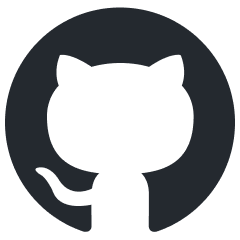
ai_quant_trade
股票AI操盘手:从学习、模拟到实盘,一站式平台。包含股票知识、策略实例、大模型、因子挖掘、传统策略、机器学习、深度学习、强化学习、图网络、高频交易、C++部署和聚宽实例代码等,可以方便学习、模拟及实盘交易
Stars: 2564
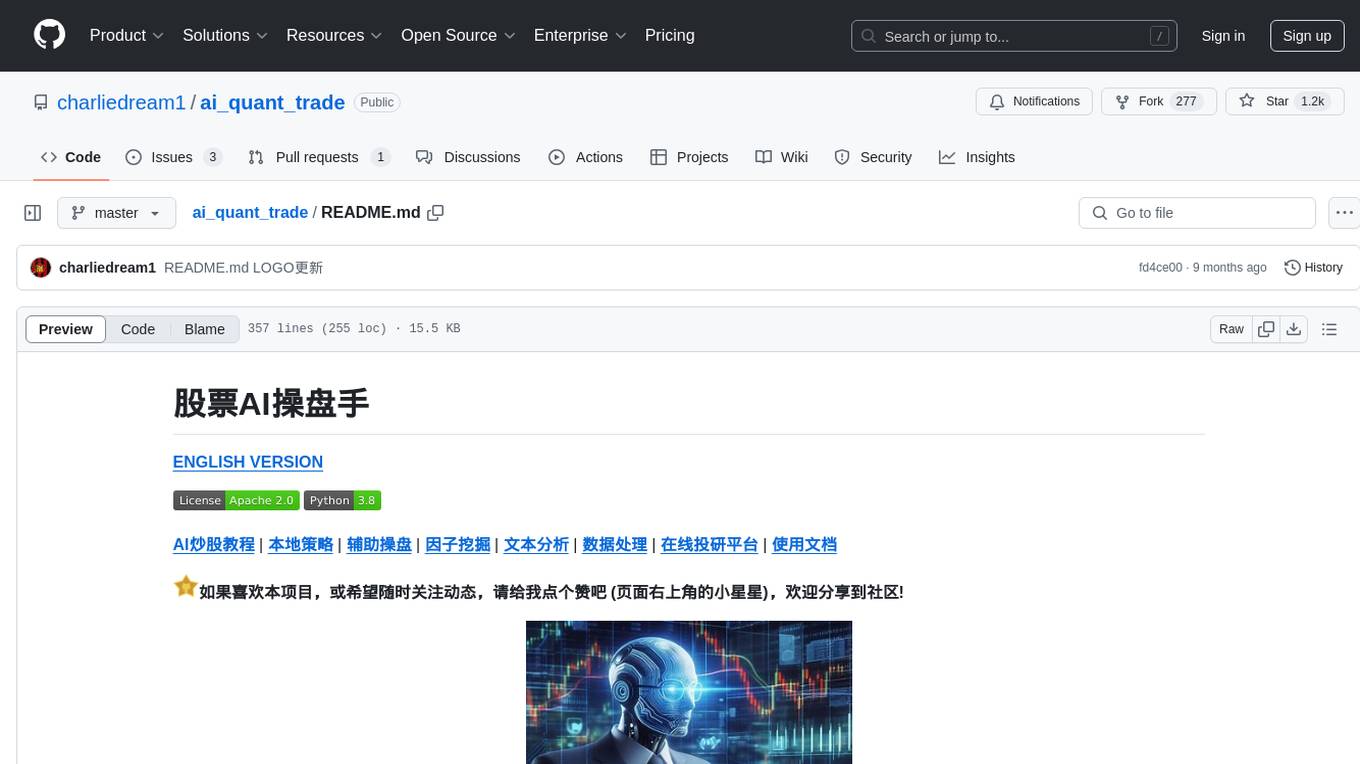
The ai_quant_trade repository is a comprehensive platform for stock AI trading, offering learning, simulation, and live trading capabilities. It includes features such as factor mining, traditional strategies, machine learning, deep learning, reinforcement learning, graph networks, and high-frequency trading. The repository provides tools for monitoring stocks, stock recommendations, and deployment tools for live trading. It also features new functionalities like sentiment analysis using StructBERT, reinforcement learning for multi-stock trading with a 53% annual return, automatic factor mining with 5000 factors, customized stock monitoring software, and local deep reinforcement learning strategies.
README:
AI量化教程 | 本地策略 | 辅助操盘 | 因子挖掘 | 文本分析 | 大模型 | 数据处理 | 在线投研平台 | 使用文档
如果喜欢本项目,或希望随时关注动态,请给我点个赞吧 (页面右上角的小星星),欢迎分享到社区!
AI量化交易操盘手
- 一站式平台:从学习、模拟到实盘
- 炒股策略:大模型、因子挖掘、传统策略、机器学习、深度学习、强化学习、图网络、高频交易等
- 资源汇总:全网资源汇总、实战案例、论文解读、代码实现
- 提供辅助操盘工具:辅助盯盘、股票推荐
- 覆盖股票、基金、加密货币等多个市场
- 实盘部署工具:python/C++/CPU/GPU等部署
本代码仓秉承收费和免费并行的原则。
知识星球
知识星球官网注册,用户权益有保障。
🔥低至每日1毛|独家速成课|无痛学课|📺视频教程|答疑解惑| 开源避坑指南|自研工具代码|3分钟视频论文速度|图书馆| 全网最低价量化类星球之一|3天不满意免费退款👇下方扫描二维码或者点击链接,都可以进入星球查看更详细的介绍🎏
星球视频介绍:
- 星球使用指南:https://mp.weixin.qq.com/s/SGc49e0xf24q5aUbf3rO0g?token=2028063978&lang=zh_CN
- 学习路线及群内资源使用:https://mp.weixin.qq.com/s/3-U048mc0riVsdETrKr77g
星球加入链接:
- AI智投星球:AI量化交易速成、前沿技术、实战案例、资源库
- AI速成营:作为子星球,深入补充编程、大模型、AI基础、原理及金融方向实战及求职等的速成和案例分享,为[AI智投星球]提供扎实的基础知识内容,形成互补
星球介绍:
福利限时放送:
🧧加入并帮助我们推广拉新可返现15元,详情请在星球或公众号内咨询管理员。🧧
🧧同时加入2个星球,立减30元(可先加入一个星球,联系管理员领取优惠卷之后,再加入另一个星球)🧧
👇扫码查看“星球”更详细的介绍(里面有搞笑漫画哦)!
🎈随着人数增加,以及内容的丰富和完善,后续会随时调整价格,请大家不要错过。欢迎大家加入!
🎯本代码仓会持续更新,但部分代码转为私有化维护仅在星球中可见,对应功能会在仓库中标注
微信公众号
🔥最新资讯实时关注
🎁关注并点赞任一篇文章,私信管理员,领取精美量化资料包一份!!!
代码仓 (永久免费)
✨AI量化交易操盘手:
- Github: https://github.com/charliedream1/ai_quant_trade
- Gitee(国内镜像): https://gitee.com/charlie1/ai_quant_trade.git
本仓库配套项目
为了便于维护,将原有的系统操作、编程基础、AI基础、AI实践等内容,独立同步至仓库[AI大模型避坑指南]
里面记录了很多我在实际中遇到的问题和解决方案,同时在实时关注更新技术发展的前沿,欢迎大家关注和star。
✨AI大模型避坑指南:
- Github: https://github.com/charliedream1/ai_wiki
- Gitee(国内镜像):https://gitee.com/charlie1/ai_wiki.git
- 简介:分享各种实用案例,追踪前沿技术发展,囊括 AI 全栈知识,涵盖大模型、编程技术、机器学习、深度学习、强化学习、图神经网络、语音识别、NLP 及图像识别等领域
时间 | 特性 |
---|---|
2025.01.03 | 大模型金融市场分析(视频教程见星球或公众号) |
2023
时间 | 特性 |
---|---|
2023.04.09 | StructBERT市场情绪分析 |
2023.03.28 | 强化学习多股票交易:年化收益53% |
2023.02.28 | 机器学习自动挖掘5000个因子及股票趋势预测 |
2023.02.05 | 利用EXCEL看盘 |
2023.01.01 | 本地深度强化学习策略 |
2022
时间 | 特性 |
---|---|
2022.11.07 | Wind本地实盘模拟 |
2022.08.03 | 基础回测框架 + 双均线策略 |
- 1. 简介
- 2. 使用
- 3. 量化资源集合
- 4. 本地量化平台
- 5. 实盘
- 6. 辅助操盘
- 7. 因子挖掘
- 8. 数据获取
- 9. 文本分析
- 10. 大模型
- 11. 编程及AI基础知识
- 12. 在线投研平台
- 打赏我
- 讨论
- 技术支持
- 常见问题
- 引用
-
本系统适合的人群:
- 机构
- 散户
- 有编程基础
- 无编程基础
-
本仓库代码结构和内容简介
ai_quant_trade ├── ai_notes (金融量化交易知识,以Markdown, Jupyter Notebook汇总知识体系) │ ├── 资源:持续收录全网优秀资源 │ ├── 实战:各类工具、框架、库的使用及踩坑实录 │ ├── 热点:金融市场热点、技术热点、论文解读 ├── docs (本仓库使用说明文档) ├── egs_aide (辅助操盘工具) │ ├── 看盘神器 ├── egs_alpha (因子库) ├── egs_data (数据获取及处理) │ ├── wind (Wind万得数据处理) │ ├── 开源工具 (各类开源数据获取工具使用介绍) ├── egs_fin_nlp (文本分析) │ ├── emotion_analysis (情感分析) ├── egs_llm (大模型应用) ├── egs_online_platform (在线投研平台策略) │ ├── 优矿_Uqer │ ├── 聚宽_JoinQuant ├── egs_trade (本地量化炒股策略) │ ├── paper_trade (实盘模拟) │ ├── wind万得实盘模拟 │ ├── rl (强化学习炒股) │ ├── vanilla (传统规则类策略) ├── quant_brain (核心算法库) ├── runtime (模型的部署和实际使用) ├── tools (辅助工具) ├── requirements.txt └── README.md
本仓库暂未进行封装成python包,拷贝整个项目源代码,在每个egs下的示例中均有详细的使用说明和原理介绍。
安装依赖包
pip install -r requirements.txt
(我们在知乎上2.6万阅读的文章)史上最全AI股票量化交易工具和开源项目汇总
以上是我们在知乎上2.6万阅读的文章,我们将所有工具重新进行了分类,并进行了点评, 收录在ai_notes文件夹下,方便大家查找。
🎯开发中
- 陆续对所有工具进行点评,方便选择
- 陆续记录各个工具的优缺点,形成一个对比表,方便选型
- 陆续记录使用方法,方便使用:我们不做大而全的使用教程,那会让你迷失,我们只列举最常用且实用的功能,让你快速上手
⛳⛳⛳AI基础知识
为了便于维护,将原有的ai_wiki目录下内容,包括系统操作、编程基础、AI基础、AI实践等内容,独立同步至仓库[AI大模型避坑指南]
里面记录了很多我在实际中遇到的问题和解决方案,同时在实时关注更新技术发展的前沿,欢迎大家关注和star。
✨AI大模型避坑指南:
- Github: https://github.com/charliedream1/ai_wiki
- Gitee(国内镜像):https://gitee.com/charlie1/ai_wiki.git
- 简介:分享各种实用案例,追踪前沿技术发展,囊括 AI 全栈知识,涵盖大模型、编程技术、机器学习、深度学习、强化学习、图神经网络、语音识别、NLP 及图像识别等领域
🎯 每个实例我都尽可能配备完善的教程,从原理、使用到代码解读。
可在本地可构建一套独立的量化交易系统,包含的策略:
- AI策略
- 强化学习
- 图网络
- 深度学习
- 机器学习
- 高频交易
- 因子挖掘
- 大模型
- 传统规则类策略
代码详细参见目录:egs_trade/rl
自从2017年AlphaGo与柯洁围棋大战之后,深度强化学习大火。
相比于机器学习和深度学习, 强化学习是以最终目标为导向 (以交互作为目标) , 而很多其他方法是考虑孤立的子问题 (如“股价预测”,“大盘预测”,“交易决策”等) , 这并不能直接获得交互的动作, 比如“命令机器人炒股盈利”, 这个任务包含了“股价预测”,”大盘预测”等等, 而强化学习的目标则是“完成命令者的任务”, 可以直接得到“炒股盈利”的一连贯动作。
-
样例介绍:
序号 策略 论文 1 原型 无 2 FinRL教程0-NeurIPS2018 Practical Deep Reinforcement Learning Approach for Stock Trading (https://arxiv.org/abs/1811.07522) -
样例回测详情
序号 策略 市场 年化收益 最大回撤 夏普率 1 原型 中国A股 2 FinRL教程0-NeurIPS2018 美股道儿琼斯30 53.1% -10.4% 2.17
图网络可以更好的构建股票和股票之间的关系,同时关联股票、新闻、情绪等各类信息,能更好的挖掘全局关系网。
(构建中,尽请期待。。。)
自从2012年AlexNet在图像分类任务上,性能碾压传统机器学习性能后,深度学习大火, 随机开启第一波人工智能热潮。其主要用于股价和大盘的预测等。
(构建中,尽请期待。。。)
机器学习以统计学为基础,以其坚实的数据基础,可解性,数据依赖少,资源占用低,训练速度快,在表格任务上, 仍然可以追平深度学习等优势,任有其应用价值。
(构建中,尽请期待。。。)
(构建中,尽请期待。。。)
传统策略虽然看似昨日黄花,但其可操作性更强,仍又一定使用价值。深度学习和机器学习,往往需要配合规则使用。
-
- 详细使用教程
- 包含策略代码+自建纯手写回测框架
- 包含良好的绘图,指示买点和卖点
- 目标:通过这个实例了解量化交易的完整框架构建方式
代码详细参见目录:egs_trade
-
Wind本地实盘模拟:双均线策略
- 利用wind软件实现的实盘模拟
- wind常作为各大金融机构的首选数据源,由于价格高额,不适合散户使用
- 使用对象:机构
代码详细参见目录:egs_aide
-
利用EXCEL看盘
- 看盘是不容易被发现
- 可以自己加入要盯盘的股票
- 可以方便利用excel快速计算和处理数据
代码详细参见目录:egs_alpha
序号 | 策略 | 论文 |
---|---|---|
1 | 机器学习自动挖掘5000个因子及股票趋势预测 |
序号 | 因子库 |
---|---|
1 | alpha101 |
2 | stockstats |
3 | ta_lib |
- 各类常见数据源使用详解
- 统一数据源接口
代码详细参见目录:egs_fin_nlp
序号 | 工具 |
---|---|
1 | StructBERT市场情绪分析 |
代码详细参见目录:egs_llm
序号 | 工具 |
---|---|
1 | 大模型金融市场分析(视频教程见星球或公众号) |
为了便于维护,将原有的ai_wiki目录下内容,包括系统操作、编程基础、AI基础、AI实践等内容,独立同步至仓库[AI大模型避坑指南]
里面记录了很多我在实际中遇到的问题和解决方案,同时在实时关注更新技术发展的前沿,欢迎大家关注和star。
✨AI大模型避坑指南:
- Github: https://github.com/charliedream1/ai_wiki
- Gitee(国内镜像):https://gitee.com/charlie1/ai_wiki.git
- 简介:分享各种实用案例,追踪前沿技术发展,囊括 AI 全栈知识,涵盖大模型、编程技术、机器学习、深度学习、强化学习、图神经网络、语音识别、NLP 及图像识别等领域
国内量化平台,如聚宽、优矿、米筐、果仁和BigQuant等,如果感兴趣,也可以自行尝试。
投研平台是为量化爱好者(宽客)量身打造的云平台,提供免费股票数据获取、精准的回测功能、 高速实盘交易接口、易用的API文档、由易入难的策略库,便于快速实现和验证策略。( 注:如下策略仅在所述回测段有效,没有进行详细的调优和全周期验证。另外,没有策略能保证全周期有效的, 如果实盘使用如下策略,请慎重使用)
欢迎在聚宽平台关注我:量客攻城狮
- 具体策略详细介绍和源码请单击如下对应策略链接访问查看
- 聚宽使用介绍请查看: egs_online_platform/聚宽_JoinQuant
- 该部分代码仅能在 聚宽平台 运行
-
股票量化策略
策略 收益 最大回撤 机器学习-动态因子选择策略 12.3% 38.93% 小市值+多均线量化炒股 58.4% 46.61% 龙虎榜-看长做短 41.82% 26.89% 强势股+趋势线判断+止损止盈 10.09% 21.449% -
股票分析研究
您的支持是我前进的动力,即便“1毛钱”我也很开心啊,感谢您的打赏和支持 (^o^)/
欢迎在 Github Discussions 中发起讨论。
- 欢迎在 Github Issues 中提交问题。
- 加入知识星球,获取更多技术支持。
请查看文档常见问题
@misc{ai_quant_trade,
author={Yi Li},
title={ai_quant_trade},
year={2022},
publisher = {GitHub},
journal = {GitHub repository},
howpublished = {\url{https://github.com/charliedream1/ai_quant_trade}},
}
For Tasks:
Click tags to check more tools for each tasksFor Jobs:
Alternative AI tools for ai_quant_trade
Similar Open Source Tools
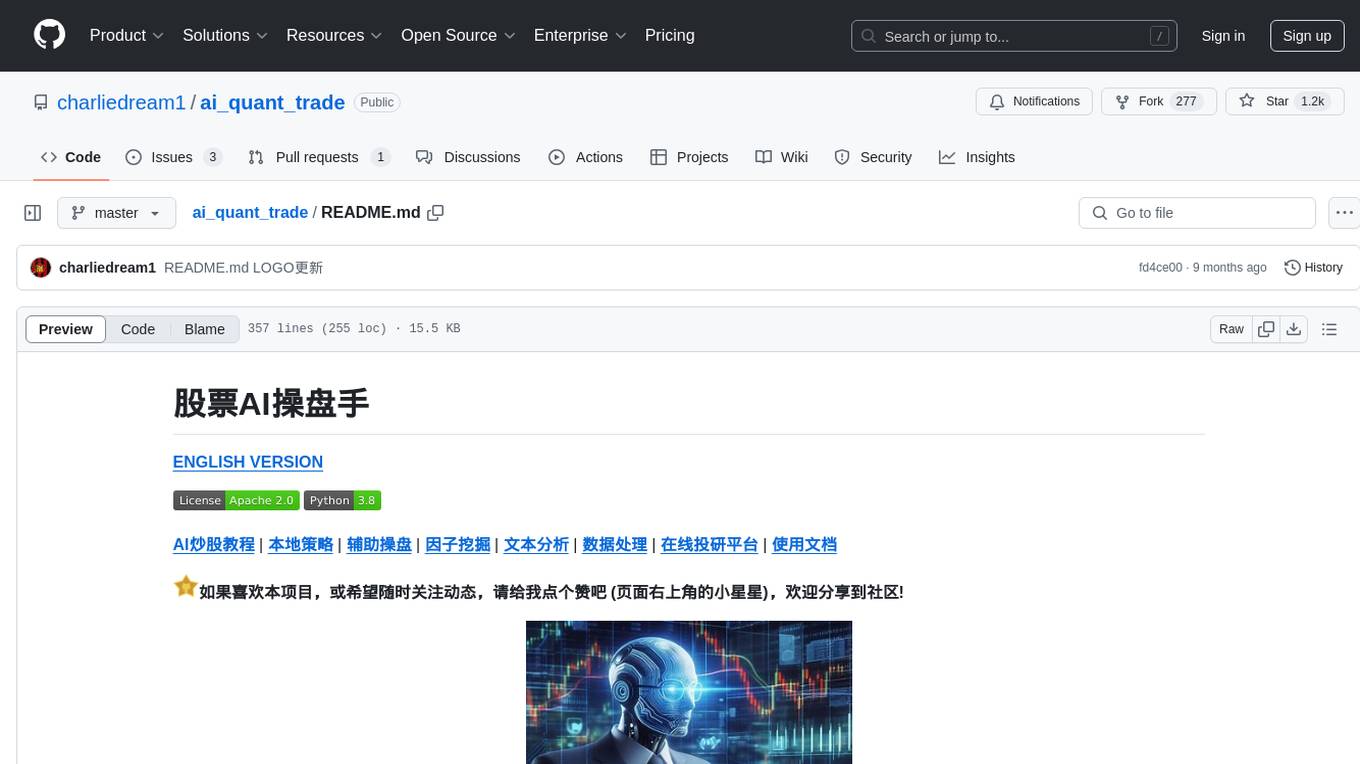
ai_quant_trade
The ai_quant_trade repository is a comprehensive platform for stock AI trading, offering learning, simulation, and live trading capabilities. It includes features such as factor mining, traditional strategies, machine learning, deep learning, reinforcement learning, graph networks, and high-frequency trading. The repository provides tools for monitoring stocks, stock recommendations, and deployment tools for live trading. It also features new functionalities like sentiment analysis using StructBERT, reinforcement learning for multi-stock trading with a 53% annual return, automatic factor mining with 5000 factors, customized stock monitoring software, and local deep reinforcement learning strategies.
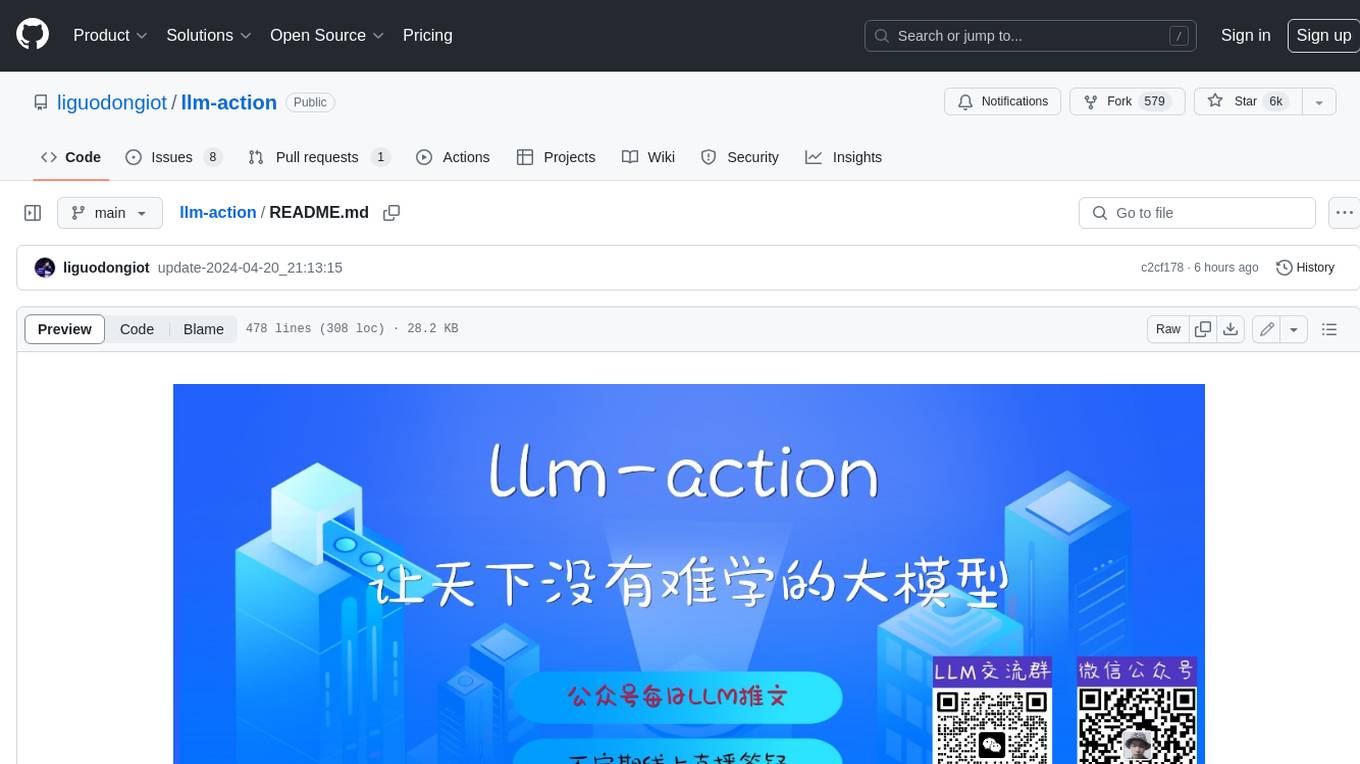
llm-action
This repository provides a comprehensive guide to large language models (LLMs), covering various aspects such as training, fine-tuning, compression, and applications. It includes detailed tutorials, code examples, and explanations of key concepts and techniques. The repository is maintained by Liguo Dong, an AI researcher and engineer with expertise in LLM research and development.
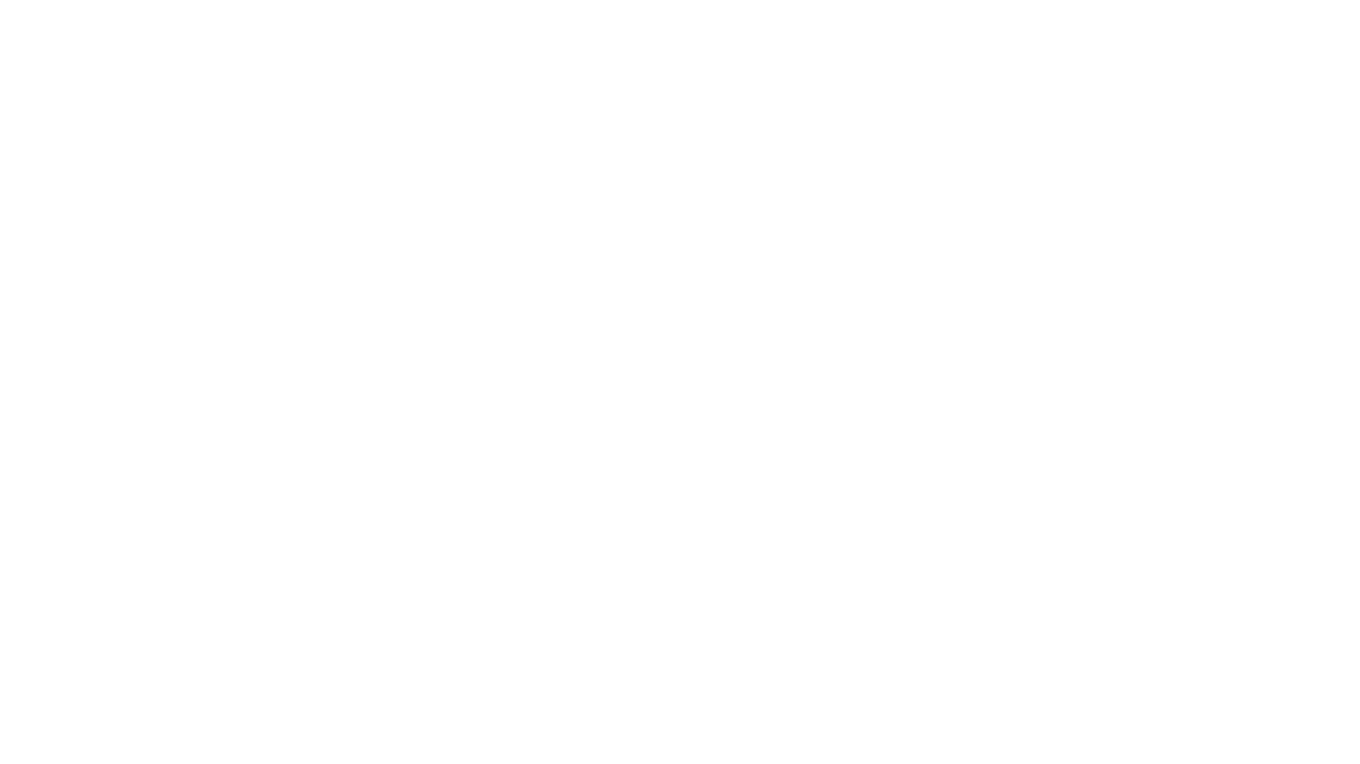
ChatGPT-Next-Web-Pro
ChatGPT-Next-Web-Pro is a tool that provides an enhanced version of ChatGPT-Next-Web with additional features and functionalities. It offers complete ChatGPT-Next-Web functionality, file uploading and storage capabilities, drawing and video support, multi-modal support, reverse model support, knowledge base integration, translation, customizations, and more. The tool can be deployed with or without a backend, allowing users to interact with AI models, manage accounts, create models, manage API keys, handle orders, manage memberships, and more. It supports various cloud services like Aliyun OSS, Tencent COS, and Minio for file storage, and integrates with external APIs like Azure, Google Gemini Pro, and Luma. The tool also provides options for customizing website titles, subtitles, icons, and plugin buttons, and offers features like voice input, file uploading, real-time token count display, and more.
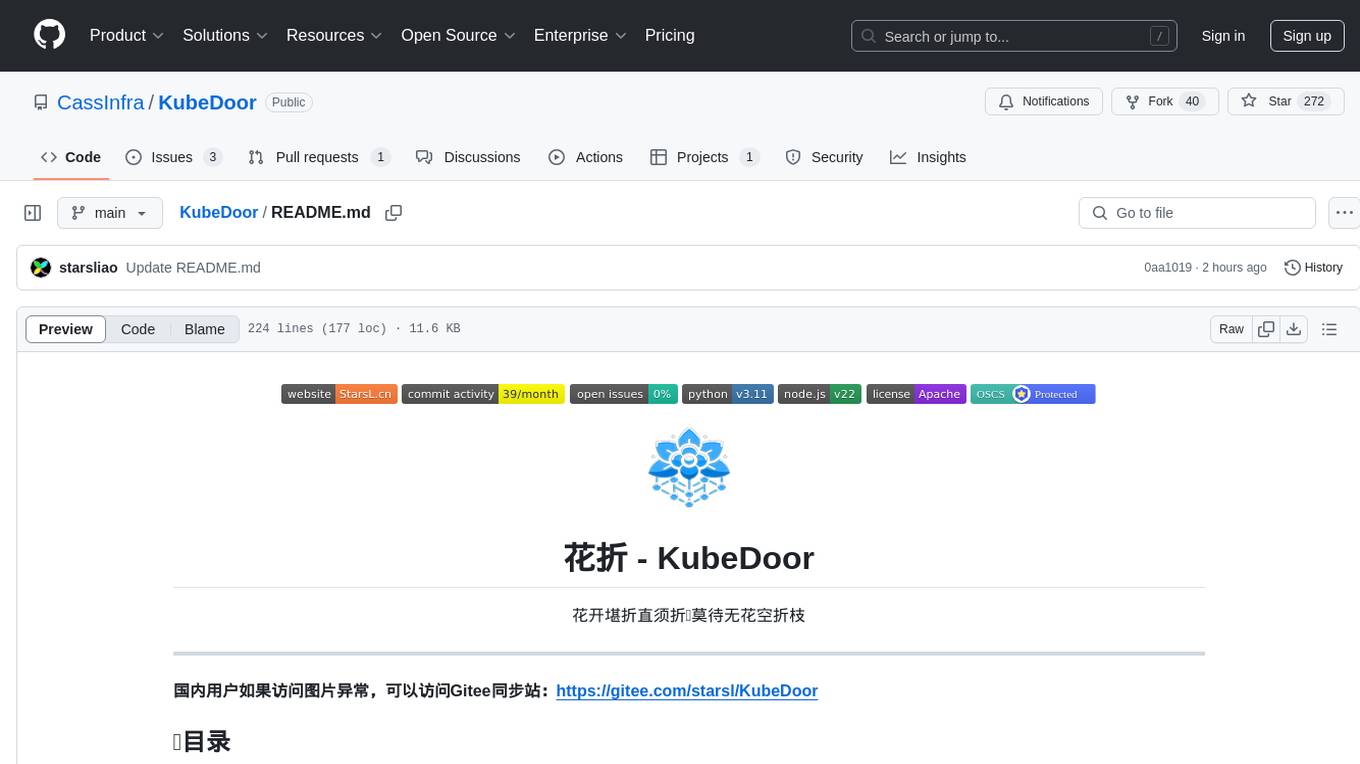
KubeDoor
KubeDoor is a microservice resource management platform developed using Python and Vue, based on K8S admission control mechanism. It supports unified remote storage, monitoring, alerting, notification, and display for multiple K8S clusters. The platform focuses on resource analysis and control during daily peak hours of microservices, ensuring consistency between resource request rate and actual usage rate.
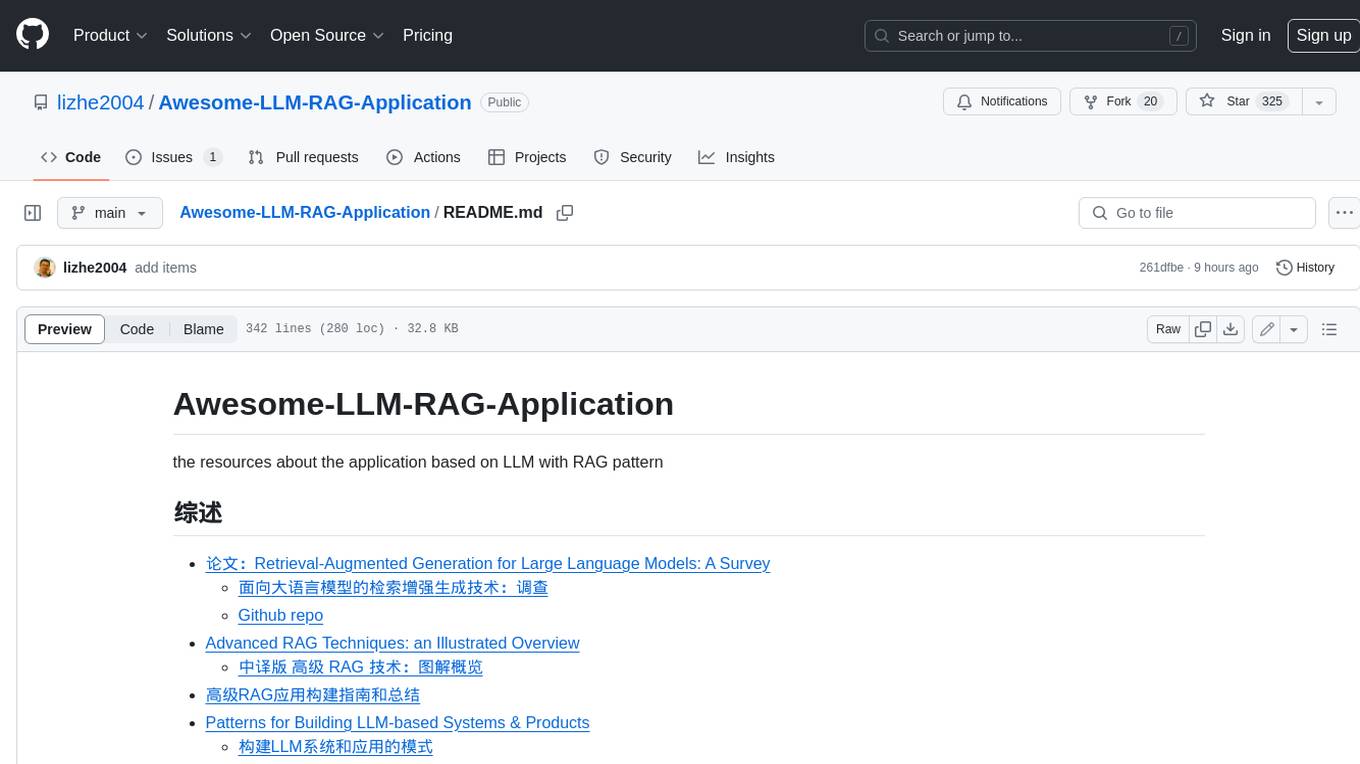
Awesome-LLM-RAG-Application
Awesome-LLM-RAG-Application is a repository that provides resources and information about applications based on Large Language Models (LLM) with Retrieval-Augmented Generation (RAG) pattern. It includes a survey paper, GitHub repo, and guides on advanced RAG techniques. The repository covers various aspects of RAG, including academic papers, evaluation benchmarks, downstream tasks, tools, and technologies. It also explores different frameworks, preprocessing tools, routing mechanisms, evaluation frameworks, embeddings, security guardrails, prompting tools, SQL enhancements, LLM deployment, observability tools, and more. The repository aims to offer comprehensive knowledge on RAG for readers interested in exploring and implementing LLM-based systems and products.
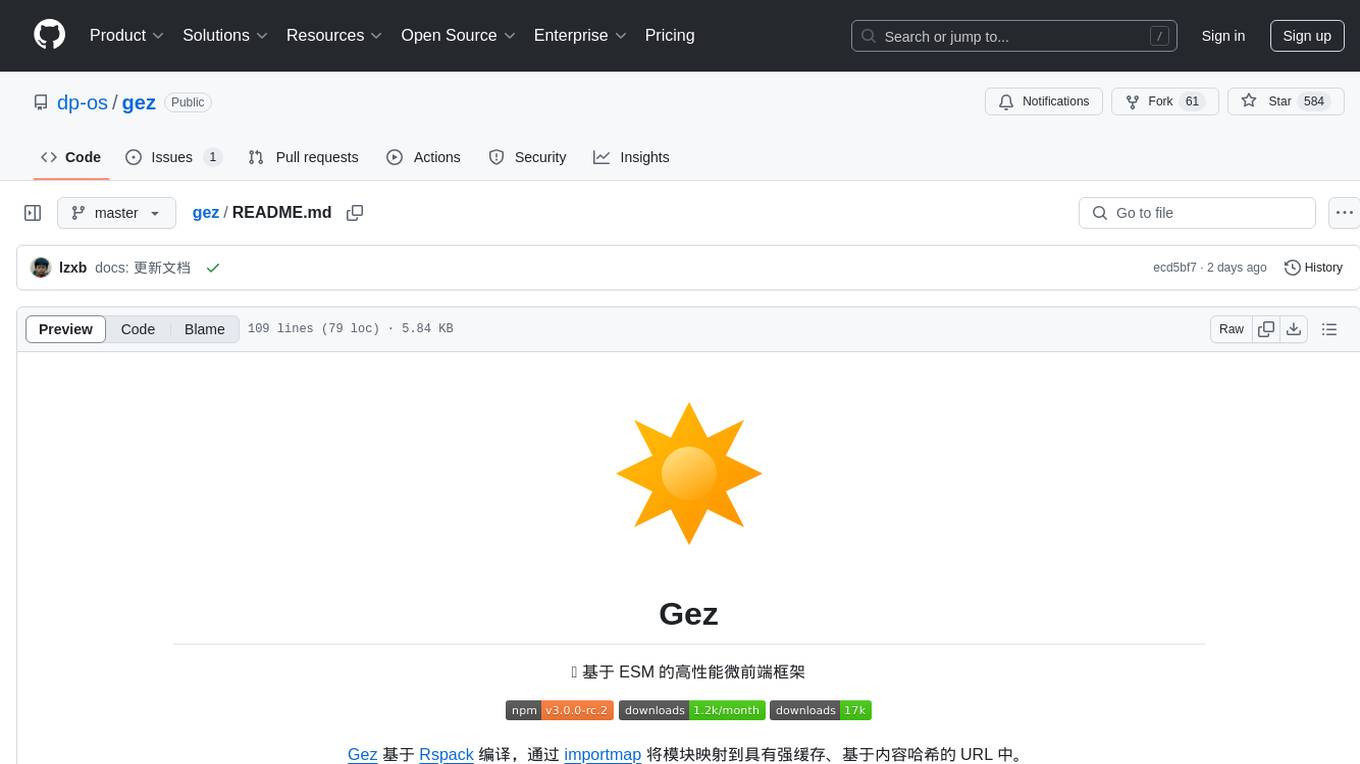
gez
Gez is a high-performance micro frontend framework based on ESM. It uses Rspack compilation and maps modules to URLs with strong caching and content-based hashing. Gez embraces modern micro frontend architecture by leveraging ESM and importmap for dependency management, providing reliable isolation with module scope, seamless integration with any modern frontend framework, intuitive development experience, and optimal performance with zero runtime overhead and reliable caching strategies.
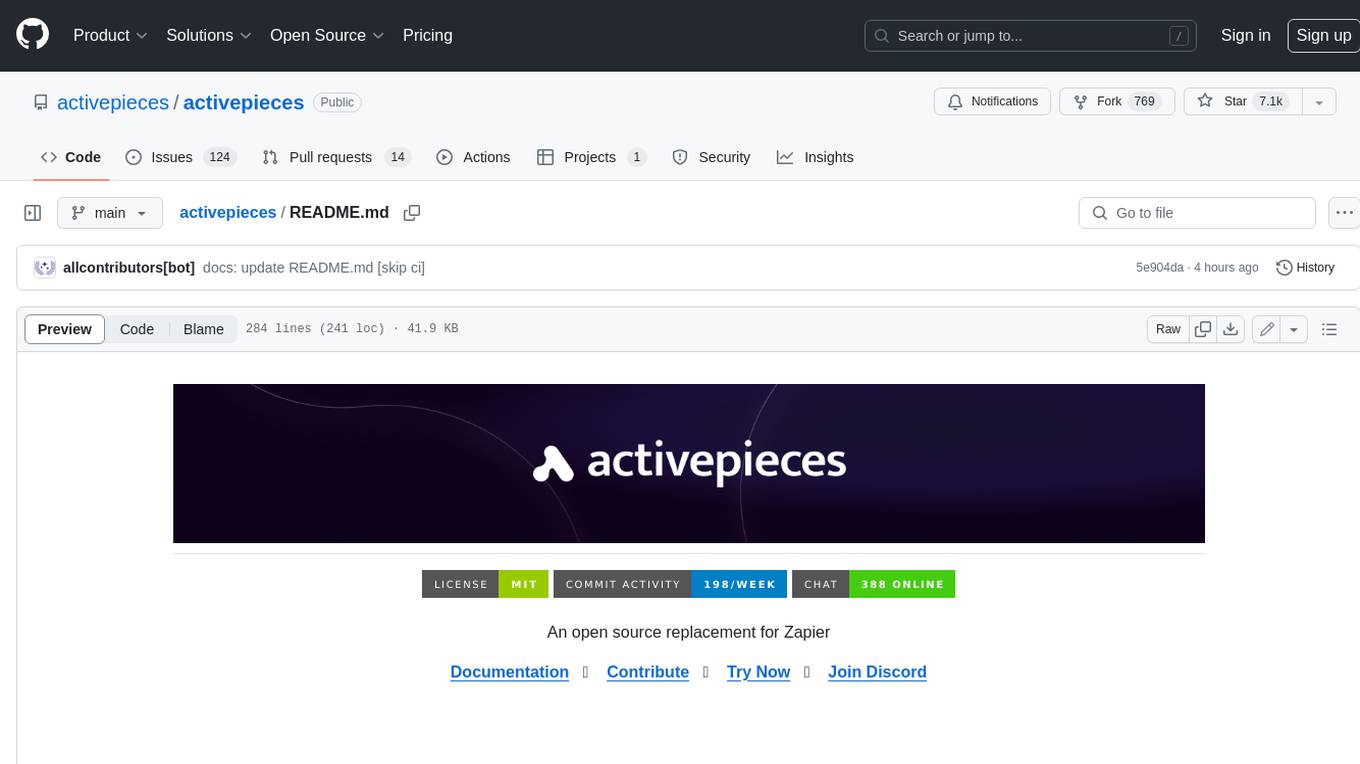
activepieces
Activepieces is an open source replacement for Zapier, designed to be extensible through a type-safe pieces framework written in Typescript. It features a user-friendly Workflow Builder with support for Branches, Loops, and Drag and Drop. Activepieces integrates with Google Sheets, OpenAI, Discord, and RSS, along with 80+ other integrations. The list of supported integrations continues to grow rapidly, thanks to valuable contributions from the community. Activepieces is an open ecosystem; all piece source code is available in the repository, and they are versioned and published directly to npmjs.com upon contributions. If you cannot find a specific piece on the pieces roadmap, please submit a request by visiting the following link: Request Piece Alternatively, if you are a developer, you can quickly build your own piece using our TypeScript framework. For guidance, please refer to the following guide: Contributor's Guide
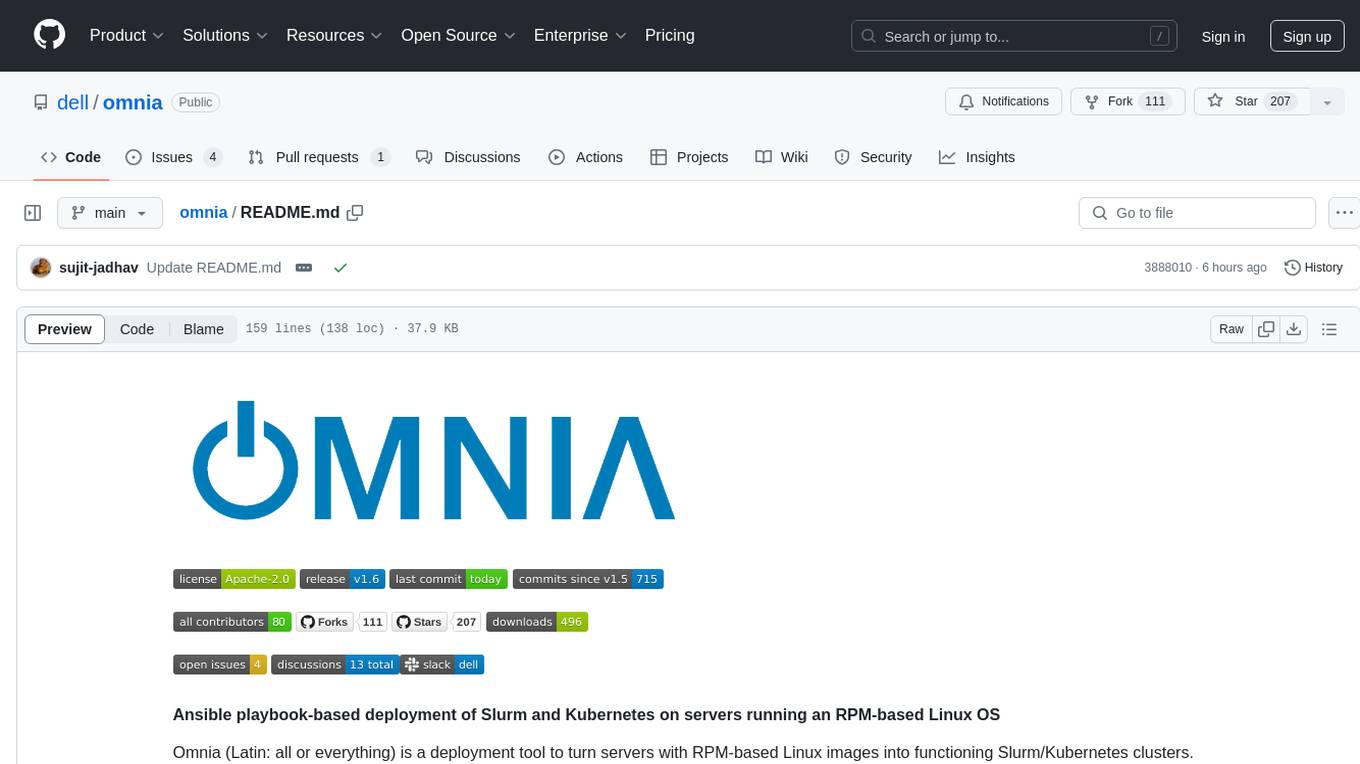
omnia
Omnia is a deployment tool designed to turn servers with RPM-based Linux images into functioning Slurm/Kubernetes clusters. It provides an Ansible playbook-based deployment for Slurm and Kubernetes on servers running an RPM-based Linux OS. The tool simplifies the process of setting up and managing clusters, making it easier for users to deploy and maintain their infrastructure.
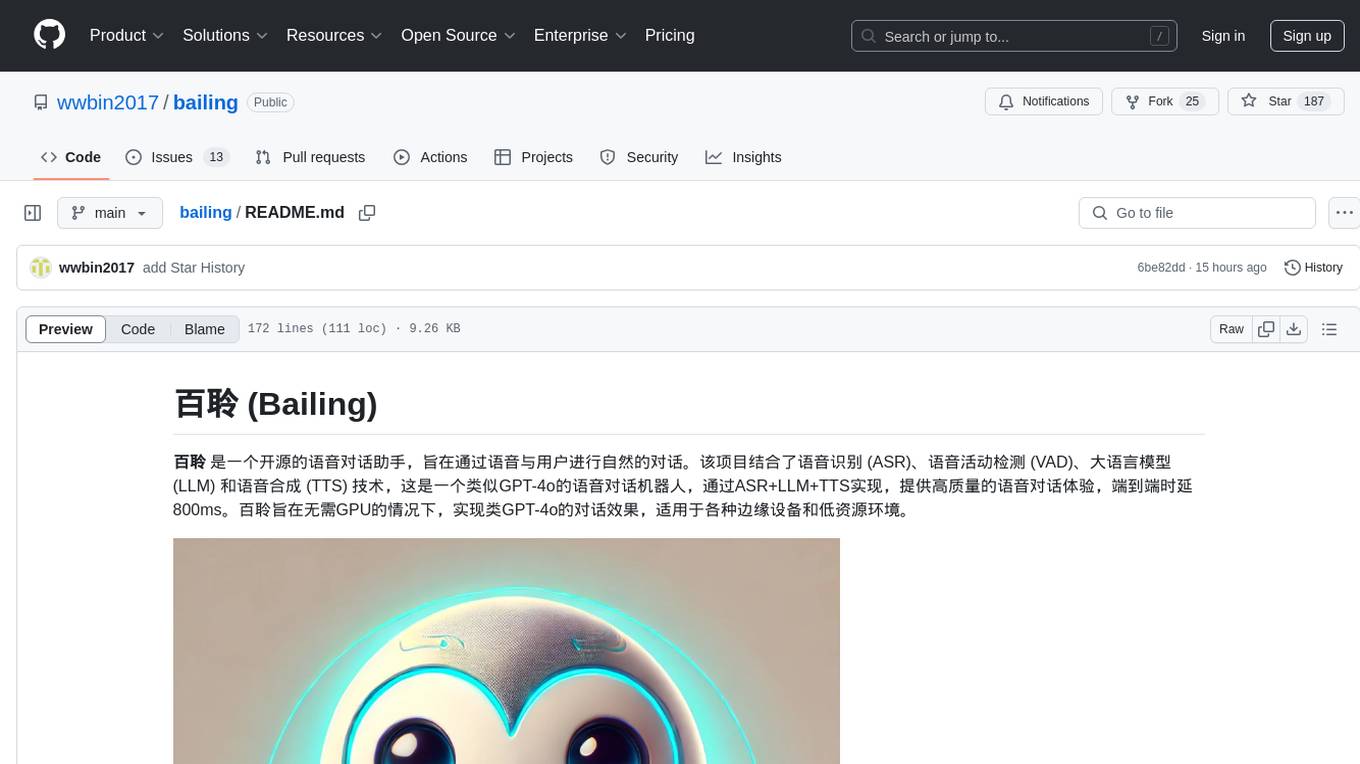
bailing
Bailing is an open-source voice assistant designed for natural conversations with users. It combines Automatic Speech Recognition (ASR), Voice Activity Detection (VAD), Large Language Model (LLM), and Text-to-Speech (TTS) technologies to provide a high-quality voice interaction experience similar to GPT-4o. Bailing aims to achieve GPT-4o-like conversation effects without the need for GPU, making it suitable for various edge devices and low-resource environments. The project features efficient open-source models, modular design allowing for module replacement and upgrades, support for memory function, tool integration for information retrieval and task execution via voice commands, and efficient task management with progress tracking and reminders.
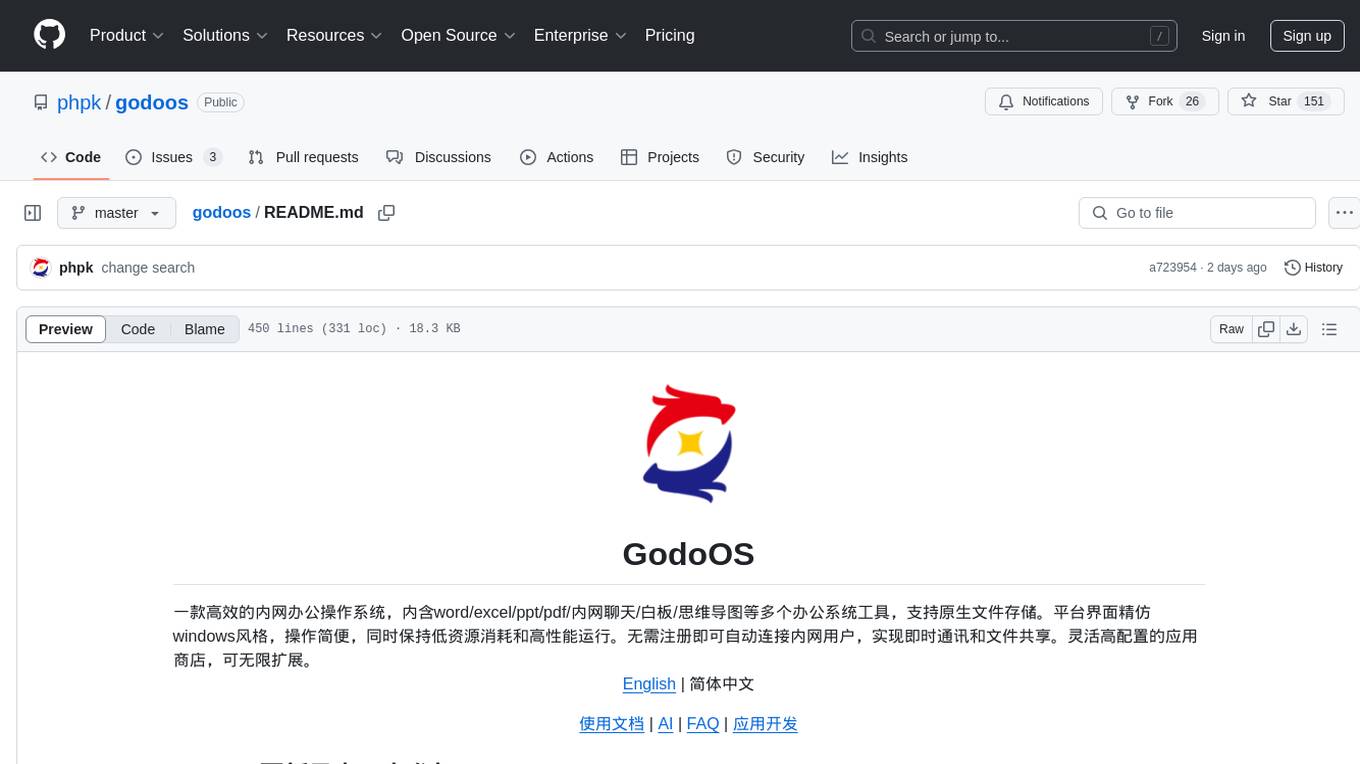
godoos
GodoOS is an efficient intranet office operating system that includes various office tools such as word/excel/ppt/pdf/internal chat/whiteboard/mind map, with native file storage support. The platform interface mimics the Windows style, making it easy to operate while maintaining low resource consumption and high performance. It automatically connects to intranet users without registration, enabling instant communication and file sharing. The flexible and highly configurable app store allows for unlimited expansion.
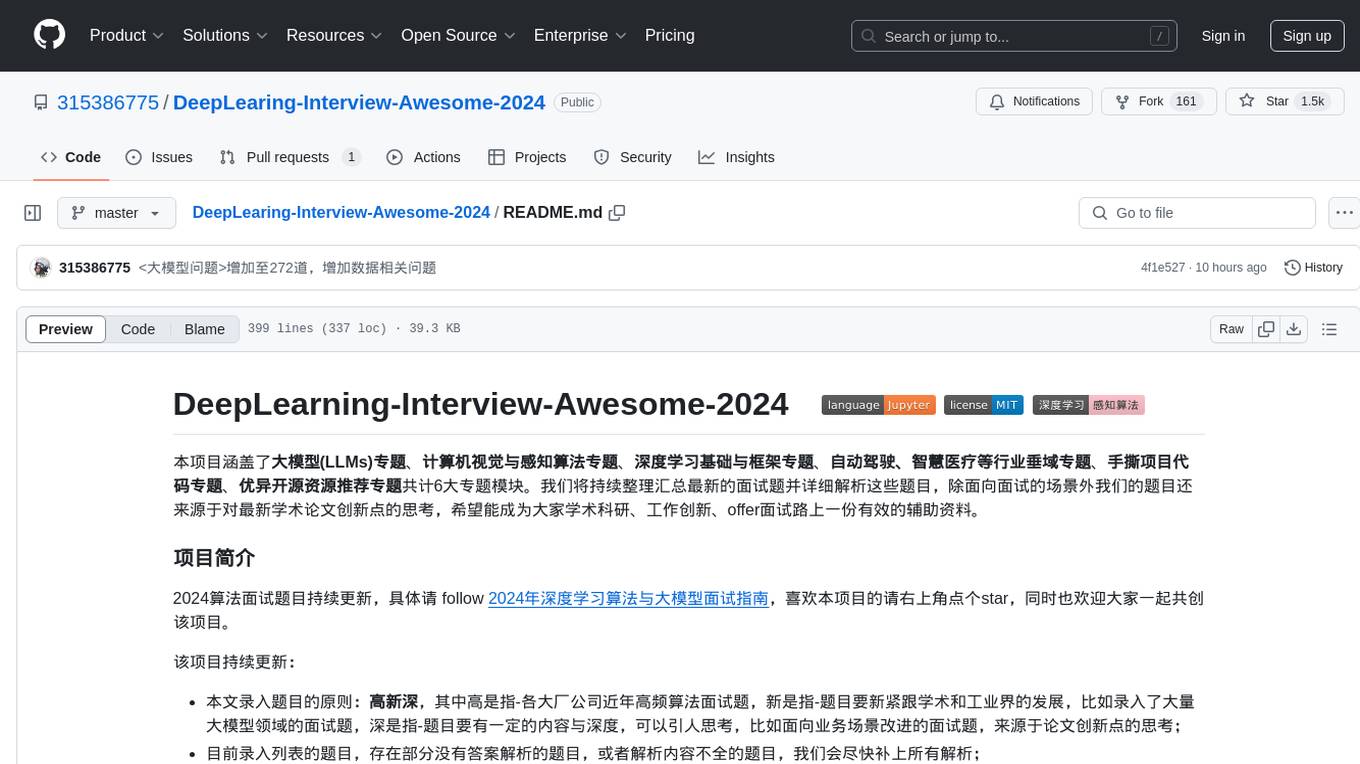
DeepLearing-Interview-Awesome-2024
DeepLearning-Interview-Awesome-2024 is a repository that covers various topics related to deep learning, computer vision, big models (LLMs), autonomous driving, smart healthcare, and more. It provides a collection of interview questions with detailed explanations sourced from recent academic papers and industry developments. The repository is aimed at assisting individuals in academic research, work innovation, and job interviews. It includes six major modules covering topics such as large language models (LLMs), computer vision models, common problems in computer vision and perception algorithms, deep learning basics and frameworks, as well as specific tasks like 3D object detection, medical image segmentation, and more.
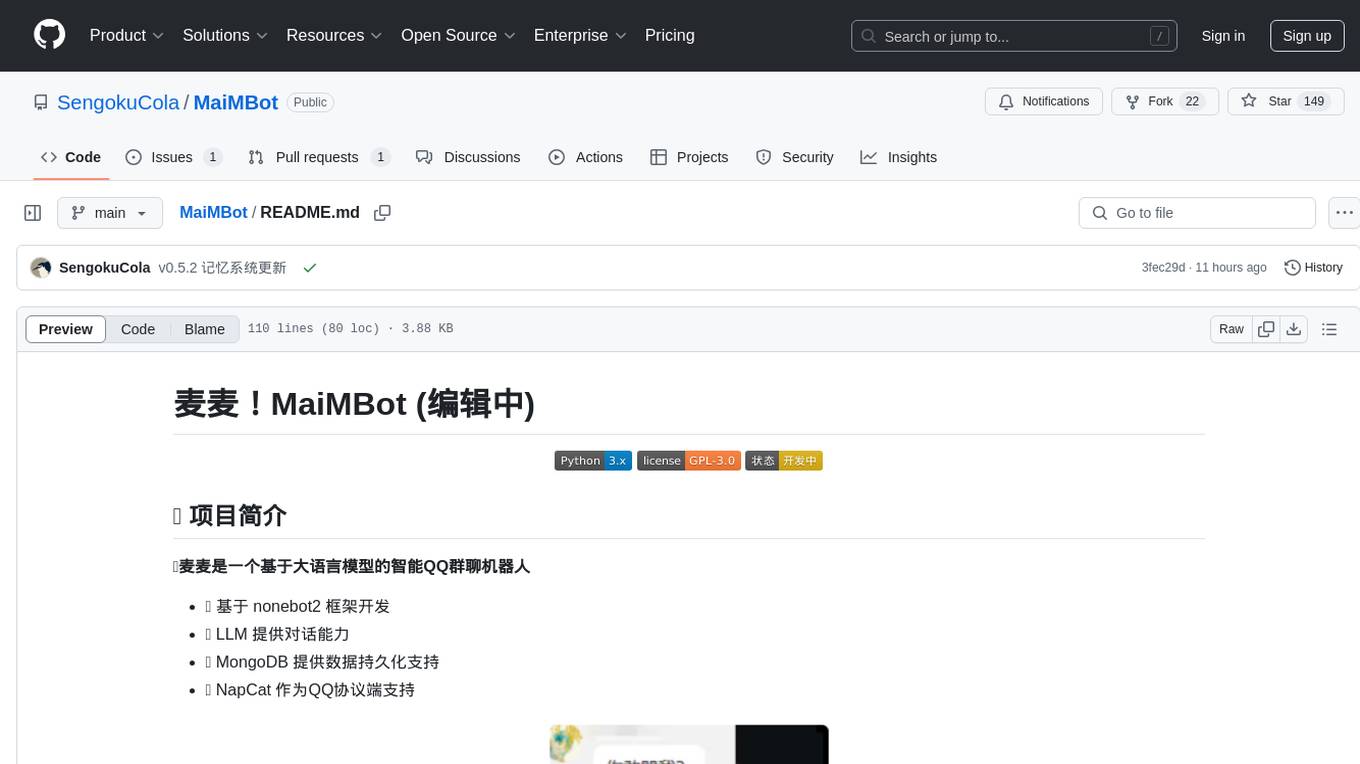
MaiMBot
MaiMBot is an intelligent QQ group chat bot based on a large language model. It is developed using the nonebot2 framework, utilizes LLM for conversation abilities, MongoDB for data persistence, and NapCat for QQ protocol support. The bot features keyword-triggered proactive responses, dynamic prompt construction, support for images and message forwarding, typo generation, multiple replies, emotion-based emoji responses, daily schedule generation, user relationship management, knowledge base, and group impressions. Work-in-progress features include personality, group atmosphere, image handling, humor, meme functions, and Minecraft interactions. The tool is in active development with plans for GIF compatibility, mini-program link parsing, bug fixes, documentation improvements, and logic enhancements for emoji sending.
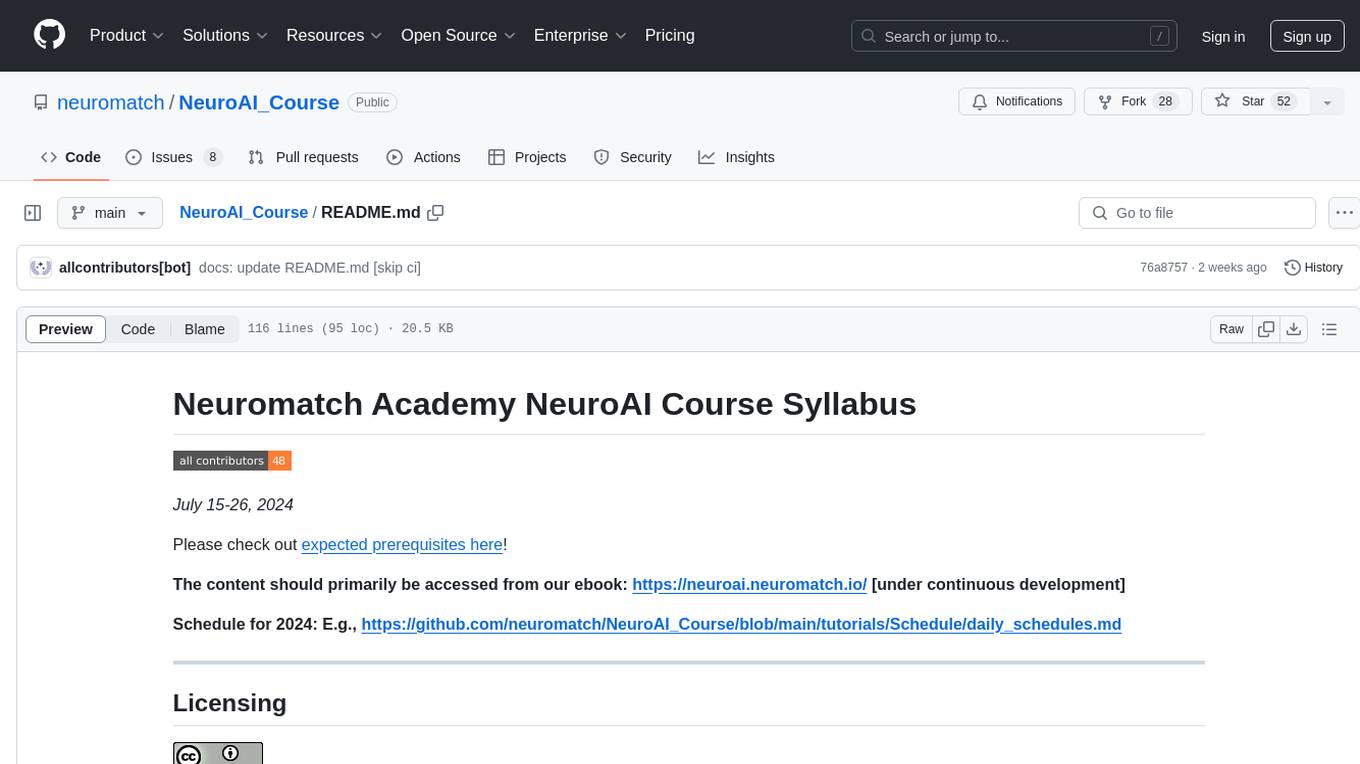
NeuroAI_Course
Neuromatch Academy NeuroAI Course Syllabus is a repository that contains the schedule and licensing information for the NeuroAI course. The course is designed to provide participants with a comprehensive understanding of artificial intelligence in neuroscience. It covers various topics related to AI applications in neuroscience, including machine learning, data analysis, and computational modeling. The content is primarily accessed from the ebook provided in the repository, and the course is scheduled for July 15-26, 2024. The repository is shared under a Creative Commons Attribution 4.0 International License and software elements are additionally licensed under the BSD (3-Clause) License. Contributors to the project are acknowledged and welcomed to contribute further.
For similar tasks
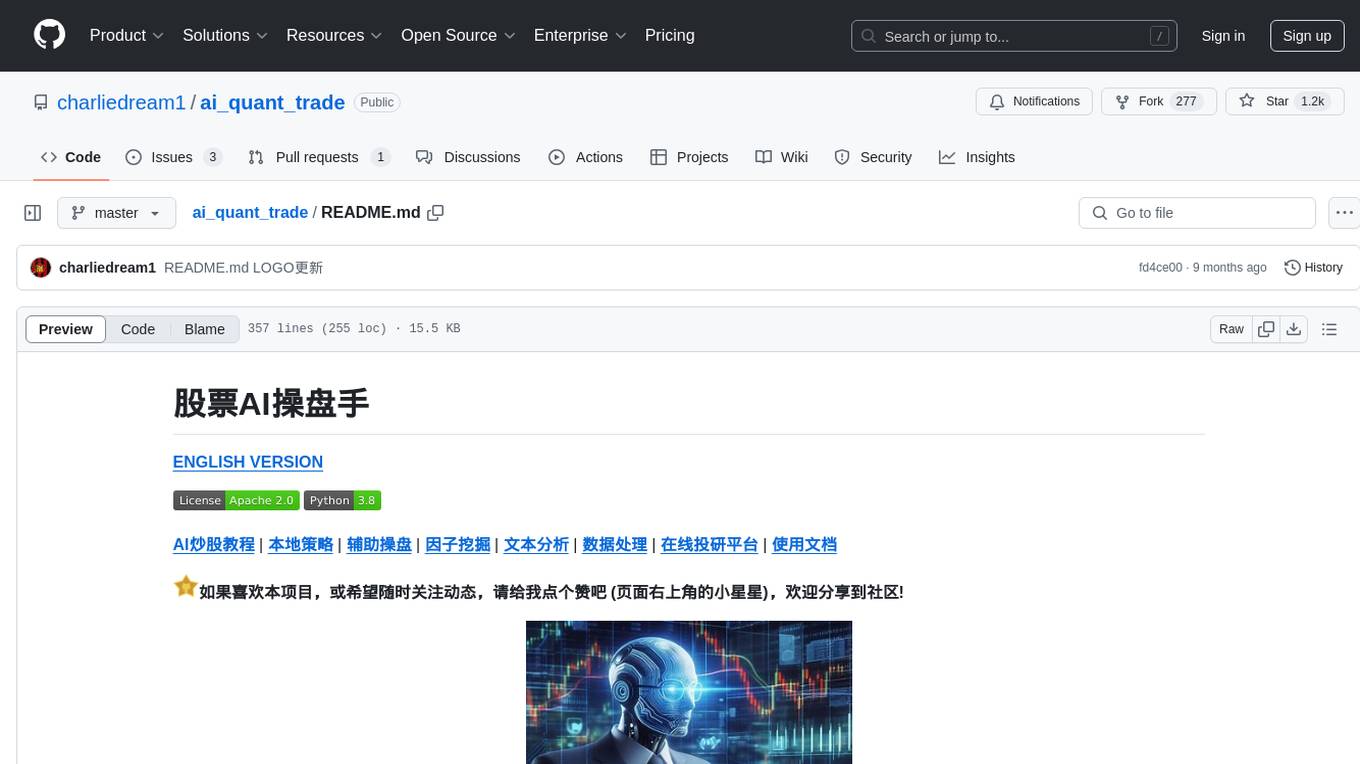
ai_quant_trade
The ai_quant_trade repository is a comprehensive platform for stock AI trading, offering learning, simulation, and live trading capabilities. It includes features such as factor mining, traditional strategies, machine learning, deep learning, reinforcement learning, graph networks, and high-frequency trading. The repository provides tools for monitoring stocks, stock recommendations, and deployment tools for live trading. It also features new functionalities like sentiment analysis using StructBERT, reinforcement learning for multi-stock trading with a 53% annual return, automatic factor mining with 5000 factors, customized stock monitoring software, and local deep reinforcement learning strategies.
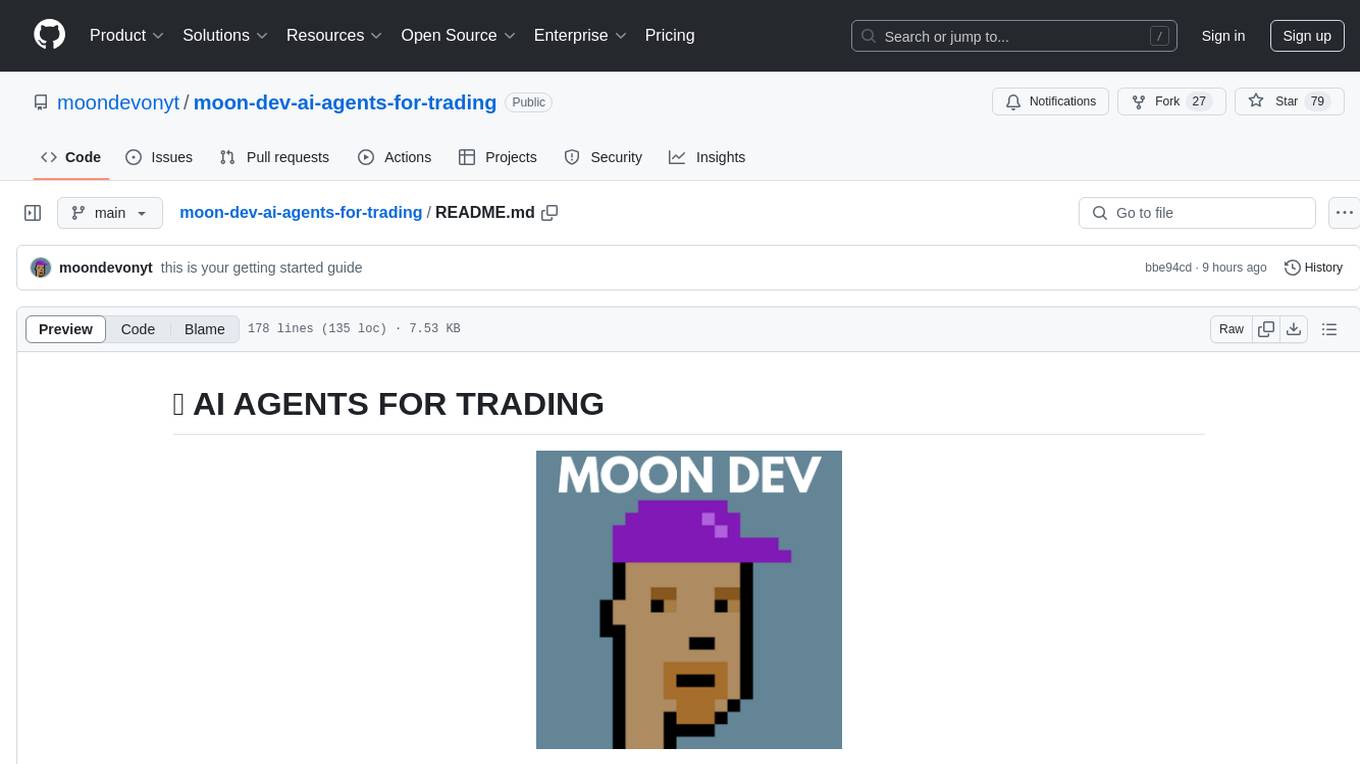
moon-dev-ai-agents-for-trading
Moon Dev AI Agents for Trading is an experimental project exploring the potential of artificial financial intelligence for trading and investing research. The project aims to develop AI agents to complement and potentially replace human trading operations by addressing common trading challenges such as emotional reactions, ego-driven decisions, inconsistent execution, fatigue effects, impatience, and fear & greed cycles. The project focuses on research areas like risk control, exit timing, entry strategies, sentiment collection, and strategy execution. It is important to note that this project is not a profitable trading solution and involves substantial risk of loss.
For similar jobs
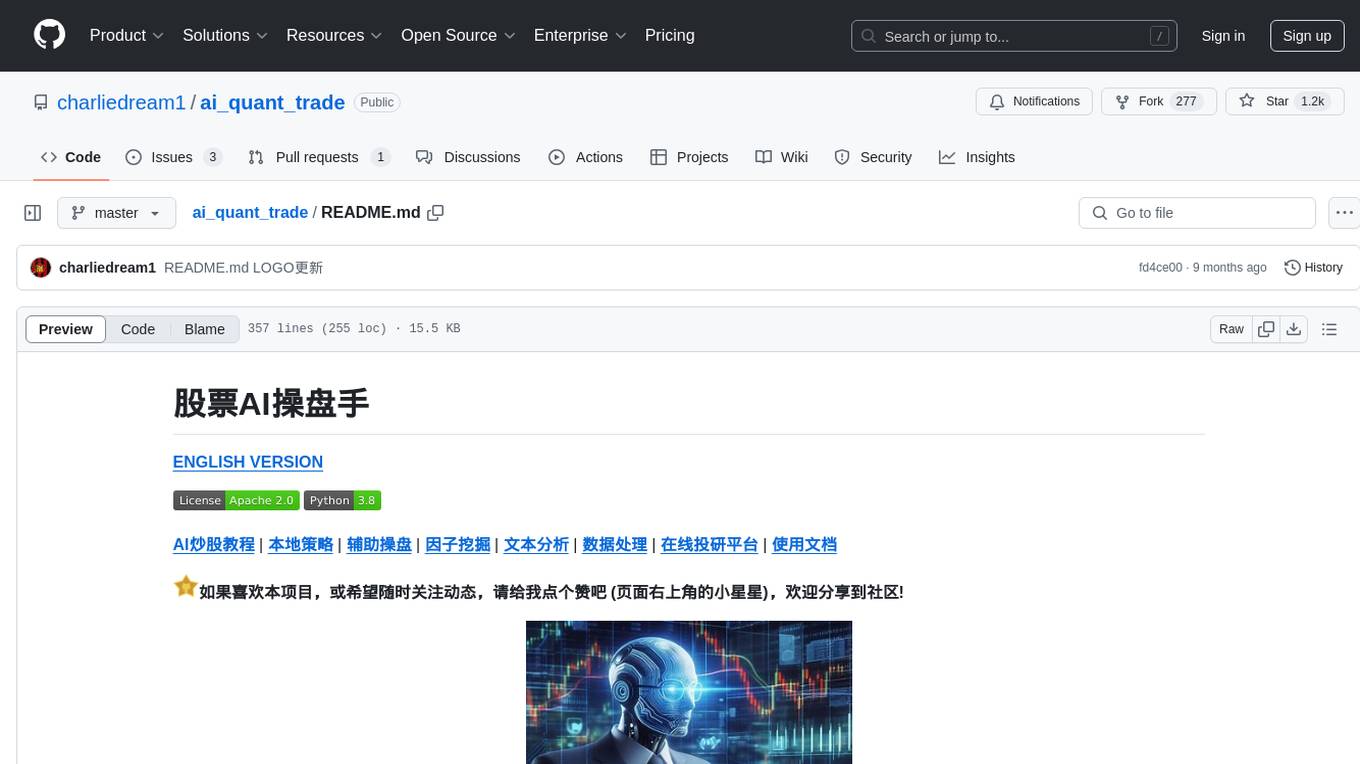
ai_quant_trade
The ai_quant_trade repository is a comprehensive platform for stock AI trading, offering learning, simulation, and live trading capabilities. It includes features such as factor mining, traditional strategies, machine learning, deep learning, reinforcement learning, graph networks, and high-frequency trading. The repository provides tools for monitoring stocks, stock recommendations, and deployment tools for live trading. It also features new functionalities like sentiment analysis using StructBERT, reinforcement learning for multi-stock trading with a 53% annual return, automatic factor mining with 5000 factors, customized stock monitoring software, and local deep reinforcement learning strategies.
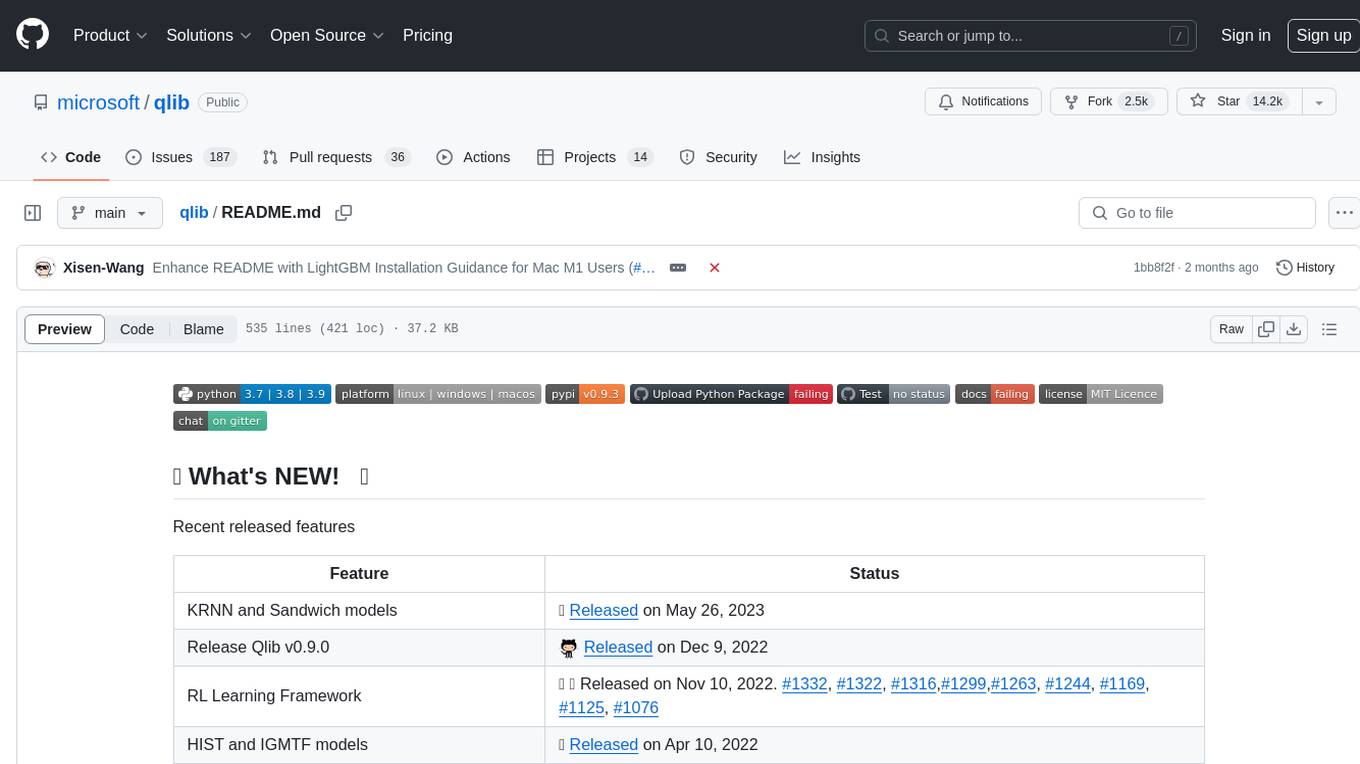
qlib
Qlib is an open-source, AI-oriented quantitative investment platform that supports diverse machine learning modeling paradigms, including supervised learning, market dynamics modeling, and reinforcement learning. It covers the entire chain of quantitative investment, from alpha seeking to order execution. The platform empowers researchers to explore ideas and implement productions using AI technologies in quantitative investment. Qlib collaboratively solves key challenges in quantitative investment by releasing state-of-the-art research works in various paradigms. It provides a full ML pipeline for data processing, model training, and back-testing, enabling users to perform tasks such as forecasting market patterns, adapting to market dynamics, and modeling continuous investment decisions.
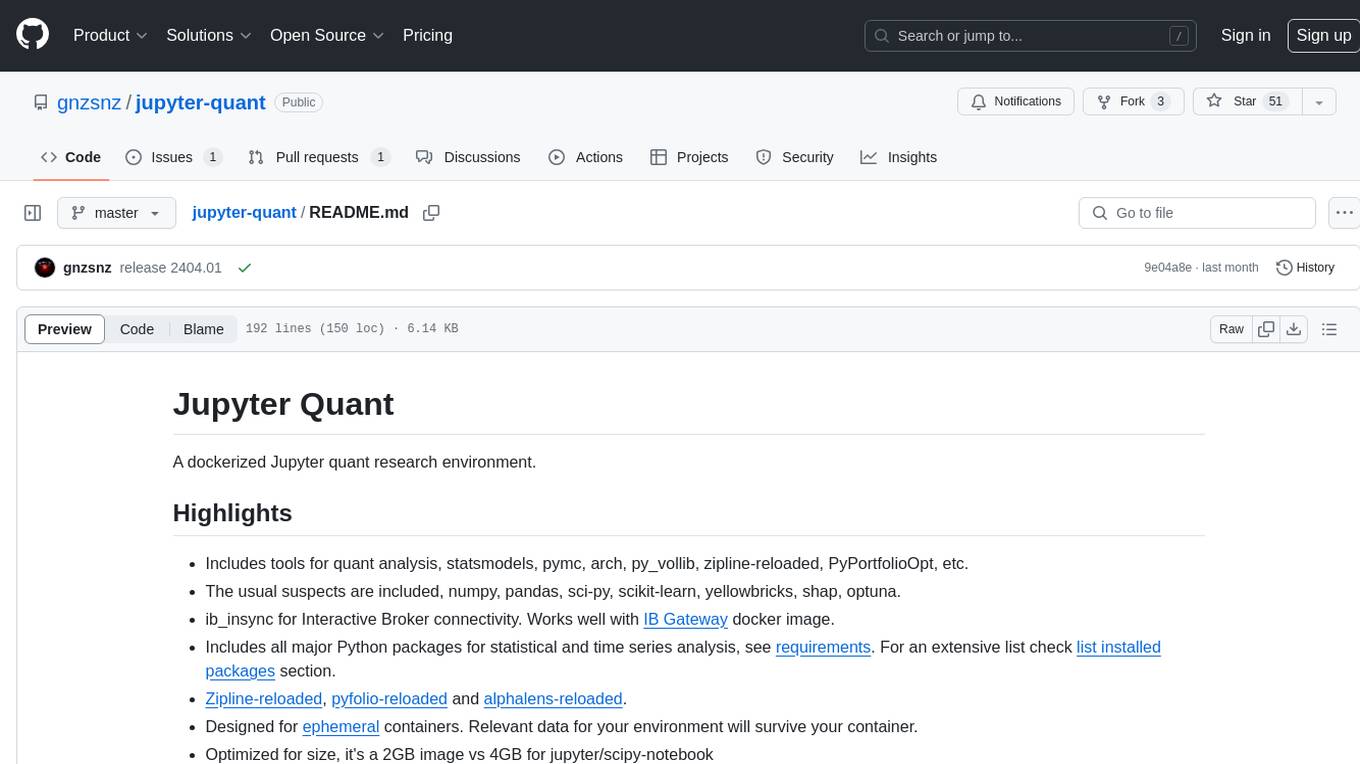
jupyter-quant
Jupyter Quant is a dockerized environment tailored for quantitative research, equipped with essential tools like statsmodels, pymc, arch, py_vollib, zipline-reloaded, PyPortfolioOpt, numpy, pandas, sci-py, scikit-learn, yellowbricks, shap, optuna, ib_insync, Cython, Numba, bottleneck, numexpr, jedi language server, jupyterlab-lsp, black, isort, and more. It does not include conda/mamba and relies on pip for package installation. The image is optimized for size, includes common command line utilities, supports apt cache, and allows for the installation of additional packages. It is designed for ephemeral containers, ensuring data persistence, and offers volumes for data, configuration, and notebooks. Common tasks include setting up the server, managing configurations, setting passwords, listing installed packages, passing parameters to jupyter-lab, running commands in the container, building wheels outside the container, installing dotfiles and SSH keys, and creating SSH tunnels.
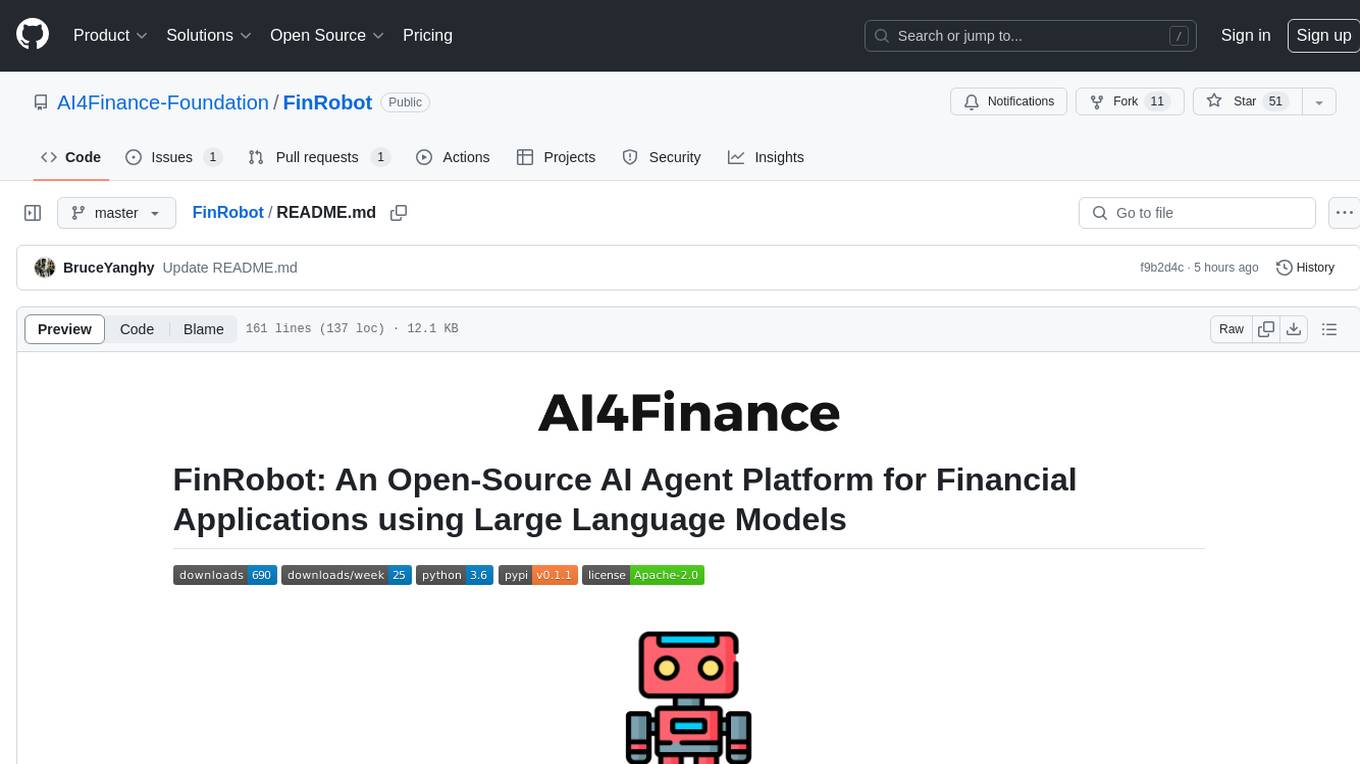
FinRobot
FinRobot is an open-source AI agent platform designed for financial applications using large language models. It transcends the scope of FinGPT, offering a comprehensive solution that integrates a diverse array of AI technologies. The platform's versatility and adaptability cater to the multifaceted needs of the financial industry. FinRobot's ecosystem is organized into four layers, including Financial AI Agents Layer, Financial LLMs Algorithms Layer, LLMOps and DataOps Layers, and Multi-source LLM Foundation Models Layer. The platform's agent workflow involves Perception, Brain, and Action modules to capture, process, and execute financial data and insights. The Smart Scheduler optimizes model diversity and selection for tasks, managed by components like Director Agent, Agent Registration, Agent Adaptor, and Task Manager. The tool provides a structured file organization with subfolders for agents, data sources, and functional modules, along with installation instructions and hands-on tutorials.
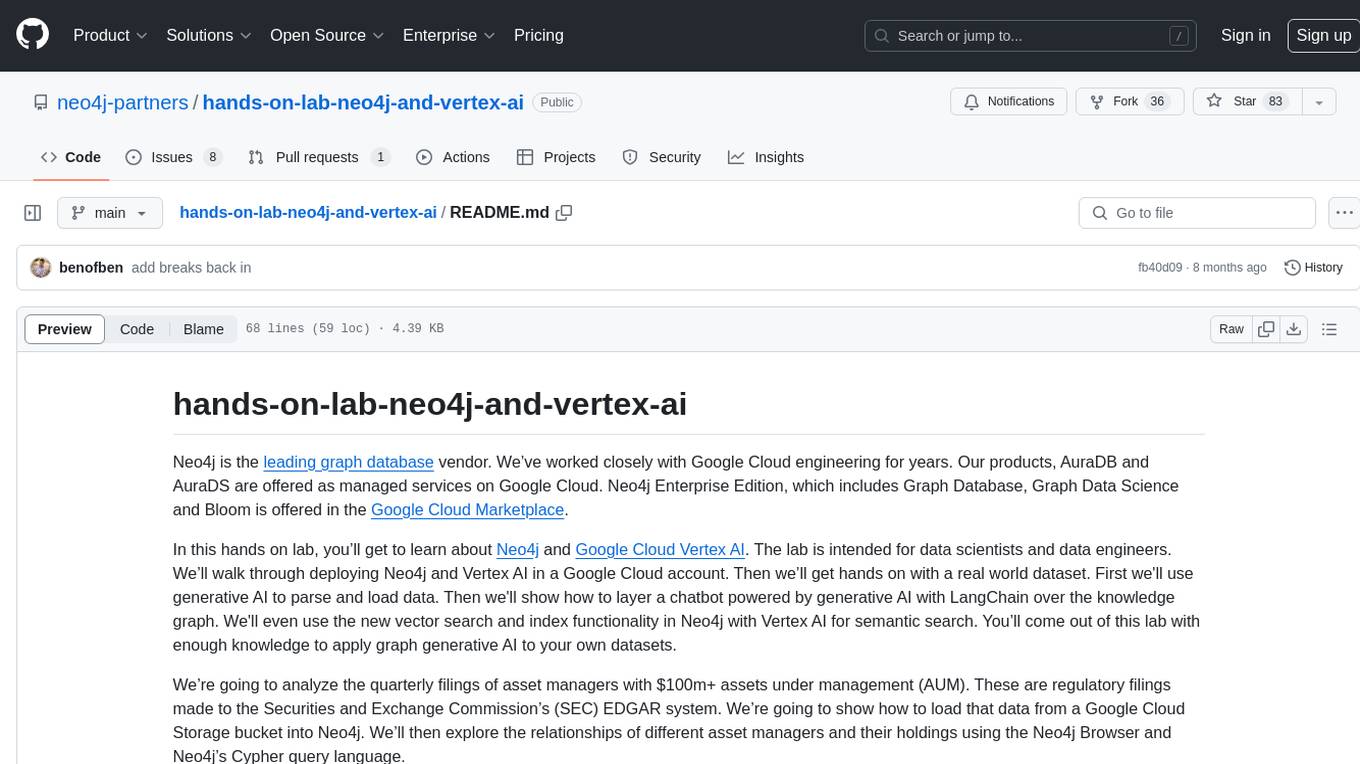
hands-on-lab-neo4j-and-vertex-ai
This repository provides a hands-on lab for learning about Neo4j and Google Cloud Vertex AI. It is intended for data scientists and data engineers to deploy Neo4j and Vertex AI in a Google Cloud account, work with real-world datasets, apply generative AI, build a chatbot over a knowledge graph, and use vector search and index functionality for semantic search. The lab focuses on analyzing quarterly filings of asset managers with $100m+ assets under management, exploring relationships using Neo4j Browser and Cypher query language, and discussing potential applications in capital markets such as algorithmic trading and securities master data management.
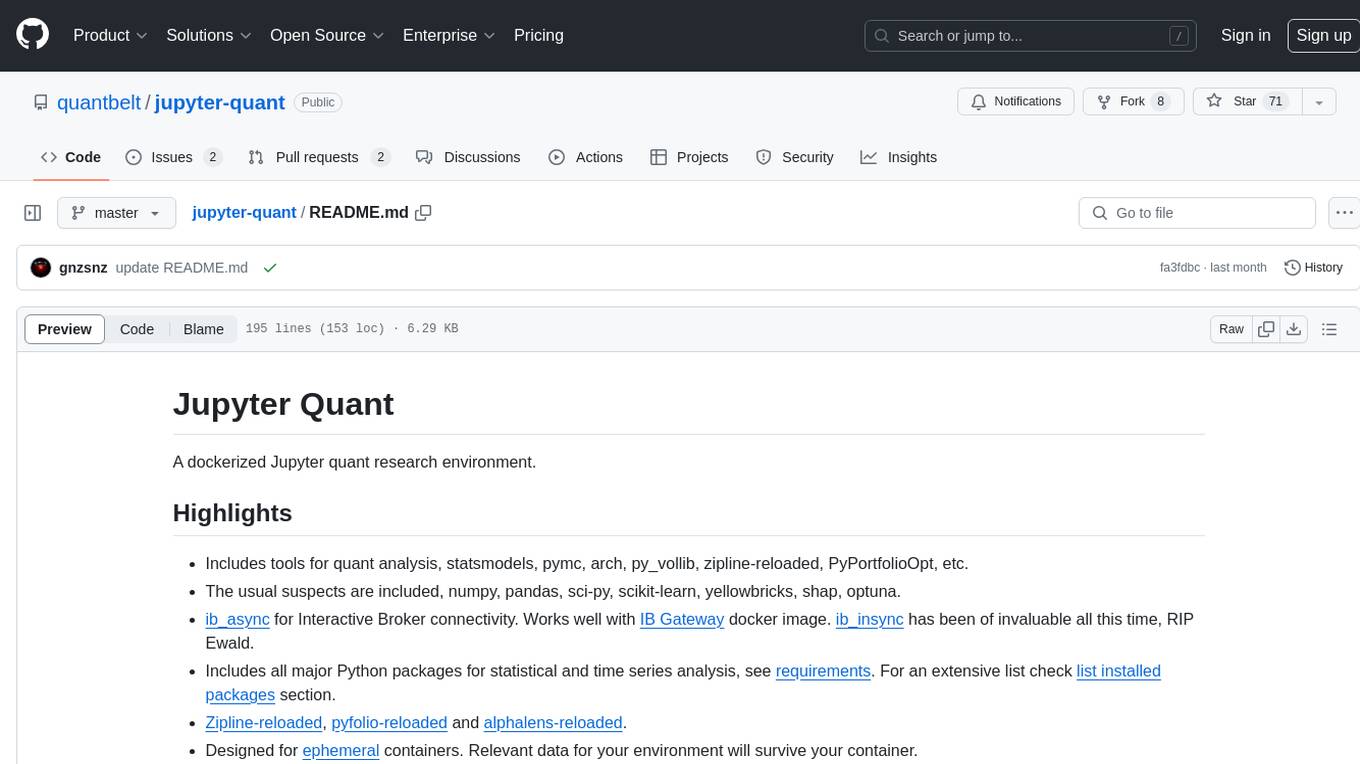
jupyter-quant
Jupyter Quant is a dockerized environment tailored for quantitative research, equipped with essential tools like statsmodels, pymc, arch, py_vollib, zipline-reloaded, PyPortfolioOpt, numpy, pandas, sci-py, scikit-learn, yellowbricks, shap, optuna, and more. It provides Interactive Broker connectivity via ib_async and includes major Python packages for statistical and time series analysis. The image is optimized for size, includes jedi language server, jupyterlab-lsp, and common command line utilities. Users can install new packages with sudo, leverage apt cache, and bring their own dot files and SSH keys. The tool is designed for ephemeral containers, ensuring data persistence and flexibility for quantitative analysis tasks.
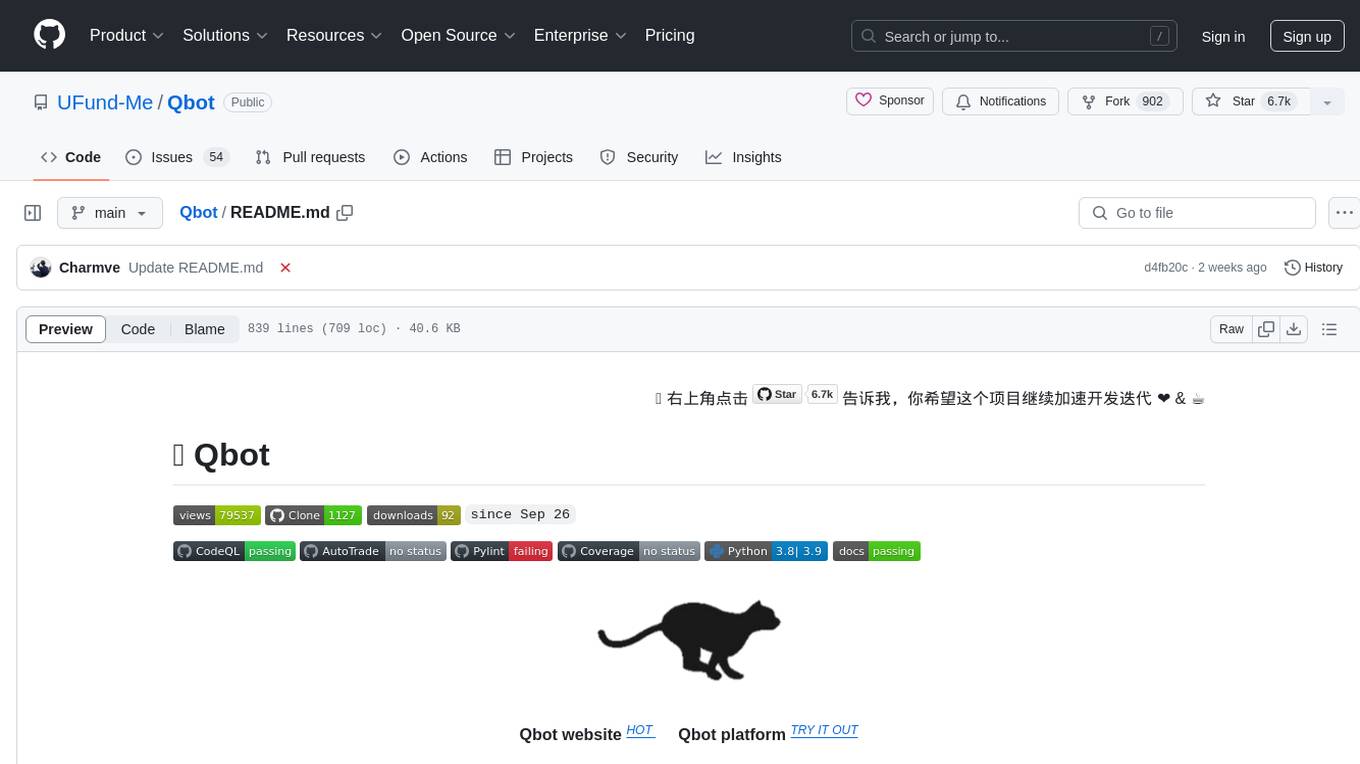
Qbot
Qbot is an AI-oriented automated quantitative investment platform that supports diverse machine learning modeling paradigms, including supervised learning, market dynamics modeling, and reinforcement learning. It provides a full closed-loop process from data acquisition, strategy development, backtesting, simulation trading to live trading. The platform emphasizes AI strategies such as machine learning, reinforcement learning, and deep learning, combined with multi-factor models to enhance returns. Users with some Python knowledge and trading experience can easily utilize the platform to address trading pain points and gaps in the market.
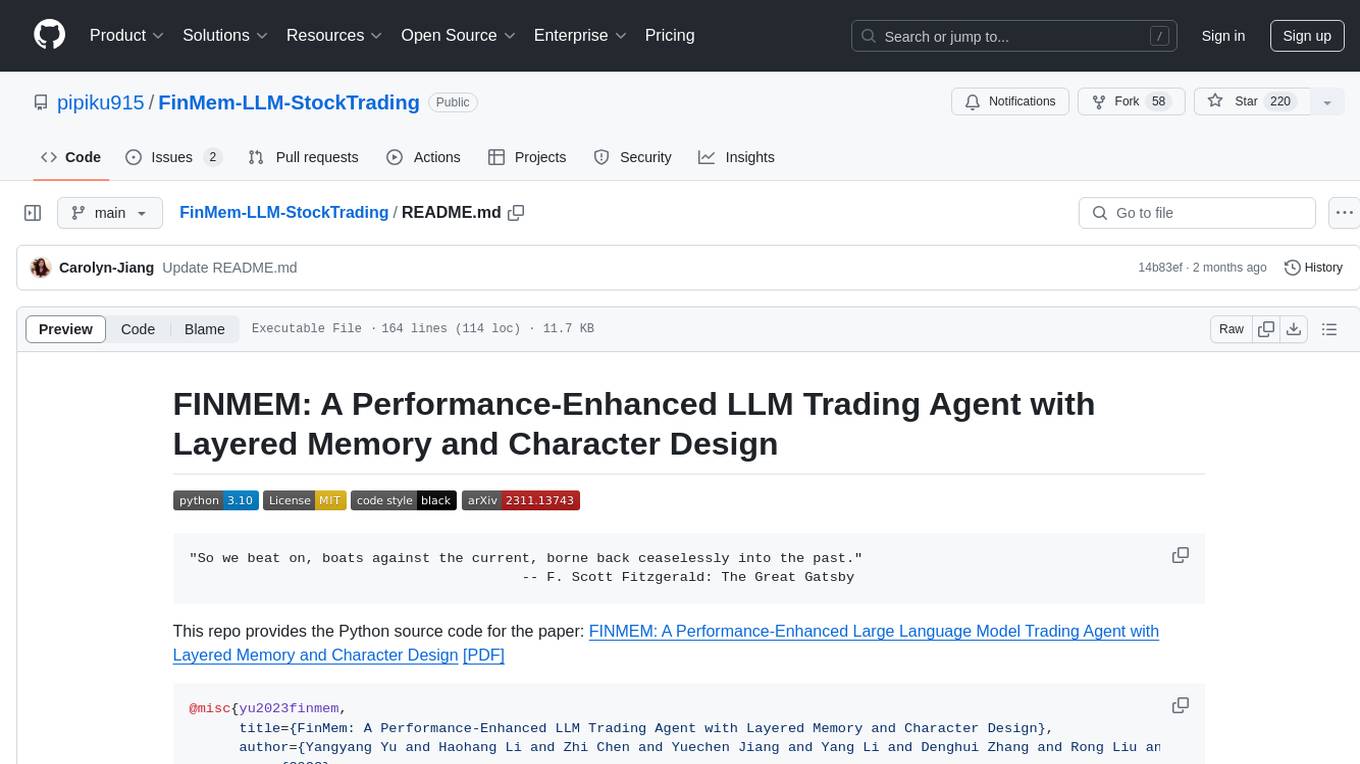
FinMem-LLM-StockTrading
This repository contains the Python source code for FINMEM, a Performance-Enhanced Large Language Model Trading Agent with Layered Memory and Character Design. It introduces FinMem, a novel LLM-based agent framework devised for financial decision-making, encompassing three core modules: Profiling, Memory with layered processing, and Decision-making. FinMem's memory module aligns closely with the cognitive structure of human traders, offering robust interpretability and real-time tuning. The framework enables the agent to self-evolve its professional knowledge, react agilely to new investment cues, and continuously refine trading decisions in the volatile financial environment. It presents a cutting-edge LLM agent framework for automated trading, boosting cumulative investment returns.