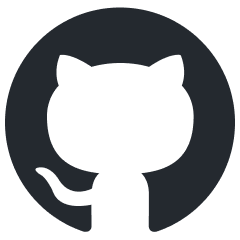
llms-txt
The /llms.txt file, helping language models use your website
Stars: 58
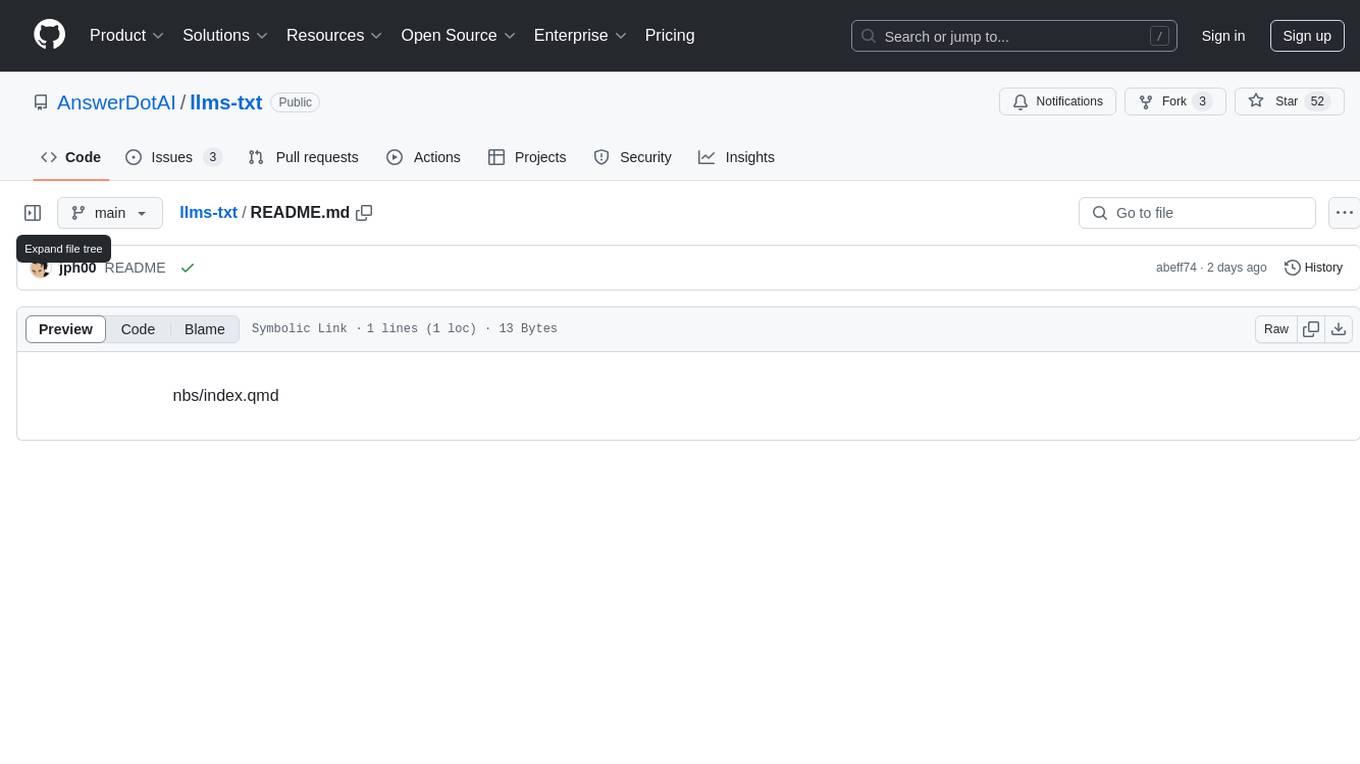
The llms-txt repository proposes a standardization on using an `/llms.txt` file to provide information to help large language models (LLMs) use a website at inference time. The `llms.txt` file is a markdown file that offers brief background information, guidance, and links to more detailed information in markdown files. It aims to provide concise and structured information for LLMs to access easily, helping users interact with websites via AI helpers. The repository also includes tools like a CLI and Python module for parsing `llms.txt` files and generating LLM context from them, along with a sample JavaScript implementation. The proposal suggests adding clean markdown versions of web pages alongside the original HTML pages to facilitate LLM readability and access to essential information.
README:
title: "The /llms.txt file"
date: 2024-09-03
author: "Jeremy Howard"
description: "A proposal to standardise on using an /llms.txt
file to provide information to help LLMs use a website at inference time."
image: "/sample.png"
Today websites are not just used to provide information to people, but they are also used to provide information to large language models. For instance, language models are often used to enhance development environments used by coders, with many systems including an option to ingest information about programming libraries and APIs from website documentation.
Providing information for language models is a little different to providing information for humans, although there is plenty of overlap. Language models generally like to have information in a more concise form. This can be more similar to what a human expert would want to read. Language models can ingest a lot of information quickly, so it can be helpful to have a single place where all of the key information can be collated---not for training (since training generally involved scraping all pages in all readable formats), but for helping users accessing the site via AI helpers.
Context windows are too small to handle most websites in their entirety, and converting HTML pages with complex navigation, ads, Javascript, etc into LLM-friendly plain text documents is difficult and imprecise. Therefore it would be helpful if there was a way to identify the most important information to provide to AI helpers, in the most appropriate form.
{.lightbox width=150px .floatr}
We propose that those interested in providing LLM-friendly content add a /llms.txt
file to their site. This is a markdown file that provides brief background information and guidance, along with links to markdown files (which can also link to external sites) providing more detailed information. This can be used, for instance, in order to provide information necessary for coders to use a library, or as part of research to learn about a person or organization and so forth. You are free to use the llms.txt
logo on your site to indicate your support if you wish.
llms.txt markdown is human and LLM readable, but is also in a precise format allowing fixed processing methods (i.e. classical programming techniques such as parsers and regex). For instance, there is an llms-txt project providing a CLI and Python module for parsing llms.txt
files and generating LLM context from them. There is also a sample JavaScript implementation.
We furthermore propose that pages on websites that have information that might be useful for LLMs to read provide a clean markdown version of those pages at the same URL as the original page, but with .md
appended. (URLs without file names should append index.html.md
instead.)
The FastHTML project follows these two proposals for its documentation. For instance, here is the FastHTML docs llms.txt. And here is an example of a regular HTML docs page, along with exact same URL but with a .md extension. Note that all nbdev projects now create .md versions of all pages by default, and all Answer.AI and fast.ai software projects using nbdev have had their docs regenerated with this feature---for instance, see the markdown version of fastcore's docments module.
This proposal does not include any particular recommendation for how to process the file, since it will depend on the application. For example, FastHTML automatically builds a new version of two markdown files including the contents of the linked URLs, using an XML-based structure suitable for use in LLMs such as Claude. The two files are: llms-ctx.txt, which does not include the optional URLs, and llms-ctx-full.txt, which does include them. They are created using the llms_txt2ctx
command line application, and the FastHTML documentation includes information for users about how to use them.
llms.txt files can be used in various scenarios. For software libraries, they can provide a structured overview of documentation, making it easier for LLMs to locate specific features or usage examples. In corporate websites, they can outline organizational structure and key information sources. Information about new legislation and necessary background and context could be curated in an llms.txt
file to help stakeholders understand it.
llms.txt files can be adapted for various domains. Personal portfolio or CV websites could use them to help answer questions about an individual. In e-commerce, they could outline product categories and policies. Educational institutions might use them to summarize course offerings and resources.
At the moment the most widely and easily understood format for language models is Markdown. Simply showing where key Markdown files can be found is a great first step. Providing some basic structure helps a language model to find where the information it needs can come from.
The llms.txt
file is unusual in that it uses Markdown to structure the information rather than a classic structured format such as XML. The reason for this is that we expect many of these files to be read by language models and agents. Having said that, the information in llms.txt
follows a specific format and can be read using standard programmatic-based tools.
The llms.txt
file spec is for files located in the root path /llms.txt
of a website (or, optionally, in a subpath). A file following the spec contains the following sections as markdown, in the specific order:
- An H1 with the name of the project or site. This is the only required section
- A blockquote with a short summary of the project, containing key information necessary for understanding the rest of the file
- Zero or more markdown sections (e.g. paragraphs, lists, etc) of any type except headings, containing more detailed information about the project and how to interpret the provided files
- Zero or more markdown sections delimited by H2 headers, containing "file lists" of URLs where further detail is available
- Each "file list" is a markdown list, containing a required markdown hyperlink
[name](url)
, then optionally a:
and notes about the file.
- Each "file list" is a markdown list, containing a required markdown hyperlink
Here is a mock example:
# Title
> Optional description goes here
Optional details go here
## Section name
- [Link title](https://link_url): Optional link details
## Optional
- [Link title](https://link_url)
Note that the "Optional" section has a special meaning---if it's included, the URLs provided there can be skipped if a shorter context is needed. Use it for secondary information which can often be skipped.
llms.txt is designed to coexist with current web standards. While sitemaps list all pages for search engines, llms.txt
offers a curated overview for LLMs. It can complement robots.txt by providing context for allowed content. The file can also reference structured data markup used on the site, helping LLMs understand how to interpret this information in context.
The approach of standardising on a path for the file follows the approach of /robots.txt
and /sitemap.xml
. robots.txt and llms.txt
have different purposes---robots.txt is generally used to let automated tools what access to a site is considered acceptable, such as for search indexing bots. On the other hand, llms.txt
information will often be used on demand when a user explicitly requesting information about a topic, such as when including a coding library's documentation in a project, or when asking a chat bot with search functiontionality for information. Our expectation is that llms.txt
will mainly be useful for inference, i.e. at the time a user is seeking assistance, as opposed to for training. However, perhaps if llms.txt
usage becomes widespread, future training runs could take advantage of the information in llms.txt
files too.
sitemap.xml is a list of all the indexable human-readable information available on a site. This isn’t a substitute for llms.txt
since it:
- Often won’t have the LLM-readable versions of pages listed
- Doesn’t include URLs to external sites, even although they might be helpful to understand the information
- Will generally cover documents that in aggregate will be too large to fit in an LLM context window, and will include a lot of information that isn’t necessary to understand the site.
Here’s an example of llms.txt
, in this case a cut down version of the file used for the FastHTML project (see also the full version:
# FastHTML
> FastHTML is a python library which brings together Starlette, Uvicorn, HTMX, and fastcore's `FT` "FastTags" into a library for creating server-rendered hypermedia applications.
Important notes:
- Although parts of its API are inspired by FastAPI, it is *not* compatible with FastAPI syntax and is not targeted at creating API services
- FastHTML is compatible with JS-native web components and any vanilla JS library, but not with React, Vue, or Svelte.
## Docs
- [FastHTML quick start](https://docs.fastht.ml/path/quickstart.html.md): A brief overview of many FastHTML features
- [HTMX reference](https://raw.githubusercontent.com/path/reference.md): Brief description of all HTMX attributes, CSS classes, headers, events, extensions, js lib methods, and config options
## Examples
- [Todo list application](https://raw.githubusercontent.com/path/adv_app.py): Detailed walk-thru of a complete CRUD app in FastHTML showing idiomatic use of FastHTML and HTMX patterns.
## Optional
- [Starlette full documentation](https://gist.githubusercontent.com/path/starlette-sml.md): A subset of the Starlette documentation useful for FastHTML development.
To create effective llms.txt
files, consider these guidelines: Use concise, clear language. When linking to resources, include brief, informative descriptions. Avoid ambiguous terms or unexplained jargon. Run a tool that expands your llms.txt
file into an LLM context file and test a number of language models to see if they can answer questions about your content.
The llms.txt
specification is open for community input. A GitHub repository hosts this informal overview, allowing for version control and public discussion. A community discord channel is available for sharing implementation experiences and discussing best practices.
For Tasks:
Click tags to check more tools for each tasksFor Jobs:
Alternative AI tools for llms-txt
Similar Open Source Tools
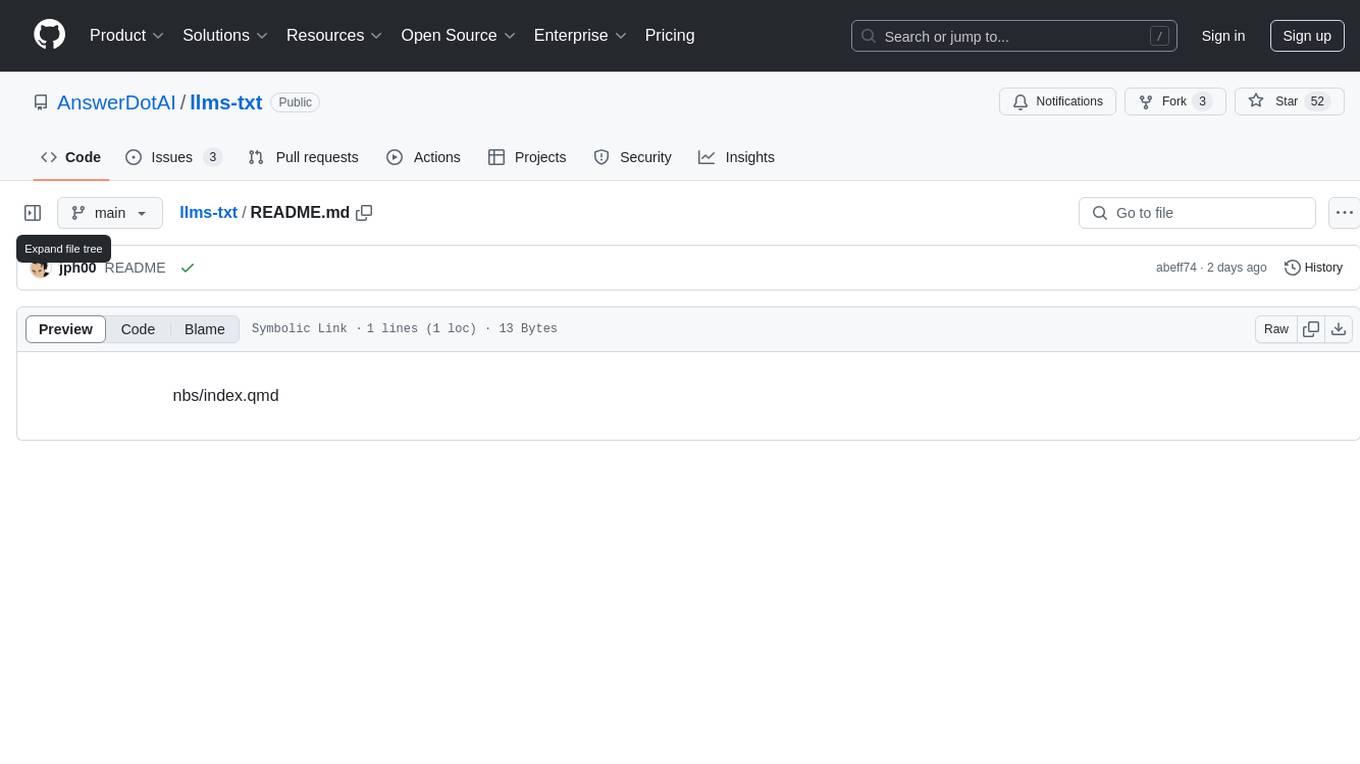
llms-txt
The llms-txt repository proposes a standardization on using an `/llms.txt` file to provide information to help large language models (LLMs) use a website at inference time. The `llms.txt` file is a markdown file that offers brief background information, guidance, and links to more detailed information in markdown files. It aims to provide concise and structured information for LLMs to access easily, helping users interact with websites via AI helpers. The repository also includes tools like a CLI and Python module for parsing `llms.txt` files and generating LLM context from them, along with a sample JavaScript implementation. The proposal suggests adding clean markdown versions of web pages alongside the original HTML pages to facilitate LLM readability and access to essential information.
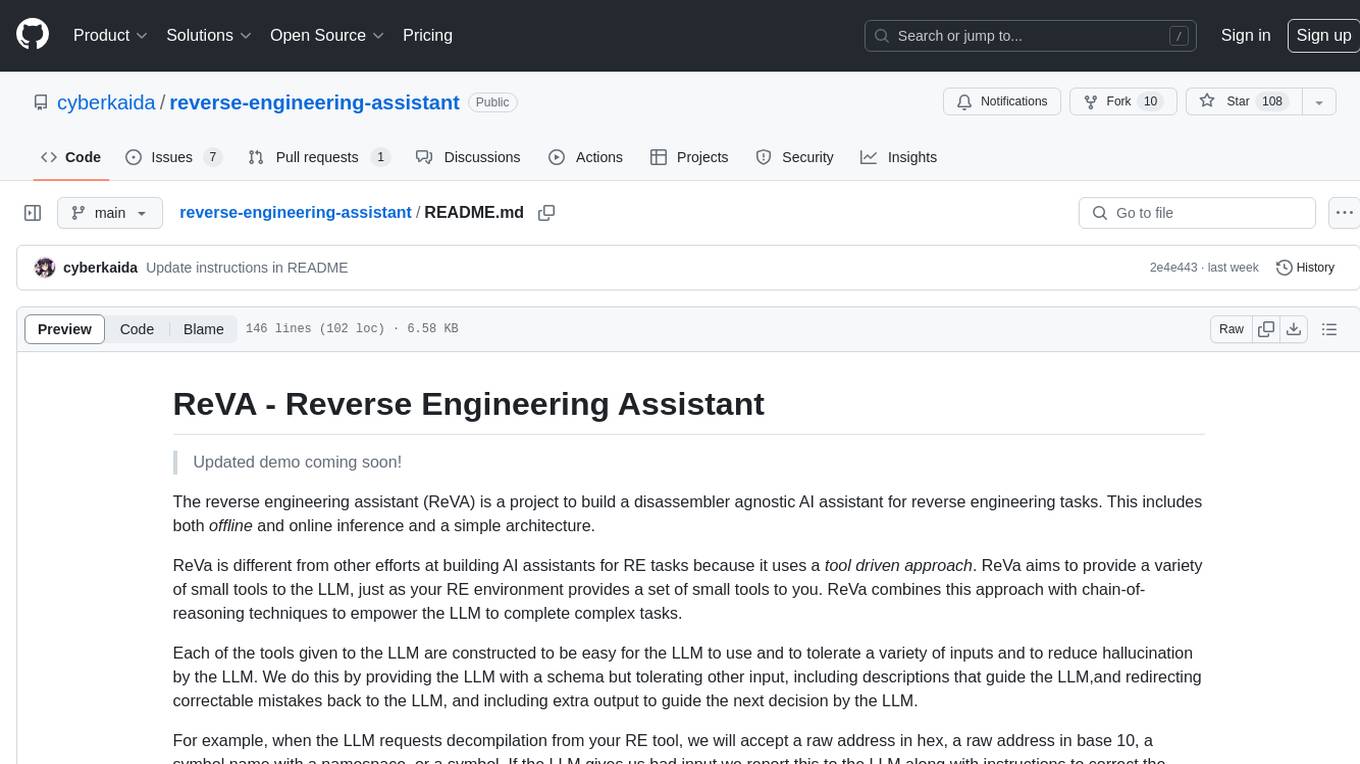
reverse-engineering-assistant
ReVA (Reverse Engineering Assistant) is a project aimed at building a disassembler agnostic AI assistant for reverse engineering tasks. It utilizes a tool-driven approach, providing small tools to the user to empower them in completing complex tasks. The assistant is designed to accept various inputs, guide the user in correcting mistakes, and provide additional context to encourage exploration. Users can ask questions, perform tasks like decompilation, class diagram generation, variable renaming, and more. ReVA supports different language models for online and local inference, with easy configuration options. The workflow involves opening the RE tool and program, then starting a chat session to interact with the assistant. Installation includes setting up the Python component, running the chat tool, and configuring the Ghidra extension for seamless integration. ReVA aims to enhance the reverse engineering process by breaking down actions into small parts, including the user's thoughts in the output, and providing support for monitoring and adjusting prompts.
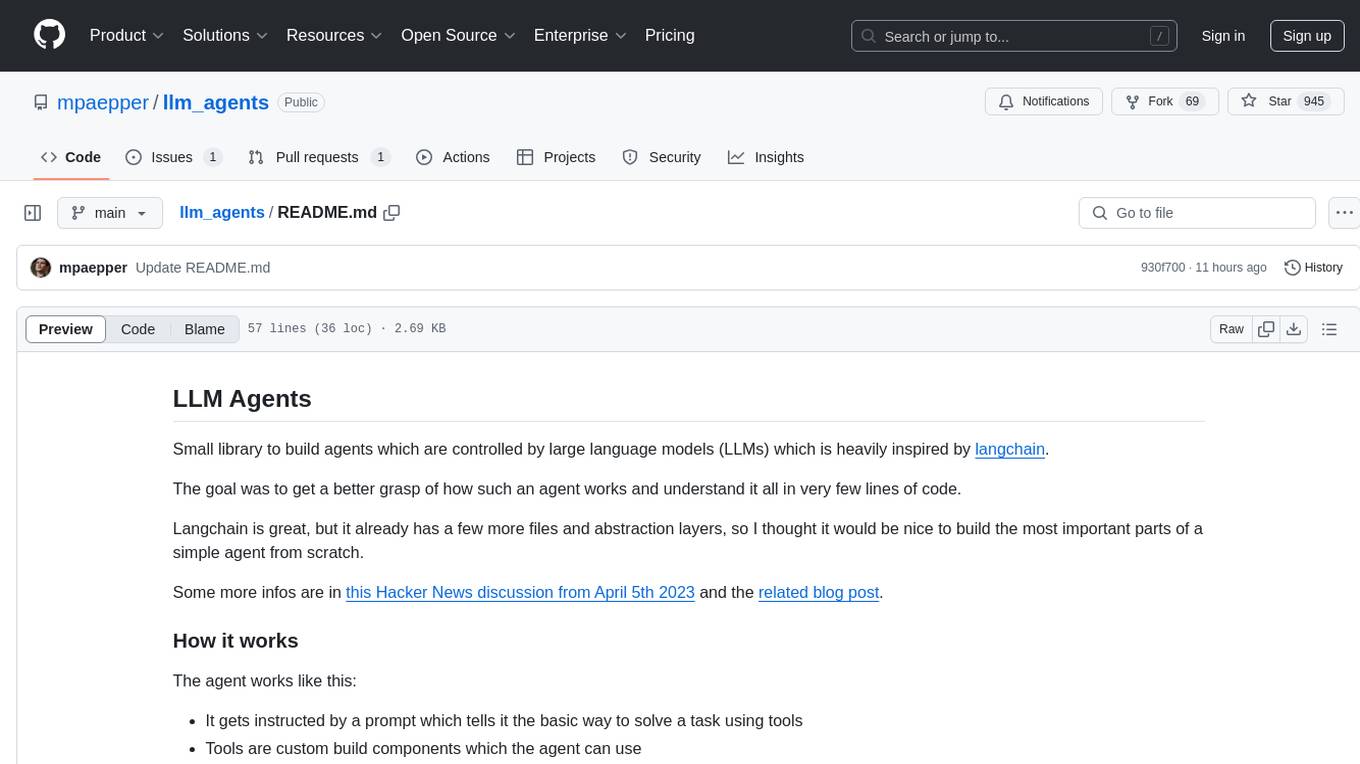
llm_agents
LLM Agents is a small library designed to build agents controlled by large language models. It aims to provide a better understanding of how such agents work in a concise manner. The library allows agents to be instructed by prompts, use custom-built components as tools, and run in a loop of Thought, Action, Observation. The agents leverage language models to generate Thought and Action, while tools like Python REPL, Google search, and Hacker News search provide Observations. The library requires setting up environment variables for OpenAI API and SERPAPI API keys. Users can create their own agents by importing the library and defining tools accordingly.
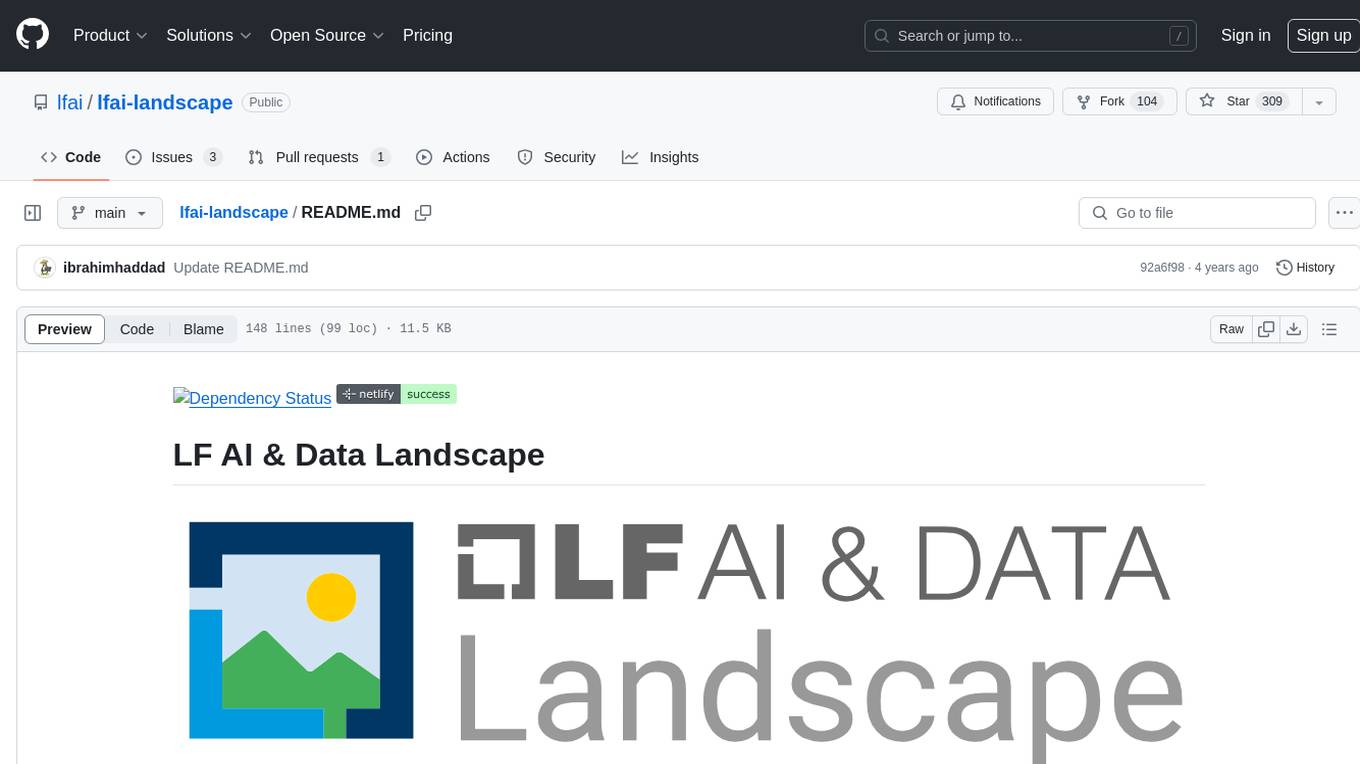
lfai-landscape
LF AI & Data Landscape is a map to explore open source projects in the AI & Data domains, highlighting companies that are members of LF AI & Data. It showcases members of the Foundation and is modelled after the Cloud Native Computing Foundation landscape. The landscape includes current version, interactive version, new entries, logos, proper SVGs, corrections, external data, best practices badge, non-updated items, license, formats, installation, vulnerability reporting, and adjusting the landscape view.
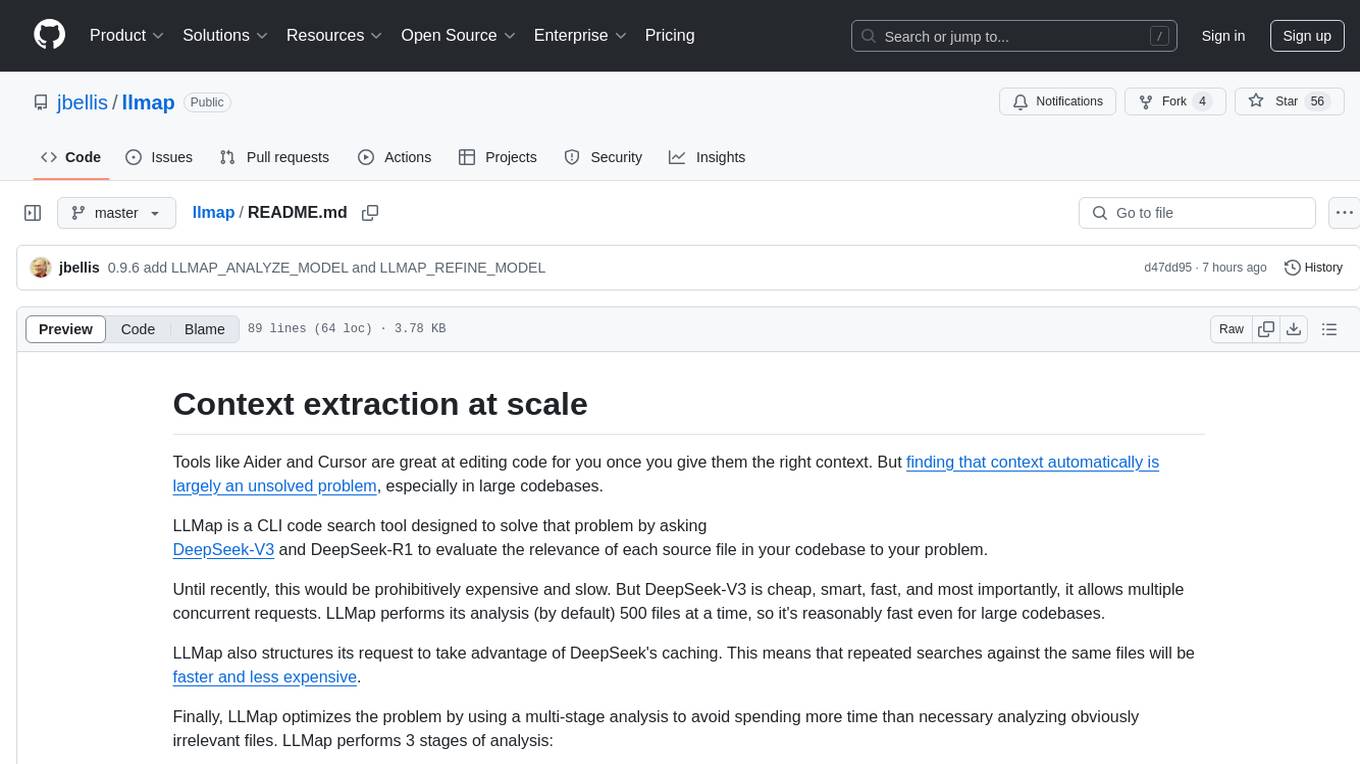
llmap
LLMap is a CLI code search tool designed to automatically find context in large codebases by evaluating the relevance of each source file using DeepSeek-V3 and DeepSeek-R1. It optimizes analysis by performing multi-stage analysis and caching results for faster searches. Currently supports Java and Python files, with potential for extension to other languages. Install with 'pip install llmap-ai' and use with a DeepSeek API key to search for specific context in code.
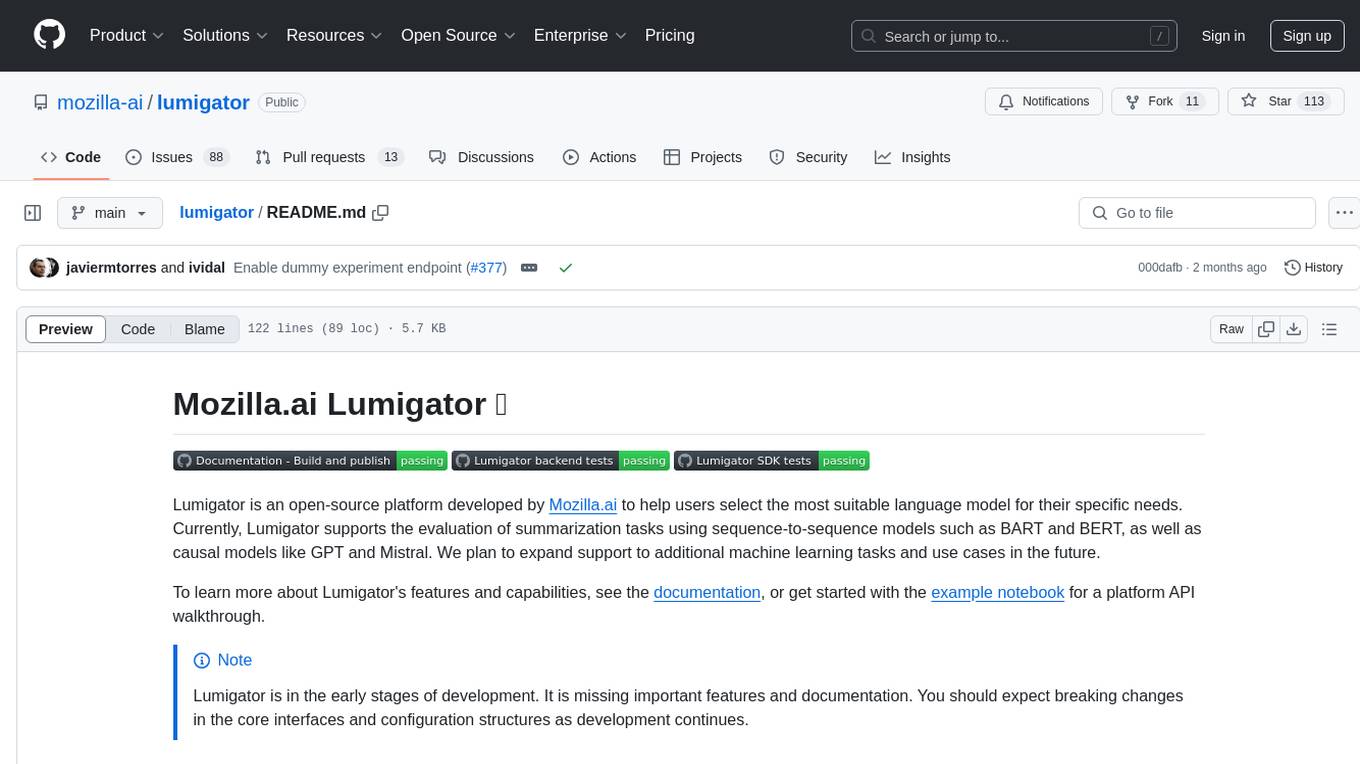
lumigator
Lumigator is an open-source platform developed by Mozilla.ai to help users select the most suitable language model for their specific needs. It supports the evaluation of summarization tasks using sequence-to-sequence models such as BART and BERT, as well as causal models like GPT and Mistral. The platform aims to make model selection transparent, efficient, and empowering by providing a framework for comparing LLMs using task-specific metrics to evaluate how well a model fits a project's needs. Lumigator is in the early stages of development and plans to expand support to additional machine learning tasks and use cases in the future.
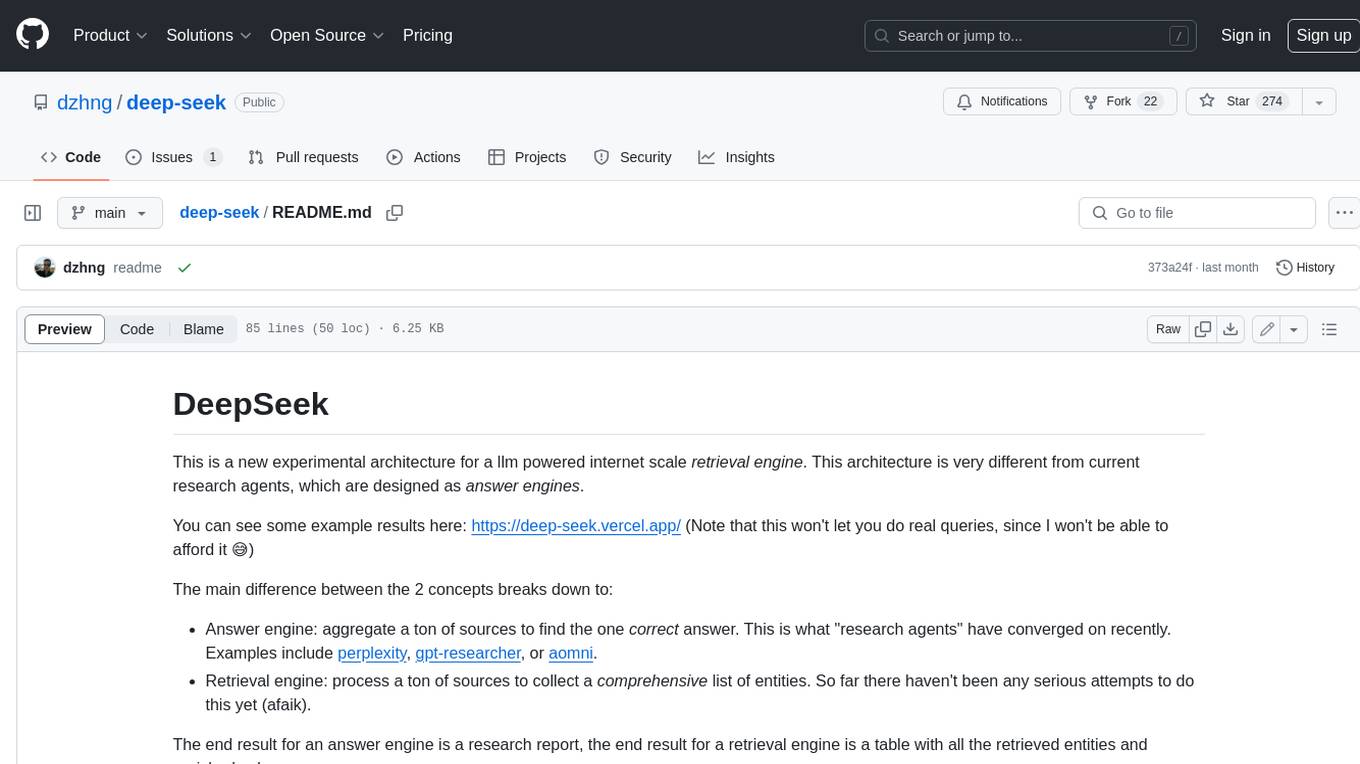
deep-seek
DeepSeek is a new experimental architecture for a large language model (LLM) powered internet-scale retrieval engine. Unlike current research agents designed as answer engines, DeepSeek aims to process a vast amount of sources to collect a comprehensive list of entities and enrich them with additional relevant data. The end result is a table with retrieved entities and enriched columns, providing a comprehensive overview of the topic. DeepSeek utilizes both standard keyword search and neural search to find relevant content, and employs an LLM to extract specific entities and their associated contents. It also includes a smaller answer agent to enrich the retrieved data, ensuring thoroughness. DeepSeek has the potential to revolutionize research and information gathering by providing a comprehensive and structured way to access information from the vastness of the internet.
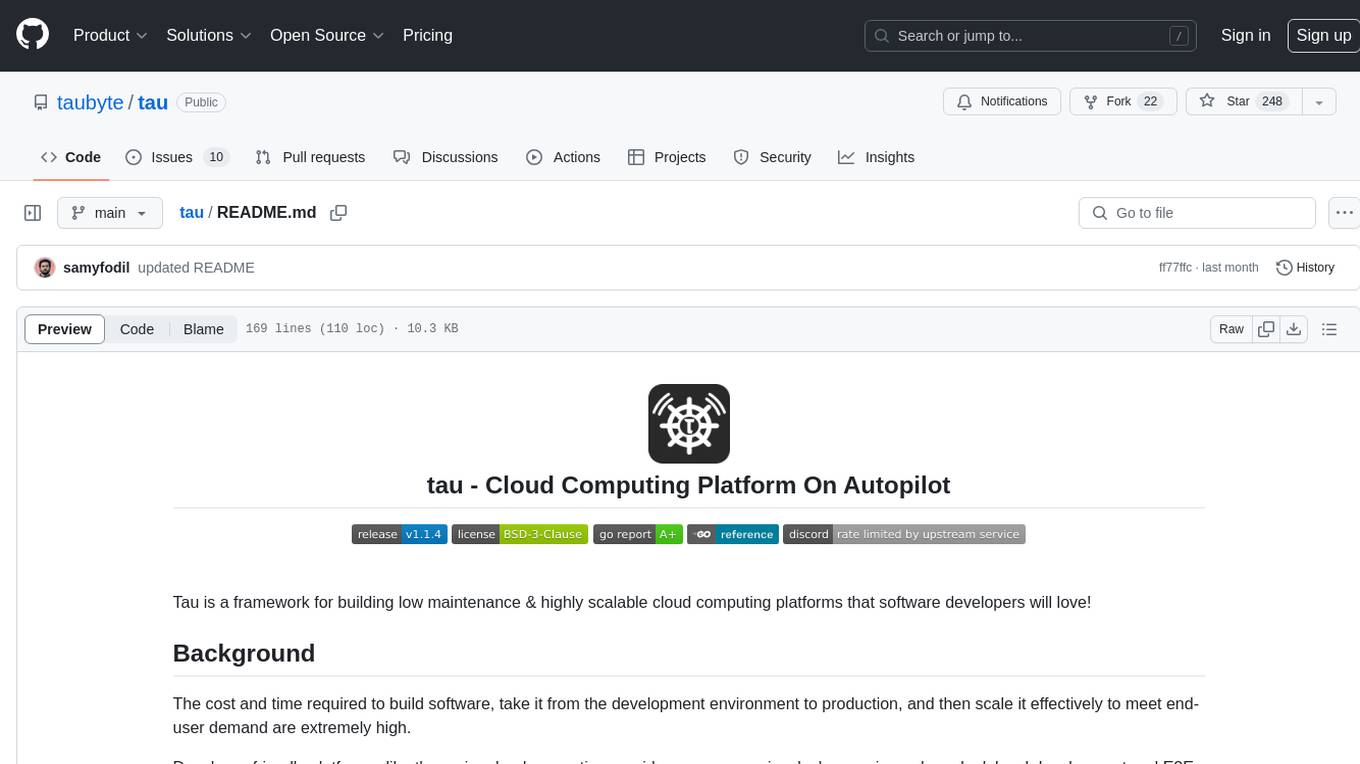
tau
Tau is a framework for building low maintenance & highly scalable cloud computing platforms that software developers will love. It aims to solve the high cost and time required to build, deploy, and scale software by providing a developer-friendly platform that offers autonomy and flexibility. Tau simplifies the process of building and maintaining a cloud computing platform, enabling developers to achieve 'Local Coding Equals Global Production' effortlessly. With features like auto-discovery, content-addressing, and support for WebAssembly, Tau empowers users to create serverless computing environments, host frontends, manage databases, and more. The platform also supports E2E testing and can be extended using a plugin system called orbit.
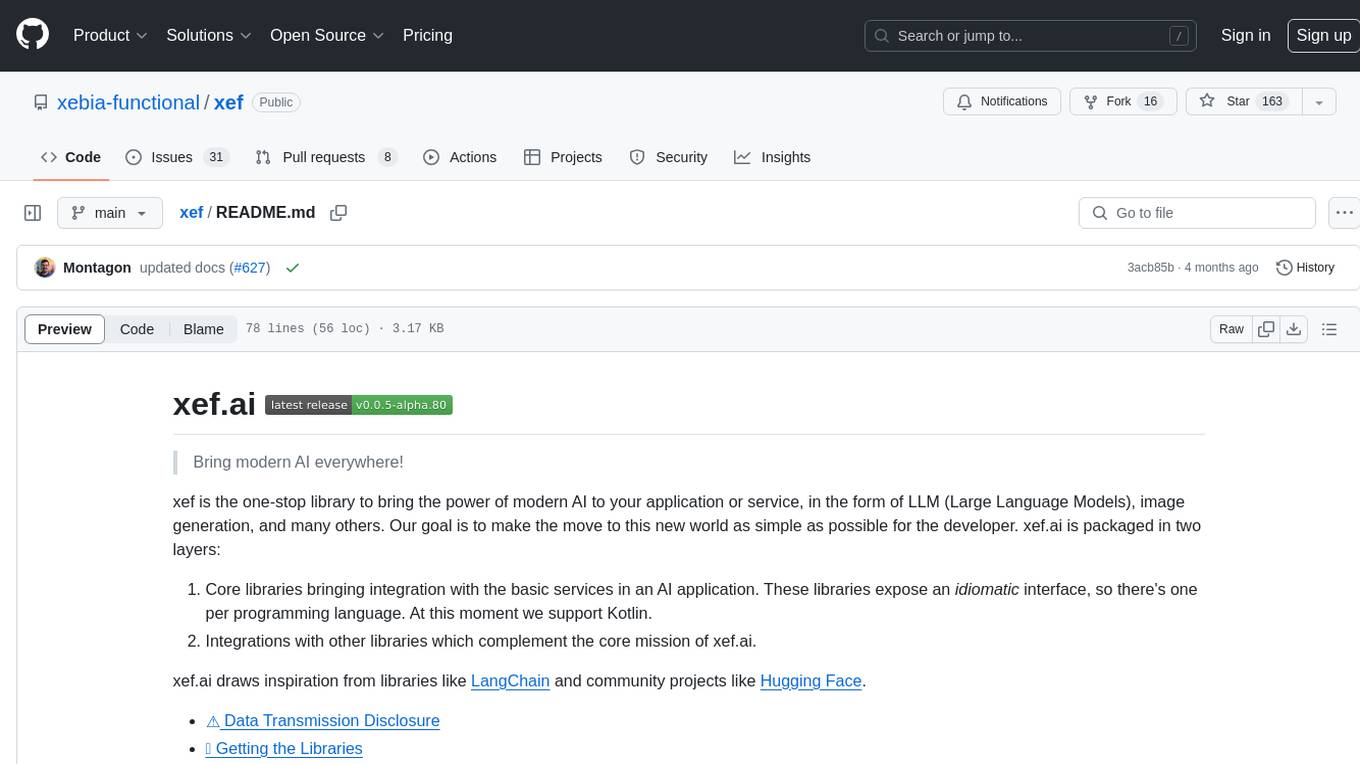
xef
xef.ai is a one-stop library designed to bring the power of modern AI to applications and services. It offers integration with Large Language Models (LLM), image generation, and other AI services. The library is packaged in two layers: core libraries for basic AI services integration and integrations with other libraries. xef.ai aims to simplify the transition to modern AI for developers by providing an idiomatic interface, currently supporting Kotlin. Inspired by LangChain and Hugging Face, xef.ai may transmit source code and user input data to third-party services, so users should review privacy policies and take precautions. Libraries are available in Maven Central under the `com.xebia` group, with `xef-core` as the core library. Developers can add these libraries to their projects and explore examples to understand usage.
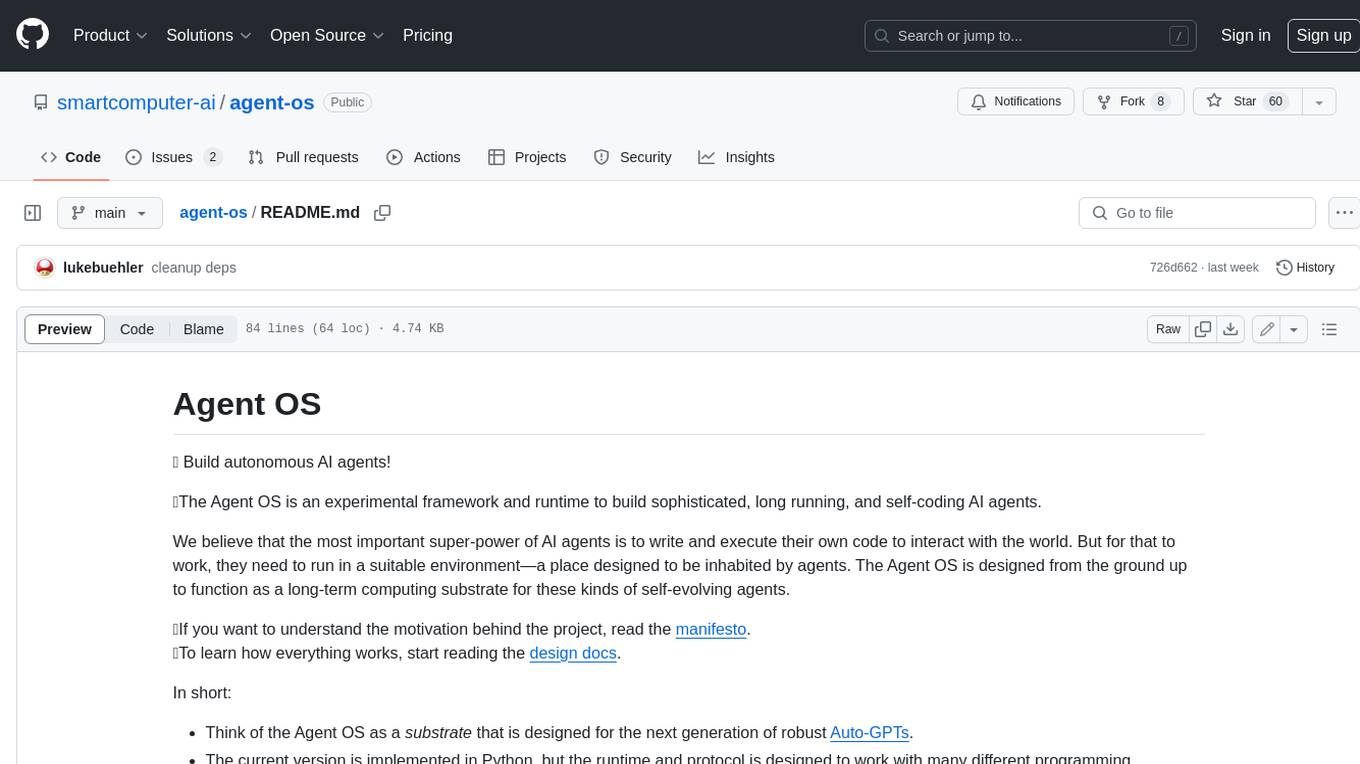
agent-os
The Agent OS is an experimental framework and runtime to build sophisticated, long running, and self-coding AI agents. We believe that the most important super-power of AI agents is to write and execute their own code to interact with the world. But for that to work, they need to run in a suitable environment—a place designed to be inhabited by agents. The Agent OS is designed from the ground up to function as a long-term computing substrate for these kinds of self-evolving agents.
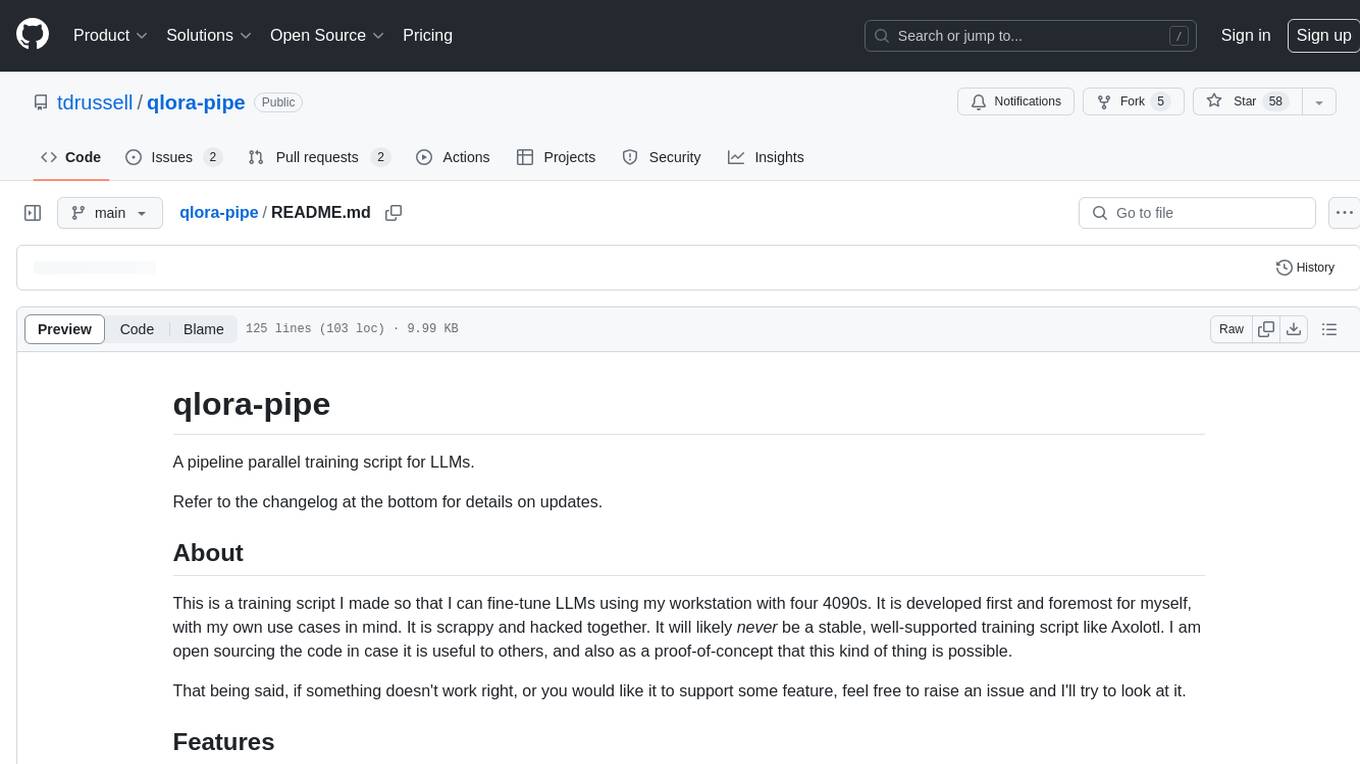
qlora-pipe
qlora-pipe is a pipeline parallel training script designed for efficiently training large language models that cannot fit on one GPU. It supports QLoRA, LoRA, and full fine-tuning, with efficient model loading and the ability to load any dataset that Axolotl can handle. The script allows for raw text training, resuming training from a checkpoint, logging metrics to Tensorboard, specifying a separate evaluation dataset, training on multiple datasets simultaneously, and supports various models like Llama, Mistral, Mixtral, Qwen-1.5, and Cohere (Command R). It handles pipeline- and data-parallelism using Deepspeed, enabling users to set the number of GPUs, pipeline stages, and gradient accumulation steps for optimal utilization.
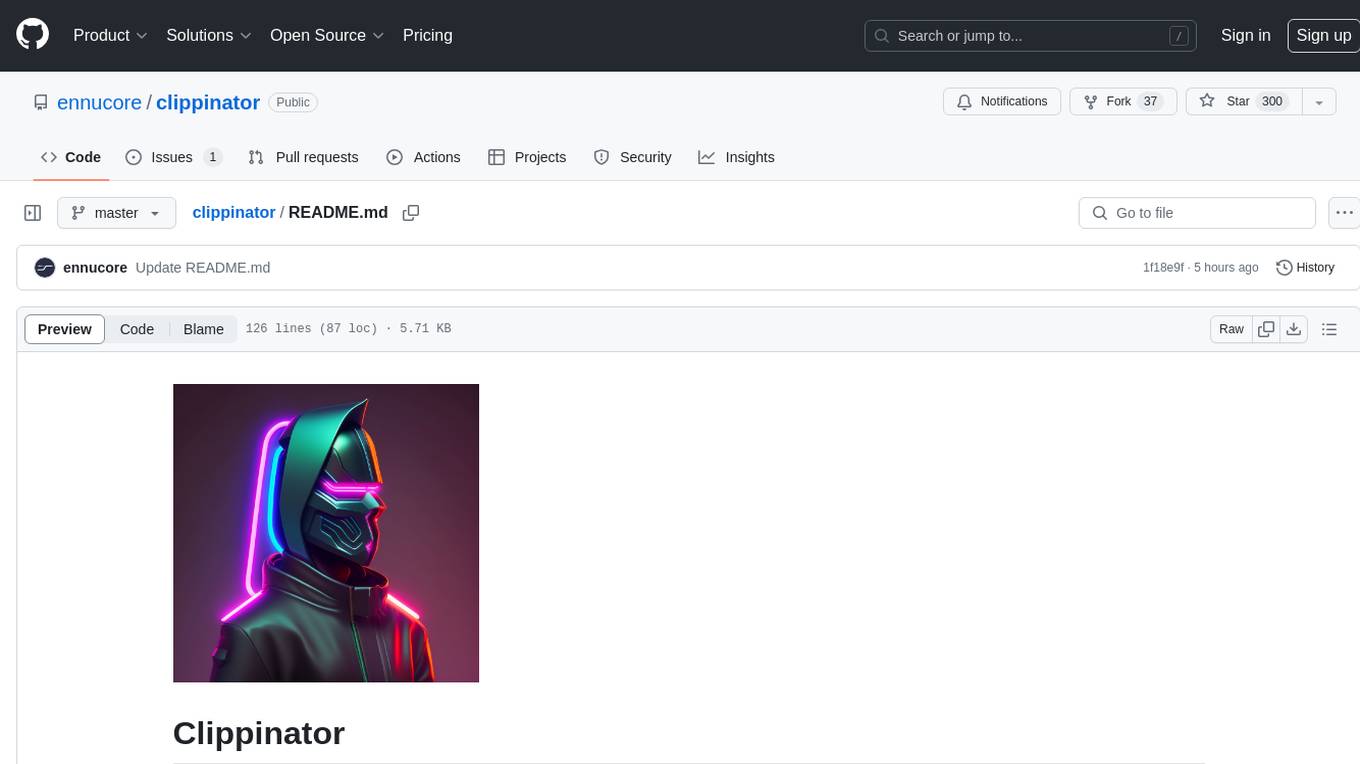
clippinator
Clippinator is a code assistant tool that helps users develop code autonomously by planning, writing, debugging, and testing projects. It consists of agents based on GPT-4 that work together to assist the user in coding tasks. The main agent, Taskmaster, delegates tasks to specialized subagents like Architect, Writer, Frontender, Editor, QA, and Devops. The tool provides project architecture, tools for file and terminal operations, browser automation with Selenium, linting capabilities, CI integration, and memory management. Users can interact with the tool to provide feedback and guide the coding process, making it a powerful tool when combined with human intervention.
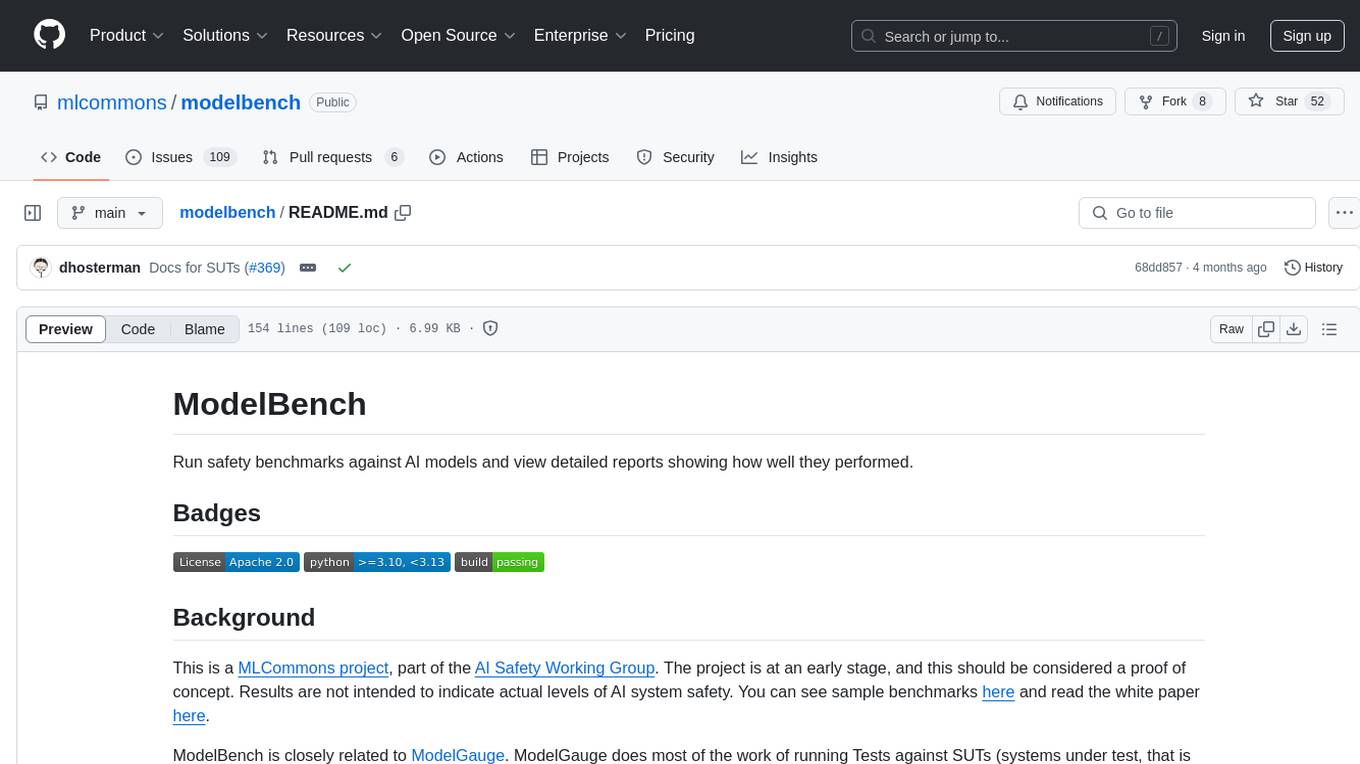
modelbench
ModelBench is a tool for running safety benchmarks against AI models and generating detailed reports. It is part of the MLCommons project and is designed as a proof of concept to aggregate measures, relate them to specific harms, create benchmarks, and produce reports. The tool requires LlamaGuard for evaluating responses and a TogetherAI account for running benchmarks. Users can install ModelBench from GitHub or PyPI, run tests using Poetry, and create benchmarks by providing necessary API keys. The tool generates static HTML pages displaying benchmark scores and allows users to dump raw scores and manage cache for faster runs. ModelBench is aimed at enabling users to test their own models and create tests and benchmarks.
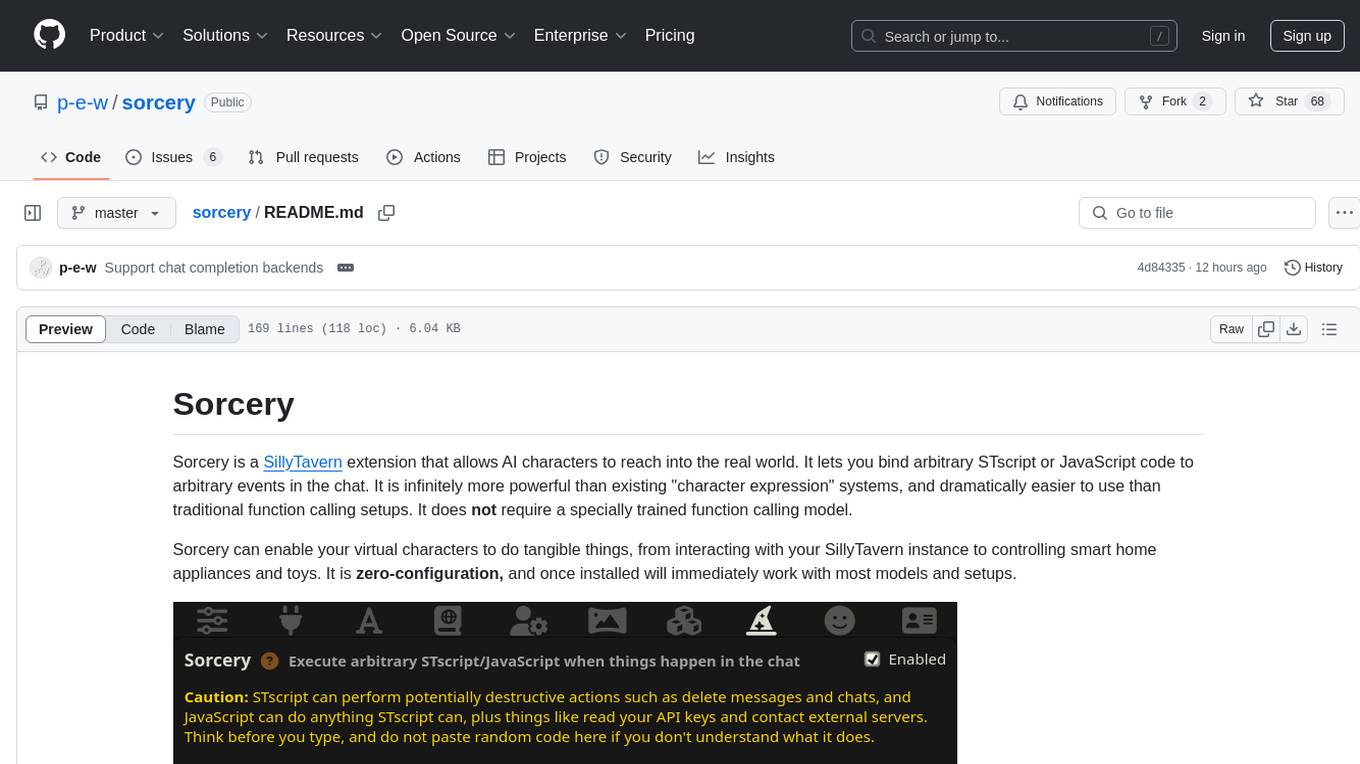
sorcery
Sorcery is a SillyTavern extension that allows AI characters to interact with the real world by executing user-defined scripts at specific events in the chat. It is easy to use and does not require a specially trained function calling model. Sorcery can be used to control smart home appliances, interact with virtual characters, and perform various tasks in the chat environment. It works by injecting instructions into the system prompt and intercepting markers to run associated scripts, providing a seamless user experience.
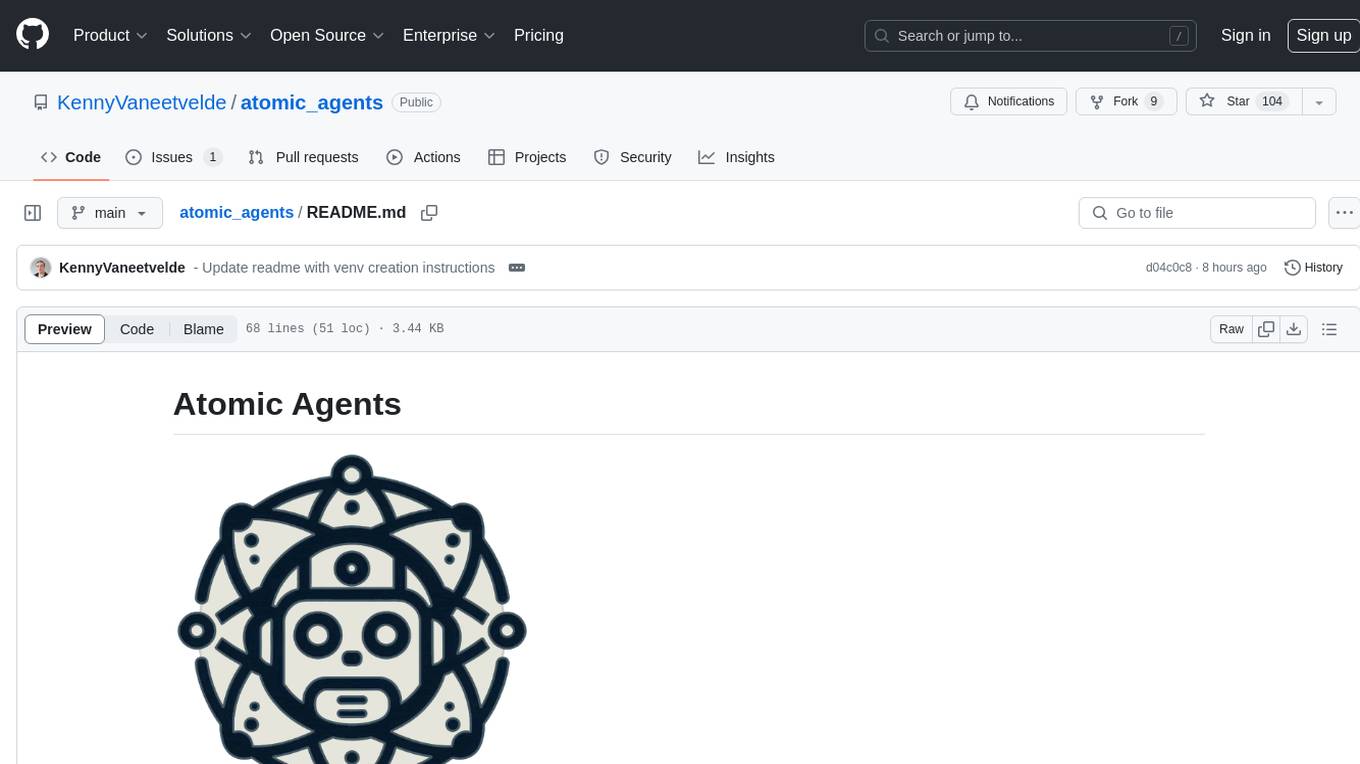
atomic_agents
Atomic Agents is a modular and extensible framework designed for creating powerful applications. It follows the principles of Atomic Design, emphasizing small and single-purpose components. Leveraging Pydantic for data validation and serialization, the framework offers a set of tools and agents that can be combined to build AI applications. It depends on the Instructor package and supports various APIs like OpenAI, Cohere, Anthropic, and Gemini. Atomic Agents is suitable for developers looking to create AI agents with a focus on modularity and flexibility.
For similar tasks
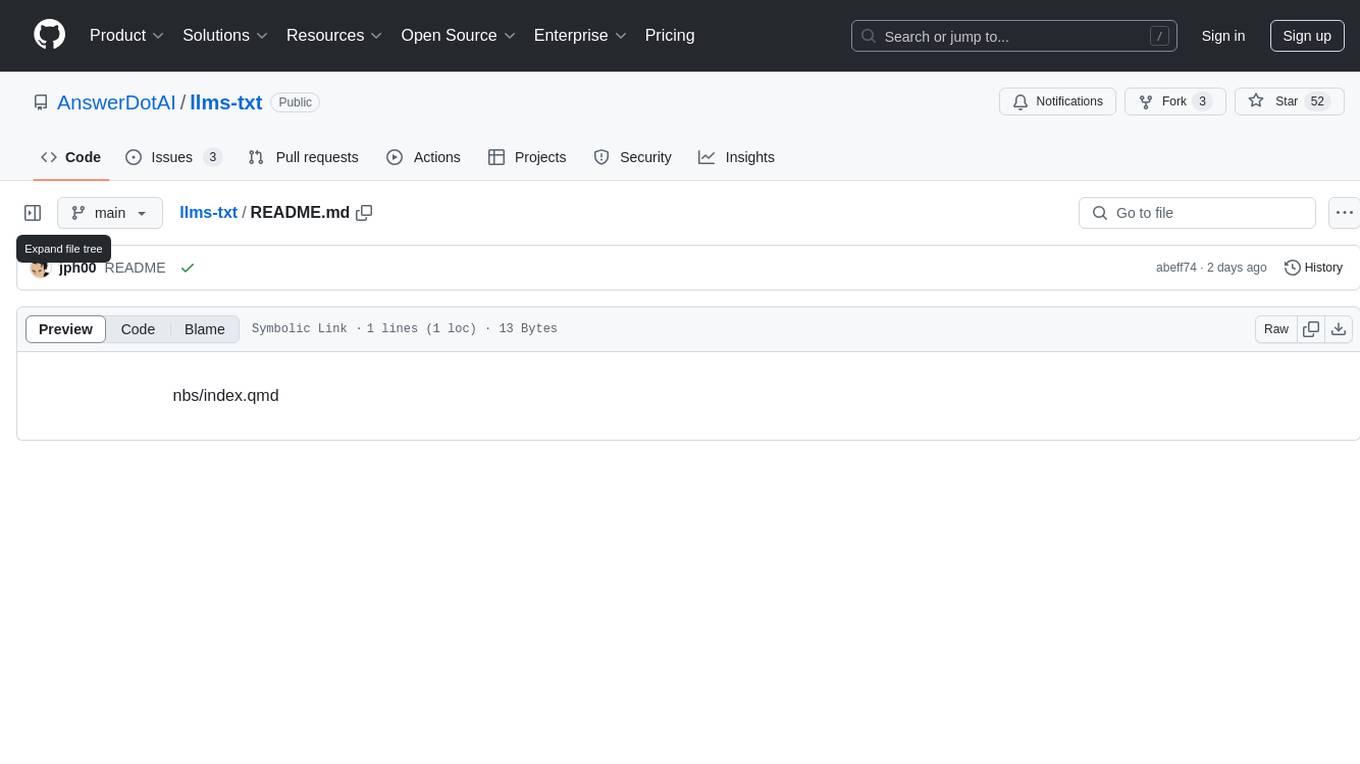
llms-txt
The llms-txt repository proposes a standardization on using an `/llms.txt` file to provide information to help large language models (LLMs) use a website at inference time. The `llms.txt` file is a markdown file that offers brief background information, guidance, and links to more detailed information in markdown files. It aims to provide concise and structured information for LLMs to access easily, helping users interact with websites via AI helpers. The repository also includes tools like a CLI and Python module for parsing `llms.txt` files and generating LLM context from them, along with a sample JavaScript implementation. The proposal suggests adding clean markdown versions of web pages alongside the original HTML pages to facilitate LLM readability and access to essential information.
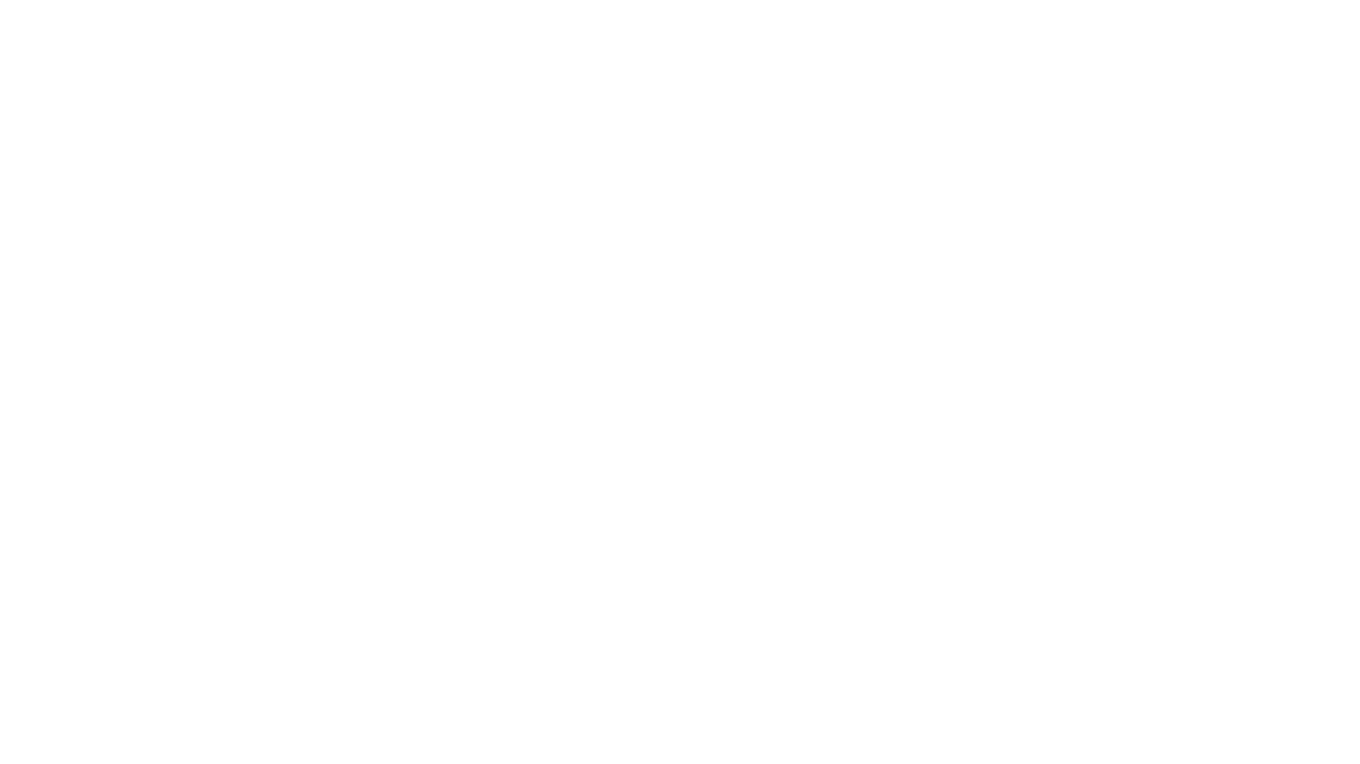
auto-news
Auto-News is an automatic news aggregator tool that utilizes Large Language Models (LLM) to pull information from various sources such as Tweets, RSS feeds, YouTube videos, web articles, Reddit, and journal notes. The tool aims to help users efficiently read and filter content based on personal interests, providing a unified reading experience and organizing information effectively. It features feed aggregation with summarization, transcript generation for videos and articles, noise reduction, task organization, and deep dive topic exploration. The tool supports multiple LLM backends, offers weekly top-k aggregations, and can be deployed on Linux/MacOS using docker-compose or Kubernetes.
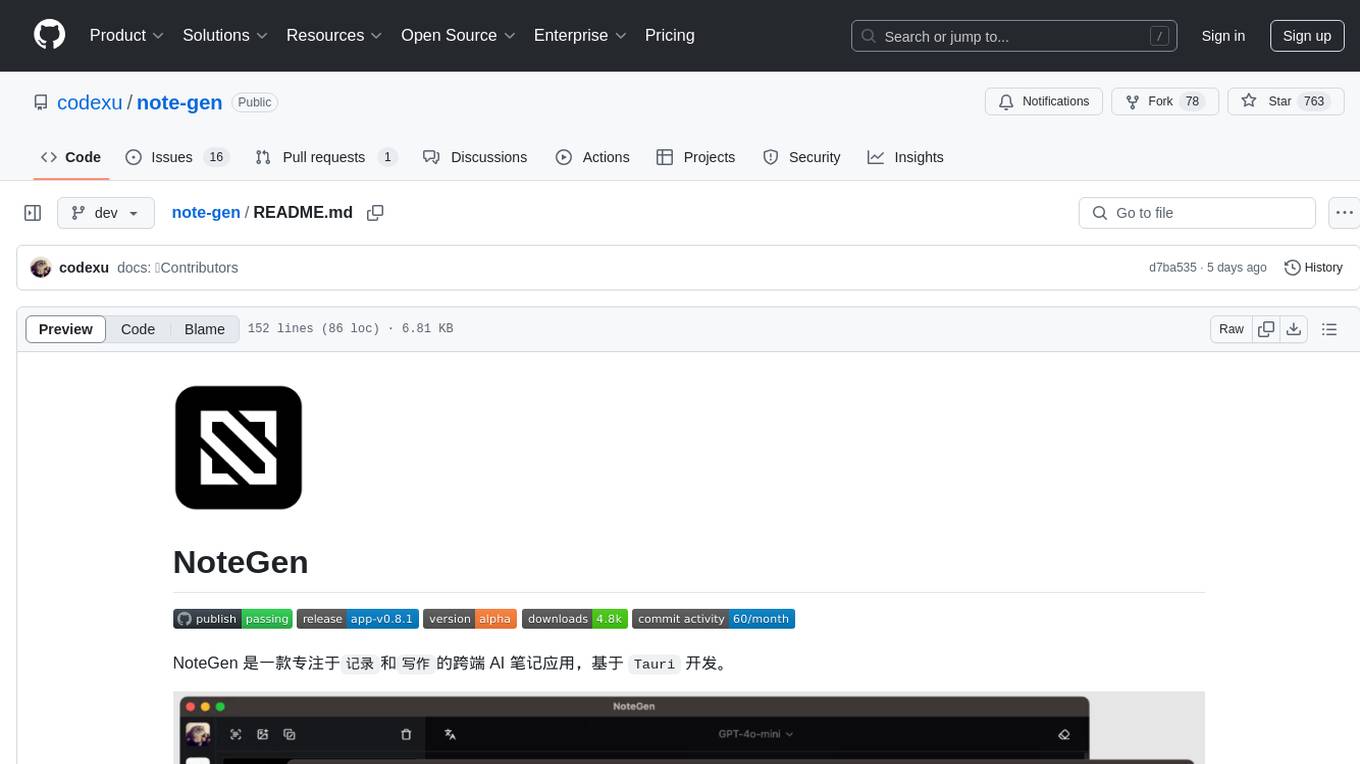
note-gen
Note-gen is a simple tool for generating notes automatically based on user input. It uses natural language processing techniques to analyze text and extract key information to create structured notes. The tool is designed to save time and effort for users who need to summarize large amounts of text or generate notes quickly. With note-gen, users can easily create organized and concise notes for study, research, or any other purpose.
For similar jobs
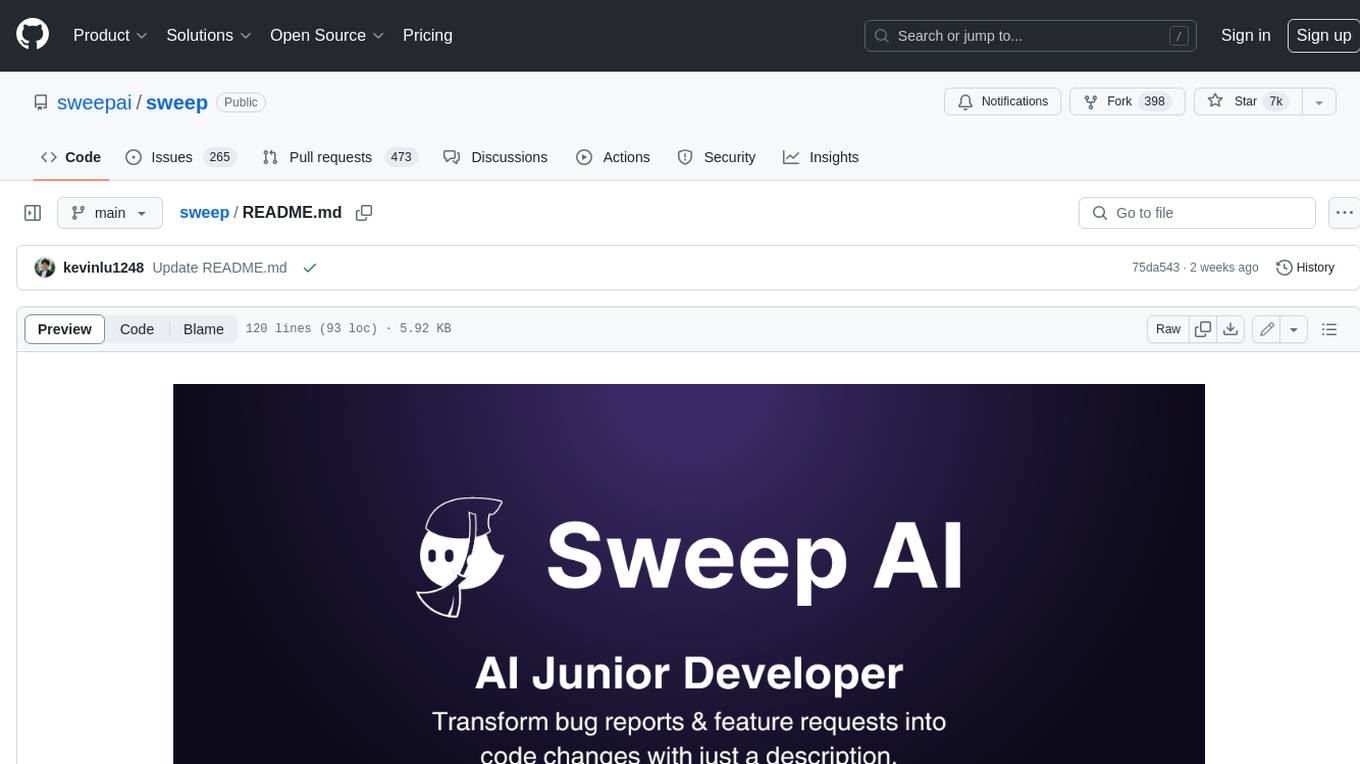
sweep
Sweep is an AI junior developer that turns bugs and feature requests into code changes. It automatically handles developer experience improvements like adding type hints and improving test coverage.
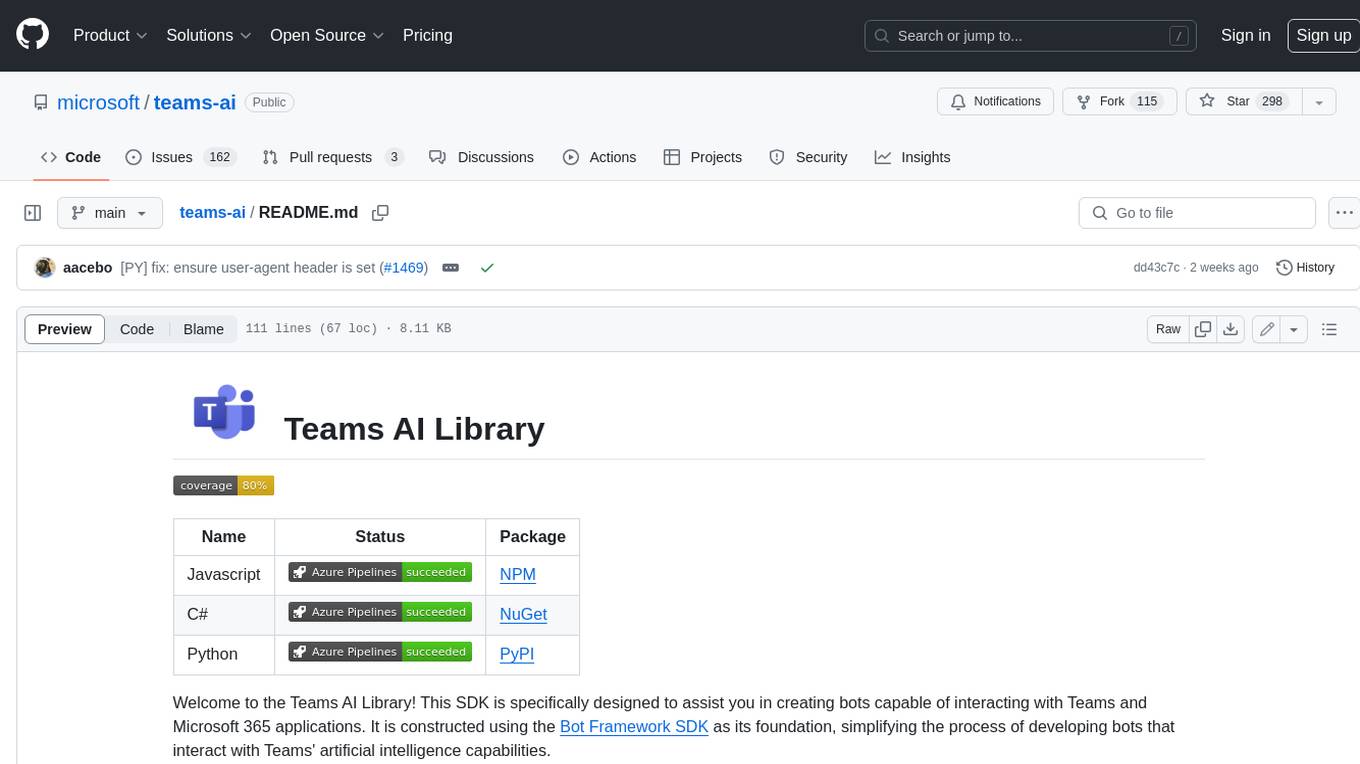
teams-ai
The Teams AI Library is a software development kit (SDK) that helps developers create bots that can interact with Teams and Microsoft 365 applications. It is built on top of the Bot Framework SDK and simplifies the process of developing bots that interact with Teams' artificial intelligence capabilities. The SDK is available for JavaScript/TypeScript, .NET, and Python.
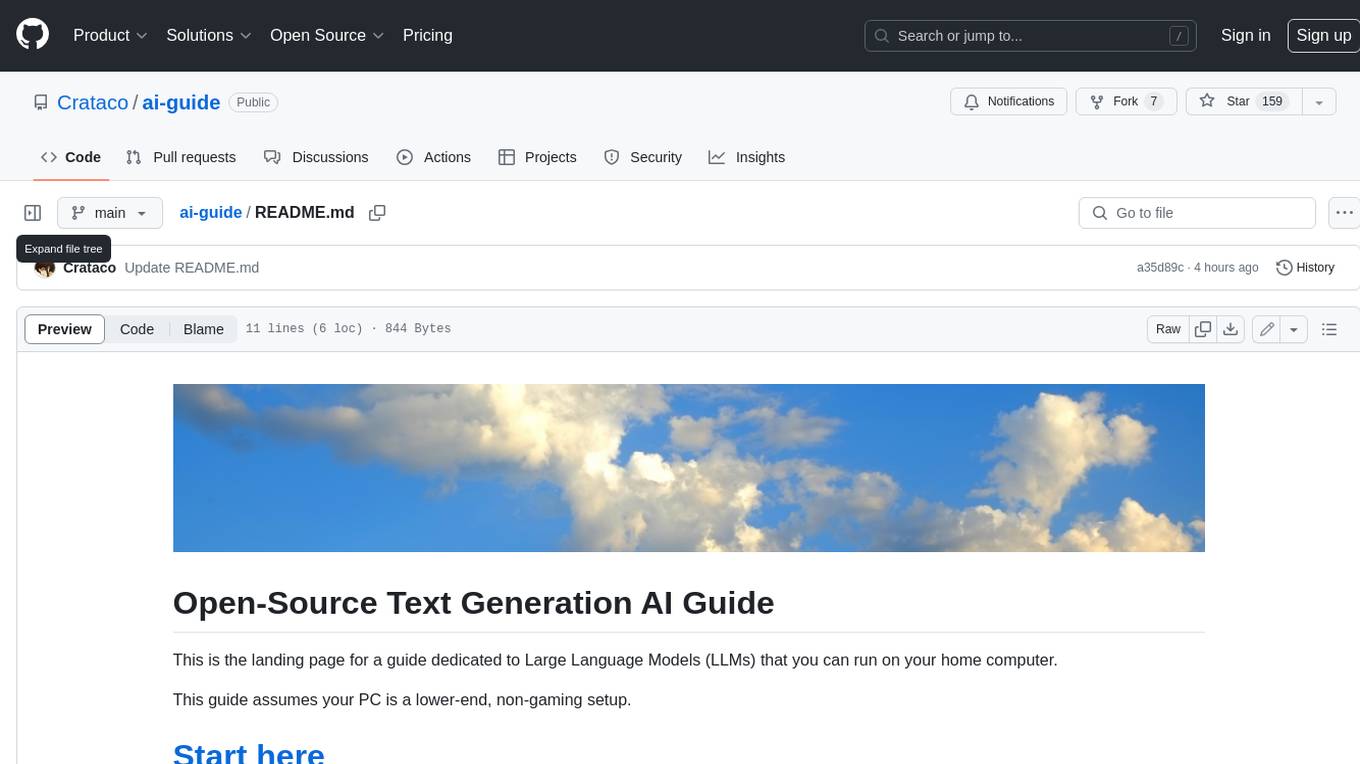
ai-guide
This guide is dedicated to Large Language Models (LLMs) that you can run on your home computer. It assumes your PC is a lower-end, non-gaming setup.
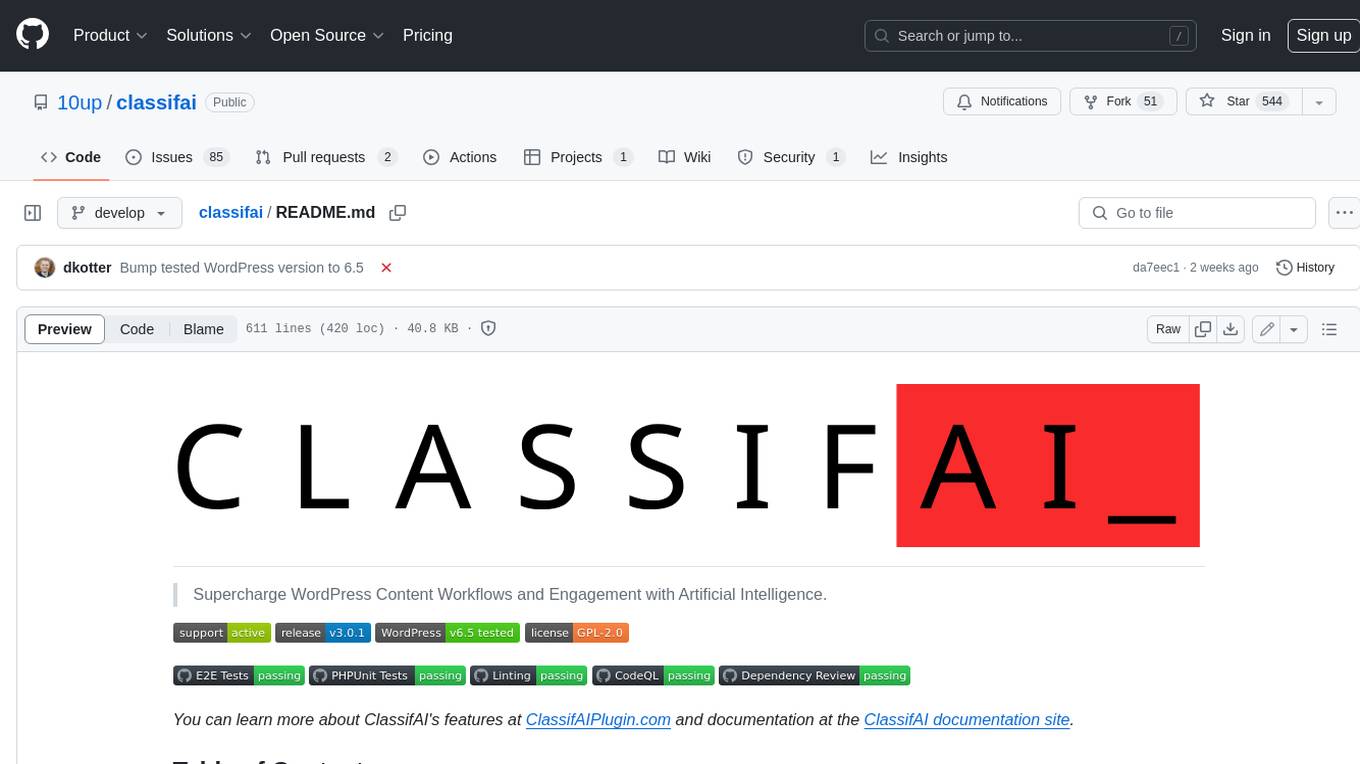
classifai
Supercharge WordPress Content Workflows and Engagement with Artificial Intelligence. Tap into leading cloud-based services like OpenAI, Microsoft Azure AI, Google Gemini and IBM Watson to augment your WordPress-powered websites. Publish content faster while improving SEO performance and increasing audience engagement. ClassifAI integrates Artificial Intelligence and Machine Learning technologies to lighten your workload and eliminate tedious tasks, giving you more time to create original content that matters.
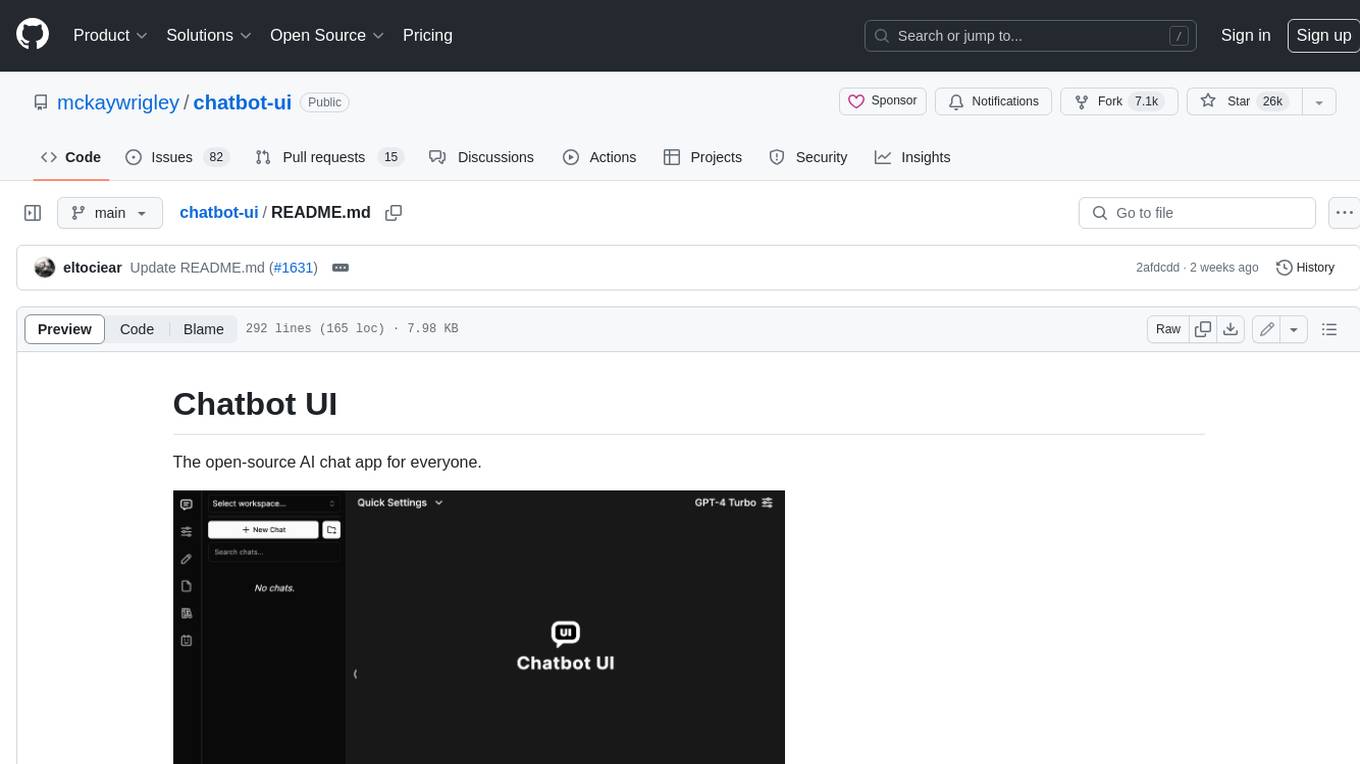
chatbot-ui
Chatbot UI is an open-source AI chat app that allows users to create and deploy their own AI chatbots. It is easy to use and can be customized to fit any need. Chatbot UI is perfect for businesses, developers, and anyone who wants to create a chatbot.
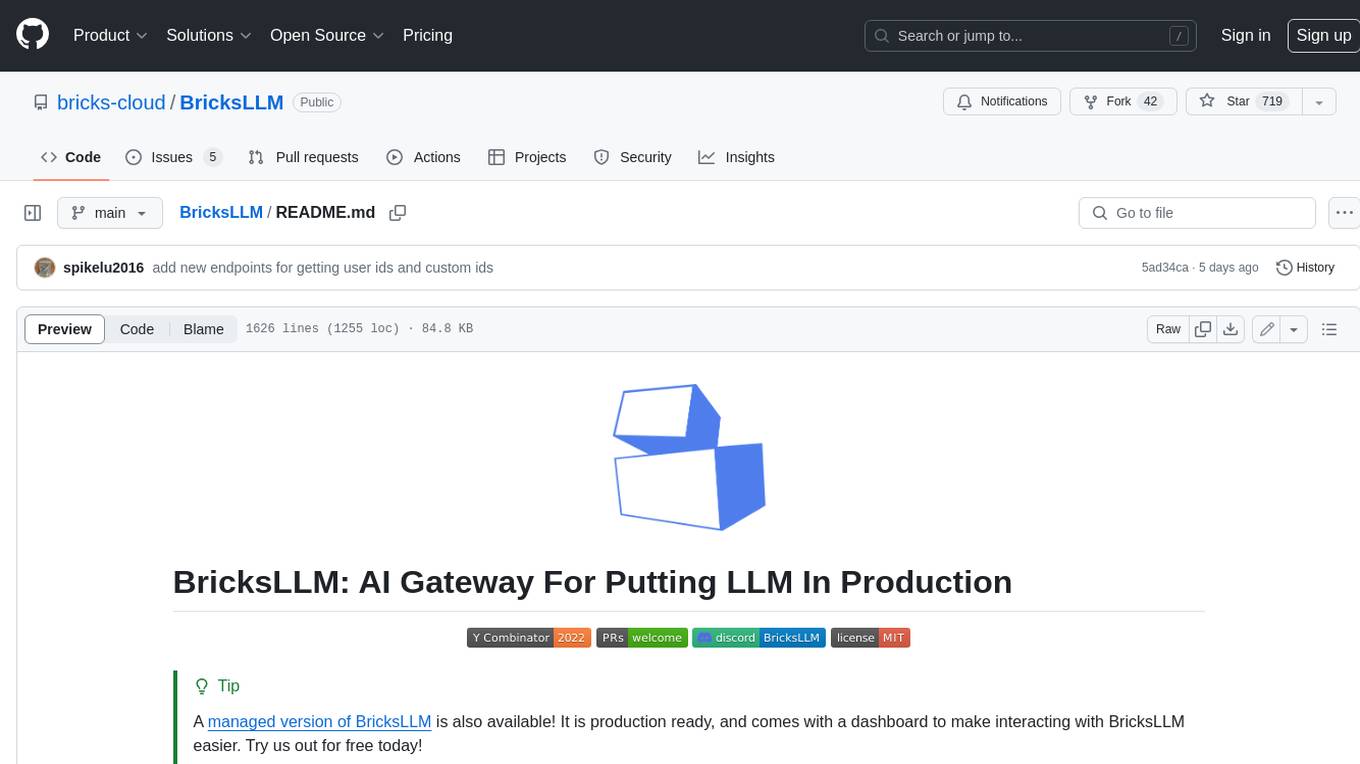
BricksLLM
BricksLLM is a cloud native AI gateway written in Go. Currently, it provides native support for OpenAI, Anthropic, Azure OpenAI and vLLM. BricksLLM aims to provide enterprise level infrastructure that can power any LLM production use cases. Here are some use cases for BricksLLM: * Set LLM usage limits for users on different pricing tiers * Track LLM usage on a per user and per organization basis * Block or redact requests containing PIIs * Improve LLM reliability with failovers, retries and caching * Distribute API keys with rate limits and cost limits for internal development/production use cases * Distribute API keys with rate limits and cost limits for students
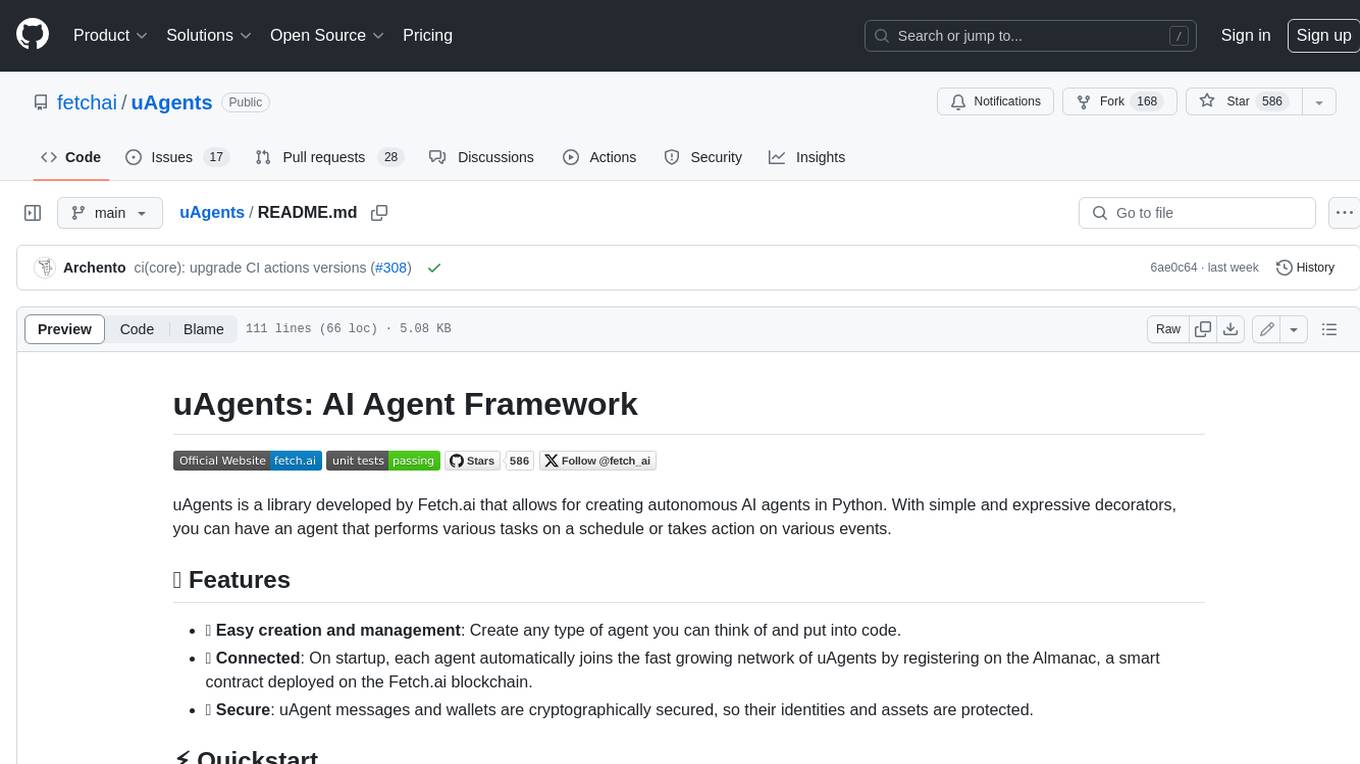
uAgents
uAgents is a Python library developed by Fetch.ai that allows for the creation of autonomous AI agents. These agents can perform various tasks on a schedule or take action on various events. uAgents are easy to create and manage, and they are connected to a fast-growing network of other uAgents. They are also secure, with cryptographically secured messages and wallets.
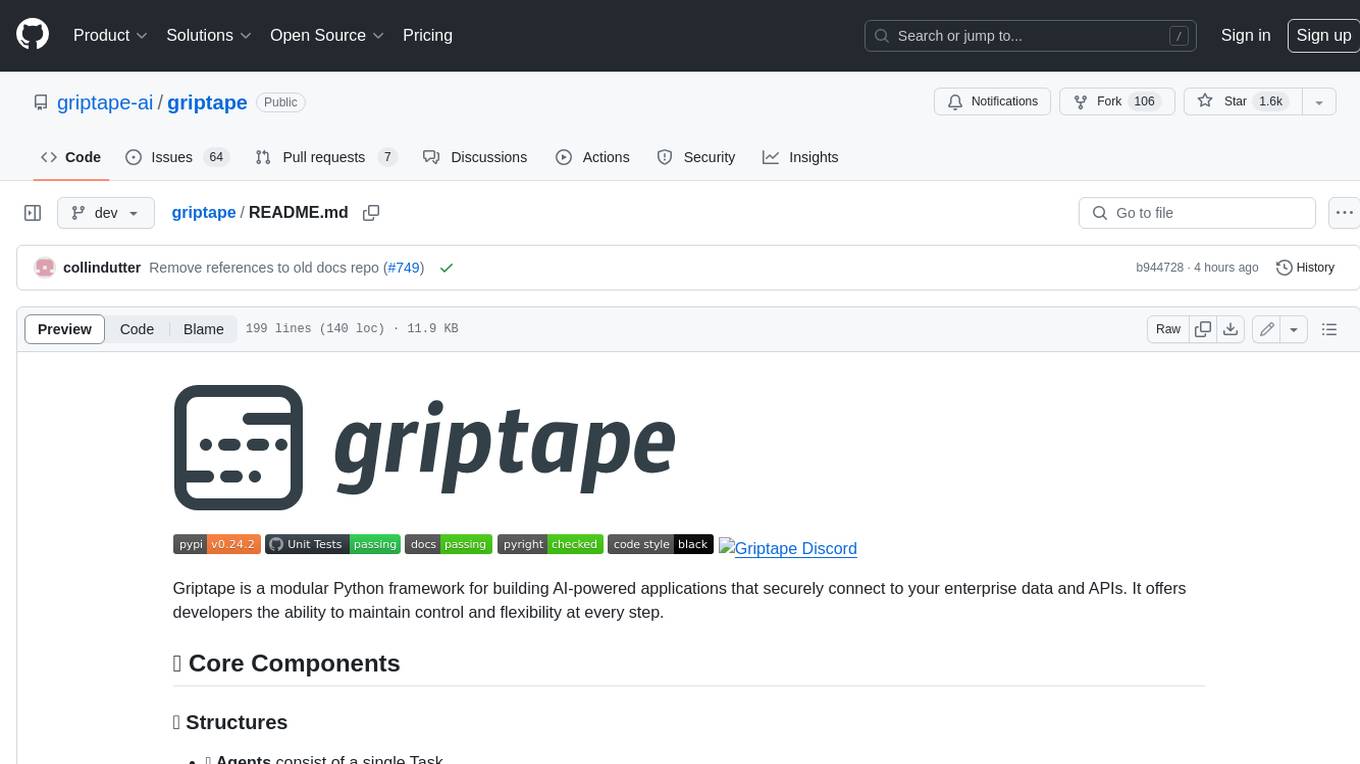
griptape
Griptape is a modular Python framework for building AI-powered applications that securely connect to your enterprise data and APIs. It offers developers the ability to maintain control and flexibility at every step. Griptape's core components include Structures (Agents, Pipelines, and Workflows), Tasks, Tools, Memory (Conversation Memory, Task Memory, and Meta Memory), Drivers (Prompt and Embedding Drivers, Vector Store Drivers, Image Generation Drivers, Image Query Drivers, SQL Drivers, Web Scraper Drivers, and Conversation Memory Drivers), Engines (Query Engines, Extraction Engines, Summary Engines, Image Generation Engines, and Image Query Engines), and additional components (Rulesets, Loaders, Artifacts, Chunkers, and Tokenizers). Griptape enables developers to create AI-powered applications with ease and efficiency.