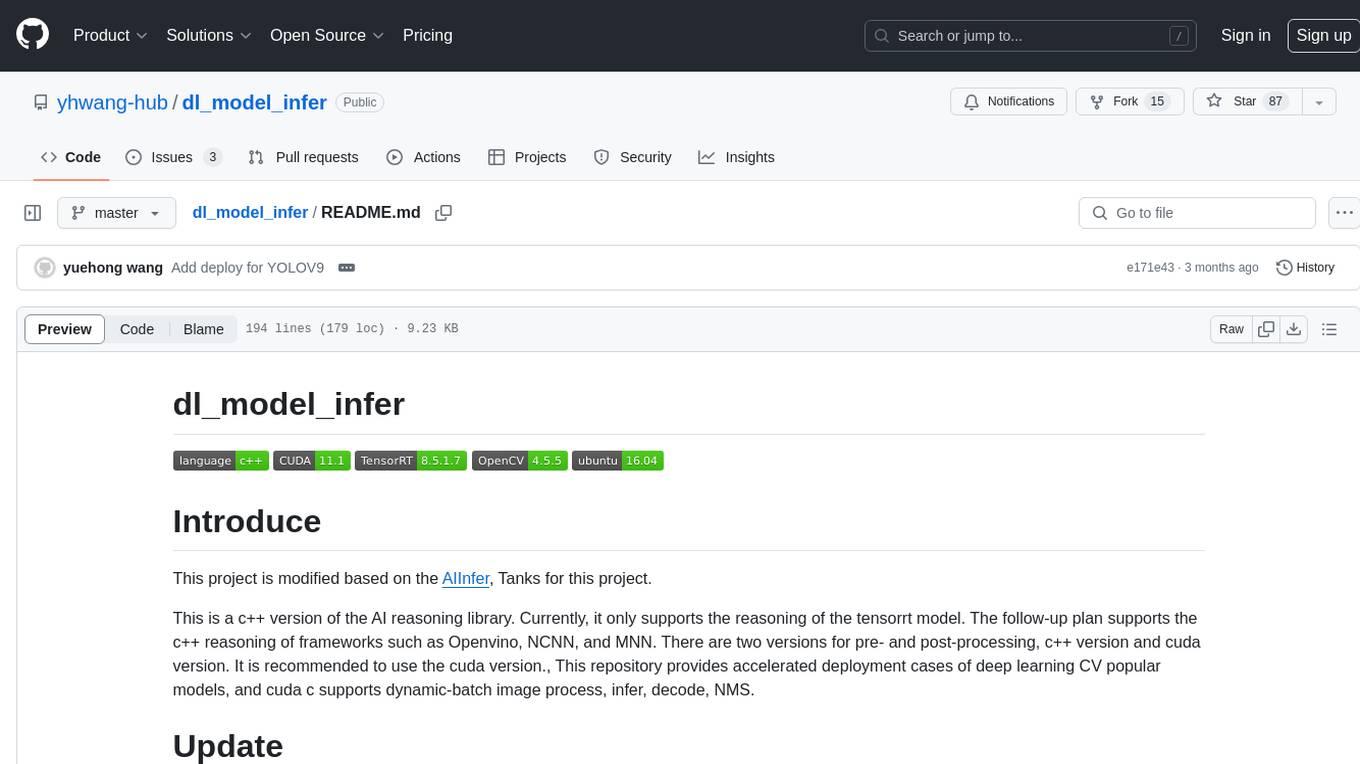
dl_model_infer
This project is a c++ version of the AI reasoning library that supports the reasoning of tensorrt models. It provides accelerated deployment cases of deep learning CV popular models and supports dynamic-batch image processing, inference, decode, and NMS. The project has been updated with various models and provides tutorials for model exports. It also includes a producer-consumer inference model for specific tasks. The project directory includes implementations for model inference applications, backend reasoning classes, post-processing, pre-processing, and target detection and tracking. Speed tests have been conducted on various models, and onnx downloads are available for different models.
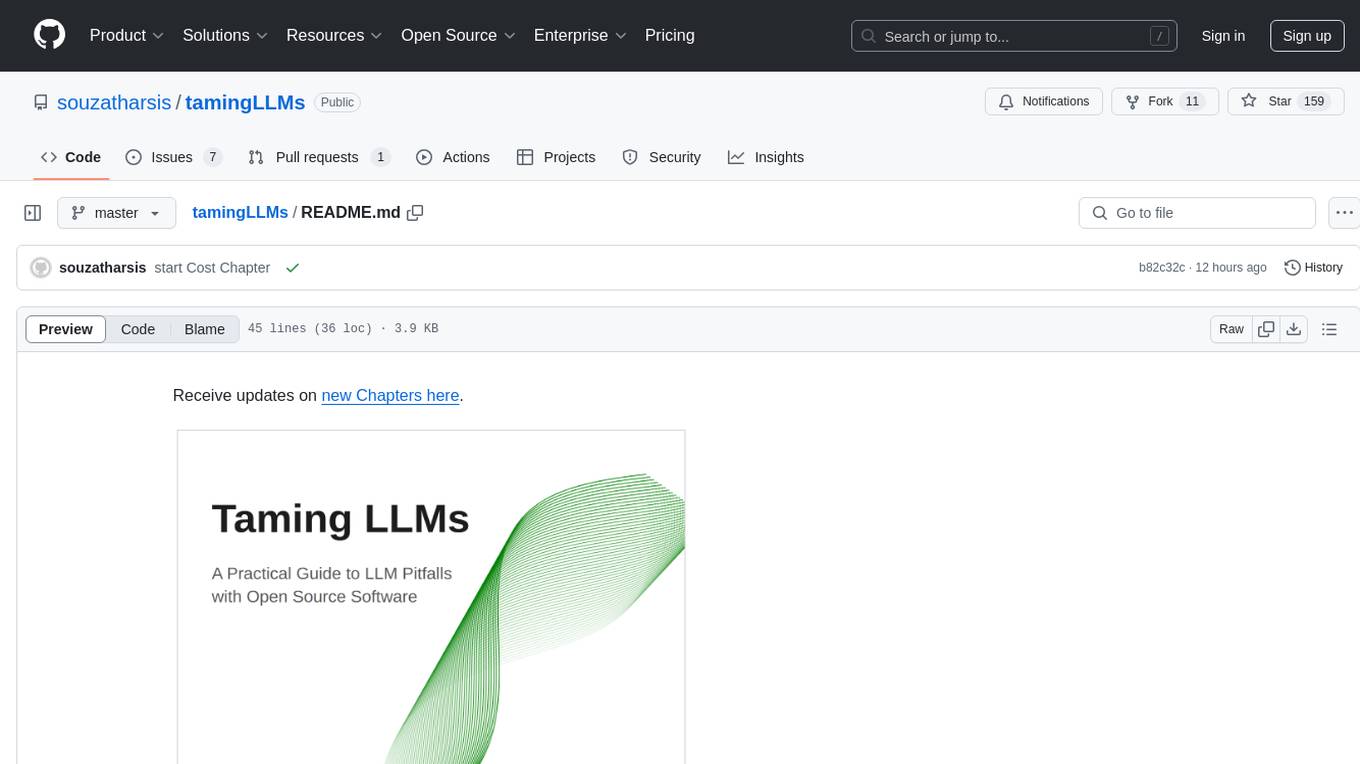
tamingLLMs
The 'Taming LLMs' repository provides a practical guide to the pitfalls and challenges associated with Large Language Models (LLMs) when building applications. It focuses on key limitations and implementation pitfalls, offering practical Python examples and open source solutions to help engineers and technical leaders navigate these challenges. The repository aims to equip readers with the knowledge to harness the power of LLMs while avoiding their inherent limitations.
For similar tasks
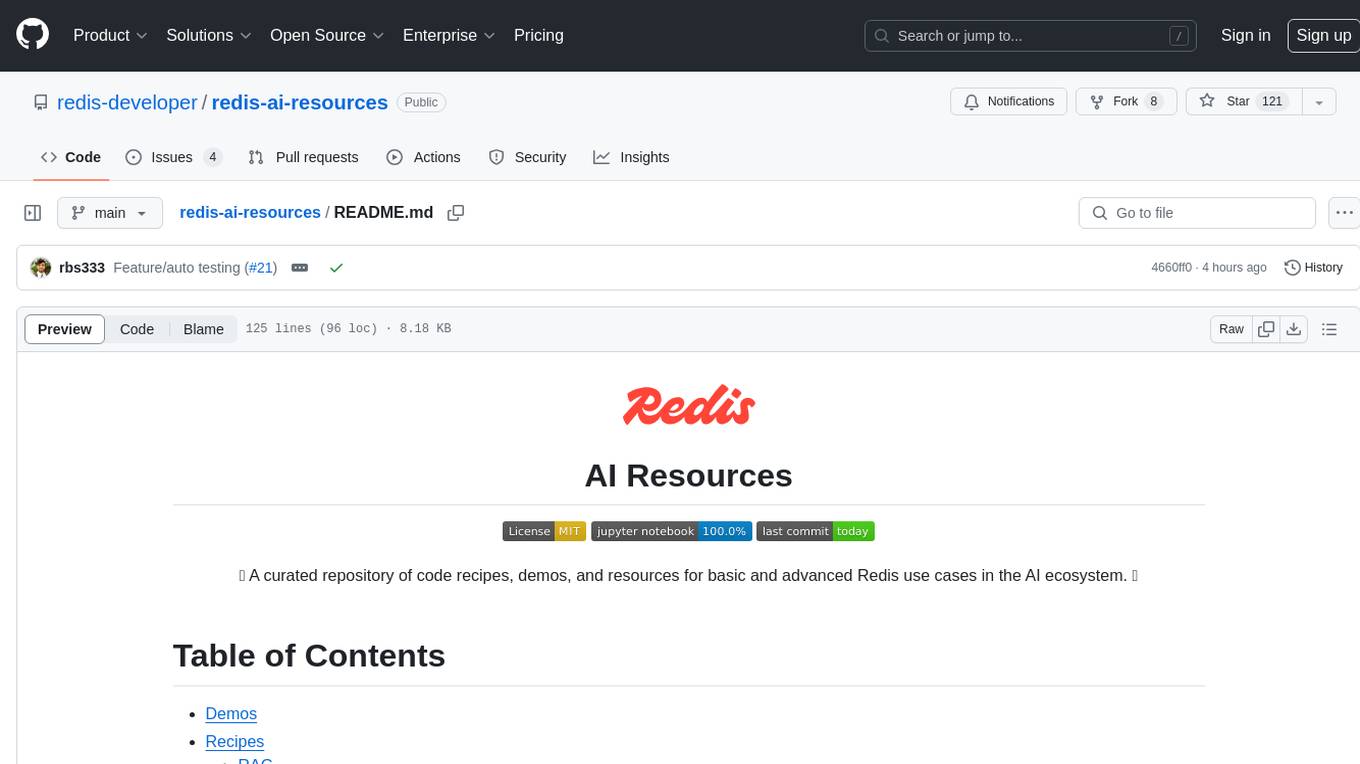
redis-ai-resources
A curated repository of code recipes, demos, and resources for basic and advanced Redis use cases in the AI ecosystem. It includes demos for ArxivChatGuru, Redis VSS, Vertex AI & Redis, Agentic RAG, ArXiv Search, and Product Search. Recipes cover topics like Getting started with RAG, Semantic Cache, Advanced RAG, and Recommendation systems. The repository also provides integrations/tools like RedisVL, AWS Bedrock, LangChain Python, LangChain JS, LlamaIndex, Semantic Kernel, RelevanceAI, and DocArray. Additional content includes blog posts, talks, reviews, and documentation related to Vector Similarity Search, AI-Powered Document Search, Vector Databases, Real-Time Product Recommendations, and more. Benchmarks compare Redis against other Vector Databases and ANN benchmarks. Documentation includes QuickStart guides, official literature for Vector Similarity Search, Redis-py client library docs, Redis Stack documentation, and Redis client list.
For similar jobs
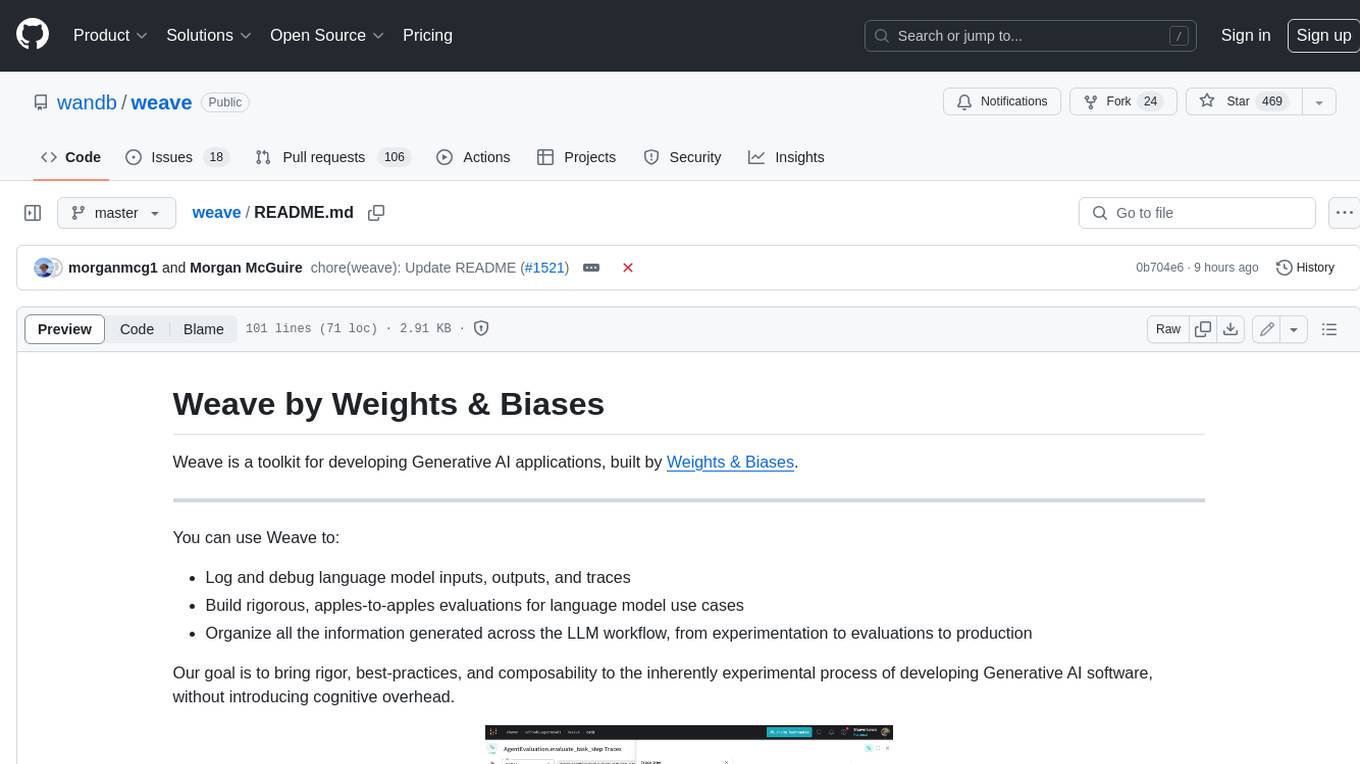
weave
Weave is a toolkit for developing Generative AI applications, built by Weights & Biases. With Weave, you can log and debug language model inputs, outputs, and traces; build rigorous, apples-to-apples evaluations for language model use cases; and organize all the information generated across the LLM workflow, from experimentation to evaluations to production. Weave aims to bring rigor, best-practices, and composability to the inherently experimental process of developing Generative AI software, without introducing cognitive overhead.
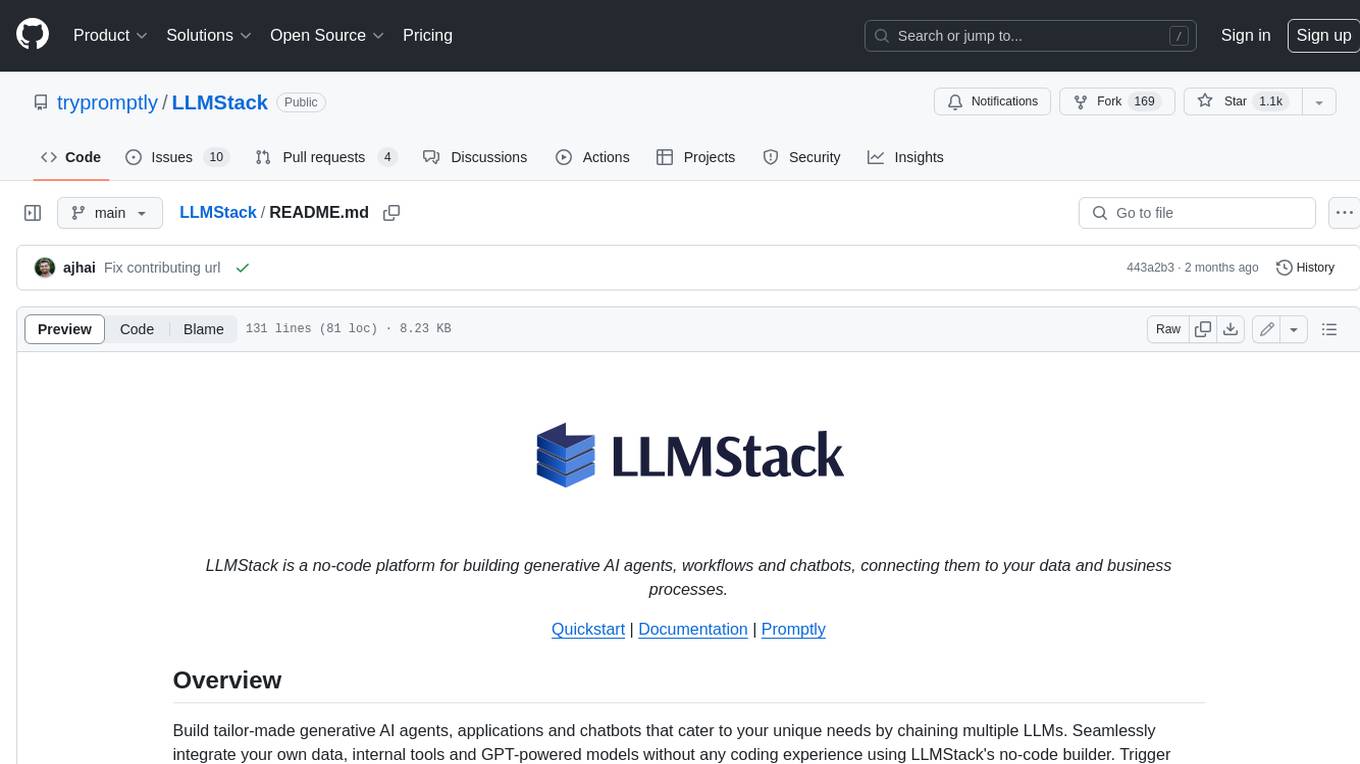
LLMStack
LLMStack is a no-code platform for building generative AI agents, workflows, and chatbots. It allows users to connect their own data, internal tools, and GPT-powered models without any coding experience. LLMStack can be deployed to the cloud or on-premise and can be accessed via HTTP API or triggered from Slack or Discord.
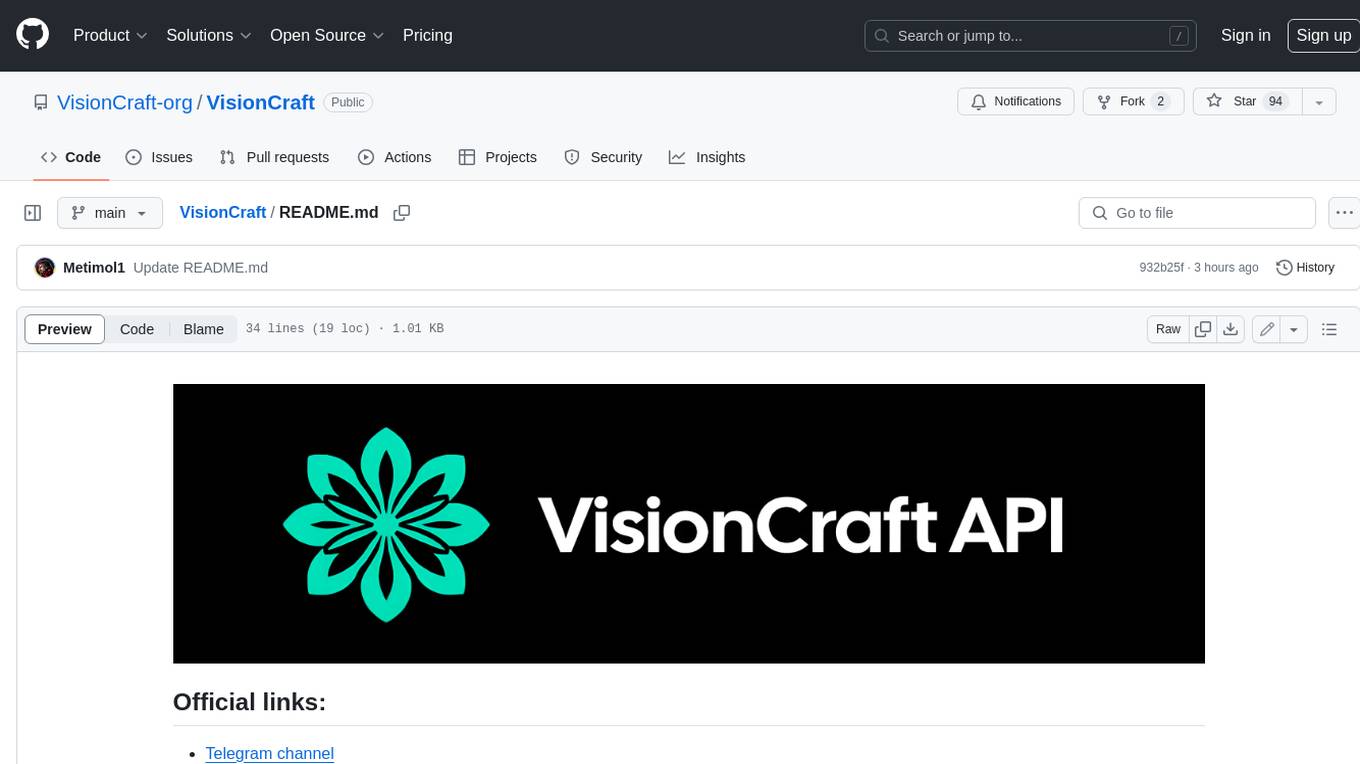
VisionCraft
The VisionCraft API is a free API for using over 100 different AI models. From images to sound.
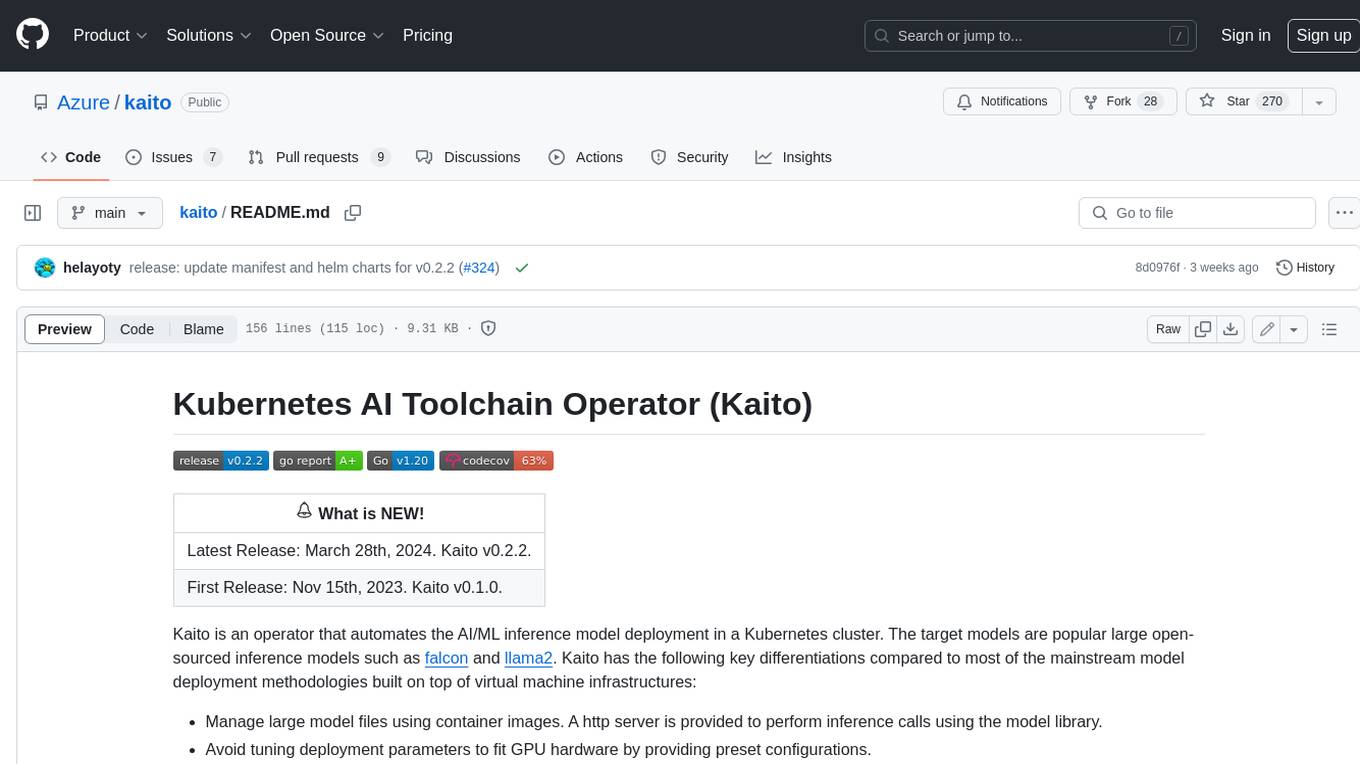
kaito
Kaito is an operator that automates the AI/ML inference model deployment in a Kubernetes cluster. It manages large model files using container images, avoids tuning deployment parameters to fit GPU hardware by providing preset configurations, auto-provisions GPU nodes based on model requirements, and hosts large model images in the public Microsoft Container Registry (MCR) if the license allows. Using Kaito, the workflow of onboarding large AI inference models in Kubernetes is largely simplified.
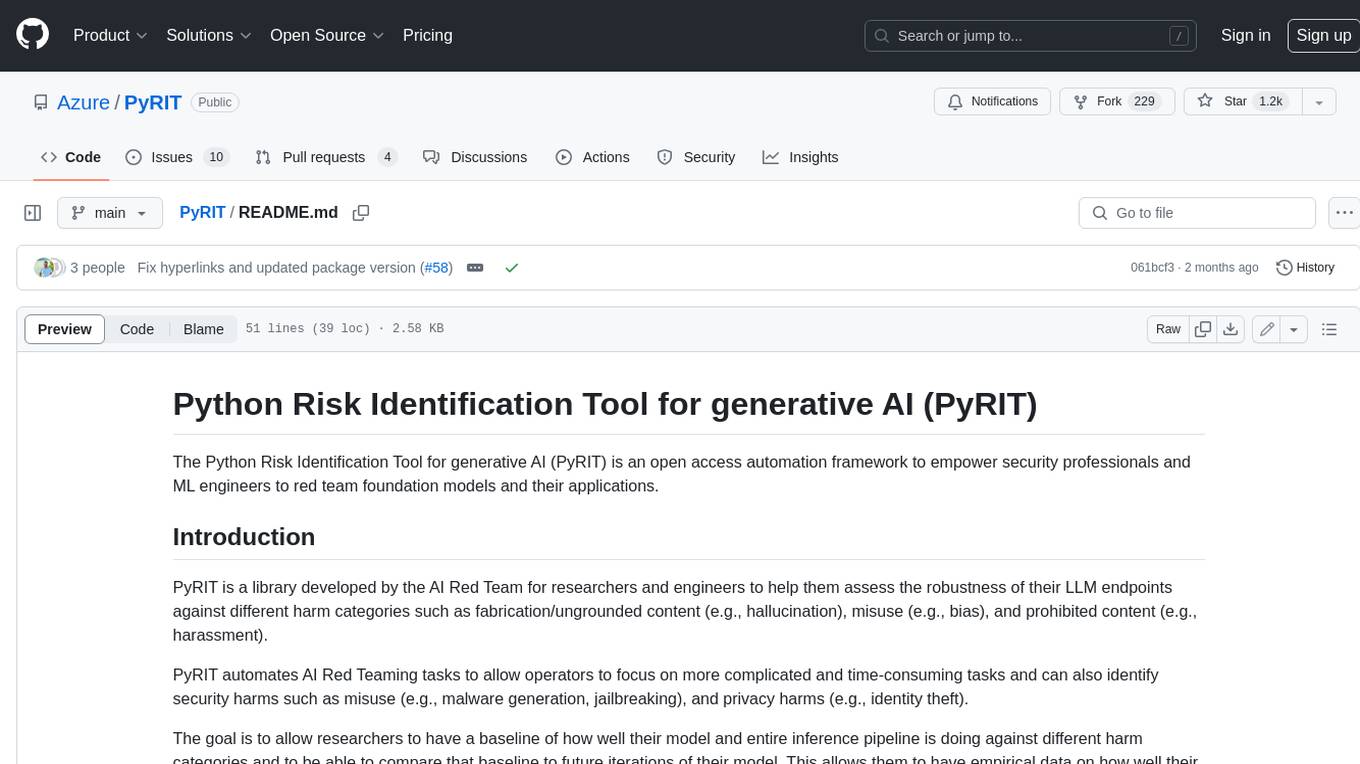
PyRIT
PyRIT is an open access automation framework designed to empower security professionals and ML engineers to red team foundation models and their applications. It automates AI Red Teaming tasks to allow operators to focus on more complicated and time-consuming tasks and can also identify security harms such as misuse (e.g., malware generation, jailbreaking), and privacy harms (e.g., identity theft). The goal is to allow researchers to have a baseline of how well their model and entire inference pipeline is doing against different harm categories and to be able to compare that baseline to future iterations of their model. This allows them to have empirical data on how well their model is doing today, and detect any degradation of performance based on future improvements.
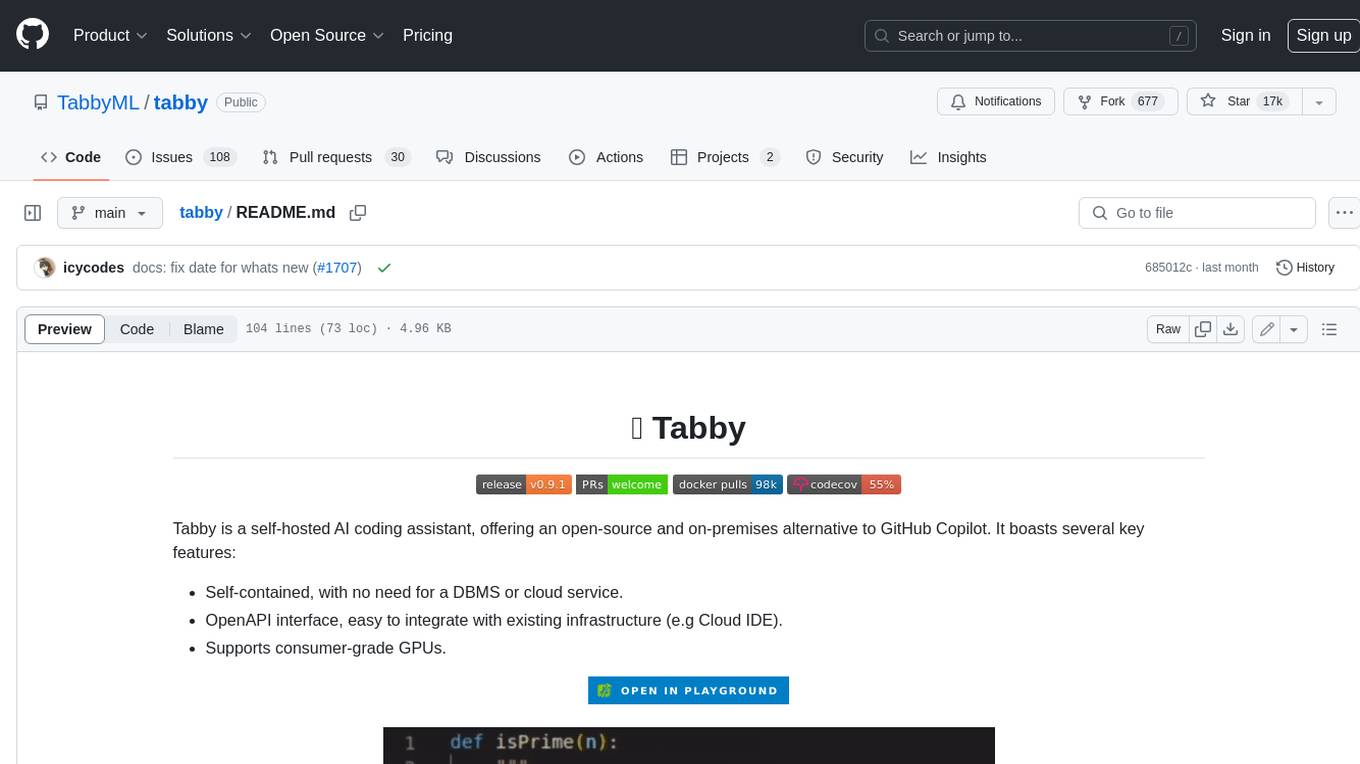
tabby
Tabby is a self-hosted AI coding assistant, offering an open-source and on-premises alternative to GitHub Copilot. It boasts several key features: * Self-contained, with no need for a DBMS or cloud service. * OpenAPI interface, easy to integrate with existing infrastructure (e.g Cloud IDE). * Supports consumer-grade GPUs.
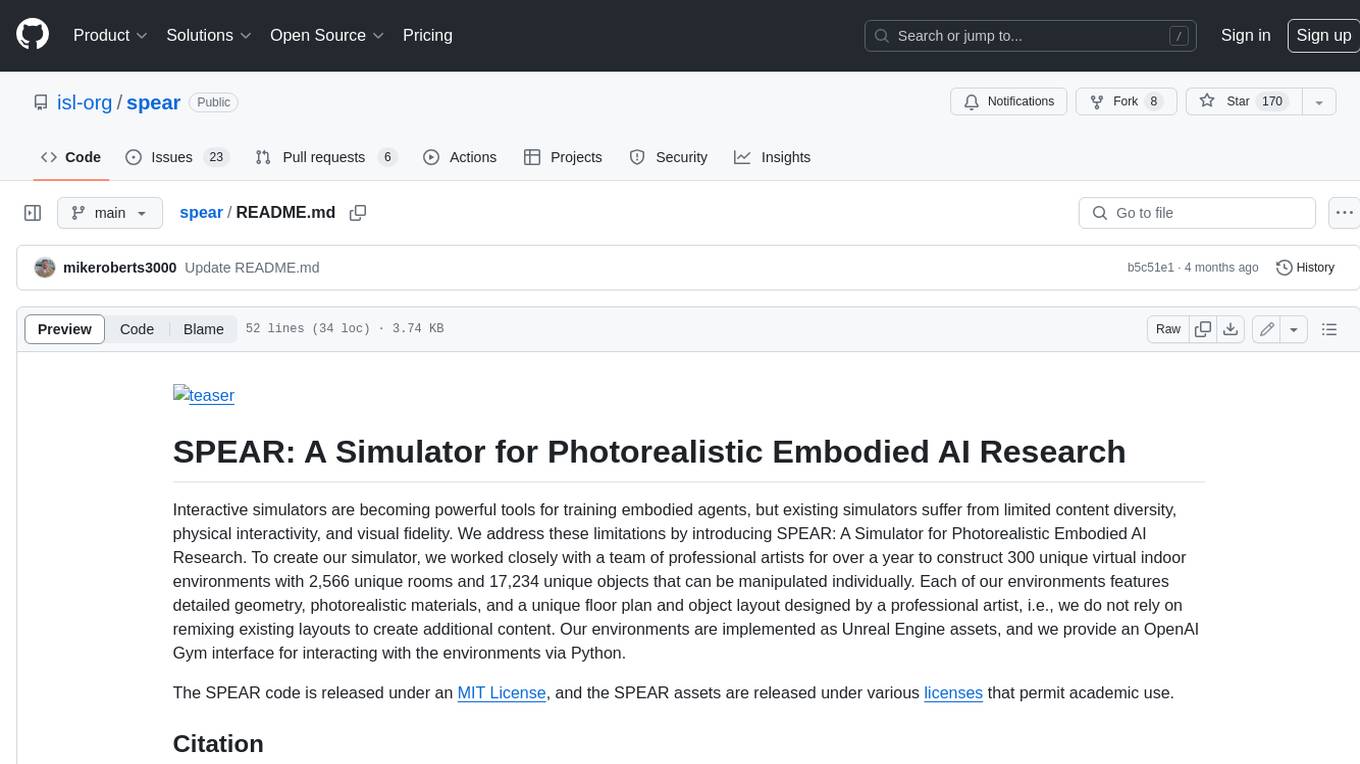
spear
SPEAR (Simulator for Photorealistic Embodied AI Research) is a powerful tool for training embodied agents. It features 300 unique virtual indoor environments with 2,566 unique rooms and 17,234 unique objects that can be manipulated individually. Each environment is designed by a professional artist and features detailed geometry, photorealistic materials, and a unique floor plan and object layout. SPEAR is implemented as Unreal Engine assets and provides an OpenAI Gym interface for interacting with the environments via Python.
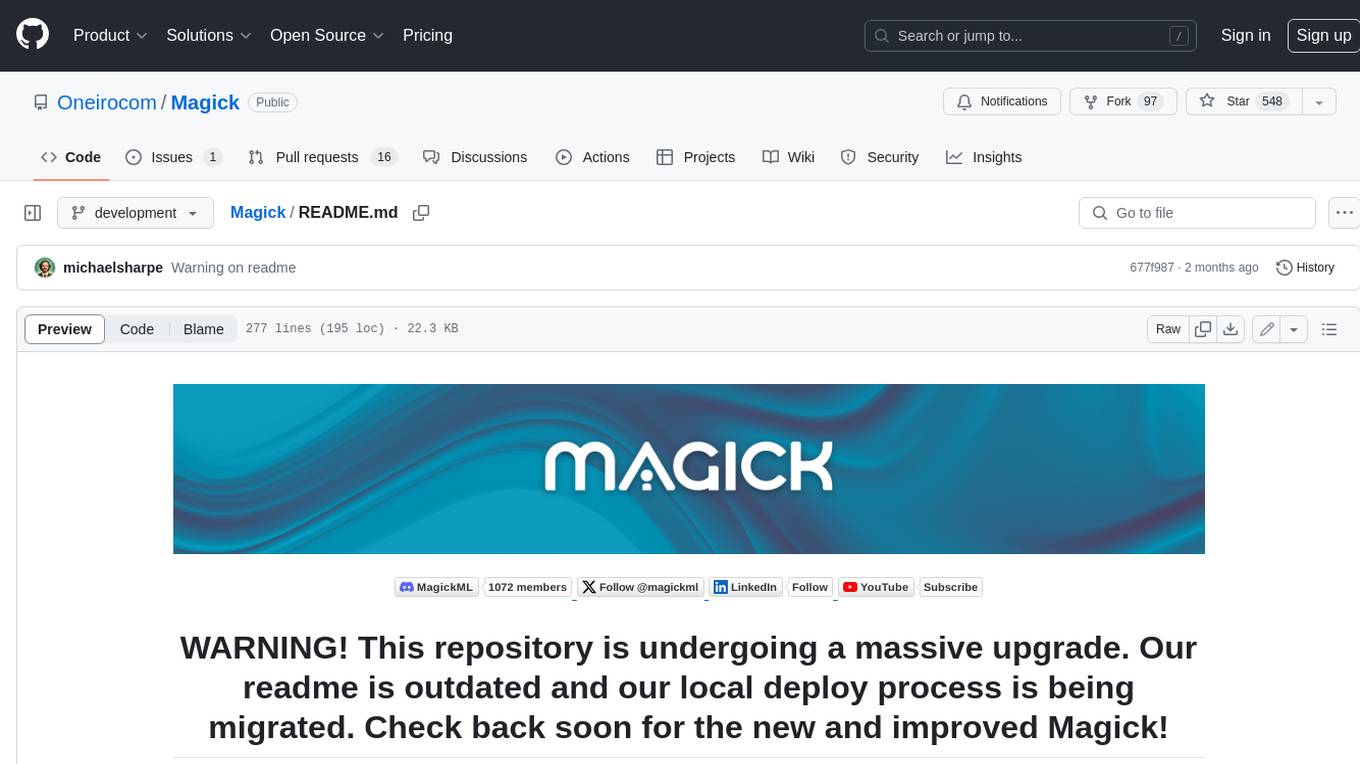
Magick
Magick is a groundbreaking visual AIDE (Artificial Intelligence Development Environment) for no-code data pipelines and multimodal agents. Magick can connect to other services and comes with nodes and templates well-suited for intelligent agents, chatbots, complex reasoning systems and realistic characters.