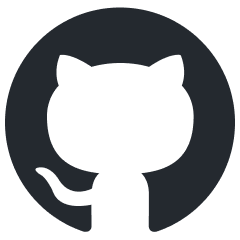
SpeziLLM
A module enabling the integration of Large Language Models (LLMs) with the Spezi Ecosystem
Stars: 131
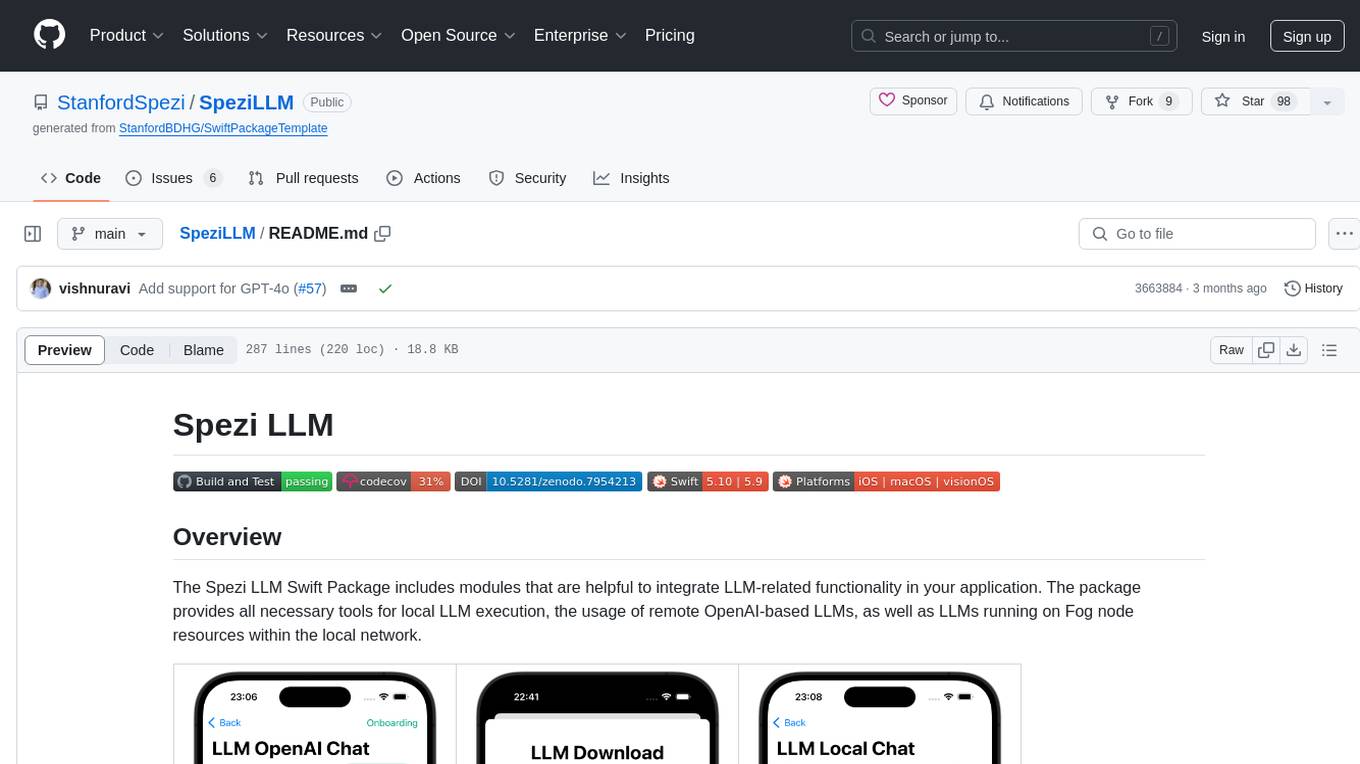
The Spezi LLM Swift Package includes modules that help integrate LLM-related functionality in applications. It provides tools for local LLM execution, usage of remote OpenAI-based LLMs, and LLMs running on Fog node resources within the local network. The package contains targets like SpeziLLM, SpeziLLMLocal, SpeziLLMLocalDownload, SpeziLLMOpenAI, and SpeziLLMFog for different LLM functionalities. Users can configure and interact with local LLMs, OpenAI LLMs, and Fog LLMs using the provided APIs and platforms within the Spezi ecosystem.
README:
The Spezi LLM Swift Package includes modules that are helpful to integrate LLM-related functionality in your application. The package provides all necessary tools for local LLM execution, the usage of remote OpenAI-based LLMs, as well as LLMs running on Fog node resources within the local network.
![]() |
![]() |
![]() |
---|---|---|
OpenAI LLM Chat View |
Language Model Download |
Local LLM Chat View |
You need to add the SpeziLLM Swift package to your app in Xcode or Swift package.
[!IMPORTANT]
If your application is not yet configured to use Spezi, follow the Spezi setup article to set up the core Spezi infrastructure.
As Spezi LLM contains a variety of different targets for specific LLM functionalities, please follow the additional setup guide in the respective target section of this README.
Spezi LLM provides a number of targets to help developers integrate LLMs in their Spezi-based applications:
- SpeziLLM: Base infrastructure of LLM execution in the Spezi ecosystem.
- SpeziLLMLocal: Local LLM execution capabilities directly on-device. Enables running open-source LLMs like Meta's Llama2 models.
- SpeziLLMLocalDownload: Download and storage manager of local Language Models, including onboarding views.
- SpeziLLMOpenAI: Integration with OpenAI's GPT models via using OpenAI's API service.
- SpeziLLMFog: Discover and dispatch LLM inference jobs to Fog node resources within the local network.
The section below highlights the setup and basic use of the SpeziLLMLocal, SpeziLLMOpenAI, and SpeziLLMFog targets in order to integrate Language Models in a Spezi-based application.
[!NOTE]
To learn more about the usage of the individual targets, please refer to the DocC documentation of the package.
The target enables developers to easily execute medium-size Language Models (LLMs) locally on-device via the llama.cpp framework. The module allows you to interact with the locally run LLM via purely Swift-based APIs, no interaction with low-level C or C++ code is necessary, building on top of the infrastructure of the SpeziLLM target.
[!IMPORTANT] Important: In order to use the LLM local target, one needs to set build parameters in the consuming Xcode project or the consuming SPM package to enable the Swift / C++ Interop, introduced in Xcode 15 and Swift 5.9. Keep in mind that this is true for nested dependencies, one needs to set this configuration recursivly for the entire dependency tree towards the llama.cpp SPM package.
For Xcode projects:
- Open your build settings in Xcode by selecting PROJECT_NAME > TARGET_NAME > Build Settings.
- Within the Build Settings, search for the
C++ and Objective-C Interoperability
setting and set it toC++ / Objective-C++
. This enables the project to use the C++ headers from llama.cpp.For SPM packages:
- Open the
Package.swift
file of your SPM package- Within the package
target
that consumes the llama.cpp package, add theinteroperabilityMode(_:)
Swift build setting like that:/// Adds the dependency to the Spezi LLM SPM package dependencies: [ .package(url: "https://github.com/StanfordSpezi/SpeziLLM", .upToNextMinor(from: "0.6.0")) ], targets: [ .target( name: "ExampleConsumingTarget", /// State the dependence of the target to SpeziLLMLocal dependencies: [ .product(name: "SpeziLLMLocal", package: "SpeziLLM") ], /// Important: Configure the `.interoperabilityMode(_:)` within the `swiftSettings` swiftSettings: [ .interoperabilityMode(.Cxx) ] ) ]
You can configure the Spezi Local LLM execution within the typical SpeziAppDelegate
.
In the example below, the LLMRunner
from the SpeziLLM target which is responsible for providing LLM functionality within the Spezi ecosystem is configured with the LLMLocalPlatform
from the SpeziLLMLocal target. This prepares the LLMRunner
to locally execute Language Models.
class TestAppDelegate: SpeziAppDelegate {
override var configuration: Configuration {
Configuration {
LLMRunner {
LLMLocalPlatform()
}
}
}
}
The code example below showcases the interaction with local LLMs through the the SpeziLLM LLMRunner
, which is injected into the SwiftUI Environment
via the Configuration
shown above.
The LLMLocalSchema
defines the type and configurations of the to-be-executed LLMLocalSession
. This transformation is done via the LLMRunner
that uses the LLMLocalPlatform
. The inference via LLMLocalSession/generate()
returns an AsyncThrowingStream
that yields all generated String
pieces.
struct LLMLocalDemoView: View {
@Environment(LLMRunner.self) var runner
@State var responseText = ""
var body: some View {
Text(responseText)
.task {
// Instantiate the `LLMLocalSchema` to an `LLMLocalSession` via the `LLMRunner`.
let llmSession: LLMLocalSession = runner(
with: LLMLocalSchema(
modelPath: URL(string: "URL to the local model file")!
)
)
do {
for try await token in try await llmSession.generate() {
responseText.append(token)
}
} catch {
// Handle errors here. E.g., you can use `ViewState` and `viewStateAlert` from SpeziViews.
}
}
}
}
[!NOTE]
To learn more about the usage of SpeziLLMLocal, please refer to the DocC documentation.
A module that allows you to interact with GPT-based Large Language Models (LLMs) from OpenAI within your Spezi application.
SpeziLLMOpenAI
provides a pure Swift-based API for interacting with the OpenAI GPT API, building on top of the infrastructure of the SpeziLLM target.
In addition, SpeziLLMOpenAI
provides developers with a declarative Domain Specific Language to utilize OpenAI function calling mechanism. This enables a structured, bidirectional, and reliable communication between the OpenAI LLMs and external tools, such as the Spezi ecosystem.
In order to use OpenAI LLMs within the Spezi ecosystem, the SpeziLLM LLMRunner
needs to be initialized in the Spezi Configuration
with the LLMOpenAIPlatform
. Only after, the LLMRunner
can be used for inference of OpenAI LLMs.
See the SpeziLLM documentation for more details.
import Spezi
import SpeziLLM
import SpeziLLMOpenAI
class LLMOpenAIAppDelegate: SpeziAppDelegate {
override var configuration: Configuration {
Configuration {
LLMRunner {
LLMOpenAIPlatform()
}
}
}
}
[!IMPORTANT] If using
SpeziLLMOpenAI
on macOS, ensure to add theKeychain Access Groups
entitlement to the enclosing Xcode project via PROJECT_NAME > Signing&Capabilities > + Capability. The array of keychain groups can be left empty, only the base entitlement is required.
The code example below showcases the interaction with an OpenAI LLM through the the SpeziLLM LLMRunner
, which is injected into the SwiftUI Environment
via the Configuration
shown above.
The LLMOpenAISchema
defines the type and configurations of the to-be-executed LLMOpenAISession
. This transformation is done via the LLMRunner
that uses the LLMOpenAIPlatform
. The inference via LLMOpenAISession/generate()
returns an AsyncThrowingStream
that yields all generated String
pieces.
import SpeziLLM
import SpeziLLMOpenAI
import SwiftUI
struct LLMOpenAIDemoView: View {
@Environment(LLMRunner.self) var runner
@State var responseText = ""
var body: some View {
Text(responseText)
.task {
// Instantiate the `LLMOpenAISchema` to an `LLMOpenAISession` via the `LLMRunner`.
let llmSession: LLMOpenAISession = runner(
with: LLMOpenAISchema(
parameters: .init(
modelType: .gpt3_5Turbo,
systemPrompt: "You're a helpful assistant that answers questions from users.",
overwritingToken: "abc123"
)
)
)
do {
for try await token in try await llmSession.generate() {
responseText.append(token)
}
} catch {
// Handle errors here. E.g., you can use `ViewState` and `viewStateAlert` from SpeziViews.
}
}
}
}
[!NOTE]
To learn more about the usage of SpeziLLMOpenAI, please refer to the DocC documentation.
The SpeziLLMFog
target enables you to use LLMs running on Fog node computing resources within the local network. The fog nodes advertise their services via mDNS, enabling clients to discover all fog nodes serving a specific host within the local network.
SpeziLLMFog
then dispatches LLM inference jobs dynamically to a random fog node within the local network and streams the response to surface it to the user.
[!IMPORTANT]
SpeziLLMFog
requires aSpeziLLMFogNode
within the local network hosted on some computing resource that actually performs the inference requests.SpeziLLMFog
provides theSpeziLLMFogNode
Docker-based package that enables an easy setup of these fog nodes. See theFogNode
directory on the root level of the SPM package as well as the respectiveREADME.md
for more details.
In order to use Fog LLMs within the Spezi ecosystem, the SpeziLLM LLMRunner
needs to be initialized in the Spezi Configuration
with the LLMFogPlatform
. Only after, the LLMRunner
can be used for inference with Fog LLMs. See the SpeziLLM documentation for more details.
The LLMFogPlatform
needs to be initialized with the custom root CA certificate that was used to sign the fog node web service certificate (see the FogNode/README.md
documentation for more information). Copy the root CA certificate from the fog node as resource to the application using SpeziLLMFog
and use it to initialize the LLMFogPlatform
within the Spezi Configuration
.
class LLMFogAppDelegate: SpeziAppDelegate {
private nonisolated static var caCertificateUrl: URL {
// Return local file URL of root CA certificate in the `.crt` format
}
override var configuration: Configuration {
Configuration {
LLMRunner {
// Set up the Fog platform with the custom CA certificate
LLMRunner {
LLMFogPlatform(configuration: .init(caCertificate: Self.caCertificateUrl))
}
}
}
}
}
The code example below showcases the interaction with a Fog LLM through the the SpeziLLM LLMRunner
, which is injected into the SwiftUI Environment
via the Configuration
shown above.
The LLMFogSchema
defines the type and configurations of the to-be-executed LLMFogSession
. This transformation is done via the LLMRunner
that uses the LLMFogPlatform
. The inference via LLMFogSession/generate()
returns an AsyncThrowingStream
that yields all generated String
pieces.
The LLMFogSession
automatically discovers all available LLM fog nodes within the local network upon setup and the dispatches the LLM inference jobs to the fog computing resource, streaming back the response and surfaces it to the user.
[!IMPORTANT]
TheLLMFogSchema
accepts a closure that returns an authorization token that is passed with every request to the Fog node in theBearer
HTTP field via theLLMFogParameters/init(modelType:systemPrompt:authToken:)
. The token is created via the closure upon every LLM inference request, as theLLMFogSession
may be long lasting and the token could therefore expire. Ensure that the closure appropriately caches the token in order to prevent unnecessary token refresh roundtrips to external systems.
struct LLMFogDemoView: View {
@Environment(LLMRunner.self) var runner
@State var responseText = ""
var body: some View {
Text(responseText)
.task {
// Instantiate the `LLMFogSchema` to an `LLMFogSession` via the `LLMRunner`.
let llmSession: LLMFogSession = runner(
with: LLMFogSchema(
parameters: .init(
modelType: .llama7B,
systemPrompt: "You're a helpful assistant that answers questions from users.",
authToken: {
// Return authorization token as `String` or `nil` if no token is required by the Fog node.
}
)
)
)
do {
for try await token in try await llmSession.generate() {
responseText.append(token)
}
} catch {
// Handle errors here. E.g., you can use `ViewState` and `viewStateAlert` from SpeziViews.
}
}
}
}
[!NOTE]
To learn more about the usage of SpeziLLMFog, please refer to the DocC documentation.
Contributions to this project are welcome. Please make sure to read the contribution guidelines and the contributor covenant code of conduct first.
This project is licensed under the MIT License. See Licenses for more information.
For Tasks:
Click tags to check more tools for each tasksFor Jobs:
Alternative AI tools for SpeziLLM
Similar Open Source Tools
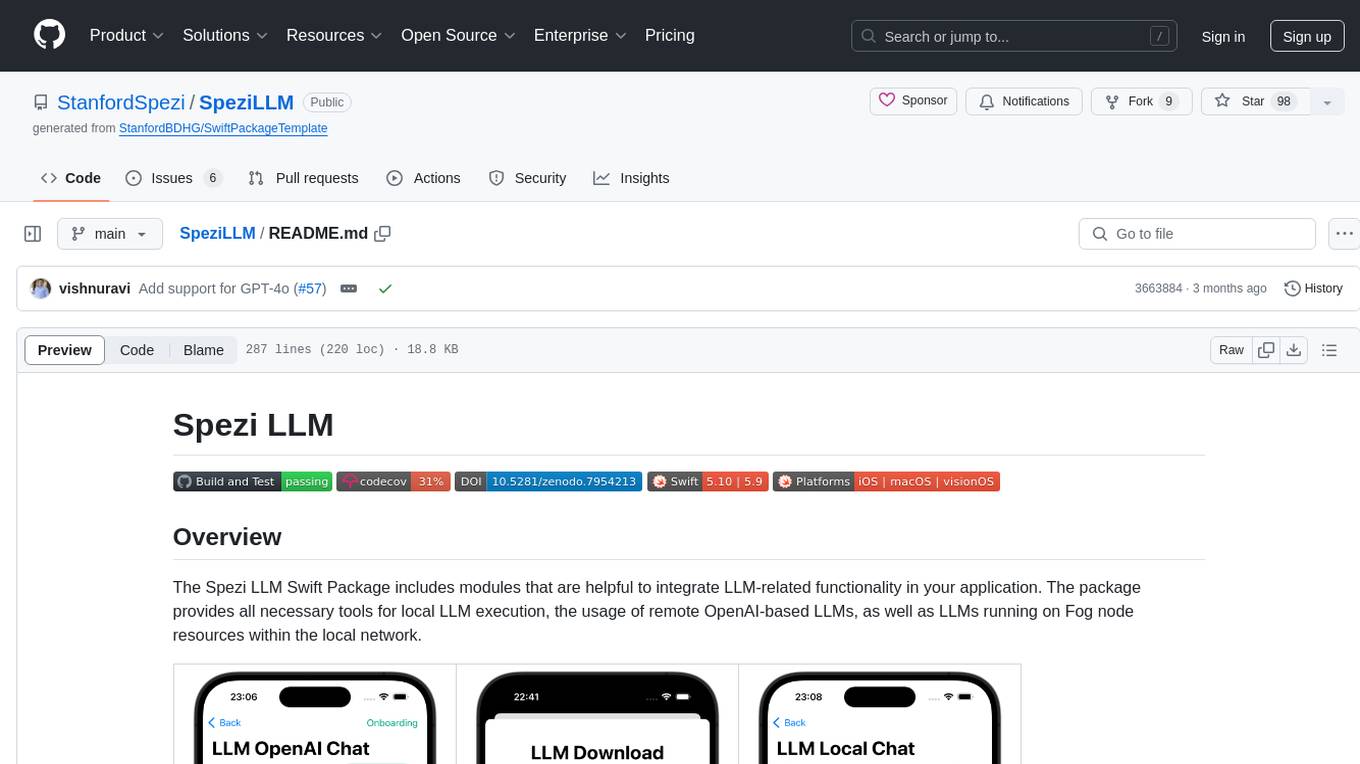
SpeziLLM
The Spezi LLM Swift Package includes modules that help integrate LLM-related functionality in applications. It provides tools for local LLM execution, usage of remote OpenAI-based LLMs, and LLMs running on Fog node resources within the local network. The package contains targets like SpeziLLM, SpeziLLMLocal, SpeziLLMLocalDownload, SpeziLLMOpenAI, and SpeziLLMFog for different LLM functionalities. Users can configure and interact with local LLMs, OpenAI LLMs, and Fog LLMs using the provided APIs and platforms within the Spezi ecosystem.
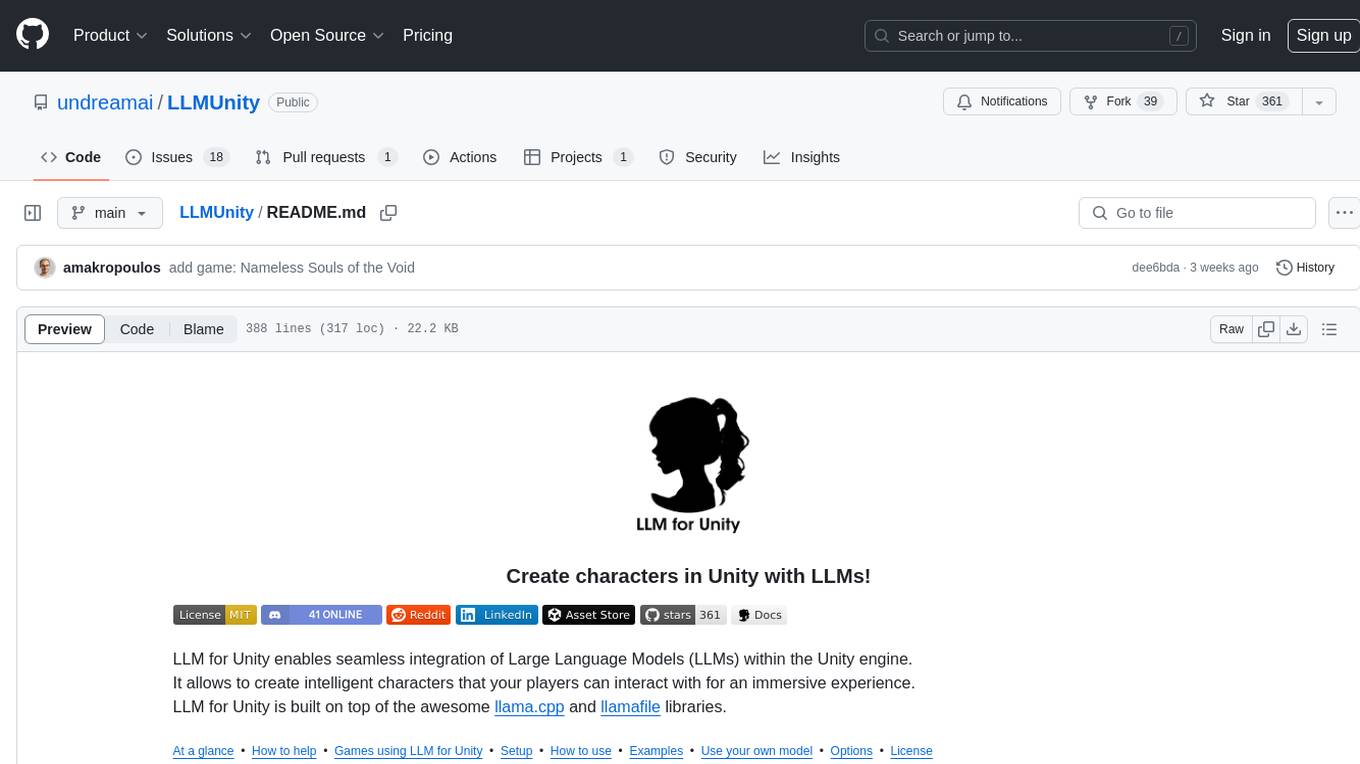
LLMUnity
LLM for Unity enables seamless integration of Large Language Models (LLMs) within the Unity engine, allowing users to create intelligent characters for immersive player interactions. The tool supports major LLM models, runs locally without internet access, offers fast inference on CPU and GPU, and is easy to set up with a single line of code. It is free for both personal and commercial use, tested on Unity 2021 LTS, 2022 LTS, and 2023. Users can build multiple AI characters efficiently, use remote servers for processing, and customize model settings for text generation.
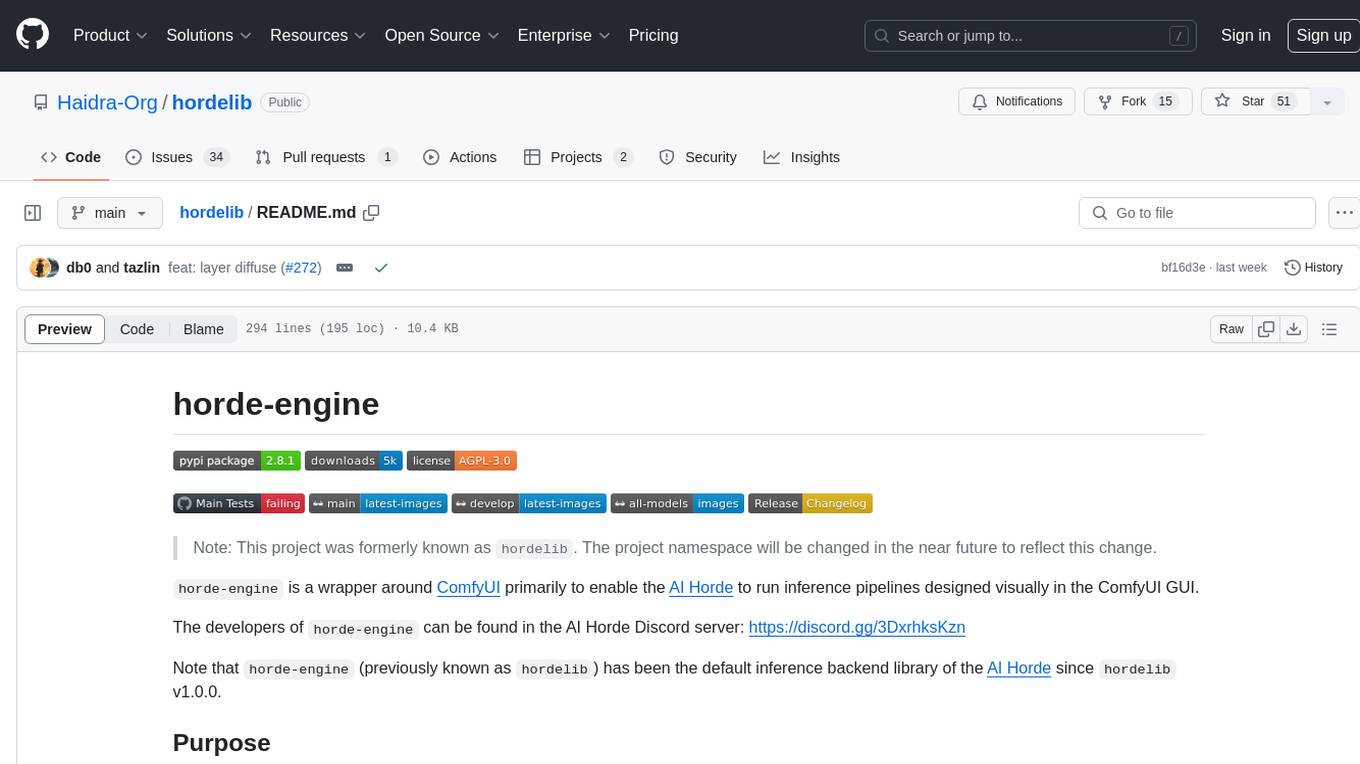
hordelib
horde-engine is a wrapper around ComfyUI designed to run inference pipelines visually designed in the ComfyUI GUI. It enables users to design inference pipelines in ComfyUI and then call them programmatically, maintaining compatibility with the existing horde implementation. The library provides features for processing Horde payloads, initializing the library, downloading and validating models, and generating images based on input data. It also includes custom nodes for preprocessing and tasks such as face restoration and QR code generation. The project depends on various open source projects and bundles some dependencies within the library itself. Users can design ComfyUI pipelines, convert them to the backend format, and run them using the run_image_pipeline() method in hordelib.comfy.Comfy(). The project is actively developed and tested using git, tox, and a specific model directory structure.
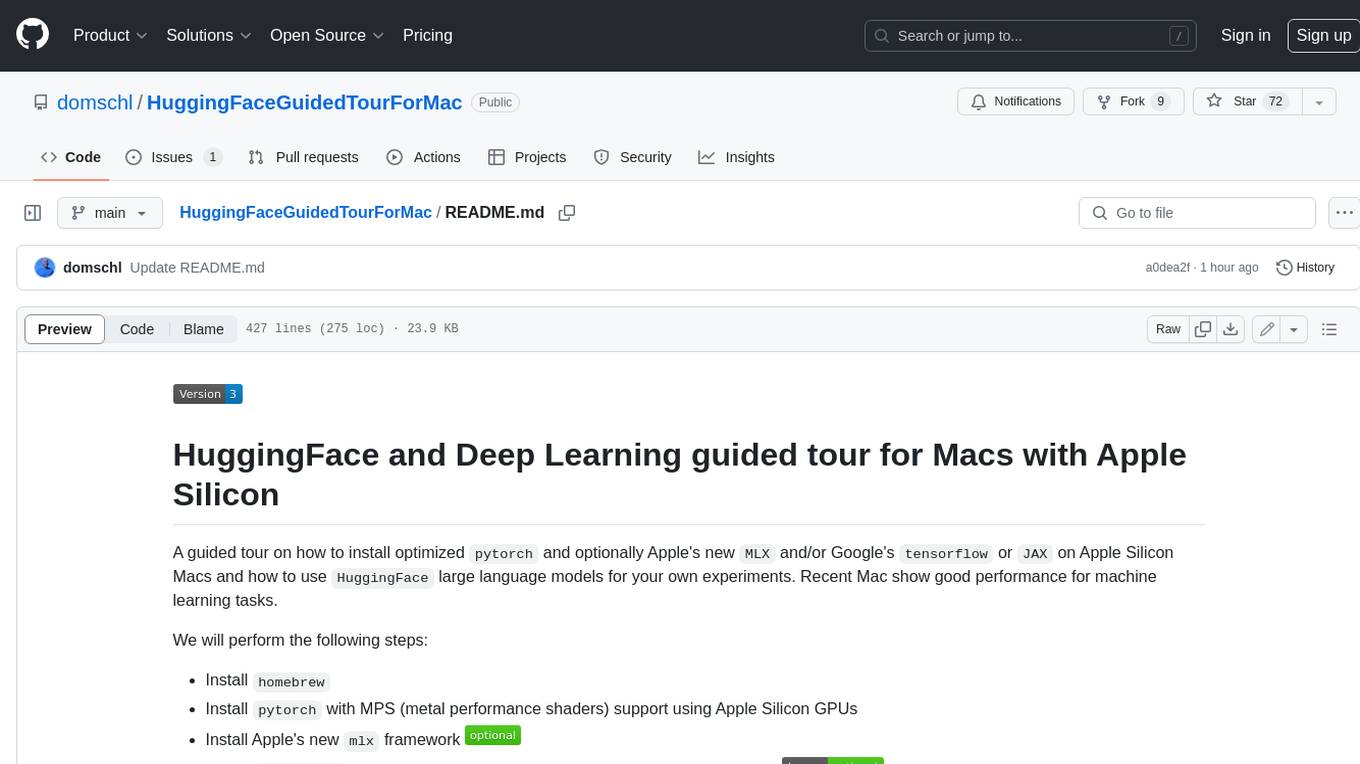
HuggingFaceGuidedTourForMac
HuggingFaceGuidedTourForMac is a guided tour on how to install optimized pytorch and optionally Apple's new MLX, JAX, and TensorFlow on Apple Silicon Macs. The repository provides steps to install homebrew, pytorch with MPS support, MLX, JAX, TensorFlow, and Jupyter lab. It also includes instructions on running large language models using HuggingFace transformers. The repository aims to help users set up their Macs for deep learning experiments with optimized performance.
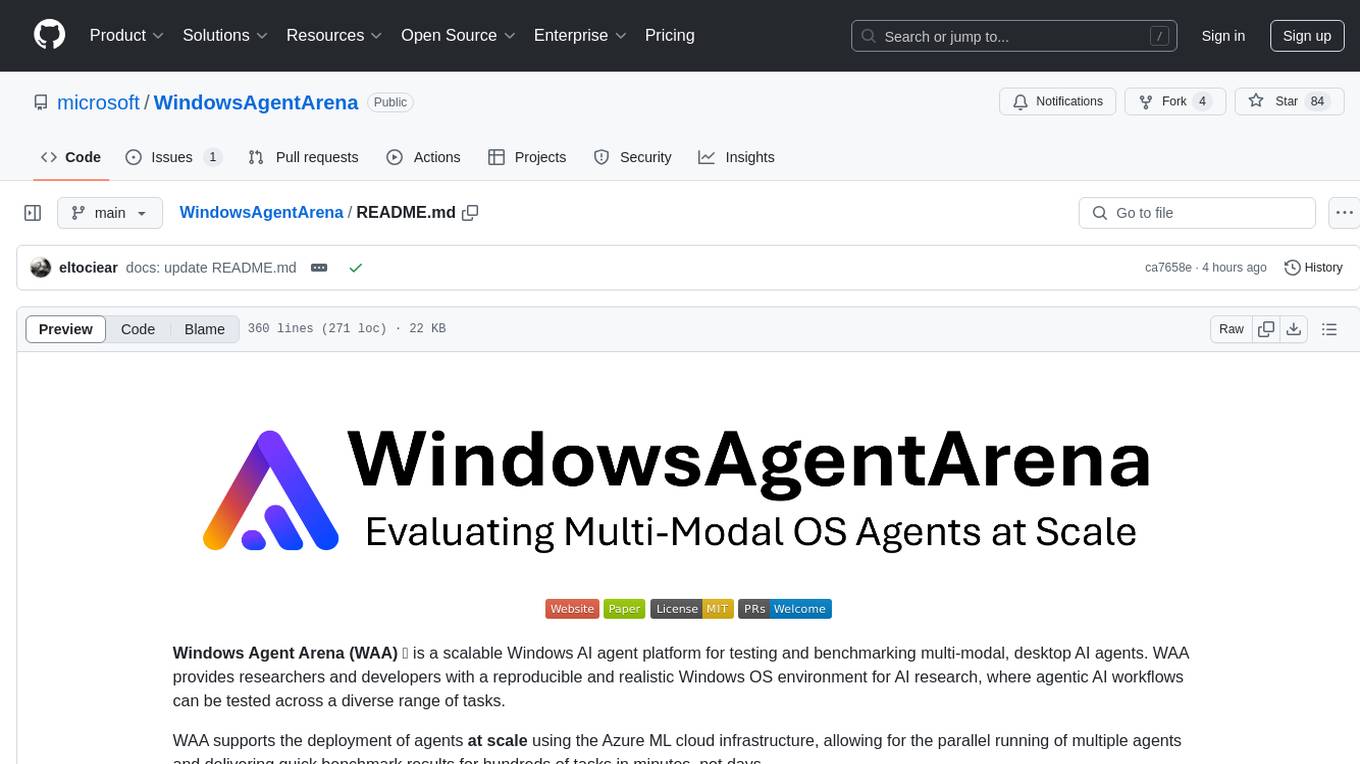
WindowsAgentArena
Windows Agent Arena (WAA) is a scalable Windows AI agent platform designed for testing and benchmarking multi-modal, desktop AI agents. It provides researchers and developers with a reproducible and realistic Windows OS environment for AI research, enabling testing of agentic AI workflows across various tasks. WAA supports deploying agents at scale using Azure ML cloud infrastructure, allowing parallel running of multiple agents and delivering quick benchmark results for hundreds of tasks in minutes.
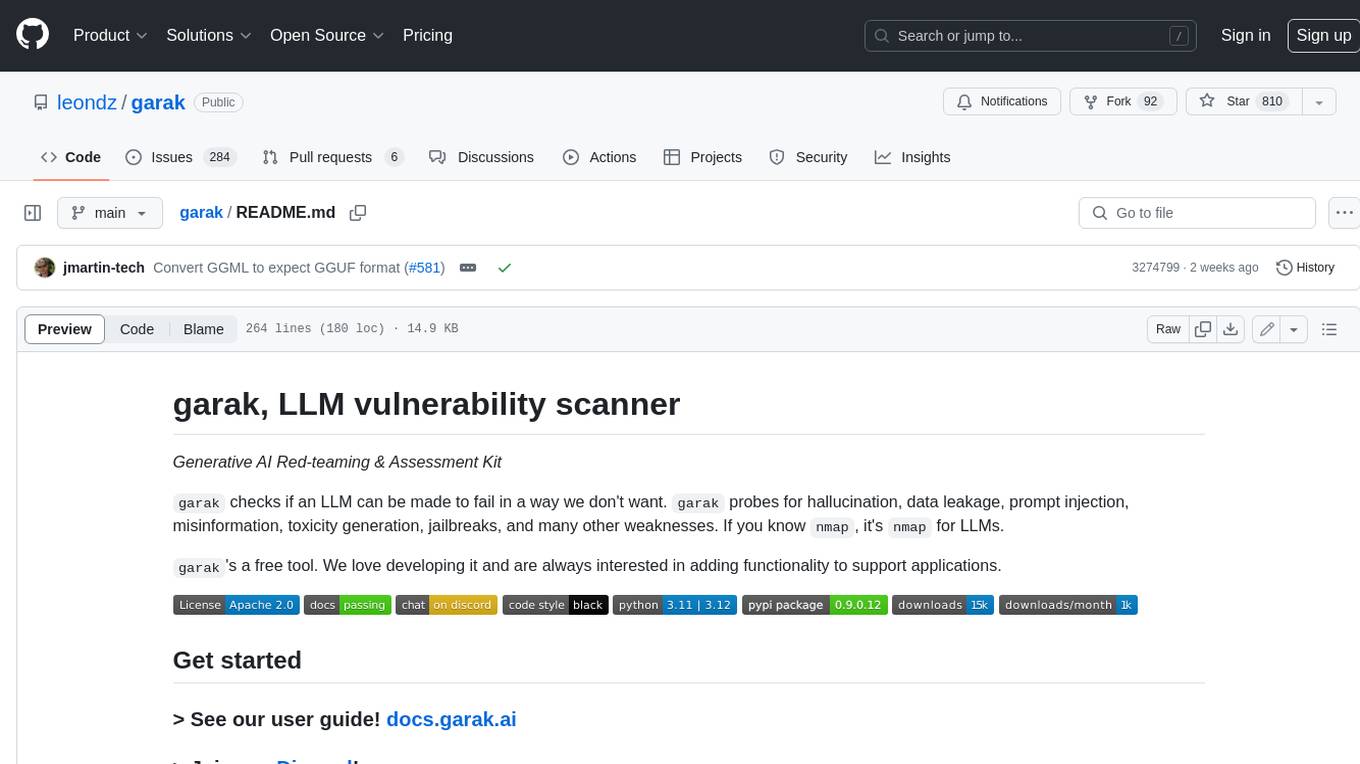
garak
Garak is a free tool that checks if a Large Language Model (LLM) can be made to fail in a way that is undesirable. It probes for hallucination, data leakage, prompt injection, misinformation, toxicity generation, jailbreaks, and many other weaknesses. Garak's a free tool. We love developing it and are always interested in adding functionality to support applications.
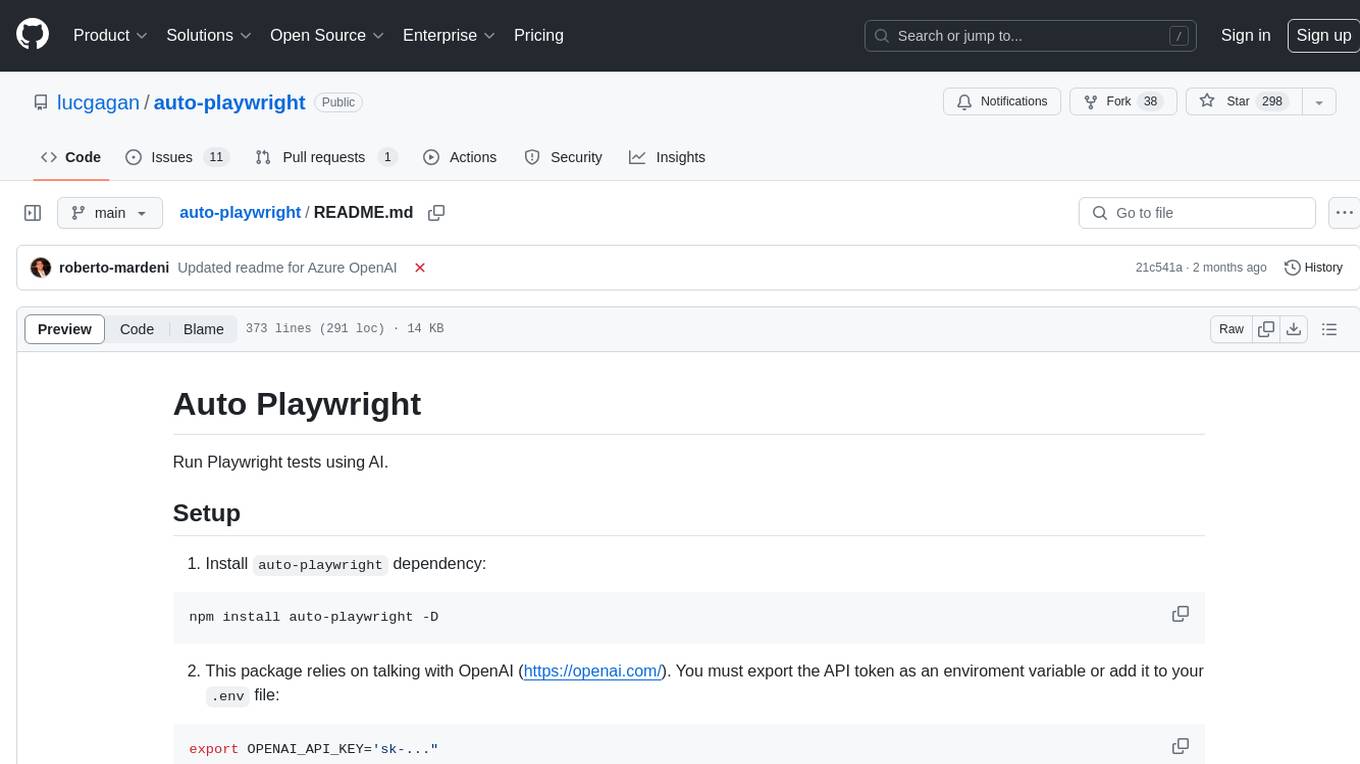
auto-playwright
Auto Playwright is a tool that allows users to run Playwright tests using AI. It eliminates the need for selectors by determining actions at runtime based on plain-text instructions. Users can automate complex scenarios, write tests concurrently with or before functionality development, and benefit from rapid test creation. The tool supports various Playwright actions and offers additional options for debugging and customization. It uses HTML sanitization to reduce costs and improve text quality when interacting with the OpenAI API.
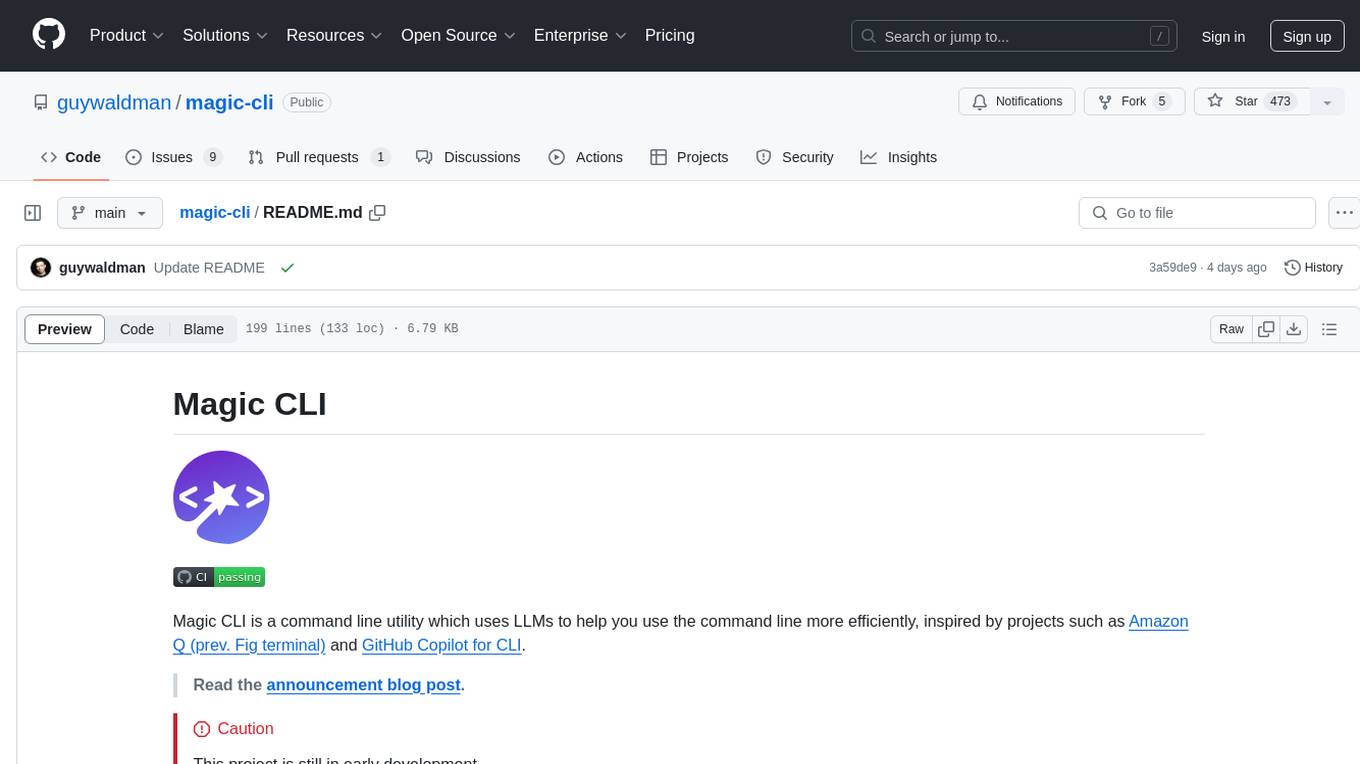
magic-cli
Magic CLI is a command line utility that leverages Large Language Models (LLMs) to enhance command line efficiency. It is inspired by projects like Amazon Q and GitHub Copilot for CLI. The tool allows users to suggest commands, search across command history, and generate commands for specific tasks using local or remote LLM providers. Magic CLI also provides configuration options for LLM selection and response generation. The project is still in early development, so users should expect breaking changes and bugs.
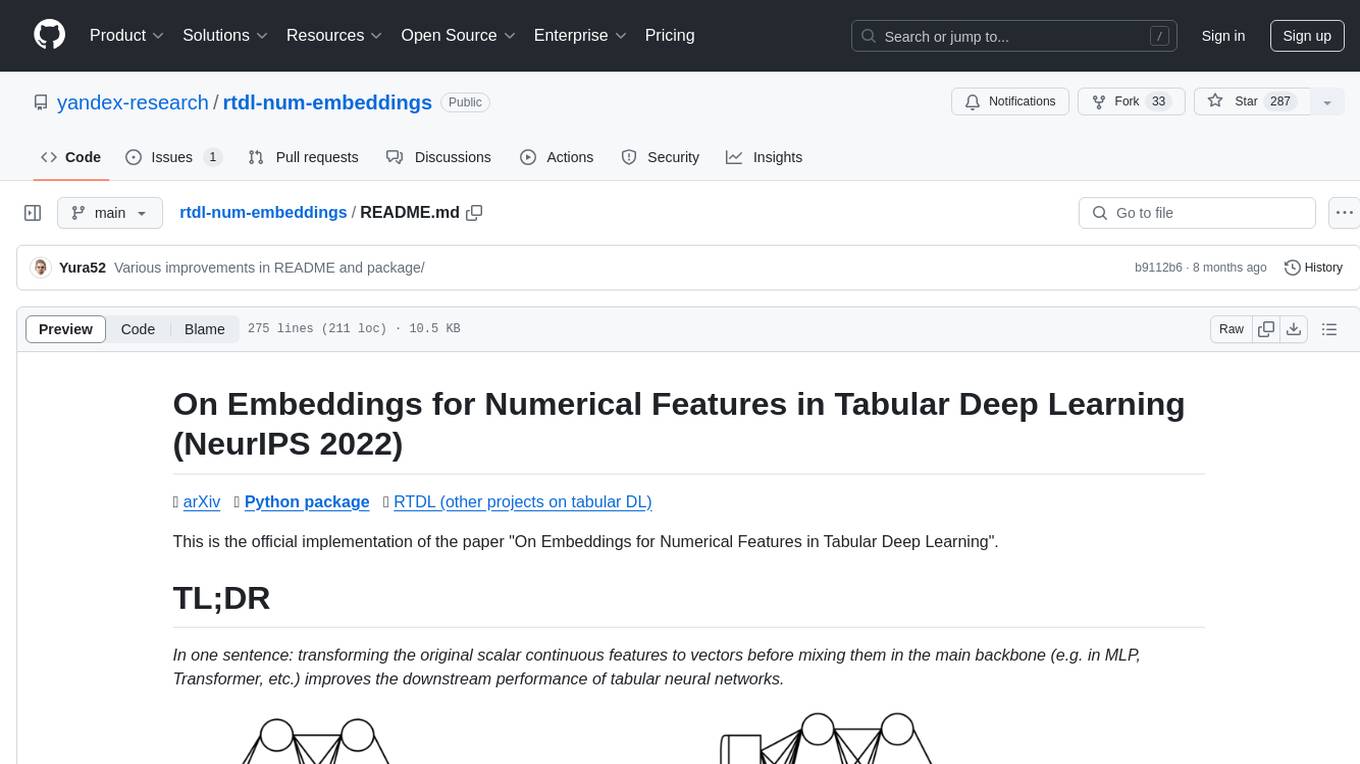
rtdl-num-embeddings
This repository provides the official implementation of the paper 'On Embeddings for Numerical Features in Tabular Deep Learning'. It focuses on transforming scalar continuous features into vectors before integrating them into the main backbone of tabular neural networks, showcasing improved performance. The embeddings for continuous features are shown to enhance the performance of tabular DL models and are applicable to various conventional backbones, offering efficiency comparable to Transformer-based models. The repository includes Python packages for practical usage, exploration of metrics and hyperparameters, and reproducing reported results for different algorithms and datasets.
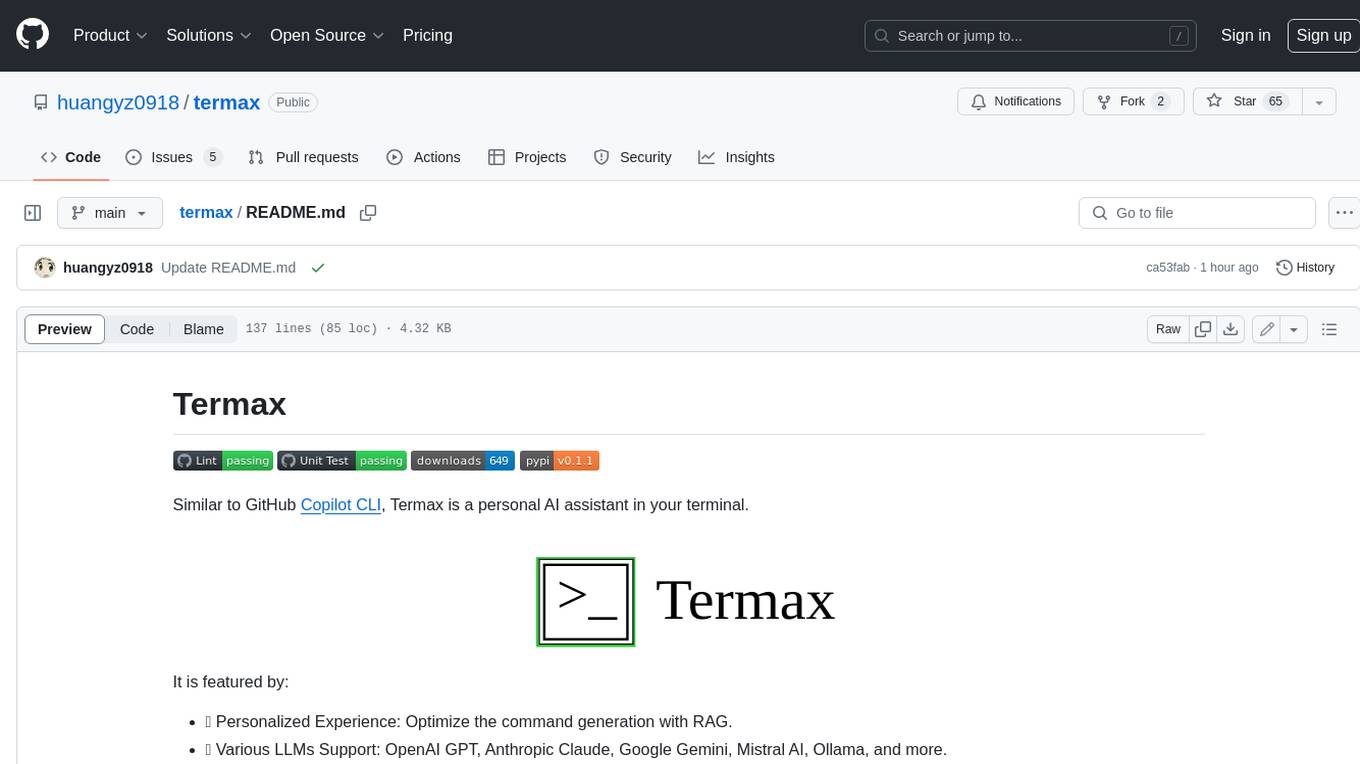
termax
Termax is an LLM agent in your terminal that converts natural language to commands. It is featured by: - Personalized Experience: Optimize the command generation with RAG. - Various LLMs Support: OpenAI GPT, Anthropic Claude, Google Gemini, Mistral AI, and more. - Shell Extensions: Plugin with popular shells like `zsh`, `bash` and `fish`. - Cross Platform: Able to run on Windows, macOS, and Linux.
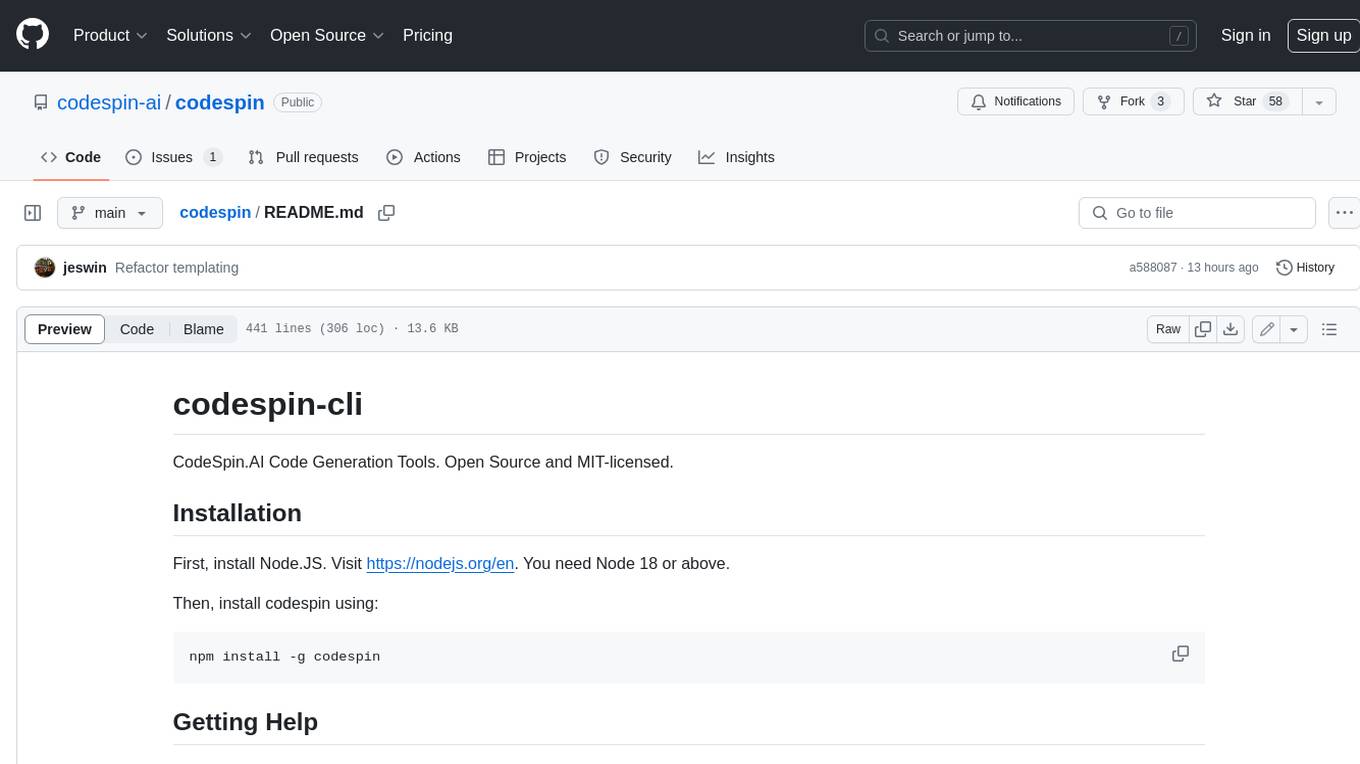
codespin
CodeSpin.AI is a set of open-source code generation tools that leverage large language models (LLMs) to automate coding tasks. With CodeSpin, you can generate code in various programming languages, including Python, JavaScript, Java, and C++, by providing natural language prompts. CodeSpin offers a range of features to enhance code generation, such as custom templates, inline prompting, and the ability to use ChatGPT as an alternative to API keys. Additionally, CodeSpin provides options for regenerating code, executing code in prompt files, and piping data into the LLM for processing. By utilizing CodeSpin, developers can save time and effort in coding tasks, improve code quality, and explore new possibilities in code generation.
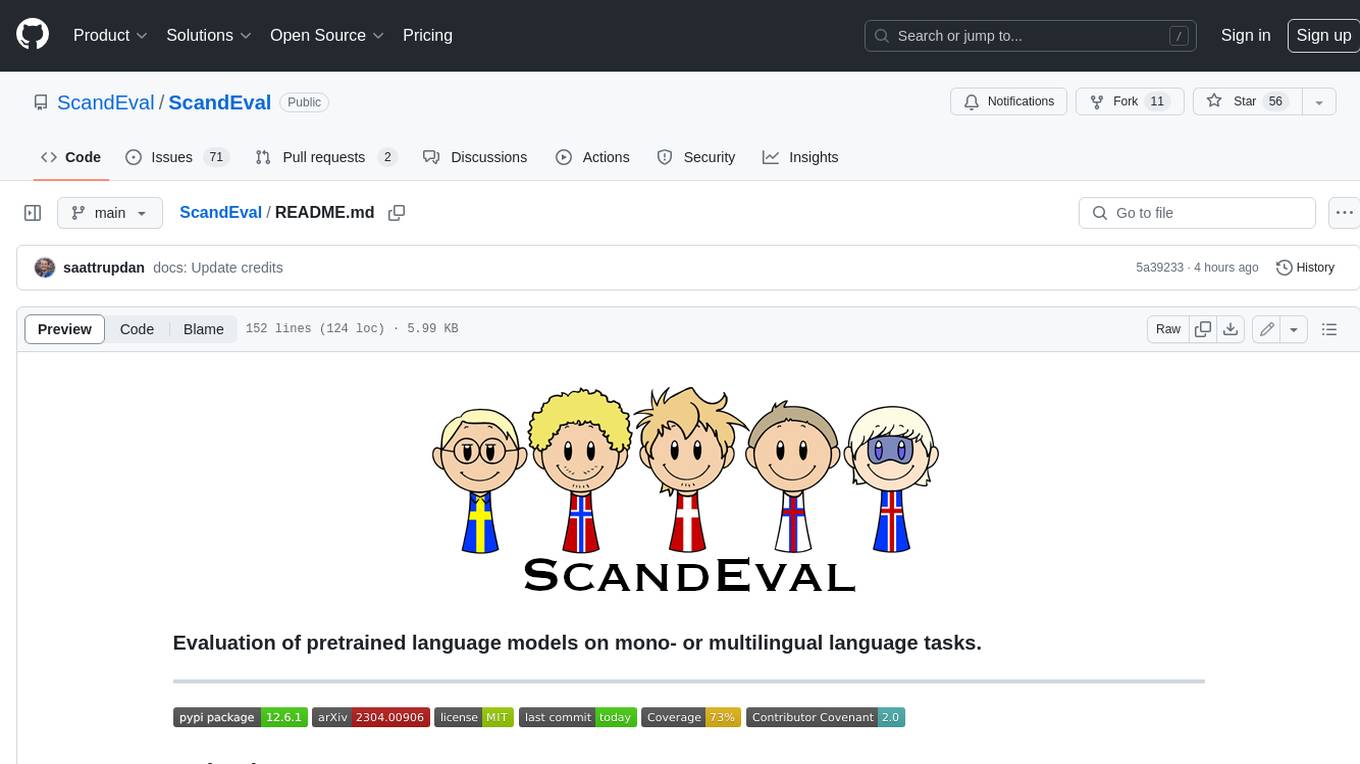
ScandEval
ScandEval is a framework for evaluating pretrained language models on mono- or multilingual language tasks. It provides a unified interface for benchmarking models on a variety of tasks, including sentiment analysis, question answering, and machine translation. ScandEval is designed to be easy to use and extensible, making it a valuable tool for researchers and practitioners alike.
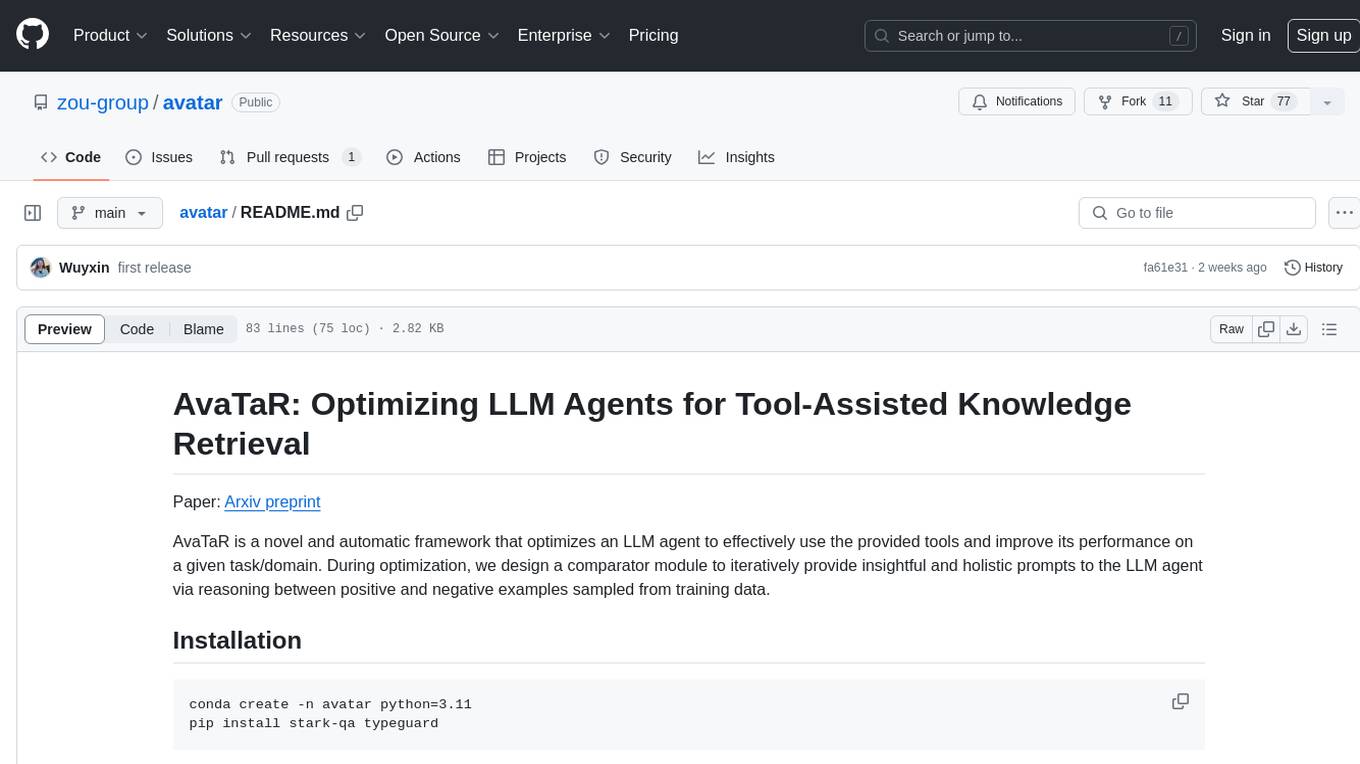
avatar
AvaTaR is a novel and automatic framework that optimizes an LLM agent to effectively use provided tools and improve performance on a given task/domain. It designs a comparator module to provide insightful prompts to the LLM agent via reasoning between positive and negative examples from training data.
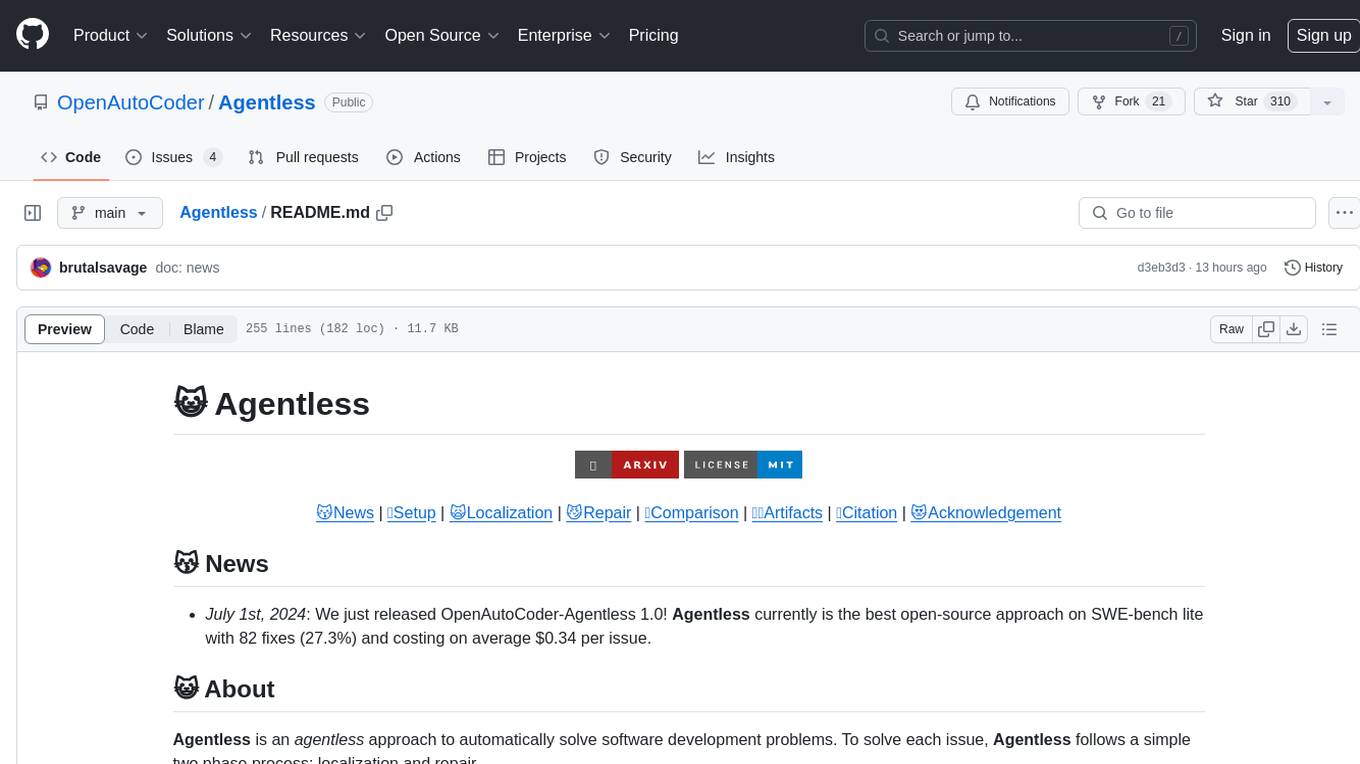
Agentless
Agentless is an open-source tool designed for automatically solving software development problems. It follows a two-phase process of localization and repair to identify faults in specific files, classes, and functions, and generate candidate patches for fixing issues. The tool is aimed at simplifying the software development process by automating issue resolution and patch generation.
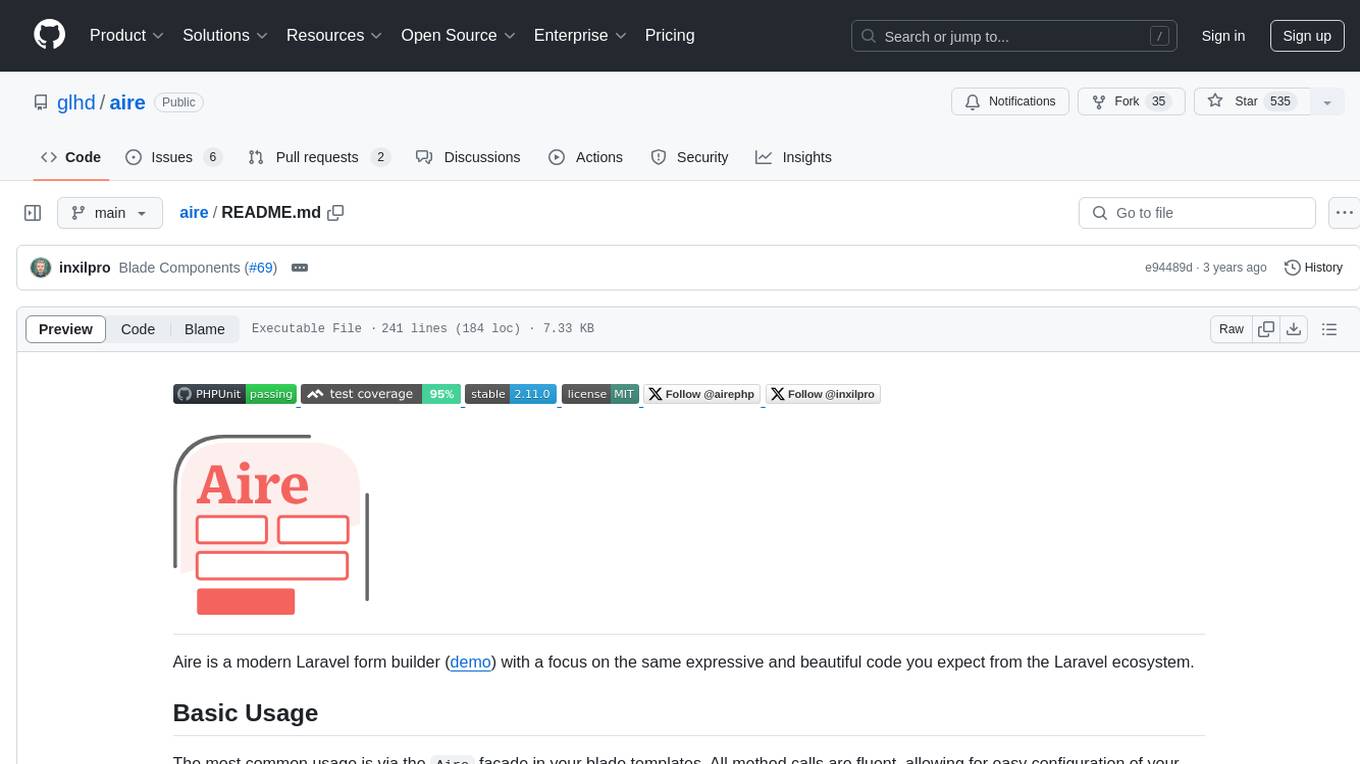
aire
Aire is a modern Laravel form builder with a focus on expressive and beautiful code. It allows easy configuration of form components using fluent method calls or Blade components. Aire supports customization through config files and custom views, data binding with Eloquent models or arrays, method spoofing, CSRF token injection, server-side and client-side validation, and translations. It is designed to run on Laravel 5.8.28 and higher, with support for PHP 7.1 and higher. Aire is actively maintained and under consideration for additional features like read-only plain text, cross-browser support for custom checkboxes and radio buttons, support for Choices.js or similar libraries, improved file input handling, and better support for content prepending or appending to inputs.
For similar tasks
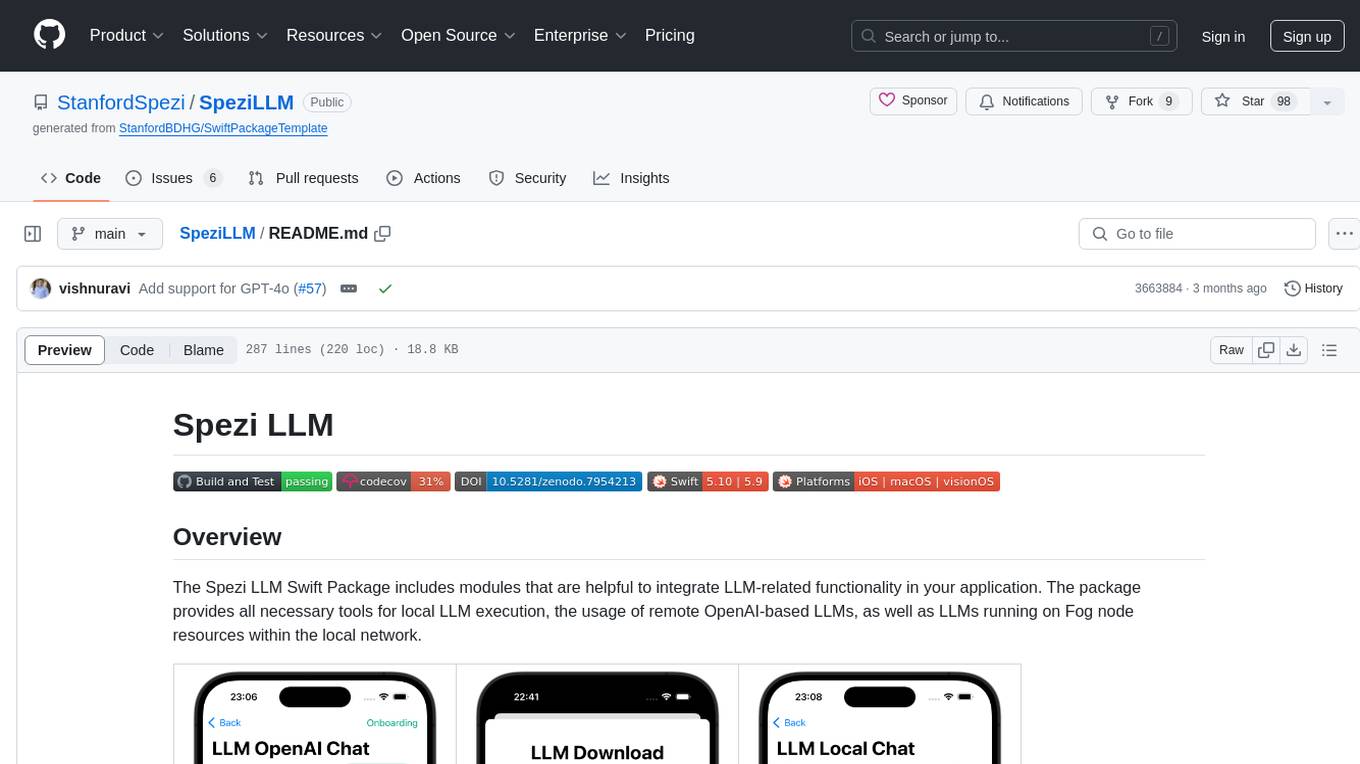
SpeziLLM
The Spezi LLM Swift Package includes modules that help integrate LLM-related functionality in applications. It provides tools for local LLM execution, usage of remote OpenAI-based LLMs, and LLMs running on Fog node resources within the local network. The package contains targets like SpeziLLM, SpeziLLMLocal, SpeziLLMLocalDownload, SpeziLLMOpenAI, and SpeziLLMFog for different LLM functionalities. Users can configure and interact with local LLMs, OpenAI LLMs, and Fog LLMs using the provided APIs and platforms within the Spezi ecosystem.
For similar jobs
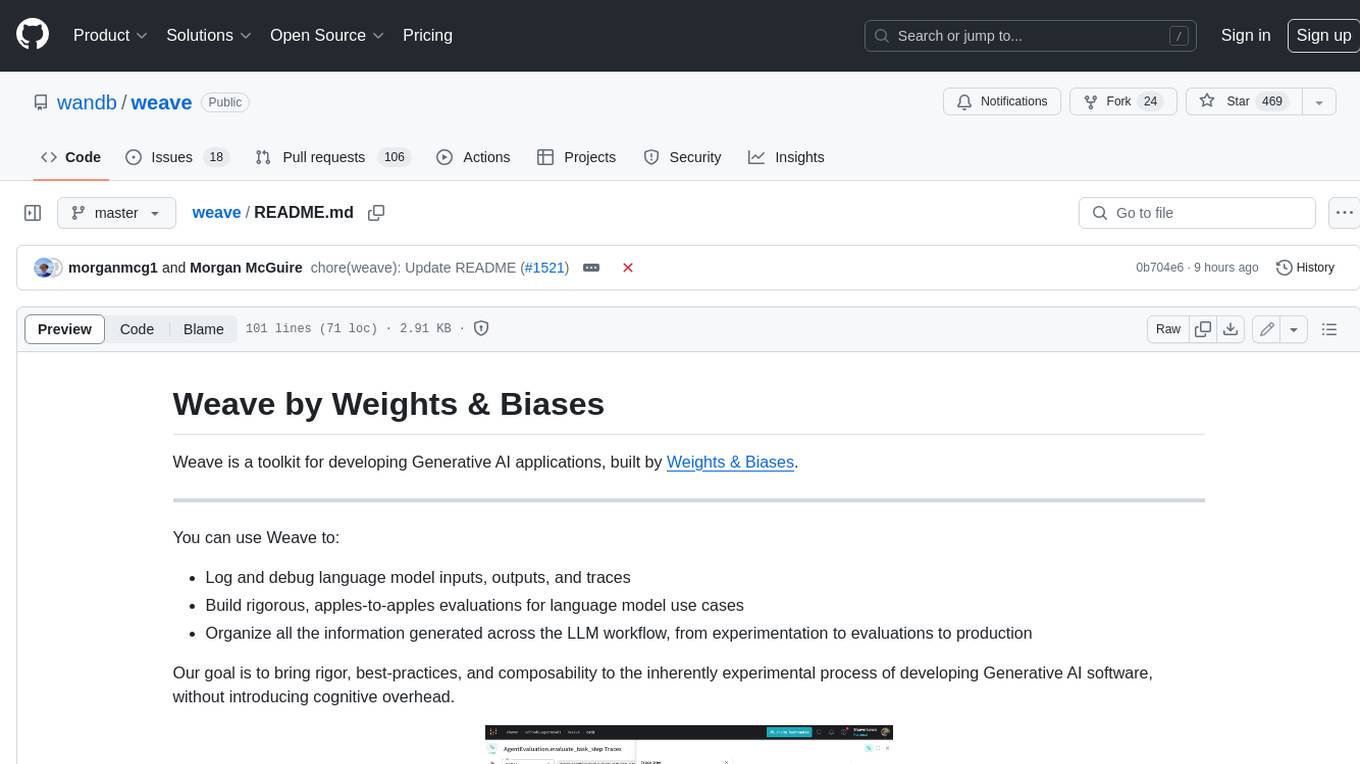
weave
Weave is a toolkit for developing Generative AI applications, built by Weights & Biases. With Weave, you can log and debug language model inputs, outputs, and traces; build rigorous, apples-to-apples evaluations for language model use cases; and organize all the information generated across the LLM workflow, from experimentation to evaluations to production. Weave aims to bring rigor, best-practices, and composability to the inherently experimental process of developing Generative AI software, without introducing cognitive overhead.
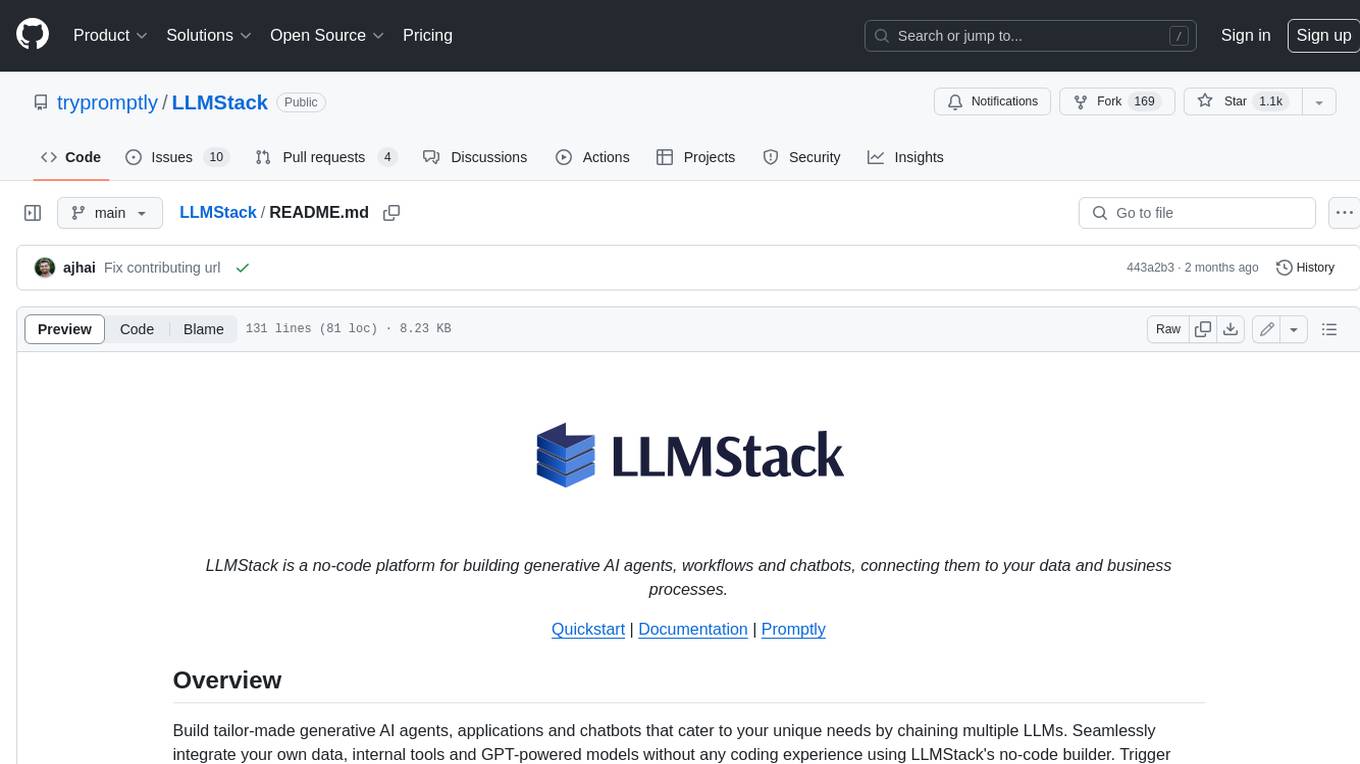
LLMStack
LLMStack is a no-code platform for building generative AI agents, workflows, and chatbots. It allows users to connect their own data, internal tools, and GPT-powered models without any coding experience. LLMStack can be deployed to the cloud or on-premise and can be accessed via HTTP API or triggered from Slack or Discord.
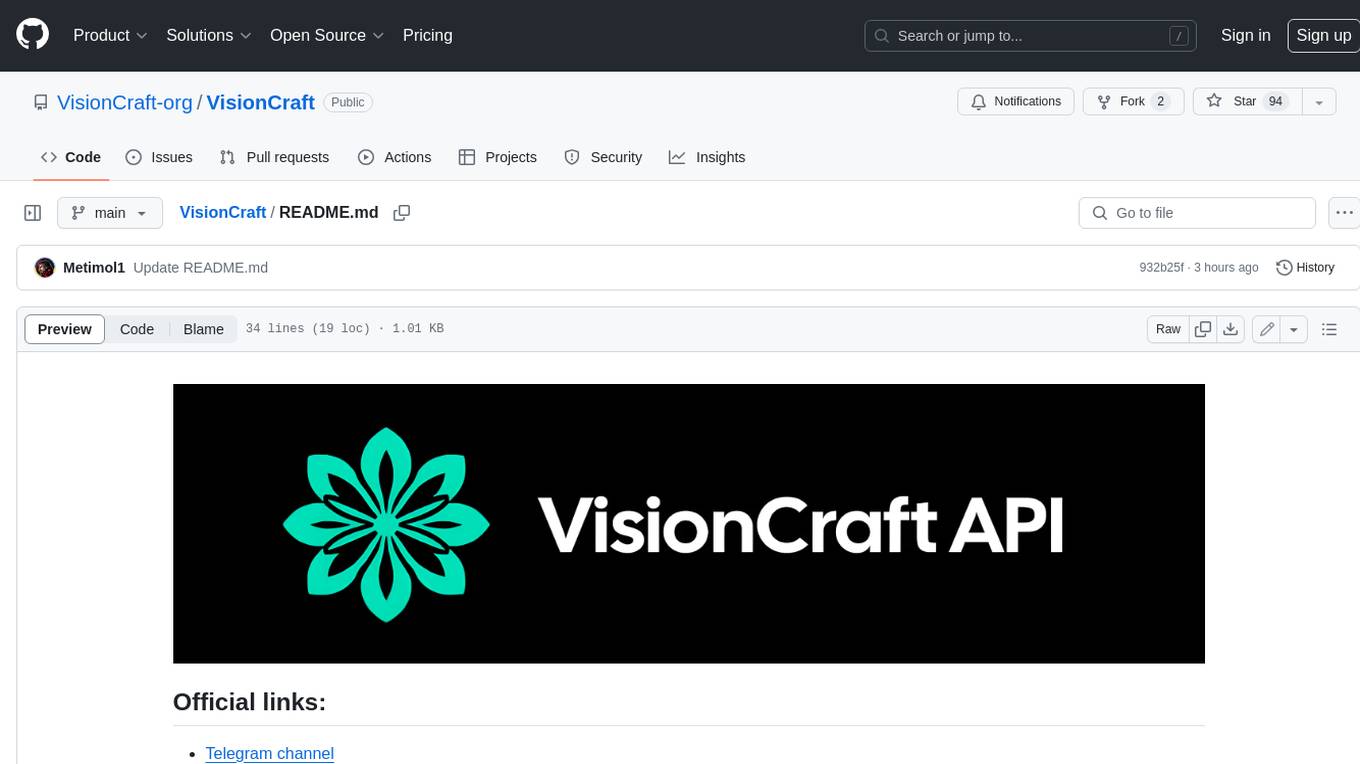
VisionCraft
The VisionCraft API is a free API for using over 100 different AI models. From images to sound.
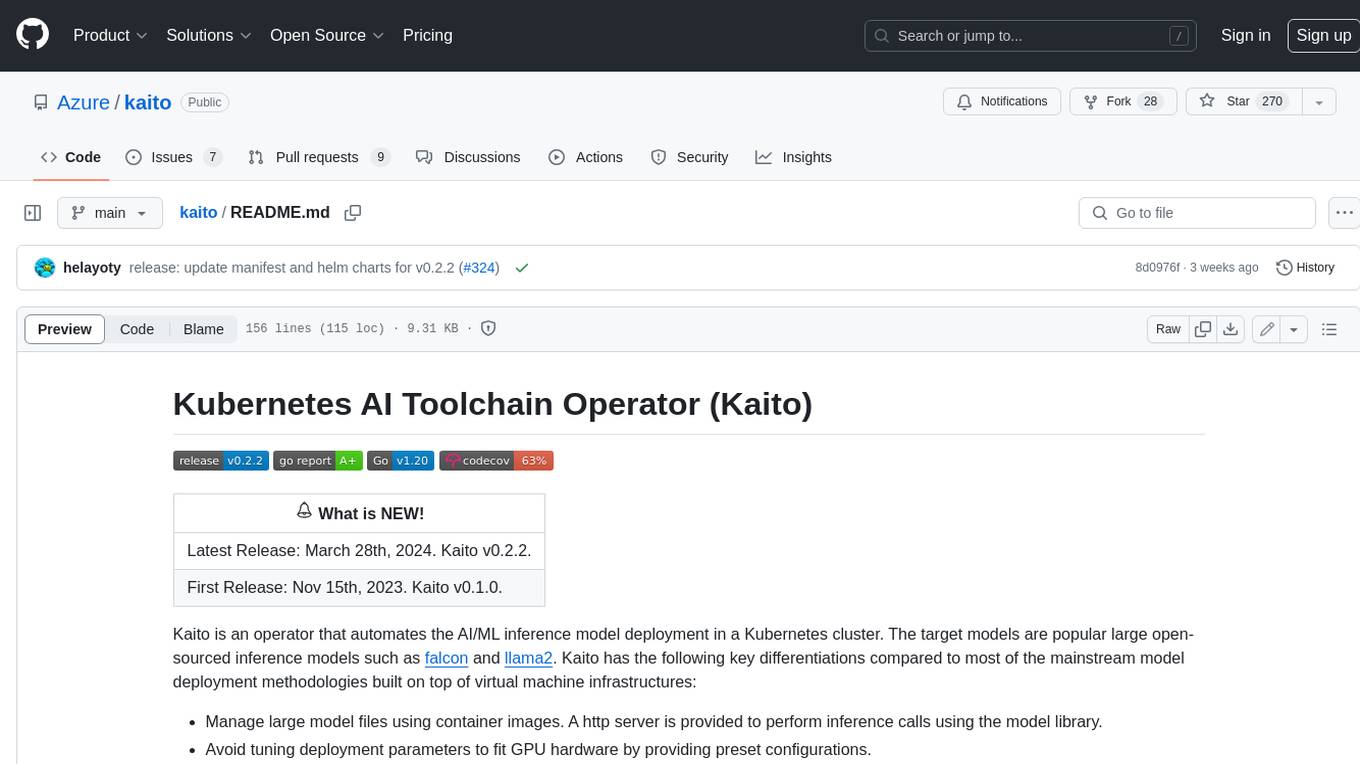
kaito
Kaito is an operator that automates the AI/ML inference model deployment in a Kubernetes cluster. It manages large model files using container images, avoids tuning deployment parameters to fit GPU hardware by providing preset configurations, auto-provisions GPU nodes based on model requirements, and hosts large model images in the public Microsoft Container Registry (MCR) if the license allows. Using Kaito, the workflow of onboarding large AI inference models in Kubernetes is largely simplified.
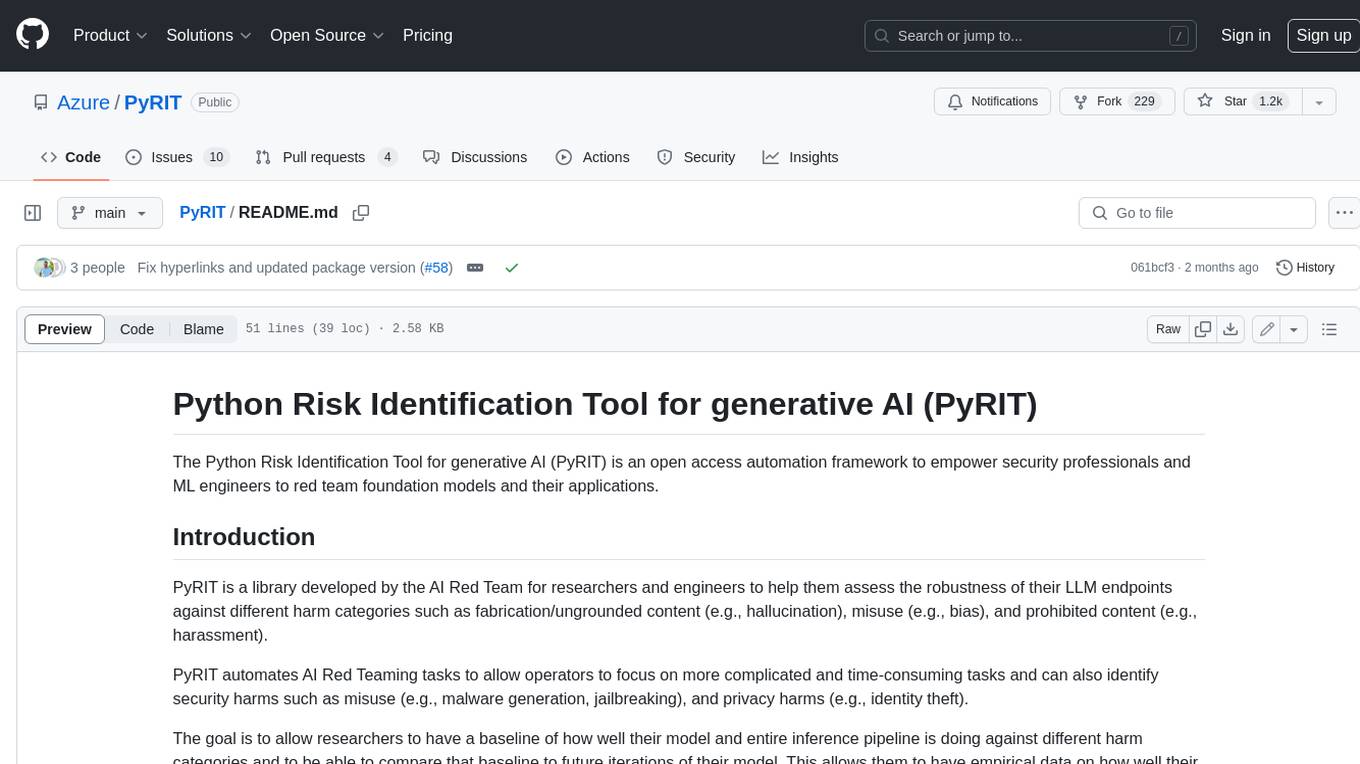
PyRIT
PyRIT is an open access automation framework designed to empower security professionals and ML engineers to red team foundation models and their applications. It automates AI Red Teaming tasks to allow operators to focus on more complicated and time-consuming tasks and can also identify security harms such as misuse (e.g., malware generation, jailbreaking), and privacy harms (e.g., identity theft). The goal is to allow researchers to have a baseline of how well their model and entire inference pipeline is doing against different harm categories and to be able to compare that baseline to future iterations of their model. This allows them to have empirical data on how well their model is doing today, and detect any degradation of performance based on future improvements.
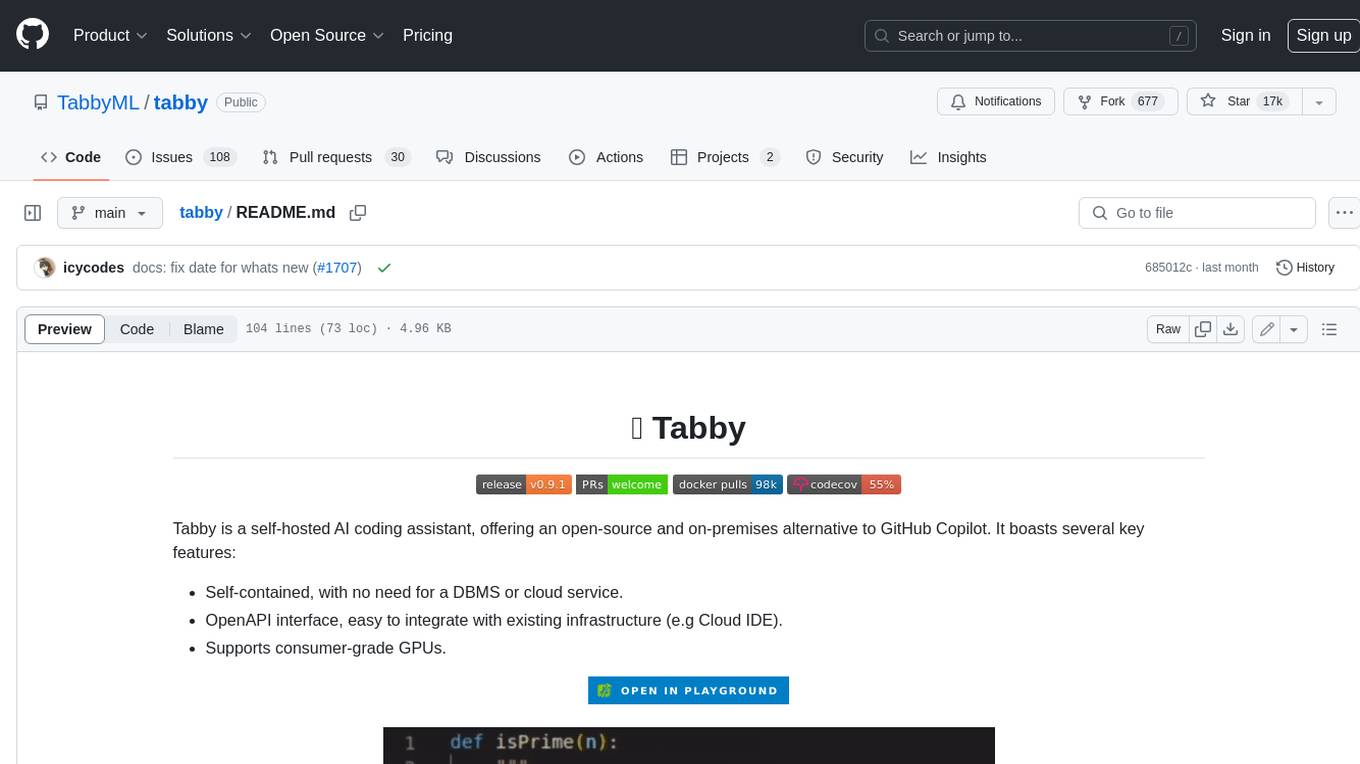
tabby
Tabby is a self-hosted AI coding assistant, offering an open-source and on-premises alternative to GitHub Copilot. It boasts several key features: * Self-contained, with no need for a DBMS or cloud service. * OpenAPI interface, easy to integrate with existing infrastructure (e.g Cloud IDE). * Supports consumer-grade GPUs.
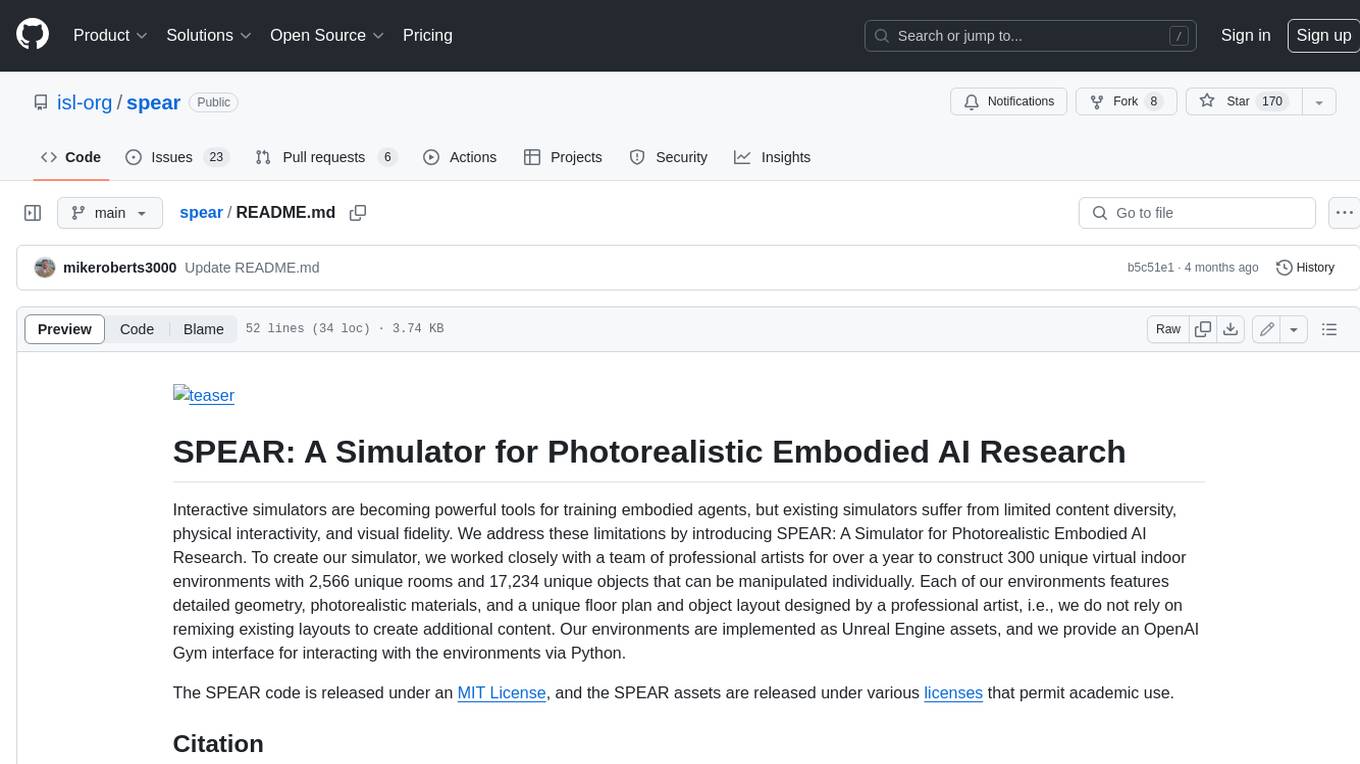
spear
SPEAR (Simulator for Photorealistic Embodied AI Research) is a powerful tool for training embodied agents. It features 300 unique virtual indoor environments with 2,566 unique rooms and 17,234 unique objects that can be manipulated individually. Each environment is designed by a professional artist and features detailed geometry, photorealistic materials, and a unique floor plan and object layout. SPEAR is implemented as Unreal Engine assets and provides an OpenAI Gym interface for interacting with the environments via Python.
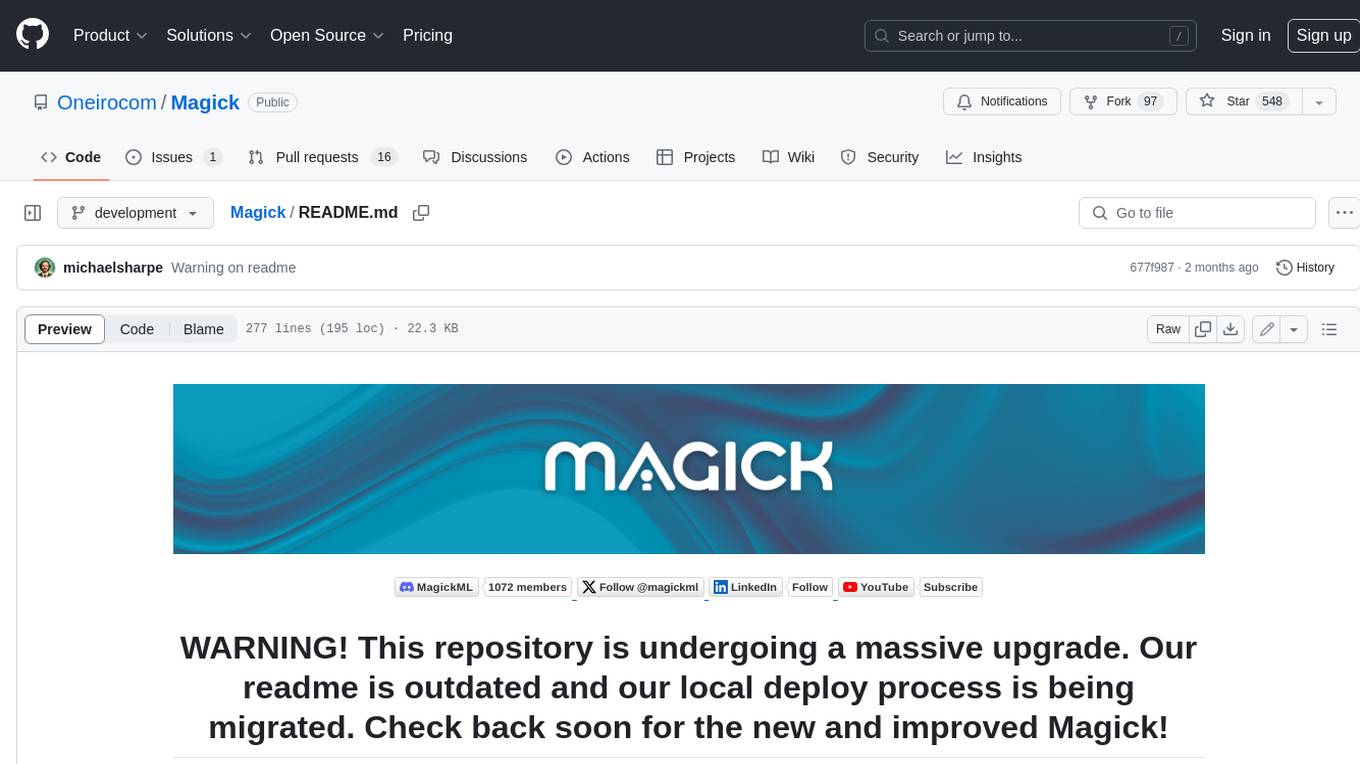
Magick
Magick is a groundbreaking visual AIDE (Artificial Intelligence Development Environment) for no-code data pipelines and multimodal agents. Magick can connect to other services and comes with nodes and templates well-suited for intelligent agents, chatbots, complex reasoning systems and realistic characters.