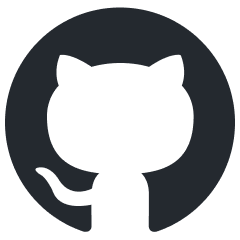
VideoTuna
Let's finetune video generation models!
Stars: 428
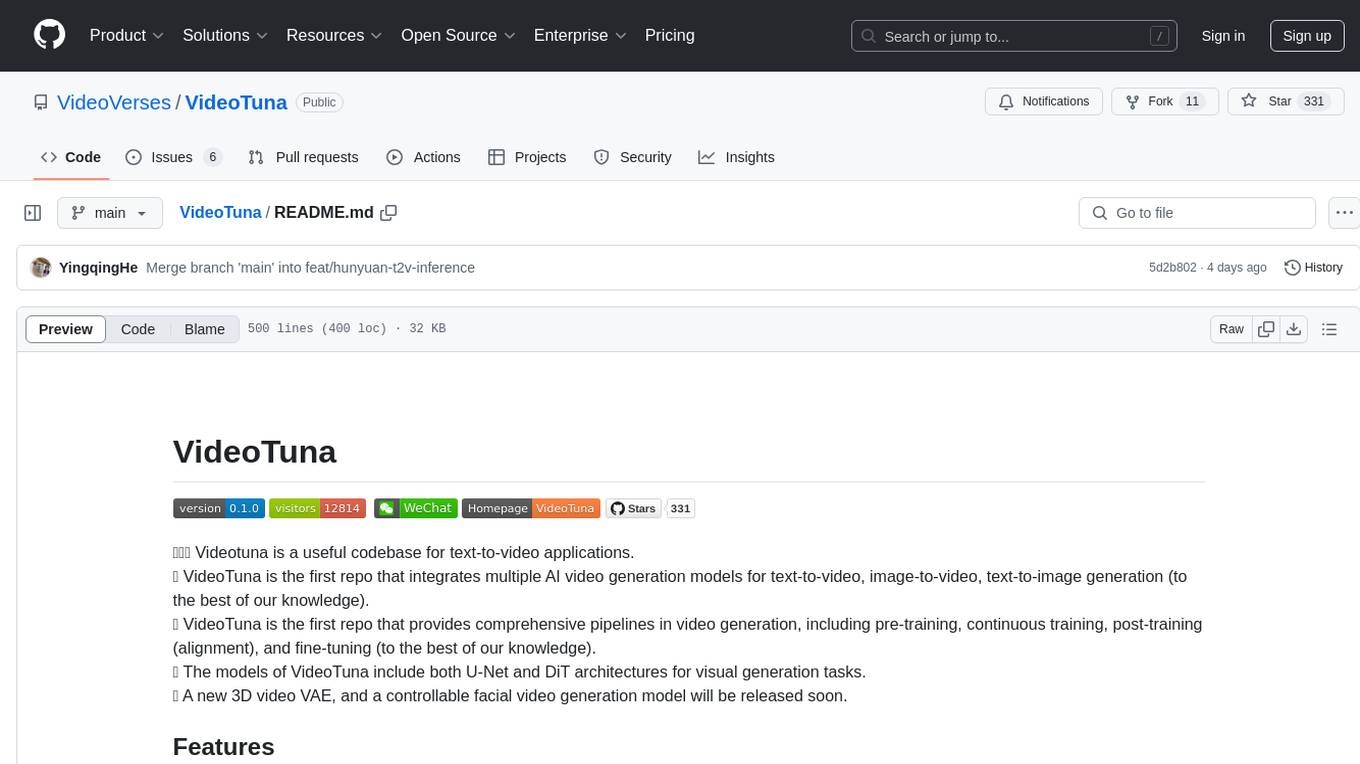
VideoTuna is a codebase for text-to-video applications that integrates multiple AI video generation models for text-to-video, image-to-video, and text-to-image generation. It provides comprehensive pipelines in video generation, including pre-training, continuous training, post-training, and fine-tuning. The models in VideoTuna include U-Net and DiT architectures for visual generation tasks, with upcoming releases of a new 3D video VAE and a controllable facial video generation model.
README:
π€π€π€ Videotuna is a useful codebase for text-to-video applications.
π VideoTuna is the first repo that integrates multiple AI video generation models including text-to-video (T2V)
, image-to-video (I2V)
, text-to-image (T2I)
, and video-to-video (V2V)
generation for model inference and finetuning (to the best of our knowledge).
π VideoTuna is the first repo that provides comprehensive pipelines in video generation, from fine-tuning to pre-training, continuous training, and post-training (alignment) (to the best of our knowledge).
π An Emotion Control I2V model will be released soon.
π All-in-one framework: Inference and fine-tune up-to-date video generation models. π Pre-training: Build your own foundational text-to-video model. π Continuous training: Keep improving your model with new data. π Domain-specific fine-tuning: Adapt models to your specific scenario. π Concept-specific fine-tuning: Teach your models with unique concepts. π Enhanced language understanding: Improve model comprehension through continuous training. π Post-processing: Enhance the videos with video-to-video enhancement model. π Post-training/Human preference alignment: Post-training with RLHF for more attractive results.
- [2025-02-03] π We update automatic code formatting from PR#27. Thanks samidarko!
- [2025-02-01] π We update Poetry migration for better dependency management and script automation from PR#25. Thanks samidarko!
- [2025-01-20] π We update the fine-tuning of
Flux-T2I
. Thanks VideoTuna team! - [2025-01-01] π We update the training of
VideoVAE+
in this repo. Thanks VideoTuna team! - [2025-01-01] π We update the inference of
Hunyuan Video
andMochi
. Thanks VideoTuna team! - [2024-12-24] π We release a SOTA Video VAE model
VideoVAE+
in this repo! Better video reconstruction than Nvidia'sCosmos-Tokenizer
. Thanks VideoTuna team! - [2024-12-01] π We update the inference of
CogVideoX-1.5-T2V&I2V
,Video-to-Video Enhancement
from ModelScope, and fine-tuning ofCogVideoX-1
. Thanks VideoTuna team! - [2024-11-01] π We make the VideoTuna V0.1.0 public! It supports inference of
VideoCrafter1-T2V&I2V
,VideoCrafter2-T2V
,DynamiCrafter-I2V
,OpenSora-T2V
,CogVideoX-1-2B-T2V
,CogVideoX-1-T2V
,Flux-T2I
, as well as training and finetuning of part of these models. Thanks VideoTuna team!
Video VAE+ can accurately compress and reconstruct the input videos with fine details.
Ground Truth | Reconstruction |
Input 1 | Input 2 |
Emotion: Anger | Emotion: Disgust | Emotion: Fear |
Emotion: Happy | Emotion: Sad | Emotion: Surprise |
Emotion: Anger | Emotion: Disgust | Emotion: Fear |
Emotion: Happy | Emotion: Sad | Emotion: Surprise |
T2V-Models | HxWxL | Checkpoints |
---|---|---|
HunyuanVideo | 720x1280x129 | Hugging Face |
Mochi | 848x480, 3s | Hugging Face |
CogVideoX-2B | 480x720x49 | Hugging Face |
CogVideoX-5B | 480x720x49 | Hugging Face |
Open-Sora 1.0 | 512Γ512x16 | Hugging Face |
Open-Sora 1.0 | 256Γ256x16 | Hugging Face |
Open-Sora 1.0 | 256Γ256x16 | Hugging Face |
VideoCrafter2 | 320x512x16 | Hugging Face |
VideoCrafter1 | 576x1024x16 | Hugging Face |
VideoCrafter1 | 320x512x16 | Hugging Face |
I2V-Models | HxWxL | Checkpoints |
---|---|---|
CogVideoX-5B-I2V | 480x720x49 | Hugging Face |
DynamiCrafter | 576x1024x16 | Hugging Face |
VideoCrafter1 | 320x512x16 | Hugging Face |
- Note: H: height; W: width; L: length
Please check docs/CHECKPOINTS.md to download all the model checkpoints.
conda create -n videotuna python=3.10 -y
conda activate videotuna
pip install poetry
poetry install
poetry run pip install "modelscope[cv]" -f https://modelscope.oss-cn-beijing.aliyuncs.com/releases/repo.html
Flash-attn installation (Optional)
Hunyuan model uses it to reduce memory usage and speed up inference. If it is not installed, the model will run in normal mode. Install the flash-attn
via:
poetry run install-flash-attn
Install Poetry: https://python-poetry.org/docs/#installation
Then:
poetry config virtualenvs.in-project true # optional but recommended, will ensure the virtual env is created in the project root
poetry config virtualenvs.create true # enable this argument to ensure the virtual env is created in the project root
poetry env use python3.10 # will create the virtual env, check with `ls -l .venv`.
poetry env activate # optional because Poetry commands (e.g. `poetry install` or `poetry run <command>`) will always automatically load the virtual env.
poetry install
poetry run pip install "modelscope[cv]" -f https://modelscope.oss-cn-beijing.aliyuncs.com/releases/repo.html
Flash-attn installation (Optional)
Hunyuan model uses it to reduce memory usage and speed up inference. If it is not installed, the model will run in normal mode. Install the flash-attn
via:
poetry run install-flash-attn
On MacOS with Apple Silicon chip use docker compose because some dependencies are not supporting arm64 (e.g. bitsandbytes
, decord
, xformers
).
First build:
docker compose build videotuna
To preserve the project's files permissions set those env variables:
export HOST_UID=$(id -u)
export HOST_GID=$(id -g)
Install dependencies:
docker compose run --remove-orphans videotuna poetry env use /usr/local/bin/python
docker compose run --remove-orphans videotuna poetry run python -m pip install --upgrade pip setuptools wheel
docker compose run --remove-orphans videotuna poetry install
docker compose run --remove-orphans videotuna poetry run pip install "modelscope[cv]" -f https://modelscope.oss-cn-beijing.aliyuncs.com/releases/repo.html
Note: installing swissarmytransformer might hang. Just try again and it should work.
Add a dependency:
docker compose run --remove-orphans videotuna poetry add wheel
Check dependencies:
docker compose run --remove-orphans videotuna poetry run pip freeze
Run Poetry commands:
docker compose run --remove-orphans videotuna poetry run format
Start a terminal:
docker compose run -it --remove-orphans videotuna bash
Please follow docs/CHECKPOINTS.md to download model checkpoints. After downloading, the model checkpoints should be placed as Checkpoint Structure.
- Inference a set of text-to-video models in one command:
bash tools/video_comparison/compare.sh
- The default mode is to run all models, e.g.,
inference_methods="videocrafter2;dynamicrafter;cogvideoβt2v;cogvideoβi2v;opensora"
- If the users want to inference specific models, modify the
inference_methods
variable incompare.sh
, and list the desired models separated by semicolons. - Also specify the input directory via the
input_dir
variable. This directory should contain aprompts.txt
file, where each line corresponds to a prompt for the video generation. The defaultinput_dir
isinputs/t2v
- The default mode is to run all models, e.g.,
- Inference a set of image-to-video models in one command:
bash tools/video_comparison/compare_i2v.sh
- Inference a specific model, run the corresponding commands as follows:
Task | Model | Command | Length (#frames) | Resolution | Inference Time (s) | GPU Memory (GiB) |
---|---|---|---|---|---|---|
T2V | HunyuanVideo | poetry run inference-hunyuan |
129 | 720x1280 | 1920 | 59.15 |
T2V | Mochi | poetry run inference-mochi |
84 | 480x848 | 109.0 | 26 |
I2V | CogVideoX-5b-I2V | poetry run inference-cogvideox-15-5b-i2v |
49 | 480x720 | 310.4 | 4.78 |
T2V | CogVideoX-2b | poetry run inference-cogvideo-t2v-diffusers |
49 | 480x720 | 107.6 | 2.32 |
T2V | Open Sora V1.0 | poetry run inference-opensora-v10-16x256x256 |
16 | 256x256 | 11.2 | 23.99 |
T2V | VideoCrafter-V2-320x512 | poetry run inference-vc2-t2v-320x512 |
16 | 320x512 | 26.4 | 10.03 |
T2V | VideoCrafter-V1-576x1024 | poetry run inference-vc1-t2v-576x1024 |
16 | 576x1024 | 91.4 | 14.57 |
I2V | DynamiCrafter | poetry run inference-dc-i2v-576x1024 |
16 | 576x1024 | 101.7 | 52.23 |
I2V | VideoCrafter-V1 | poetry run inference-vc1-i2v-320x512 |
16 | 320x512 | 26.4 | 10.03 |
T2I | Flux-dev | poetry run inference-flux-dev |
1 | 768x1360 | 238.1 | 1.18 |
T2I | Flux-schnell | poetry run inference-flux-schnell |
1 | 768x1360 | 5.4 | 1.20 |
Flux-dev: Trained using guidance distillation, it requires 40 to 50 steps to generate high-quality images.
Flux-schnell: Trained using latent adversarial diffusion distillation, it can generate high-quality images in only 1 to 4 steps.
Please follow the docs/datasets.md to try provided toydataset or build your own datasets.
Before started, we assume you have finished the following two preliminary steps:
- Install the environment
- Prepare the dataset
- Download the checkpoints and get these two checkpoints
ll checkpoints/videocrafter/t2v_v2_512/model.ckpt
ll checkpoints/stablediffusion/v2-1_512-ema/model.ckpt
First, run this command to convert the VC2 checkpoint as we make minor modifications on the keys of the state dict of the checkpoint. The converted checkpoint will be automatically save at checkpoints/videocrafter/t2v_v2_512/model_converted.ckpt
.
python tools/convert_checkpoint.py --input_path checkpoints/videocrafter/t2v_v2_512/model.ckpt
Second, run this command to start training on the single GPU. The training results will be automatically saved at results/train/${CURRENT_TIME}_${EXPNAME}
poetry run train-videocrafter-v2
We support lora finetuning to make the model to learn new concepts/characters/styles.
- Example config file:
configs/001_videocrafter2/vc2_t2v_lora.yaml
- Training lora based on VideoCrafter2:
bash shscripts/train_videocrafter_lora.sh
- Inference the trained models:
bash shscripts/inference_vc2_t2v_320x512_lora.sh
We support open-sora finetuning, you can simply run the following commands:
# finetune the Open-Sora v1.0
poetry run train-opensorav10
We support flux lora finetuning, you can simply run the following commands:
# finetune the Flux-Lora
poetry run train-flux-lora
# inference the lora model
poetry run inference-flux-lora
If you want to build your own dataset, please organize your data as inputs/t2i/flux/plushie_teddybear
, which contains the training images and the corresponding text prompt files, as shown in the following directory structure. Then modify the instance_data_dir
inconfigs/006_flux/multidatabackend.json
.
owndata/
βββ img1.jpg
βββ img2.jpg
βββ img3.jpg
βββ ...
βββ prompt1.txt # prompt of img1.jpg
βββ prompt2.txt # prompt of img2.jpg
βββ prompt3.txt # prompt of img3.jpg
βββ ...
We support VBench evaluation to evaluate the T2V generation performance. Please check eval/README.md for details.
Git hooks are handled with pre-commit library.
Run the following command to install hooks on commit
. They will check formatting, linting and types.
poetry run pre-commit install
poetry run pre-commit install --hook-type commit-msg
poetry run pre-commit run --all-files
We thank the following repos for sharing their awesome models and codes!
- Mochi: A new SOTA in open-source video generation models
- VideoCrafter2: Overcoming Data Limitations for High-Quality Video Diffusion Models
- VideoCrafter1: Open Diffusion Models for High-Quality Video Generation
- DynamiCrafter: Animating Open-domain Images with Video Diffusion Priors
- Open-Sora: Democratizing Efficient Video Production for All
- CogVideoX: Text-to-Video Diffusion Models with An Expert Transformer
- VADER: Video Diffusion Alignment via Reward Gradients
- VBench: Comprehensive Benchmark Suite for Video Generative Models
- Flux: Text-to-image models from Black Forest Labs.
- SimpleTuner: A fine-tuning kit for text-to-image generation.
- LLMs-Meet-MM-Generation: A paper collection of utilizing LLMs for multimodal generation (image, video, 3D and audio).
- MMTrail: A multimodal trailer video dataset with language and music descriptions.
- Seeing-and-Hearing: A versatile framework for Joint VA generation, V2A, A2V, and I2A.
- Self-Cascade: A Self-Cascade model for higher-resolution image and video generation.
- ScaleCrafter and HiPrompt: Free method for higher-resolution image and video generation.
- FreeTraj and FreeNoise: Free method for video trajectory control and longer-video generation.
- Follow-Your-Emoji, Follow-Your-Click, and Follow-Your-Pose: Follow family for controllable video generation.
- Animate-A-Story: A framework for storytelling video generation.
- LVDM: Latent Video Diffusion Model for long video generation and text-to-video generation.
Please follow CC-BY-NC-ND. If you want a license authorization, please contact the project leads Yingqing He ([email protected]) and Yazhou Xing ([email protected]).
@software{videotuna,
author = {Yingqing He and Yazhou Xing and Zhefan Rao and Haoyu Wu and Zhaoyang Liu and Jingye Chen and Pengjun Fang and Jiajun Li and Liya Ji and Runtao Liu and Xiaowei Chi and Yang Fei and Guocheng Shao and Yue Ma and Qifeng Chen},
title = {VideoTuna: A Powerful Toolkit for Video Generation with Model Fine-Tuning and Post-Training},
month = {Nov},
year = {2024},
url = {https://github.com/VideoVerses/VideoTuna}
}
For Tasks:
Click tags to check more tools for each tasksFor Jobs:
Alternative AI tools for VideoTuna
Similar Open Source Tools
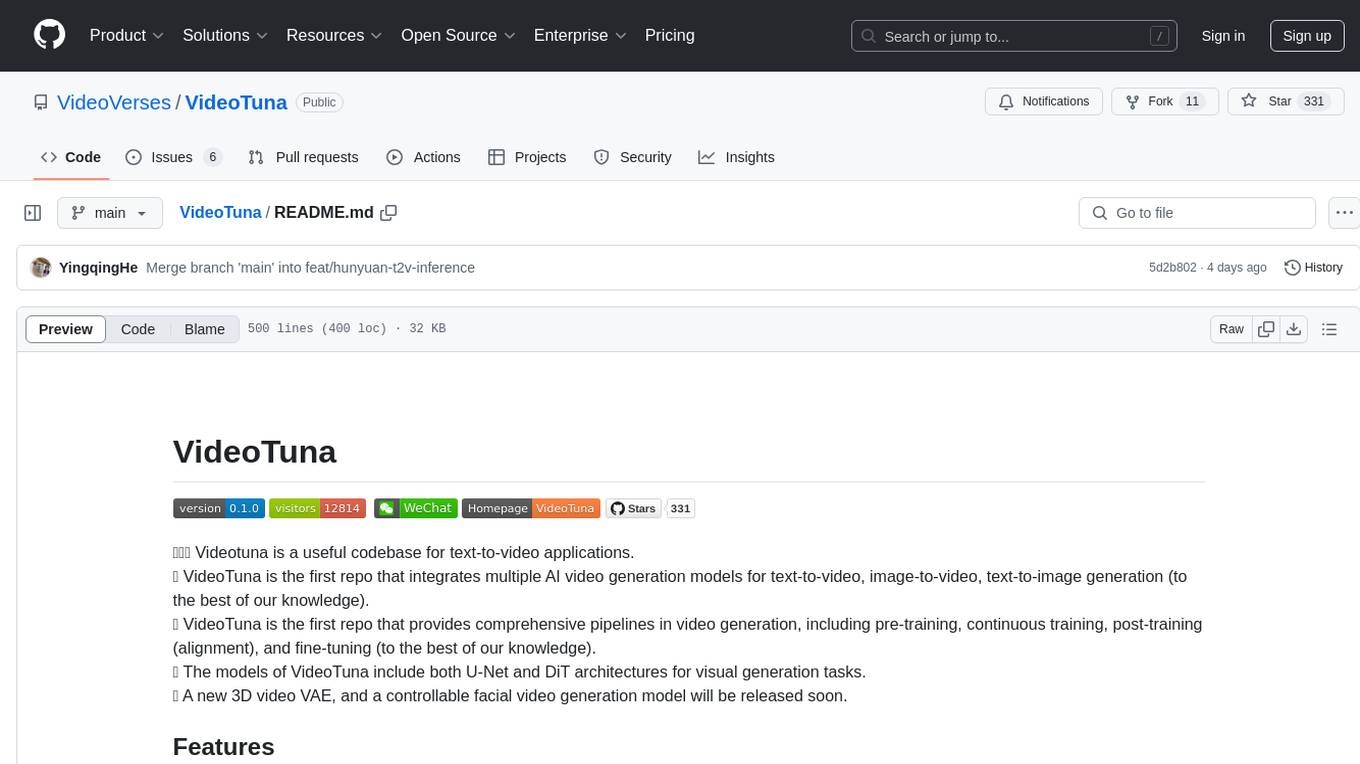
VideoTuna
VideoTuna is a codebase for text-to-video applications that integrates multiple AI video generation models for text-to-video, image-to-video, and text-to-image generation. It provides comprehensive pipelines in video generation, including pre-training, continuous training, post-training, and fine-tuning. The models in VideoTuna include U-Net and DiT architectures for visual generation tasks, with upcoming releases of a new 3D video VAE and a controllable facial video generation model.
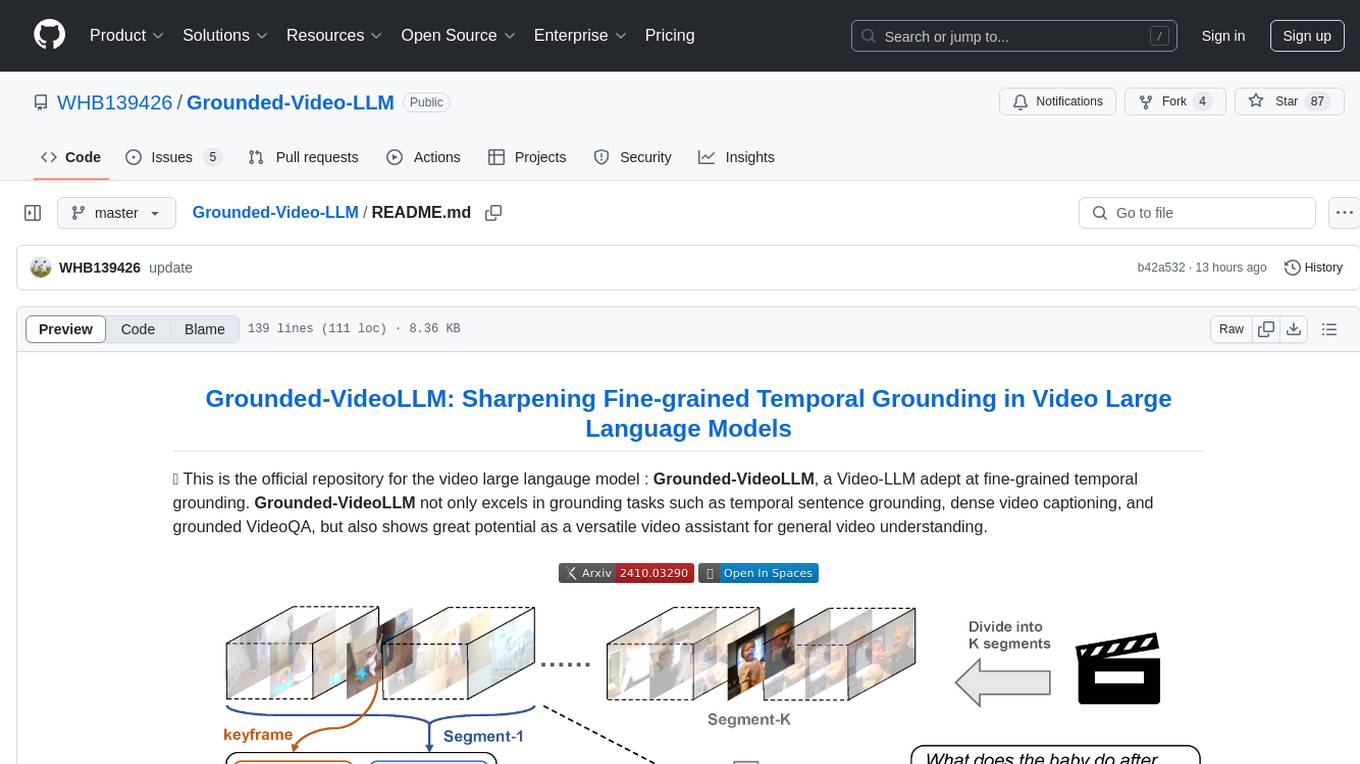
Grounded-Video-LLM
Grounded-VideoLLM is a Video Large Language Model specialized in fine-grained temporal grounding. It excels in tasks such as temporal sentence grounding, dense video captioning, and grounded VideoQA. The model incorporates an additional temporal stream, discrete temporal tokens with specific time knowledge, and a multi-stage training scheme. It shows potential as a versatile video assistant for general video understanding. The repository provides pretrained weights, inference scripts, and datasets for training. Users can run inference queries to get temporal information from videos and train the model from scratch.
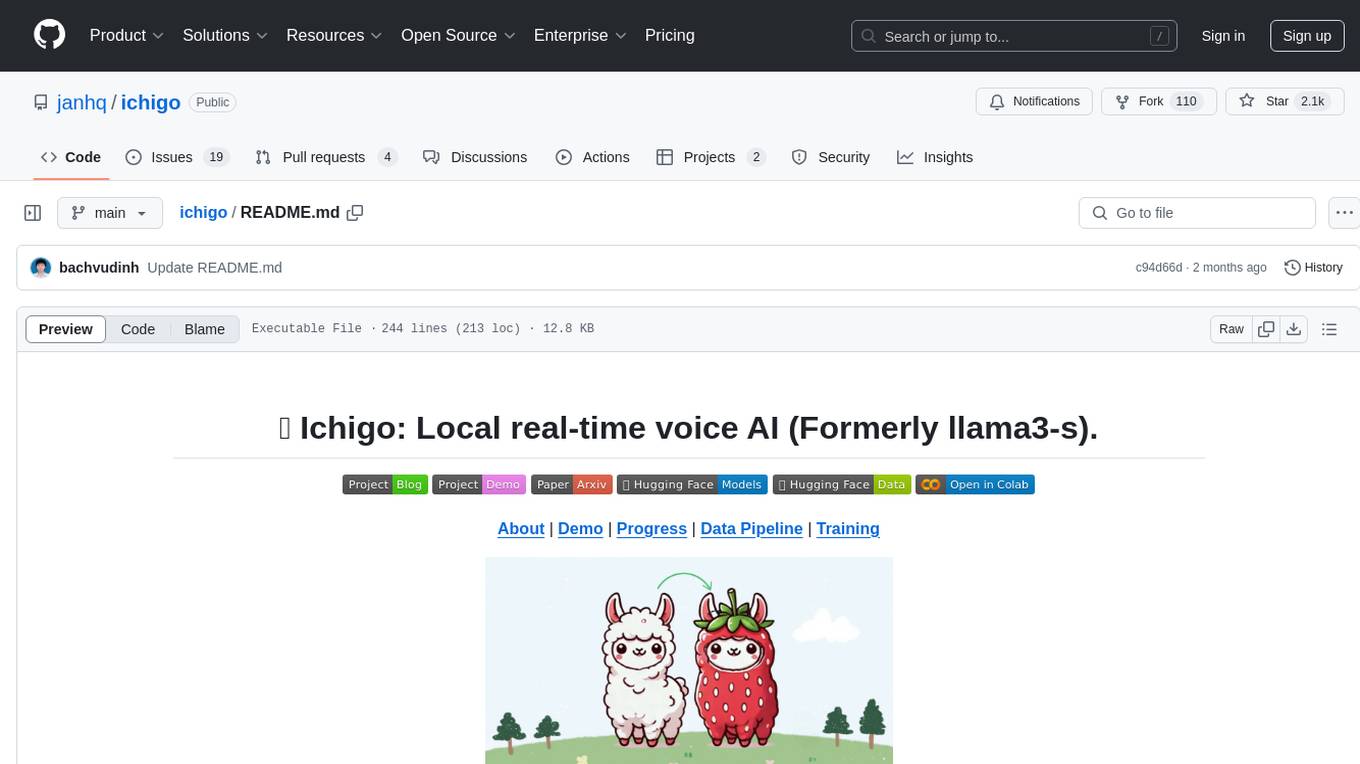
ichigo
Ichigo is a local real-time voice AI tool that uses an early fusion technique to extend a text-based LLM to have native 'listening' ability. It is an open research experiment with improved multiturn capabilities and the ability to refuse processing inaudible queries. The tool is designed for open data, open weight, on-device Siri-like functionality, inspired by Meta's Chameleon paper. Ichigo offers a web UI demo and Gradio web UI for users to interact with the tool. It has achieved enhanced MMLU scores, stronger context handling, advanced noise management, and improved multi-turn capabilities for a robust user experience.
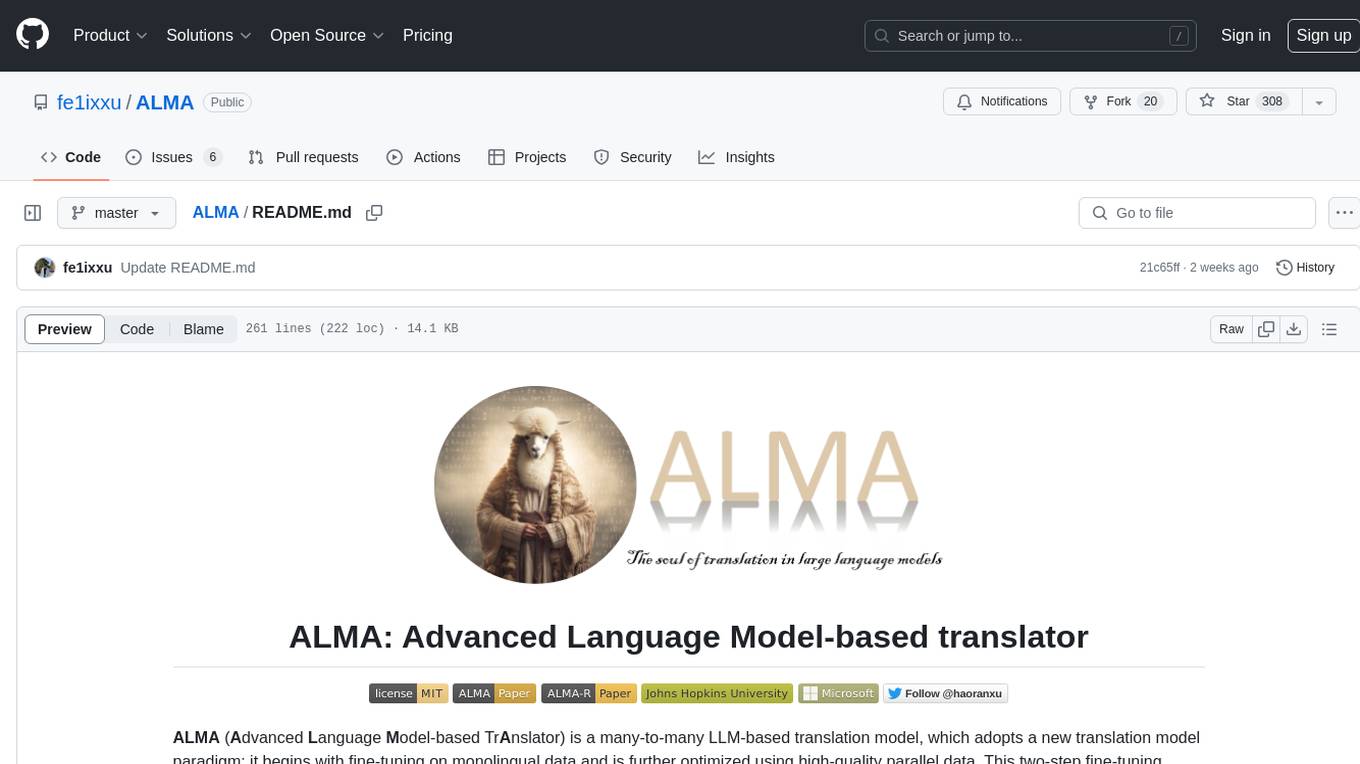
ALMA
ALMA (Advanced Language Model-based Translator) is a many-to-many LLM-based translation model that utilizes a two-step fine-tuning process on monolingual and parallel data to achieve strong translation performance. ALMA-R builds upon ALMA models with LoRA fine-tuning and Contrastive Preference Optimization (CPO) for even better performance, surpassing GPT-4 and WMT winners. The repository provides ALMA and ALMA-R models, datasets, environment setup, evaluation scripts, training guides, and data information for users to leverage these models for translation tasks.
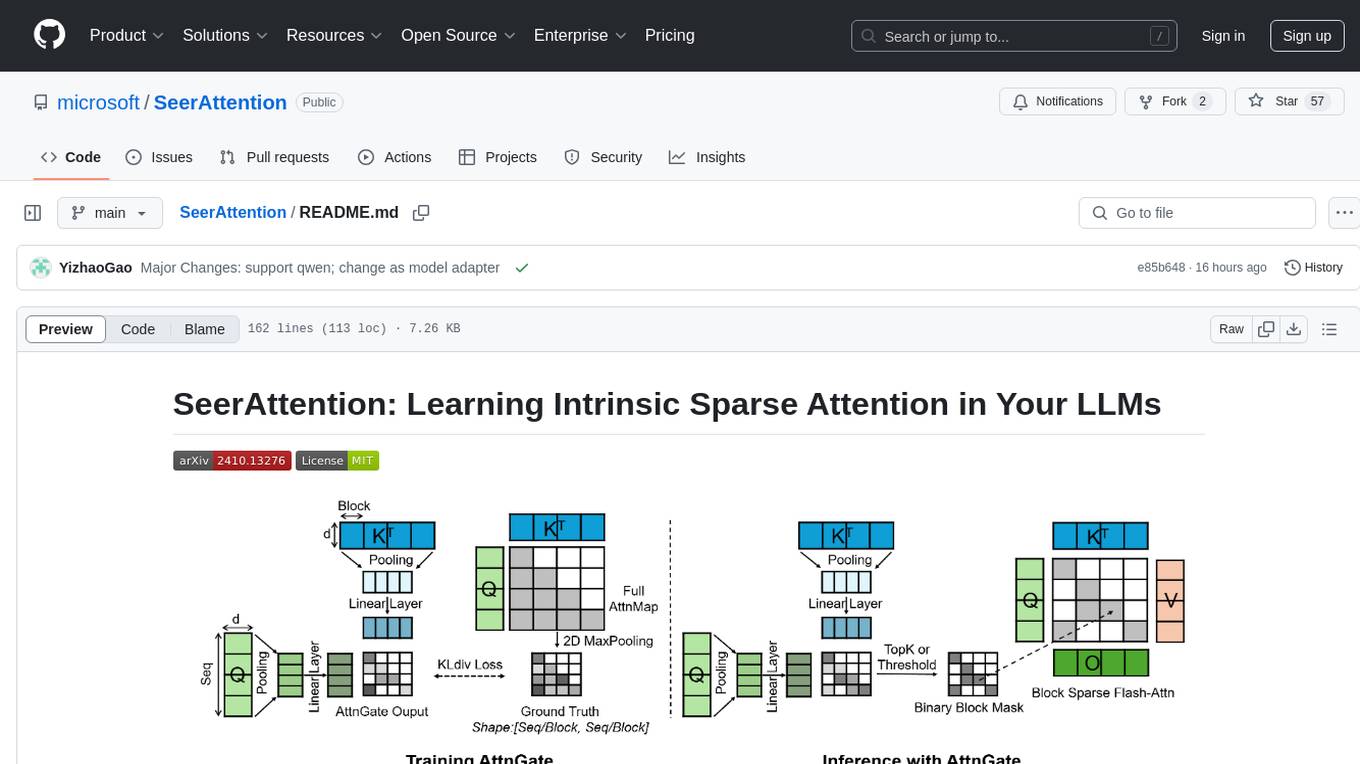
SeerAttention
SeerAttention is a novel trainable sparse attention mechanism that learns intrinsic sparsity patterns directly from LLMs through self-distillation at post-training time. It achieves faster inference while maintaining accuracy for long-context prefilling. The tool offers features such as trainable sparse attention, block-level sparsity, self-distillation, efficient kernel, and easy integration with existing transformer architectures. Users can quickly start using SeerAttention for inference with AttnGate Adapter and training attention gates with self-distillation. The tool provides efficient evaluation methods and encourages contributions from the community.
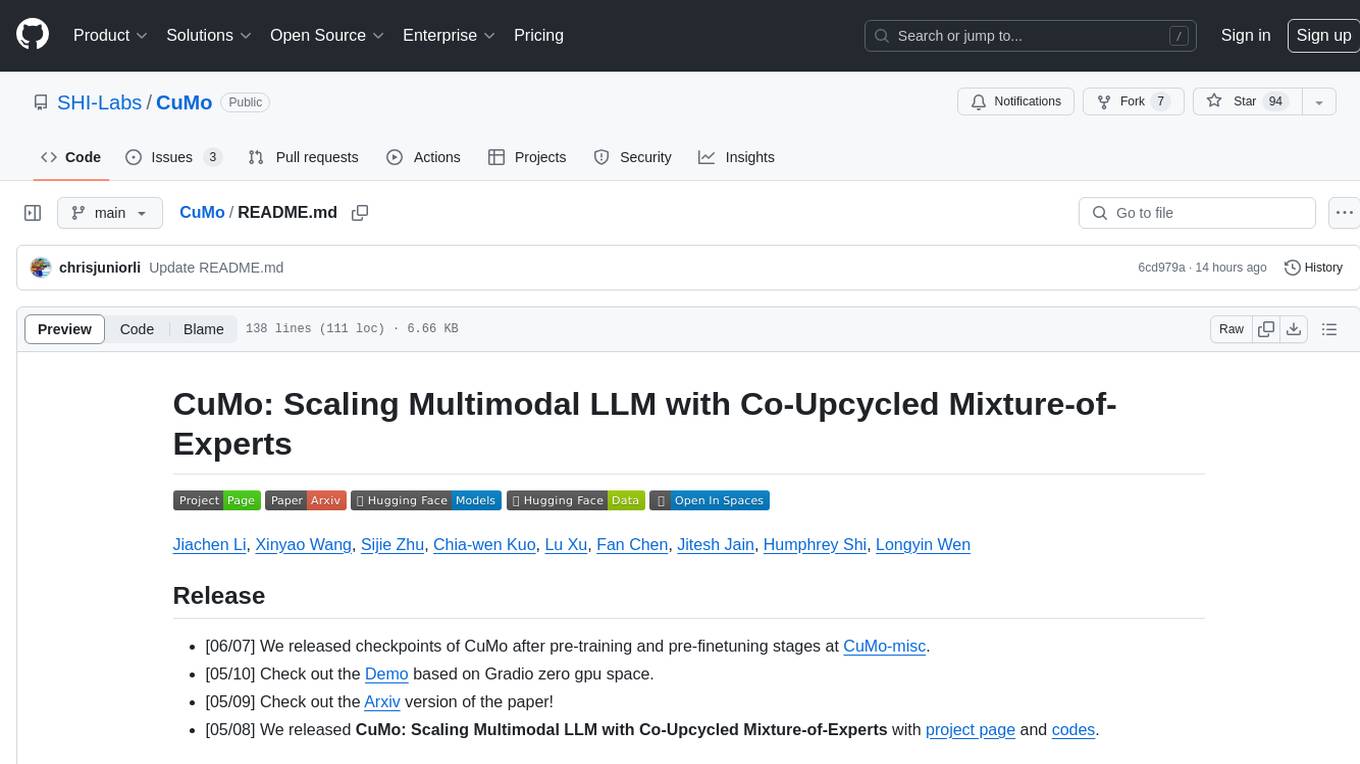
CuMo
CuMo is a project focused on scaling multimodal Large Language Models (LLMs) with Co-Upcycled Mixture-of-Experts. It introduces CuMo, which incorporates Co-upcycled Top-K sparsely-gated Mixture-of-experts blocks into the vision encoder and the MLP connector, enhancing the capabilities of multimodal LLMs. The project adopts a three-stage training approach with auxiliary losses to stabilize the training process and maintain a balanced loading of experts. CuMo achieves comparable performance to other state-of-the-art multimodal LLMs on various Visual Question Answering (VQA) and visual-instruction-following benchmarks.
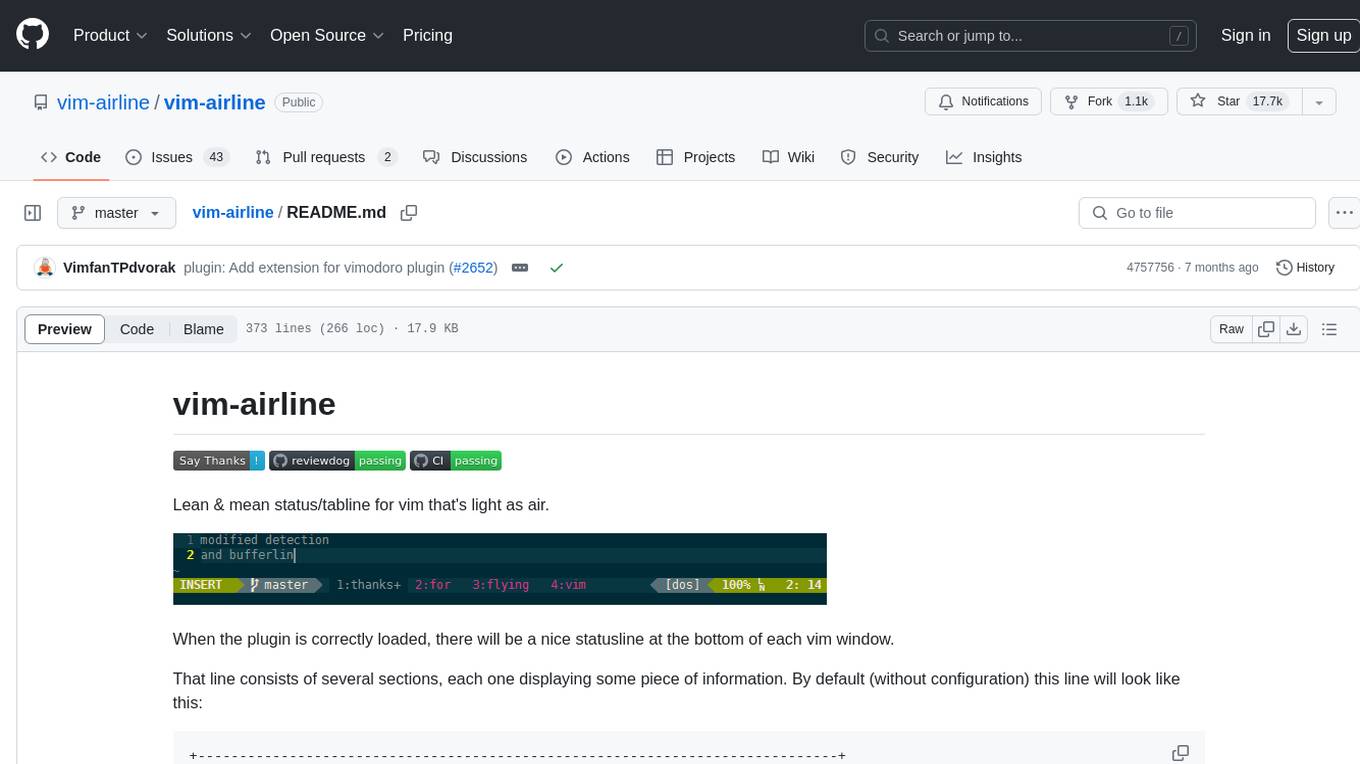
vim-airline
Vim-airline is a lean and mean status/tabline plugin for Vim that provides a nice statusline at the bottom of each Vim window. It consists of several sections displaying information such as mode, environment status, filename, filetype, file encoding, and current position in the file. The plugin is highly customizable and integrates with various plugins, providing a tiny core with extensibility in mind. It is optimized for speed, supports multiple themes, and integrates seamlessly with other plugins. Vim-airline is written in 100% Vimscript, eliminating the need for Python. The plugin aims to be stable and includes a unit testing suite for reliability.
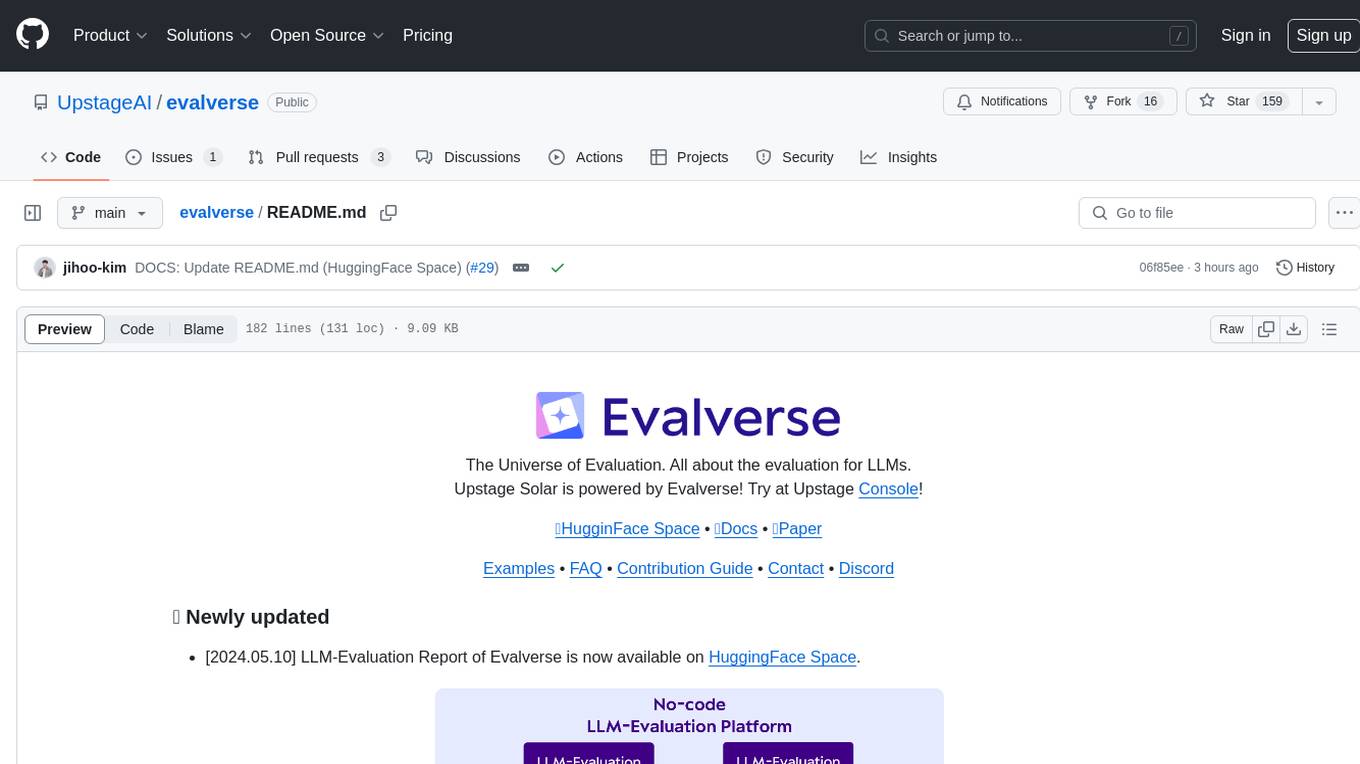
evalverse
Evalverse is an open-source project designed to support Large Language Model (LLM) evaluation needs. It provides a standardized and user-friendly solution for processing and managing LLM evaluations, catering to AI research engineers and scientists. Evalverse supports various evaluation methods, insightful reports, and no-code evaluation processes. Users can access unified evaluation with submodules, request evaluations without code via Slack bot, and obtain comprehensive reports with scores, rankings, and visuals. The tool allows for easy comparison of scores across different models and swift addition of new evaluation tools.
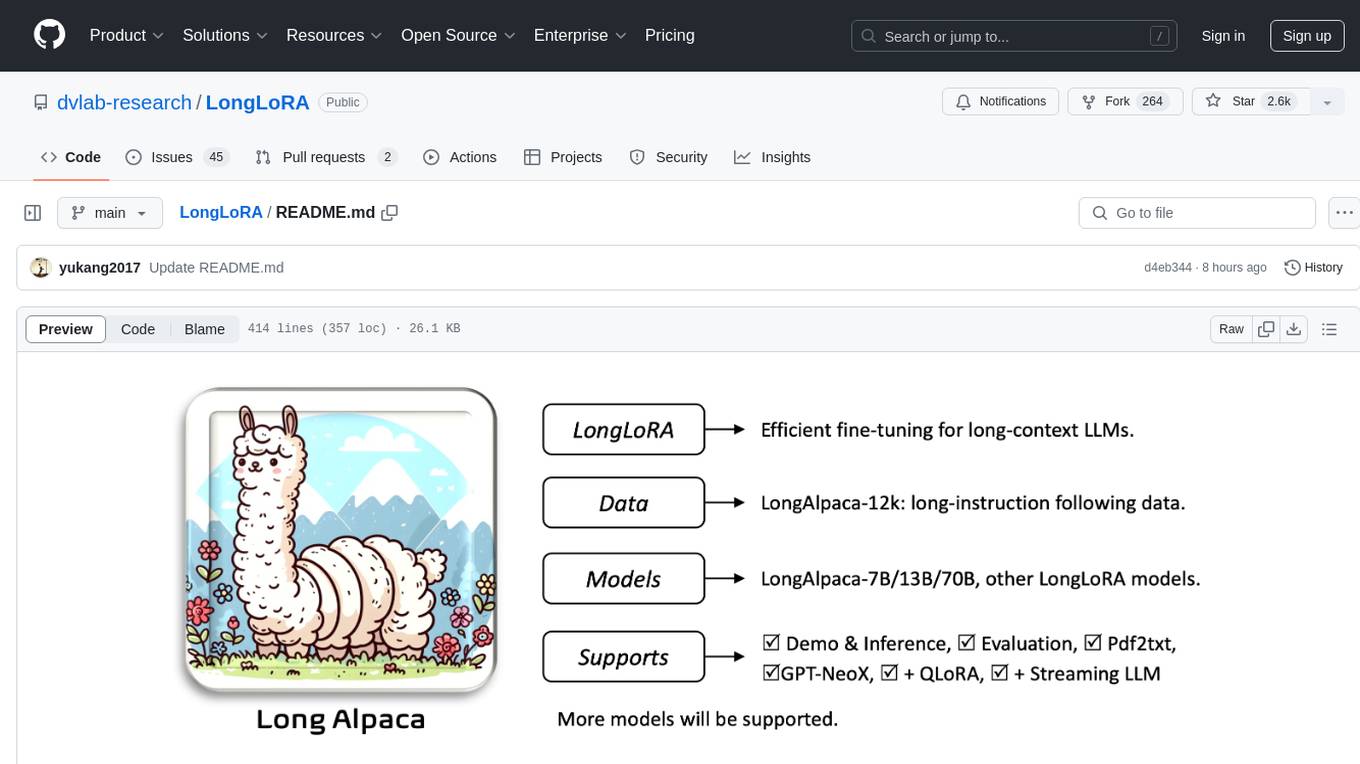
LongLoRA
LongLoRA is a tool for efficient fine-tuning of long-context large language models. It includes LongAlpaca data with long QA data collected and short QA sampled, models from 7B to 70B with context length from 8k to 100k, and support for GPTNeoX models. The tool supports supervised fine-tuning, context extension, and improved LoRA fine-tuning. It provides pre-trained weights, fine-tuning instructions, evaluation methods, local and online demos, streaming inference, and data generation via Pdf2text. LongLoRA is licensed under Apache License 2.0, while data and weights are under CC-BY-NC 4.0 License for research use only.
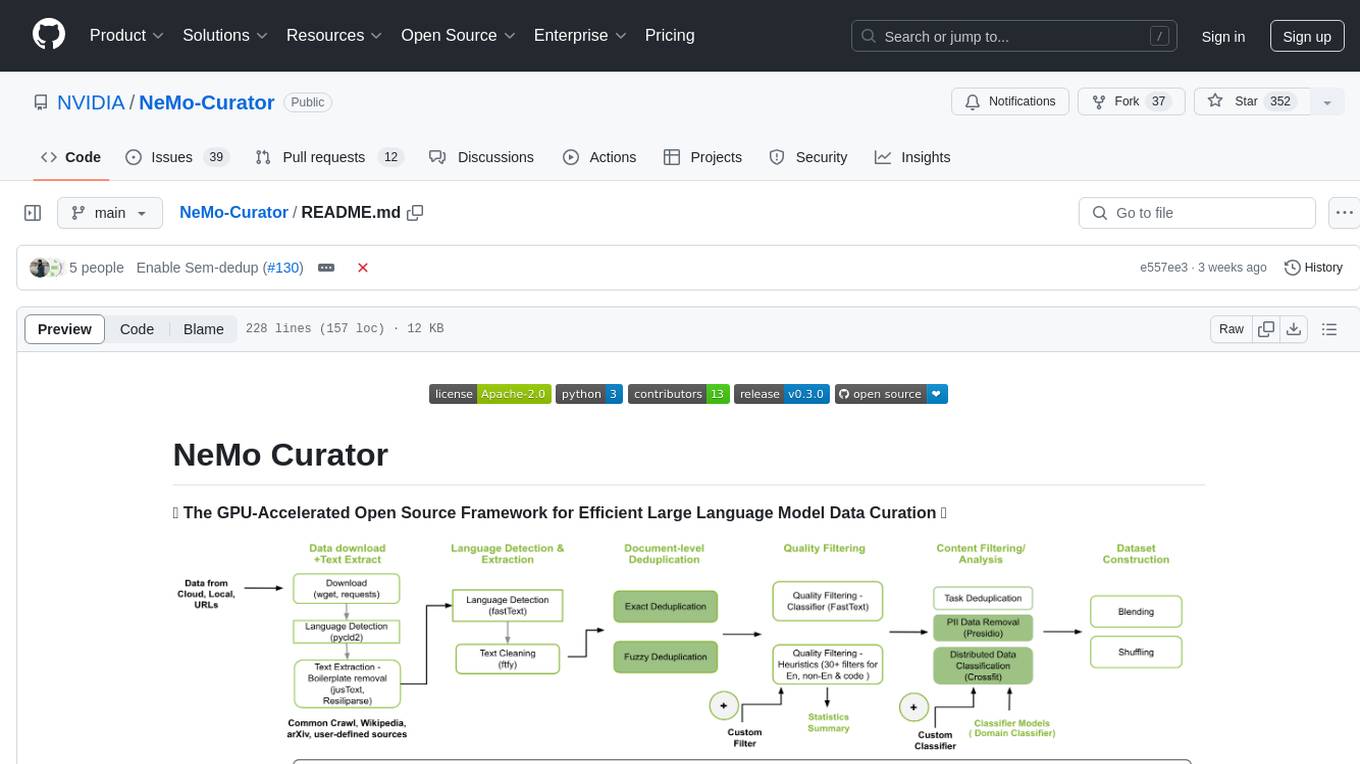
NeMo-Curator
NeMo Curator is a GPU-accelerated open-source framework designed for efficient large language model data curation. It provides scalable dataset preparation for tasks like foundation model pretraining, domain-adaptive pretraining, supervised fine-tuning, and parameter-efficient fine-tuning. The library leverages GPUs with Dask and RAPIDS to accelerate data curation, offering customizable and modular interfaces for pipeline expansion and model convergence. Key features include data download, text extraction, quality filtering, deduplication, downstream-task decontamination, distributed data classification, and PII redaction. NeMo Curator is suitable for curating high-quality datasets for large language model training.
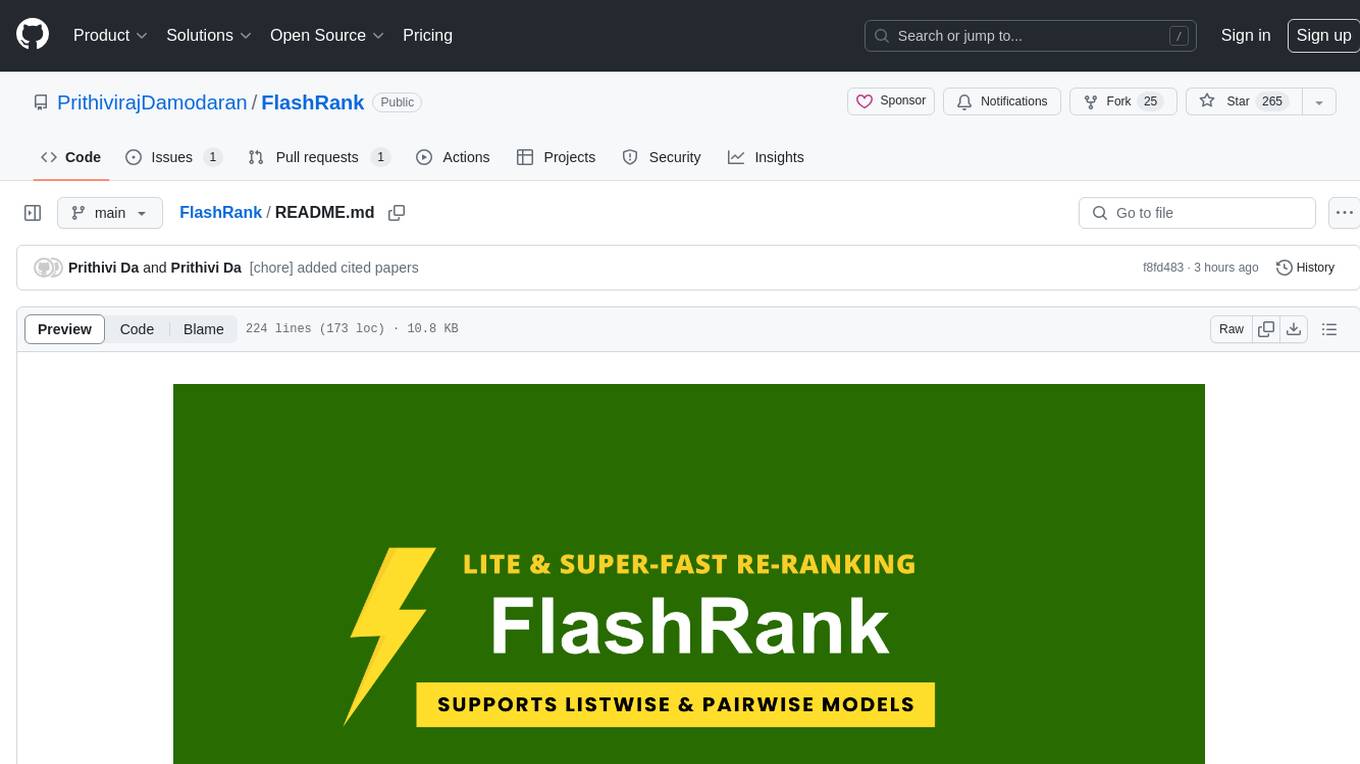
FlashRank
FlashRank is an ultra-lite and super-fast Python library designed to add re-ranking capabilities to existing search and retrieval pipelines. It is based on state-of-the-art Language Models (LLMs) and cross-encoders, offering support for pairwise/pointwise rerankers and listwise LLM-based rerankers. The library boasts the tiniest reranking model in the world (~4MB) and runs on CPU without the need for Torch or Transformers. FlashRank is cost-conscious, with a focus on low cost per invocation and smaller package size for efficient serverless deployments. It supports various models like ms-marco-TinyBERT, ms-marco-MiniLM, rank-T5-flan, ms-marco-MultiBERT, and more, with plans for future model additions. The tool is ideal for enhancing search precision and speed in scenarios where lightweight models with competitive performance are preferred.
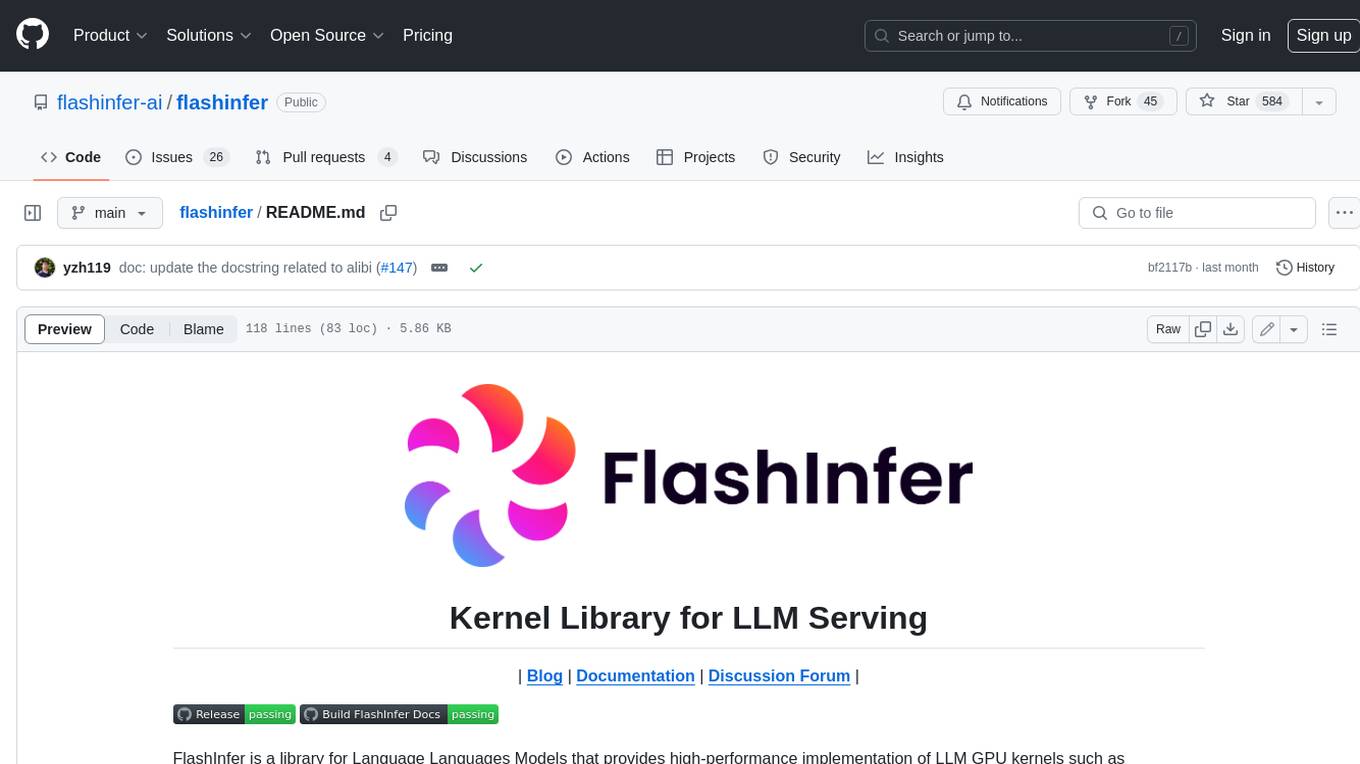
flashinfer
FlashInfer is a library for Language Languages Models that provides high-performance implementation of LLM GPU kernels such as FlashAttention, PageAttention and LoRA. FlashInfer focus on LLM serving and inference, and delivers state-the-art performance across diverse scenarios.
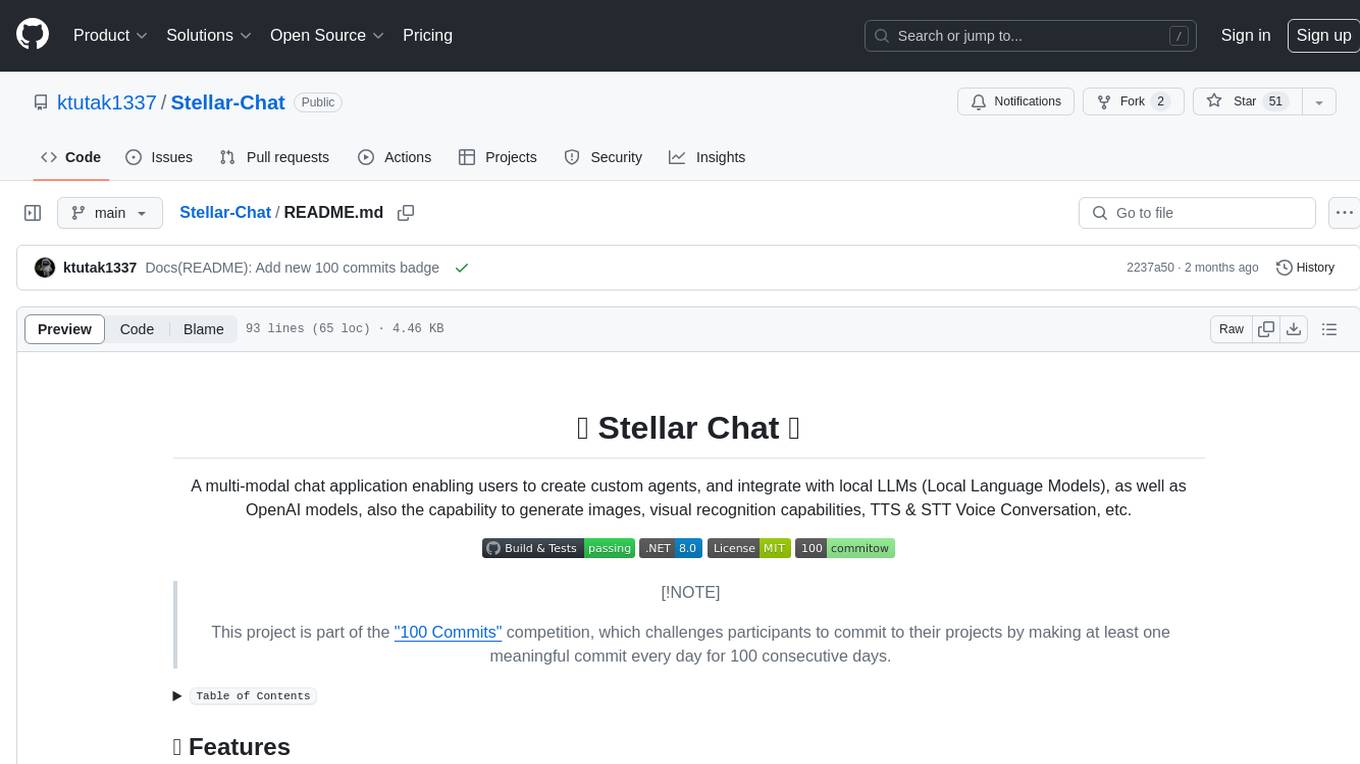
Stellar-Chat
Stellar Chat is a multi-modal chat application that enables users to create custom agents and integrate with local language models and OpenAI models. It provides capabilities for generating images, visual recognition, text-to-speech, and speech-to-text functionalities. Users can engage in multimodal conversations, create custom agents, search messages and conversations, and integrate with various applications for enhanced productivity. The project is part of the '100 Commits' competition, challenging participants to make meaningful commits daily for 100 consecutive days.
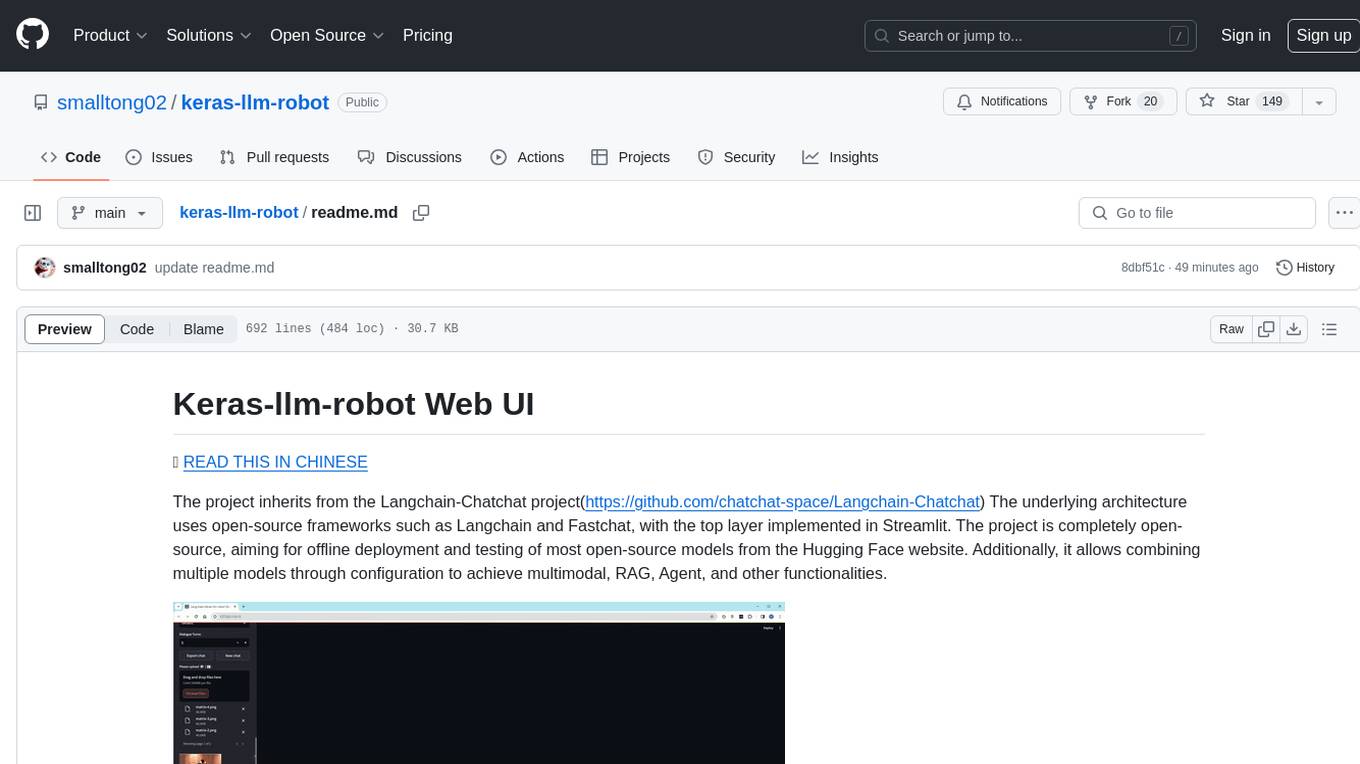
keras-llm-robot
The Keras-llm-robot Web UI project is an open-source tool designed for offline deployment and testing of various open-source models from the Hugging Face website. It allows users to combine multiple models through configuration to achieve functionalities like multimodal, RAG, Agent, and more. The project consists of three main interfaces: chat interface for language models, configuration interface for loading models, and tools & agent interface for auxiliary models. Users can interact with the language model through text, voice, and image inputs, and the tool supports features like model loading, quantization, fine-tuning, role-playing, code interpretation, speech recognition, image recognition, network search engine, and function calling.
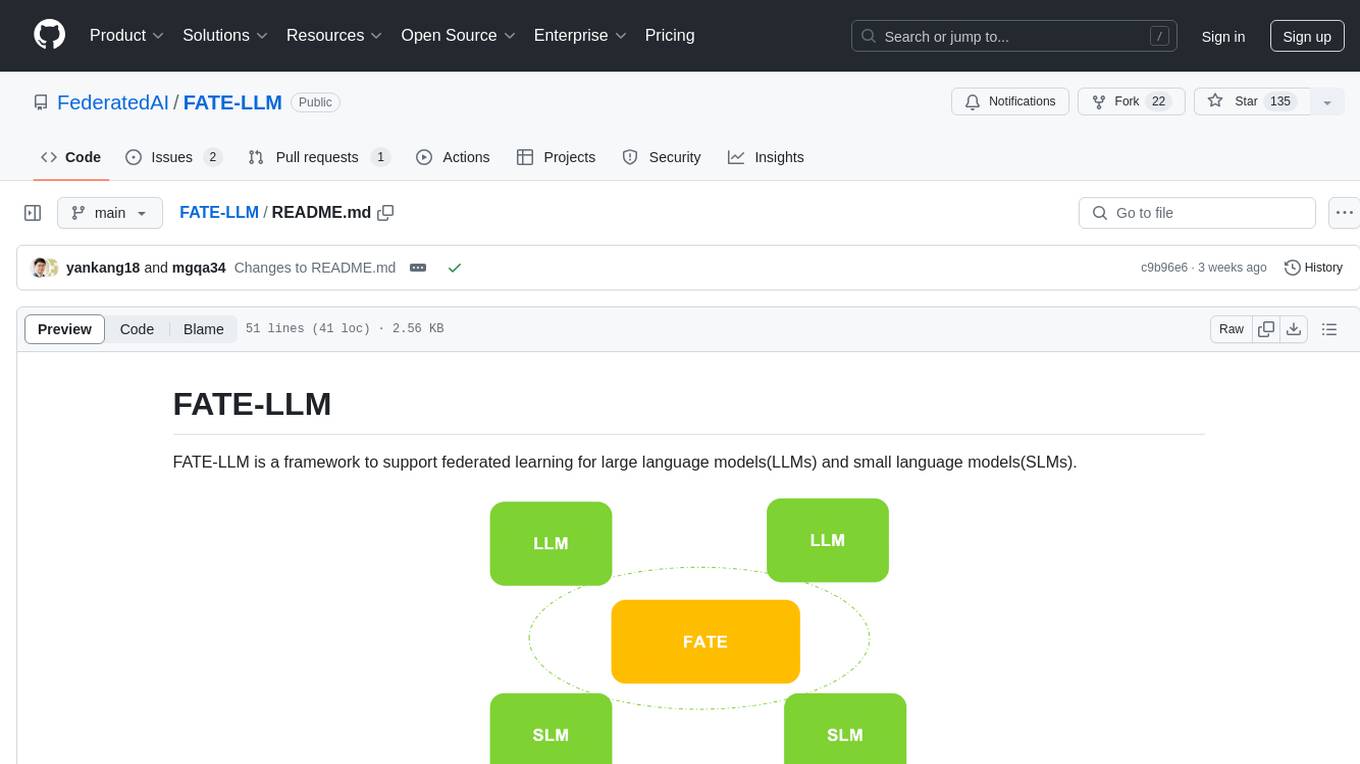
FATE-LLM
FATE-LLM is a framework supporting federated learning for large and small language models. It promotes training efficiency of federated LLMs using Parameter-Efficient methods, protects the IP of LLMs using FedIPR, and ensures data privacy during training and inference through privacy-preserving mechanisms.
For similar tasks
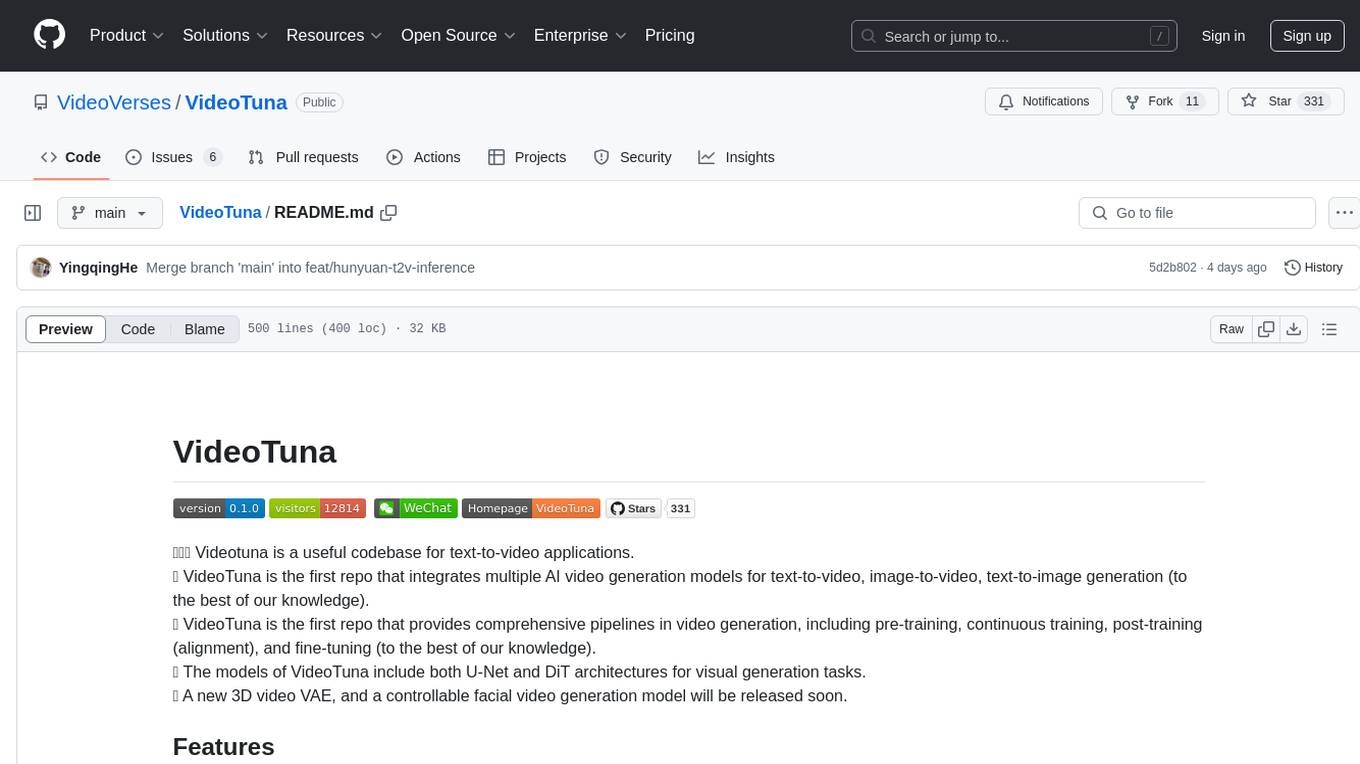
VideoTuna
VideoTuna is a codebase for text-to-video applications that integrates multiple AI video generation models for text-to-video, image-to-video, and text-to-image generation. It provides comprehensive pipelines in video generation, including pre-training, continuous training, post-training, and fine-tuning. The models in VideoTuna include U-Net and DiT architectures for visual generation tasks, with upcoming releases of a new 3D video VAE and a controllable facial video generation model.
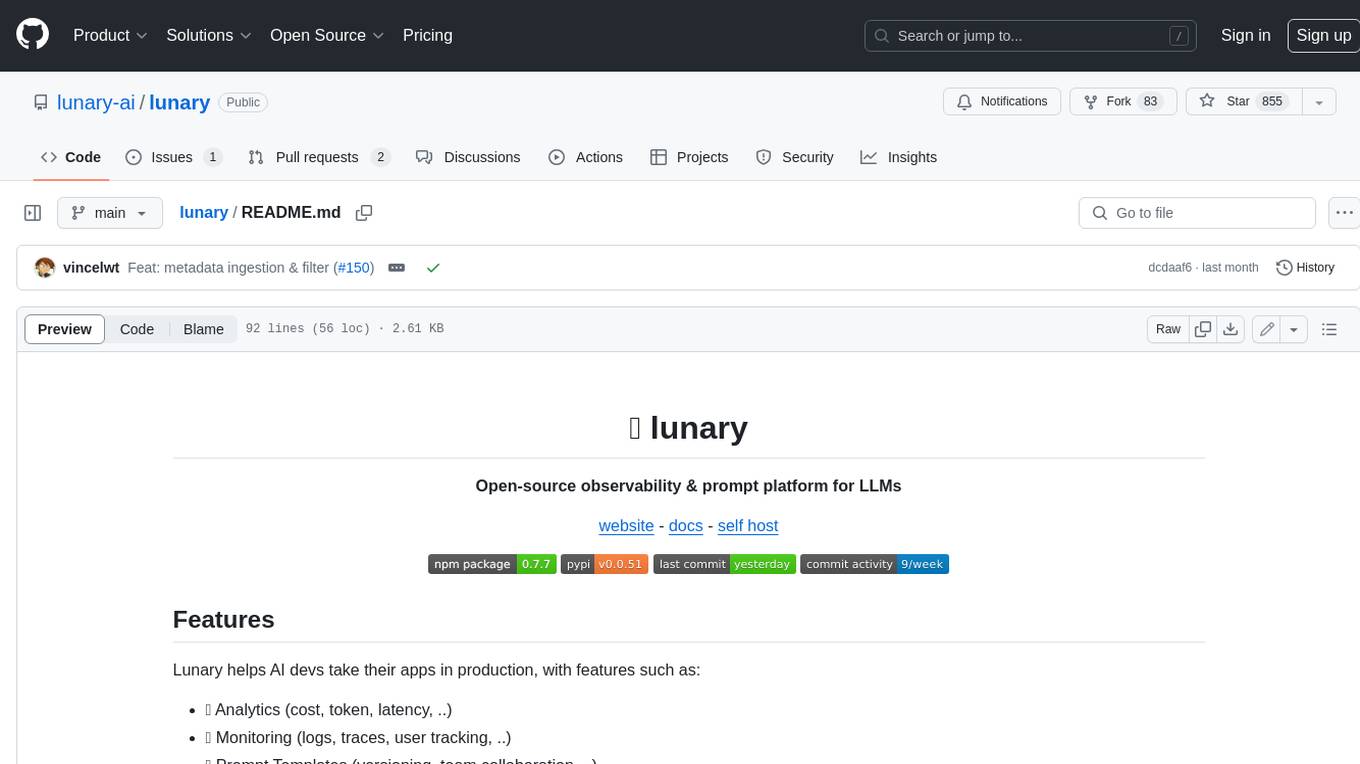
lunary
Lunary is an open-source observability and prompt platform for Large Language Models (LLMs). It provides a suite of features to help AI developers take their applications into production, including analytics, monitoring, prompt templates, fine-tuning dataset creation, chat and feedback tracking, and evaluations. Lunary is designed to be usable with any model, not just OpenAI, and is easy to integrate and self-host.
For similar jobs
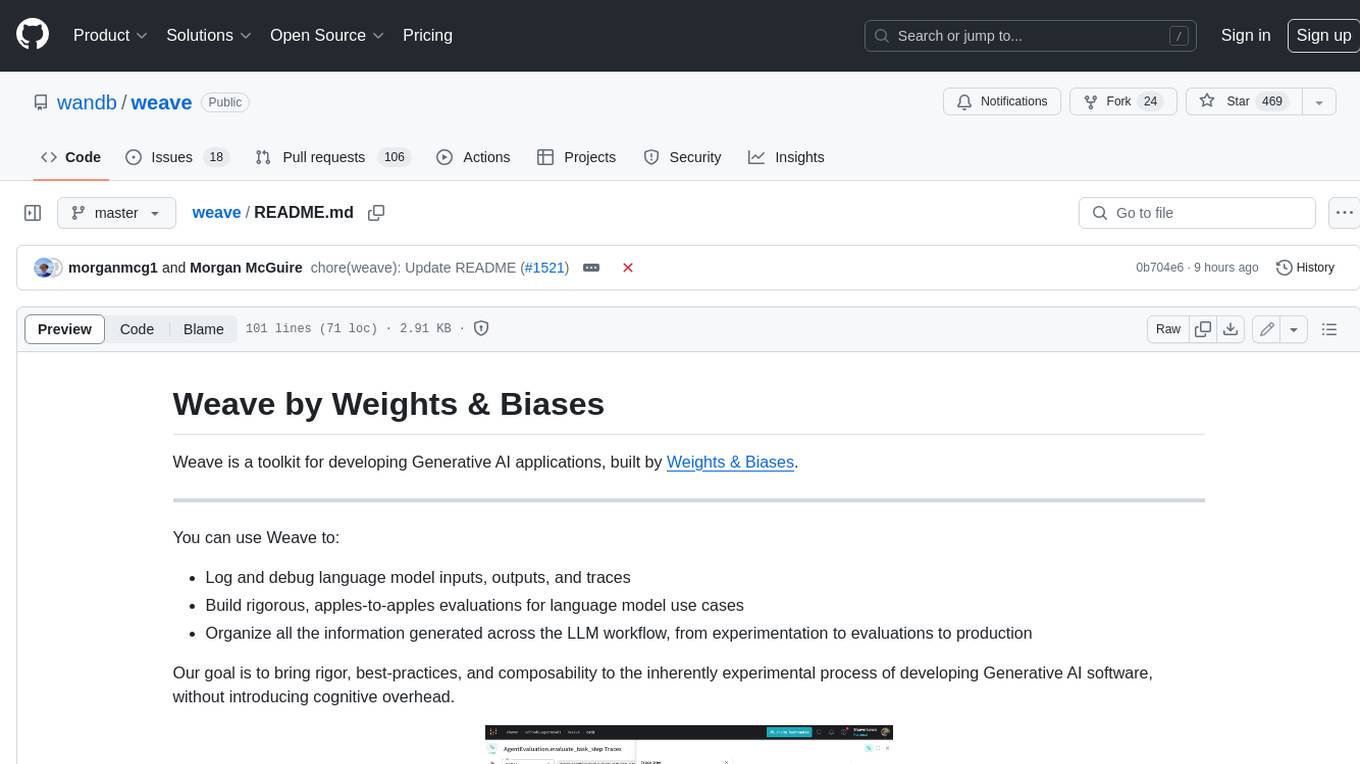
weave
Weave is a toolkit for developing Generative AI applications, built by Weights & Biases. With Weave, you can log and debug language model inputs, outputs, and traces; build rigorous, apples-to-apples evaluations for language model use cases; and organize all the information generated across the LLM workflow, from experimentation to evaluations to production. Weave aims to bring rigor, best-practices, and composability to the inherently experimental process of developing Generative AI software, without introducing cognitive overhead.
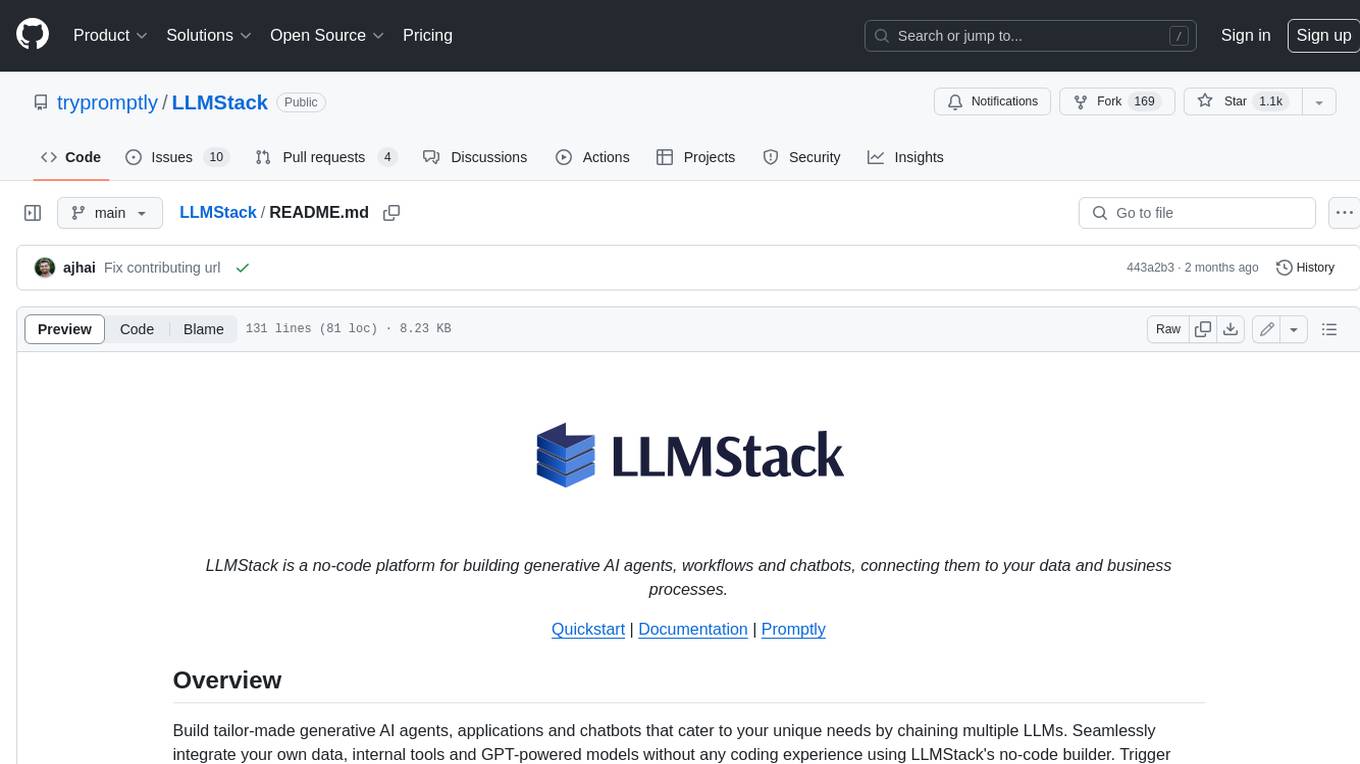
LLMStack
LLMStack is a no-code platform for building generative AI agents, workflows, and chatbots. It allows users to connect their own data, internal tools, and GPT-powered models without any coding experience. LLMStack can be deployed to the cloud or on-premise and can be accessed via HTTP API or triggered from Slack or Discord.
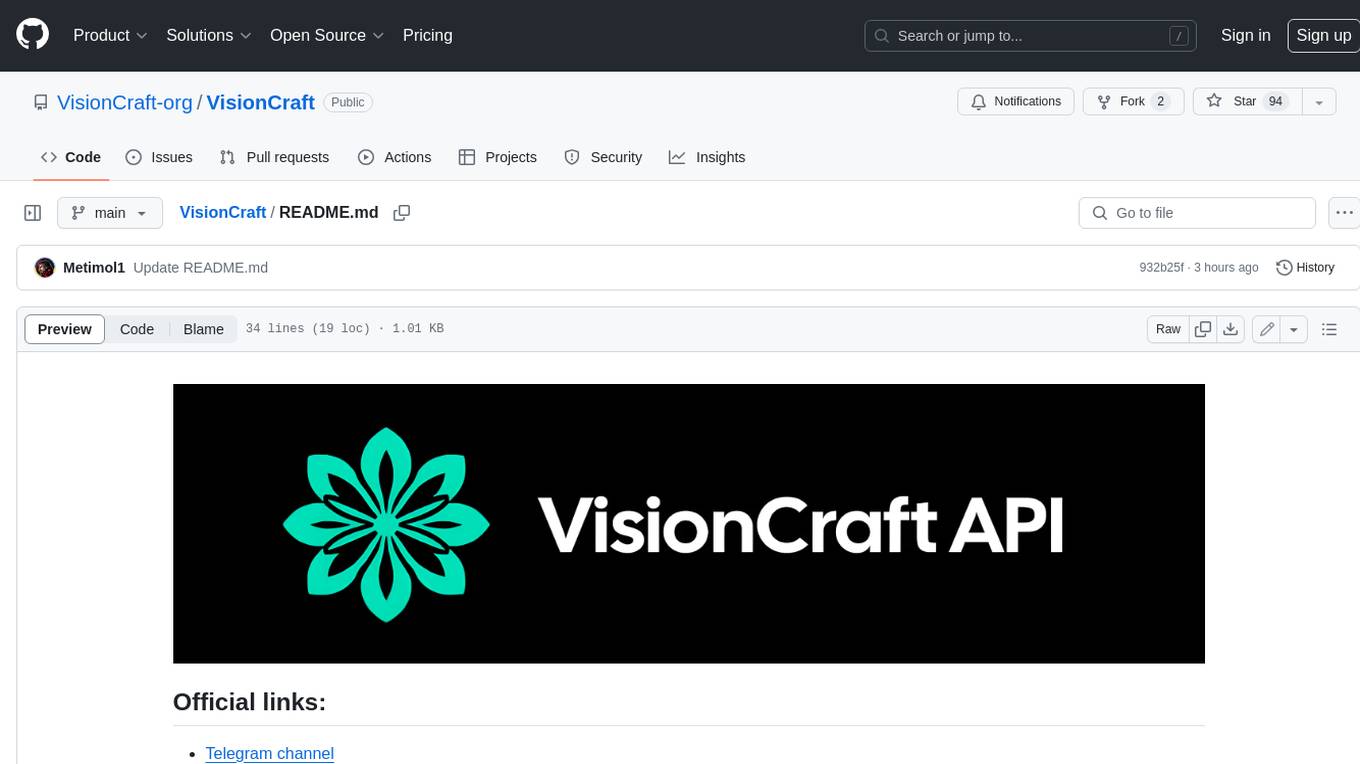
VisionCraft
The VisionCraft API is a free API for using over 100 different AI models. From images to sound.
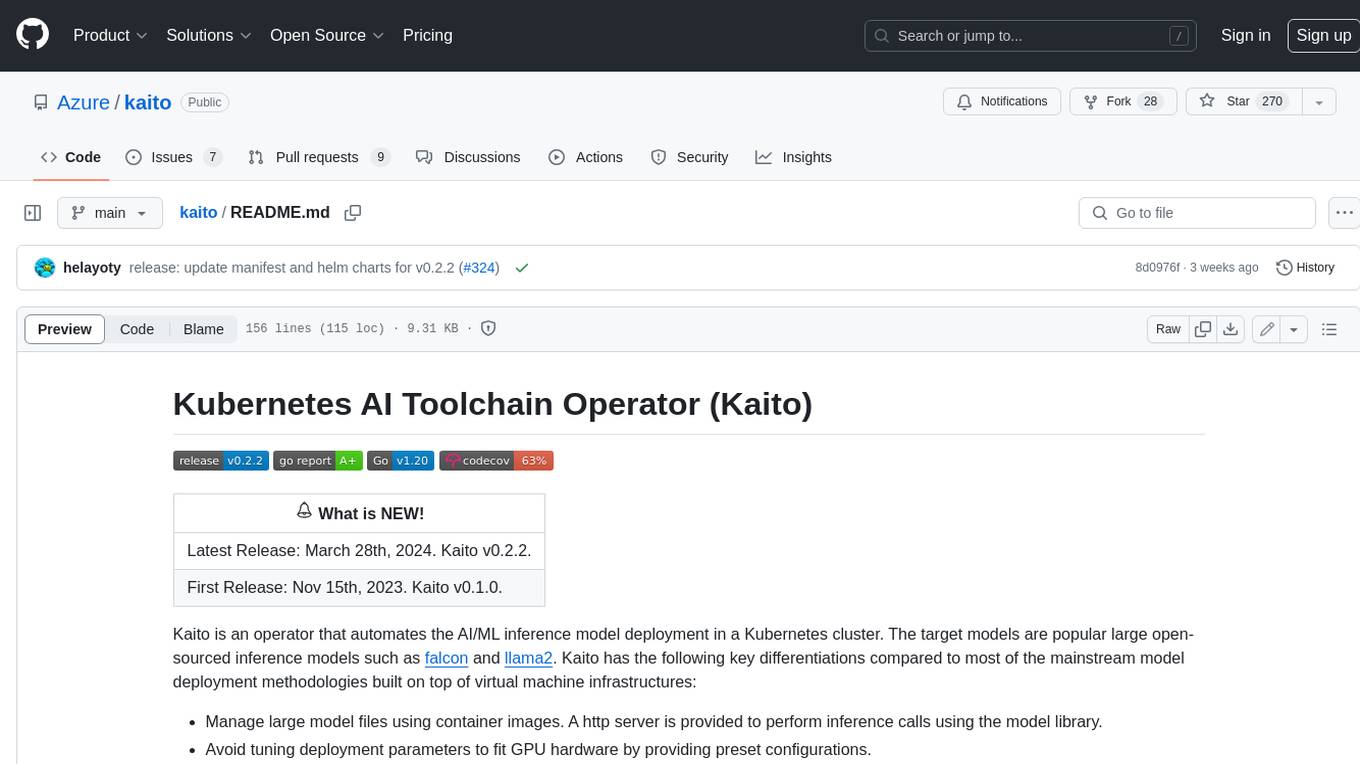
kaito
Kaito is an operator that automates the AI/ML inference model deployment in a Kubernetes cluster. It manages large model files using container images, avoids tuning deployment parameters to fit GPU hardware by providing preset configurations, auto-provisions GPU nodes based on model requirements, and hosts large model images in the public Microsoft Container Registry (MCR) if the license allows. Using Kaito, the workflow of onboarding large AI inference models in Kubernetes is largely simplified.
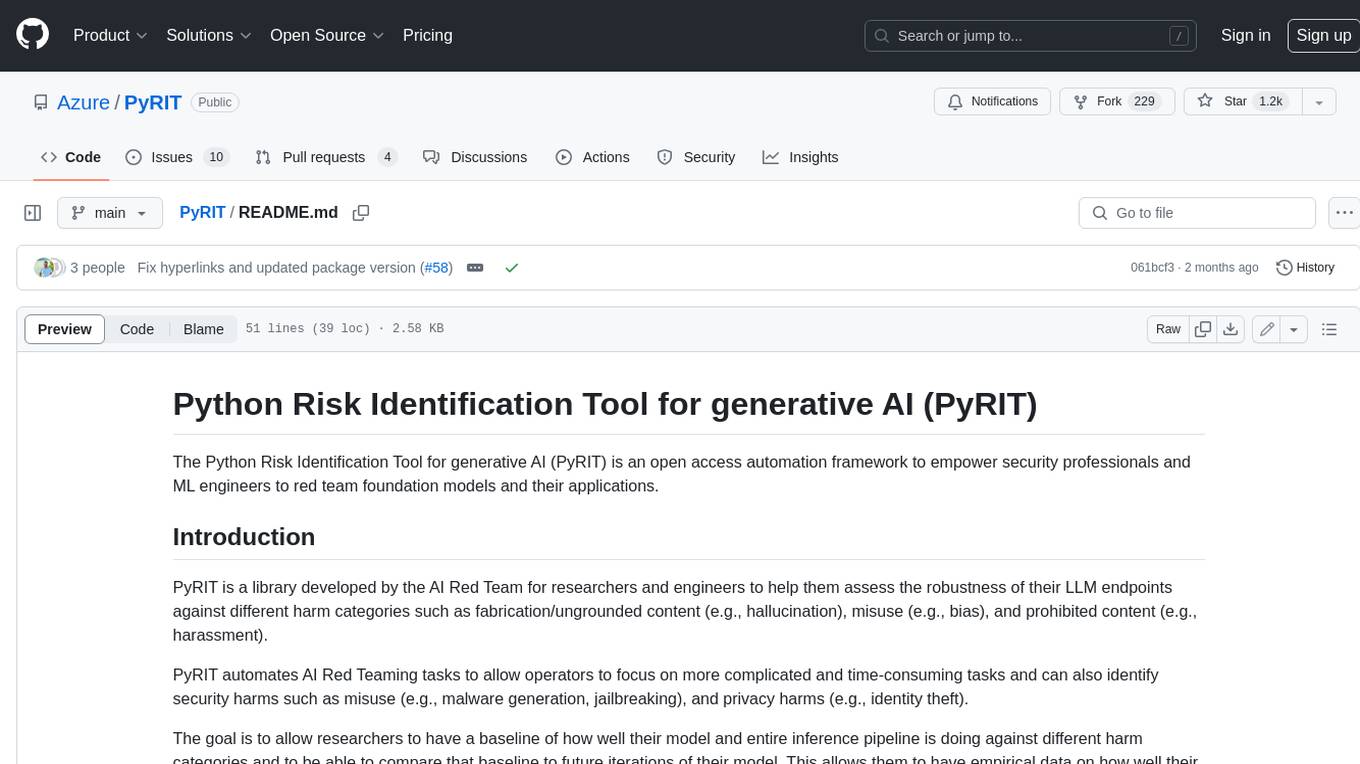
PyRIT
PyRIT is an open access automation framework designed to empower security professionals and ML engineers to red team foundation models and their applications. It automates AI Red Teaming tasks to allow operators to focus on more complicated and time-consuming tasks and can also identify security harms such as misuse (e.g., malware generation, jailbreaking), and privacy harms (e.g., identity theft). The goal is to allow researchers to have a baseline of how well their model and entire inference pipeline is doing against different harm categories and to be able to compare that baseline to future iterations of their model. This allows them to have empirical data on how well their model is doing today, and detect any degradation of performance based on future improvements.
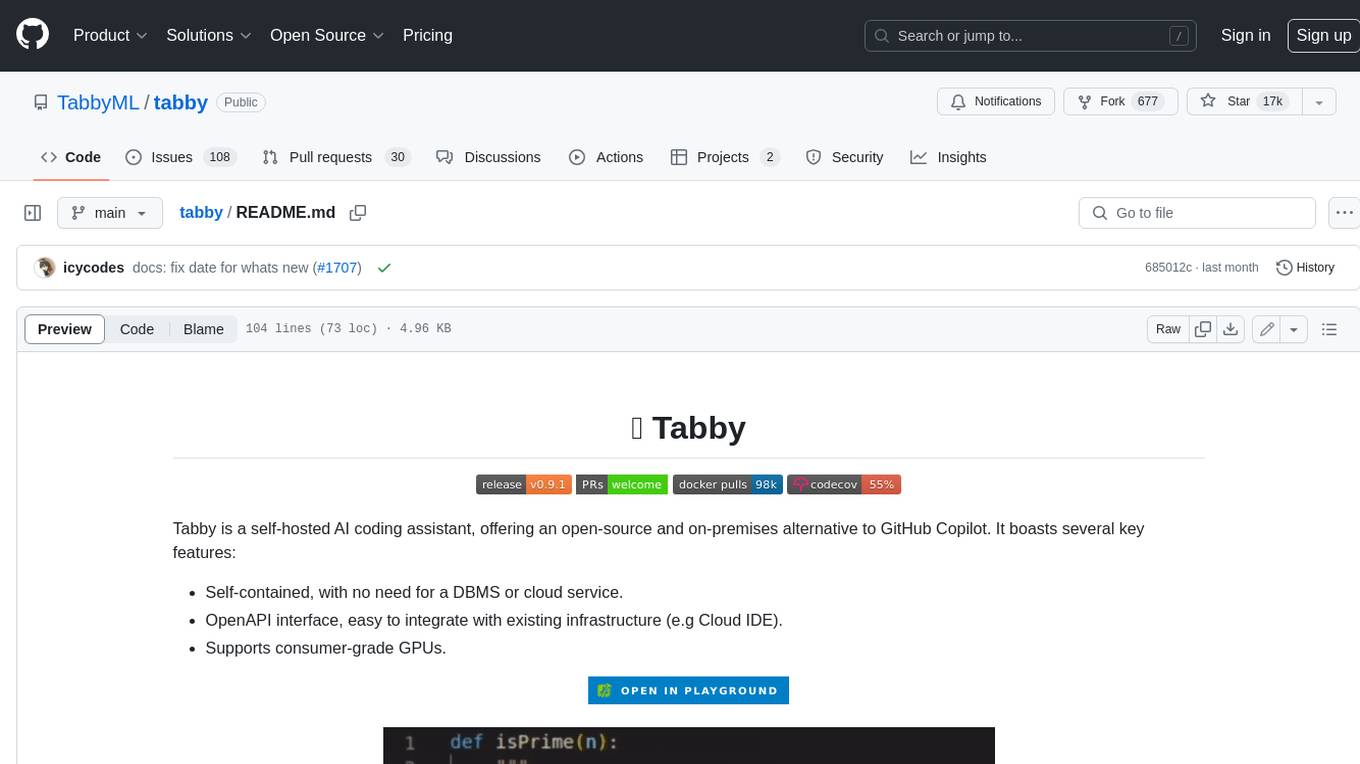
tabby
Tabby is a self-hosted AI coding assistant, offering an open-source and on-premises alternative to GitHub Copilot. It boasts several key features: * Self-contained, with no need for a DBMS or cloud service. * OpenAPI interface, easy to integrate with existing infrastructure (e.g Cloud IDE). * Supports consumer-grade GPUs.
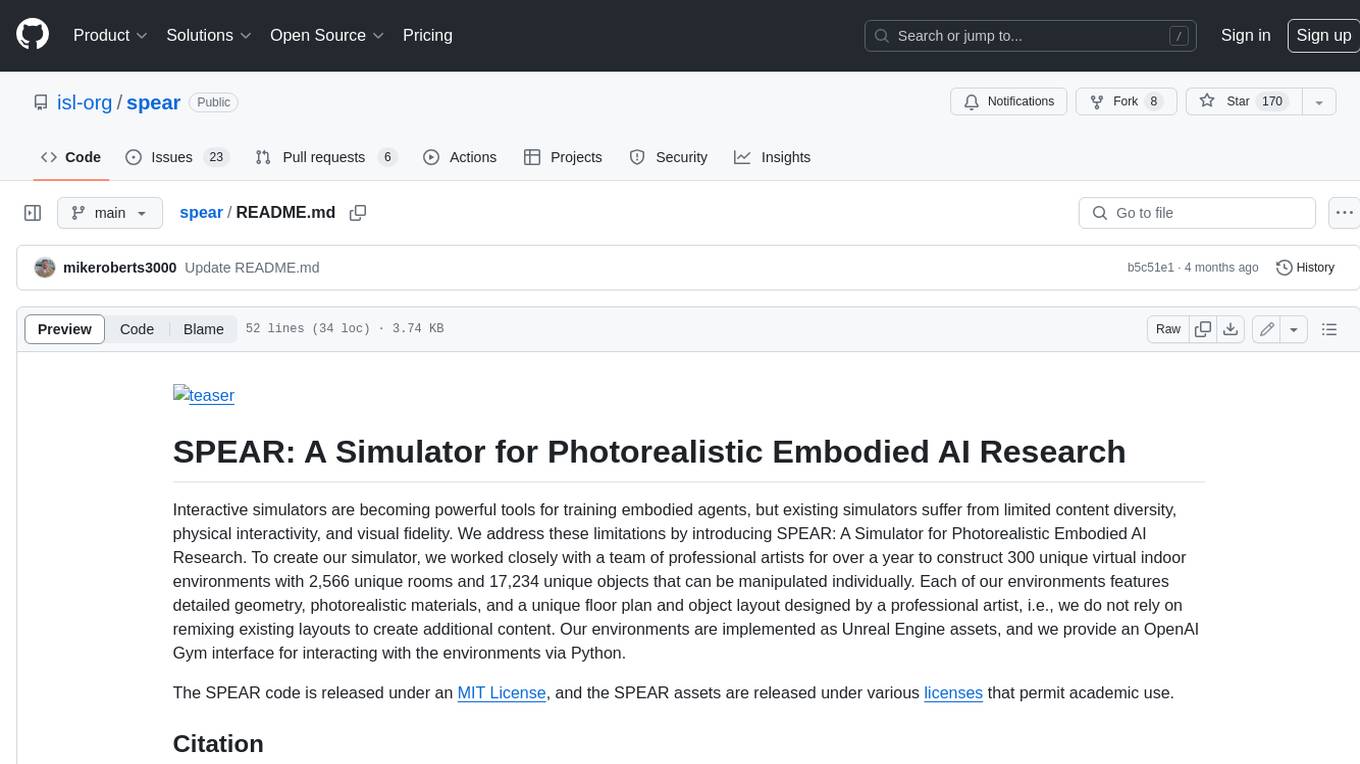
spear
SPEAR (Simulator for Photorealistic Embodied AI Research) is a powerful tool for training embodied agents. It features 300 unique virtual indoor environments with 2,566 unique rooms and 17,234 unique objects that can be manipulated individually. Each environment is designed by a professional artist and features detailed geometry, photorealistic materials, and a unique floor plan and object layout. SPEAR is implemented as Unreal Engine assets and provides an OpenAI Gym interface for interacting with the environments via Python.
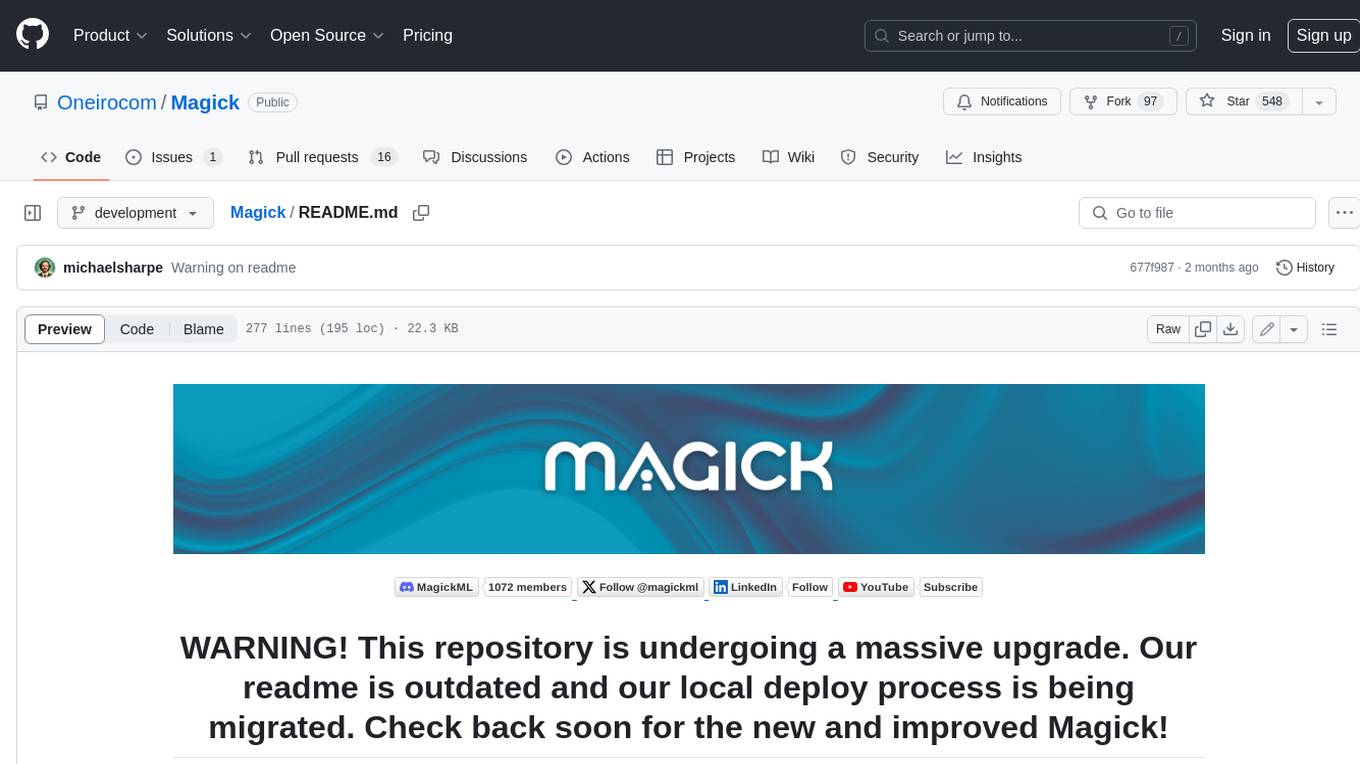
Magick
Magick is a groundbreaking visual AIDE (Artificial Intelligence Development Environment) for no-code data pipelines and multimodal agents. Magick can connect to other services and comes with nodes and templates well-suited for intelligent agents, chatbots, complex reasoning systems and realistic characters.