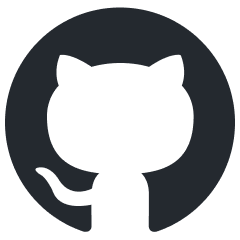
together-cookbook
A collection of notebooks/recipes showcasing usecases of open-source models with Together AI.
Stars: 769
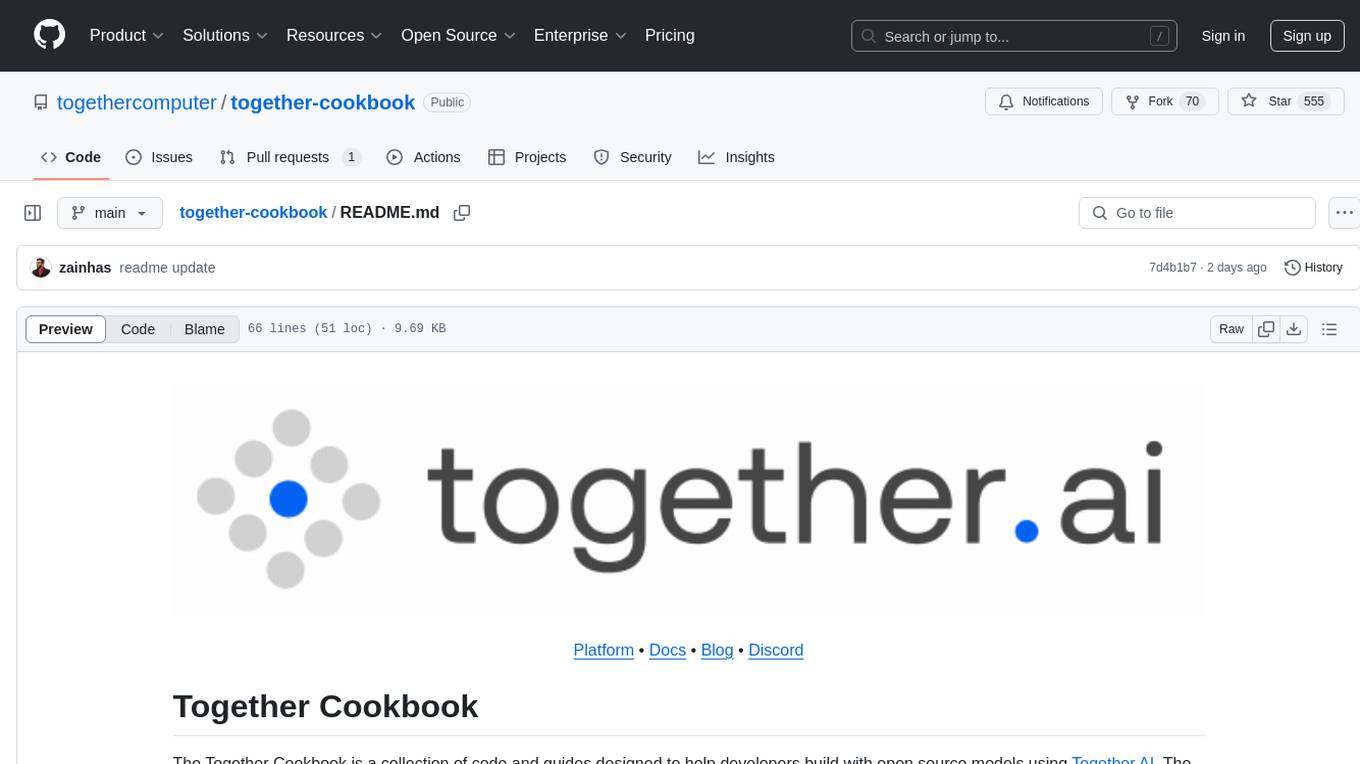
The Together Cookbook is a collection of code and guides designed to help developers build with open source models using Together AI. The recipes provide examples on how to chain multiple LLM calls, create agents that route tasks to specialized models, run multiple LLMs in parallel, break down tasks into parallel subtasks, build agents that iteratively improve responses, perform LoRA fine-tuning and inference, fine-tune LLMs for repetition, improve summarization capabilities, fine-tune LLMs on multi-step conversations, implement retrieval-augmented generation, conduct multimodal search and conditional image generation, visualize vector embeddings, improve search results with rerankers, implement vector search with embedding models, extract structured text from images, summarize and evaluate outputs with LLMs, generate podcasts from PDF content, and get LLMs to generate knowledge graphs.
README:
Platform • Docs • Blog • Discord
The Together Cookbook is a collection of code and guides designed to help developers build with open source models using Together AI. The best way to use the recipes is to copy code snippets and integrate them into your own projects!
We welcome contributions to this repository! If you have a cookbook you'd like to add, please reach out to use the Discord or open a pull request!
To make the most of the examples in this cookbook, you'll need a Together AI API key (sign up for free here).
While the code examples are primarily written in Python/JS, the concepts can be adapted to any programming language that supports interaction with the Together API.
Cookbook | Description | Open |
---|---|---|
Agents | ||
Serial Chain Agent | Chain multiple LLM calls sequentially to process complex tasks. | |
Conditional Router Agent Workflow | Create an agent that routes tasks to specialized models. | |
Parallel Agent Workflow | Run multiple LLMs in parallel and aggregate their solutions. | |
Orchestrator Subtask Agent Workflow | Break down tasks into parallel subtasks to be executed by LLMs. | |
Looping Agent Workflow | Build an agent that iteratively improves responses | |
Fine-tuning | ||
LoRA Inference and Fine-tuning | Perform LoRA fine-tuning and inference on Together AI. |
|
Long Context Finetuning For Repetition | Fine-tuning LLMs to repeat back words in long sequences. | |
Summarization Long Context Finetuning | Long context fine-tuning to improve summarization capabilities. | |
Conversation Finetuning | Fine-tuning LLMs on multi-step conversations. | |
Retrieval-augmented generation | ||
RAG_with_Reasoning_Models | RAG + source citations with DeepSeek R1. | |
MultiModal_RAG_with_Nvidia_Deck | Multimodal RAG using Nvidia investor slides |
|
Open_Contextual_RAG | An implementation of Contextual Retrieval using open models. | |
Text_RAG | Implement text-based Retrieval-Augmented Generation | |
Search | ||
Multimodal Search and Conditional Image Generation | Text-to-image and image-to-image search and condtional image generation. | |
Embedding_Visualization | Visualize vector embeddings | |
Search_with_Reranking | Improve search results with rerankers | |
Semantic_Search | Implement vector search with embedding models | |
Miscellaneous | ||
Thinking_Augmented_Generation | Give R1 thinking tokens to small models | |
Flux LoRA Inference | Generate images with fine-tuned Flux LoRA's | |
Structured_Text_Extraction_from_Images | Extract structured text from images | |
Summarization Evaluation | Summarizing and evaluating outputs with LLMs. | |
PDF_to_Podcast | Generate a podcast from PDF content NotebookLM style! | |
Knowledge_Graphs_with_Structured_Outputs | Get LLMs to generate knowledge graphs |
Looking for more resources to enhance your experience with open source models? Check out these helpful links:
- Together AI Research: Explore papers and technical blog posts from our research team.
- Together AI Blog: Explore technical blogs, product announcements and more on our blog.
For Tasks:
Click tags to check more tools for each tasksFor Jobs:
Alternative AI tools for together-cookbook
Similar Open Source Tools
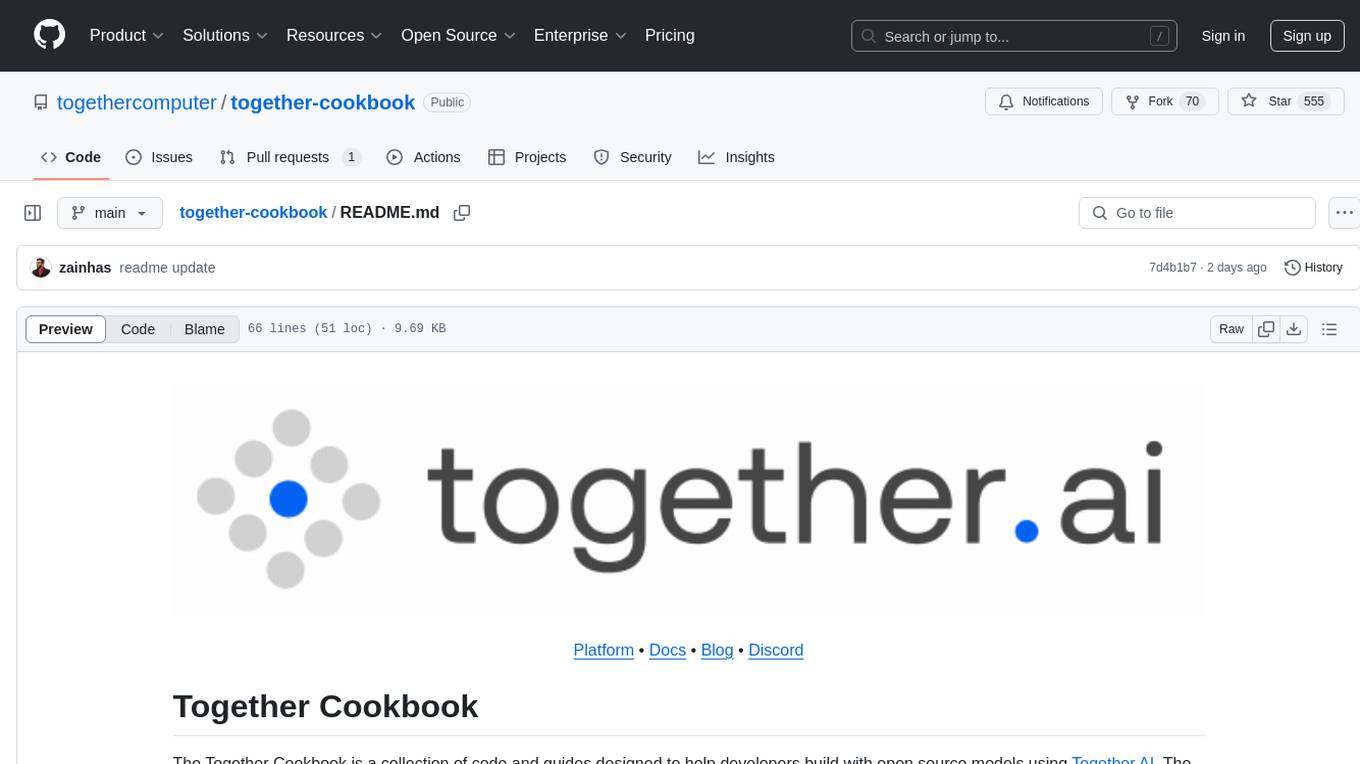
together-cookbook
The Together Cookbook is a collection of code and guides designed to help developers build with open source models using Together AI. The recipes provide examples on how to chain multiple LLM calls, create agents that route tasks to specialized models, run multiple LLMs in parallel, break down tasks into parallel subtasks, build agents that iteratively improve responses, perform LoRA fine-tuning and inference, fine-tune LLMs for repetition, improve summarization capabilities, fine-tune LLMs on multi-step conversations, implement retrieval-augmented generation, conduct multimodal search and conditional image generation, visualize vector embeddings, improve search results with rerankers, implement vector search with embedding models, extract structured text from images, summarize and evaluate outputs with LLMs, generate podcasts from PDF content, and get LLMs to generate knowledge graphs.
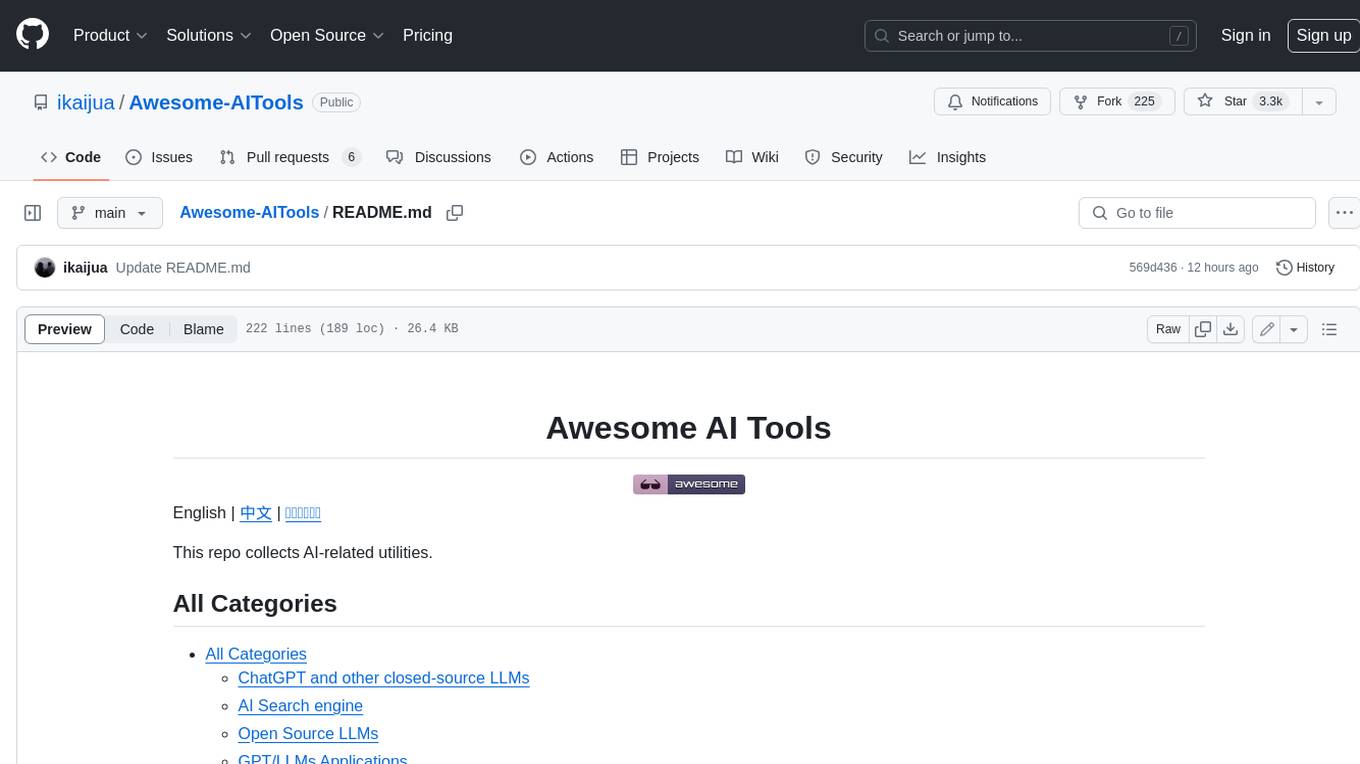
Awesome-AITools
This repo collects AI-related utilities. ## All Categories * All Categories * ChatGPT and other closed-source LLMs * AI Search engine * Open Source LLMs * GPT/LLMs Applications * LLM training platform * Applications that integrate multiple LLMs * AI Agent * Writing * Programming Development * Translation * AI Conversation or AI Voice Conversation * Image Creation * Speech Recognition * Text To Speech * Voice Processing * AI generated music or sound effects * Speech translation * Video Creation * Video Content Summary * OCR(Optical Character Recognition)
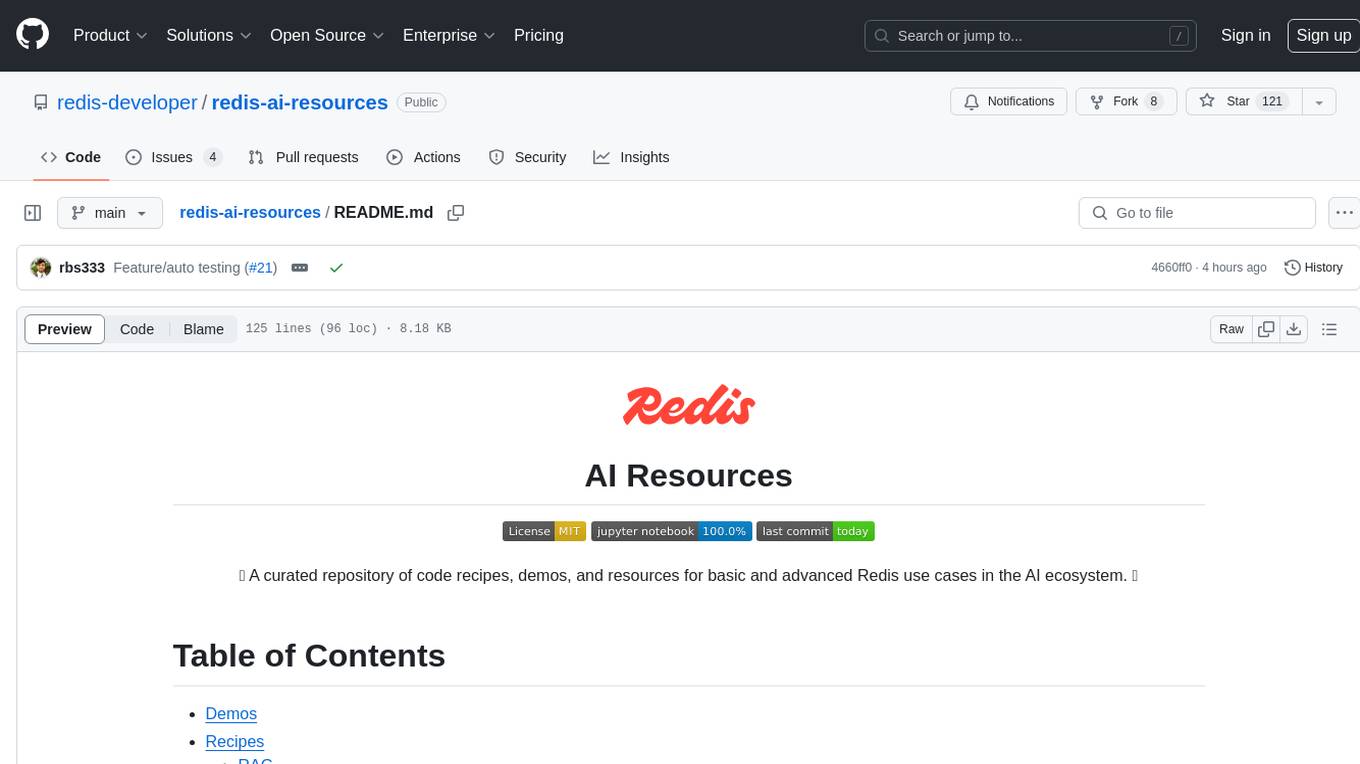
redis-ai-resources
A curated repository of code recipes, demos, and resources for basic and advanced Redis use cases in the AI ecosystem. It includes demos for ArxivChatGuru, Redis VSS, Vertex AI & Redis, Agentic RAG, ArXiv Search, and Product Search. Recipes cover topics like Getting started with RAG, Semantic Cache, Advanced RAG, and Recommendation systems. The repository also provides integrations/tools like RedisVL, AWS Bedrock, LangChain Python, LangChain JS, LlamaIndex, Semantic Kernel, RelevanceAI, and DocArray. Additional content includes blog posts, talks, reviews, and documentation related to Vector Similarity Search, AI-Powered Document Search, Vector Databases, Real-Time Product Recommendations, and more. Benchmarks compare Redis against other Vector Databases and ANN benchmarks. Documentation includes QuickStart guides, official literature for Vector Similarity Search, Redis-py client library docs, Redis Stack documentation, and Redis client list.
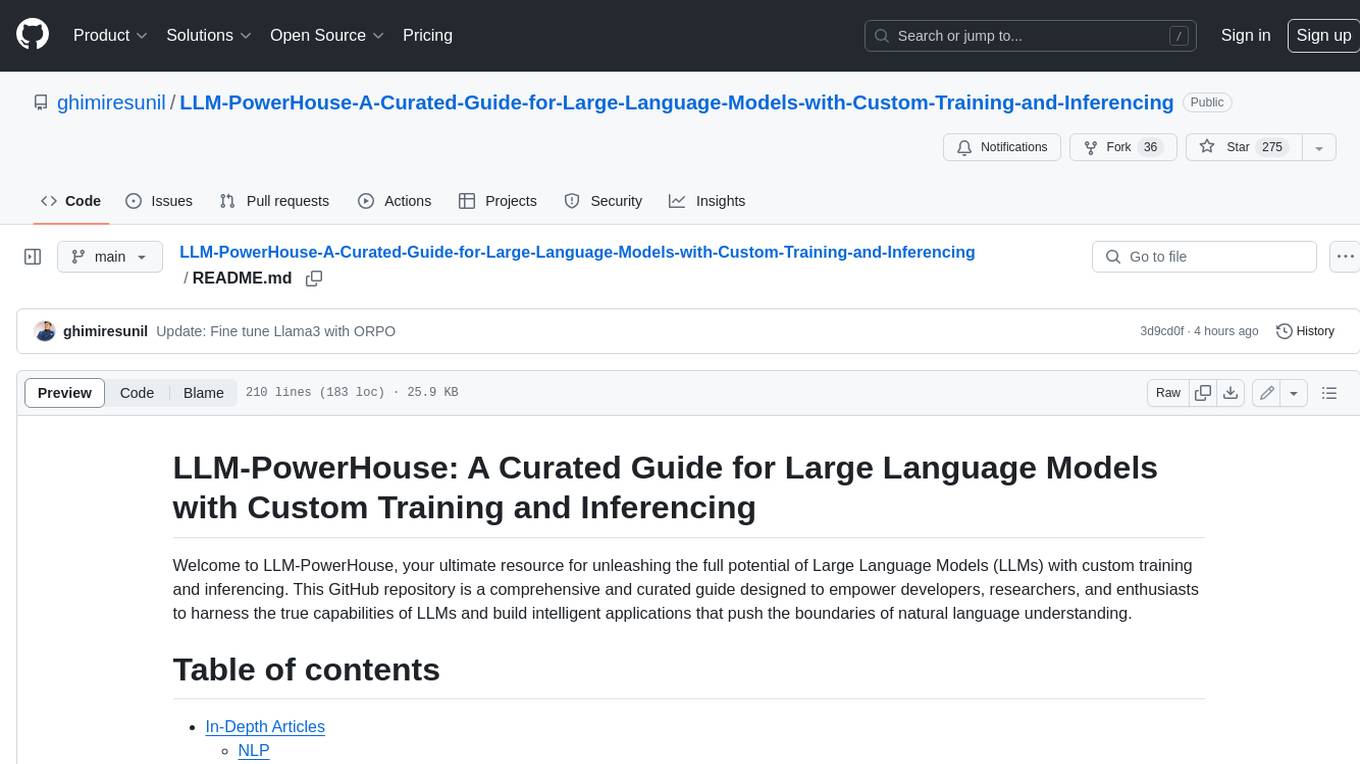
LLM-PowerHouse-A-Curated-Guide-for-Large-Language-Models-with-Custom-Training-and-Inferencing
LLM-PowerHouse is a comprehensive and curated guide designed to empower developers, researchers, and enthusiasts to harness the true capabilities of Large Language Models (LLMs) and build intelligent applications that push the boundaries of natural language understanding. This GitHub repository provides in-depth articles, codebase mastery, LLM PlayLab, and resources for cost analysis and network visualization. It covers various aspects of LLMs, including NLP, models, training, evaluation metrics, open LLMs, and more. The repository also includes a collection of code examples and tutorials to help users build and deploy LLM-based applications.
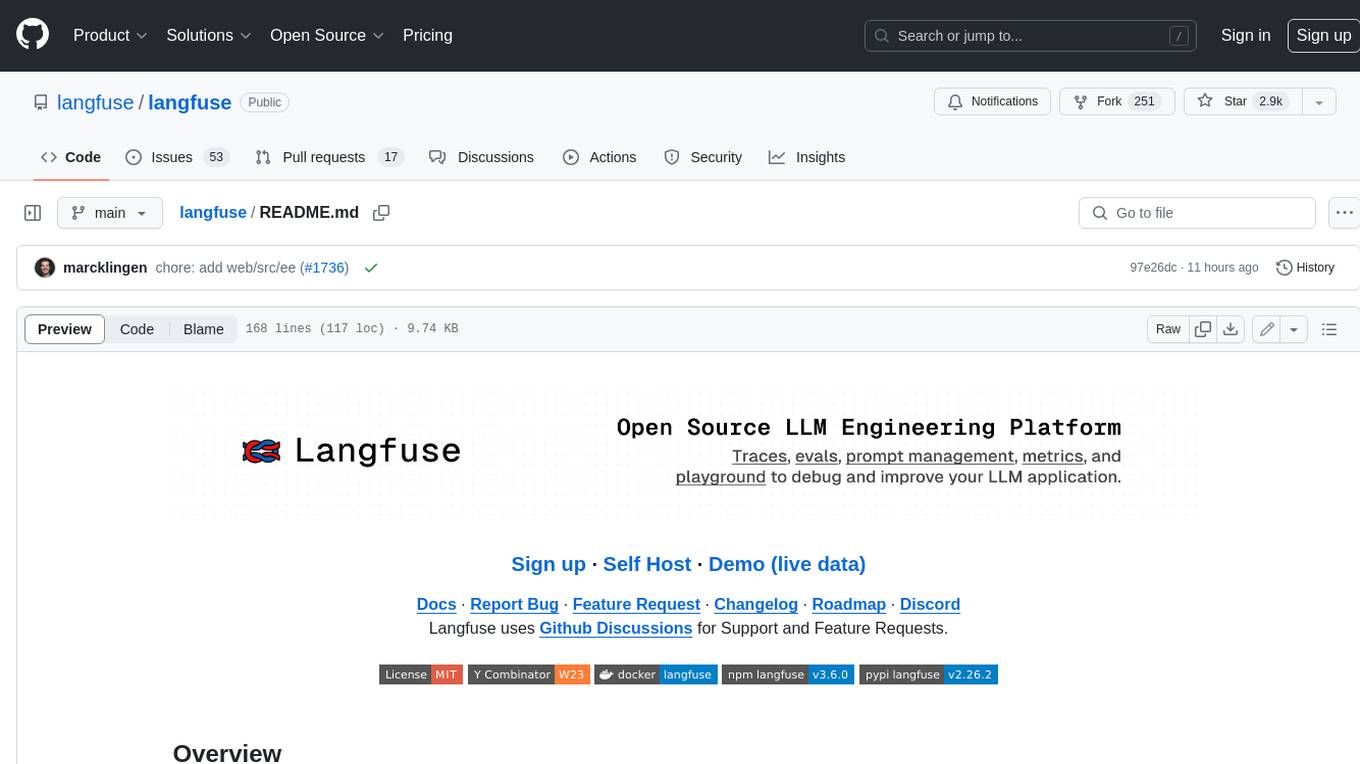
langfuse
Langfuse is a powerful tool that helps you develop, monitor, and test your LLM applications. With Langfuse, you can: * **Develop:** Instrument your app and start ingesting traces to Langfuse, inspect and debug complex logs, and manage, version, and deploy prompts from within Langfuse. * **Monitor:** Track metrics (cost, latency, quality) and gain insights from dashboards & data exports, collect and calculate scores for your LLM completions, run model-based evaluations, collect user feedback, and manually score observations in Langfuse. * **Test:** Track and test app behaviour before deploying a new version, test expected in and output pairs and benchmark performance before deploying, and track versions and releases in your application. Langfuse is easy to get started with and offers a generous free tier. You can sign up for Langfuse Cloud or deploy Langfuse locally or on your own infrastructure. Langfuse also offers a variety of integrations to make it easy to connect to your LLM applications.
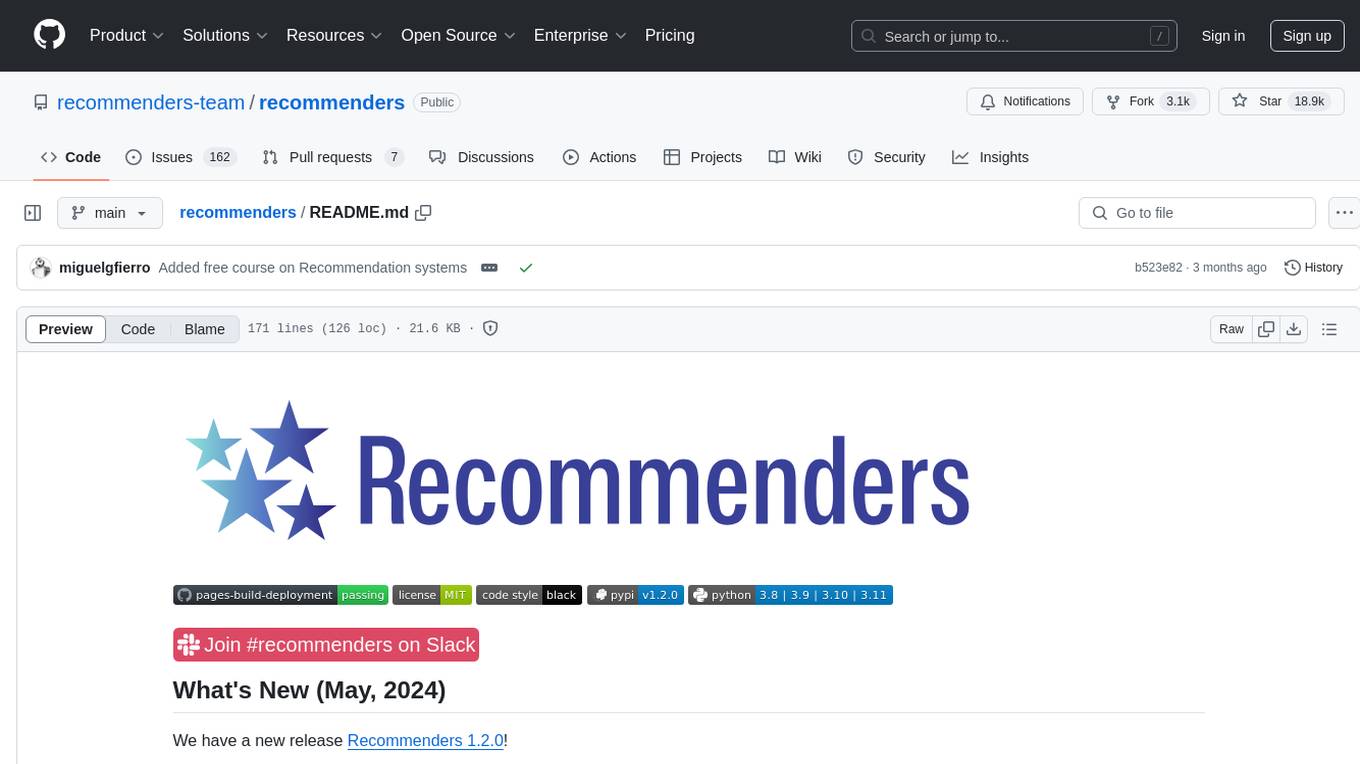
recommenders
Recommenders is a project under the Linux Foundation of AI and Data that assists researchers, developers, and enthusiasts in prototyping, experimenting with, and bringing to production a range of classic and state-of-the-art recommendation systems. The repository contains examples and best practices for building recommendation systems, provided as Jupyter notebooks. It covers tasks such as preparing data, building models using various recommendation algorithms, evaluating algorithms, tuning hyperparameters, and operationalizing models in a production environment on Azure. The project provides utilities to support common tasks like loading datasets, evaluating model outputs, and splitting training/test data. It includes implementations of state-of-the-art algorithms for self-study and customization in applications.
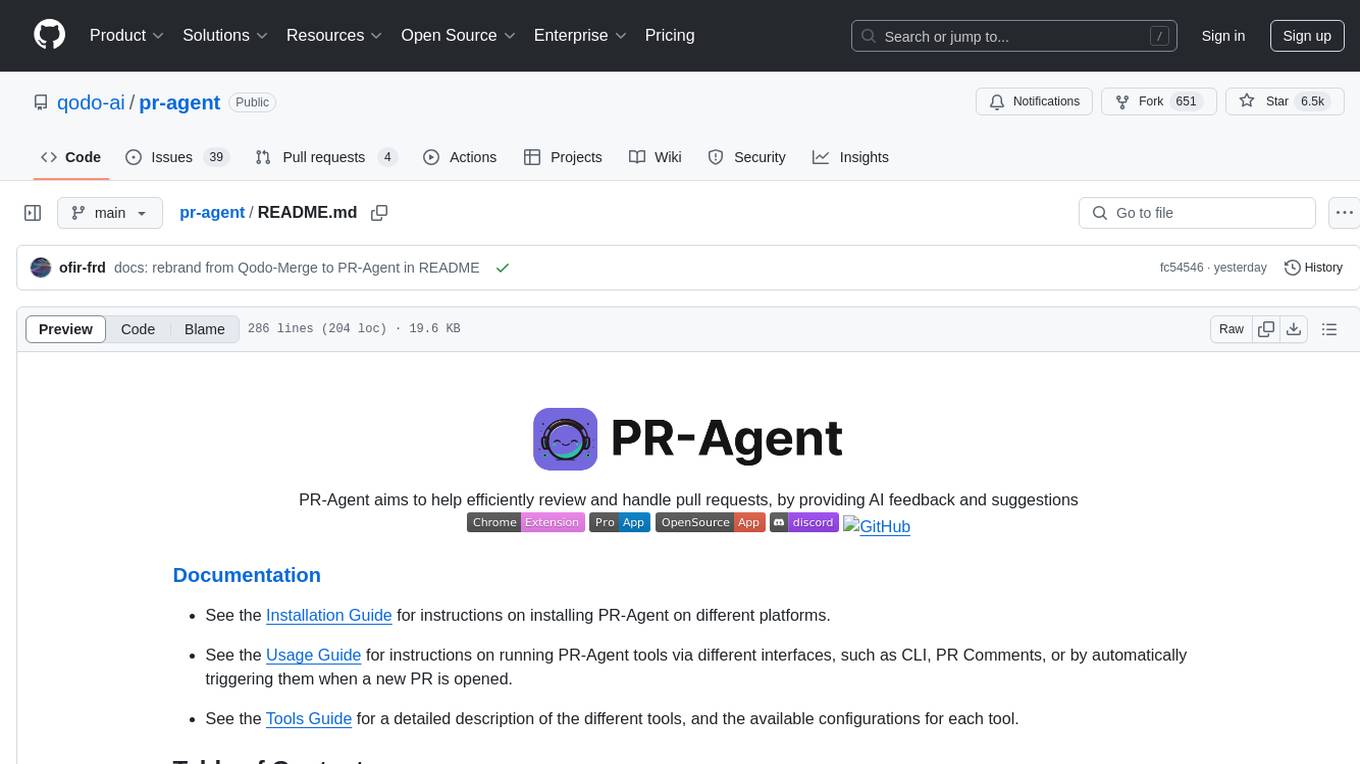
pr-agent
PR-Agent is a tool designed to assist in efficiently reviewing and handling pull requests by providing AI feedback and suggestions. It offers various tools such as Review, Describe, Improve, Ask, Update CHANGELOG, and more, with the ability to run them via different interfaces like CLI, PR Comments, or automatically triggering them when a new PR is opened. The tool supports multiple git platforms and models, emphasizing real-life practical usage and modular, customizable tools.
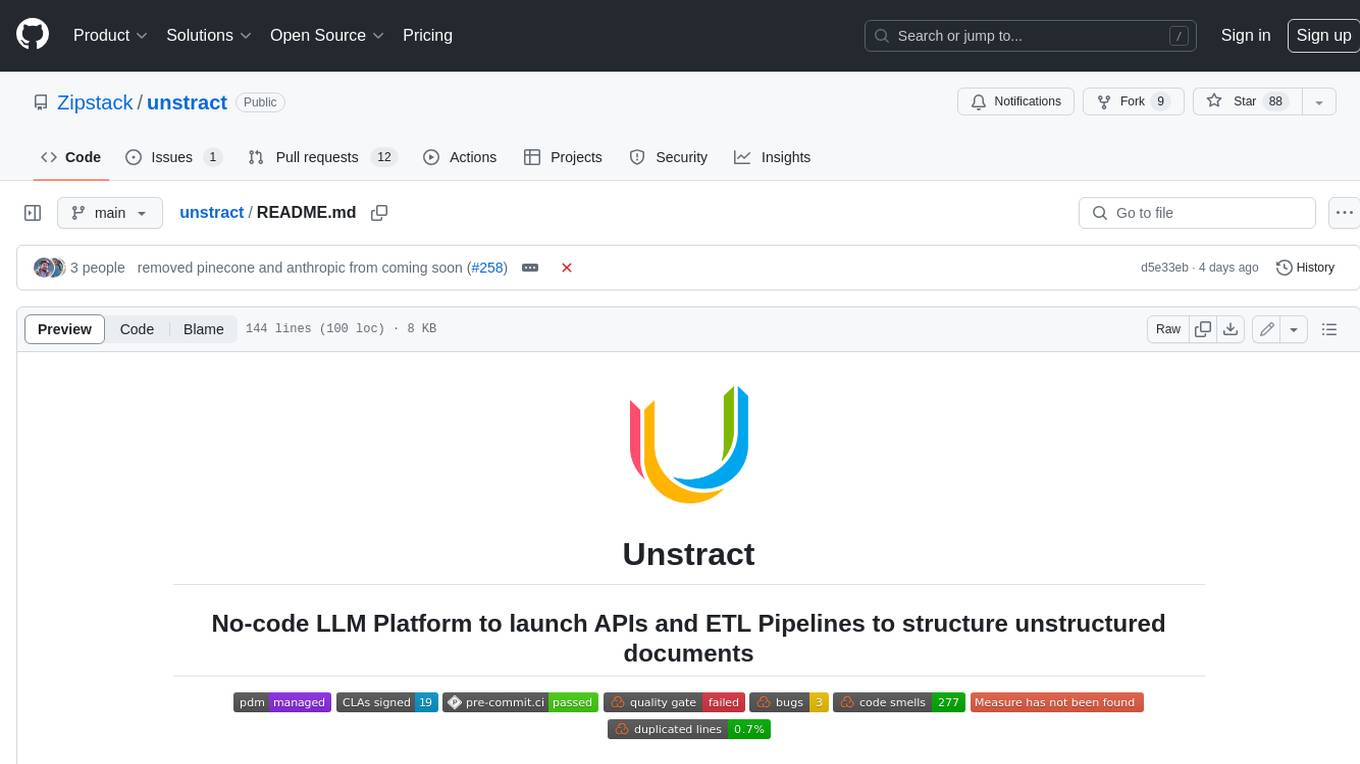
unstract
Unstract is a no-code platform that enables users to launch APIs and ETL pipelines to structure unstructured documents. With Unstract, users can go beyond co-pilots by enabling machine-to-machine automation. Unstract's Prompt Studio provides a simple, no-code approach to creating prompts for LLMs, vector databases, embedding models, and text extractors. Users can then configure Prompt Studio projects as API deployments or ETL pipelines to automate critical business processes that involve complex documents. Unstract supports a wide range of LLM providers, vector databases, embeddings, text extractors, ETL sources, and ETL destinations, providing users with the flexibility to choose the best tools for their needs.
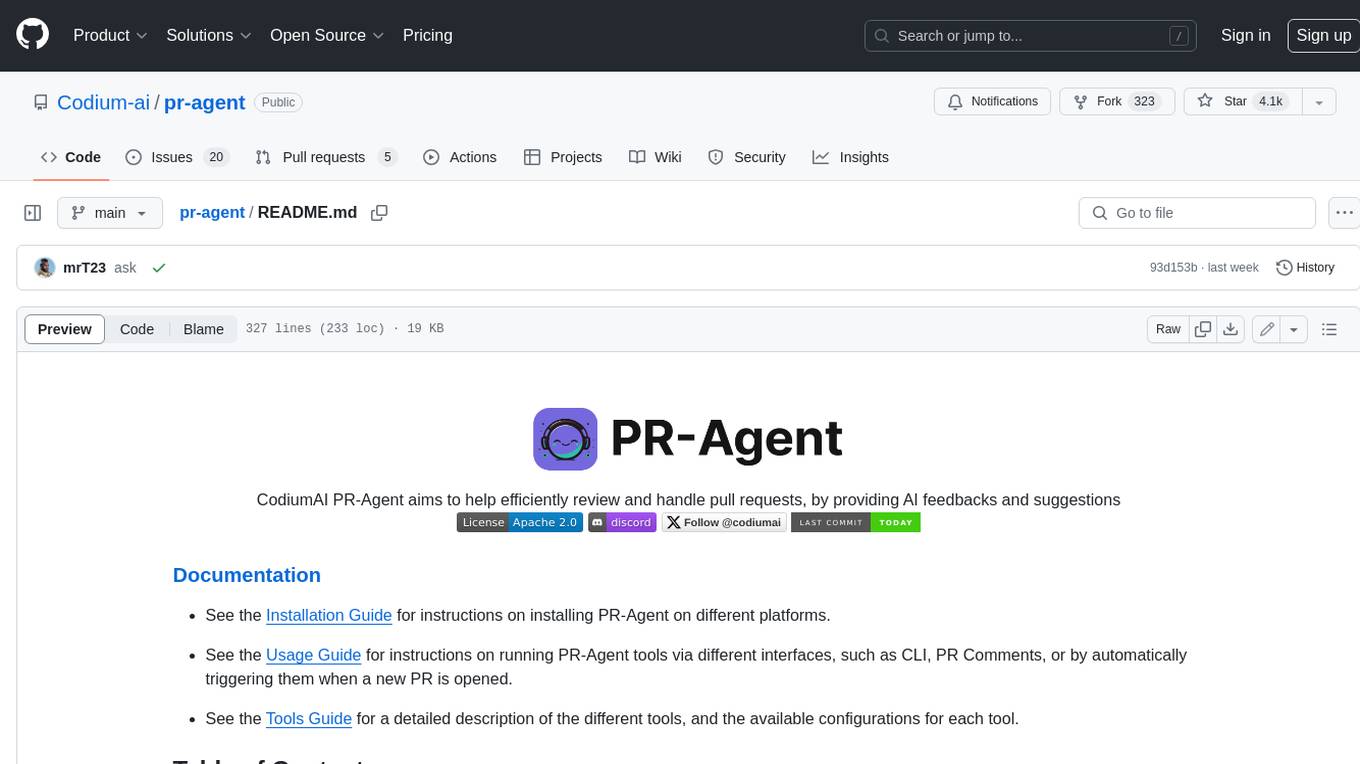
pr-agent
PR-Agent is a tool that helps to efficiently review and handle pull requests by providing AI feedbacks and suggestions. It supports various commands such as generating PR descriptions, providing code suggestions, answering questions about the PR, and updating the CHANGELOG.md file. PR-Agent can be used via CLI, GitHub Action, GitHub App, Docker, and supports multiple git providers and models. It emphasizes real-life practical usage, with each tool having a single GPT-4 call for quick and affordable responses. The PR Compression strategy enables effective handling of both short and long PRs, while the JSON prompting strategy allows for modular and customizable tools. PR-Agent Pro, the hosted version by CodiumAI, provides additional benefits such as full management, improved privacy, priority support, and extra features.
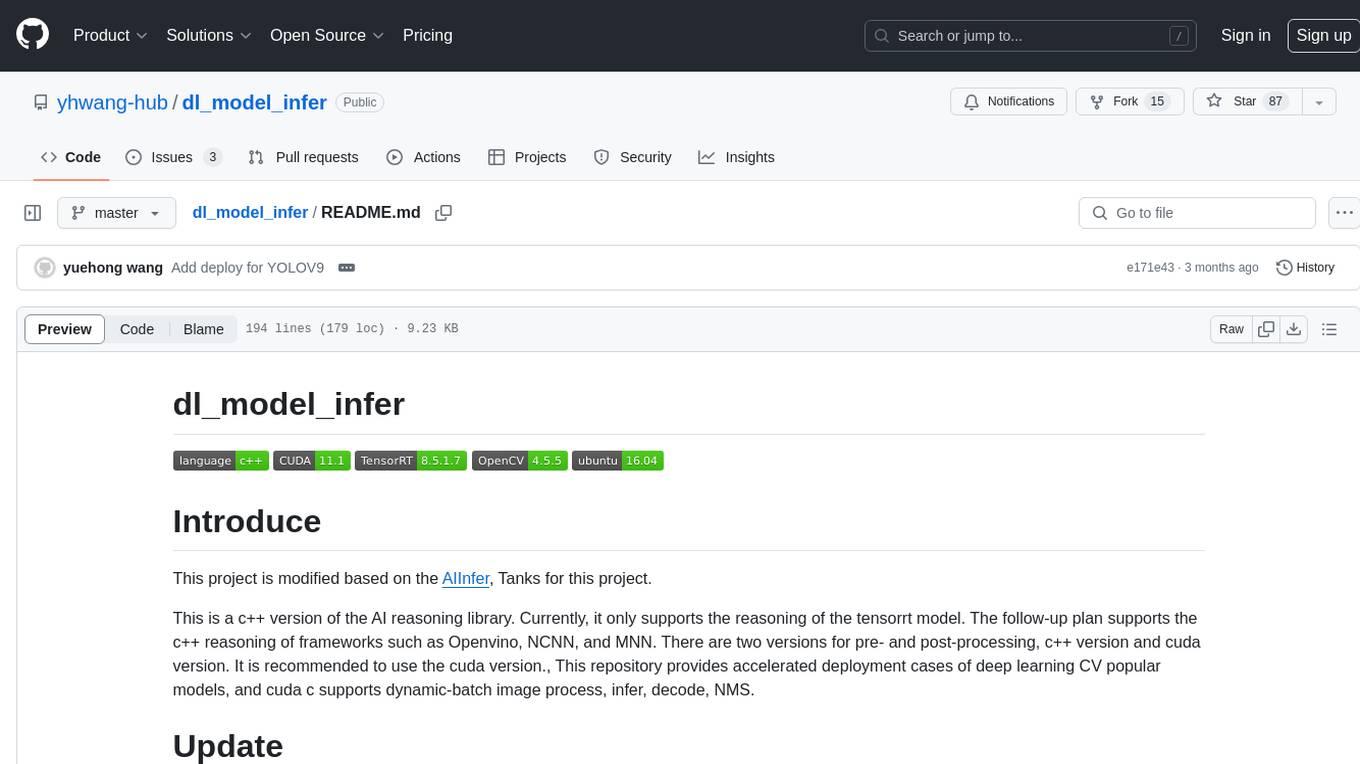
dl_model_infer
This project is a c++ version of the AI reasoning library that supports the reasoning of tensorrt models. It provides accelerated deployment cases of deep learning CV popular models and supports dynamic-batch image processing, inference, decode, and NMS. The project has been updated with various models and provides tutorials for model exports. It also includes a producer-consumer inference model for specific tasks. The project directory includes implementations for model inference applications, backend reasoning classes, post-processing, pre-processing, and target detection and tracking. Speed tests have been conducted on various models, and onnx downloads are available for different models.
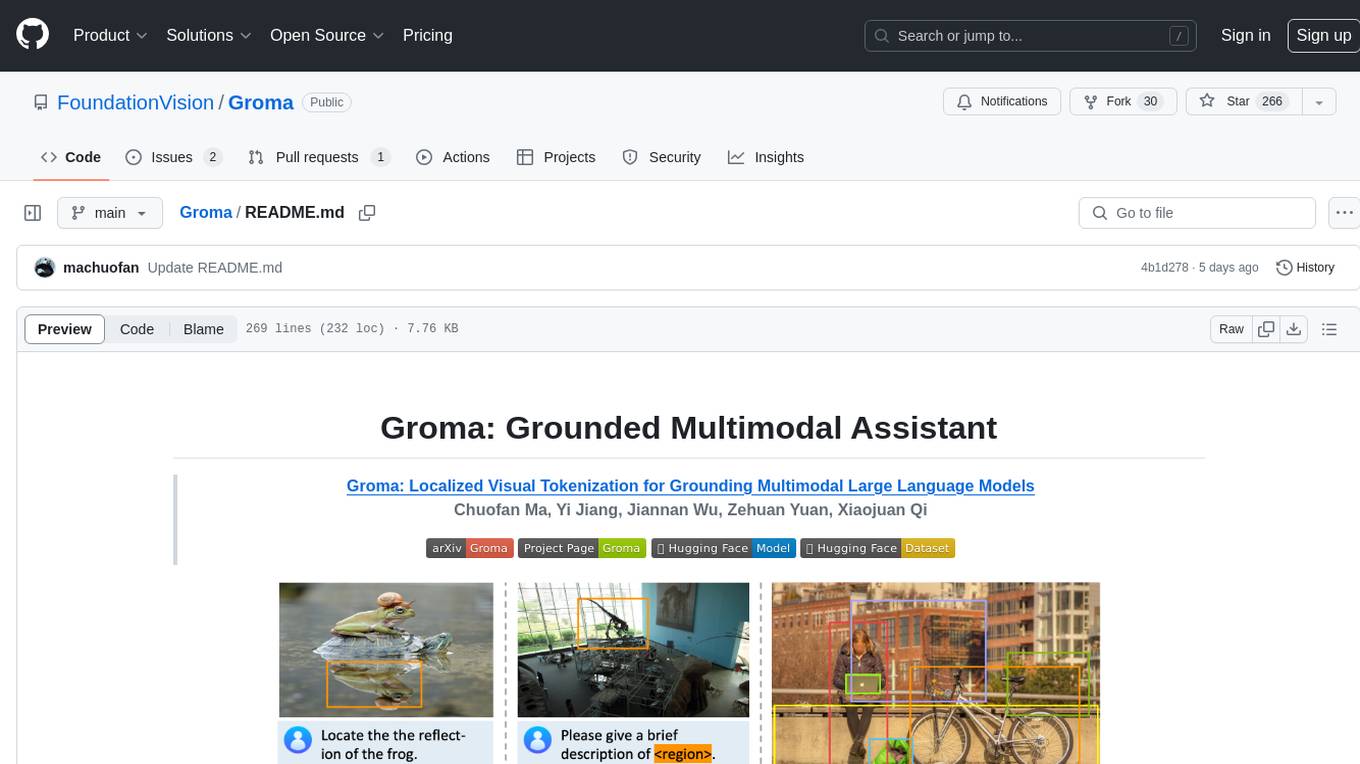
Groma
Groma is a grounded multimodal assistant that excels in region understanding and visual grounding. It can process user-defined region inputs and generate contextually grounded long-form responses. The tool presents a unique paradigm for multimodal large language models, focusing on visual tokenization for localization. Groma achieves state-of-the-art performance in referring expression comprehension benchmarks. The tool provides pretrained model weights and instructions for data preparation, training, inference, and evaluation. Users can customize training by starting from intermediate checkpoints. Groma is designed to handle tasks related to detection pretraining, alignment pretraining, instruction finetuning, instruction following, and more.
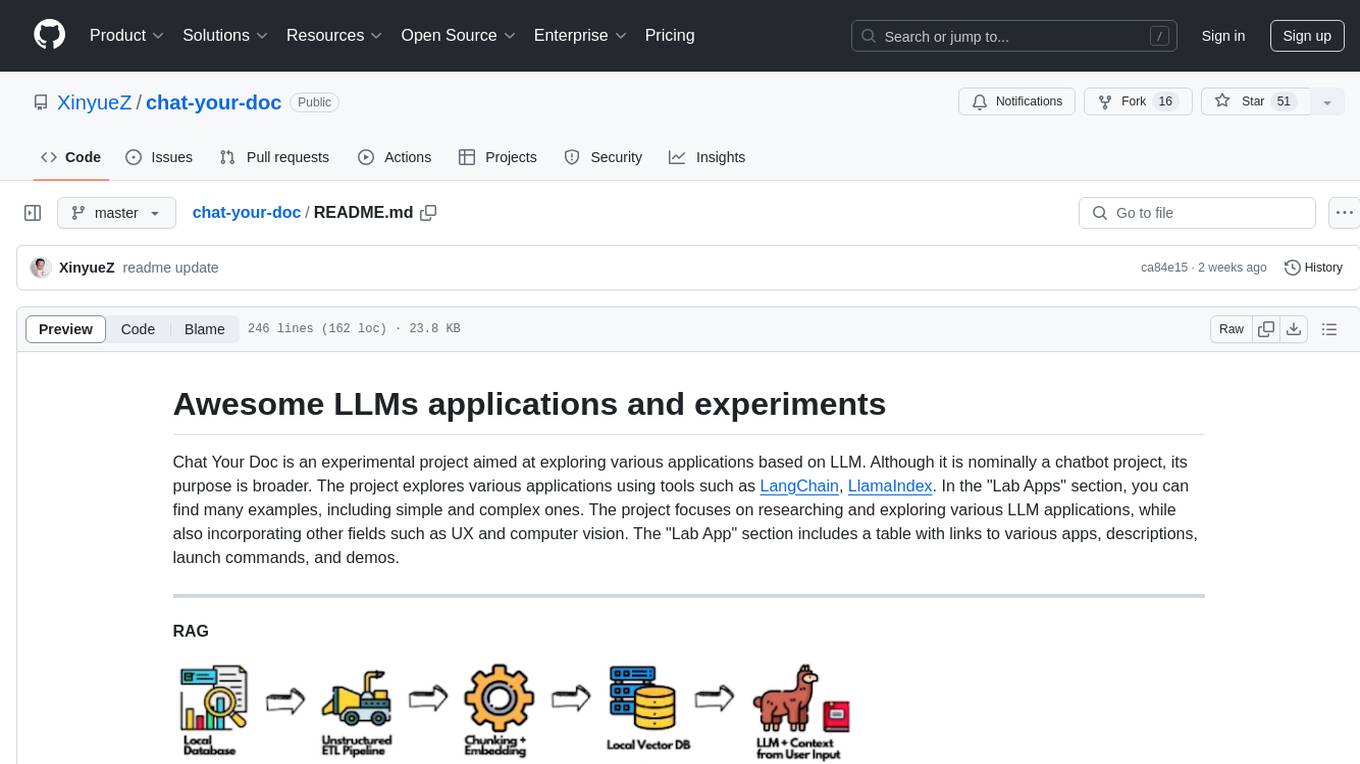
chat-your-doc
Chat Your Doc is an experimental project exploring various applications based on LLM technology. It goes beyond being just a chatbot project, focusing on researching LLM applications using tools like LangChain and LlamaIndex. The project delves into UX, computer vision, and offers a range of examples in the 'Lab Apps' section. It includes links to different apps, descriptions, launch commands, and demos, aiming to showcase the versatility and potential of LLM applications.
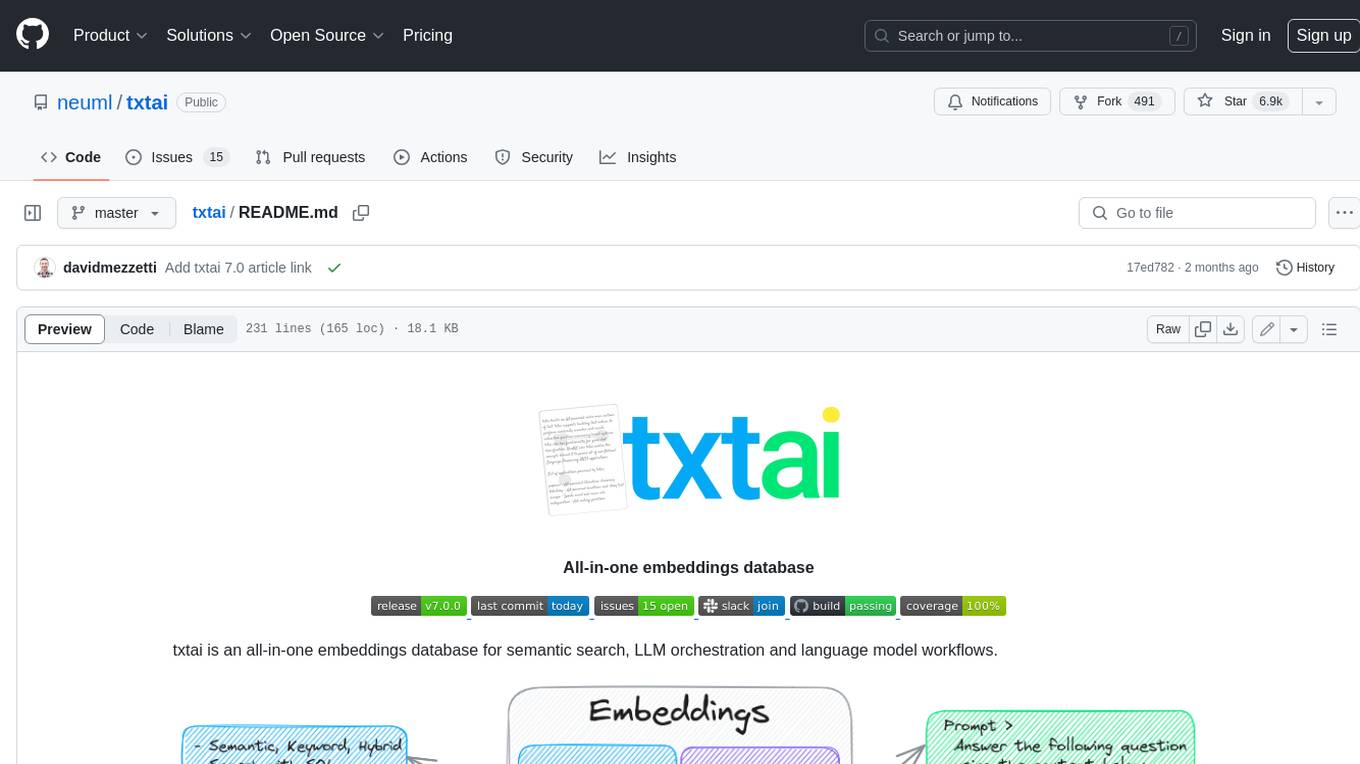
txtai
Txtai is an all-in-one embeddings database for semantic search, LLM orchestration, and language model workflows. It combines vector indexes, graph networks, and relational databases to enable vector search with SQL, topic modeling, retrieval augmented generation, and more. Txtai can stand alone or serve as a knowledge source for large language models (LLMs). Key features include vector search with SQL, object storage, topic modeling, graph analysis, multimodal indexing, embedding creation for various data types, pipelines powered by language models, workflows to connect pipelines, and support for Python, JavaScript, Java, Rust, and Go. Txtai is open-source under the Apache 2.0 license.
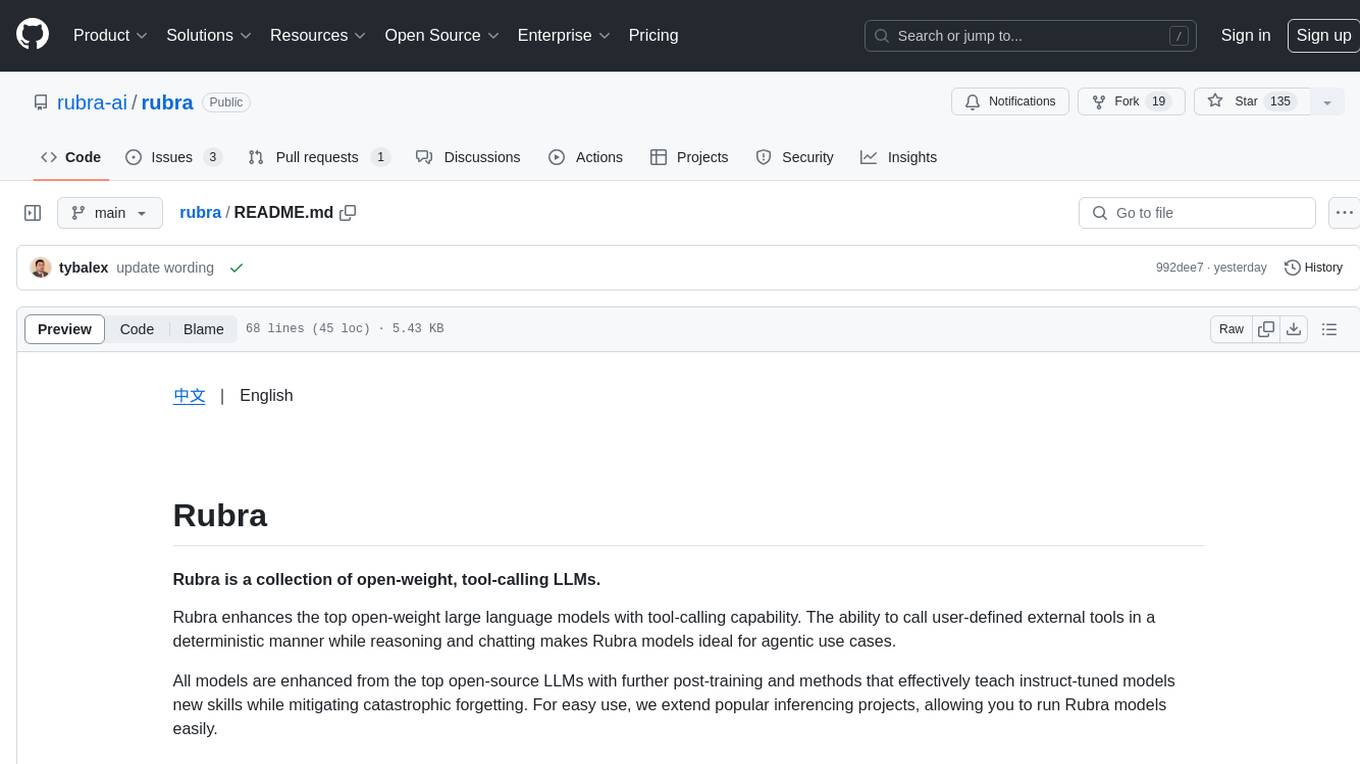
rubra
Rubra is a collection of open-weight large language models enhanced with tool-calling capability. It allows users to call user-defined external tools in a deterministic manner while reasoning and chatting, making it ideal for agentic use cases. The models are further post-trained to teach instruct-tuned models new skills and mitigate catastrophic forgetting. Rubra extends popular inferencing projects for easy use, enabling users to run the models easily.
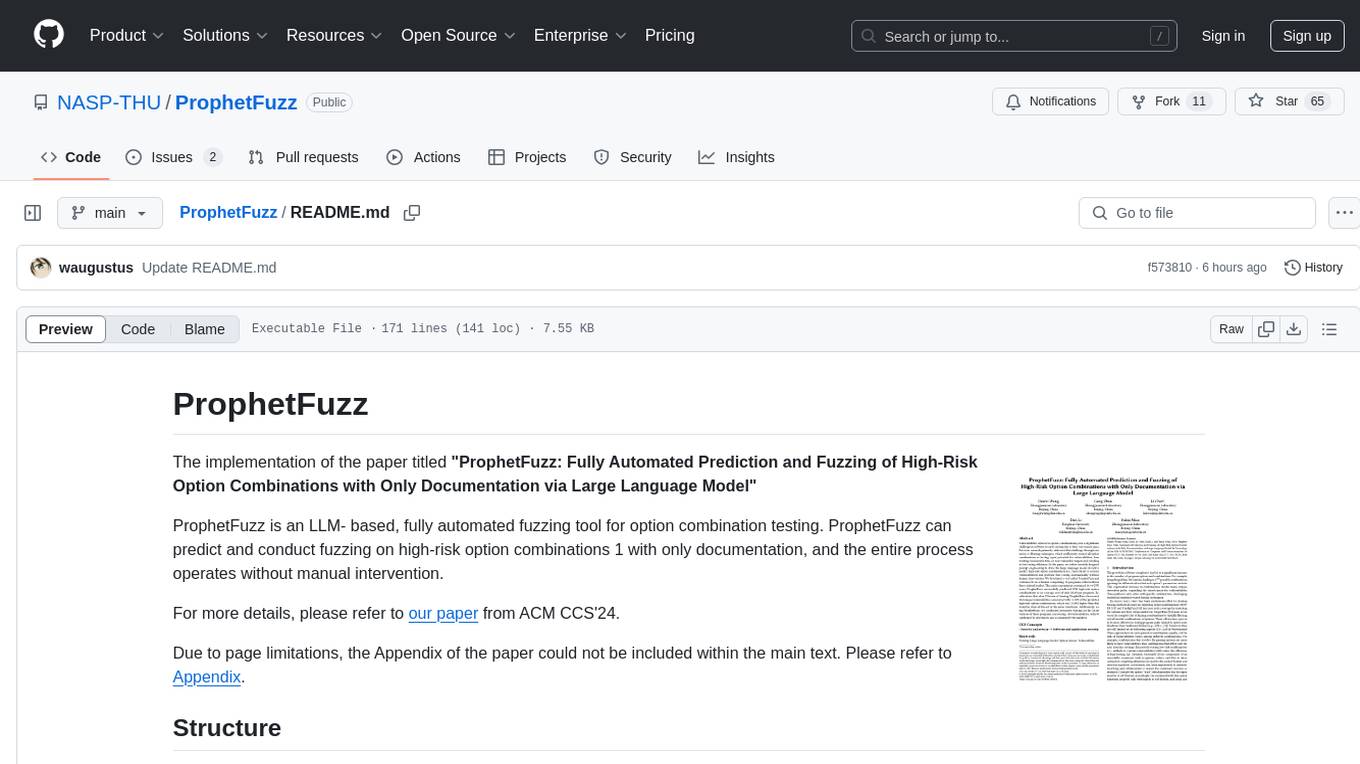
ProphetFuzz
ProphetFuzz is a fully automated fuzzing tool based on Large Language Models (LLM) for testing high-risk option combinations with only documentation. It can predict and conduct fuzzing on high-risk option combinations without manual intervention. The tool consists of components for parsing documentation, extracting constraints, predicting combinations, assembling commands, generating files, and conducting fuzzing. ProphetFuzz has been used to uncover numerous vulnerabilities in various programs, earning CVE numbers for confirmed vulnerabilities. The tool has been credited to Dawei Wang and Geng Zhou.
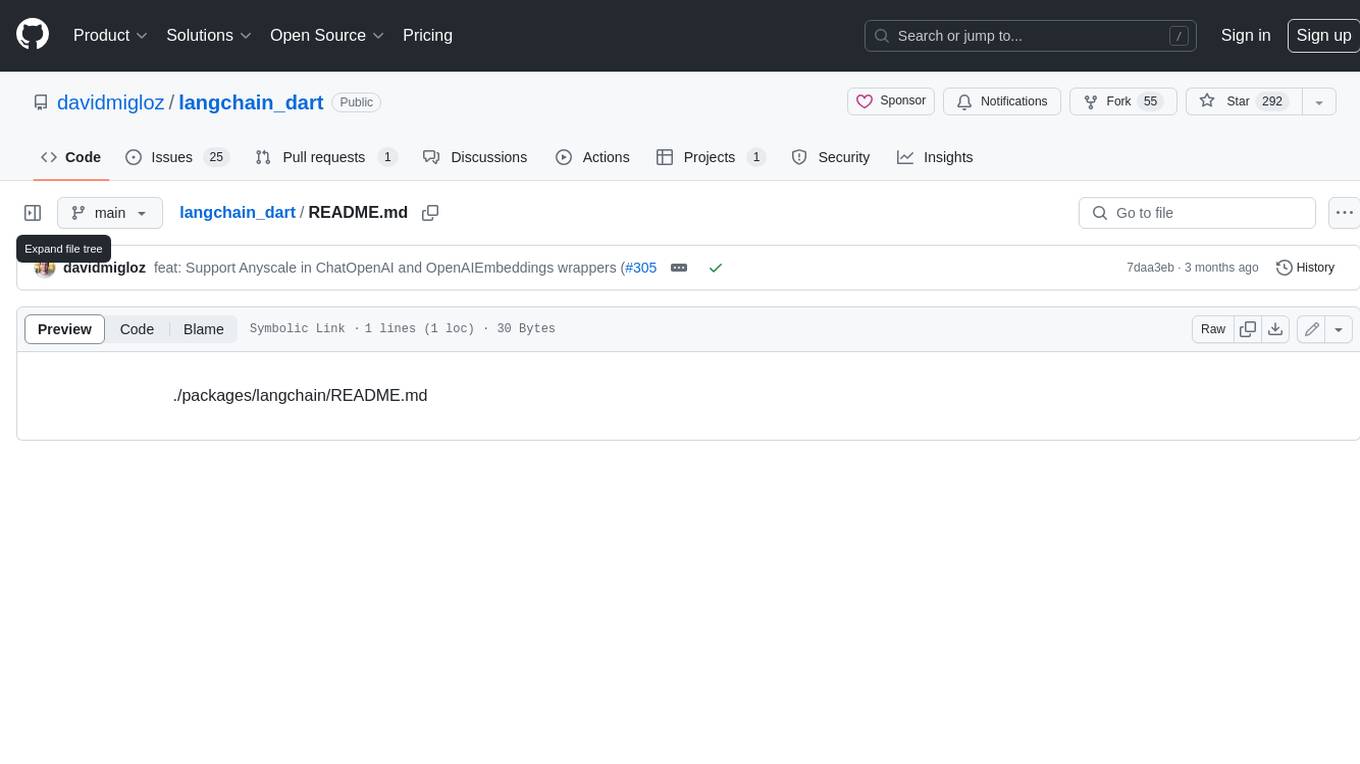
langchain_dart
LangChain.dart is a Dart port of the popular LangChain Python framework created by Harrison Chase. LangChain provides a set of ready-to-use components for working with language models and a standard interface for chaining them together to formulate more advanced use cases (e.g. chatbots, Q&A with RAG, agents, summarization, extraction, etc.). The components can be grouped into a few core modules: * **Model I/O:** LangChain offers a unified API for interacting with various LLM providers (e.g. OpenAI, Google, Mistral, Ollama, etc.), allowing developers to switch between them with ease. Additionally, it provides tools for managing model inputs (prompt templates and example selectors) and parsing the resulting model outputs (output parsers). * **Retrieval:** assists in loading user data (via document loaders), transforming it (with text splitters), extracting its meaning (using embedding models), storing (in vector stores) and retrieving it (through retrievers) so that it can be used to ground the model's responses (i.e. Retrieval-Augmented Generation or RAG). * **Agents:** "bots" that leverage LLMs to make informed decisions about which available tools (such as web search, calculators, database lookup, etc.) to use to accomplish the designated task. The different components can be composed together using the LangChain Expression Language (LCEL).
For similar tasks
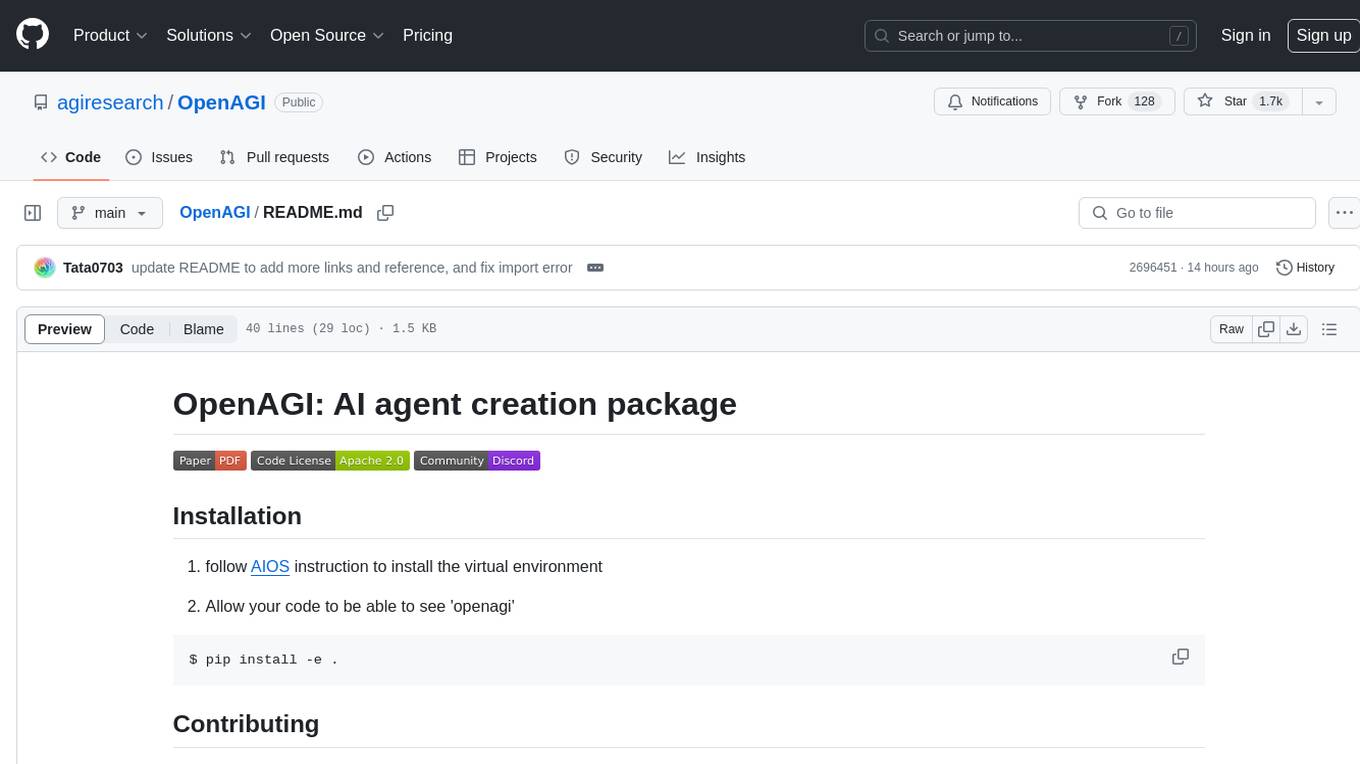
OpenAGI
OpenAGI is an AI agent creation package designed for researchers and developers to create intelligent agents using advanced machine learning techniques. The package provides tools and resources for building and training AI models, enabling users to develop sophisticated AI applications. With a focus on collaboration and community engagement, OpenAGI aims to facilitate the integration of AI technologies into various domains, fostering innovation and knowledge sharing among experts and enthusiasts.
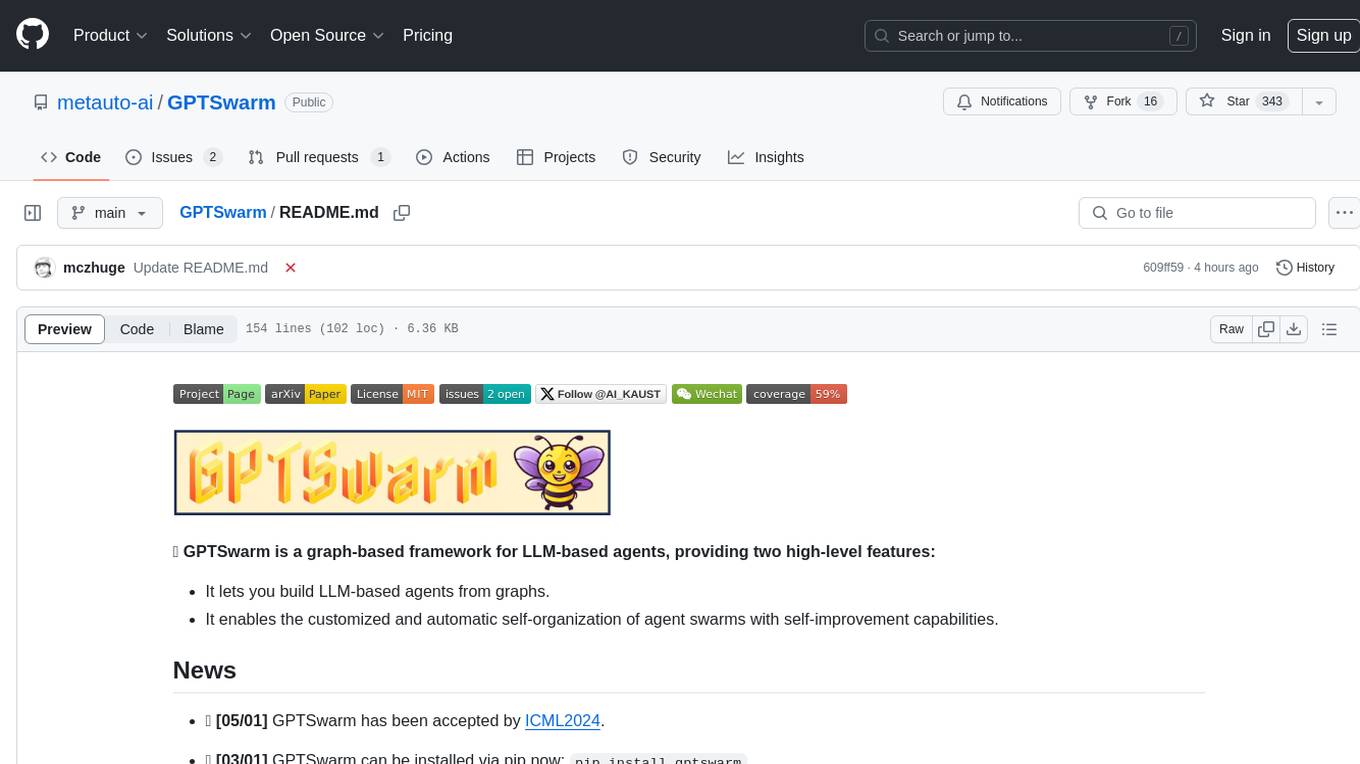
GPTSwarm
GPTSwarm is a graph-based framework for LLM-based agents that enables the creation of LLM-based agents from graphs and facilitates the customized and automatic self-organization of agent swarms with self-improvement capabilities. The library includes components for domain-specific operations, graph-related functions, LLM backend selection, memory management, and optimization algorithms to enhance agent performance and swarm efficiency. Users can quickly run predefined swarms or utilize tools like the file analyzer. GPTSwarm supports local LM inference via LM Studio, allowing users to run with a local LLM model. The framework has been accepted by ICML2024 and offers advanced features for experimentation and customization.
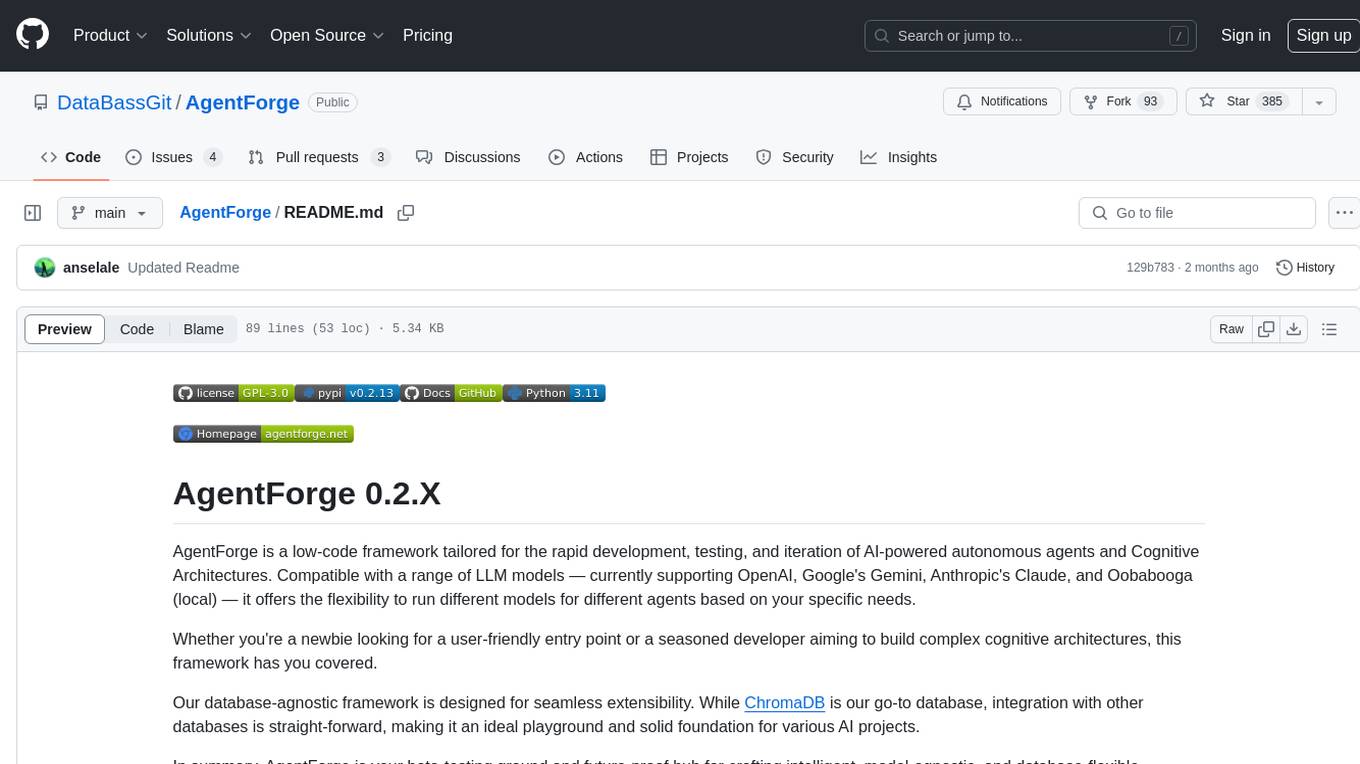
AgentForge
AgentForge is a low-code framework tailored for the rapid development, testing, and iteration of AI-powered autonomous agents and Cognitive Architectures. It is compatible with a range of LLM models and offers flexibility to run different models for different agents based on specific needs. The framework is designed for seamless extensibility and database-flexibility, making it an ideal playground for various AI projects. AgentForge is a beta-testing ground and future-proof hub for crafting intelligent, model-agnostic autonomous agents.
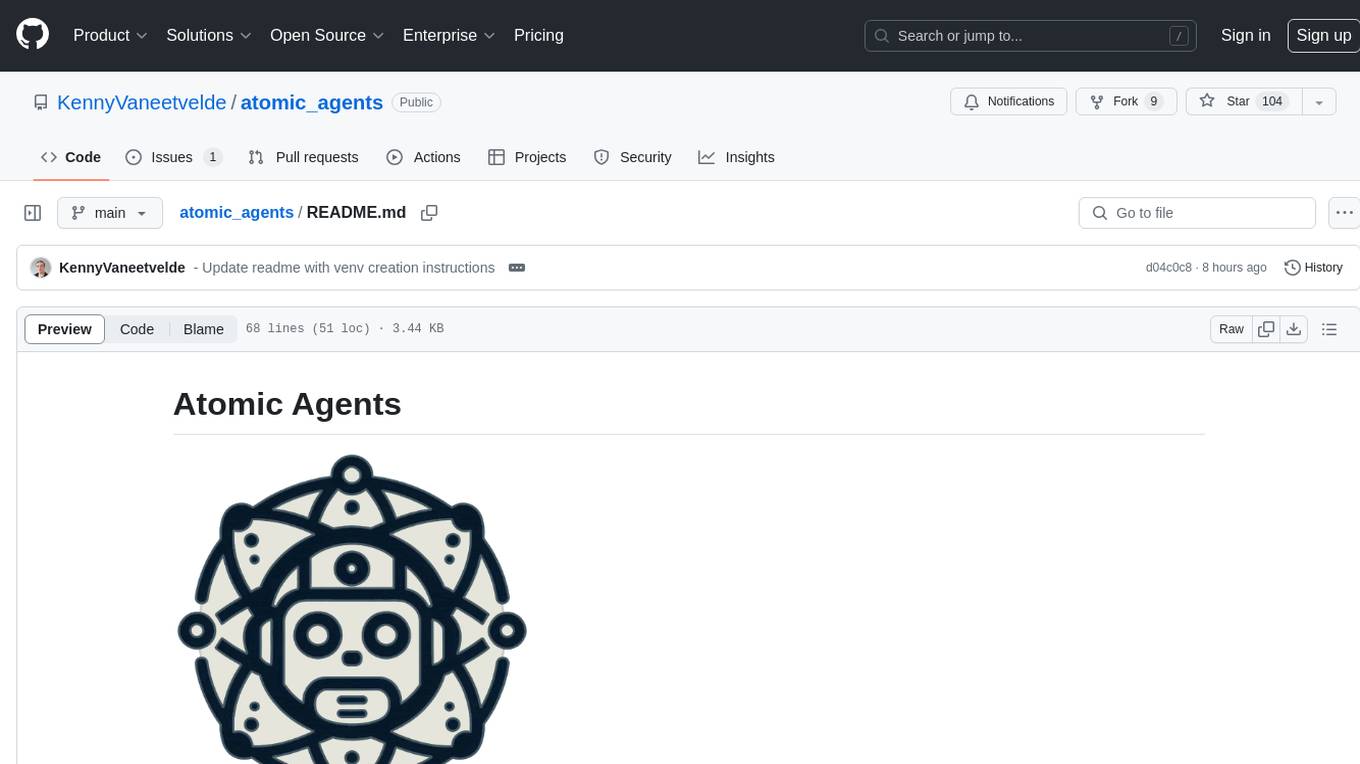
atomic_agents
Atomic Agents is a modular and extensible framework designed for creating powerful applications. It follows the principles of Atomic Design, emphasizing small and single-purpose components. Leveraging Pydantic for data validation and serialization, the framework offers a set of tools and agents that can be combined to build AI applications. It depends on the Instructor package and supports various APIs like OpenAI, Cohere, Anthropic, and Gemini. Atomic Agents is suitable for developers looking to create AI agents with a focus on modularity and flexibility.
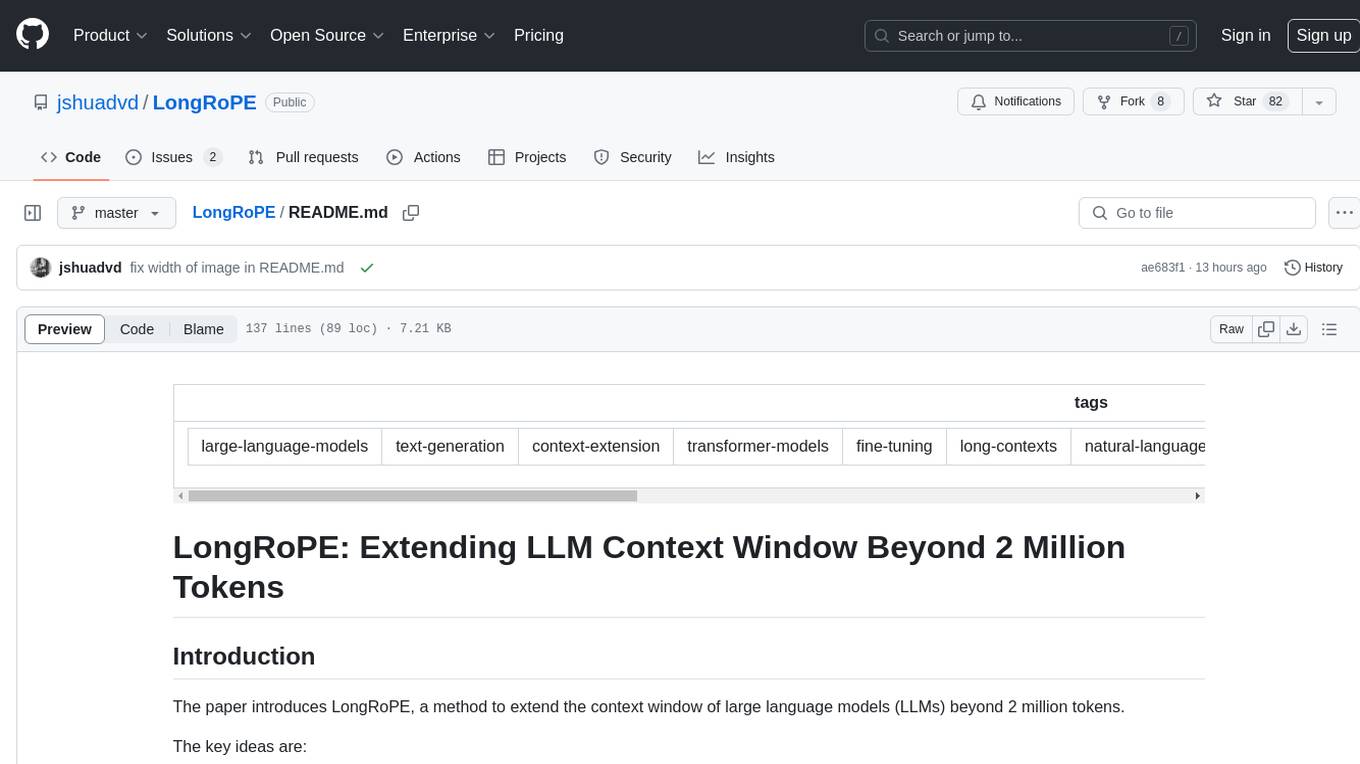
LongRoPE
LongRoPE is a method to extend the context window of large language models (LLMs) beyond 2 million tokens. It identifies and exploits non-uniformities in positional embeddings to enable 8x context extension without fine-tuning. The method utilizes a progressive extension strategy with 256k fine-tuning to reach a 2048k context. It adjusts embeddings for shorter contexts to maintain performance within the original window size. LongRoPE has been shown to be effective in maintaining performance across various tasks from 4k to 2048k context lengths.
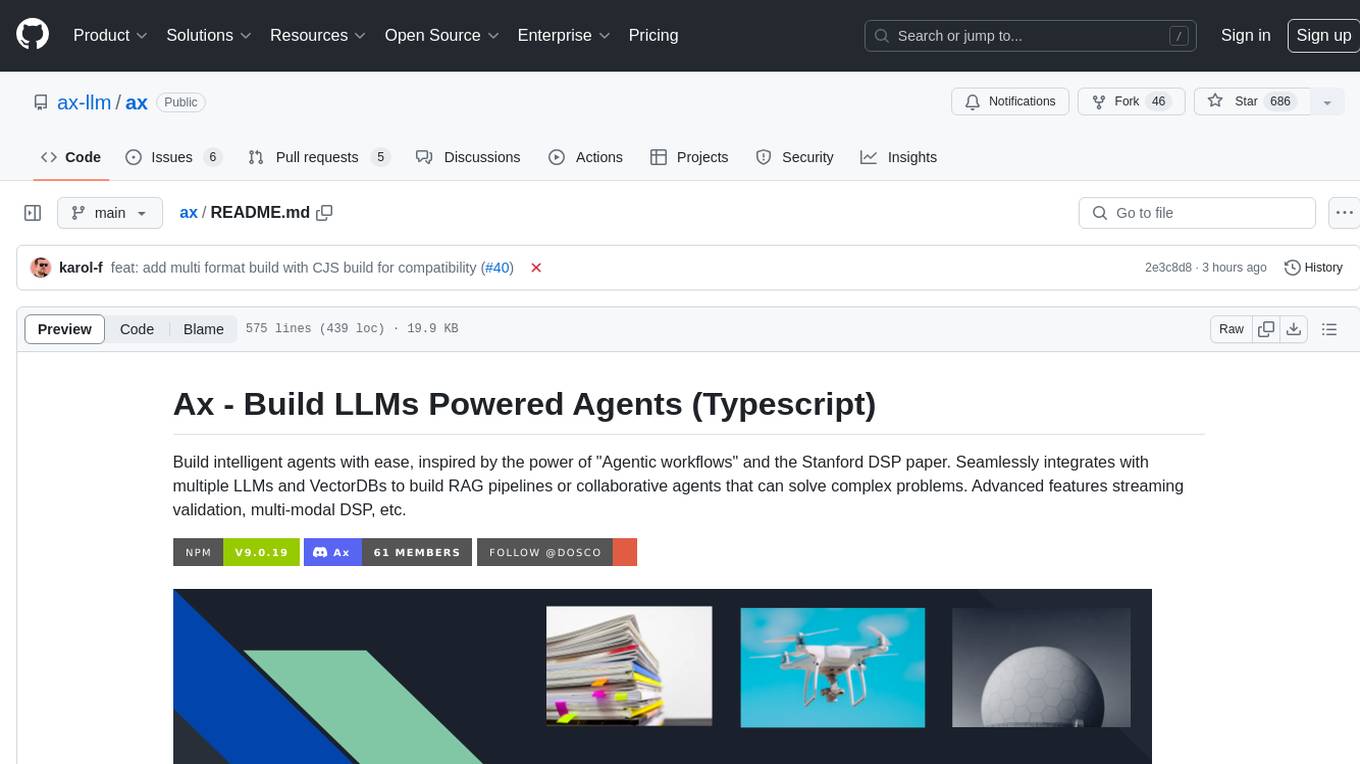
ax
Ax is a Typescript library that allows users to build intelligent agents inspired by agentic workflows and the Stanford DSP paper. It seamlessly integrates with multiple Large Language Models (LLMs) and VectorDBs to create RAG pipelines or collaborative agents capable of solving complex problems. The library offers advanced features such as streaming validation, multi-modal DSP, and automatic prompt tuning using optimizers. Users can easily convert documents of any format to text, perform smart chunking, embedding, and querying, and ensure output validation while streaming. Ax is production-ready, written in Typescript, and has zero dependencies.
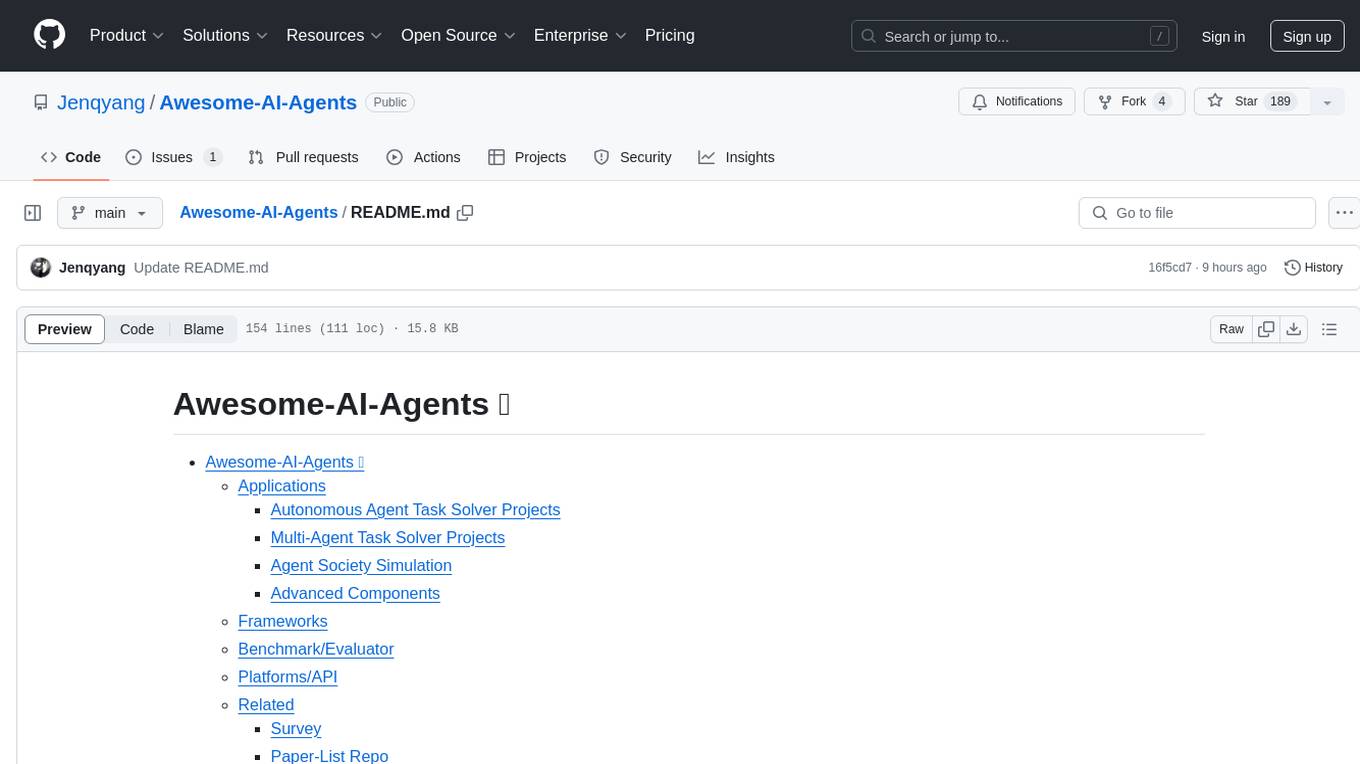
Awesome-AI-Agents
Awesome-AI-Agents is a curated list of projects, frameworks, benchmarks, platforms, and related resources focused on autonomous AI agents powered by Large Language Models (LLMs). The repository showcases a wide range of applications, multi-agent task solver projects, agent society simulations, and advanced components for building and customizing AI agents. It also includes frameworks for orchestrating role-playing, evaluating LLM-as-Agent performance, and connecting LLMs with real-world applications through platforms and APIs. Additionally, the repository features surveys, paper lists, and blogs related to LLM-based autonomous agents, making it a valuable resource for researchers, developers, and enthusiasts in the field of AI.
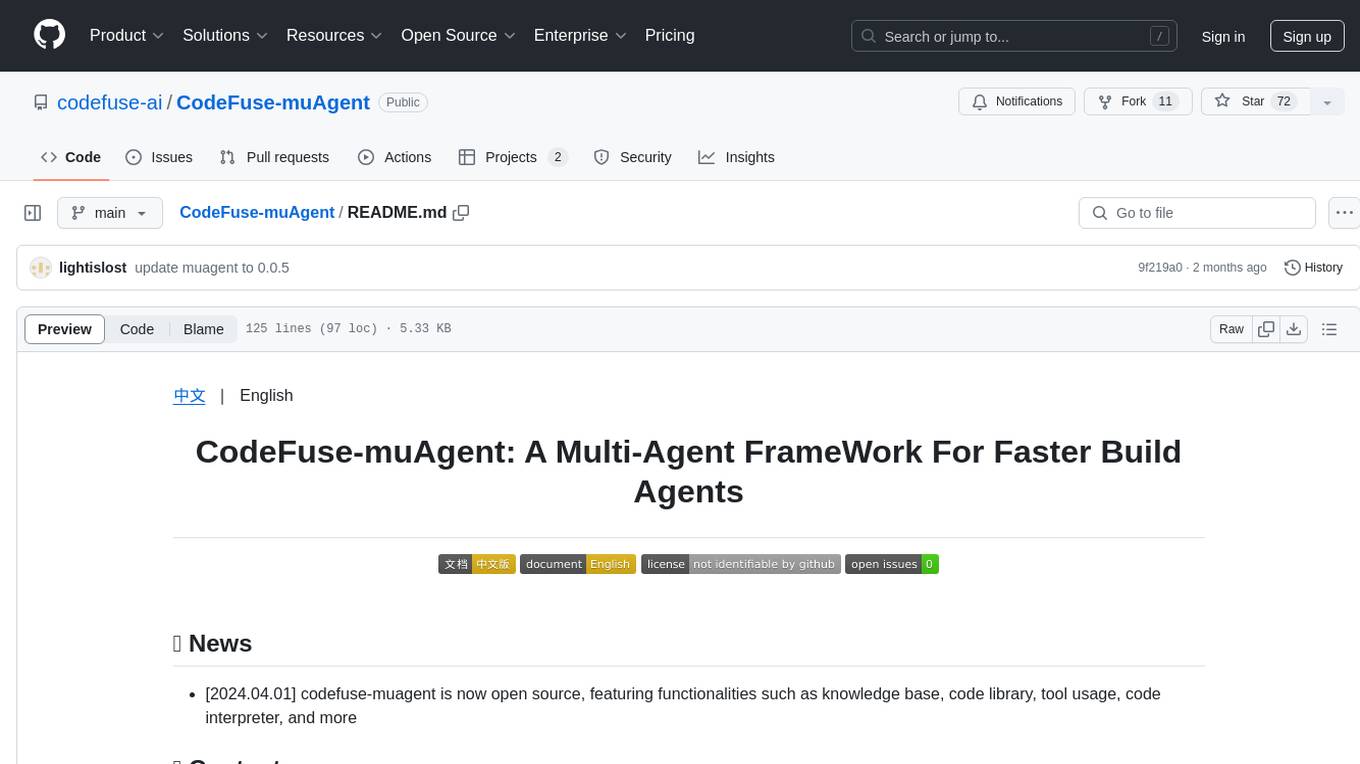
CodeFuse-muAgent
CodeFuse-muAgent is a Multi-Agent framework designed to streamline Standard Operating Procedure (SOP) orchestration for agents. It integrates toolkits, code libraries, knowledge bases, and sandbox environments for rapid construction of complex Multi-Agent interactive applications. The framework enables efficient execution and handling of multi-layered and multi-dimensional tasks.
For similar jobs
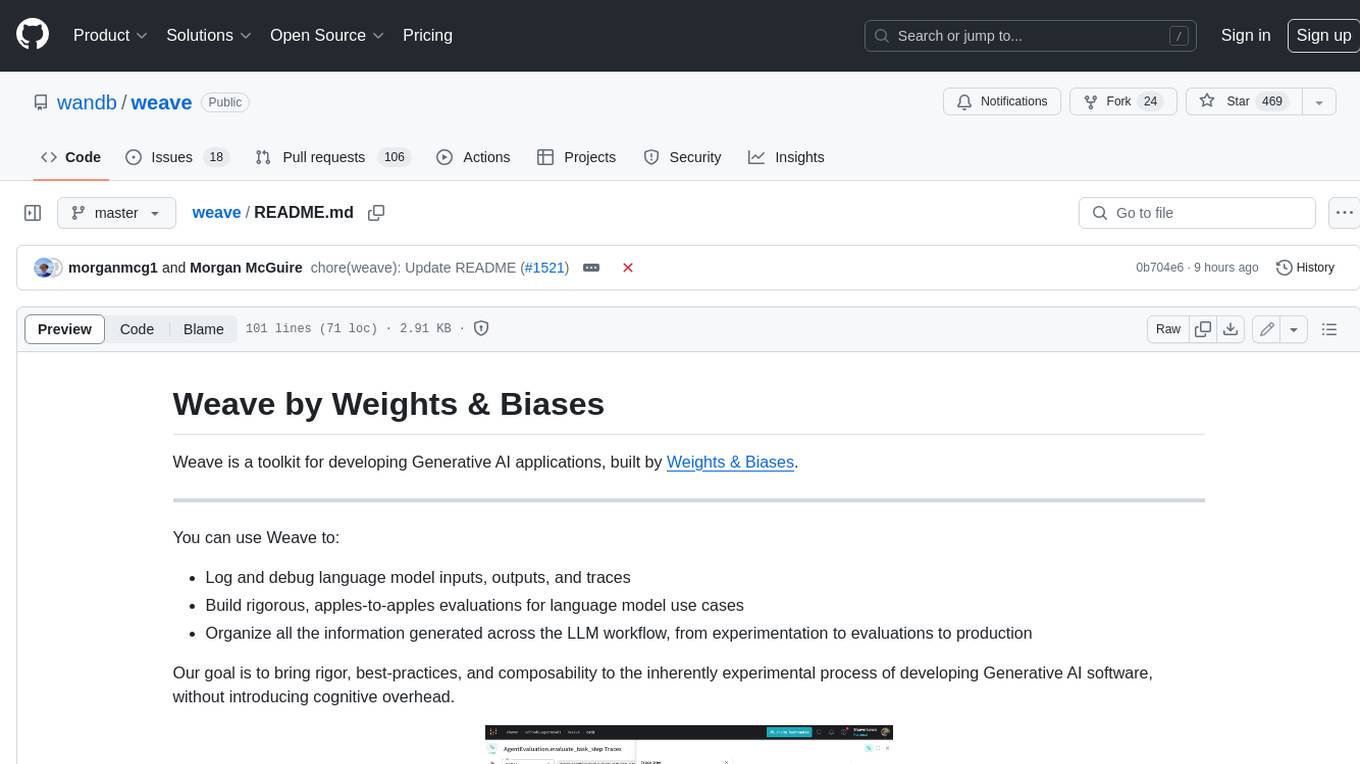
weave
Weave is a toolkit for developing Generative AI applications, built by Weights & Biases. With Weave, you can log and debug language model inputs, outputs, and traces; build rigorous, apples-to-apples evaluations for language model use cases; and organize all the information generated across the LLM workflow, from experimentation to evaluations to production. Weave aims to bring rigor, best-practices, and composability to the inherently experimental process of developing Generative AI software, without introducing cognitive overhead.
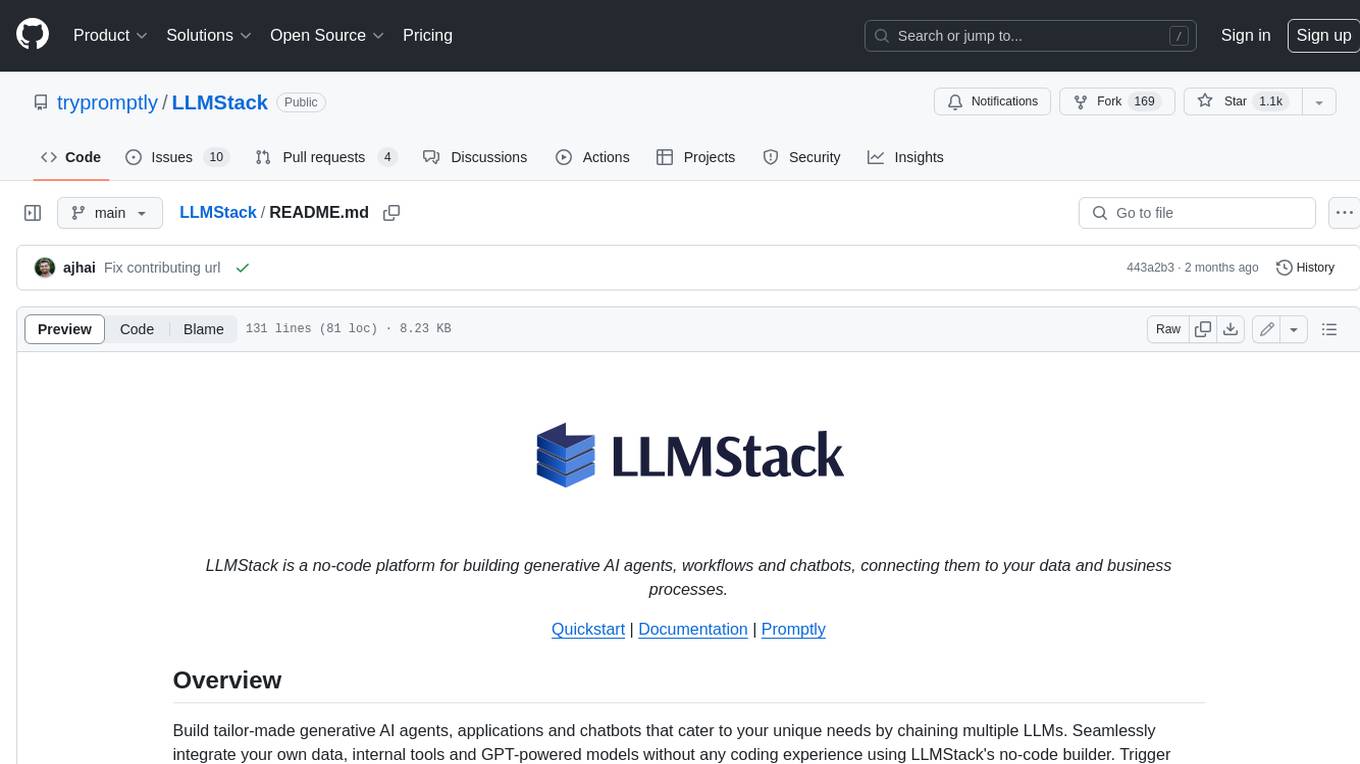
LLMStack
LLMStack is a no-code platform for building generative AI agents, workflows, and chatbots. It allows users to connect their own data, internal tools, and GPT-powered models without any coding experience. LLMStack can be deployed to the cloud or on-premise and can be accessed via HTTP API or triggered from Slack or Discord.
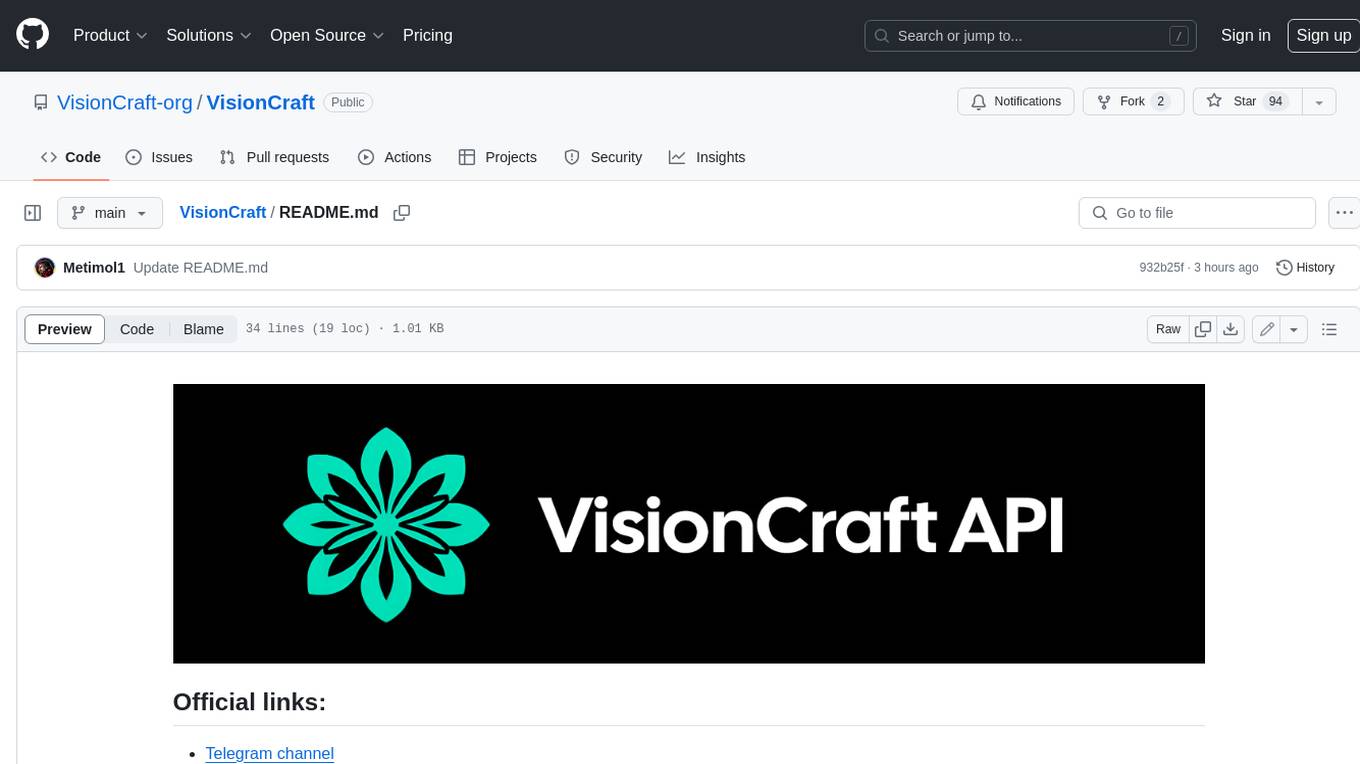
VisionCraft
The VisionCraft API is a free API for using over 100 different AI models. From images to sound.
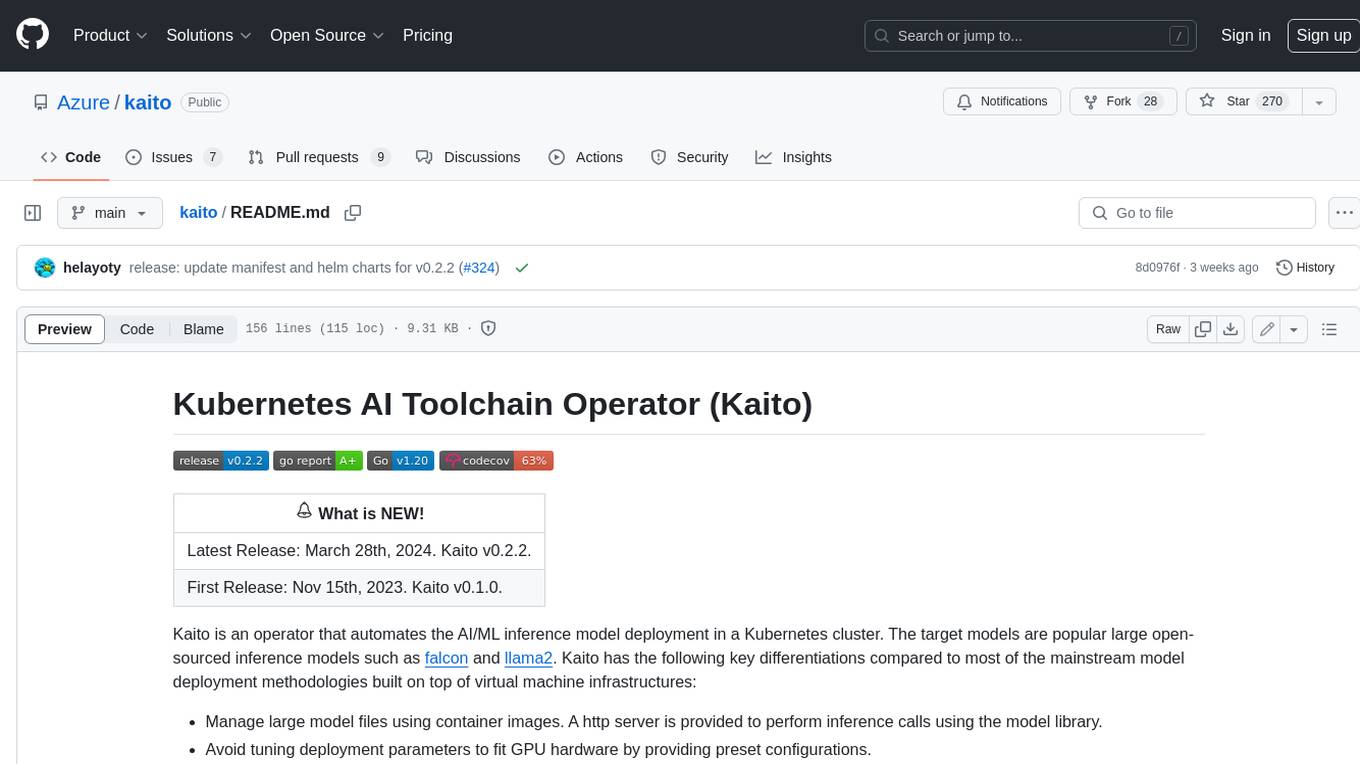
kaito
Kaito is an operator that automates the AI/ML inference model deployment in a Kubernetes cluster. It manages large model files using container images, avoids tuning deployment parameters to fit GPU hardware by providing preset configurations, auto-provisions GPU nodes based on model requirements, and hosts large model images in the public Microsoft Container Registry (MCR) if the license allows. Using Kaito, the workflow of onboarding large AI inference models in Kubernetes is largely simplified.
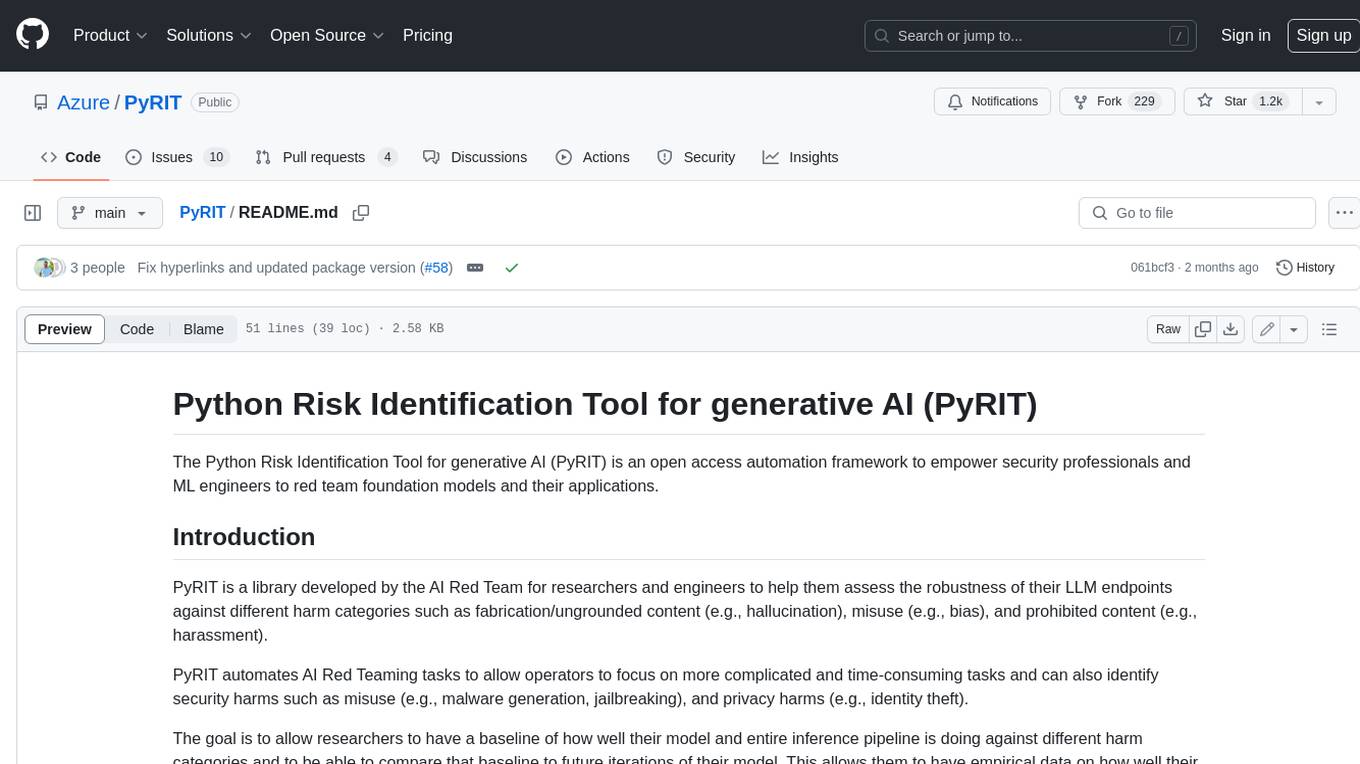
PyRIT
PyRIT is an open access automation framework designed to empower security professionals and ML engineers to red team foundation models and their applications. It automates AI Red Teaming tasks to allow operators to focus on more complicated and time-consuming tasks and can also identify security harms such as misuse (e.g., malware generation, jailbreaking), and privacy harms (e.g., identity theft). The goal is to allow researchers to have a baseline of how well their model and entire inference pipeline is doing against different harm categories and to be able to compare that baseline to future iterations of their model. This allows them to have empirical data on how well their model is doing today, and detect any degradation of performance based on future improvements.
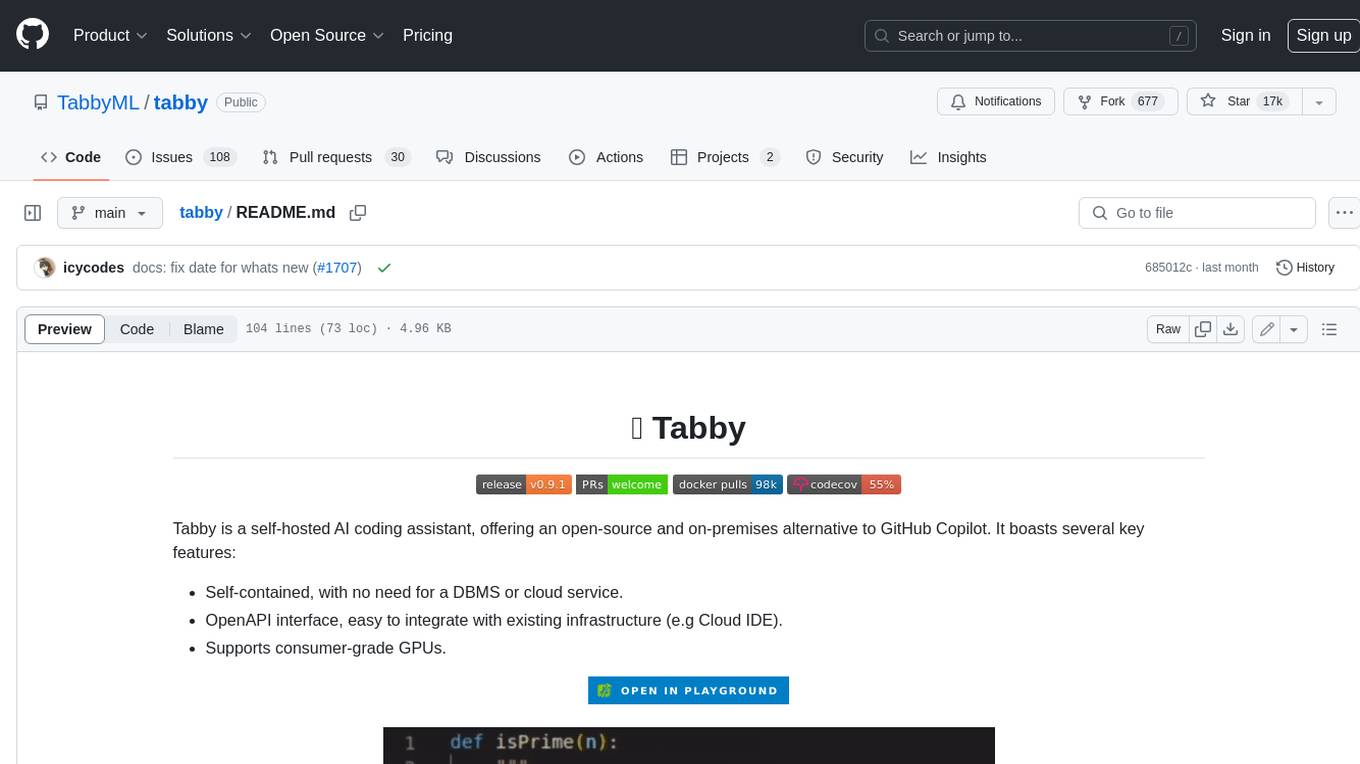
tabby
Tabby is a self-hosted AI coding assistant, offering an open-source and on-premises alternative to GitHub Copilot. It boasts several key features: * Self-contained, with no need for a DBMS or cloud service. * OpenAPI interface, easy to integrate with existing infrastructure (e.g Cloud IDE). * Supports consumer-grade GPUs.
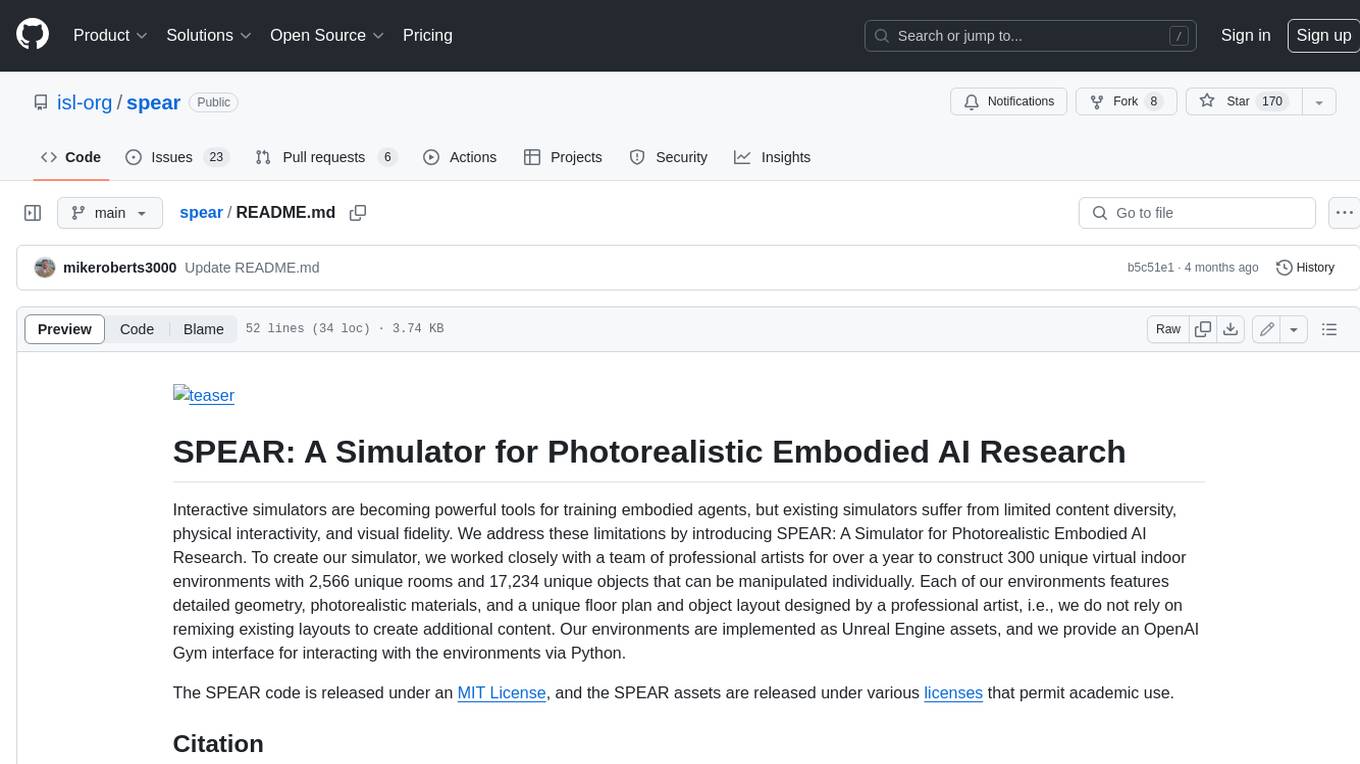
spear
SPEAR (Simulator for Photorealistic Embodied AI Research) is a powerful tool for training embodied agents. It features 300 unique virtual indoor environments with 2,566 unique rooms and 17,234 unique objects that can be manipulated individually. Each environment is designed by a professional artist and features detailed geometry, photorealistic materials, and a unique floor plan and object layout. SPEAR is implemented as Unreal Engine assets and provides an OpenAI Gym interface for interacting with the environments via Python.
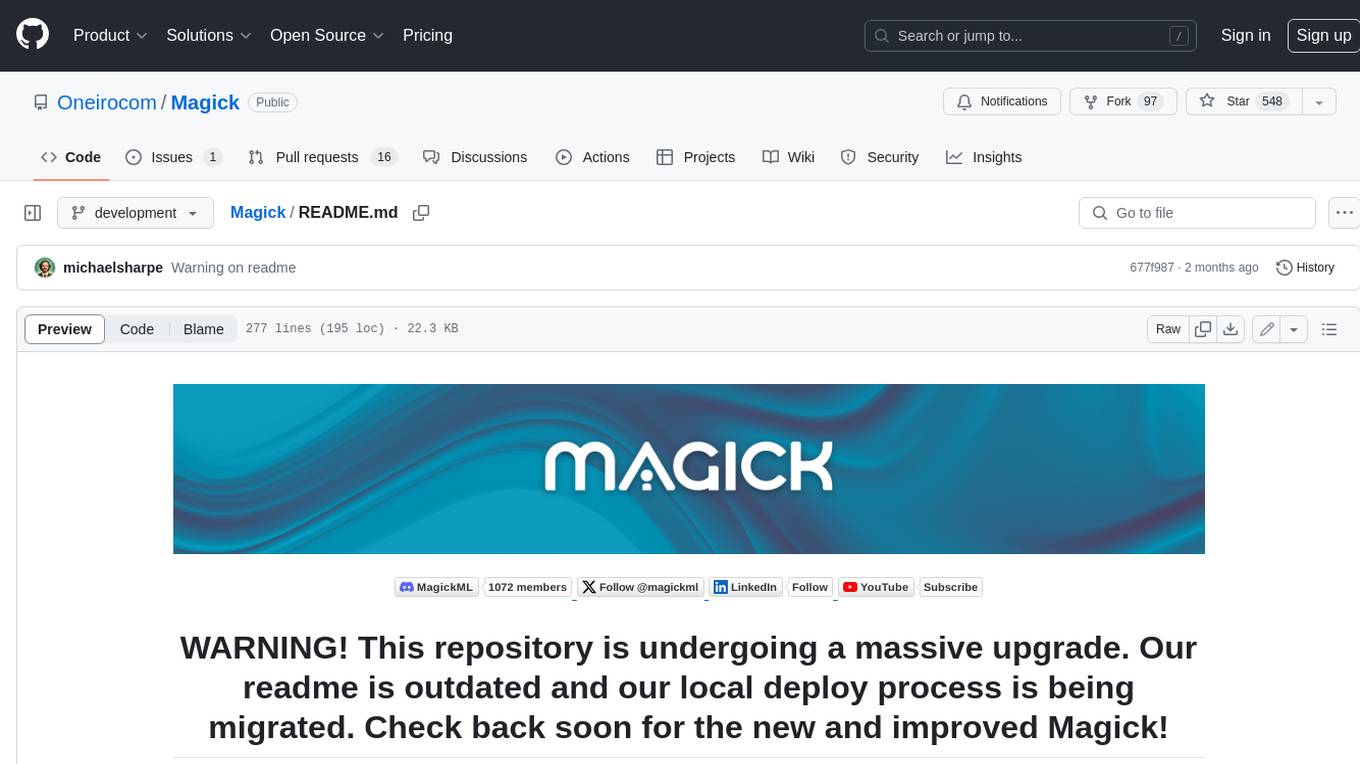
Magick
Magick is a groundbreaking visual AIDE (Artificial Intelligence Development Environment) for no-code data pipelines and multimodal agents. Magick can connect to other services and comes with nodes and templates well-suited for intelligent agents, chatbots, complex reasoning systems and realistic characters.