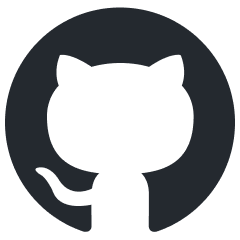
llm-universe
本项目是一个面向小白开发者的大模型应用开发教程,在线阅读地址:https://datawhalechina.github.io/llm-universe/
Stars: 4408
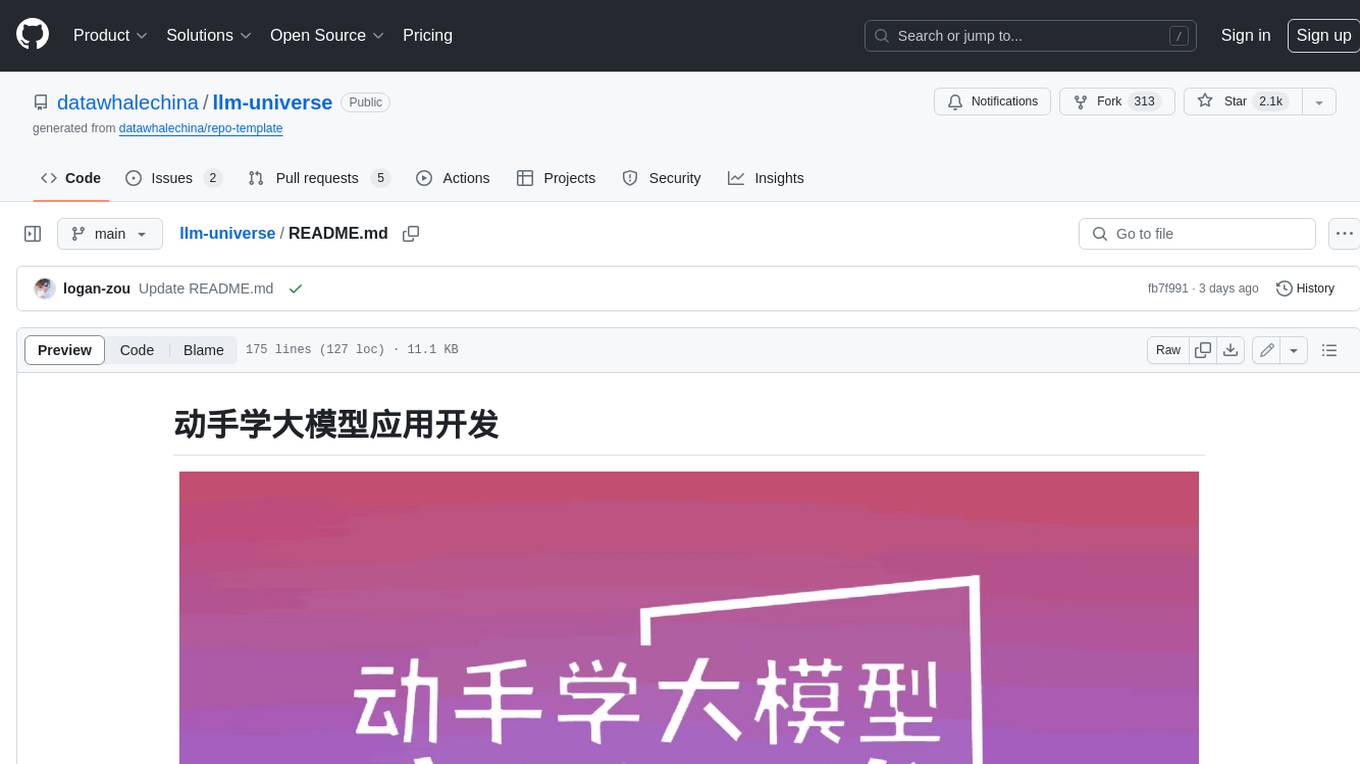
This project is a tutorial on developing large model applications for novice developers. It aims to provide a comprehensive introduction to large model development, focusing on Alibaba Cloud servers and integrating personal knowledge assistant projects. The tutorial covers the following topics: 1. **Introduction to Large Models**: A simplified introduction for novice developers on what large models are, their characteristics, what LangChain is, and how to develop an LLM application. 2. **How to Call Large Model APIs**: This section introduces various methods for calling APIs of well-known domestic and foreign large model products, including calling native APIs, encapsulating them as LangChain LLMs, and encapsulating them as Fastapi calls. It also provides a unified encapsulation for various large model APIs, such as Baidu Wenxin, Xunfei Xinghuo, and Zh譜AI. 3. **Knowledge Base Construction**: Loading, processing, and vector database construction of different types of knowledge base documents. 4. **Building RAG Applications**: Integrating LLM into LangChain to build a retrieval question and answer chain, and deploying applications using Streamlit. 5. **Verification and Iteration**: How to implement verification and iteration in large model development, and common evaluation methods. The project consists of three main parts: 1. **Introduction to LLM Development**: A simplified version of V1 aims to help beginners get started with LLM development quickly and conveniently, understand the general process of LLM development, and build a simple demo. 2. **LLM Development Techniques**: More advanced LLM development techniques, including but not limited to: Prompt Engineering, processing of multiple types of source data, optimizing retrieval, recall ranking, Agent framework, etc. 3. **LLM Application Examples**: Introduce some successful open source cases, analyze the ideas, core concepts, and implementation frameworks of these application examples from the perspective of this course, and help beginners understand what kind of applications they can develop through LLM. Currently, the first part has been completed, and everyone is welcome to read and learn; the second and third parts are under creation. **Directory Structure Description**: requirements.txt: Installation dependencies in the official environment notebook: Notebook source code file docs: Markdown documentation file figures: Pictures data_base: Knowledge base source file used
README:
本项目是一个面向小白开发者的大模型应用开发教程,旨在基于阿里云服务器,结合个人知识库助手项目,通过一个课程完成大模型开发的重点入门,主要内容包括:
- 大模型简介,何为大模型、大模型特点是什么、LangChain 是什么,如何开发一个 LLM 应用,针对小白开发者的简单介绍;
- 如何调用大模型 API,本节介绍了国内外知名大模型产品 API 的多种调用方式,包括调用原生 API、封装为 LangChain LLM、封装为 Fastapi 等调用方式,同时将包括百度文心、讯飞星火、智谱AI等多种大模型 API 进行了统一形式封装;
- 知识库搭建,不同类型知识库文档的加载、处理,向量数据库的搭建;
- 构建 RAG 应用,包括将 LLM 接入到 LangChain 构建检索问答链,使用 Streamlit 进行应用部署
- 验证迭代,大模型开发如何实现验证迭代,一般的评估方法有什么;
本项目主要包括三部分内容:
- LLM 开发入门。V1 版本的简化版,旨在帮助初学者最快、最便捷地入门 LLM 开发,理解 LLM 开发的一般流程,可以搭建出一个简单的 Demo。
- LLM 开发技巧。LLM 开发更进阶的技巧,包括但不限于:Prompt Engineering、多类型源数据的处理、优化检索、召回精排、Agent 框架等
- LLM 应用实例。引入一些成功的开源案例,从本课程的角度出发,解析这些应用范例的 Idea、核心思路、实现框架,帮助初学者明白其可以通过 LLM 开发什么样的应用。
目前,第一部分已经完稿,欢迎大家阅读学习;第二、三部分正在创作中。
目录结构说明:
requirements.txt:官方环境下的安装依赖
notebook:Notebook 源代码文件
docs:Markdown 文档文件
figures:图片
data_base:所使用的知识库源文件
LLM 正逐步成为信息世界的新革命力量,其通过强大的自然语言理解、自然语言生成能力,为开发者提供了新的、更强大的应用开发选择。随着国内外井喷式的 LLM API 服务开放,如何基于 LLM API 快速、便捷地开发具备更强能力、集成 LLM 的应用,开始成为开发者的一项重要技能。
目前,关于 LLM 的介绍以及零散的 LLM 开发技能课程已有不少,但质量参差不齐,且没有很好地整合,开发者需要搜索大量教程并阅读大量相关性不强、必要性较低的内容,才能初步掌握大模型开发的必备技能,学习效率低,学习门槛也较高。
本项目从实践出发,结合最常见、通用的个人知识库助手项目,深入浅出逐步拆解 LLM 开发的一般流程、步骤,旨在帮助没有算法基础的小白通过一个课程完成大模型开发的基础入门。同时,我们也会加入 RAG 开发的进阶技巧以及一些成功的 LLM 应用案例的解读,帮助完成第一部分学习的读者进一步掌握更高阶的 RAG 开发技巧,并能够通过对已有成功项目的借鉴开发自己的、好玩的应用。
所有具备基础 Python 能力,想要掌握 LLM 应用开发技能的开发者。
本项目对学习者的人工智能基础、算法基础没有任何要求,仅需要掌握基本 Python 语法、掌握初级 Python 开发技能即可。
考虑到环境搭建问题,本项目提供了阿里云服务器学生免费领取方式,学生读者可以免费领取阿里云服务器,并通过阿里云服务器完成本课程的学习;本项目同时也提供了个人电脑及非阿里云服务器的环境搭建指南;本项目对本地硬件基本没有要求,不需要 GPU 环境,个人电脑及服务器均可用于学习。
注:本项目主要使用各大模型厂商提供的 API 来进行应用开发,如果你想要学习部署应用本地开源 LLM,欢迎学习同样由 Datawhale 出品的 Self LLM | 开源大模型食用指南,该项目将手把手教你如何速通开源 LLM 部署微调全链路!
注:考虑到学习难度,本项目主要面向初学者,介绍如何使用 LLM 来搭建应用。如果你想要进一步深入学习 LLM 的理论基础,并在理论的基础上进一步认识、应用 LLM,欢迎学习同样由 Datawhale 出品的 So Large LM | 大模型基础,该项目将为你提供全面而深入的 LLM 理论知识及实践方法!
-
充分面向实践,动手学习大模型开发。相较于其他从理论入手、与实践代差较大的类似教程,本教程基于具有通用性的个人知识库助手项目打造,将普适的大模型开发理念融合在项目实践中,帮助学习者通过动手搭建个人项目来掌握大模型开发技能。
-
从零开始,全面又简短的大模型教程。本项目针对个人知识库助手项目,对相关大模型开发理论、概念和基本技能进行了项目主导的重构,删去不需要理解的底层原理和算法细节,涵盖所有大模型开发的核心技能。教程整体时长在数小时之内,但学习完本教程,可以掌握基础大模型开发的所有核心技能。
-
兼具统一性与拓展性。本项目对 GPT、百度文心、讯飞星火、智谱GLM 等国内外主要 LLM API 进行了统一封装,支持一键调用不同的 LLM,帮助开发者将更多的精力放在学习应用与模型本身的优化上,而不需要花时间在繁琐的调用细节上;同时,本教程拟上线 奇想星球 | AIGC共创社区平台,支持学习者自定义项目为本教程增加拓展内容,具备充分的拓展性。
https://datawhalechina.github.io/llm-universe/
https://github.com/datawhalechina/llm-universe/releases/tag/v1
负责人:邹雨衡
- LLM 介绍 @高立业
-
使用 LLM API 开发应用 @毛雨
- 基本概念
-
使用 LLM API
- ChatGPT
- 文心一言
- 讯飞星火
- 智谱 GLM
- Prompt Engineering
- 搭建知识库 @娄天奥
-
构建 RAG 应用 @徐虎
-
将 LLM 接入 LangChain
- ChatGPT
- 文心一言
- 讯飞星火
- 智谱 GLM
- 基于 LangChain 搭建检索问答链
- 基于 Streamlit 部署知识库助手
-
将 LLM 接入 LangChain
- 系统评估与优化 @邹雨衡
负责人:高立业
- 背景
- 架构概览
- 存在的问题
- 解决方法
- 数据处理
- 多类型文档处理
- 分块优化
- 向量模型的选择
- 微调向量模型(进阶)
- 索引层面
- 索引结构
- 混合检索
- 假设性问题
- 检索阶段
- query 过滤
- 对齐 query 和 文档
- 对齐检索和 LLM
- 生成阶段
- 后处理
- 微调 LLM(进阶)
- 参考引用
- 增强阶段
- 上下文增强
- 增强流程
- RAG 工程化评估
负责人:徐虎
- ChatWithDatawhale——个人知识库助手解读
- 天机——人情世故大模型解读
核心贡献者
- 邹雨衡-项目负责人(Datawhale成员-对外经济贸易大学研究生)
- 高立业-第二部分负责人(DataWhale成员-算法工程师)
- 徐虎-第三部分负责人(Datawhale成员-算法工程师)
主要贡献者
- 毛雨-内容创作者(后端开发工程师)
- 娄天奥-内容创作者(Datawhale鲸英助教-中国科学院大学研究生)
- 崔腾松-项目支持者(Datawhale成员-奇想星球联合发起人)
- June-项目支持者(Datawhale成员-奇想星球联合发起人)
其他
- 特别感谢 @Sm1les、@LSGOMYP 对本项目的帮助与支持;
- 特别感谢奇想星球 | AIGC共创社区平台提供的支持,欢迎大家关注;
- 如果有任何想法可以联系我们 DataWhale 也欢迎大家多多提出 issue;
- 特别感谢以下为教程做出贡献的同学!
Made with contrib.rocks.
For Tasks:
Click tags to check more tools for each tasksFor Jobs:
Alternative AI tools for llm-universe
Similar Open Source Tools
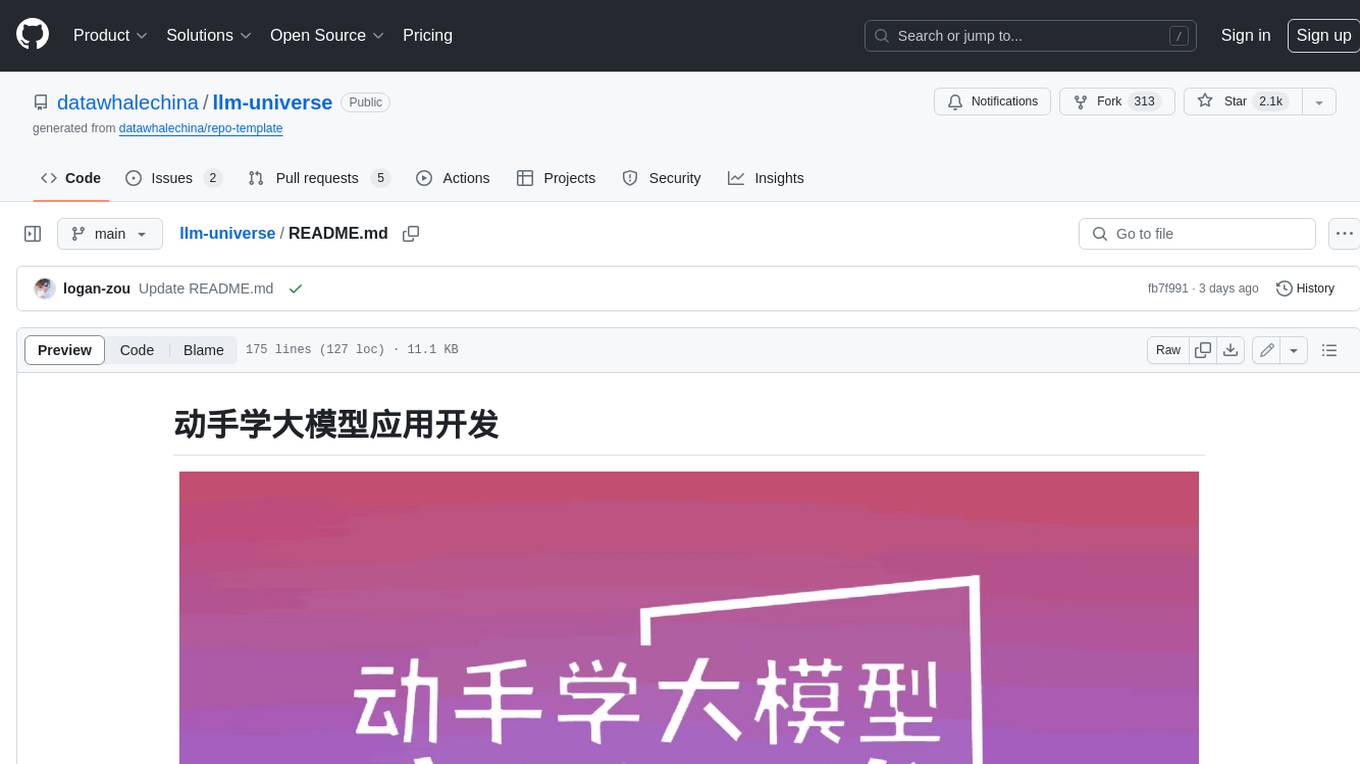
llm-universe
This project is a tutorial on developing large model applications for novice developers. It aims to provide a comprehensive introduction to large model development, focusing on Alibaba Cloud servers and integrating personal knowledge assistant projects. The tutorial covers the following topics: 1. **Introduction to Large Models**: A simplified introduction for novice developers on what large models are, their characteristics, what LangChain is, and how to develop an LLM application. 2. **How to Call Large Model APIs**: This section introduces various methods for calling APIs of well-known domestic and foreign large model products, including calling native APIs, encapsulating them as LangChain LLMs, and encapsulating them as Fastapi calls. It also provides a unified encapsulation for various large model APIs, such as Baidu Wenxin, Xunfei Xinghuo, and Zh譜AI. 3. **Knowledge Base Construction**: Loading, processing, and vector database construction of different types of knowledge base documents. 4. **Building RAG Applications**: Integrating LLM into LangChain to build a retrieval question and answer chain, and deploying applications using Streamlit. 5. **Verification and Iteration**: How to implement verification and iteration in large model development, and common evaluation methods. The project consists of three main parts: 1. **Introduction to LLM Development**: A simplified version of V1 aims to help beginners get started with LLM development quickly and conveniently, understand the general process of LLM development, and build a simple demo. 2. **LLM Development Techniques**: More advanced LLM development techniques, including but not limited to: Prompt Engineering, processing of multiple types of source data, optimizing retrieval, recall ranking, Agent framework, etc. 3. **LLM Application Examples**: Introduce some successful open source cases, analyze the ideas, core concepts, and implementation frameworks of these application examples from the perspective of this course, and help beginners understand what kind of applications they can develop through LLM. Currently, the first part has been completed, and everyone is welcome to read and learn; the second and third parts are under creation. **Directory Structure Description**: requirements.txt: Installation dependencies in the official environment notebook: Notebook source code file docs: Markdown documentation file figures: Pictures data_base: Knowledge base source file used
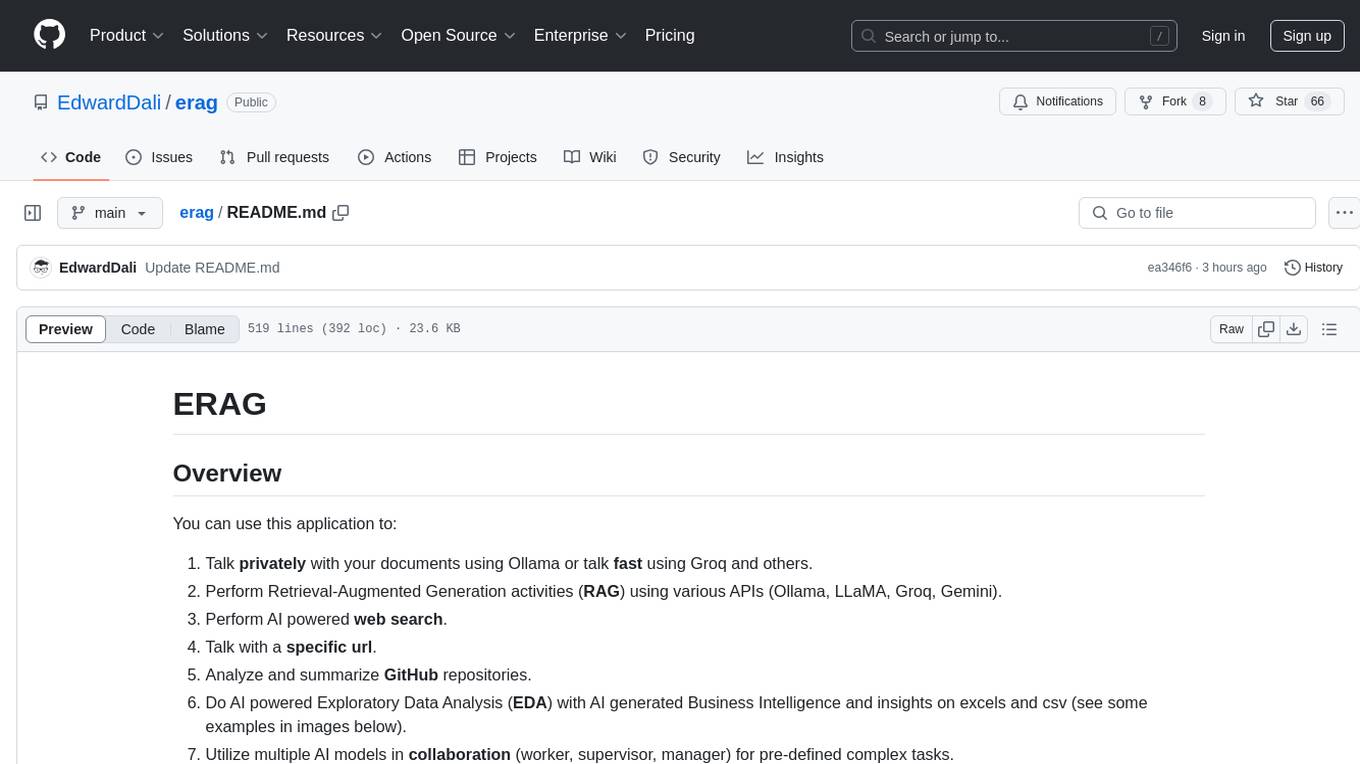
erag
ERAG is an advanced system that combines lexical, semantic, text, and knowledge graph searches with conversation context to provide accurate and contextually relevant responses. This tool processes various document types, creates embeddings, builds knowledge graphs, and uses this information to answer user queries intelligently. It includes modules for interacting with web content, GitHub repositories, and performing exploratory data analysis using various language models.
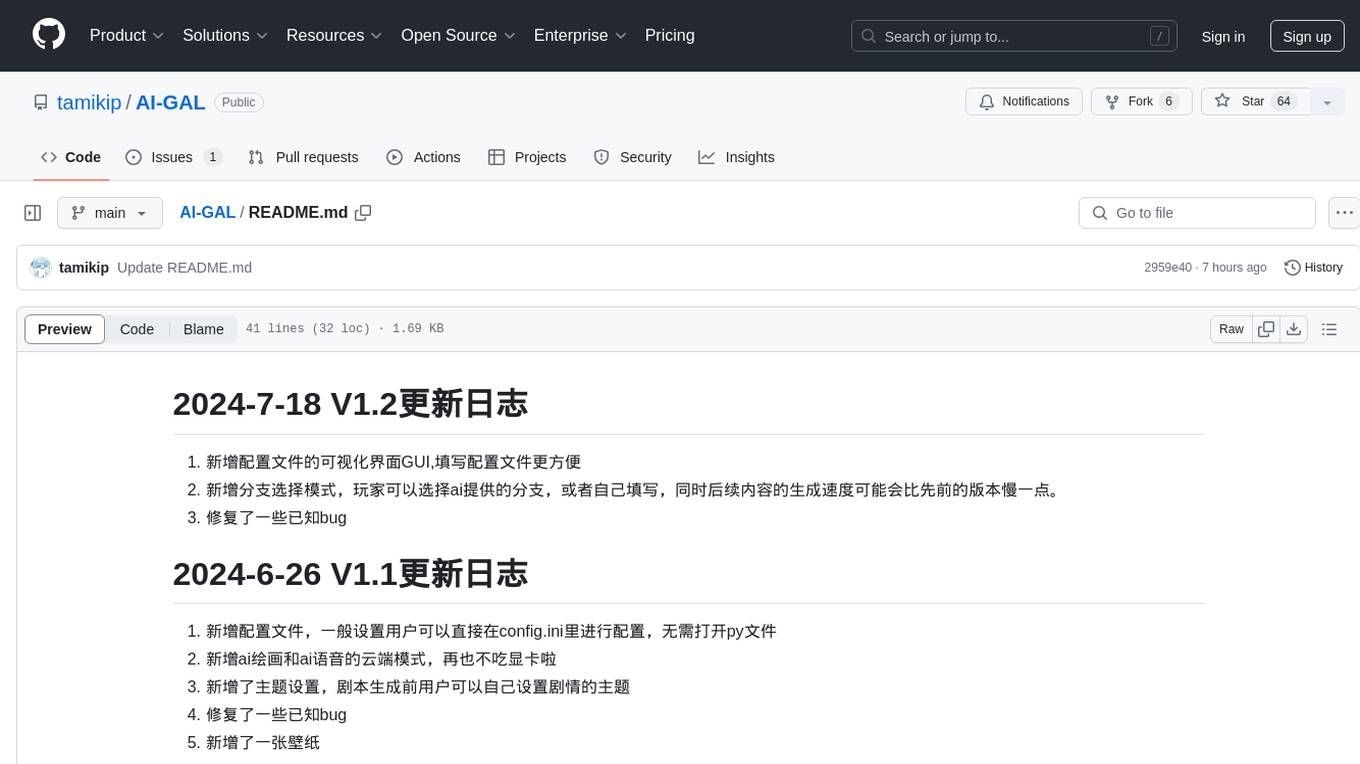
AI-GAL
AI-GAL is a tool that offers a visual GUI for easier configuration file editing, branch selection mode for content generation, and bug fixes. Users can configure settings in config.ini, utilize cloud-based AI drawing and voice modes, set themes for script generation, and enjoy a wallpaper. Prior to usage, ensure a 4GB+ GPU, chatgpt key or local LLM deployment, and installation of stable diffusion, gpt-sovits, and rembg. To start, fill out the config.ini file and run necessary APIs. Restart a storyline by clearing story.txt in the game directory. Encounter errors? Copy the log.txt details and send them for assistance.
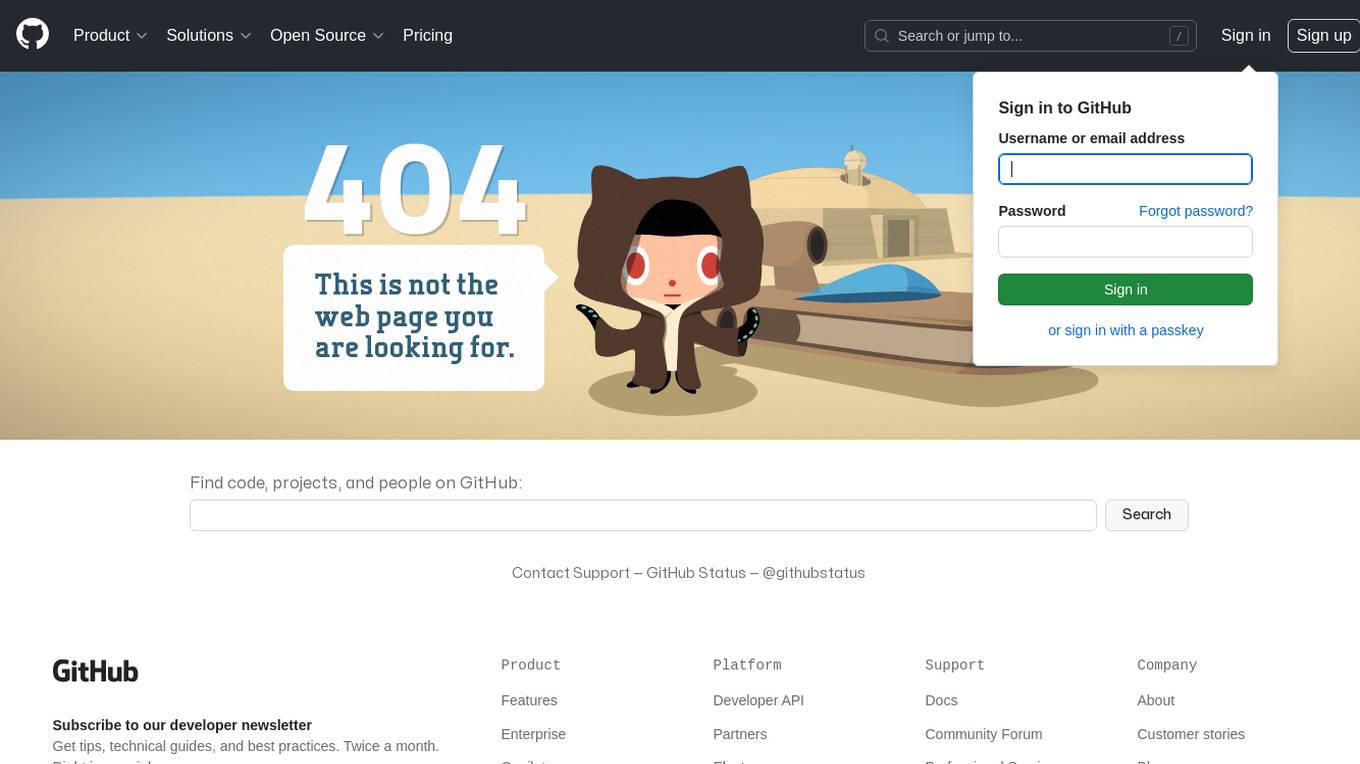
CHATPGT-MEV-BOT
The 𝓜𝓔𝓥-𝓑𝓞𝓣 is a revolutionary tool that empowers users to maximize their ETH earnings through advanced slippage techniques within the Ethereum ecosystem. Its user-centric design, optimized earning mechanism, and comprehensive security measures make it an indispensable tool for traders seeking to enhance their crypto trading strategies. With its current free access, there's no better time to explore the 𝓜𝓔𝓥-𝓑𝓞𝓣's capabilities and witness the transformative impact it can have on your crypto trading journey.
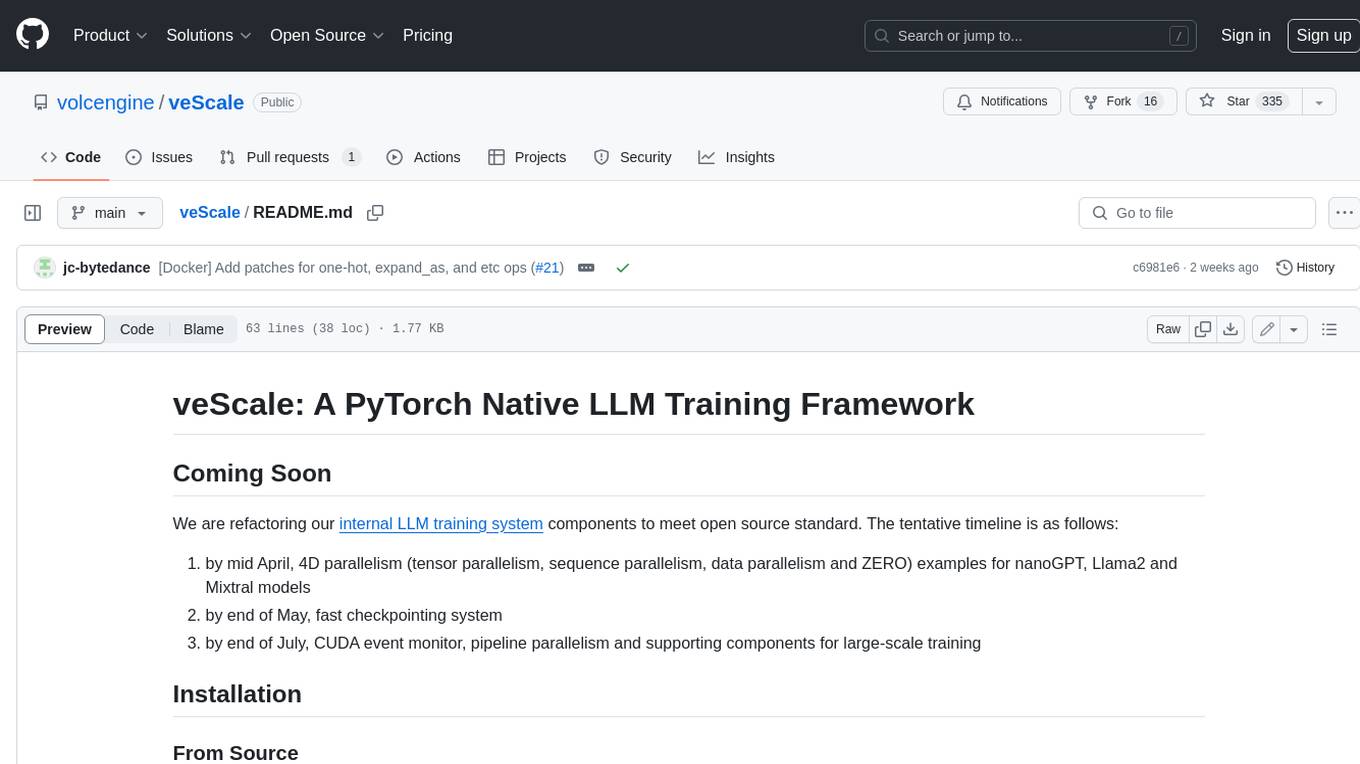
veScale
veScale is a PyTorch Native LLM Training Framework. It provides a set of tools and components to facilitate the training of large language models (LLMs) using PyTorch. veScale includes features such as 4D parallelism, fast checkpointing, and a CUDA event monitor. It is designed to be scalable and efficient, and it can be used to train LLMs on a variety of hardware platforms.
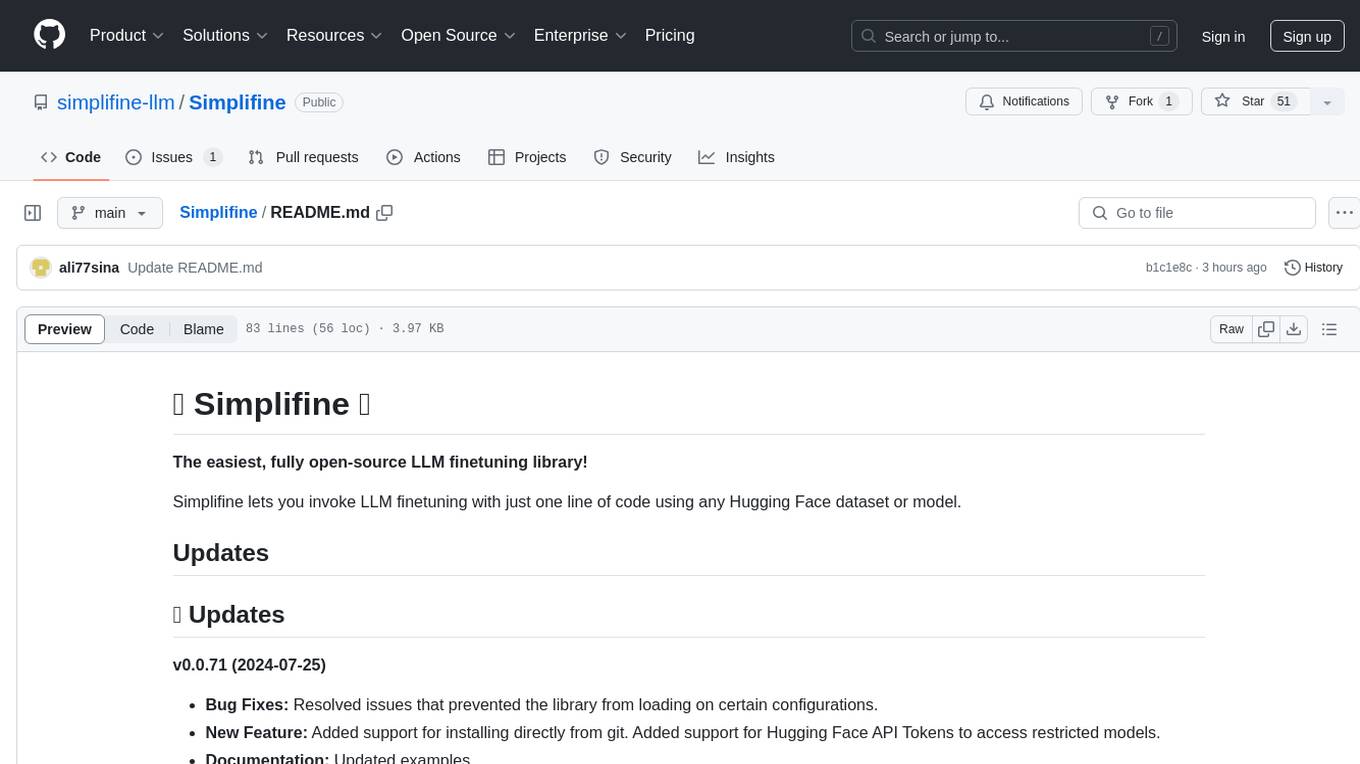
Simplifine
Simplifine is an open-source library designed for easy LLM finetuning, enabling users to perform tasks such as supervised fine tuning, question-answer finetuning, contrastive loss for embedding tasks, multi-label classification finetuning, and more. It provides features like WandB logging, in-built evaluation tools, automated finetuning parameters, and state-of-the-art optimization techniques. The library offers bug fixes, new features, and documentation updates in its latest version. Users can install Simplifine via pip or directly from GitHub. The project welcomes contributors and provides comprehensive documentation and support for users.
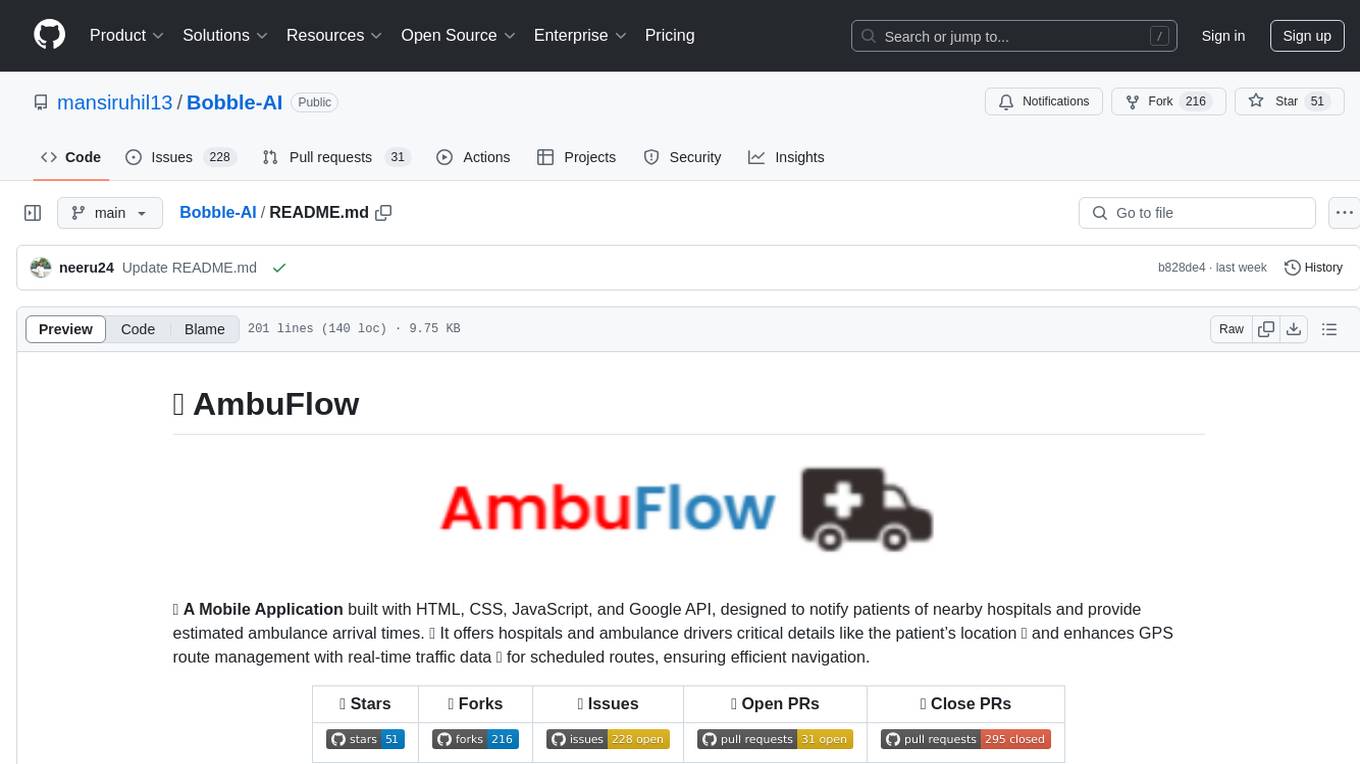
Bobble-AI
AmbuFlow is a mobile application developed using HTML, CSS, JavaScript, and Google API to notify patients of nearby hospitals and provide estimated ambulance arrival times. It offers critical details like patient's location and enhances GPS route management with real-time traffic data for efficient navigation. The app helps users find nearby hospitals, track ambulances in real-time, and manage ambulance routes based on traffic and distance. It ensures quick emergency response, real-time tracking, enhanced communication, resource management, and a user-friendly interface for seamless navigation in high-stress situations.
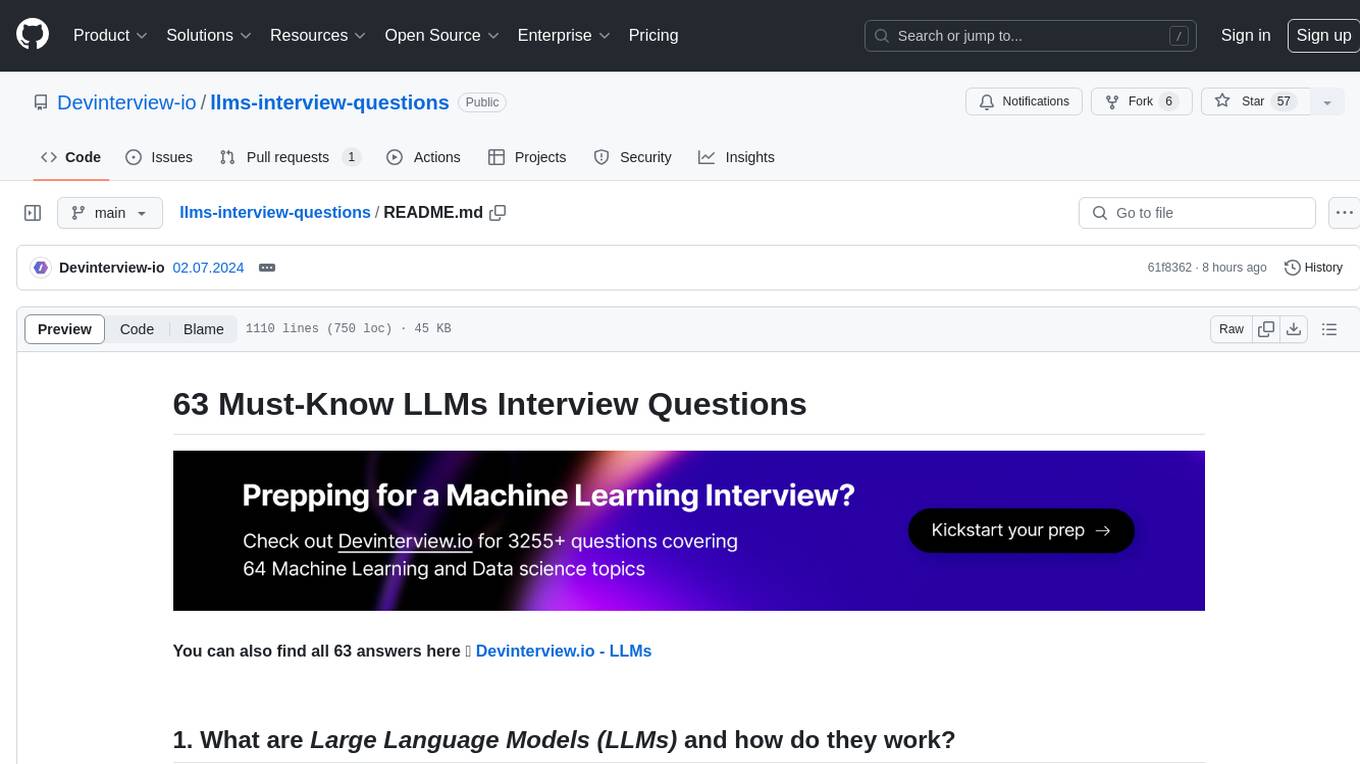
llms-interview-questions
This repository contains a comprehensive collection of 63 must-know Large Language Models (LLMs) interview questions. It covers topics such as the architecture of LLMs, transformer models, attention mechanisms, training processes, encoder-decoder frameworks, differences between LLMs and traditional statistical language models, handling context and long-term dependencies, transformers for parallelization, applications of LLMs, sentiment analysis, language translation, conversation AI, chatbots, and more. The readme provides detailed explanations, code examples, and insights into utilizing LLMs for various tasks.
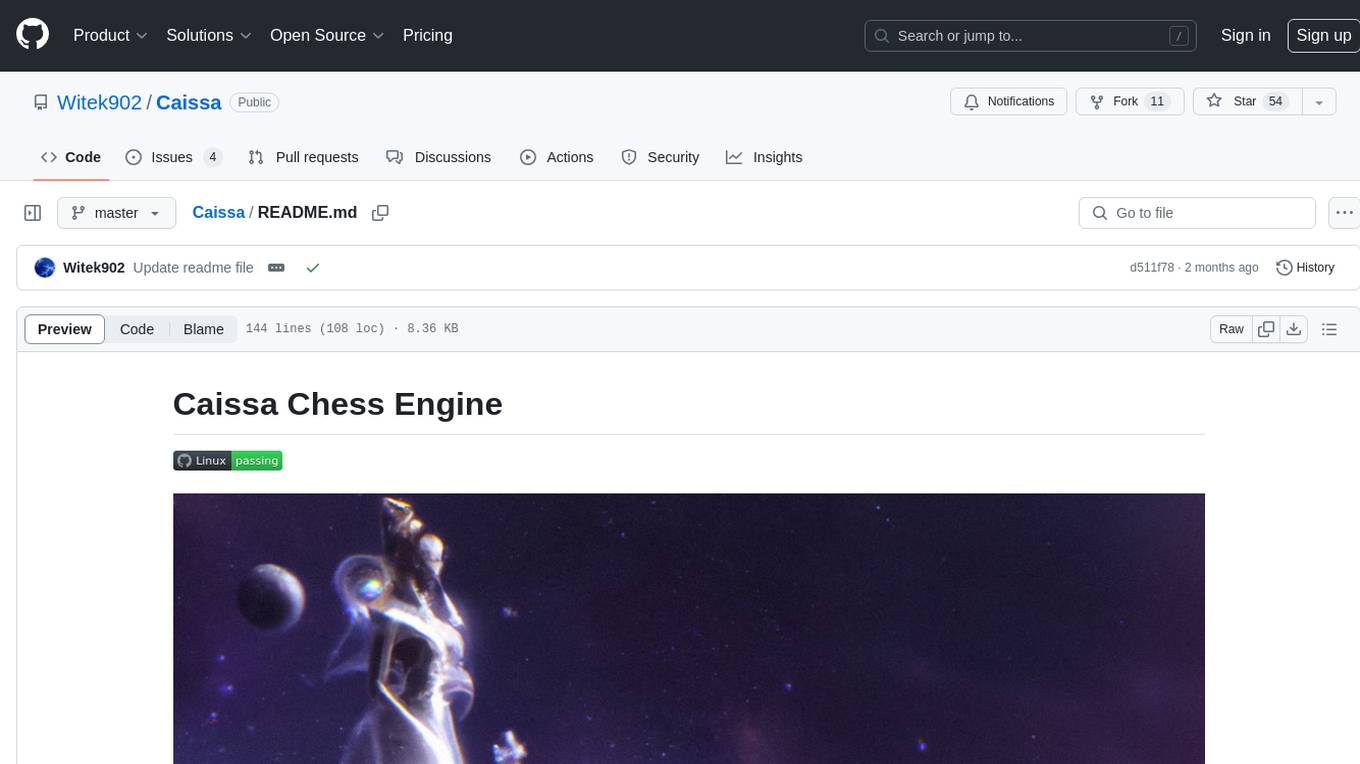
Caissa
Caissa is a strong, UCI command-line chess engine optimized for regular chess, FRC, and DFRC. It features its own neural network trained with self-play games, supports various UCI options, and provides different EXE versions for different CPU architectures. The engine uses advanced search algorithms, neural network evaluation, and endgame tablebases. It offers outstanding performance in ultra-short games and is written in C++ with modules for backend, frontend, and utilities like neural network trainer and self-play data generator.
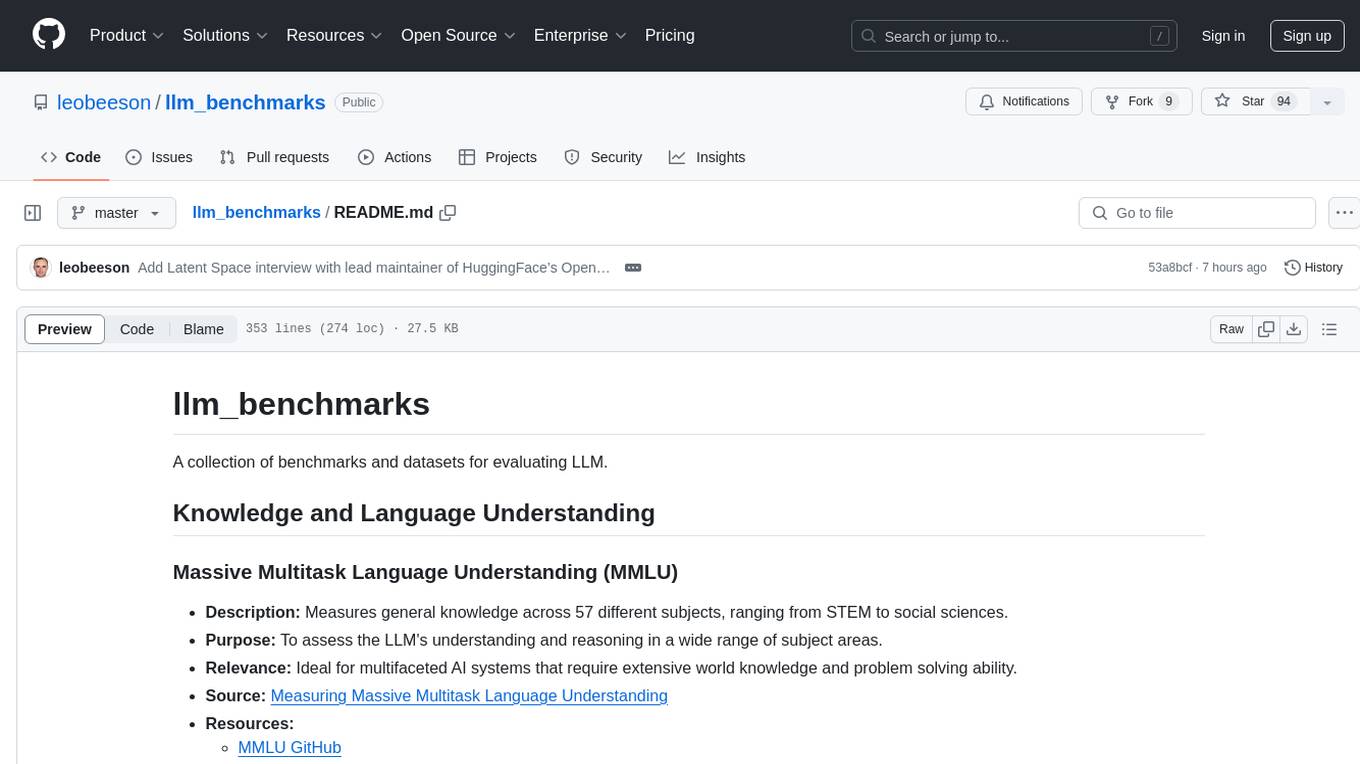
llm_benchmarks
llm_benchmarks is a collection of benchmarks and datasets for evaluating Large Language Models (LLMs). It includes various tasks and datasets to assess LLMs' knowledge, reasoning, language understanding, and conversational abilities. The repository aims to provide comprehensive evaluation resources for LLMs across different domains and applications, such as education, healthcare, content moderation, coding, and conversational AI. Researchers and developers can leverage these benchmarks to test and improve the performance of LLMs in various real-world scenarios.
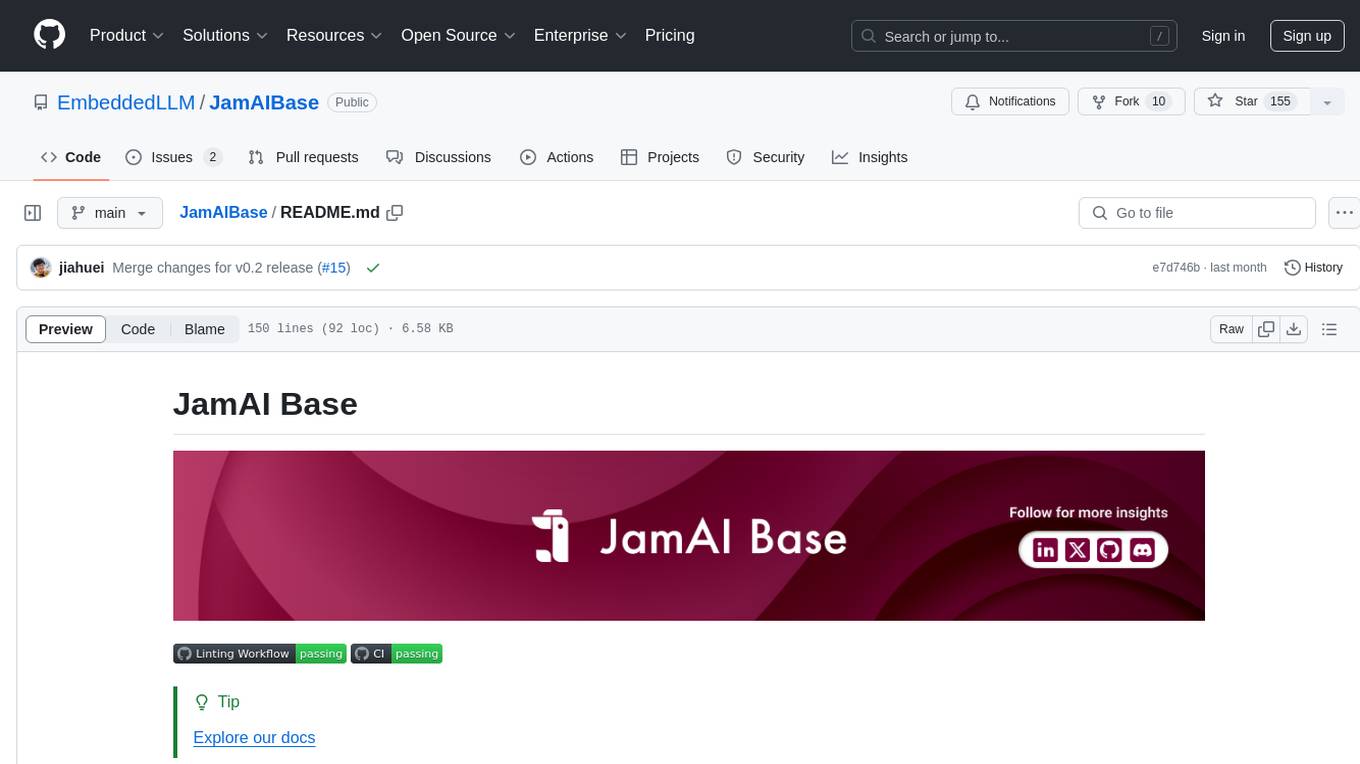
JamAIBase
JamAI Base is an open-source platform integrating SQLite and LanceDB databases with managed memory and RAG capabilities. It offers built-in LLM, vector embeddings, and reranker orchestration accessible through a spreadsheet-like UI and REST API. Users can transform static tables into dynamic entities, facilitate real-time interactions, manage structured data, and simplify chatbot development. The tool focuses on ease of use, scalability, flexibility, declarative paradigm, and innovative RAG techniques, making complex data operations accessible to users with varying technical expertise.
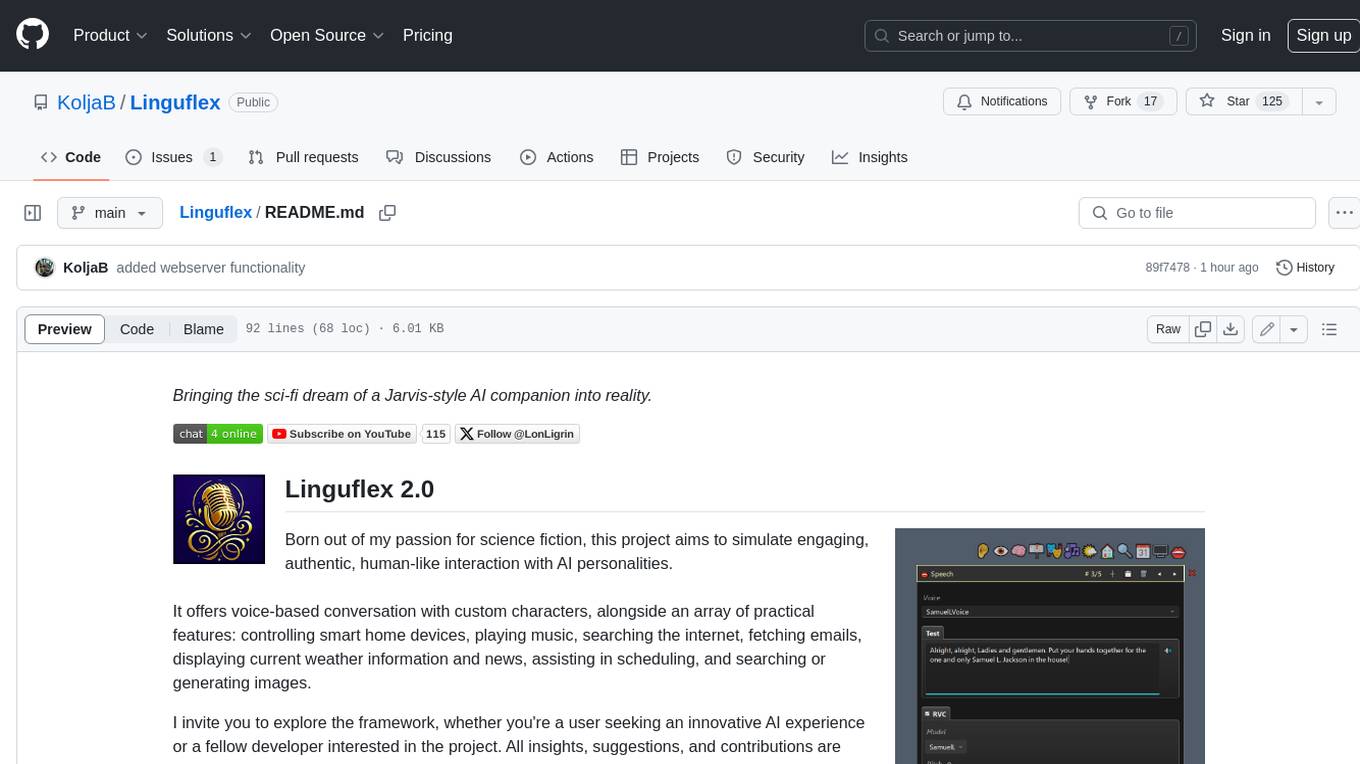
Linguflex
Linguflex is a project that aims to simulate engaging, authentic, human-like interaction with AI personalities. It offers voice-based conversation with custom characters, alongside an array of practical features such as controlling smart home devices, playing music, searching the internet, fetching emails, displaying current weather information and news, assisting in scheduling, and searching or generating images.
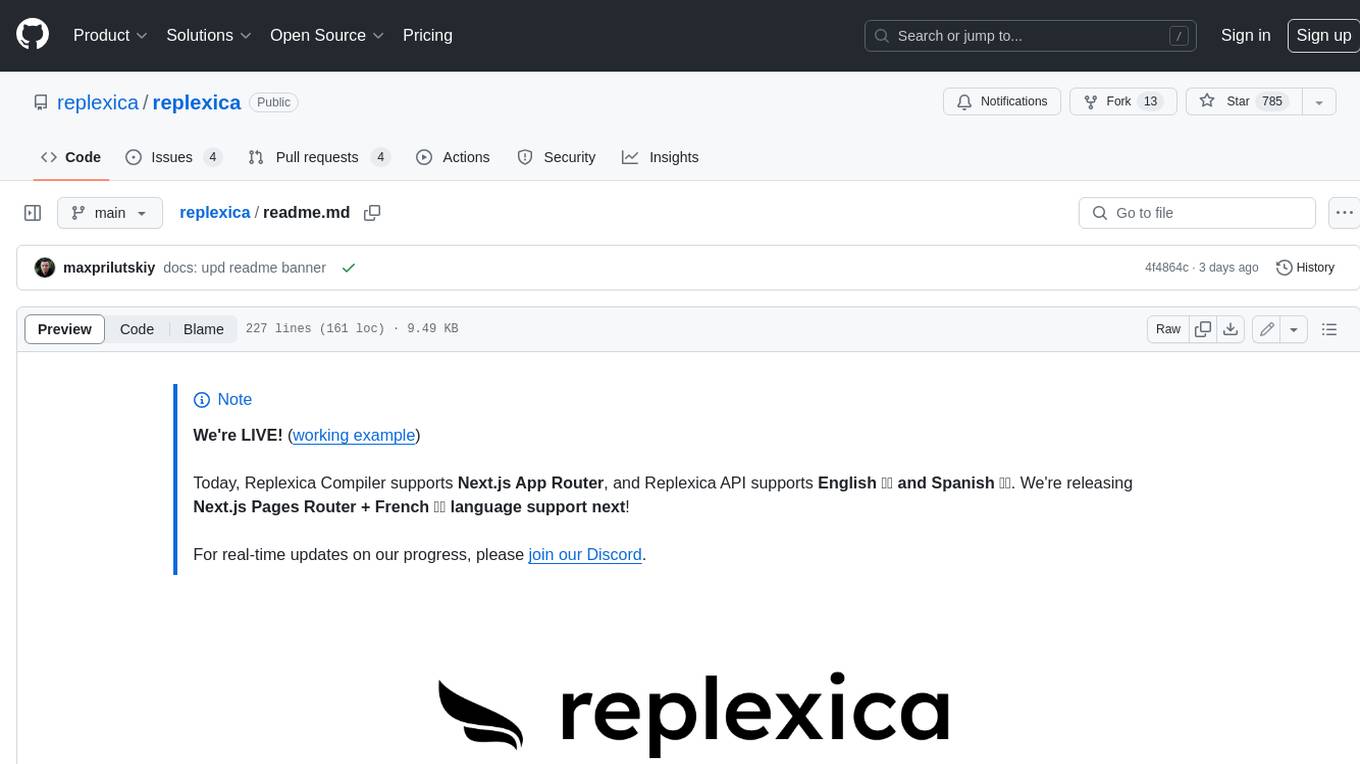
replexica
Replexica is an i18n toolkit for React, to ship multi-language apps fast. It doesn't require extracting text into JSON files, and uses AI-powered API for content processing. It comes in two parts: 1. Replexica Compiler - an open-source compiler plugin for React; 2. Replexica API - an i18n API in the cloud that performs translations using LLMs. (Usage based, has a free tier.) Replexica supports several i18n formats: 1. JSON-free Replexica compiler format; 2. .md files for Markdown content; 3. Legacy JSON and YAML-based formats.
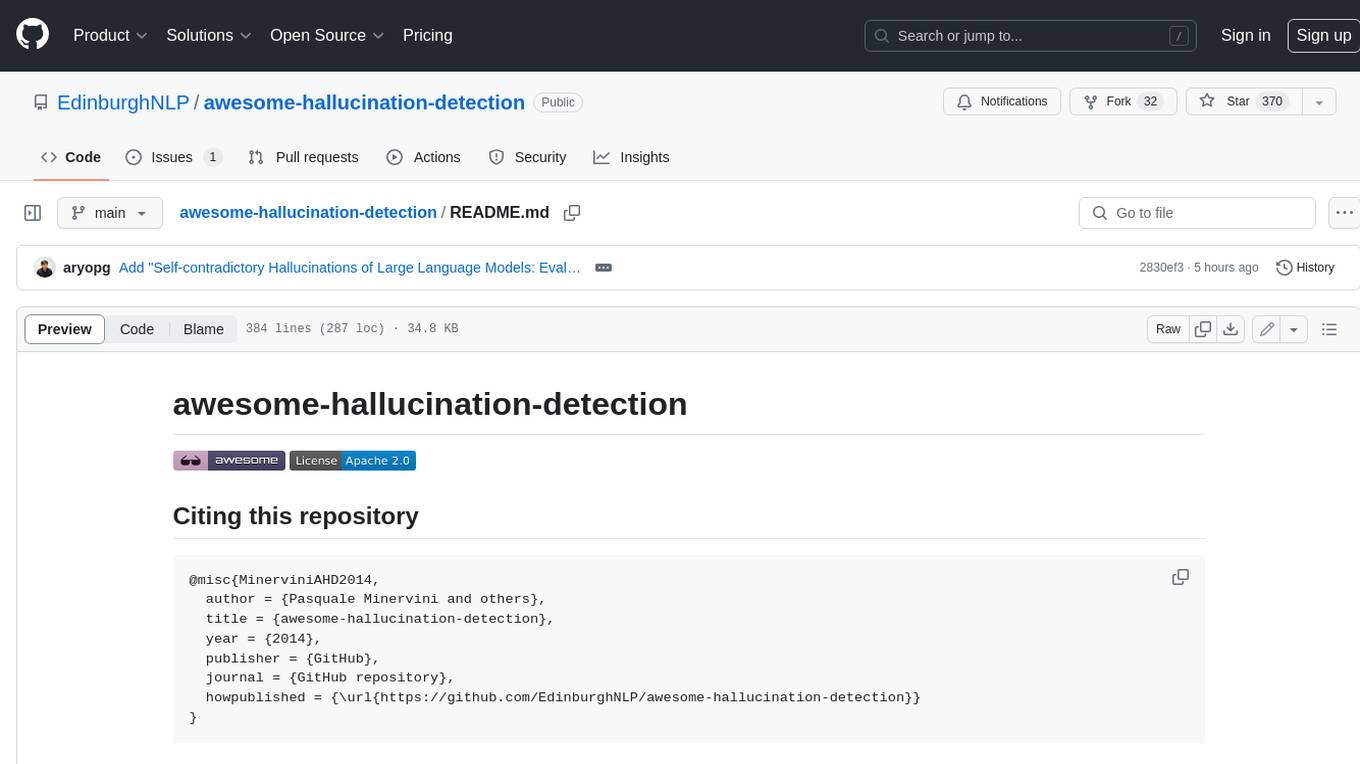
awesome-hallucination-detection
This repository provides a curated list of papers, datasets, and resources related to the detection and mitigation of hallucinations in large language models (LLMs). Hallucinations refer to the generation of factually incorrect or nonsensical text by LLMs, which can be a significant challenge for their use in real-world applications. The resources in this repository aim to help researchers and practitioners better understand and address this issue.
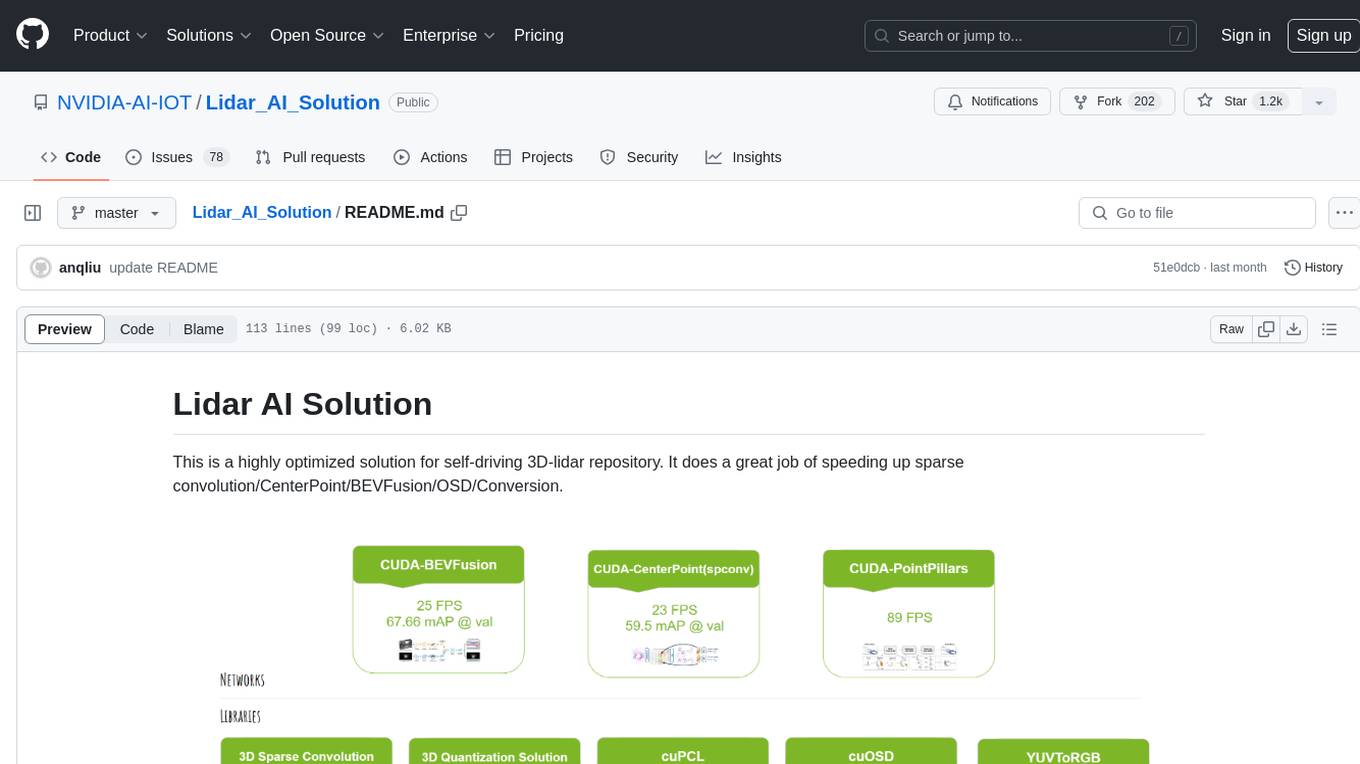
Lidar_AI_Solution
Lidar AI Solution is a highly optimized repository for self-driving 3D lidar, providing solutions for sparse convolution, BEVFusion, CenterPoint, OSD, and Conversion. It includes CUDA and TensorRT implementations for various tasks such as 3D sparse convolution, BEVFusion, CenterPoint, PointPillars, V2XFusion, cuOSD, cuPCL, and YUV to RGB conversion. The repository offers easy-to-use solutions, high accuracy, low memory usage, and quantization options for different tasks related to self-driving technology.
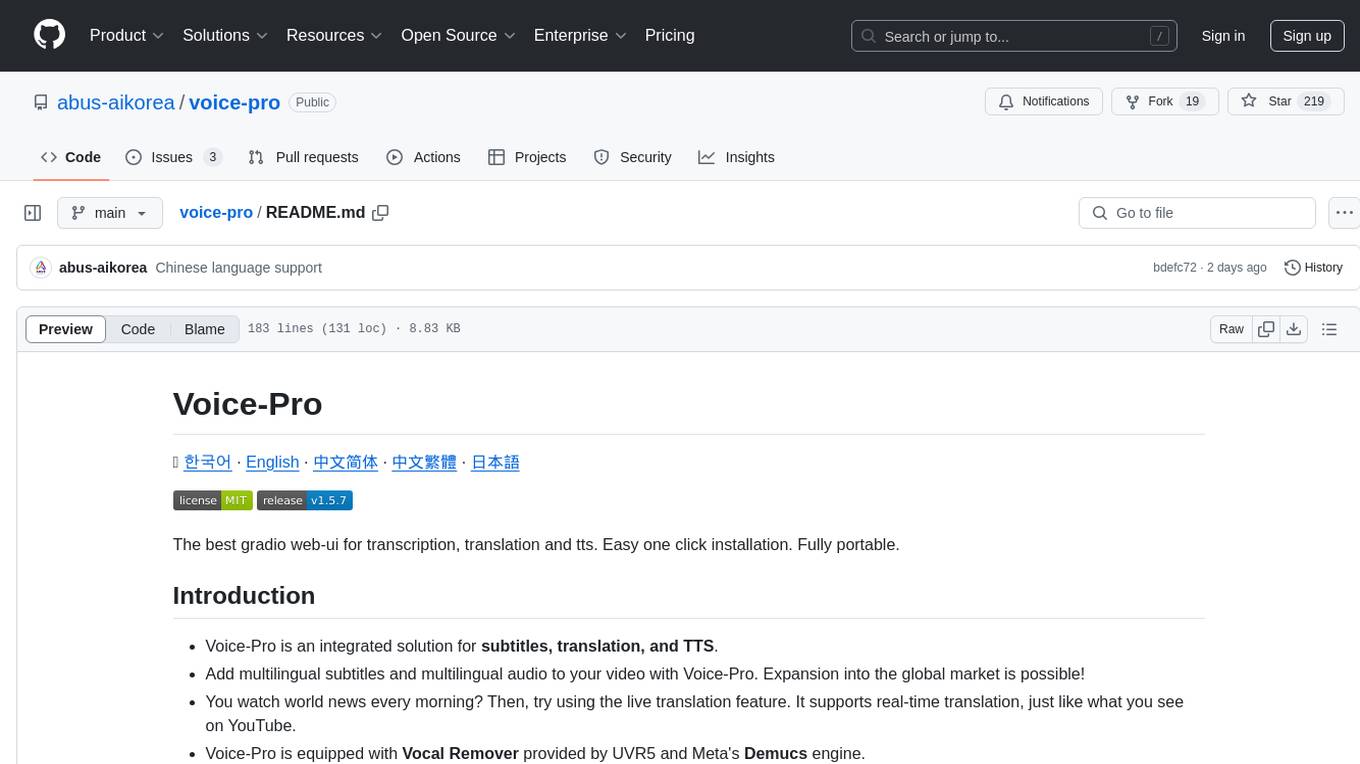
voice-pro
Voice-Pro is an integrated solution for subtitles, translation, and TTS. It offers features like multilingual subtitles, live translation, vocal remover, and supports OpenAI Whisper and Open-Source Translator. The tool provides a Studio tab for various functions, Whisper Caption tab for subtitle creation, Translate tab for translation, TTS tab for text-to-speech, Live Translation tab for real-time voice recognition, and Batch tab for processing multiple files. Users can download YouTube videos, improve voice recognition accuracy, create automatic subtitles, and produce multilingual videos with ease. The tool is easy to install with one-click and offers a Web-UI for user convenience.
For similar tasks
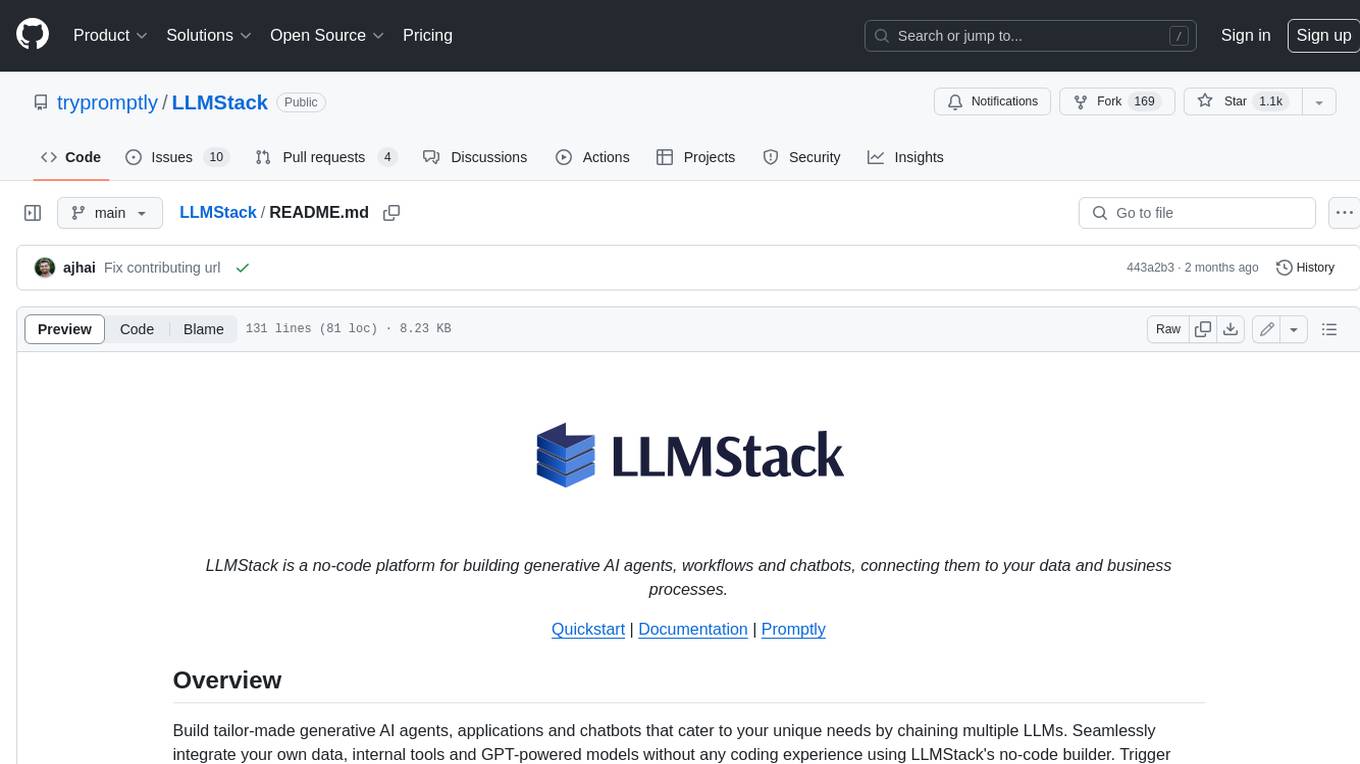
LLMStack
LLMStack is a no-code platform for building generative AI agents, workflows, and chatbots. It allows users to connect their own data, internal tools, and GPT-powered models without any coding experience. LLMStack can be deployed to the cloud or on-premise and can be accessed via HTTP API or triggered from Slack or Discord.
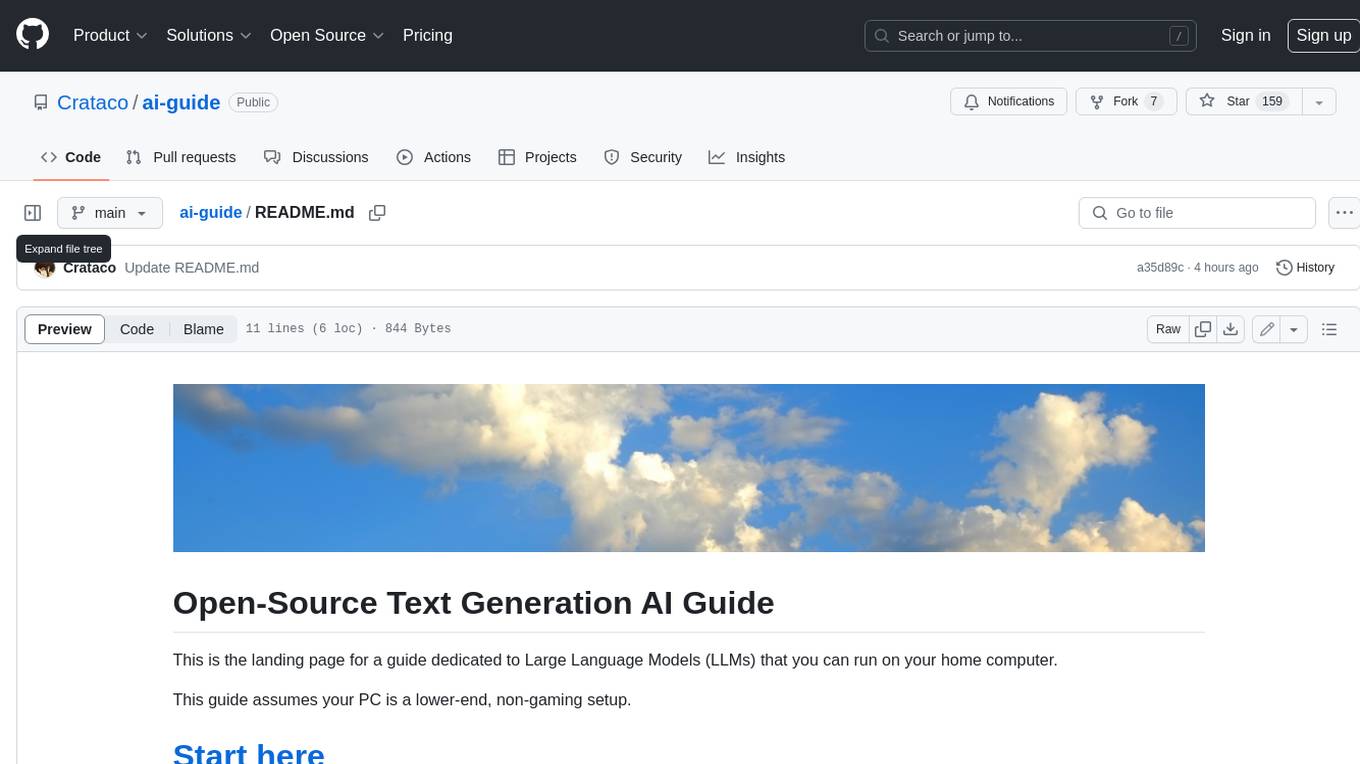
ai-guide
This guide is dedicated to Large Language Models (LLMs) that you can run on your home computer. It assumes your PC is a lower-end, non-gaming setup.
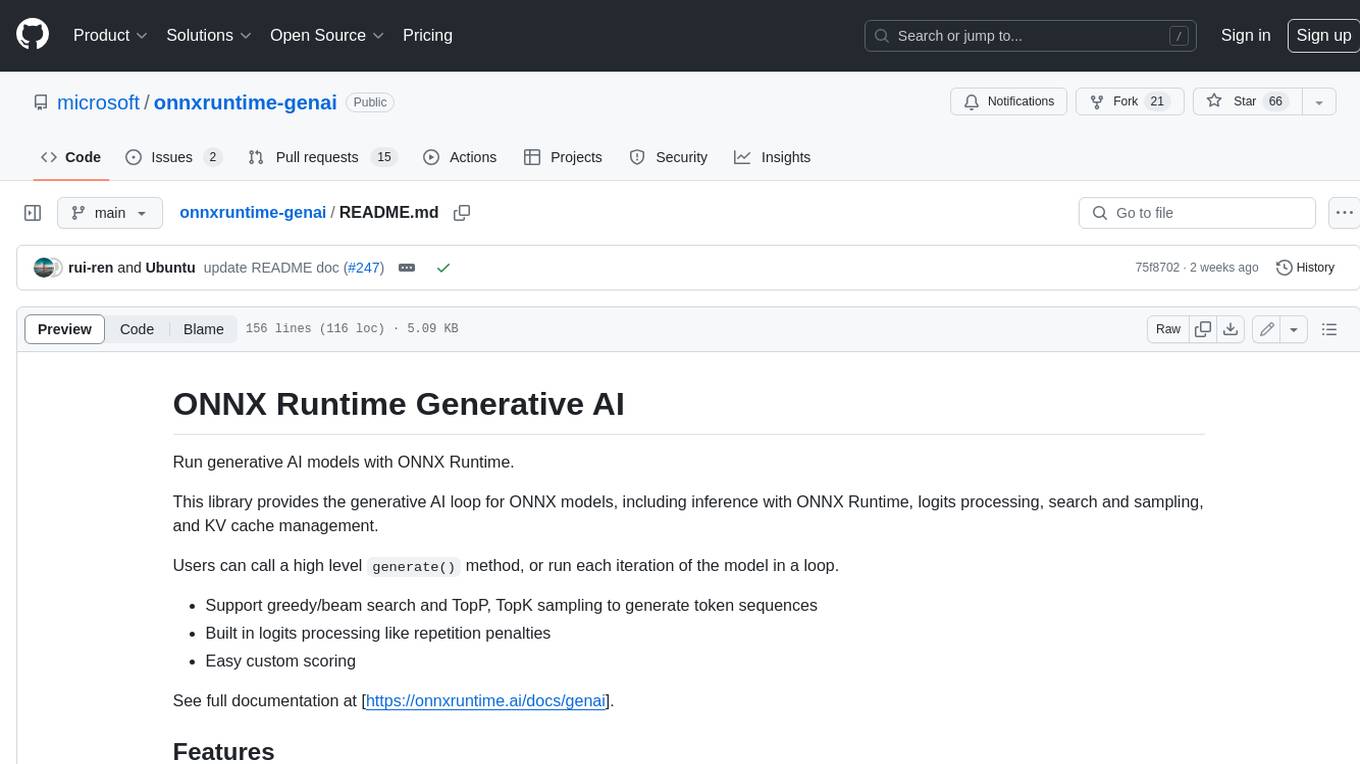
onnxruntime-genai
ONNX Runtime Generative AI is a library that provides the generative AI loop for ONNX models, including inference with ONNX Runtime, logits processing, search and sampling, and KV cache management. Users can call a high level `generate()` method, or run each iteration of the model in a loop. It supports greedy/beam search and TopP, TopK sampling to generate token sequences, has built in logits processing like repetition penalties, and allows for easy custom scoring.
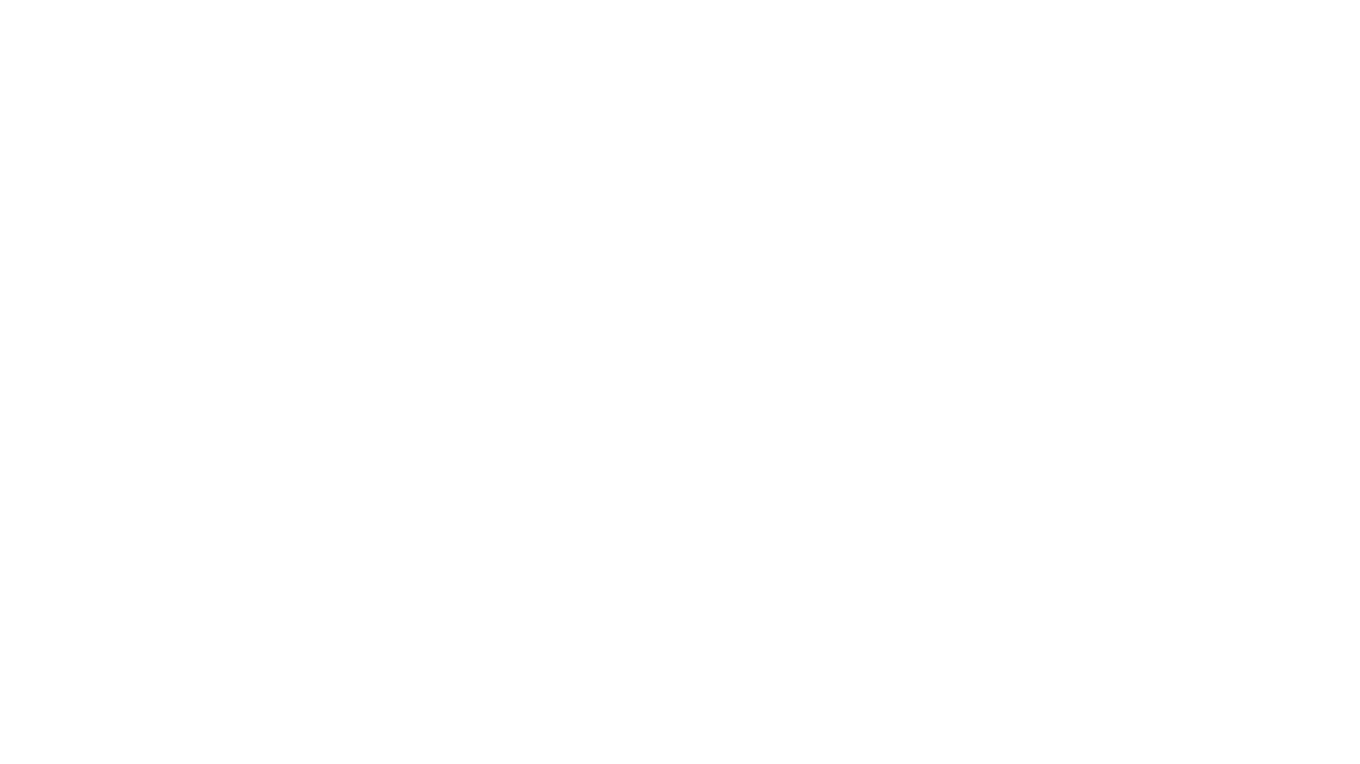
jupyter-ai
Jupyter AI connects generative AI with Jupyter notebooks. It provides a user-friendly and powerful way to explore generative AI models in notebooks and improve your productivity in JupyterLab and the Jupyter Notebook. Specifically, Jupyter AI offers: * An `%%ai` magic that turns the Jupyter notebook into a reproducible generative AI playground. This works anywhere the IPython kernel runs (JupyterLab, Jupyter Notebook, Google Colab, Kaggle, VSCode, etc.). * A native chat UI in JupyterLab that enables you to work with generative AI as a conversational assistant. * Support for a wide range of generative model providers, including AI21, Anthropic, AWS, Cohere, Gemini, Hugging Face, NVIDIA, and OpenAI. * Local model support through GPT4All, enabling use of generative AI models on consumer grade machines with ease and privacy.
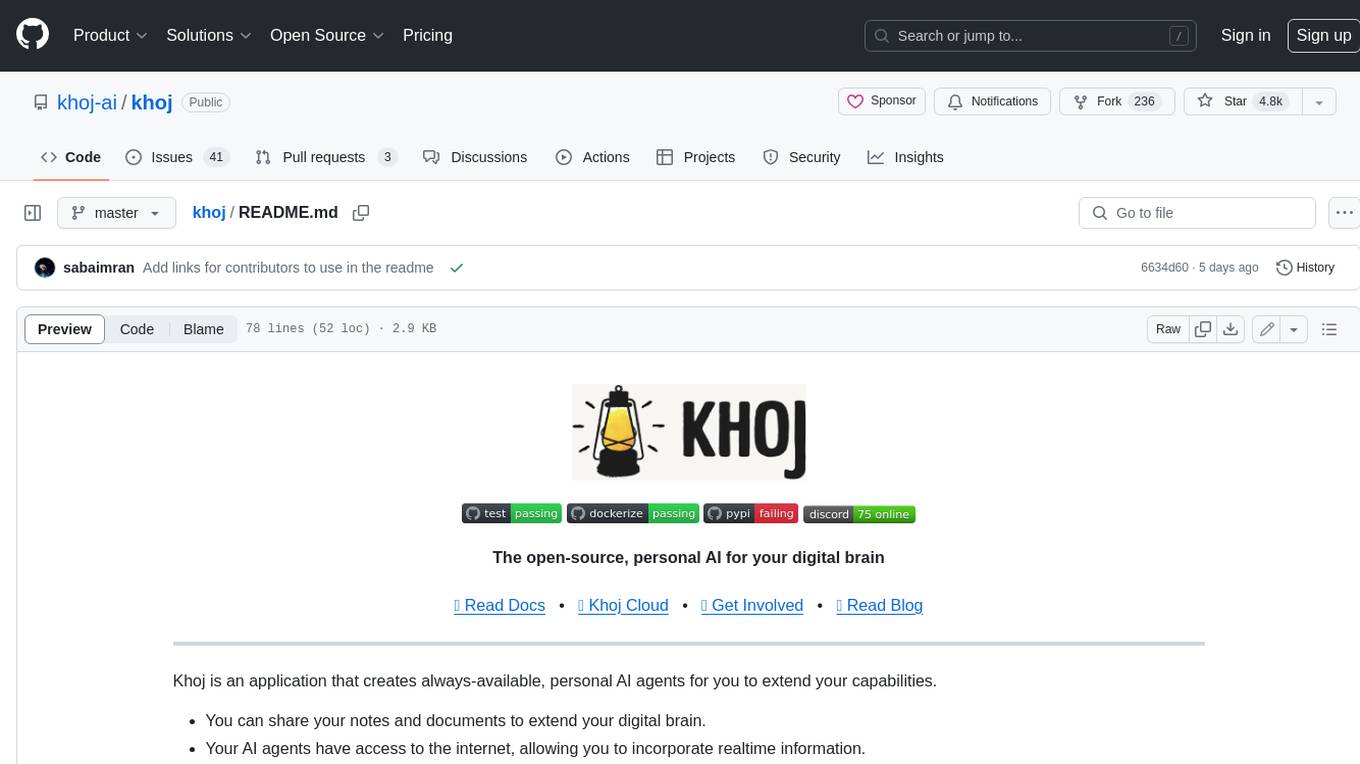
khoj
Khoj is an open-source, personal AI assistant that extends your capabilities by creating always-available AI agents. You can share your notes and documents to extend your digital brain, and your AI agents have access to the internet, allowing you to incorporate real-time information. Khoj is accessible on Desktop, Emacs, Obsidian, Web, and Whatsapp, and you can share PDF, markdown, org-mode, notion files, and GitHub repositories. You'll get fast, accurate semantic search on top of your docs, and your agents can create deeply personal images and understand your speech. Khoj is self-hostable and always will be.
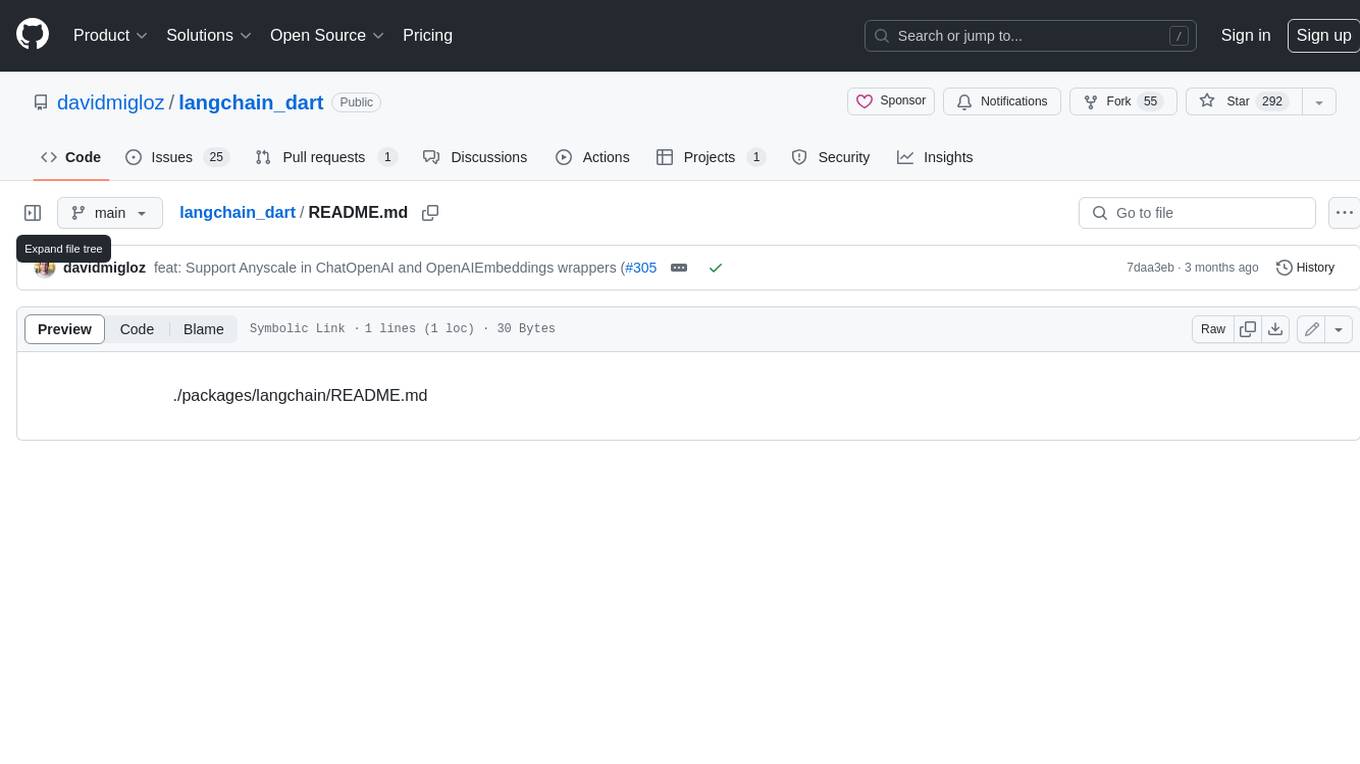
langchain_dart
LangChain.dart is a Dart port of the popular LangChain Python framework created by Harrison Chase. LangChain provides a set of ready-to-use components for working with language models and a standard interface for chaining them together to formulate more advanced use cases (e.g. chatbots, Q&A with RAG, agents, summarization, extraction, etc.). The components can be grouped into a few core modules: * **Model I/O:** LangChain offers a unified API for interacting with various LLM providers (e.g. OpenAI, Google, Mistral, Ollama, etc.), allowing developers to switch between them with ease. Additionally, it provides tools for managing model inputs (prompt templates and example selectors) and parsing the resulting model outputs (output parsers). * **Retrieval:** assists in loading user data (via document loaders), transforming it (with text splitters), extracting its meaning (using embedding models), storing (in vector stores) and retrieving it (through retrievers) so that it can be used to ground the model's responses (i.e. Retrieval-Augmented Generation or RAG). * **Agents:** "bots" that leverage LLMs to make informed decisions about which available tools (such as web search, calculators, database lookup, etc.) to use to accomplish the designated task. The different components can be composed together using the LangChain Expression Language (LCEL).
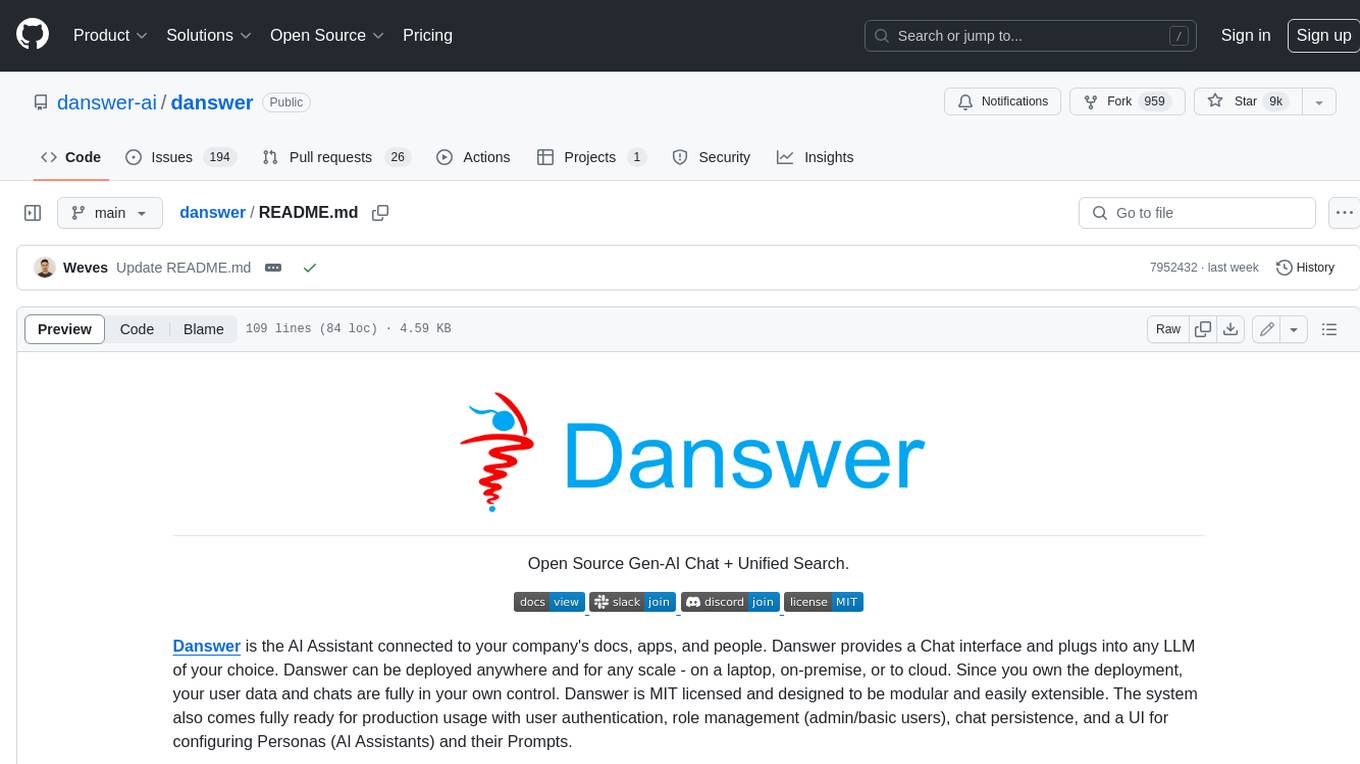
danswer
Danswer is an open-source Gen-AI Chat and Unified Search tool that connects to your company's docs, apps, and people. It provides a Chat interface and plugs into any LLM of your choice. Danswer can be deployed anywhere and for any scale - on a laptop, on-premise, or to cloud. Since you own the deployment, your user data and chats are fully in your own control. Danswer is MIT licensed and designed to be modular and easily extensible. The system also comes fully ready for production usage with user authentication, role management (admin/basic users), chat persistence, and a UI for configuring Personas (AI Assistants) and their Prompts. Danswer also serves as a Unified Search across all common workplace tools such as Slack, Google Drive, Confluence, etc. By combining LLMs and team specific knowledge, Danswer becomes a subject matter expert for the team. Imagine ChatGPT if it had access to your team's unique knowledge! It enables questions such as "A customer wants feature X, is this already supported?" or "Where's the pull request for feature Y?"
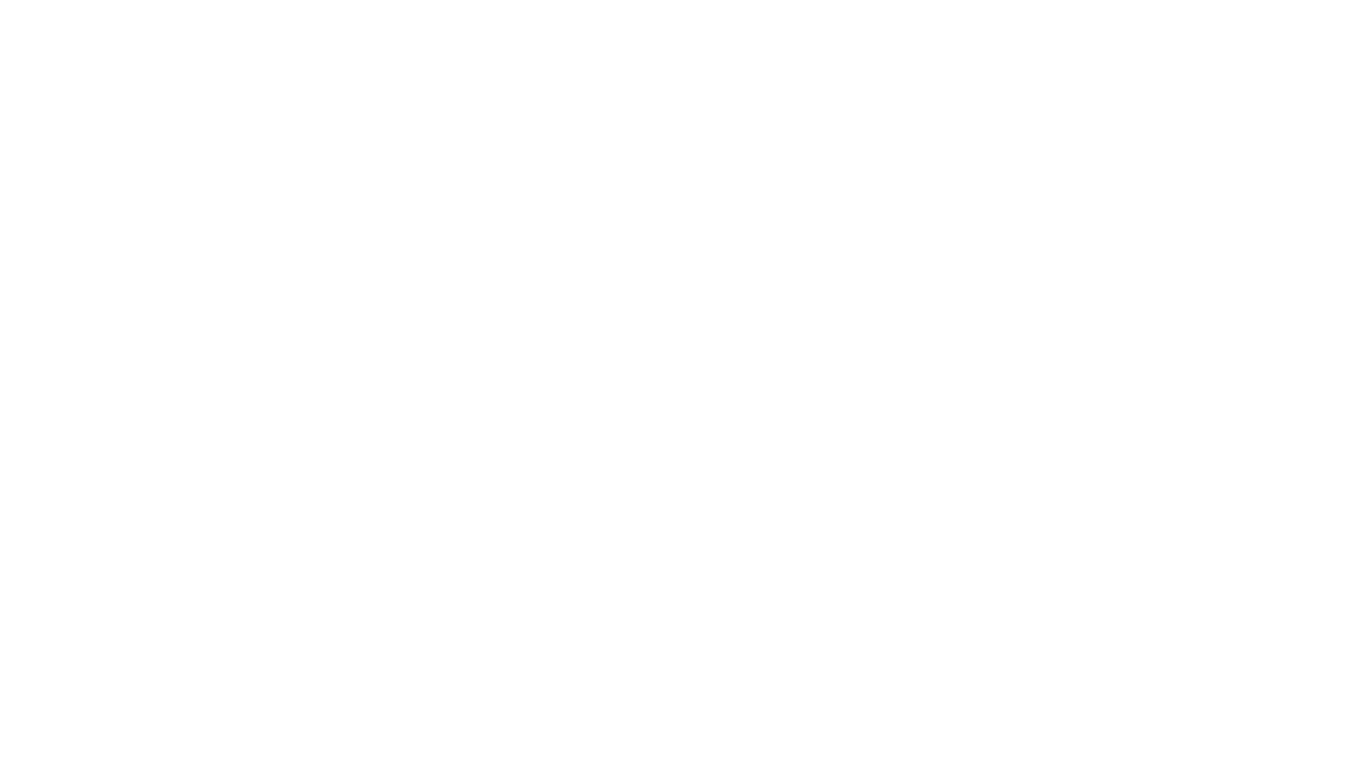
infinity
Infinity is an AI-native database designed for LLM applications, providing incredibly fast full-text and vector search capabilities. It supports a wide range of data types, including vectors, full-text, and structured data, and offers a fused search feature that combines multiple embeddings and full text. Infinity is easy to use, with an intuitive Python API and a single-binary architecture that simplifies deployment. It achieves high performance, with 0.1 milliseconds query latency on million-scale vector datasets and up to 15K QPS.
For similar jobs
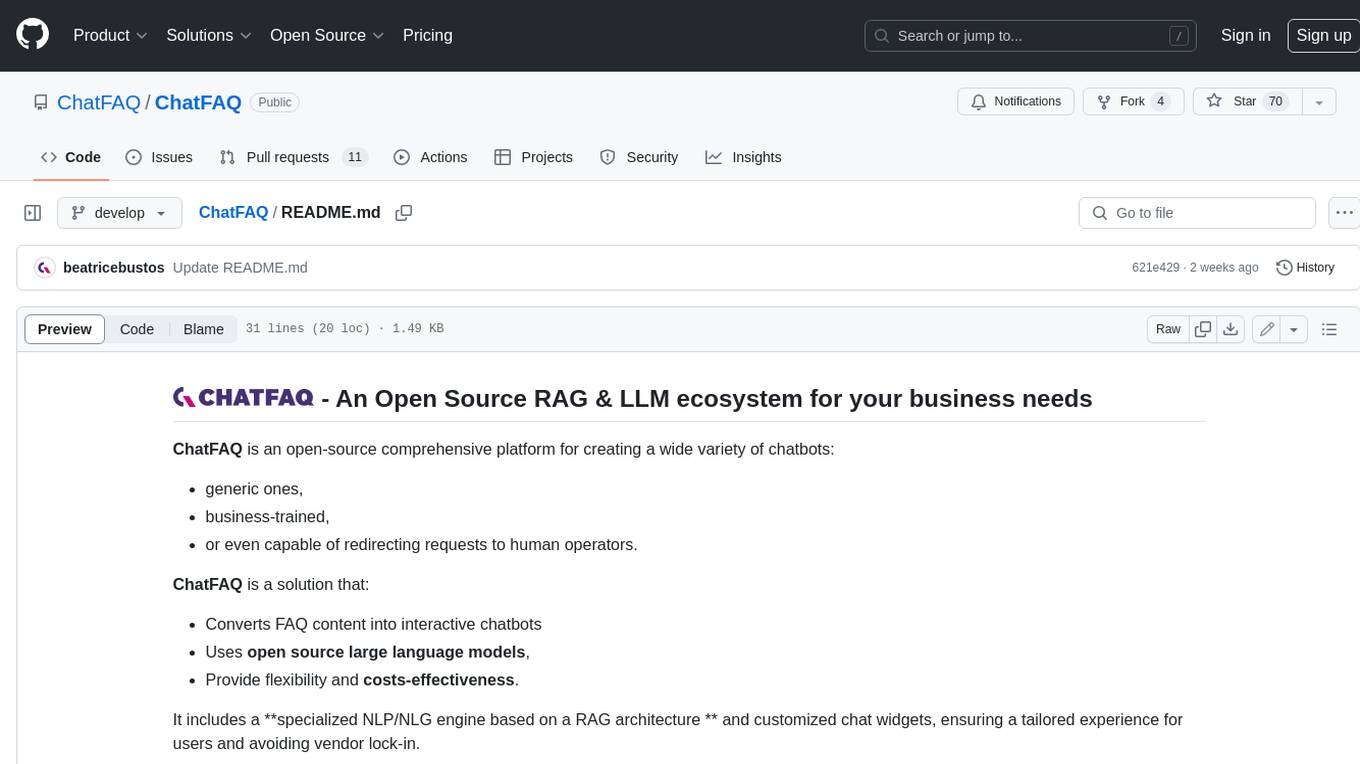
ChatFAQ
ChatFAQ is an open-source comprehensive platform for creating a wide variety of chatbots: generic ones, business-trained, or even capable of redirecting requests to human operators. It includes a specialized NLP/NLG engine based on a RAG architecture and customized chat widgets, ensuring a tailored experience for users and avoiding vendor lock-in.
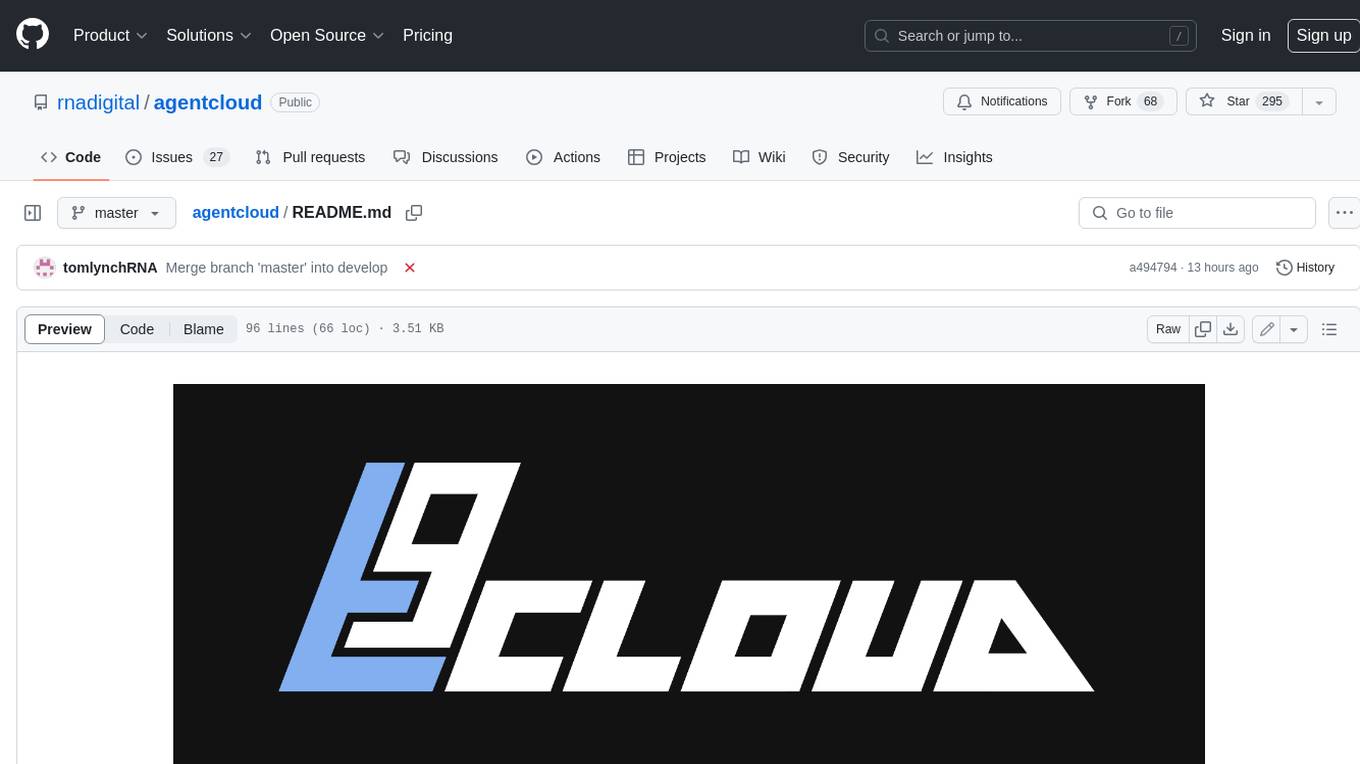
agentcloud
AgentCloud is an open-source platform that enables companies to build and deploy private LLM chat apps, empowering teams to securely interact with their data. It comprises three main components: Agent Backend, Webapp, and Vector Proxy. To run this project locally, clone the repository, install Docker, and start the services. The project is licensed under the GNU Affero General Public License, version 3 only. Contributions and feedback are welcome from the community.
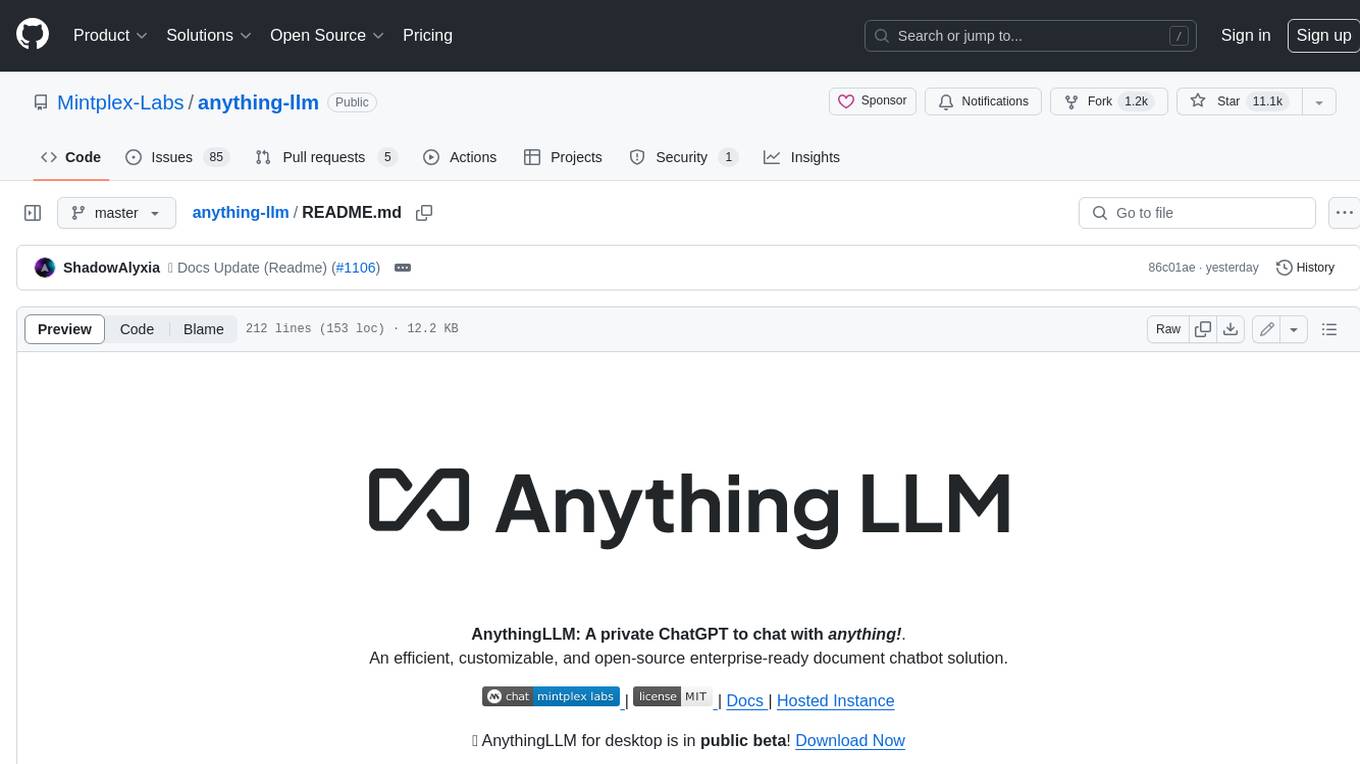
anything-llm
AnythingLLM is a full-stack application that enables you to turn any document, resource, or piece of content into context that any LLM can use as references during chatting. This application allows you to pick and choose which LLM or Vector Database you want to use as well as supporting multi-user management and permissions.
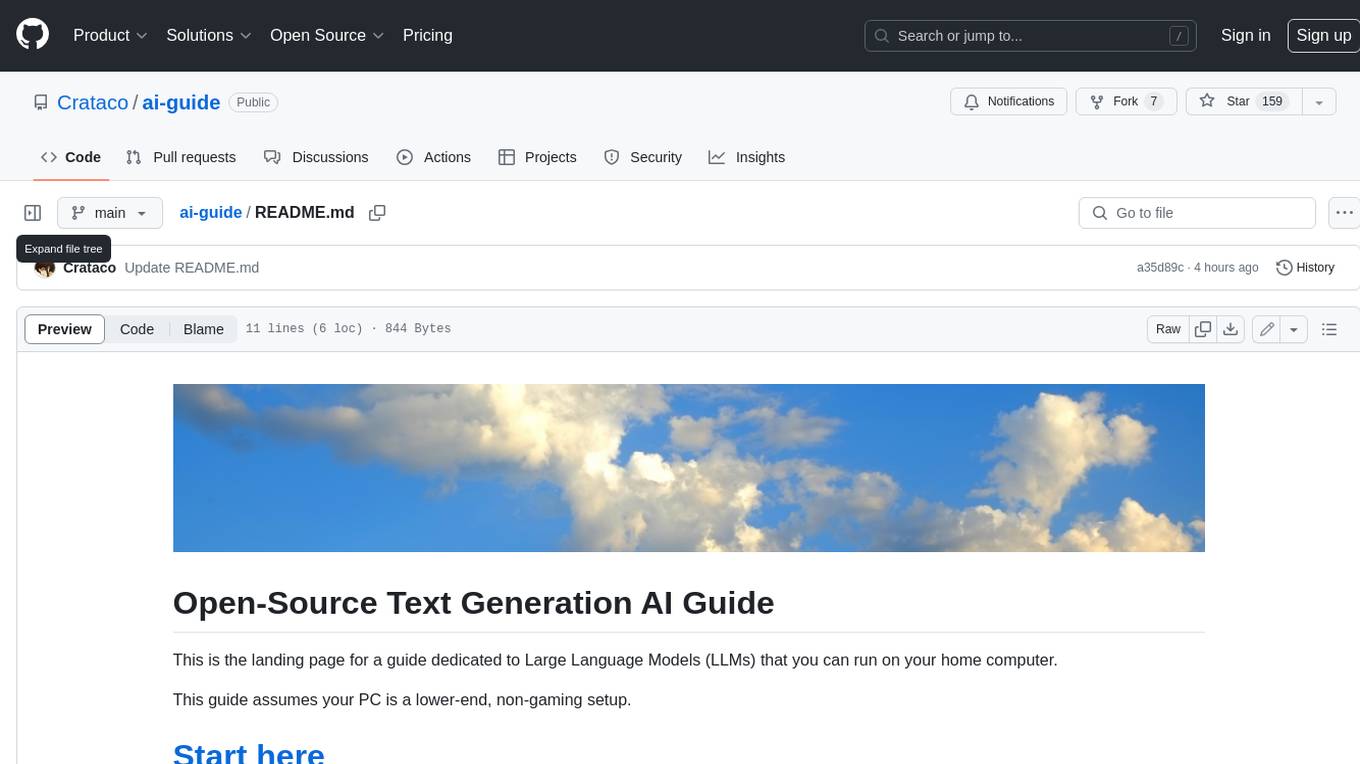
ai-guide
This guide is dedicated to Large Language Models (LLMs) that you can run on your home computer. It assumes your PC is a lower-end, non-gaming setup.
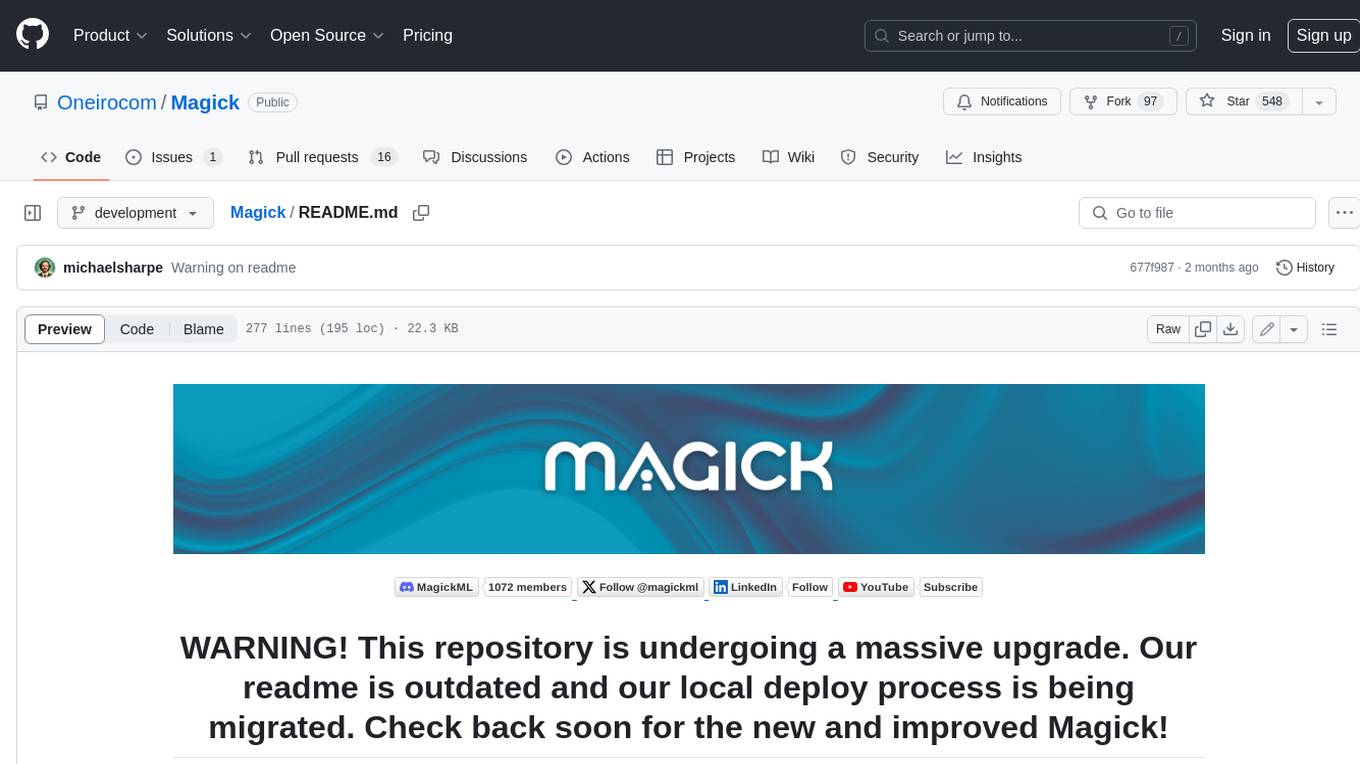
Magick
Magick is a groundbreaking visual AIDE (Artificial Intelligence Development Environment) for no-code data pipelines and multimodal agents. Magick can connect to other services and comes with nodes and templates well-suited for intelligent agents, chatbots, complex reasoning systems and realistic characters.
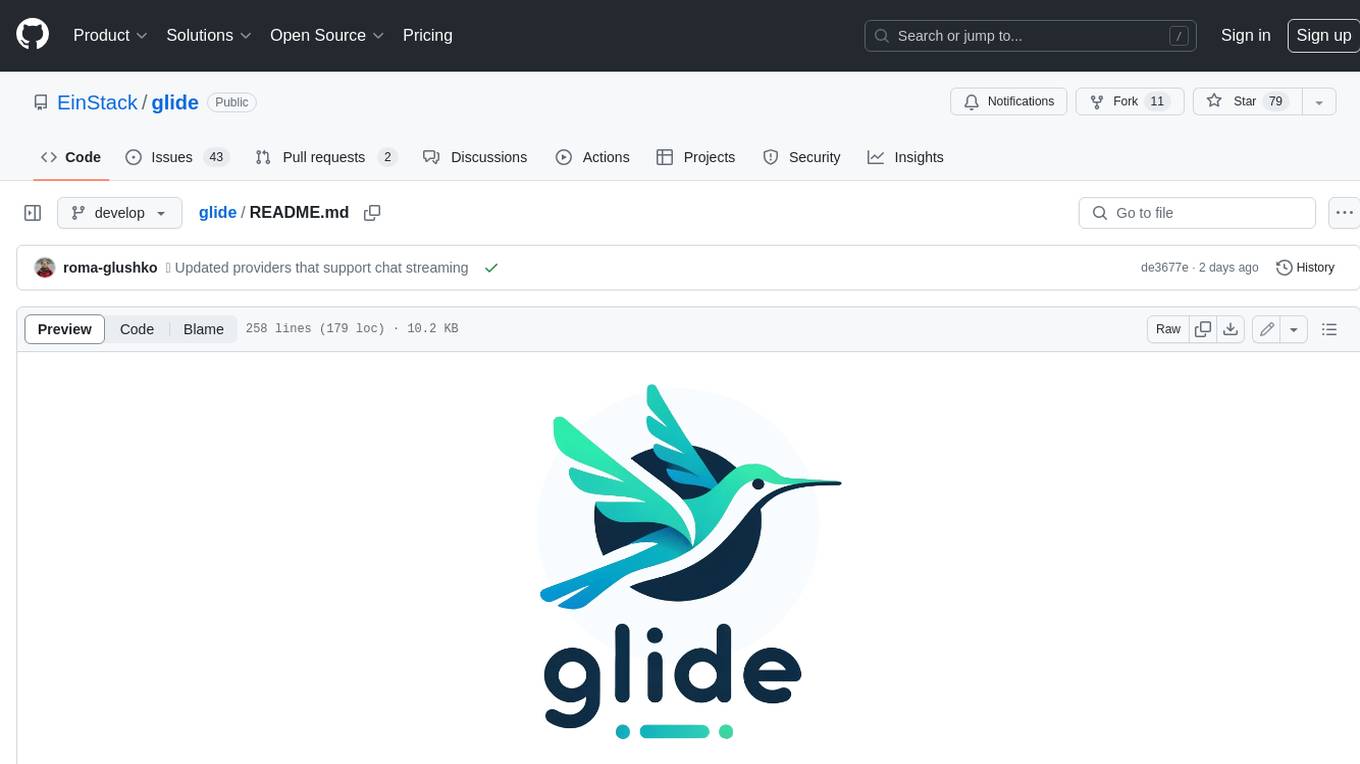
glide
Glide is a cloud-native LLM gateway that provides a unified REST API for accessing various large language models (LLMs) from different providers. It handles LLMOps tasks such as model failover, caching, key management, and more, making it easy to integrate LLMs into applications. Glide supports popular LLM providers like OpenAI, Anthropic, Azure OpenAI, AWS Bedrock (Titan), Cohere, Google Gemini, OctoML, and Ollama. It offers high availability, performance, and observability, and provides SDKs for Python and NodeJS to simplify integration.
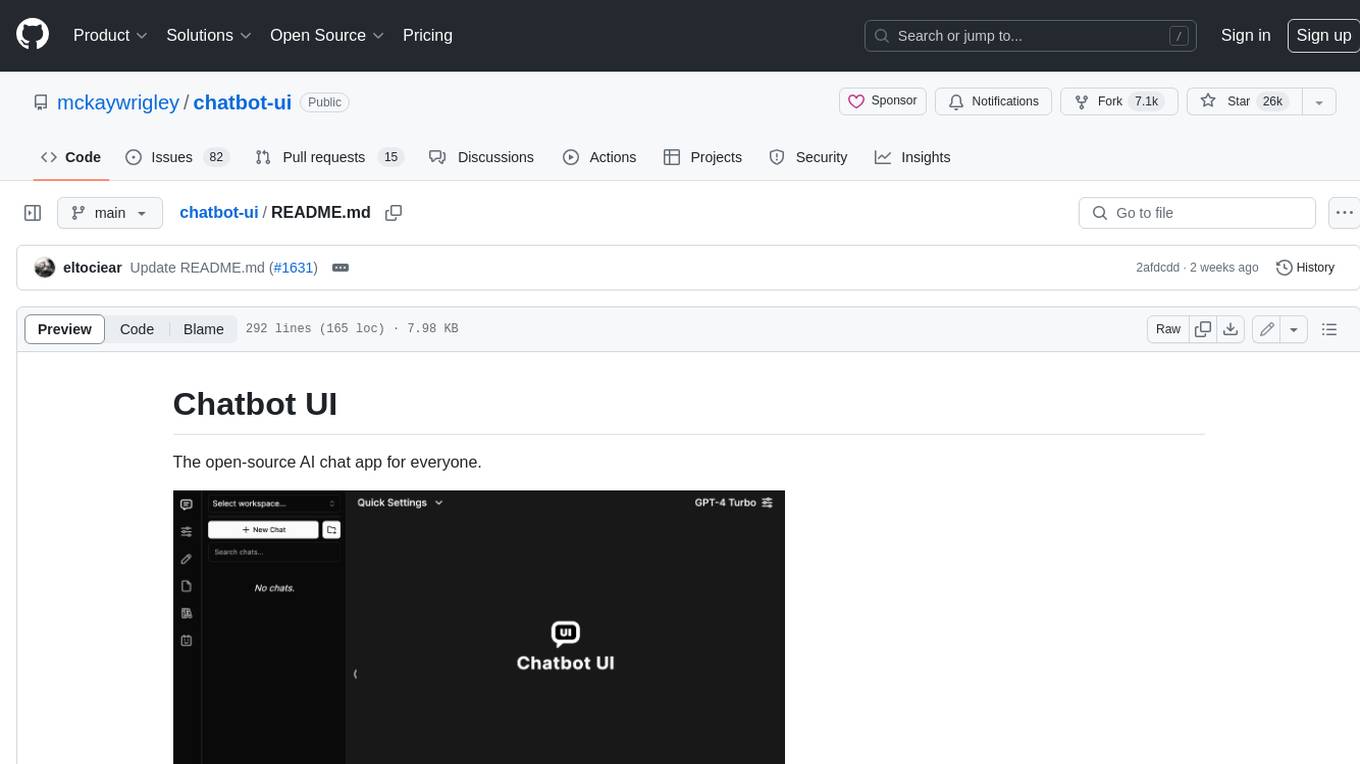
chatbot-ui
Chatbot UI is an open-source AI chat app that allows users to create and deploy their own AI chatbots. It is easy to use and can be customized to fit any need. Chatbot UI is perfect for businesses, developers, and anyone who wants to create a chatbot.
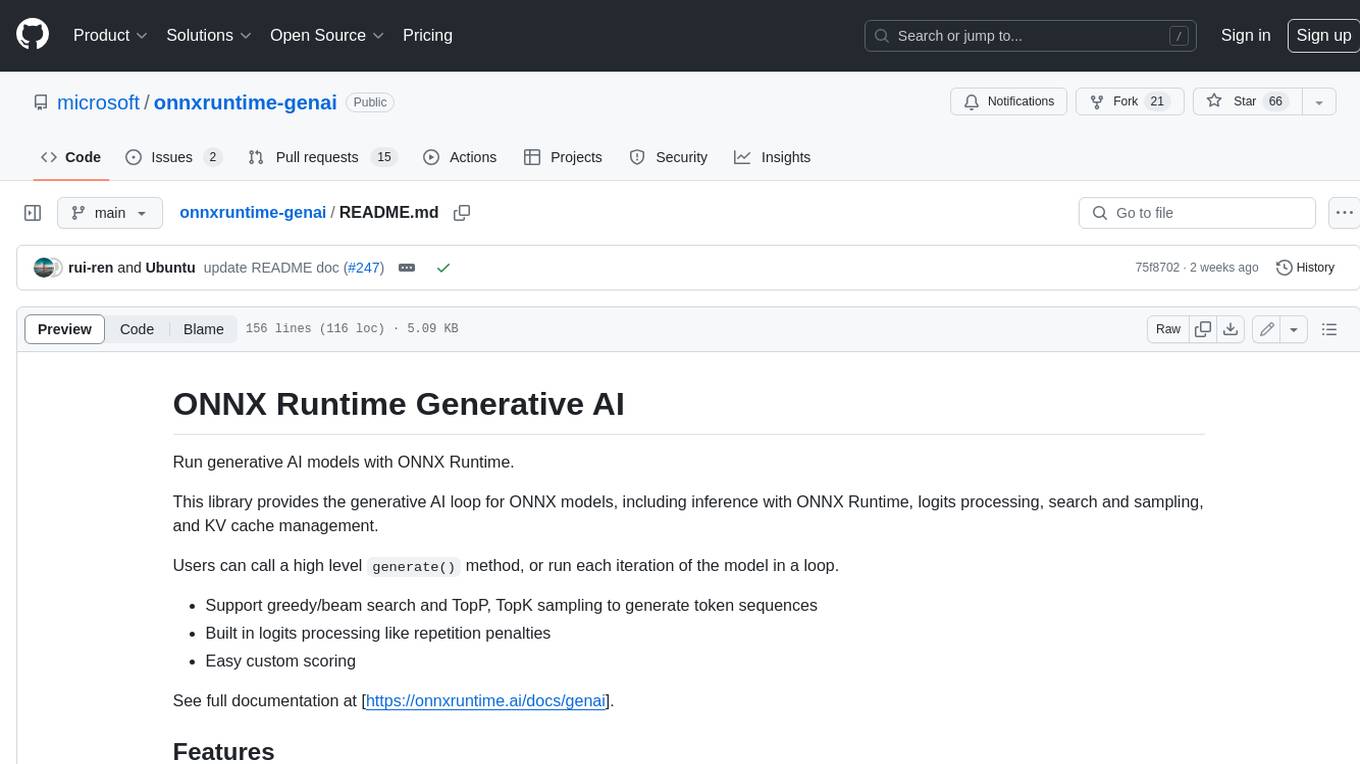
onnxruntime-genai
ONNX Runtime Generative AI is a library that provides the generative AI loop for ONNX models, including inference with ONNX Runtime, logits processing, search and sampling, and KV cache management. Users can call a high level `generate()` method, or run each iteration of the model in a loop. It supports greedy/beam search and TopP, TopK sampling to generate token sequences, has built in logits processing like repetition penalties, and allows for easy custom scoring.