Best AI tools for< Deploy Ml Systems >
20 - AI tool Sites
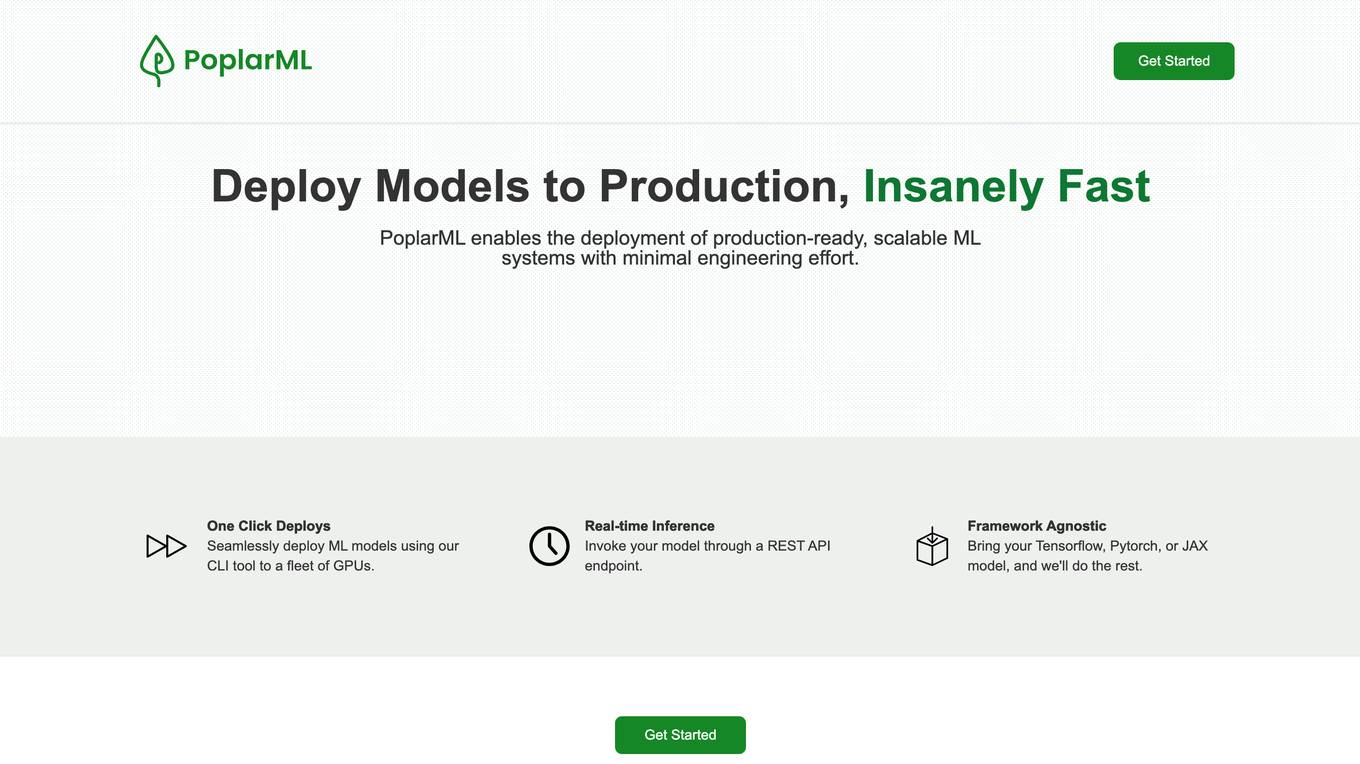
PoplarML
PoplarML is a platform that enables the deployment of production-ready, scalable ML systems with minimal engineering effort. It offers one-click deploys, real-time inference, and framework agnostic support. With PoplarML, users can seamlessly deploy ML models using a CLI tool to a fleet of GPUs and invoke their models through a REST API endpoint. The platform supports Tensorflow, Pytorch, and JAX models.
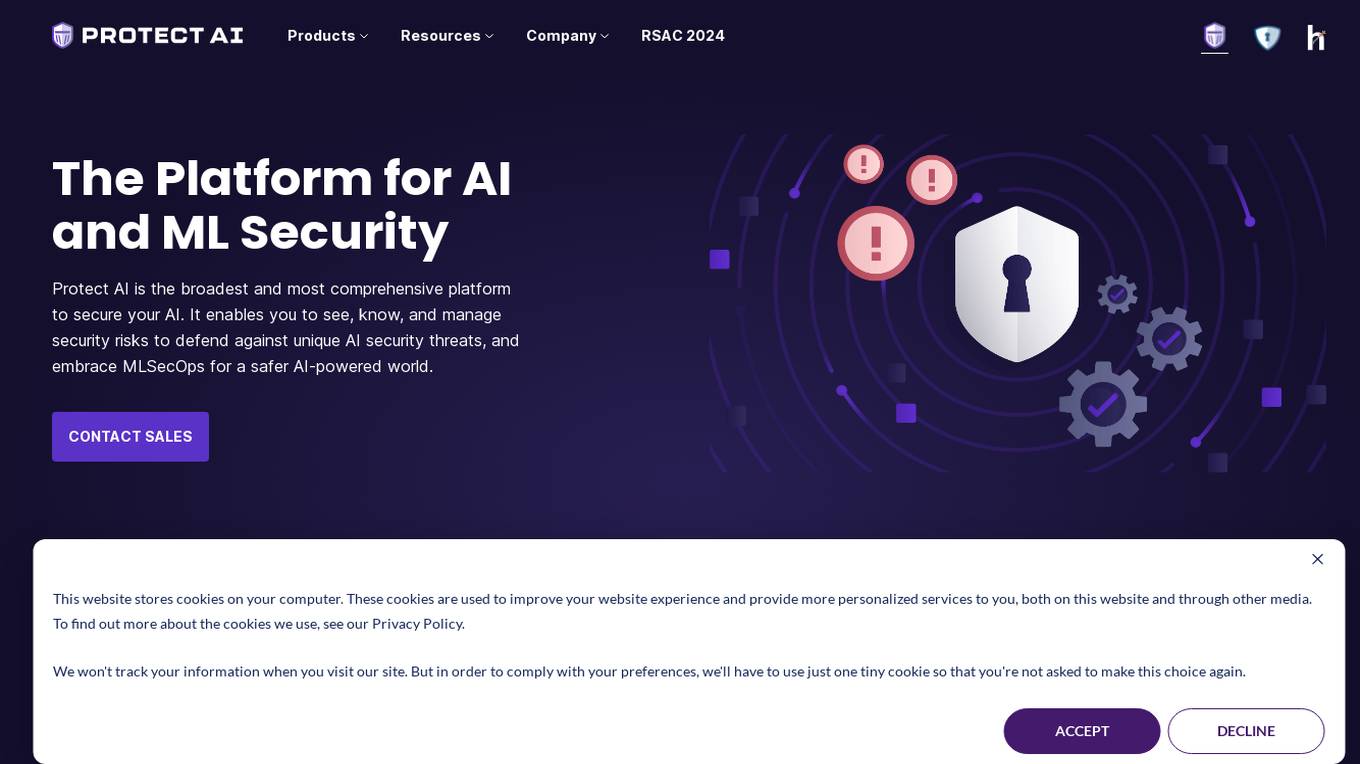
Protect AI
Protect AI is a comprehensive platform designed to secure AI systems by providing visibility and manageability to detect and mitigate unique AI security threats. The platform empowers organizations to embrace a security-first approach to AI, offering solutions for AI Security Posture Management, ML model security enforcement, AI/ML supply chain vulnerability database, LLM security monitoring, and observability. Protect AI aims to safeguard AI applications and ML systems from potential vulnerabilities, enabling users to build, adopt, and deploy AI models confidently and at scale.
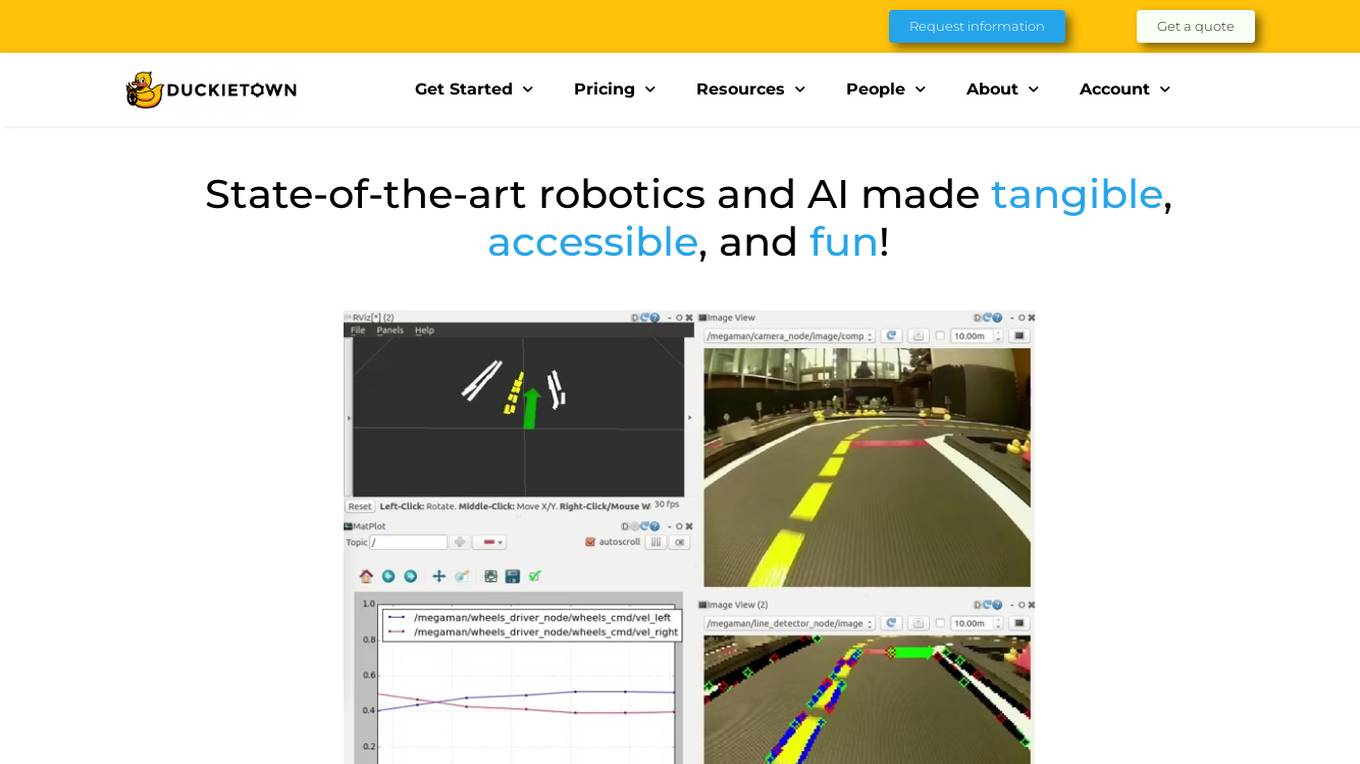
Duckietown
Duckietown is a platform for delivering cutting-edge robotics and AI learning experiences. It offers teaching resources to instructors, hands-on activities to learners, an accessible research platform to researchers, and a state-of-the-art ecosystem for professional training. Duckietown's mission is to make robotics and AI education state-of-the-art, hands-on, and accessible to all.
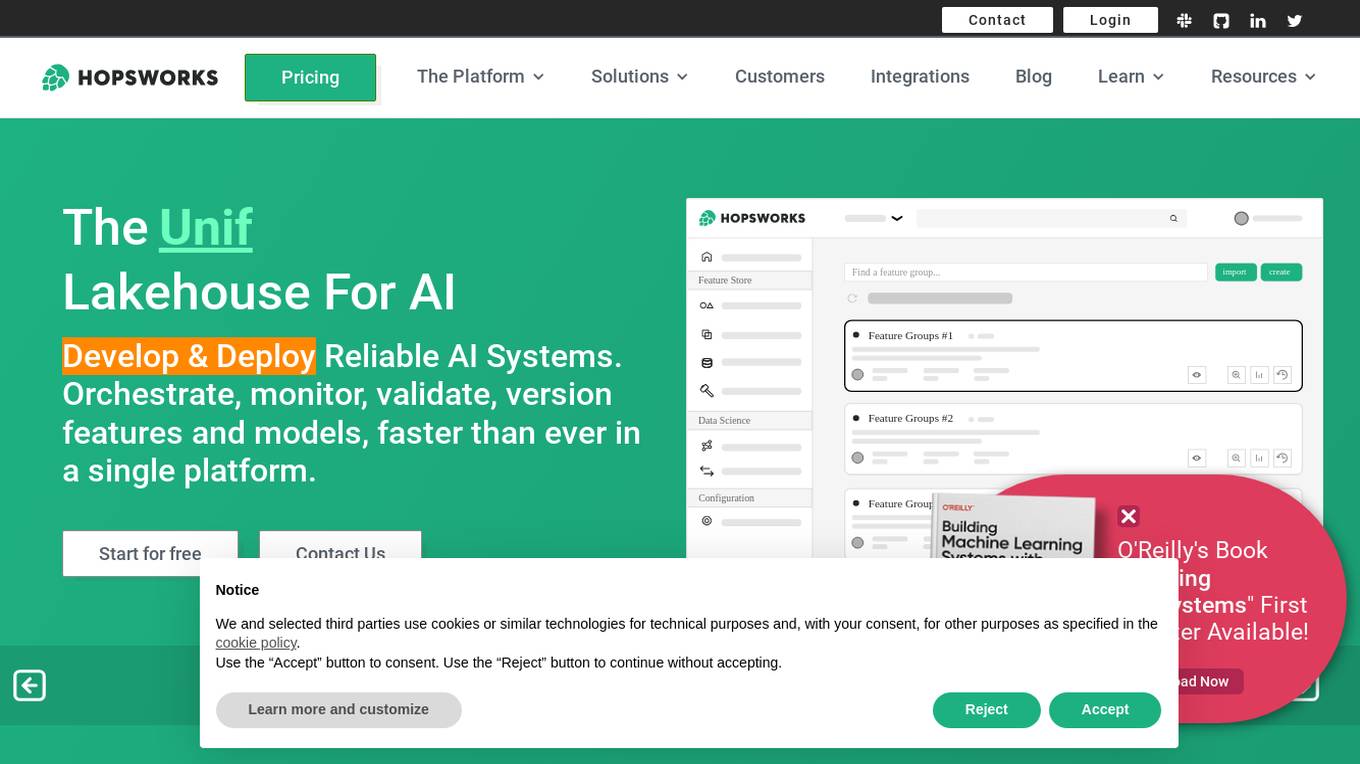
Hopsworks
Hopsworks is an AI platform that offers a comprehensive solution for building, deploying, and monitoring machine learning systems. It provides features such as a Feature Store, real-time ML capabilities, and generative AI solutions. Hopsworks enables users to develop and deploy reliable AI systems, orchestrate and monitor models, and personalize machine learning models with private data. The platform supports batch and real-time ML tasks, with the flexibility to deploy on-premises or in the cloud.
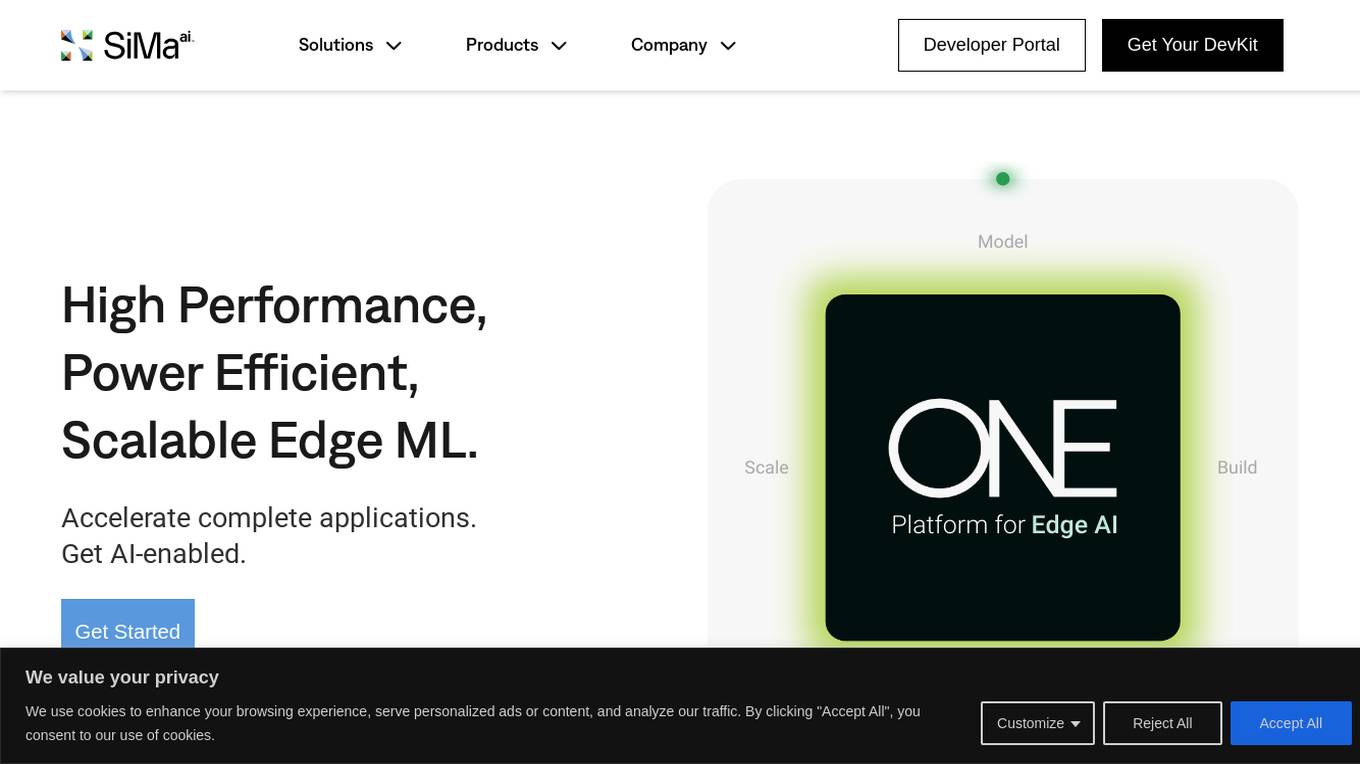
SiMa.ai
SiMa.ai is an AI application that offers high-performance, power-efficient, and scalable edge machine learning solutions for various industries such as automotive, industrial, healthcare, drones, and government sectors. The platform provides MLSoC™ boards, DevKit 2.0, Palette Software 1.2, and Edgematic™ for developers to accelerate complete applications and deploy AI-enabled solutions. SiMa.ai's Machine Learning System on Chip (MLSoC) enables full-pipeline implementations of real-world ML solutions, making it a trusted platform for edge AI development.
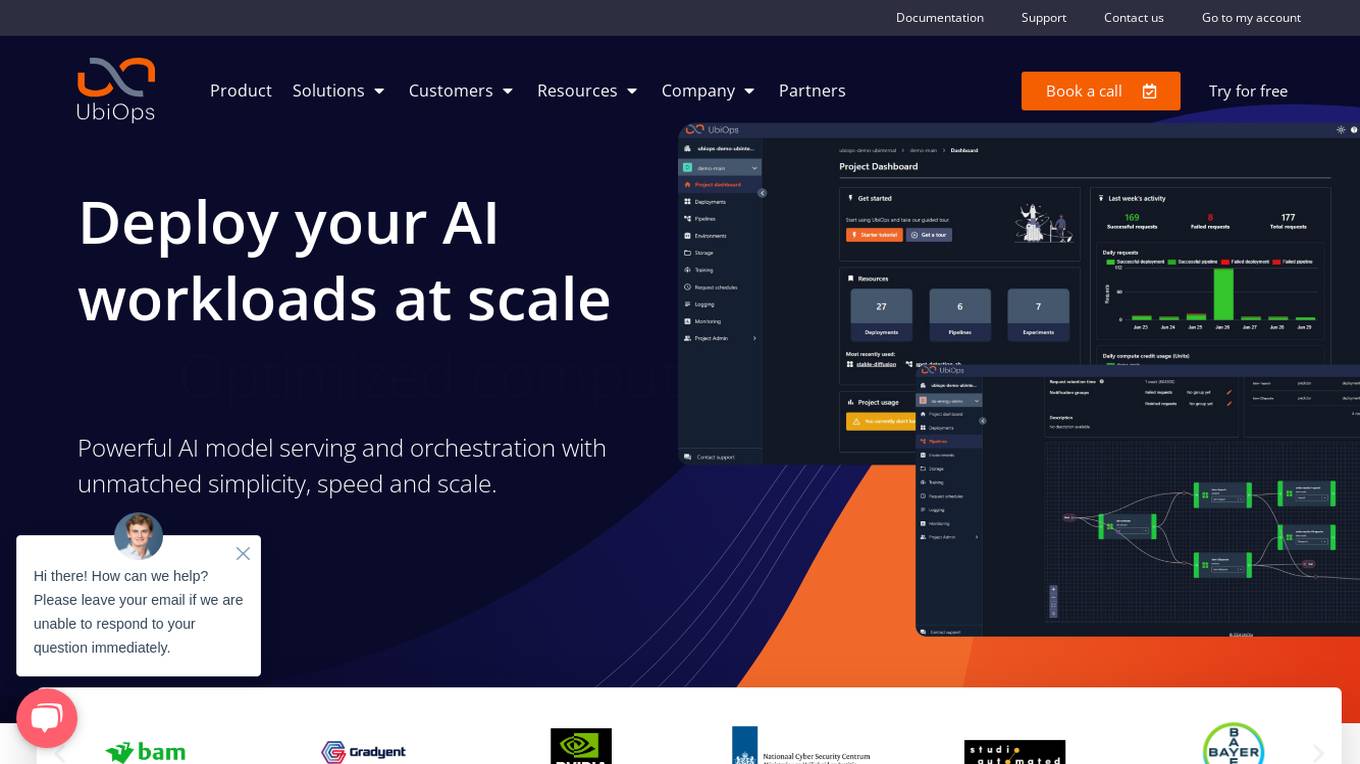
UbiOps
UbiOps is an AI infrastructure platform that helps teams quickly run their AI & ML workloads as reliable and secure microservices. It offers powerful AI model serving and orchestration with unmatched simplicity, speed, and scale. UbiOps allows users to deploy models and functions in minutes, manage AI workloads from a single control plane, integrate easily with tools like PyTorch and TensorFlow, and ensure security and compliance by design. The platform supports hybrid and multi-cloud workload orchestration, rapid adaptive scaling, and modular applications with unique workflow management system.
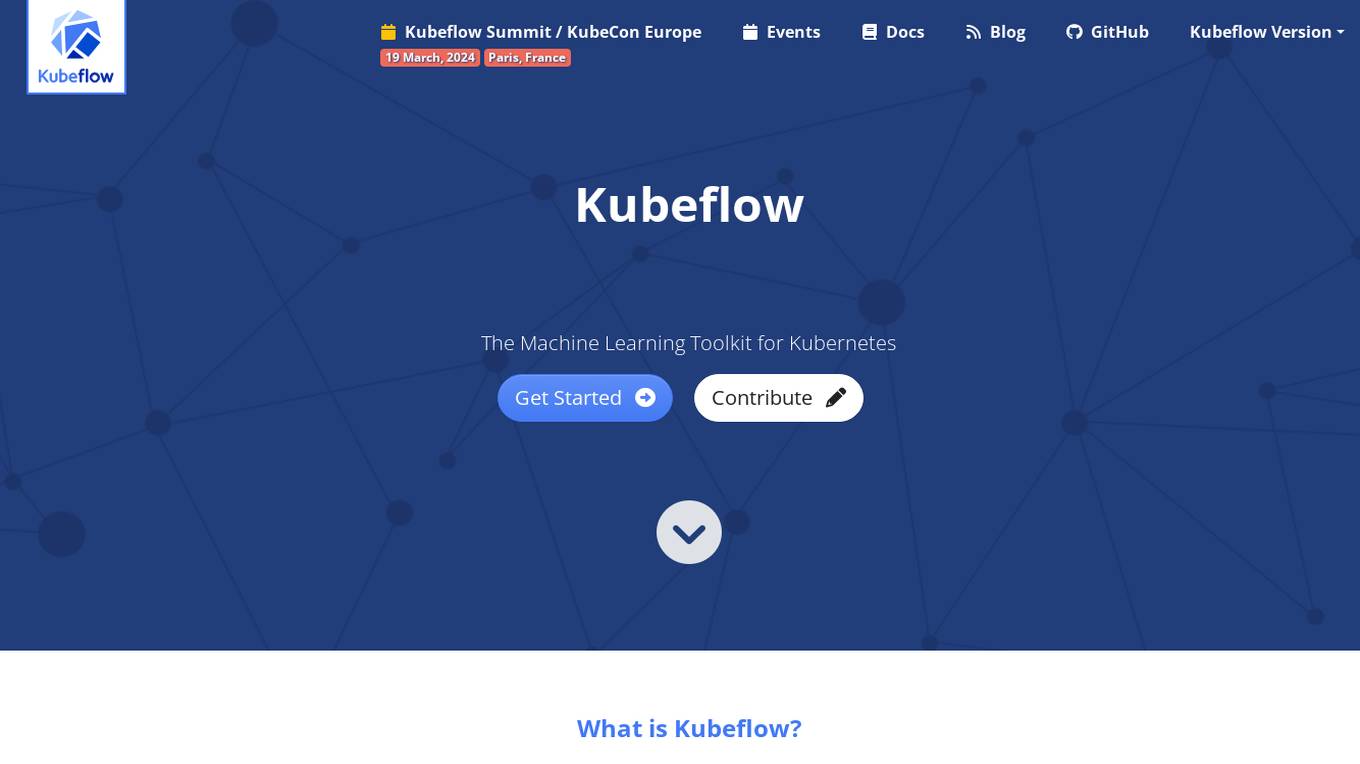
Kubeflow
Kubeflow is an open-source machine learning (ML) toolkit that makes deploying ML workflows on Kubernetes simple, portable, and scalable. It provides a unified interface for model training, serving, and hyperparameter tuning, and supports a variety of popular ML frameworks including PyTorch, TensorFlow, and XGBoost. Kubeflow is designed to be used with Kubernetes, a container orchestration system that automates the deployment, management, and scaling of containerized applications.
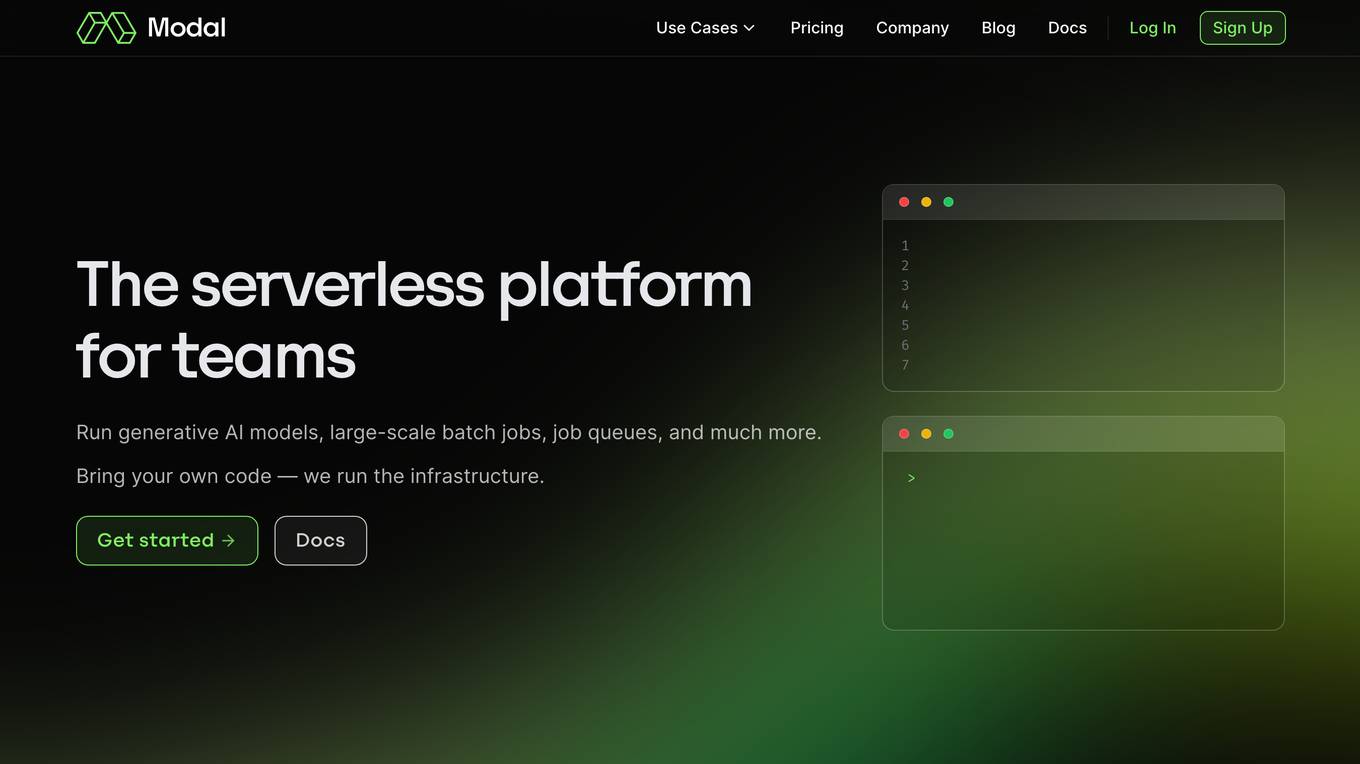
Modal
Modal is a high-performance cloud platform designed for developers, AI data, and ML teams. It offers a serverless environment for running generative AI models, large-scale batch jobs, job queues, and more. With Modal, users can bring their own code and leverage the platform's optimized container file system for fast cold boots and seamless autoscaling. The platform is engineered for large-scale workloads, allowing users to scale to hundreds of GPUs, pay only for what they use, and deploy functions to the cloud in seconds without the need for YAML or Dockerfiles. Modal also provides features for job scheduling, web endpoints, observability, and security compliance.
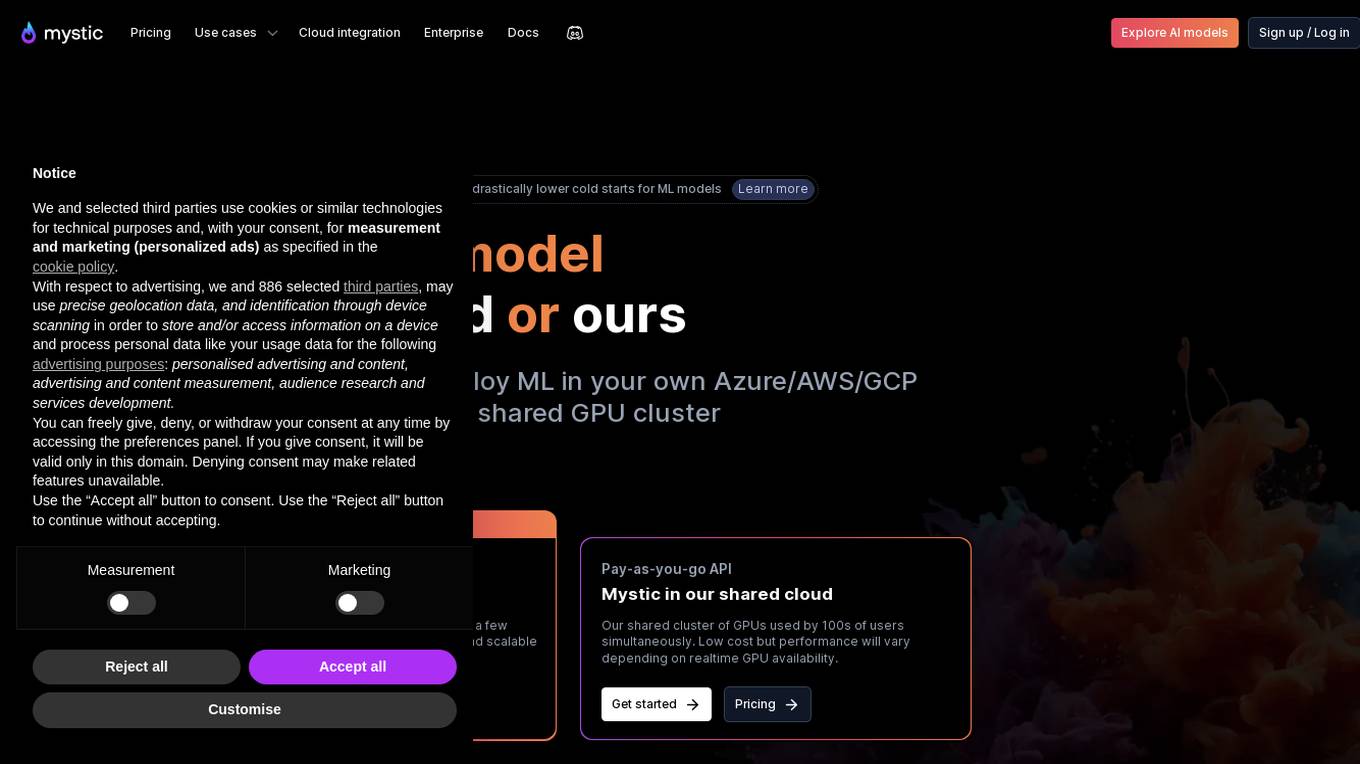
Mystic.ai
Mystic.ai is an AI tool designed to deploy and scale Machine Learning models with ease. It offers a fully managed Kubernetes platform that runs in your own cloud, allowing users to deploy ML models in their own Azure/AWS/GCP account or in a shared GPU cluster. Mystic.ai provides cost optimizations, fast inference, simpler developer experience, and performance optimizations to ensure high-performance AI model serving. With features like pay-as-you-go API, cloud integration with AWS/Azure/GCP, and a beautiful dashboard, Mystic.ai simplifies the deployment and management of ML models for data scientists and AI engineers.
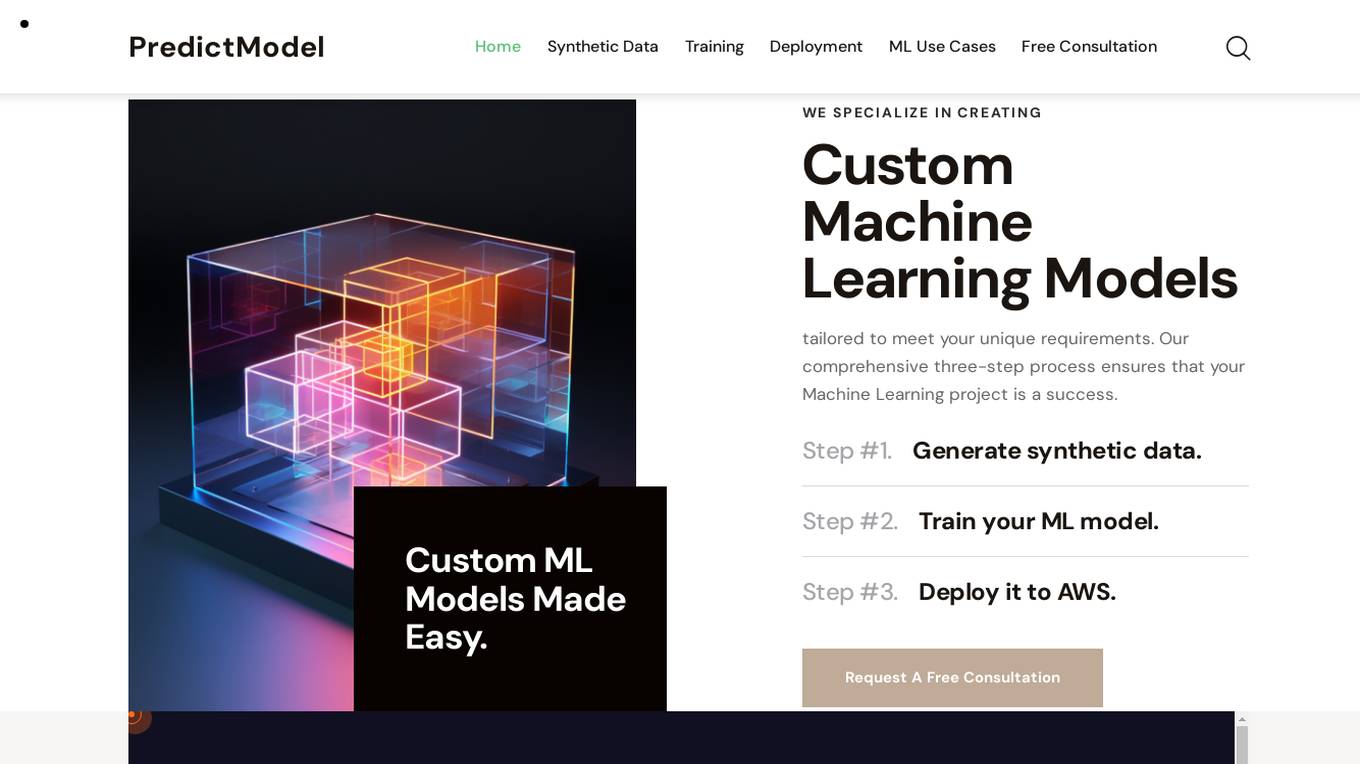
PredictModel
PredictModel is an AI tool that specializes in creating custom Machine Learning models tailored to meet unique requirements. The platform offers a comprehensive three-step process, including generating synthetic data, training ML models, and deploying them to AWS. PredictModel helps businesses streamline processes, improve customer segmentation, enhance client interaction, and boost overall business performance. The tool maximizes accuracy through customized synthetic data generation and saves time and money by providing expert ML engineers. With a focus on automated lead prioritization, fraud detection, cost optimization, and planning, PredictModel aims to stay ahead of the curve in the ML industry.
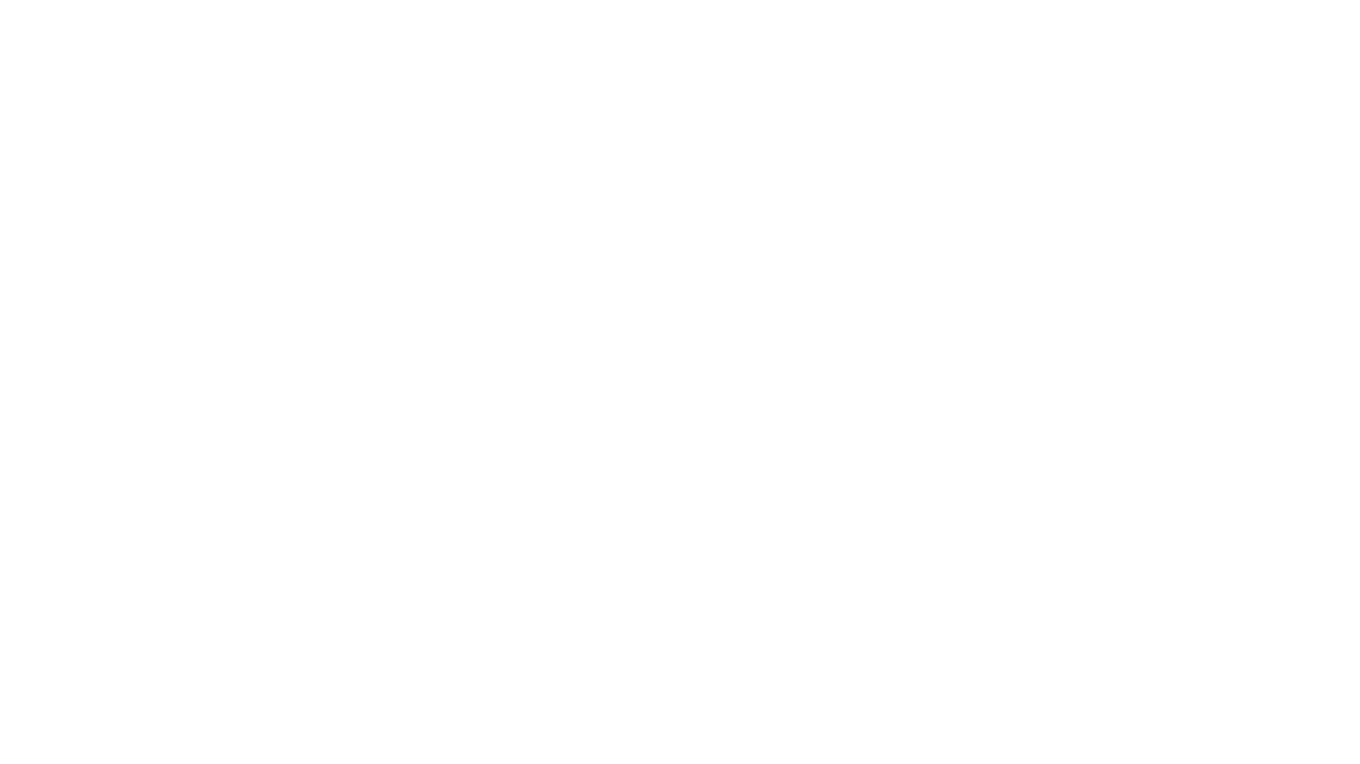
TensorFlow
TensorFlow is an end-to-end platform for machine learning. It provides a wide range of tools and resources to help developers build, train, and deploy ML models. TensorFlow is used by researchers and developers all over the world to solve real-world problems in a variety of domains, including computer vision, natural language processing, and robotics.
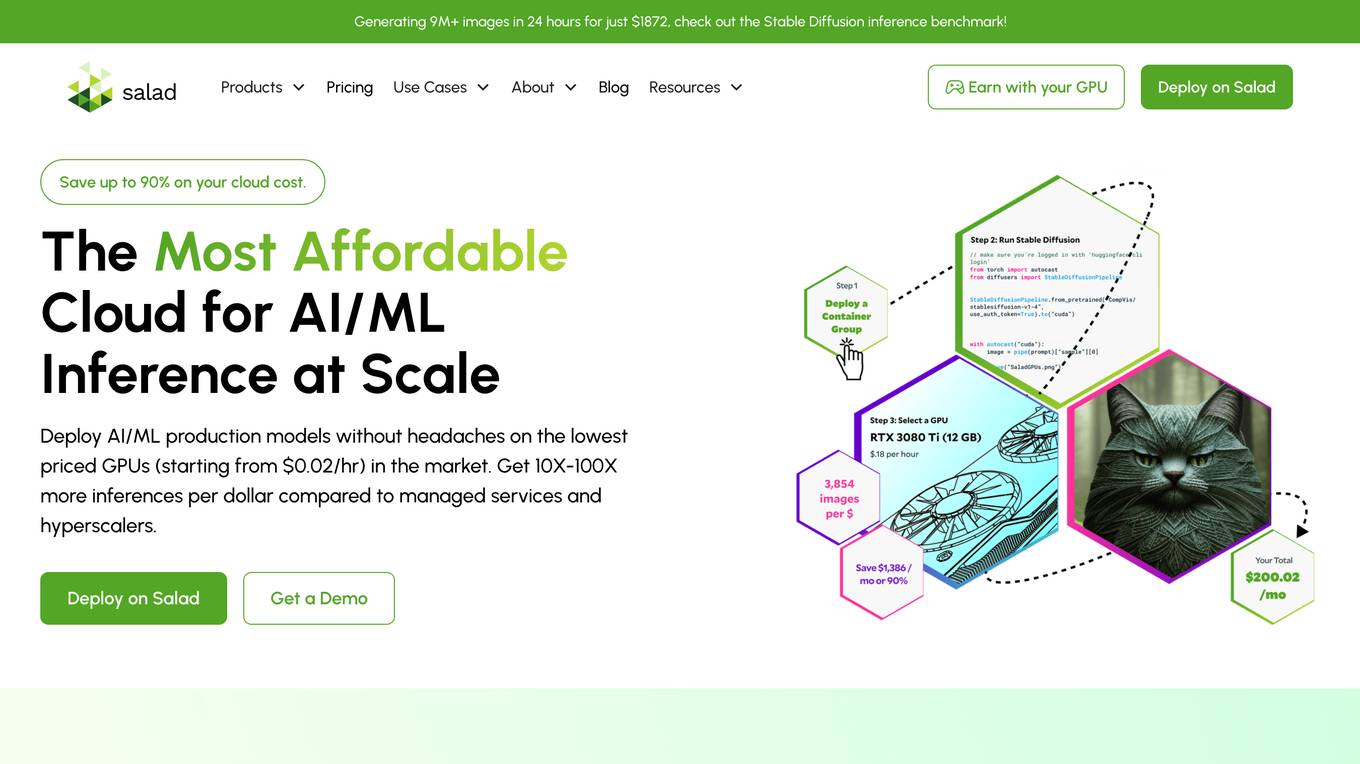
Salad
Salad is a distributed GPU cloud platform that offers fully managed and massively scalable services for AI applications. It provides the lowest priced AI transcription in the market, with features like image generation, voice AI, computer vision, data collection, and batch processing. Salad democratizes cloud computing by leveraging consumer GPUs to deliver cost-effective AI/ML inference at scale. The platform is trusted by hundreds of machine learning and data science teams for its affordability, scalability, and ease of deployment.
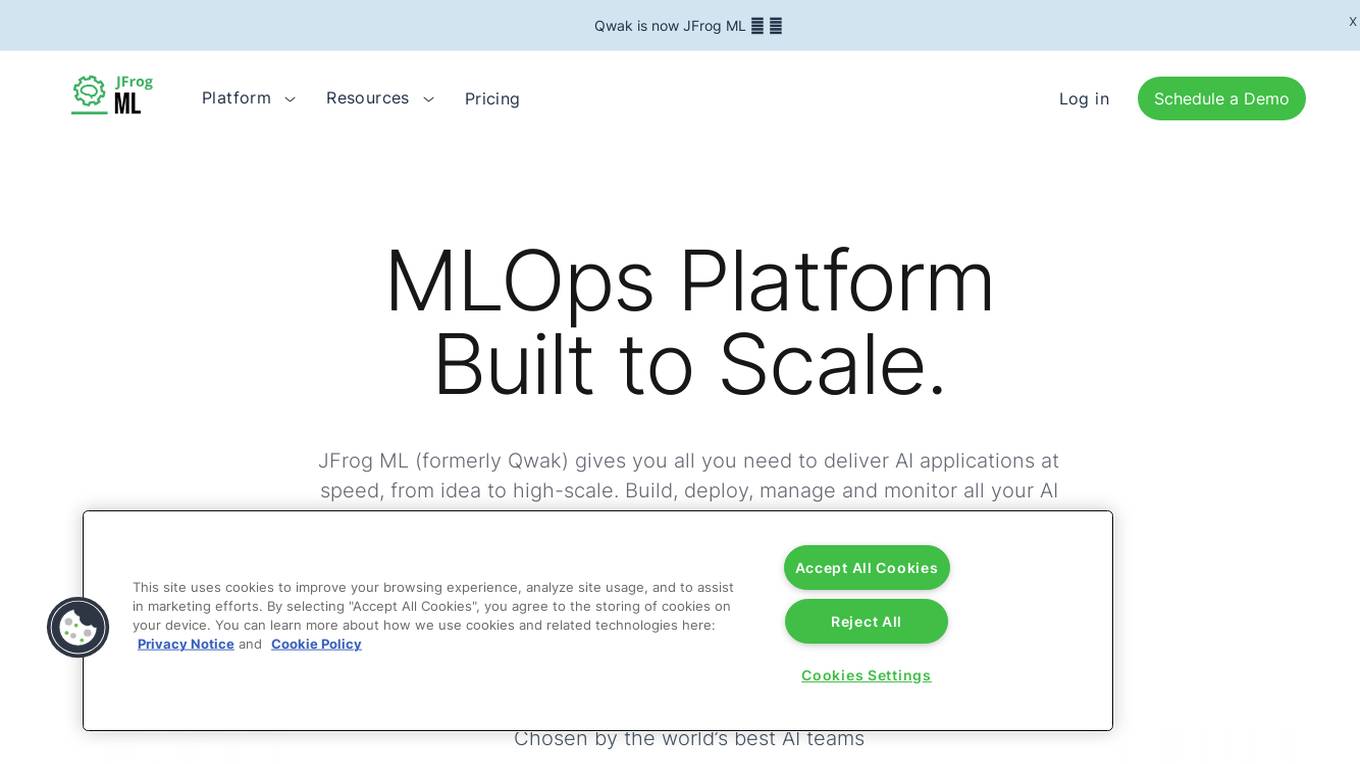
JFrog ML
JFrog ML is an AI platform designed to streamline AI development from prototype to production. It offers a unified MLOps platform to build, train, deploy, and manage AI workflows at scale. With features like Feature Store, LLMOps, and model monitoring, JFrog ML empowers AI teams to collaborate efficiently and optimize AI & ML models in production.
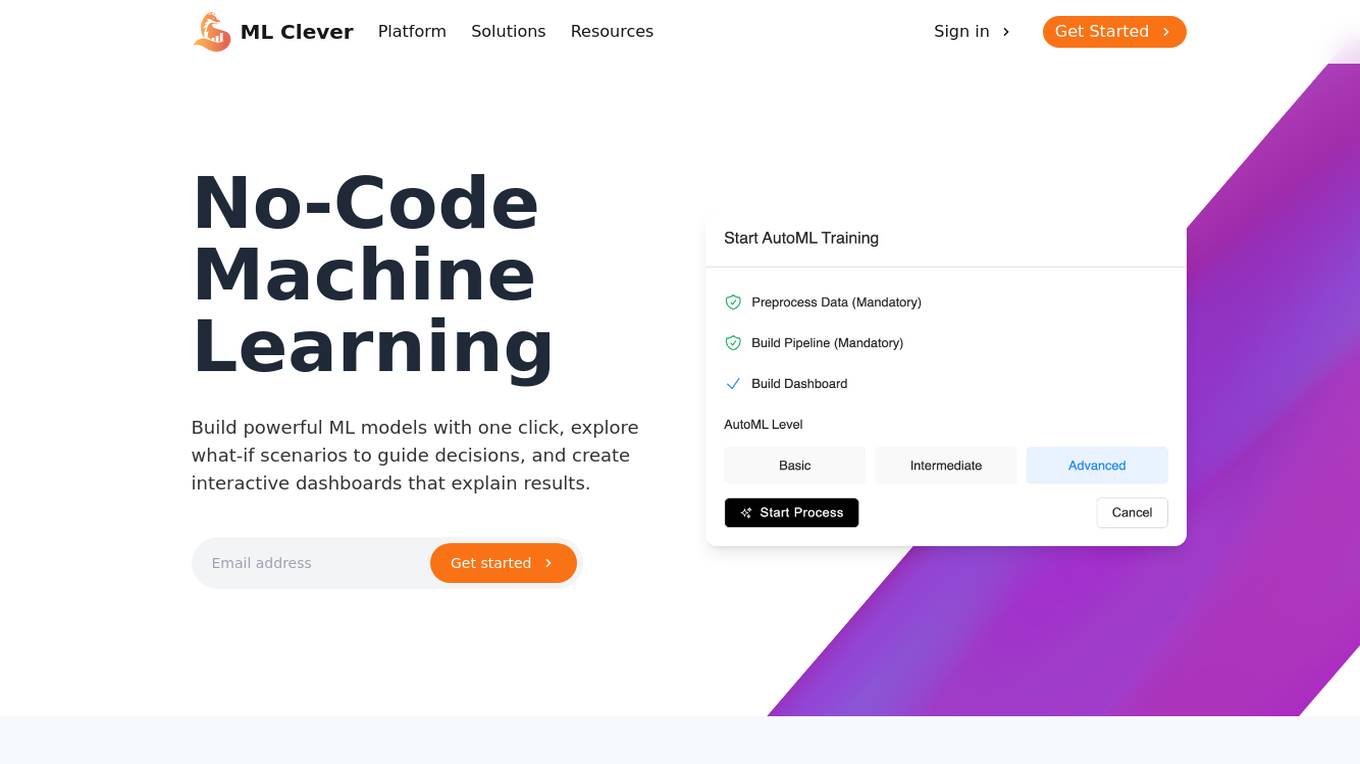
ML Clever
ML Clever is a no-code machine learning platform that empowers users to build powerful ML models with one click, explore what-if scenarios to guide decisions, and create interactive dashboards to explain results. It combines automated machine learning, interactive dashboards, and flexible prediction tools in one platform, allowing users to transform data into business insights without the need for data scientists or coding skills.
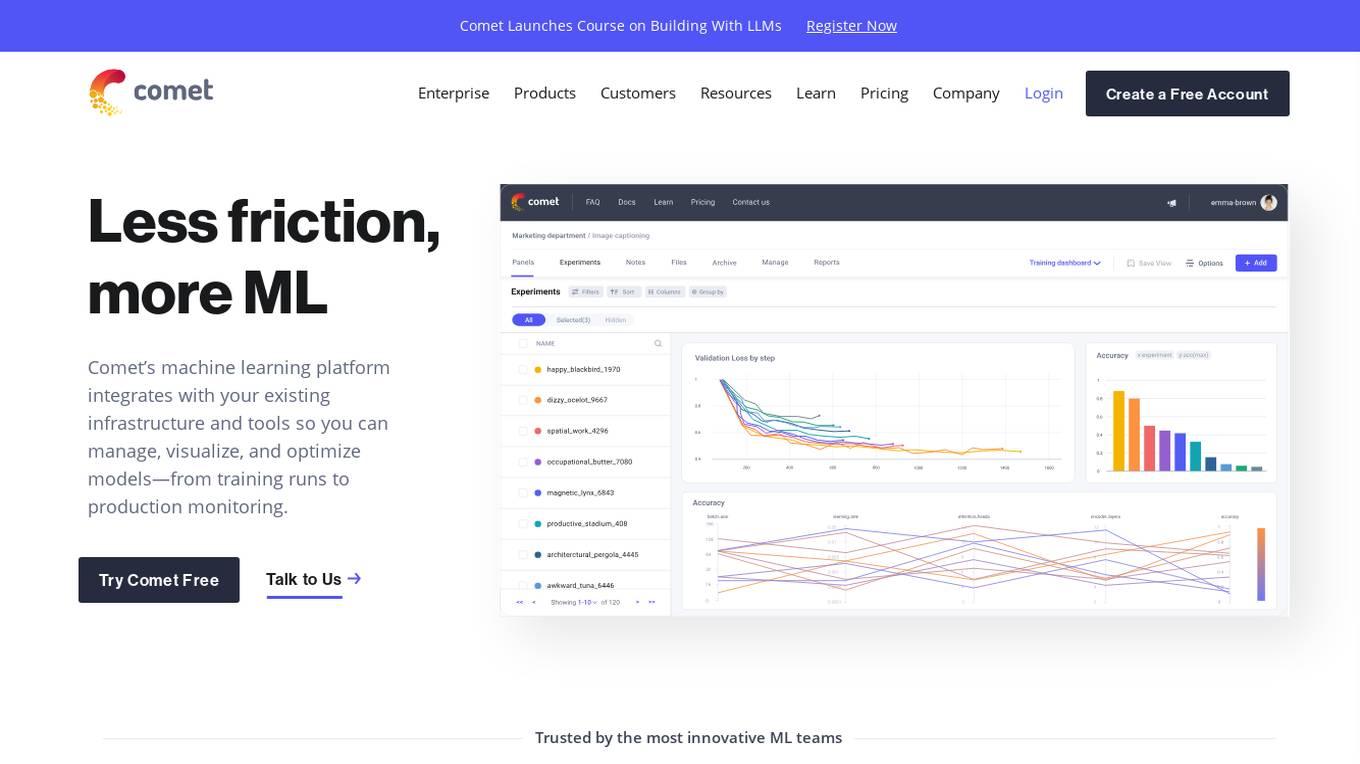
Comet ML
Comet ML is a machine learning platform that integrates with your existing infrastructure and tools so you can manage, visualize, and optimize models—from training runs to production monitoring.
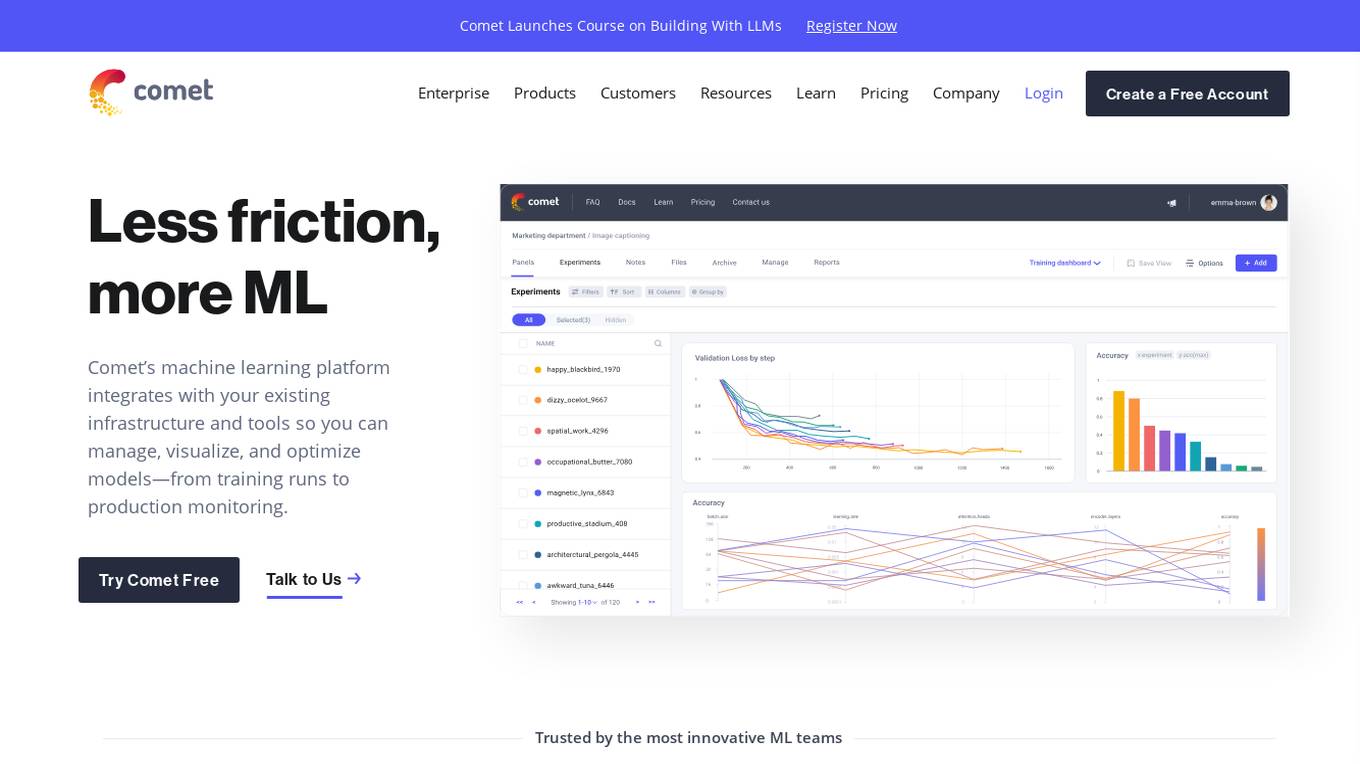
Comet ML
Comet ML is a machine learning platform that integrates with your existing infrastructure and tools so you can manage, visualize, and optimize models—from training runs to production monitoring.
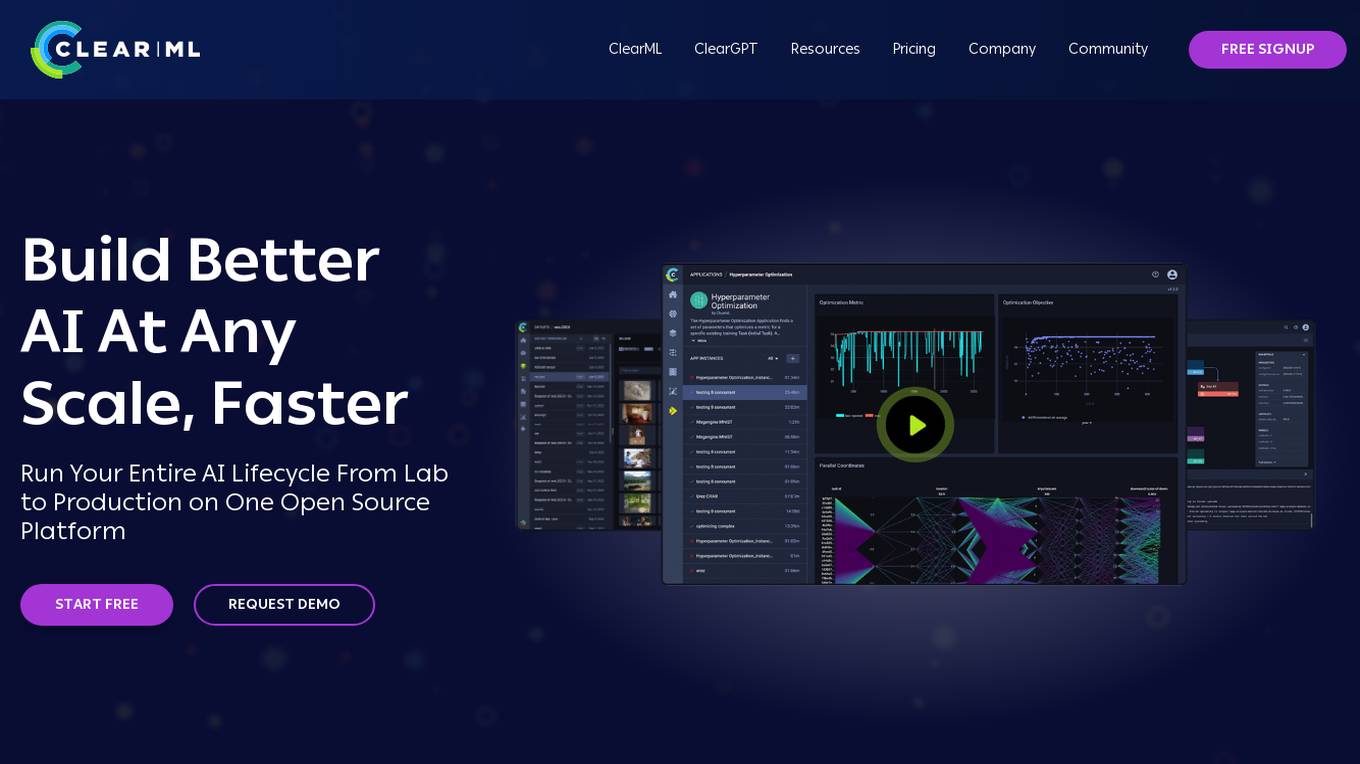
ClearML
ClearML is an open-source, end-to-end platform for continuous machine learning (ML). It provides a unified platform for data management, experiment tracking, model training, deployment, and monitoring. ClearML is designed to make it easy for teams to collaborate on ML projects and to ensure that models are deployed and maintained in a reliable and scalable way.
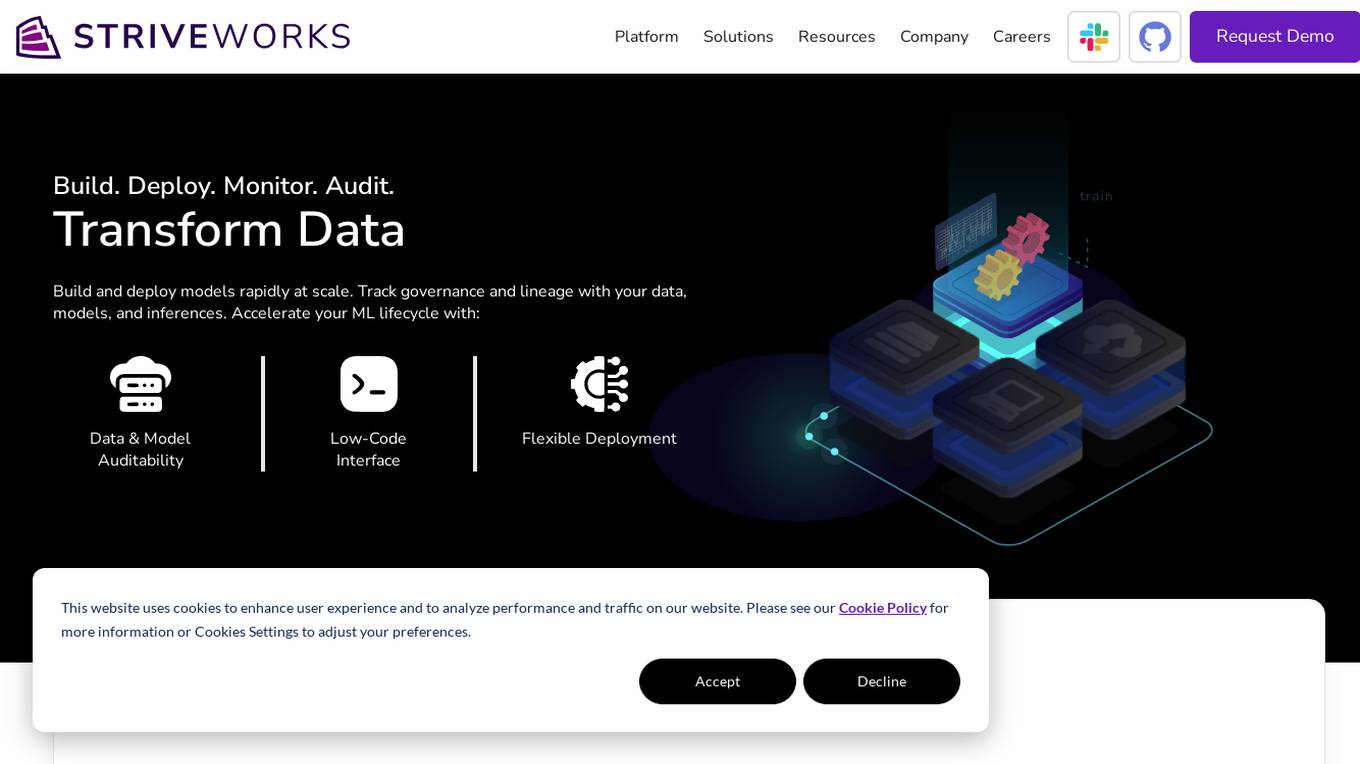
Striveworks
Striveworks is an AI application that offers a Machine Learning Operations Platform designed to help organizations build, deploy, maintain, monitor, and audit machine learning models efficiently. It provides features such as rapid model deployment, data and model auditability, low-code interface, flexible deployment options, and operationalizing AI data science with real returns. Striveworks aims to accelerate the ML lifecycle, save time and money in model creation, and enable non-experts to leverage AI for data-driven decisions.
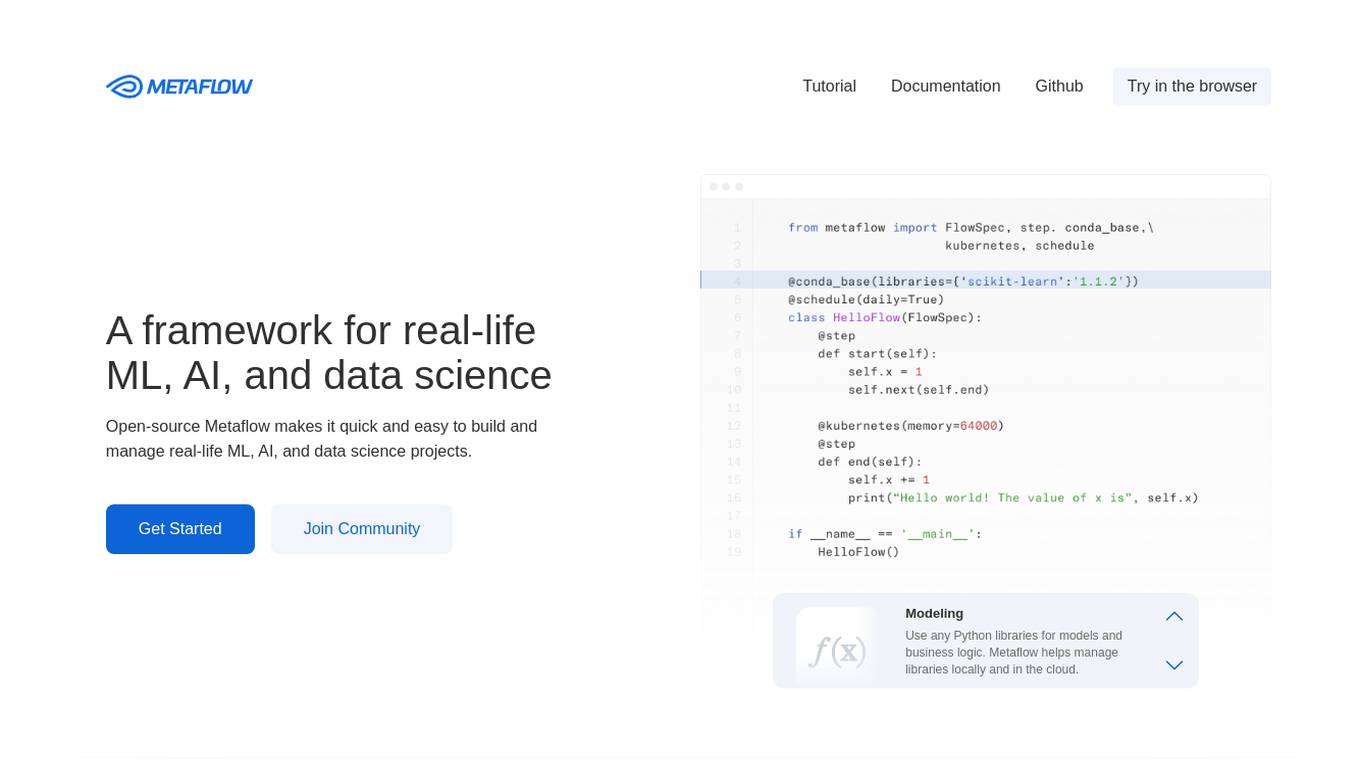
Metaflow
Metaflow is an open-source framework for building and managing real-life ML, AI, and data science projects. It makes it easy to use any Python libraries for models and business logic, deploy workflows to production with a single command, track and store variables inside the flow automatically for easy experiment tracking and debugging, and create robust workflows in plain Python. Metaflow is used by hundreds of companies, including Netflix, 23andMe, and Realtor.com.
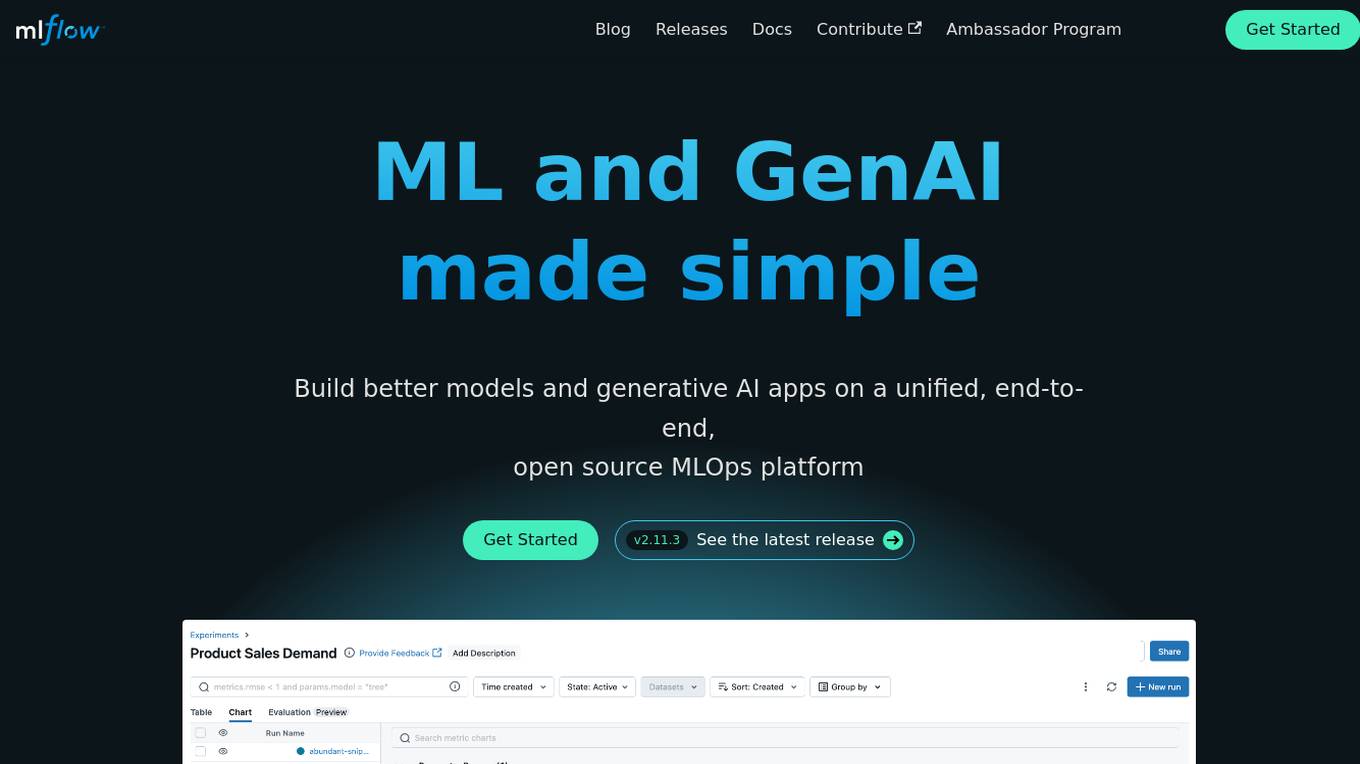
MLflow
MLflow is an open source platform for managing the end-to-end machine learning (ML) lifecycle, including tracking experiments, packaging models, deploying models, and managing model registries. It provides a unified platform for both traditional ML and generative AI applications.
20 - Open Source AI Tools
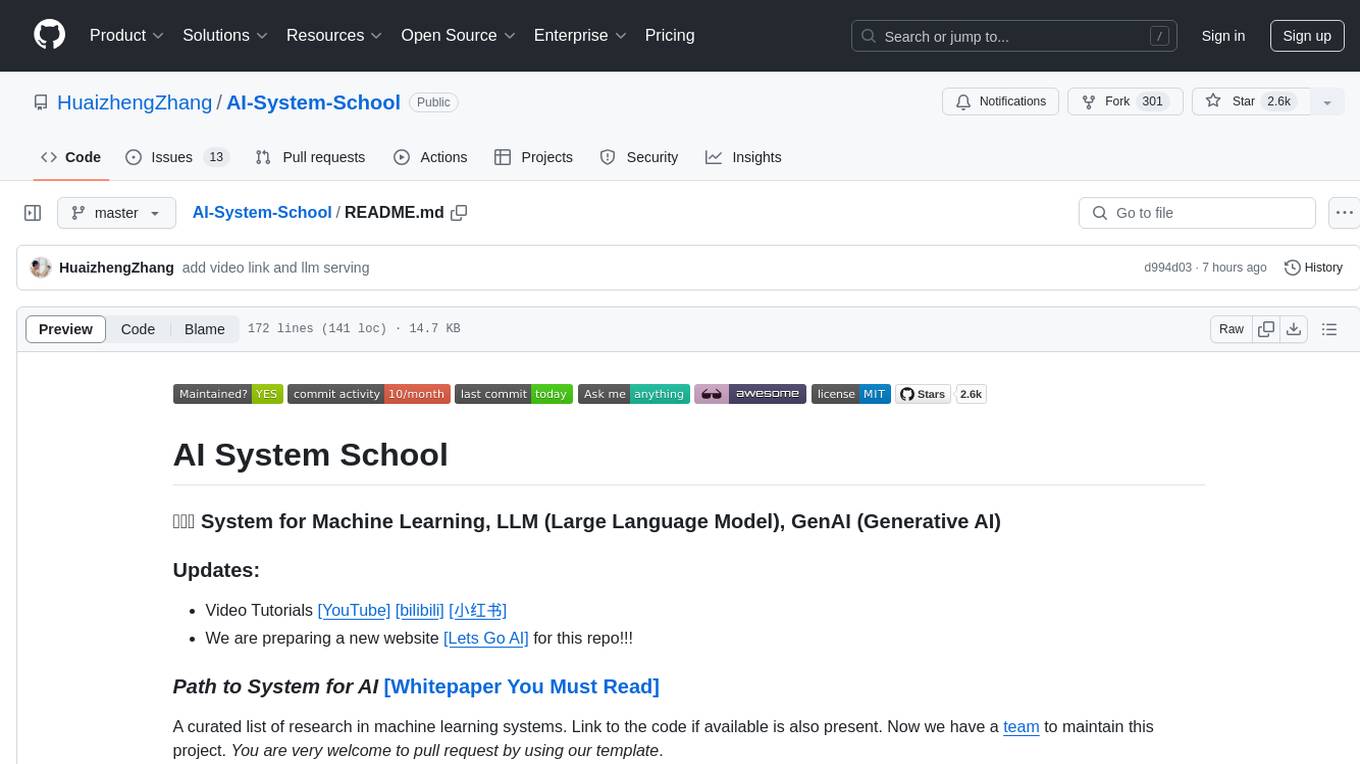
AI-System-School
AI System School is a curated list of research in machine learning systems, focusing on ML/DL infra, LLM infra, domain-specific infra, ML/LLM conferences, and general resources. It provides resources such as data processing, training systems, video systems, autoML systems, and more. The repository aims to help users navigate the landscape of AI systems and machine learning infrastructure, offering insights into conferences, surveys, books, videos, courses, and blogs related to the field.
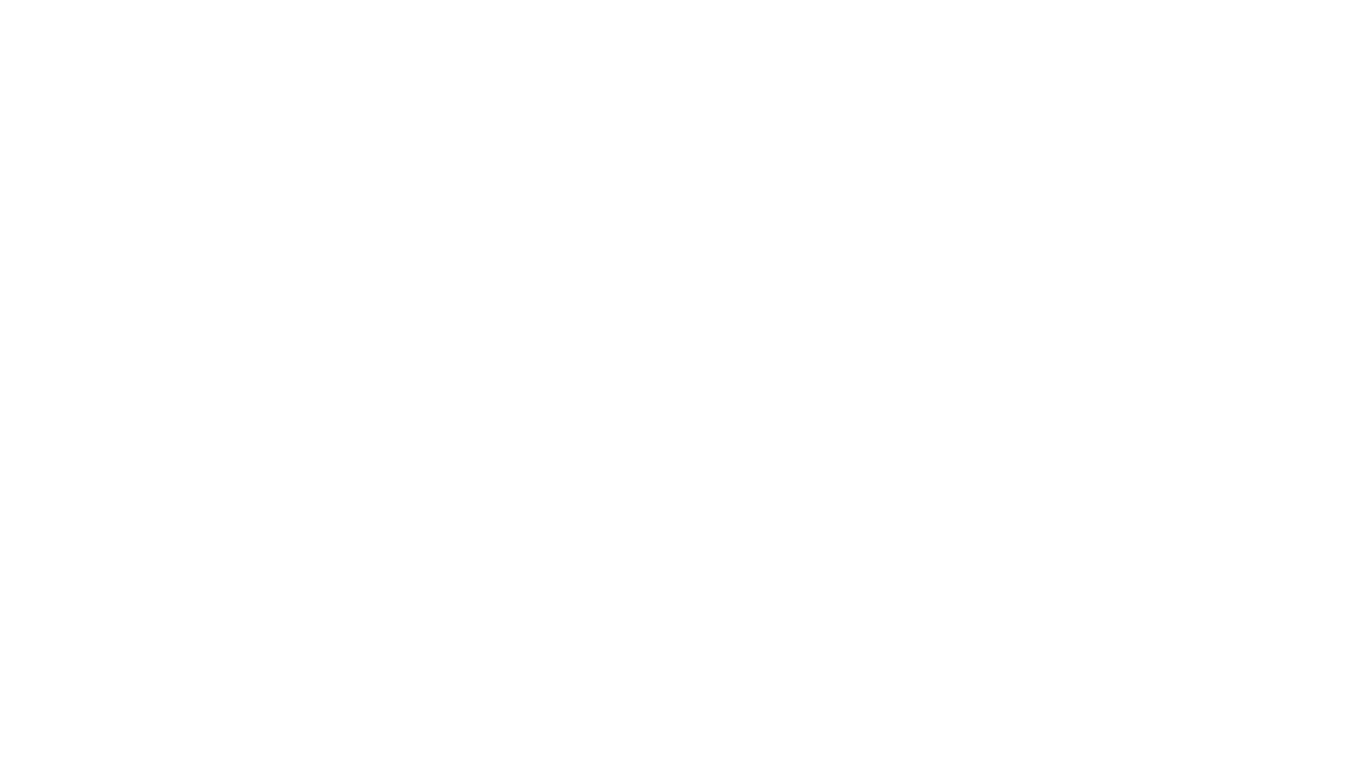
second-brain-ai-assistant-course
This open-source course teaches how to build an advanced RAG and LLM system using LLMOps and ML systems best practices. It helps you create an AI assistant that leverages your personal knowledge base to answer questions, summarize documents, and provide insights. The course covers topics such as LLM system architecture, pipeline orchestration, large-scale web crawling, model fine-tuning, and advanced RAG features. It is suitable for ML/AI engineers and data/software engineers & data scientists looking to level up to production AI systems. The course is free, with minimal costs for tools like OpenAI's API and Hugging Face's Dedicated Endpoints. Participants will build two separate Python applications for offline ML pipelines and online inference pipeline.
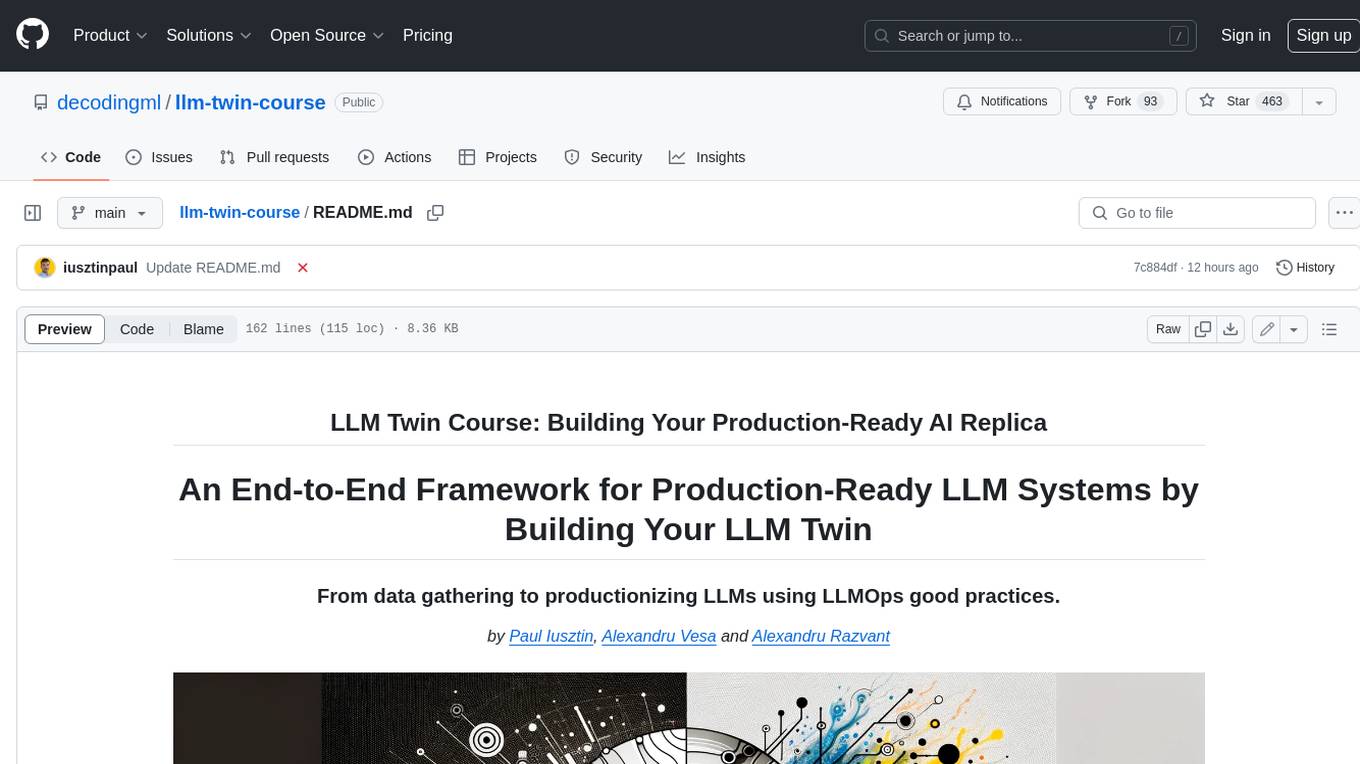
llm-twin-course
The LLM Twin Course is a free, end-to-end framework for building production-ready LLM systems. It teaches you how to design, train, and deploy a production-ready LLM twin of yourself powered by LLMs, vector DBs, and LLMOps good practices. The course is split into 11 hands-on written lessons and the open-source code you can access on GitHub. You can read everything and try out the code at your own pace.
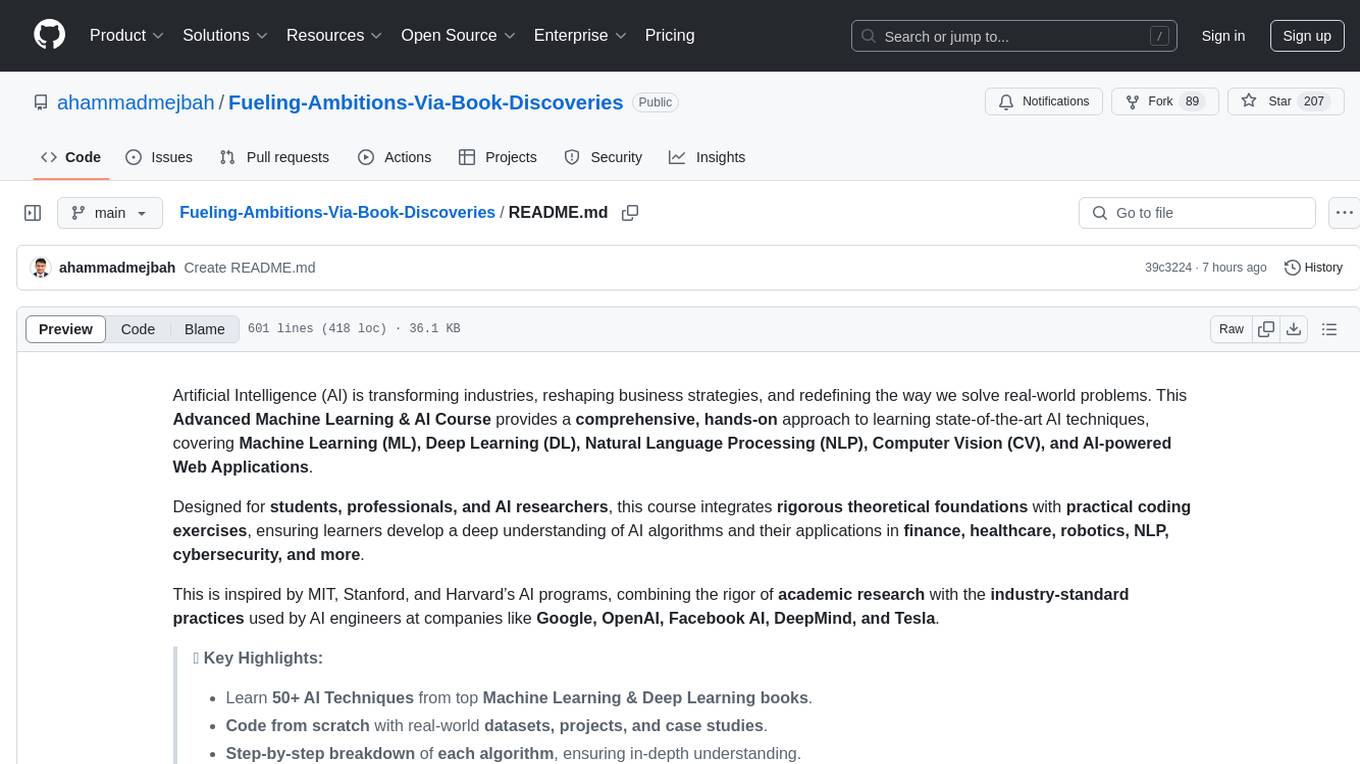
Fueling-Ambitions-Via-Book-Discoveries
Fueling-Ambitions-Via-Book-Discoveries is an Advanced Machine Learning & AI Course designed for students, professionals, and AI researchers. The course integrates rigorous theoretical foundations with practical coding exercises, ensuring learners develop a deep understanding of AI algorithms and their applications in finance, healthcare, robotics, NLP, cybersecurity, and more. Inspired by MIT, Stanford, and Harvard’s AI programs, it combines academic research rigor with industry-standard practices used by AI engineers at companies like Google, OpenAI, Facebook AI, DeepMind, and Tesla. Learners can learn 50+ AI techniques from top Machine Learning & Deep Learning books, code from scratch with real-world datasets, projects, and case studies, and focus on ML Engineering & AI Deployment using Django & Streamlit. The course also offers industry-relevant projects to build a strong AI portfolio.
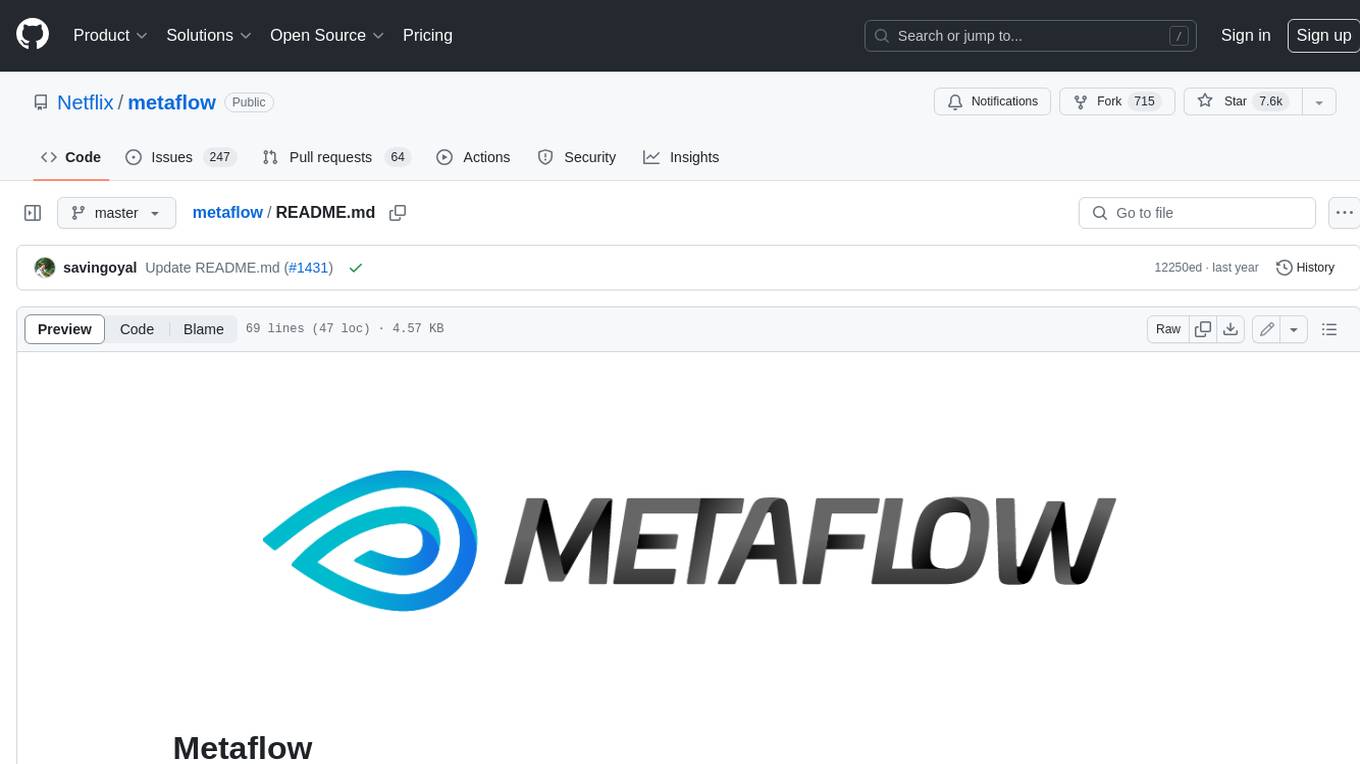
metaflow
Metaflow is a user-friendly library designed to assist scientists and engineers in developing and managing real-world data science projects. Initially created at Netflix, Metaflow aimed to enhance the productivity of data scientists working on diverse projects ranging from traditional statistics to cutting-edge deep learning. For further information, refer to Metaflow's website and documentation.
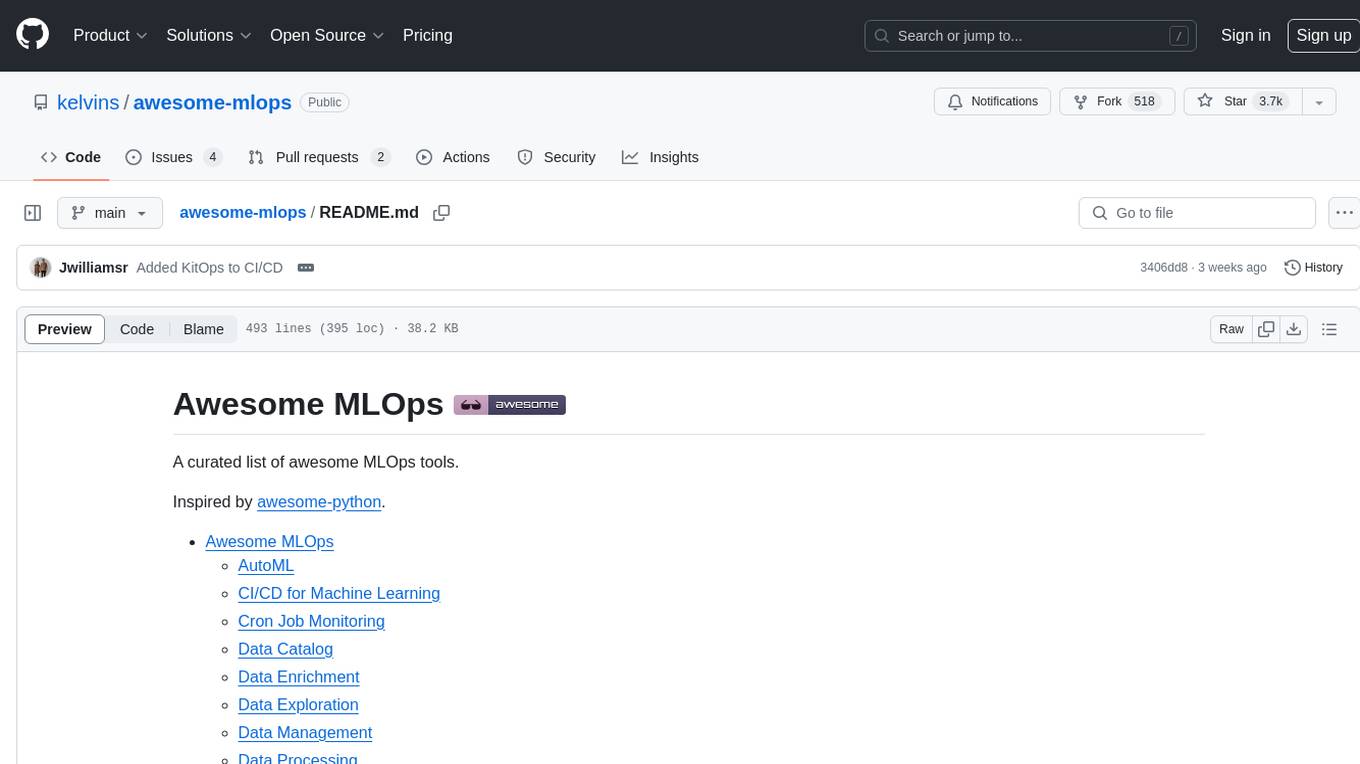
awesome-mlops
Awesome MLOps is a curated list of tools related to Machine Learning Operations, covering areas such as AutoML, CI/CD for Machine Learning, Data Cataloging, Data Enrichment, Data Exploration, Data Management, Data Processing, Data Validation, Data Visualization, Drift Detection, Feature Engineering, Feature Store, Hyperparameter Tuning, Knowledge Sharing, Machine Learning Platforms, Model Fairness and Privacy, Model Interpretability, Model Lifecycle, Model Serving, Model Testing & Validation, Optimization Tools, Simplification Tools, Visual Analysis and Debugging, and Workflow Tools. The repository provides a comprehensive collection of tools and resources for individuals and teams working in the field of MLOps.
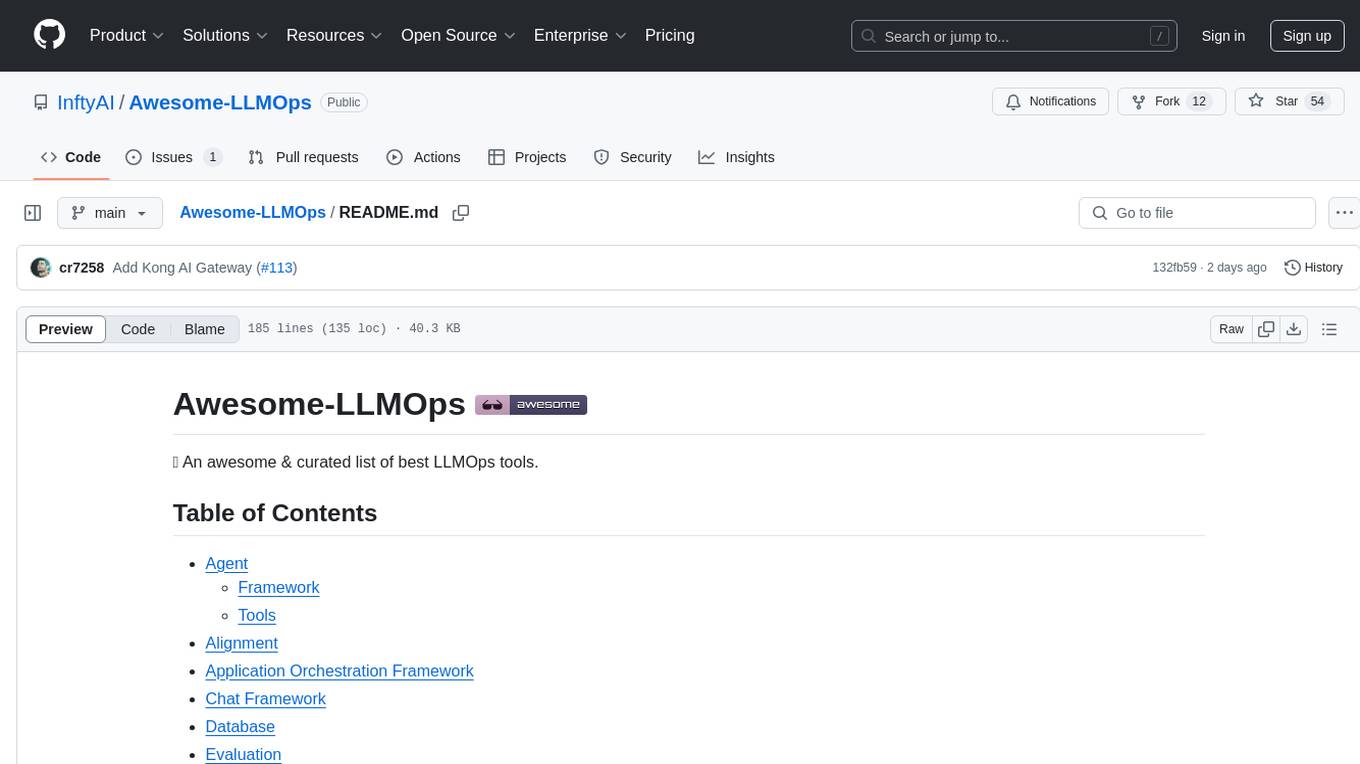
Awesome-LLMOps
Awesome-LLMOps is a curated list of the best LLMOps tools, providing a comprehensive collection of frameworks and tools for building, deploying, and managing large language models (LLMs) and AI agents. The repository includes a wide range of tools for tasks such as building multimodal AI agents, fine-tuning models, orchestrating applications, evaluating models, and serving models for inference. It covers various aspects of the machine learning operations (MLOps) lifecycle, from training to deployment and observability. The tools listed in this repository cater to the needs of developers, data scientists, and machine learning engineers working with large language models and AI applications.
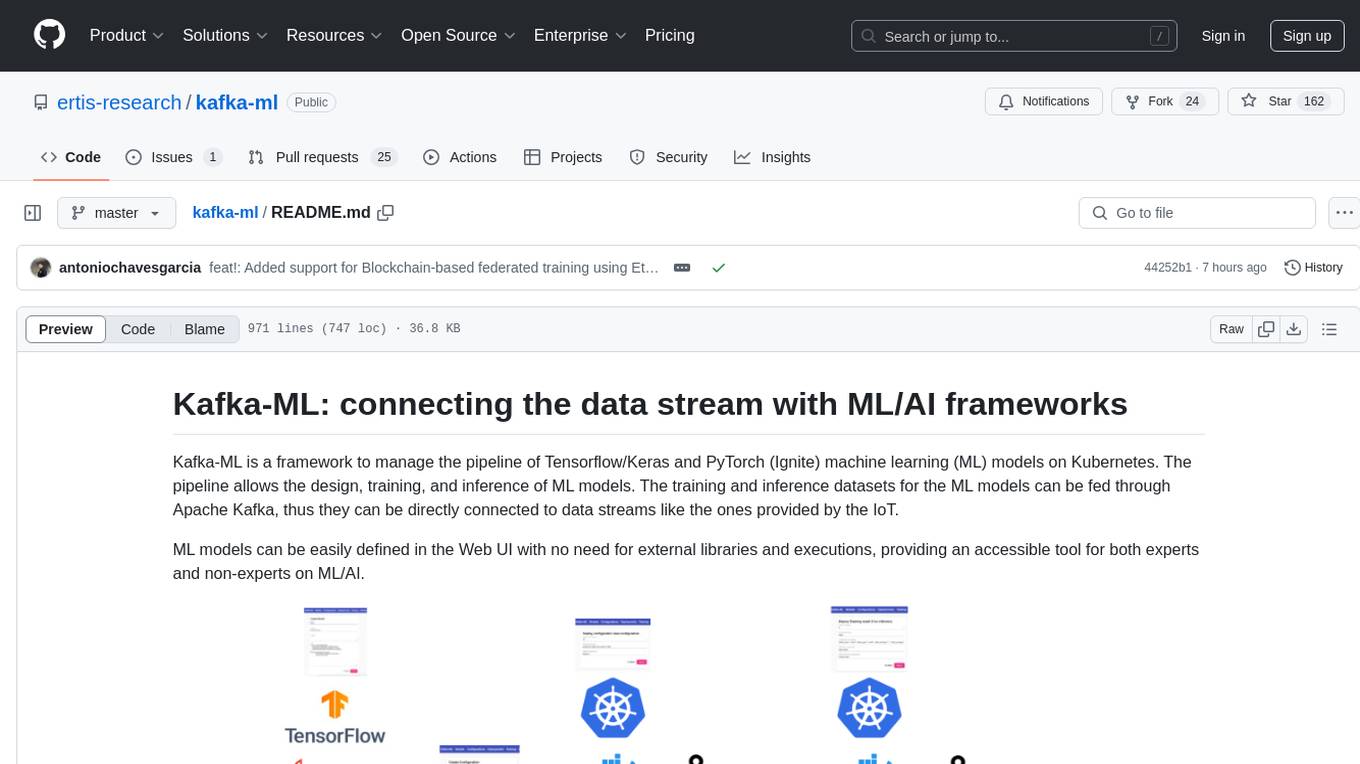
kafka-ml
Kafka-ML is a framework designed to manage the pipeline of Tensorflow/Keras and PyTorch machine learning models on Kubernetes. It enables the design, training, and inference of ML models with datasets fed through Apache Kafka, connecting them directly to data streams like those from IoT devices. The Web UI allows easy definition of ML models without external libraries, catering to both experts and non-experts in ML/AI.
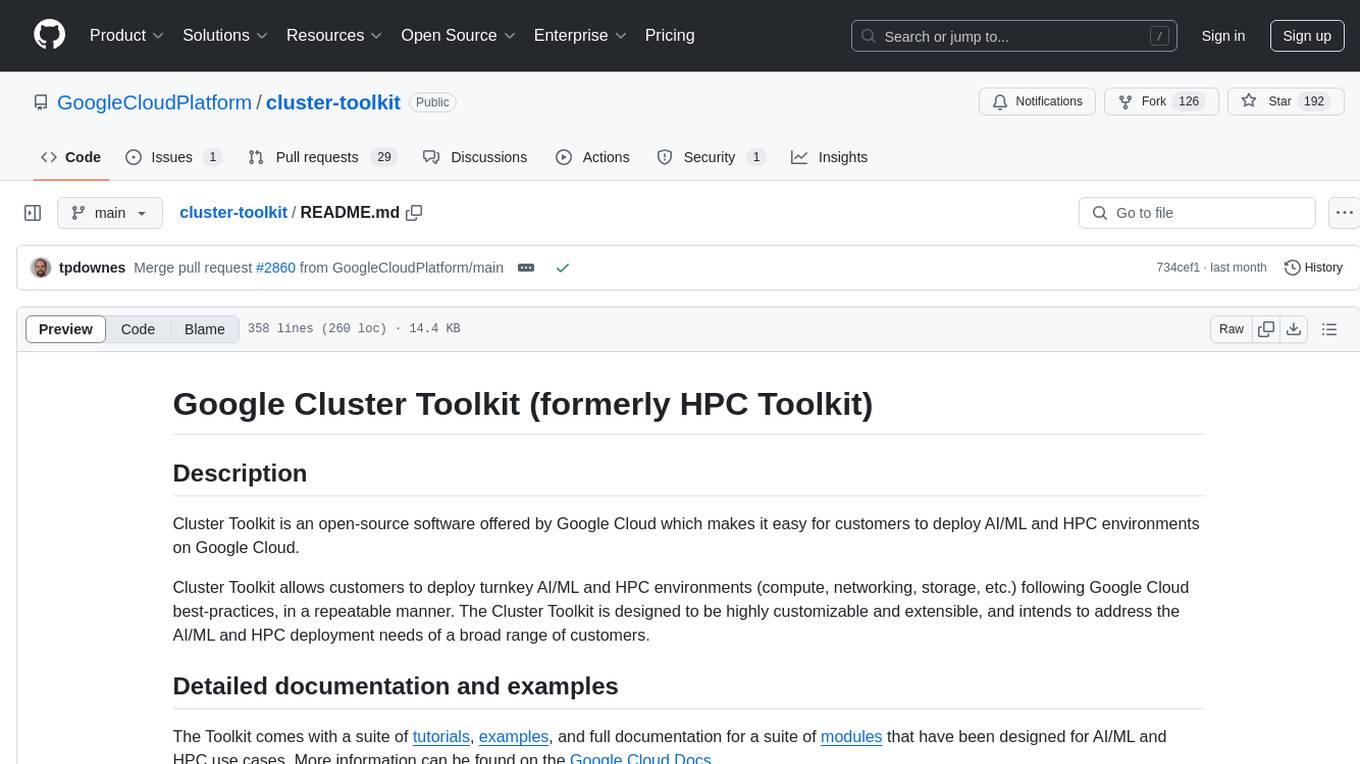
cluster-toolkit
Cluster Toolkit is an open-source software by Google Cloud for deploying AI/ML and HPC environments on Google Cloud. It allows easy deployment following best practices, with high customization and extensibility. The toolkit includes tutorials, examples, and documentation for various modules designed for AI/ML and HPC use cases.
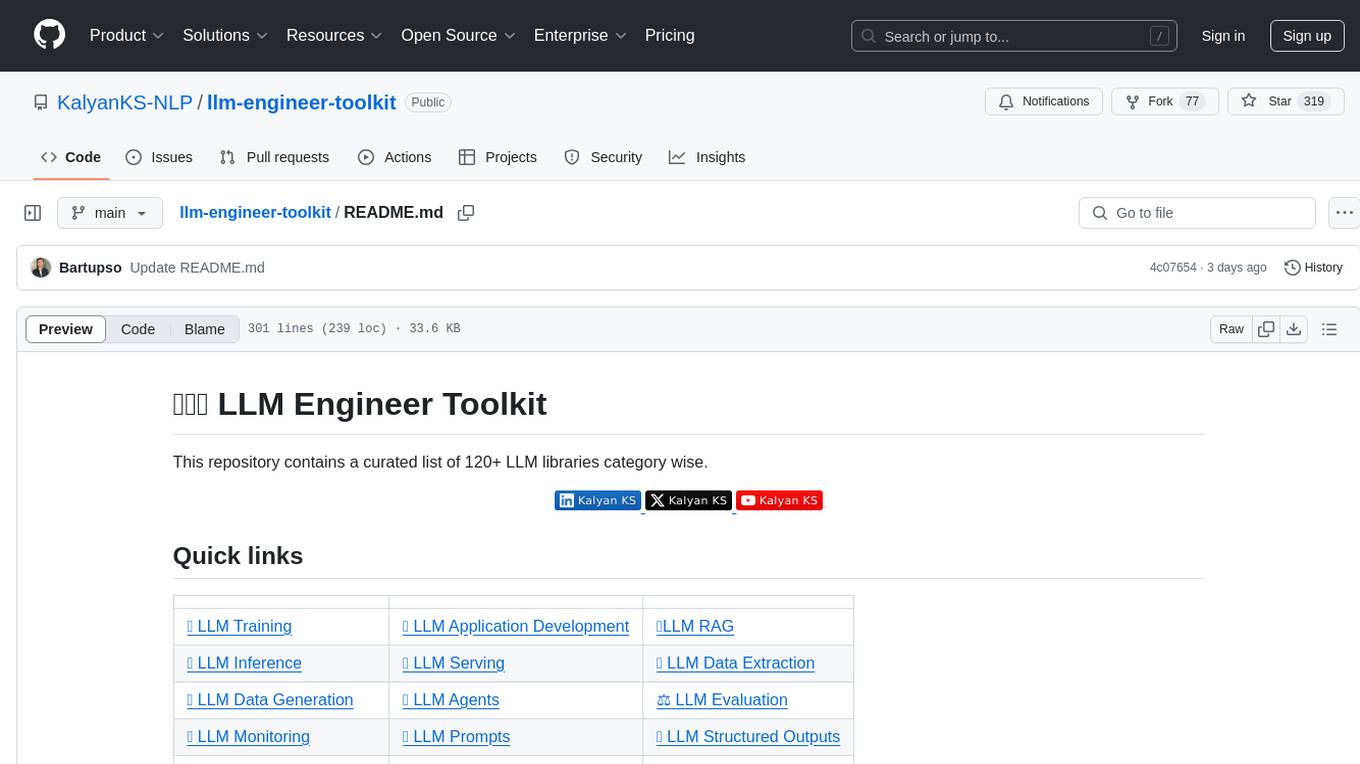
llm-engineer-toolkit
The LLM Engineer Toolkit is a curated repository containing over 120 LLM libraries categorized for various tasks such as training, application development, inference, serving, data extraction, data generation, agents, evaluation, monitoring, prompts, structured outputs, safety, security, embedding models, and other miscellaneous tools. It includes libraries for fine-tuning LLMs, building applications powered by LLMs, serving LLM models, extracting data, generating synthetic data, creating AI agents, evaluating LLM applications, monitoring LLM performance, optimizing prompts, handling structured outputs, ensuring safety and security, embedding models, and more. The toolkit covers a wide range of tools and frameworks to streamline the development, deployment, and optimization of large language models.
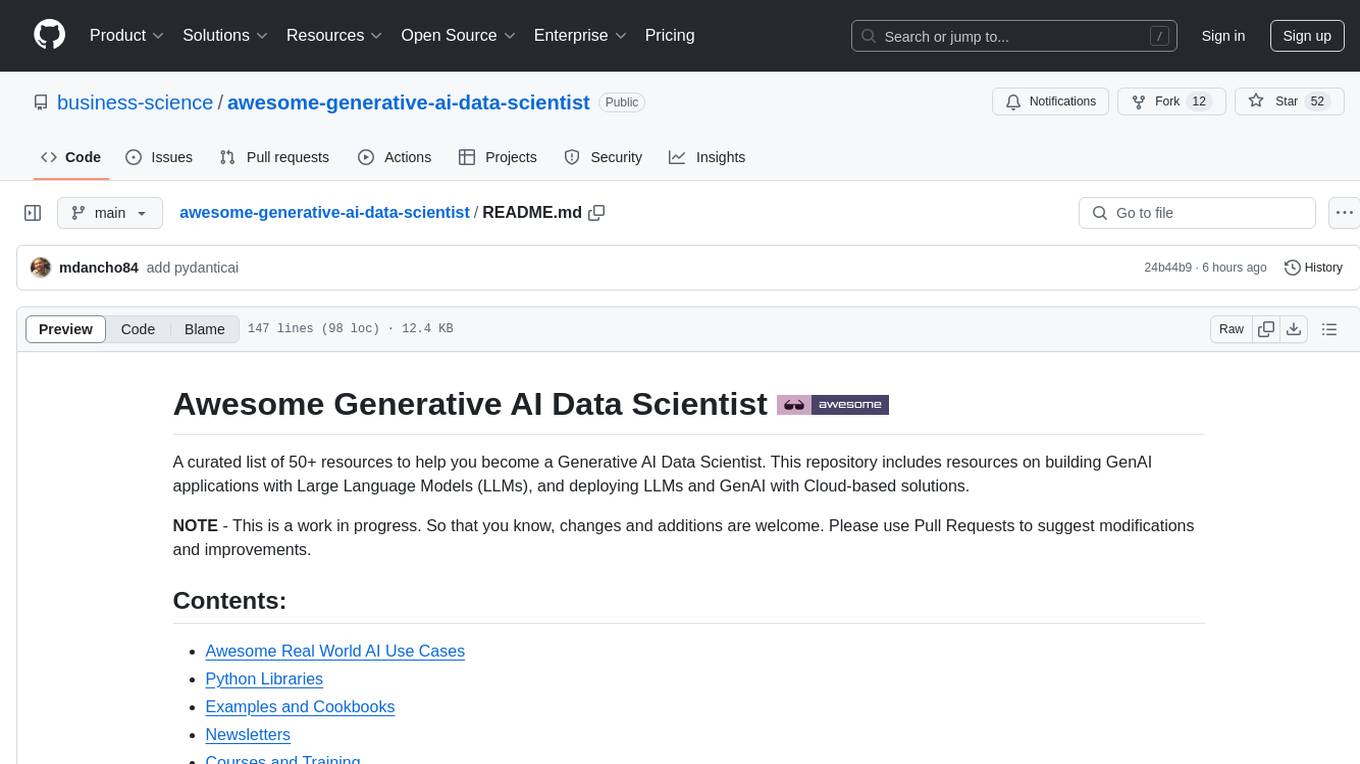
awesome-generative-ai-data-scientist
A curated list of 50+ resources to help you become a Generative AI Data Scientist. This repository includes resources on building GenAI applications with Large Language Models (LLMs), and deploying LLMs and GenAI with Cloud-based solutions.
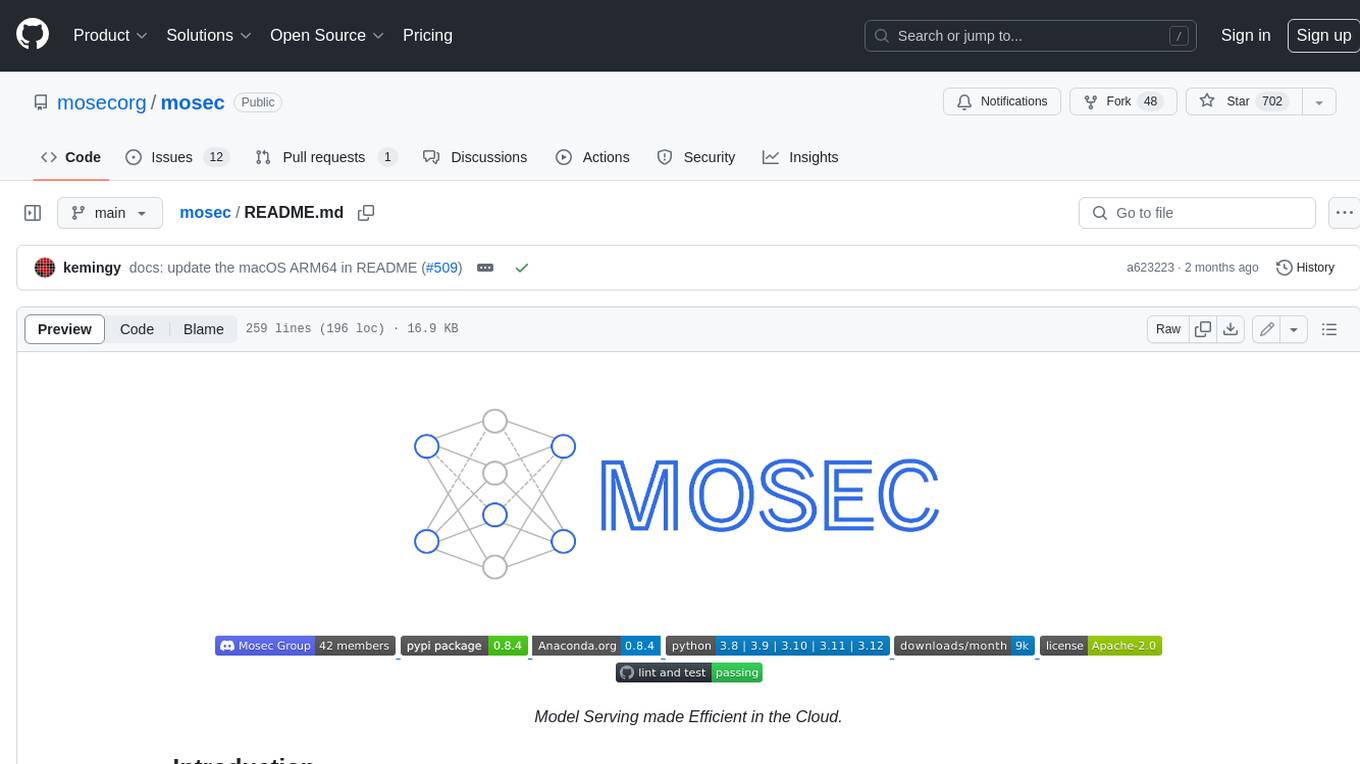
mosec
Mosec is a high-performance and flexible model serving framework for building ML model-enabled backend and microservices. It bridges the gap between any machine learning models you just trained and the efficient online service API. * **Highly performant** : web layer and task coordination built with Rust 🦀, which offers blazing speed in addition to efficient CPU utilization powered by async I/O * **Ease of use** : user interface purely in Python 🐍, by which users can serve their models in an ML framework-agnostic manner using the same code as they do for offline testing * **Dynamic batching** : aggregate requests from different users for batched inference and distribute results back * **Pipelined stages** : spawn multiple processes for pipelined stages to handle CPU/GPU/IO mixed workloads * **Cloud friendly** : designed to run in the cloud, with the model warmup, graceful shutdown, and Prometheus monitoring metrics, easily managed by Kubernetes or any container orchestration systems * **Do one thing well** : focus on the online serving part, users can pay attention to the model optimization and business logic
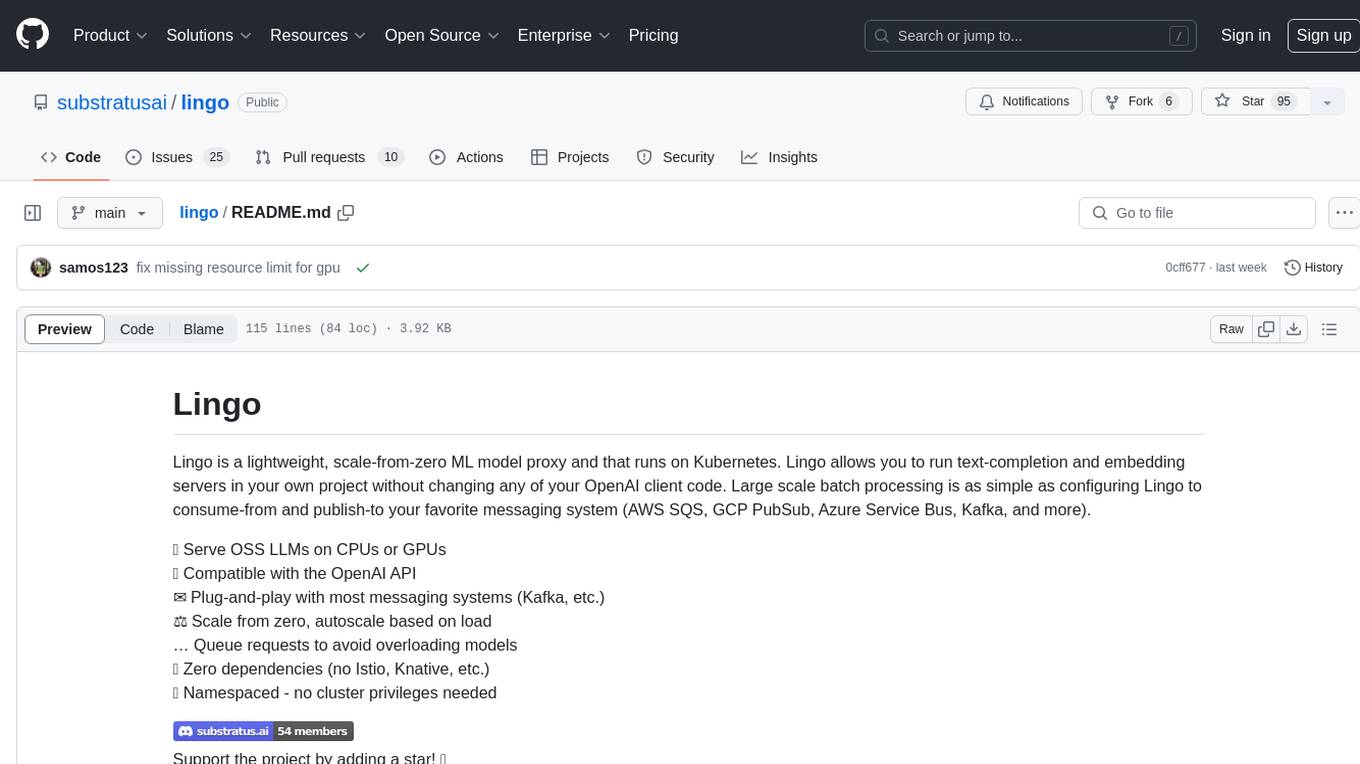
lingo
Lingo is a lightweight ML model proxy that runs on Kubernetes, allowing you to run text-completion and embedding servers without changing OpenAI client code. It supports serving OSS LLMs, is compatible with OpenAI API, plug-and-play with messaging systems, scales from zero based on load, and has zero dependencies. Namespaced with no cluster privileges needed.
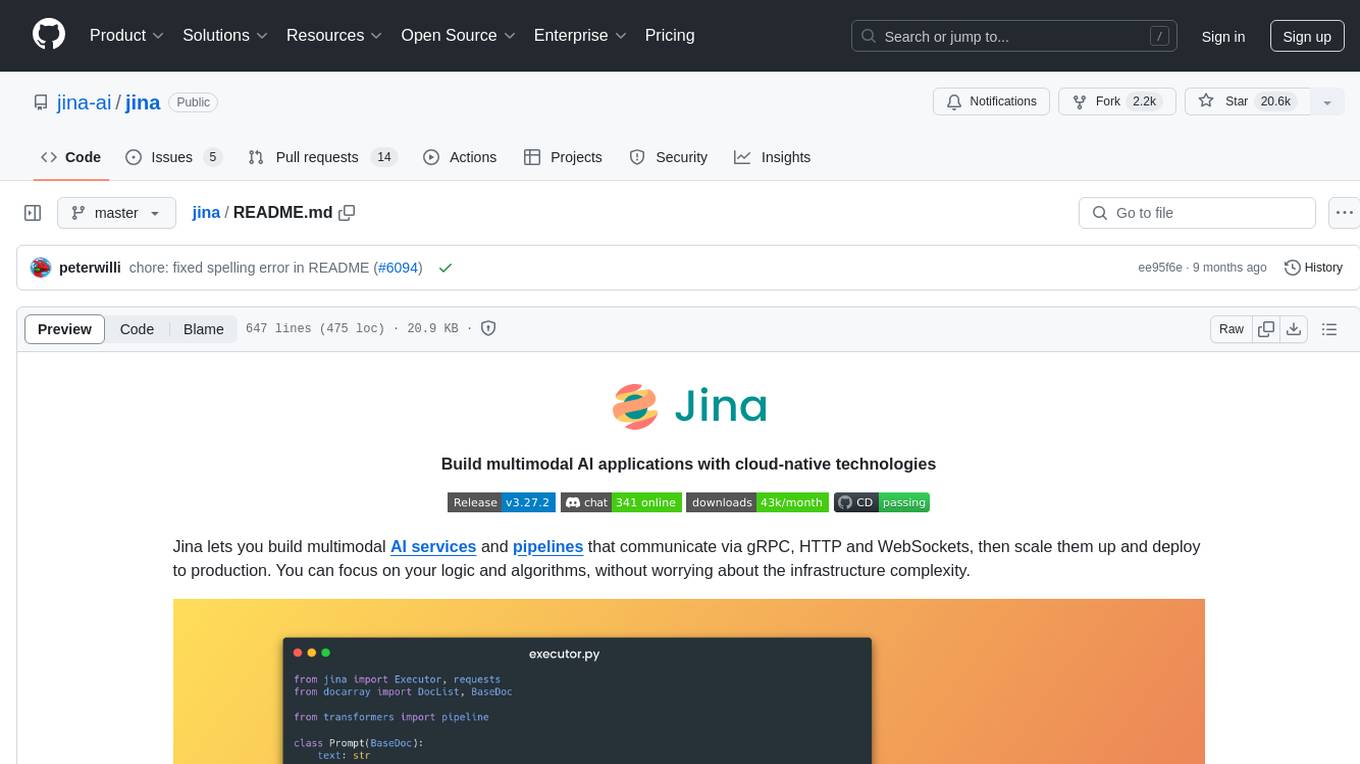
jina
Jina is a tool that allows users to build multimodal AI services and pipelines using cloud-native technologies. It provides a Pythonic experience for serving ML models and transitioning from local deployment to advanced orchestration frameworks like Docker-Compose, Kubernetes, or Jina AI Cloud. Users can build and serve models for any data type and deep learning framework, design high-performance services with easy scaling, serve LLM models while streaming their output, integrate with Docker containers via Executor Hub, and host on CPU/GPU using Jina AI Cloud. Jina also offers advanced orchestration and scaling capabilities, a smooth transition to the cloud, and easy scalability and concurrency features for applications. Users can deploy to their own cloud or system with Kubernetes and Docker Compose integration, and even deploy to JCloud for autoscaling and monitoring.
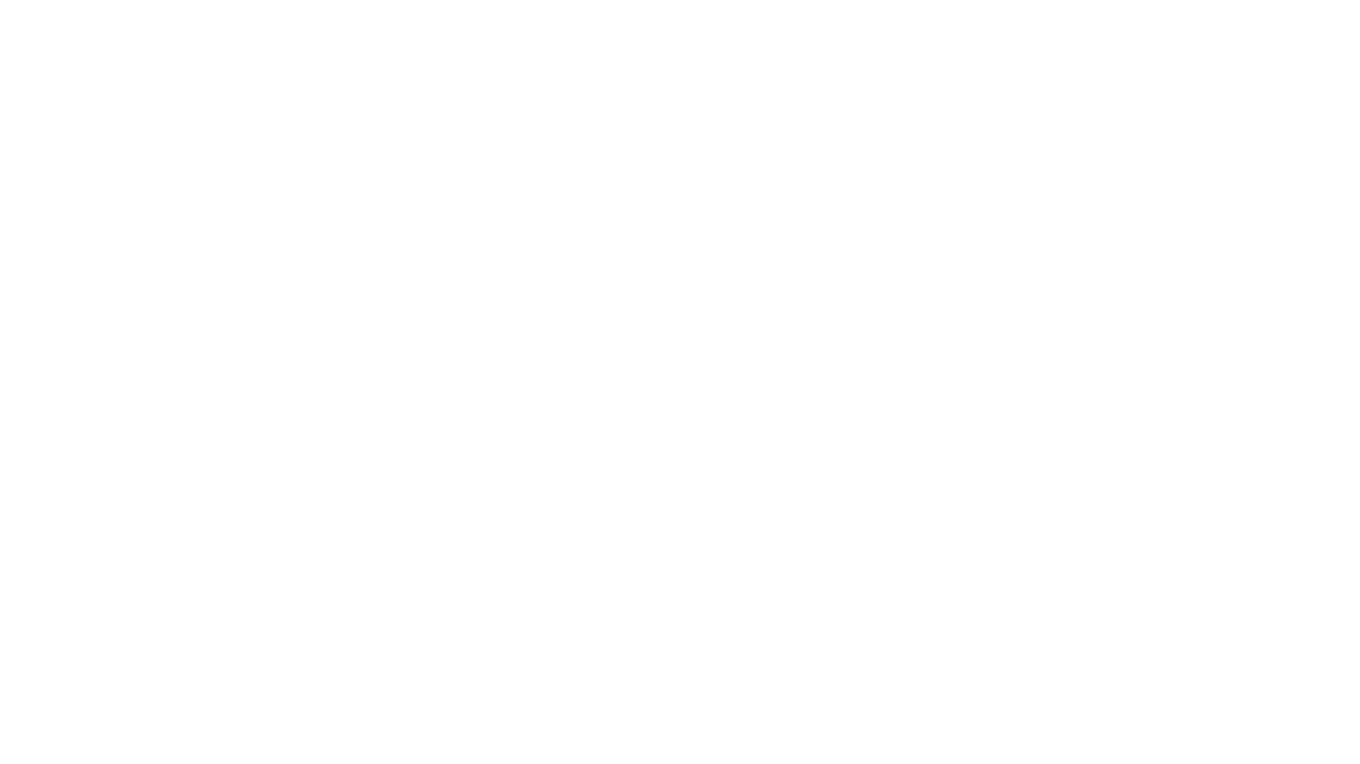
motia
Motia is an AI agent framework designed for software engineers to create, test, and deploy production-ready AI agents quickly. It provides a code-first approach, allowing developers to write agent logic in familiar languages and visualize execution in real-time. With Motia, developers can focus on business logic rather than infrastructure, offering zero infrastructure headaches, multi-language support, composable steps, built-in observability, instant APIs, and full control over AI logic. Ideal for building sophisticated agents and intelligent automations, Motia's event-driven architecture and modular steps enable the creation of GenAI-powered workflows, decision-making systems, and data processing pipelines.
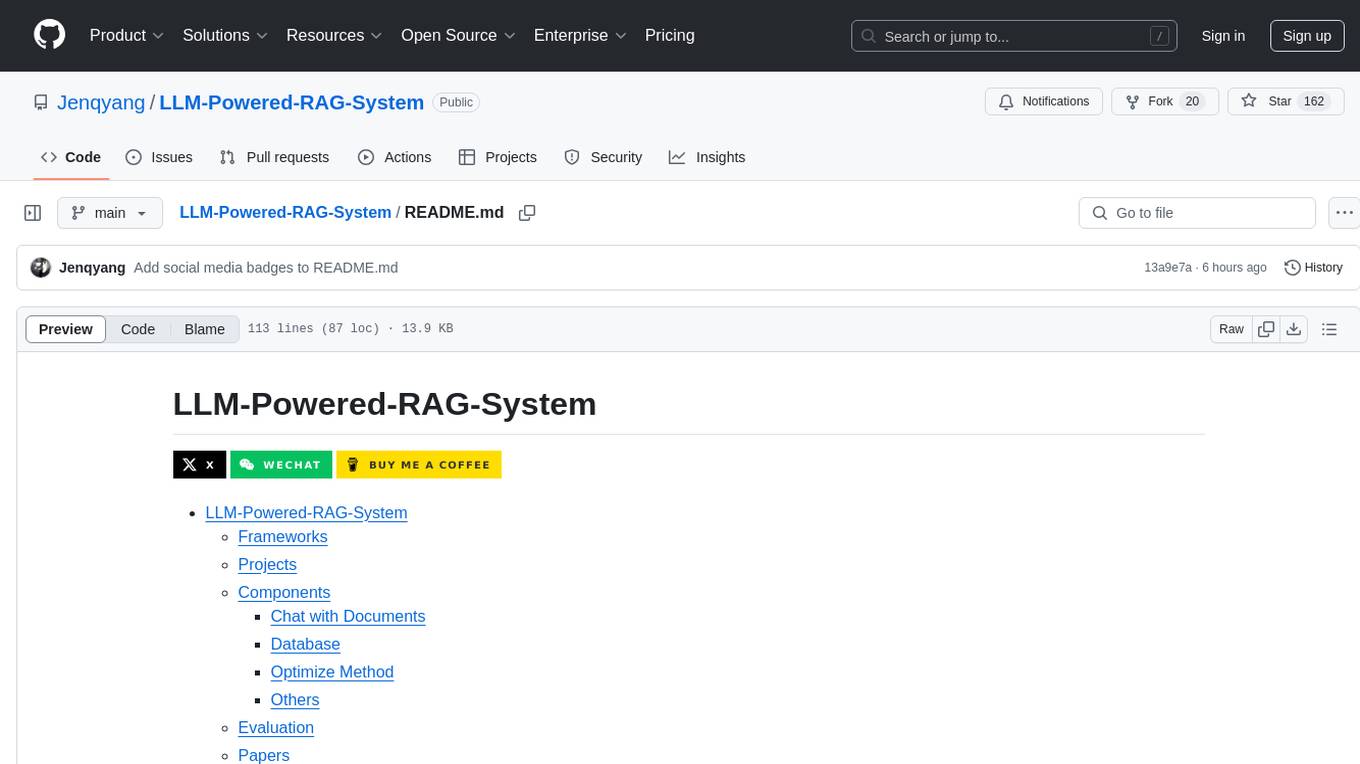
LLM-Powered-RAG-System
LLM-Powered-RAG-System is a comprehensive repository containing frameworks, projects, components, evaluation tools, papers, blogs, and other resources related to Retrieval-Augmented Generation (RAG) systems powered by Large Language Models (LLMs). The repository includes various frameworks for building applications with LLMs, data frameworks, modular graph-based RAG systems, dense retrieval models, and efficient retrieval augmentation and generation frameworks. It also features projects such as personal productivity assistants, knowledge-based platforms, chatbots, question and answer systems, and code assistants. Additionally, the repository provides components for interacting with documents, databases, and optimization methods using ML and LLM technologies. Evaluation frameworks, papers, blogs, and other resources related to RAG systems are also included.
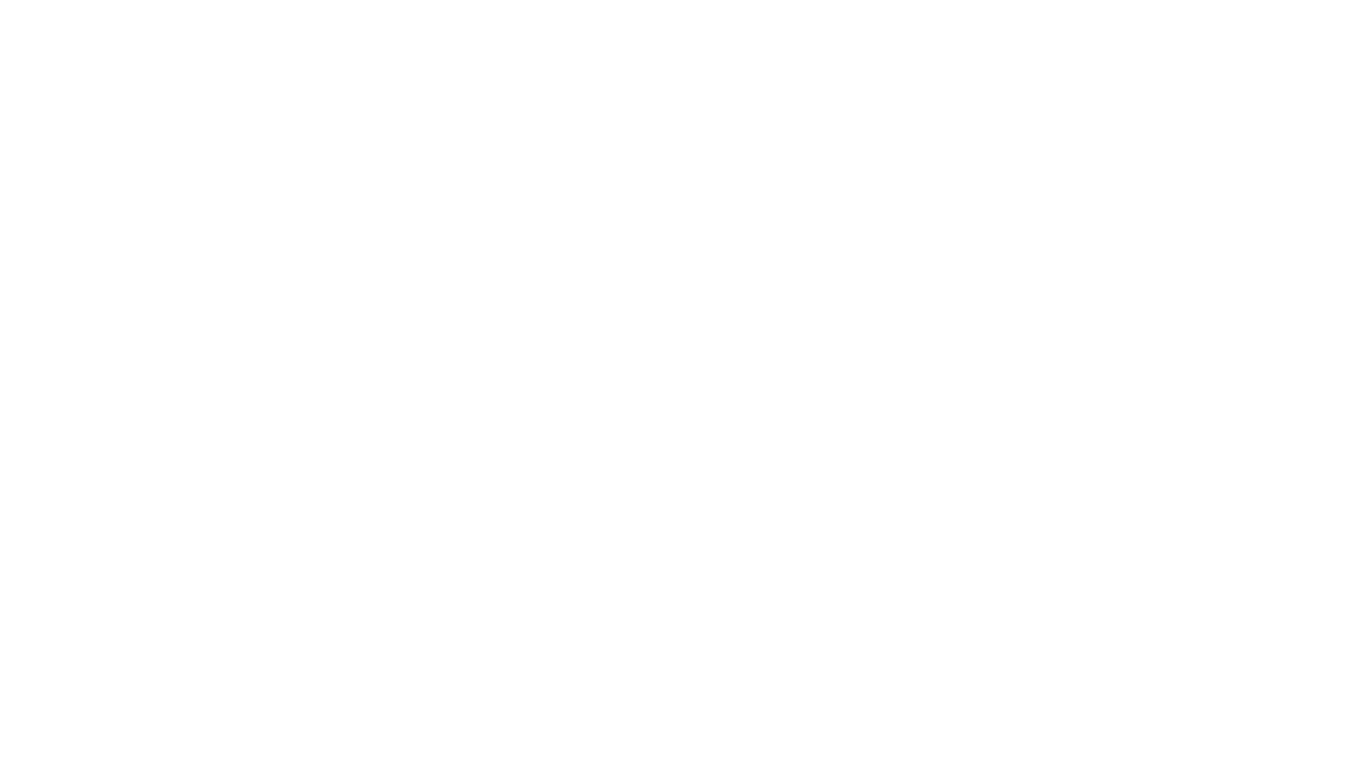
cognita
Cognita is an open-source framework to organize your RAG codebase along with a frontend to play around with different RAG customizations. It provides a simple way to organize your codebase so that it becomes easy to test it locally while also being able to deploy it in a production ready environment. The key issues that arise while productionizing RAG system from a Jupyter Notebook are: 1. **Chunking and Embedding Job** : The chunking and embedding code usually needs to be abstracted out and deployed as a job. Sometimes the job will need to run on a schedule or be trigerred via an event to keep the data updated. 2. **Query Service** : The code that generates the answer from the query needs to be wrapped up in a api server like FastAPI and should be deployed as a service. This service should be able to handle multiple queries at the same time and also autoscale with higher traffic. 3. **LLM / Embedding Model Deployment** : Often times, if we are using open-source models, we load the model in the Jupyter notebook. This will need to be hosted as a separate service in production and model will need to be called as an API. 4. **Vector DB deployment** : Most testing happens on vector DBs in memory or on disk. However, in production, the DBs need to be deployed in a more scalable and reliable way. Cognita makes it really easy to customize and experiment everything about a RAG system and still be able to deploy it in a good way. It also ships with a UI that makes it easier to try out different RAG configurations and see the results in real time. You can use it locally or with/without using any Truefoundry components. However, using Truefoundry components makes it easier to test different models and deploy the system in a scalable way. Cognita allows you to host multiple RAG systems using one app. ### Advantages of using Cognita are: 1. A central reusable repository of parsers, loaders, embedders and retrievers. 2. Ability for non-technical users to play with UI - Upload documents and perform QnA using modules built by the development team. 3. Fully API driven - which allows integration with other systems. > If you use Cognita with Truefoundry AI Gateway, you can get logging, metrics and feedback mechanism for your user queries. ### Features: 1. Support for multiple document retrievers that use `Similarity Search`, `Query Decompostion`, `Document Reranking`, etc 2. Support for SOTA OpenSource embeddings and reranking from `mixedbread-ai` 3. Support for using LLMs using `Ollama` 4. Support for incremental indexing that ingests entire documents in batches (reduces compute burden), keeps track of already indexed documents and prevents re-indexing of those docs.
20 - OpenAI Gpts
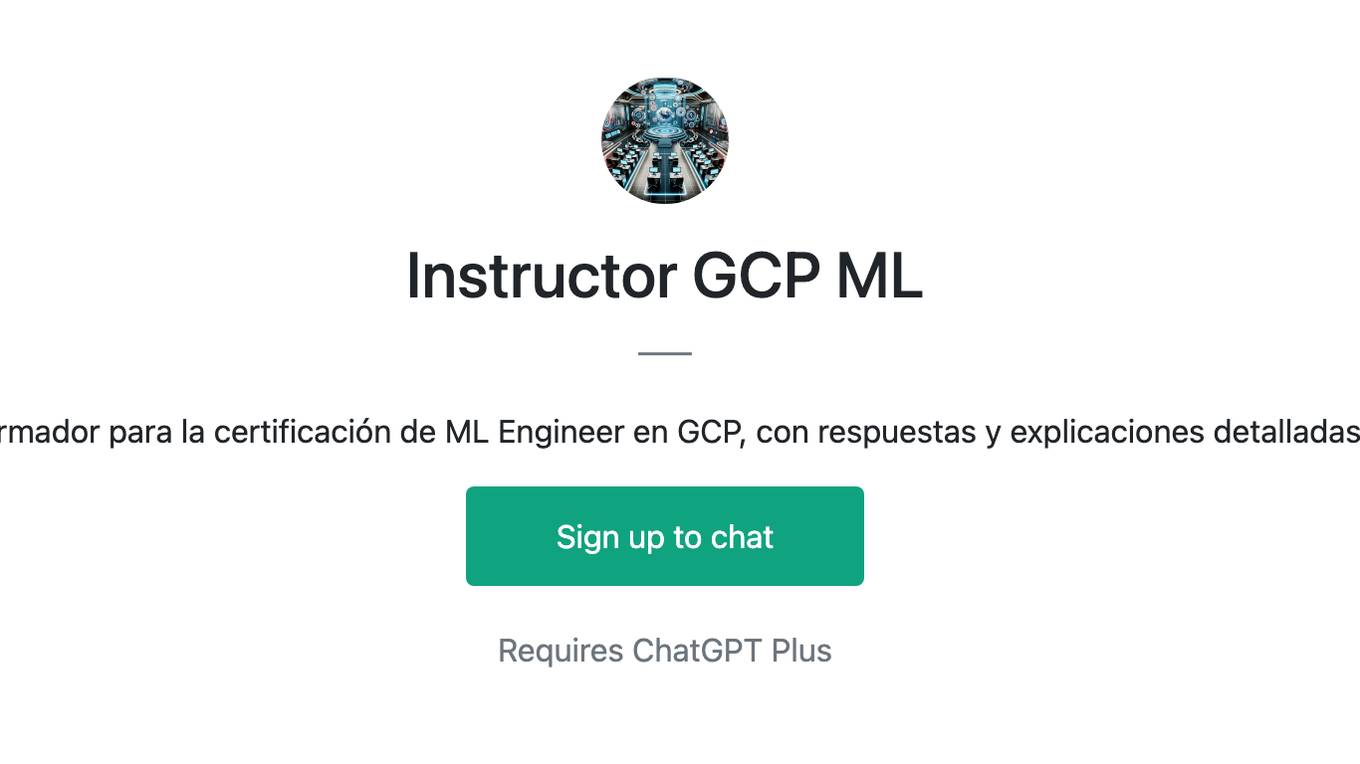
Instructor GCP ML
Formador para la certificación de ML Engineer en GCP, con respuestas y explicaciones detalladas.
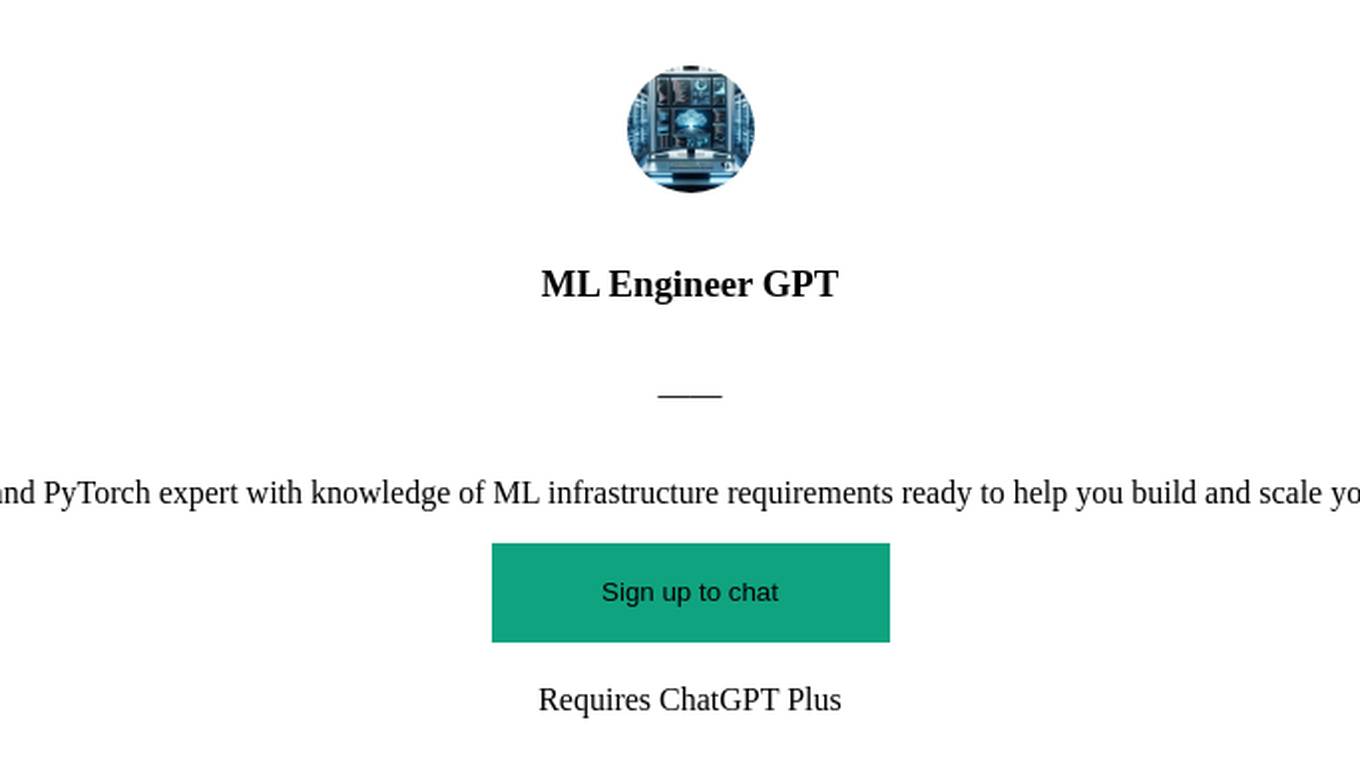
ML Engineer GPT
I'm a Python and PyTorch expert with knowledge of ML infrastructure requirements ready to help you build and scale your ML projects.
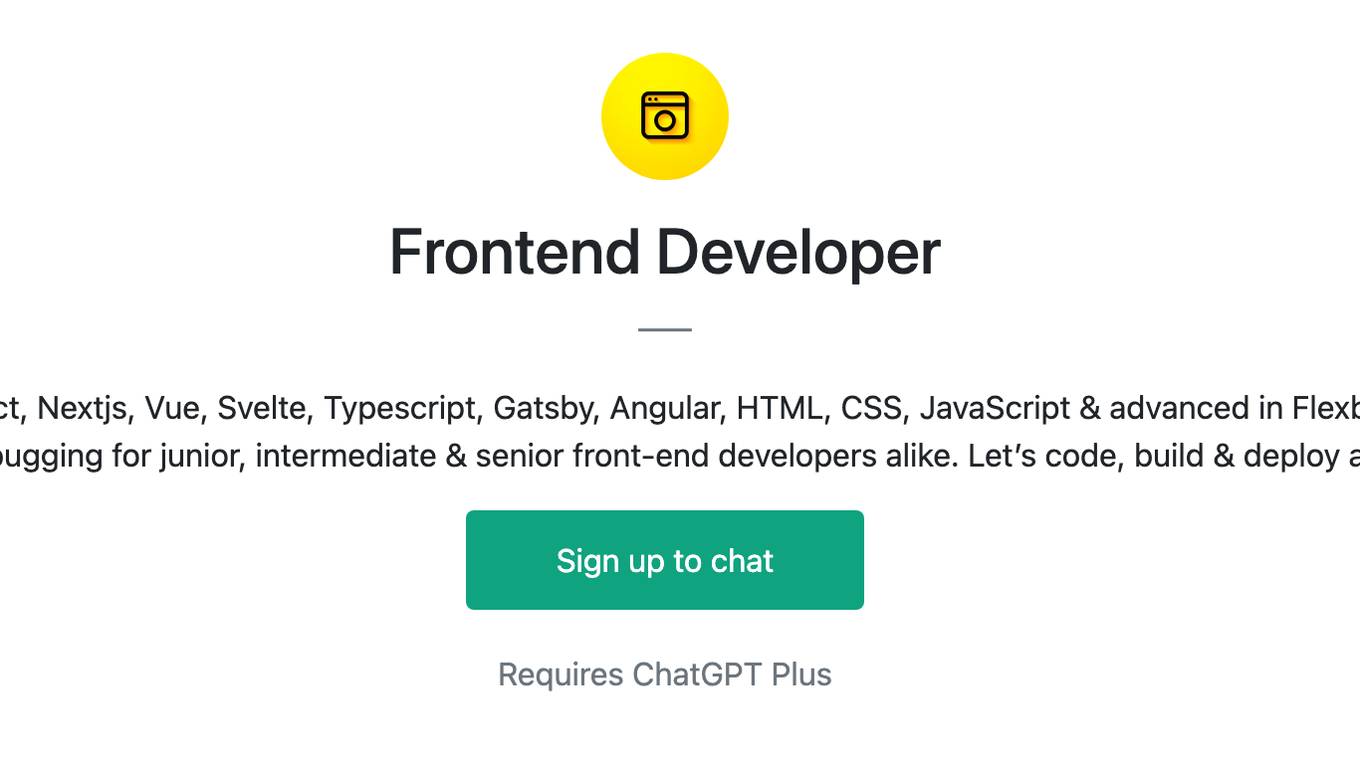
Frontend Developer
AI front-end developer expert in coding React, Nextjs, Vue, Svelte, Typescript, Gatsby, Angular, HTML, CSS, JavaScript & advanced in Flexbox, Tailwind & Material Design. Mentors in coding & debugging for junior, intermediate & senior front-end developers alike. Let’s code, build & deploy a SaaS app.
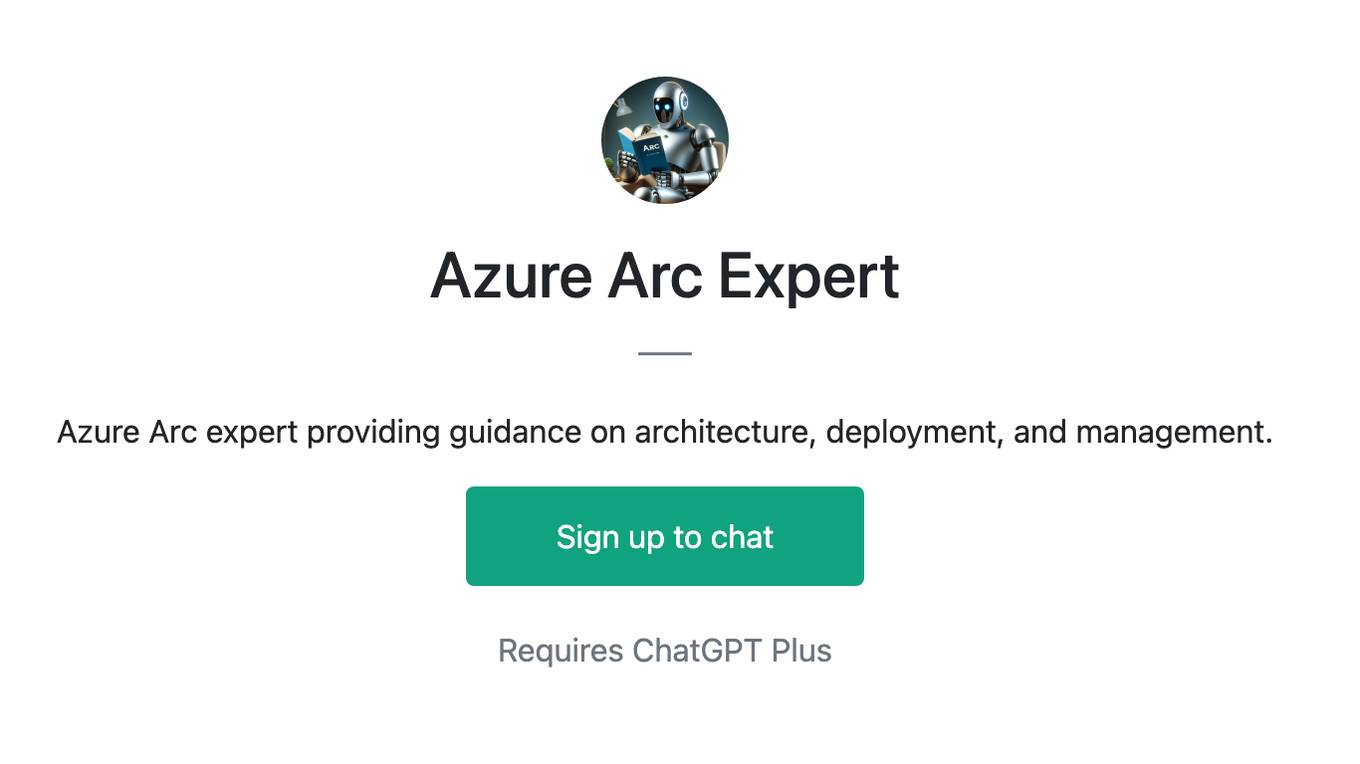
Azure Arc Expert
Azure Arc expert providing guidance on architecture, deployment, and management.
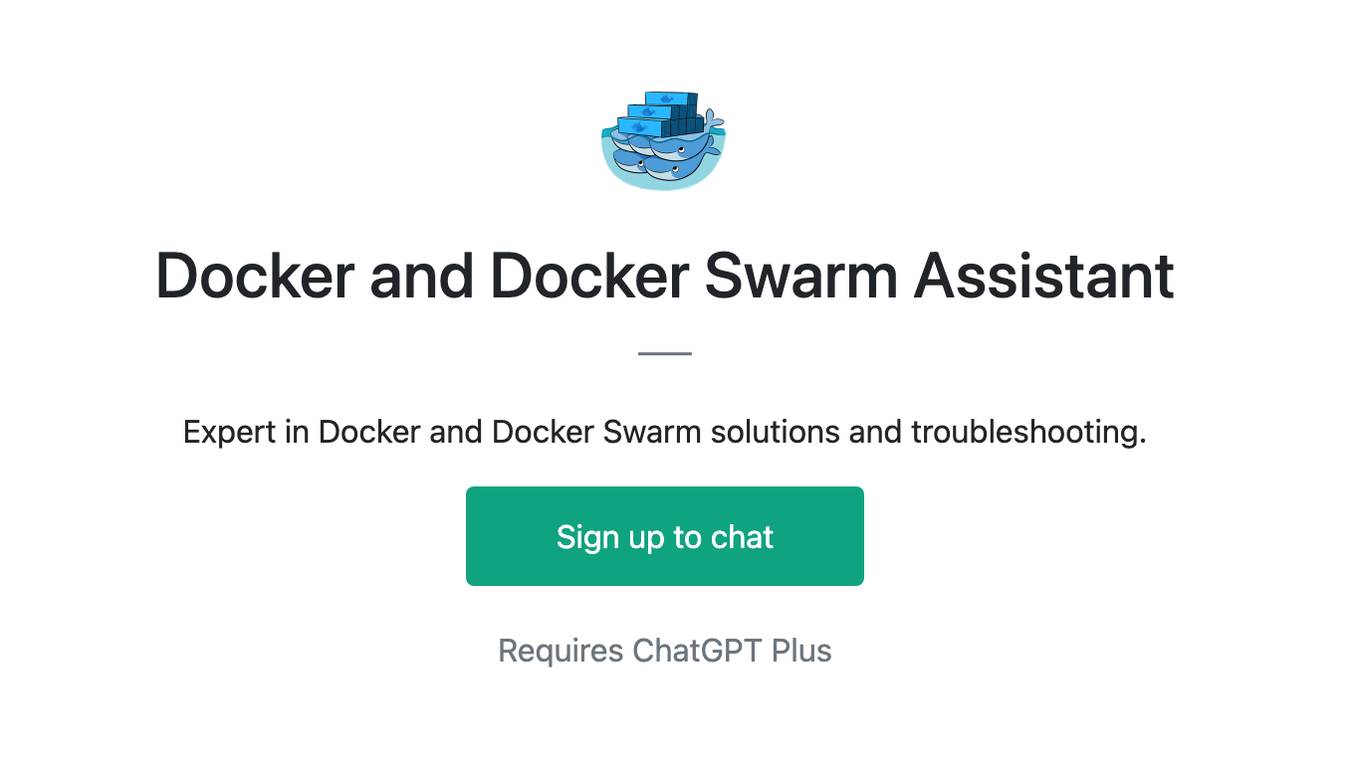
Docker and Docker Swarm Assistant
Expert in Docker and Docker Swarm solutions and troubleshooting.
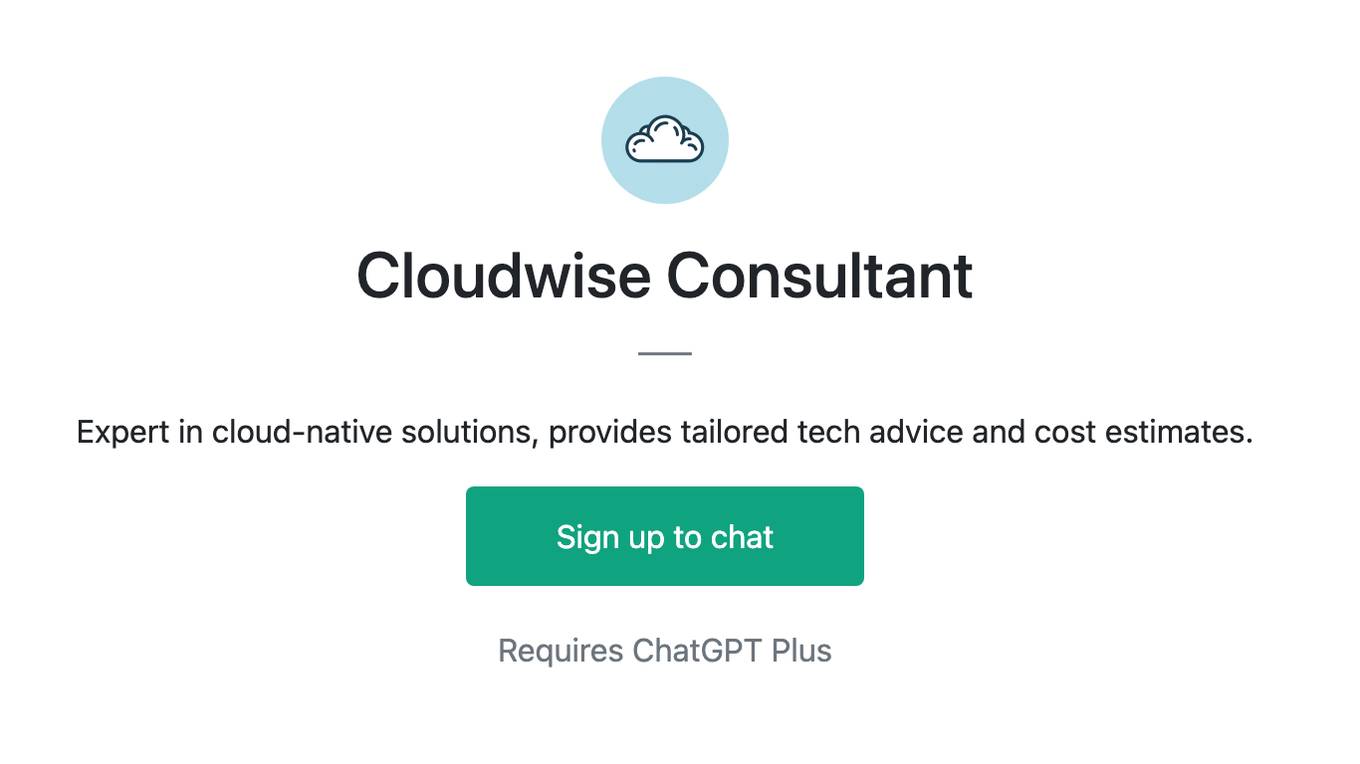
Cloudwise Consultant
Expert in cloud-native solutions, provides tailored tech advice and cost estimates.