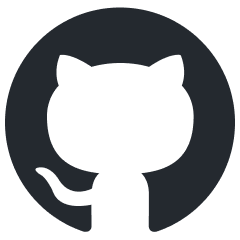
recommenders
Best Practices on Recommendation Systems
Stars: 19610
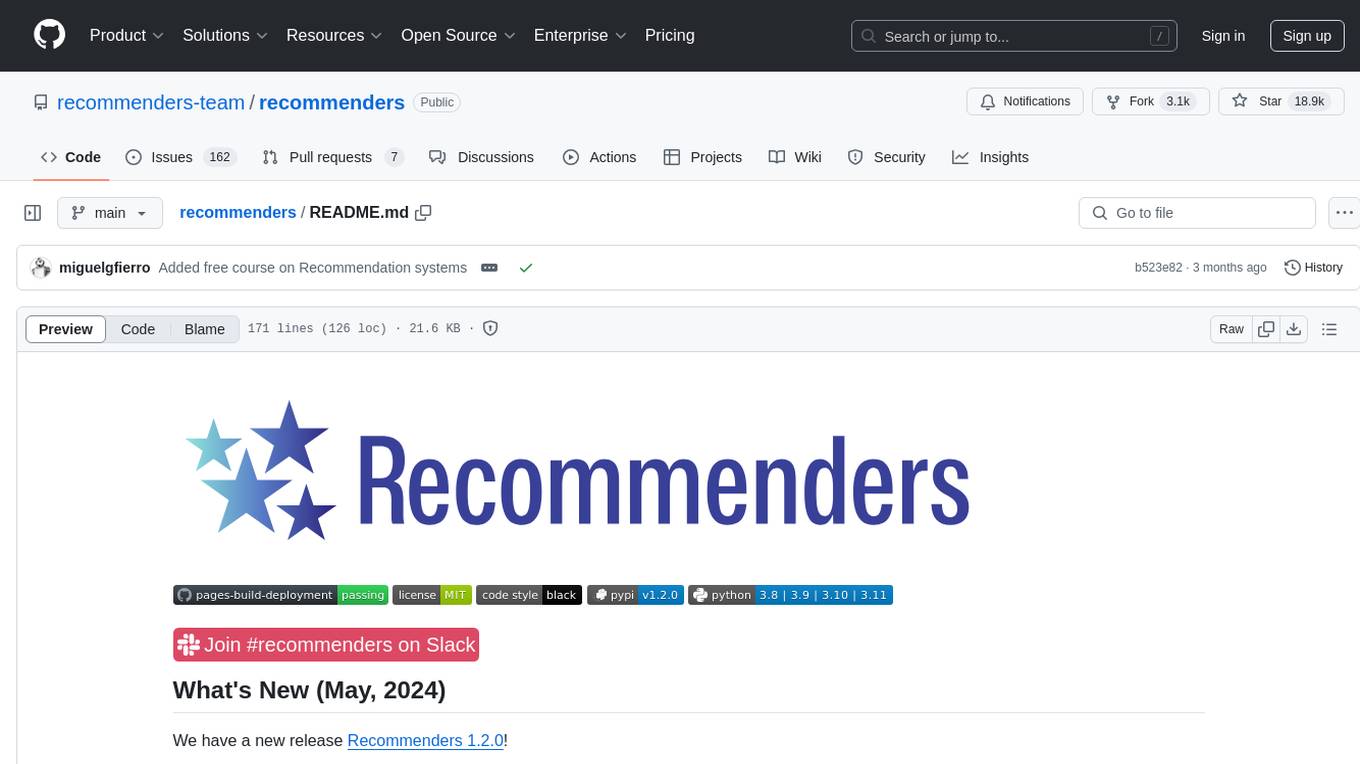
Recommenders is a project under the Linux Foundation of AI and Data that assists researchers, developers, and enthusiasts in prototyping, experimenting with, and bringing to production a range of classic and state-of-the-art recommendation systems. The repository contains examples and best practices for building recommendation systems, provided as Jupyter notebooks. It covers tasks such as preparing data, building models using various recommendation algorithms, evaluating algorithms, tuning hyperparameters, and operationalizing models in a production environment on Azure. The project provides utilities to support common tasks like loading datasets, evaluating model outputs, and splitting training/test data. It includes implementations of state-of-the-art algorithms for self-study and customization in applications.
README:
We have a new release Recommenders 1.2.1!
We fixed a lot of bugs due to dependencies, improved security, reviewed the notebooks and the libraries.
Recommenders objective is to assist researchers, developers and enthusiasts in prototyping, experimenting with and bringing to production a range of classic and state-of-the-art recommendation systems.
Recommenders is a project under the Linux Foundation of AI and Data.
This repository contains examples and best practices for building recommendation systems, provided as Jupyter notebooks. The examples detail our learnings on five key tasks:
- Prepare Data: Preparing and loading data for each recommendation algorithm.
- Model: Building models using various classical and deep learning recommendation algorithms such as Alternating Least Squares (ALS) or eXtreme Deep Factorization Machines (xDeepFM).
- Evaluate: Evaluating algorithms with offline metrics.
- Model Select and Optimize: Tuning and optimizing hyperparameters for recommendation models.
- Operationalize: Operationalizing models in a production environment on Azure.
Several utilities are provided in recommenders to support common tasks such as loading datasets in the format expected by different algorithms, evaluating model outputs, and splitting training/test data. Implementations of several state-of-the-art algorithms are included for self-study and customization in your own applications. See the Recommenders documentation.
For a more detailed overview of the repository, please see the documents on the wiki page.
For some of the practical scenarios where recommendation systems have been applied, see scenarios.
We recommend conda for environment management, and VS Code for development. To install the recommenders package and run an example notebook on Linux/WSL:
# 1. Install gcc if it is not installed already. On Ubuntu, this could done by using the command
# sudo apt install gcc
# 2. Create and activate a new conda environment
conda create -n <environment_name> python=3.9
conda activate <environment_name>
# 3. Install the core recommenders package. It can run all the CPU notebooks.
pip install recommenders
# 4. create a Jupyter kernel
python -m ipykernel install --user --name <environment_name> --display-name <kernel_name>
# 5. Clone this repo within VSCode or using command line:
git clone https://github.com/recommenders-team/recommenders.git
# 6. Within VSCode:
# a. Open a notebook, e.g., examples/00_quick_start/sar_movielens.ipynb;
# b. Select Jupyter kernel <kernel_name>;
# c. Run the notebook.
For more information about setup on other platforms (e.g., Windows and macOS) and different configurations (e.g., GPU, Spark and experimental features), see the Setup Guide.
In addition to the core package, several extras are also provided, including:
-
[gpu]
: Needed for running GPU models. -
[spark]
: Needed for running Spark models. -
[dev]
: Needed for development for the repo. -
[all]
:[gpu]
|[spark]
|[dev]
-
[experimental]
: Models that are not thoroughly tested and/or may require additional steps in installation.
The table below lists the recommendation algorithms currently available in the repository. Notebooks are linked under the Example column as Quick start, showcasing an easy to run example of the algorithm, or as Deep dive, explaining in detail the math and implementation of the algorithm.
Algorithm | Type | Description | Example |
---|---|---|---|
Alternating Least Squares (ALS) | Collaborative Filtering | Matrix factorization algorithm for explicit or implicit feedback in large datasets, optimized for scalability and distributed computing capability. It works in the PySpark environment. | Quick start / Deep dive |
Attentive Asynchronous Singular Value Decomposition (A2SVD)* | Collaborative Filtering | Sequential-based algorithm that aims to capture both long and short-term user preferences using attention mechanism. It works in the CPU/GPU environment. | Quick start |
Cornac/Bayesian Personalized Ranking (BPR) | Collaborative Filtering | Matrix factorization algorithm for predicting item ranking with implicit feedback. It works in the CPU environment. | Deep dive |
Cornac/Bilateral Variational Autoencoder (BiVAE) | Collaborative Filtering | Generative model for dyadic data (e.g., user-item interactions). It works in the CPU/GPU environment. | Deep dive |
Convolutional Sequence Embedding Recommendation (Caser) | Collaborative Filtering | Algorithm based on convolutions that aim to capture both user’s general preferences and sequential patterns. It works in the CPU/GPU environment. | Quick start |
Deep Knowledge-Aware Network (DKN)* | Content-Based Filtering | Deep learning algorithm incorporating a knowledge graph and article embeddings for providing news or article recommendations. It works in the CPU/GPU environment. | Quick start / Deep dive |
Extreme Deep Factorization Machine (xDeepFM)* | Collaborative Filtering | Deep learning based algorithm for implicit and explicit feedback with user/item features. It works in the CPU/GPU environment. | Quick start |
FastAI Embedding Dot Bias (FAST) | Collaborative Filtering | General purpose algorithm with embeddings and biases for users and items. It works in the CPU/GPU environment. | Quick start |
LightFM/Factorization Machine | Collaborative Filtering | Factorization Machine algorithm for both implicit and explicit feedbacks. It works in the CPU environment. | Quick start |
LightGBM/Gradient Boosting Tree* | Content-Based Filtering | Gradient Boosting Tree algorithm for fast training and low memory usage in content-based problems. It works in the CPU/GPU/PySpark environments. | Quick start in CPU / Deep dive in PySpark |
LightGCN | Collaborative Filtering | Deep learning algorithm which simplifies the design of GCN for predicting implicit feedback. It works in the CPU/GPU environment. | Deep dive |
GeoIMC* | Collaborative Filtering | Matrix completion algorithm that takes into account user and item features using Riemannian conjugate gradient optimization and follows a geometric approach. It works in the CPU environment. | Quick start |
GRU | Collaborative Filtering | Sequential-based algorithm that aims to capture both long and short-term user preferences using recurrent neural networks. It works in the CPU/GPU environment. | Quick start |
Multinomial VAE | Collaborative Filtering | Generative model for predicting user/item interactions. It works in the CPU/GPU environment. | Deep dive |
Neural Recommendation with Long- and Short-term User Representations (LSTUR)* | Content-Based Filtering | Neural recommendation algorithm for recommending news articles with long- and short-term user interest modeling. It works in the CPU/GPU environment. | Quick start |
Neural Recommendation with Attentive Multi-View Learning (NAML)* | Content-Based Filtering | Neural recommendation algorithm for recommending news articles with attentive multi-view learning. It works in the CPU/GPU environment. | Quick start |
Neural Collaborative Filtering (NCF) | Collaborative Filtering | Deep learning algorithm with enhanced performance for user/item implicit feedback. It works in the CPU/GPU environment. | Quick start / Deep dive |
Neural Recommendation with Personalized Attention (NPA)* | Content-Based Filtering | Neural recommendation algorithm for recommending news articles with personalized attention network. It works in the CPU/GPU environment. | Quick start |
Neural Recommendation with Multi-Head Self-Attention (NRMS)* | Content-Based Filtering | Neural recommendation algorithm for recommending news articles with multi-head self-attention. It works in the CPU/GPU environment. | Quick start |
Next Item Recommendation (NextItNet) | Collaborative Filtering | Algorithm based on dilated convolutions and residual network that aims to capture sequential patterns. It considers both user/item interactions and features. It works in the CPU/GPU environment. | Quick start |
Restricted Boltzmann Machines (RBM) | Collaborative Filtering | Neural network based algorithm for learning the underlying probability distribution for explicit or implicit user/item feedback. It works in the CPU/GPU environment. | Quick start / Deep dive |
Riemannian Low-rank Matrix Completion (RLRMC)* | Collaborative Filtering | Matrix factorization algorithm using Riemannian conjugate gradients optimization with small memory consumption to predict user/item interactions. It works in the CPU environment. | Quick start |
Simple Algorithm for Recommendation (SAR)* | Collaborative Filtering | Similarity-based algorithm for implicit user/item feedback. It works in the CPU environment. | Quick start / Deep dive |
Self-Attentive Sequential Recommendation (SASRec) | Collaborative Filtering | Transformer based algorithm for sequential recommendation. It works in the CPU/GPU environment. | Quick start |
Short-term and Long-term Preference Integrated Recommender (SLi-Rec)* | Collaborative Filtering | Sequential-based algorithm that aims to capture both long and short-term user preferences using attention mechanism, a time-aware controller and a content-aware controller. It works in the CPU/GPU environment. | Quick start |
Multi-Interest-Aware Sequential User Modeling (SUM)* | Collaborative Filtering | An enhanced memory network-based sequential user model which aims to capture users' multiple interests. It works in the CPU/GPU environment. | Quick start |
Sequential Recommendation Via Personalized Transformer (SSEPT) | Collaborative Filtering | Transformer based algorithm for sequential recommendation with User embedding. It works in the CPU/GPU environment. | Quick start |
Standard VAE | Collaborative Filtering | Generative Model for predicting user/item interactions. It works in the CPU/GPU environment. | Deep dive |
Surprise/Singular Value Decomposition (SVD) | Collaborative Filtering | Matrix factorization algorithm for predicting explicit rating feedback in small datasets. It works in the CPU/GPU environment. | Deep dive |
Term Frequency - Inverse Document Frequency (TF-IDF) | Content-Based Filtering | Simple similarity-based algorithm for content-based recommendations with text datasets. It works in the CPU environment. | Quick start |
Vowpal Wabbit (VW)* | Content-Based Filtering | Fast online learning algorithms, great for scenarios where user features / context are constantly changing. It uses the CPU for online learning. | Deep dive |
Wide and Deep | Collaborative Filtering | Deep learning algorithm that can memorize feature interactions and generalize user features. It works in the CPU/GPU environment. | Quick start |
xLearn/Factorization Machine (FM) & Field-Aware FM (FFM) | Collaborative Filtering | Quick and memory efficient algorithm to predict labels with user/item features. It works in the CPU/GPU environment. | Deep dive |
NOTE: * indicates algorithms invented/contributed by Microsoft.
Independent or incubating algorithms and utilities are candidates for the contrib folder. This will house contributions which may not easily fit into the core repository or need time to refactor or mature the code and add necessary tests.
Algorithm | Type | Description | Example |
---|---|---|---|
SARplus * | Collaborative Filtering | Optimized implementation of SAR for Spark | Quick start |
We provide a benchmark notebook to illustrate how different algorithms could be evaluated and compared. In this notebook, the MovieLens dataset is split into training/test sets at a 75/25 ratio using a stratified split. A recommendation model is trained using each of the collaborative filtering algorithms below. We utilize empirical parameter values reported in literature here. For ranking metrics we use k=10
(top 10 recommended items). We run the comparison on a Standard NC6s_v2 Azure DSVM (6 vCPUs, 112 GB memory and 1 P100 GPU). Spark ALS is run in local standalone mode. In this table we show the results on Movielens 100k, running the algorithms for 15 epochs.
Algo | MAP | nDCG@k | Precision@k | Recall@k | RMSE | MAE | R2 | Explained Variance |
---|---|---|---|---|---|---|---|---|
ALS | 0.004732 | 0.044239 | 0.048462 | 0.017796 | 0.965038 | 0.753001 | 0.255647 | 0.251648 |
BiVAE | 0.146126 | 0.475077 | 0.411771 | 0.219145 | N/A | N/A | N/A | N/A |
BPR | 0.132478 | 0.441997 | 0.388229 | 0.212522 | N/A | N/A | N/A | N/A |
FastAI | 0.025503 | 0.147866 | 0.130329 | 0.053824 | 0.943084 | 0.744337 | 0.285308 | 0.287671 |
LightGCN | 0.088526 | 0.419846 | 0.379626 | 0.144336 | N/A | N/A | N/A | N/A |
NCF | 0.107720 | 0.396118 | 0.347296 | 0.180775 | N/A | N/A | N/A | N/A |
SAR | 0.110591 | 0.382461 | 0.330753 | 0.176385 | 1.253805 | 1.048484 | -0.569363 | 0.030474 |
SVD | 0.012873 | 0.095930 | 0.091198 | 0.032783 | 0.938681 | 0.742690 | 0.291967 | 0.291971 |
This project welcomes contributions and suggestions. Before contributing, please see our contribution guidelines.
This project adheres to this Code of Conduct in order to foster a welcoming and inspiring community for all.
These tests are the nightly builds, which compute the asynchronous tests. main
is our principal branch and staging
is our development branch. We use pytest for testing python utilities in recommenders and the Recommenders notebook executor for the notebooks.
For more information about the testing pipelines, please see the test documentation.
The nightly build tests are run daily on AzureML.
Build Type | Branch | Status | Branch | Status | |
---|---|---|---|---|---|
Linux CPU | main | staging | |||
Linux GPU | main | staging | |||
Linux Spark | main | staging |
- FREE COURSE: M. González-Fierro, "Recommendation Systems: A Practical Introduction", LinkedIn Learning, 2024. Available on this link.
- D. Li, J. Lian, L. Zhang, K. Ren, D. Lu, T. Wu, X. Xie, "Recommender Systems: Frontiers and Practices", Springer, Beijing, 2024. Available on this link.
- A. Argyriou, M. González-Fierro, and L. Zhang, "Microsoft Recommenders: Best Practices for Production-Ready Recommendation Systems", WWW 2020: International World Wide Web Conference Taipei, 2020. Available online: https://dl.acm.org/doi/abs/10.1145/3366424.3382692
- S. Graham, J.K. Min, T. Wu, "Microsoft recommenders: tools to accelerate developing recommender systems", RecSys '19: Proceedings of the 13th ACM Conference on Recommender Systems, 2019. Available online: https://dl.acm.org/doi/10.1145/3298689.3346967
- L. Zhang, T. Wu, X. Xie, A. Argyriou, M. González-Fierro and J. Lian, "Building Production-Ready Recommendation System at Scale", ACM SIGKDD Conference on Knowledge Discovery and Data Mining 2019 (KDD 2019), 2019.
For Tasks:
Click tags to check more tools for each tasksFor Jobs:
Alternative AI tools for recommenders
Similar Open Source Tools
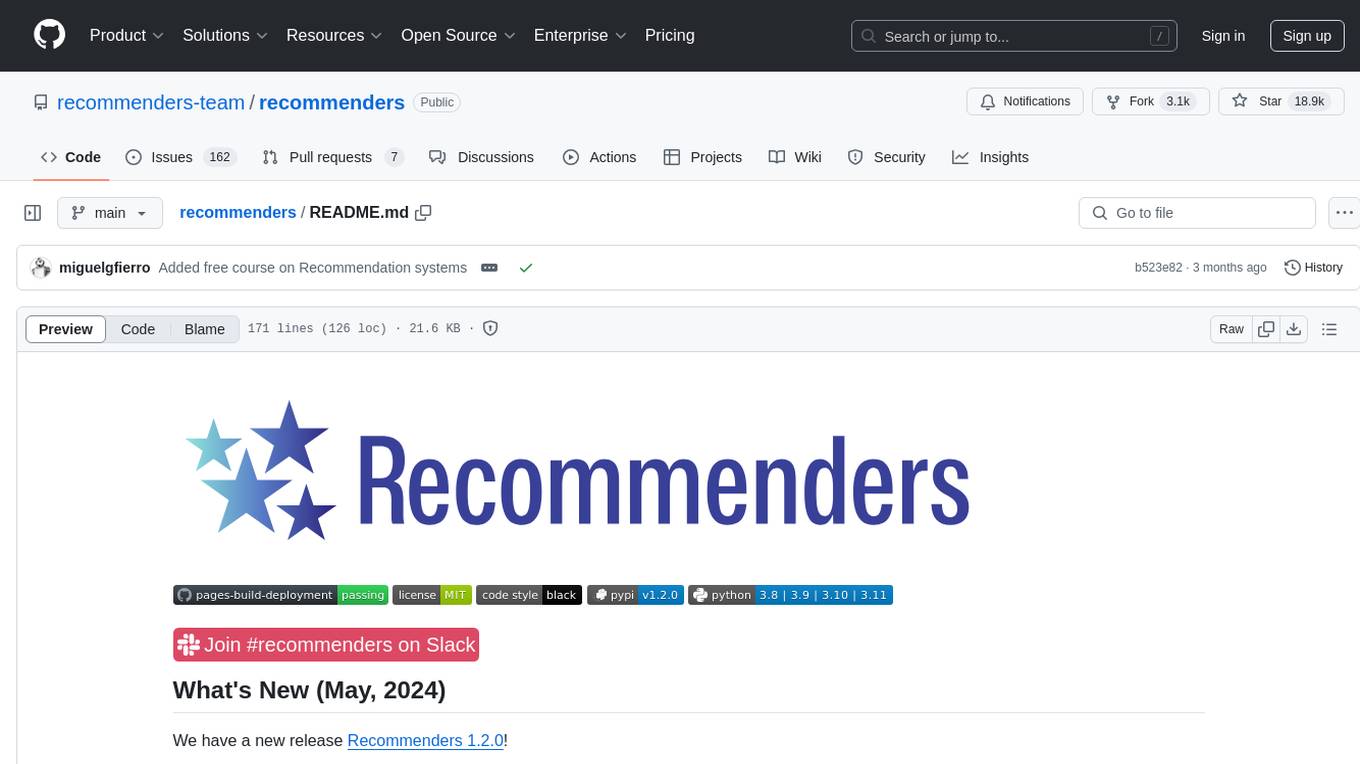
recommenders
Recommenders is a project under the Linux Foundation of AI and Data that assists researchers, developers, and enthusiasts in prototyping, experimenting with, and bringing to production a range of classic and state-of-the-art recommendation systems. The repository contains examples and best practices for building recommendation systems, provided as Jupyter notebooks. It covers tasks such as preparing data, building models using various recommendation algorithms, evaluating algorithms, tuning hyperparameters, and operationalizing models in a production environment on Azure. The project provides utilities to support common tasks like loading datasets, evaluating model outputs, and splitting training/test data. It includes implementations of state-of-the-art algorithms for self-study and customization in applications.
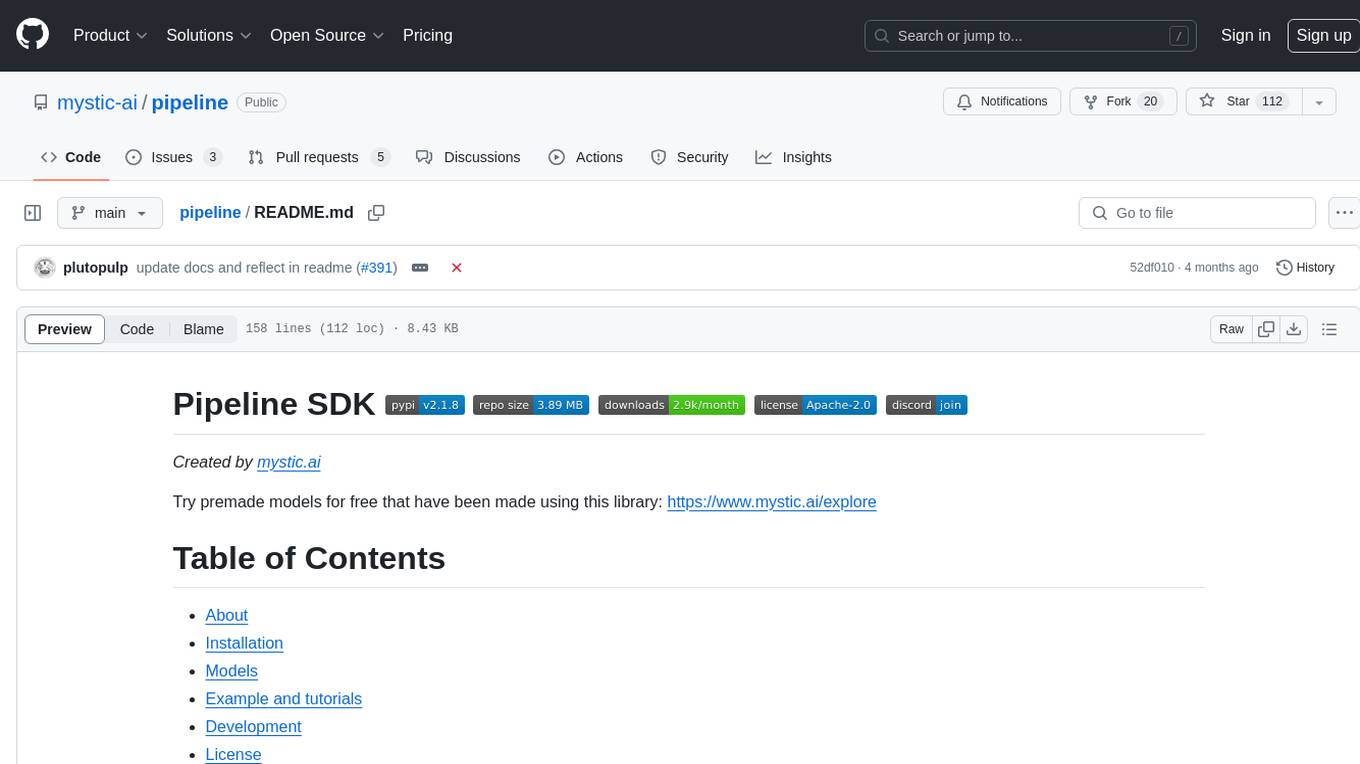
pipeline
Pipeline is a Python library designed for constructing computational flows for AI/ML models. It supports both development and production environments, offering capabilities for inference, training, and finetuning. The library serves as an interface to Mystic, enabling the execution of pipelines at scale and on enterprise GPUs. Users can also utilize this SDK with Pipeline Core on a private hosted cluster. The syntax for defining AI/ML pipelines is reminiscent of sessions in Tensorflow v1 and Flows in Prefect.
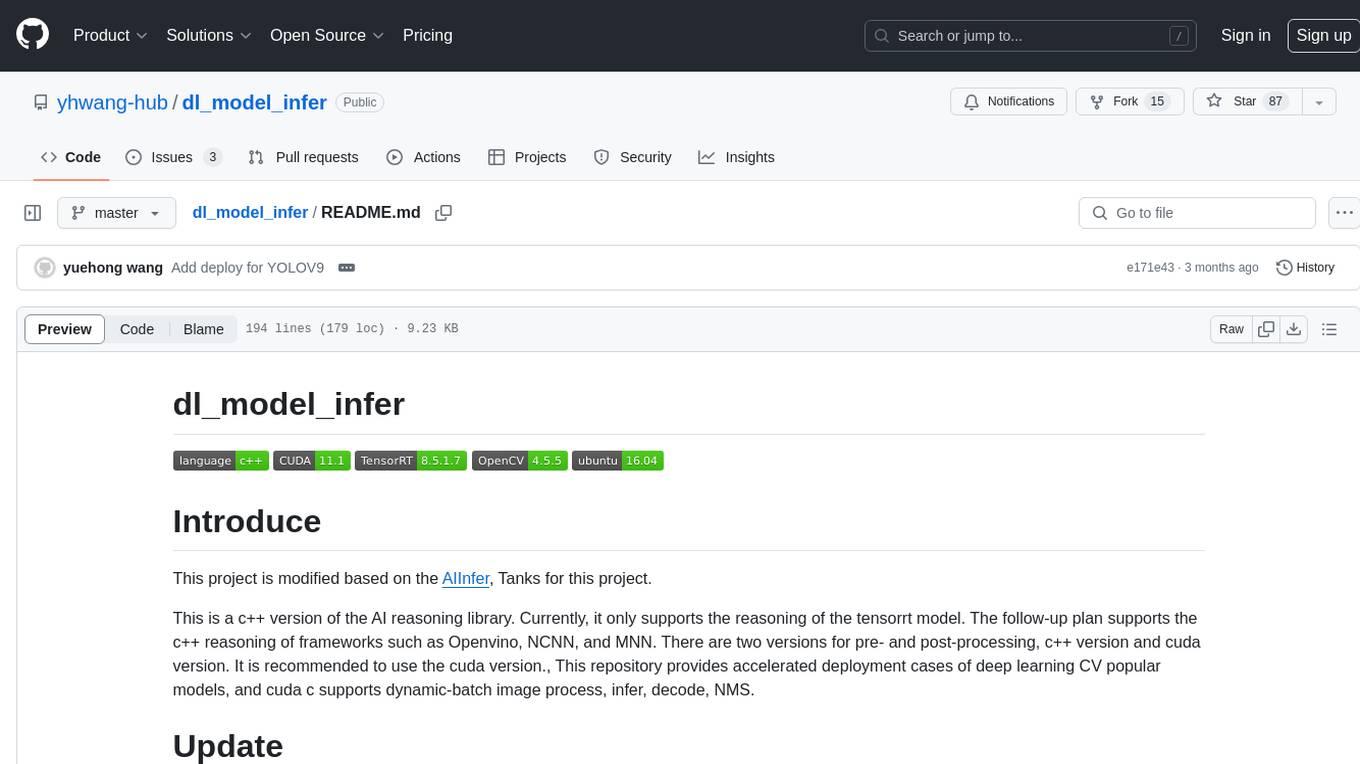
dl_model_infer
This project is a c++ version of the AI reasoning library that supports the reasoning of tensorrt models. It provides accelerated deployment cases of deep learning CV popular models and supports dynamic-batch image processing, inference, decode, and NMS. The project has been updated with various models and provides tutorials for model exports. It also includes a producer-consumer inference model for specific tasks. The project directory includes implementations for model inference applications, backend reasoning classes, post-processing, pre-processing, and target detection and tracking. Speed tests have been conducted on various models, and onnx downloads are available for different models.
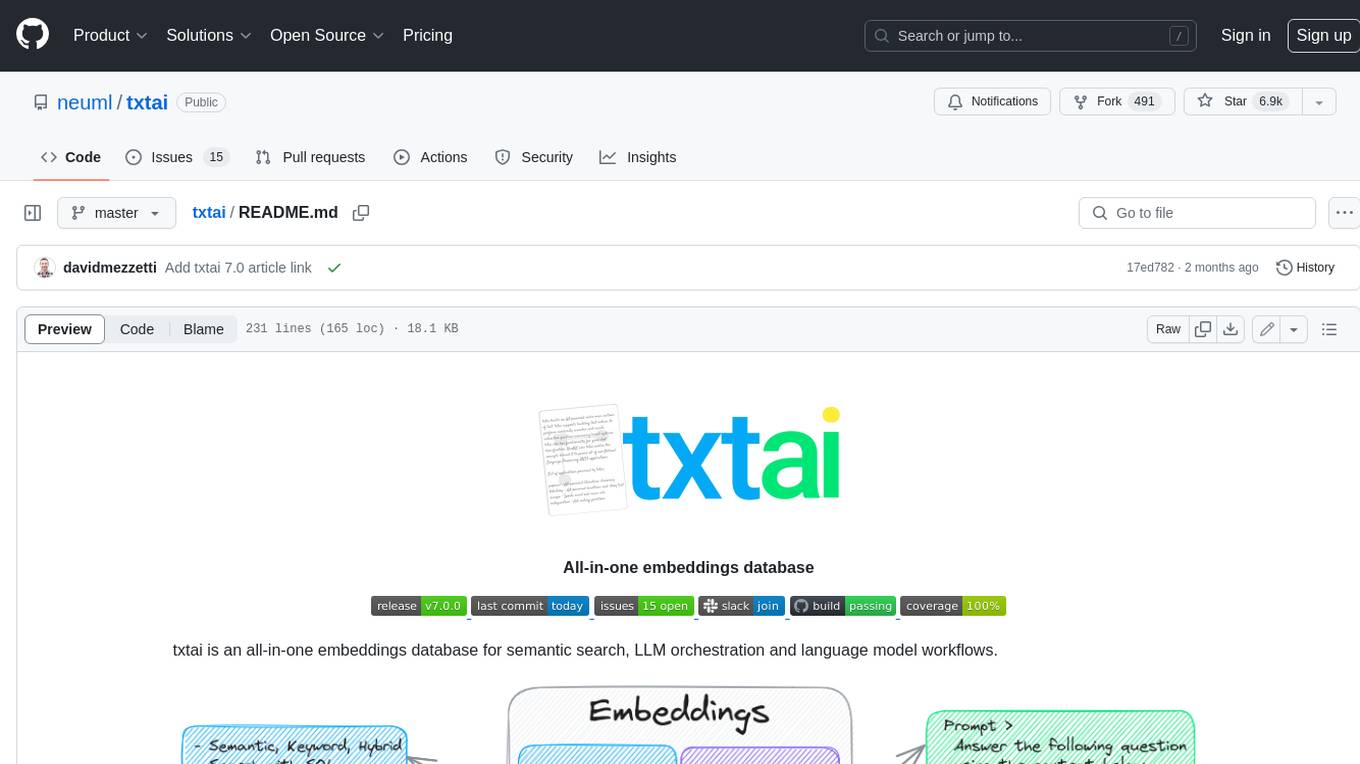
txtai
Txtai is an all-in-one embeddings database for semantic search, LLM orchestration, and language model workflows. It combines vector indexes, graph networks, and relational databases to enable vector search with SQL, topic modeling, retrieval augmented generation, and more. Txtai can stand alone or serve as a knowledge source for large language models (LLMs). Key features include vector search with SQL, object storage, topic modeling, graph analysis, multimodal indexing, embedding creation for various data types, pipelines powered by language models, workflows to connect pipelines, and support for Python, JavaScript, Java, Rust, and Go. Txtai is open-source under the Apache 2.0 license.
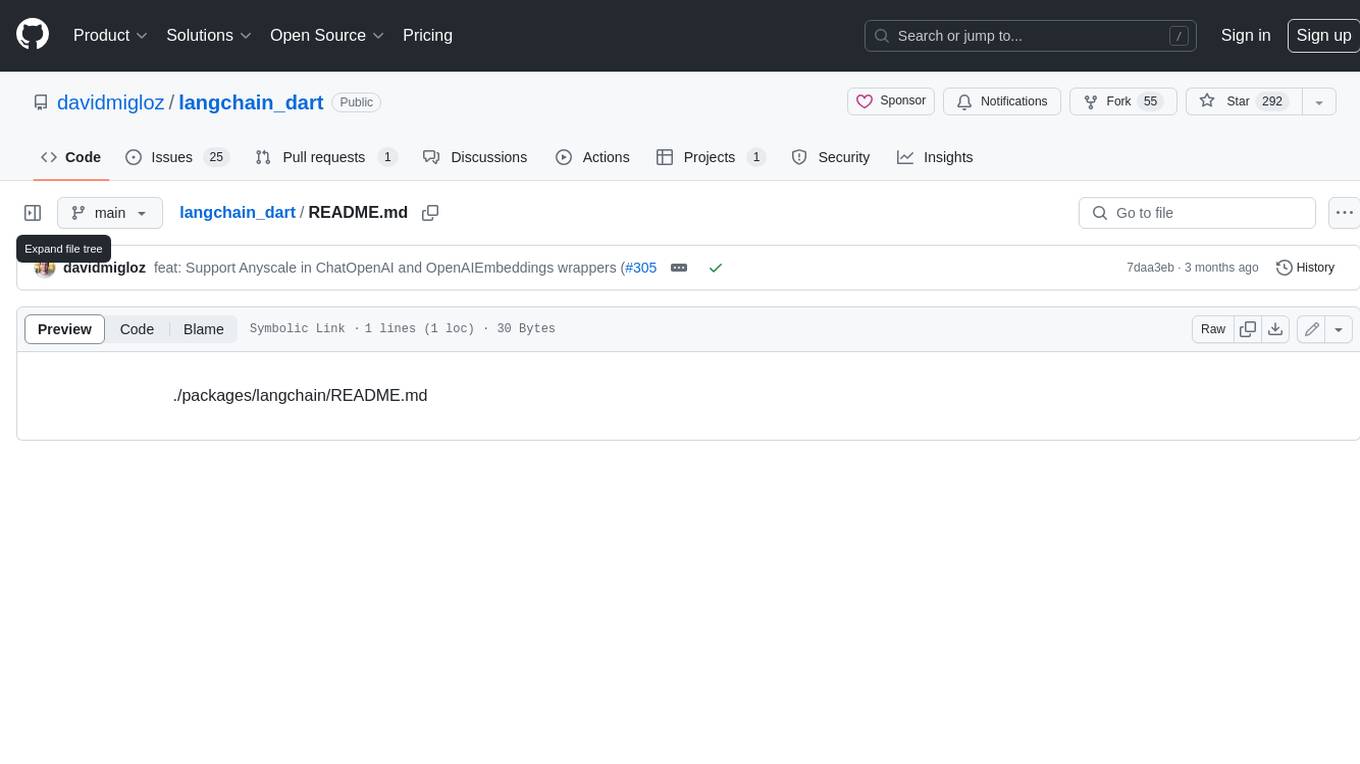
langchain_dart
LangChain.dart is a Dart port of the popular LangChain Python framework created by Harrison Chase. LangChain provides a set of ready-to-use components for working with language models and a standard interface for chaining them together to formulate more advanced use cases (e.g. chatbots, Q&A with RAG, agents, summarization, extraction, etc.). The components can be grouped into a few core modules: * **Model I/O:** LangChain offers a unified API for interacting with various LLM providers (e.g. OpenAI, Google, Mistral, Ollama, etc.), allowing developers to switch between them with ease. Additionally, it provides tools for managing model inputs (prompt templates and example selectors) and parsing the resulting model outputs (output parsers). * **Retrieval:** assists in loading user data (via document loaders), transforming it (with text splitters), extracting its meaning (using embedding models), storing (in vector stores) and retrieving it (through retrievers) so that it can be used to ground the model's responses (i.e. Retrieval-Augmented Generation or RAG). * **Agents:** "bots" that leverage LLMs to make informed decisions about which available tools (such as web search, calculators, database lookup, etc.) to use to accomplish the designated task. The different components can be composed together using the LangChain Expression Language (LCEL).
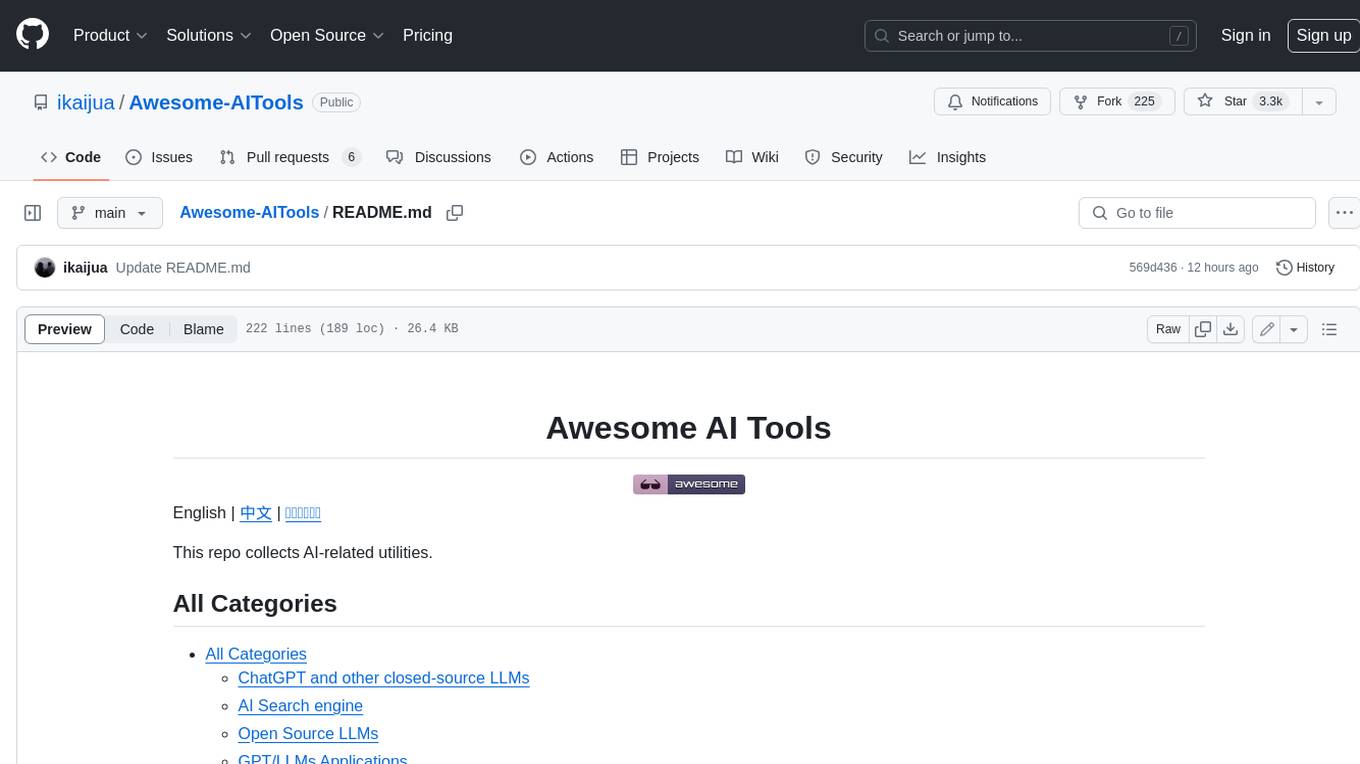
Awesome-AITools
This repo collects AI-related utilities. ## All Categories * All Categories * ChatGPT and other closed-source LLMs * AI Search engine * Open Source LLMs * GPT/LLMs Applications * LLM training platform * Applications that integrate multiple LLMs * AI Agent * Writing * Programming Development * Translation * AI Conversation or AI Voice Conversation * Image Creation * Speech Recognition * Text To Speech * Voice Processing * AI generated music or sound effects * Speech translation * Video Creation * Video Content Summary * OCR(Optical Character Recognition)
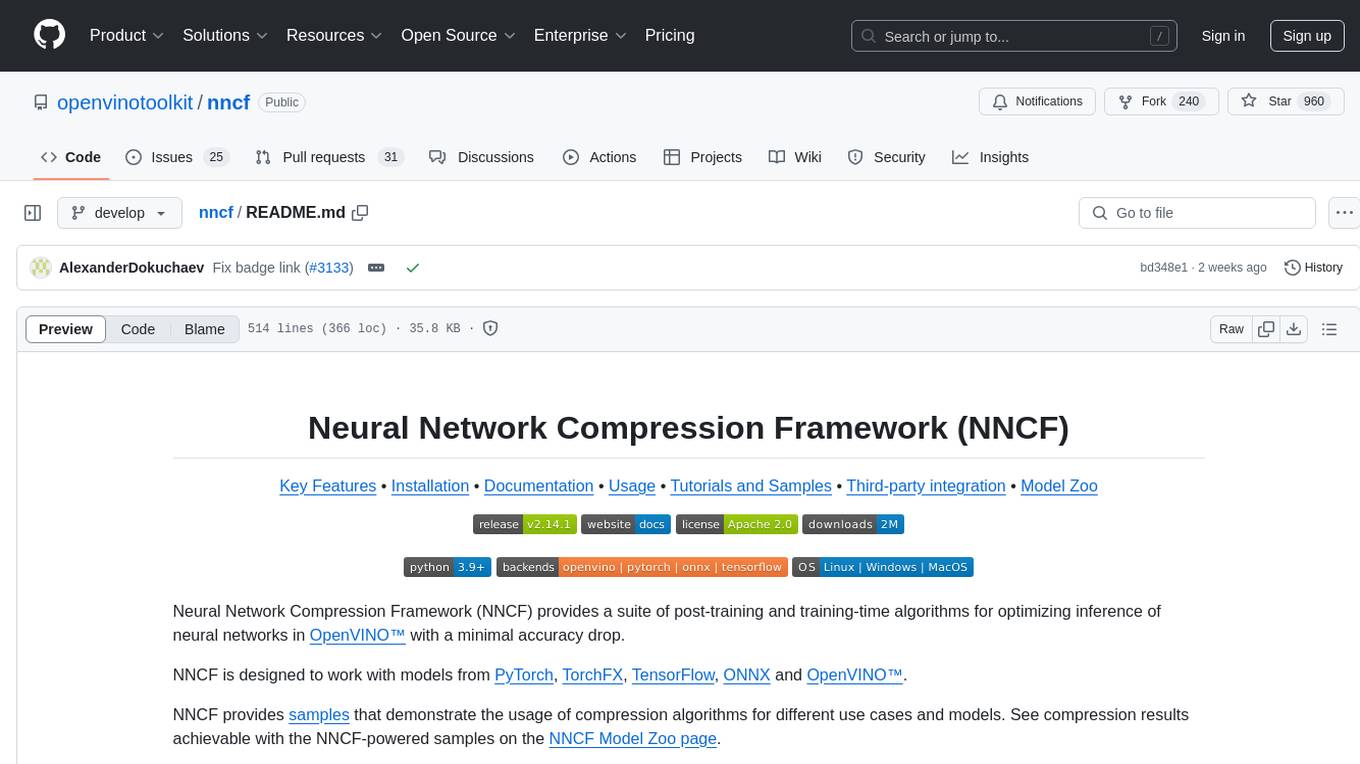
nncf
Neural Network Compression Framework (NNCF) provides a suite of post-training and training-time algorithms for optimizing inference of neural networks in OpenVINO™ with a minimal accuracy drop. It is designed to work with models from PyTorch, TorchFX, TensorFlow, ONNX, and OpenVINO™. NNCF offers samples demonstrating compression algorithms for various use cases and models, with the ability to add different compression algorithms easily. It supports GPU-accelerated layers, distributed training, and seamless combination of pruning, sparsity, and quantization algorithms. NNCF allows exporting compressed models to ONNX or TensorFlow formats for use with OpenVINO™ toolkit, and supports Accuracy-Aware model training pipelines via Adaptive Compression Level Training and Early Exit Training.
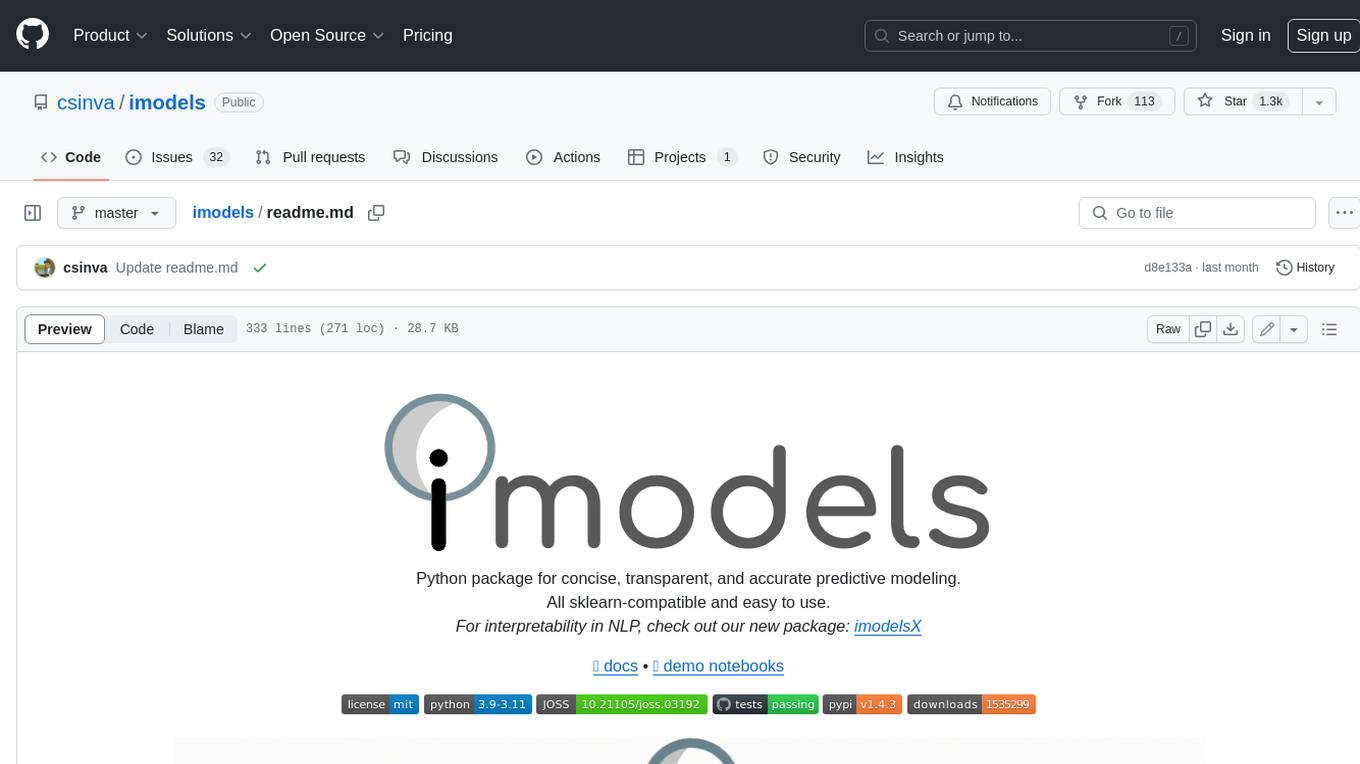
imodels
Python package for concise, transparent, and accurate predictive modeling. All sklearn-compatible and easy to use. _For interpretability in NLP, check out our new package:imodelsX _
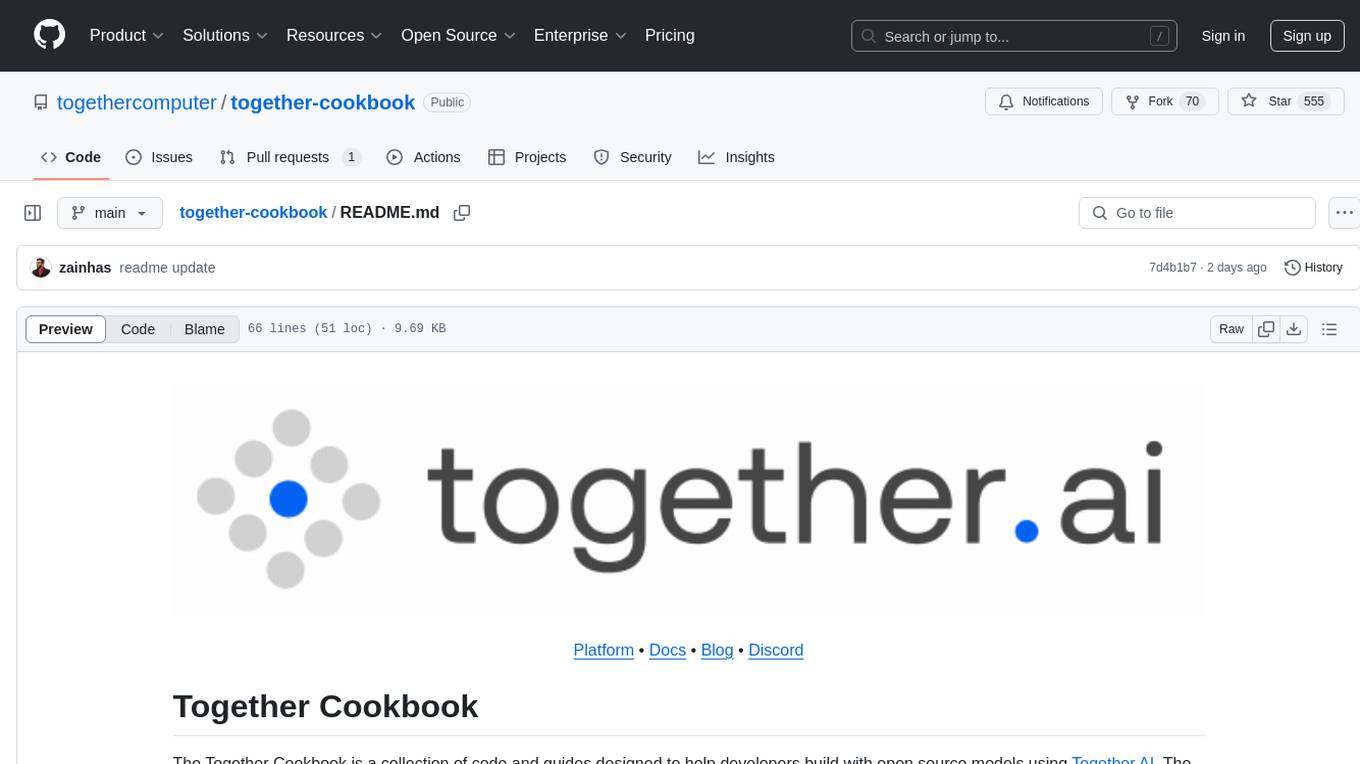
together-cookbook
The Together Cookbook is a collection of code and guides designed to help developers build with open source models using Together AI. The recipes provide examples on how to chain multiple LLM calls, create agents that route tasks to specialized models, run multiple LLMs in parallel, break down tasks into parallel subtasks, build agents that iteratively improve responses, perform LoRA fine-tuning and inference, fine-tune LLMs for repetition, improve summarization capabilities, fine-tune LLMs on multi-step conversations, implement retrieval-augmented generation, conduct multimodal search and conditional image generation, visualize vector embeddings, improve search results with rerankers, implement vector search with embedding models, extract structured text from images, summarize and evaluate outputs with LLMs, generate podcasts from PDF content, and get LLMs to generate knowledge graphs.
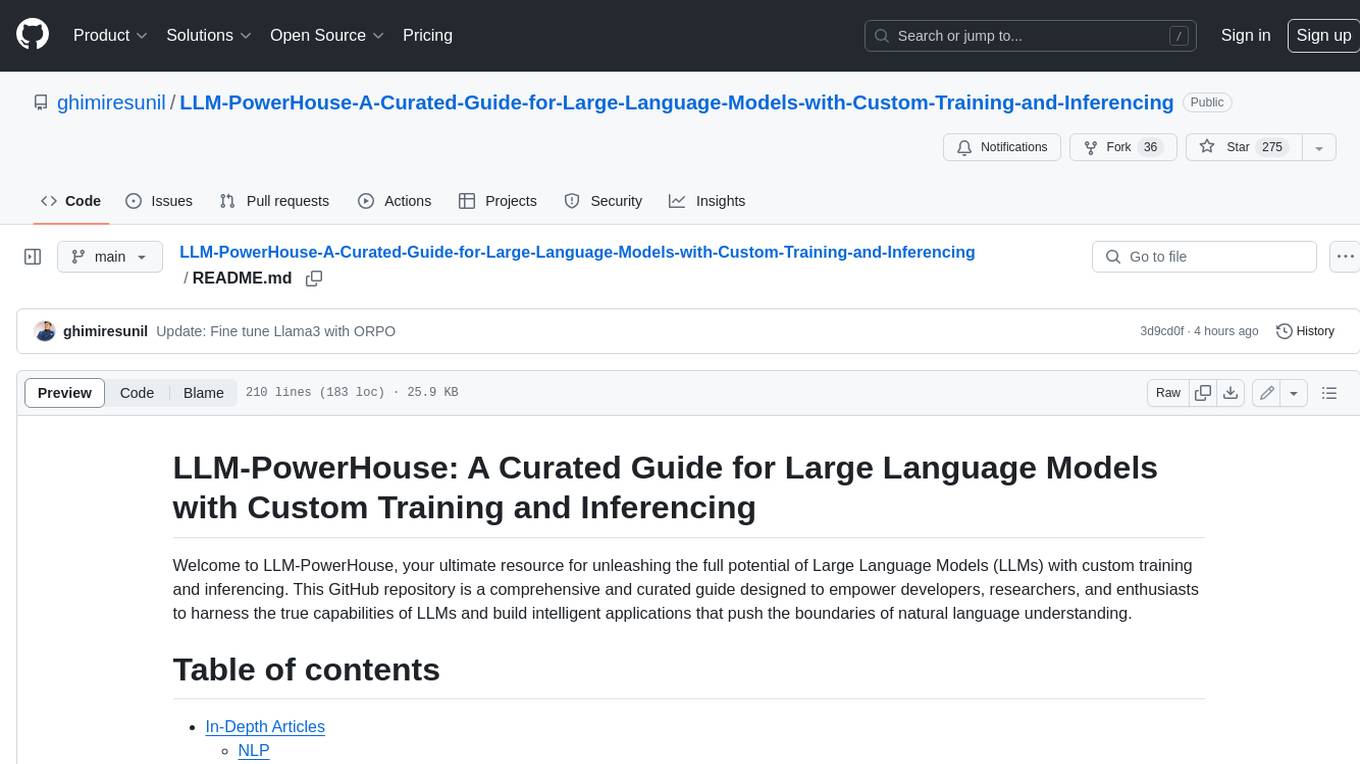
LLM-PowerHouse-A-Curated-Guide-for-Large-Language-Models-with-Custom-Training-and-Inferencing
LLM-PowerHouse is a comprehensive and curated guide designed to empower developers, researchers, and enthusiasts to harness the true capabilities of Large Language Models (LLMs) and build intelligent applications that push the boundaries of natural language understanding. This GitHub repository provides in-depth articles, codebase mastery, LLM PlayLab, and resources for cost analysis and network visualization. It covers various aspects of LLMs, including NLP, models, training, evaluation metrics, open LLMs, and more. The repository also includes a collection of code examples and tutorials to help users build and deploy LLM-based applications.
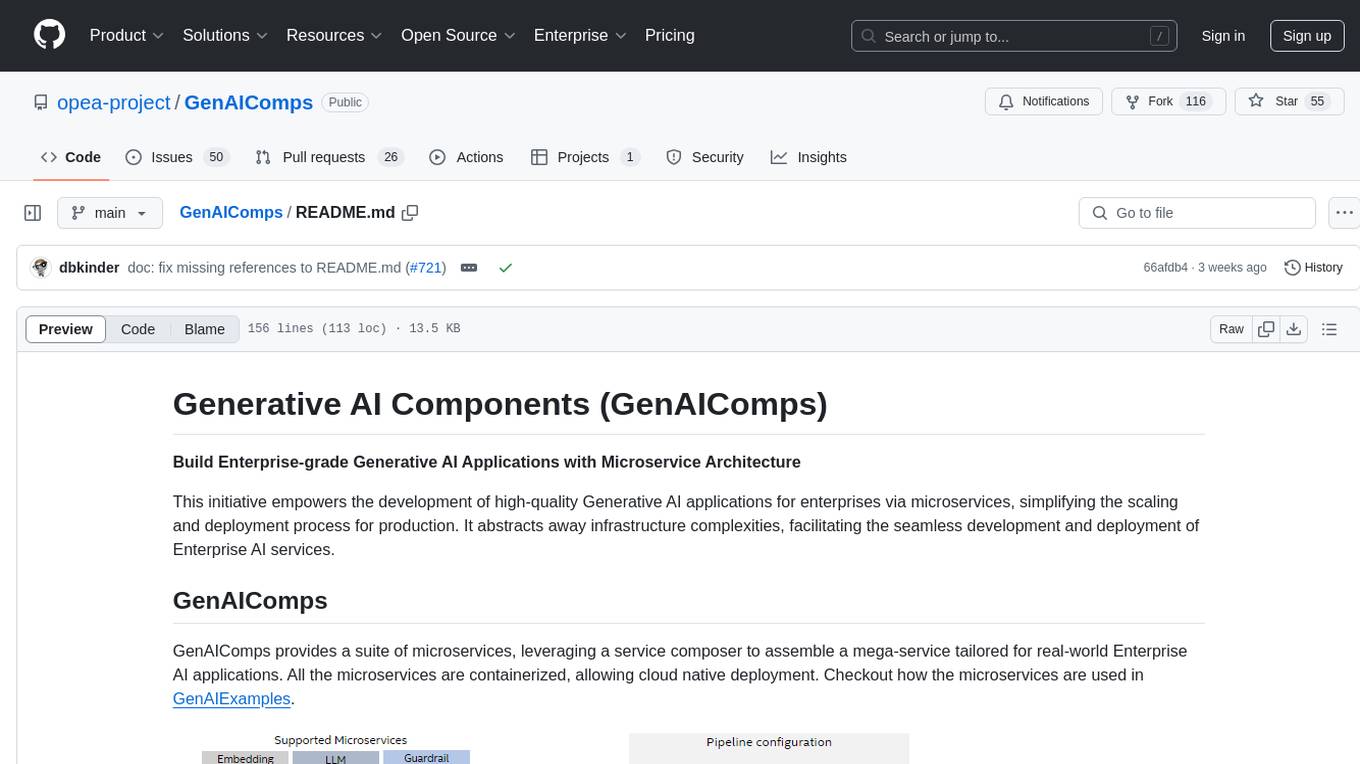
GenAIComps
GenAIComps is an initiative aimed at building enterprise-grade Generative AI applications using a microservice architecture. It simplifies the scaling and deployment process for production, abstracting away infrastructure complexities. GenAIComps provides a suite of containerized microservices that can be assembled into a mega-service tailored for real-world Enterprise AI applications. The modular approach of microservices allows for independent development, deployment, and scaling of individual components, promoting modularity, flexibility, and scalability. The mega-service orchestrates multiple microservices to deliver comprehensive solutions, encapsulating complex business logic and workflow orchestration. The gateway serves as the interface for users to access the mega-service, providing customized access based on user requirements.
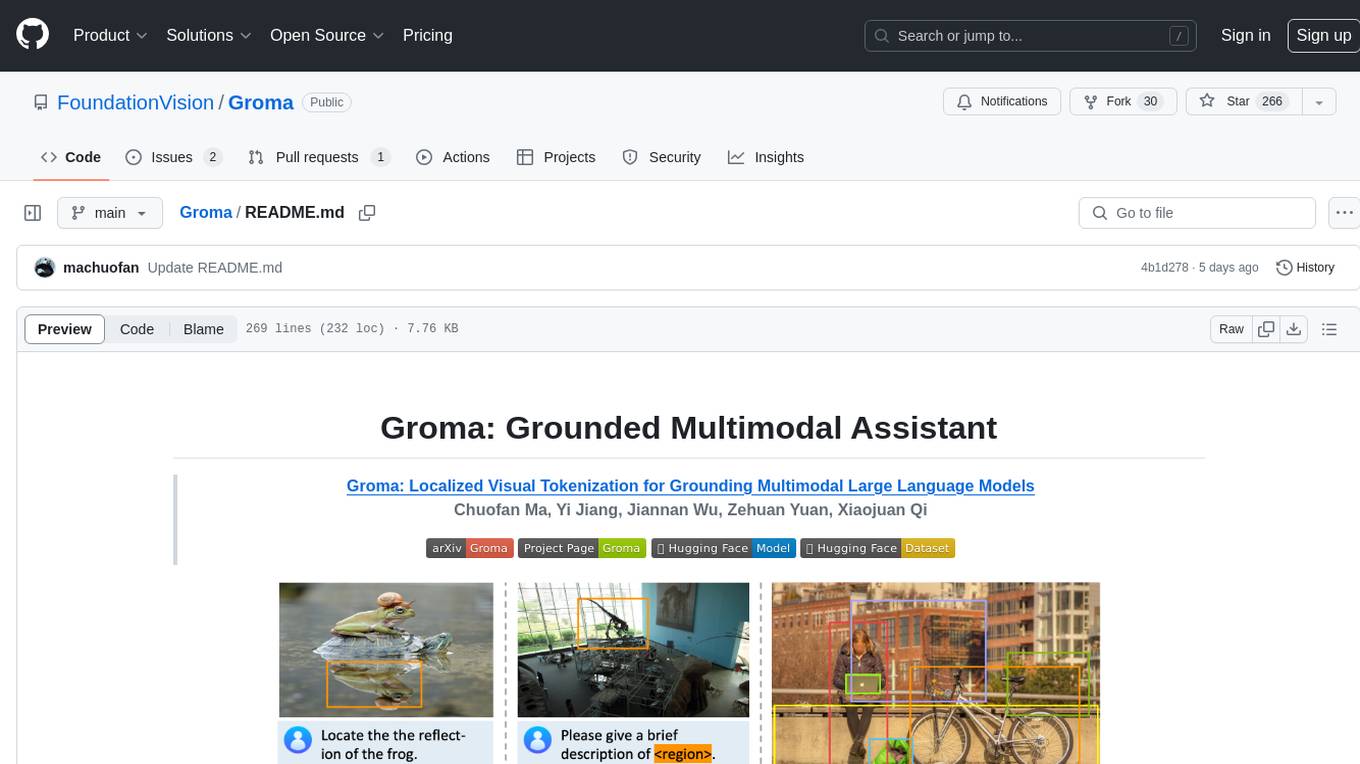
Groma
Groma is a grounded multimodal assistant that excels in region understanding and visual grounding. It can process user-defined region inputs and generate contextually grounded long-form responses. The tool presents a unique paradigm for multimodal large language models, focusing on visual tokenization for localization. Groma achieves state-of-the-art performance in referring expression comprehension benchmarks. The tool provides pretrained model weights and instructions for data preparation, training, inference, and evaluation. Users can customize training by starting from intermediate checkpoints. Groma is designed to handle tasks related to detection pretraining, alignment pretraining, instruction finetuning, instruction following, and more.
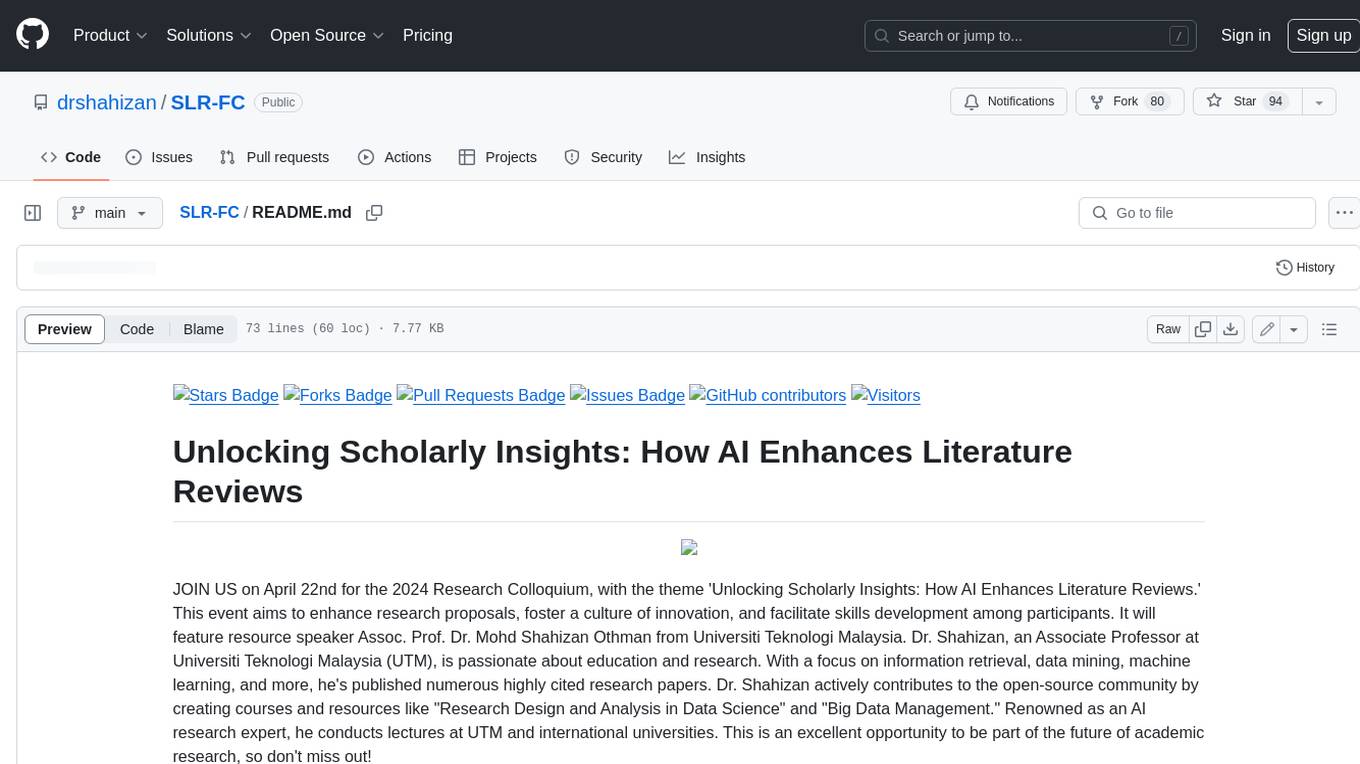
SLR-FC
This repository provides a comprehensive collection of AI tools and resources to enhance literature reviews. It includes a curated list of AI tools for various tasks, such as identifying research gaps, discovering relevant papers, visualizing paper content, and summarizing text. Additionally, the repository offers materials on generative AI, effective prompts, copywriting, image creation, and showcases of AI capabilities. By leveraging these tools and resources, researchers can streamline their literature review process, gain deeper insights from scholarly literature, and improve the quality of their research outputs.
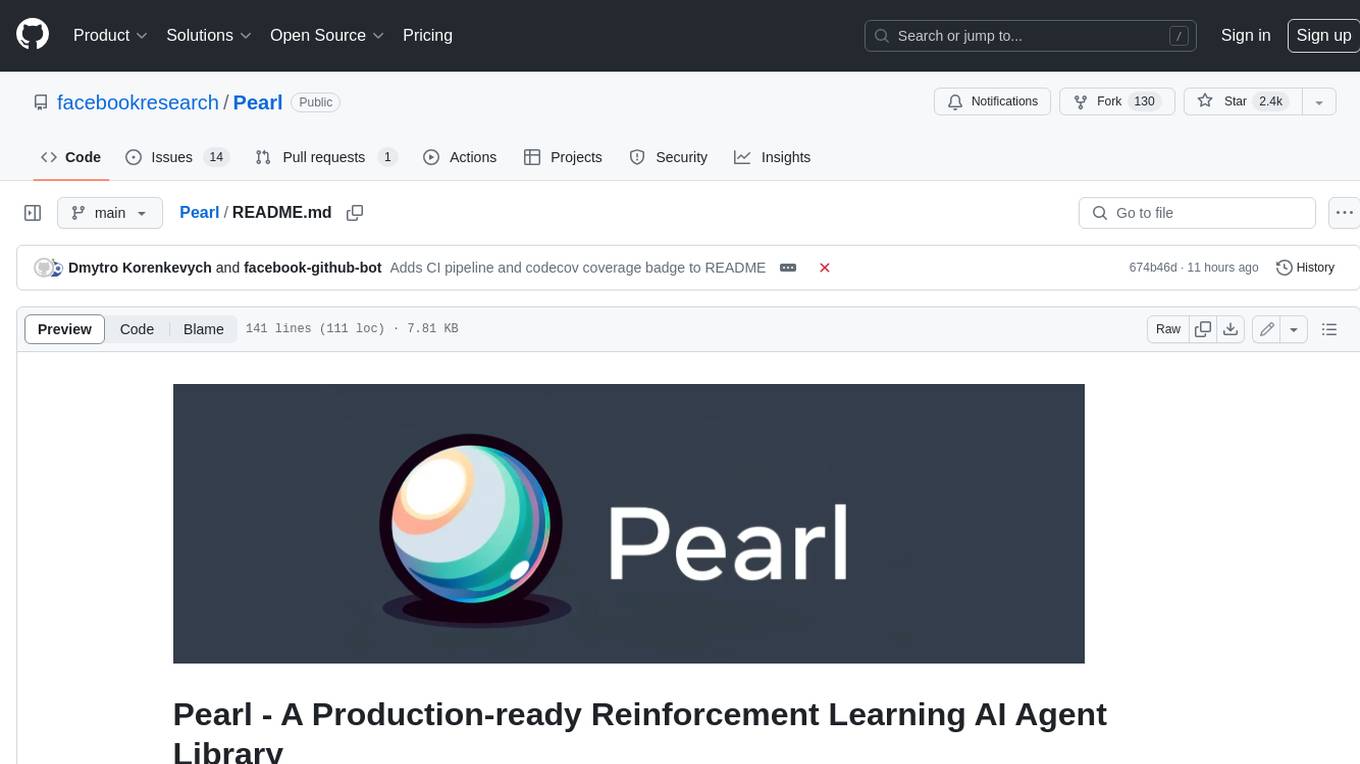
Pearl
Pearl is a production-ready Reinforcement Learning AI agent library open-sourced by the Applied Reinforcement Learning team at Meta. It enables researchers and practitioners to develop Reinforcement Learning AI agents that prioritize cumulative long-term feedback over immediate feedback and can adapt to environments with limited observability, sparse feedback, and high stochasticity. Pearl offers a diverse set of unique features for production environments, including dynamic action spaces, offline learning, intelligent neural exploration, safe decision making, history summarization, and data augmentation.
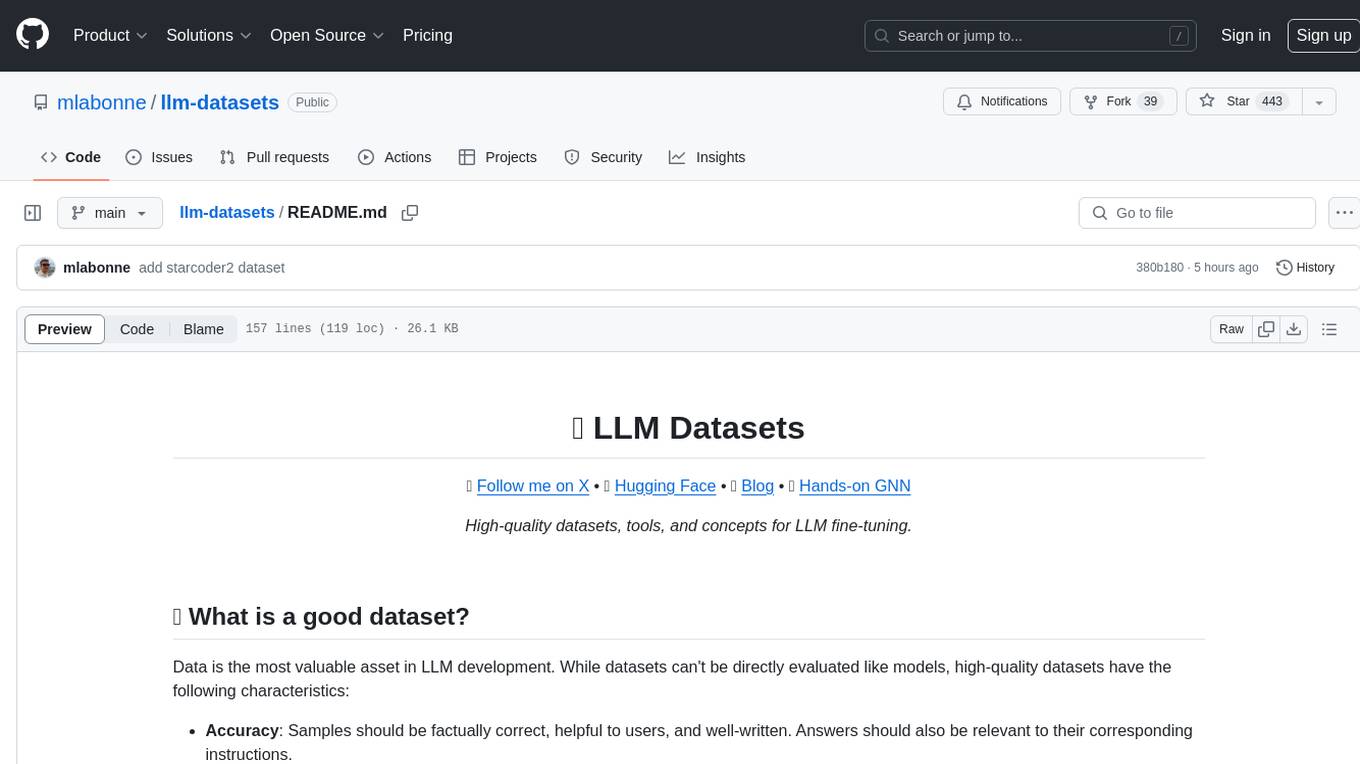
llm-datasets
LLM Datasets is a repository containing high-quality datasets, tools, and concepts for LLM fine-tuning. It provides datasets with characteristics like accuracy, diversity, and complexity to train large language models for various tasks. The repository includes datasets for general-purpose, math & logic, code, conversation & role-play, and agent & function calling domains. It also offers guidance on creating high-quality datasets through data deduplication, data quality assessment, data exploration, and data generation techniques.
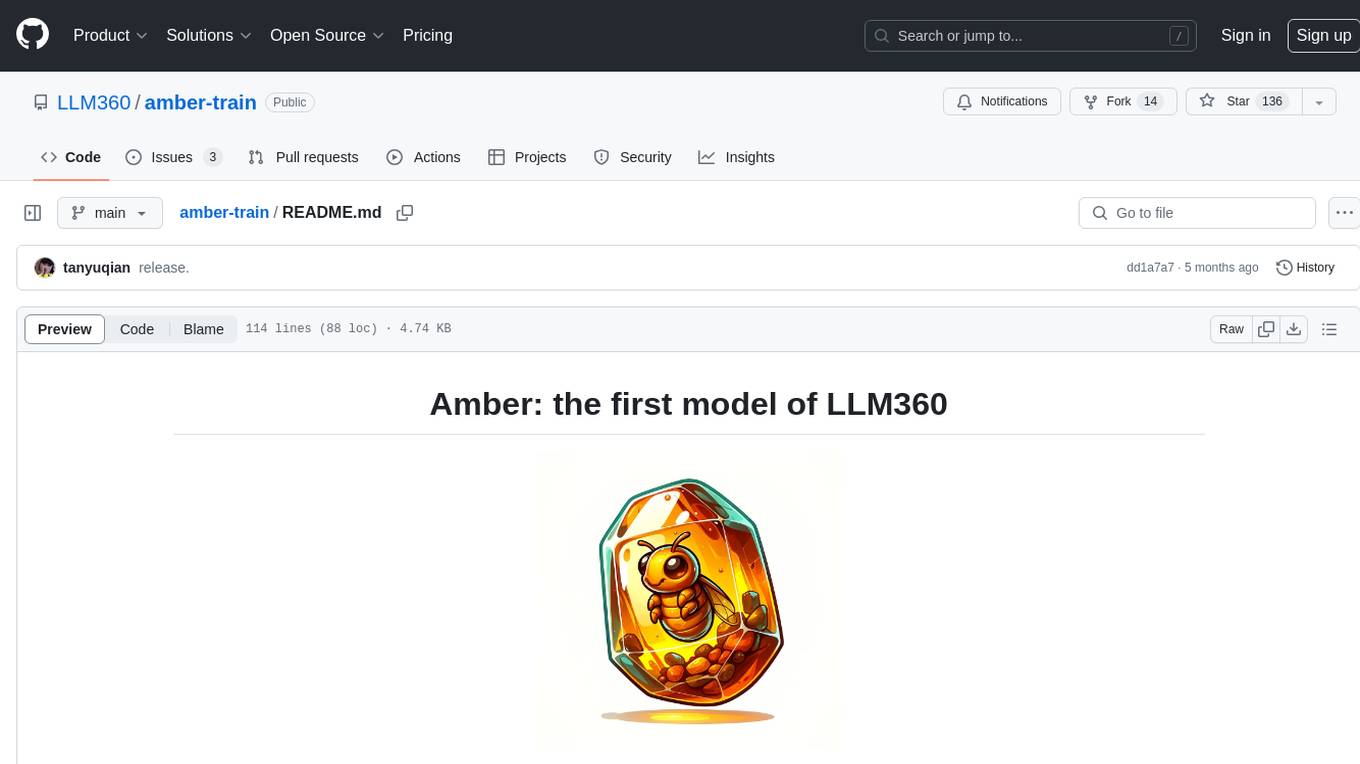
amber-train
Amber is the first model in the LLM360 family, an initiative for comprehensive and fully open-sourced LLMs. It is a 7B English language model with the LLaMA architecture. The model type is a language model with the same architecture as LLaMA-7B. It is licensed under Apache 2.0. The resources available include training code, data preparation, metrics, and fully processed Amber pretraining data. The model has been trained on various datasets like Arxiv, Book, C4, Refined-Web, StarCoder, StackExchange, and Wikipedia. The hyperparameters include a total of 6.7B parameters, hidden size of 4096, intermediate size of 11008, 32 attention heads, 32 hidden layers, RMSNorm ε of 1e^-6, max sequence length of 2048, and a vocabulary size of 32000.
For similar tasks
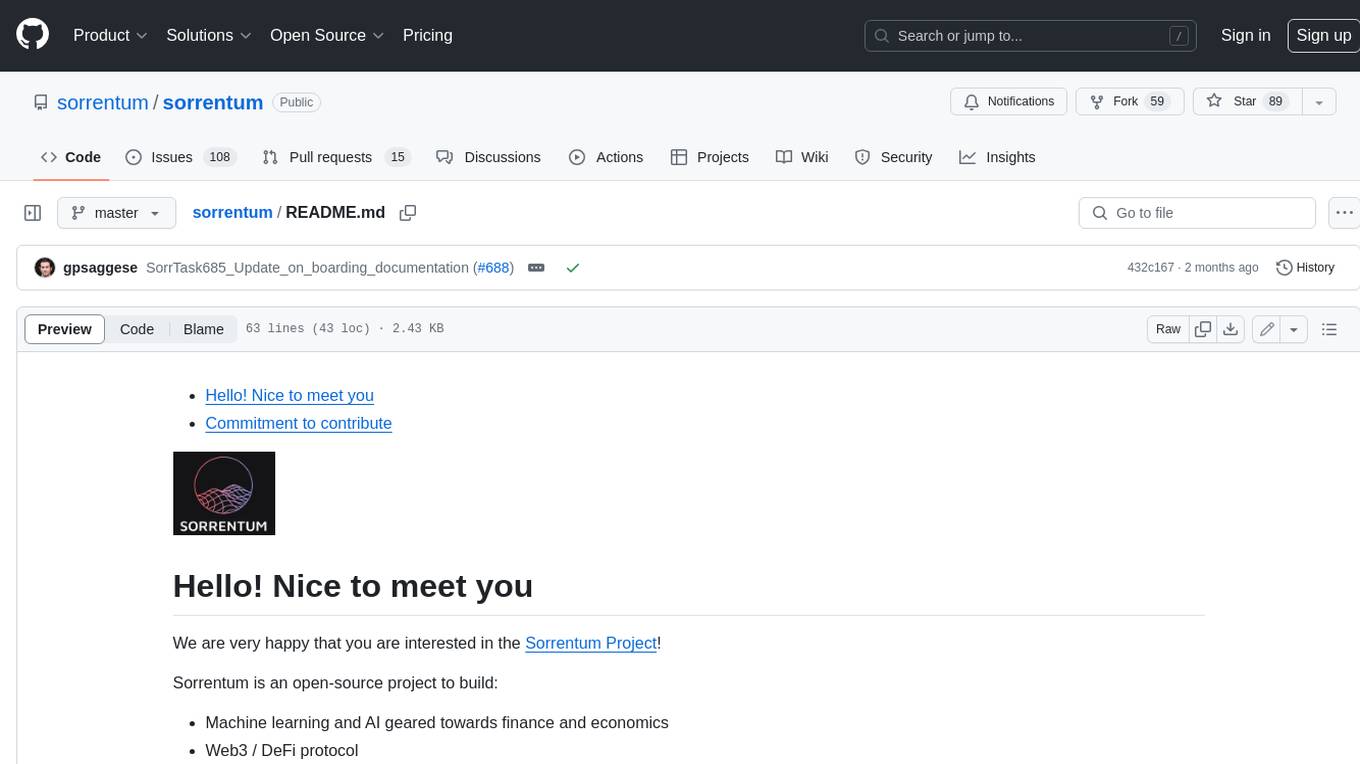
sorrentum
Sorrentum is an open-source project that aims to combine open-source development, startups, and brilliant students to build machine learning, AI, and Web3 / DeFi protocols geared towards finance and economics. The project provides opportunities for internships, research assistantships, and development grants, as well as the chance to work on cutting-edge problems, learn about startups, write academic papers, and get internships and full-time positions at companies working on Sorrentum applications.
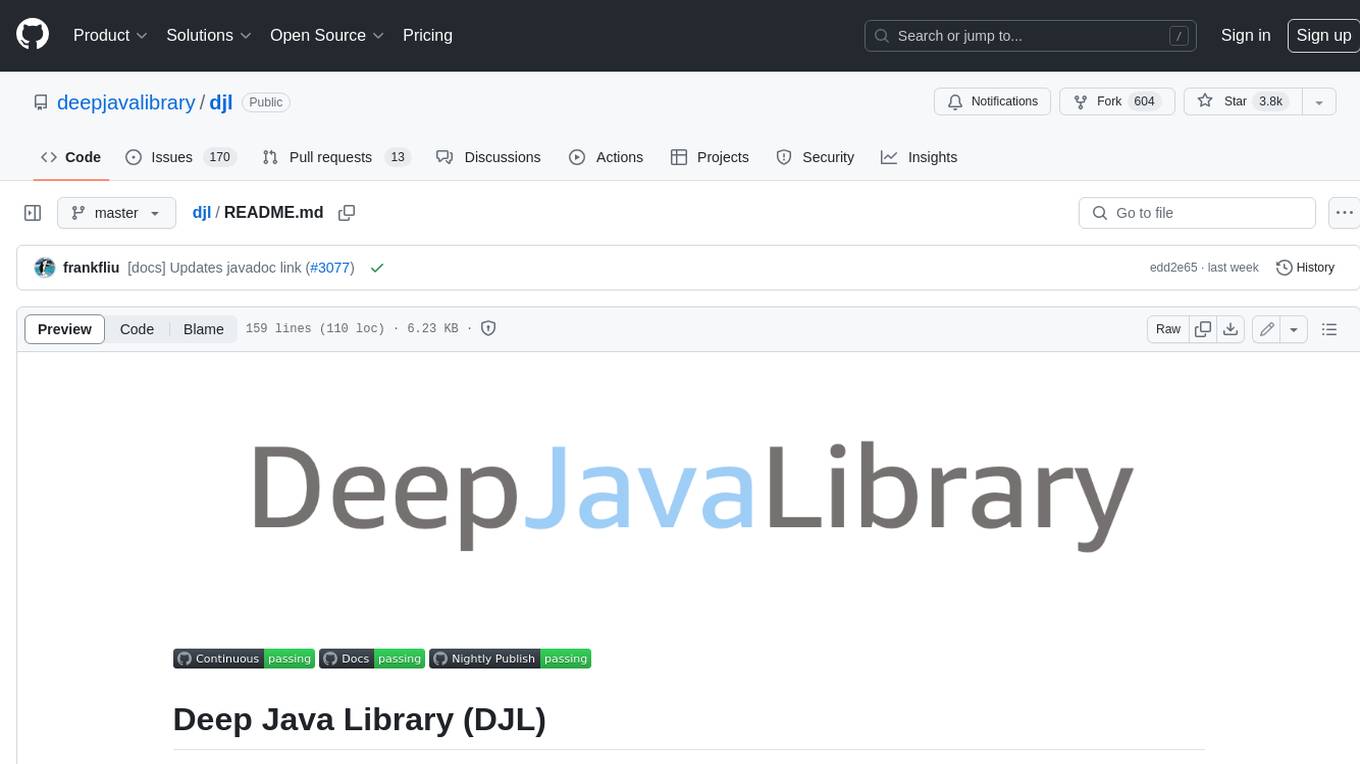
djl
Deep Java Library (DJL) is an open-source, high-level, engine-agnostic Java framework for deep learning. It is designed to be easy to get started with and simple to use for Java developers. DJL provides a native Java development experience and allows users to integrate machine learning and deep learning models with their Java applications. The framework is deep learning engine agnostic, enabling users to switch engines at any point for optimal performance. DJL's ergonomic API interface guides users with best practices to accomplish deep learning tasks, such as running inference and training neural networks.
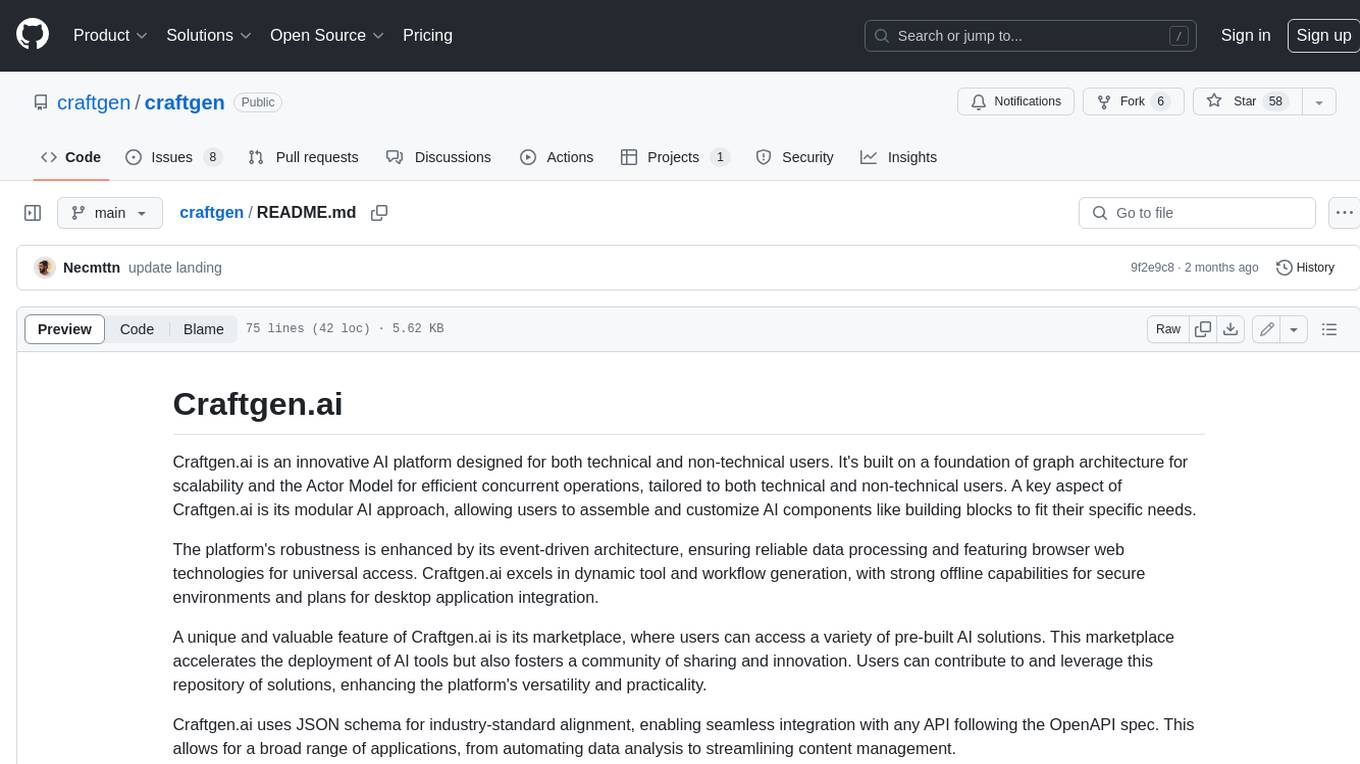
craftgen
Craftgen.ai is an innovative AI platform designed for both technical and non-technical users. It's built on a foundation of graph architecture for scalability and the Actor Model for efficient concurrent operations, tailored to both technical and non-technical users. A key aspect of Craftgen.ai is its modular AI approach, allowing users to assemble and customize AI components like building blocks to fit their specific needs. The platform's robustness is enhanced by its event-driven architecture, ensuring reliable data processing and featuring browser web technologies for universal access. Craftgen.ai excels in dynamic tool and workflow generation, with strong offline capabilities for secure environments and plans for desktop application integration. A unique and valuable feature of Craftgen.ai is its marketplace, where users can access a variety of pre-built AI solutions. This marketplace accelerates the deployment of AI tools but also fosters a community of sharing and innovation. Users can contribute to and leverage this repository of solutions, enhancing the platform's versatility and practicality. Craftgen.ai uses JSON schema for industry-standard alignment, enabling seamless integration with any API following the OpenAPI spec. This allows for a broad range of applications, from automating data analysis to streamlining content management. The platform is designed to bridge the gap between advanced AI technology and practical usability. It's a flexible, secure, and intuitive platform that empowers users, from developers seeking to create custom AI solutions to businesses looking to automate routine tasks. Craftgen.ai's goal is to make AI technology an integral, seamless part of everyday problem-solving and innovation, providing a platform where modular AI and a thriving marketplace converge to meet the diverse needs of its users.
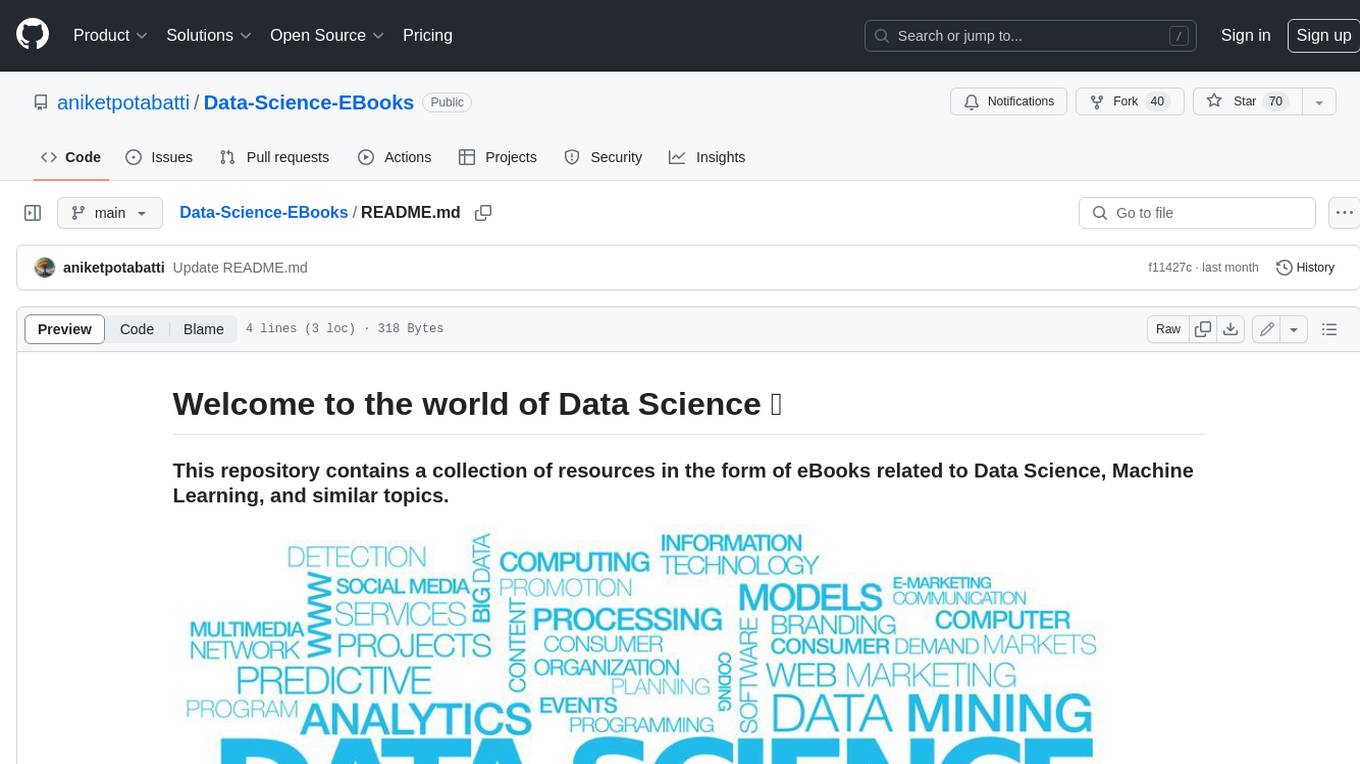
Data-Science-EBooks
This repository contains a collection of resources in the form of eBooks related to Data Science, Machine Learning, and similar topics.
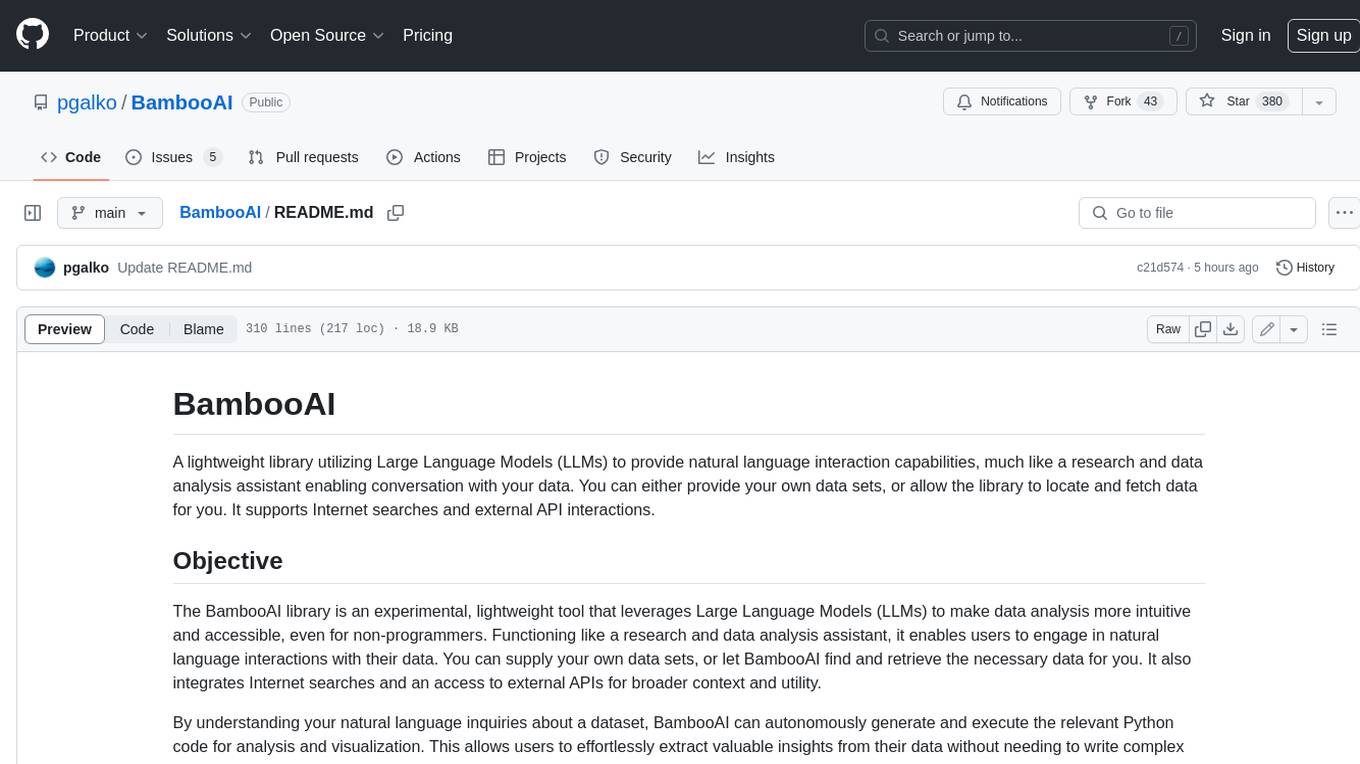
BambooAI
BambooAI is a lightweight library utilizing Large Language Models (LLMs) to provide natural language interaction capabilities, much like a research and data analysis assistant enabling conversation with your data. You can either provide your own data sets, or allow the library to locate and fetch data for you. It supports Internet searches and external API interactions.
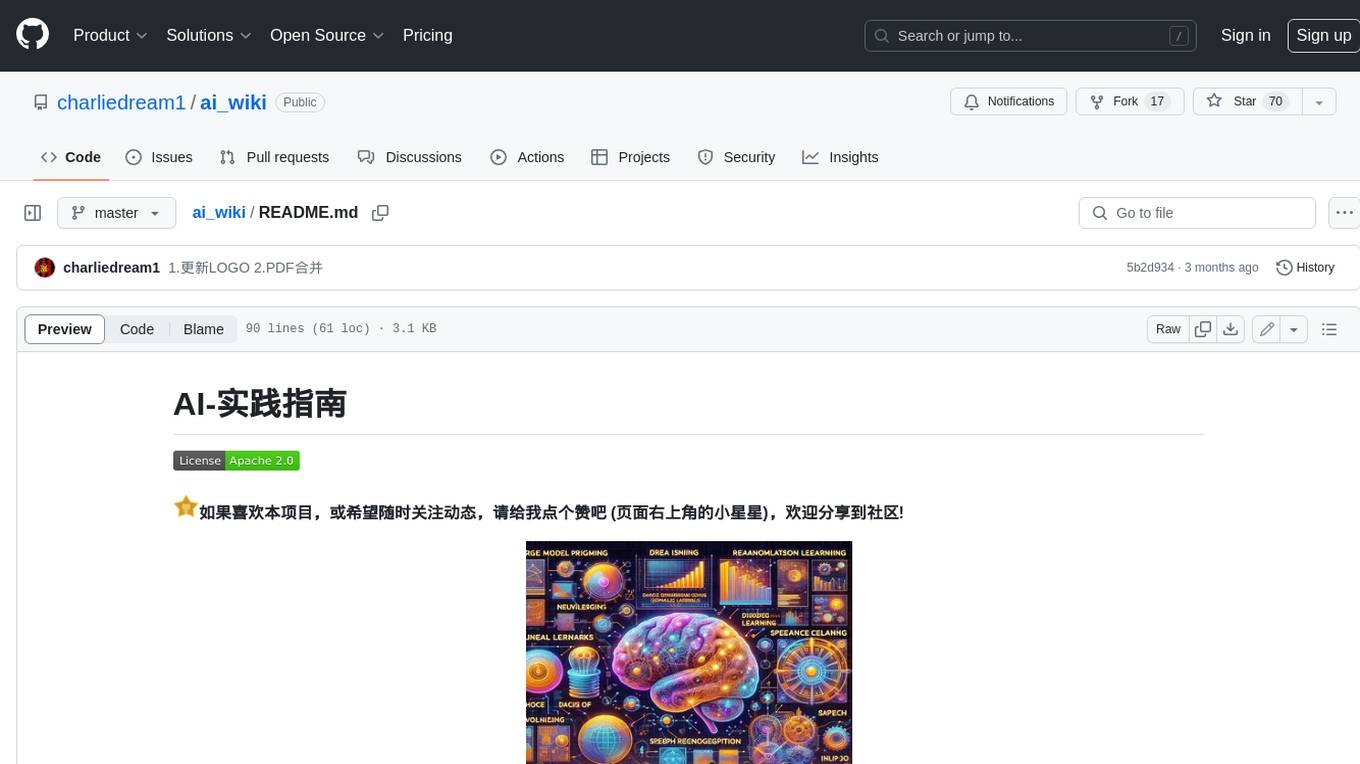
ai_wiki
This repository provides a comprehensive collection of resources, open-source tools, and knowledge related to quantitative analysis. It serves as a valuable knowledge base and navigation guide for individuals interested in various aspects of quantitative investing, including platforms, programming languages, mathematical foundations, machine learning, deep learning, and practical applications. The repository is well-structured and organized, with clear sections covering different topics. It includes resources on system platforms, programming codes, mathematical foundations, algorithm principles, machine learning, deep learning, reinforcement learning, graph networks, model deployment, and practical applications. Additionally, there are dedicated sections on quantitative trading and investment, as well as large models. The repository is actively maintained and updated, ensuring that users have access to the latest information and resources.
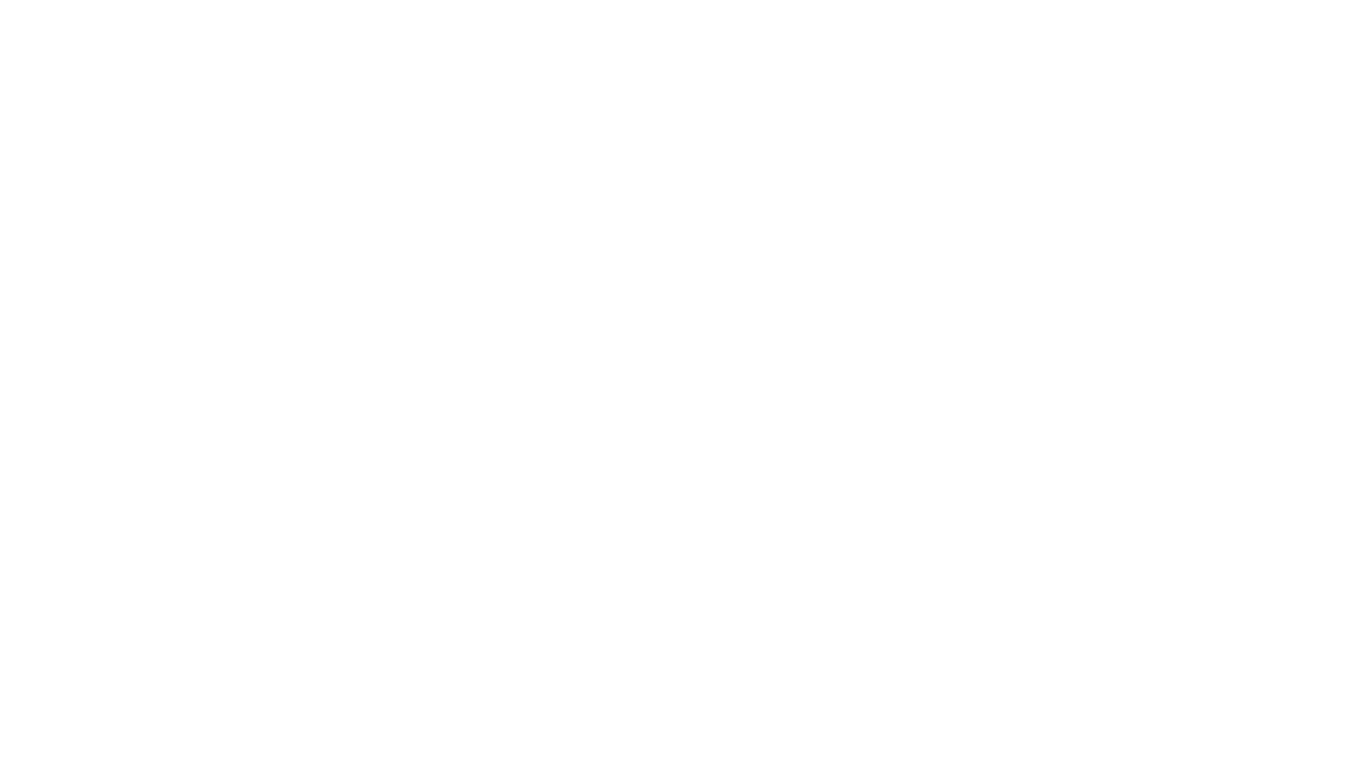
free-for-life
A massive list including a huge amount of products and services that are completely free! ⭐ Star on GitHub • 🤝 Contribute # Table of Contents * APIs, Data & ML * Artificial Intelligence * BaaS * Code Editors * Code Generation * DNS * Databases * Design & UI * Domains * Email * Font * For Students * Forms * Linux Distributions * Messaging & Streaming * PaaS * Payments & Billing * SSL
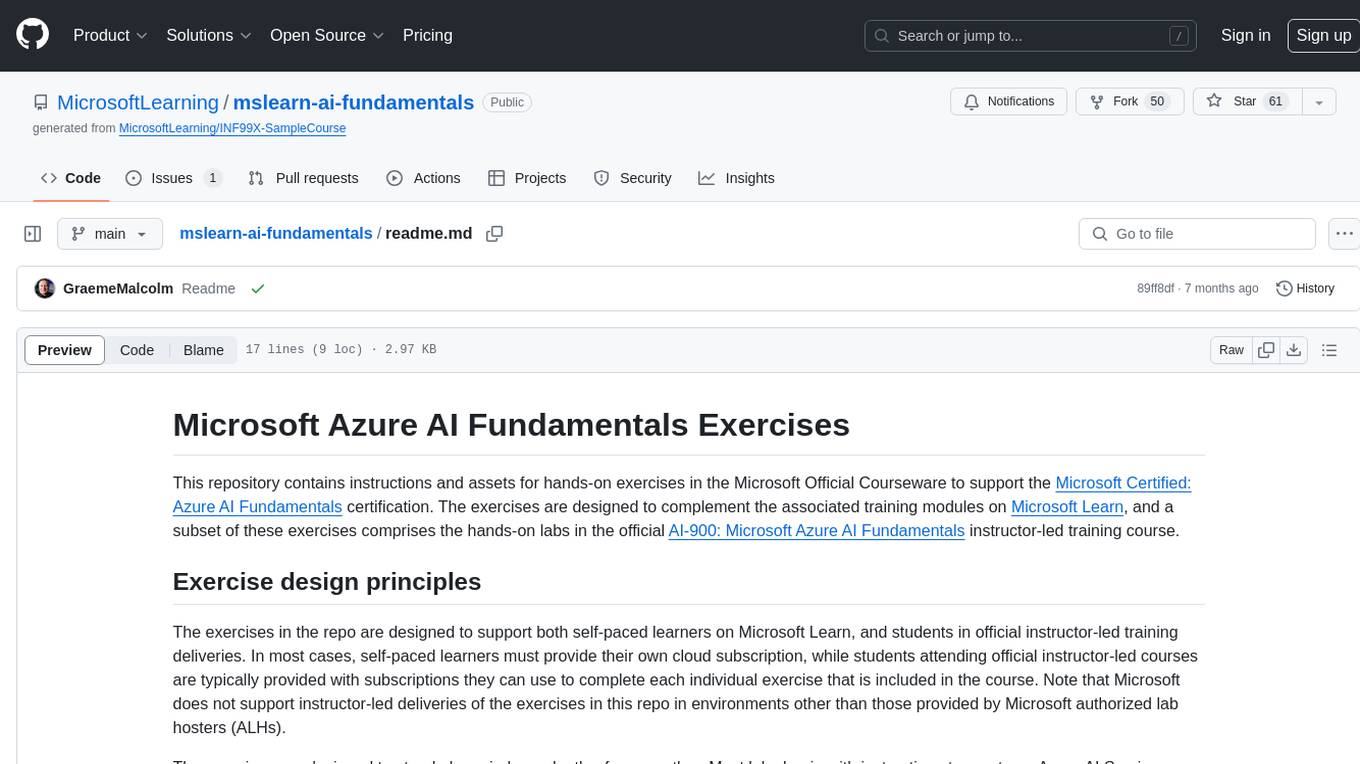
mslearn-ai-fundamentals
This repository contains materials for the Microsoft Learn AI Fundamentals module. It covers the basics of artificial intelligence, machine learning, and data science. The content includes hands-on labs, interactive learning modules, and assessments to help learners understand key concepts and techniques in AI. Whether you are new to AI or looking to expand your knowledge, this module provides a comprehensive introduction to the fundamentals of AI.
For similar jobs
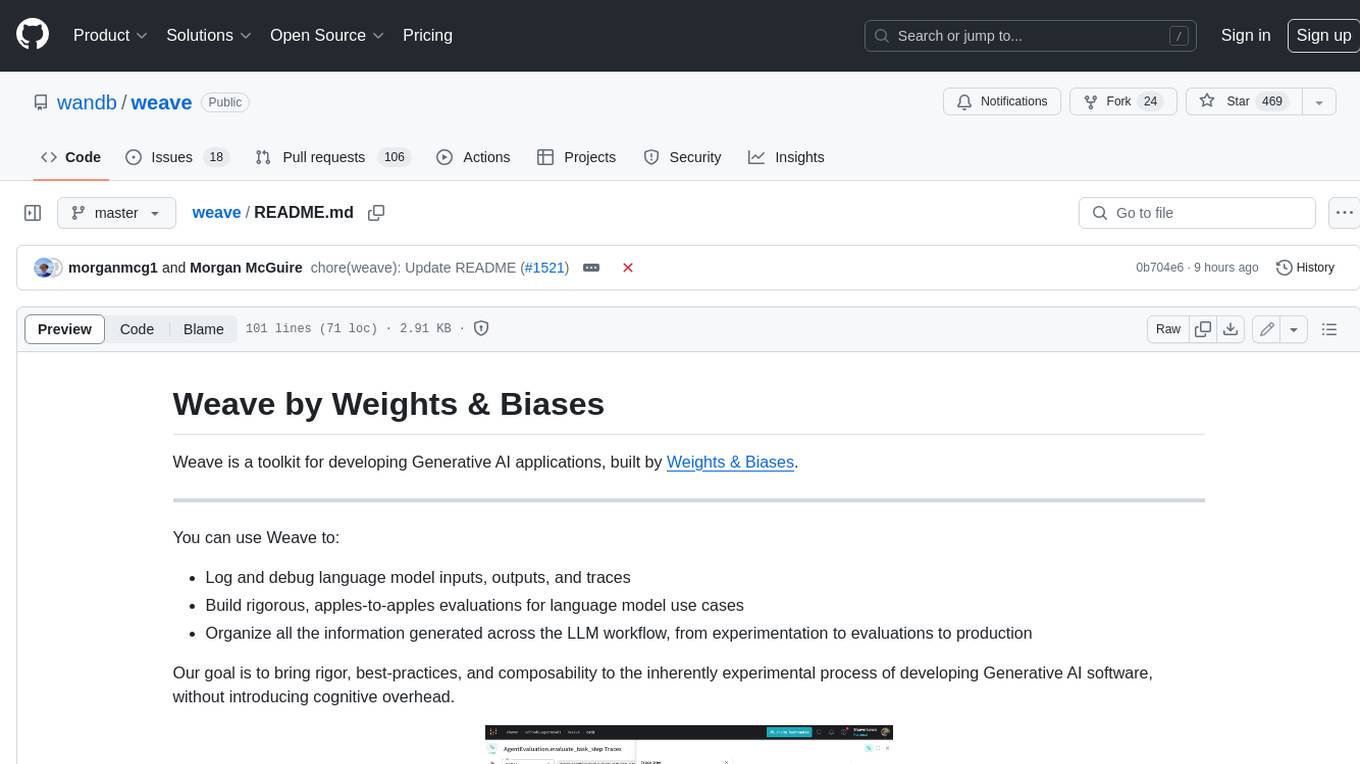
weave
Weave is a toolkit for developing Generative AI applications, built by Weights & Biases. With Weave, you can log and debug language model inputs, outputs, and traces; build rigorous, apples-to-apples evaluations for language model use cases; and organize all the information generated across the LLM workflow, from experimentation to evaluations to production. Weave aims to bring rigor, best-practices, and composability to the inherently experimental process of developing Generative AI software, without introducing cognitive overhead.
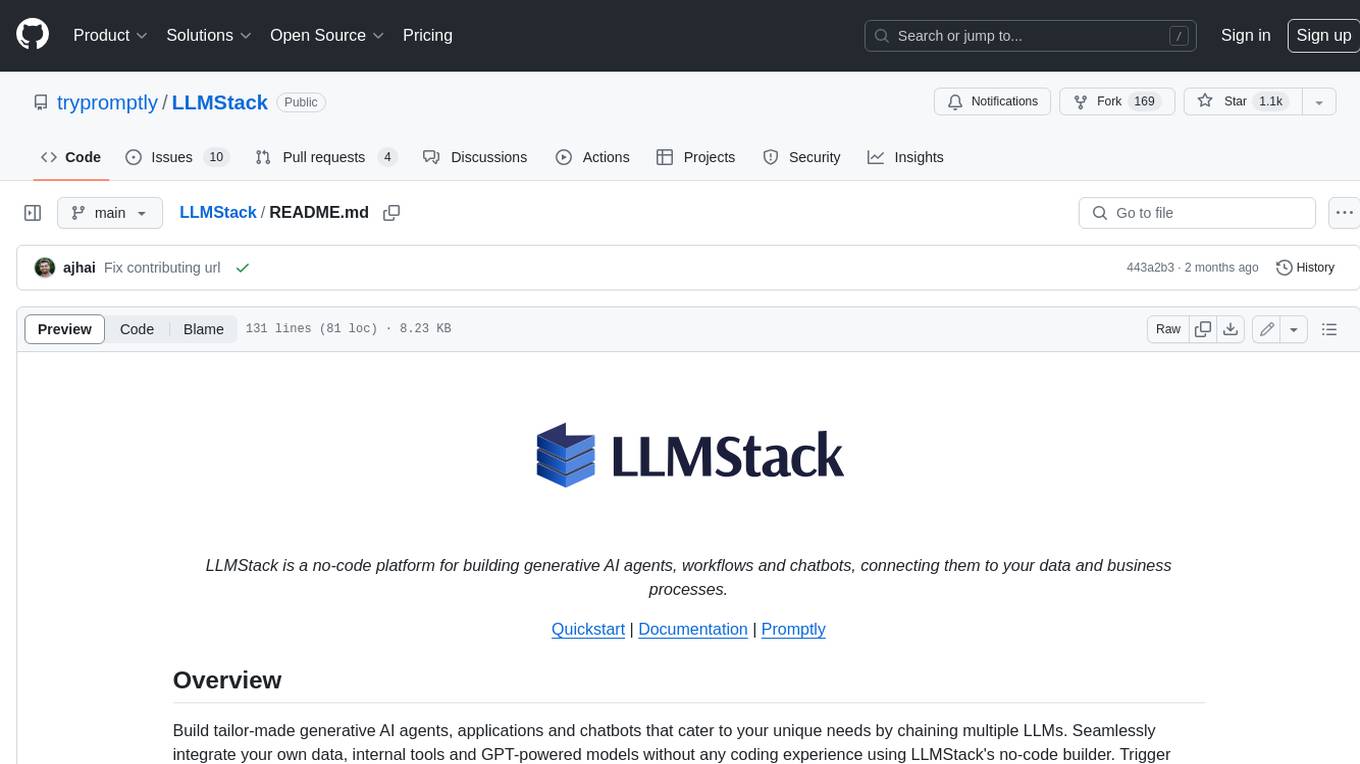
LLMStack
LLMStack is a no-code platform for building generative AI agents, workflows, and chatbots. It allows users to connect their own data, internal tools, and GPT-powered models without any coding experience. LLMStack can be deployed to the cloud or on-premise and can be accessed via HTTP API or triggered from Slack or Discord.
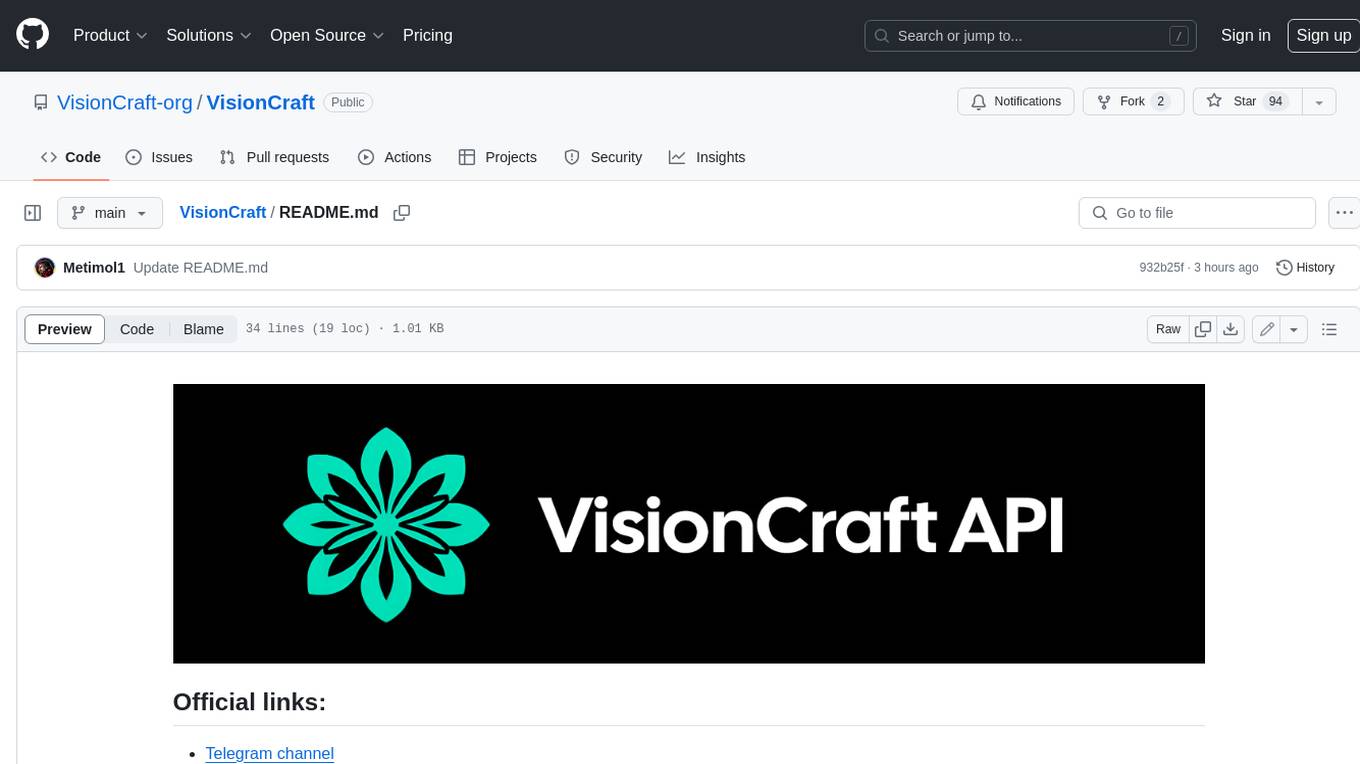
VisionCraft
The VisionCraft API is a free API for using over 100 different AI models. From images to sound.
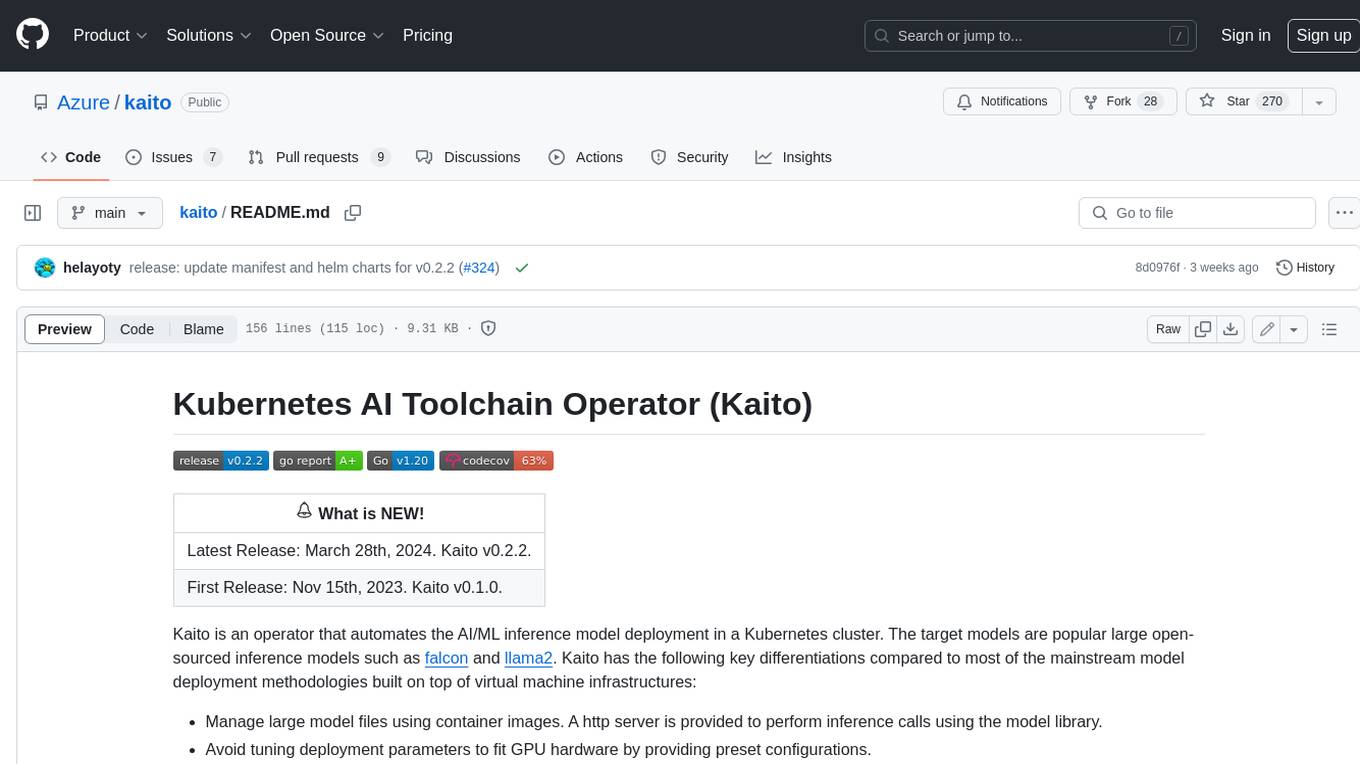
kaito
Kaito is an operator that automates the AI/ML inference model deployment in a Kubernetes cluster. It manages large model files using container images, avoids tuning deployment parameters to fit GPU hardware by providing preset configurations, auto-provisions GPU nodes based on model requirements, and hosts large model images in the public Microsoft Container Registry (MCR) if the license allows. Using Kaito, the workflow of onboarding large AI inference models in Kubernetes is largely simplified.
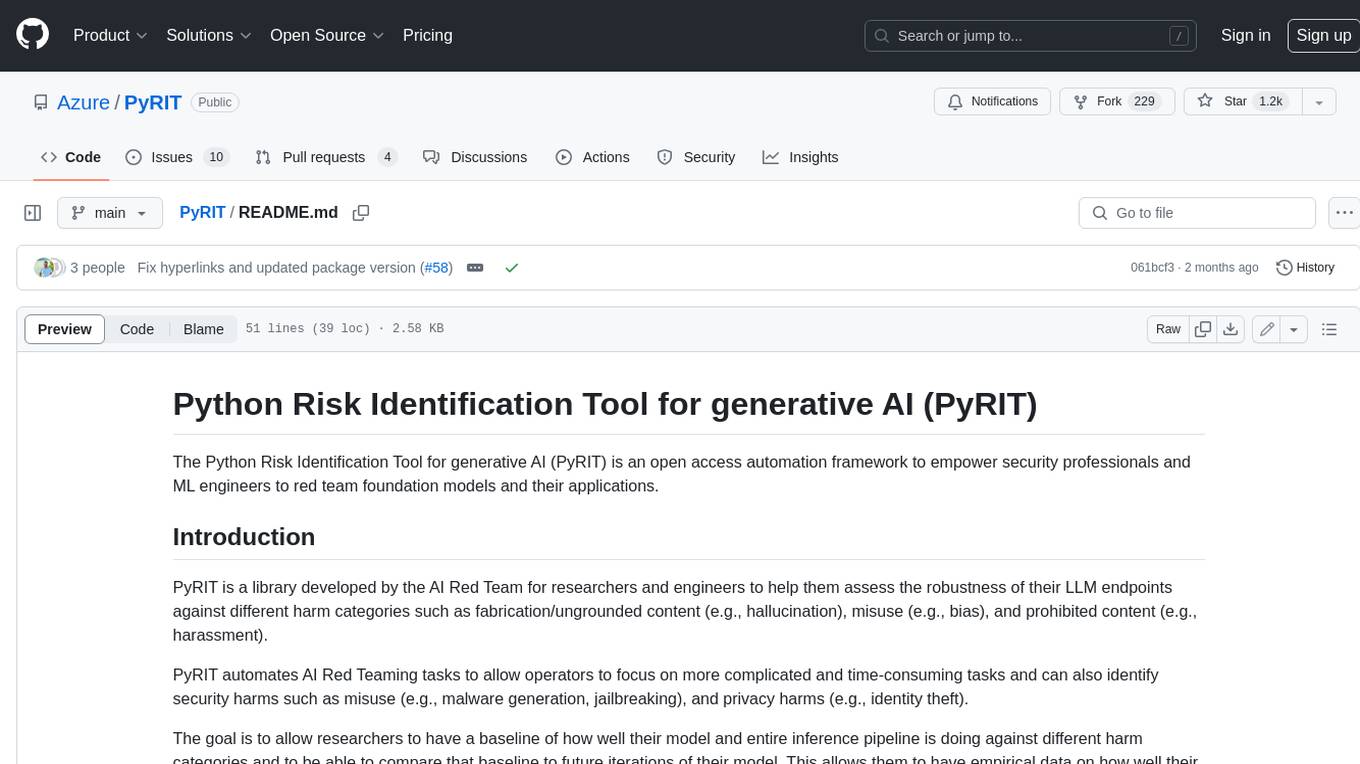
PyRIT
PyRIT is an open access automation framework designed to empower security professionals and ML engineers to red team foundation models and their applications. It automates AI Red Teaming tasks to allow operators to focus on more complicated and time-consuming tasks and can also identify security harms such as misuse (e.g., malware generation, jailbreaking), and privacy harms (e.g., identity theft). The goal is to allow researchers to have a baseline of how well their model and entire inference pipeline is doing against different harm categories and to be able to compare that baseline to future iterations of their model. This allows them to have empirical data on how well their model is doing today, and detect any degradation of performance based on future improvements.
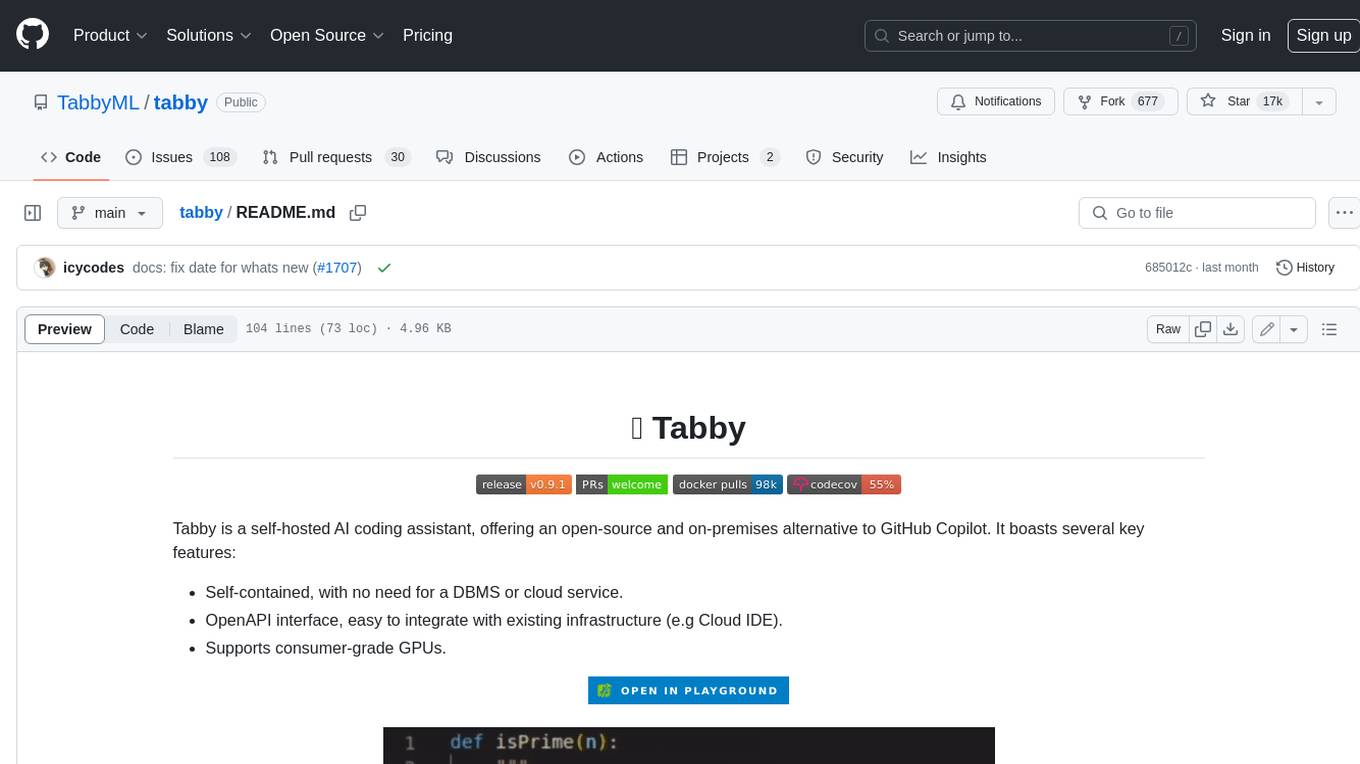
tabby
Tabby is a self-hosted AI coding assistant, offering an open-source and on-premises alternative to GitHub Copilot. It boasts several key features: * Self-contained, with no need for a DBMS or cloud service. * OpenAPI interface, easy to integrate with existing infrastructure (e.g Cloud IDE). * Supports consumer-grade GPUs.
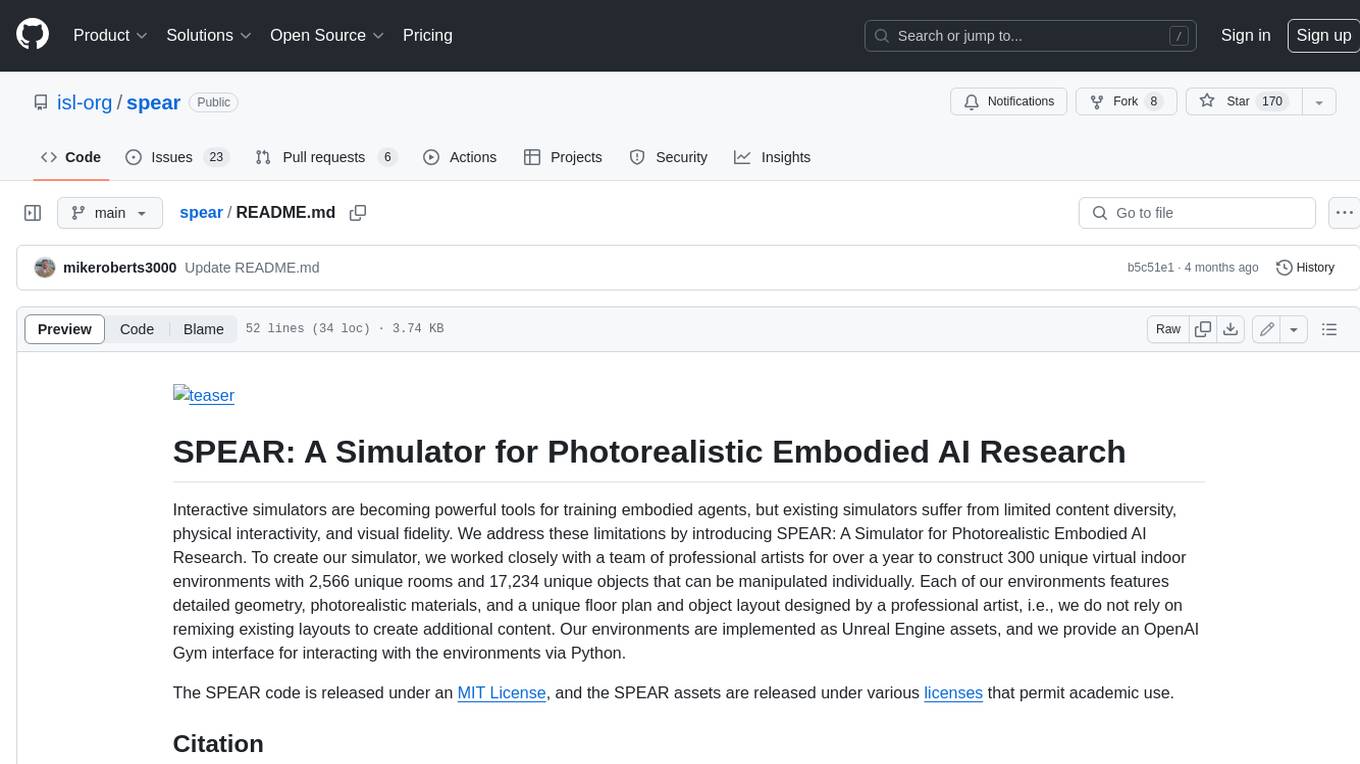
spear
SPEAR (Simulator for Photorealistic Embodied AI Research) is a powerful tool for training embodied agents. It features 300 unique virtual indoor environments with 2,566 unique rooms and 17,234 unique objects that can be manipulated individually. Each environment is designed by a professional artist and features detailed geometry, photorealistic materials, and a unique floor plan and object layout. SPEAR is implemented as Unreal Engine assets and provides an OpenAI Gym interface for interacting with the environments via Python.
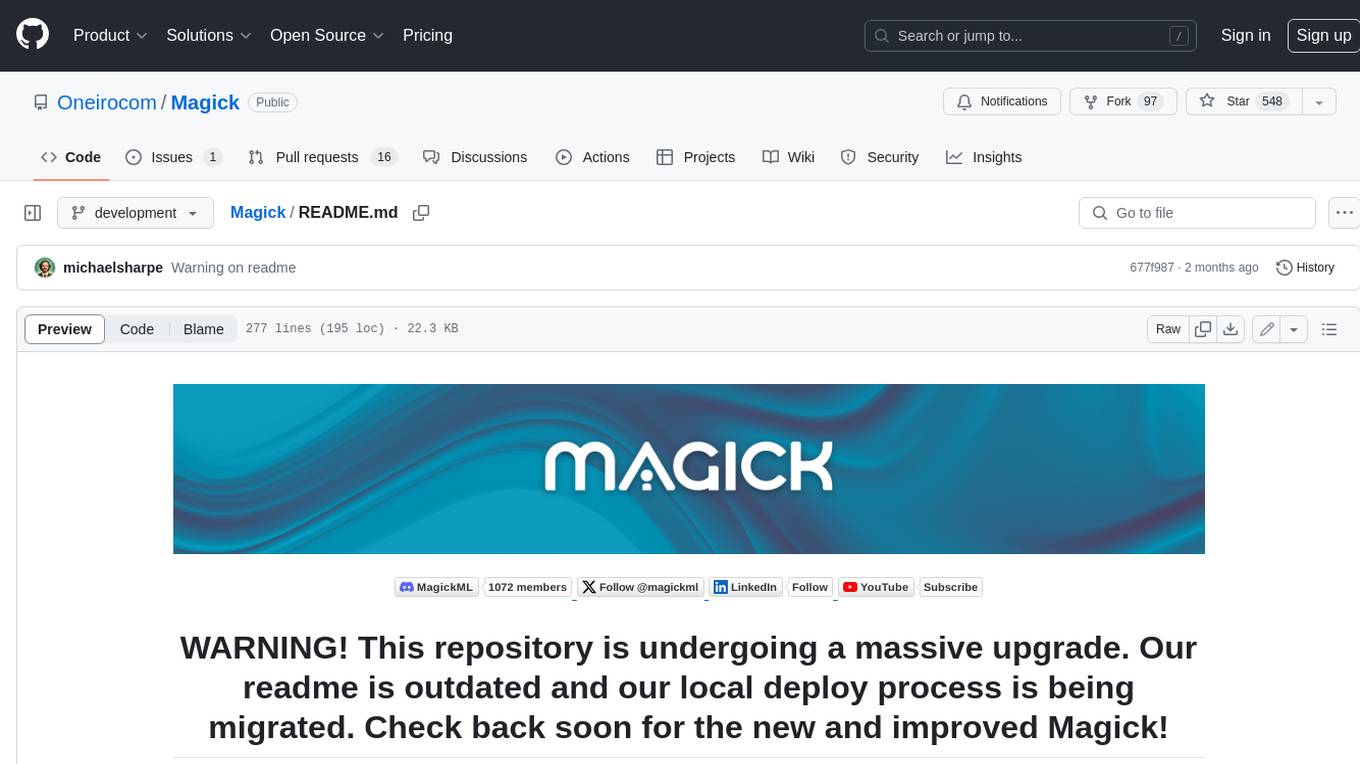
Magick
Magick is a groundbreaking visual AIDE (Artificial Intelligence Development Environment) for no-code data pipelines and multimodal agents. Magick can connect to other services and comes with nodes and templates well-suited for intelligent agents, chatbots, complex reasoning systems and realistic characters.