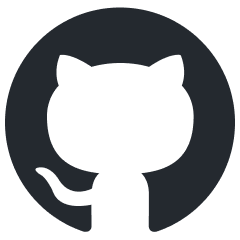
Awesome-Tabular-LLMs
We collect papers about "large language models (LLM) for table-related tasks", e.g., using LLM for Table QA task. “表格+LLM”相关论文整理
Stars: 151
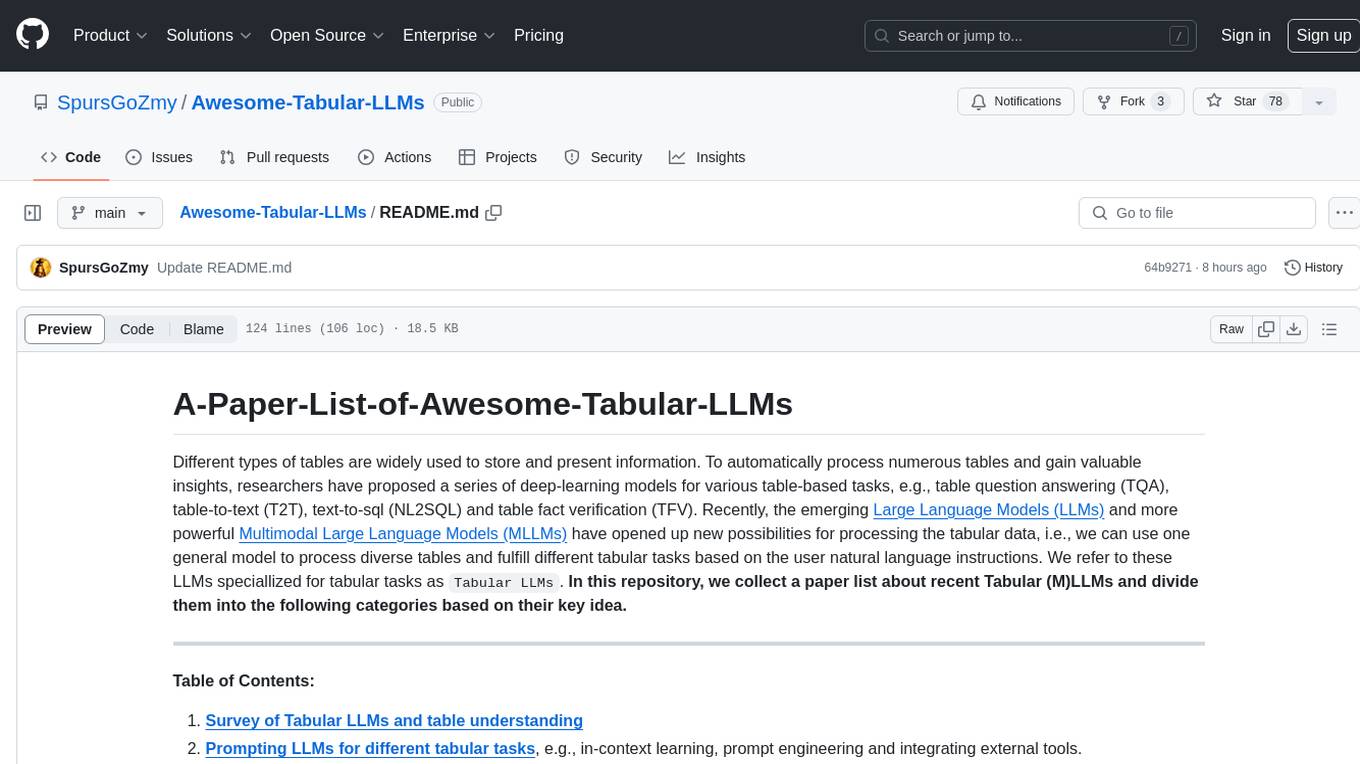
This repository is a collection of papers on Tabular Large Language Models (LLMs) specialized for processing tabular data. It includes surveys, models, and applications related to table understanding tasks such as Table Question Answering, Table-to-Text, Text-to-SQL, and more. The repository categorizes the papers based on key ideas and provides insights into the advancements in using LLMs for processing diverse tables and fulfilling various tabular tasks based on natural language instructions.
README:
Different types of tables are widely used to store and present information. To automatically process numerous tables and gain valuable insights, researchers have proposed a series of deep-learning models for various table-based tasks, e.g., table question answering (TQA), table-to-text (T2T), text-to-sql (NL2SQL) and table fact verification (TFV). Recently, the emerging Large Language Models (LLMs) and more powerful Multimodal Large Language Models (MLLMs) have opened up new possibilities for processing the tabular data, i.e., we can use one general model to process diverse tables and fulfill different tabular tasks based on the user natural language instructions. We refer to these LLMs speciallized for tabular tasks as Tabular LLMs
. In this repository, we collect a paper list about recent Tabular (M)LLMs and divide them into the following categories based on their key idea.
Table of Contents:
- Survey of Tabular LLMs and table understanding
- Prompting LLMs for different tabular tasks, e.g., in-context learning, prompt engineering and integrating external tools.
- Training LLMs for better table understanding ability, e.g., training existing LLMs by instruction fine-tuning or post-pretraining.
- Developing agents for processing tabular data, e.g., devolping copilot for processing excel tables.
- Empirical study or benchmarks for evaluating LLMs' table understanding ability, e.g., exploring the influence of various table types or table formats.
- Multimodal table understanding, e.g., training MLLMs to understand diverse table images and textual user requests.
- Table Understanding datasets, e.g., valuable datasets for model training and evaluation.
Task Names and Abbreviations:
Task Names | Abbreviations | Task Descriptions |
---|---|---|
Table Question Answering | TQA | Answering questions based on the table(s), e.g., answer look-up or computation questions about table(s). |
Table-to-Text | Table2Text or T2T | Generate a text based on the table(s), e.g., generate a analysis report given a financial statement. |
Text-to-Table | Text2Table | Generate structured tables based on input text, e.g., generate a statistical table based on the game summary. |
Table Fact Verification | TFV | Judging if a statement is true or false (or not enough evidence) based on the table(s) |
Text-to-SQL | NL2SQL | Generate a SQL statement to answer the user question based on the database schema |
Tabular Mathematical Reasoning | TMR | Solving mathematical reasoning problems based on the table(s), e.g., solve math word problems related to a table |
Table-and-Text Question Answering | TAT-QA | Answering questions based on both table(s) and their related texts, e.g., answer questions given wikipedia tables and their surrounding texts. |
Table Interpretation | TI | Interpreting basic table content and structure information, e.g., column type annotation, entity linking, relation extraction, cell type classification et al. |
Table Augmentation | TA | Augmenting existing tables with new data, e.g., schema augmentation, row population, et al. |
Title | Conference | Date | Pages |
---|---|---|---|
Language Modeling on Tabular Data: A Survey of Foundations, Techniques and Evolution | arxiv | 2024-08-20 | 49 |
Large Language Model for Table Processing: A Survey | arxiv | 2024-02-04 | 9 |
A Survey of Table Reasoning with Large Language Models | arxiv | 2024-02-13 | 9 |
Large Language Models(LLMs) on Tabular Data: Prediction, Generation, and Understanding -- A Survey | arxiv | 2024-03-01 | 41 |
Transformers for Tabular Data Representation: A Survey of Models and Applications | TACL 2023 | 23 | |
Table Pre-training: A Survey on Model Architectures, Pre-training Objectives, and Downstream Tasks | IJCAI 2022 | 2022-01-24 | 15 |
Title | Conference | Date | Task | Code |
---|---|---|---|---|
HYTREL: Hypergraph-enhanced Tabular Data Representation Learning |
NIPS 2023 | 2023-07-14 | TA, TI | Github |
FLAME: A small language model for spreadsheet formulas | AAAI 2024 | 2023-01-31 | Generating Excel Formulas | Github |
Title | Conference | Date | Task | Code |
---|---|---|---|---|
SheetAgent: A Generalist Agent for Spreadsheet Reasoning and Manipulation via Large Language Models | arxiv | 2024-03-06 | Manipulating Excels with LLM | Github |
EHRAgent: Code Empowers Large Language Models for Few-shot Complex Tabular Reasoning on Electronic Health Records |
arxiv | 2024-01-13 | TQA | Github |
InfiAgent-DABench: Evaluating Agents on Data Analysis Tasks |
arxiv | 2024-01-10 | Data Analysis | Github |
DB-GPT: Empowering Database Interactions with Private Large Language Models |
arxiv | 2023-12-29 | Data Analysis | Github |
ReAcTable: Enhancing ReAct for Table Question Answering | arxiv | 2023-10-01 | TQA | |
SheetCopilot: Bringing Software Productivity to the Next Level through Large Language Models |
NIPS 2023 | 2023-05-30 | Manipulating Excels with LLM | Github |
TableGPT: Towards Unifying Tables, Nature Language and Commands into One GPT | arxiv | 2023-07-17 | Manipulating CSV table with LLM |
Title | Conference | Date | Task | Code |
---|---|---|---|---|
PixT3: Pixel-based Table-To-Text Generation |
ACL 2024 | 2023-11-16 | T2T | Github |
TabPedia: Towards Comprehensive Visual Table Understanding with Concept Synergy | arxiv | 2024-06-03 | TQA,TI | |
TableVQA-Bench: A Visual Question Answering Benchmark on Multiple Table Domains |
arxiv | 2024-04-30 | TQA, TFV | Github |
Tables as Texts or Images: Evaluating the Table Reasoning Ability of LLMs and MLLMs | ACL 2024 | 2024-02-19 | TQA,TFV,T2T | |
Multimodal Table Understanding |
ACL 2024 | 2024-02-15 | TQA, TFV, T2T, TI, TAT-QA, TMR | Github |
Title | Conference | Date | Task | Data Volume | Domain | Table Type | Data and Code |
---|---|---|---|---|---|---|---|
ENTRANT: A Large Financial Dataset for Table Understanding | Sci Data | 2024-07-04 | Cell Type Classification, Header Extraction, et al | Millions of tables with cell attributes, as well as positional and hierarchical information | Financial | Flat tables and hierarchical tables | Github |
TableBench: A Comprehensive and Complex Benchmark for Table Question Answering | arxiv | 2024-08-17 | TMR, TFV, Trend Forecasting and Chart Generation | 3681 tables and 20K samples | Collect tables from academic datasets like WTQ and FeTaQA | Flat tables and a small number of hierarchical tables | Github |
DocTabQA: Answering Questions from Long Documents Using Tables | arxiv | 2024-08-21 | Table Generation based on question and document | 300 documents and 1.5k question-table pairs | Financial | Flat tables and hierarchical tables | Github |
For Tasks:
Click tags to check more tools for each tasksFor Jobs:
Alternative AI tools for Awesome-Tabular-LLMs
Similar Open Source Tools
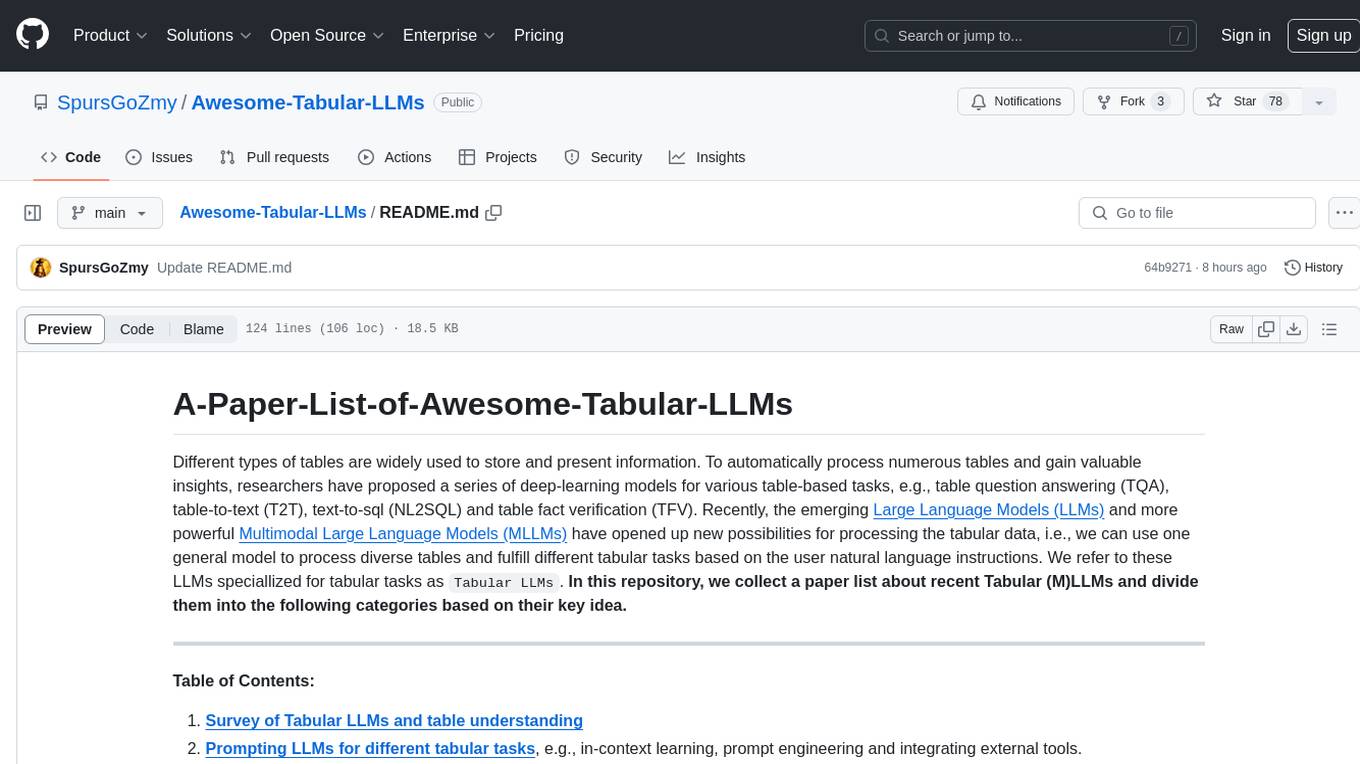
Awesome-Tabular-LLMs
This repository is a collection of papers on Tabular Large Language Models (LLMs) specialized for processing tabular data. It includes surveys, models, and applications related to table understanding tasks such as Table Question Answering, Table-to-Text, Text-to-SQL, and more. The repository categorizes the papers based on key ideas and provides insights into the advancements in using LLMs for processing diverse tables and fulfilling various tabular tasks based on natural language instructions.
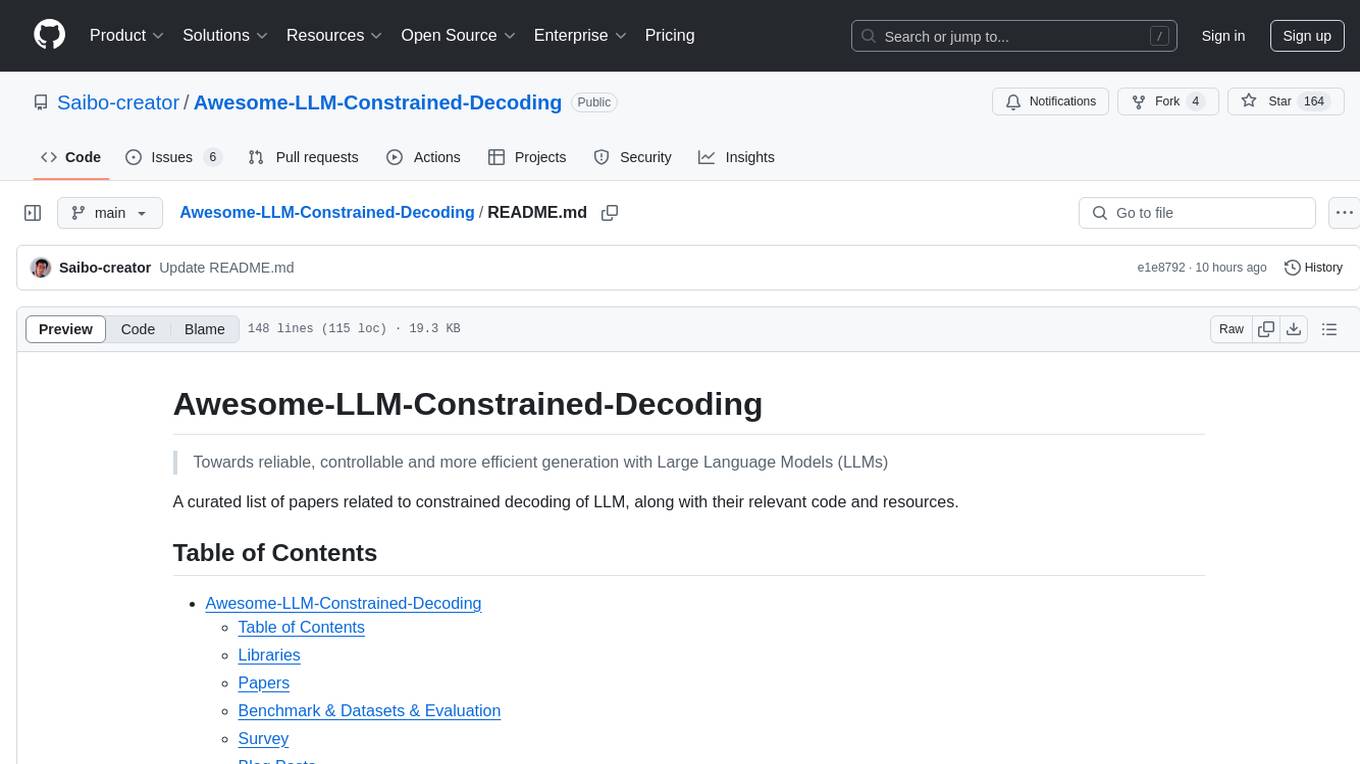
Awesome-LLM-Constrained-Decoding
Awesome-LLM-Constrained-Decoding is a curated list of papers, code, and resources related to constrained decoding of Large Language Models (LLMs). The repository aims to facilitate reliable, controllable, and efficient generation with LLMs by providing a comprehensive collection of materials in this domain.
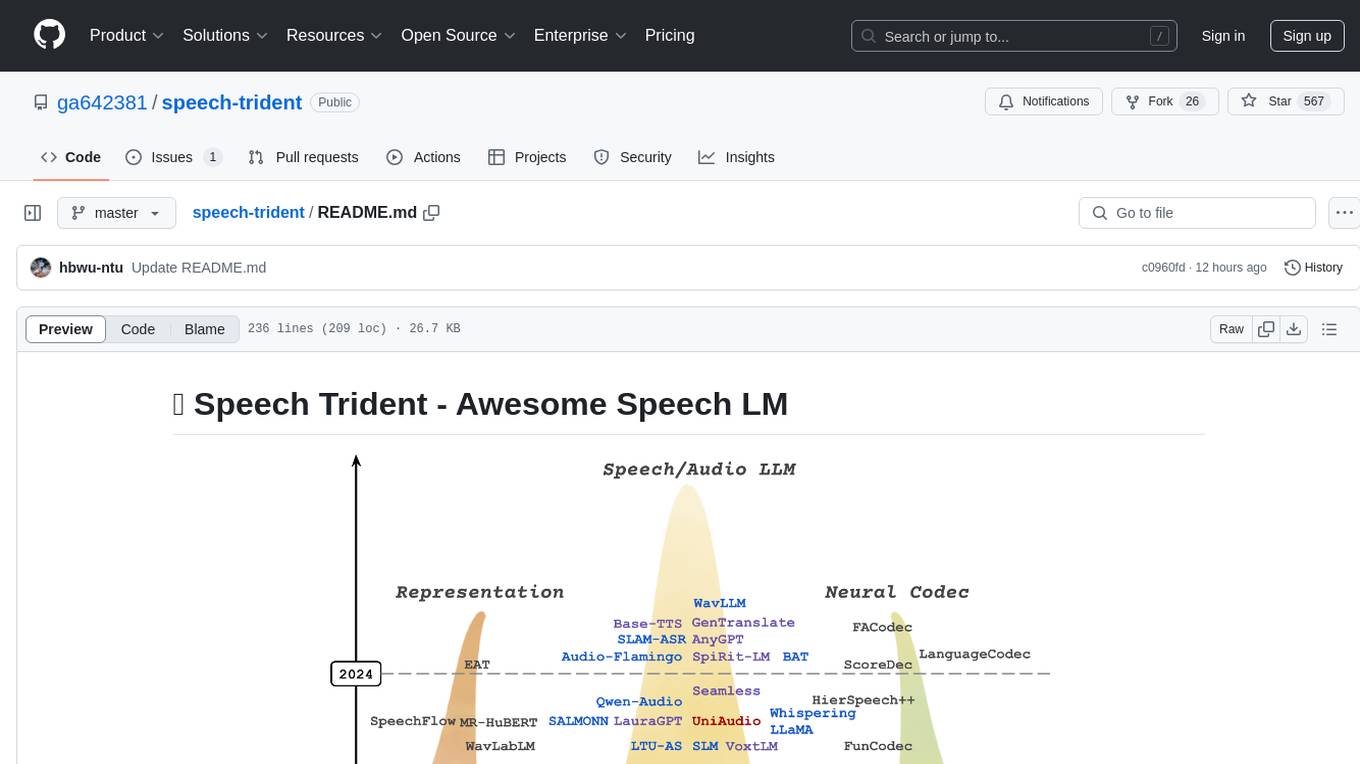
speech-trident
Speech Trident is a repository focusing on speech/audio large language models, covering representation learning, neural codec, and language models. It explores speech representation models, speech neural codec models, and speech large language models. The repository includes contributions from various researchers and provides a comprehensive list of speech/audio language models, representation models, and codec models.
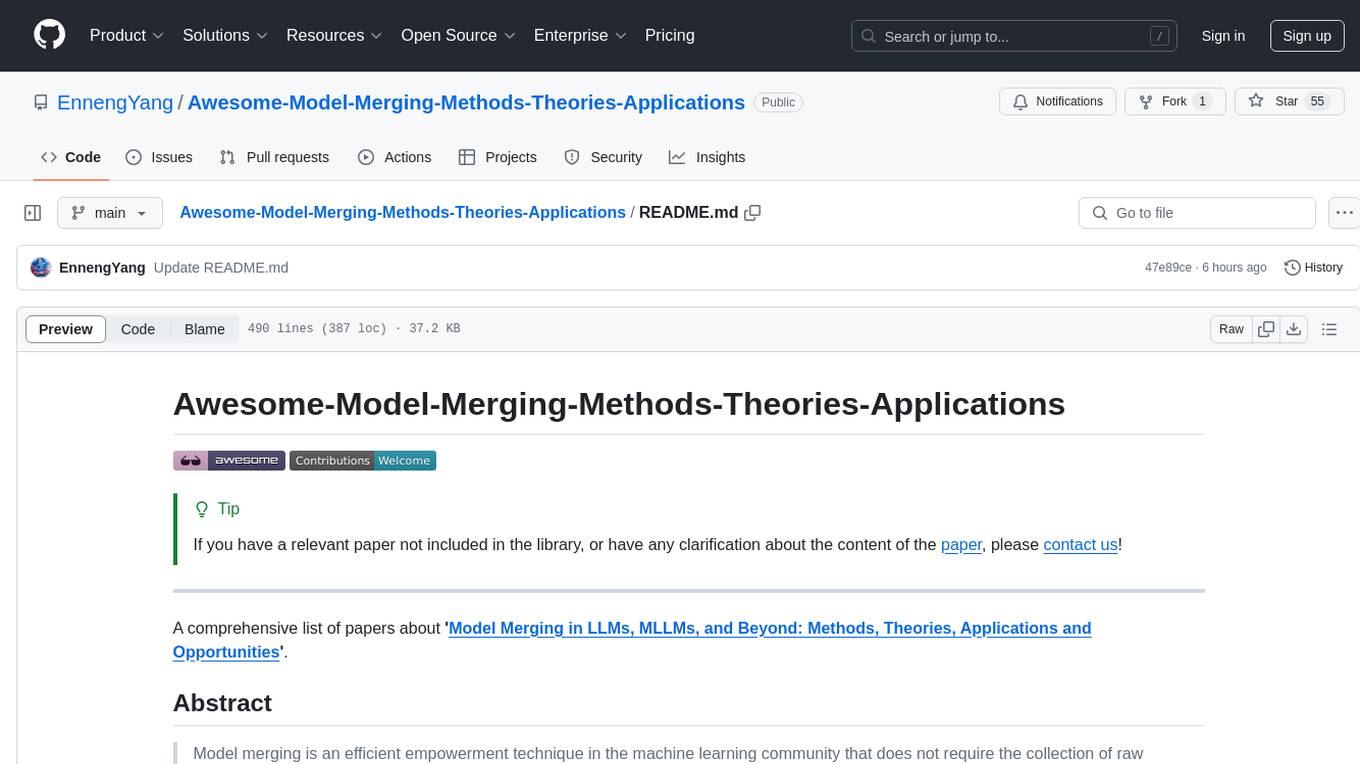
Awesome-Model-Merging-Methods-Theories-Applications
A comprehensive repository focusing on 'Model Merging in LLMs, MLLMs, and Beyond', providing an exhaustive overview of model merging methods, theories, applications, and future research directions. The repository covers various advanced methods, applications in foundation models, different machine learning subfields, and tasks like pre-merging methods, architecture transformation, weight alignment, basic merging methods, and more.
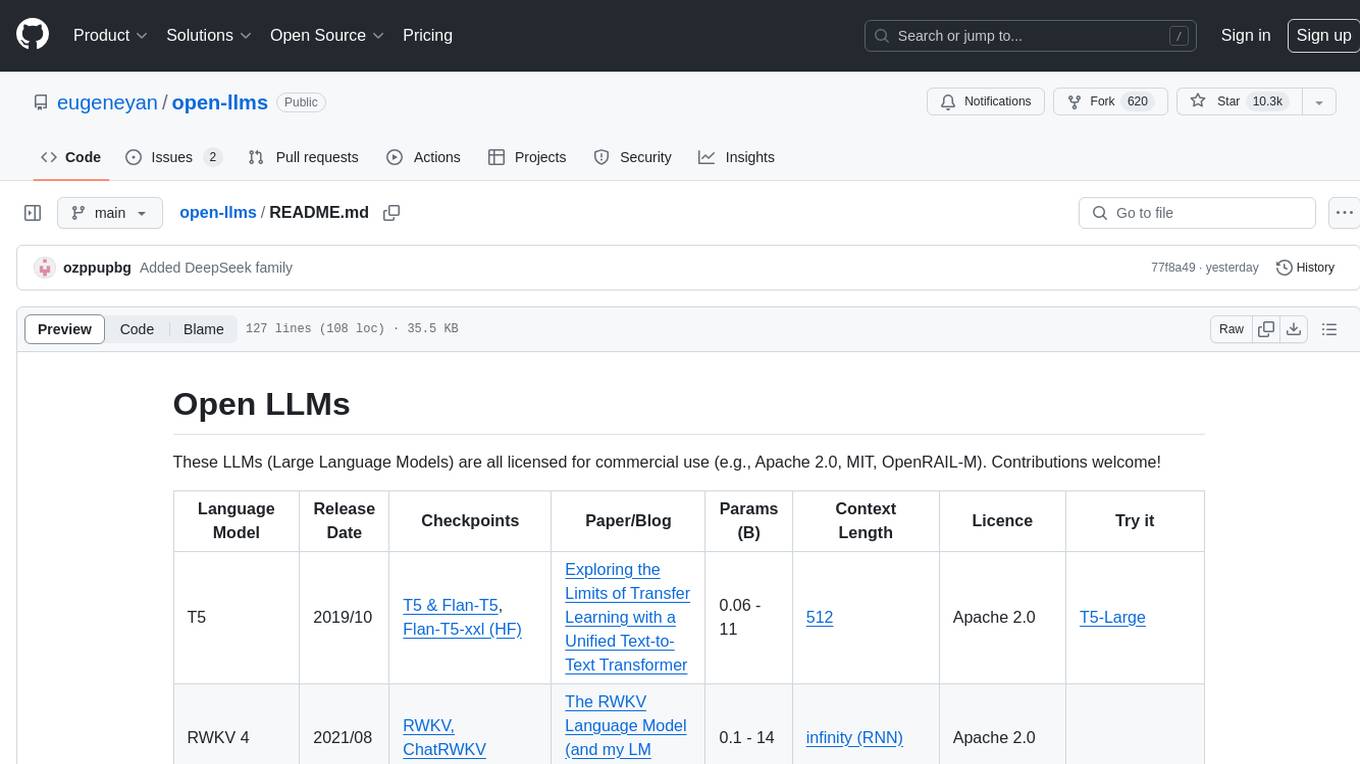
open-llms
Open LLMs is a repository containing various Large Language Models licensed for commercial use. It includes models like T5, GPT-NeoX, UL2, Bloom, Cerebras-GPT, Pythia, Dolly, and more. These models are designed for tasks such as transfer learning, language understanding, chatbot development, code generation, and more. The repository provides information on release dates, checkpoints, papers/blogs, parameters, context length, and licenses for each model. Contributions to the repository are welcome, and it serves as a resource for exploring the capabilities of different language models.
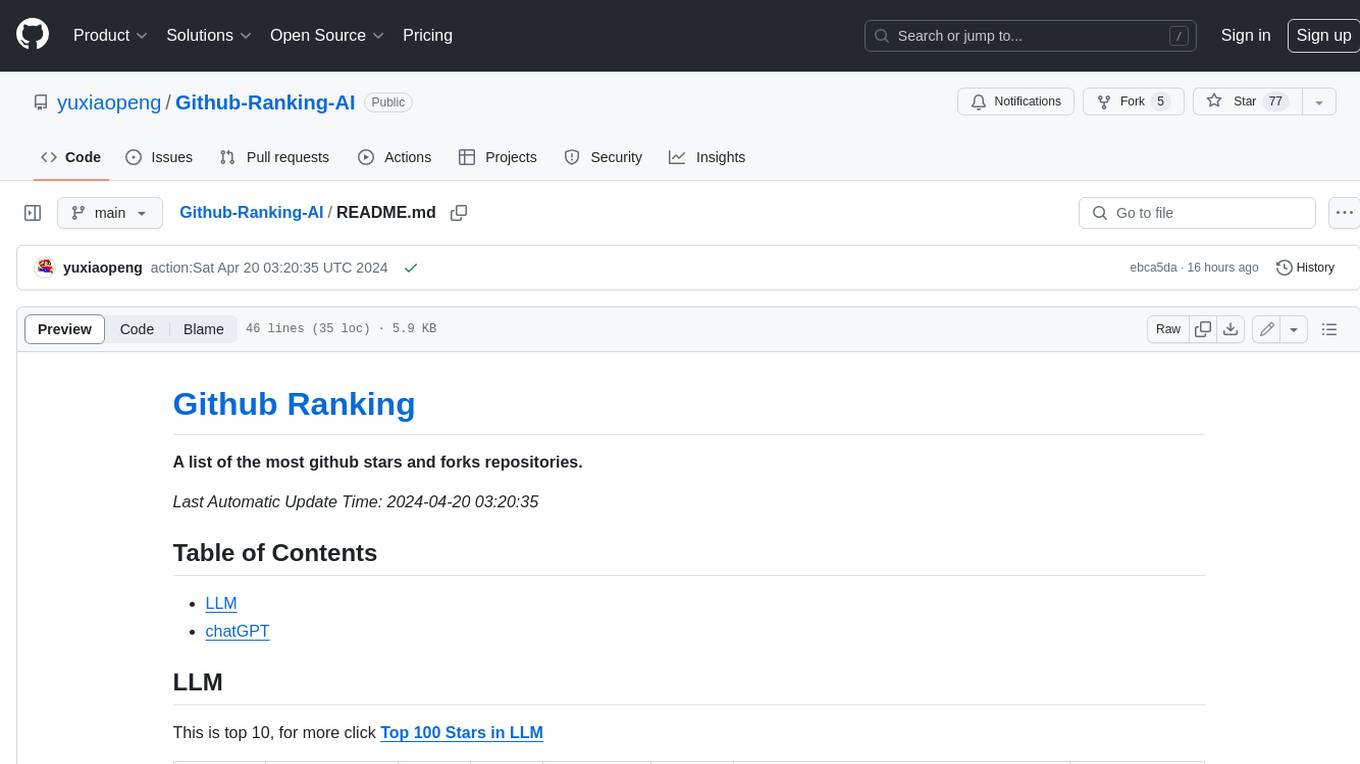
Github-Ranking-AI
This repository provides a list of the most starred and forked repositories on GitHub. It is updated automatically and includes information such as the project name, number of stars, number of forks, language, number of open issues, description, and last commit date. The repository is divided into two sections: LLM and chatGPT. The LLM section includes repositories related to large language models, while the chatGPT section includes repositories related to the chatGPT chatbot.
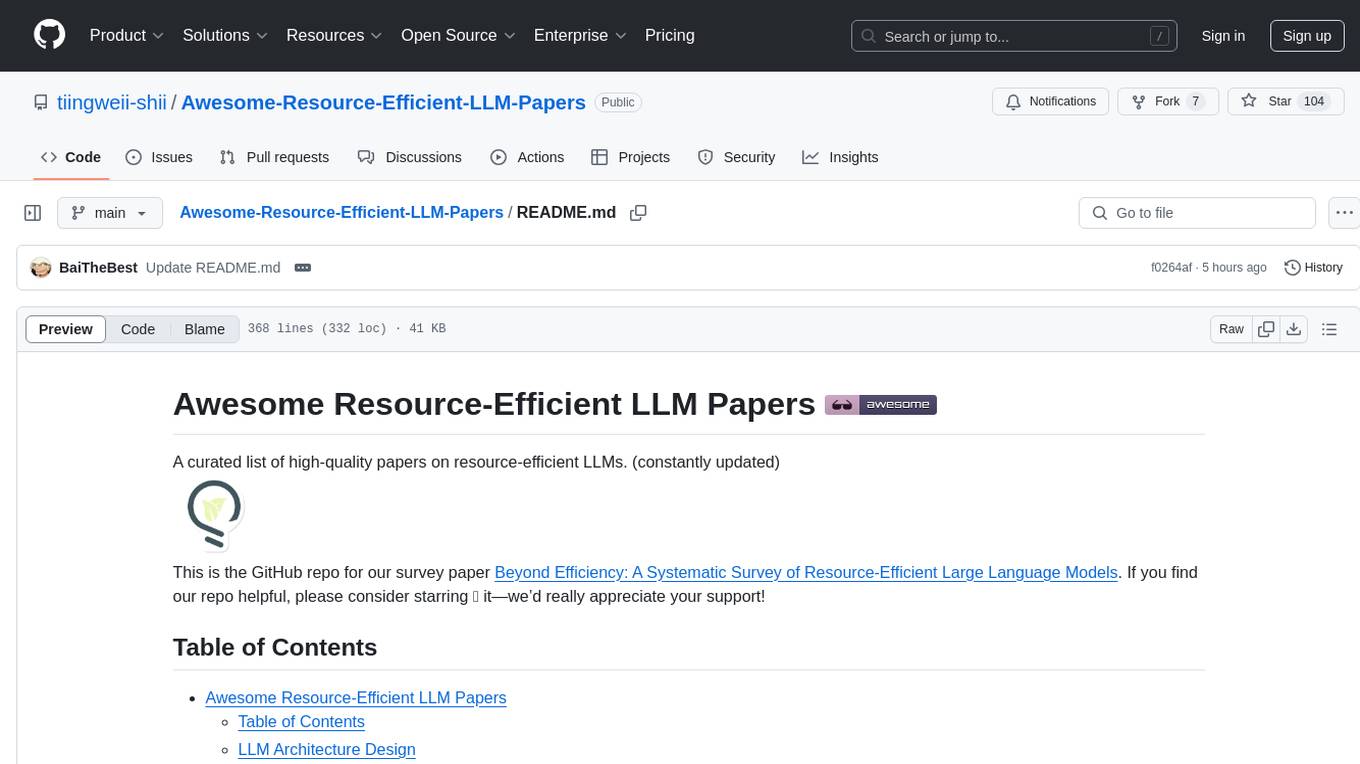
Awesome-Resource-Efficient-LLM-Papers
A curated list of high-quality papers on resource-efficient Large Language Models (LLMs) with a focus on various aspects such as architecture design, pre-training, fine-tuning, inference, system design, and evaluation metrics. The repository covers topics like efficient transformer architectures, non-transformer architectures, memory efficiency, data efficiency, model compression, dynamic acceleration, deployment optimization, support infrastructure, and other related systems. It also provides detailed information on computation metrics, memory metrics, energy metrics, financial cost metrics, network communication metrics, and other metrics relevant to resource-efficient LLMs. The repository includes benchmarks for evaluating the efficiency of NLP models and references for further reading.
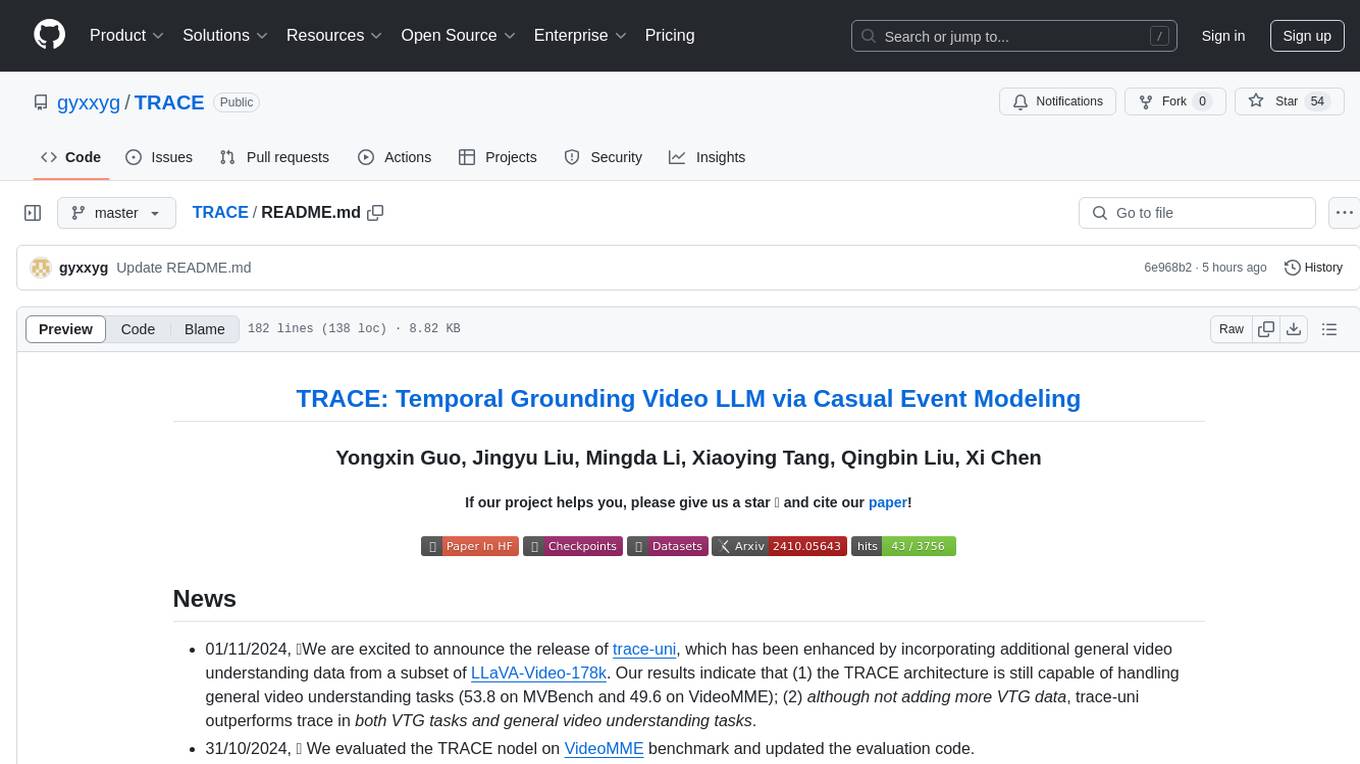
TRACE
TRACE is a temporal grounding video model that utilizes causal event modeling to capture videos' inherent structure. It presents a task-interleaved video LLM model tailored for sequential encoding/decoding of timestamps, salient scores, and textual captions. The project includes various model checkpoints for different stages and fine-tuning on specific datasets. It provides evaluation codes for different tasks like VTG, MVBench, and VideoMME. The repository also offers annotation files and links to raw videos preparation projects. Users can train the model on different tasks and evaluate the performance based on metrics like CIDER, METEOR, SODA_c, F1, mAP, Hit@1, etc. TRACE has been enhanced with trace-retrieval and trace-uni models, showing improved performance on dense video captioning and general video understanding tasks.
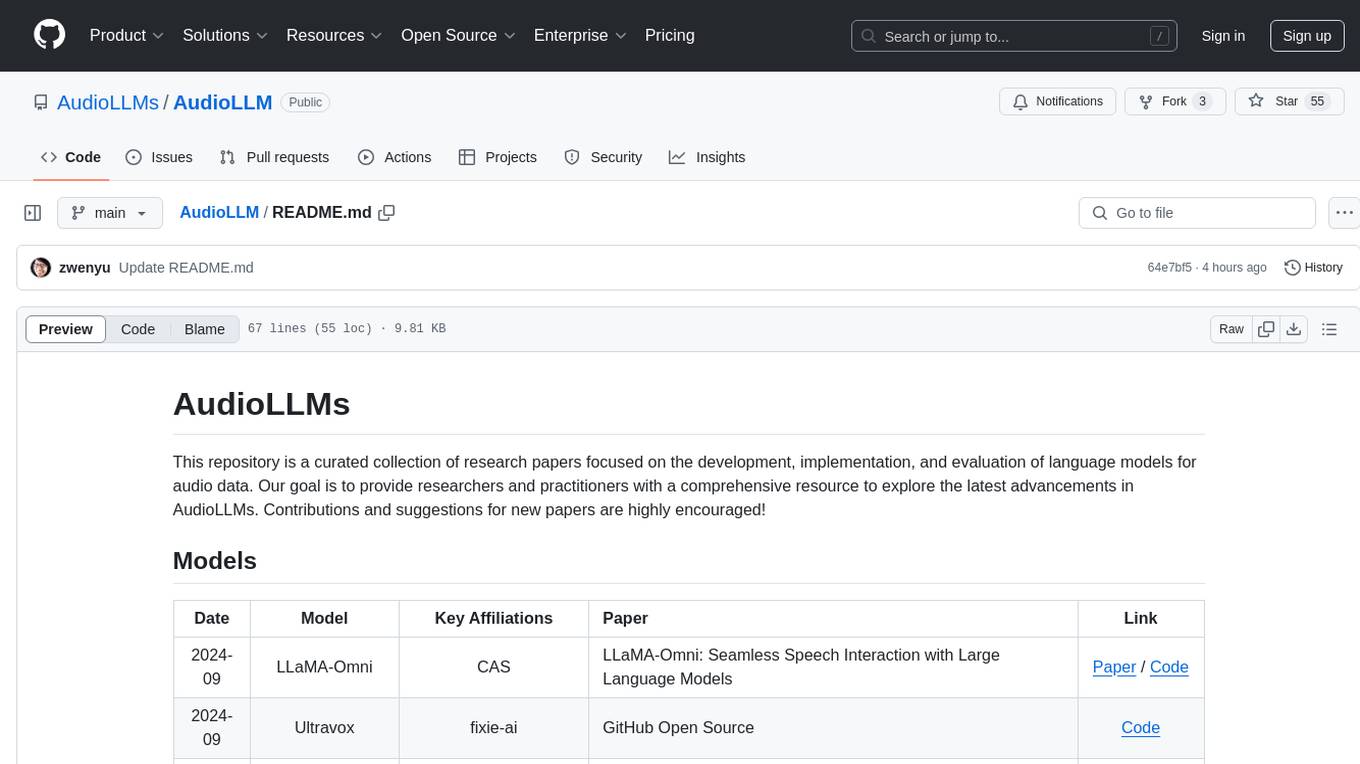
AudioLLM
AudioLLMs is a curated collection of research papers focusing on developing, implementing, and evaluating language models for audio data. The repository aims to provide researchers and practitioners with a comprehensive resource to explore the latest advancements in AudioLLMs. It includes models for speech interaction, speech recognition, speech translation, audio generation, and more. Additionally, it covers methodologies like multitask audioLLMs and segment-level Q-Former, as well as evaluation benchmarks like AudioBench and AIR-Bench. Adversarial attacks such as VoiceJailbreak are also discussed.
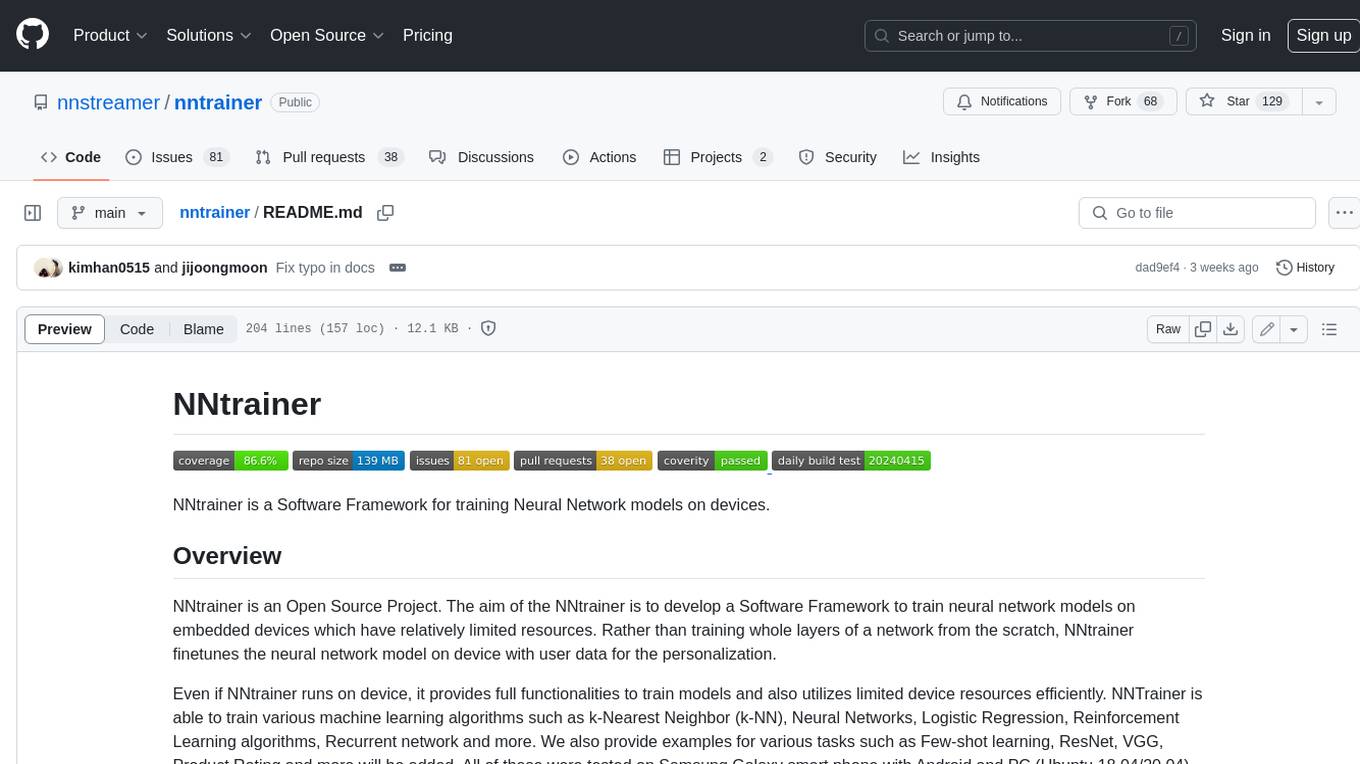
nntrainer
NNtrainer is a software framework for training neural network models on devices with limited resources. It enables on-device fine-tuning of neural networks using user data for personalization. NNtrainer supports various machine learning algorithms and provides examples for tasks such as few-shot learning, ResNet, VGG, and product rating. It is optimized for embedded devices and utilizes CBLAS and CUBLAS for accelerated calculations. NNtrainer is open source and released under the Apache License version 2.0.
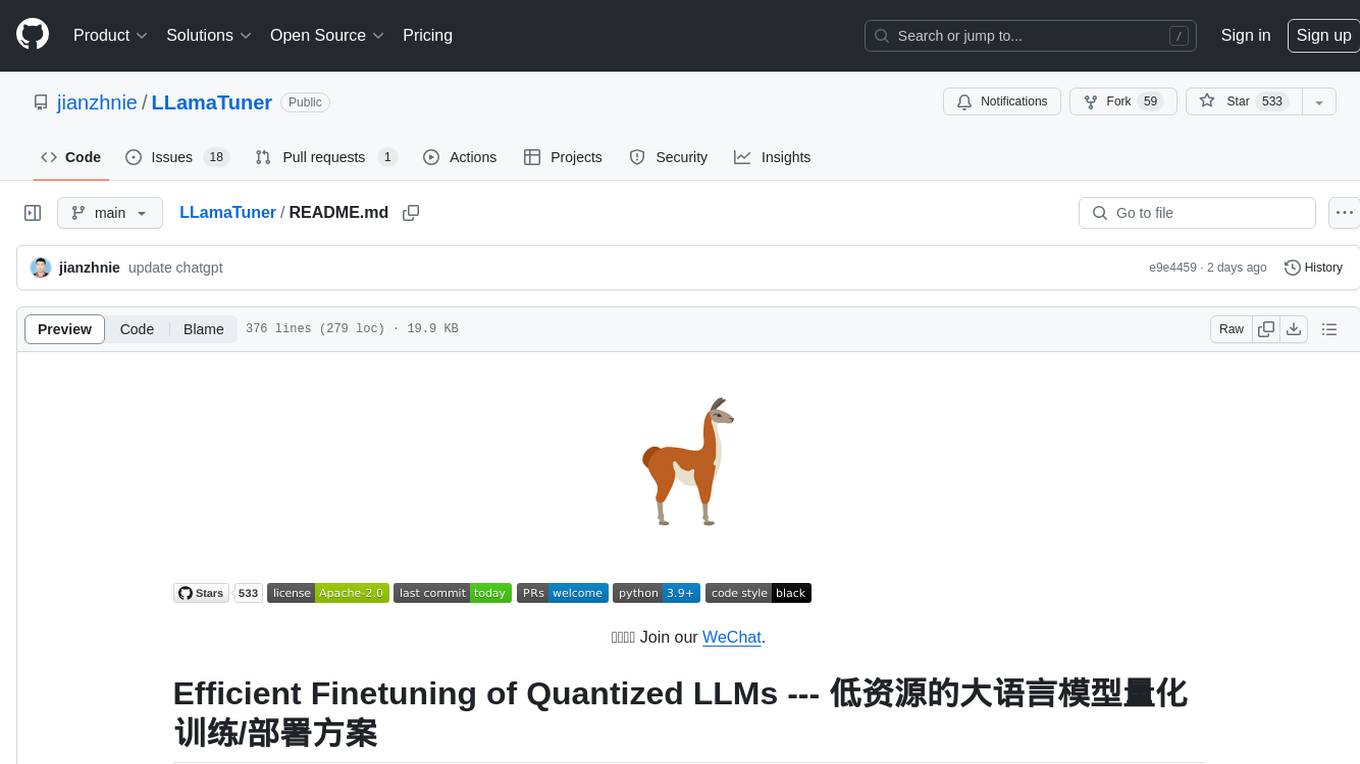
LLamaTuner
LLamaTuner is a repository for the Efficient Finetuning of Quantized LLMs project, focusing on building and sharing instruction-following Chinese baichuan-7b/LLaMA/Pythia/GLM model tuning methods. The project enables training on a single Nvidia RTX-2080TI and RTX-3090 for multi-round chatbot training. It utilizes bitsandbytes for quantization and is integrated with Huggingface's PEFT and transformers libraries. The repository supports various models, training approaches, and datasets for supervised fine-tuning, LoRA, QLoRA, and more. It also provides tools for data preprocessing and offers models in the Hugging Face model hub for inference and finetuning. The project is licensed under Apache 2.0 and acknowledges contributions from various open-source contributors.
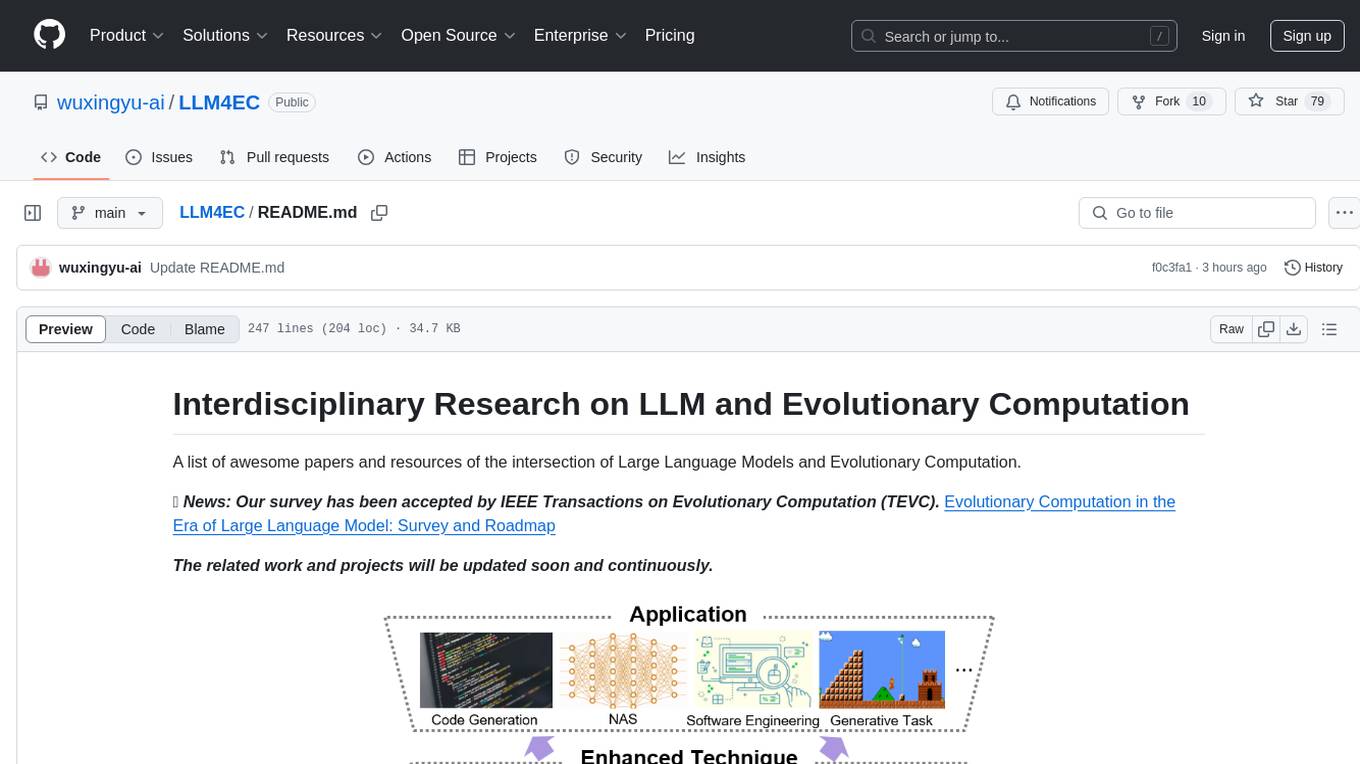
LLM4EC
LLM4EC is an interdisciplinary research repository focusing on the intersection of Large Language Models (LLM) and Evolutionary Computation (EC). It provides a comprehensive collection of papers and resources exploring various applications, enhancements, and synergies between LLM and EC. The repository covers topics such as LLM-assisted optimization, EA-based LLM architecture search, and applications in code generation, software engineering, neural architecture search, and other generative tasks. The goal is to facilitate research and development in leveraging LLM and EC for innovative solutions in diverse domains.
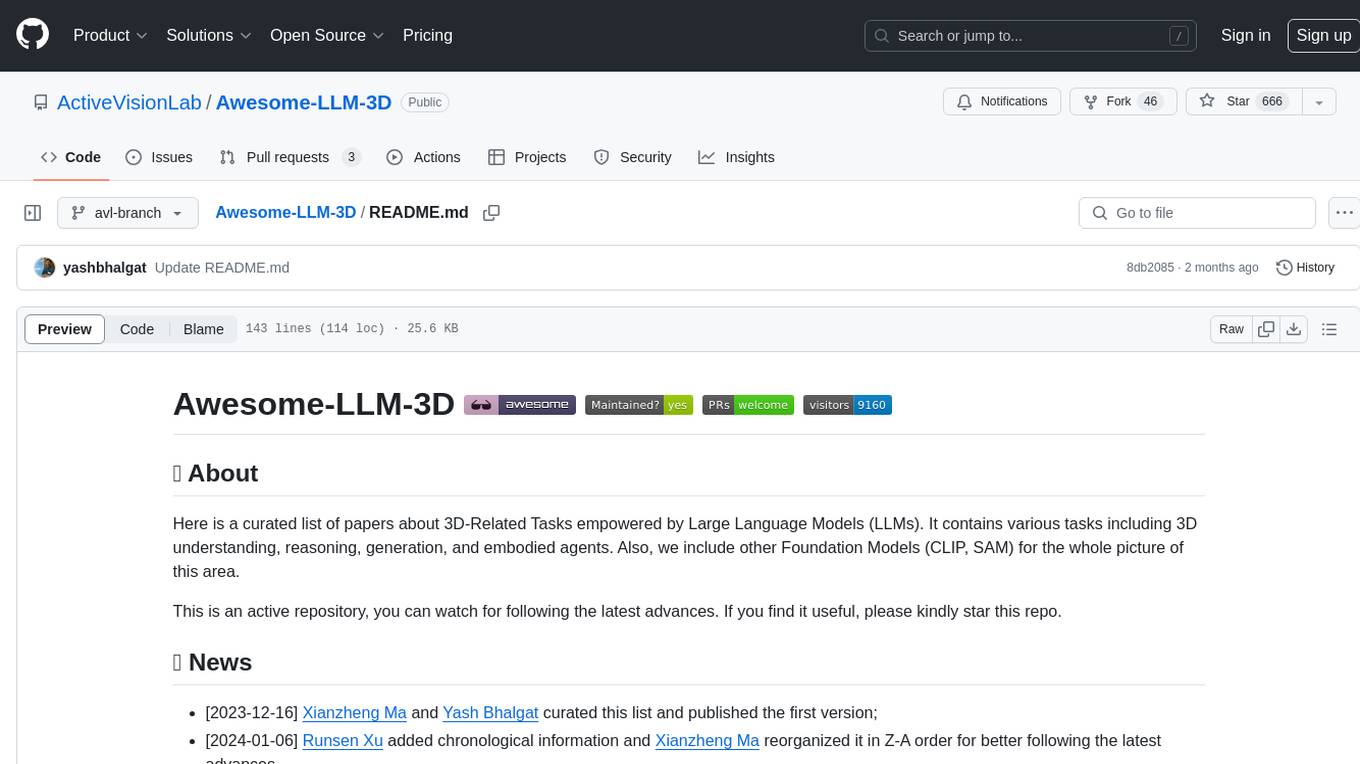
Awesome-LLM-3D
This repository is a curated list of papers related to 3D tasks empowered by Large Language Models (LLMs). It covers tasks such as 3D understanding, reasoning, generation, and embodied agents. The repository also includes other Foundation Models like CLIP and SAM to provide a comprehensive view of the area. It is actively maintained and updated to showcase the latest advances in the field. Users can find a variety of research papers and projects related to 3D tasks and LLMs in this repository.
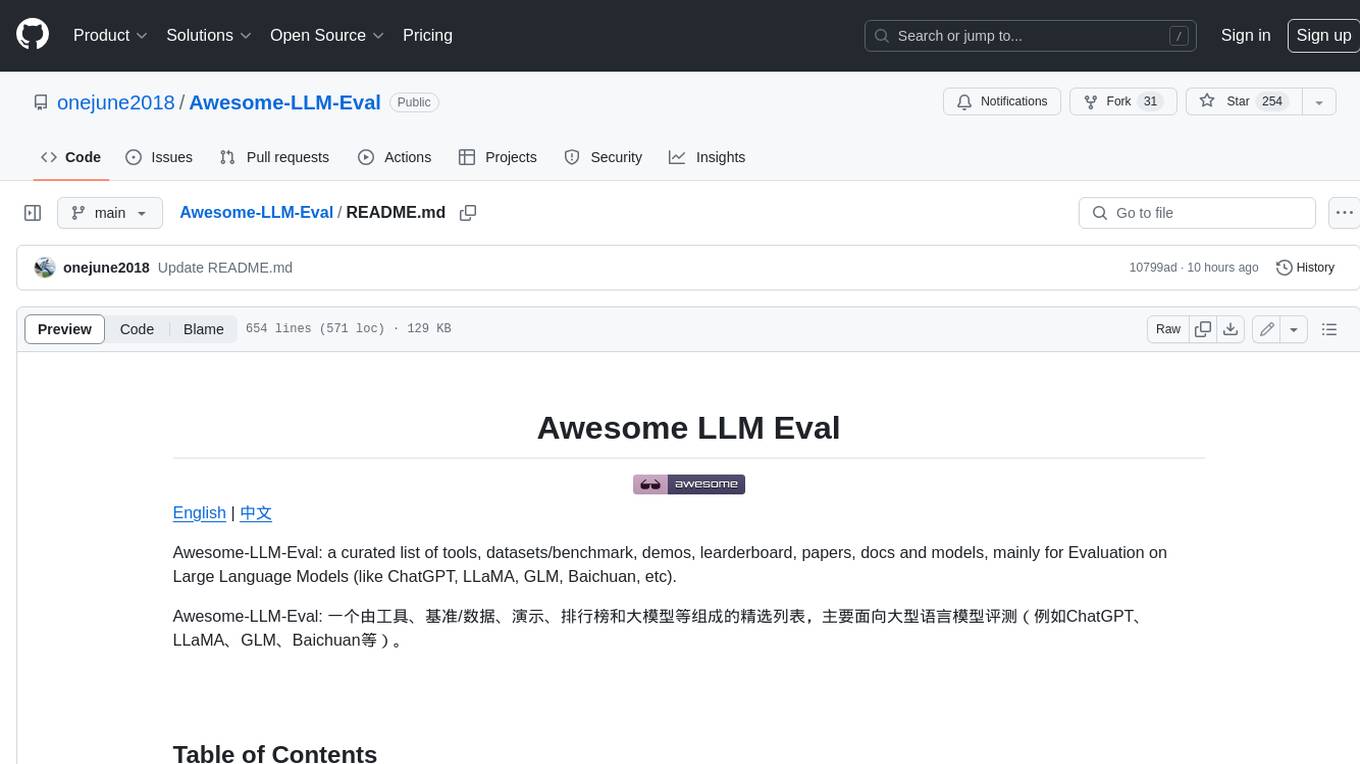
Awesome-LLM-Eval
Awesome-LLM-Eval: a curated list of tools, benchmarks, demos, papers for Large Language Models (like ChatGPT, LLaMA, GLM, Baichuan, etc) Evaluation on Language capabilities, Knowledge, Reasoning, Fairness and Safety.
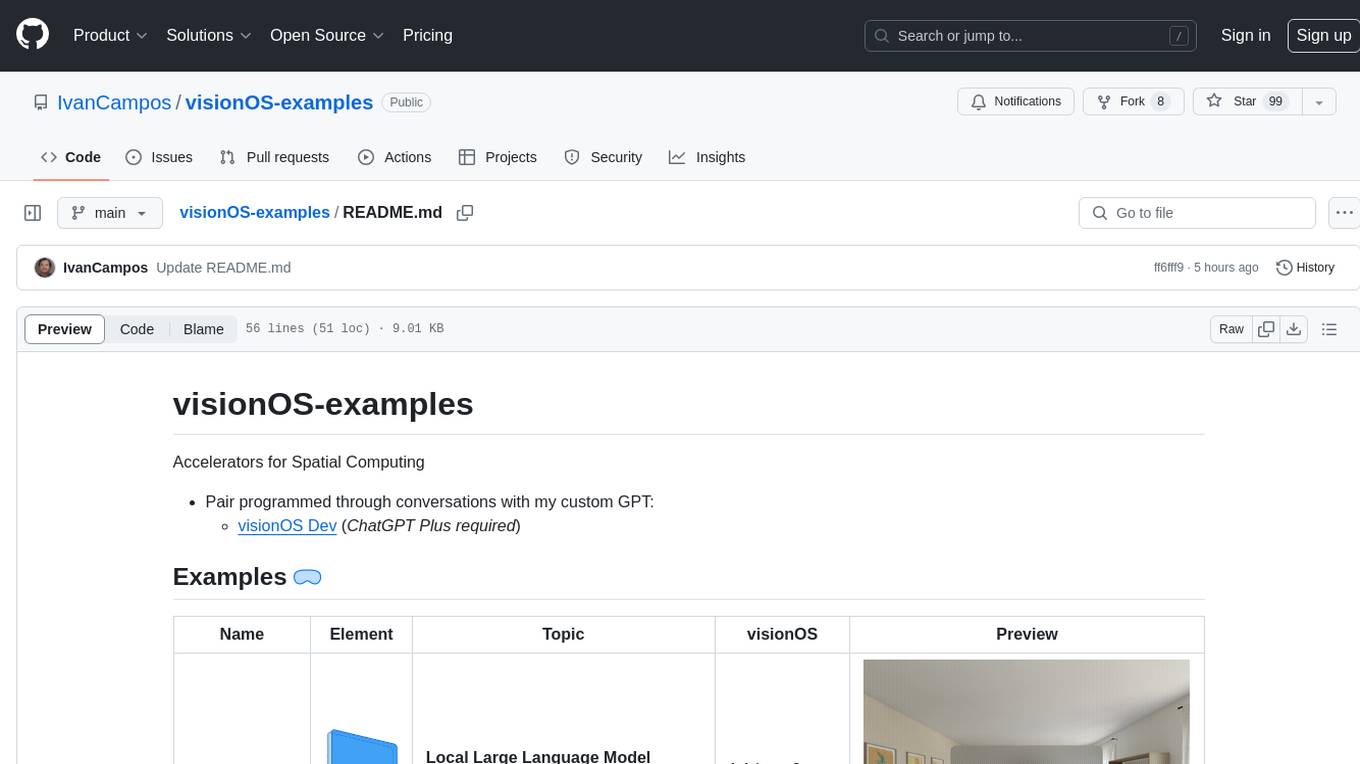
visionOS-examples
visionOS-examples is a repository containing accelerators for Spatial Computing. It includes examples such as Local Large Language Model, Chat Apple Vision Pro, WebSockets, Anchor To Head, Hand Tracking, Battery Life, Countdown, Plane Detection, Timer Vision, and PencilKit for visionOS. The repository showcases various functionalities and features for Apple Vision Pro, offering tools for developers to enhance their visionOS apps with capabilities like hand tracking, plane detection, and real-time cryptocurrency prices.
For similar tasks
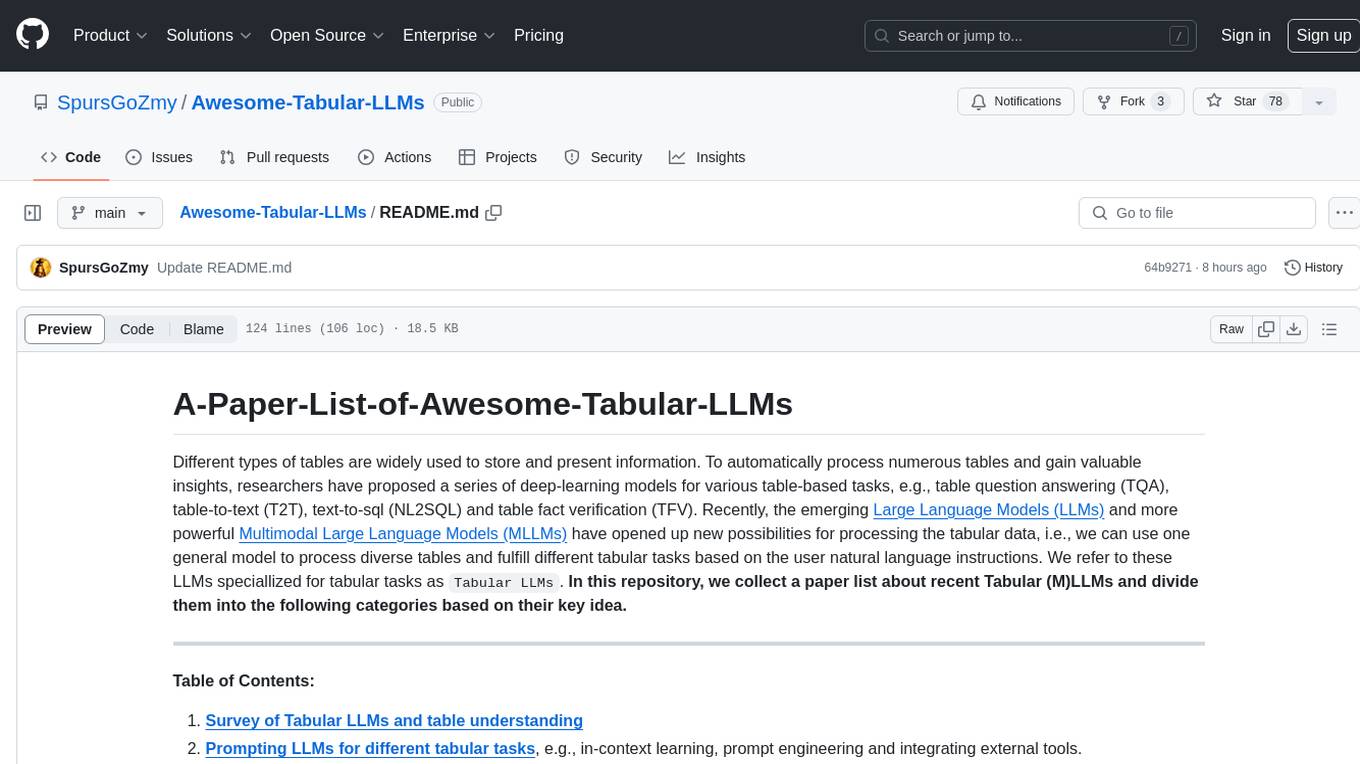
Awesome-Tabular-LLMs
This repository is a collection of papers on Tabular Large Language Models (LLMs) specialized for processing tabular data. It includes surveys, models, and applications related to table understanding tasks such as Table Question Answering, Table-to-Text, Text-to-SQL, and more. The repository categorizes the papers based on key ideas and provides insights into the advancements in using LLMs for processing diverse tables and fulfilling various tabular tasks based on natural language instructions.
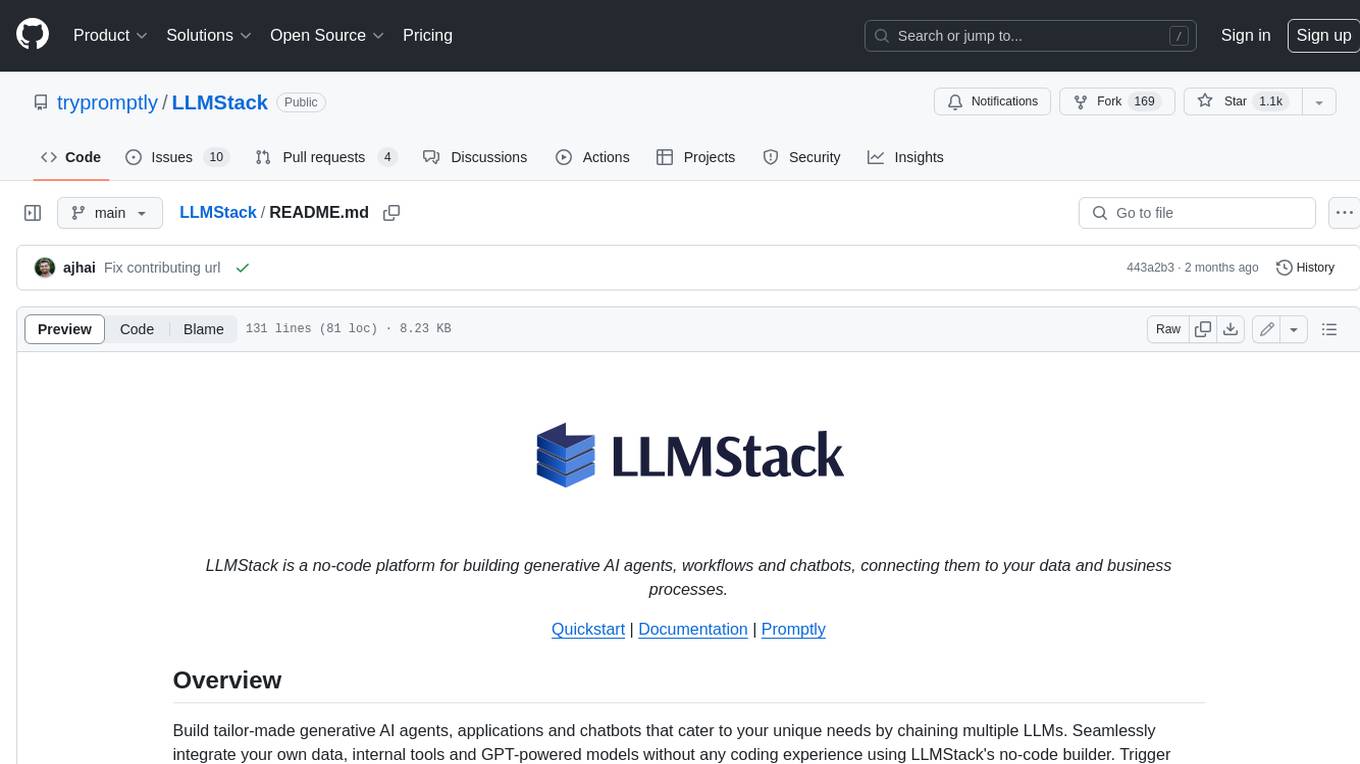
LLMStack
LLMStack is a no-code platform for building generative AI agents, workflows, and chatbots. It allows users to connect their own data, internal tools, and GPT-powered models without any coding experience. LLMStack can be deployed to the cloud or on-premise and can be accessed via HTTP API or triggered from Slack or Discord.
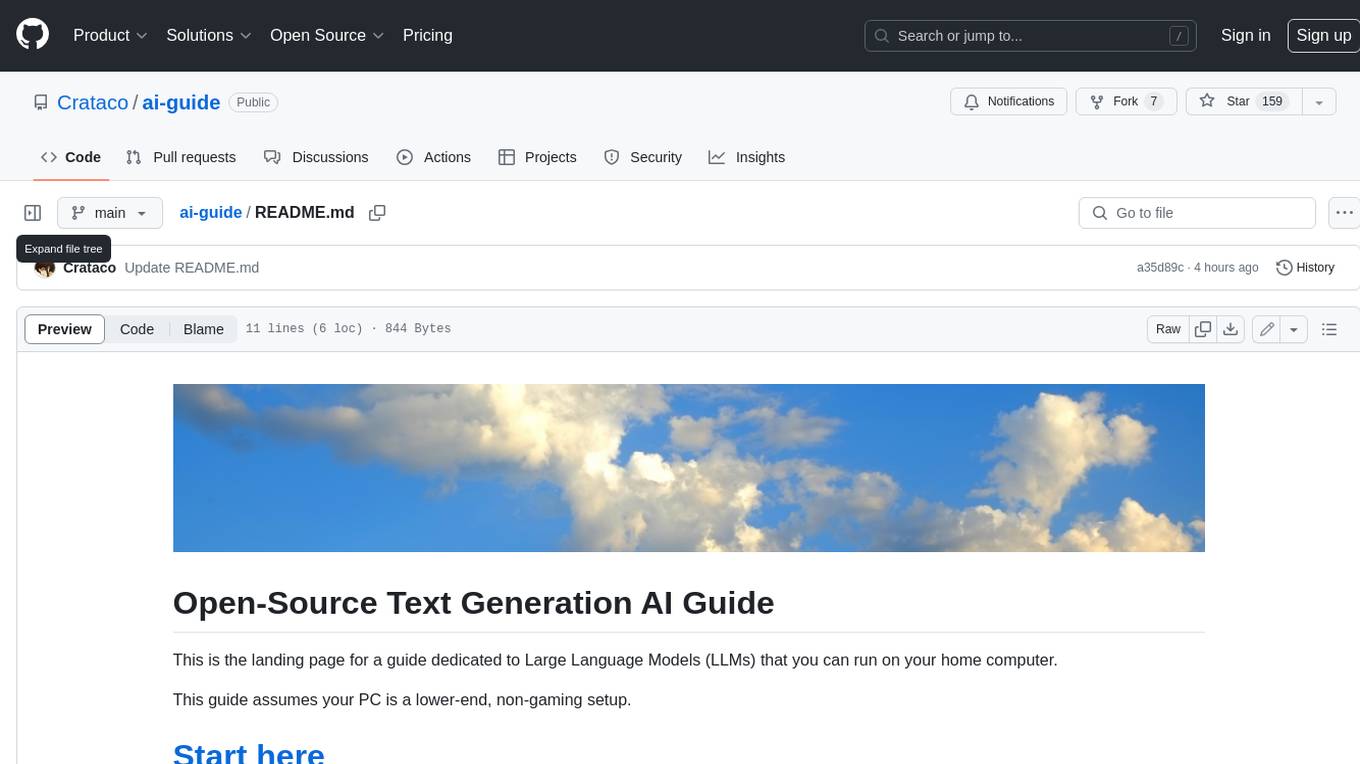
ai-guide
This guide is dedicated to Large Language Models (LLMs) that you can run on your home computer. It assumes your PC is a lower-end, non-gaming setup.
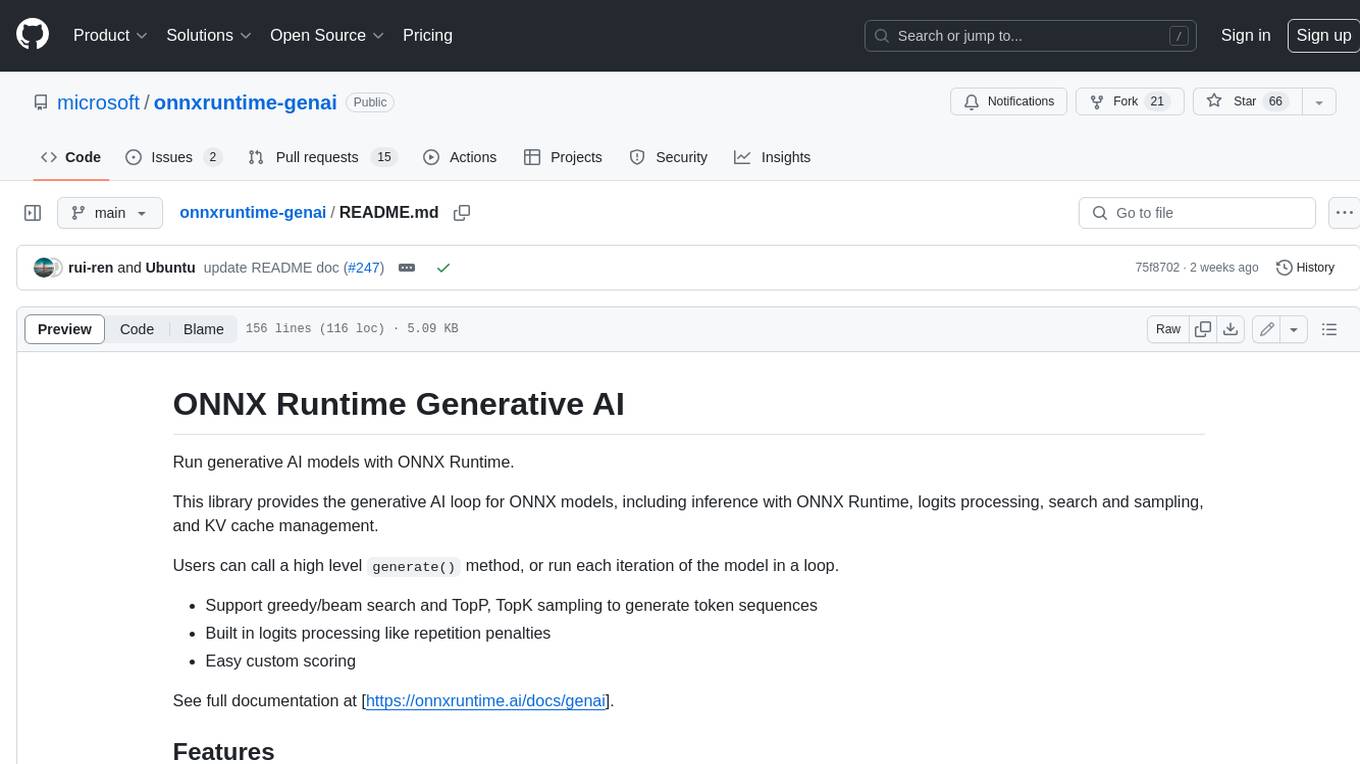
onnxruntime-genai
ONNX Runtime Generative AI is a library that provides the generative AI loop for ONNX models, including inference with ONNX Runtime, logits processing, search and sampling, and KV cache management. Users can call a high level `generate()` method, or run each iteration of the model in a loop. It supports greedy/beam search and TopP, TopK sampling to generate token sequences, has built in logits processing like repetition penalties, and allows for easy custom scoring.
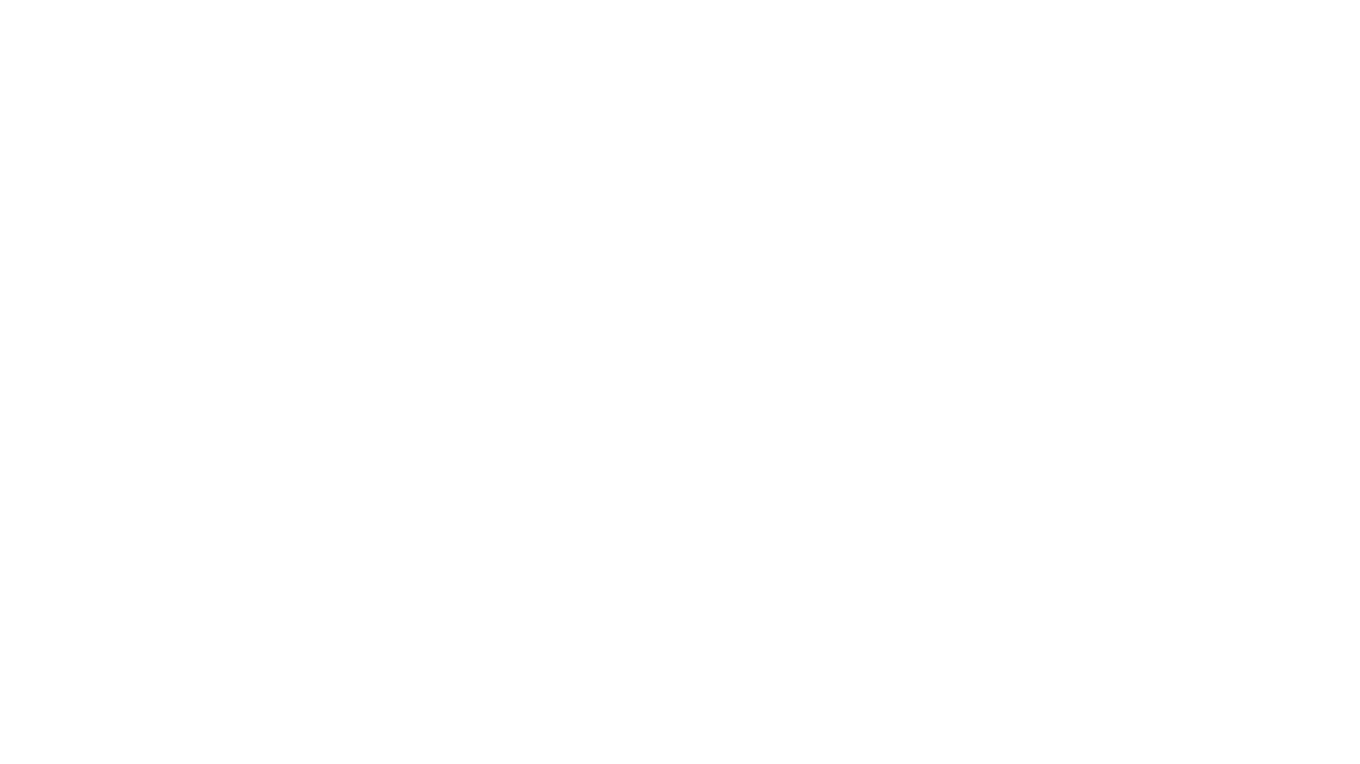
jupyter-ai
Jupyter AI connects generative AI with Jupyter notebooks. It provides a user-friendly and powerful way to explore generative AI models in notebooks and improve your productivity in JupyterLab and the Jupyter Notebook. Specifically, Jupyter AI offers: * An `%%ai` magic that turns the Jupyter notebook into a reproducible generative AI playground. This works anywhere the IPython kernel runs (JupyterLab, Jupyter Notebook, Google Colab, Kaggle, VSCode, etc.). * A native chat UI in JupyterLab that enables you to work with generative AI as a conversational assistant. * Support for a wide range of generative model providers, including AI21, Anthropic, AWS, Cohere, Gemini, Hugging Face, NVIDIA, and OpenAI. * Local model support through GPT4All, enabling use of generative AI models on consumer grade machines with ease and privacy.
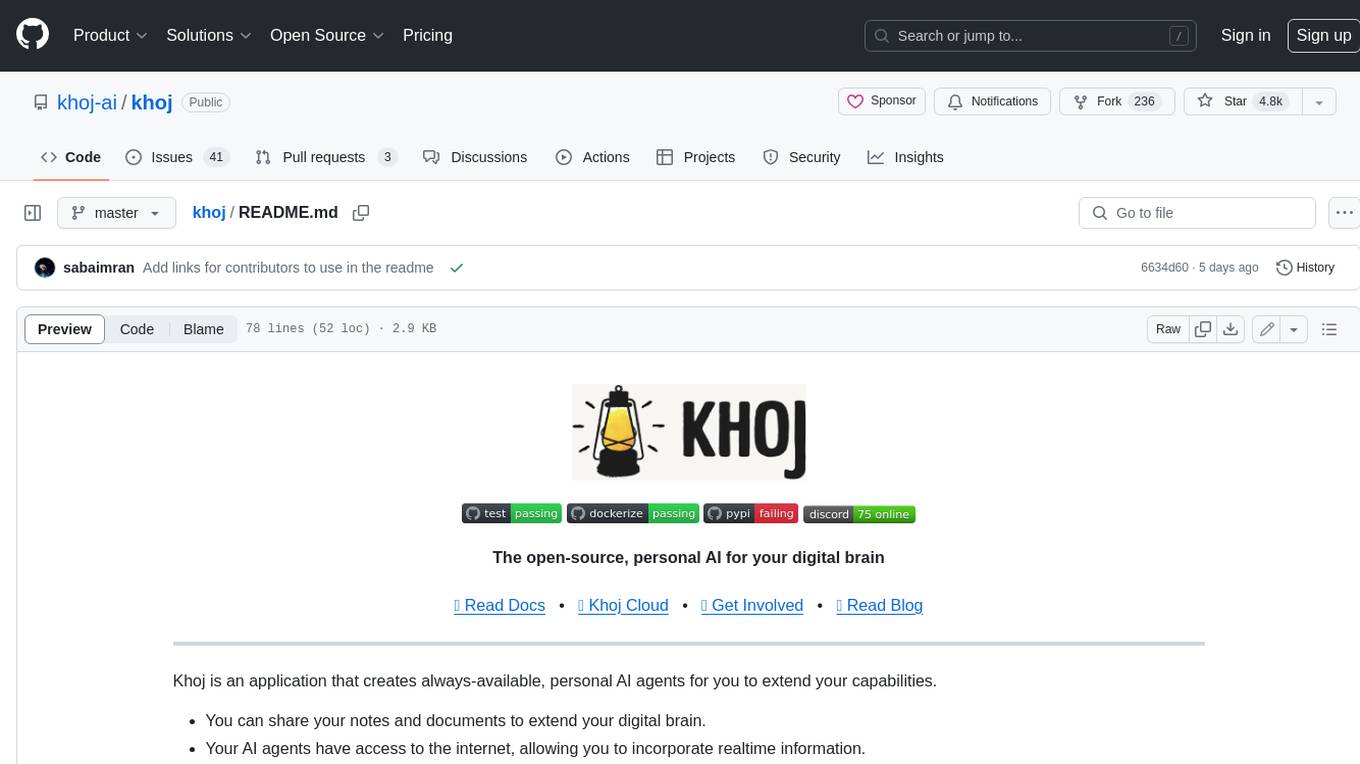
khoj
Khoj is an open-source, personal AI assistant that extends your capabilities by creating always-available AI agents. You can share your notes and documents to extend your digital brain, and your AI agents have access to the internet, allowing you to incorporate real-time information. Khoj is accessible on Desktop, Emacs, Obsidian, Web, and Whatsapp, and you can share PDF, markdown, org-mode, notion files, and GitHub repositories. You'll get fast, accurate semantic search on top of your docs, and your agents can create deeply personal images and understand your speech. Khoj is self-hostable and always will be.
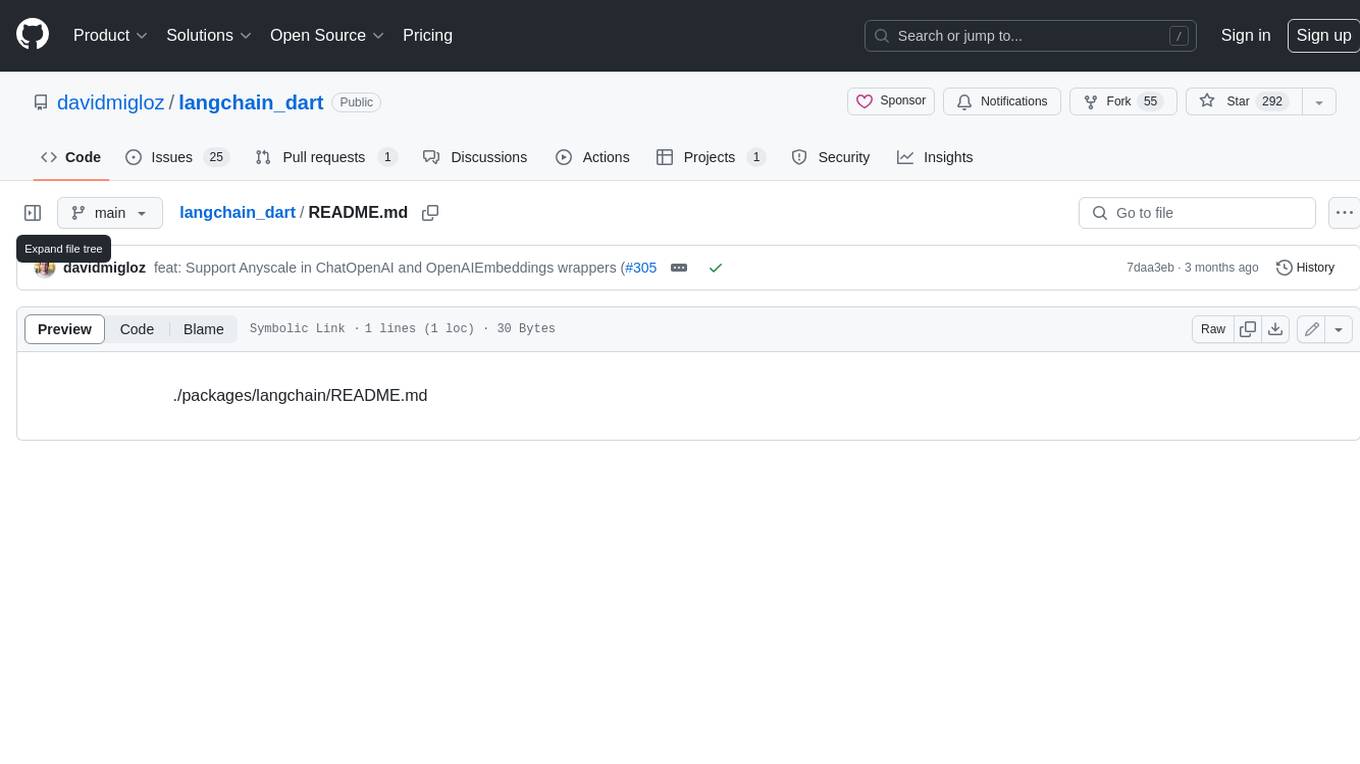
langchain_dart
LangChain.dart is a Dart port of the popular LangChain Python framework created by Harrison Chase. LangChain provides a set of ready-to-use components for working with language models and a standard interface for chaining them together to formulate more advanced use cases (e.g. chatbots, Q&A with RAG, agents, summarization, extraction, etc.). The components can be grouped into a few core modules: * **Model I/O:** LangChain offers a unified API for interacting with various LLM providers (e.g. OpenAI, Google, Mistral, Ollama, etc.), allowing developers to switch between them with ease. Additionally, it provides tools for managing model inputs (prompt templates and example selectors) and parsing the resulting model outputs (output parsers). * **Retrieval:** assists in loading user data (via document loaders), transforming it (with text splitters), extracting its meaning (using embedding models), storing (in vector stores) and retrieving it (through retrievers) so that it can be used to ground the model's responses (i.e. Retrieval-Augmented Generation or RAG). * **Agents:** "bots" that leverage LLMs to make informed decisions about which available tools (such as web search, calculators, database lookup, etc.) to use to accomplish the designated task. The different components can be composed together using the LangChain Expression Language (LCEL).
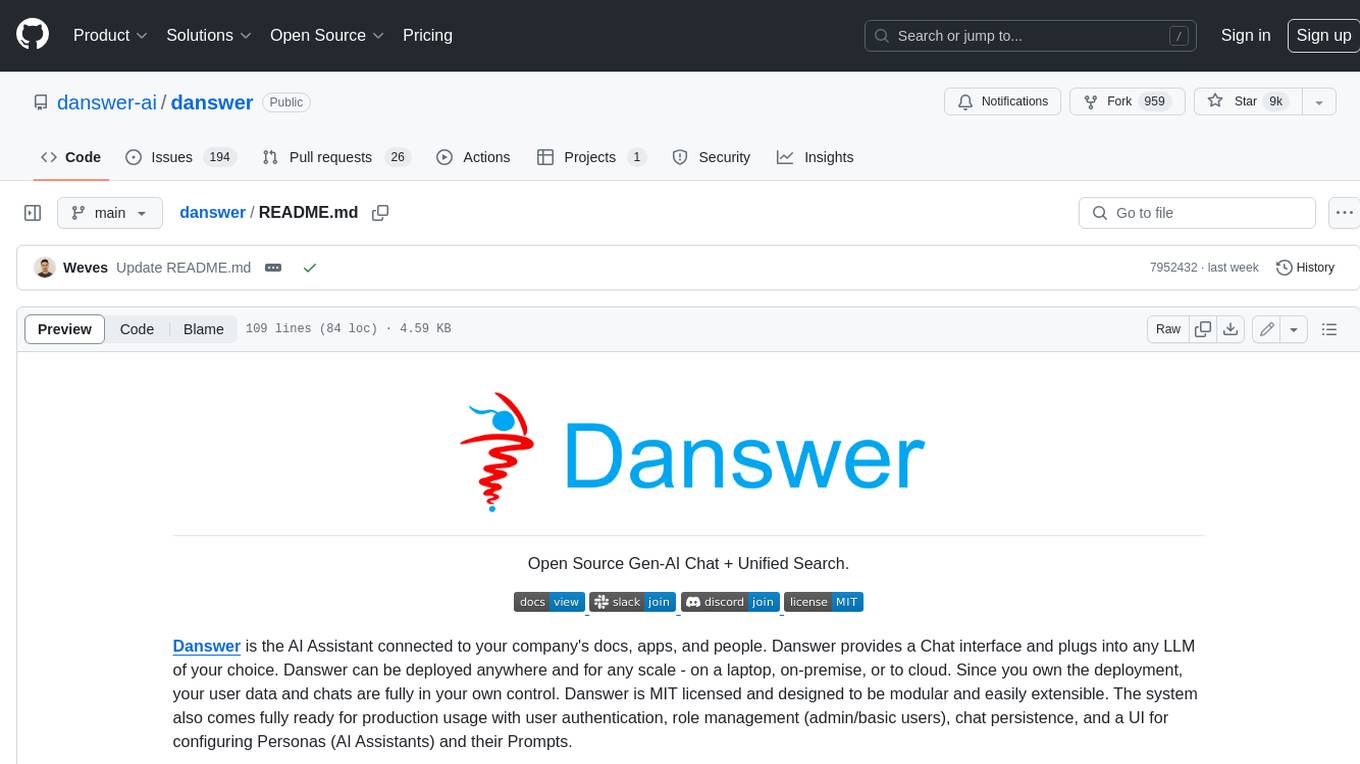
danswer
Danswer is an open-source Gen-AI Chat and Unified Search tool that connects to your company's docs, apps, and people. It provides a Chat interface and plugs into any LLM of your choice. Danswer can be deployed anywhere and for any scale - on a laptop, on-premise, or to cloud. Since you own the deployment, your user data and chats are fully in your own control. Danswer is MIT licensed and designed to be modular and easily extensible. The system also comes fully ready for production usage with user authentication, role management (admin/basic users), chat persistence, and a UI for configuring Personas (AI Assistants) and their Prompts. Danswer also serves as a Unified Search across all common workplace tools such as Slack, Google Drive, Confluence, etc. By combining LLMs and team specific knowledge, Danswer becomes a subject matter expert for the team. Imagine ChatGPT if it had access to your team's unique knowledge! It enables questions such as "A customer wants feature X, is this already supported?" or "Where's the pull request for feature Y?"
For similar jobs
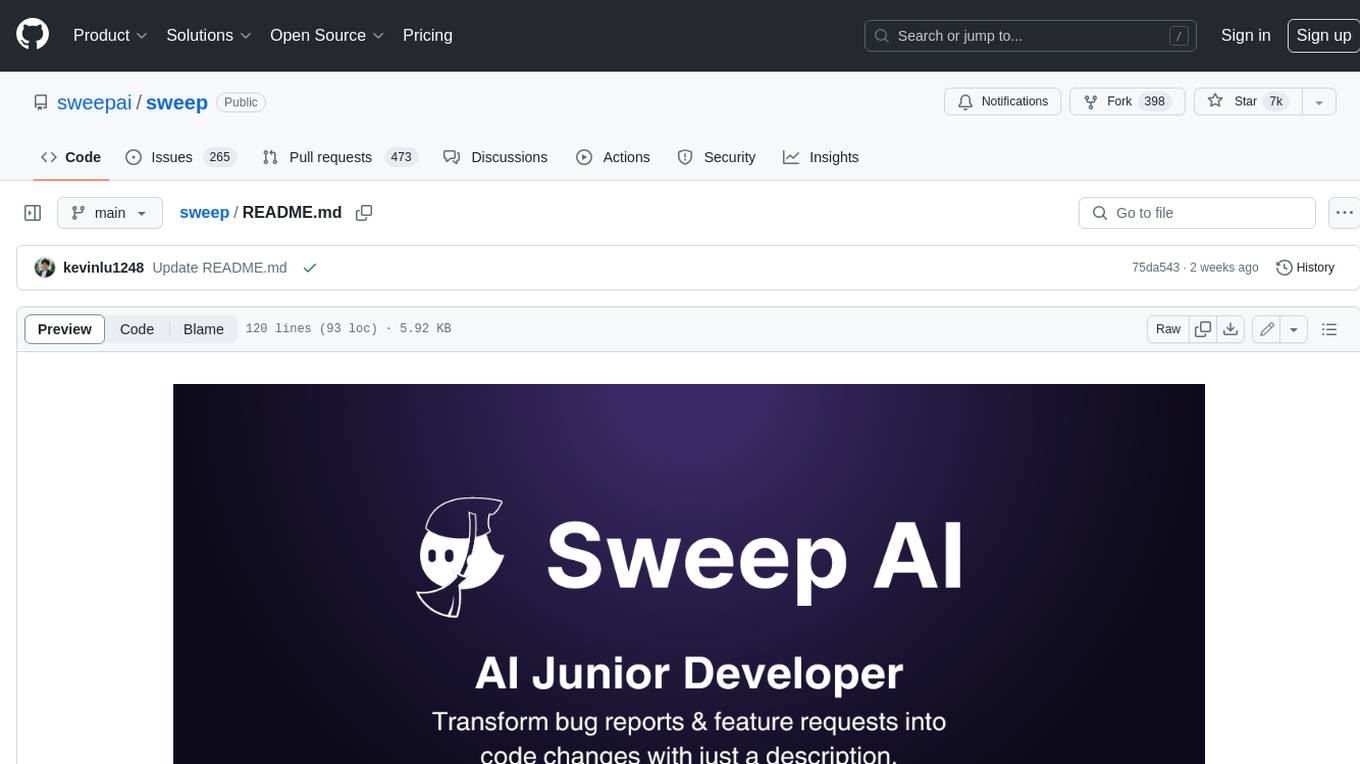
sweep
Sweep is an AI junior developer that turns bugs and feature requests into code changes. It automatically handles developer experience improvements like adding type hints and improving test coverage.
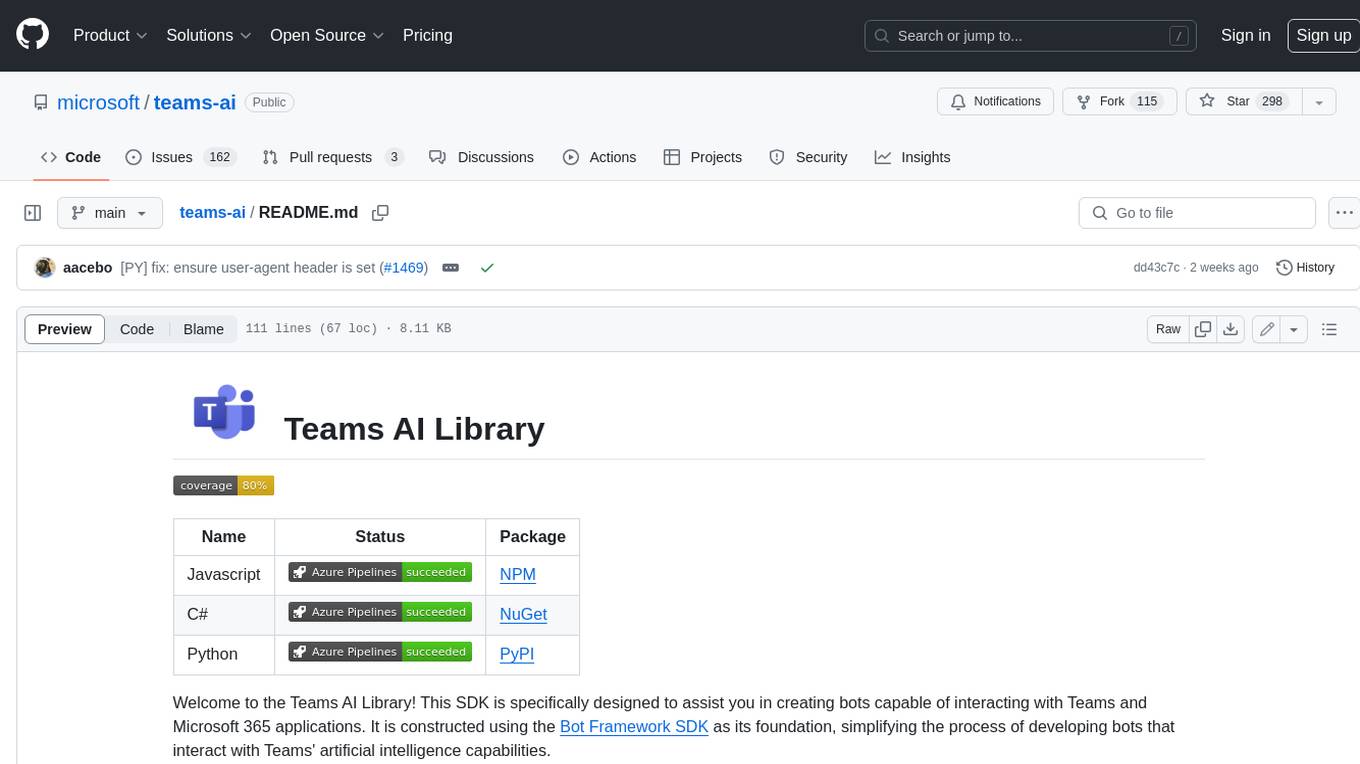
teams-ai
The Teams AI Library is a software development kit (SDK) that helps developers create bots that can interact with Teams and Microsoft 365 applications. It is built on top of the Bot Framework SDK and simplifies the process of developing bots that interact with Teams' artificial intelligence capabilities. The SDK is available for JavaScript/TypeScript, .NET, and Python.
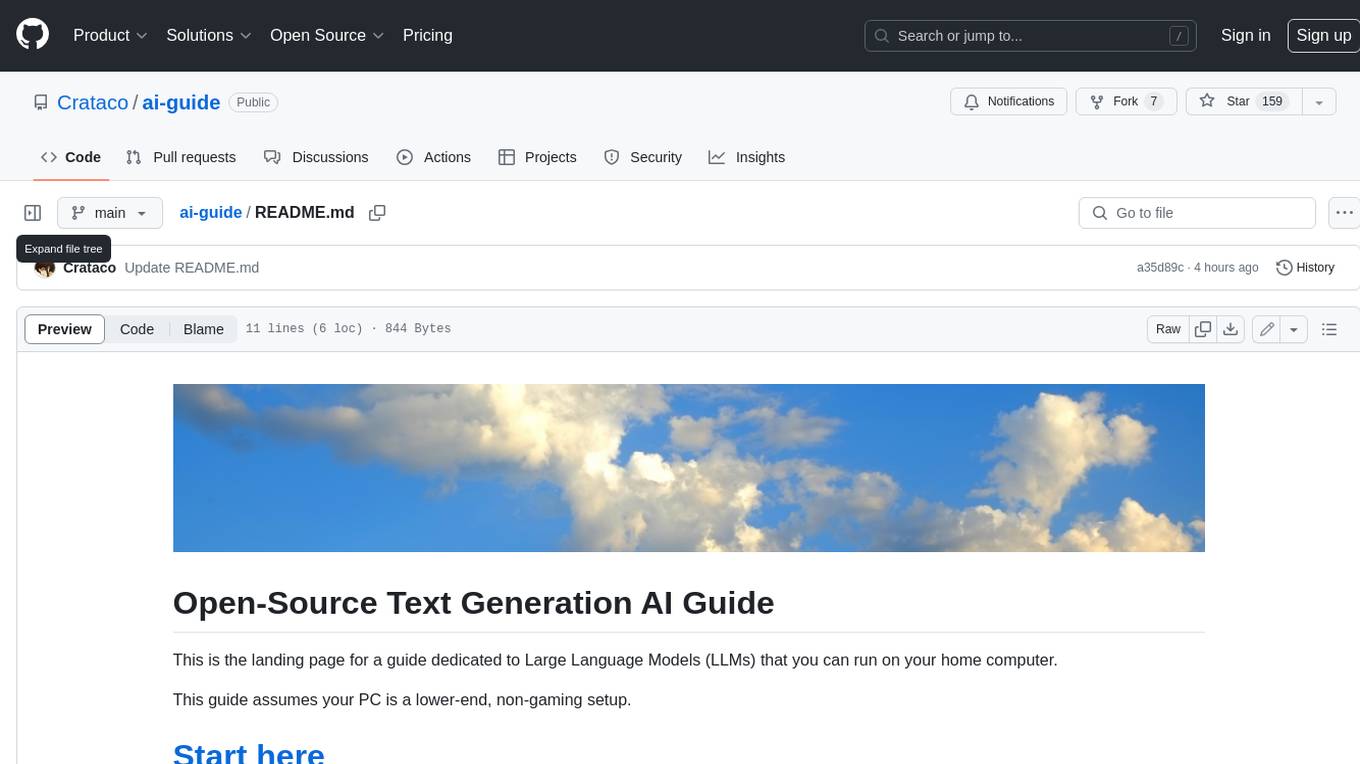
ai-guide
This guide is dedicated to Large Language Models (LLMs) that you can run on your home computer. It assumes your PC is a lower-end, non-gaming setup.
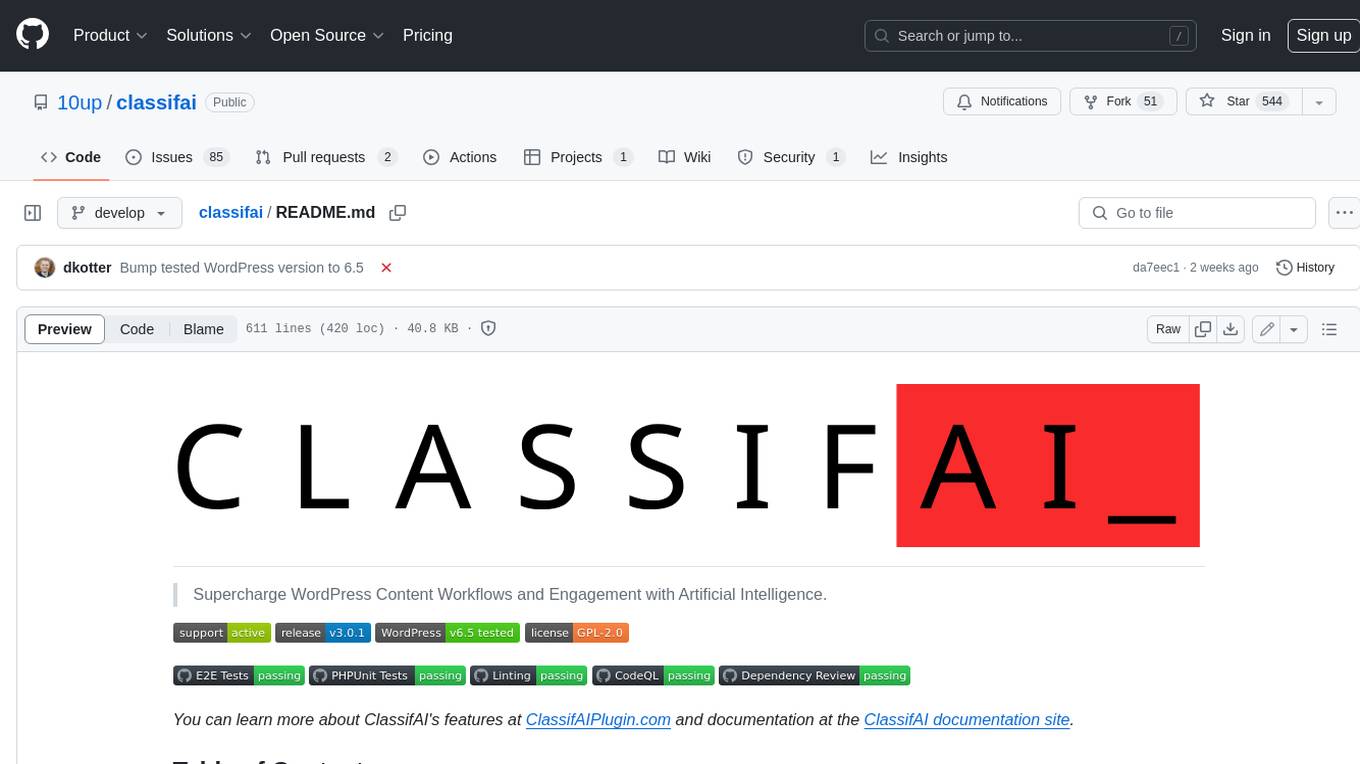
classifai
Supercharge WordPress Content Workflows and Engagement with Artificial Intelligence. Tap into leading cloud-based services like OpenAI, Microsoft Azure AI, Google Gemini and IBM Watson to augment your WordPress-powered websites. Publish content faster while improving SEO performance and increasing audience engagement. ClassifAI integrates Artificial Intelligence and Machine Learning technologies to lighten your workload and eliminate tedious tasks, giving you more time to create original content that matters.
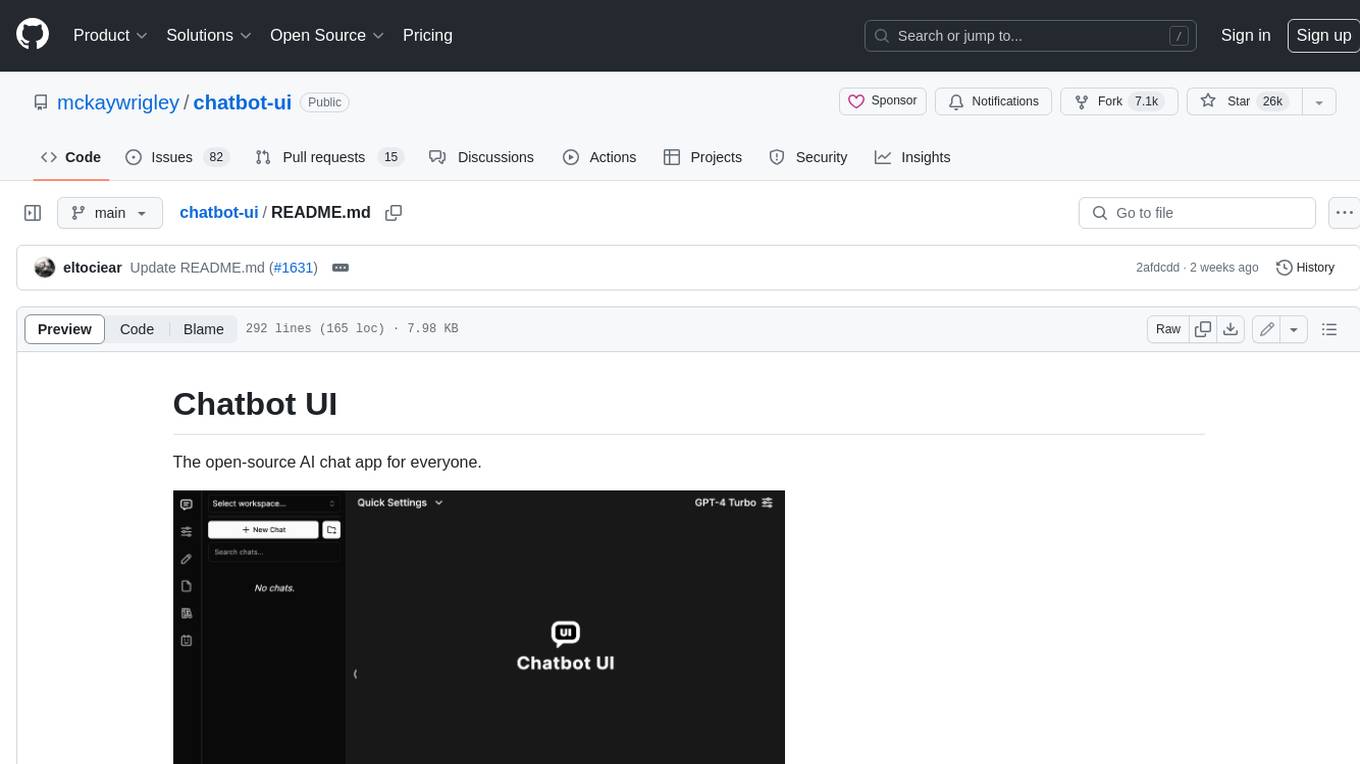
chatbot-ui
Chatbot UI is an open-source AI chat app that allows users to create and deploy their own AI chatbots. It is easy to use and can be customized to fit any need. Chatbot UI is perfect for businesses, developers, and anyone who wants to create a chatbot.
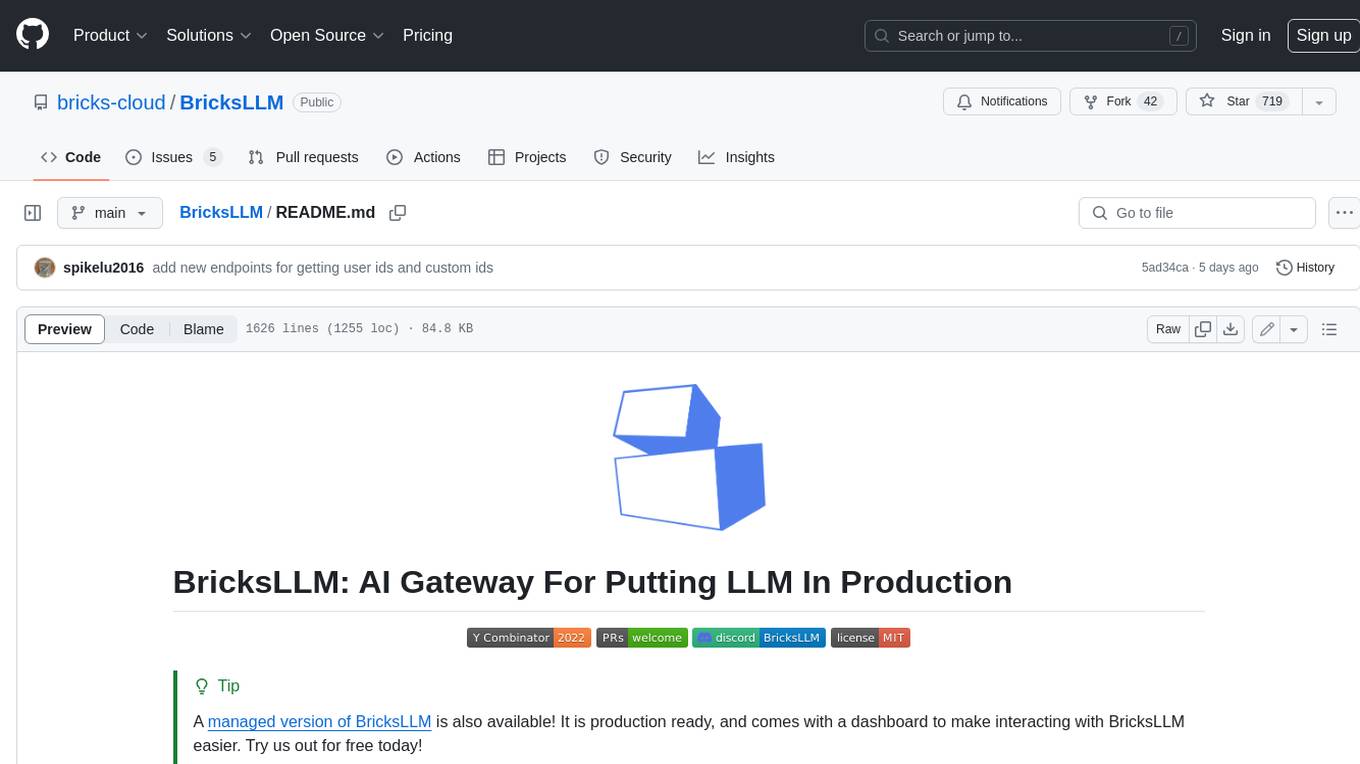
BricksLLM
BricksLLM is a cloud native AI gateway written in Go. Currently, it provides native support for OpenAI, Anthropic, Azure OpenAI and vLLM. BricksLLM aims to provide enterprise level infrastructure that can power any LLM production use cases. Here are some use cases for BricksLLM: * Set LLM usage limits for users on different pricing tiers * Track LLM usage on a per user and per organization basis * Block or redact requests containing PIIs * Improve LLM reliability with failovers, retries and caching * Distribute API keys with rate limits and cost limits for internal development/production use cases * Distribute API keys with rate limits and cost limits for students
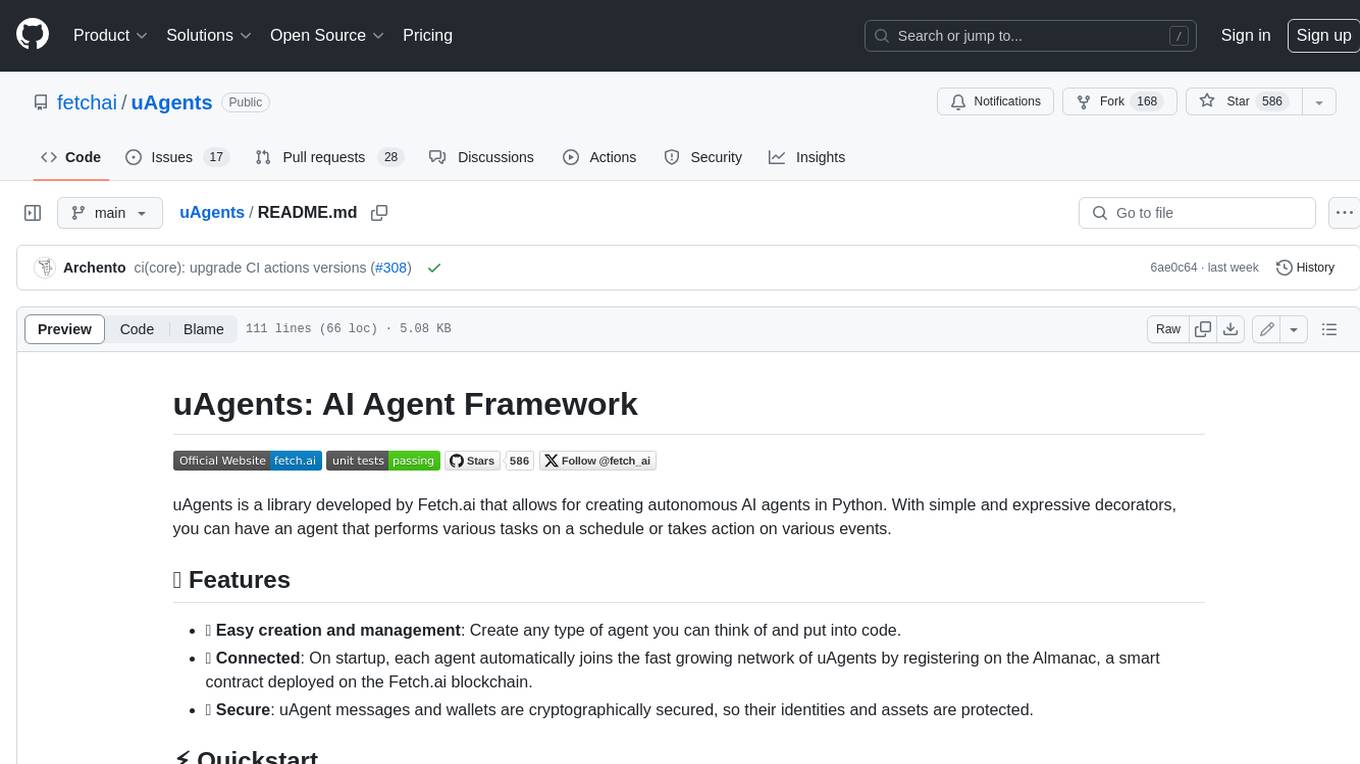
uAgents
uAgents is a Python library developed by Fetch.ai that allows for the creation of autonomous AI agents. These agents can perform various tasks on a schedule or take action on various events. uAgents are easy to create and manage, and they are connected to a fast-growing network of other uAgents. They are also secure, with cryptographically secured messages and wallets.
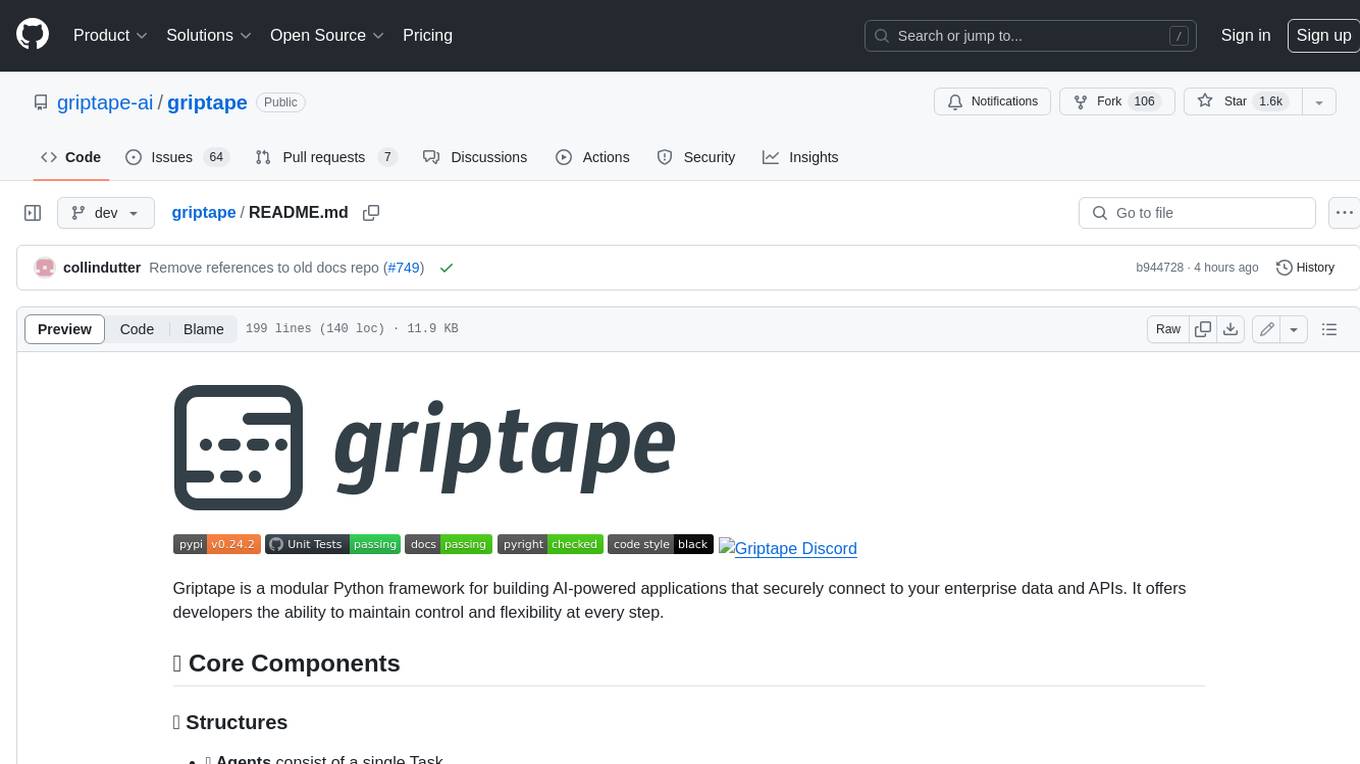
griptape
Griptape is a modular Python framework for building AI-powered applications that securely connect to your enterprise data and APIs. It offers developers the ability to maintain control and flexibility at every step. Griptape's core components include Structures (Agents, Pipelines, and Workflows), Tasks, Tools, Memory (Conversation Memory, Task Memory, and Meta Memory), Drivers (Prompt and Embedding Drivers, Vector Store Drivers, Image Generation Drivers, Image Query Drivers, SQL Drivers, Web Scraper Drivers, and Conversation Memory Drivers), Engines (Query Engines, Extraction Engines, Summary Engines, Image Generation Engines, and Image Query Engines), and additional components (Rulesets, Loaders, Artifacts, Chunkers, and Tokenizers). Griptape enables developers to create AI-powered applications with ease and efficiency.