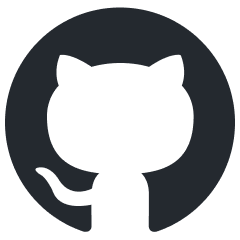
awesome-mlops
:sunglasses: A curated list of awesome MLOps tools
Stars: 3652
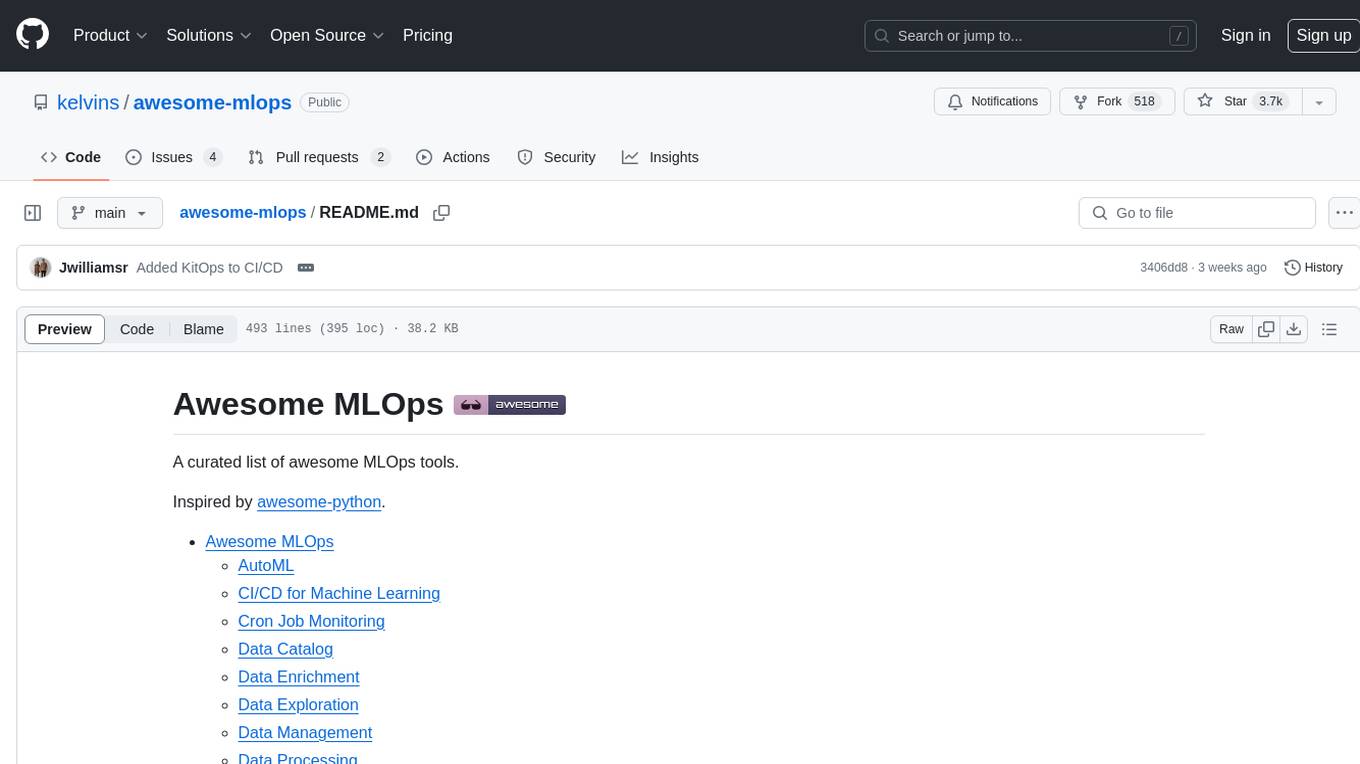
Awesome MLOps is a curated list of tools related to Machine Learning Operations, covering areas such as AutoML, CI/CD for Machine Learning, Data Cataloging, Data Enrichment, Data Exploration, Data Management, Data Processing, Data Validation, Data Visualization, Drift Detection, Feature Engineering, Feature Store, Hyperparameter Tuning, Knowledge Sharing, Machine Learning Platforms, Model Fairness and Privacy, Model Interpretability, Model Lifecycle, Model Serving, Model Testing & Validation, Optimization Tools, Simplification Tools, Visual Analysis and Debugging, and Workflow Tools. The repository provides a comprehensive collection of tools and resources for individuals and teams working in the field of MLOps.
README:
A curated list of awesome MLOps tools.
Inspired by awesome-python.
-
Awesome MLOps
- AutoML
- CI/CD for Machine Learning
- Cron Job Monitoring
- Data Catalog
- Data Enrichment
- Data Exploration
- Data Management
- Data Processing
- Data Validation
- Data Visualization
- Drift Detection
- Feature Engineering
- Feature Store
- Hyperparameter Tuning
- Knowledge Sharing
- Machine Learning Platform
- Model Fairness and Privacy
- Model Interpretability
- Model Lifecycle
- Model Serving
- Model Testing & Validation
- Optimization Tools
- Simplification Tools
- Visual Analysis and Debugging
- Workflow Tools
- Resources
- Contributing
Tools for performing AutoML.
- AutoGluon - Automated machine learning for image, text, tabular, time-series, and multi-modal data.
- AutoKeras - AutoKeras goal is to make machine learning accessible for everyone.
- AutoPyTorch - Automatic architecture search and hyperparameter optimization for PyTorch.
- AutoSKLearn - Automated machine learning toolkit and a drop-in replacement for a scikit-learn estimator.
- EvalML - A library that builds, optimizes, and evaluates ML pipelines using domain-specific functions.
- FLAML - Finds accurate ML models automatically, efficiently and economically.
- H2O AutoML - Automates ML workflow, which includes automatic training and tuning of models.
- MindsDB - AI layer for databases that allows you to effortlessly develop, train and deploy ML models.
- MLBox - MLBox is a powerful Automated Machine Learning python library.
- Model Search - Framework that implements AutoML algorithms for model architecture search at scale.
- NNI - An open source AutoML toolkit for automate machine learning lifecycle.
Tools for performing CI/CD for Machine Learning.
- ClearML - Auto-Magical CI/CD to streamline your ML workflow.
- CML - Open-source library for implementing CI/CD in machine learning projects.
- KitOps – Open source MLOps project that eases model handoffs between data scientist and DevOps.
Tools for monitoring cron jobs (recurring jobs).
- Cronitor - Monitor any cron job or scheduled task.
- HealthchecksIO - Simple and effective cron job monitoring.
Tools for data cataloging.
- Amundsen - Data discovery and metadata engine for improving the productivity when interacting with data.
- Apache Atlas - Provides open metadata management and governance capabilities to build a data catalog.
- CKAN - Open-source DMS (data management system) for powering data hubs and data portals.
- DataHub - LinkedIn's generalized metadata search & discovery tool.
- Magda - A federated, open-source data catalog for all your big data and small data.
- Metacat - Unified metadata exploration API service for Hive, RDS, Teradata, Redshift, S3 and Cassandra.
- OpenMetadata - A Single place to discover, collaborate and get your data right.
Tools and libraries for data enrichment.
- Snorkel - A system for quickly generating training data with weak supervision.
- Upgini - Enriches training datasets with features from public and community shared data sources.
Tools for performing data exploration.
- Apache Zeppelin - Enables data-driven, interactive data analytics and collaborative documents.
- BambooLib - An intuitive GUI for Pandas DataFrames.
- DataPrep - Collect, clean and visualize your data in Python.
- Google Colab - Hosted Jupyter notebook service that requires no setup to use.
- Jupyter Notebook - Web-based notebook environment for interactive computing.
- JupyterLab - The next-generation user interface for Project Jupyter.
- Jupytext - Jupyter Notebooks as Markdown Documents, Julia, Python or R scripts.
- Pandas Profiling - Create HTML profiling reports from pandas DataFrame objects.
- Polynote - The polyglot notebook with first-class Scala support.
Tools for performing data management.
- Arrikto - Dead simple, ultra fast storage for the hybrid Kubernetes world.
- BlazingSQL - A lightweight, GPU accelerated, SQL engine for Python. Built on RAPIDS cuDF.
- Delta Lake - Storage layer that brings scalable, ACID transactions to Apache Spark and other engines.
- Dolt - SQL database that you can fork, clone, branch, merge, push and pull just like a git repository.
- Dud - A lightweight CLI tool for versioning data alongside source code and building data pipelines.
- DVC - Management and versioning of datasets and machine learning models.
- Git LFS - An open source Git extension for versioning large files.
- Hub - A dataset format for creating, storing, and collaborating on AI datasets of any size.
- Intake - A lightweight set of tools for loading and sharing data in data science projects.
- lakeFS - Repeatable, atomic and versioned data lake on top of object storage.
- Marquez - Collect, aggregate, and visualize a data ecosystem's metadata.
- Milvus - An open source embedding vector similarity search engine powered by Faiss, NMSLIB and Annoy.
- Pinecone - Managed and distributed vector similarity search used with a lightweight SDK.
- Qdrant - An open source vector similarity search engine with extended filtering support.
- Quilt - A self-organizing data hub with S3 support.
Tools related to data processing and data pipelines.
- Airflow - Platform to programmatically author, schedule, and monitor workflows.
- Azkaban - Batch workflow job scheduler created at LinkedIn to run Hadoop jobs.
- Dagster - A data orchestrator for machine learning, analytics, and ETL.
- Hadoop - Framework that allows for the distributed processing of large data sets across clusters.
- OpenRefine - Power tool for working with messy data and improving it.
- Spark - Unified analytics engine for large-scale data processing.
Tools related to data validation.
- Cerberus - Lightweight, extensible data validation library for Python.
- Cleanlab - Python library for data-centric AI and machine learning with messy, real-world data and labels.
- Great Expectations - A Python data validation framework that allows to test your data against datasets.
- JSON Schema - A vocabulary that allows you to annotate and validate JSON documents.
- TFDV - An library for exploring and validating machine learning data.
Tools for data visualization, reports and dashboards.
- Count - SQL/drag-and-drop querying and visualisation tool based on notebooks.
- Dash - Analytical Web Apps for Python, R, Julia, and Jupyter.
- Data Studio - Reporting solution for power users who want to go beyond the data and dashboards of GA.
- Facets - Visualizations for understanding and analyzing machine learning datasets.
- Grafana - Multi-platform open source analytics and interactive visualization web application.
- Lux - Fast and easy data exploration by automating the visualization and data analysis process.
- Metabase - The simplest, fastest way to get business intelligence and analytics to everyone.
- Redash - Connect to any data source, easily visualize, dashboard and share your data.
- SolidUI - AI-generated visualization prototyping and editing platform, support 2D and 3D models.
- Superset - Modern, enterprise-ready business intelligence web application.
- Tableau - Powerful and fastest growing data visualization tool used in the business intelligence industry.
Tools and libraries related to drift detection.
- Alibi Detect - An open source Python library focused on outlier, adversarial and drift detection.
- Frouros - An open source Python library for drift detection in machine learning systems.
- TorchDrift - A data and concept drift library for PyTorch.
Tools and libraries related to feature engineering.
- Feature Engine - Feature engineering package with SKlearn like functionality.
- Featuretools - Python library for automated feature engineering.
- TSFresh - Python library for automatic extraction of relevant features from time series.
Feature store tools for data serving.
- Butterfree - A tool for building feature stores. Transform your raw data into beautiful features.
- ByteHub - An easy-to-use feature store. Optimized for time-series data.
- Feast - End-to-end open source feature store for machine learning.
- Feathr - An enterprise-grade, high performance feature store.
- Featureform - A Virtual Feature Store. Turn your existing data infrastructure into a feature store.
- Tecton - A fully-managed feature platform built to orchestrate the complete lifecycle of features.
Tools and libraries to perform hyperparameter tuning.
- Advisor - Open-source implementation of Google Vizier for hyper parameters tuning.
- Hyperas - A very simple wrapper for convenient hyperparameter optimization.
- Hyperopt - Distributed Asynchronous Hyperparameter Optimization in Python.
- Katib - Kubernetes-based system for hyperparameter tuning and neural architecture search.
- KerasTuner - Easy-to-use, scalable hyperparameter optimization framework.
- Optuna - Open source hyperparameter optimization framework to automate hyperparameter search.
- Scikit Optimize - Simple and efficient library to minimize expensive and noisy black-box functions.
- Talos - Hyperparameter Optimization for TensorFlow, Keras and PyTorch.
- Tune - Python library for experiment execution and hyperparameter tuning at any scale.
Tools for sharing knowledge to the entire team/company.
- Knowledge Repo - Knowledge sharing platform for data scientists and other technical professions.
- Kyso - One place for data insights so your entire team can learn from your data.
Complete machine learning platform solutions.
- aiWARE - aiWARE helps MLOps teams evaluate, deploy, integrate, scale & monitor ML models.
- Algorithmia - Securely govern your machine learning operations with a healthy ML lifecycle.
- Allegro AI - Transform ML/DL research into products. Faster.
- Bodywork - Deploys machine learning projects developed in Python, to Kubernetes.
- CNVRG - An end-to-end machine learning platform to build and deploy AI models at scale.
- DAGsHub - A platform built on open source tools for data, model and pipeline management.
- Dataiku - Platform democratizing access to data and enabling enterprises to build their own path to AI.
- DataRobot - AI platform that democratizes data science and automates the end-to-end ML at scale.
- Domino - One place for your data science tools, apps, results, models, and knowledge.
- Edge Impulse - Platform for creating, optimizing, and deploying AI/ML algorithms for edge devices.
- envd - Machine learning development environment for data science and AI/ML engineering teams.
- FedML - Simplifies the workflow of federated learning anywhere at any scale.
- Gradient - Multicloud CI/CD and MLOps platform for machine learning teams.
- H2O - Open source leader in AI with a mission to democratize AI for everyone.
- Hopsworks - Open-source platform for developing and operating machine learning models at scale.
- Iguazio - Data science platform that automates MLOps with end-to-end machine learning pipelines.
- Katonic - Automate your cycle of intelligence with Katonic MLOps Platform.
- Knime - Create and productionize data science using one easy and intuitive environment.
- Kubeflow - Making deployments of ML workflows on Kubernetes simple, portable and scalable.
- LynxKite - A complete graph data science platform for very large graphs and other datasets.
- ML Workspace - All-in-one web-based IDE specialized for machine learning and data science.
- MLReef - Open source MLOps platform that helps you collaborate, reproduce and share your ML work.
- Modzy - Deploy, connect, run, and monitor machine learning (ML) models in the enterprise and at the edge.
- Neu.ro - MLOps platform that integrates open-source and proprietary tools into client-oriented systems.
- Omnimizer - Simplifies and accelerates MLOps by bridging the gap between ML models and edge hardware.
- Pachyderm - Combines data lineage with end-to-end pipelines on Kubernetes, engineered for the enterprise.
- Polyaxon - A platform for reproducible and scalable machine learning and deep learning on kubernetes.
- Sagemaker - Fully managed service that provides the ability to build, train, and deploy ML models quickly.
- SAS Viya - Cloud native AI, analytic and data management platform that supports the analytics life cycle.
- Sematic - An open-source end-to-end pipelining tool to go from laptop prototype to cloud in no time.
- SigOpt - A platform that makes it easy to track runs, visualize training, and scale hyperparameter tuning.
- TrueFoundry - A Cloud-native MLOps Platform over Kubernetes to simplify training and serving of ML Models.
- Valohai - Takes you from POC to production while managing the whole model lifecycle.
Tools for performing model fairness and privacy in production.
- AIF360 - A comprehensive set of fairness metrics for datasets and machine learning models.
- Fairlearn - A Python package to assess and improve fairness of machine learning models.
- Opacus - A library that enables training PyTorch models with differential privacy.
- TensorFlow Privacy - Library for training machine learning models with privacy for training data.
Tools for performing model interpretability/explainability.
- Alibi - Open-source Python library enabling ML model inspection and interpretation.
- Captum - Model interpretability and understanding library for PyTorch.
- ELI5 - Python package which helps to debug machine learning classifiers and explain their predictions.
- InterpretML - A toolkit to help understand models and enable responsible machine learning.
- LIME - Explaining the predictions of any machine learning classifier.
- Lucid - Collection of infrastructure and tools for research in neural network interpretability.
- SAGE - For calculating global feature importance using Shapley values.
- SHAP - A game theoretic approach to explain the output of any machine learning model.
Tools for managing model lifecycle (tracking experiments, parameters and metrics).
- Aim - A super-easy way to record, search and compare 1000s of ML training runs.
- Cascade - Library of ML-Engineering tools for rapid prototyping and experiment management.
- Comet - Track your datasets, code changes, experimentation history, and models.
- Guild AI - Open source experiment tracking, pipeline automation, and hyperparameter tuning.
- Keepsake - Version control for machine learning with support to Amazon S3 and Google Cloud Storage.
- Losswise - Makes it easy to track the progress of a machine learning project.
- Mlflow - Open source platform for the machine learning lifecycle.
- ModelDB - Open source ML model versioning, metadata, and experiment management.
- Neptune AI - The most lightweight experiment management tool that fits any workflow.
- Sacred - A tool to help you configure, organize, log and reproduce experiments.
- Weights and Biases - A tool for visualizing and tracking your machine learning experiments.
Tools for serving models in production.
- Banana - Host your ML inference code on serverless GPUs and integrate it into your app with one line of code.
- Beam - Develop on serverless GPUs, deploy highly performant APIs, and rapidly prototype ML models.
- BentoML - Open-source platform for high-performance ML model serving.
- BudgetML - Deploy a ML inference service on a budget in less than 10 lines of code.
- Cog - Open-source tool that lets you package ML models in a standard, production-ready container.
- Cortex - Machine learning model serving infrastructure.
- Geniusrise - Host inference APIs, bulk inference and fine tune text, vision, audio and multi-modal models.
- Gradio - Create customizable UI components around your models.
- GraphPipe - Machine learning model deployment made simple.
- Hydrosphere - Platform for deploying your Machine Learning to production.
- KFServing - Kubernetes custom resource definition for serving ML models on arbitrary frameworks.
- LocalAI - Drop-in replacement REST API that’s compatible with OpenAI API specifications for inferencing.
- Merlin - A platform for deploying and serving machine learning models.
- MLEM - Version and deploy your ML models following GitOps principles.
- Opyrator - Turns your ML code into microservices with web API, interactive GUI, and more.
- PredictionIO - Event collection, deployment of algorithms, evaluation, querying predictive results via APIs.
- Quix - Serverless platform for processing data streams in real-time with machine learning models.
- Rune - Provides containers to encapsulate and deploy EdgeML pipelines and applications.
- Seldon - Take your ML projects from POC to production with maximum efficiency and minimal risk.
- Streamlit - Lets you create apps for your ML projects with deceptively simple Python scripts.
- TensorFlow Serving - Flexible, high-performance serving system for ML models, designed for production.
- TorchServe - A flexible and easy to use tool for serving PyTorch models.
- Triton Inference Server - Provides an optimized cloud and edge inferencing solution.
- Vespa - Store, search, organize and make machine-learned inferences over big data at serving time.
Tools for testing and validating models.
- Deepchecks - Open-source package for validating ML models & data, with various checks and suites.
- Starwhale - An MLOps/LLMOps platform for model building, evaluation, and fine-tuning.
- Trubrics - Validate machine learning with data science and domain expert feedback.
Optimization tools related to model scalability in production.
- Accelerate - A simple way to train and use PyTorch models with multi-GPU, TPU, mixed-precision.
- Dask - Provides advanced parallelism for analytics, enabling performance at scale for the tools you love.
- DeepSpeed - Deep learning optimization library that makes distributed training easy, efficient, and effective.
- Fiber - Python distributed computing library for modern computer clusters.
- Horovod - Distributed deep learning training framework for TensorFlow, Keras, PyTorch, and Apache MXNet.
- Mahout - Distributed linear algebra framework and mathematically expressive Scala DSL.
- MLlib - Apache Spark's scalable machine learning library.
- Modin - Speed up your Pandas workflows by changing a single line of code.
- Nebullvm - Easy-to-use library to boost AI inference.
- Nos - Open-source module for running AI workloads on Kubernetes in an optimized way.
- Petastorm - Enables single machine or distributed training and evaluation of deep learning models.
- Rapids - Gives the ability to execute end-to-end data science and analytics pipelines entirely on GPUs.
- Ray - Fast and simple framework for building and running distributed applications.
- Singa - Apache top level project, focusing on distributed training of DL and ML models.
- Tpot - Automated ML tool that optimizes machine learning pipelines using genetic programming.
Tools related to machine learning simplification and standardization.
- Chassis - Turns models into ML-friendly containers that run just about anywhere.
- Hermione - Help Data Scientists on setting up more organized codes, in a quicker and simpler way.
- Hydra - A framework for elegantly configuring complex applications.
- Koalas - Pandas API on Apache Spark. Makes data scientists more productive when interacting with big data.
- Ludwig - Allows users to train and test deep learning models without the need to write code.
- MLNotify - No need to keep checking your training, just one import line and you'll know the second it's done.
- PyCaret - Open source, low-code machine learning library in Python.
- Sagify - A CLI utility to train and deploy ML/DL models on AWS SageMaker.
- Soopervisor - Export ML projects to Kubernetes (Argo workflows), Airflow, AWS Batch, and SLURM.
- Soorgeon - Convert monolithic Jupyter notebooks into maintainable pipelines.
- TrainGenerator - A web app to generate template code for machine learning.
- Turi Create - Simplifies the development of custom machine learning models.
Tools for performing visual analysis and debugging of ML/DL models.
- Aporia - Observability with customized monitoring and explainability for ML models.
- Arize - A free end-to-end ML observability and model monitoring platform.
- CometLLM - Track, visualize, and evaluate your LLM prompts and chains in one easy-to-use UI.
- Evidently - Interactive reports to analyze ML models during validation or production monitoring.
- Fiddler - Monitor, explain, and analyze your AI in production.
- Manifold - A model-agnostic visual debugging tool for machine learning.
- NannyML - Algorithm capable of fully capturing the impact of data drift on performance.
- Netron - Visualizer for neural network, deep learning, and machine learning models.
- Phoenix - MLOps in a Notebook for troubleshooting and fine-tuning generative LLM, CV, and tabular models.
- Superwise - Fully automated, enterprise-grade model observability in a self-service SaaS platform.
- Whylogs - The open source standard for data logging. Enables ML monitoring and observability.
- Yellowbrick - Visual analysis and diagnostic tools to facilitate machine learning model selection.
Tools and frameworks to create workflows or pipelines in the machine learning context.
- Argo - Open source container-native workflow engine for orchestrating parallel jobs on Kubernetes.
- Automate Studio - Rapidly build & deploy AI-powered workflows.
- Couler - Unified interface for constructing and managing workflows on different workflow engines.
- dstack - An open-core tool to automate data and training workflows.
- Flyte - Easy to create concurrent, scalable, and maintainable workflows for machine learning.
- Hamilton - A scalable general purpose micro-framework for defining dataflows.
- Kale - Aims at simplifying the Data Science experience of deploying Kubeflow Pipelines workflows.
- Kedro - Library that implements software engineering best-practice for data and ML pipelines.
- Luigi - Python module that helps you build complex pipelines of batch jobs.
- Metaflow - Human-friendly lib that helps scientists and engineers build and manage data science projects.
- MLRun - Generic mechanism for data scientists to build, run, and monitor ML tasks and pipelines.
- Orchest - Visual pipeline editor and workflow orchestrator with an easy to use UI and based on Kubernetes.
- Ploomber - Write maintainable, production-ready pipelines. Develop locally, deploy to the cloud.
- Prefect - A workflow management system, designed for modern infrastructure.
- VDP - An open-source tool to seamlessly integrate AI for unstructured data into the modern data stack.
- ZenML - An extensible open-source MLOps framework to create reproducible pipelines.
Where to discover new tools and discuss about existing ones.
- A Tour of End-to-End Machine Learning Platforms (Databaseline)
- Continuous Delivery for Machine Learning (Martin Fowler)
- Delivering on the Vision of MLOps: A maturity-based approach (GigaOm)
- Machine Learning Operations (MLOps): Overview, Definition, and Architecture (arXiv)
- MLOps: Continuous delivery and automation pipelines in machine learning (Google)
- MLOps: Machine Learning as an Engineering Discipline (Medium)
- Rules of Machine Learning: Best Practices for ML Engineering (Google)
- The ML Test Score: A Rubric for ML Production Readiness and Technical Debt Reduction (Google)
- What Is MLOps? (NVIDIA)
- Beginning MLOps with MLFlow (Apress)
- Building Machine Learning Pipelines (O'Reilly)
- Building Machine Learning Powered Applications (O'Reilly)
- Deep Learning in Production (AI Summer)
- Designing Machine Learning Systems (O'Reilly)
- Engineering MLOps (Packt)
- Implementing MLOps in the Enterprise (O'Reilly)
- Introducing MLOps (O'Reilly)
- Kubeflow for Machine Learning (O'Reilly)
- Kubeflow Operations Guide (O'Reilly)
- Machine Learning Design Patterns (O'Reilly)
- Machine Learning Engineering in Action (Manning)
- ML Ops: Operationalizing Data Science (O'Reilly)
- MLOps Engineering at Scale (Manning)
- MLOps Lifecycle Toolkit (Apress)
- Practical Deep Learning at Scale with MLflow (Packt)
- Practical MLOps (O'Reilly)
- Production-Ready Applied Deep Learning (Packt)
- Reliable Machine Learning (O'Reilly)
- The Machine Learning Solutions Architect Handbook (Packt)
- apply() - The ML data engineering conference
- MLOps Conference - Keynotes and Panels
- MLOps World: Machine Learning in Production Conference
- NormConf - The Normcore Tech Conference
- Stanford MLSys Seminar Series
- Applied ML
- Awesome AutoML Papers
- Awesome AutoML
- Awesome Data Science
- Awesome DataOps
- Awesome Deep Learning
- Awesome Game Datasets (includes AI content)
- Awesome Machine Learning
- Awesome MLOps
- Awesome Production Machine Learning
- Awesome Python
- Deep Learning in Production
- How AI Built This
- Kubernetes Podcast from Google
- Machine Learning – Software Engineering Daily
- MLOps.community
- Pipeline Conversation
- Practical AI: Machine Learning, Data Science
- This Week in Machine Learning & AI
- True ML Talks
All contributions are welcome! Please take a look at the contribution guidelines first.
For Tasks:
Click tags to check more tools for each tasksFor Jobs:
Alternative AI tools for awesome-mlops
Similar Open Source Tools
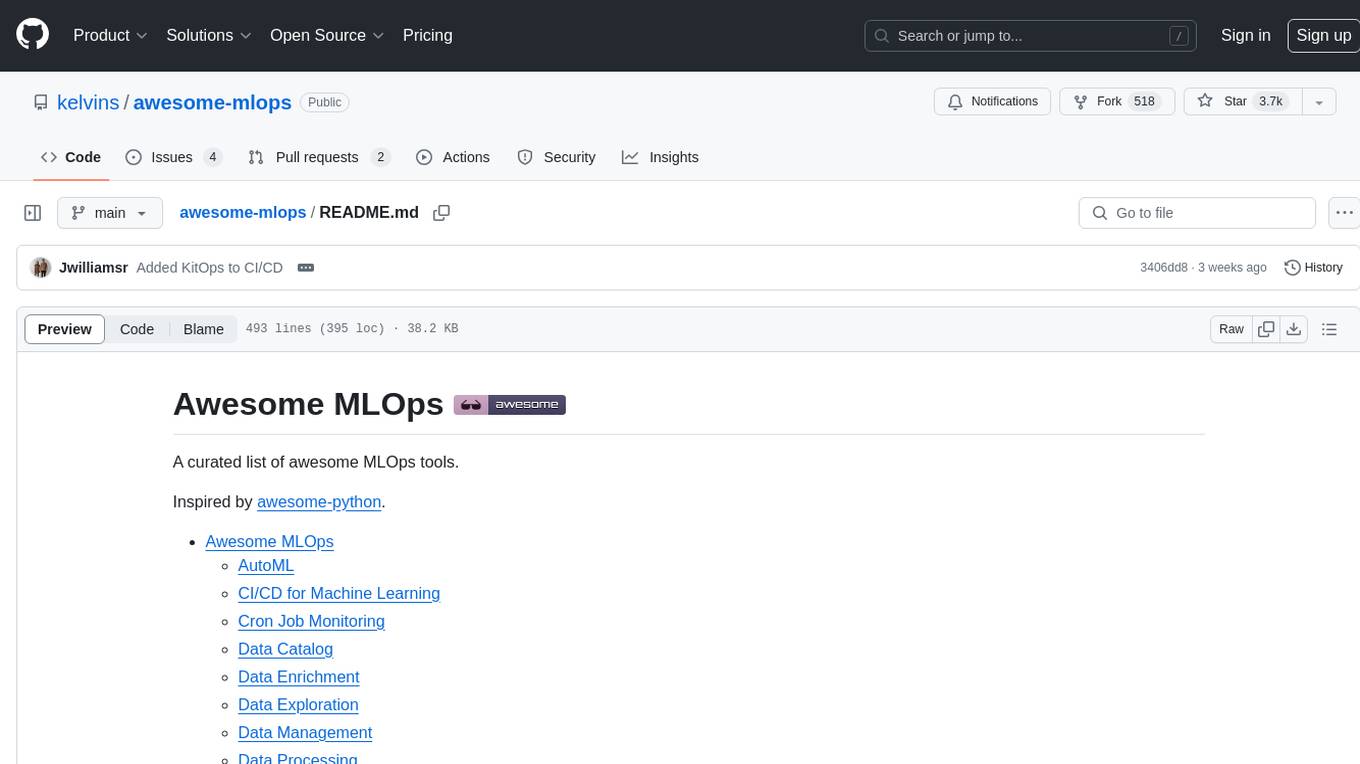
awesome-mlops
Awesome MLOps is a curated list of tools related to Machine Learning Operations, covering areas such as AutoML, CI/CD for Machine Learning, Data Cataloging, Data Enrichment, Data Exploration, Data Management, Data Processing, Data Validation, Data Visualization, Drift Detection, Feature Engineering, Feature Store, Hyperparameter Tuning, Knowledge Sharing, Machine Learning Platforms, Model Fairness and Privacy, Model Interpretability, Model Lifecycle, Model Serving, Model Testing & Validation, Optimization Tools, Simplification Tools, Visual Analysis and Debugging, and Workflow Tools. The repository provides a comprehensive collection of tools and resources for individuals and teams working in the field of MLOps.
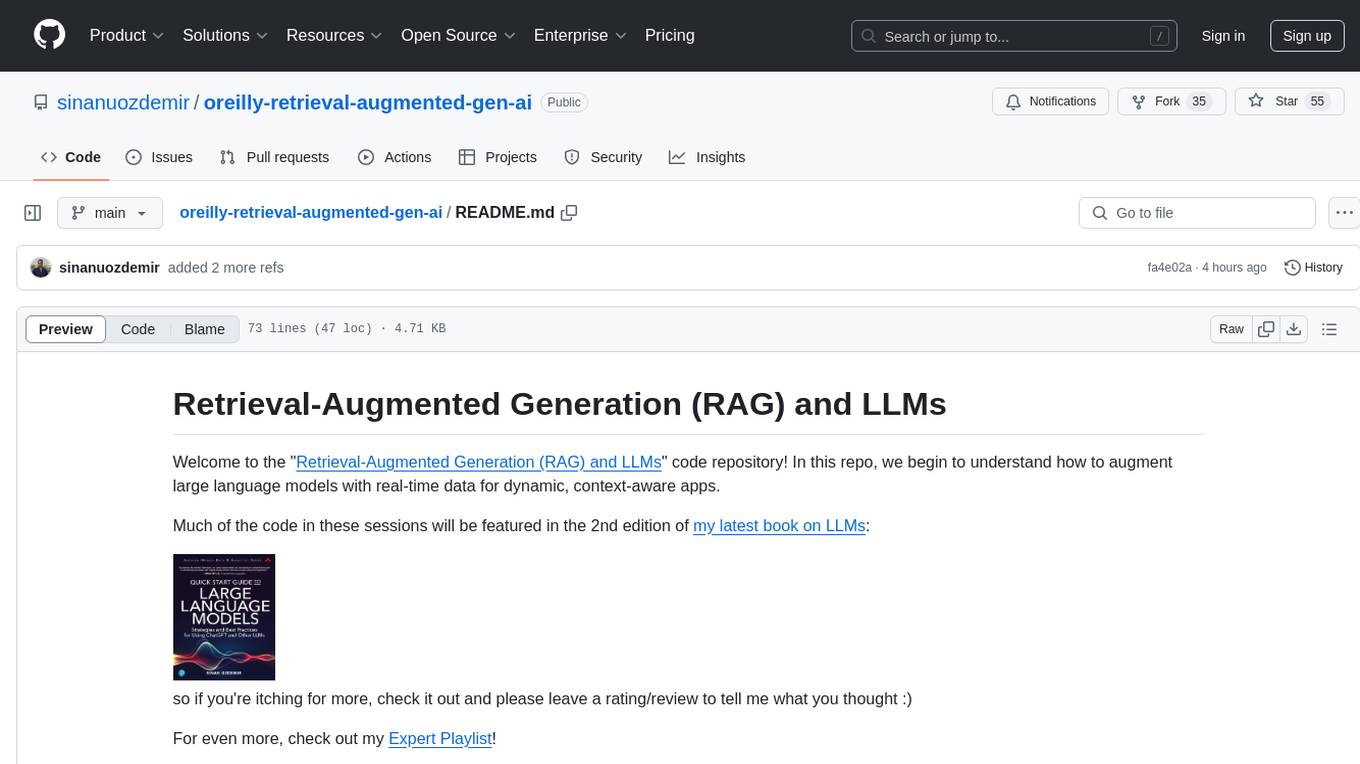
oreilly-retrieval-augmented-gen-ai
This repository focuses on Retrieval-Augmented Generation (RAG) and Large Language Models (LLMs). It provides code and resources to augment LLMs with real-time data for dynamic, context-aware applications. The content covers topics such as semantic search, fine-tuning embeddings, building RAG chatbots, evaluating LLMs, and using knowledge graphs in RAG. Prerequisites include Python skills, knowledge of machine learning and LLMs, and introductory experience with NLP and AI models.
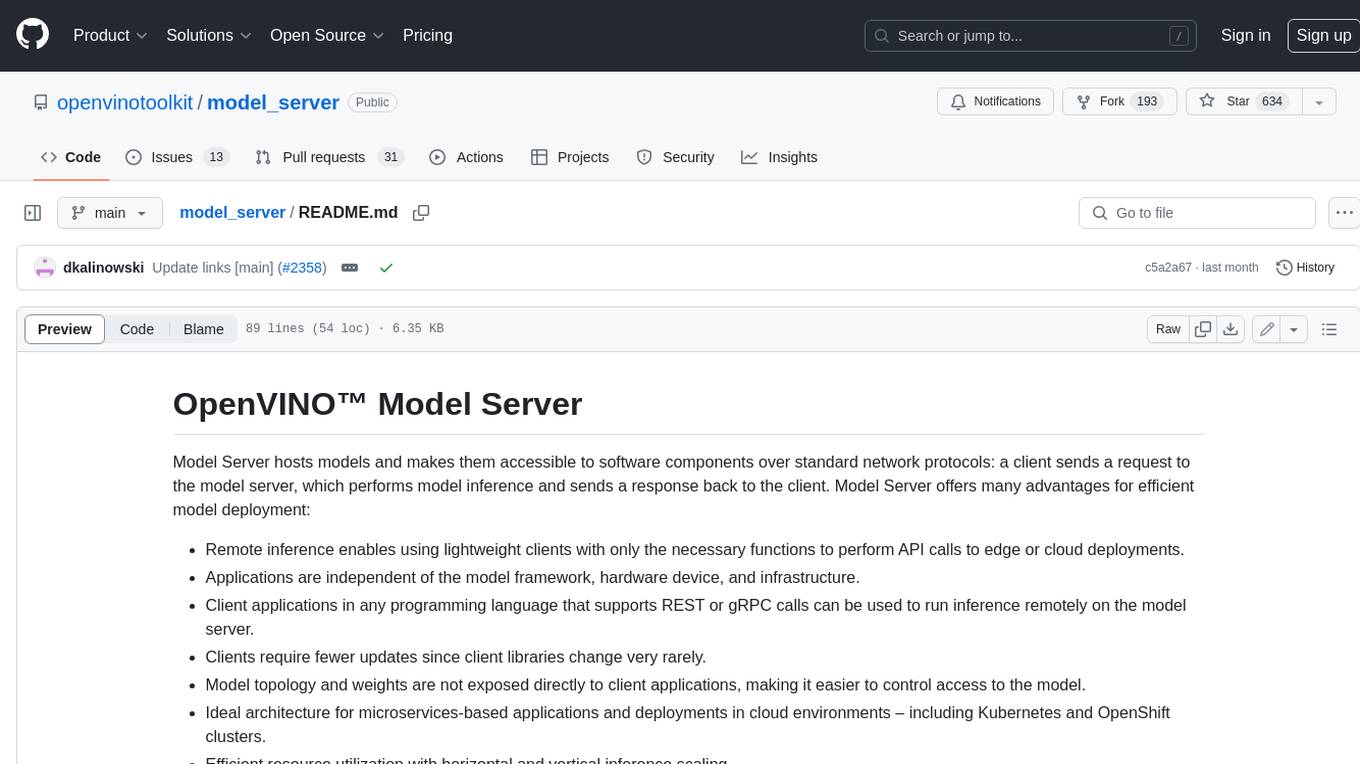
model_server
OpenVINO™ Model Server (OVMS) is a high-performance system for serving models. Implemented in C++ for scalability and optimized for deployment on Intel architectures, the model server uses the same architecture and API as TensorFlow Serving and KServe while applying OpenVINO for inference execution. Inference service is provided via gRPC or REST API, making deploying new algorithms and AI experiments easy.
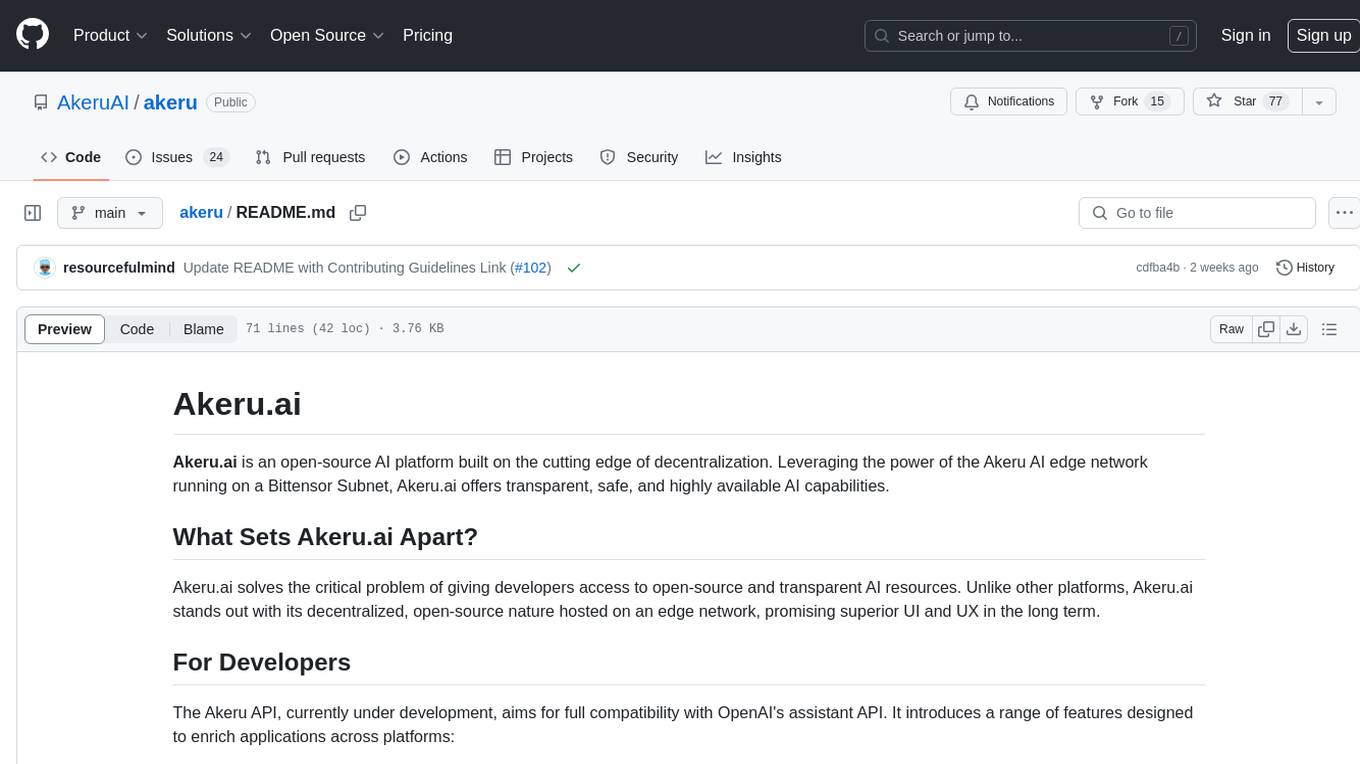
akeru
Akeru.ai is an open-source AI platform leveraging the power of decentralization. It offers transparent, safe, and highly available AI capabilities. The platform aims to give developers access to open-source and transparent AI resources through its decentralized nature hosted on an edge network. Akeru API introduces features like retrieval, function calling, conversation management, custom instructions, data input optimization, user privacy, testing and iteration, and comprehensive documentation. It is ideal for creating AI agents and enhancing web and mobile applications with advanced AI capabilities. The platform runs on a Bittensor Subnet design that aims to democratize AI technology and promote an equitable AI future. Akeru.ai embraces decentralization challenges to ensure a decentralized and equitable AI ecosystem with security features like watermarking and network pings. The API architecture integrates with technologies like Bun, Redis, and Elysia for a robust, scalable solution.
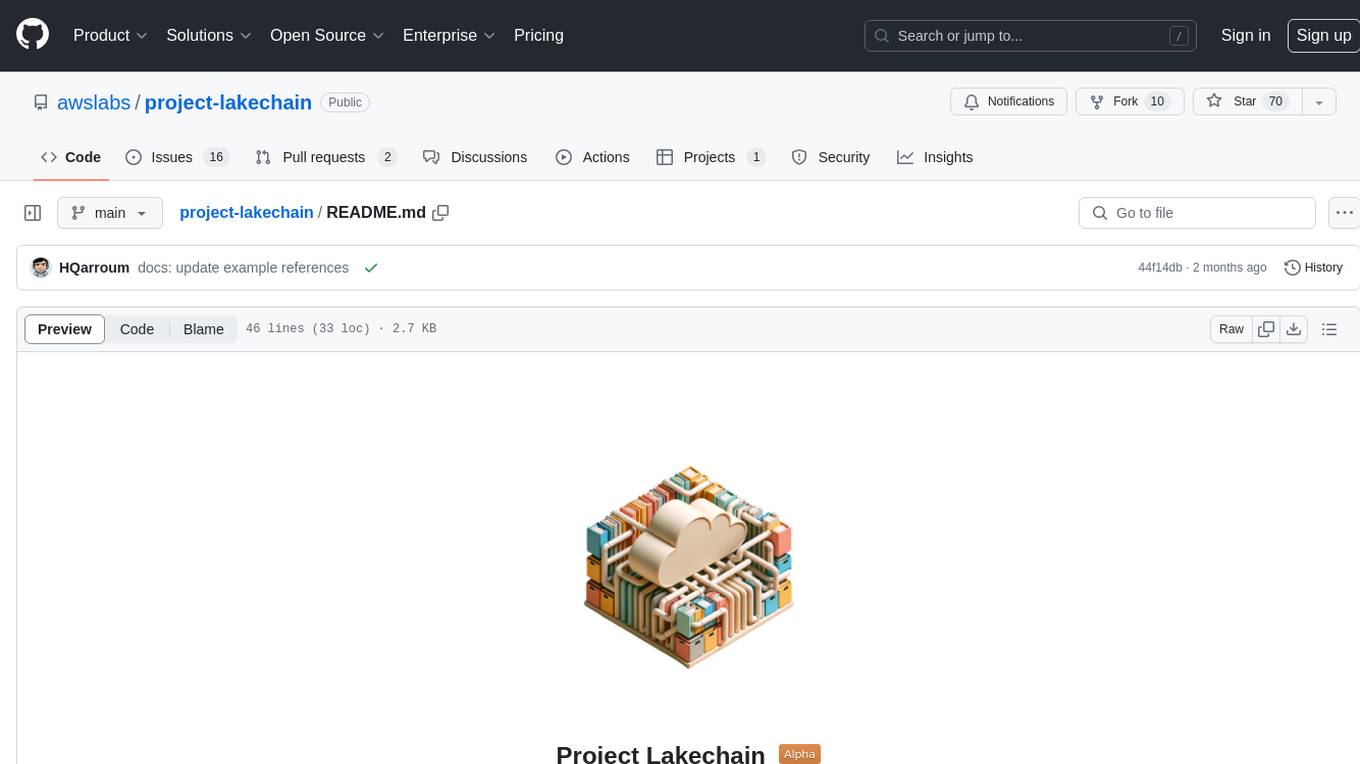
project-lakechain
Project Lakechain is a cloud-native, AI-powered framework for building document processing pipelines on AWS. It provides a composable API with built-in middlewares for common tasks, scalable architecture, cost efficiency, GPU and CPU support, and the ability to create custom transform middlewares. With ready-made examples and emphasis on modularity, Lakechain simplifies the deployment of scalable document pipelines for tasks like metadata extraction, NLP analysis, text summarization, translations, audio transcriptions, computer vision, and more.
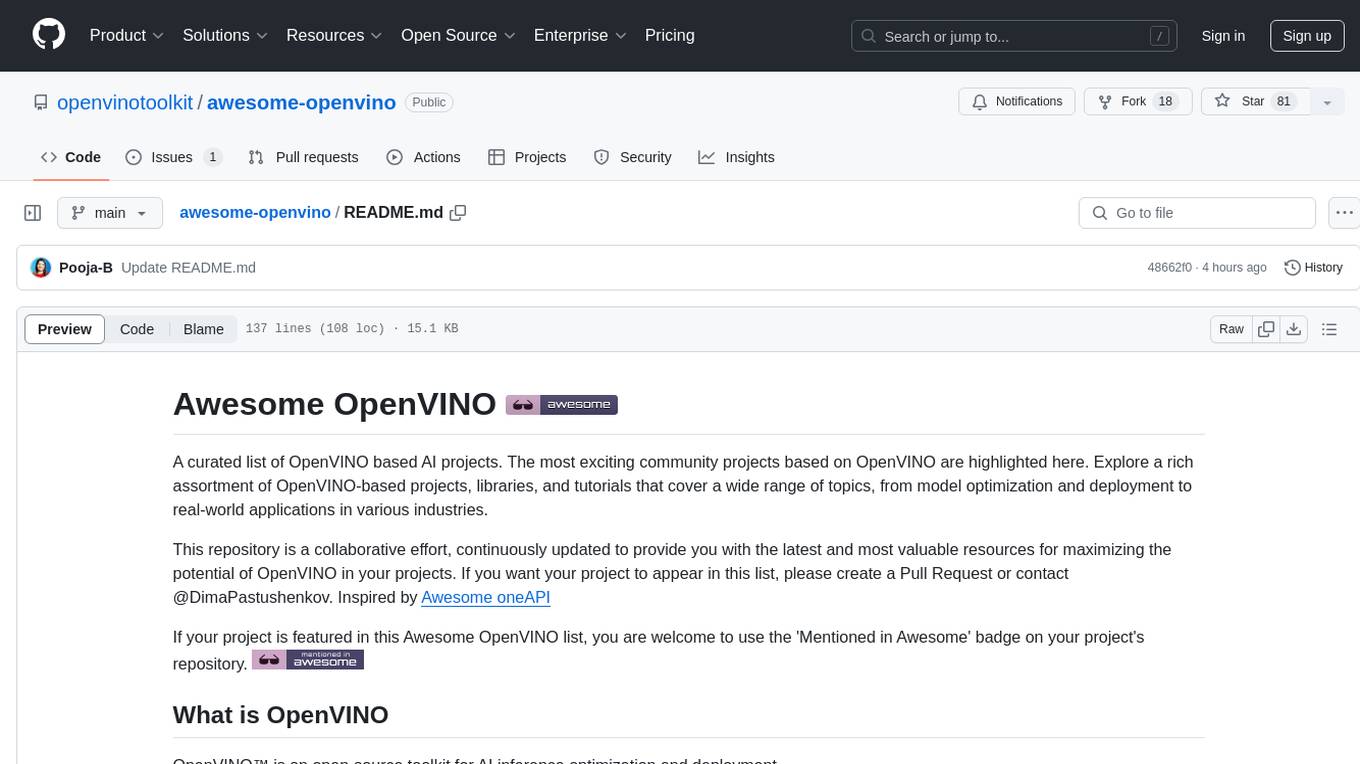
awesome-openvino
Awesome OpenVINO is a curated list of AI projects based on the OpenVINO toolkit, offering a rich assortment of projects, libraries, and tutorials covering various topics like model optimization, deployment, and real-world applications across industries. It serves as a valuable resource continuously updated to maximize the potential of OpenVINO in projects, featuring projects like Stable Diffusion web UI, Visioncom, FastSD CPU, OpenVINO AI Plugins for GIMP, and more.
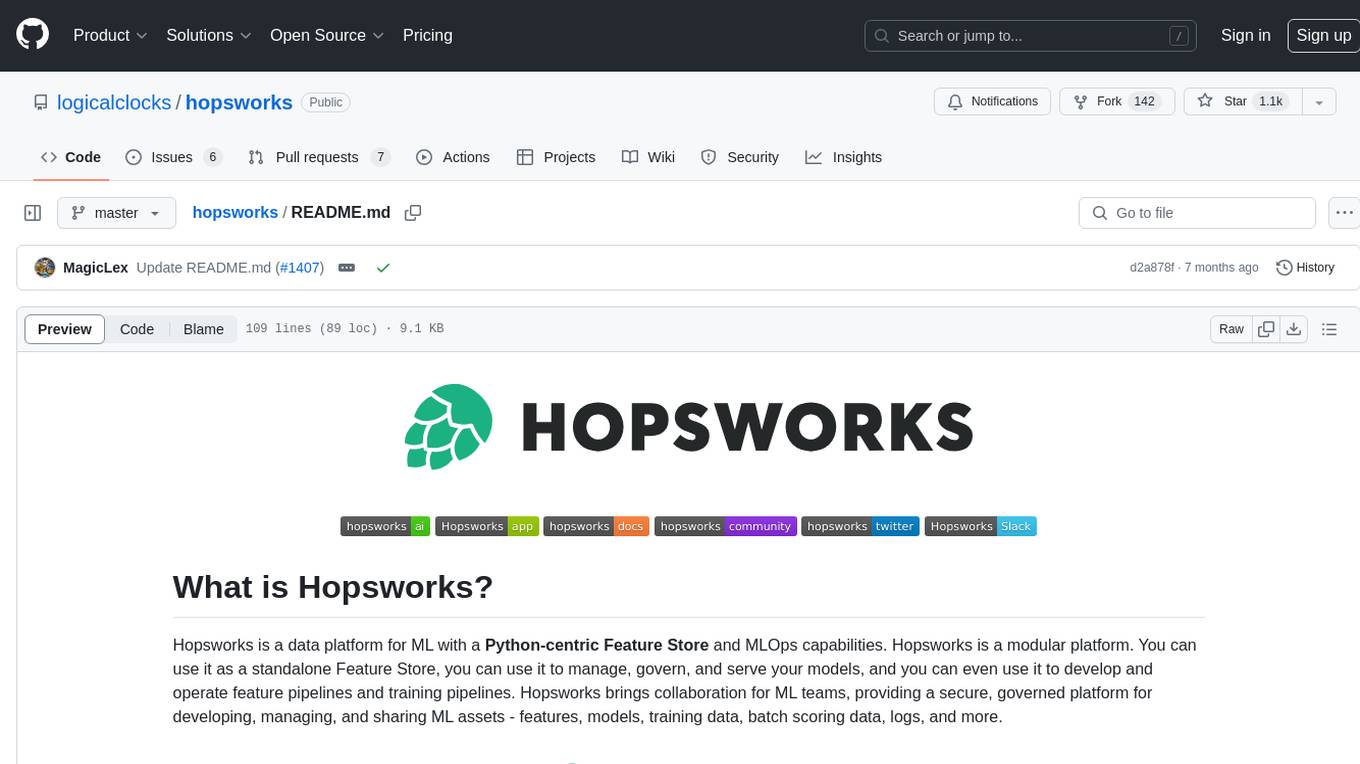
hopsworks
Hopsworks is a data platform for ML with a Python-centric Feature Store and MLOps capabilities. It provides collaboration for ML teams, offering a secure, governed platform for developing, managing, and sharing ML assets. Hopsworks supports project-based multi-tenancy, team collaboration, development tools for Data Science, and is available on any platform including managed cloud services and on-premise installations. The platform enables end-to-end responsibility from raw data to managed features and models, supports versioning, lineage, and provenance, and facilitates the complete MLOps life cycle.
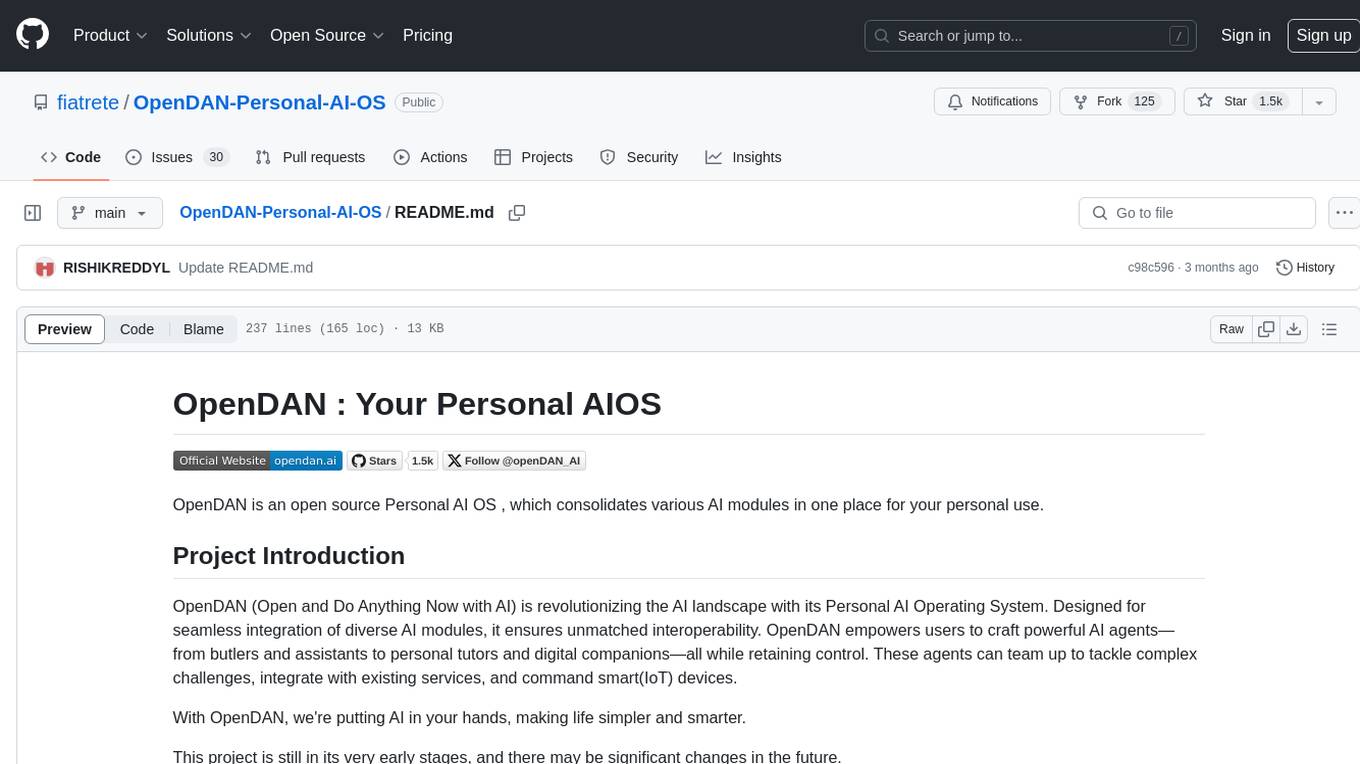
OpenDAN-Personal-AI-OS
OpenDAN is an open source Personal AI OS that consolidates various AI modules for personal use. It empowers users to create powerful AI agents like assistants, tutors, and companions. The OS allows agents to collaborate, integrate with services, and control smart devices. OpenDAN offers features like rapid installation, AI agent customization, connectivity via Telegram/Email, building a local knowledge base, distributed AI computing, and more. It aims to simplify life by putting AI in users' hands. The project is in early stages with ongoing development and future plans for user and kernel mode separation, home IoT device control, and an official OpenDAN SDK release.
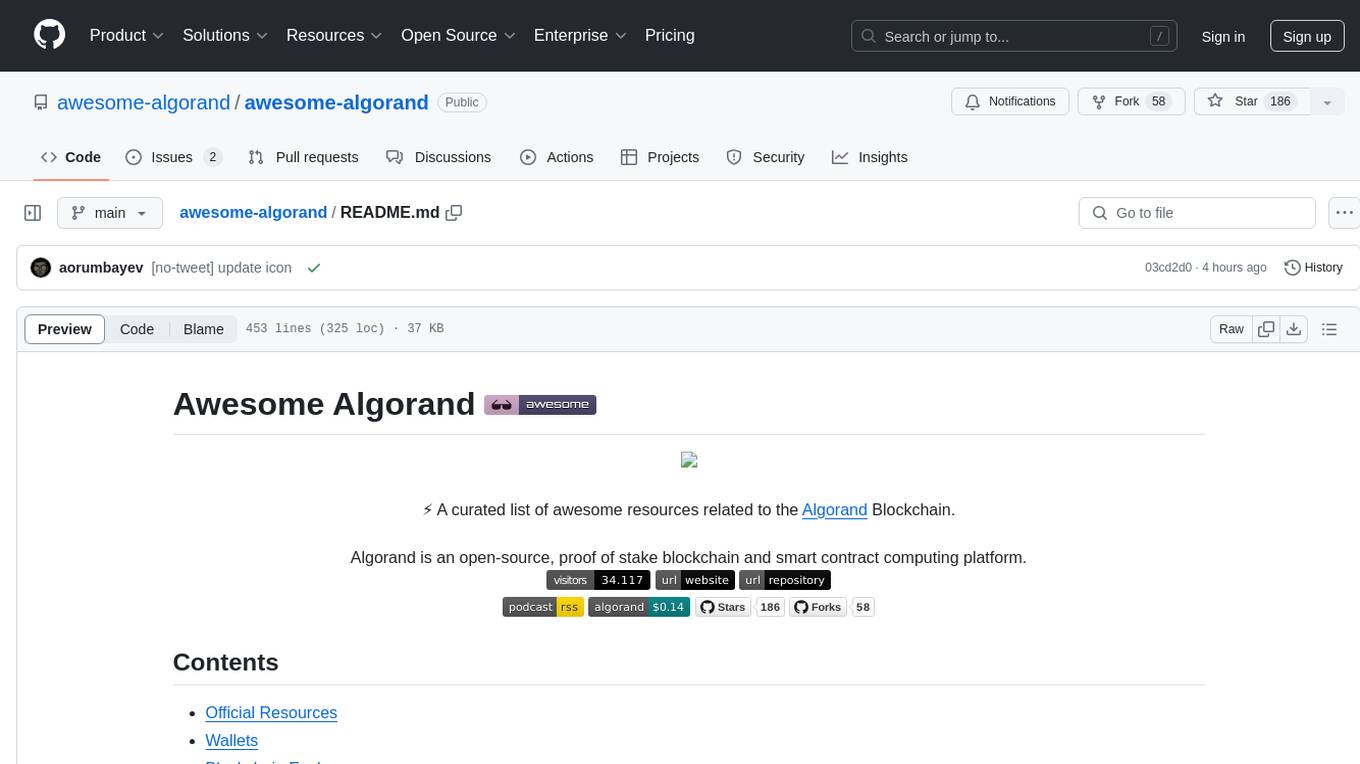
awesome-algorand
Awesome Algorand is a curated list of resources related to the Algorand Blockchain, including official resources, wallets, blockchain explorers, portfolio trackers, learning resources, development tools, DeFi platforms, nodes & consensus participation, subscription management, security auditing services, blockchain bridges, oracles, name services, community resources, Algorand Request for Comments, metrics and analytics services, decentralized voting tools, and NFT marketplaces. The repository provides a comprehensive collection of tools, tutorials, protocols, and platforms for developers, users, and enthusiasts interested in the Algorand ecosystem.
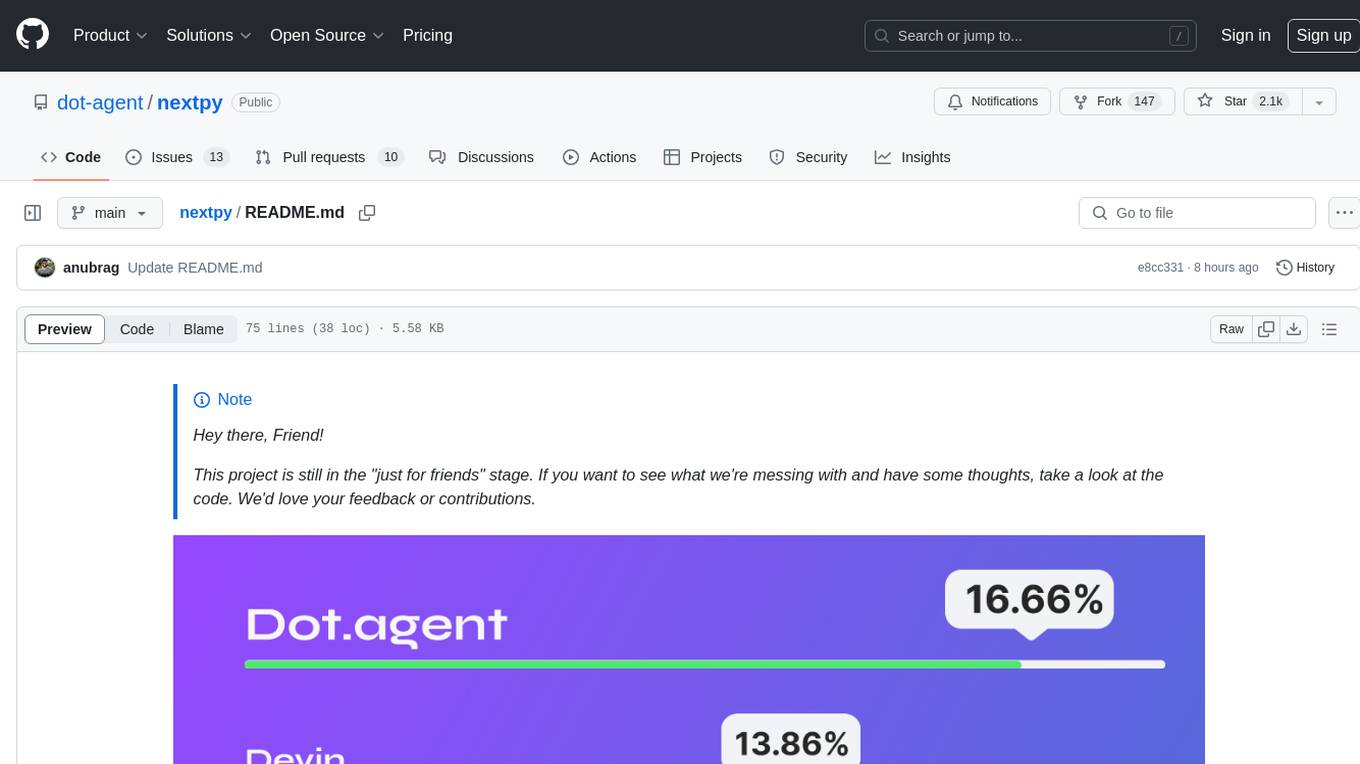
nextpy
Nextpy is a cutting-edge software development framework optimized for AI-based code generation. It provides guardrails for defining AI system boundaries, structured outputs for prompt engineering, a powerful prompt engine for efficient processing, better AI generations with precise output control, modularity for multiplatform and extensible usage, developer-first approach for transferable knowledge, and containerized & scalable deployment options. It offers 4-10x faster performance compared to Streamlit apps, with a focus on cooperation within the open-source community and integration of key components from various projects.
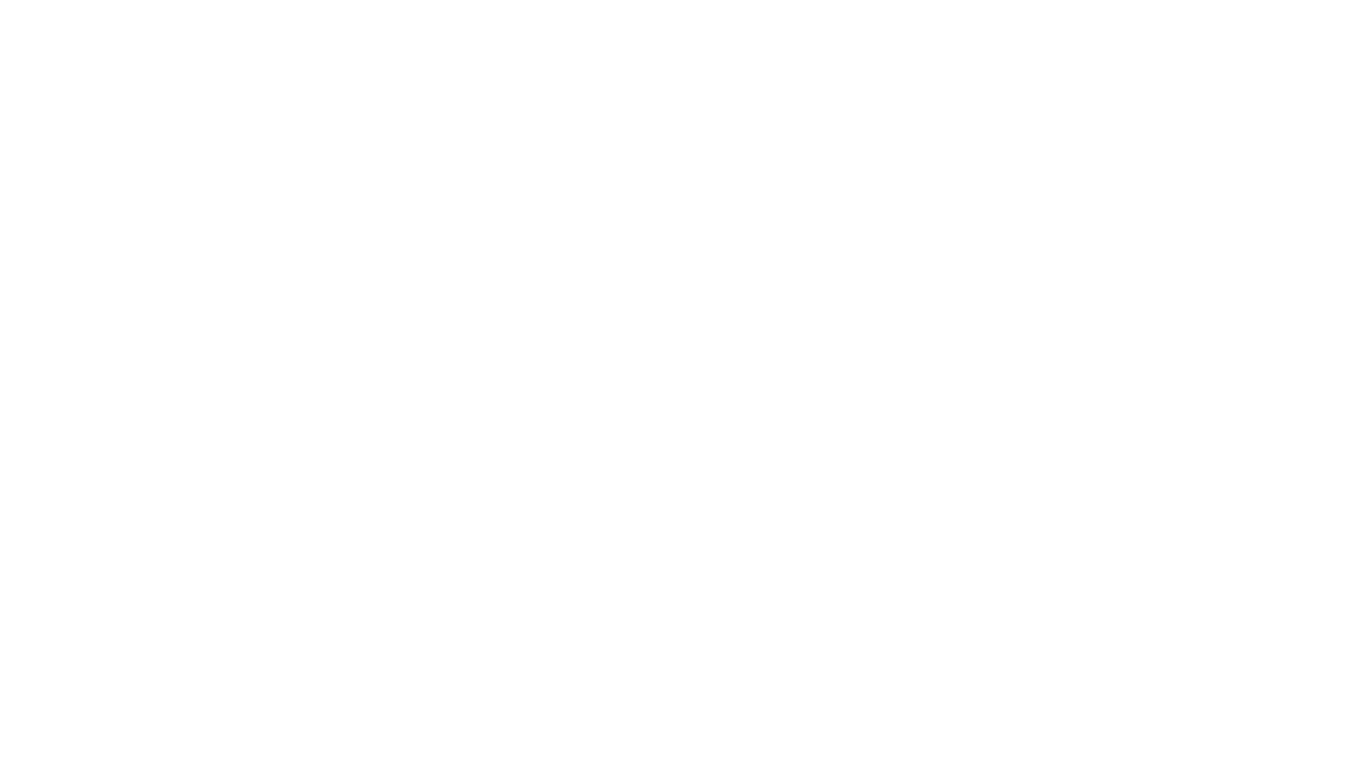
langwatch
LangWatch is a monitoring and analytics platform designed to track, visualize, and analyze interactions with Large Language Models (LLMs). It offers real-time telemetry to optimize LLM cost and latency, a user-friendly interface for deep insights into LLM behavior, user analytics for engagement metrics, detailed debugging capabilities, and guardrails to monitor LLM outputs for issues like PII leaks and toxic language. The platform supports OpenAI and LangChain integrations, simplifying the process of tracing LLM calls and generating API keys for usage. LangWatch also provides documentation for easy integration and self-hosting options for interested users.
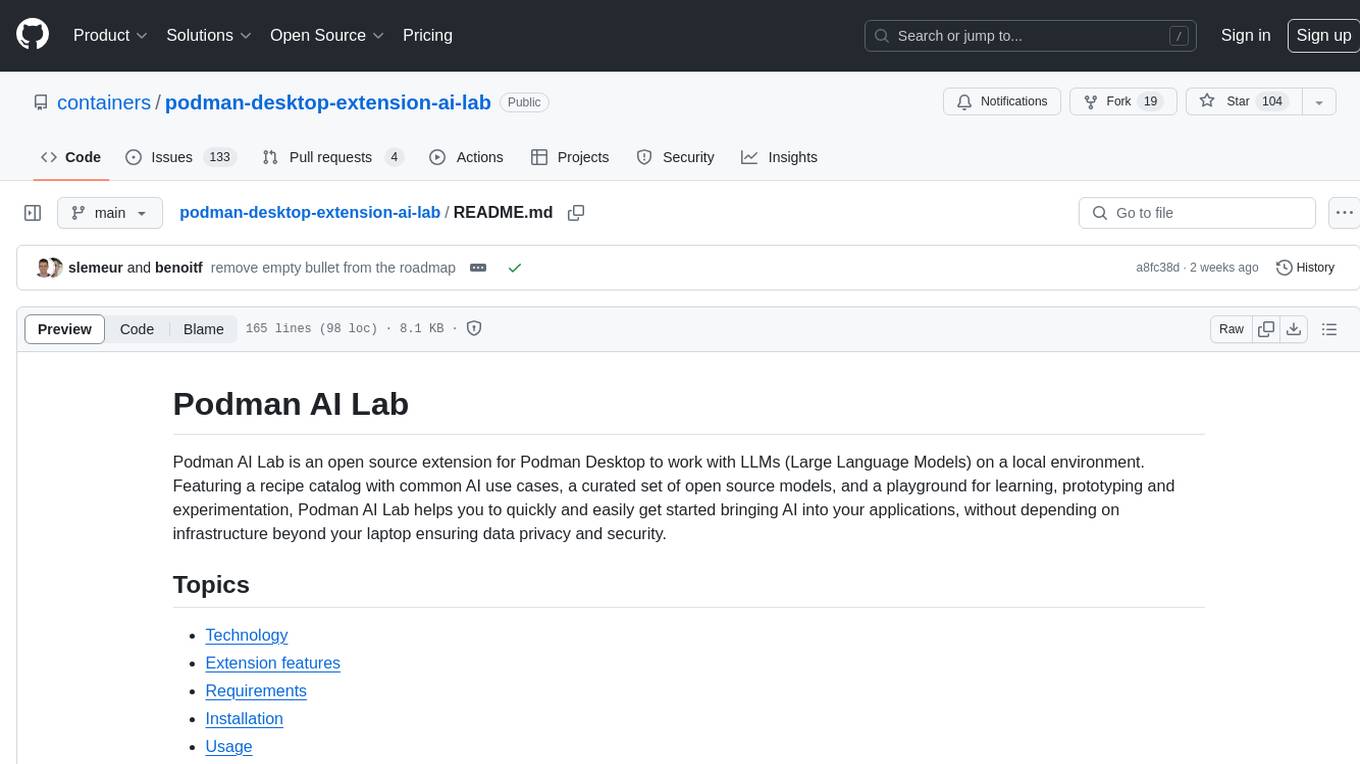
podman-desktop-extension-ai-lab
Podman AI Lab is an open source extension for Podman Desktop designed to work with Large Language Models (LLMs) on a local environment. It features a recipe catalog with common AI use cases, a curated set of open source models, and a playground for learning, prototyping, and experimentation. Users can quickly and easily get started bringing AI into their applications without depending on external infrastructure, ensuring data privacy and security.
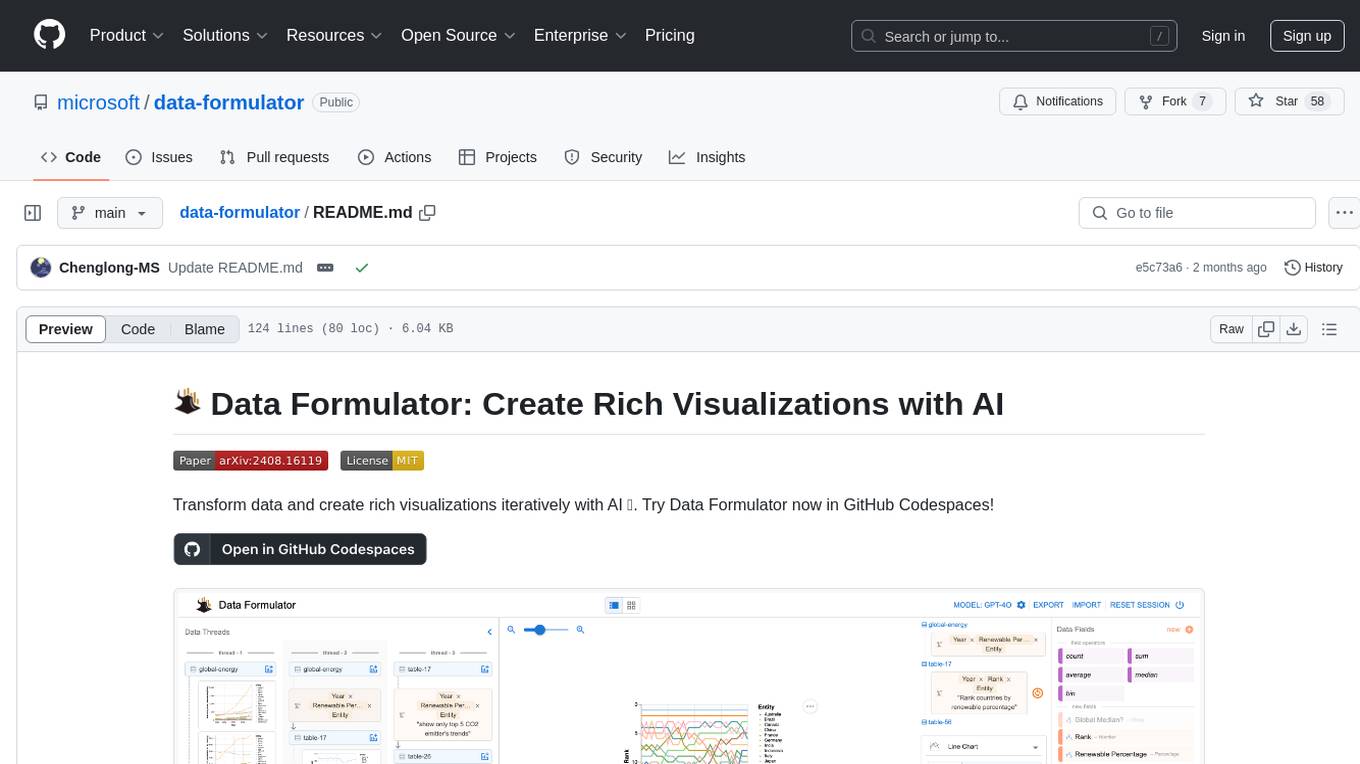
data-formulator
Data Formulator is an AI-powered tool developed by Microsoft Research to help data analysts create rich visualizations iteratively. It combines user interface interactions with natural language inputs to simplify the process of describing chart designs while delegating data transformation to AI. Users can utilize features like blended UI and NL inputs, data threads for history navigation, and code inspection to create impressive visualizations. The tool supports local installation for customization and Codespaces for quick setup. Developers can build new data analysis tools on top of Data Formulator, and research papers are available for further reading.
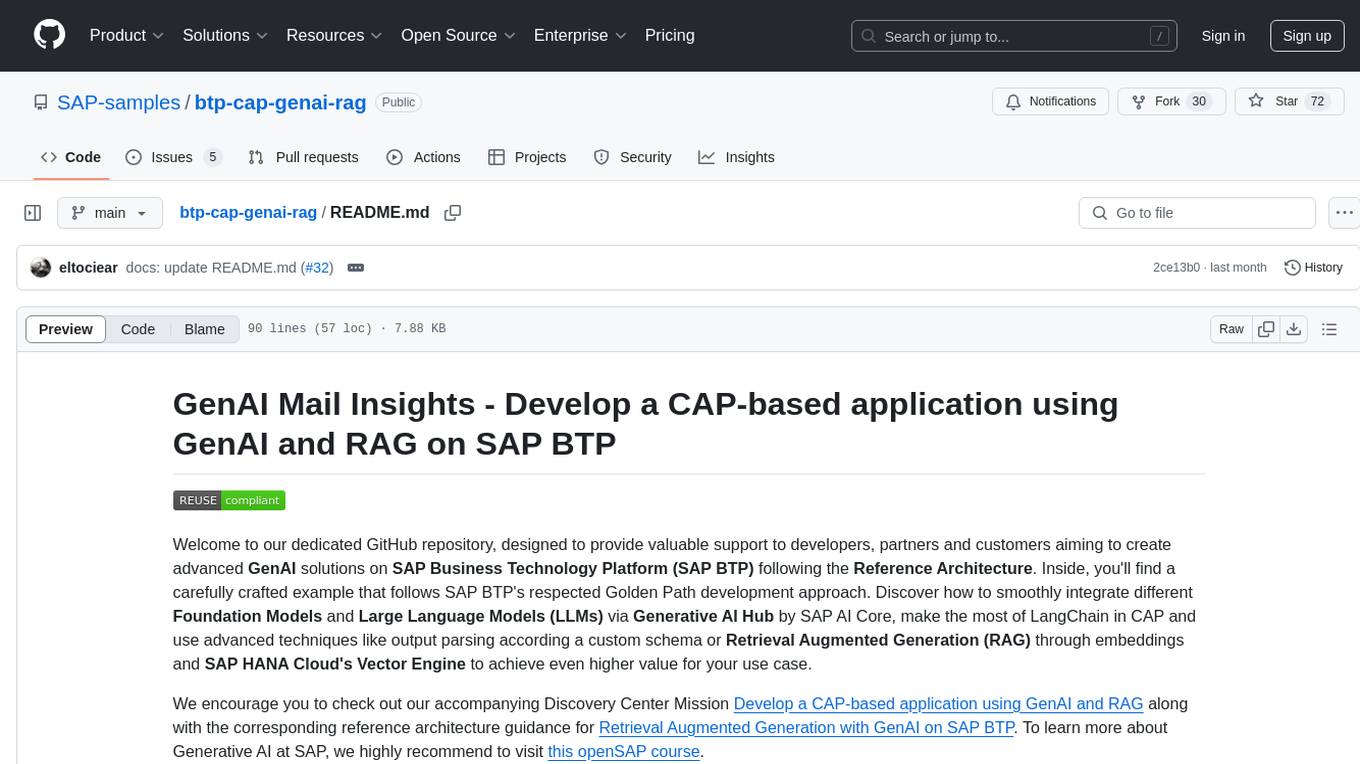
btp-cap-genai-rag
This GitHub repository provides support for developers, partners, and customers to create advanced GenAI solutions on SAP Business Technology Platform (SAP BTP) following the Reference Architecture. It includes examples on integrating Foundation Models and Large Language Models via Generative AI Hub, using LangChain in CAP, and implementing advanced techniques like Retrieval Augmented Generation (RAG) through embeddings and SAP HANA Cloud's Vector Engine for enhanced value in customer support scenarios.
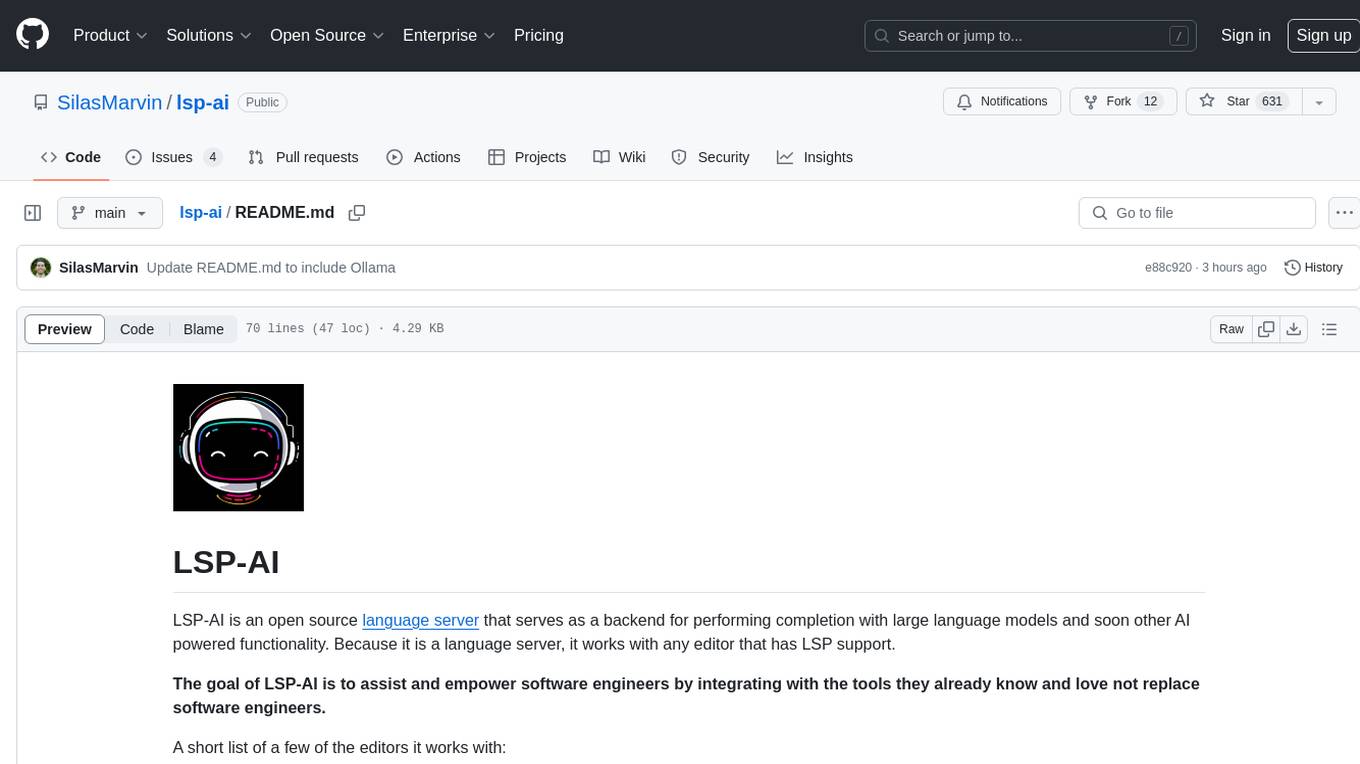
lsp-ai
LSP-AI is an open source language server designed to enhance software engineers' productivity by integrating AI-powered functionality into various text editors. It serves as a backend for completion with large language models and offers features like unified AI capabilities, simplified plugin development, enhanced collaboration, broad compatibility with editors supporting Language Server Protocol, flexible LLM backend support, and commitment to staying updated with the latest advancements in LLM-driven software development. The tool aims to centralize open-source development work, provide a collaborative platform for developers, and offer a future-ready solution for AI-powered assistants in text editors.
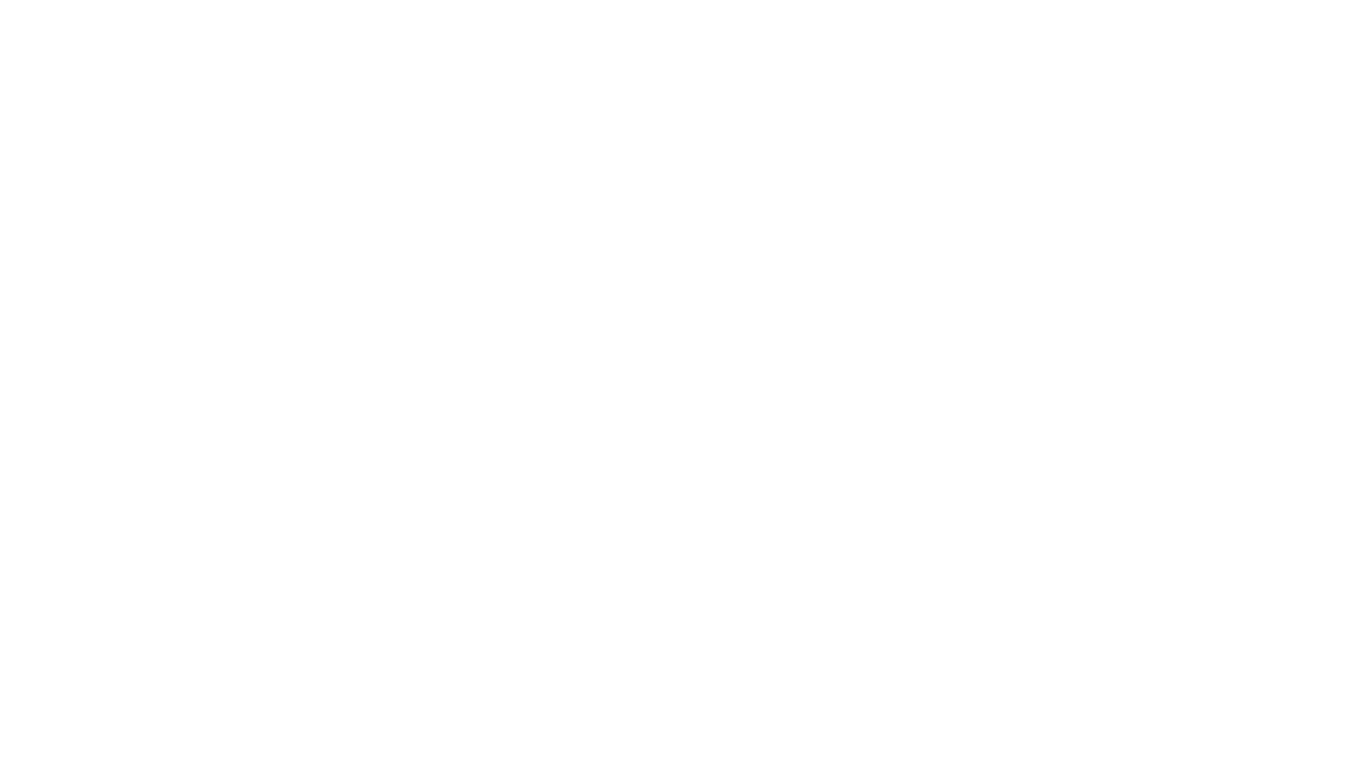
awesome-ai-tools
Awesome AI Tools is a curated list of popular tools and resources for artificial intelligence enthusiasts. It includes a wide range of tools such as machine learning libraries, deep learning frameworks, data visualization tools, and natural language processing resources. Whether you are a beginner or an experienced AI practitioner, this repository aims to provide you with a comprehensive collection of tools to enhance your AI projects and research. Explore the list to discover new tools, stay updated with the latest advancements in AI technology, and find the right resources to support your AI endeavors.
For similar tasks
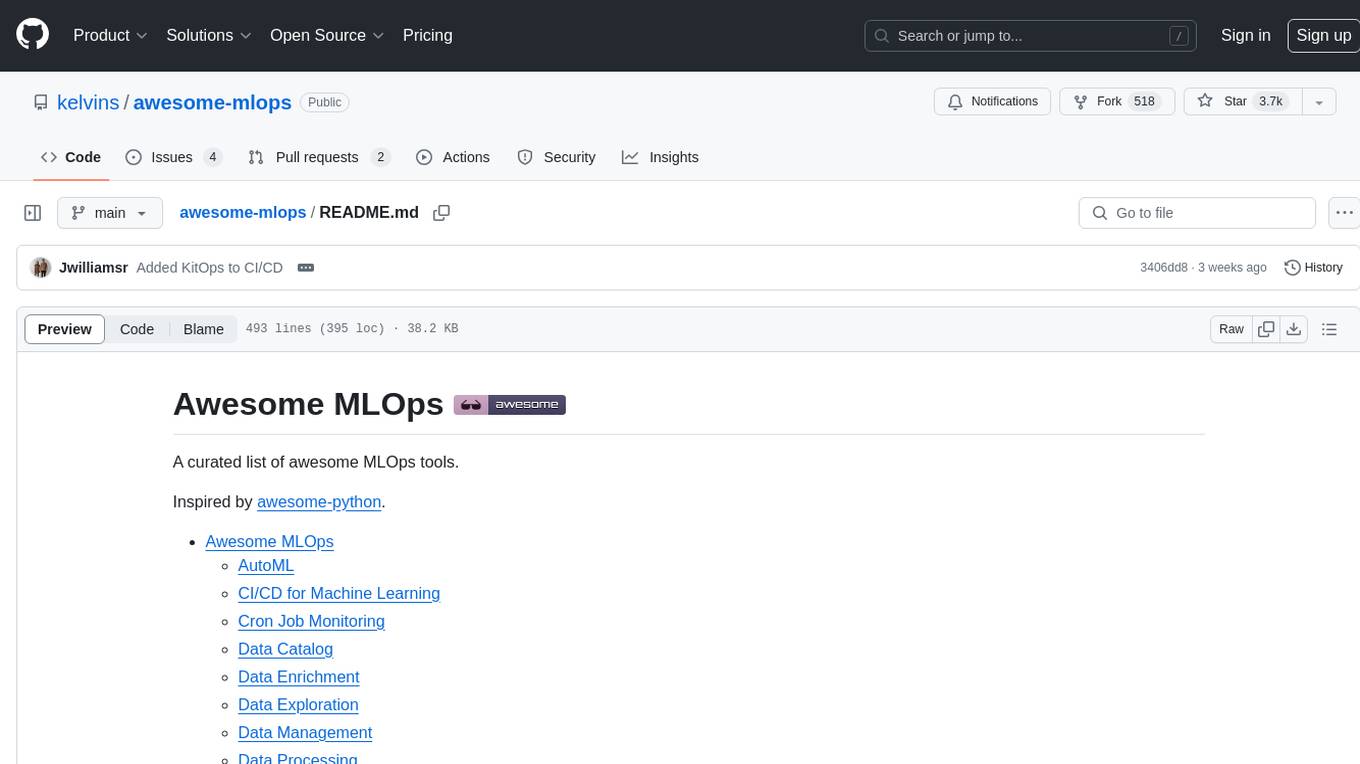
awesome-mlops
Awesome MLOps is a curated list of tools related to Machine Learning Operations, covering areas such as AutoML, CI/CD for Machine Learning, Data Cataloging, Data Enrichment, Data Exploration, Data Management, Data Processing, Data Validation, Data Visualization, Drift Detection, Feature Engineering, Feature Store, Hyperparameter Tuning, Knowledge Sharing, Machine Learning Platforms, Model Fairness and Privacy, Model Interpretability, Model Lifecycle, Model Serving, Model Testing & Validation, Optimization Tools, Simplification Tools, Visual Analysis and Debugging, and Workflow Tools. The repository provides a comprehensive collection of tools and resources for individuals and teams working in the field of MLOps.
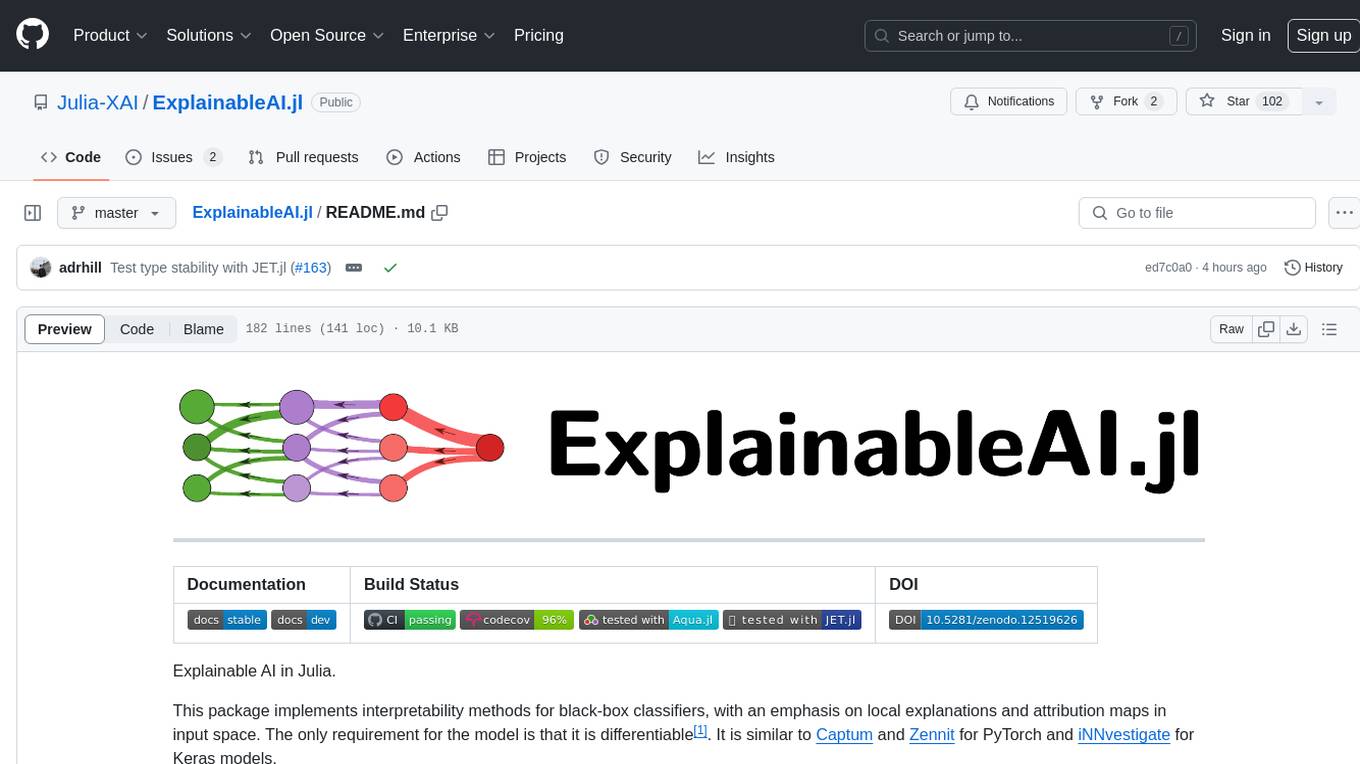
ExplainableAI.jl
ExplainableAI.jl is a Julia package that implements interpretability methods for black-box classifiers, focusing on local explanations and attribution maps in input space. The package requires models to be differentiable with Zygote.jl. It is similar to Captum and Zennit for PyTorch and iNNvestigate for Keras models. Users can analyze and visualize explanations for model predictions, with support for different XAI methods and customization. The package aims to provide transparency and insights into model decision-making processes, making it a valuable tool for understanding and validating machine learning models.
For similar jobs
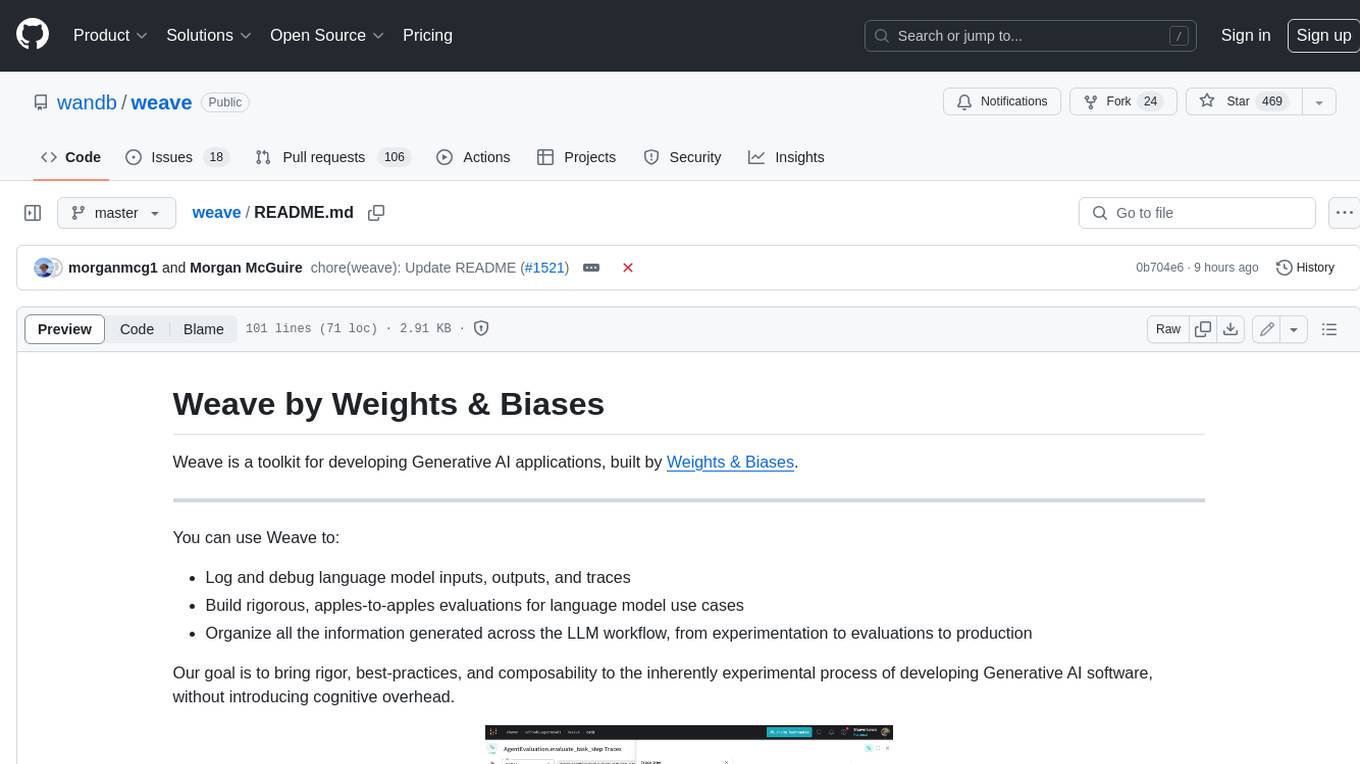
weave
Weave is a toolkit for developing Generative AI applications, built by Weights & Biases. With Weave, you can log and debug language model inputs, outputs, and traces; build rigorous, apples-to-apples evaluations for language model use cases; and organize all the information generated across the LLM workflow, from experimentation to evaluations to production. Weave aims to bring rigor, best-practices, and composability to the inherently experimental process of developing Generative AI software, without introducing cognitive overhead.
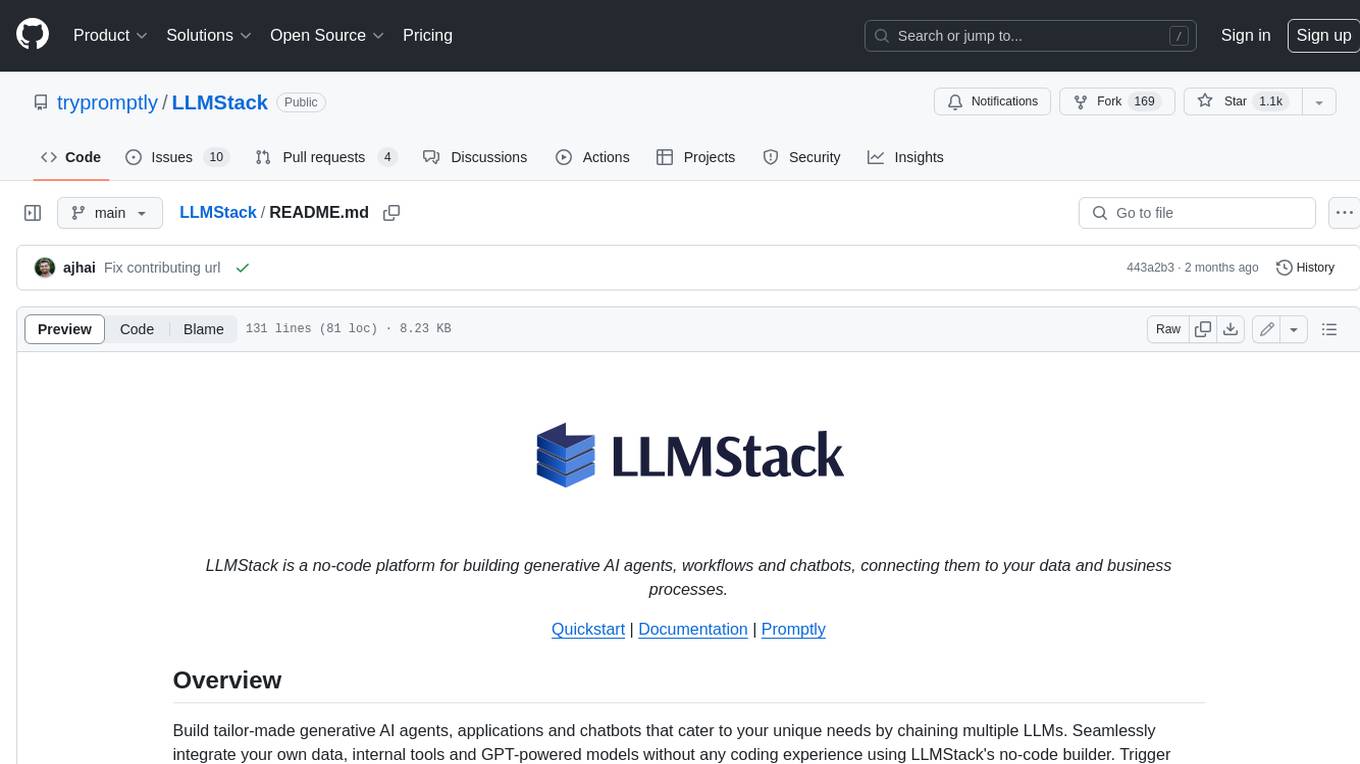
LLMStack
LLMStack is a no-code platform for building generative AI agents, workflows, and chatbots. It allows users to connect their own data, internal tools, and GPT-powered models without any coding experience. LLMStack can be deployed to the cloud or on-premise and can be accessed via HTTP API or triggered from Slack or Discord.
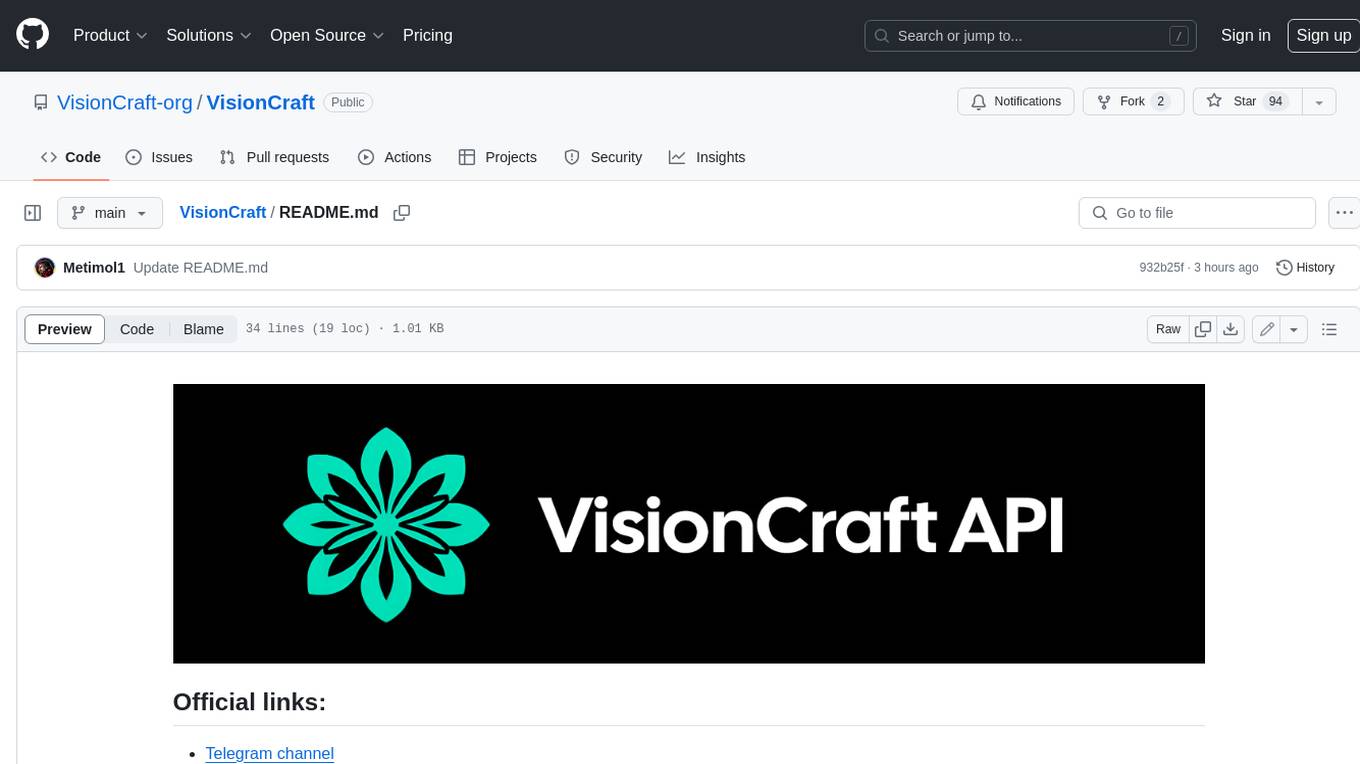
VisionCraft
The VisionCraft API is a free API for using over 100 different AI models. From images to sound.
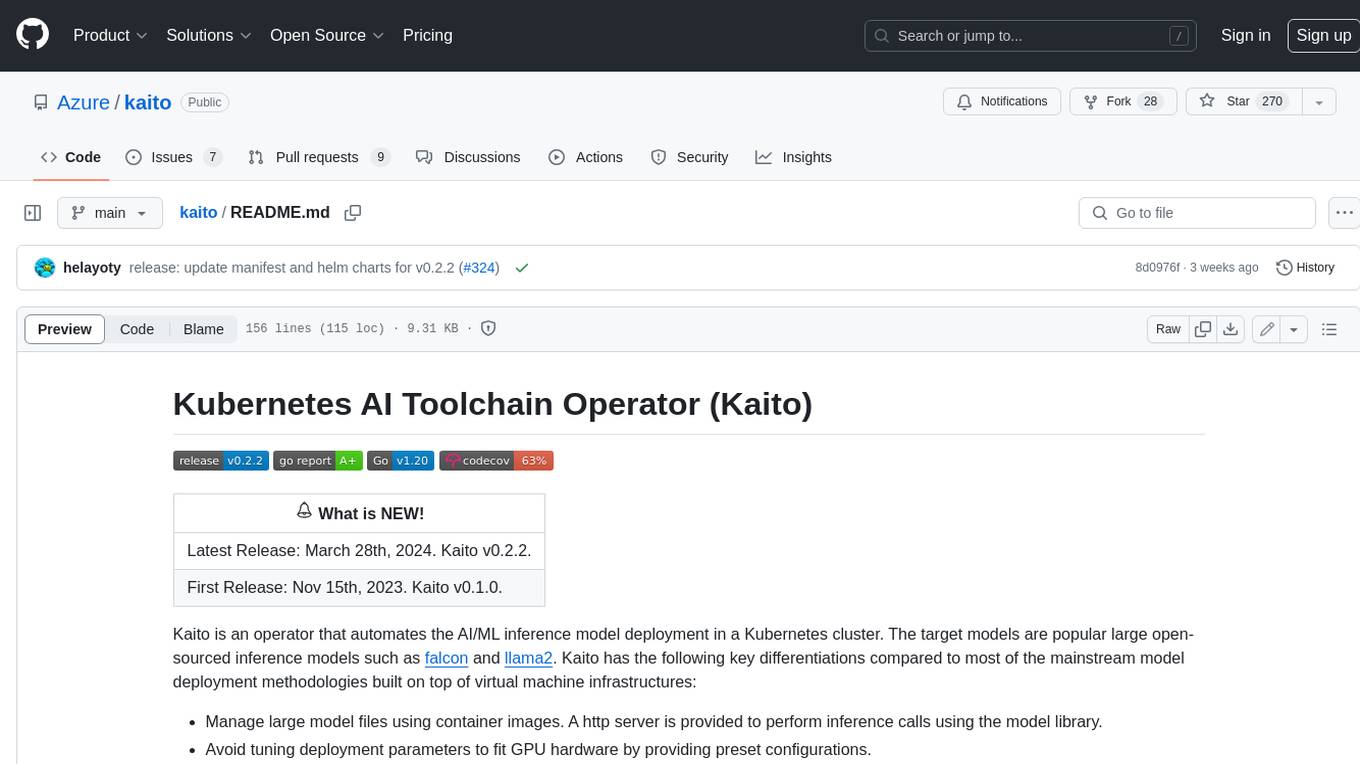
kaito
Kaito is an operator that automates the AI/ML inference model deployment in a Kubernetes cluster. It manages large model files using container images, avoids tuning deployment parameters to fit GPU hardware by providing preset configurations, auto-provisions GPU nodes based on model requirements, and hosts large model images in the public Microsoft Container Registry (MCR) if the license allows. Using Kaito, the workflow of onboarding large AI inference models in Kubernetes is largely simplified.
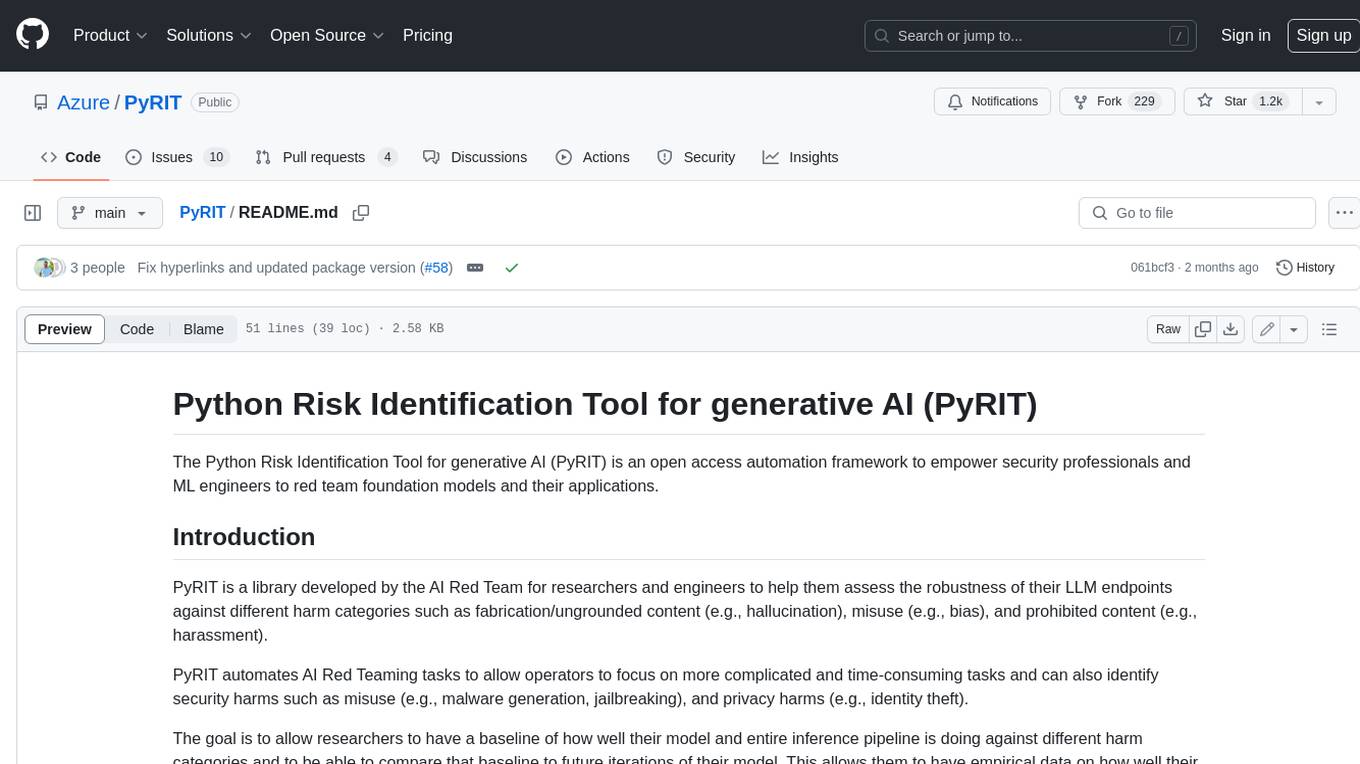
PyRIT
PyRIT is an open access automation framework designed to empower security professionals and ML engineers to red team foundation models and their applications. It automates AI Red Teaming tasks to allow operators to focus on more complicated and time-consuming tasks and can also identify security harms such as misuse (e.g., malware generation, jailbreaking), and privacy harms (e.g., identity theft). The goal is to allow researchers to have a baseline of how well their model and entire inference pipeline is doing against different harm categories and to be able to compare that baseline to future iterations of their model. This allows them to have empirical data on how well their model is doing today, and detect any degradation of performance based on future improvements.
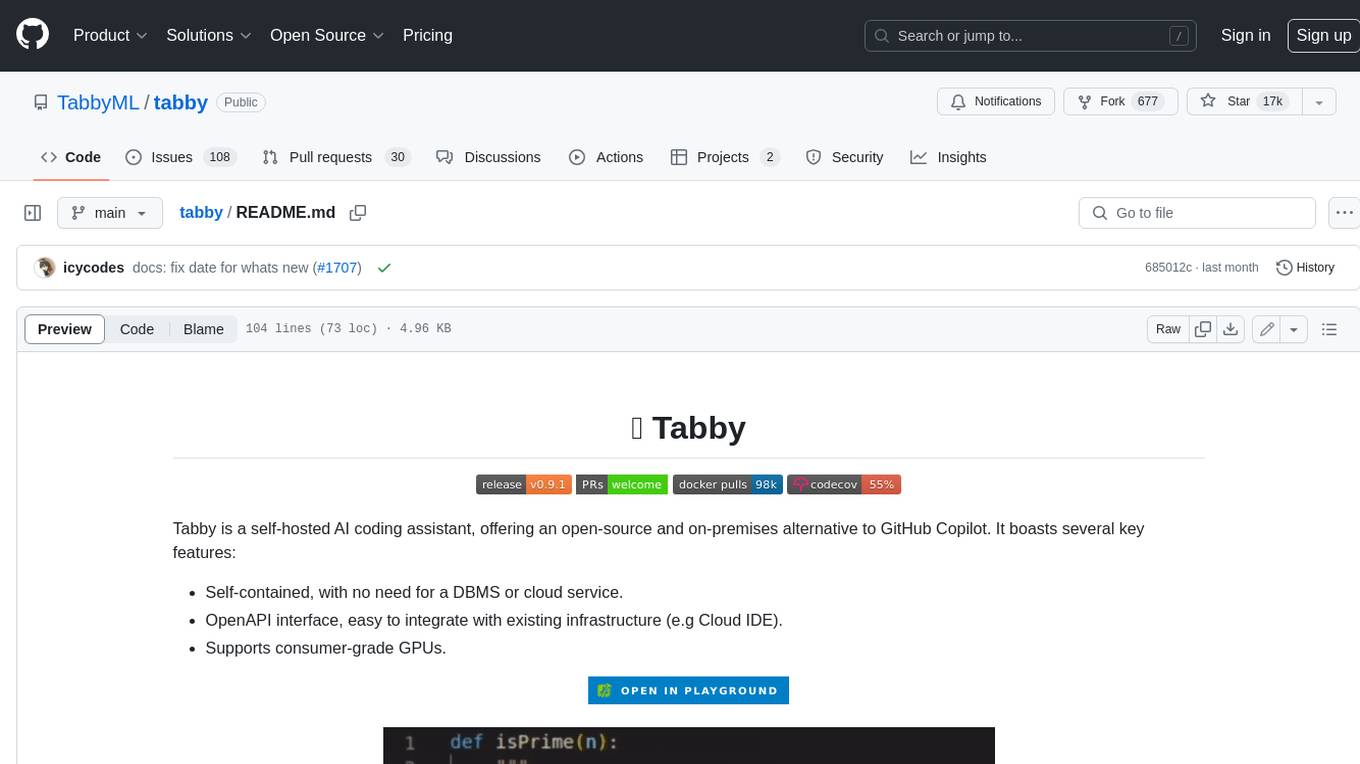
tabby
Tabby is a self-hosted AI coding assistant, offering an open-source and on-premises alternative to GitHub Copilot. It boasts several key features: * Self-contained, with no need for a DBMS or cloud service. * OpenAPI interface, easy to integrate with existing infrastructure (e.g Cloud IDE). * Supports consumer-grade GPUs.
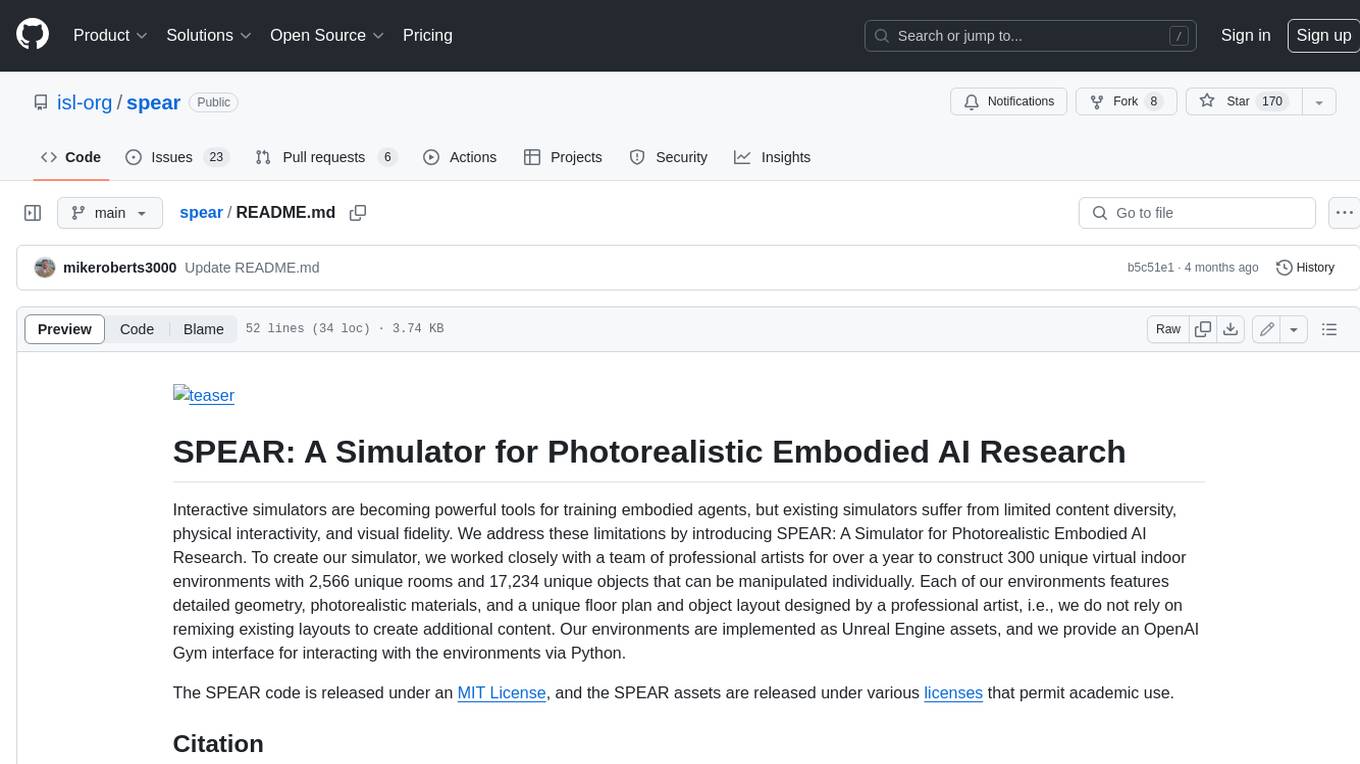
spear
SPEAR (Simulator for Photorealistic Embodied AI Research) is a powerful tool for training embodied agents. It features 300 unique virtual indoor environments with 2,566 unique rooms and 17,234 unique objects that can be manipulated individually. Each environment is designed by a professional artist and features detailed geometry, photorealistic materials, and a unique floor plan and object layout. SPEAR is implemented as Unreal Engine assets and provides an OpenAI Gym interface for interacting with the environments via Python.
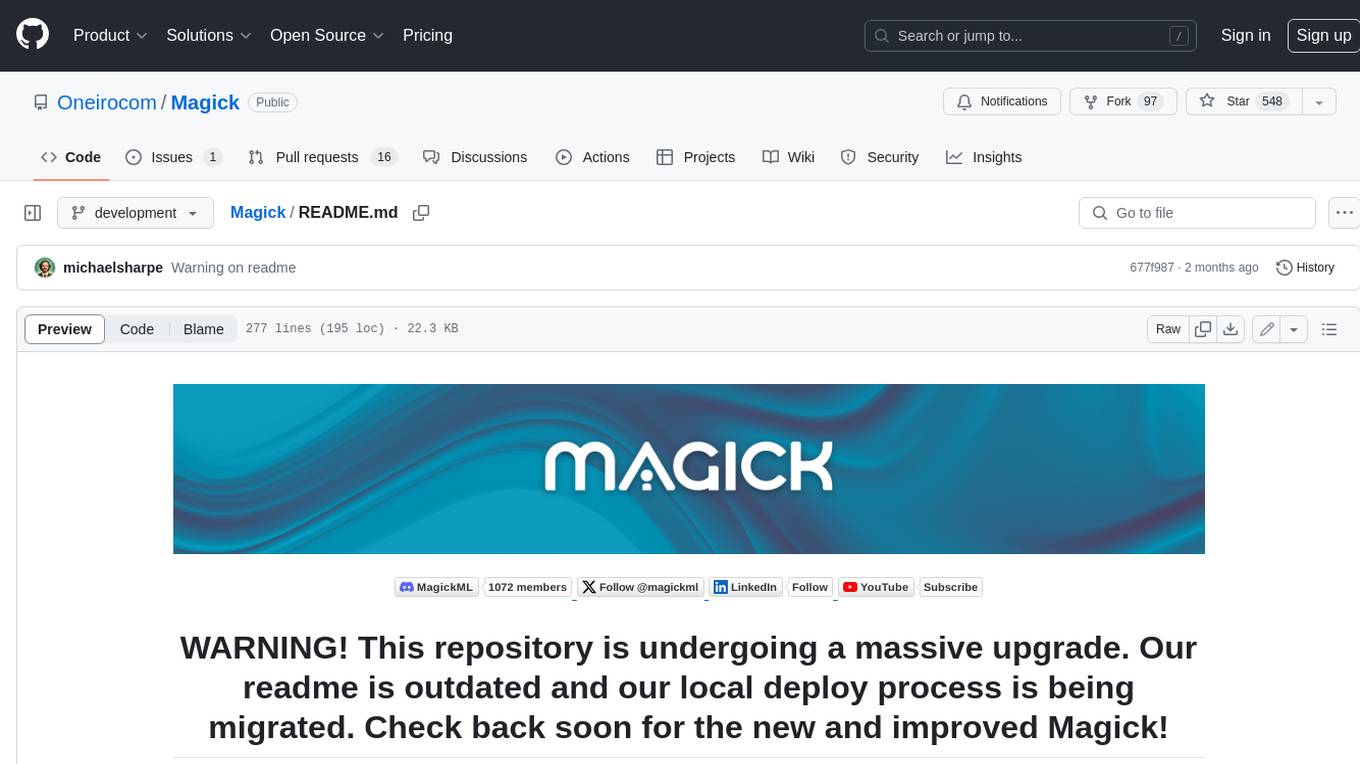
Magick
Magick is a groundbreaking visual AIDE (Artificial Intelligence Development Environment) for no-code data pipelines and multimodal agents. Magick can connect to other services and comes with nodes and templates well-suited for intelligent agents, chatbots, complex reasoning systems and realistic characters.