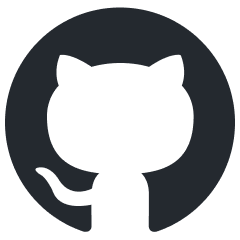
MyScaleDB
An open-source, high-performance SQL vector database built on ClickHouse.
Stars: 689
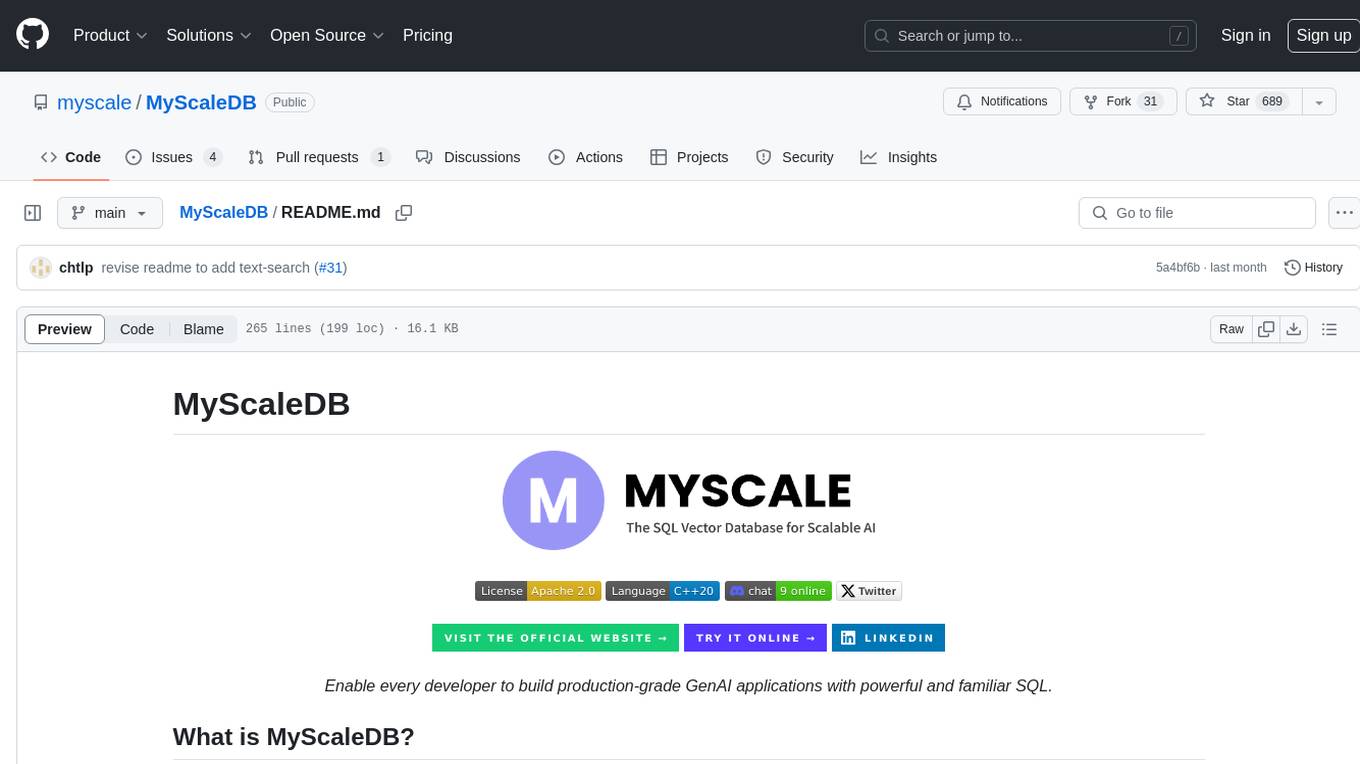
MyScaleDB is a SQL vector database optimized for AI applications, enabling developers to manage and process massive volumes of data efficiently. It offers fast and powerful vector search, filtered search, and SQL-vector join queries, making it fully SQL-compatible. MyScaleDB provides unmatched performance and scalability by leveraging cutting-edge OLAP database architecture and advanced vector algorithms. It is production-ready for AI applications, supporting structured data, text, vector, JSON, geospatial, and time-series data. MyScale Cloud offers fully-managed MyScaleDB with premium features on billion-scale data, making it cost-effective and simpler to use compared to specialized vector databases. Built on top of ClickHouse, MyScaleDB combines structured and vector search efficiently, ensuring high accuracy and performance in filtered search operations.
README:
MyScaleDB is the SQL vector database that enables developers to build production-ready and scalable AI applications using familiar SQL. It is built on top of ClickHouse and optimized for AI applications and solutions, allowing developers to effectively manage and process massive volumes of data.
Key benefits of using MyScaleDB include:
-
Fully SQL-Compatible
- Fast, powerful, and efficient vector search, filtered search, and SQL-vector join queries.
- Use SQL with vector-related functions to interact with MyScaleDB. No need to learn complex new tools or frameworks – stick with what you know and love.
-
Production-Ready for AI applications
- A unified and time-tested platform to manage and process structured data, text, vector, JSON, geospatial, time-series data, and more. See supported data types and functions
- Improved RAG accuracy by combining vectors with rich metadata, full-text search, and performing high-precision, high-efficiency filtered search at any ratio1.
-
Unmatched performance and scalability
- MyScaleDB leverages cutting-edge OLAP database architecture and advanced vector algorithms for lightning-fast vector operations.
- Scale your applications effortlessly and cost-effectively as your data grows.
MyScale Cloud provides fully-managed MyScaleDB with premium features on billion-scale data2. Compared with specialized vector databases that use custom APIs, MyScale is more powerful, performant, and cost-effective while remaining simpler to use. This makes it suitable for a large community of programmers. Additionally, when compared to integrated vector databases like PostgreSQL with pgvector or ElasticSearch with vector extensions, MyScale consumes fewer resources and achieves better accuracy and speed for structured and vector joint queries, such as filtered searches.
- Fully SQL compatible
- Unified structured and vectorized data management
- Millisecond search on billion-scale vectors
- Highly reliable & linearly scalable
- Powerful text-search and text/vector hybrid search functions
- Complex SQL vector queries
See our documentation and blogs for more about MyScale’s unique features and advantages. Our open-source benchmark provides detailed comparison with other vector database products.
ClickHouse is a popular open-source analytical database that excels at big data processing and analytics due to its columnar storage with advanced compression, skip indexing, and SIMD processing. Unlike transactional databases like PostgreSQL and MySQL, which use row storage and main optimzies for transactional processing, ClickHouse has significantly faster analytical and data scanning speeds.
One of the key operations in combining structured and vector search is filtered search, which involves filtering by other attributes first and then performing vector search on the remaining data. Columnar storage and pre-filtering are crucial for ensuring high accuracy and high performance in filtered search, which is why we chose to build MyScaleDB on top of ClickHouse.
While we have modified ClickHouse's execution and storage engine in many ways to ensure fast and cost-effective SQL vector queries, many of the features (#37893, #38048, #37859, #56728, #58223) related to general SQL processing have been contributed back to the ClickHouse open source community.
The simplest way to use MyScaleDB is to create an instance on MyScale Cloud service. You can start from a free pod supporting 5M 768D vectors. Sign up here and checkout MyScaleDB QuickStart for more instructions.
To quickly get a MyScaleDB instance up and running, simply pull and run the latest Docker image:
docker run --name myscaledb --net=host myscale/myscaledb:1.5
Note: Myscale's default configuration only allows localhost ip access. For the docker run startup method, you need to specify
--net=host
to access services deployed in docker mode on the current node.
This will start a MyScaleDB instance with default user default
and no password. You can then connect to the database using clickhouse-client
:
docker exec -it myscaledb clickhouse-client
- Use the following recommended directory structure and the location of the
docker-compose.yaml
file:
> tree myscaledb
myscaledb
├── docker-compose.yaml
└── volumes
└── config
└── users.d
└── custom_users_config.xml
3 directories, 2 files
- Define the configuration for your deployment. We recommend starting with the following configuration in your
docker-compose.yaml
file, which you can adjust based on your specific requirements:
version: '3.7'
services:
myscaledb:
image: myscale/myscaledb:1.5
tty: true
ports:
- '8123:8123'
- '9000:9000'
- '8998:8998'
- '9363:9363'
- '9116:9116'
networks:
myscaledb_network:
ipv4_address: 10.0.0.2
volumes:
- ${DOCKER_VOLUME_DIRECTORY:-.}/volumes/data:/var/lib/clickhouse
- ${DOCKER_VOLUME_DIRECTORY:-.}/volumes/log:/var/log/clickhouse-server
- ${DOCKER_VOLUME_DIRECTORY:-.}/volumes/config/users.d/custom_users_config.xml:/etc/clickhouse-server/users.d/custom_users_config.xml
deploy:
resources:
limits:
cpus: "16.00"
memory: 32Gb
networks:
myscaledb_network:
driver: bridge
ipam:
driver: default
config:
- subnet: 10.0.0.0/24
custom_users_config.xml
:
<clickhouse>
<users>
<default>
<password></password>
<networks>
<ip>::1</ip>
<ip>127.0.0.1</ip>
<ip>10.0.0.0/24</ip>
</networks>
<profile>default</profile>
<quota>default</quota>
<access_management>1</access_management>
</default>
</users>
</clickhouse>
Note: The custom_users_config configuration allows you to use the default user to access the database on the node where the database service is deployed using docker compose. If you want to access the database service on other nodes, it is recommended to create a user that can be accessed through other IPs. For detailed settings, see: MyScaleDB Create User. You can also customize the configuration file of MyScaleDB. Copy the
/etc/clickhouse-server
directory from yourmyscaledb
container to your local drive, modify the configuration, and add a directory mapping to thedocker-compose.yaml
file to make the configuration take effect:- ${DOCKER_VOLUME_DIRECTORY:-.}/volumes/config:/etc/clickhouse-server
- Use the following command to get it running:
cd myscaledb
docker compose up -d
- Access the MyScaleDB command line interface using the following command.
docker exec -it myscaledb-myscaledb-1 clickhouse-client
- You can now run SQL statements. See Executing SQL Queries.
See Vector Search Documentation for how to create a SQL table with vector index and perform vector search. It's recommended to specify TYPE SCANN
when creating a vector index in open source MyScaleDB.
-- Create a table with body_vector of length 384
CREATE TABLE default.wiki_abstract
(
`id` UInt64,
`body` String,
`title` String,
`url` String,
`body_vector` Array(Float32),
CONSTRAINT check_length CHECK length(body_vector) = 384
)
ENGINE = MergeTree
ORDER BY id;
-- Insert data from parquet files on S3
INSERT INTO default.wiki_abstract SELECT * FROM s3('https://myscale-datasets.s3.ap-southeast-1.amazonaws.com/wiki_abstract_with_vector.parquet','Parquet');
-- Build a SCANN vector index with Cosine metric on the body_vector
ALTER TABLE default.wiki_abstract ADD VECTOR INDEX vec_idx body_vector TYPE SCANN('metric_type=Cosine');
-- Query the index build progress from the `vector_indices` table
-- Wait until the index progress becomes `Built`
SELECT * FROM system.vector_indices;
-- Perform vector search return the top-5 results
SELECT
id,
title,
distance(body_vector, [-0.052, -0.0146, -0.0677, -0.0256, -0.0395, -0.0381, -0.025, 0.0911, -0.0429, -0.0592, 0.0017, -0.0358, -0.0464, -0.0189, -0.0192, 0.0544, -0.0022, -0.0292, -0.0474, -0.0286, 0.0746, -0.013, -0.0217, -0.0246, -0.0169, 0.0495, -0.0947, 0.0139, 0.0445, -0.0262, -0.0049, 0.0506, 0.004, 0.0276, 0.0063, -0.0643, 0.0059, -0.0229, -0.0315, 0.0549, 0.1427, 0.0079, 0.011, -0.0036, -0.0617, 0.0155, -0.0607, 0.0258, -0.0205, 0.0008, -0.0547, 0.0329, -0.0522, -0.0347, 0.0921, 0.0139, -0.013, 0.0716, -0.0165, 0.0257, -0.0071, 0.0084, -0.0653, 0.0091, 0.0544, -0.0192, -0.0169, -0.0017, -0.0304, 0.0427, -0.0389, 0.0921, -0.0622, -0.0196, 0.0025, 0.0214, 0.0259, -0.0493, -0.0211, -0.119, -0.0736, -0.1545, -0.0578, -0.0145, 0.0138, 0.0478, -0.0451, -0.0332, 0.0799, 0.0001, -0.0737, 0.0427, 0.0517, 0.0102, 0.0386, 0.0233, 0.0425, -0.0279, -0.0529, 0.0744, -0.0305, -0.026, 0.1229, -0.002, 0.0038, -0.0491, 0.0352, 0.0027, -0.056, -0.1044, 0.123, -0.0184, 0.1148, -0.0189, 0.0412, -0.0347, -0.0569, -0.0119, 0.0098, -0.0016, 0.0451, 0.0273, 0.0436, 0.0082, 0.0166, -0.0989, 0.0747, -0.0, 0.0306, -0.0717, -0.007, 0.0665, 0.0452, 0.0123, -0.0238, 0.0512, -0.0116, 0.0517, 0.0288, -0.0013, 0.0176, 0.0762, 0.1284, -0.031, 0.0891, -0.0286, 0.0132, 0.003, 0.0433, 0.0102, -0.0209, -0.0459, -0.0312, -0.0387, 0.0201, -0.027, 0.0243, 0.0713, 0.0359, -0.0674, -0.0747, -0.0147, 0.0489, -0.0092, -0.018, 0.0236, 0.0372, -0.0071, -0.0513, -0.0396, -0.0316, -0.0297, -0.0385, -0.062, 0.0465, 0.0539, -0.033, 0.0643, 0.061, 0.0062, 0.0245, 0.0868, 0.0523, -0.0253, 0.0157, 0.0266, 0.0124, 0.1382, -0.0107, 0.0835, -0.1057, -0.0188, -0.0786, 0.057, 0.0707, -0.0185, 0.0708, 0.0189, -0.0374, -0.0484, 0.0089, 0.0247, 0.0255, -0.0118, 0.0739, 0.0114, -0.0448, -0.016, -0.0836, 0.0107, 0.0067, -0.0535, -0.0186, -0.0042, 0.0582, -0.0731, -0.0593, 0.0299, 0.0004, -0.0299, 0.0128, -0.0549, 0.0493, 0.0, -0.0419, 0.0549, -0.0315, 0.1012, 0.0459, -0.0628, 0.0417, -0.0153, 0.0471, -0.0301, -0.0615, 0.0137, -0.0219, 0.0735, 0.083, 0.0114, -0.0326, -0.0272, 0.0642, -0.0203, 0.0557, -0.0579, 0.0883, 0.0719, 0.0007, 0.0598, -0.0431, -0.0189, -0.0593, -0.0334, 0.02, -0.0371, -0.0441, 0.0407, -0.0805, 0.0058, 0.1039, 0.0534, 0.0495, -0.0325, 0.0782, -0.0403, 0.0108, -0.0068, -0.0525, 0.0801, 0.0256, -0.0183, -0.0619, -0.0063, -0.0605, 0.0377, -0.0281, -0.0097, -0.0029, -0.106, 0.0465, -0.0033, -0.0308, 0.0357, 0.0156, -0.0406, -0.0308, 0.0013, 0.0458, 0.0231, 0.0207, -0.0828, -0.0573, 0.0298, -0.0381, 0.0935, -0.0498, -0.0979, -0.1452, 0.0835, -0.0973, -0.0172, 0.0003, 0.09, -0.0931, -0.0252, 0.008, -0.0441, -0.0938, -0.0021, 0.0885, 0.0088, 0.0034, -0.0049, 0.0217, 0.0584, -0.012, 0.059, 0.0146, -0.0, -0.0045, 0.0663, 0.0017, 0.0015, 0.0569, -0.0089, -0.0232, 0.0065, 0.0204, -0.0253, 0.1119, -0.036, 0.0125, 0.0531, 0.0584, -0.0101, -0.0593, -0.0577, -0.0656, -0.0396, 0.0525, -0.006, -0.0149, 0.003, -0.1009, -0.0281, 0.0311, -0.0088, 0.0441, -0.0056, 0.0715, 0.051, 0.0219, -0.0028, 0.0294, -0.0969, -0.0852, 0.0304, 0.0374, 0.1078, -0.0559, 0.0805, -0.0464, 0.0369, 0.0874, -0.0251, 0.0075, -0.0502, -0.0181, -0.1059, 0.0111, 0.0894, 0.0021, 0.0838, 0.0497, -0.0183, 0.0246, -0.004, -0.0828, 0.06, -0.1161, -0.0367, 0.0475, 0.0317]) AS distance
FROM default.wiki_abstract
ORDER BY distance ASC
LIMIT 5;
We're committed to continuously improving and evolving MyScaleDB to meet the ever-changing needs of the AI industry. Join us on this exciting journey and be part of the revolution in AI data management!
-
Get the latest MyScaleDB news or updates
- Follow @MyScaleDB on Twitter
- Follow @MyScale on LinkedIn
- Read MyScale Blog
- [x] Inverted index & performant keyword/vector hybrid search (supported since 1.5)
- [ ] Support more storage engines, e.g.
ReplacingMergeTree
- [ ] LLM observability with MyScaleDB
- [ ] Data-centric LLM
- [ ] Automatic data science with MyScaleDB
MyScaleDB is licensed under the Apache License, Version 2.0. View a copy of the License file.
We give special thanks for these open-source projects, upon which we have developed MyScaleDB:
- ClickHouse - A free analytics DBMS for big data.
- Faiss - A library for efficient similarity search and clustering of dense vectors, by Meta's Fundamental AI Research.
- hnswlib - Header-only C++/python library for fast approximate nearest neighbors.
- ScaNN - Scalable Nearest Neighbors library by Google Research.
- Tantivy - A full-text search engine library inspired by Apache Lucene and written in Rust.
-
See why metadata filtering is crucial for imporoving RAG accuracy here. ↩
-
The MSTG (Multi-scale Tree Graph) algorithm is provided through MyScale Cloud, achieving high data density with disk-based storage and better indexing & search performance on billion-scale vector data. ↩
For Tasks:
Click tags to check more tools for each tasksFor Jobs:
Alternative AI tools for MyScaleDB
Similar Open Source Tools
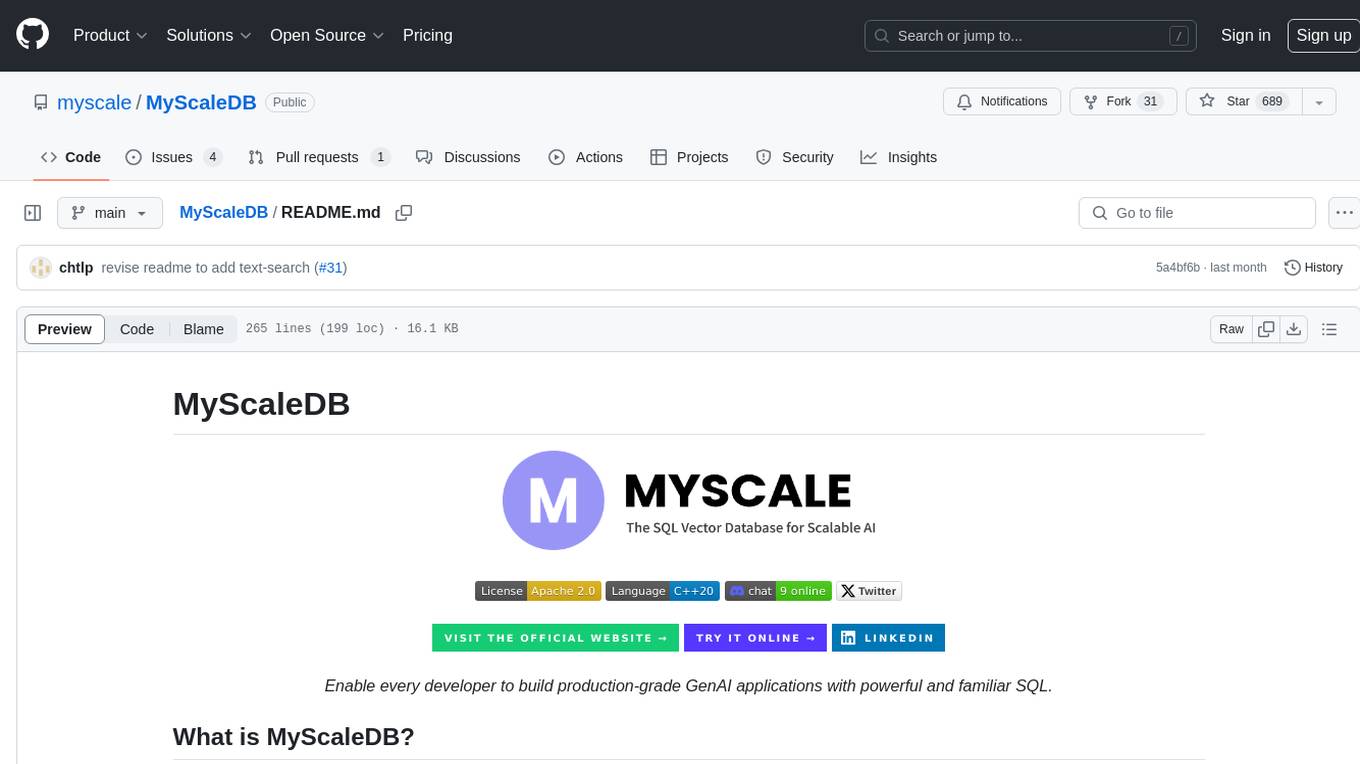
MyScaleDB
MyScaleDB is a SQL vector database optimized for AI applications, enabling developers to manage and process massive volumes of data efficiently. It offers fast and powerful vector search, filtered search, and SQL-vector join queries, making it fully SQL-compatible. MyScaleDB provides unmatched performance and scalability by leveraging cutting-edge OLAP database architecture and advanced vector algorithms. It is production-ready for AI applications, supporting structured data, text, vector, JSON, geospatial, and time-series data. MyScale Cloud offers fully-managed MyScaleDB with premium features on billion-scale data, making it cost-effective and simpler to use compared to specialized vector databases. Built on top of ClickHouse, MyScaleDB combines structured and vector search efficiently, ensuring high accuracy and performance in filtered search operations.
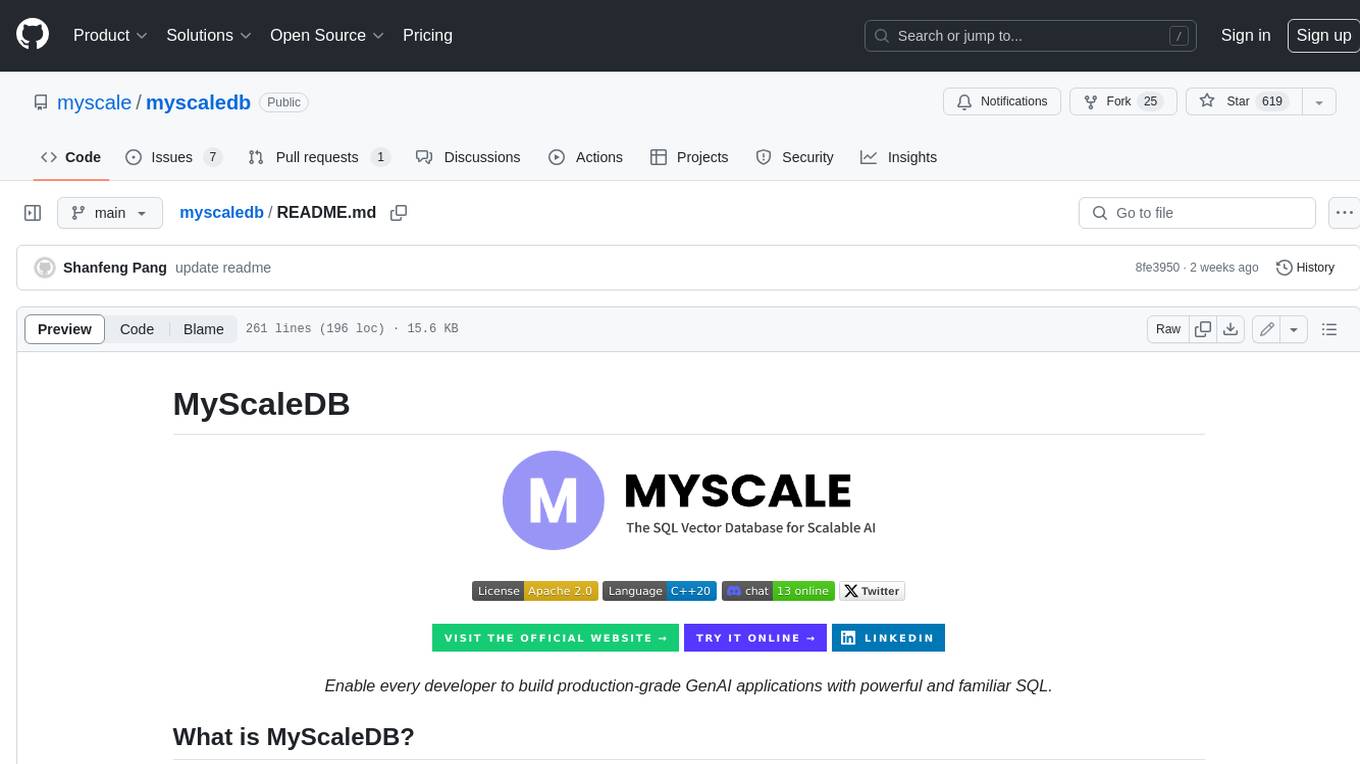
myscaledb
MyScaleDB is a SQL vector database designed for scalable AI applications, enabling developers to efficiently manage and process massive volumes of data using familiar SQL. It offers fast and efficient vector search, filtered search, and SQL-vector join queries. MyScaleDB is fully SQL-compatible and production-ready for AI applications, providing unmatched performance and scalability through cutting-edge OLAP architecture and advanced vector algorithms. Built on top of ClickHouse, it combines structured and vectorized data management for high accuracy and speed in filtered searches.
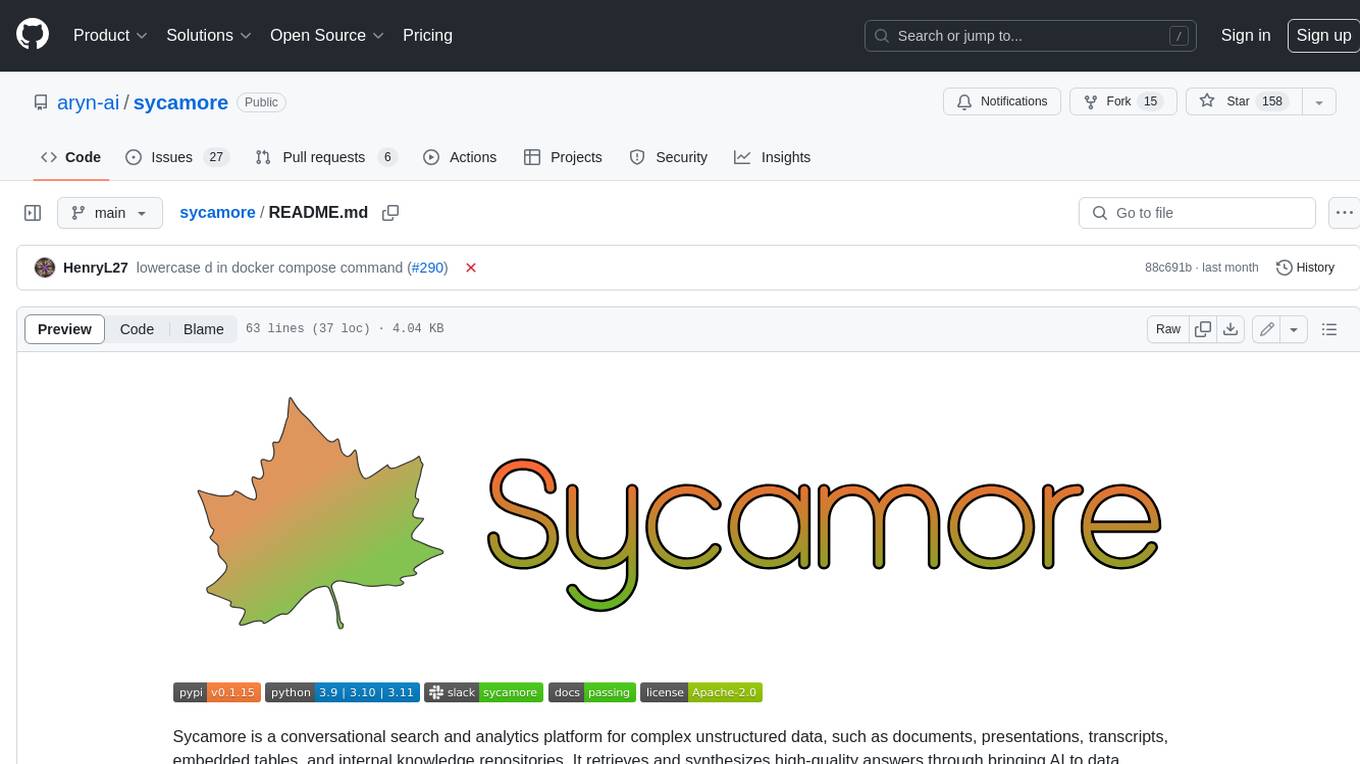
sycamore
Sycamore is a conversational search and analytics platform for complex unstructured data, such as documents, presentations, transcripts, embedded tables, and internal knowledge repositories. It retrieves and synthesizes high-quality answers through bringing AI to data preparation, indexing, and retrieval. Sycamore makes it easy to prepare unstructured data for search and analytics, providing a toolkit for data cleaning, information extraction, enrichment, summarization, and generation of vector embeddings that encapsulate the semantics of data. Sycamore uses your choice of generative AI models to make these operations simple and effective, and it enables quick experimentation and iteration. Additionally, Sycamore uses OpenSearch for indexing, enabling hybrid (vector + keyword) search, retrieval-augmented generation (RAG) pipelining, filtering, analytical functions, conversational memory, and other features to improve information retrieval.
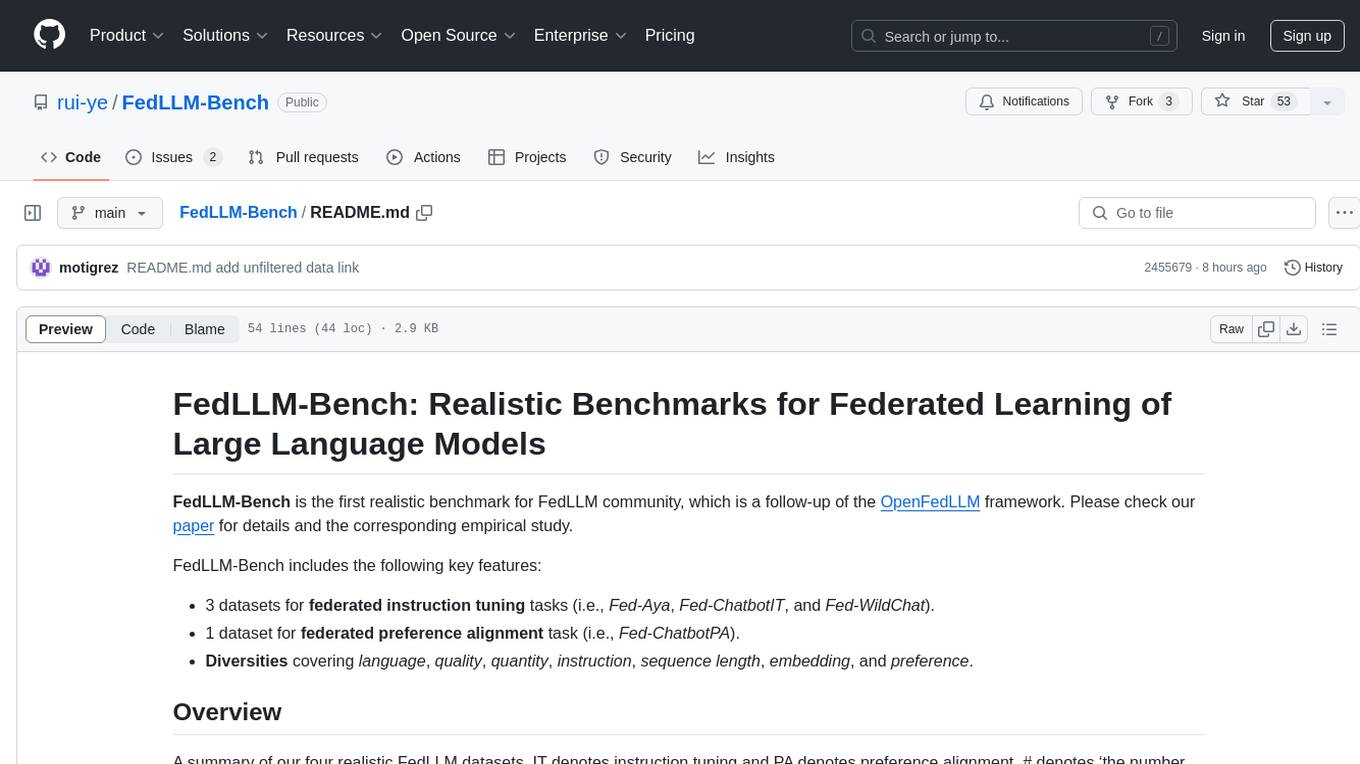
FedLLM-Bench
FedLLM-Bench is a realistic benchmark for the Federated Learning of Large Language Models community. It includes datasets for federated instruction tuning and preference alignment tasks, exhibiting diversities in language, quality, quantity, instruction, sequence length, embedding, and preference. The repository provides training scripts and code for open-ended evaluation, aiming to facilitate research and development in federated learning of large language models.
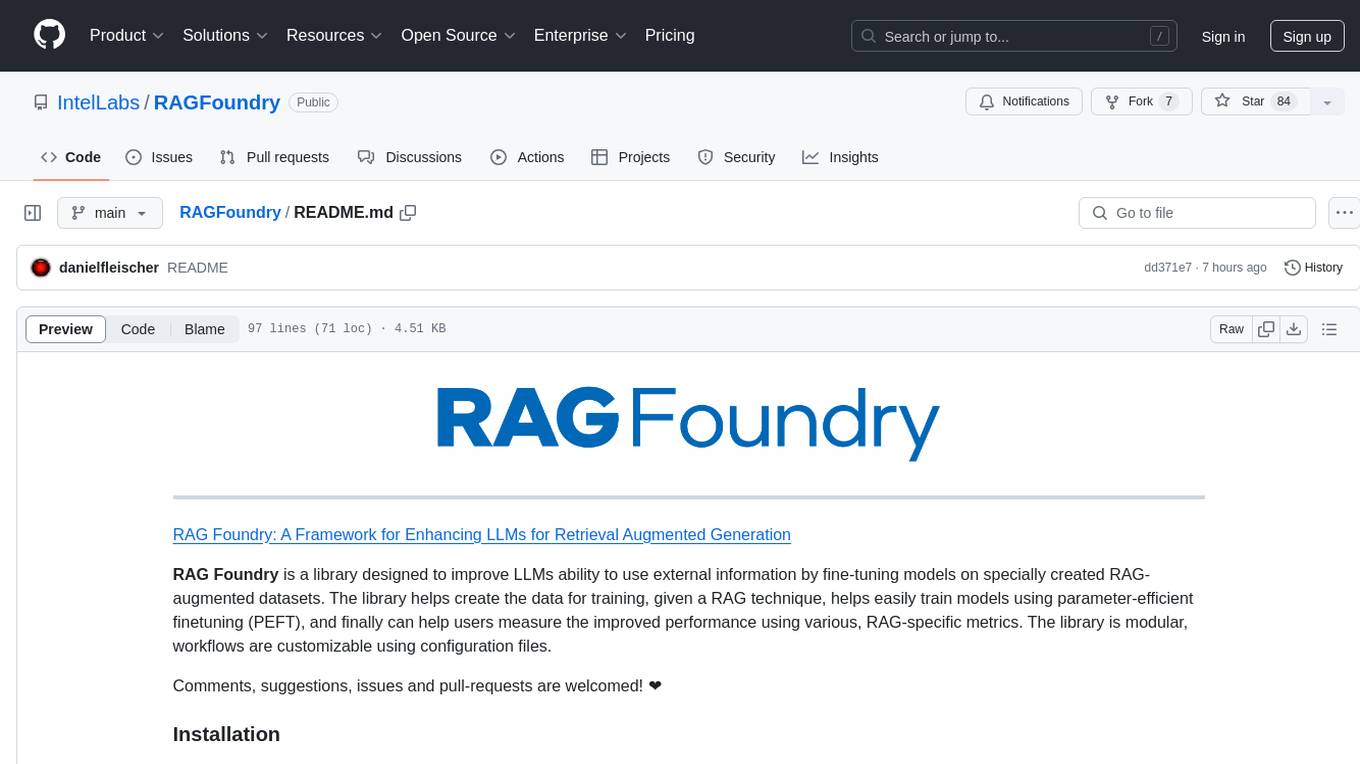
RAGFoundry
RAG Foundry is a library designed to enhance Large Language Models (LLMs) by fine-tuning models on RAG-augmented datasets. It helps create training data, train models using parameter-efficient finetuning (PEFT), and measure performance using RAG-specific metrics. The library is modular, customizable using configuration files, and facilitates prototyping with various RAG settings and configurations for tasks like data processing, retrieval, training, inference, and evaluation.
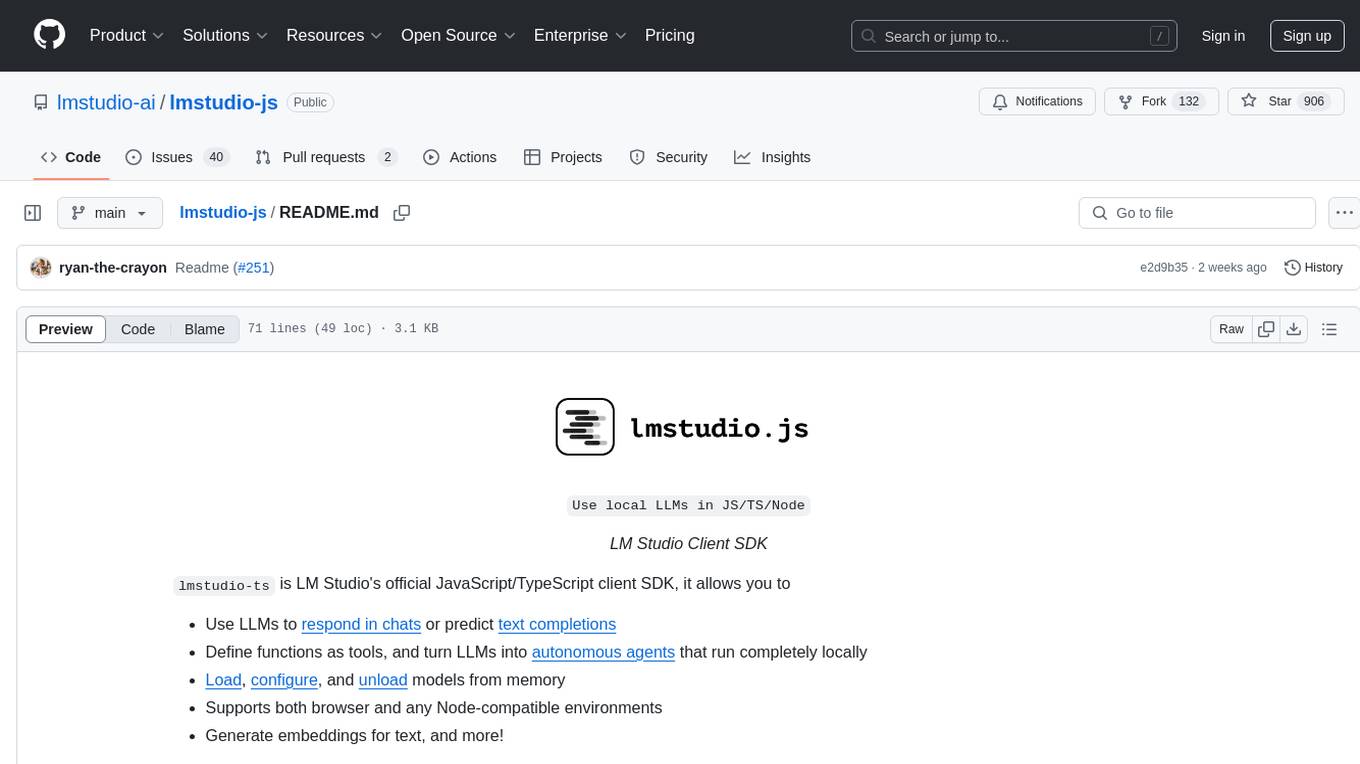
lmstudio-js
LM Studio Client SDK lmstudio-ts is LM Studio's official JavaScript/TypeScript client SDK. It allows you to use LLMs to respond in chats or predict text completions, define functions as tools, and turn LLMs into autonomous agents that run completely locally, load, configure, and unload models from memory, supports both browser and any Node-compatible environments, generate embeddings for text, and more! Why use `lmstudio-js` over `openai` sdk? Open AI's SDK is designed to use with Open AI's proprietary models. As such, it is missing many features that are essential for using LLMs in a local environment, such as managing loading and unloading models from memory, configuring load parameters (context length, gpu offload settings, etc.), speculative decoding, getting information (such as context length, model size, etc.) about a model, and more. In addition, while `openai` sdk is automatically generated, `lmstudio-js` is designed from ground-up to be clean and easy to use for TypeScript/JavaScript developers.
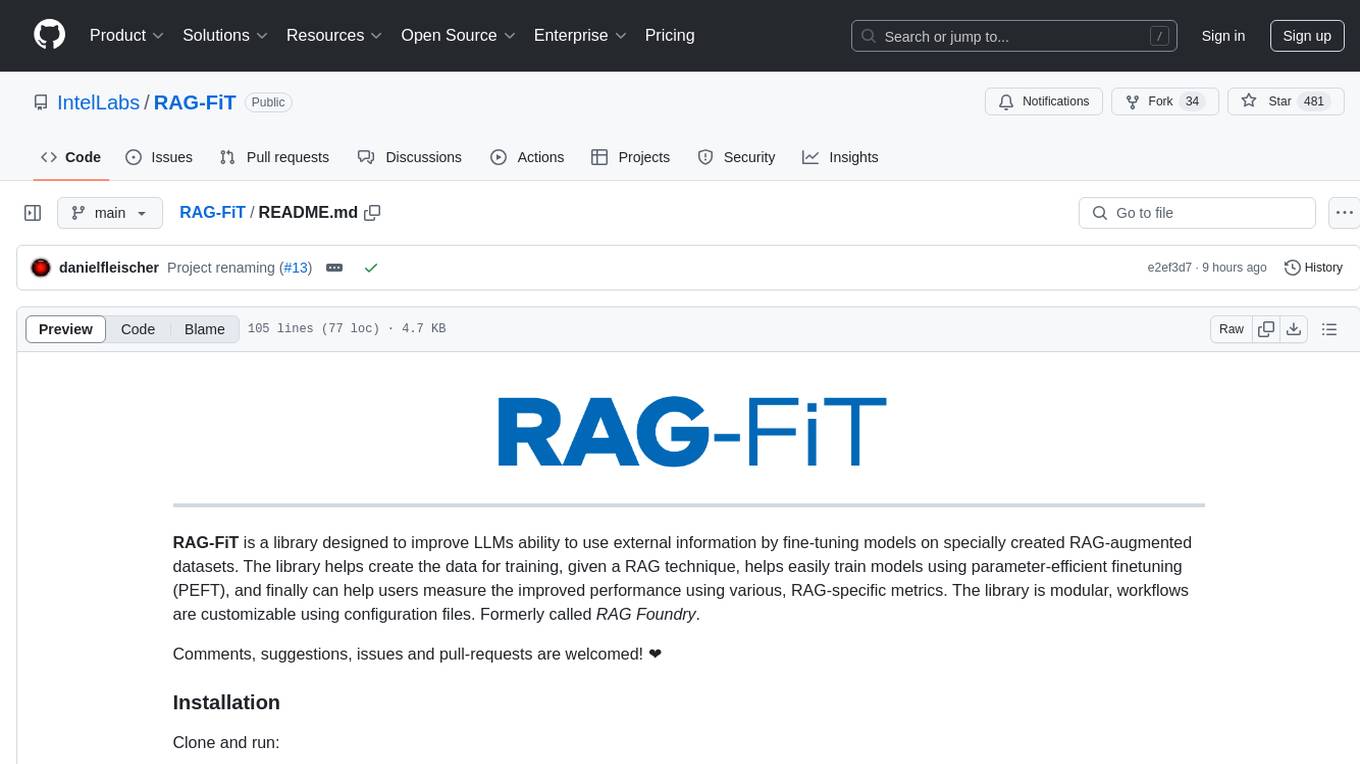
RAG-FiT
RAG-FiT is a library designed to improve Language Models' ability to use external information by fine-tuning models on specially created RAG-augmented datasets. The library assists in creating training data, training models using parameter-efficient finetuning (PEFT), and evaluating performance using RAG-specific metrics. It is modular, customizable via configuration files, and facilitates fast prototyping and experimentation with various RAG settings and configurations.
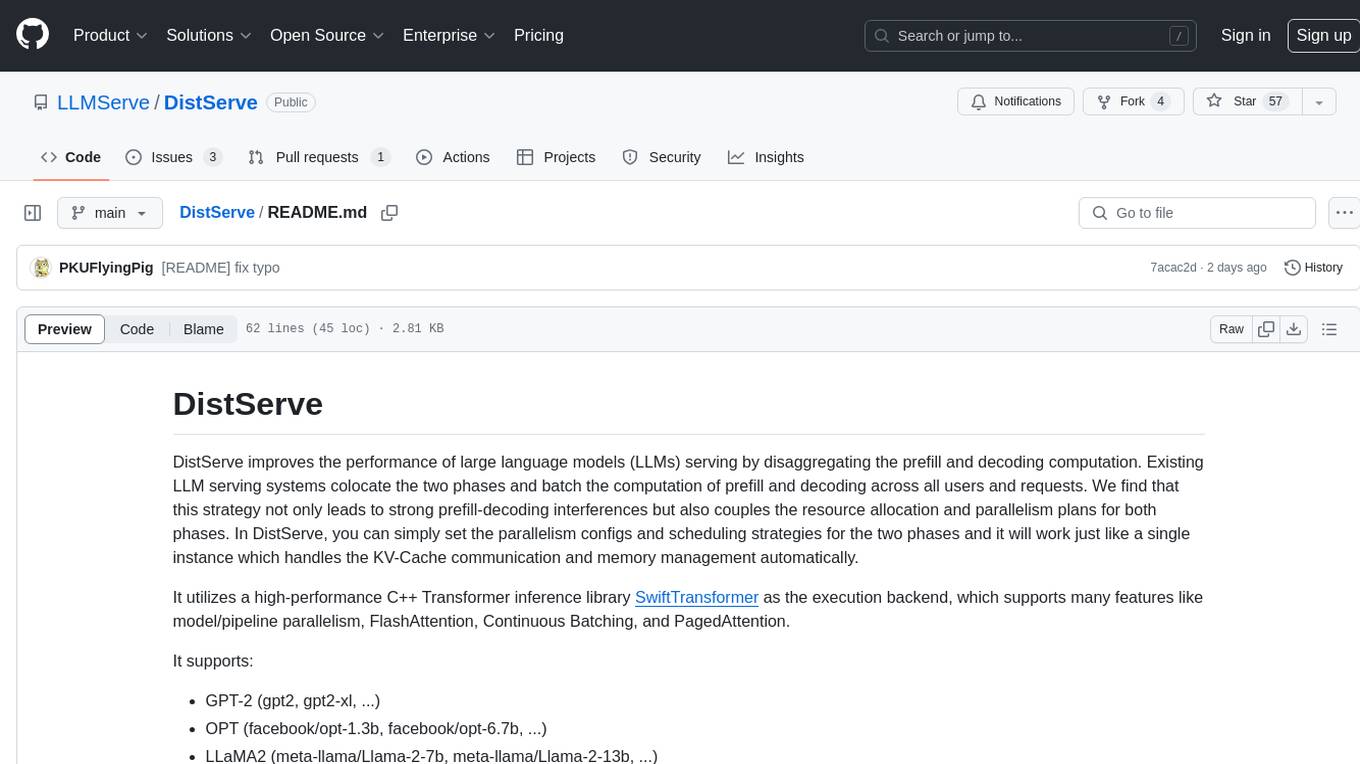
DistServe
DistServe improves the performance of large language models serving by disaggregating the prefill and decoding computation. It allows setting parallelism configs and scheduling strategies for the two phases independently, handling KV-Cache communication and memory management automatically. Utilizes a high-performance C++ Transformer inference library SwiftTransformer with features like model/pipeline parallelism, FlashAttention, Continuous Batching, and PagedAttention. Supports GPT-2, OPT, and LLaMA2 models.
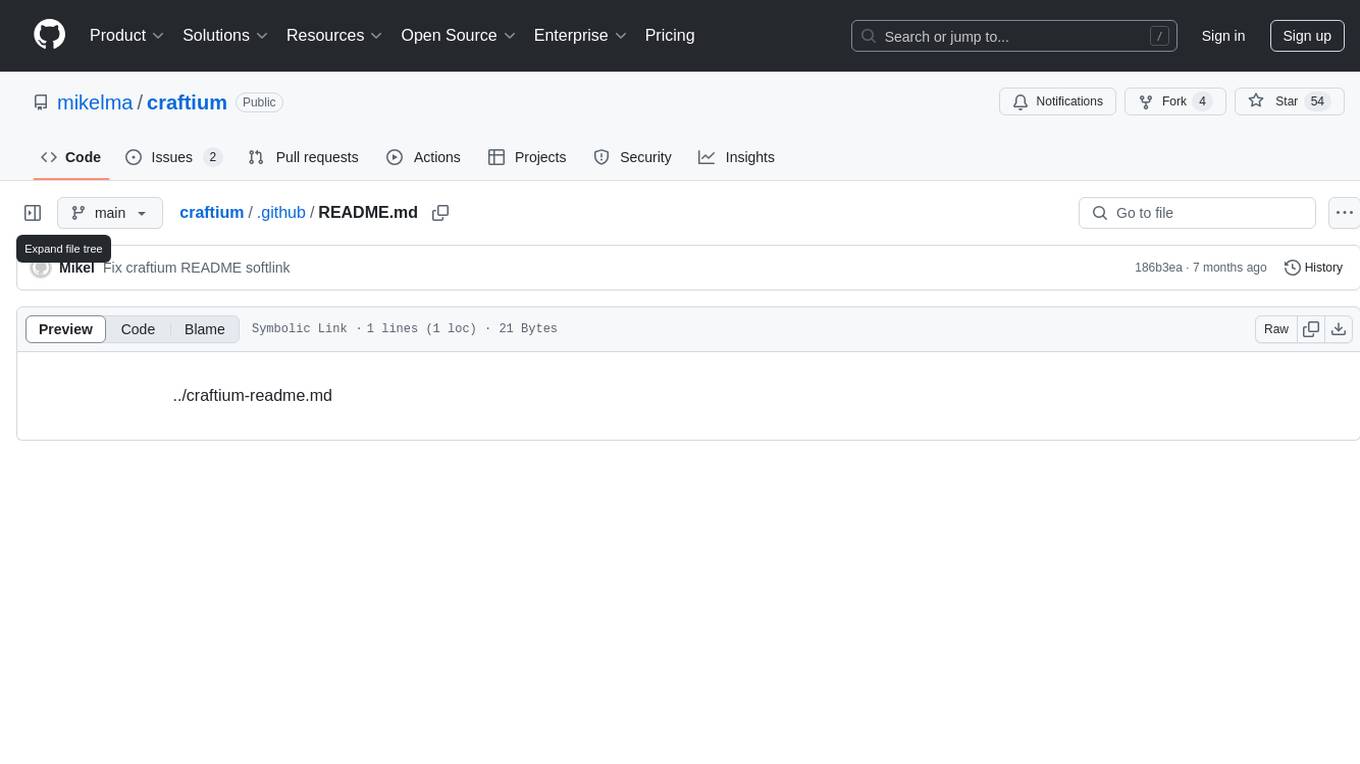
craftium
Craftium is an open-source platform based on the Minetest voxel game engine and the Gymnasium and PettingZoo APIs, designed for creating fast, rich, and diverse single and multi-agent environments. It allows for connecting to Craftium's Python process, executing actions as keyboard and mouse controls, extending the Lua API for creating RL environments and tasks, and supporting client/server synchronization for slow agents. Craftium is fully extensible, extensively documented, modern RL API compatible, fully open source, and eliminates the need for Java. It offers a variety of environments for research and development in reinforcement learning.
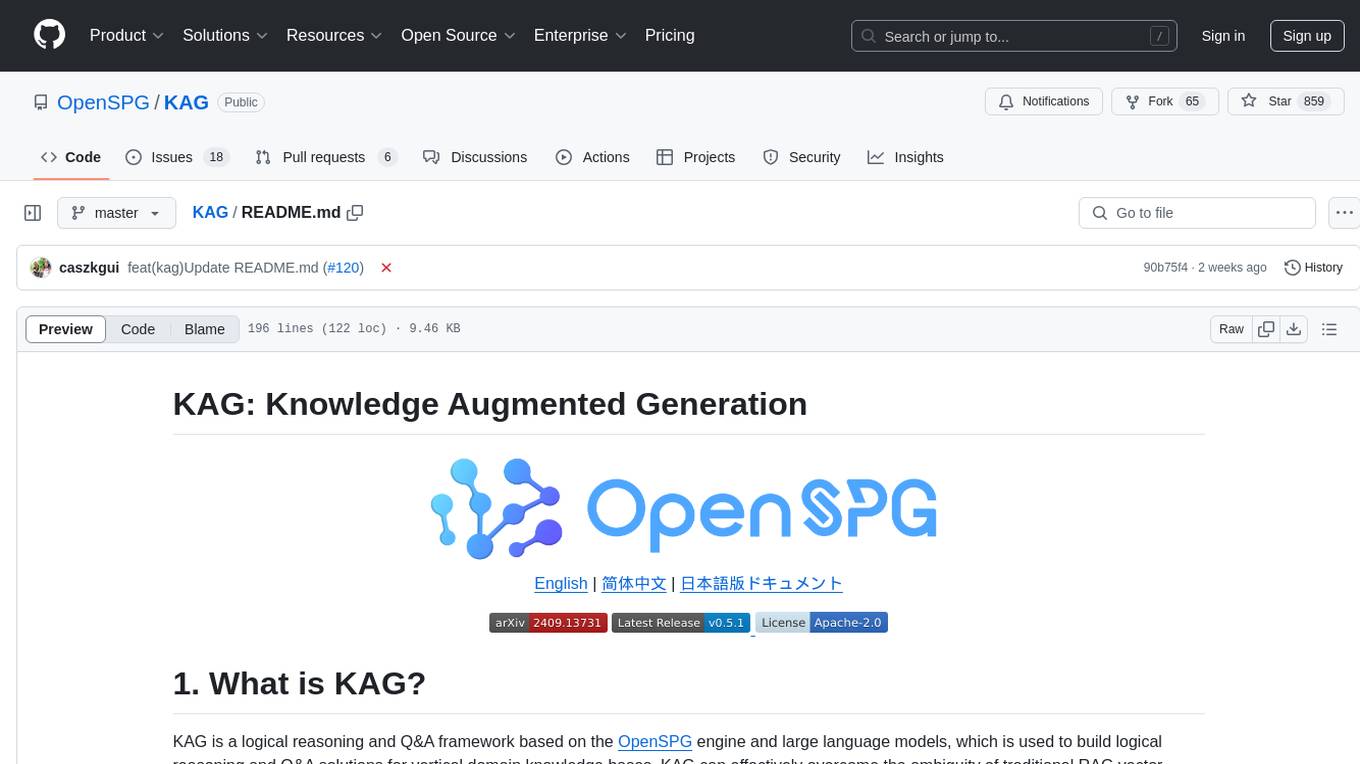
KAG
KAG is a logical reasoning and Q&A framework based on the OpenSPG engine and large language models. It is used to build logical reasoning and Q&A solutions for vertical domain knowledge bases. KAG supports logical reasoning, multi-hop fact Q&A, and integrates knowledge and chunk mutual indexing structure, conceptual semantic reasoning, schema-constrained knowledge construction, and logical form-guided hybrid reasoning and retrieval. The framework includes kg-builder for knowledge representation and kg-solver for logical symbol-guided hybrid solving and reasoning engine. KAG aims to enhance LLM service framework in professional domains by integrating logical and factual characteristics of KGs.
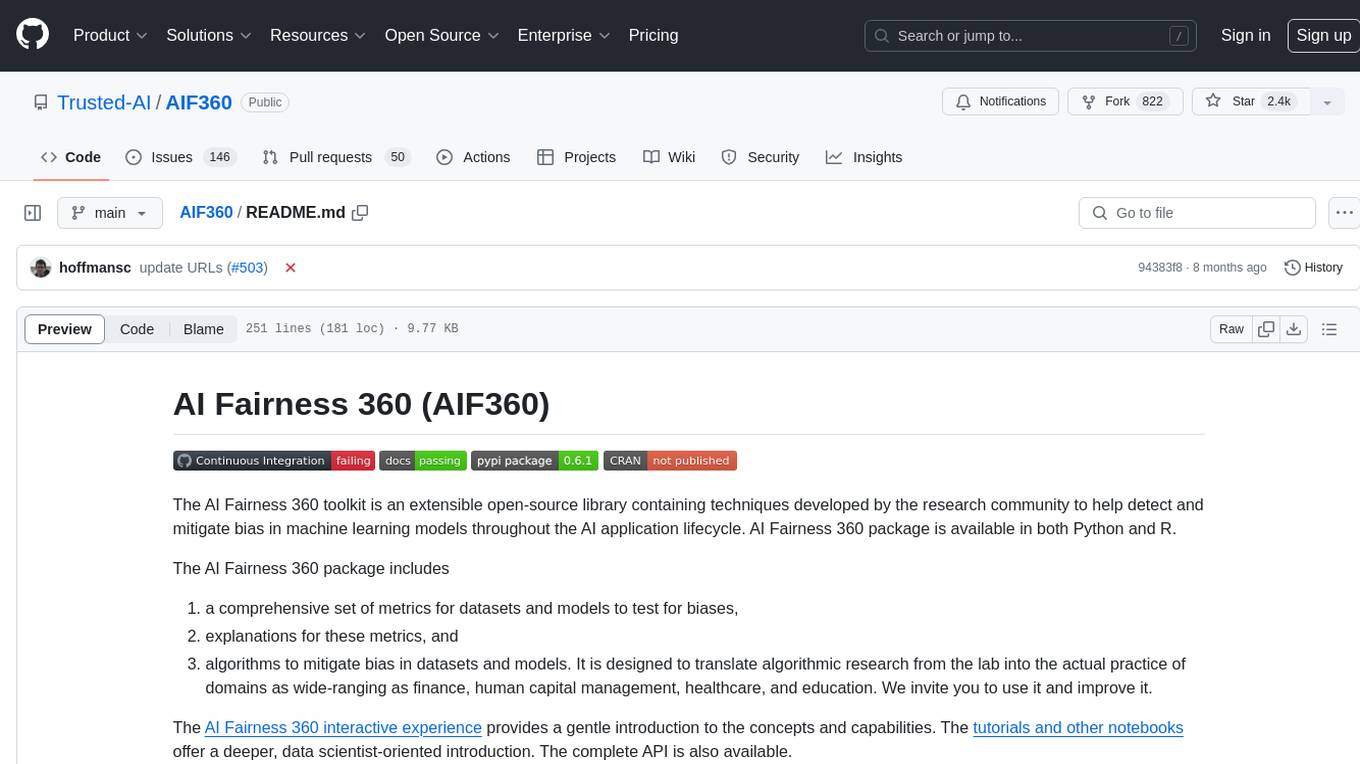
AIF360
The AI Fairness 360 toolkit is an open-source library designed to detect and mitigate bias in machine learning models. It provides a comprehensive set of metrics, explanations, and algorithms for bias mitigation in various domains such as finance, healthcare, and education. The toolkit supports multiple bias mitigation algorithms and fairness metrics, and is available in both Python and R. Users can leverage the toolkit to ensure fairness in AI applications and contribute to its development for extensibility.
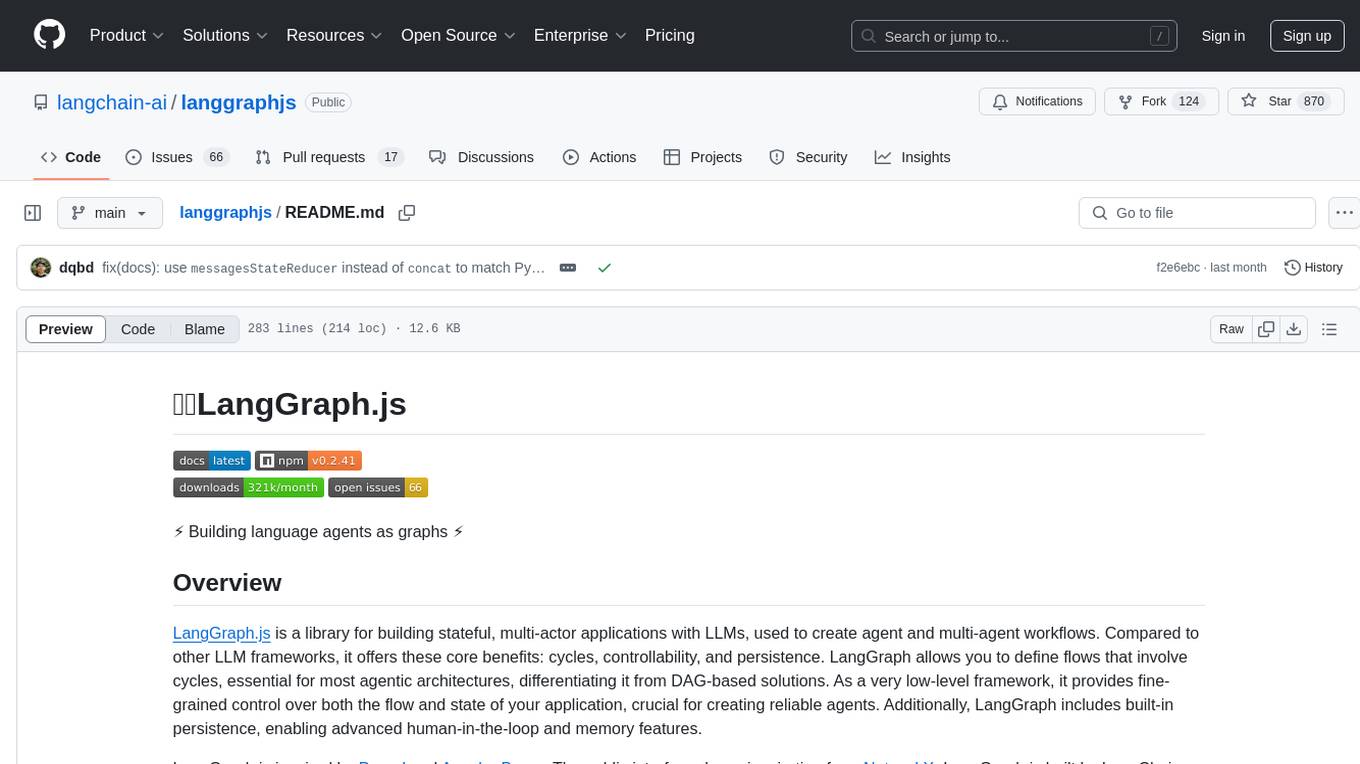
langgraphjs
LangGraph.js is a library for building stateful, multi-actor applications with LLMs, offering benefits such as cycles, controllability, and persistence. It allows defining flows involving cycles, providing fine-grained control over application flow and state. Inspired by Pregel and Apache Beam, it includes features like loops, persistence, human-in-the-loop workflows, and streaming support. LangGraph integrates seamlessly with LangChain.js and LangSmith but can be used independently.
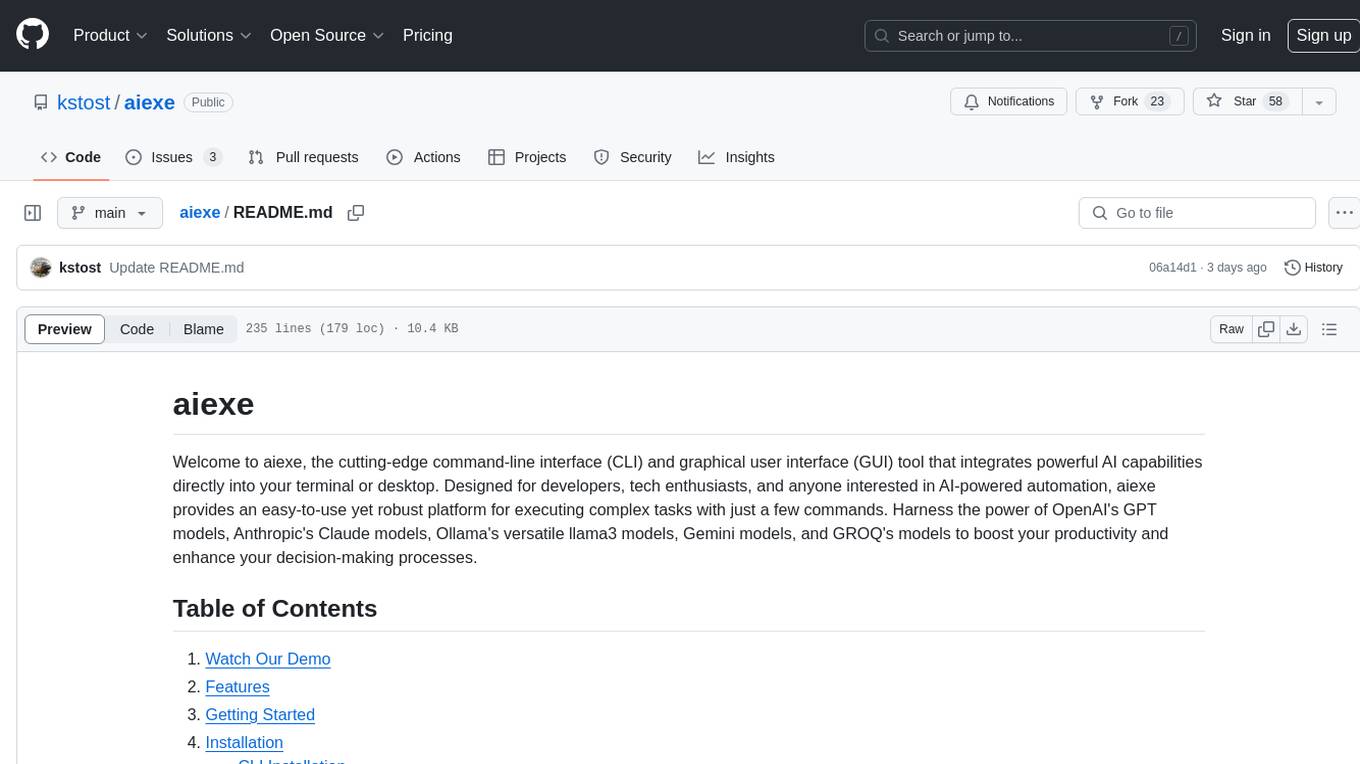
aiexe
aiexe is a cutting-edge command-line interface (CLI) and graphical user interface (GUI) tool that integrates powerful AI capabilities directly into your terminal or desktop. It is designed for developers, tech enthusiasts, and anyone interested in AI-powered automation. aiexe provides an easy-to-use yet robust platform for executing complex tasks with just a few commands. Users can harness the power of various AI models from OpenAI, Anthropic, Ollama, Gemini, and GROQ to boost productivity and enhance decision-making processes.
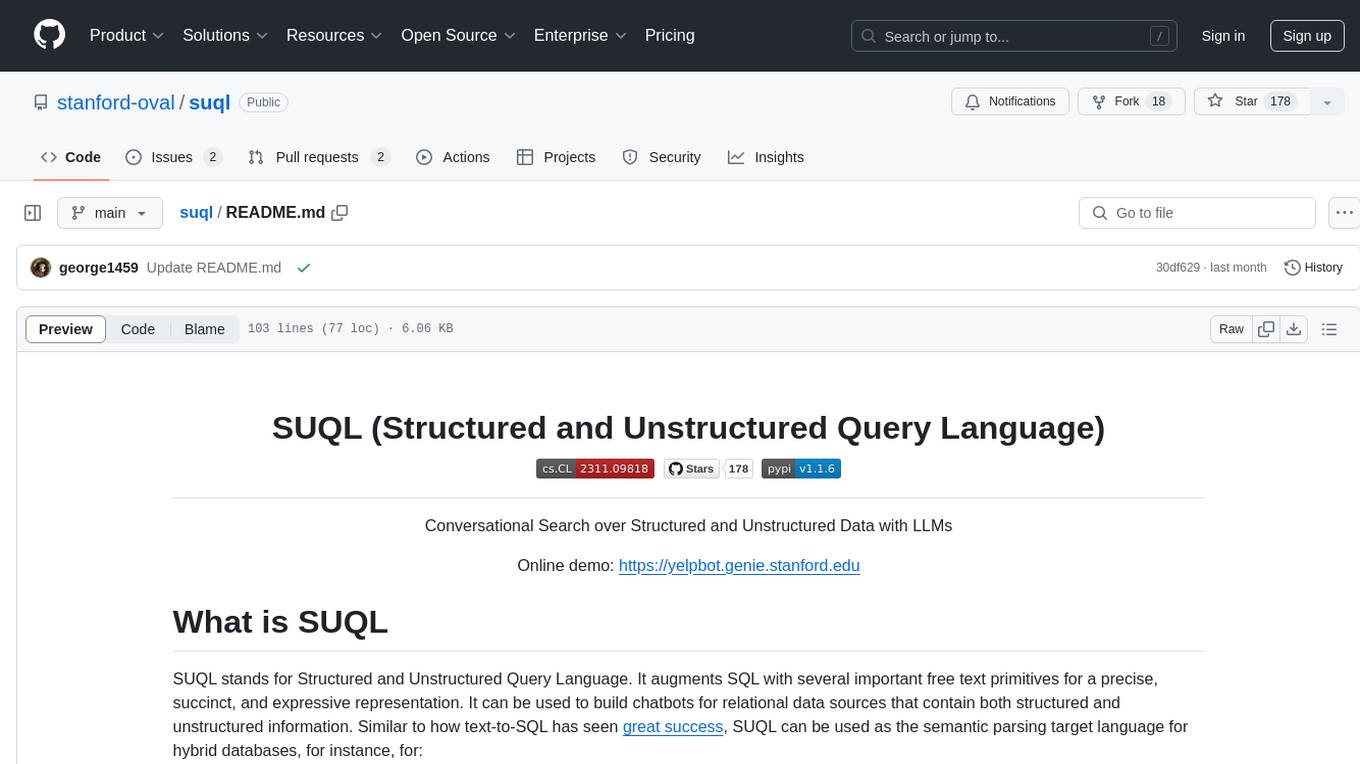
suql
SUQL (Structured and Unstructured Query Language) is a tool that augments SQL with free text primitives for building chatbots that can interact with relational data sources containing both structured and unstructured information. It seamlessly integrates retrieval models, large language models (LLMs), and traditional SQL to provide a clean interface for hybrid data access. SUQL supports optimizations to minimize expensive LLM calls, scalability to large databases with PostgreSQL, and general SQL operations like JOINs and GROUP BYs.
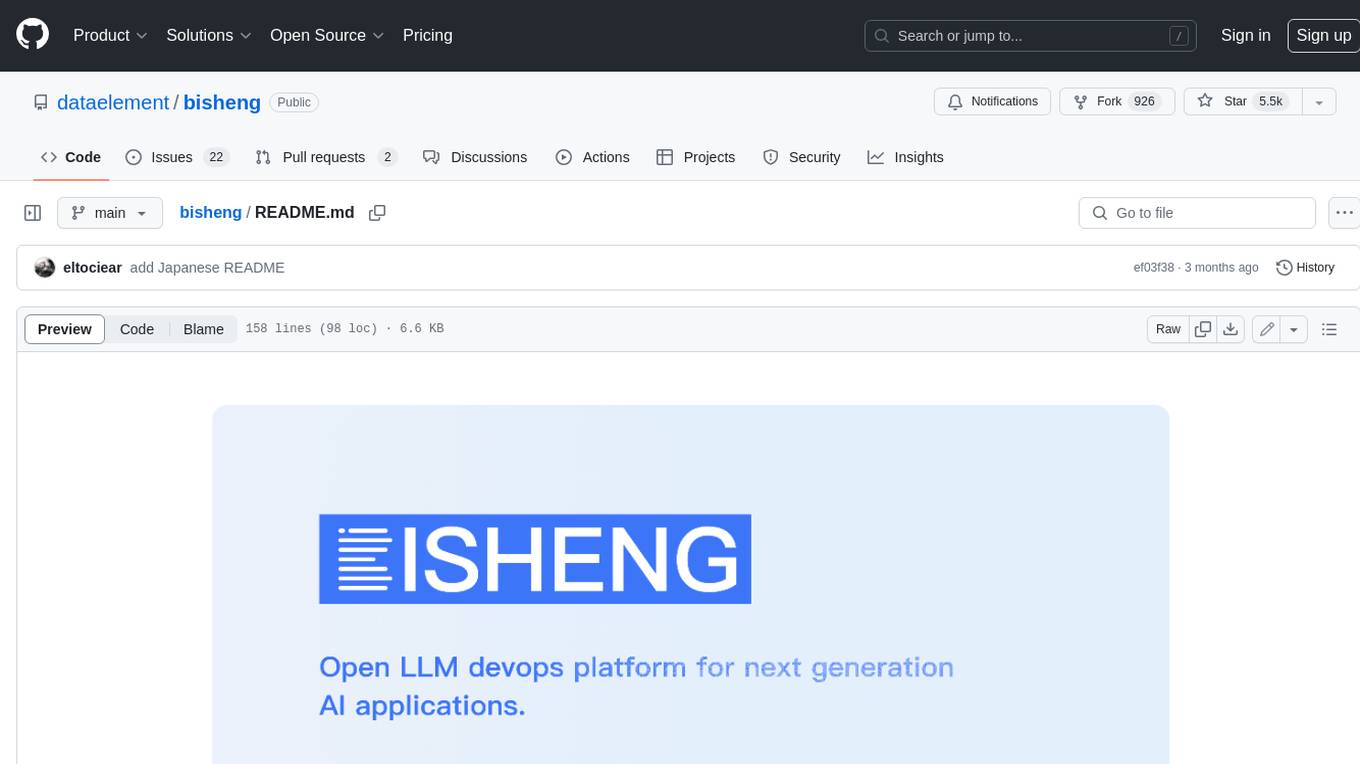
bisheng
Bisheng is a leading open-source **large model application development platform** that empowers and accelerates the development and deployment of large model applications, helping users enter the next generation of application development with the best possible experience.
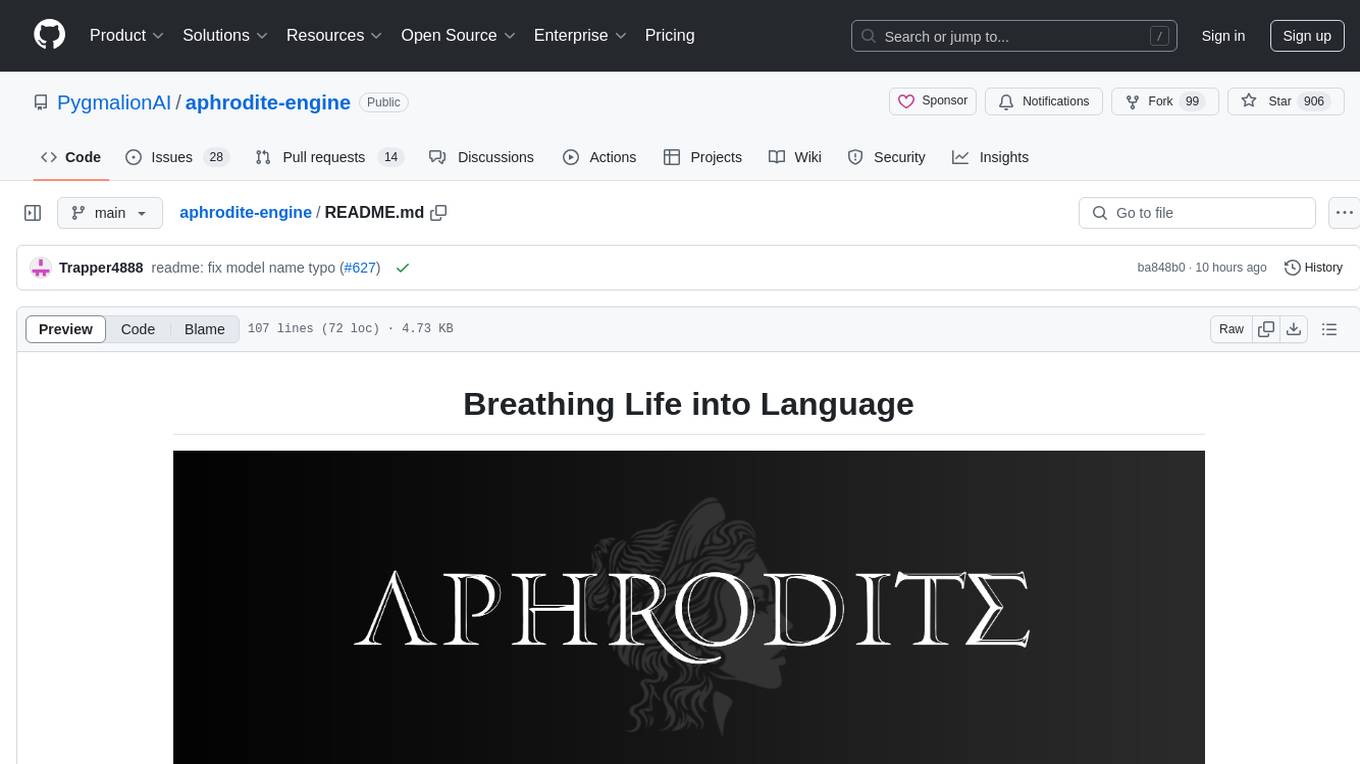
aphrodite-engine
Aphrodite is the official backend engine for PygmalionAI, serving as the inference endpoint for the website. It allows serving Hugging Face-compatible models with fast speeds. Features include continuous batching, efficient K/V management, optimized CUDA kernels, quantization support, distributed inference, and 8-bit KV Cache. The engine requires Linux OS and Python 3.8 to 3.12, with CUDA >= 11 for build requirements. It supports various GPUs, CPUs, TPUs, and Inferentia. Users can limit GPU memory utilization and access full commands via CLI.
For similar tasks
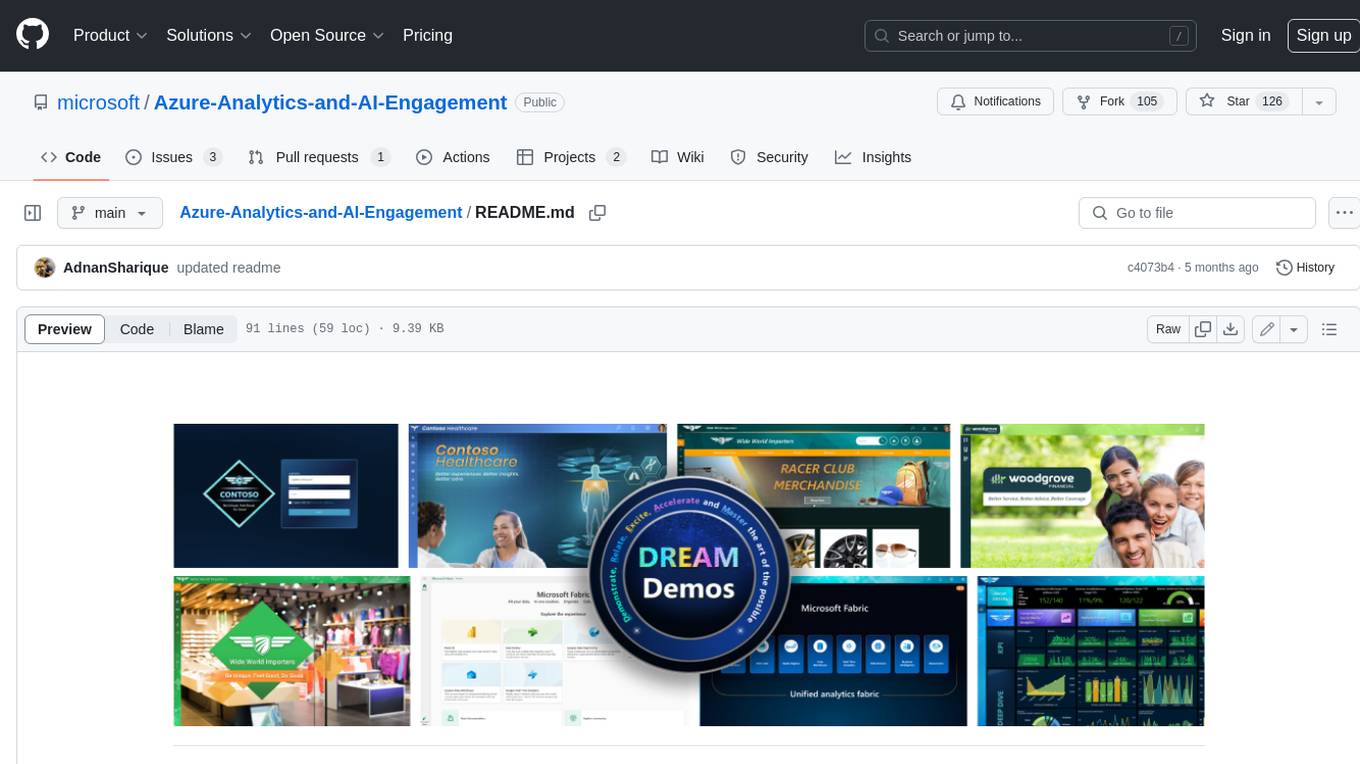
Azure-Analytics-and-AI-Engagement
The Azure-Analytics-and-AI-Engagement repository provides packaged Industry Scenario DREAM Demos with ARM templates (Containing a demo web application, Power BI reports, Synapse resources, AML Notebooks etc.) that can be deployed in a customer’s subscription using the CAPE tool within a matter of few hours. Partners can also deploy DREAM Demos in their own subscriptions using DPoC.
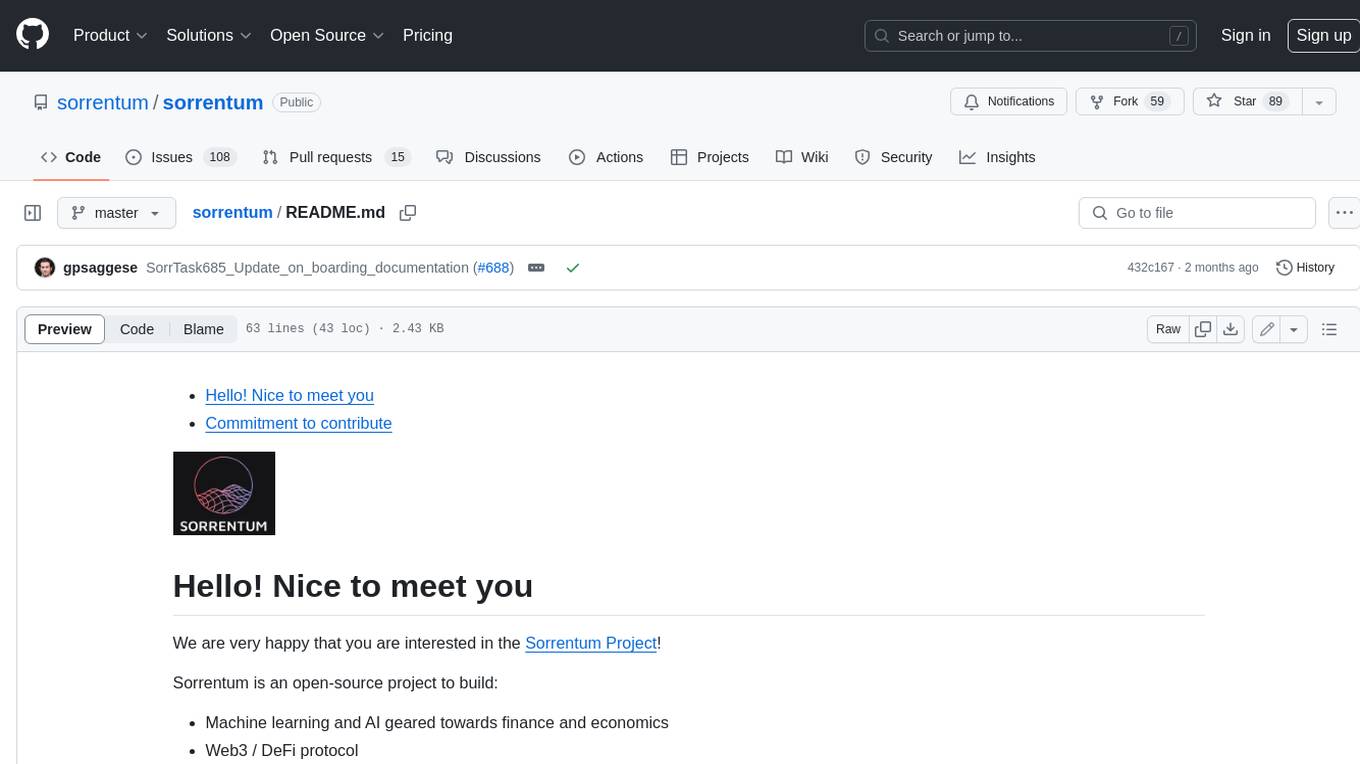
sorrentum
Sorrentum is an open-source project that aims to combine open-source development, startups, and brilliant students to build machine learning, AI, and Web3 / DeFi protocols geared towards finance and economics. The project provides opportunities for internships, research assistantships, and development grants, as well as the chance to work on cutting-edge problems, learn about startups, write academic papers, and get internships and full-time positions at companies working on Sorrentum applications.
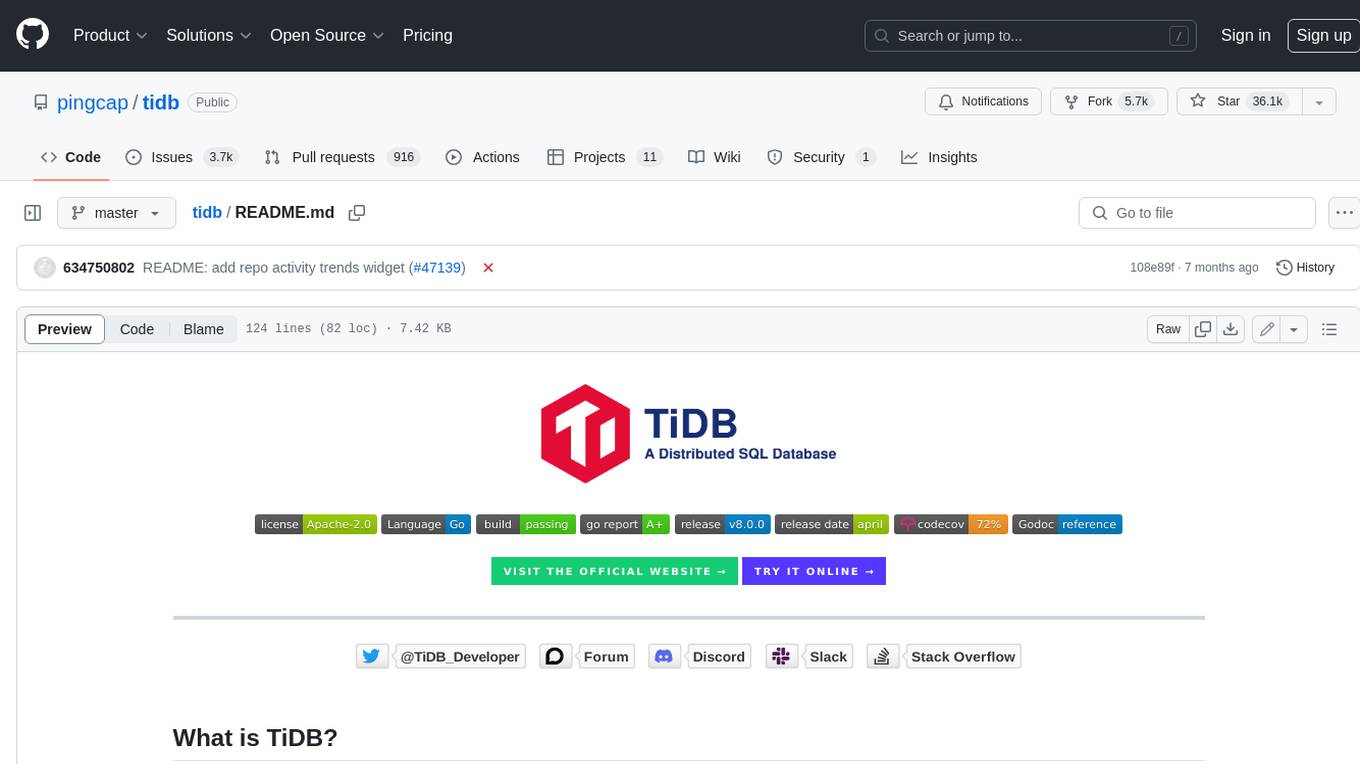
tidb
TiDB is an open-source distributed SQL database that supports Hybrid Transactional and Analytical Processing (HTAP) workloads. It is MySQL compatible and features horizontal scalability, strong consistency, and high availability.
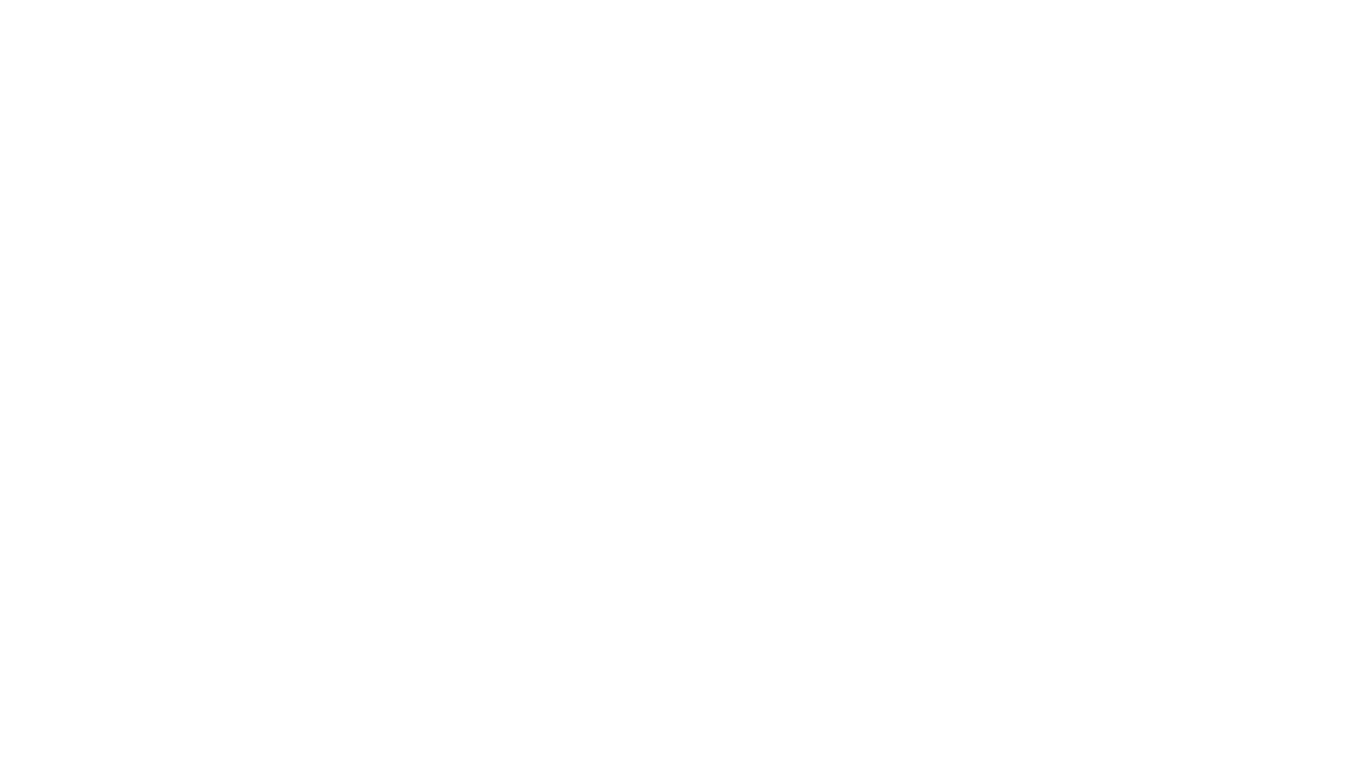
zep-python
Zep is an open-source platform for building and deploying large language model (LLM) applications. It provides a suite of tools and services that make it easy to integrate LLMs into your applications, including chat history memory, embedding, vector search, and data enrichment. Zep is designed to be scalable, reliable, and easy to use, making it a great choice for developers who want to build LLM-powered applications quickly and easily.
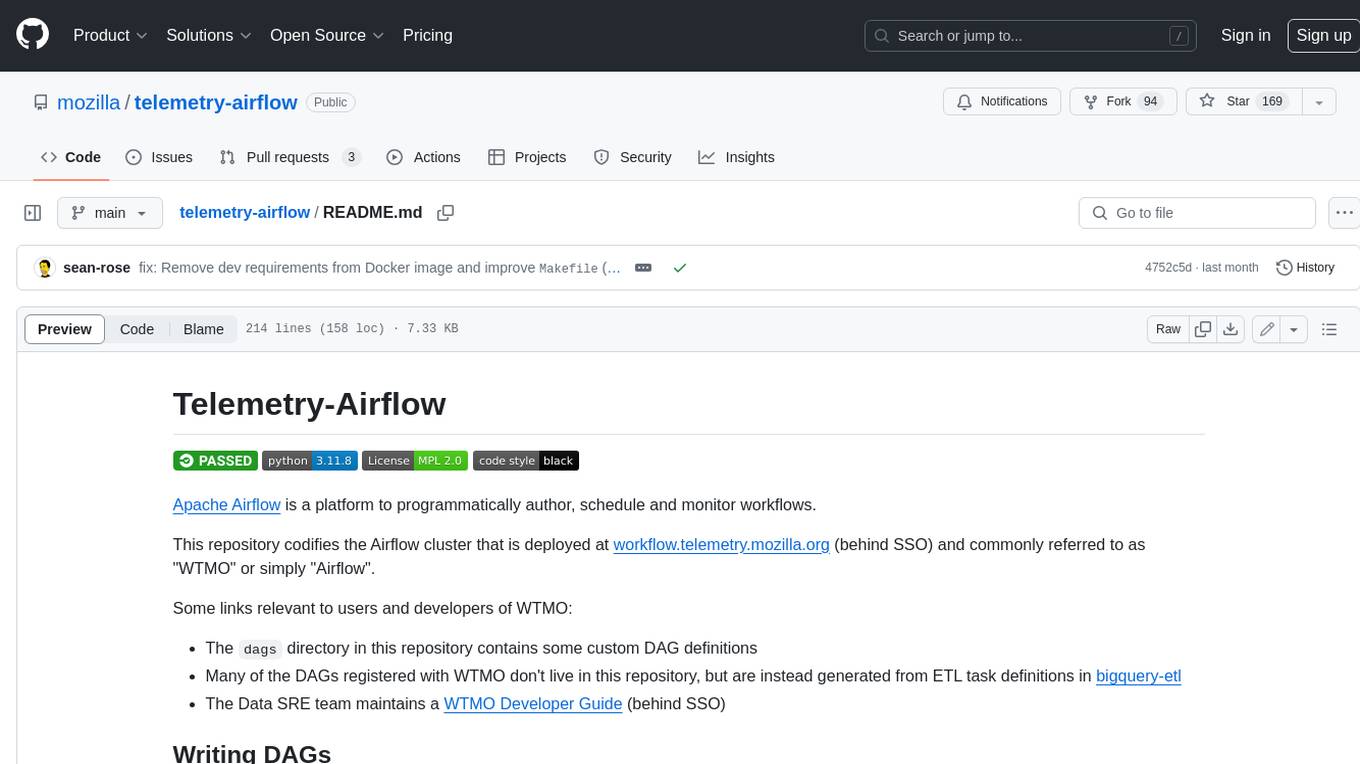
telemetry-airflow
This repository codifies the Airflow cluster that is deployed at workflow.telemetry.mozilla.org (behind SSO) and commonly referred to as "WTMO" or simply "Airflow". Some links relevant to users and developers of WTMO: * The `dags` directory in this repository contains some custom DAG definitions * Many of the DAGs registered with WTMO don't live in this repository, but are instead generated from ETL task definitions in bigquery-etl * The Data SRE team maintains a WTMO Developer Guide (behind SSO)
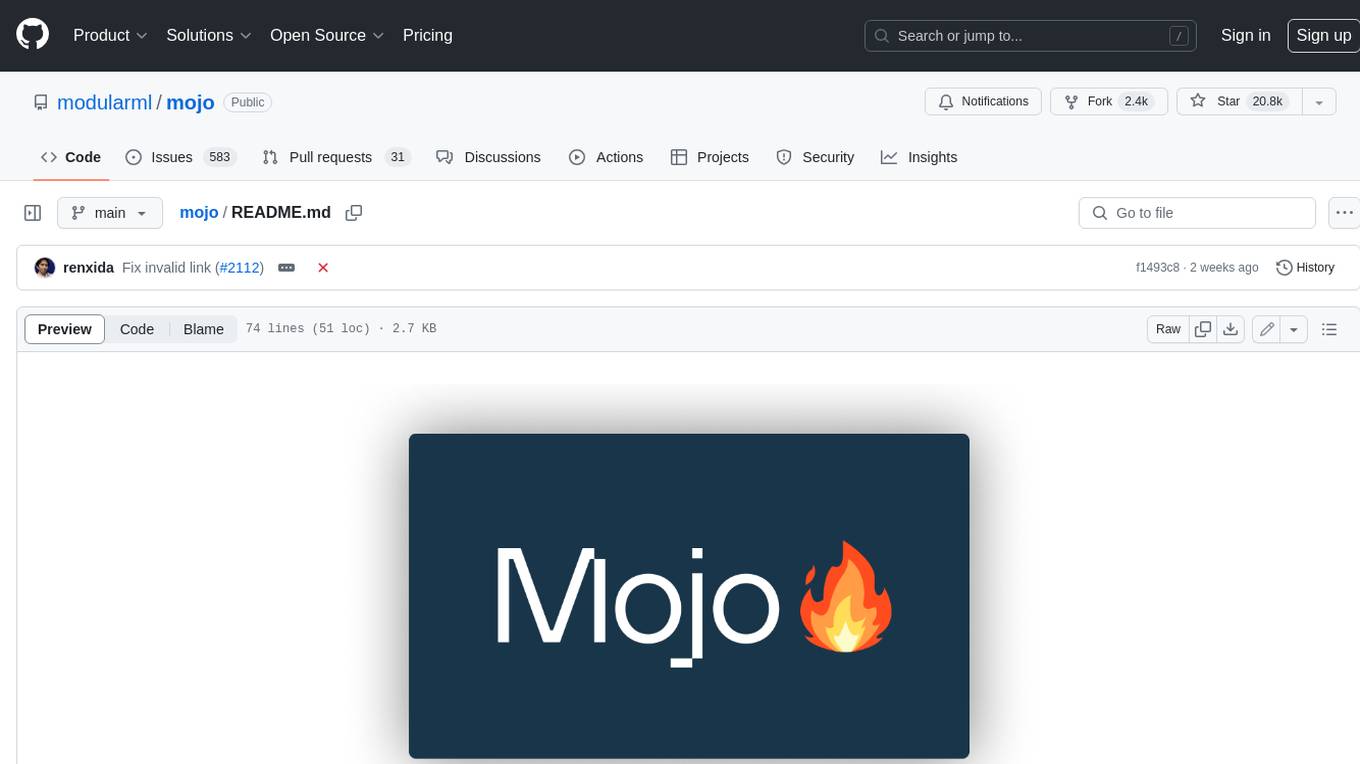
mojo
Mojo is a new programming language that bridges the gap between research and production by combining Python syntax and ecosystem with systems programming and metaprogramming features. Mojo is still young, but it is designed to become a superset of Python over time.
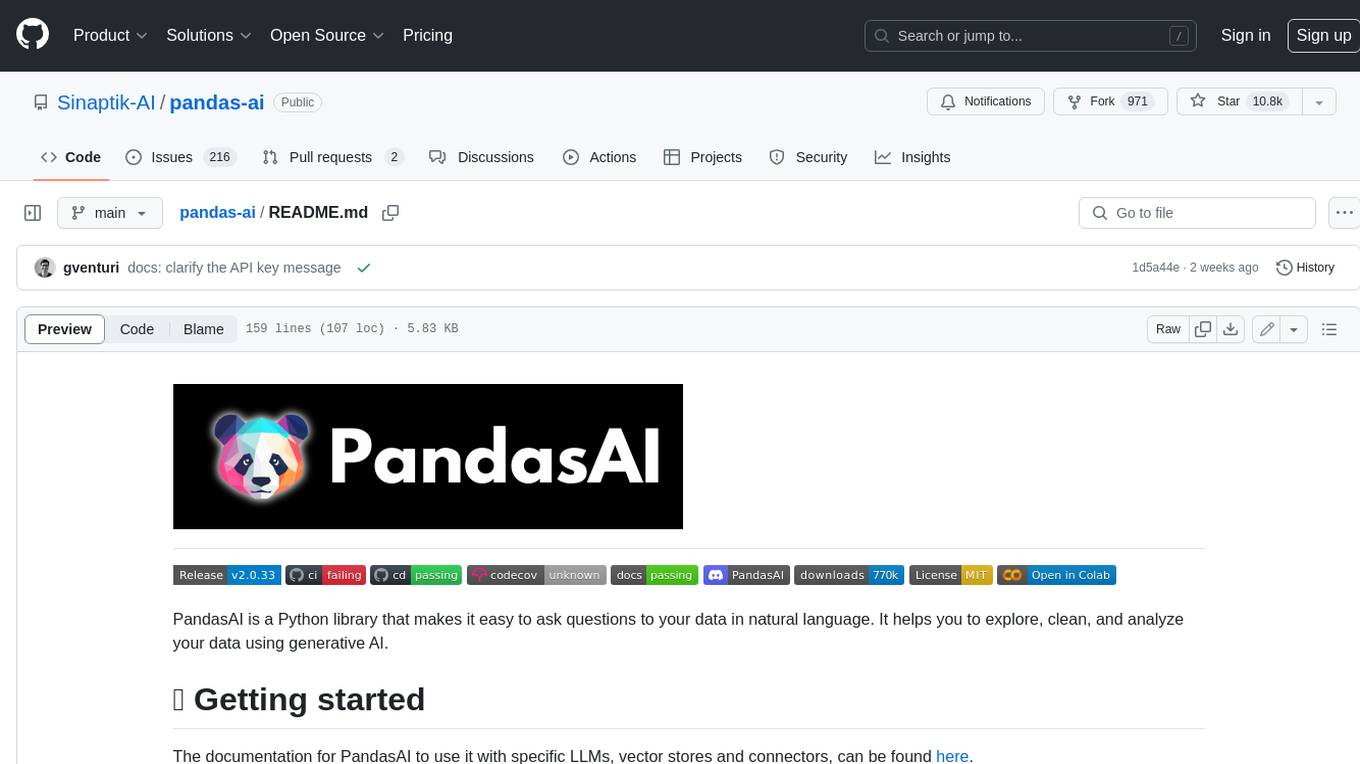
pandas-ai
PandasAI is a Python library that makes it easy to ask questions to your data in natural language. It helps you to explore, clean, and analyze your data using generative AI.
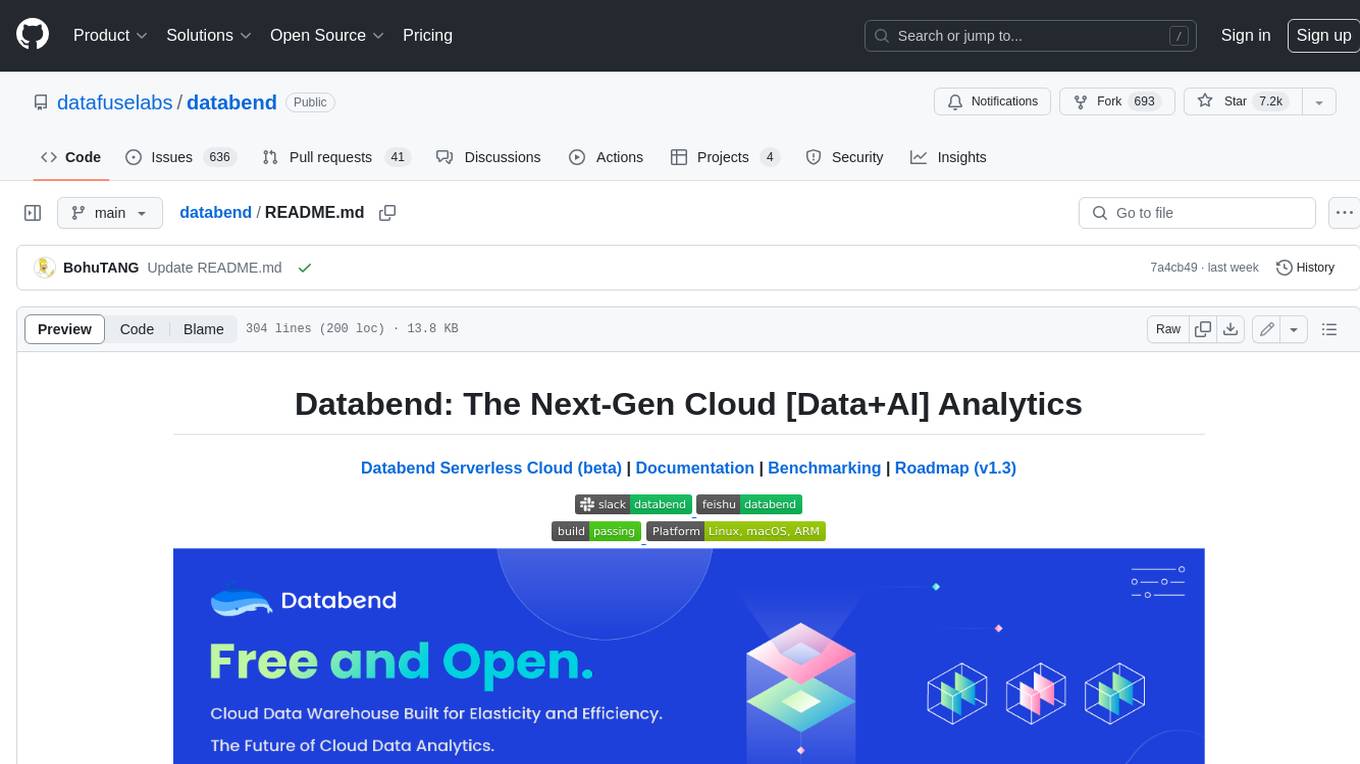
databend
Databend is an open-source cloud data warehouse that serves as a cost-effective alternative to Snowflake. With its focus on fast query execution and data ingestion, it's designed for complex analysis of the world's largest datasets.
For similar jobs
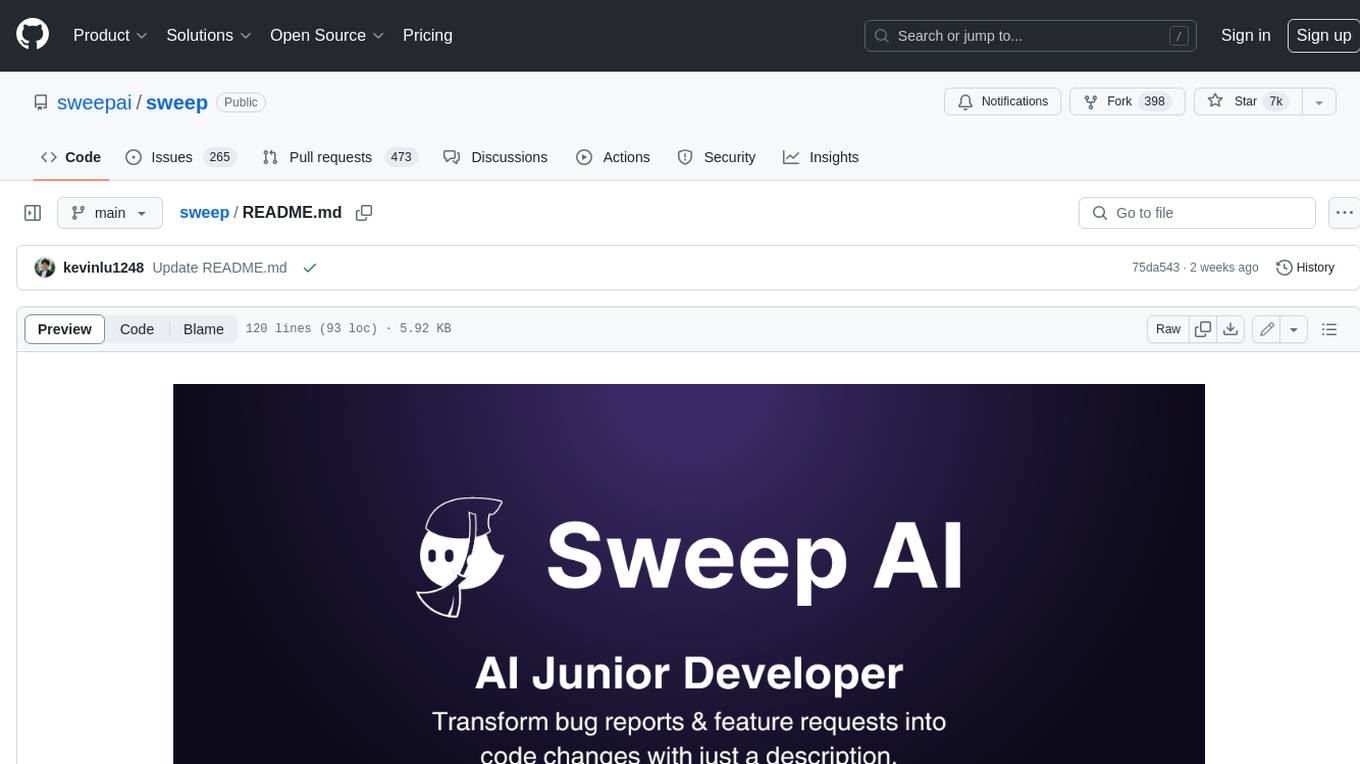
sweep
Sweep is an AI junior developer that turns bugs and feature requests into code changes. It automatically handles developer experience improvements like adding type hints and improving test coverage.
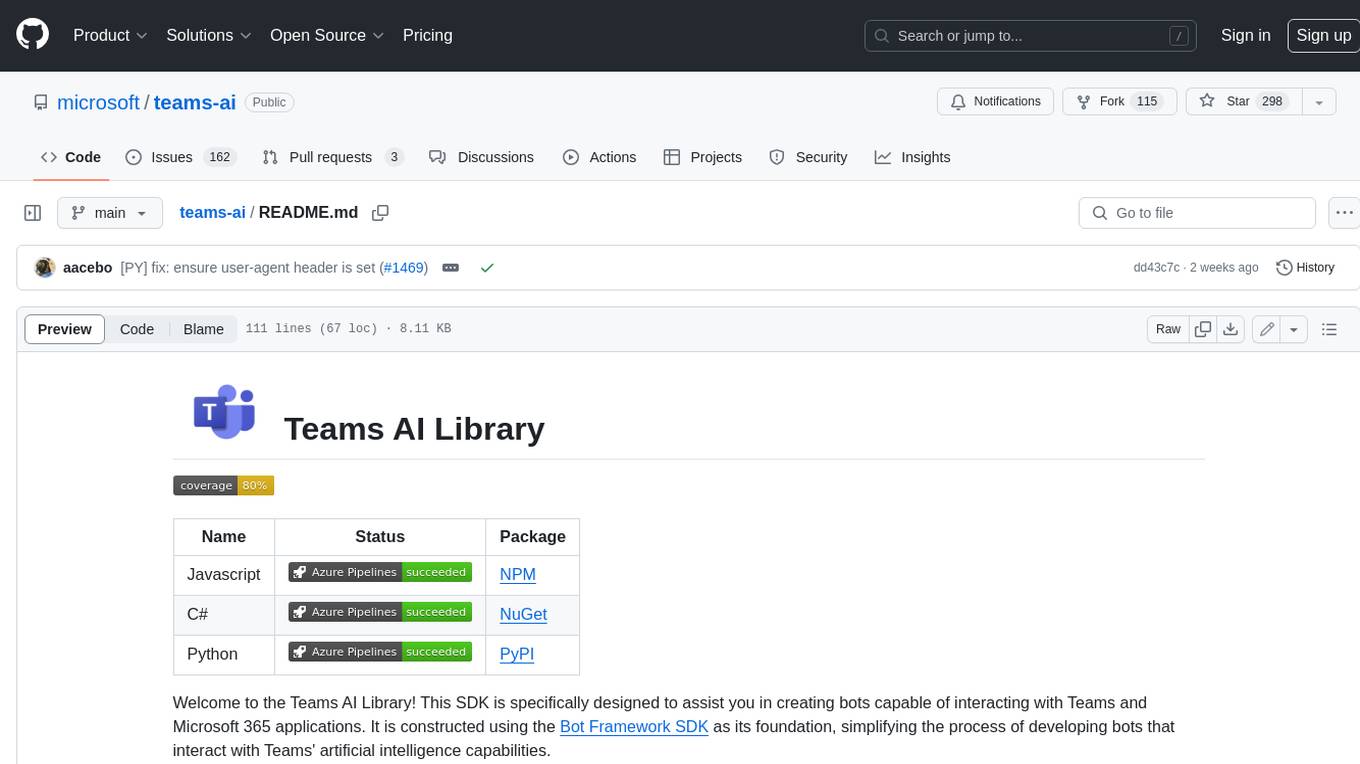
teams-ai
The Teams AI Library is a software development kit (SDK) that helps developers create bots that can interact with Teams and Microsoft 365 applications. It is built on top of the Bot Framework SDK and simplifies the process of developing bots that interact with Teams' artificial intelligence capabilities. The SDK is available for JavaScript/TypeScript, .NET, and Python.
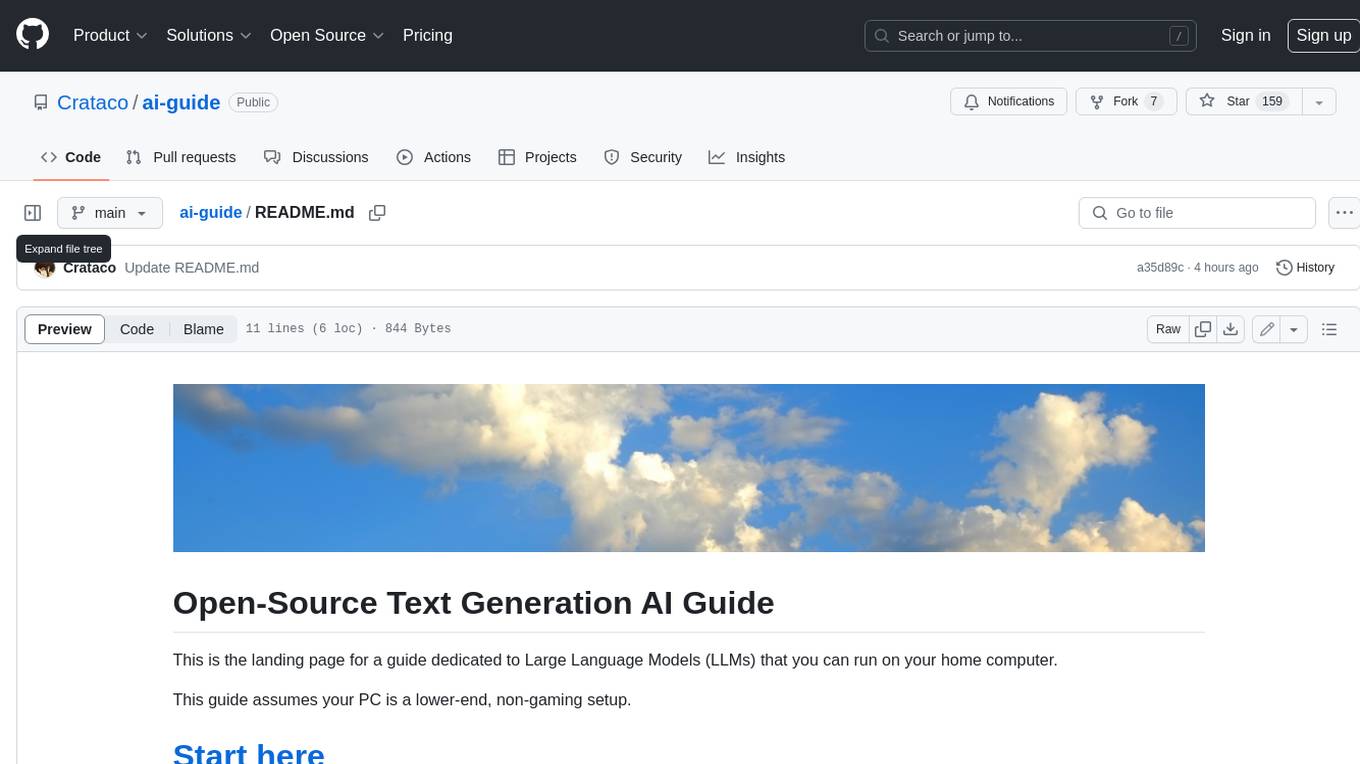
ai-guide
This guide is dedicated to Large Language Models (LLMs) that you can run on your home computer. It assumes your PC is a lower-end, non-gaming setup.
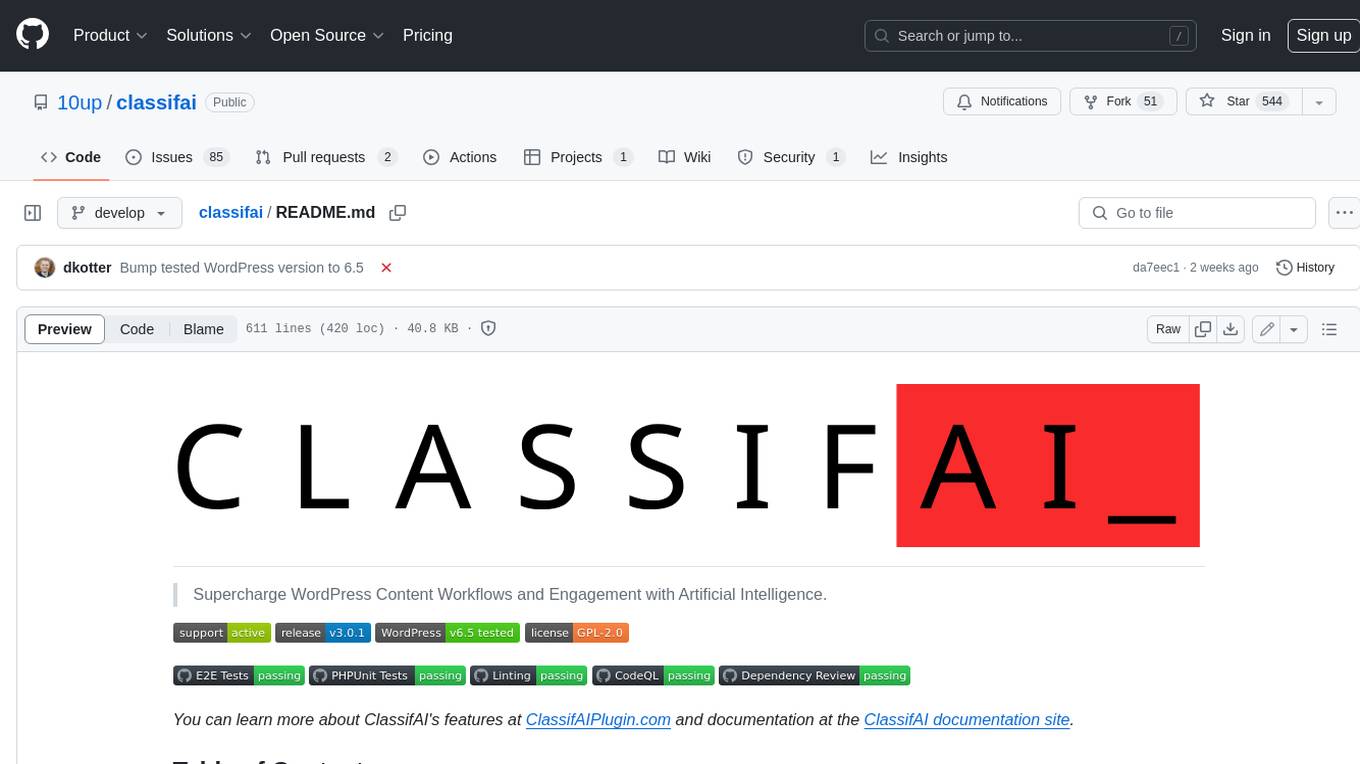
classifai
Supercharge WordPress Content Workflows and Engagement with Artificial Intelligence. Tap into leading cloud-based services like OpenAI, Microsoft Azure AI, Google Gemini and IBM Watson to augment your WordPress-powered websites. Publish content faster while improving SEO performance and increasing audience engagement. ClassifAI integrates Artificial Intelligence and Machine Learning technologies to lighten your workload and eliminate tedious tasks, giving you more time to create original content that matters.
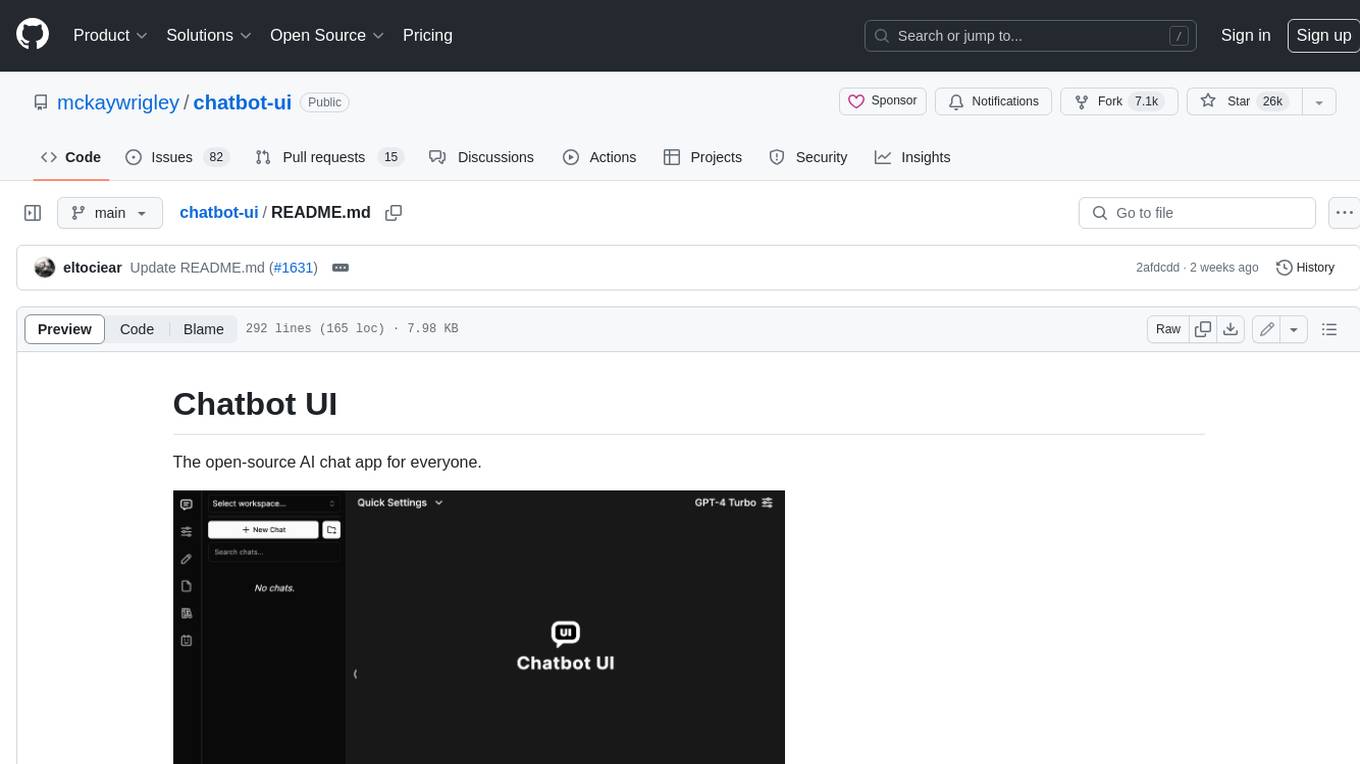
chatbot-ui
Chatbot UI is an open-source AI chat app that allows users to create and deploy their own AI chatbots. It is easy to use and can be customized to fit any need. Chatbot UI is perfect for businesses, developers, and anyone who wants to create a chatbot.
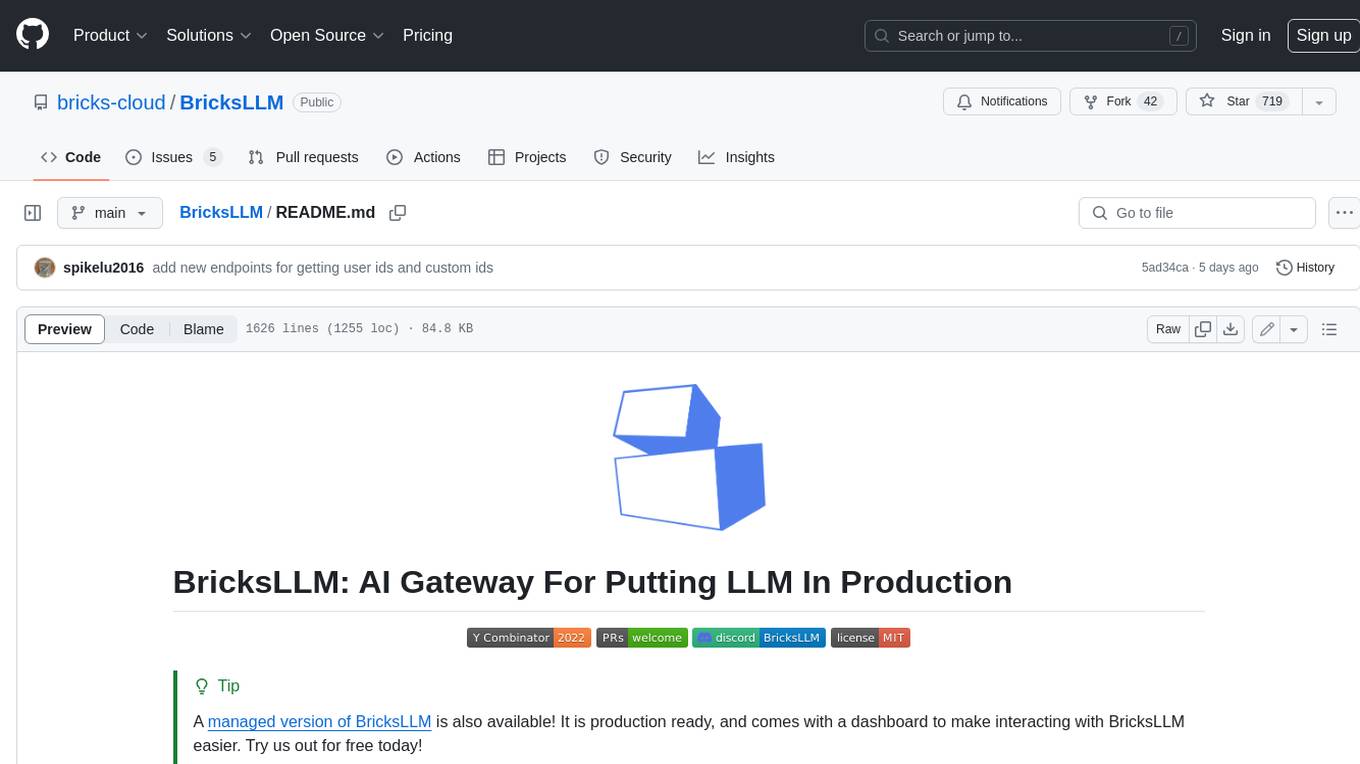
BricksLLM
BricksLLM is a cloud native AI gateway written in Go. Currently, it provides native support for OpenAI, Anthropic, Azure OpenAI and vLLM. BricksLLM aims to provide enterprise level infrastructure that can power any LLM production use cases. Here are some use cases for BricksLLM: * Set LLM usage limits for users on different pricing tiers * Track LLM usage on a per user and per organization basis * Block or redact requests containing PIIs * Improve LLM reliability with failovers, retries and caching * Distribute API keys with rate limits and cost limits for internal development/production use cases * Distribute API keys with rate limits and cost limits for students
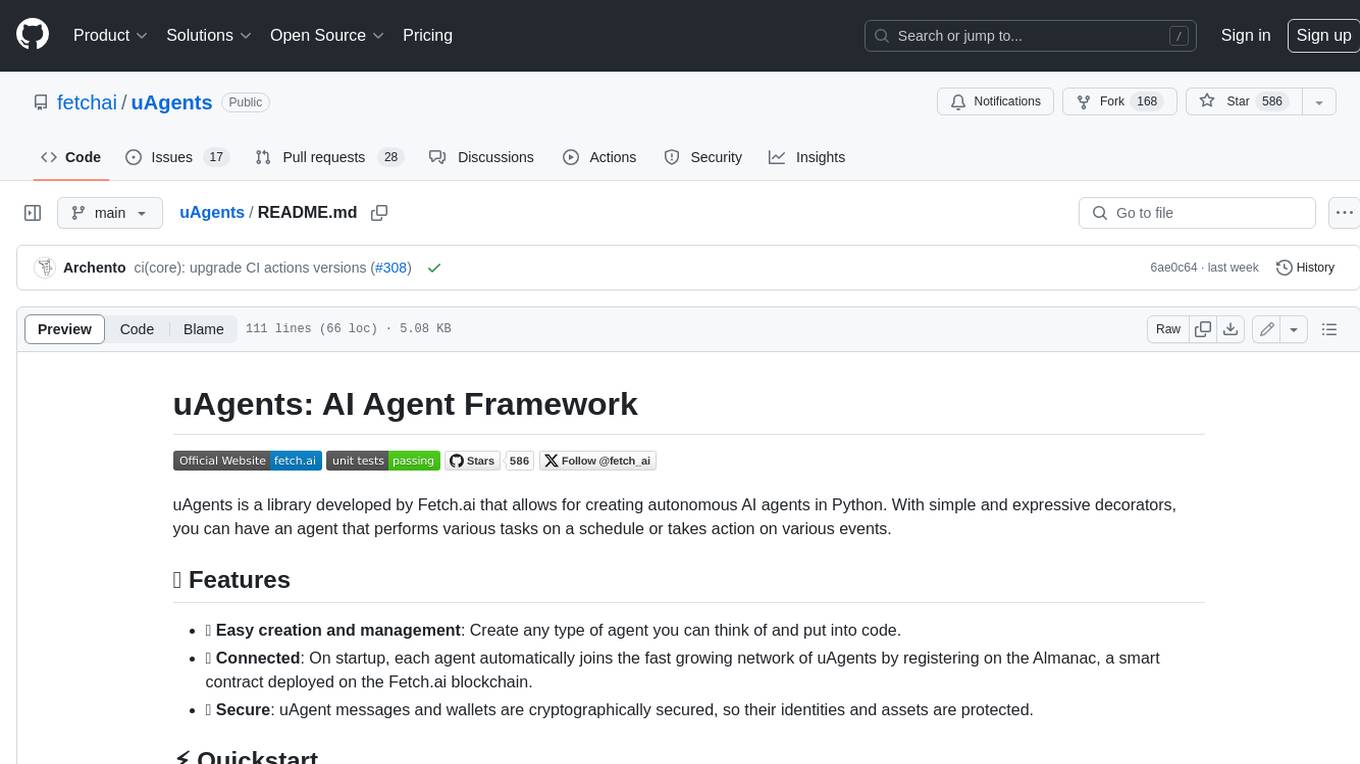
uAgents
uAgents is a Python library developed by Fetch.ai that allows for the creation of autonomous AI agents. These agents can perform various tasks on a schedule or take action on various events. uAgents are easy to create and manage, and they are connected to a fast-growing network of other uAgents. They are also secure, with cryptographically secured messages and wallets.
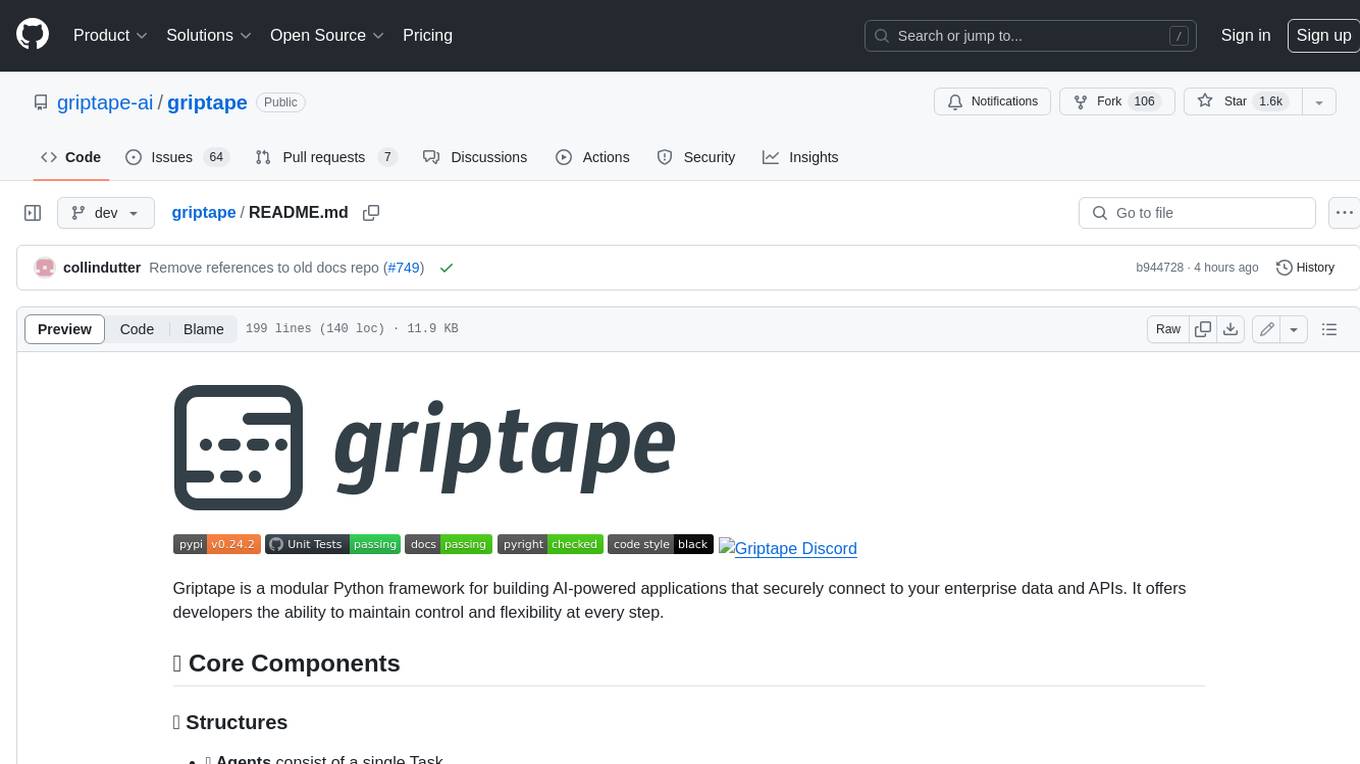
griptape
Griptape is a modular Python framework for building AI-powered applications that securely connect to your enterprise data and APIs. It offers developers the ability to maintain control and flexibility at every step. Griptape's core components include Structures (Agents, Pipelines, and Workflows), Tasks, Tools, Memory (Conversation Memory, Task Memory, and Meta Memory), Drivers (Prompt and Embedding Drivers, Vector Store Drivers, Image Generation Drivers, Image Query Drivers, SQL Drivers, Web Scraper Drivers, and Conversation Memory Drivers), Engines (Query Engines, Extraction Engines, Summary Engines, Image Generation Engines, and Image Query Engines), and additional components (Rulesets, Loaders, Artifacts, Chunkers, and Tokenizers). Griptape enables developers to create AI-powered applications with ease and efficiency.