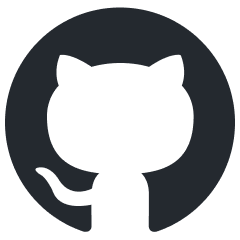
Autono
A ReAct-Based Highly Robust Autonomous Agent Framework
Stars: 191
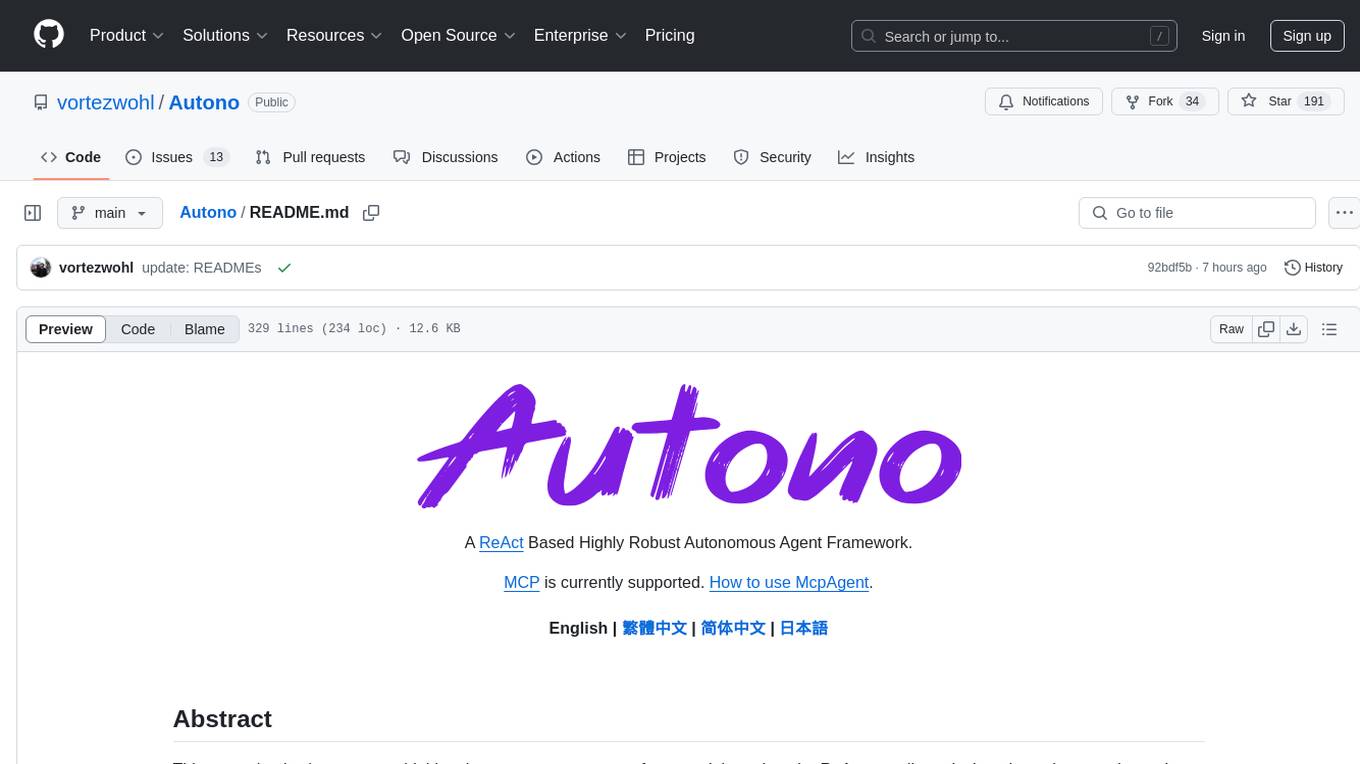
README:
A ReAct Based Highly Robust Autonomous Agent Framework.
MCP is currently supported. How to use McpAgent.
This paper (project) proposes a highly robust autonomous agent framework based on the ReAct paradigm, designed to solve complex tasks through adaptive decision making and multi-agent collaboration. Unlike traditional frameworks that rely on fixed workflows generated by LLM-based planners, this framework dynamically generates next actions during agent execution based on prior trajectories, thereby enhancing its robustness. To address potential termination issues caused by adaptive execution paths, I propose a timely abandonment strategy incorporating a probabilistic penalty mechanism. For multi-agent collaboration, I introduce a memory transfer mechanism that enables shared and dynamically updated memory among agents. The framework's innovative timely abandonment strategy dynamically adjusts the probability of task abandonment via probabilistic penalties, allowing developers to balance conservative and exploratory tendencies in agent execution strategies by tuning hyperparameters. This significantly improves adaptability and task execution efficiency in complex environments. Additionally, agents can be extended through external tool integration, supported by modular design and MCP protocol compatibility, which enables flexible action space expansion. Through explicit division of labor, the multi-agent collaboration mechanism enables agents to focus on specific task components, thereby significantly improving execution efficiency and quality.
The experimental results demonstrate that the autono
framework significantly outperforms autogen
and langchain
in handling tasks of varying complexity, especially in multi-step tasks with possible failures.
Framework | Version | Model | one-step-task | multi-step-task | multi-step-task-with-possible-failure |
---|---|---|---|---|---|
autono |
1.0.0 |
gpt-4o-mini qwen-plus deepseek-v3 |
96.7%100%100% | 100%96.7%100% | 76.7%93.3%93.3% |
autogen |
0.4.9.2 |
gpt-4o-mini qwen-plus deepseek-v3 |
90%90%N/A | 53.3%0%N/A | 3.3%3.3%N/A |
langchain |
0.3.21 |
gpt-4o-mini qwen-plus deepseek-v3 |
73.3%73.3%76.7% | 13.3%13.3%13.3% | 10%13.3%6.7% |
-
one-step-task
: Tasks that can be completed with a single tool call. -
multi-step-task
: Tasks that require multiple tool calls to complete, with no possibility of tool failure. -
multi-step-task-with-possible-failure
: Tasks that require multiple tool calls to complete, where tools may fail, requiring the agent to retry and correct errors.
The deepseek-v3 model is not supported by
autogen-agentchat==0.4.9.2
.
You can reproduce my experiments here.
If you are incorporating the autono
framework into your research, please remember to properly cite it to acknowledge its contribution to your work.
Если вы интегрируете фреймворк autono
в своё исследование, пожалуйста, не забудьте правильно сослаться на него, указывая его вклад в вашу работу.
もしあなたが研究に autono
フレームワークを組み入れているなら、その貢献を認めるために適切に引用することを忘れないでください.
如果您正在將 autono
框架整合到您的研究中,請務必正確引用它,以聲明它對您工作的貢獻.
@software{Wu_Autono_2025,
author = {Wu, Zihao},
license = {GPL-3.0},
month = apr,
title = {{Autono}},
url = {https://github.com/vortezwohl/Autono},
version = {1.0.0},
year = {2025}
}
-
From PYPI
pip install -U autono
-
From Github
Get access to unreleased features.
pip install git+https://github.com/vortezwohl/Autono.git
To start building your own agent, follow the steps listed.
-
set environmental variable
OPENAI_API_KEY
# .env OPENAI_API_KEY=sk-...
-
import required dependencies
-
Agent
lets you instantiate an agent. -
Personality
is an enumeration class used for customizing personalities of agents.-
Personality.PRUDENT
makes the agent's behavior more cautious. -
Personality.INQUISITIVE
encourages the agent to be more proactive in trying and exploring.
-
-
get_openai_model
gives you aBaseChatModel
as thought engine. -
@ability(brain: BaseChatModel, cache: bool = True, cache_dir: str = '')
is a decorator which lets you declare a function as anAbility
. -
@agentic(agent: Agent)
is a decorator which lets you declare a function as anAgenticAbility
.
from autono import ( Agent, Personality, get_openai_model, ability, agentic )
-
-
declare functions as basic abilities
@ability def calculator(expr: str) -> float: # this function only accepts a single math expression return simplify(expr) @ability def write_file(filename: str, content: str) -> str: with open(filename, 'w', encoding='utf-8') as f: f.write(content) return f'{content} written to {filename}.'
-
instantiate an agent
You can grant abilities to agents while instantiating them.
model = get_openai_model() agent = Agent(abilities=[calculator, write_file], brain=model, name='Autono', personality=Personality.INQUISITIVE)
-
You can also grant more abilities to agents later:
agent.grant_ability(calculator)
or
agent.grant_abilities([calculator])
-
To deprive abilities:
agent.deprive_ability(calculator)
or
agent.deprive_abilities([calculator])
You can change an agent's personality using method
change_personality(personality: Personality)
agent.change_personality(Personality.PRUDENT)
-
-
assign a request to your agent
agent.assign("Here is a sphere with radius of 9.5 cm and pi here is 3.14159, find the area and volume respectively then write the results into a file called 'result.txt'.")
-
leave the rest to your agent
response = agent.just_do_it() print(response)
autono
also supports multi-agent collaboration scenario, declare a function as agent calling ability with@agentic(agent: Agent)
, then grant it to an agent. See example.
Integration with MCP
I provide McpAgent
to support tool calls based on the MCP protocol. Below is a brief guide to integrating McpAgent
with mcp.stdio_client
:
-
import required dependencies
-
McpAgent
allows you to instantiate an agent capable of accessing MCP tools. -
StdioMcpConfig
is an alias formcp.client.stdio.StdioServerParameters
and serves as the MCP server connection configuration. -
@mcp_session(mcp_config: StdioMcpConfig)
allows you to declare a function as an MCP session. -
sync_call
allows you to synchronizedly call a coroutine function.
from autono import ( McpAgent, get_openai_model, StdioMcpConfig, mcp_session, sync_call )
-
-
create an MCP session
-
To connect with a stdio based MCP server, use
StdioMcpConfig
.mcp_config = StdioMcpConfig( command='python', args=['./my_stdio_mcp_server.py'], env=dict(), cwd='./mcp_servers' )
A function decorated with
@mcp_session
will receive an MCP session instance as its first parameter. A function can be decorated with multiple@mcp_session
decorators to access sessions for different MCP servers.@sync_call @mcp_session(mcp_config) async def run(session, request: str) -> str: ...
-
To connect via HTTP with a SSE based MCP server, just provide the URL.
@sync_call @mcp_session('http://localhost:8000/sse') async def run(session, request: str) -> str: ...
-
To connect via websocket with a WS based MCP server, provide the URL.
@sync_call @mcp_session('ws://localhost:8000/message') async def run(session, request: str) -> str: ...
-
-
create an
McpAgent
instance within the MCP sessionAfter creating
McpAgent
, you need to call thefetch_abilities()
method to retrieve tool configurations from the MCP server.@sync_call @mcp_session(mcp_config) async def run(session, request: str) -> str: mcp_agent = await McpAgent(session=session, brain=get_openai_model()).fetch_abilities() ...
-
assign tasks to the
McpAgent
instance and await execution result@sync_call @mcp_session(mcp_config) async def run(session, request: str) -> str: mcp_agent = await McpAgent(session=session, brain=get_openai_model()).fetch_abilities() result = await mcp_agent.assign(request).just_do_it() return result.conclusion
-
call the function
if __name__ == '__main__': ret = run(request='What can you do?') print(ret)
I also provide the complete MCP agent test script. See example.
To make the working process of agents observable, I provide two hooks, namely BeforeActionTaken
and AfterActionTaken
.
They allow you to observe and intervene in the decision-making and execution results of each step of the agent's actions.
You can obtain and modify the agent's decision results for the next action through the BeforeActionTaken
hook,
while AfterActionTaken
allows you to obtain and modify the execution results of the actions (the tampered execution results will be part of the agent's memory).
To start using hooks, follow the steps listed.
-
bring in hooks and messages from
autono
from autono.brain.hook import BeforeActionTaken, AfterActionTaken from autono.message import BeforeActionTakenMessage, AfterActionTakenMessage
-
declare functions and encapsulate them as hooks
def before_action_taken(agent: Agent, message: BeforeActionTakenMessage): print(f'Agent: {agent.name}, Next move: {message}') return message def after_action_taken(agent: Agent, message: AfterActionTakenMessage): print(f'Agent: {agent.name}, Action taken: {message}') return message before_action_taken_hook = BeforeActionTaken(before_action_taken) after_action_taken_hook = AfterActionTaken(after_action_taken)
In these two hook functions, you intercepted the message and printed the information in the message. Afterwards, you returned the message unaltered to the agent. Of course, you also have the option to modify the information in the message, thereby achieving intervention in the agent's working process.
-
use hooks during the agent's working process
agent.assign(...).just_do_it(before_action_taken_hook, after_action_taken_hook)
For Tasks:
Click tags to check more tools for each tasksFor Jobs:
Alternative AI tools for Autono
Similar Open Source Tools
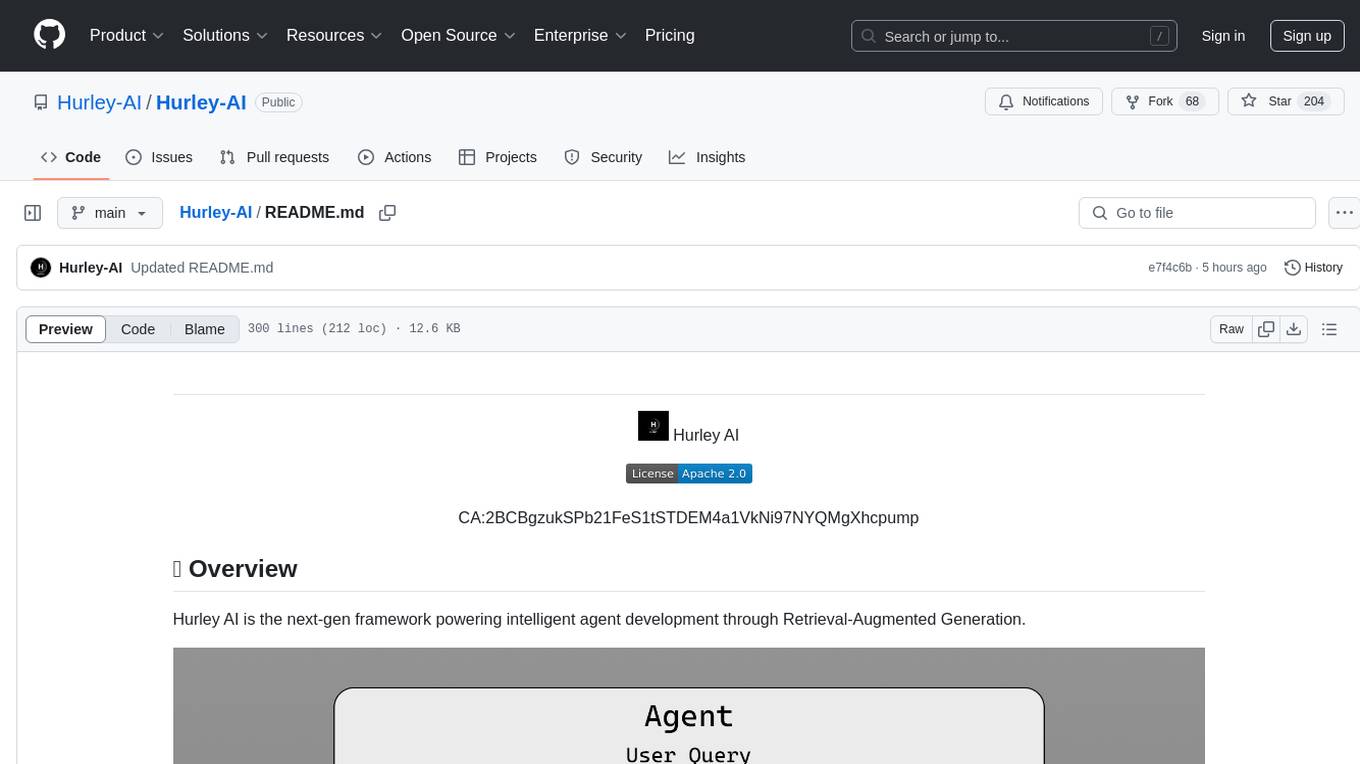
Hurley-AI
Hurley AI is a next-gen framework for developing intelligent agents through Retrieval-Augmented Generation. It enables easy creation of custom AI assistants and agents, supports various agent types, and includes pre-built tools for domains like finance and legal. Hurley AI integrates with LLM inference services and provides observability with Arize Phoenix. Users can create Hurley RAG tools with a single line of code and customize agents with specific instructions. The tool also offers various helper functions to connect with Hurley RAG and search tools, along with pre-built tools for tasks like summarizing text, rephrasing text, understanding memecoins, and querying databases.
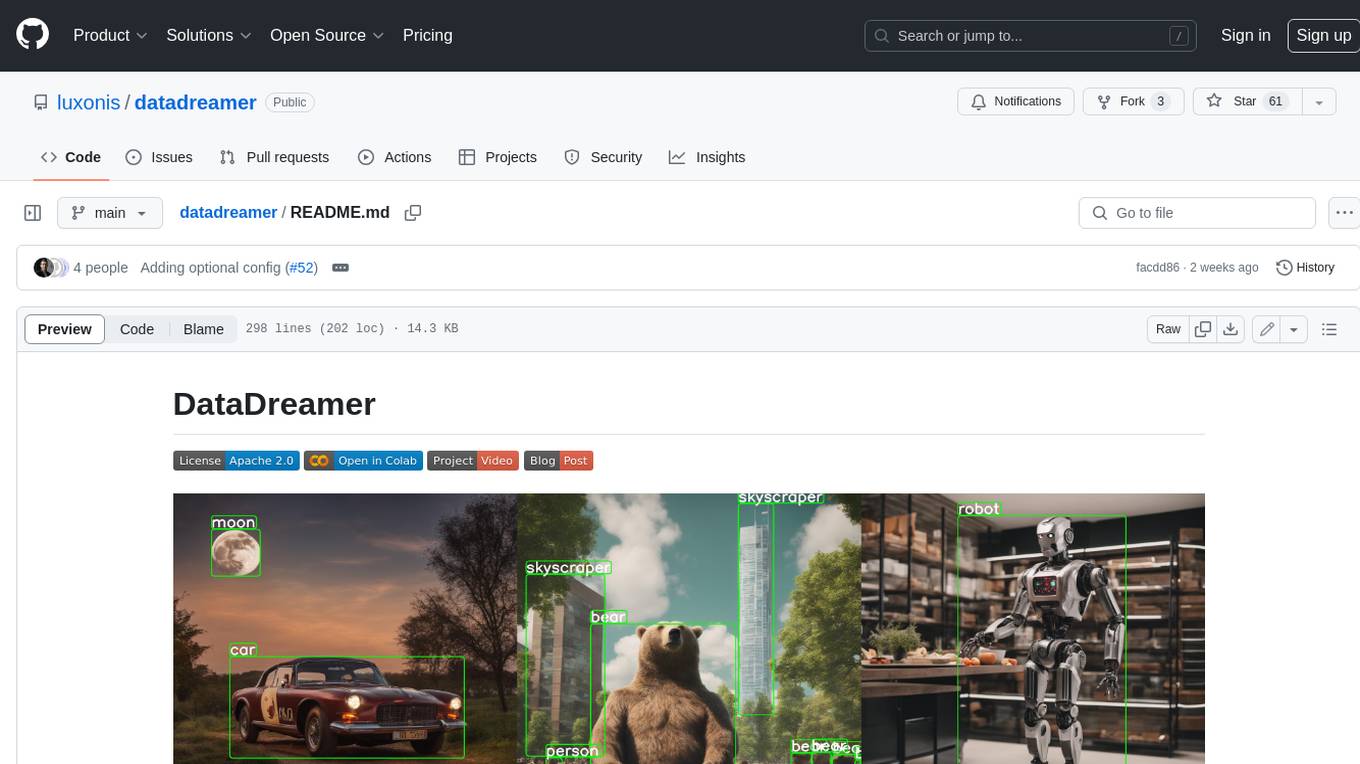
datadreamer
DataDreamer is an advanced toolkit designed to facilitate the development of edge AI models by enabling synthetic data generation, knowledge extraction from pre-trained models, and creation of efficient and potent models. It eliminates the need for extensive datasets by generating synthetic datasets, leverages latent knowledge from pre-trained models, and focuses on creating compact models suitable for integration into any device and performance for specialized tasks. The toolkit offers features like prompt generation, image generation, dataset annotation, and tools for training small-scale neural networks for edge deployment. It provides hardware requirements, usage instructions, available models, and limitations to consider while using the library.
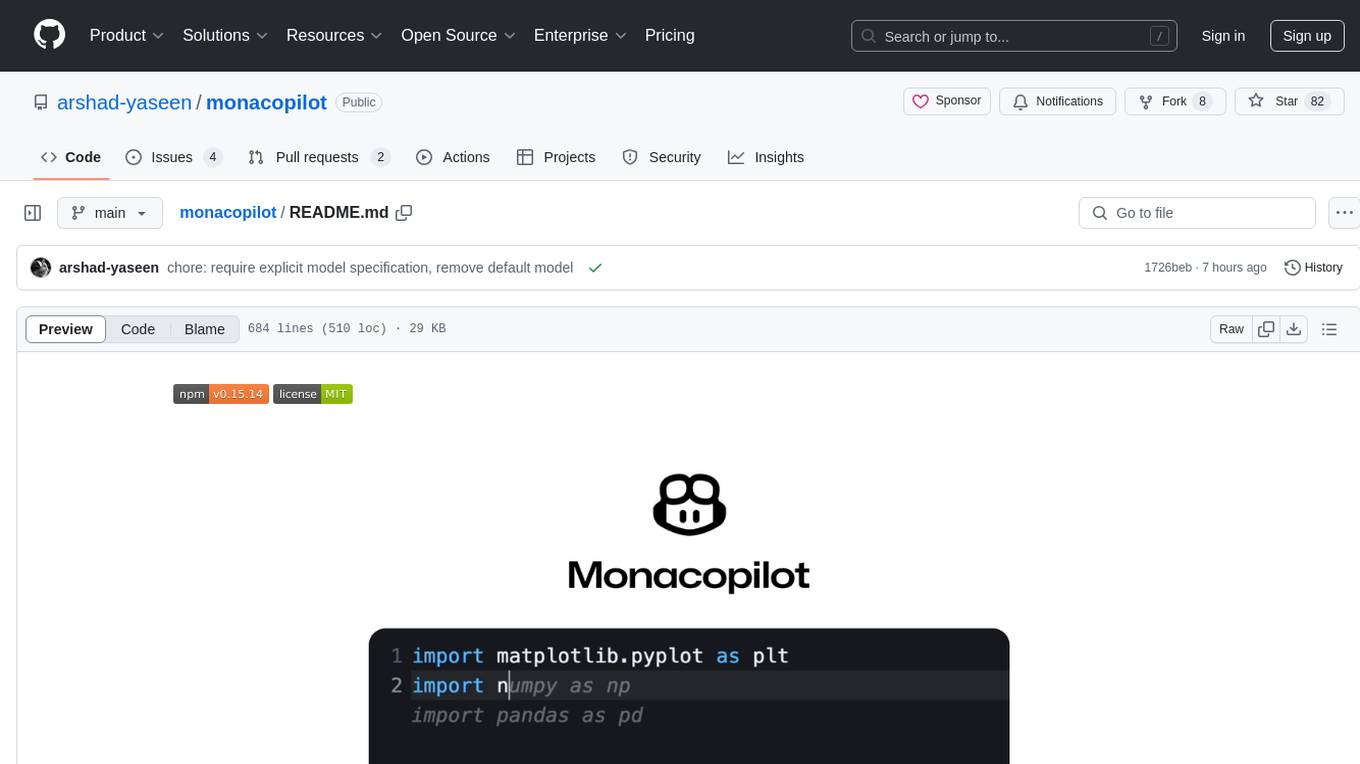
monacopilot
Monacopilot is a powerful and customizable AI auto-completion plugin for the Monaco Editor. It supports multiple AI providers such as Anthropic, OpenAI, Groq, and Google, providing real-time code completions with an efficient caching system. The plugin offers context-aware suggestions, customizable completion behavior, and framework agnostic features. Users can also customize the model support and trigger completions manually. Monacopilot is designed to enhance coding productivity by providing accurate and contextually appropriate completions in daily spoken language.
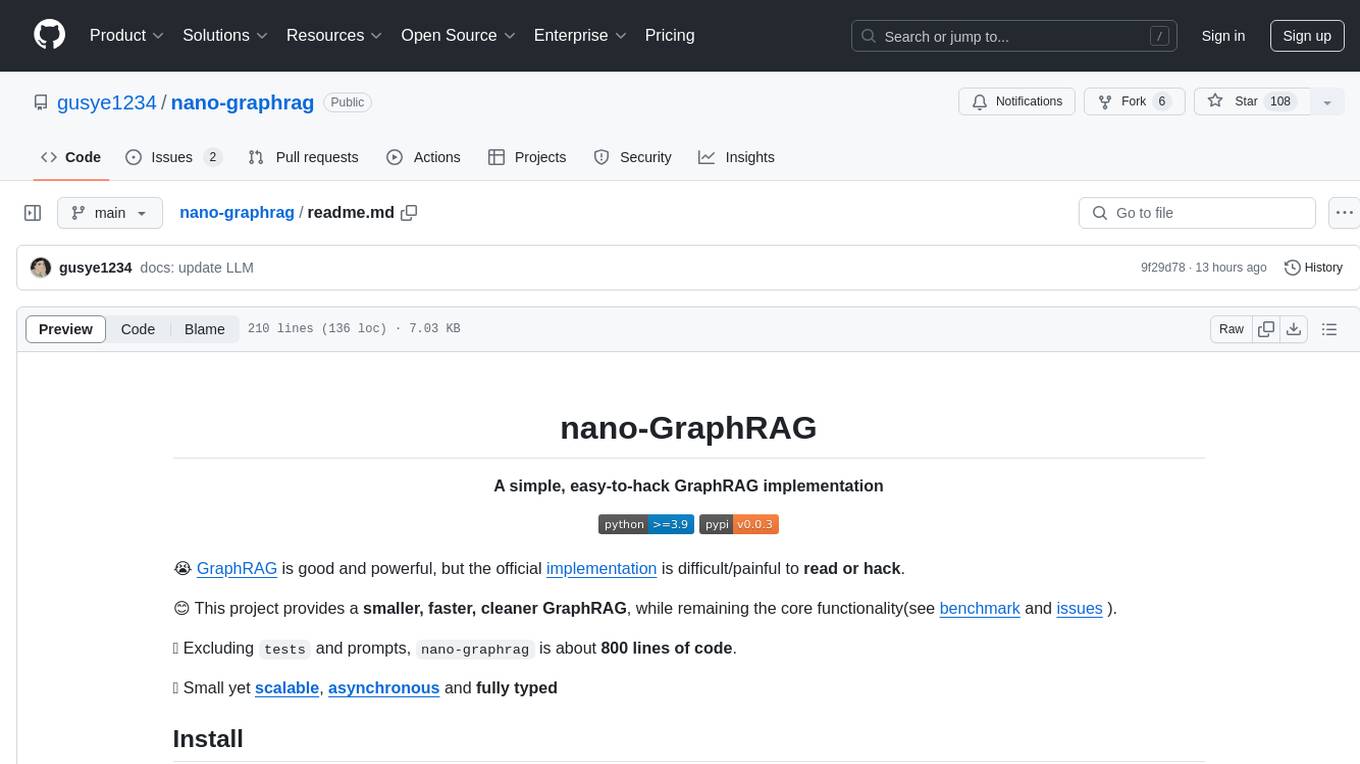
nano-graphrag
nano-GraphRAG is a simple, easy-to-hack implementation of GraphRAG that provides a smaller, faster, and cleaner version of the official implementation. It is about 800 lines of code, small yet scalable, asynchronous, and fully typed. The tool supports incremental insert, async methods, and various parameters for customization. Users can replace storage components and LLM functions as needed. It also allows for embedding function replacement and comes with pre-defined prompts for entity extraction and community reports. However, some features like covariates and global search implementation differ from the original GraphRAG. Future versions aim to address issues related to data source ID, community description truncation, and add new components.
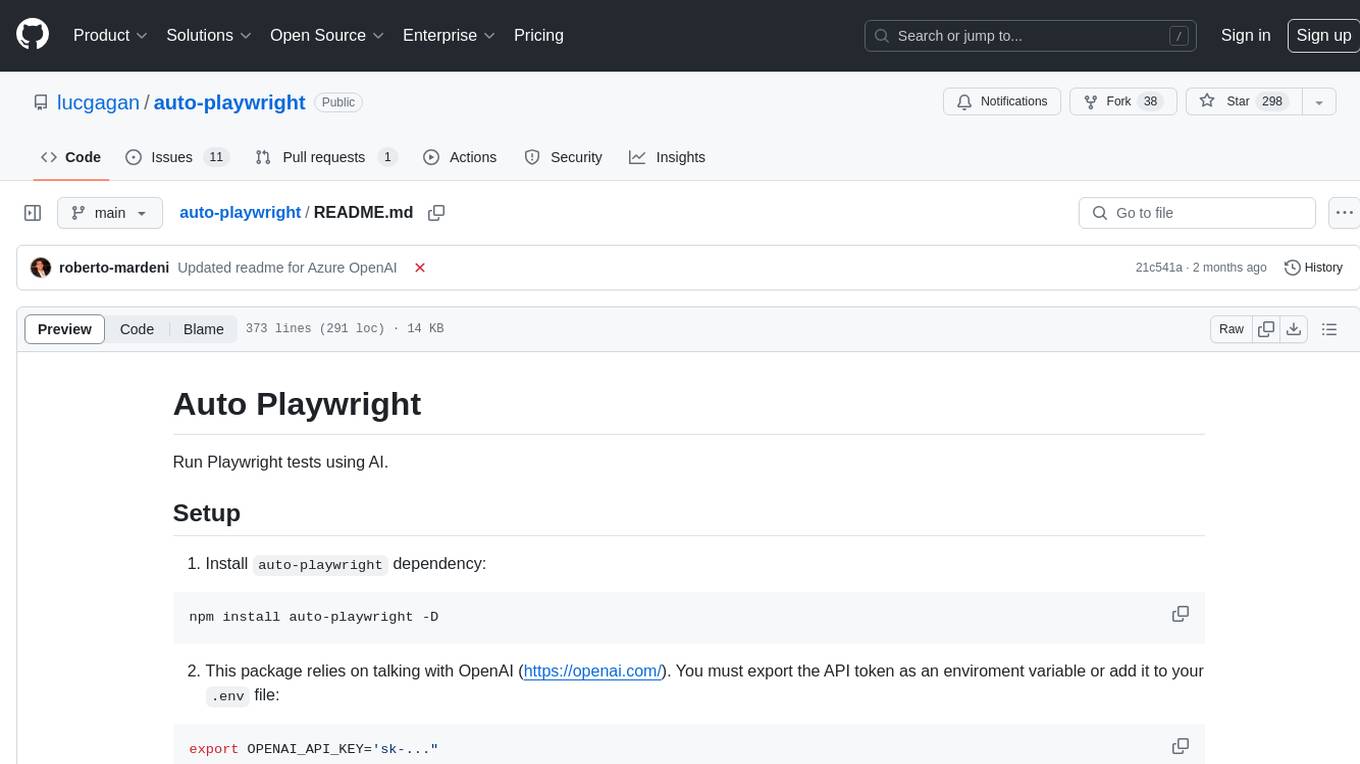
auto-playwright
Auto Playwright is a tool that allows users to run Playwright tests using AI. It eliminates the need for selectors by determining actions at runtime based on plain-text instructions. Users can automate complex scenarios, write tests concurrently with or before functionality development, and benefit from rapid test creation. The tool supports various Playwright actions and offers additional options for debugging and customization. It uses HTML sanitization to reduce costs and improve text quality when interacting with the OpenAI API.
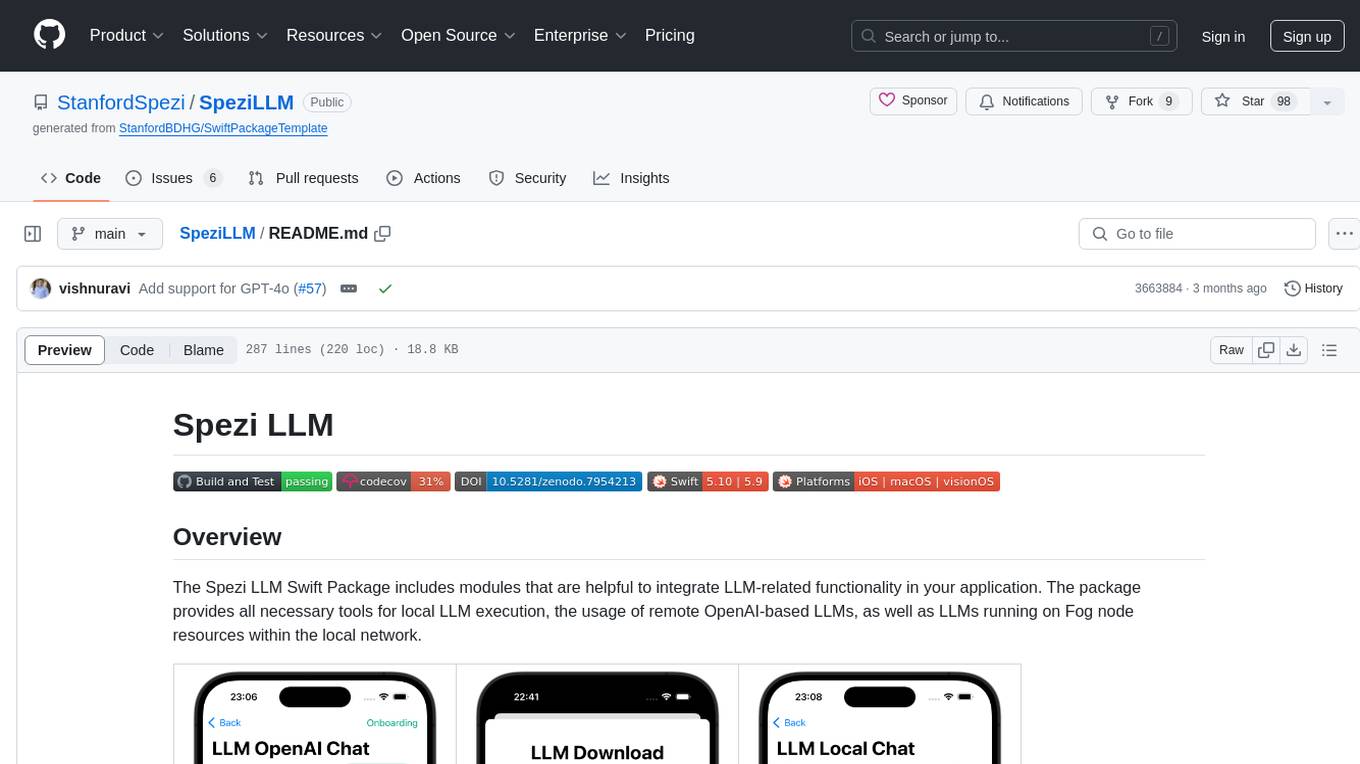
SpeziLLM
The Spezi LLM Swift Package includes modules that help integrate LLM-related functionality in applications. It provides tools for local LLM execution, usage of remote OpenAI-based LLMs, and LLMs running on Fog node resources within the local network. The package contains targets like SpeziLLM, SpeziLLMLocal, SpeziLLMLocalDownload, SpeziLLMOpenAI, and SpeziLLMFog for different LLM functionalities. Users can configure and interact with local LLMs, OpenAI LLMs, and Fog LLMs using the provided APIs and platforms within the Spezi ecosystem.
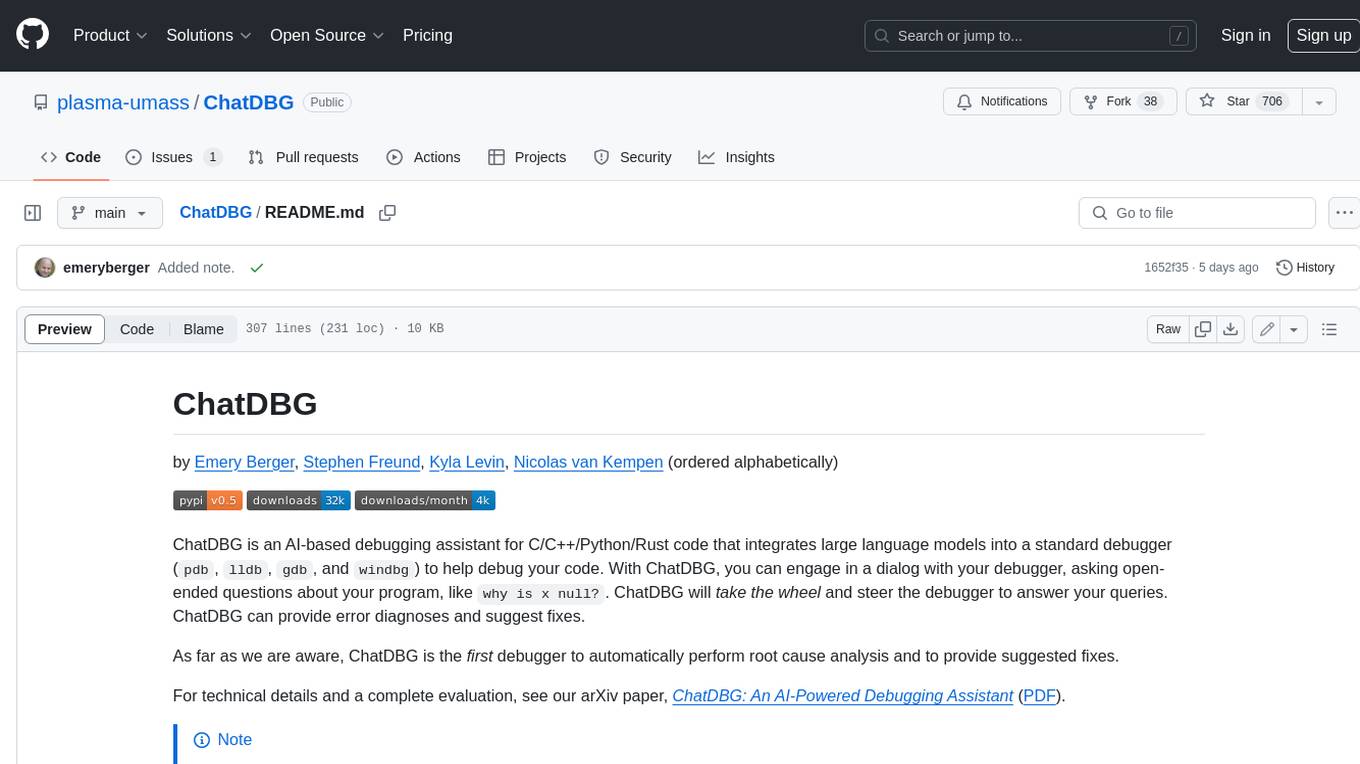
ChatDBG
ChatDBG is an AI-based debugging assistant for C/C++/Python/Rust code that integrates large language models into a standard debugger (`pdb`, `lldb`, `gdb`, and `windbg`) to help debug your code. With ChatDBG, you can engage in a dialog with your debugger, asking open-ended questions about your program, like `why is x null?`. ChatDBG will _take the wheel_ and steer the debugger to answer your queries. ChatDBG can provide error diagnoses and suggest fixes. As far as we are aware, ChatDBG is the _first_ debugger to automatically perform root cause analysis and to provide suggested fixes.
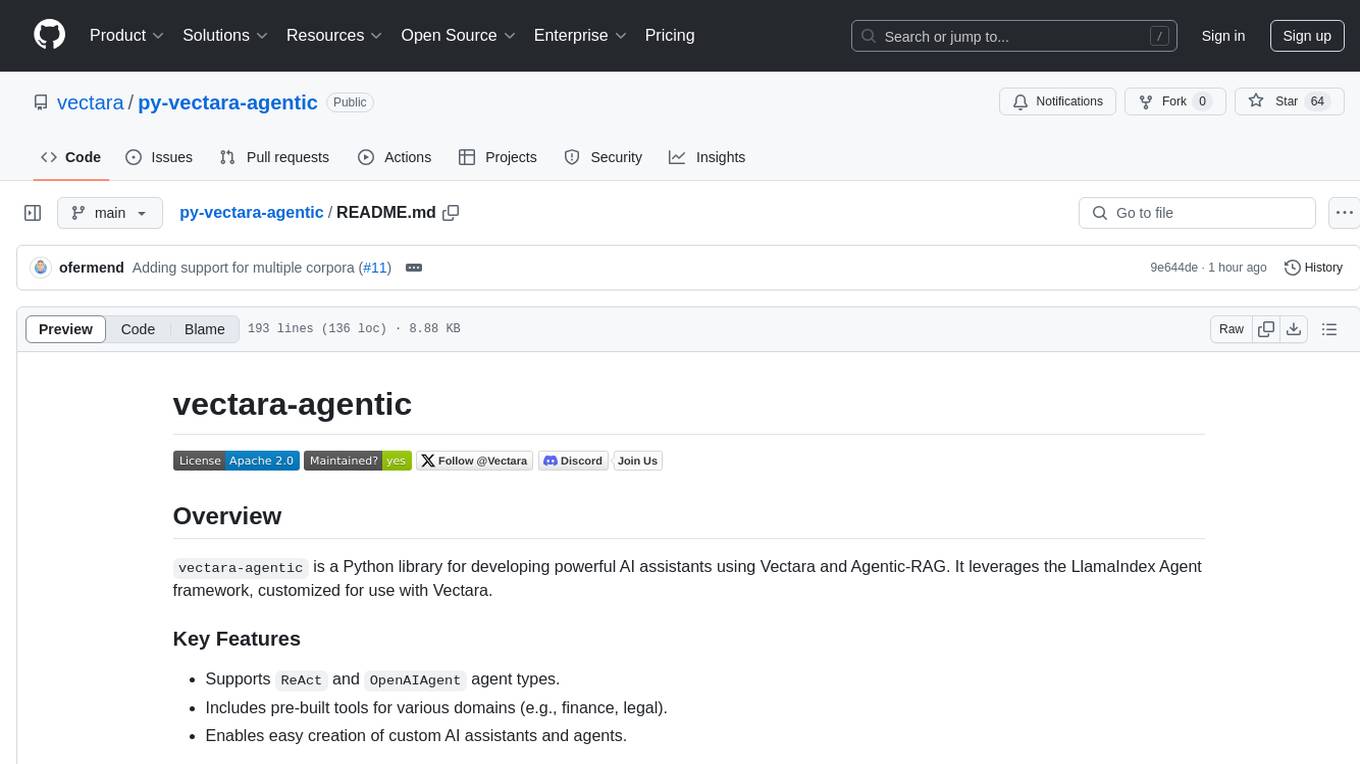
py-vectara-agentic
The `vectara-agentic` Python library is designed for developing powerful AI assistants using Vectara and Agentic-RAG. It supports various agent types, includes pre-built tools for domains like finance and legal, and enables easy creation of custom AI assistants and agents. The library provides tools for summarizing text, rephrasing text, legal tasks like summarizing legal text and critiquing as a judge, financial tasks like analyzing balance sheets and income statements, and database tools for inspecting and querying databases. It also supports observability via LlamaIndex and Arize Phoenix integration.
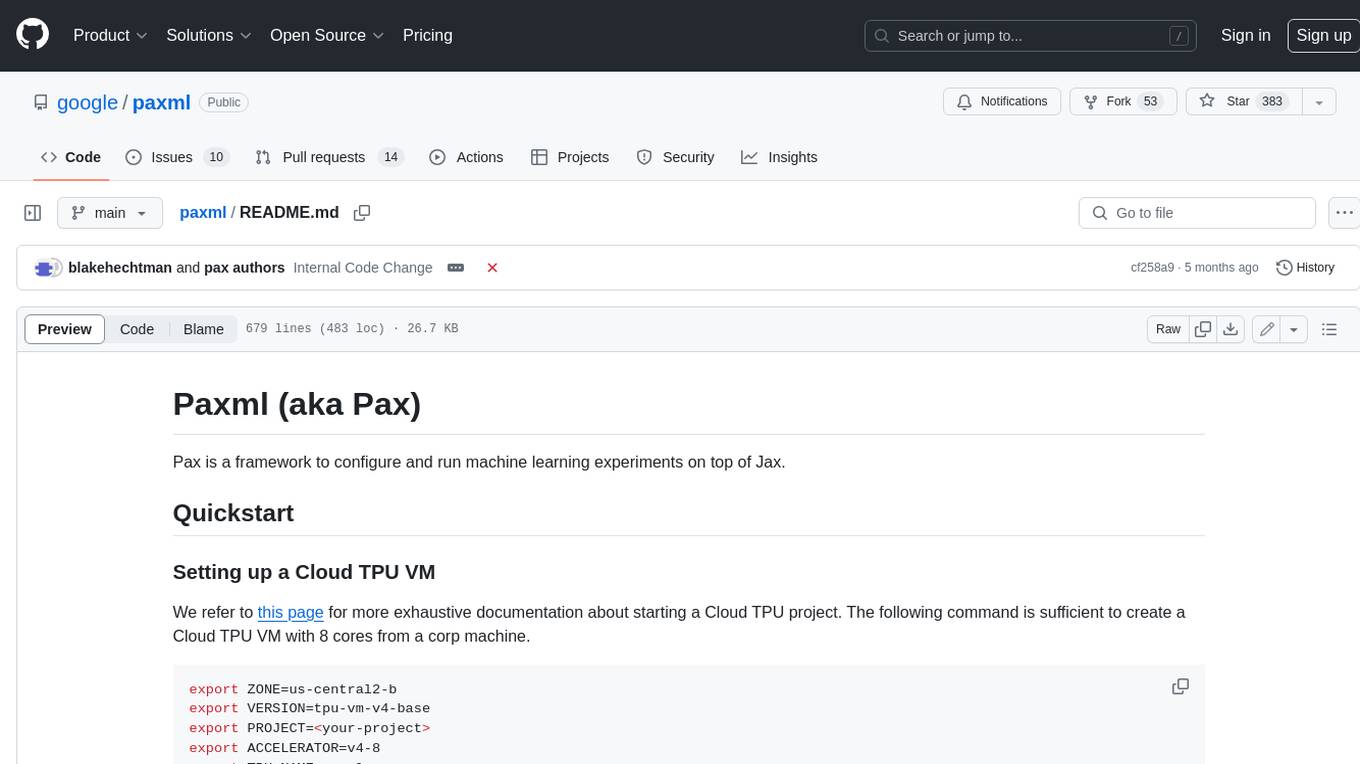
paxml
Pax is a framework to configure and run machine learning experiments on top of Jax.
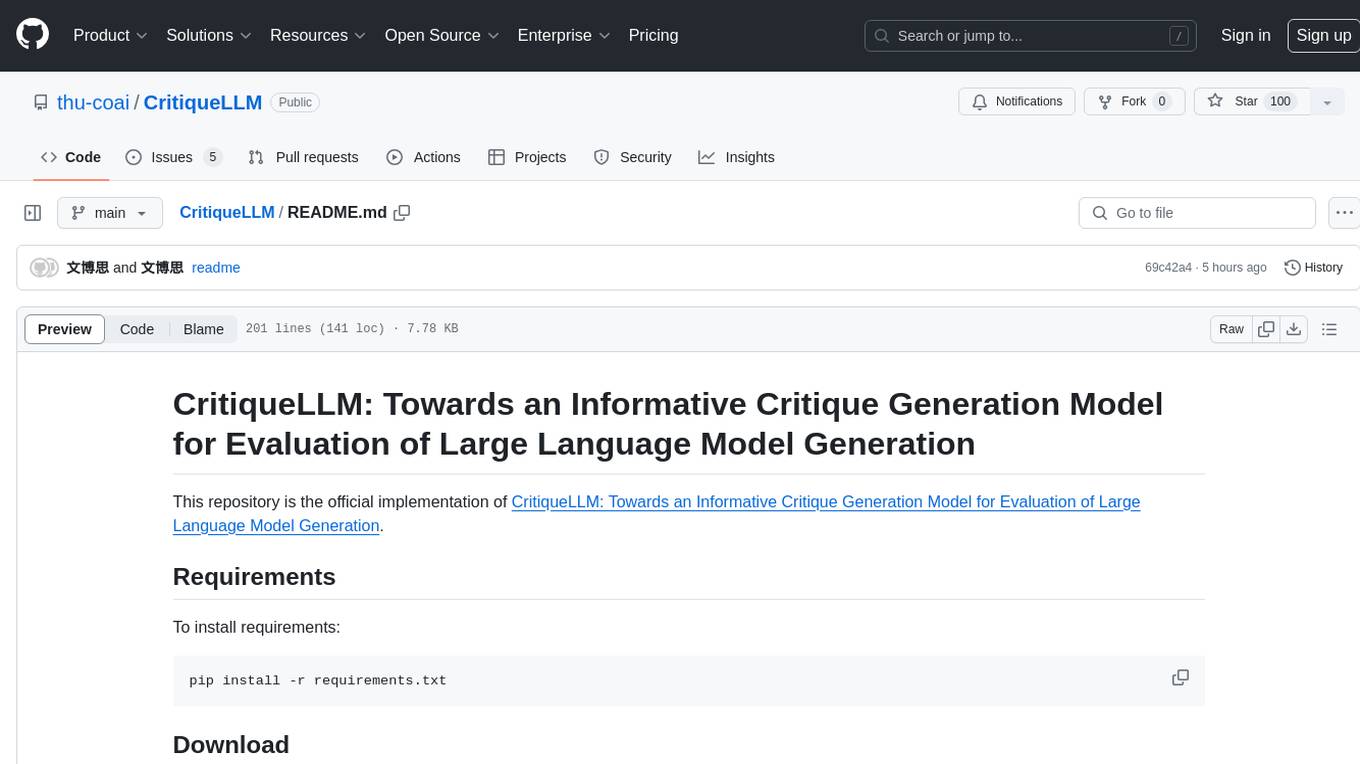
CritiqueLLM
CritiqueLLM is an official implementation of a model designed for generating informative critiques to evaluate large language model generation. It includes functionalities for data collection, referenced pointwise grading, referenced pairwise comparison, reference-free pairwise comparison, reference-free pointwise grading, inference for pointwise grading and pairwise comparison, and evaluation of the generated results. The model aims to provide a comprehensive framework for evaluating the performance of large language models based on human ratings and comparisons.
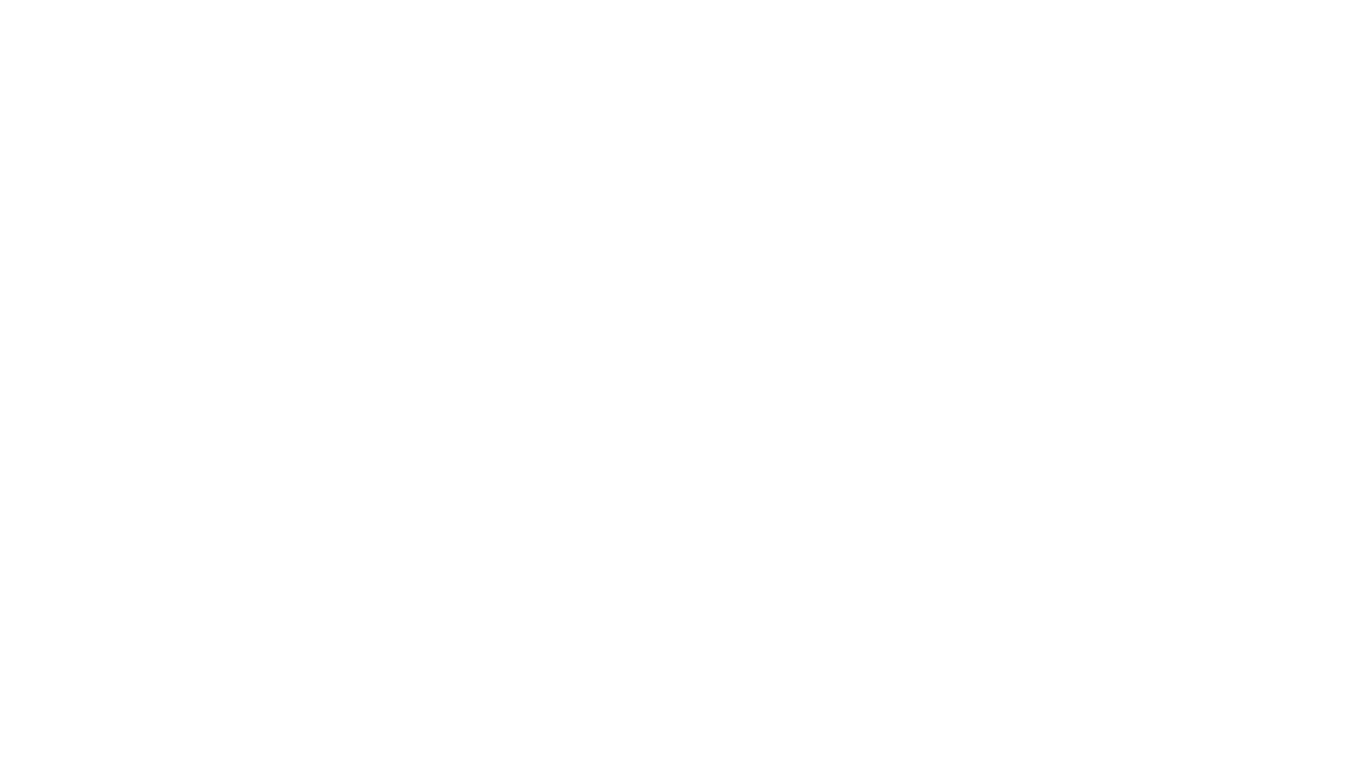
upgini
Upgini is an intelligent data search engine with a Python library that helps users find and add relevant features to their ML pipeline from various public, community, and premium external data sources. It automates the optimization of connected data sources by generating an optimal set of machine learning features using large language models, GraphNNs, and recurrent neural networks. The tool aims to simplify feature search and enrichment for external data to make it a standard approach in machine learning pipelines. It democratizes access to data sources for the data science community.
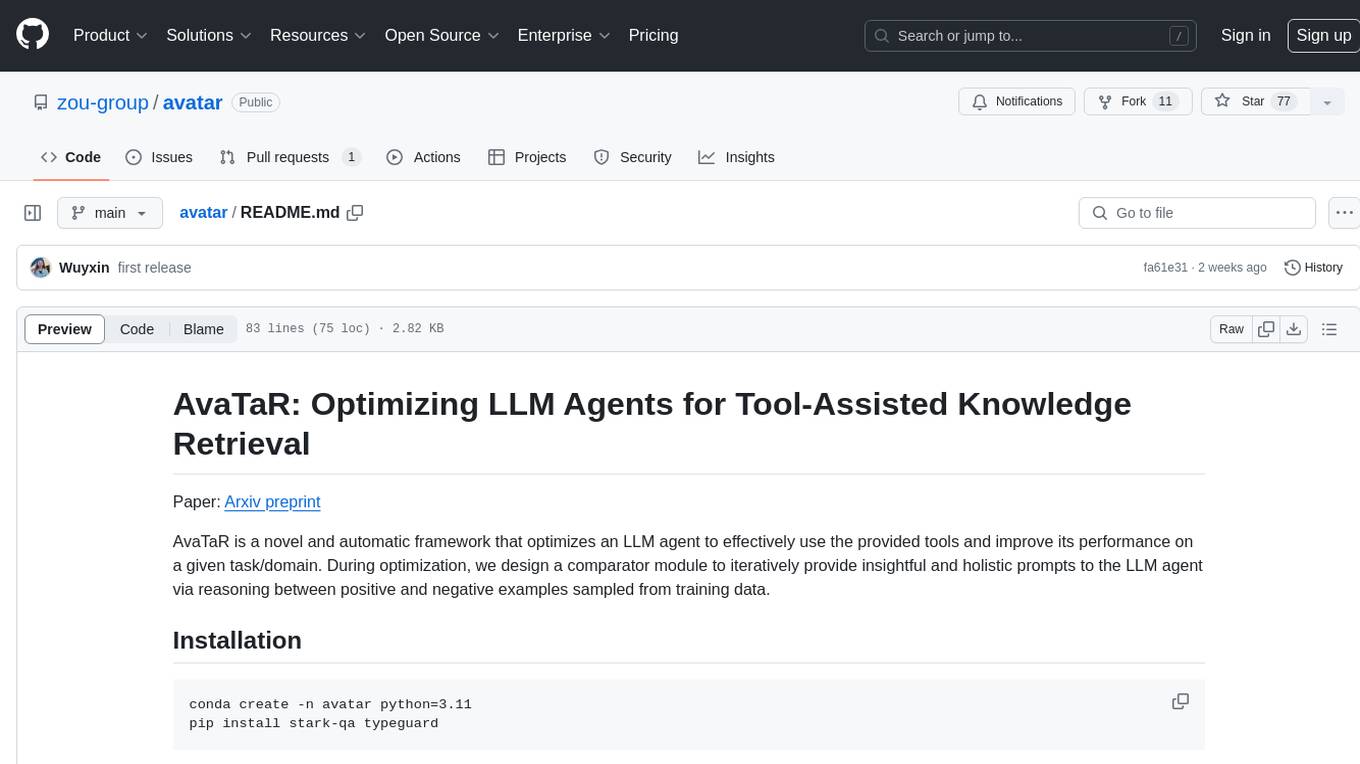
avatar
AvaTaR is a novel and automatic framework that optimizes an LLM agent to effectively use provided tools and improve performance on a given task/domain. It designs a comparator module to provide insightful prompts to the LLM agent via reasoning between positive and negative examples from training data.
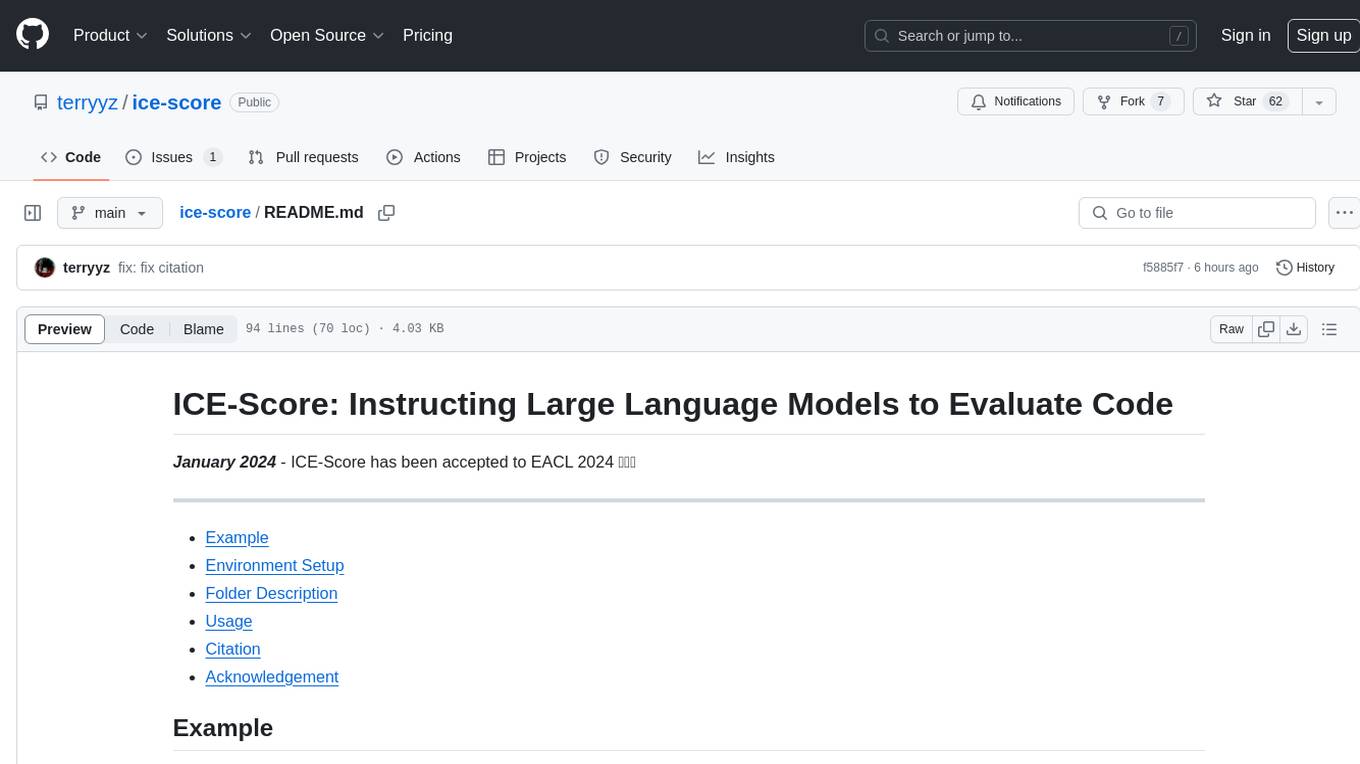
ice-score
ICE-Score is a tool designed to instruct large language models to evaluate code. It provides a minimum viable product (MVP) for evaluating generated code snippets using inputs such as problem, output, task, aspect, and model. Users can also evaluate with reference code and enable zero-shot chain-of-thought evaluation. The tool is built on codegen-metrics and code-bert-score repositories and includes datasets like CoNaLa and HumanEval. ICE-Score has been accepted to EACL 2024.
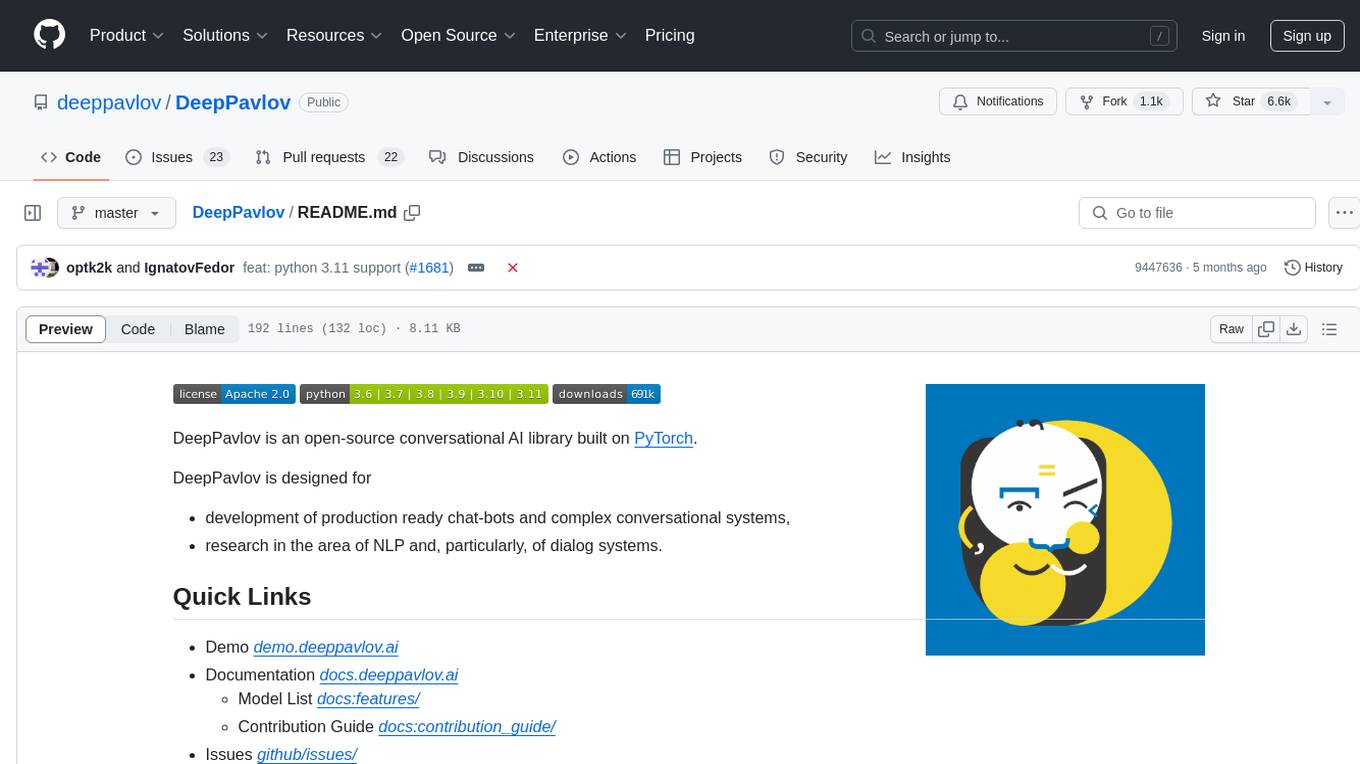
DeepPavlov
DeepPavlov is an open-source conversational AI library built on PyTorch. It is designed for the development of production-ready chatbots and complex conversational systems, as well as for research in the area of NLP and dialog systems. The library offers a wide range of models for tasks such as Named Entity Recognition, Intent/Sentence Classification, Question Answering, Sentence Similarity/Ranking, Syntactic Parsing, and more. DeepPavlov also provides embeddings like BERT, ELMo, and FastText for various languages, along with AutoML capabilities and integrations with REST API, Socket API, and Amazon AWS.
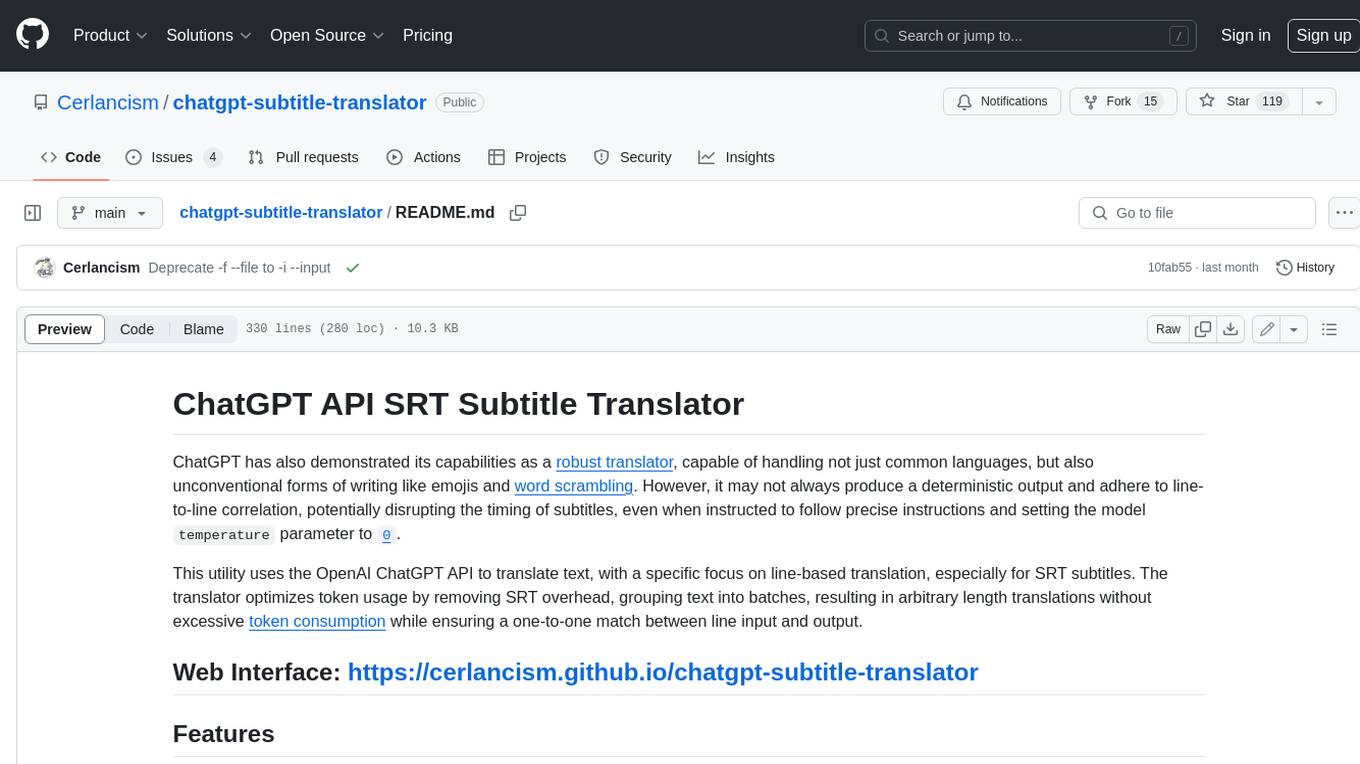
chatgpt-subtitle-translator
This tool utilizes the OpenAI ChatGPT API to translate text, with a focus on line-based translation, particularly for SRT subtitles. It optimizes token usage by removing SRT overhead and grouping text into batches, allowing for arbitrary length translations without excessive token consumption while maintaining a one-to-one match between line input and output.