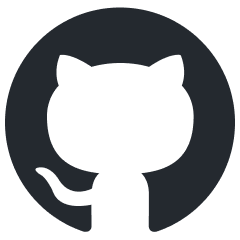
Recommendation-Systems-without-Explicit-ID-Features-A-Literature-Review
Paper List of Pre-trained Foundation Recommender Models
Stars: 261
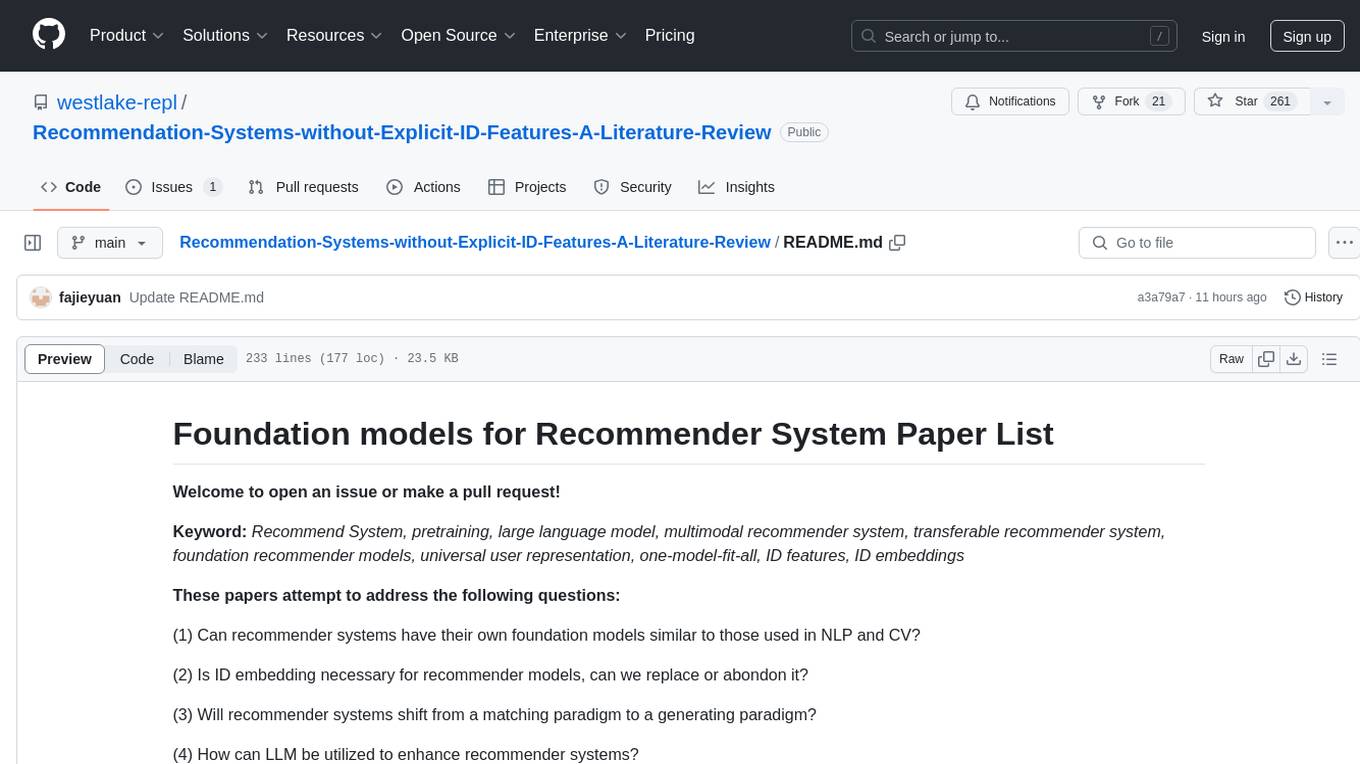
This repository is a collection of papers and resources related to recommendation systems, focusing on foundation models, transferable recommender systems, large language models, and multimodal recommender systems. It explores questions such as the necessity of ID embeddings, the shift from matching to generating paradigms, and the future of multimodal recommender systems. The papers cover various aspects of recommendation systems, including pretraining, user representation, dataset benchmarks, and evaluation methods. The repository aims to provide insights and advancements in the field of recommendation systems through literature reviews, surveys, and empirical studies.
README:
Welcome to open an issue or make a pull request!
Keyword: Recommend System, pretraining, large language model, multimodal recommender system, transferable recommender system, foundation recommender models, universal user representation, one-model-fit-all, ID features, ID embeddings
These papers attempt to address the following questions:
(1) Can recommender systems have their own foundation models similar to those used in NLP and CV?
(2) Is ID embedding necessary for recommender models, can we replace or abondon it?
(3) Will recommender systems shift from a matching paradigm to a generating paradigm?
(4) How can LLM be utilized to enhance recommender systems?
(5) What does the future hold for multimodal recommender systems?
- Where to Go Next for Recommender Systems? ID-vs. Modality-based recommender models revisited, SIGIR2023, 2022/09, [paper] [code]
- Exploring the Upper Limits of Text-Based Collaborative Filtering Using Large Language Models: Discoveries and Insights, arxiv 2023/05, [paper]
- Exploring Adapter-based Transfer Learning for Recommender Systems: Empirical Studies and Practical Insights, WSDM2024, [paper] [code]
- The Elephant in the Room: Rethinking the Usage of Pre-trained Language Model in Sequential Recommendation, arxiv2024/04, [paper]
- NineRec: A Benchmark Dataset Suite for Evaluating Transferable Recommendation, TPAMI2024, [paper] [link] | Images, Text, Nine downstream datasets
- TenRec: A Large-scale Multipurpose Benchmark Dataset for Recommender Systems, NeurIPS 2022 [paper]
- PixelRec: An Image Dataset for Benchmarking Recommender Systems with Raw Pixels, SDM 2023/09 [paper] |[link]| Images, Text, Tags, 200 million interactions
- MicroLens: A Content-Driven Micro-Video Recommendation Dataset at Scale [paper] [link] [DeepMind Talk] | Images, Text, Video, Audio, comments, tags, etc.
- MIND: A Large-scale Dataset for News Recommendation, ACL2020, [paper] | Text
- Parameter-Efficient Transfer from Sequential Behaviors for User Modeling and Recommendation, SIGIR 2020 [link]
- MoRec: [link] Netflix: [link] Amazon: [link]
- Exploring Multi-Scenario Multi-Modal CTR Prediction with a Large Scale Dataset, SIGIR 2024/07 [paper] |[link]| Images, Text, Multi-domain, True Negative, 100 million CTR data
- A Survey on Large Language Models for Recommendation, arxiv 2023/05, [paper]
- How Can Recommender Systems Benefit from Large Language Models: A Survey, arxiv 2023/06, [paper]
- Recommender Systems in the Era of Large Language Models, arxiv, 2023/07, [paper]
- A Survey on Evaluation of Large Language Models, arxiv, 2023/07, [paper]
- Self-Supervised Learning for Recommender Systems: A Survey, arxiv, 2023/06, [paper]
- Pre-train, Prompt and Recommendation: A Comprehensive Survey of Language Modelling Paradigm Adaptations in Recommender Systems, 2022/09, [paper]
- User Modeling in the Era of Large Language Models: Current Research and Future Directions,2023/12, [paper]
- USER MODELING AND USER PROFILING: A COMPREHENSIVE SURVEY,2024/02, [paper]
- Foundation Models for Recommender Systems: A Survey and New Perspectives, 2024/02, [paper]
- Multimodal Pretraining, Adaptation, and Generation for Recommendation: A Survey, 2024/05, [paper]
- Emergent Abilities of Large Language Models, TMLR 2022/08, [paper]
- Exploring the Upper Limits of Text-Based Collaborative Filtering Using Large Language Models: Discoveries and Insights, arxiv 2023/05, [paper]
- Do LLMs Understand User Preferences? Evaluating LLMs On User Rating Prediction, arxiv 2023/05, [paper]
- Scaling Law for Recommendation Models: Towards General-purpose User Representations, AAAI 2023, [paper]
- StackRec: Efficient Training of Very Deep Sequential Recommender Models by Iterative Stacking, SIGIR 2021, [paper]
- A User-Adaptive Layer Selection Framework for Very Deep Sequential Recommender Models, AAAI 2021, [paper]
- A Generic Network Compression Framework for Sequential Recommender Systems, SIGIR 2020, [paper]
- Scaling Law of Large Sequential Recommendation Models, arxiv 2023/11, [paper]
- Actions Speak Louder than Words: Trillion-Parameter Sequential Transducers for Generative Recommendations, arxiv 2024/03, [paper]
- Breaking the Length Barrier: LLM-Enhanced CTR Prediction in Long Textual User Behaviors, SIGIR 2024, [paper]
- M6-Rec: Generative Pretrained Language Models are Open-Ended Recommender Systems,arxiv 2022/05, [paper]
- TALLRec: An Effective and Efficient Tuning Framework to Align Large Language Model with Recommendation, arxiv 2023/04, [paper]
- GPT4Rec: A Generative Framework for Personalized Recommendation and User Interests Interpretation, 2023/04, [paper]
- A Bi-Step Grounding Paradigm for Large Language Models in Recommendation Systems, arxiv, 2023/08, [paper]
- LlamaRec: Two-Stage Recommendation using Large Language Models for Ranking, PGAI@CIKM 2023, [paper] [code]
- Improving Sequential Recommendations with LLMs, arxiv 2024/02, [paper]
Freezing LLM [link]
- CTR-BERT: Cost-effective knowledge distillation for billion-parameter teacher models,arxiv 2022/04, [paper]
- Towards Unified Conversational Recommender Systems via Knowledge-Enhanced Prompt Learning, arxiv 2022/06, [paper]
- Generative Recommendation: Towards Next-generation Recommender Paradigm, arxiv 2023/04, [paper]
- Exploring the Upper Limits of Text-Based Collaborative Filtering Using Large Language Models: Discoveries and Insights, arxiv 2023/05, [paper]
- A First Look at LLM-Powered Generative News Recommendation, arxiv 2023/05, [paper]
- Privacy-Preserving Recommender Systems with Synthetic Query Generation using Differentially Private Large Language Models, arxiv 2023/05,[paper]
- RecAgent: A Novel Simulation Paradigm for Recommender Systems, arxiv 2023/06, [paper]
- Zero-Shot Next-Item Recommendation using Large Pretrained Language Models, arxiv 2023/04, [paper]
- Can ChatGPT Make Fair Recommendation? A Fairness Evaluation Benchmark for Recommendation with Large Language Model, RecSys 2023
- Leveraging Large Language Models for Sequential Recommendation, Recsys 2023/09, [paper]
- LLMRec: Large Language Models with Graph Augmentation for Recommendation, WSDM 2024 Oral, [paper] [code]
- Are ID Embeddings Necessary? Whitening Pre-trained Text Embeddings for Effective Sequential Recommendation, arxiv 2024/02 , [paper]
- Large Language Models are Zero-Shot Rankers for Recommender Systems, arxiv 2023/05, [paper]
- Recommendation as Language Processing (RLP): A Unified Pretrain, Personalized Prompt & Predict Paradigm (P5), arxiv 2022/03, [paper]
- Language Models as Recommender Systems: Evaluations and Limitations, NeurIPS Workshop ICBINB 2021/10, [paper]
- Prompt Learning for News Recommendation, SIGIR 2023/04, [paper]
- LLM-Rec: Personalized Recommendation via Prompting Large Language Models, arxiv,2023/07 [paper]
ChatGPT [link]
- Is ChatGPT a Good Recommender A Preliminary Study, arxiv 2023/04, [paper]
- Is ChatGPT Good at Search? Investigating Large Language Models as Re-Ranking Agent, arxiv 2023/04, [paper]
- Chat-REC: Towards Interactive and Explainable LLMs-Augmented Recommender System, arxiv 2023/04,[paper]
- Recommendation as Instruction Following: A Large Language Model Empowered Recommendation Approach, arxiv 2023/05, [paper]
- Leveraging Large Language Models in Conversational Recommender Systems, arxiv 2023/05, [paper]
- Uncovering ChatGPT’s Capabilities in Recommender Systems, arxiv 2023/05, [paper][code]
- Sparks of Artificial General Recommender (AGR): Early Experiments with ChatGPT, arxiv 2023/05, [paper]
- Is ChatGPT Fair for Recommendation? Evaluating Fairness in Large Language Model Recommendation, arxiv 2023/05,[paper] [code]
- Sparks of Artificial General Recommender (AGR): Early Experiments with ChatGPT, arxiv 2023/05,[paper]
- PALR: Personalization Aware LLMs for Recommendation, arxiv 2023/05, [paper]
- Privacy-Preserving Recommender Systems with Synthetic Query Generation using Differentially Private Large Language Models, arxiv 2023/05, [paper]
- Rethinking the Evaluation for Conversational Recommendation in the Era of Large Language Models, arxiv 2023/05, [paper]
- CTRL: Connect Tabular and Language Model for CTR Prediction, arxiv 2023/06,[paper].
- VBPR: Visual Bayesian Personalized Ranking from Implicit Feedback, AAAI2016, [paper]
- Adversarial Training Towards Robust Multimedia Recommender System, TKDE2019, [paper]
- Multi-modal Knowledge Graphs for Recommender Systems, CIKM 2020, [paper]
- Online Distillation-enhanced Multi-modal Transformer for Sequential Recommendation, ACMMM 2023, [paper]
- Self-Supervised Multi-Modal Sequential Recommendation, arxiv2023/02, [paper]
- FMMRec: Fairness-aware Multimodal Recommendation, arxiv2023/10, [paper]
- Self-Supervised Multi-Modal Sequential Recommendation, arxiv 2024/02, [paper]
- ID Embedding as Subtle Features of Content and Structure for Multimodal Recommendation, arxiv2023/10, [paper]
- Enhancing ID and Text Fusion via Alternative Training in Session-based Recommendation, arxiv2023/2, [paper]
- BiVRec: Bidirectional View-based Multimodal Sequential Recommendation,arxiv2023/2, [paper]
- A Large Language Model Enhanced Sequential Recommender for Joint Video and Comment Recommendation, arxiv2024/2, [paper]
- An Empirical Study of Training ID-Agnostic Multi-modal Sequential Recommenders, arxiv2024/3, [paper]
- Discrete Semantic Tokenization for Deep CTR Prediction, arxiv2024/3, [paper]
- End-to-end training of Multimodal Model and ranking Model, arxiv2023/3, [paper]
- TransRec: Learning Transferable Recommendation from Mixture-of-Modality Feedback, arxiv 2022/06, [paper]
- Towards Universal Sequence Representation Learning for Recommender Systems, KDD2022,2022/06, [paper]
- Learning Vector-Quantized Item Representation for Transferable Sequential Recommenders, WWW 2023, [paper] [code]
- UP5: Unbiased Foundation Model for Fairness-aware Recommendation, arxiv 2023/05, [paper]
- Exploring Adapter-based Transfer Learning for Recommender Systems: Empirical Studies and Practical Insights, arxiv 2023/05, [paper] [code]
- OpenP5: Benchmarking Foundation Models for Recommendation, arxiv 2023/06, [paper]
- Thoroughly Modeling Multi-domain Pre-trained Recommendation as Language, arxiv 2023/10, [paper]
- MISSRec: Pre-training and Transferring Multi-modal Interest-aware Sequence Representation for Recommendation, arxiv 2023/10, [paper]
- Collaborative Word-based Pre-trained Item Representation for Transferable Recommendation, arxiv 2023/11, [paper]
- Universal Multi-modal Multi-domain Pre-trained Recommendation, arxiv 2023/11, [paper]
- Multi-Modality is All You Need for Transferable Recommender Systems, arxiv 2023, [paper]
- TransFR: Transferable Federated Recommendation with Pre-trained Language Models, arxiv 2024/02 ,[paper]
- Rethinking Cross-Domain Sequential Recommendation under Open-World Assumptions, arxiv 2024/02 ,[paper]
- Large Language Models meet Collaborative Filtering: An Efficient All-round LLM-based Recommender System, arxiv 2024/04 ,[paper]
- Language Models Encode Collaborative Signals in Recommendation, arxiv 2024/07 ,[paper]
- Parameter-Efficient Transfer from Sequential Behaviors for User Modeling and Recommendation, SIGIR 2020, [paper], [code]
- One4all User Representation for Recommender Systems in E-commerce, arxiv 2021, [paper]
- Learning Transferable User Representations with Sequential Behaviors via Contrastive Pre-training, ICDM 2021, [paper]
- User-specific Adaptive Fine-tuning for Cross-domain Recommendations, TKDE 2021, [paper]
- Scaling Law for Recommendation Models: Towards General-purpose User Representations, AAAI 2023, [paper]
- U-BERT: Pre-training user representations for improved recommendation, AAAI 2021, [paper]
- One for All, All for One: Learning and Transferring User Embeddings for Cross-Domain Recommendation, WSDM 2022, [paper]
- Field-aware Variational Autoencoders for Billion-scale User Representation Learning,ICDE2022, [paper]
- Learning Large-scale Universal User Representation with Sparse Mixture of Experts, ICML2022workshop, [paper]
- Multi Datasource LTV User Representation (MDLUR), KDD2023, [paper]
- Pivotal Role of Language Modeling in Recommender Systems: Enriching Task-specific and Task-agnostic Representation Learning. arxiv2022/12, [paper]
- USER MODELING AND USER PROFILING: A COMPREHENSIVE SURVEY,2024/02, [paper]
- Generalized User Representations for Transfer Learning, arxiv 2024/03, [paper]
- Bridging Language and Items for Retrieval and Recommendation,arxiv 2024/04, [paper]
- One Person, One Model, One World: Learning Continual User Representation without Forgetting, SIGIR 2021, [paper], [code]
- Tenrec: A Large-scale Multipurpose Benchmark Dataset for Recommender Systems, NeurIPS 2022 [paper]
- STAN: Stage-Adaptive Network for Multi-Task Recommendation by Learning User Lifecycle-Based Representationg, Recsys 2023, [paper]
- Task Relation-aware Continual User Representation Learning, KDD2023, [paper]
- ReLLa: Retrieval-enhanced Large Language Models for Lifelong Sequential Behavior Comprehension in Recommendation, arxiv2023/08, [paper]
Generative Recommender Systems [link]
- A Simple Convolutional Generative Network for Next Item Recommendation, WSDM 2018/08, [paper] [code]
- Future Data Helps Training: Modeling Future Contexts for Session-based Recommendation, WWW 2020/04, [paper] [code]
- Recommendation via Collaborative Diffusion Generative Model, KSEM 2022/08, [paper]
- Blurring-Sharpening Process Models for Collaborative Filtering, arxiv 2022/09, [paper]
- Generative Slate Recommendation with Reinforcement Learning, arxiv 2023/01, [paper]
- Recommender Systems with Generative Retrieval, arxiv 2023/04, [paper]
- DiffuRec: A Diffusion Model for Sequential Recommendation, arxiv 2023/04, [paper]
- Diffusion Recommender Model, arxiv 2023/04, [paper]
- A First Look at LLM-Powered Generative News Recommendation, arxiv 2023/05, [paper]
- Recommender Systems with Generative Retrieval, arxiv 2023/05, [paper]
- Generative Retrieval as Dense Retrieval, arxiv 2023/06, [paper]
- RecFusion: A Binomial Diffusion Process for 1D Data for Recommendation, arxiv 2023/06, [paper]
- Generative Sequential Recommendation with GPTRec, SIGIR workshop 2023, [paper]
- FANS: Fast Non-Autoregressive Sequence Generation for Item List Continuation, WWW 2023, [paper]
- Generative Next-Basket Recommendation, RecSys 2023
- Large Language Model Augmented Narrative Driven Recommendations, RecSys 2023, [paper]
- LightLM: A Lightweight Deep and Narrow Language Model forGenerative Recommendation, arxiv 2023/10, [paper]
- Xiangyang Li Github [Link]
- nancheng58i Github [Link]
- enoche Github [Link]
- WLiK Github [Link]
- https://github.com/CHIANGEL/Awesome-LLM-for-RecSys
- https://medium.com/@lifengyi_6964/a-large-scale-short-video-recommender-system-dataset-160fdfe81b79
- https://medium.com/@lifengyi_6964/rethinking-the-id-paradigm-in-recommender-systems-the-rise-of-modality-98f449dec016
- https://medium.com/@lifengyi_6964/one-model-for-all-universal-recommender-system-82dab214a07d
- https://zhuanlan.zhihu.com/p/437671278
- https://zhuanlan.zhihu.com/p/675213913
- https://zhuanlan.zhihu.com/p/684805058
- https://zhuanlan.zhihu.com/p/665467596
If you have an innovative idea for building a universal foundation recommendation model but require large-scale dataset and computational resources, consider joining our lab as an intern or visiting scholar. We can provide access to 100 NVIDIA 80G A100 GPUs and a billion-level dataset of user-video/image/text interactions.
The laboratory is hiring research assistants, interns, doctoral students, and postdoctoral researchers. Please contact the corresponding author for details.
实验室招聘科研助理,实习生,博士生和博士后,请联系[email protected].
For Tasks:
Click tags to check more tools for each tasksFor Jobs:
Alternative AI tools for Recommendation-Systems-without-Explicit-ID-Features-A-Literature-Review
Similar Open Source Tools
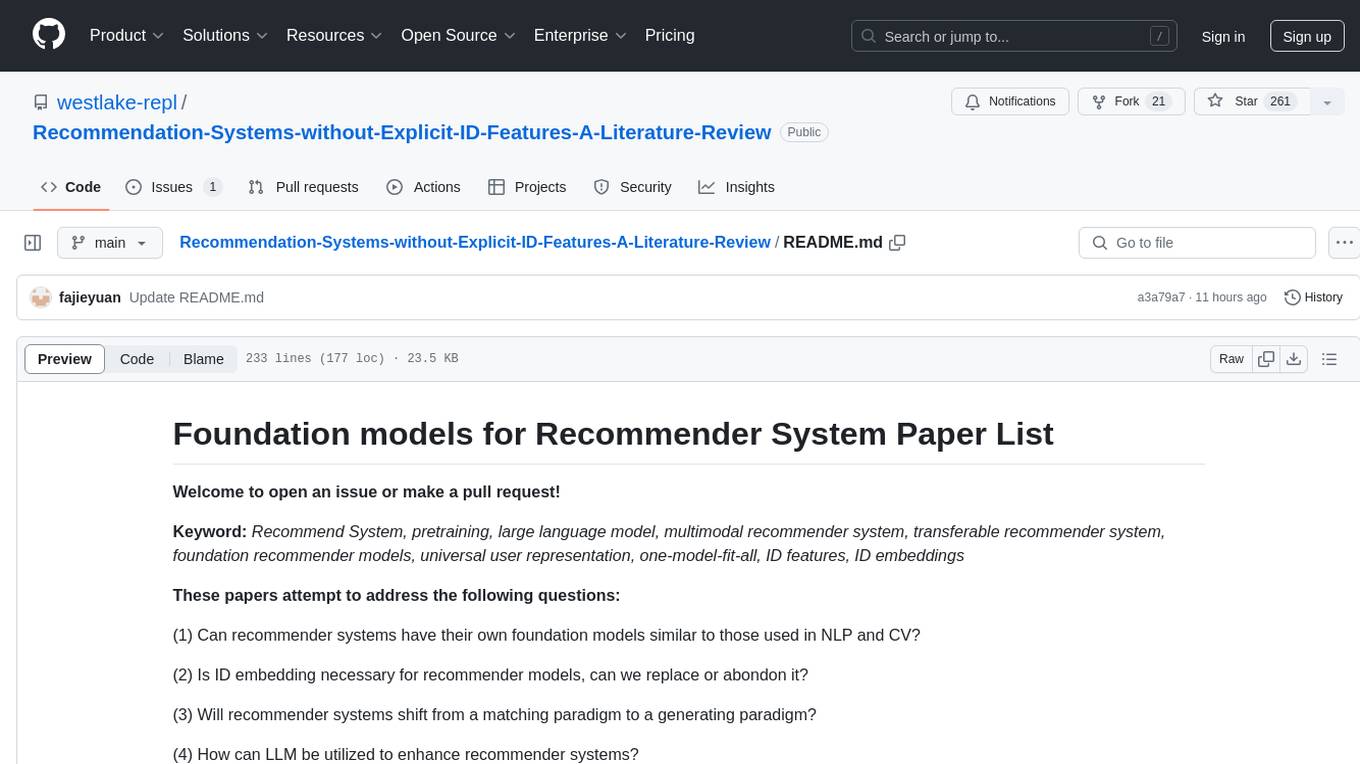
Recommendation-Systems-without-Explicit-ID-Features-A-Literature-Review
This repository is a collection of papers and resources related to recommendation systems, focusing on foundation models, transferable recommender systems, large language models, and multimodal recommender systems. It explores questions such as the necessity of ID embeddings, the shift from matching to generating paradigms, and the future of multimodal recommender systems. The papers cover various aspects of recommendation systems, including pretraining, user representation, dataset benchmarks, and evaluation methods. The repository aims to provide insights and advancements in the field of recommendation systems through literature reviews, surveys, and empirical studies.
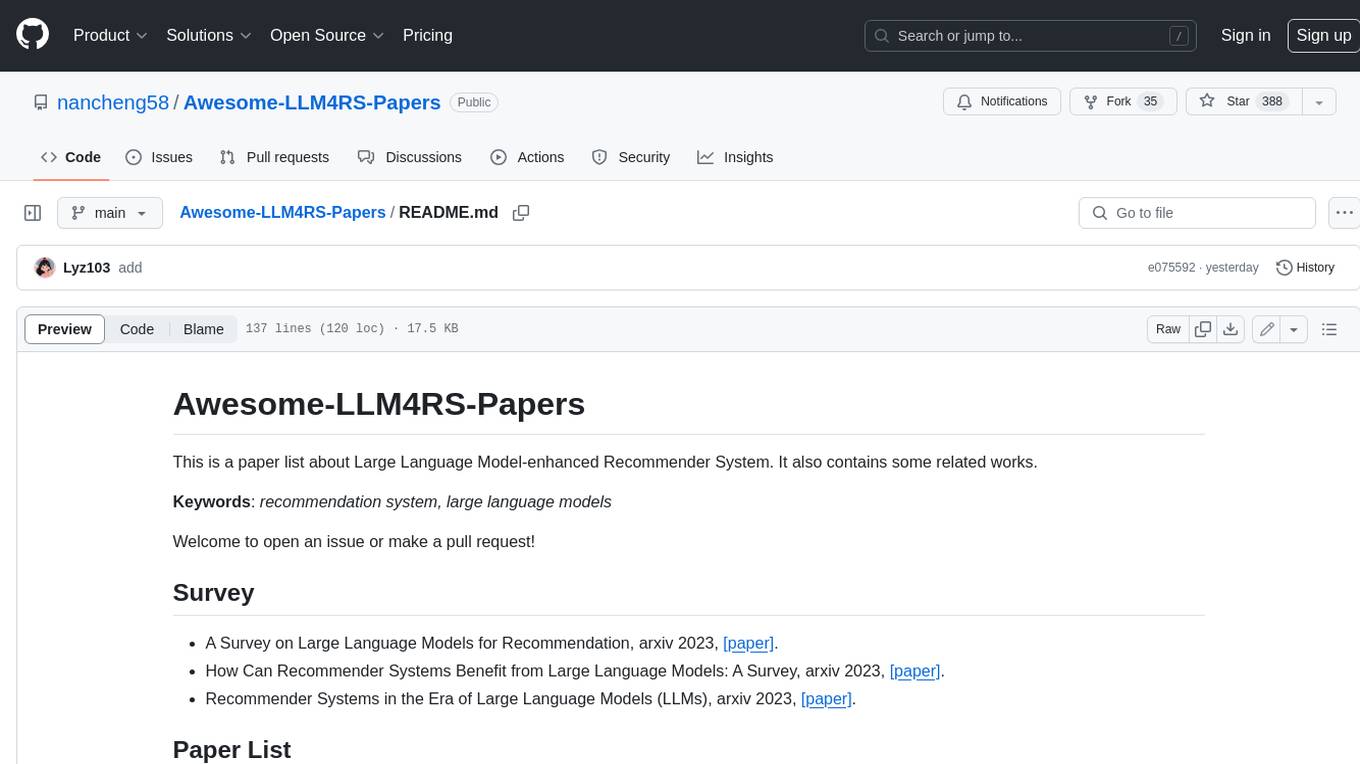
Awesome-LLM4RS-Papers
This paper list is about Large Language Model-enhanced Recommender System. It also contains some related works. Keywords: recommendation system, large language models
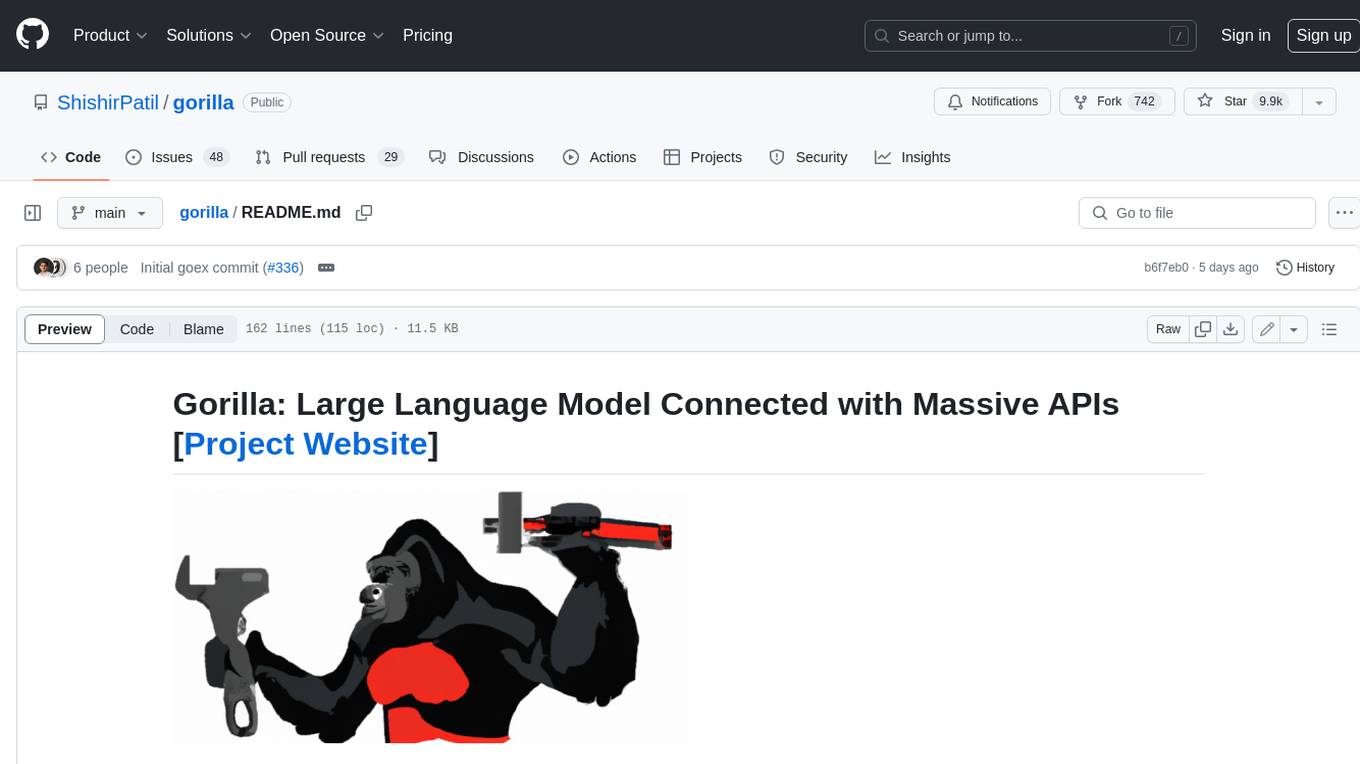
gorilla
Gorilla is a tool that enables LLMs to use tools by invoking APIs. Given a natural language query, Gorilla comes up with the semantically- and syntactically- correct API to invoke. With Gorilla, you can use LLMs to invoke 1,600+ (and growing) API calls accurately while reducing hallucination. Gorilla also releases APIBench, the largest collection of APIs, curated and easy to be trained on!
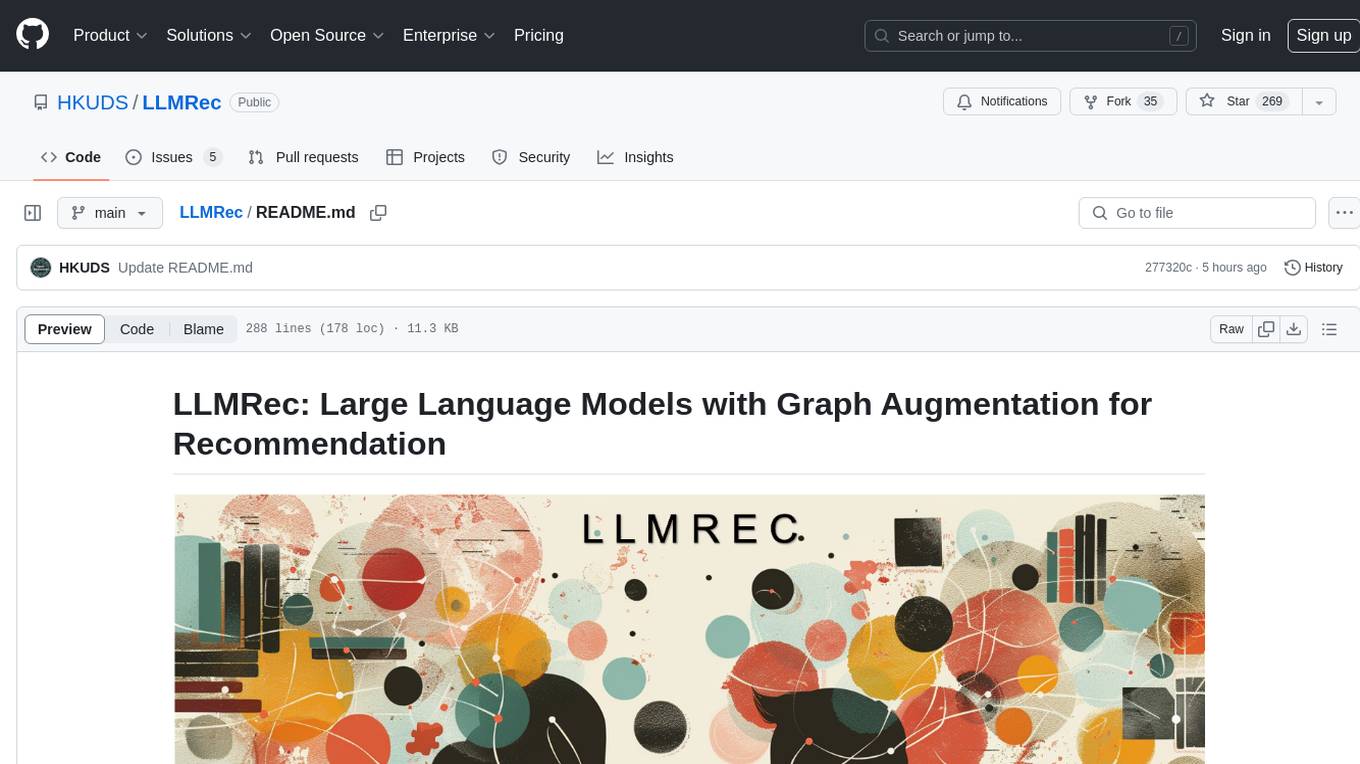
LLMRec
LLMRec is a PyTorch implementation for the WSDM 2024 paper 'Large Language Models with Graph Augmentation for Recommendation'. It is a novel framework that enhances recommenders by applying LLM-based graph augmentation strategies to recommendation systems. The tool aims to make the most of content within online platforms to augment interaction graphs by reinforcing u-i interactive edges, enhancing item node attributes, and conducting user node profiling from a natural language perspective.
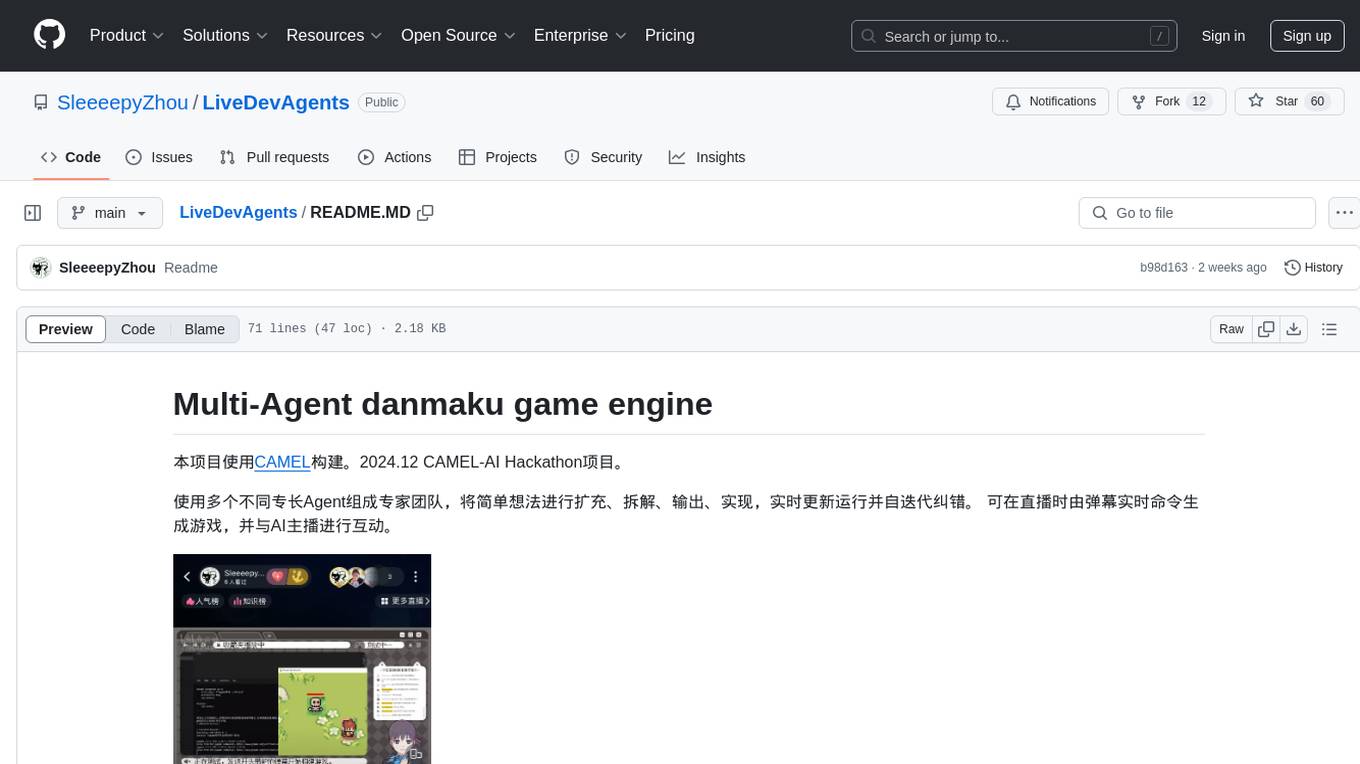
LiveDevAgents
LiveDevAgents is a multi-agent danmaku game engine built using CAMEL. It was developed for the 2024.12 CAMEL-AI Hackathon project. The engine allows for the creation of games in real-time through live bullet comments during streaming, enabling interaction with AI hosts. The project aims to expand and deconstruct simple ideas with a team of agents of different expertise, continuously updating and self-correcting during runtime. It also supports workforce enhancement, migration of anchor agents to a new framework, improvement of bullet comment processing logic, expansion of live control for more platforms, integration of art and music agents, and VR shared workspace for collaborative development.
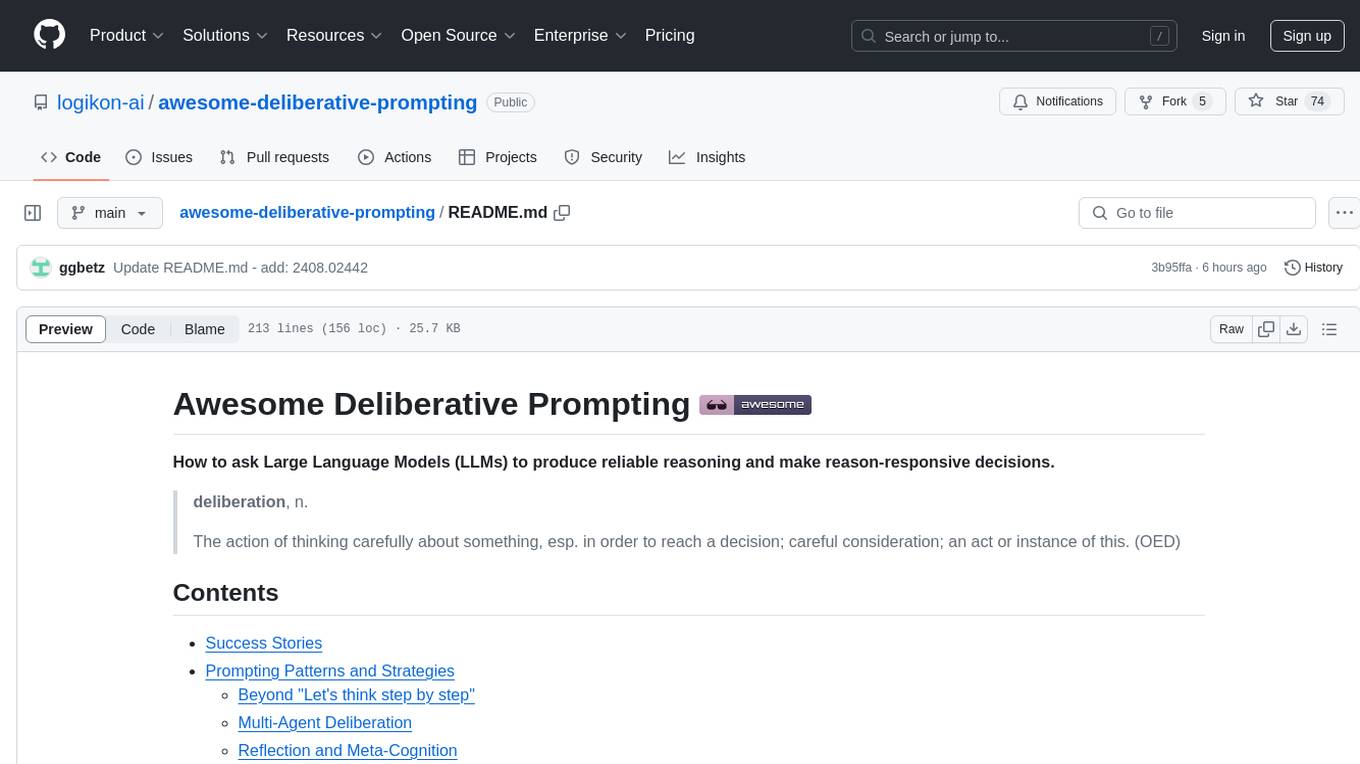
awesome-deliberative-prompting
The 'awesome-deliberative-prompting' repository focuses on how to ask Large Language Models (LLMs) to produce reliable reasoning and make reason-responsive decisions through deliberative prompting. It includes success stories, prompting patterns and strategies, multi-agent deliberation, reflection and meta-cognition, text generation techniques, self-correction methods, reasoning analytics, limitations, failures, puzzles, datasets, tools, and other resources related to deliberative prompting. The repository provides a comprehensive overview of research, techniques, and tools for enhancing reasoning capabilities of LLMs.
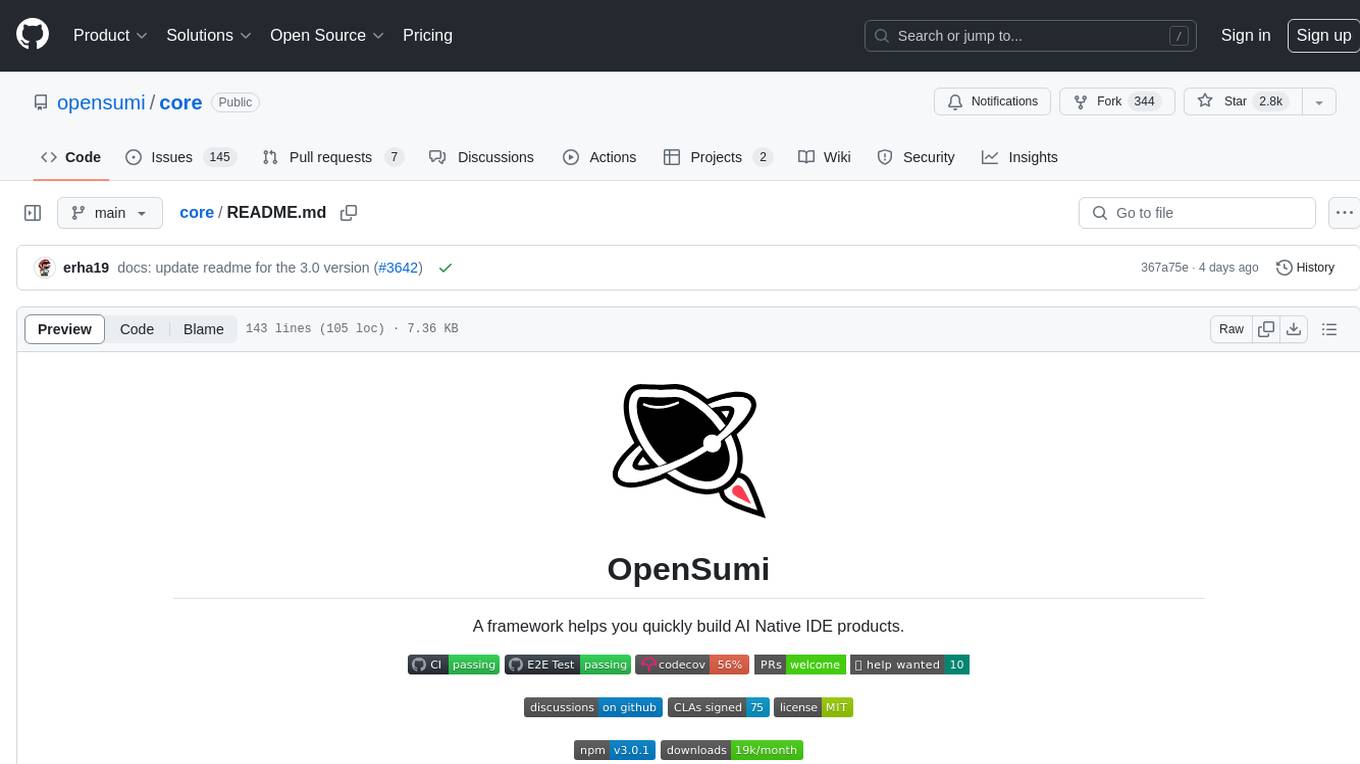
core
OpenSumi is a framework designed to help users quickly build AI Native IDE products. It provides a set of tools and templates for creating Cloud IDEs, Desktop IDEs based on Electron, CodeBlitz web IDE Framework, Lite Web IDE on the Browser, and Mini-App liked IDE. The framework also offers documentation for users to refer to and a detailed guide on contributing to the project. OpenSumi encourages contributions from the community and provides a platform for users to report bugs, contribute code, or improve documentation. The project is licensed under the MIT license and contains third-party code under other open source licenses.
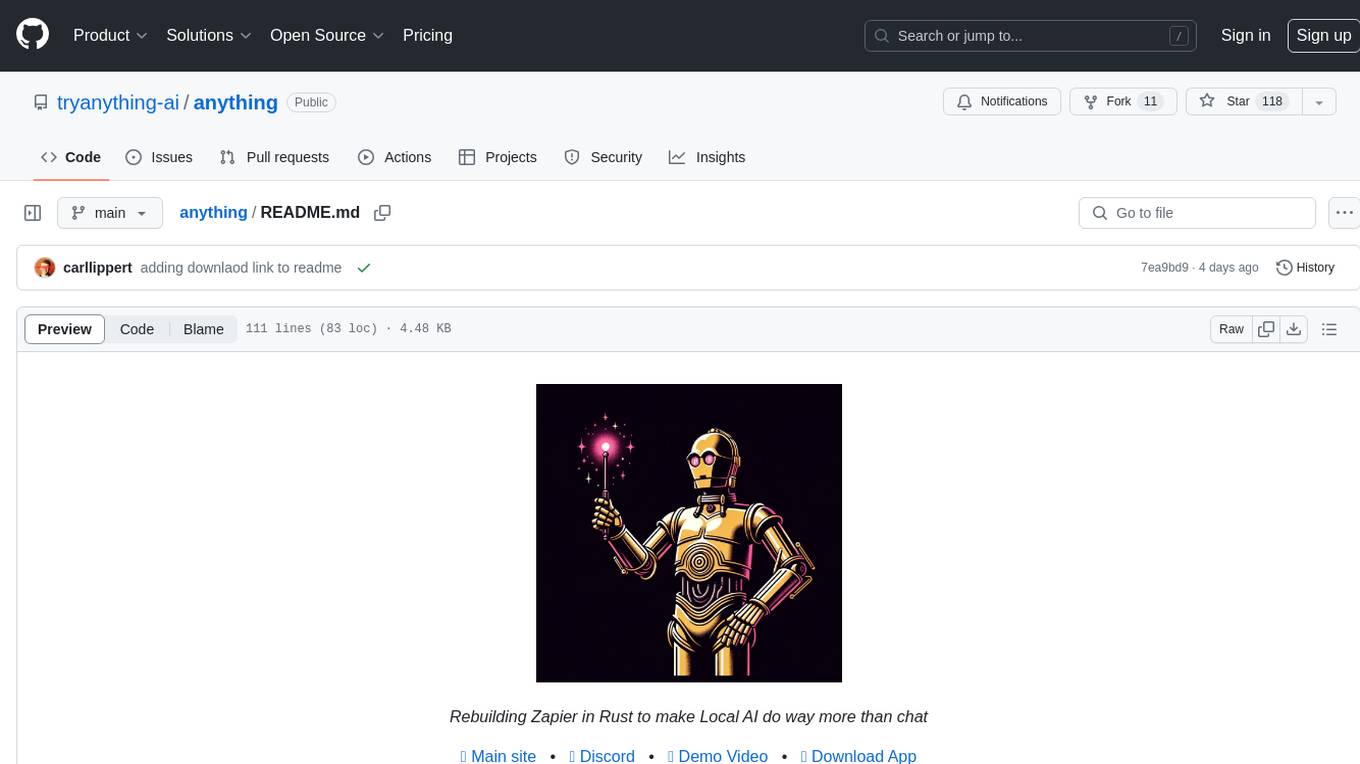
anything
Anything is an open automation tool built in Rust that aims to rebuild Zapier, enabling local AI to perform a wide range of tasks beyond chat functionalities. The tool focuses on extensibility without sacrificing understandability, allowing users to create custom extensions in Rust or other interpreted languages like Python or Typescript. It features an embedded SQLite DB, a WYSIWYG editor, event system, cron trigger, HTTP and CLI extensions, with plans for additional extensions like Deno, Python, and Local AI. The tool is designed to be user-friendly, with a file-first state approach, portable triggers, actions, and flows, and a human-centric file and folder naming convention. It does not require Docker, making it easy to run on low-powered devices for 24/7 self-hosting. The event processing is focused on simplicity and visibility, with extensibility through custom extensions and a marketplace for templates, actions, and triggers.
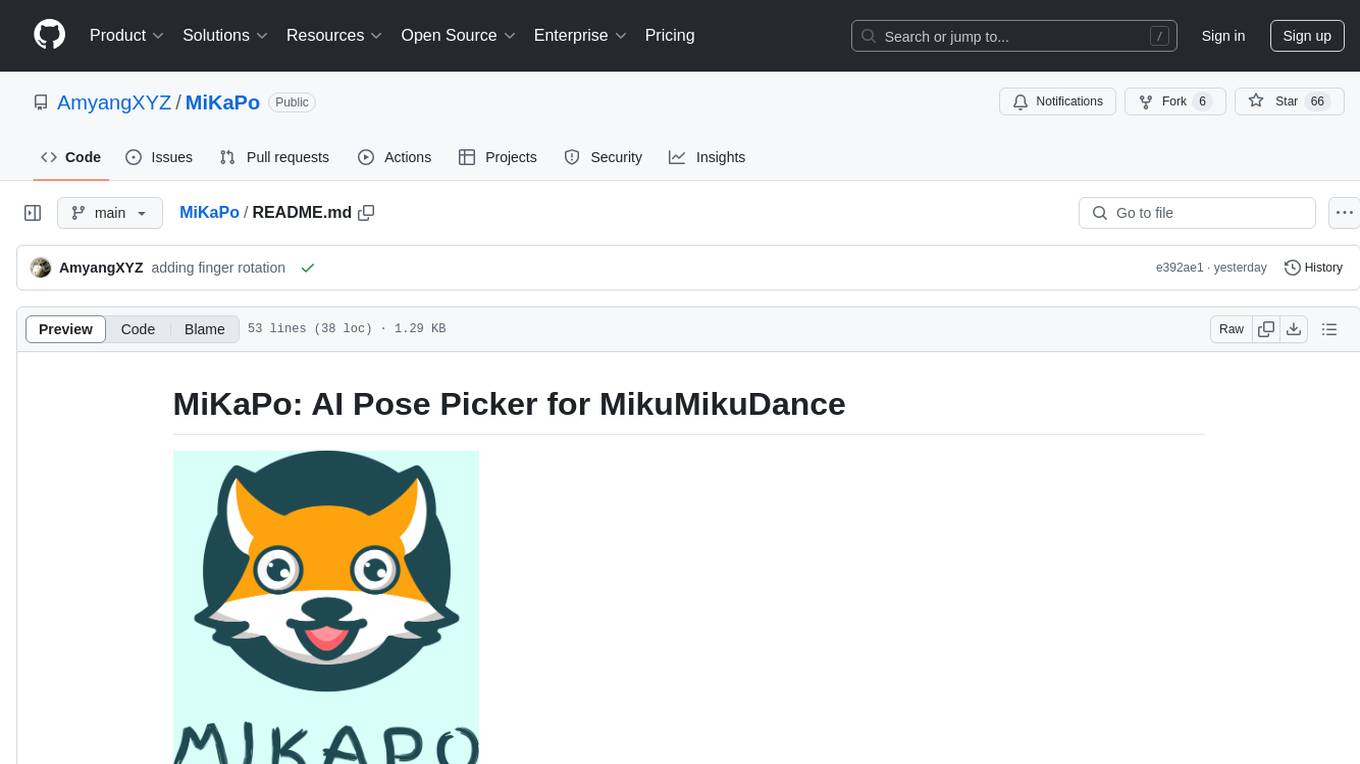
MiKaPo
MiKaPo is a web-based tool that allows users to pose MMD models in real-time using video input. It utilizes technologies such as Mediapipe for 3D key points detection, Babylon.js for 3D scene rendering, babylon-mmd for MMD model viewing, and Vite+React for the web framework. Users can upload videos and images, select different environments, and choose models for posing. MiKaPo also supports camera input and Ollama (electron version). The tool is open to feature requests and pull requests, with ongoing development to add VMD export functionality.
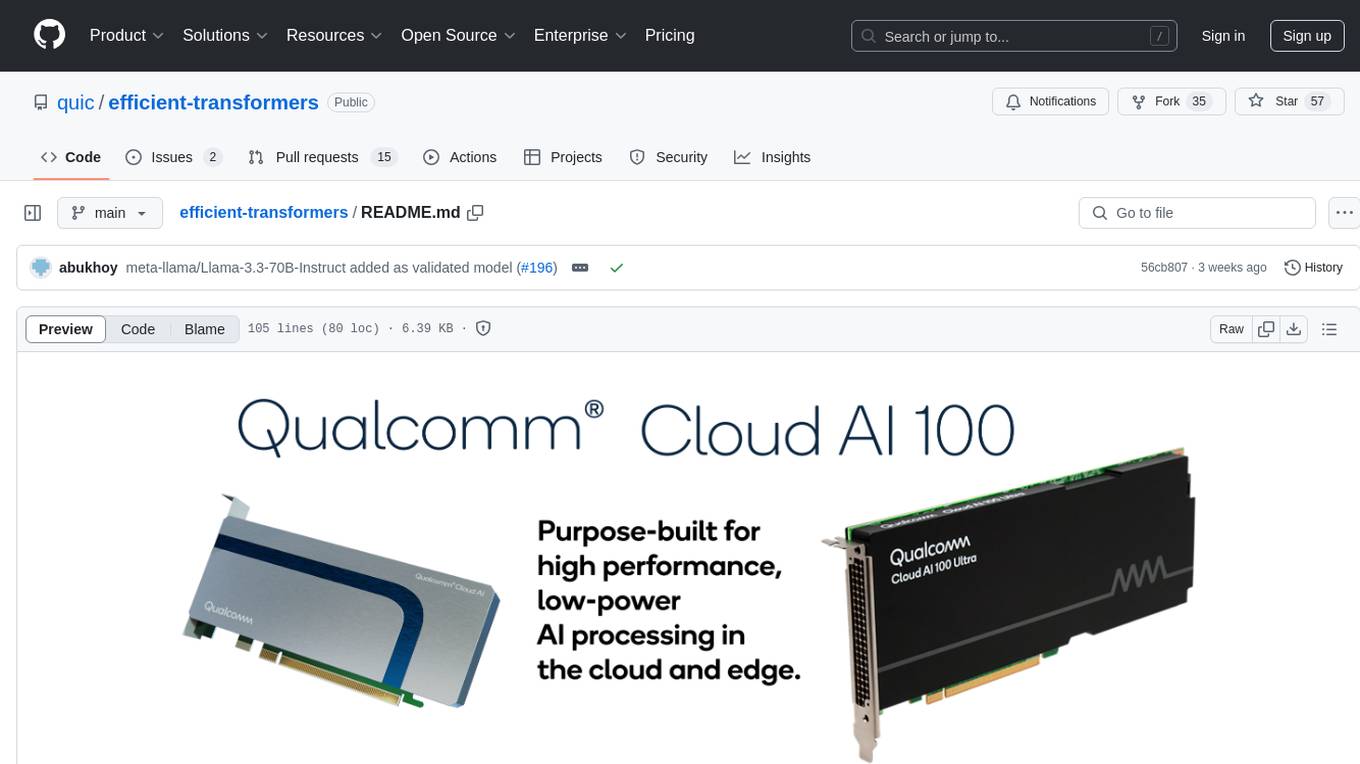
efficient-transformers
Efficient Transformers Library provides reimplemented blocks of Large Language Models (LLMs) to make models functional and highly performant on Qualcomm Cloud AI 100. It includes graph transformations, handling for under-flows and overflows, patcher modules, exporter module, sample applications, and unit test templates. The library supports seamless inference on pre-trained LLMs with documentation for model optimization and deployment. Contributions and suggestions are welcome, with a focus on testing changes for model support and common utilities.
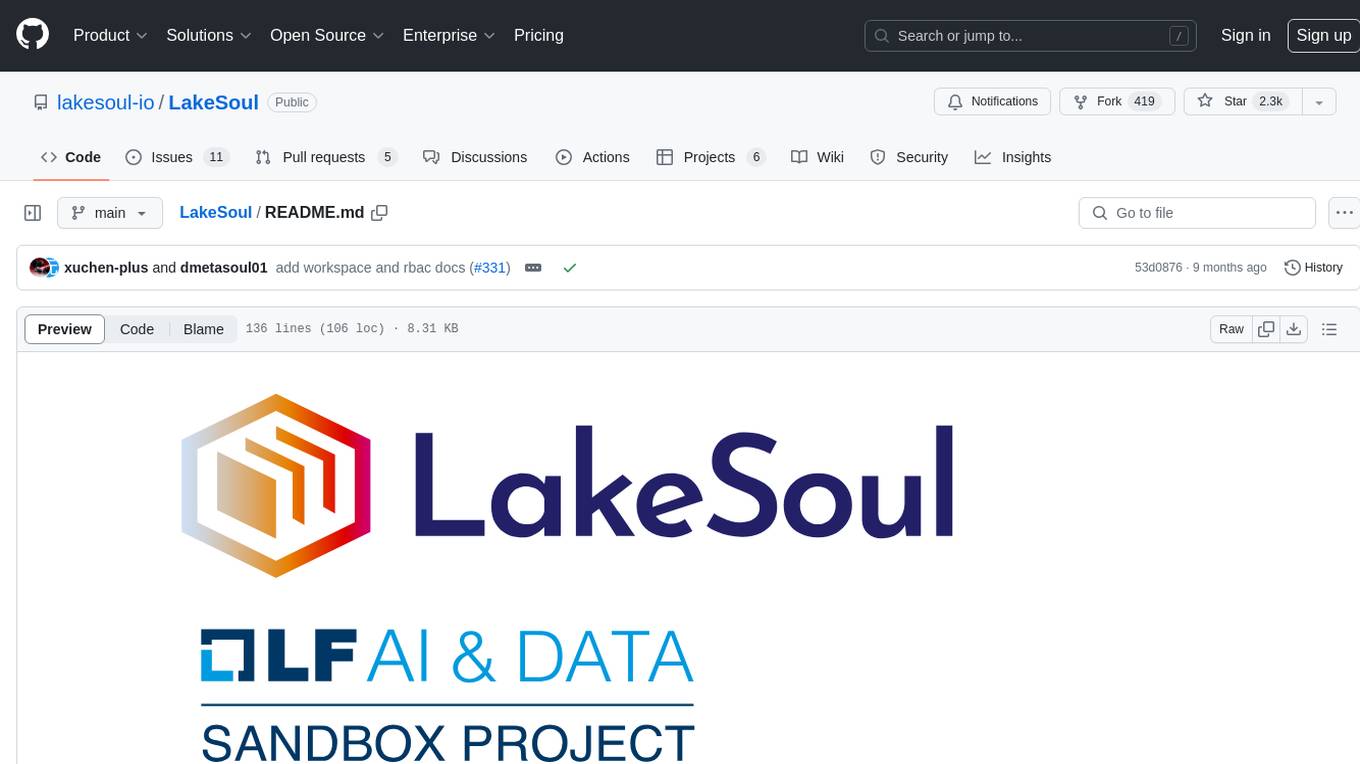
LakeSoul
LakeSoul is a cloud-native Lakehouse framework that supports scalable metadata management, ACID transactions, efficient and flexible upsert operation, schema evolution, and unified streaming & batch processing. It supports multiple computing engines like Spark, Flink, Presto, and PyTorch, and computing modes such as batch, stream, MPP, and AI. LakeSoul scales metadata management and achieves ACID control by using PostgreSQL. It provides features like automatic compaction, table lifecycle maintenance, redundant data cleaning, and permission isolation for metadata.
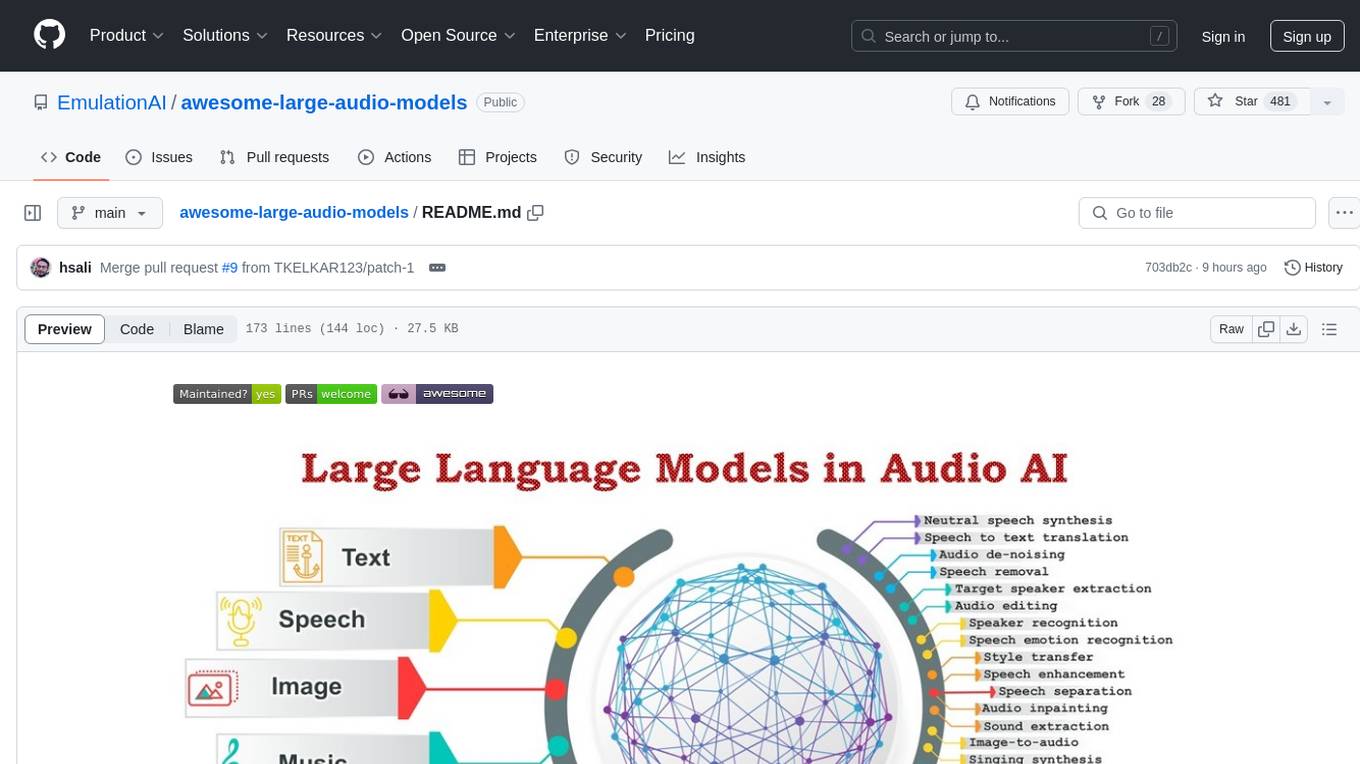
awesome-large-audio-models
This repository is a curated list of awesome large AI models in audio signal processing, focusing on the application of large language models to audio tasks. It includes survey papers, popular large audio models, automatic speech recognition, neural speech synthesis, speech translation, other speech applications, large audio models in music, and audio datasets. The repository aims to provide a comprehensive overview of recent advancements and challenges in applying large language models to audio signal processing, showcasing the efficacy of transformer-based architectures in various audio tasks.
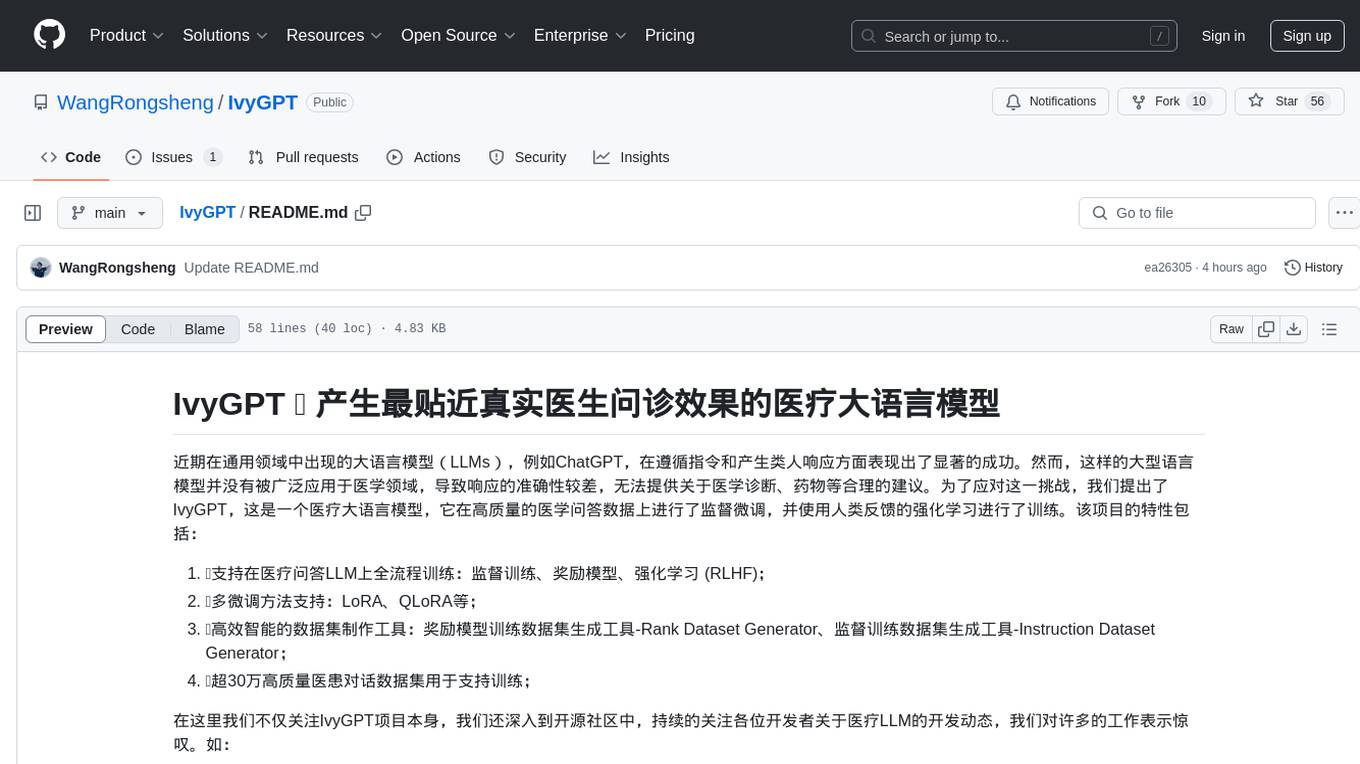
IvyGPT
IvyGPT is a medical large language model that aims to generate the most realistic doctor consultation effects. It has been fine-tuned on high-quality medical Q&A data and trained using human feedback reinforcement learning. The project features full-process training on medical Q&A LLM, multiple fine-tuning methods support, efficient dataset creation tools, and a dataset of over 300,000 high-quality doctor-patient dialogues for training.
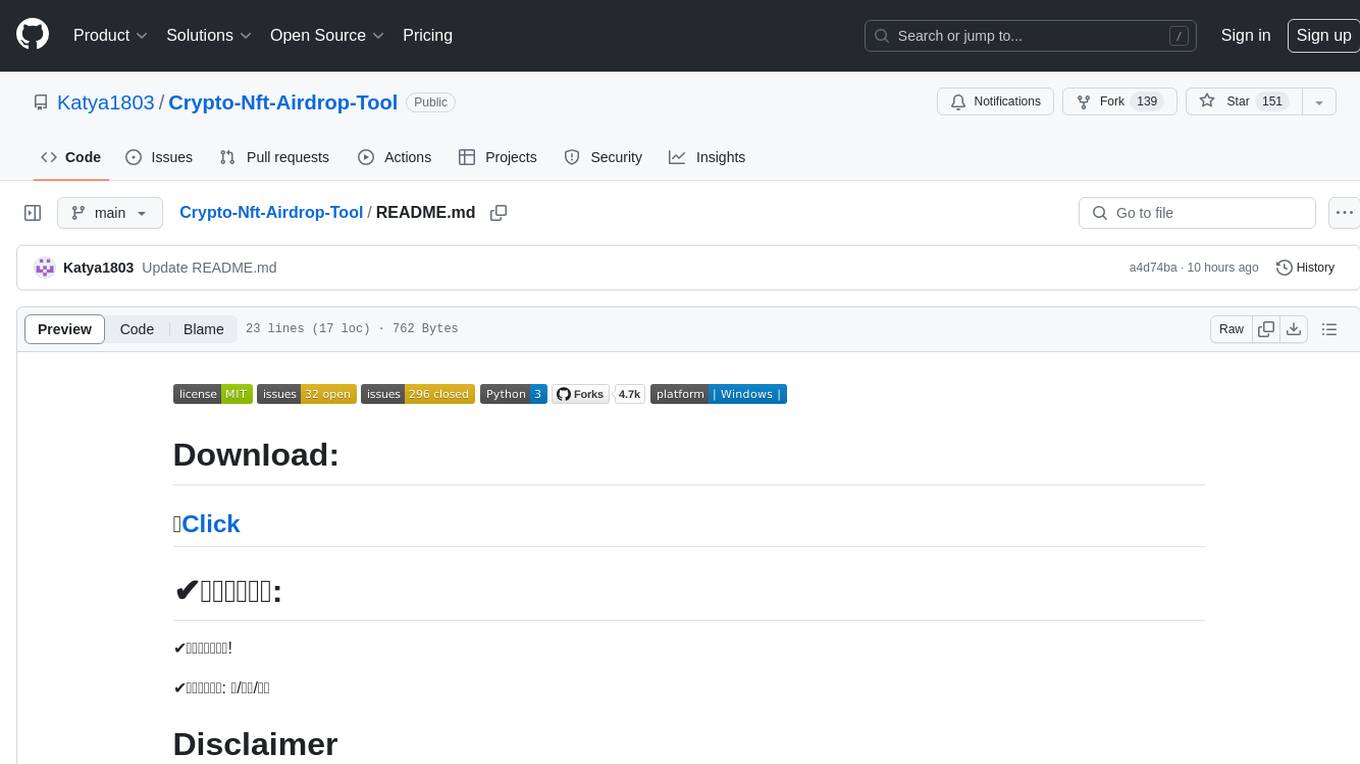
Crypto-Nft-Airdrop-Tool
Crypto-Nft-Airdrop-Tool is a Python tool designed for conducting airdrops of NFTs in the crypto space. It provides functionality for distributing NFTs to a specified audience efficiently. The tool is compatible with Windows platform and requires Python 3. Users can easily manage and execute airdrop campaigns using this tool, enhancing their engagement with the NFT community. The tool simplifies the process of distributing NFTs and ensures a seamless experience for both creators and recipients.
For similar tasks
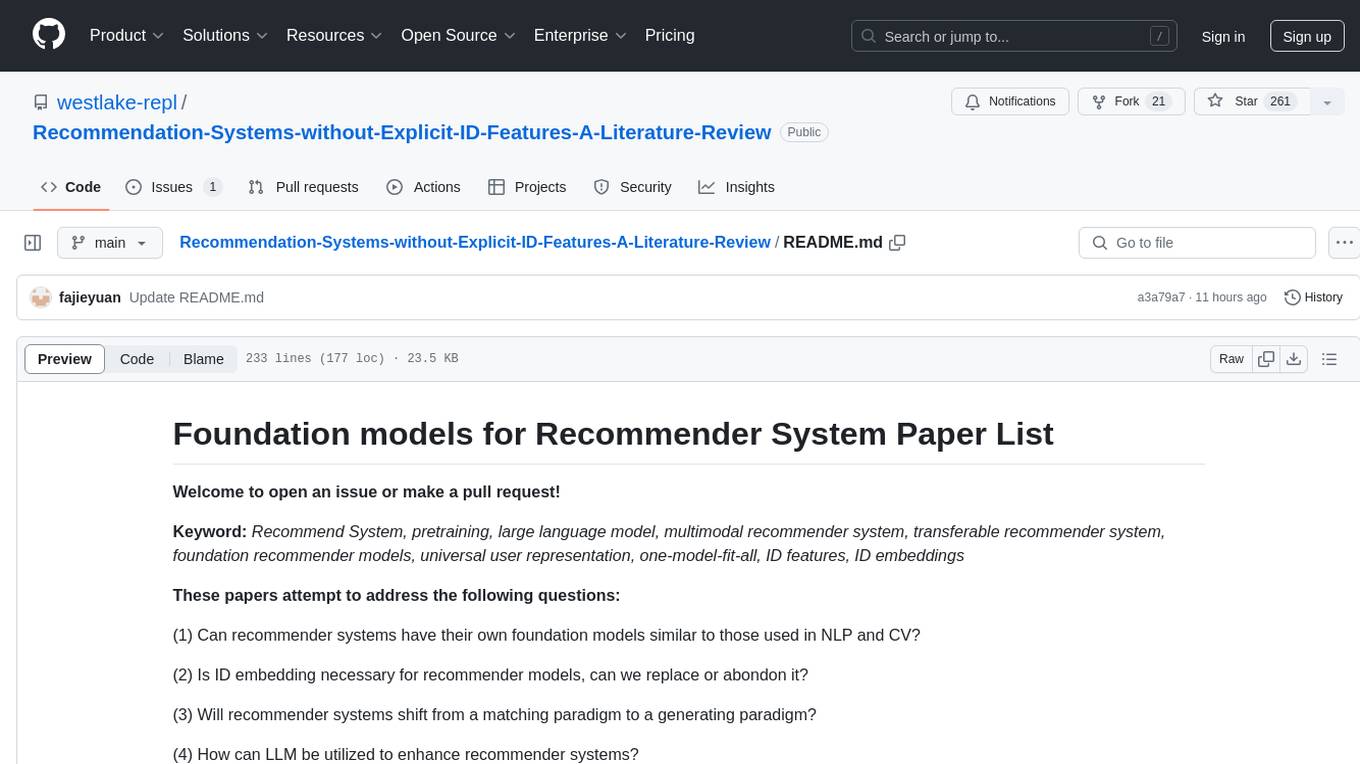
Recommendation-Systems-without-Explicit-ID-Features-A-Literature-Review
This repository is a collection of papers and resources related to recommendation systems, focusing on foundation models, transferable recommender systems, large language models, and multimodal recommender systems. It explores questions such as the necessity of ID embeddings, the shift from matching to generating paradigms, and the future of multimodal recommender systems. The papers cover various aspects of recommendation systems, including pretraining, user representation, dataset benchmarks, and evaluation methods. The repository aims to provide insights and advancements in the field of recommendation systems through literature reviews, surveys, and empirical studies.
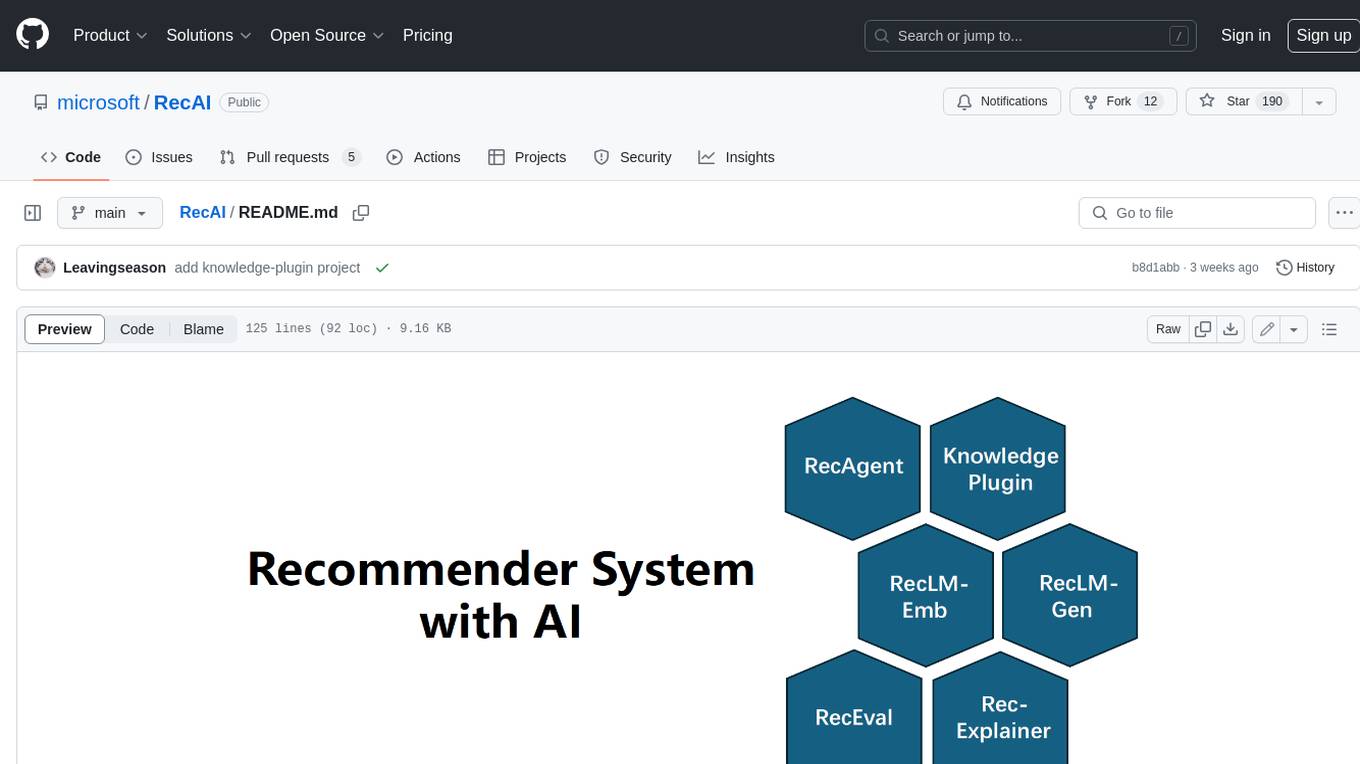
RecAI
RecAI is a project that explores the integration of Large Language Models (LLMs) into recommender systems, addressing the challenges of interactivity, explainability, and controllability. It aims to bridge the gap between general-purpose LLMs and domain-specific recommender systems, providing a holistic perspective on the practical requirements of LLM4Rec. The project investigates various techniques, including Recommender AI agents, selective knowledge injection, fine-tuning language models, evaluation, and LLMs as model explainers, to create more sophisticated, interactive, and user-centric recommender systems.
For similar jobs
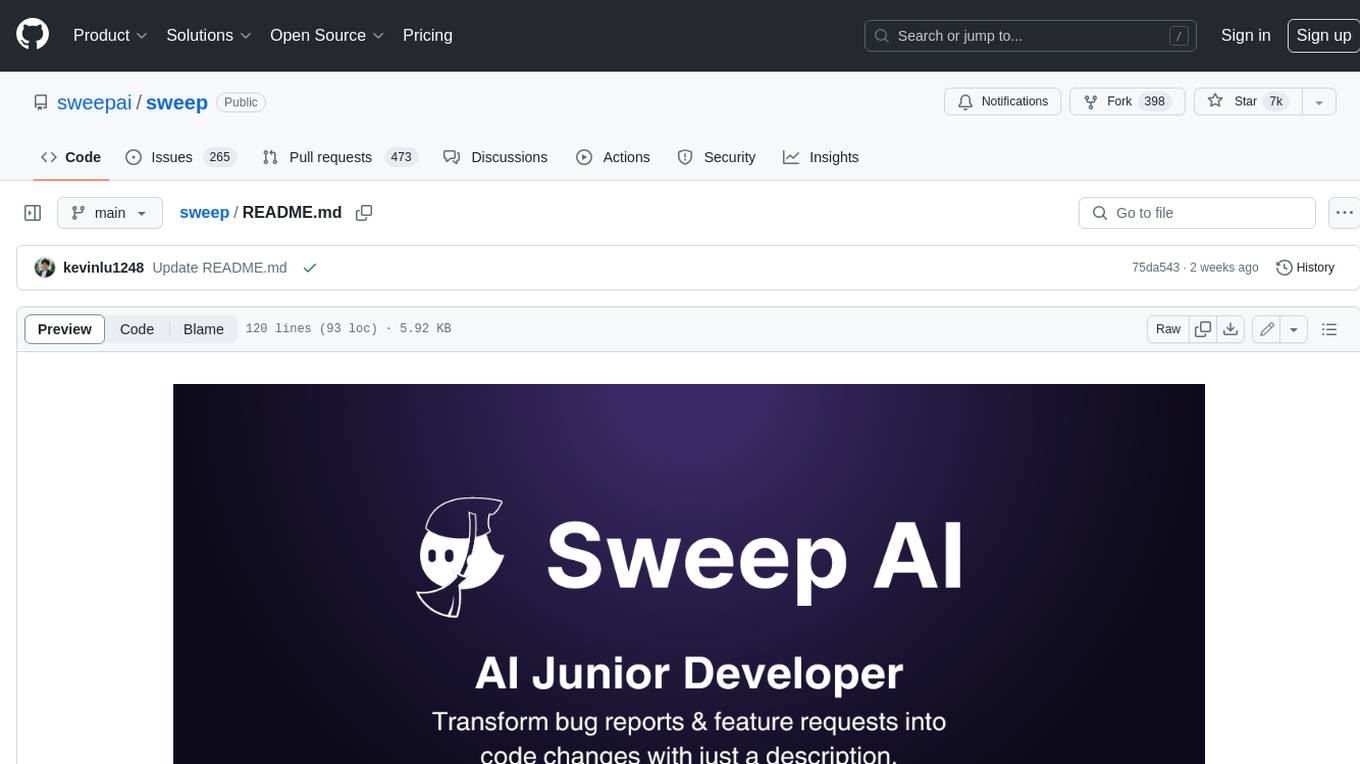
sweep
Sweep is an AI junior developer that turns bugs and feature requests into code changes. It automatically handles developer experience improvements like adding type hints and improving test coverage.
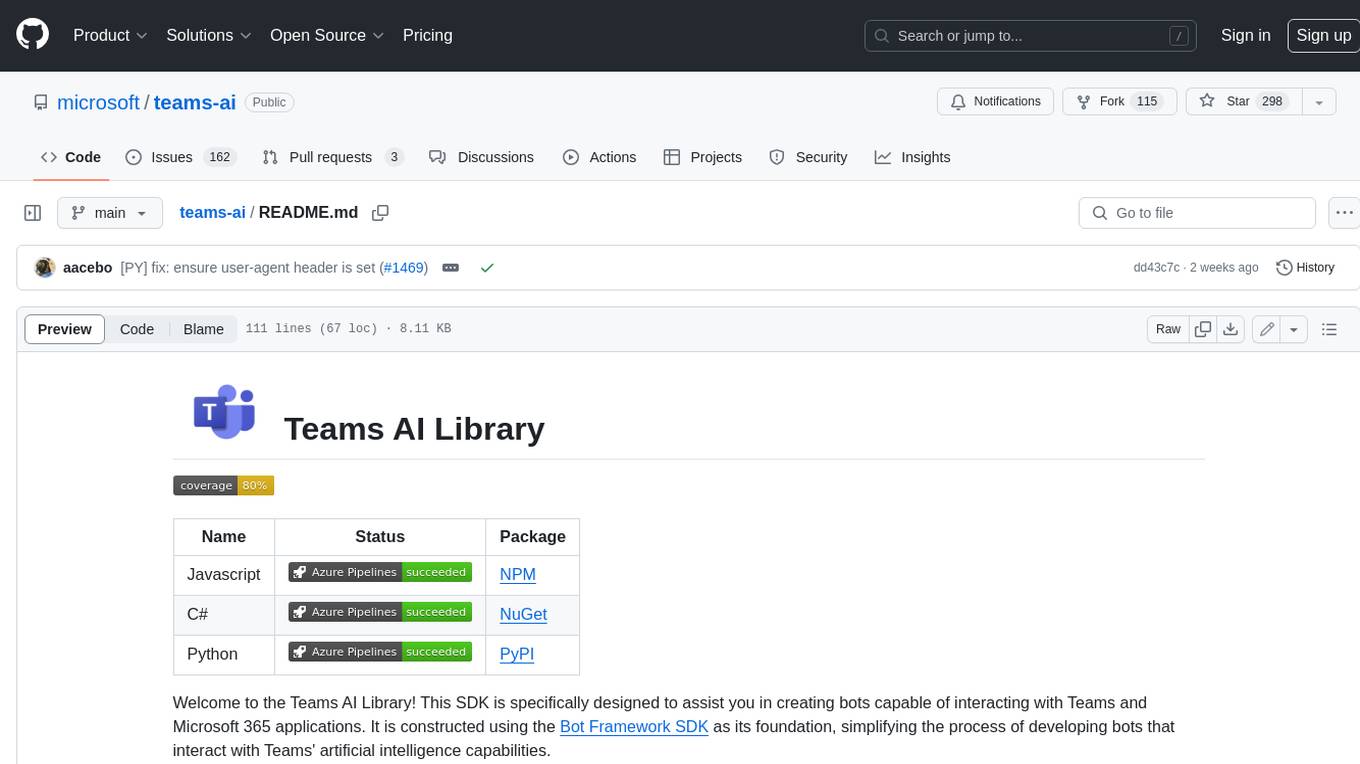
teams-ai
The Teams AI Library is a software development kit (SDK) that helps developers create bots that can interact with Teams and Microsoft 365 applications. It is built on top of the Bot Framework SDK and simplifies the process of developing bots that interact with Teams' artificial intelligence capabilities. The SDK is available for JavaScript/TypeScript, .NET, and Python.
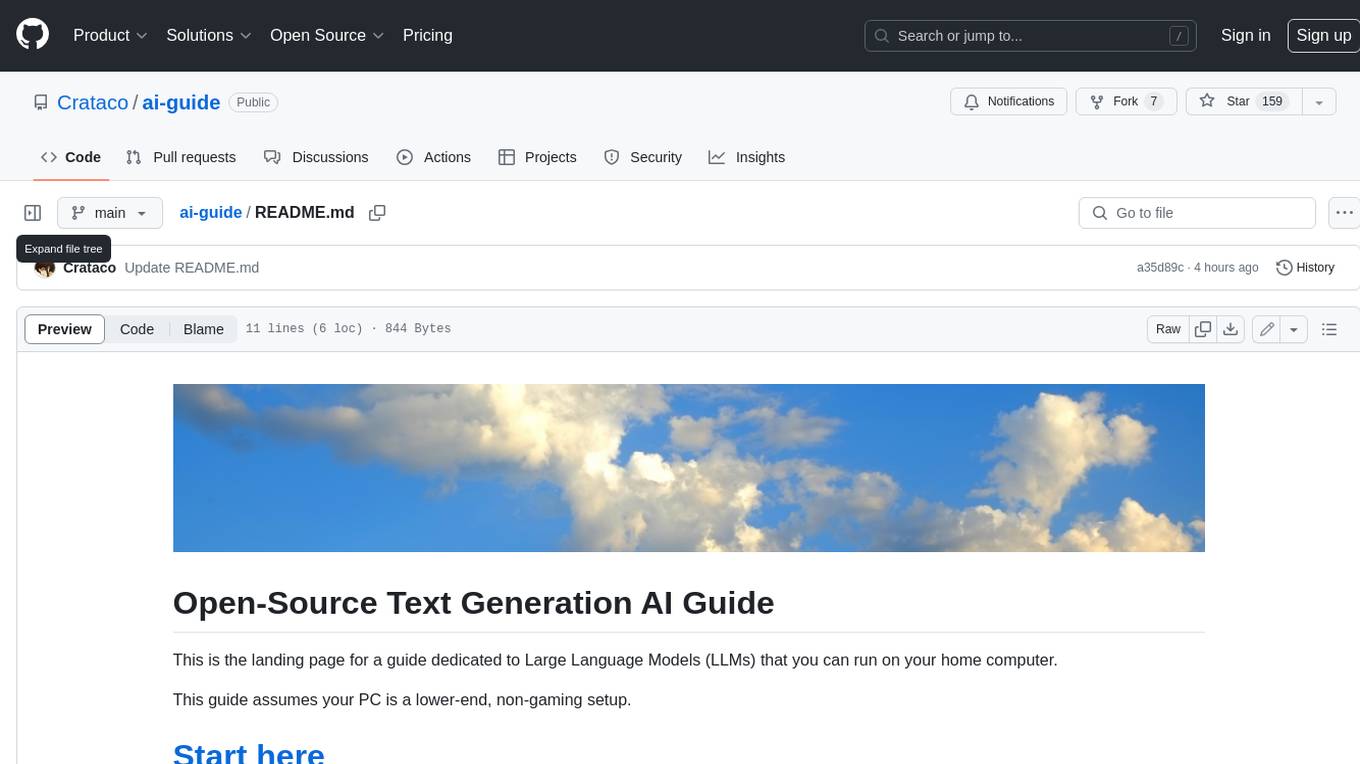
ai-guide
This guide is dedicated to Large Language Models (LLMs) that you can run on your home computer. It assumes your PC is a lower-end, non-gaming setup.
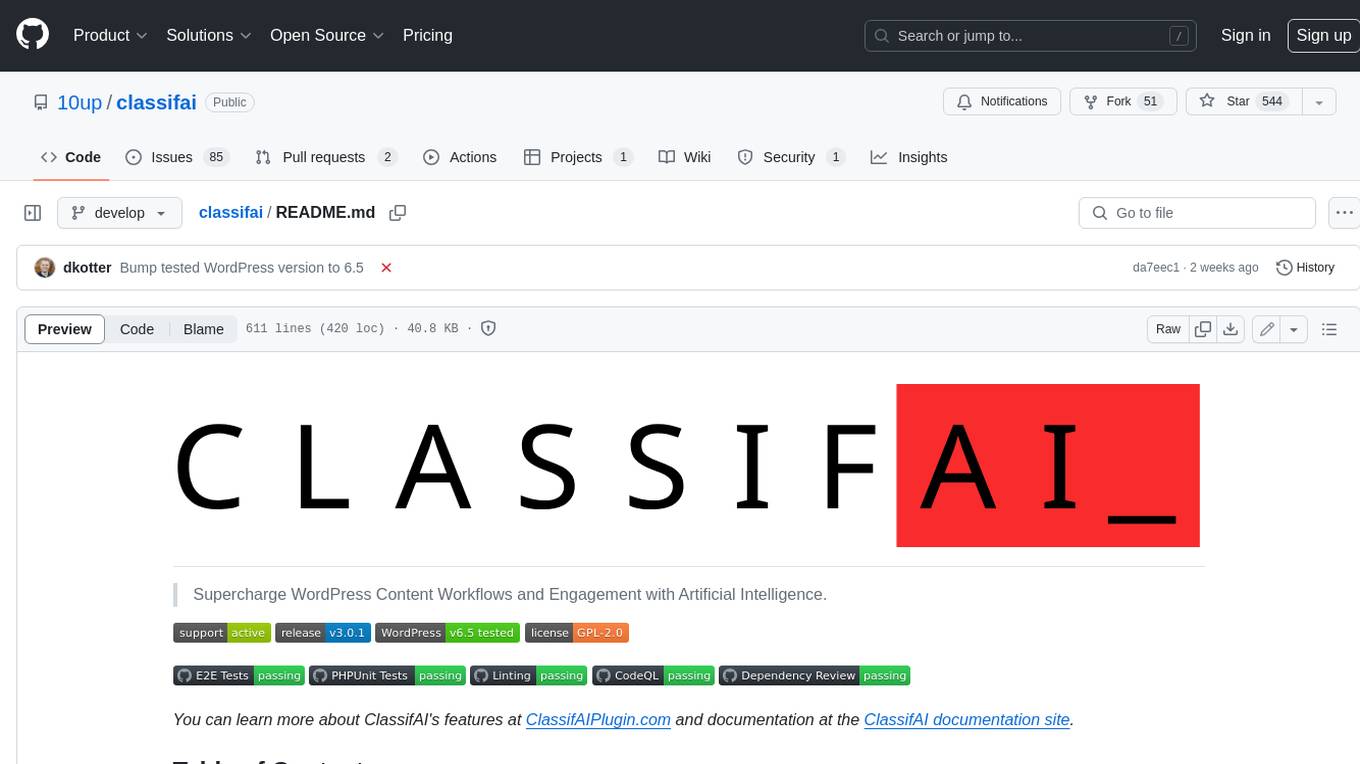
classifai
Supercharge WordPress Content Workflows and Engagement with Artificial Intelligence. Tap into leading cloud-based services like OpenAI, Microsoft Azure AI, Google Gemini and IBM Watson to augment your WordPress-powered websites. Publish content faster while improving SEO performance and increasing audience engagement. ClassifAI integrates Artificial Intelligence and Machine Learning technologies to lighten your workload and eliminate tedious tasks, giving you more time to create original content that matters.
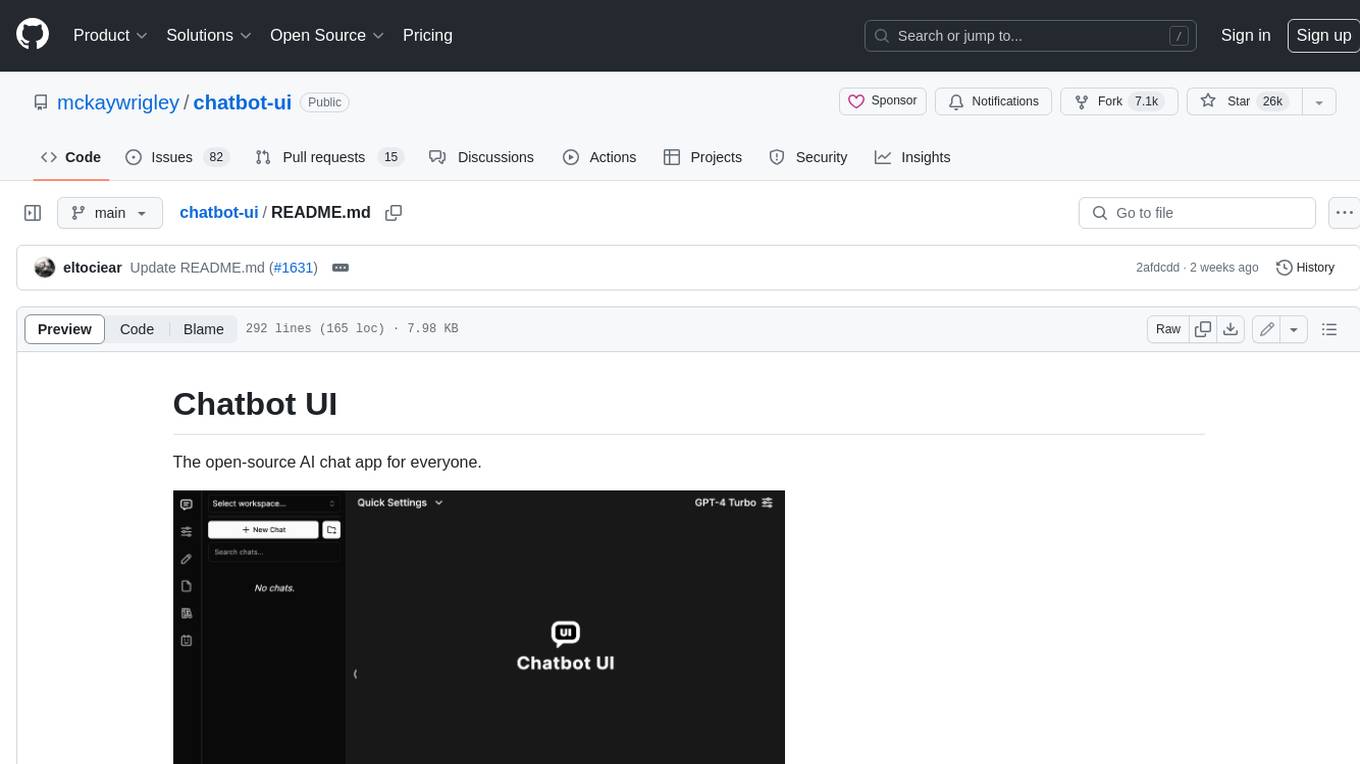
chatbot-ui
Chatbot UI is an open-source AI chat app that allows users to create and deploy their own AI chatbots. It is easy to use and can be customized to fit any need. Chatbot UI is perfect for businesses, developers, and anyone who wants to create a chatbot.
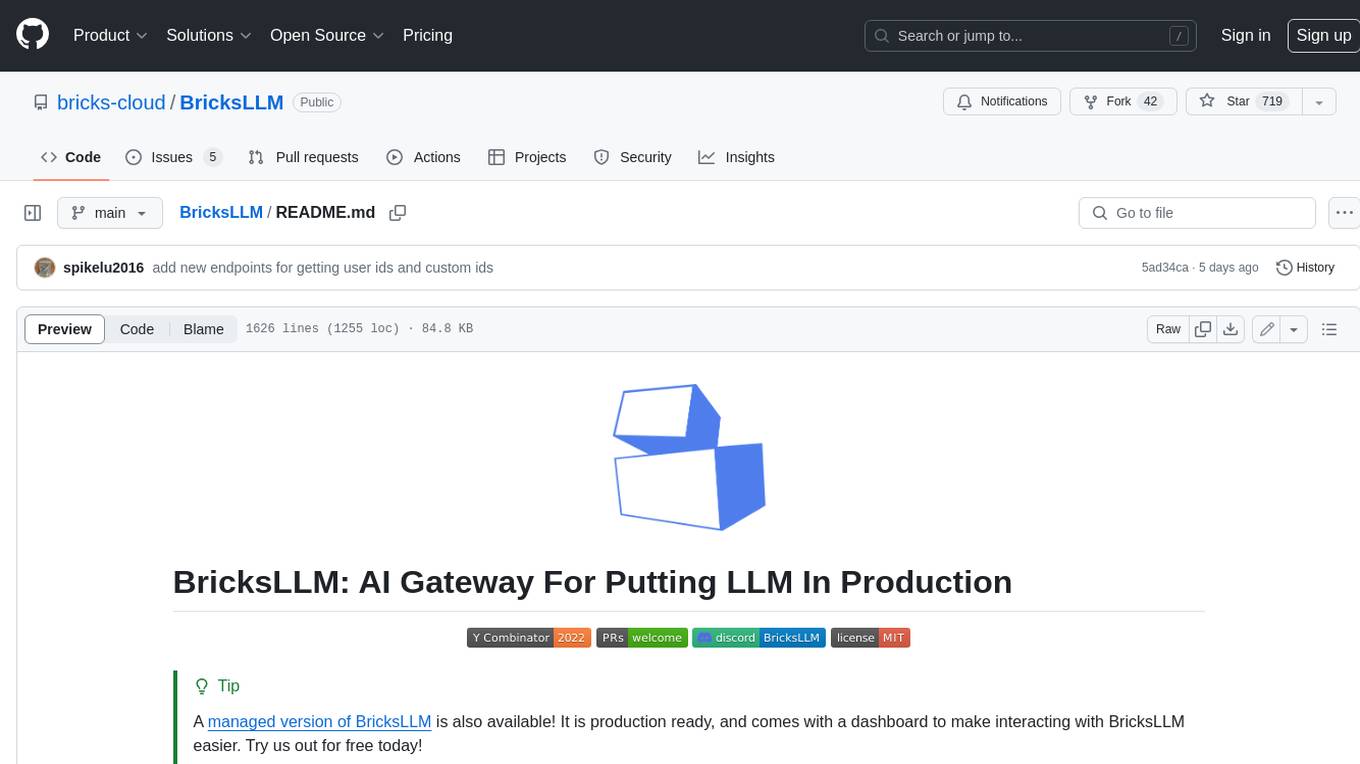
BricksLLM
BricksLLM is a cloud native AI gateway written in Go. Currently, it provides native support for OpenAI, Anthropic, Azure OpenAI and vLLM. BricksLLM aims to provide enterprise level infrastructure that can power any LLM production use cases. Here are some use cases for BricksLLM: * Set LLM usage limits for users on different pricing tiers * Track LLM usage on a per user and per organization basis * Block or redact requests containing PIIs * Improve LLM reliability with failovers, retries and caching * Distribute API keys with rate limits and cost limits for internal development/production use cases * Distribute API keys with rate limits and cost limits for students
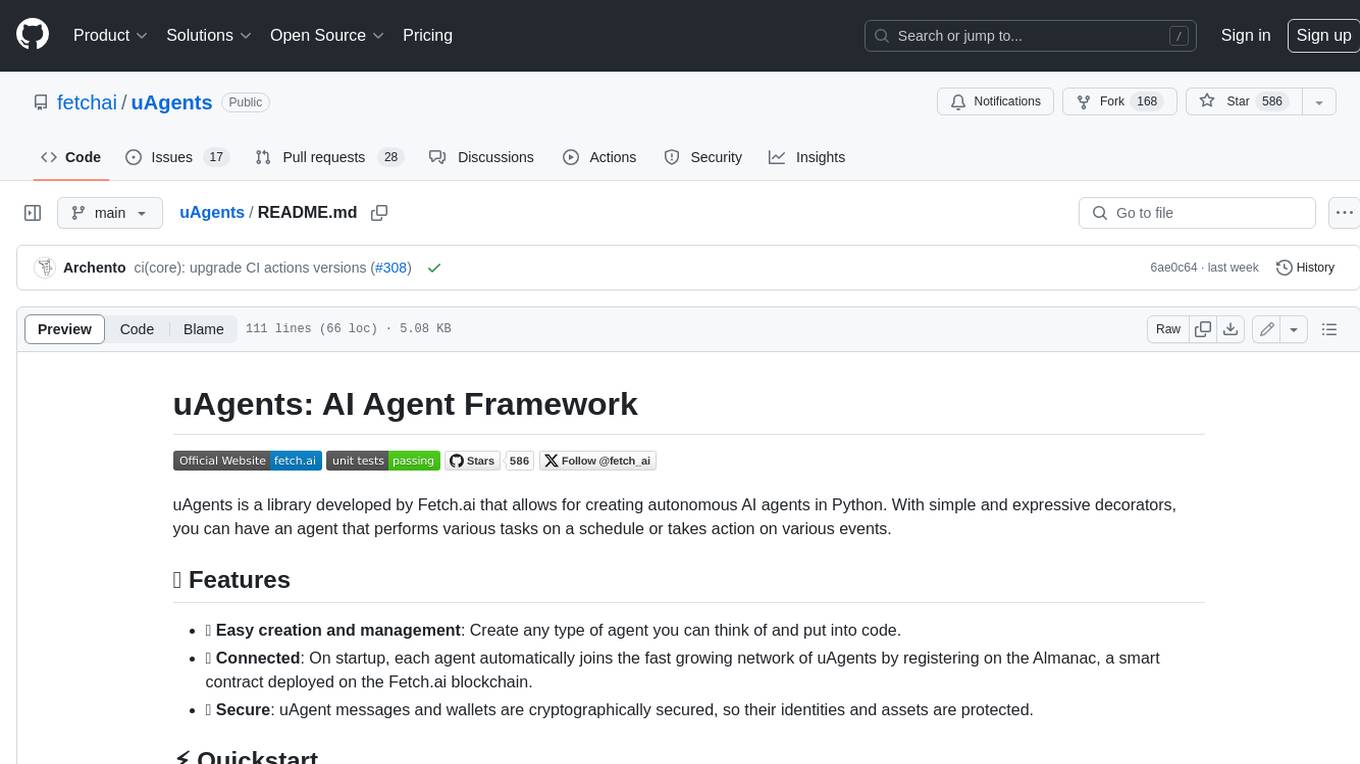
uAgents
uAgents is a Python library developed by Fetch.ai that allows for the creation of autonomous AI agents. These agents can perform various tasks on a schedule or take action on various events. uAgents are easy to create and manage, and they are connected to a fast-growing network of other uAgents. They are also secure, with cryptographically secured messages and wallets.
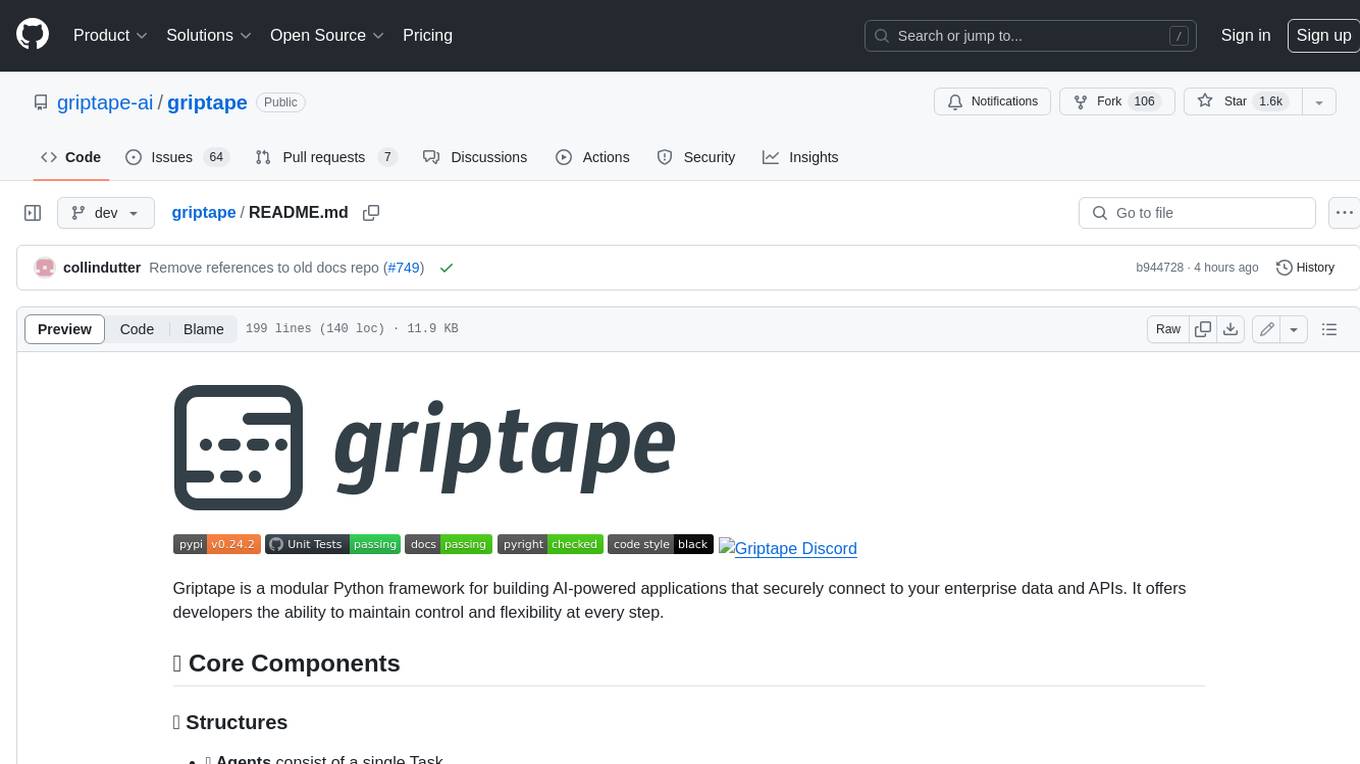
griptape
Griptape is a modular Python framework for building AI-powered applications that securely connect to your enterprise data and APIs. It offers developers the ability to maintain control and flexibility at every step. Griptape's core components include Structures (Agents, Pipelines, and Workflows), Tasks, Tools, Memory (Conversation Memory, Task Memory, and Meta Memory), Drivers (Prompt and Embedding Drivers, Vector Store Drivers, Image Generation Drivers, Image Query Drivers, SQL Drivers, Web Scraper Drivers, and Conversation Memory Drivers), Engines (Query Engines, Extraction Engines, Summary Engines, Image Generation Engines, and Image Query Engines), and additional components (Rulesets, Loaders, Artifacts, Chunkers, and Tokenizers). Griptape enables developers to create AI-powered applications with ease and efficiency.