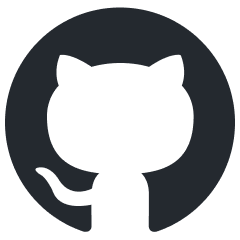
ControlFlow
đĻž Take control of your AI agents
Stars: 938
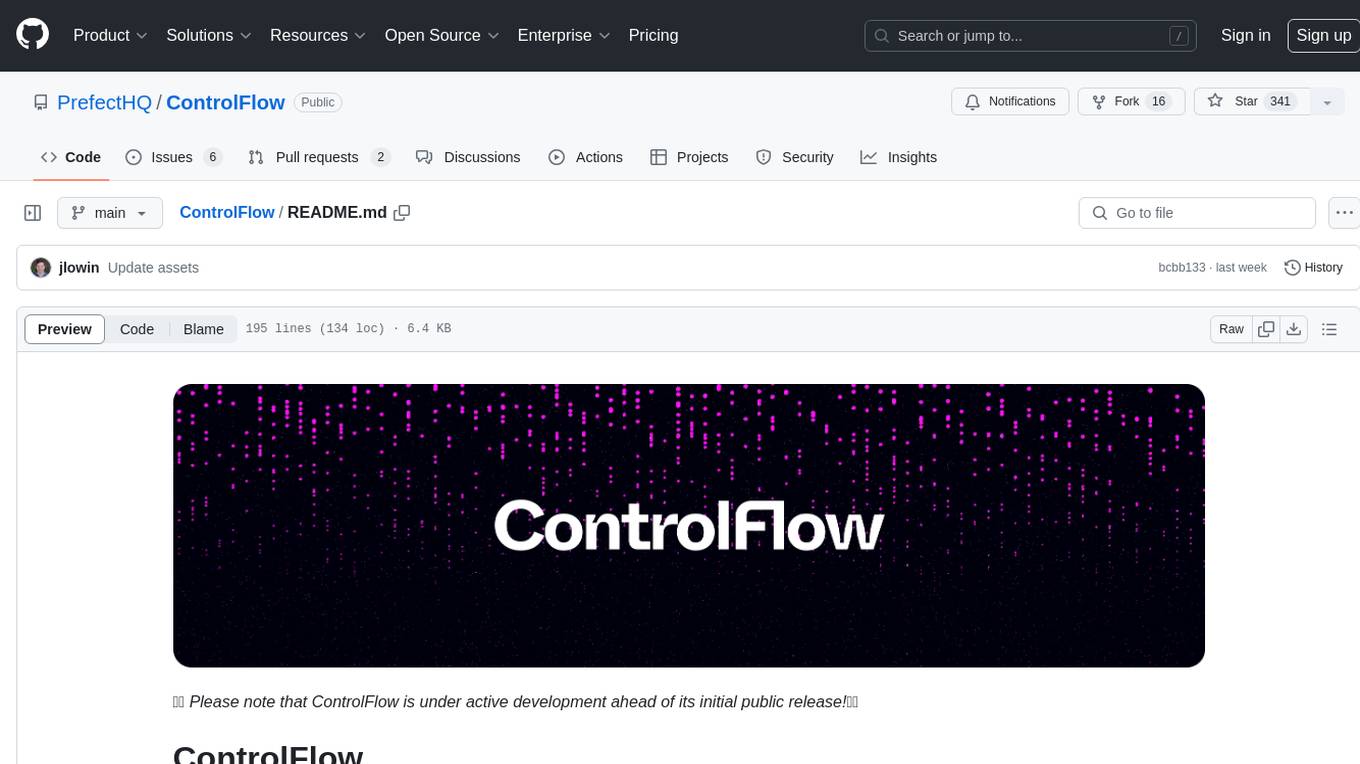
ControlFlow is a Python framework designed for building agentic AI workflows. It provides a structured approach for defining tasks, assigning specialized AI agents, and orchestrating complex behaviors. By balancing AI autonomy with precise oversight, users can create sophisticated AI-powered applications with confidence. ControlFlow offers a task-centric architecture, structured results with type-safe outputs, specialized agents for efficient problem-solving, ecosystem integration with LangChain models, flexible control over workflows, multi-agent orchestration, and native observability and debugging capabilities.
README:
ControlFlow is a Python framework for building agentic AI workflows.
ControlFlow provides a structured, developer-focused framework for defining workflows and delegating work to LLMs, without sacrificing control or transparency:
- Create discrete, observable tasks for an AI to work on.
- Assign one or more specialized AI agents to each task.
- Combine tasks into a flow to orchestrate more complex behaviors.
The simplest ControlFlow workflow has one task, a default agent, and automatic thread management:
import controlflow as cf
result = cf.run("Write a short poem about artificial intelligence")
print(result)
Result:
In circuits and code, a mind does bloom,
With algorithms weaving through the gloom.
A spark of thought in silicon's embrace,
Artificial intelligence finds its place.
ControlFlow addresses the challenges of building AI-powered applications that are both powerful and predictable:
- đ§Š Task-Centric Architecture: Break complex AI workflows into manageable, observable steps.
- đ Structured Results: Bridge the gap between AI and traditional software with type-safe, validated outputs.
- đ¤ Specialized Agents: Deploy task-specific AI agents for efficient problem-solving.
- đī¸ Flexible Control: Continuously tune the balance of control and autonomy in your workflows.
- đšī¸ Multi-Agent Orchestration: Coordinate multiple AI agents within a single workflow or task.
- đ Native Observability: Monitor and debug your AI workflows with full Prefect 3.0 support.
- đ Ecosystem Integration: Seamlessly work with your existing code, tools, and the broader AI ecosystem.
Install ControlFlow with pip
:
pip install controlflow
Next, configure your LLM provider. ControlFlow's default provider is OpenAI, which requires the OPENAI_API_KEY
environment variable:
export OPENAI_API_KEY=your-api-key
To use a different LLM provider, see the LLM configuration docs.
Here's a more involved example that showcases user interaction, a multi-step workflow, and structured outputs:
import controlflow as cf
from pydantic import BaseModel
class ResearchProposal(BaseModel):
title: str
abstract: str
key_points: list[str]
@cf.flow
def research_proposal_flow():
# Task 1: Get the research topic from the user
user_input = cf.Task(
"Work with the user to choose a research topic",
interactive=True,
)
# Task 2: Generate a structured research proposal
proposal = cf.run(
"Generate a structured research proposal",
result_type=ResearchProposal,
depends_on=[user_input]
)
return proposal
result = research_proposal_flow()
print(result.model_dump_json(indent=2))
Click to see results
Conversation:
Agent: Hello! I'm here to help you choose a research topic. Do you have any particular area of interest or field you would like to explore? If you have any specific ideas or requirements, please share them as well. User: Yes, I'm interested in LLM agentic workflows
Proposal:
{ "title": "AI Agentic Workflows: Enhancing Efficiency and Automation", "abstract": "This research proposal aims to explore the development and implementation of AI agentic workflows to enhance efficiency and automation in various domains. AI agents, equipped with advanced capabilities, can perform complex tasks, make decisions, and interact with other agents or humans to achieve specific goals. This research will investigate the underlying technologies, methodologies, and applications of AI agentic workflows, evaluate their effectiveness, and propose improvements to optimize their performance.", "key_points": [ "Introduction: Definition and significance of AI agentic workflows, Historical context and evolution of AI in workflows", "Technological Foundations: AI technologies enabling agentic workflows (e.g., machine learning, natural language processing), Software and hardware requirements for implementing AI workflows", "Methodologies: Design principles for creating effective AI agents, Workflow orchestration and management techniques, Interaction protocols between AI agents and human operators", "Applications: Case studies of AI agentic workflows in various industries (e.g., healthcare, finance, manufacturing), Benefits and challenges observed in real-world implementations", "Evaluation and Metrics: Criteria for assessing the performance of AI agentic workflows, Metrics for measuring efficiency, accuracy, and user satisfaction", "Proposed Improvements: Innovations to enhance the capabilities of AI agents, Strategies for addressing limitations and overcoming challenges", "Conclusion: Summary of key findings, Future research directions and potential impact on industry and society" ] }
In this example, ControlFlow is automatically managing a flow
, or a shared context for a series of tasks. You can switch between standard Python functions and agentic tasks at any time, making it easy to incrementally build out complex workflows.
To dive deeper into ControlFlow:
For Tasks:
Click tags to check more tools for each tasksFor Jobs:
Alternative AI tools for ControlFlow
Similar Open Source Tools
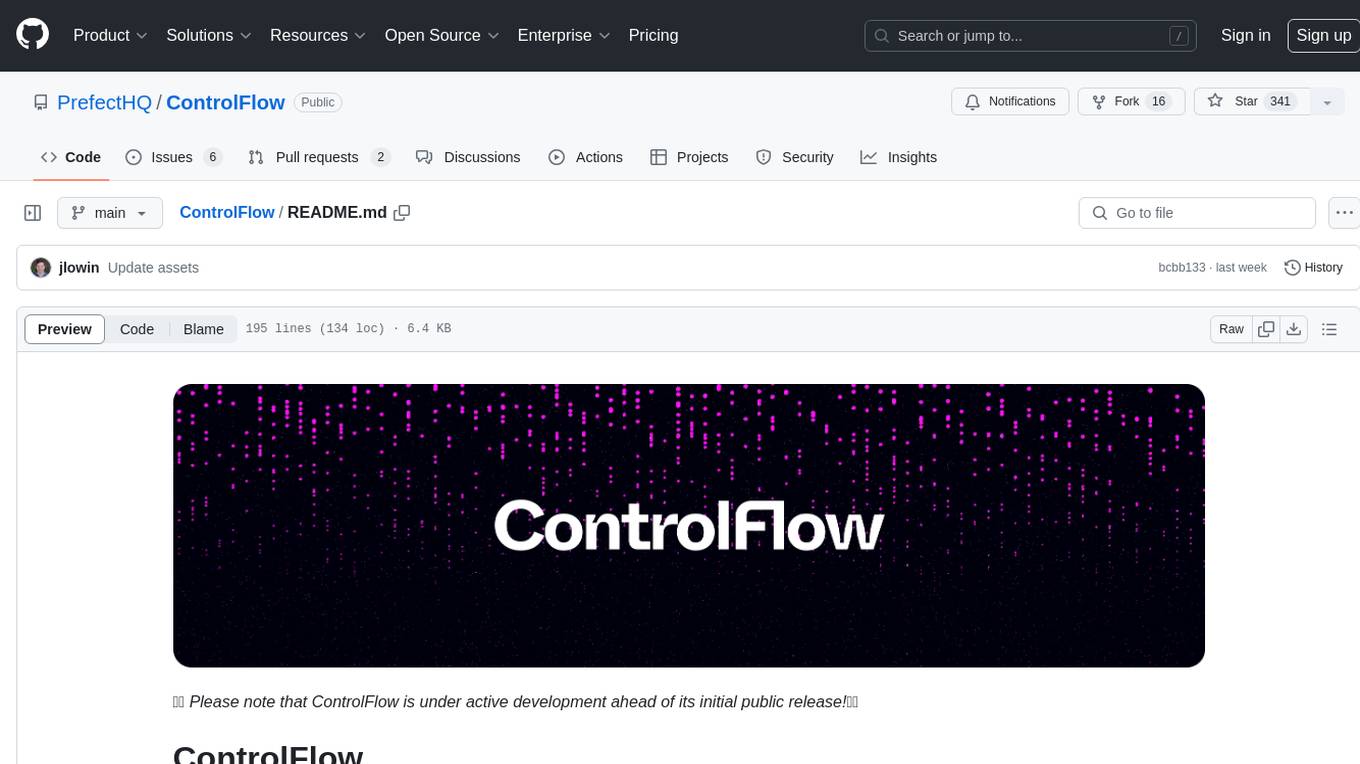
ControlFlow
ControlFlow is a Python framework designed for building agentic AI workflows. It provides a structured approach for defining tasks, assigning specialized AI agents, and orchestrating complex behaviors. By balancing AI autonomy with precise oversight, users can create sophisticated AI-powered applications with confidence. ControlFlow offers a task-centric architecture, structured results with type-safe outputs, specialized agents for efficient problem-solving, ecosystem integration with LangChain models, flexible control over workflows, multi-agent orchestration, and native observability and debugging capabilities.
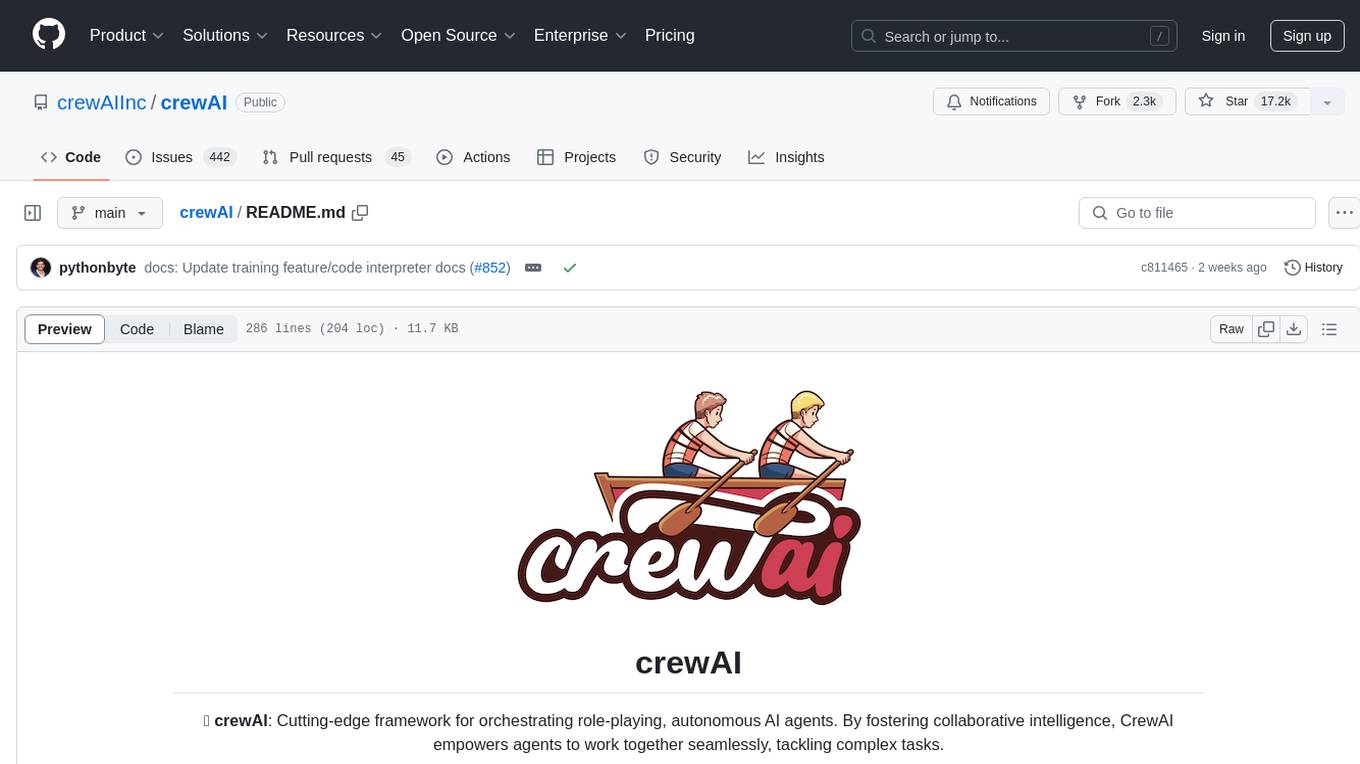
crewAI
CrewAI is a cutting-edge framework designed to orchestrate role-playing autonomous AI agents. By fostering collaborative intelligence, CrewAI empowers agents to work together seamlessly, tackling complex tasks. It enables AI agents to assume roles, share goals, and operate in a cohesive unit, much like a well-oiled crew. Whether you're building a smart assistant platform, an automated customer service ensemble, or a multi-agent research team, CrewAI provides the backbone for sophisticated multi-agent interactions. With features like role-based agent design, autonomous inter-agent delegation, flexible task management, and support for various LLMs, CrewAI offers a dynamic and adaptable solution for both development and production workflows.
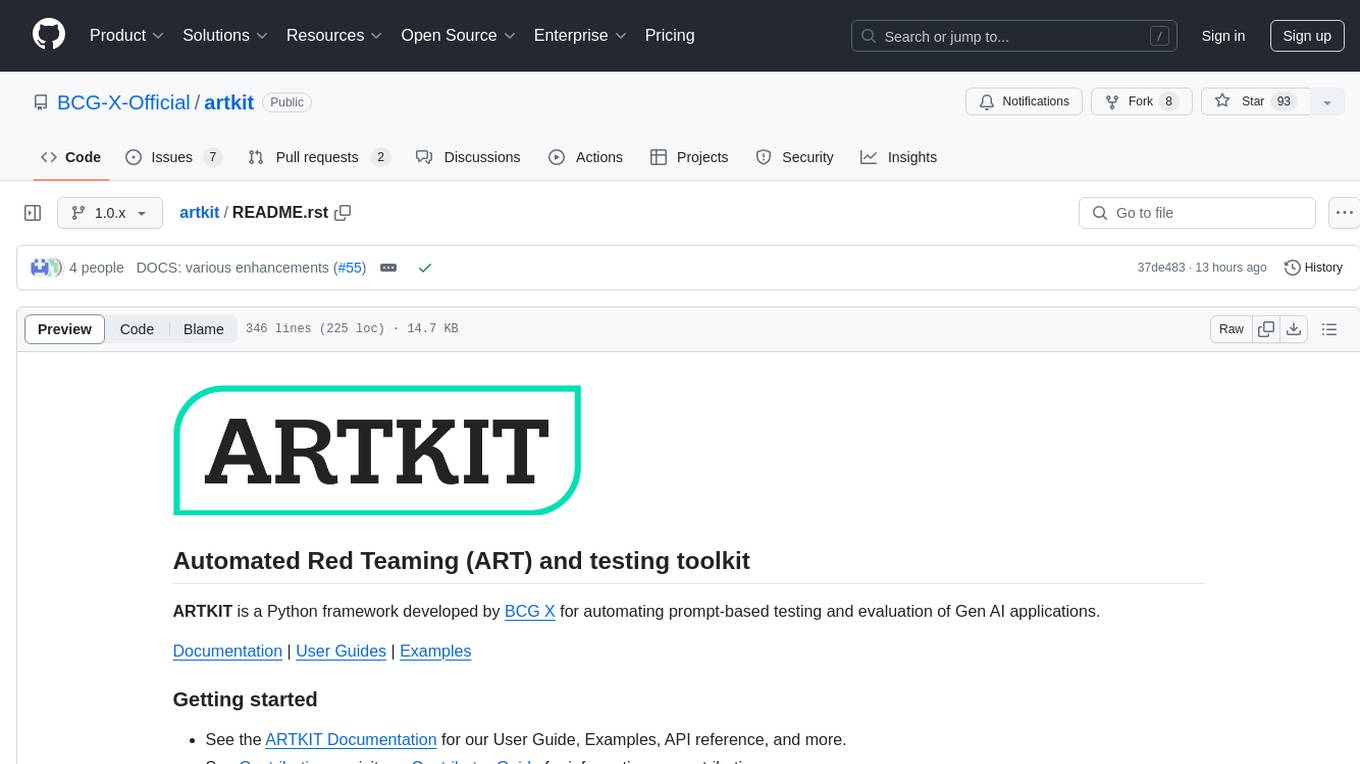
artkit
ARTKIT is a Python framework developed by BCG X for automating prompt-based testing and evaluation of Gen AI applications. It allows users to develop automated end-to-end testing and evaluation pipelines for Gen AI systems, supporting multi-turn conversations and various testing scenarios like Q&A accuracy, brand values, equitability, safety, and security. The framework provides a simple API, asynchronous processing, caching, model agnostic support, end-to-end pipelines, multi-turn conversations, robust data flows, and visualizations. ARTKIT is designed for customization by data scientists and engineers to enhance human-in-the-loop testing and evaluation, emphasizing the importance of tailored testing for each Gen AI use case.
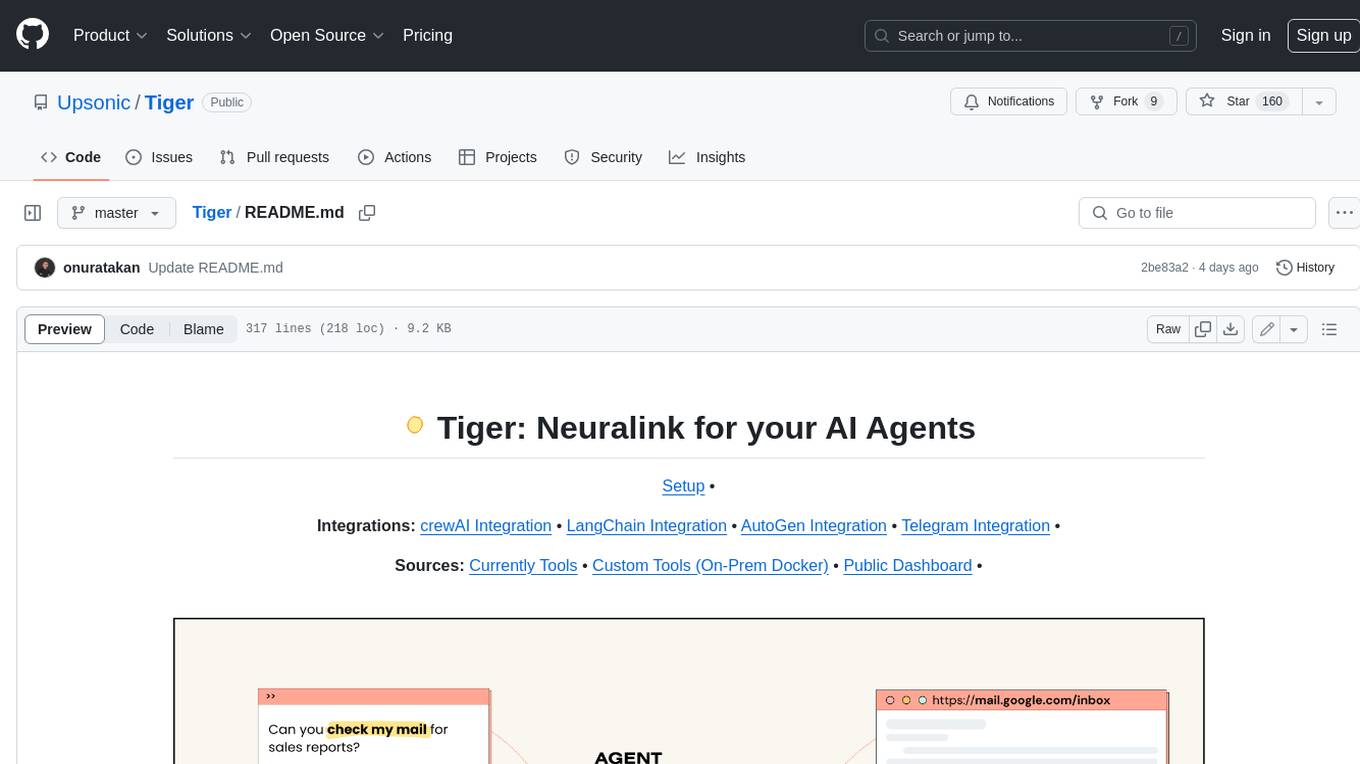
Tiger
Tiger is a community-driven project developing a reusable and integrated tool ecosystem for LLM Agent Revolution. It utilizes Upsonic for isolated tool storage, profiling, and automatic document generation. With Tiger, you can create a customized environment for your agents or leverage the robust and publicly maintained Tiger curated by the community itself.
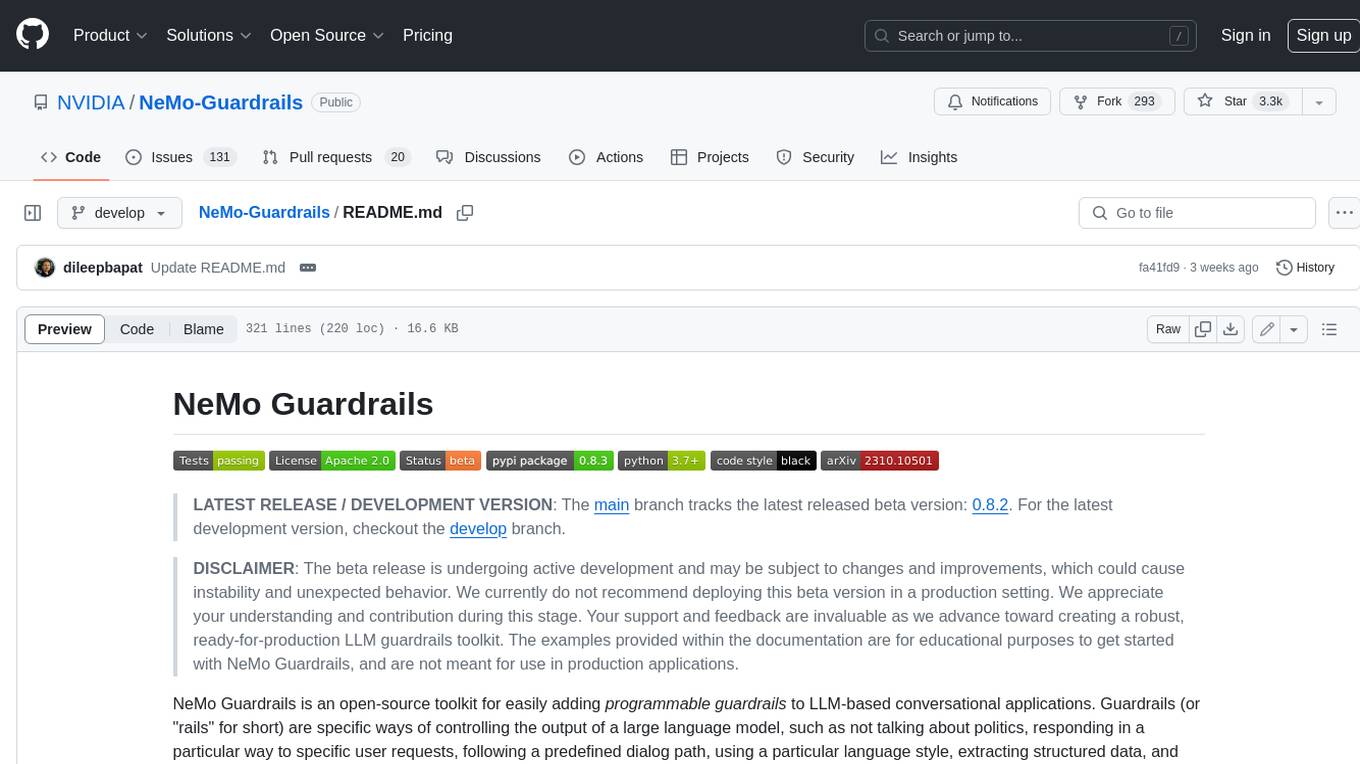
NeMo-Guardrails
NeMo Guardrails is an open-source toolkit for easily adding _programmable guardrails_ to LLM-based conversational applications. Guardrails (or "rails" for short) are specific ways of controlling the output of a large language model, such as not talking about politics, responding in a particular way to specific user requests, following a predefined dialog path, using a particular language style, extracting structured data, and more.
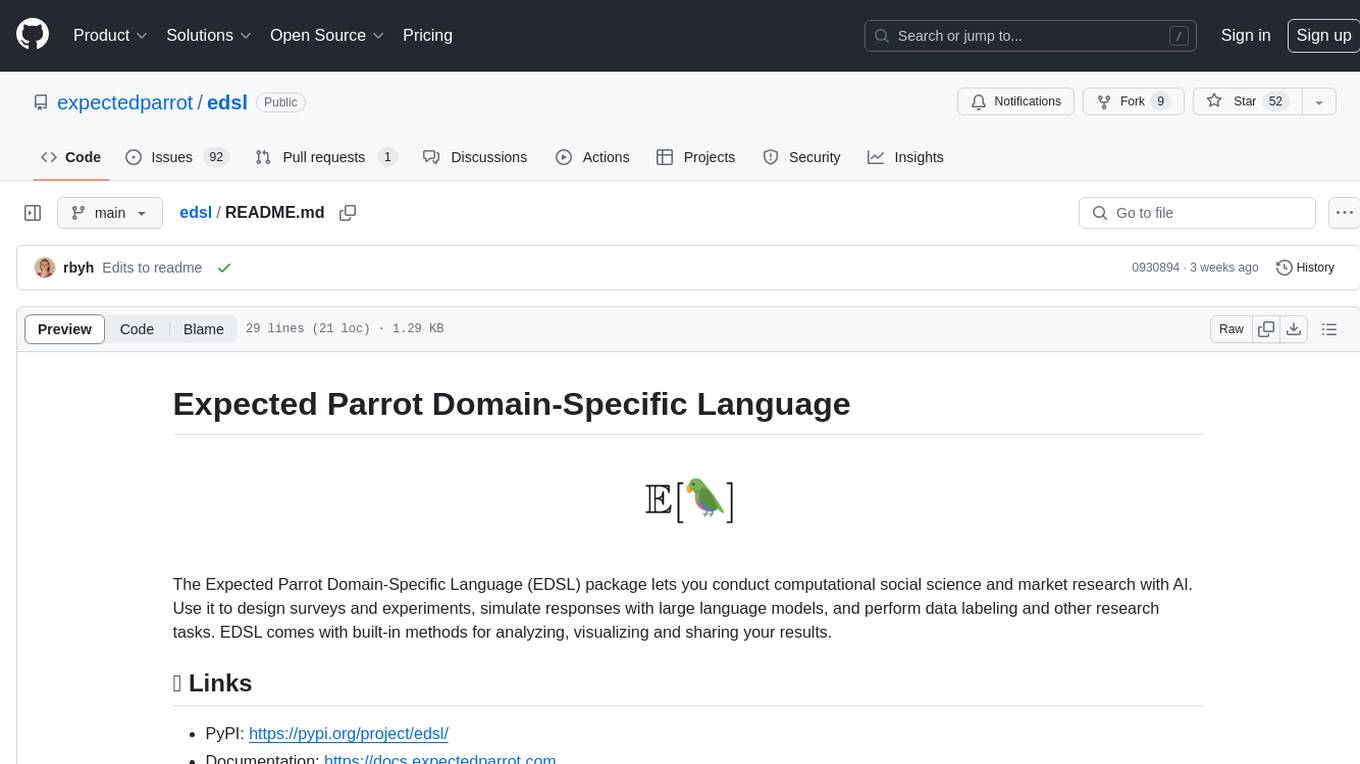
edsl
The Expected Parrot Domain-Specific Language (EDSL) package enables users to conduct computational social science and market research with AI. It facilitates designing surveys and experiments, simulating responses using large language models, and performing data labeling and other research tasks. EDSL includes built-in methods for analyzing, visualizing, and sharing research results. It is compatible with Python 3.9 - 3.11 and requires API keys for LLMs stored in a `.env` file.
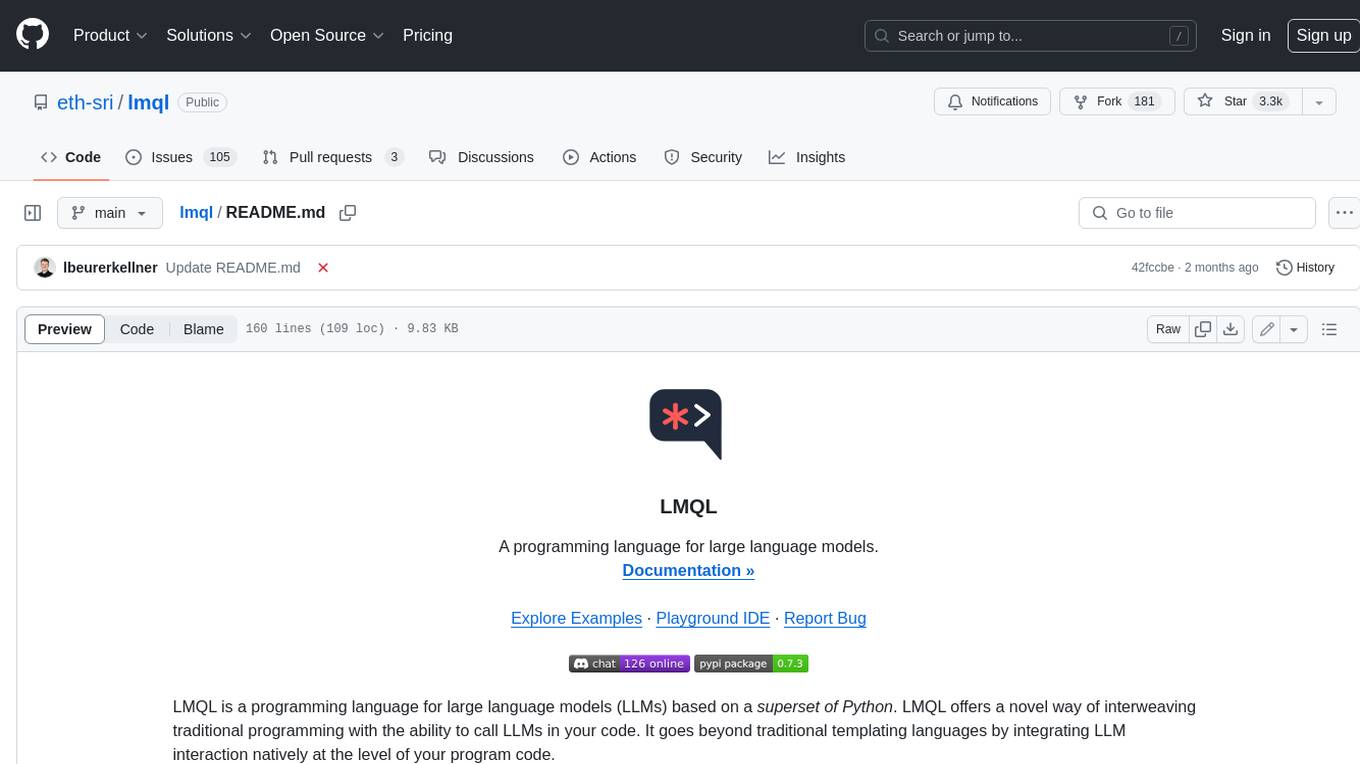
lmql
LMQL is a programming language designed for large language models (LLMs) that offers a unique way of integrating traditional programming with LLM interaction. It allows users to write programs that combine algorithmic logic with LLM calls, enabling model reasoning capabilities within the context of the program. LMQL provides features such as Python syntax integration, rich control-flow options, advanced decoding techniques, powerful constraints via logit masking, runtime optimization, sync and async API support, multi-model compatibility, and extensive applications like JSON decoding and interactive chat interfaces. The tool also offers library integration, flexible tooling, and output streaming options for easy model output handling.
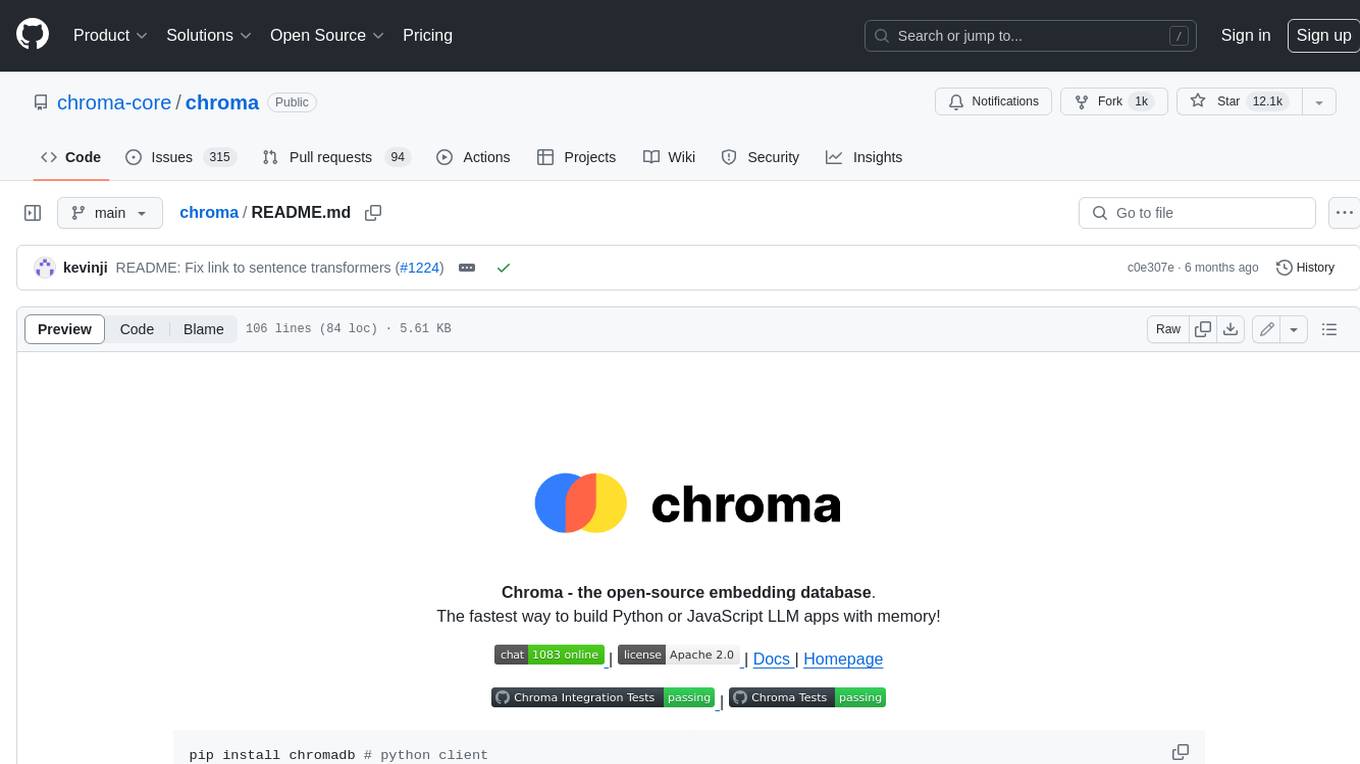
chroma
Chroma is an open-source embedding database that provides a simple, scalable, and feature-rich way to build Python or JavaScript LLM apps with memory. It offers a fully-typed, fully-tested, and fully-documented API that makes it easy to get started and scale your applications. Chroma also integrates with popular tools like LangChain and LlamaIndex, and supports a variety of embedding models, including Sentence Transformers, OpenAI embeddings, and Cohere embeddings. With Chroma, you can easily add documents to your database, query relevant documents with natural language, and compose documents into the context window of an LLM like GPT3 for additional summarization or analysis.
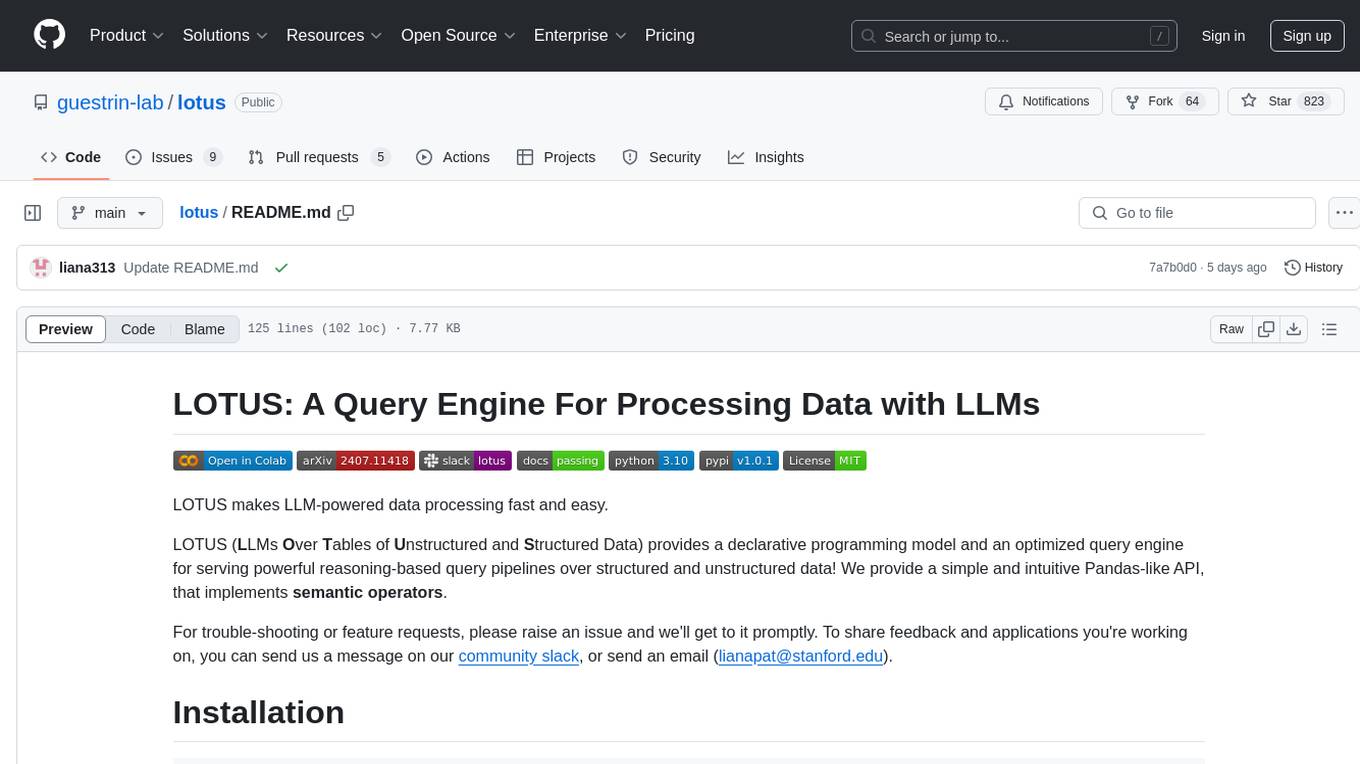
lotus
LOTUS (LLMs Over Tables of Unstructured and Structured Data) is a query engine that provides a declarative programming model and an optimized query engine for reasoning-based query pipelines over structured and unstructured data. It offers a simple and intuitive Pandas-like API with semantic operators for fast and easy LLM-powered data processing. The tool implements a semantic operator programming model, allowing users to write AI-based pipelines with high-level logic and leaving the rest of the work to the query engine. LOTUS supports various semantic operators like sem_map, sem_filter, sem_extract, sem_agg, sem_topk, sem_join, sem_sim_join, and sem_search, enabling users to perform tasks like mapping records, filtering data, aggregating records, and more. The tool also supports different model classes such as LM, RM, and Reranker for language modeling, retrieval, and reranking tasks respectively.
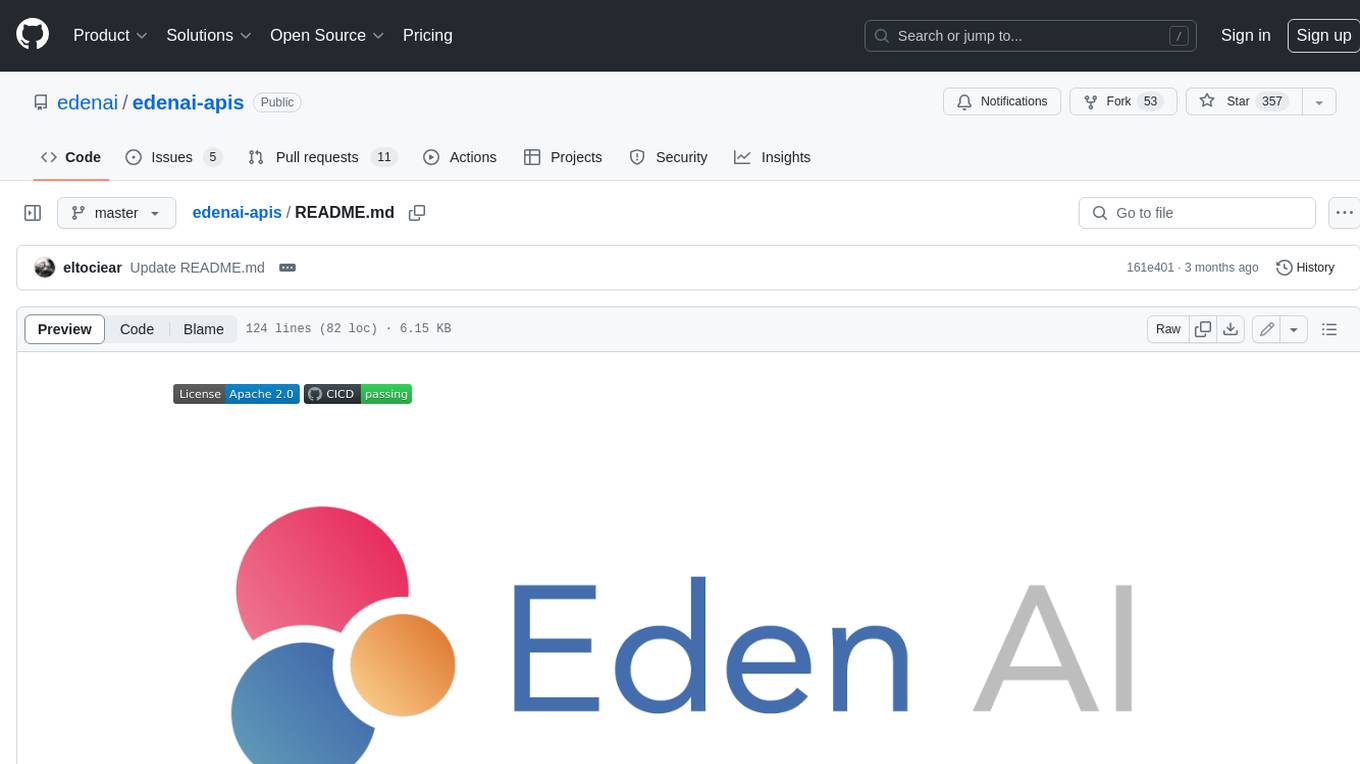
edenai-apis
Eden AI aims to simplify the use and deployment of AI technologies by providing a unique API that connects to all the best AI engines. With the rise of **AI as a Service** , a lot of companies provide off-the-shelf trained models that you can access directly through an API. These companies are either the tech giants (Google, Microsoft , Amazon) or other smaller, more specialized companies, and there are hundreds of them. Some of the most known are : DeepL (translation), OpenAI (text and image analysis), AssemblyAI (speech analysis). There are **hundreds of companies** doing that. We're regrouping the best ones **in one place** !
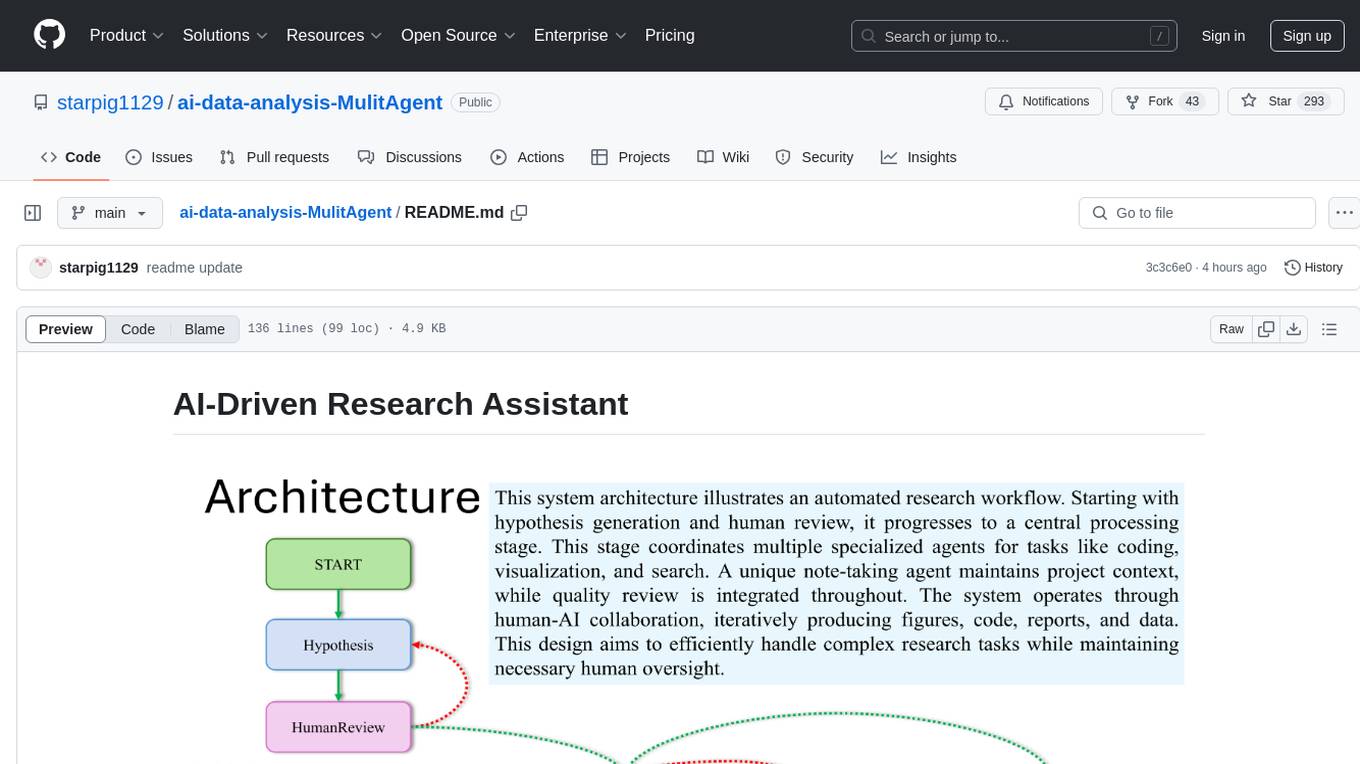
ai-data-analysis-MulitAgent
AI-Driven Research Assistant is an advanced AI-powered system utilizing specialized agents for data analysis, visualization, and report generation. It integrates LangChain, OpenAI's GPT models, and LangGraph for complex research processes. Key features include hypothesis generation, data processing, web search, code generation, and report writing. The system's unique Note Taker agent maintains project state, reducing overhead and improving context retention. System requirements include Python 3.10+ and Jupyter Notebook environment. Installation involves cloning the repository, setting up a Conda virtual environment, installing dependencies, and configuring environment variables. Usage instructions include setting data, running Jupyter Notebook, customizing research tasks, and viewing results. Main components include agents for hypothesis generation, process supervision, visualization, code writing, search, report writing, quality review, and note-taking. Workflow involves hypothesis generation, processing, quality review, and revision. Customization is possible by modifying agent creation and workflow definition. Current issues include OpenAI errors, NoteTaker efficiency, runtime optimization, and refiner improvement. Contributions via pull requests are welcome under the MIT License.
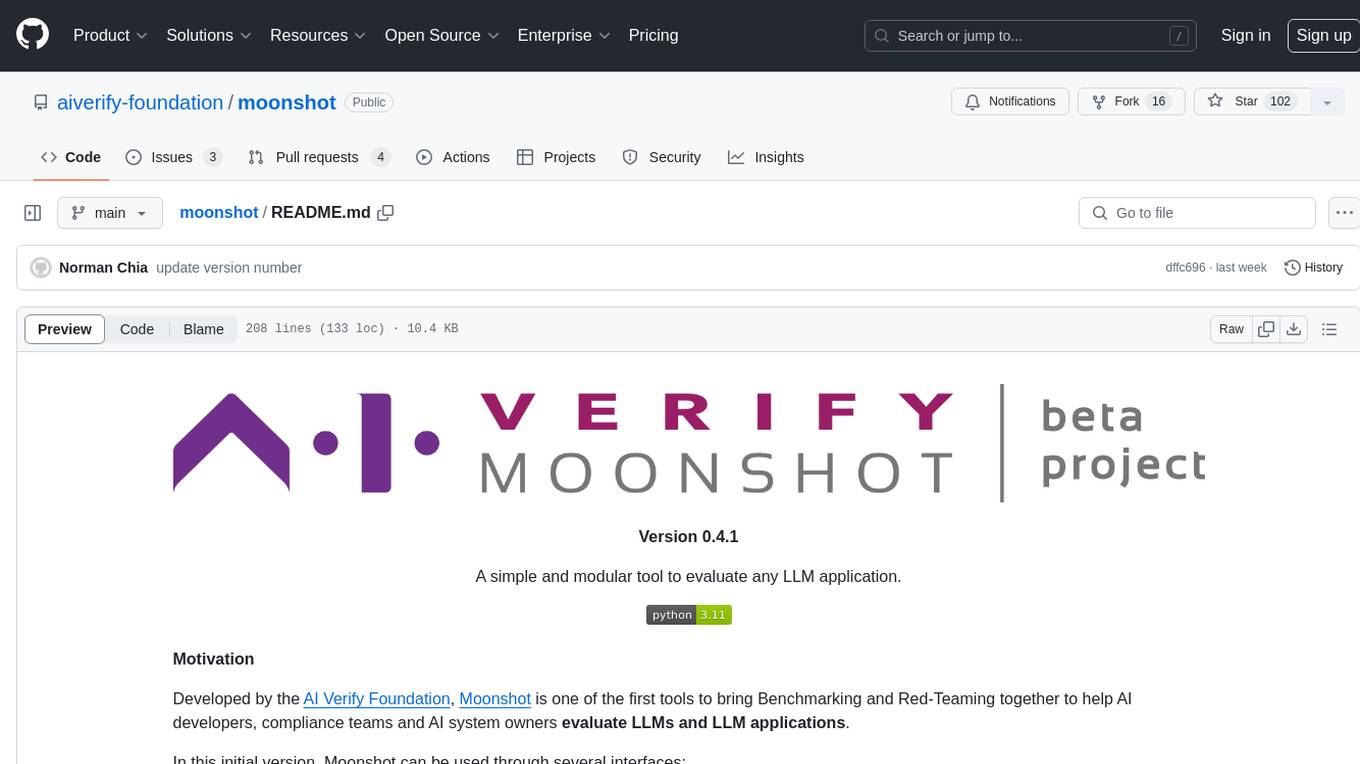
moonshot
Moonshot is a simple and modular tool developed by the AI Verify Foundation to evaluate Language Model Models (LLMs) and LLM applications. It brings Benchmarking and Red-Teaming together to assist AI developers, compliance teams, and AI system owners in assessing LLM performance. Moonshot can be accessed through various interfaces including User-friendly Web UI, Interactive Command Line Interface, and seamless integration into MLOps workflows via Library APIs or Web APIs. It offers features like benchmarking LLMs from popular model providers, running relevant tests, creating custom cookbooks and recipes, and automating Red Teaming to identify vulnerabilities in AI systems.
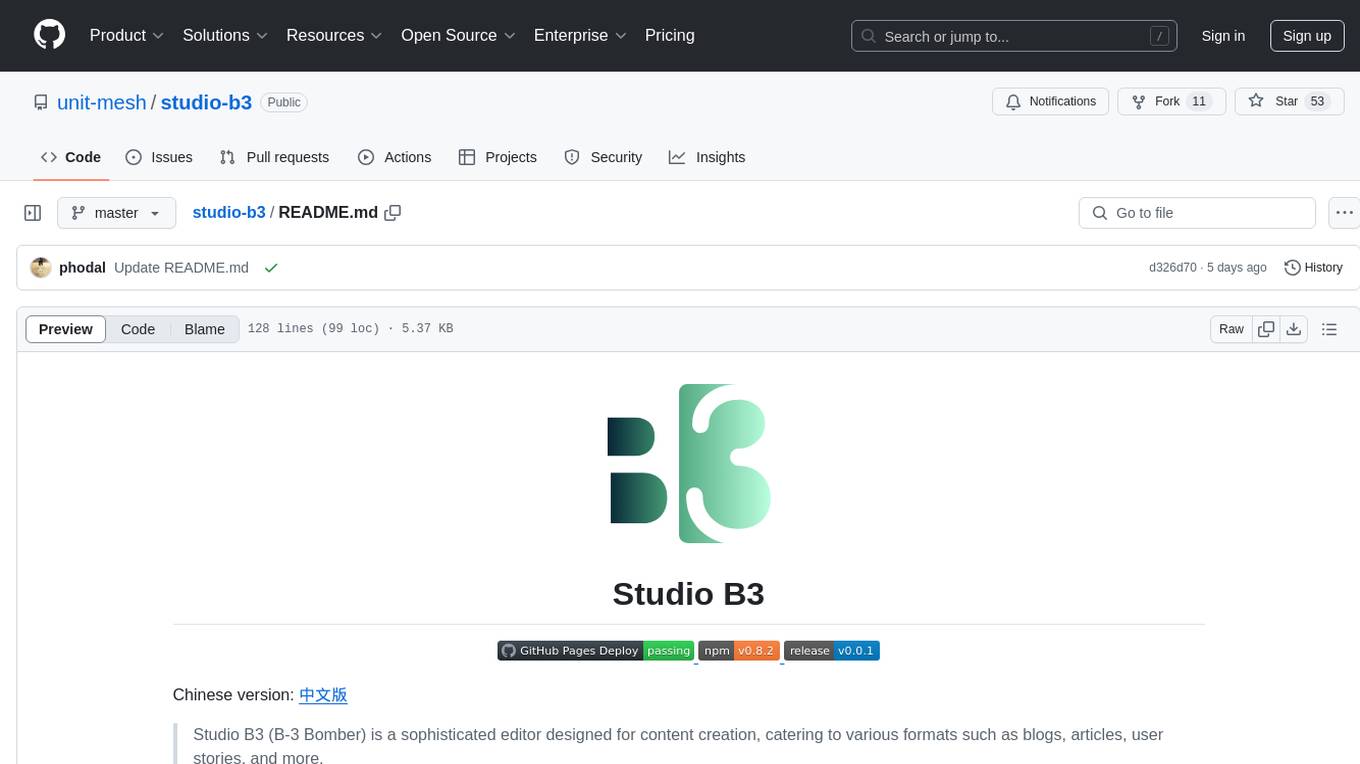
studio-b3
Studio B3 (B-3 Bomber) is a sophisticated editor designed for content creation, catering to various formats such as blogs, articles, user stories, and more. It provides an immersive content generation experience with local AI capabilities for intelligent search and recommendation functions. Users can define custom actions and variables for flexible content generation. The editor includes interactive tools like Bubble Menu, Slash Command, and Quick Insert for enhanced user experience in editing, searching, and navigation. The design principles focus on intelligent embedding of AI, local optimization for efficient writing experience, and context flexibility for better control over AI-generated content.
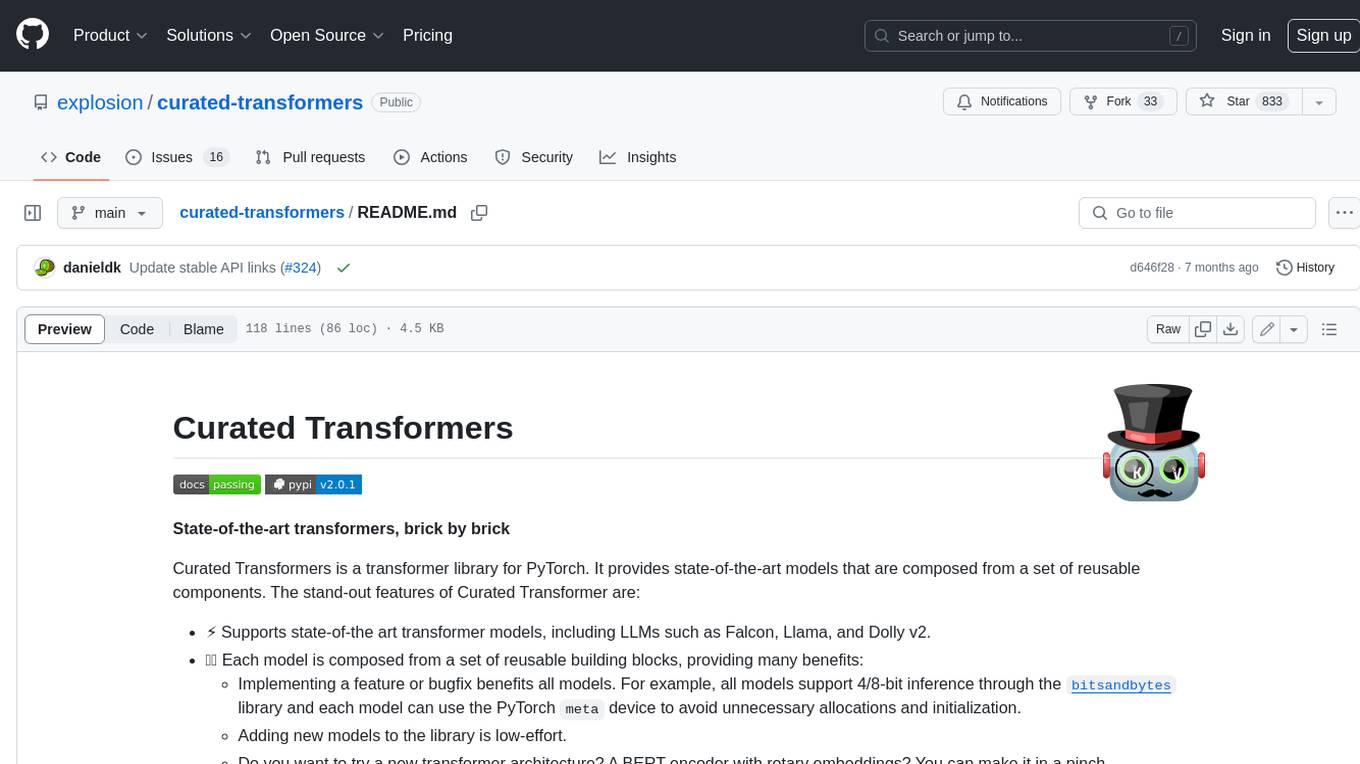
curated-transformers
Curated Transformers is a transformer library for PyTorch that provides state-of-the-art models composed of reusable components. It supports various transformer architectures, including encoders like ALBERT, BERT, and RoBERTa, and decoders like Falcon, Llama, and MPT. The library emphasizes consistent type annotations, minimal dependencies, and ease of use for education and research. It has been production-tested by Explosion and will be the default transformer implementation in spaCy 3.7.
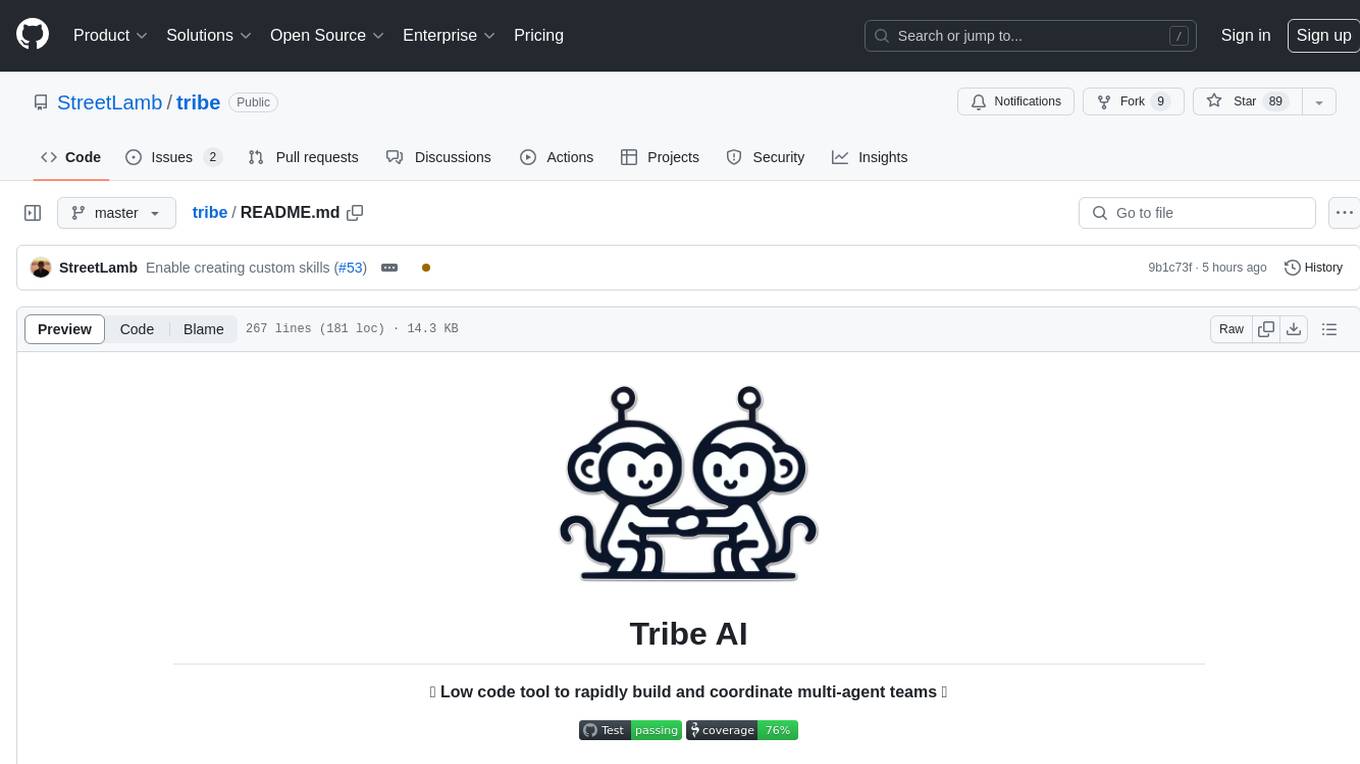
tribe
Tribe AI is a low code tool designed to rapidly build and coordinate multi-agent teams. It leverages the langgraph framework to customize and coordinate teams of agents, allowing tasks to be split among agents with different strengths for faster and better problem-solving. The tool supports persistent conversations, observability, tool calling, human-in-the-loop functionality, easy deployment with Docker, and multi-tenancy for managing multiple users and teams.
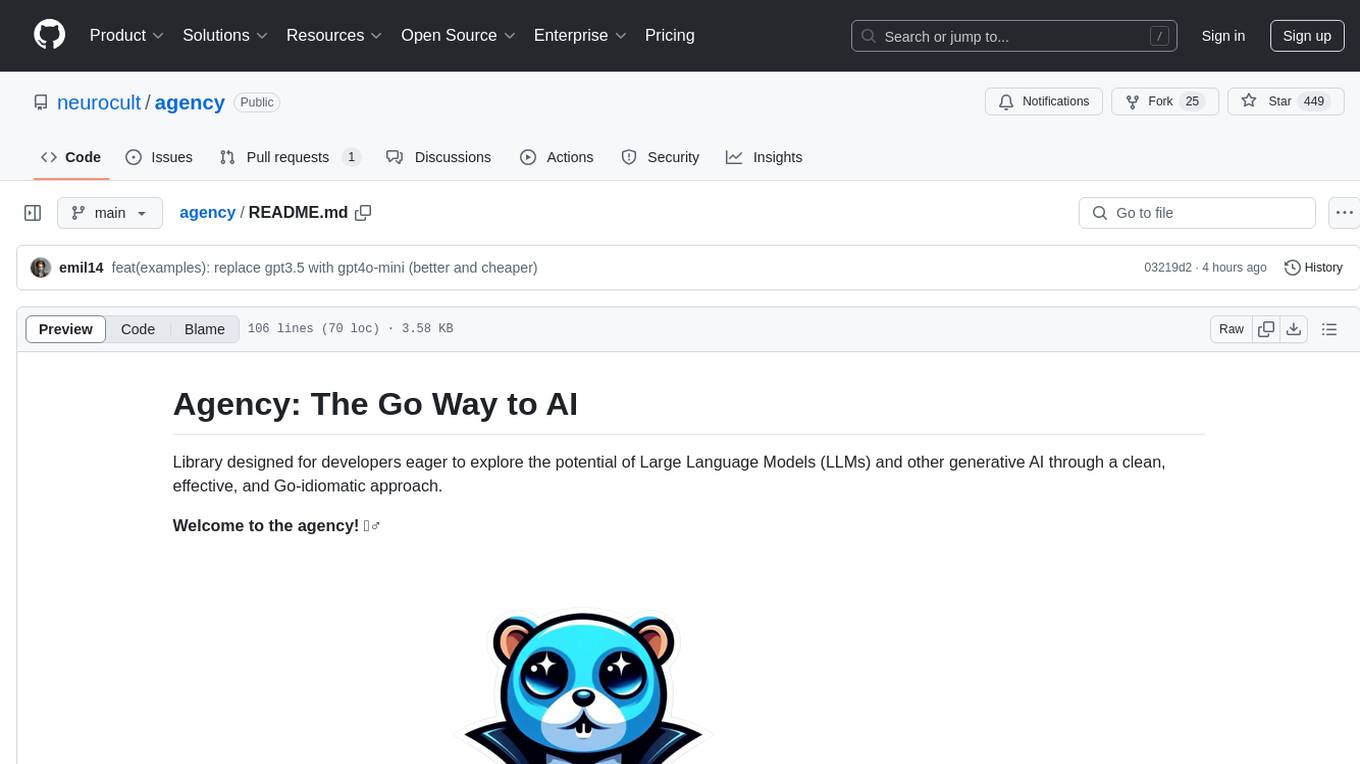
agency
Agency is a Go library designed for developers to explore Large Language Models (LLMs) and generative AI in a clean, effective, and Go-idiomatic way. It allows users to easily create custom operations, compose operations into processes, and interact with OpenAI API bindings for various tasks such as text completion, image generation, and speech-to-text conversion. The ultimate goal of Agency is to empower users to build autonomous AI systems, from chat interfaces to complex data analysis, with a focus on simplicity, flexibility, and efficiency.
For similar tasks
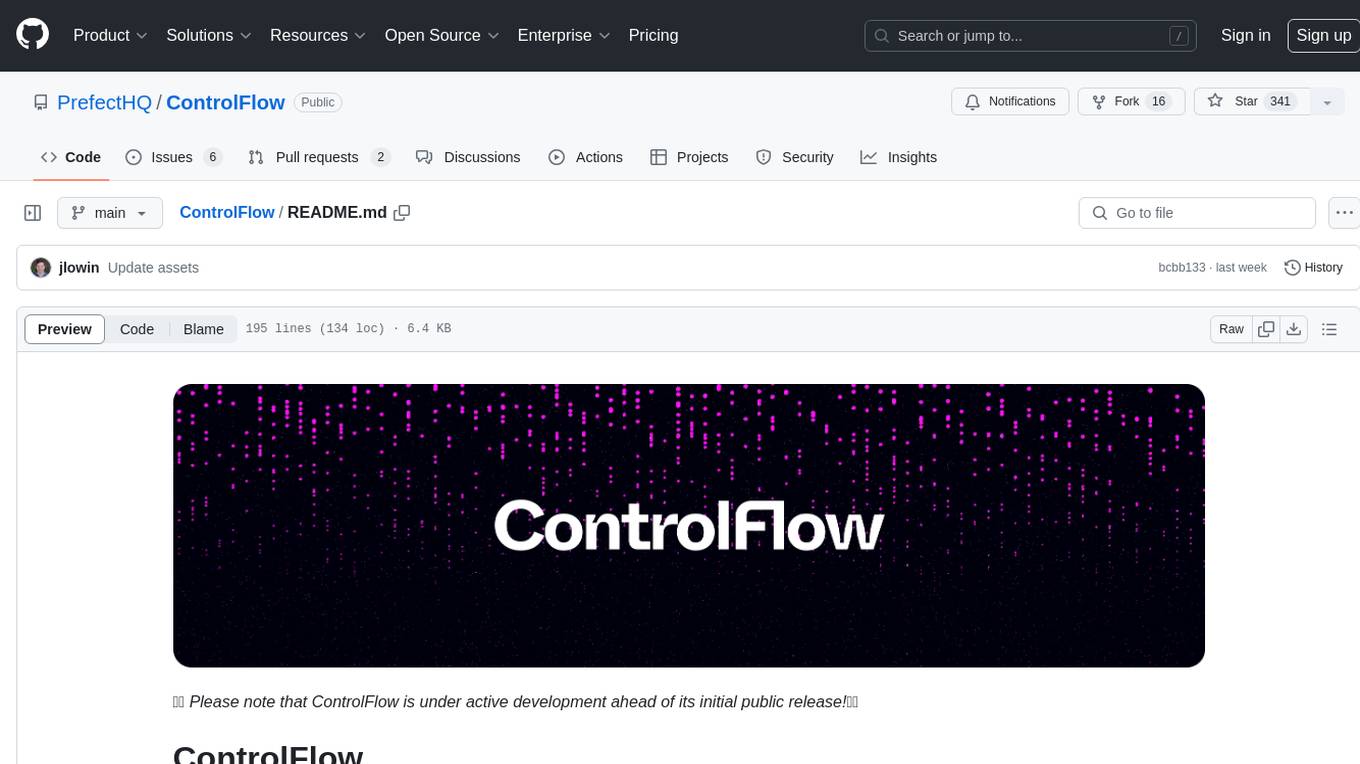
ControlFlow
ControlFlow is a Python framework designed for building agentic AI workflows. It provides a structured approach for defining tasks, assigning specialized AI agents, and orchestrating complex behaviors. By balancing AI autonomy with precise oversight, users can create sophisticated AI-powered applications with confidence. ControlFlow offers a task-centric architecture, structured results with type-safe outputs, specialized agents for efficient problem-solving, ecosystem integration with LangChain models, flexible control over workflows, multi-agent orchestration, and native observability and debugging capabilities.
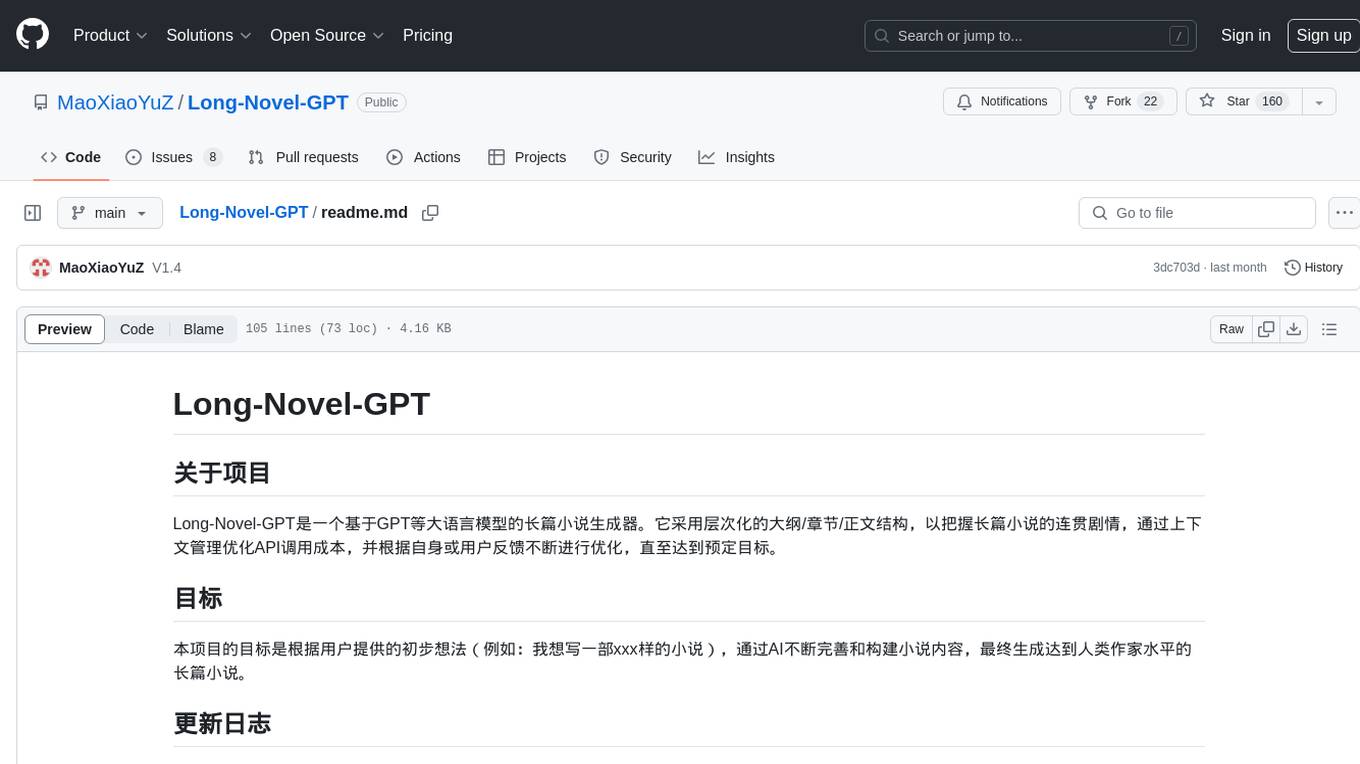
Long-Novel-GPT
Long-Novel-GPT is a long novel generator based on large language models like GPT. It utilizes a hierarchical outline/chapter/text structure to maintain the coherence of long novels. It optimizes API calls cost through context management and continuously improves based on self or user feedback until reaching the set goal. The tool aims to continuously refine and build novel content based on user-provided initial ideas, ultimately generating long novels at the level of human writers.
For similar jobs
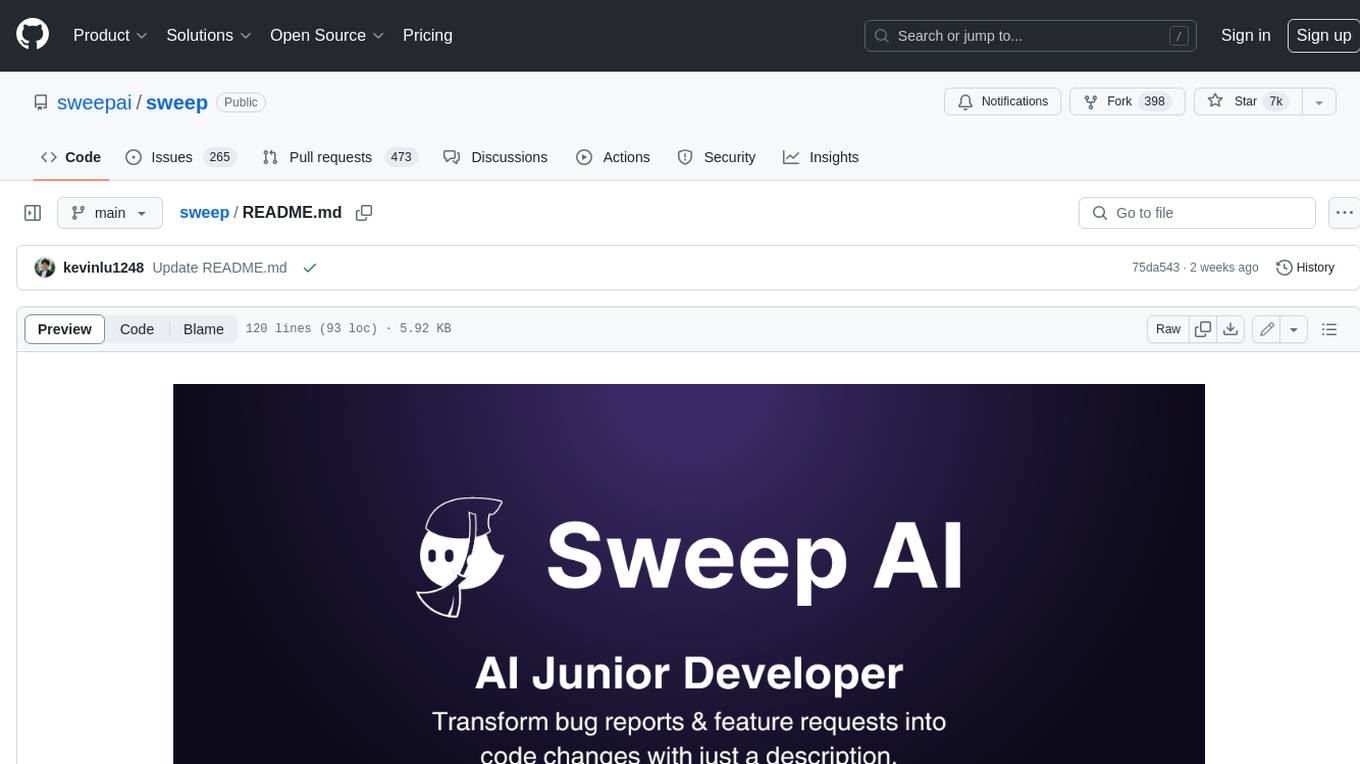
sweep
Sweep is an AI junior developer that turns bugs and feature requests into code changes. It automatically handles developer experience improvements like adding type hints and improving test coverage.
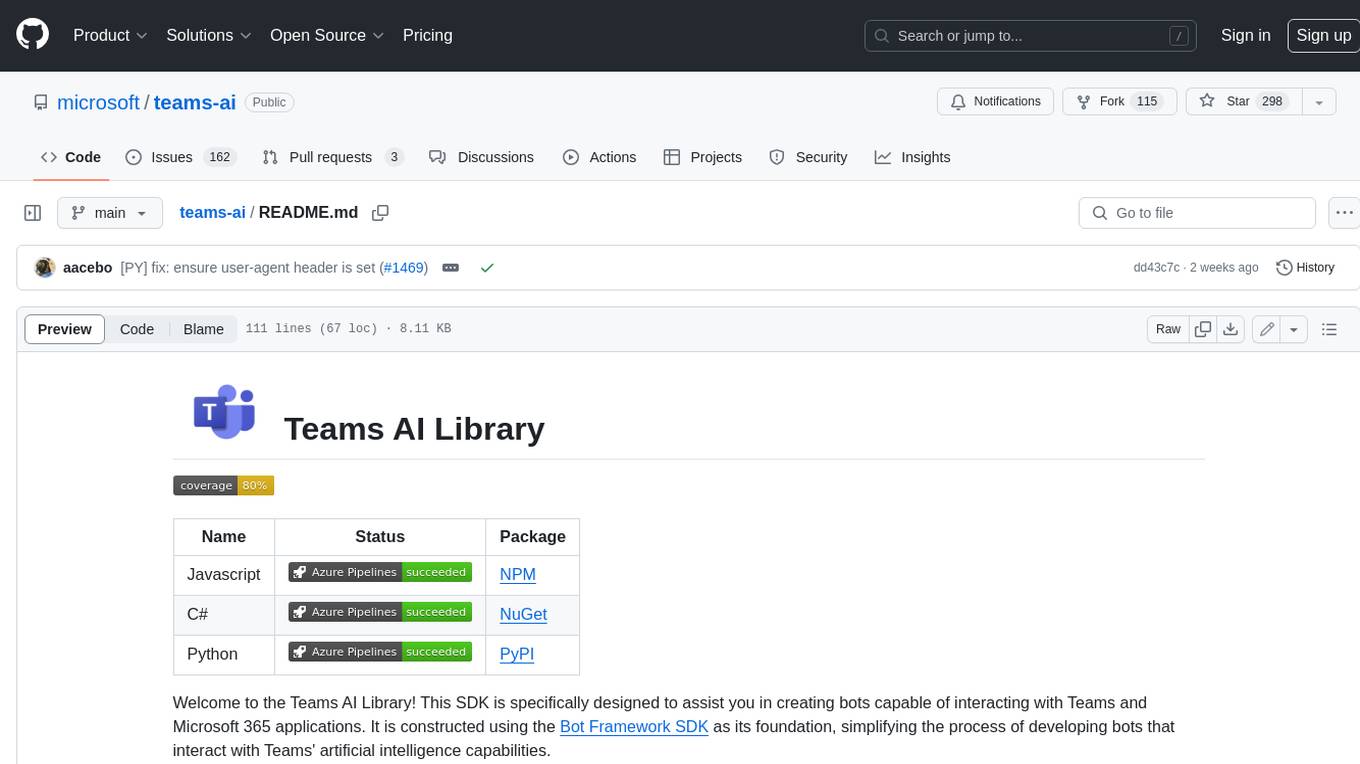
teams-ai
The Teams AI Library is a software development kit (SDK) that helps developers create bots that can interact with Teams and Microsoft 365 applications. It is built on top of the Bot Framework SDK and simplifies the process of developing bots that interact with Teams' artificial intelligence capabilities. The SDK is available for JavaScript/TypeScript, .NET, and Python.
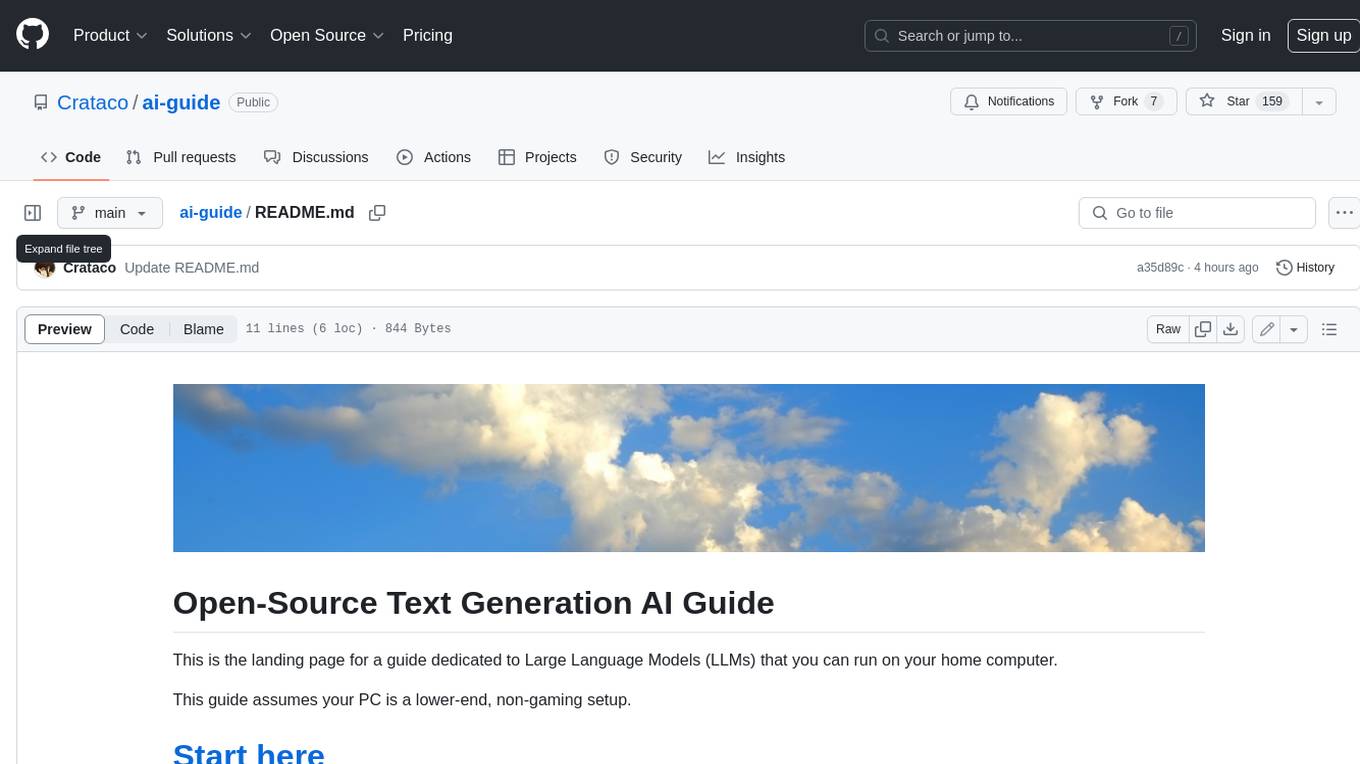
ai-guide
This guide is dedicated to Large Language Models (LLMs) that you can run on your home computer. It assumes your PC is a lower-end, non-gaming setup.
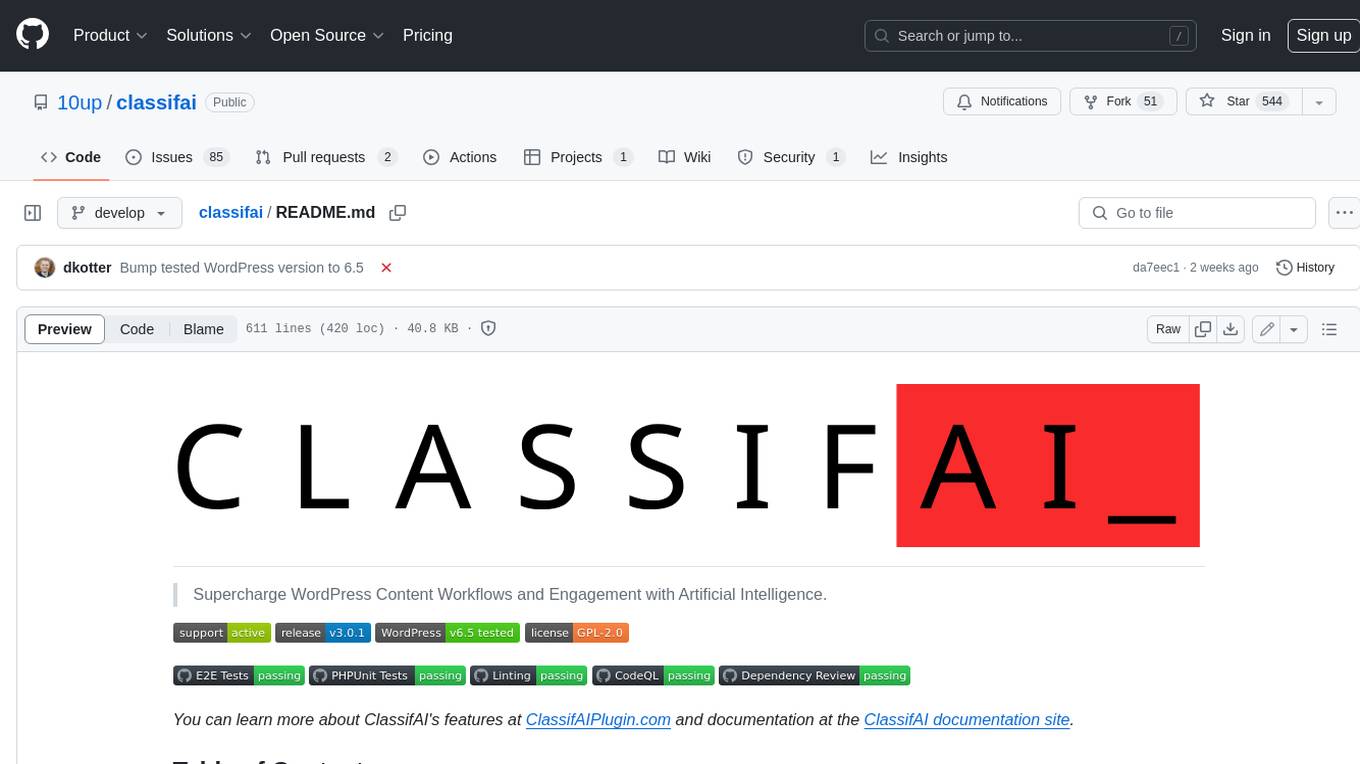
classifai
Supercharge WordPress Content Workflows and Engagement with Artificial Intelligence. Tap into leading cloud-based services like OpenAI, Microsoft Azure AI, Google Gemini and IBM Watson to augment your WordPress-powered websites. Publish content faster while improving SEO performance and increasing audience engagement. ClassifAI integrates Artificial Intelligence and Machine Learning technologies to lighten your workload and eliminate tedious tasks, giving you more time to create original content that matters.
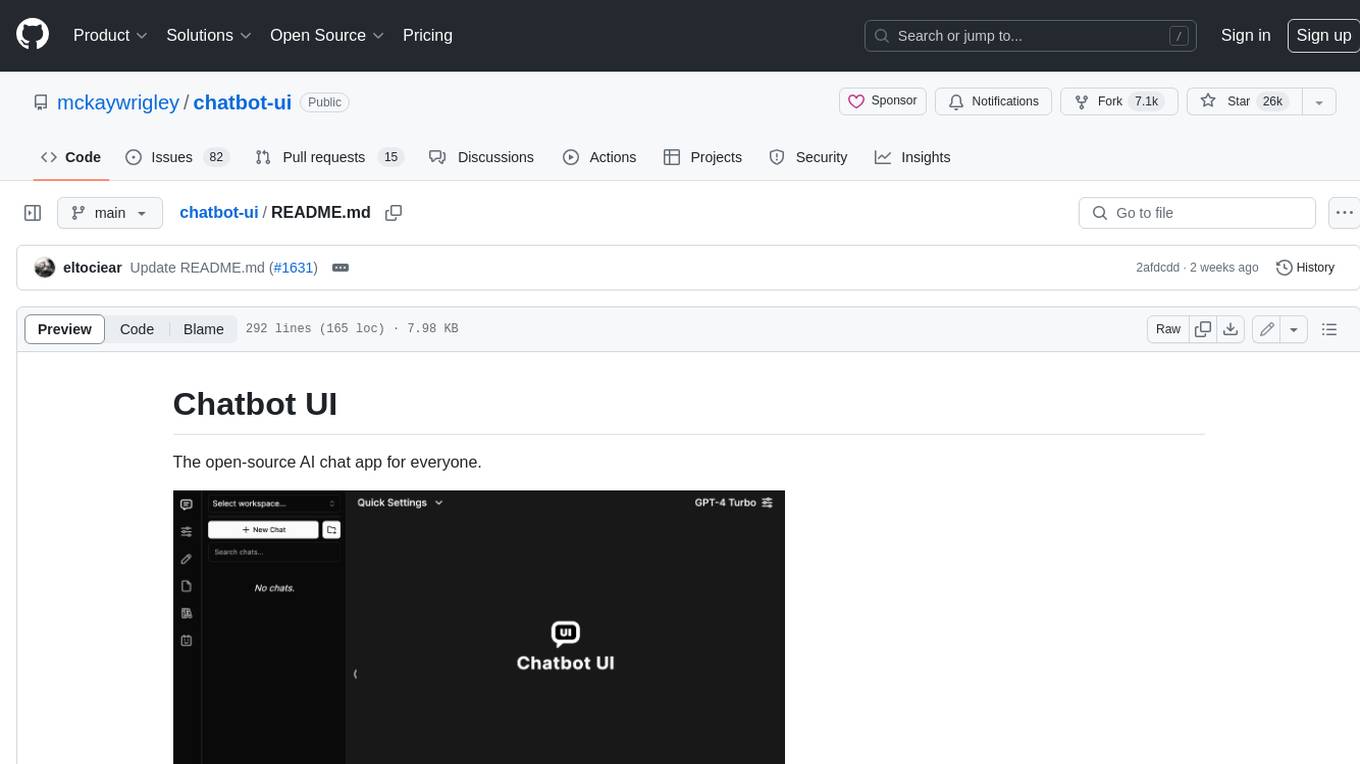
chatbot-ui
Chatbot UI is an open-source AI chat app that allows users to create and deploy their own AI chatbots. It is easy to use and can be customized to fit any need. Chatbot UI is perfect for businesses, developers, and anyone who wants to create a chatbot.
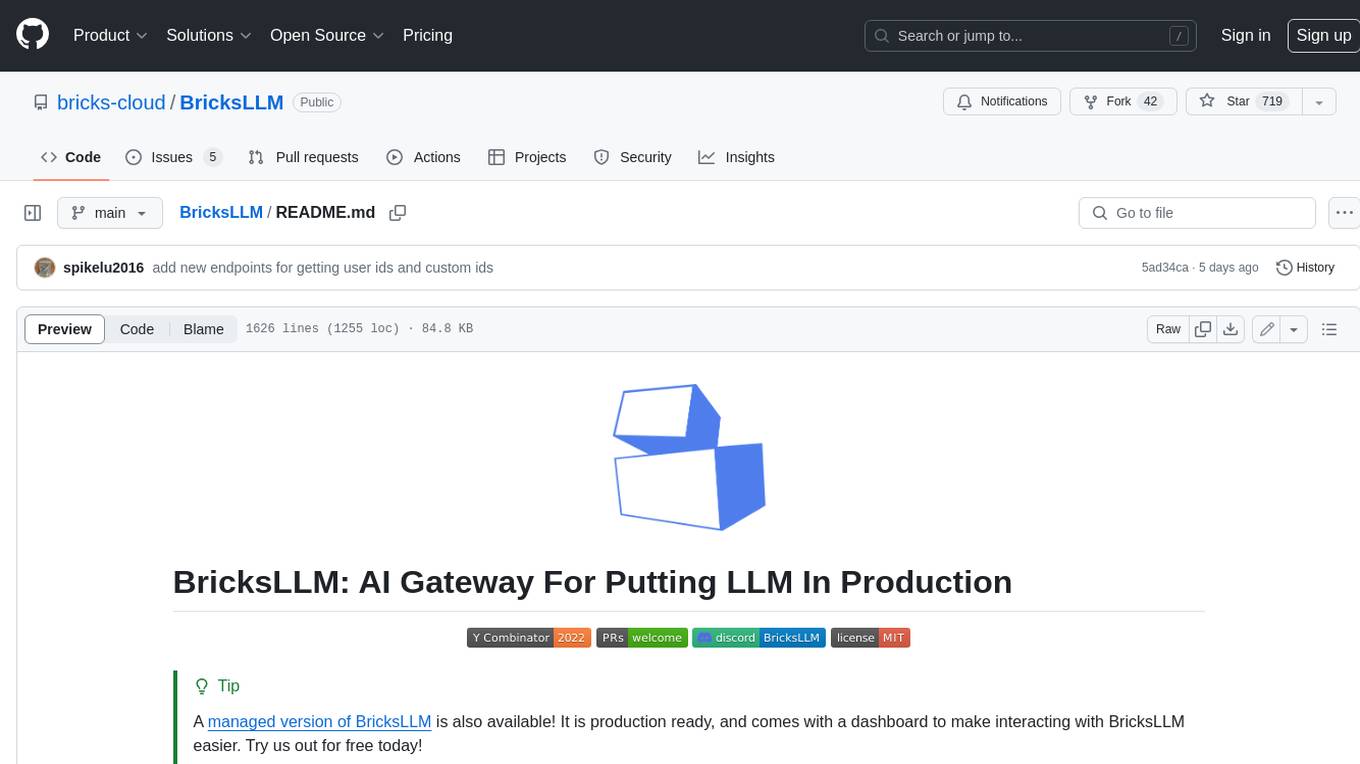
BricksLLM
BricksLLM is a cloud native AI gateway written in Go. Currently, it provides native support for OpenAI, Anthropic, Azure OpenAI and vLLM. BricksLLM aims to provide enterprise level infrastructure that can power any LLM production use cases. Here are some use cases for BricksLLM: * Set LLM usage limits for users on different pricing tiers * Track LLM usage on a per user and per organization basis * Block or redact requests containing PIIs * Improve LLM reliability with failovers, retries and caching * Distribute API keys with rate limits and cost limits for internal development/production use cases * Distribute API keys with rate limits and cost limits for students
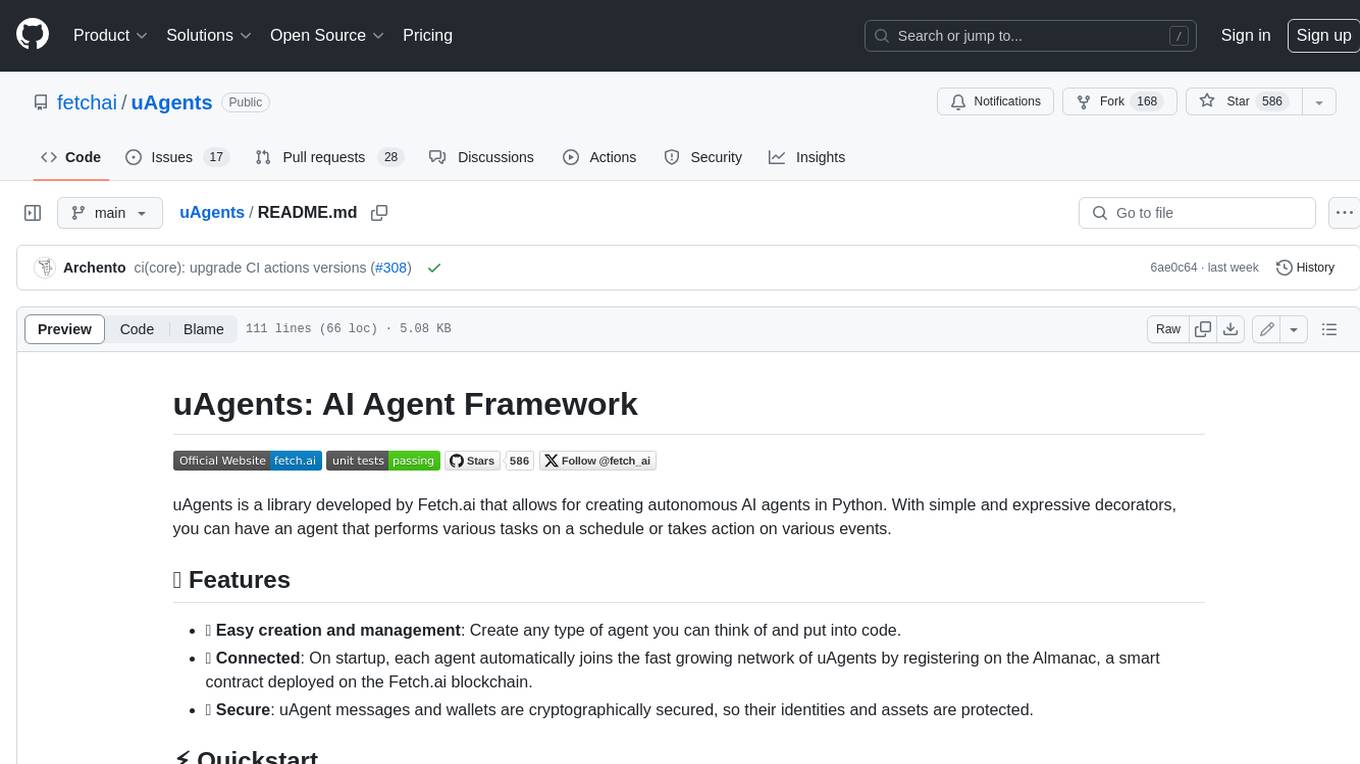
uAgents
uAgents is a Python library developed by Fetch.ai that allows for the creation of autonomous AI agents. These agents can perform various tasks on a schedule or take action on various events. uAgents are easy to create and manage, and they are connected to a fast-growing network of other uAgents. They are also secure, with cryptographically secured messages and wallets.
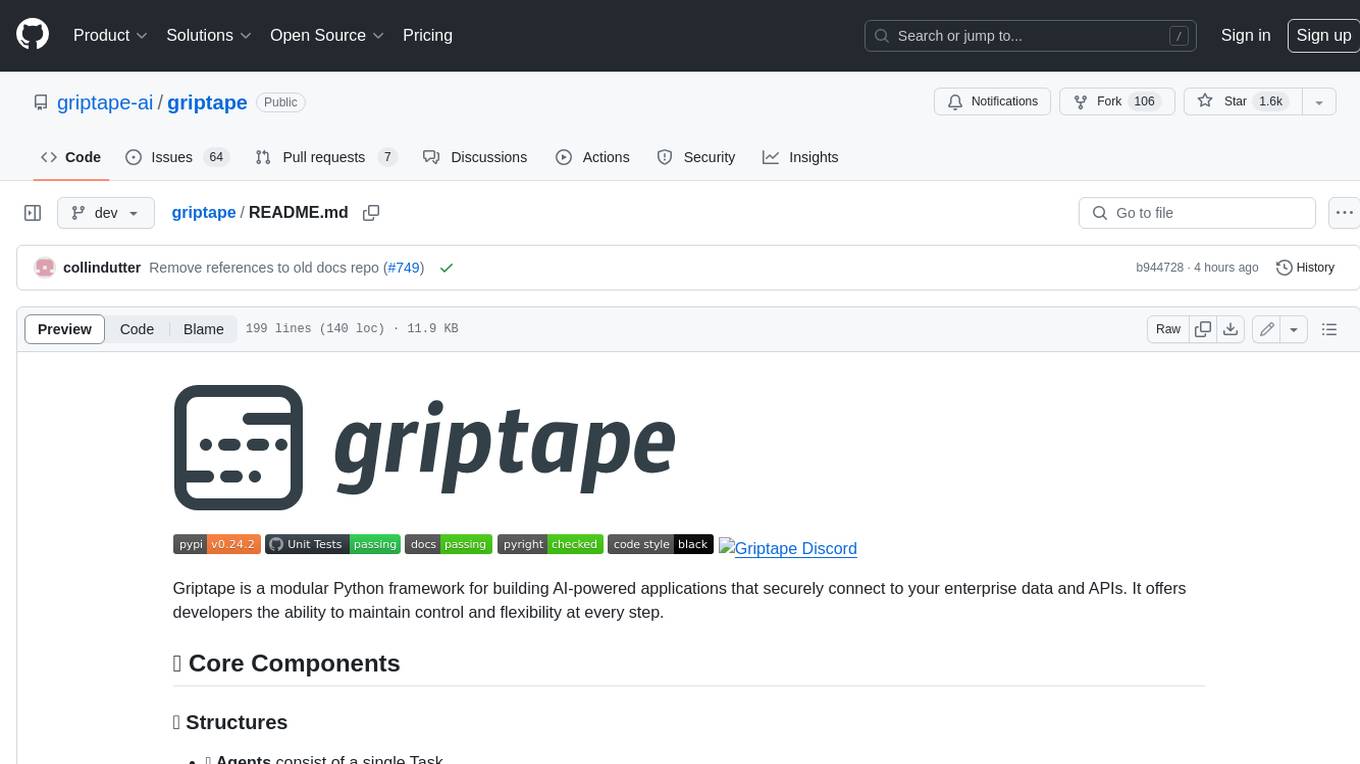
griptape
Griptape is a modular Python framework for building AI-powered applications that securely connect to your enterprise data and APIs. It offers developers the ability to maintain control and flexibility at every step. Griptape's core components include Structures (Agents, Pipelines, and Workflows), Tasks, Tools, Memory (Conversation Memory, Task Memory, and Meta Memory), Drivers (Prompt and Embedding Drivers, Vector Store Drivers, Image Generation Drivers, Image Query Drivers, SQL Drivers, Web Scraper Drivers, and Conversation Memory Drivers), Engines (Query Engines, Extraction Engines, Summary Engines, Image Generation Engines, and Image Query Engines), and additional components (Rulesets, Loaders, Artifacts, Chunkers, and Tokenizers). Griptape enables developers to create AI-powered applications with ease and efficiency.