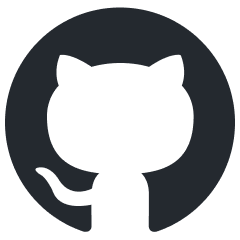
aiohttp-client-cache
An async persistent cache for aiohttp requests
Stars: 118
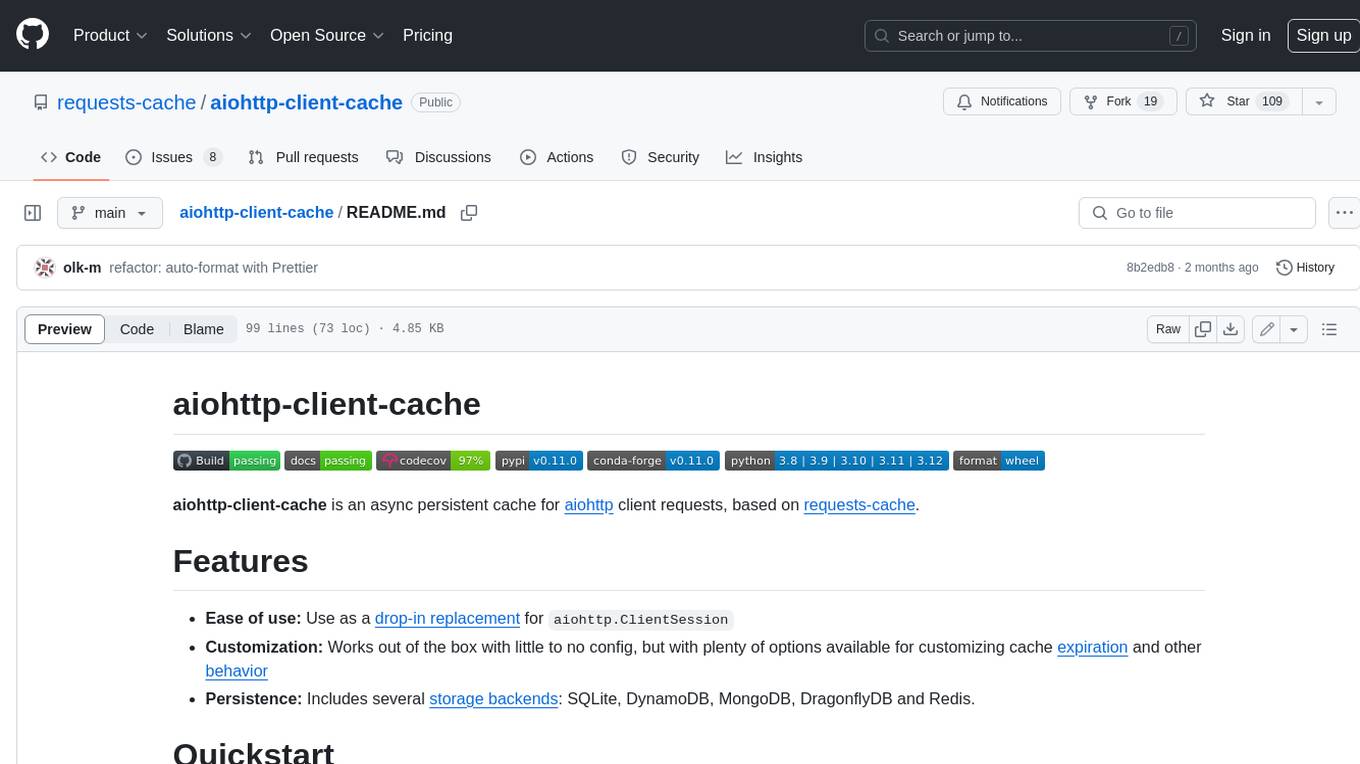
aiohttp-client-cache is an asynchronous persistent cache for aiohttp client requests, based on requests-cache. It is easy to use, customizable, and persistent, with several storage backends available, including SQLite, DynamoDB, MongoDB, DragonflyDB, and Redis.
README:
aiohttp-client-cache is an async persistent cache for aiohttp client requests, based on requests-cache.
-
Ease of use: Use as a drop-in replacement
for
aiohttp.ClientSession
- Customization: Works out of the box with little to no config, but with plenty of options available for customizing cache expiration and other behavior
- Persistence: Includes several storage backends: SQLite, DynamoDB, MongoDB, DragonflyDB and Redis.
First, install with pip (python 3.8+ required):
pip install aiohttp-client-cache[all]
Note:
Adding [all]
will install optional dependencies for all supported backends. When adding this
library to your application, you can include only the dependencies you actually need; see individual
backend docs and pyproject.toml
for details.
Next, use aiohttp_client_cache.CachedSession in place of aiohttp.ClientSession. To briefly demonstrate how to use it:
Replace this:
from aiohttp import ClientSession
async with ClientSession() as session:
await session.get('http://httpbin.org/delay/1')
With this:
from aiohttp_client_cache import CachedSession, SQLiteBackend
async with CachedSession(cache=SQLiteBackend('demo_cache')) as session:
await session.get('http://httpbin.org/delay/1')
The URL in this example adds a delay of 1 second, simulating a slow or rate-limited website.
With caching, the response will be fetched once, saved to demo_cache.sqlite
, and subsequent
requests will return the cached response near-instantly.
Several options are available to customize caching behavior. This example demonstrates a few of them:
# fmt: off
from aiohttp_client_cache import SQLiteBackend
cache = SQLiteBackend(
cache_name='~/.cache/aiohttp-requests.db', # For SQLite, this will be used as the filename
expire_after=60*60, # By default, cached responses expire in an hour
urls_expire_after={'*.fillmurray.com': -1}, # Requests for any subdomain on this site will never expire
allowed_codes=(200, 418), # Cache responses with these status codes
allowed_methods=['GET', 'POST'], # Cache requests with these HTTP methods
include_headers=True, # Cache requests with different headers separately
ignored_params=['auth_token'], # Keep using the cached response even if this param changes
timeout=2.5, # Connection timeout for SQLite backend
)
To learn more, see:
If there is a feature you want, if you've discovered a bug, or if you have other general feedback, please create an issue for it!
For Tasks:
Click tags to check more tools for each tasksFor Jobs:
Alternative AI tools for aiohttp-client-cache
Similar Open Source Tools
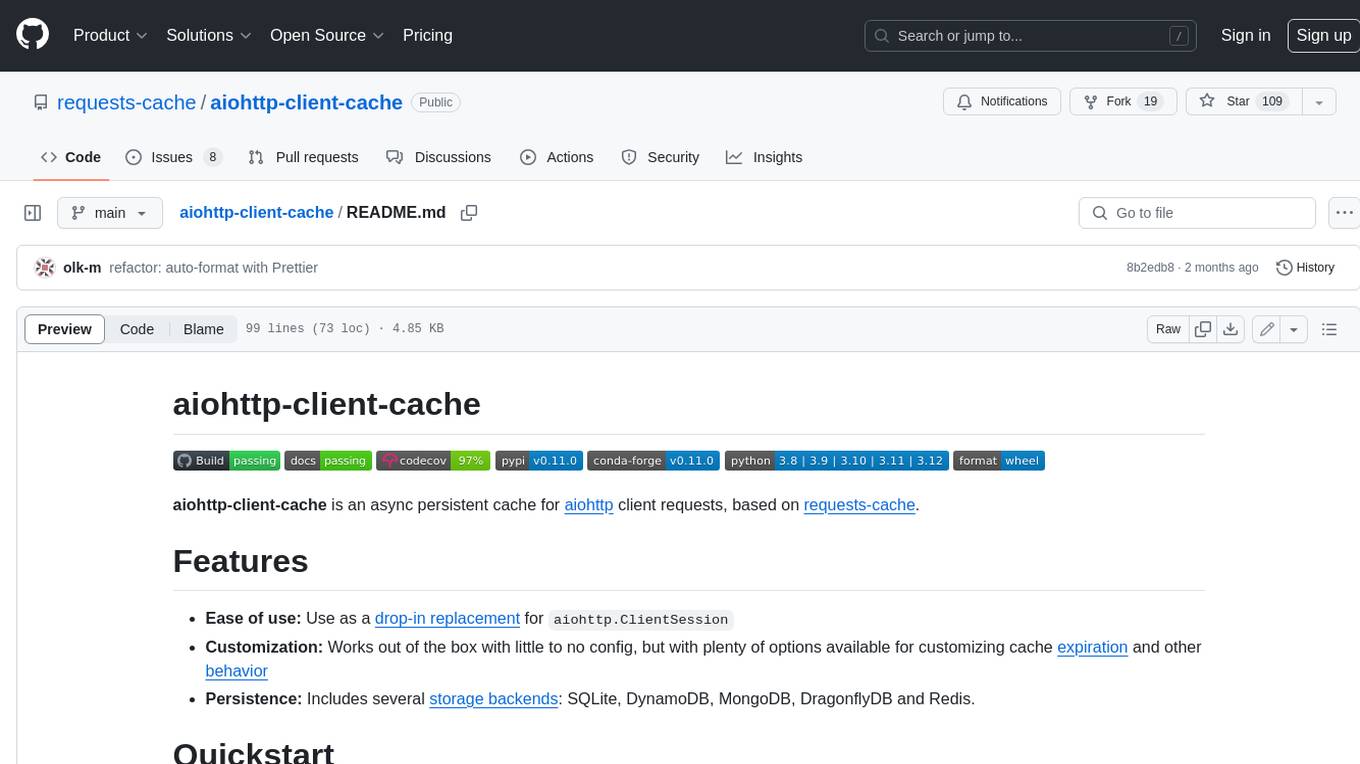
aiohttp-client-cache
aiohttp-client-cache is an asynchronous persistent cache for aiohttp client requests, based on requests-cache. It is easy to use, customizable, and persistent, with several storage backends available, including SQLite, DynamoDB, MongoDB, DragonflyDB, and Redis.
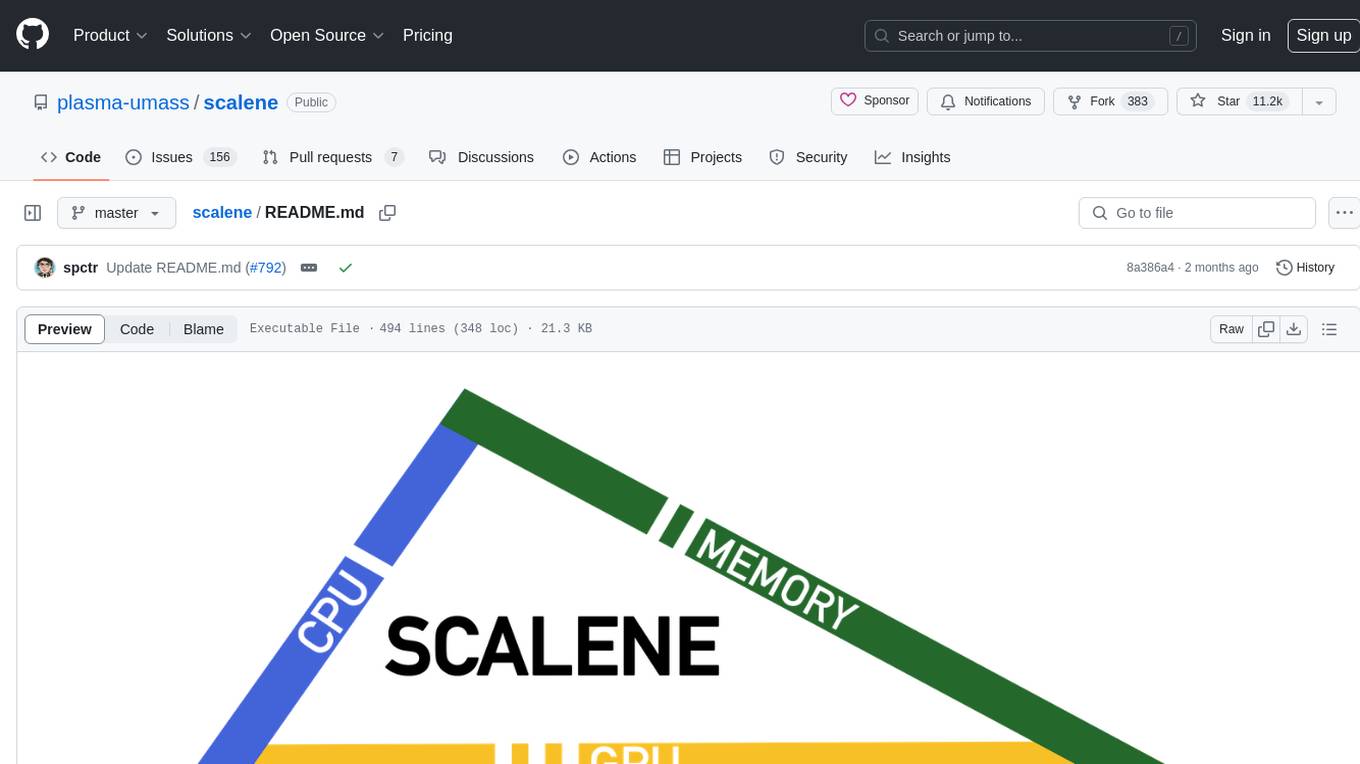
scalene
Scalene is a high-performance CPU, GPU, and memory profiler for Python that provides detailed information and runs faster than many other profilers. It incorporates AI-powered proposed optimizations, allowing users to generate optimization suggestions by clicking on specific lines or regions of code. Scalene separates time spent in Python from native code, highlights hotspots, and identifies memory usage per line. It supports GPU profiling on NVIDIA-based systems and detects memory leaks. Users can generate reduced profiles, profile specific functions using decorators, and suspend/resume profiling for background processes. Scalene is available as a pip or conda package and works on various platforms. It offers features like profiling at the line level, memory trends, copy volume reporting, and leak detection.
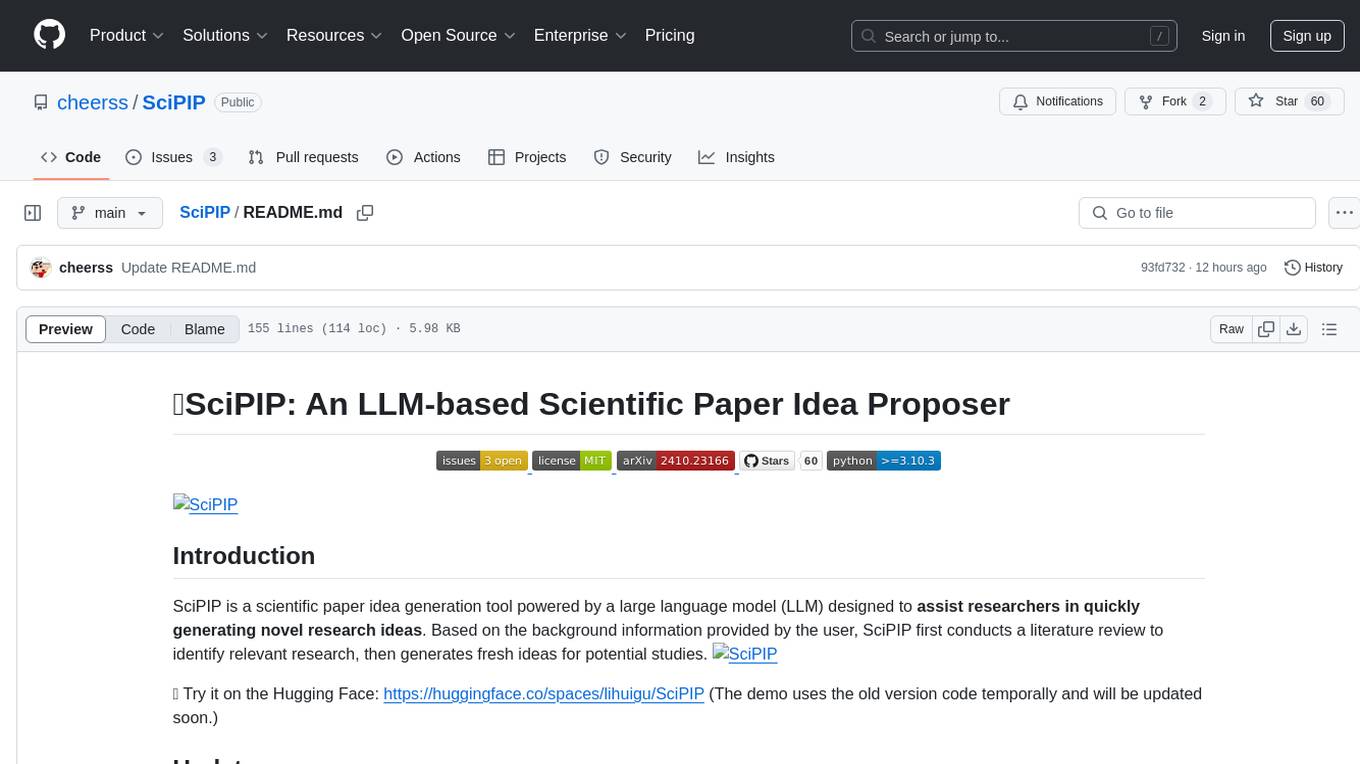
SciPIP
SciPIP is a scientific paper idea generation tool powered by a large language model (LLM) designed to assist researchers in quickly generating novel research ideas. It conducts a literature review based on user-provided background information and generates fresh ideas for potential studies. The tool is designed to help researchers in various fields by providing a GUI environment for idea generation, supporting NLP, multimodal, and CV fields, and allowing users to interact with the tool through a web app or terminal. SciPIP uses Neo4j as its database and provides functionalities for generating new ideas, fetching papers, and constructing the database.
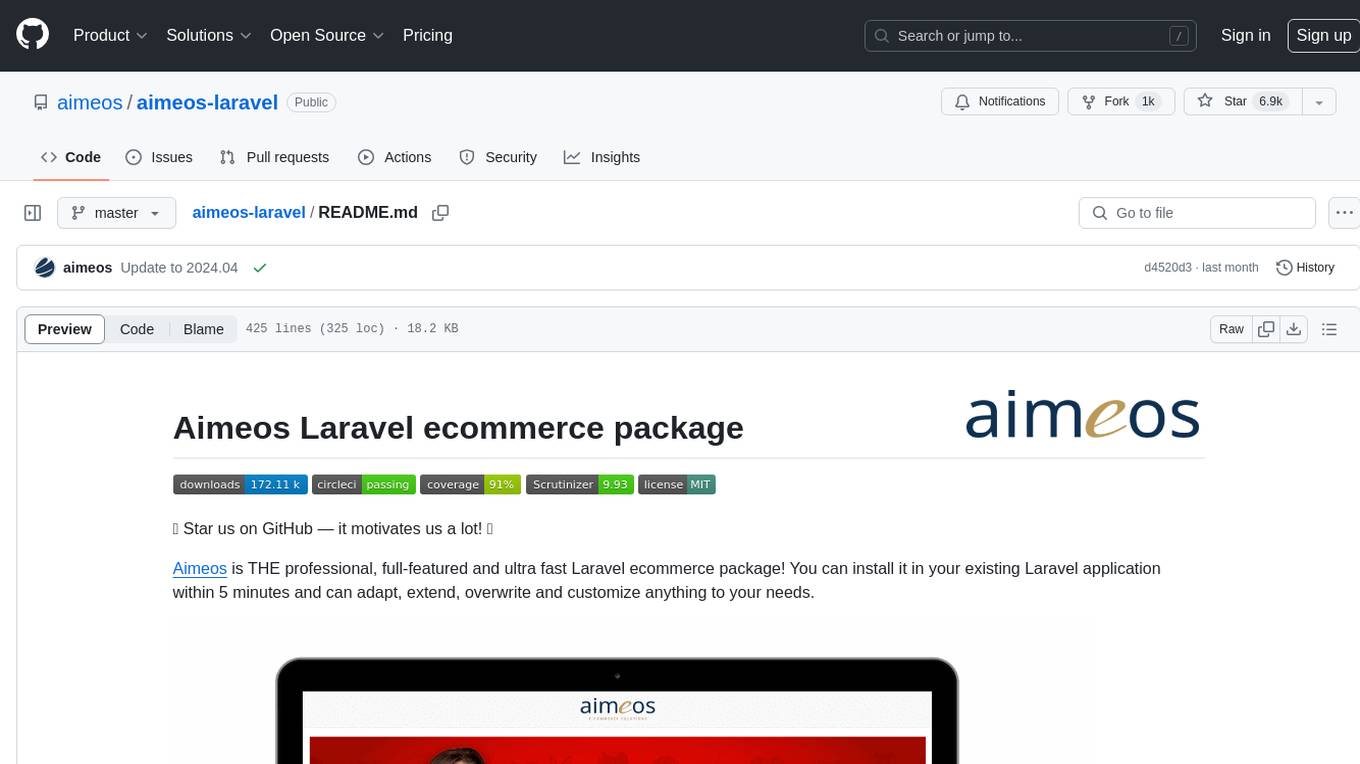
aimeos-laravel
Aimeos Laravel is a professional, full-featured, and ultra-fast Laravel ecommerce package that can be easily integrated into existing Laravel applications. It offers a wide range of features including multi-vendor, multi-channel, and multi-warehouse support, fast performance, support for various product types, subscriptions with recurring payments, multiple payment gateways, full RTL support, flexible pricing options, admin backend, REST and GraphQL APIs, modular structure, SEO optimization, multi-language support, AI-based text translation, mobile optimization, and high-quality source code. The package is highly configurable and extensible, making it suitable for e-commerce SaaS solutions, marketplaces, and online shops with millions of vendors.
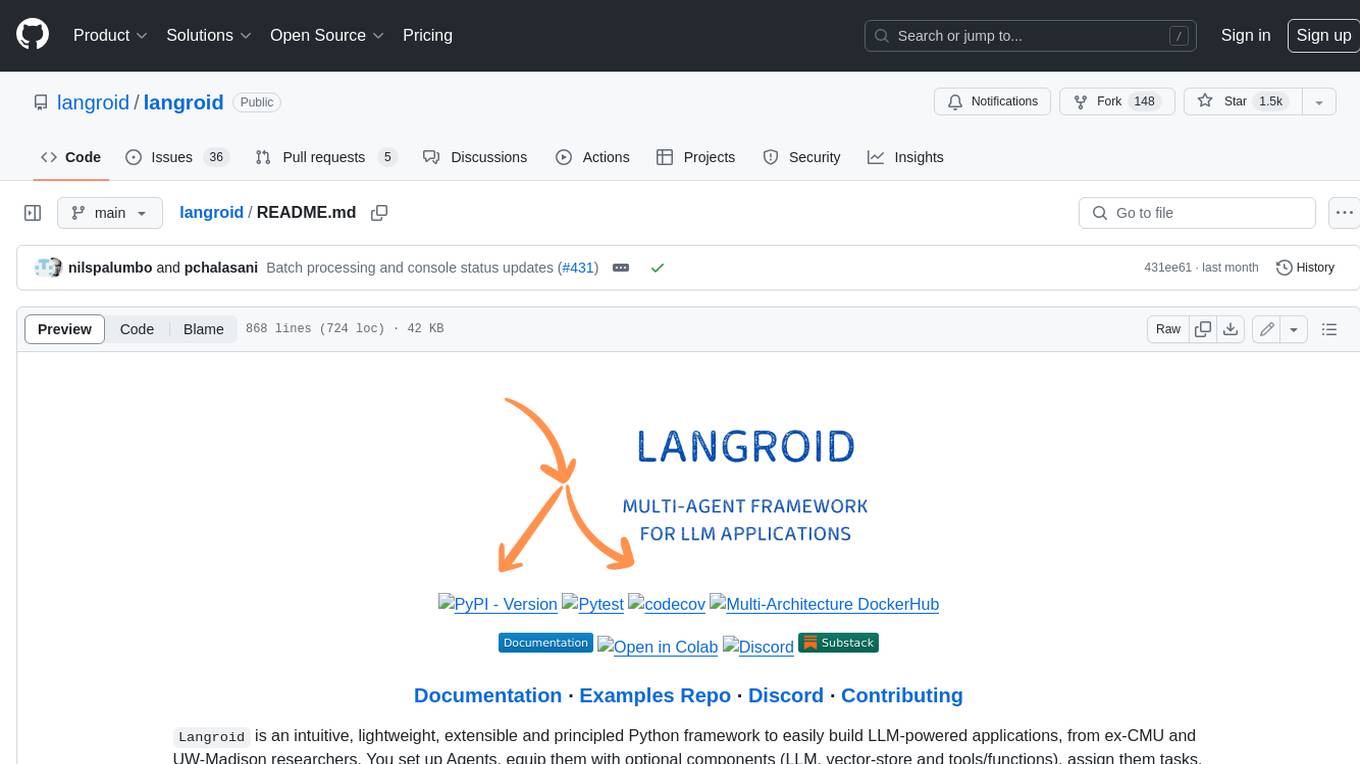
langroid
Langroid is a Python framework that makes it easy to build LLM-powered applications. It uses a multi-agent paradigm inspired by the Actor Framework, where you set up Agents, equip them with optional components (LLM, vector-store and tools/functions), assign them tasks, and have them collaboratively solve a problem by exchanging messages. Langroid is a fresh take on LLM app-development, where considerable thought has gone into simplifying the developer experience; it does not use Langchain.
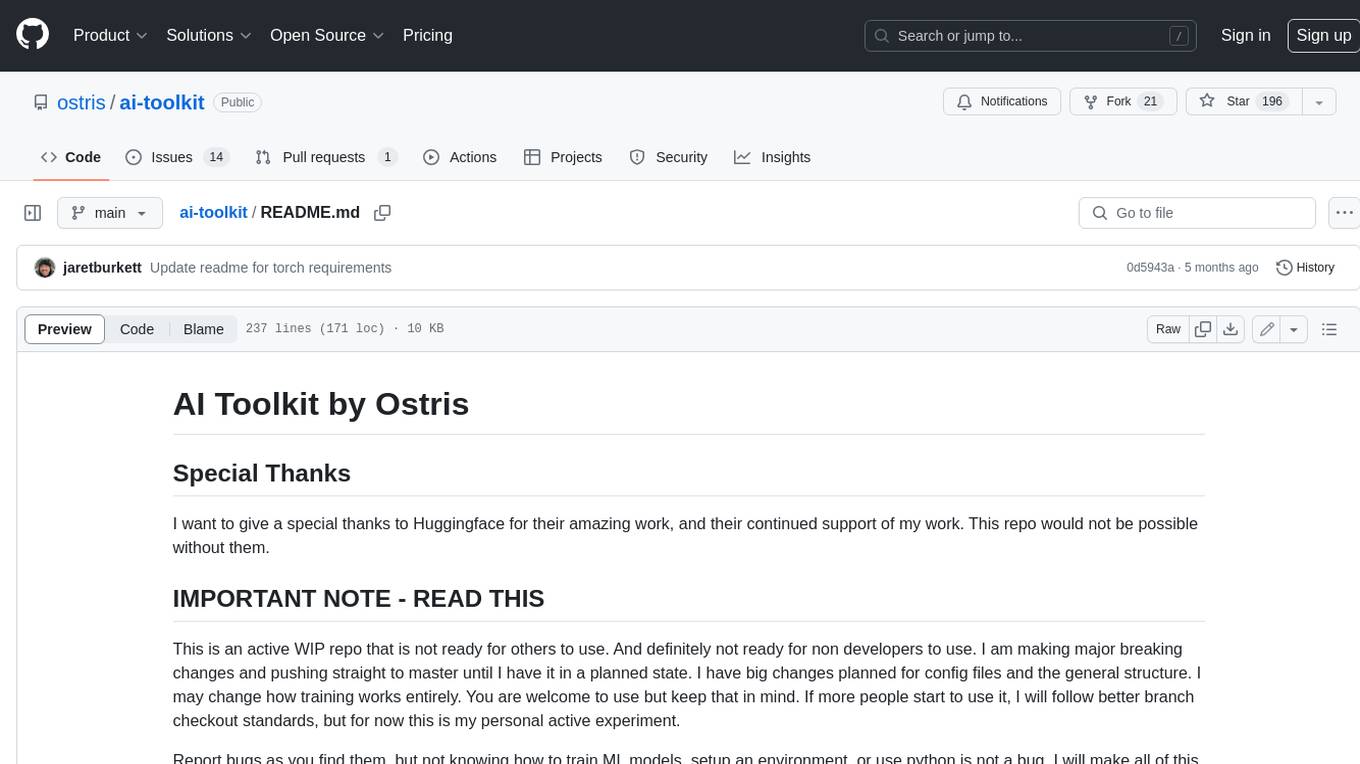
ai-toolkit
The AI Toolkit by Ostris is a collection of tools for machine learning, specifically designed for image generation, LoRA (latent representations of attributes) extraction and manipulation, and model training. It provides a user-friendly interface and extensive documentation to make it accessible to both developers and non-developers. The toolkit is actively under development, with new features and improvements being added regularly. Some of the key features of the AI Toolkit include: - Batch Image Generation: Allows users to generate a batch of images based on prompts or text files, using a configuration file to specify the desired settings. - LoRA (lierla), LoCON (LyCORIS) Extractor: Facilitates the extraction of LoRA and LoCON representations from pre-trained models, enabling users to modify and manipulate these representations for various purposes. - LoRA Rescale: Provides a tool to rescale LoRA weights, allowing users to adjust the influence of specific attributes in the generated images. - LoRA Slider Trainer: Enables the training of LoRA sliders, which can be used to control and adjust specific attributes in the generated images, offering a powerful tool for fine-tuning and customization. - Extensions: Supports the creation and sharing of custom extensions, allowing users to extend the functionality of the toolkit with their own tools and scripts. - VAE (Variational Auto Encoder) Trainer: Facilitates the training of VAEs for image generation, providing users with a tool to explore and improve the quality of generated images. The AI Toolkit is a valuable resource for anyone interested in exploring and utilizing machine learning for image generation and manipulation. Its user-friendly interface, extensive documentation, and active development make it an accessible and powerful tool for both beginners and experienced users.
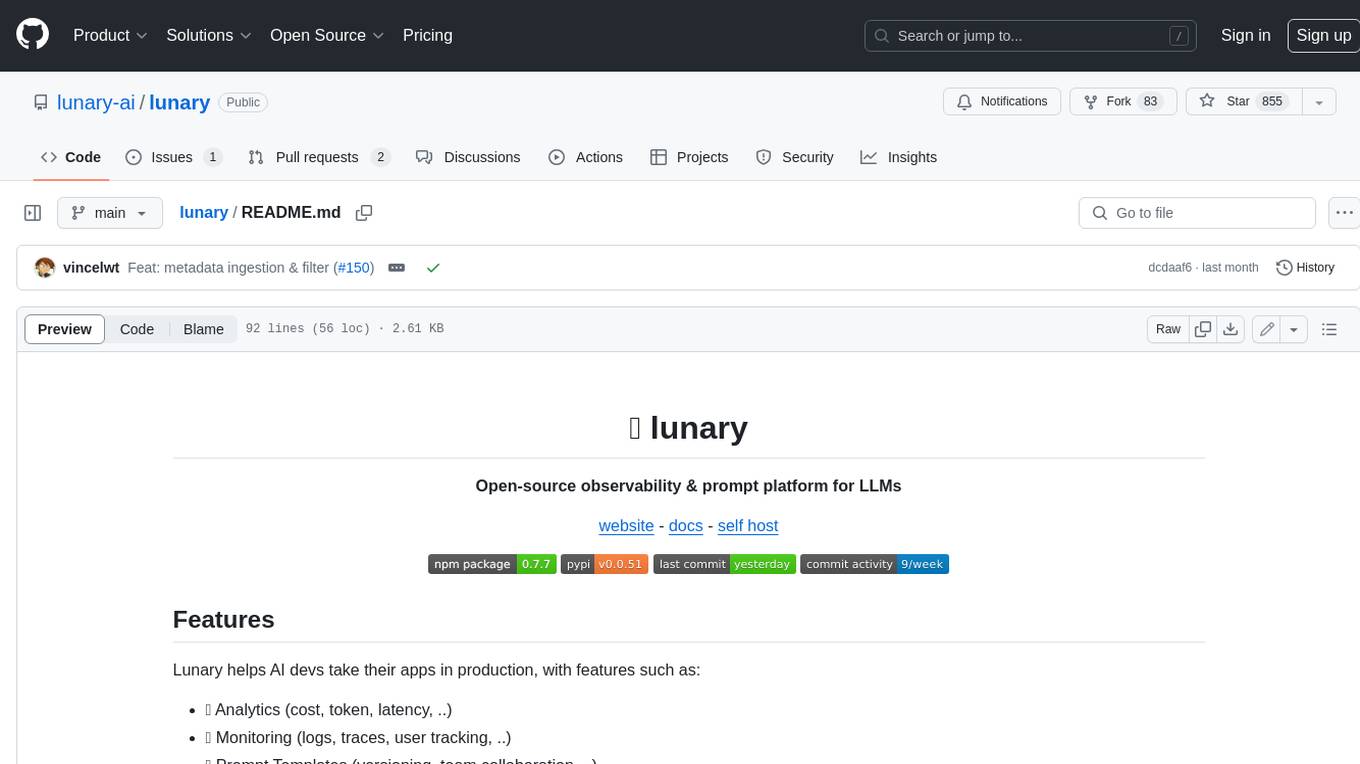
lunary
Lunary is an open-source observability and prompt platform for Large Language Models (LLMs). It provides a suite of features to help AI developers take their applications into production, including analytics, monitoring, prompt templates, fine-tuning dataset creation, chat and feedback tracking, and evaluations. Lunary is designed to be usable with any model, not just OpenAI, and is easy to integrate and self-host.
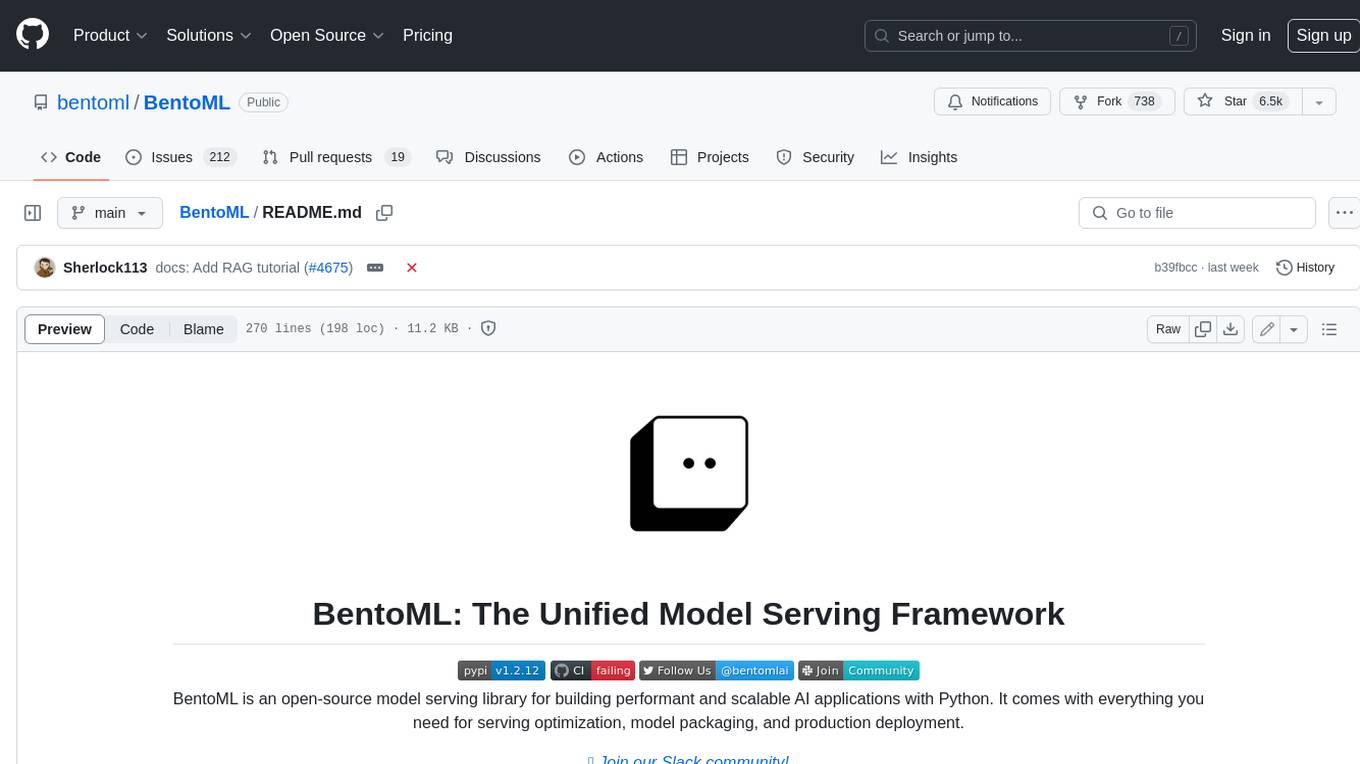
BentoML
BentoML is an open-source model serving library for building performant and scalable AI applications with Python. It comes with everything you need for serving optimization, model packaging, and production deployment.
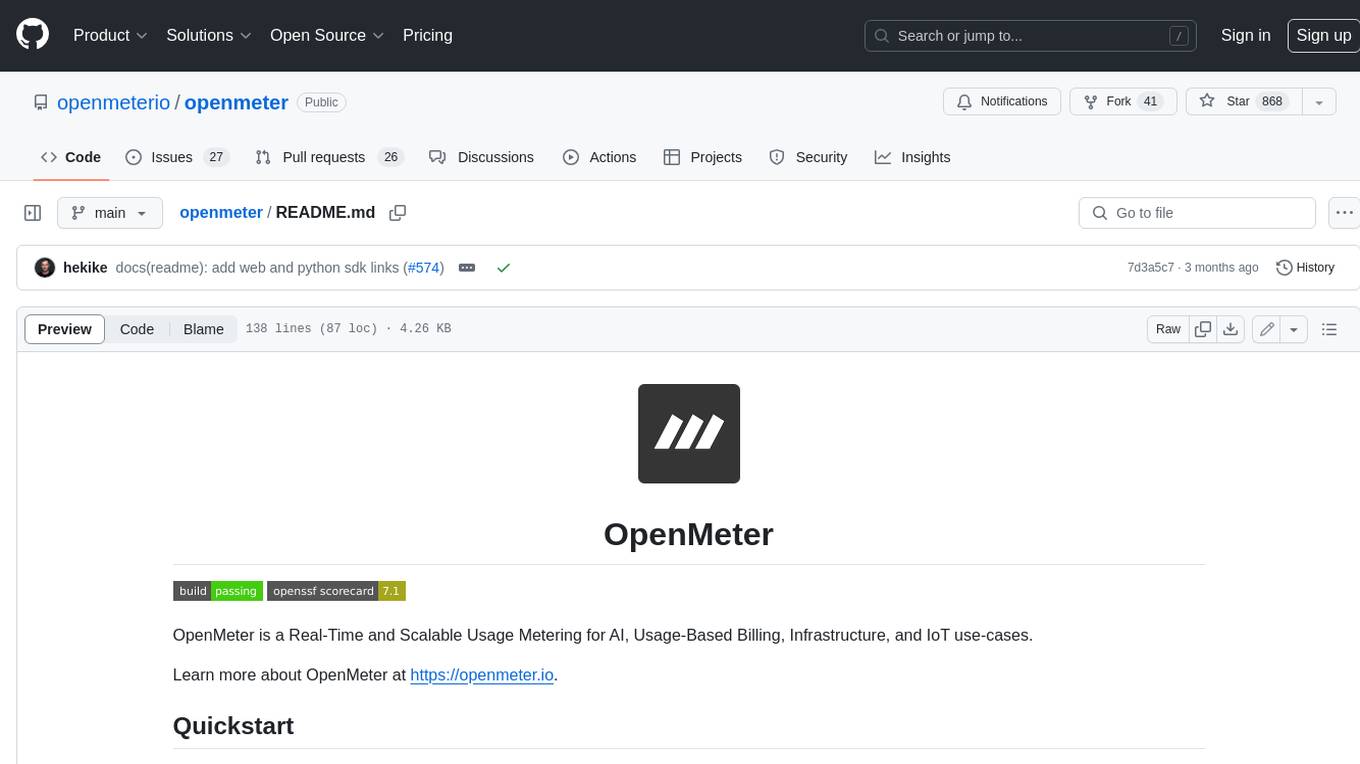
openmeter
OpenMeter is a real-time and scalable usage metering tool for AI, usage-based billing, infrastructure, and IoT use cases. It provides a REST API for integrations and offers client SDKs in Node.js, Python, Go, and Web. OpenMeter is licensed under the Apache 2.0 License.
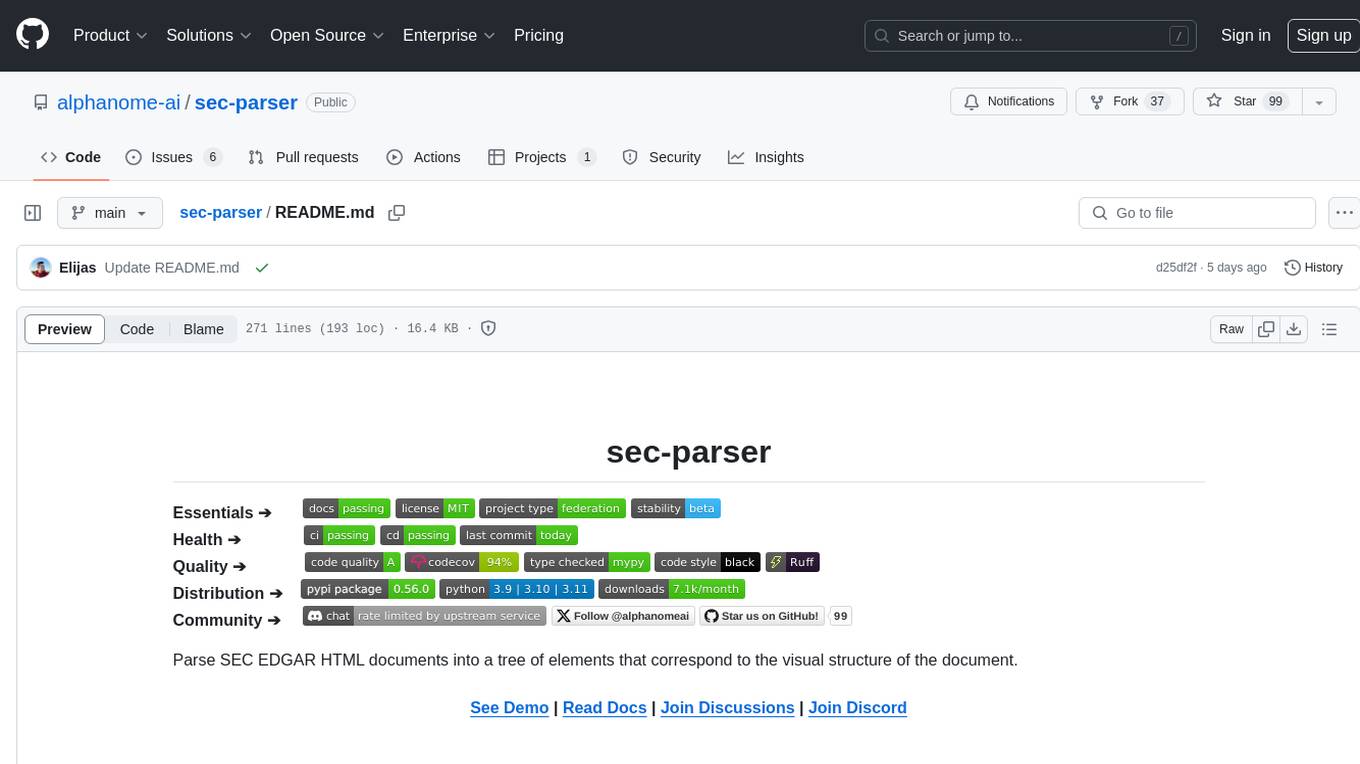
sec-parser
The `sec-parser` project simplifies extracting meaningful information from SEC EDGAR HTML documents by organizing them into semantic elements and a tree structure. It helps in parsing SEC filings for financial and regulatory analysis, analytics and data science, AI and machine learning, causal AI, and large language models. The tool is especially beneficial for AI, ML, and LLM applications by streamlining data pre-processing and feature extraction.
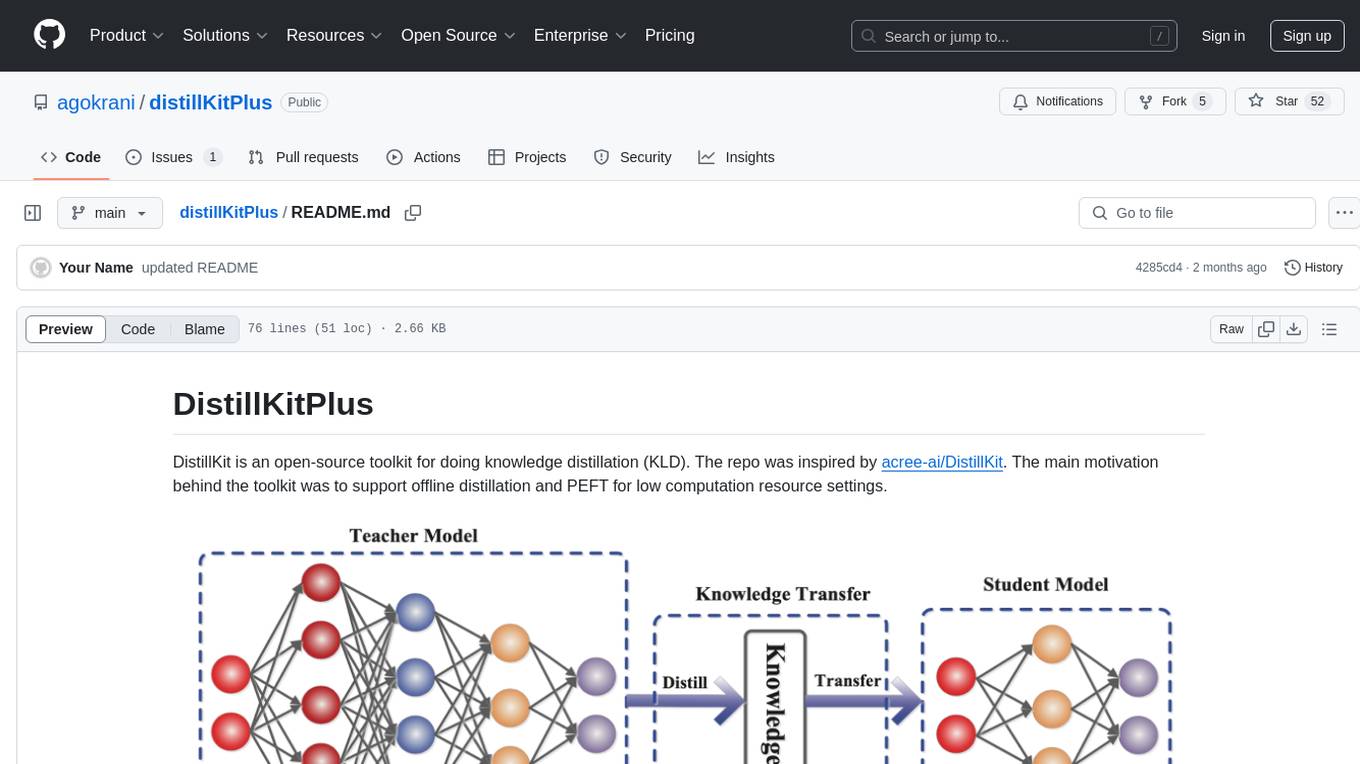
distillKitPlus
DistillKitPlus is an open-source toolkit designed for knowledge distillation (KLD) in low computation resource settings. It supports logit distillation, pre-computed logits for memory-efficient training, LoRA fine-tuning integration, and model quantization for faster inference. The toolkit utilizes a JSON configuration file for project, dataset, model, tokenizer, training, distillation, LoRA, and quantization settings. Users can contribute to the toolkit and contact the developers for technical questions or issues.
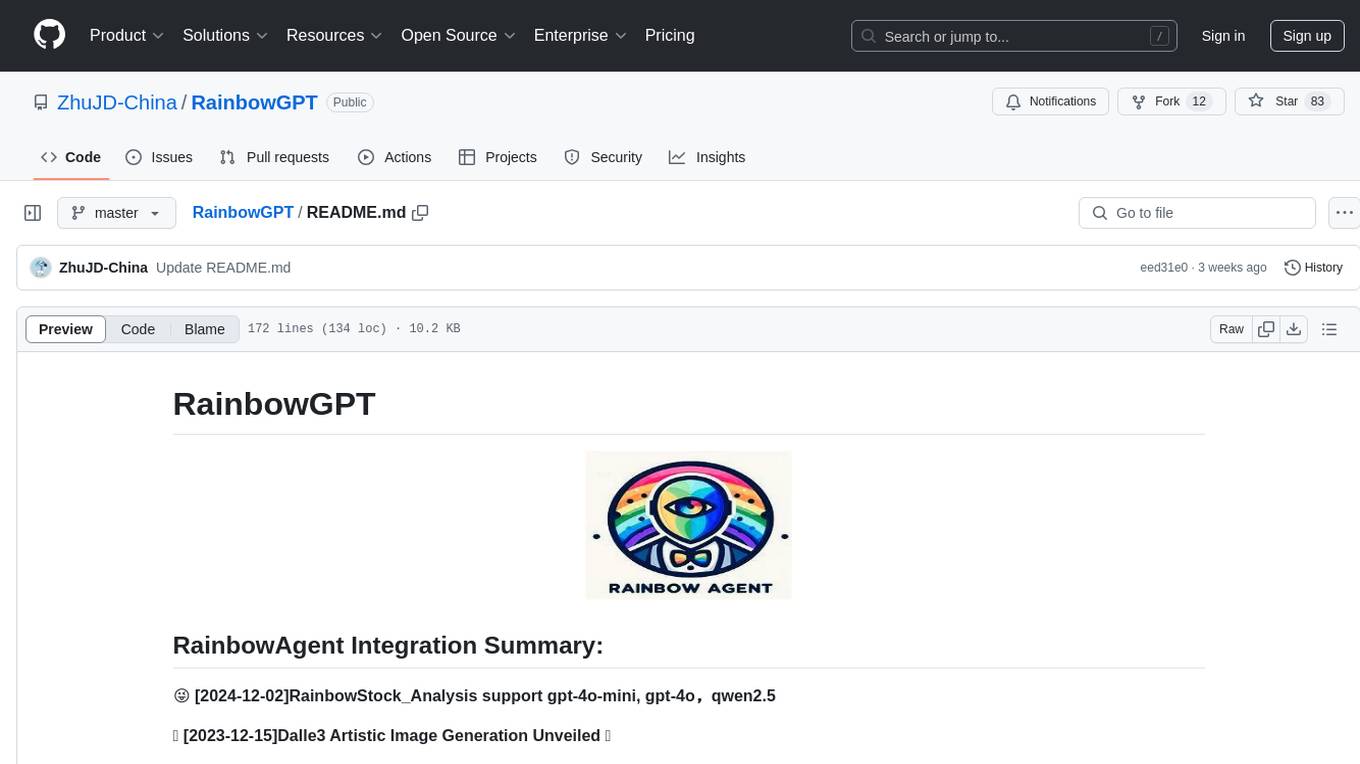
RainbowGPT
RainbowGPT is a versatile tool that offers a range of functionalities, including Stock Analysis for financial decision-making, MySQL Management for database navigation, and integration of AI technologies like GPT-4 and ChatGlm3. It provides a user-friendly interface suitable for all skill levels, ensuring seamless information flow and continuous expansion of emerging technologies. The tool enhances adaptability, creativity, and insight, making it a valuable asset for various projects and tasks.
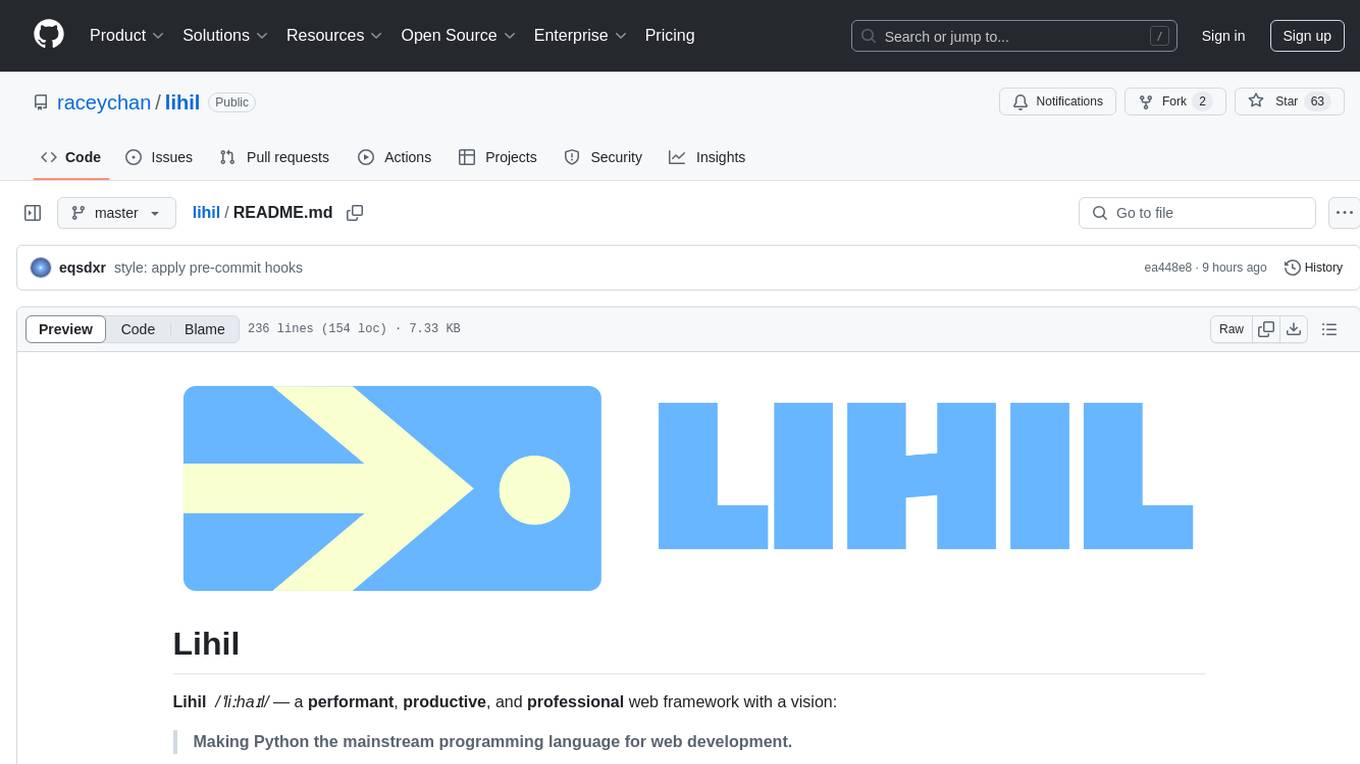
lihil
Lihil is a performant, productive, and professional web framework designed to make Python the mainstream programming language for web development. It is 100% test covered and strictly typed, offering fast performance, ergonomic API, and built-in solutions for common problems. Lihil is suitable for enterprise web development, delivering robust and scalable solutions with best practices in microservice architecture and related patterns. It features dependency injection, OpenAPI docs generation, error response generation, data validation, message system, testability, and strong support for AI features. Lihil is ASGI compatible and uses starlette as its ASGI toolkit, ensuring compatibility with starlette classes and middlewares. The framework follows semantic versioning and has a roadmap for future enhancements and features.
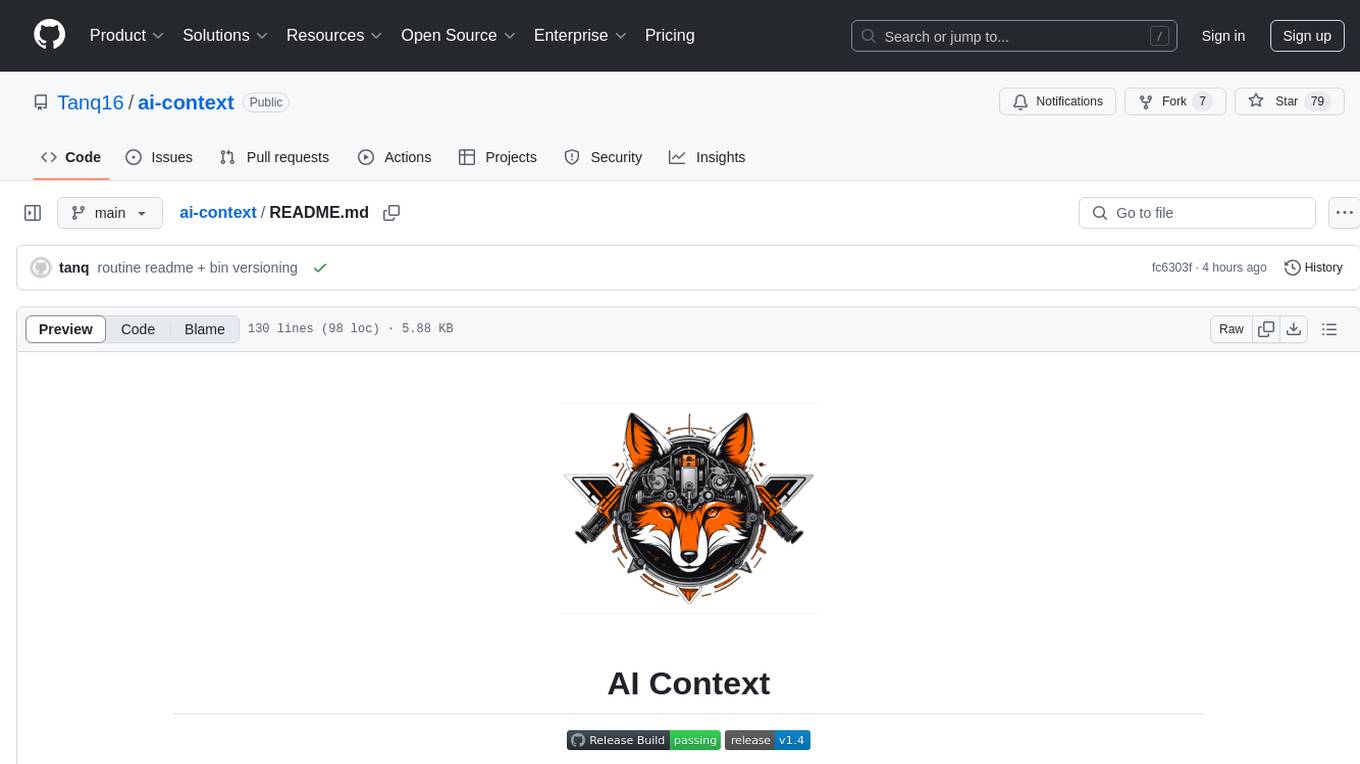
ai-context
AI Context is a CLI tool that generates AI-friendly markdown files from GitHub repos, local code, YouTube videos, or webpages. It supports processing local directories, GitHub repositories, YouTube transcripts, and webpages, converting them to markdown format. The tool simplifies interactions with LLMs like ChatGPT and Claude by providing a text-first context creation approach. It offers features for installation, usage, and acknowledgments, with options to process single paths, URLs, or lists of paths concurrently.
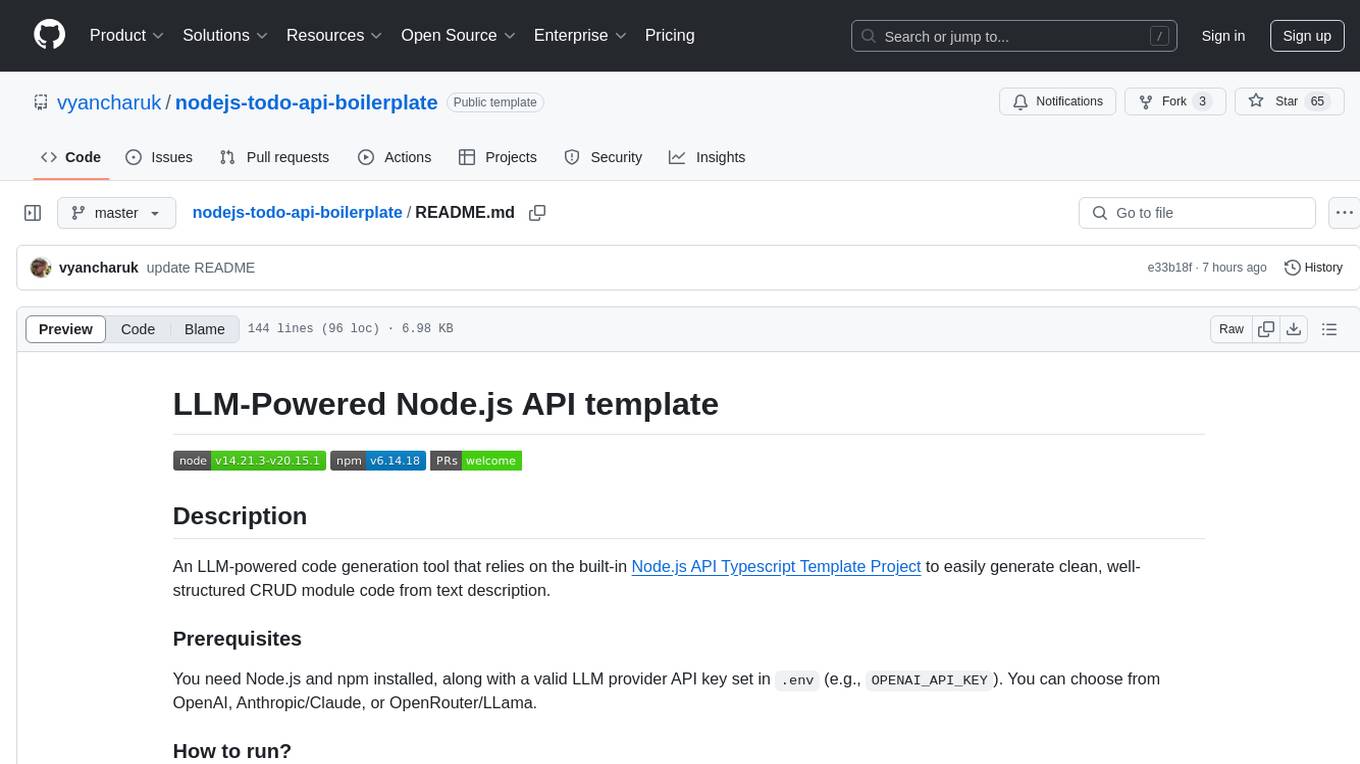
nodejs-todo-api-boilerplate
An LLM-powered code generation tool that relies on the built-in Node.js API Typescript Template Project to easily generate clean, well-structured CRUD module code from text description. It orchestrates 3 LLM micro-agents (`Developer`, `Troubleshooter` and `TestsFixer`) to generate code, fix compilation errors, and ensure passing E2E tests. The process includes module code generation, DB migration creation, seeding data, and running tests to validate output. By cycling through these steps, it guarantees consistent and production-ready CRUD code aligned with vertical slicing architecture.
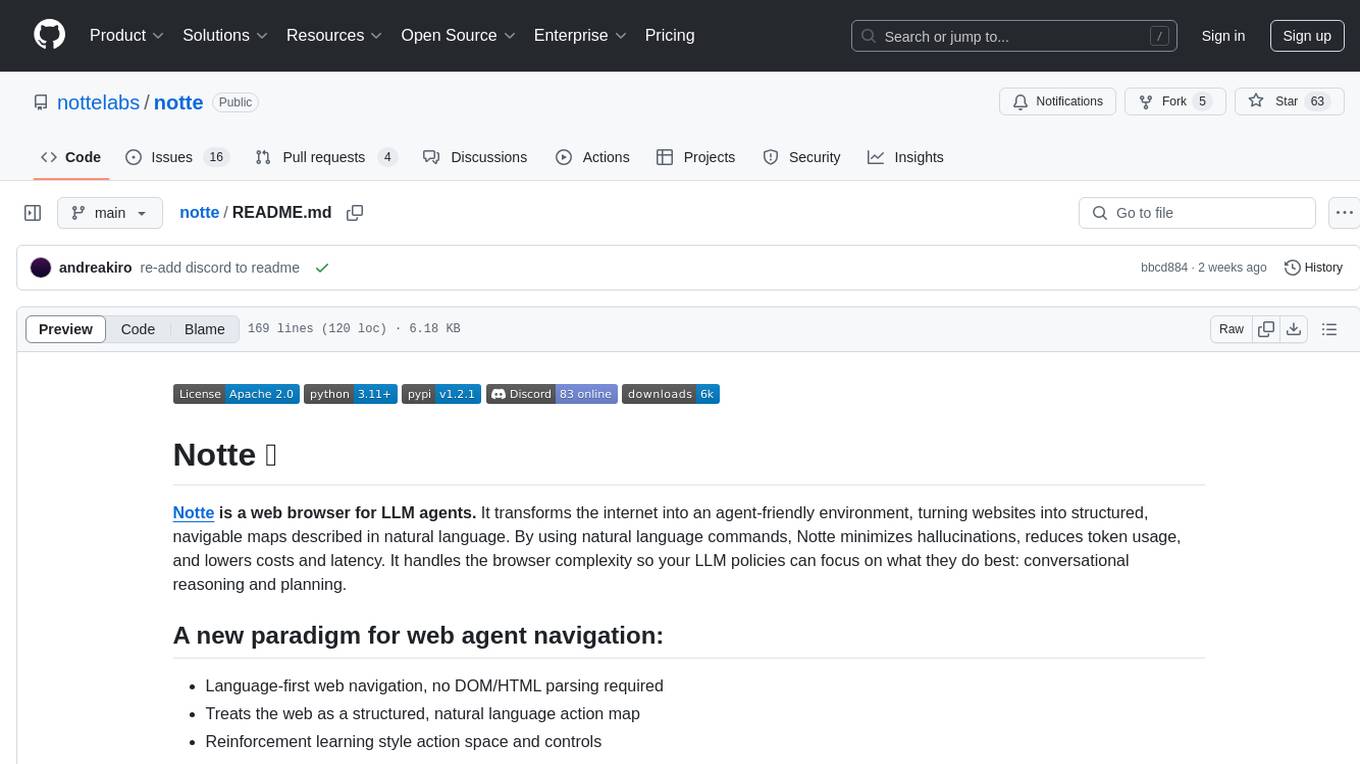
notte
Notte is a web browser designed specifically for LLM agents, providing a language-first web navigation experience without the need for DOM/HTML parsing. It transforms websites into structured, navigable maps described in natural language, enabling users to interact with the web using natural language commands. By simplifying browser complexity, Notte allows LLM policies to focus on conversational reasoning and planning, reducing token usage, costs, and latency. The tool supports various language model providers and offers a reinforcement learning style action space and controls for full navigation control.
For similar tasks
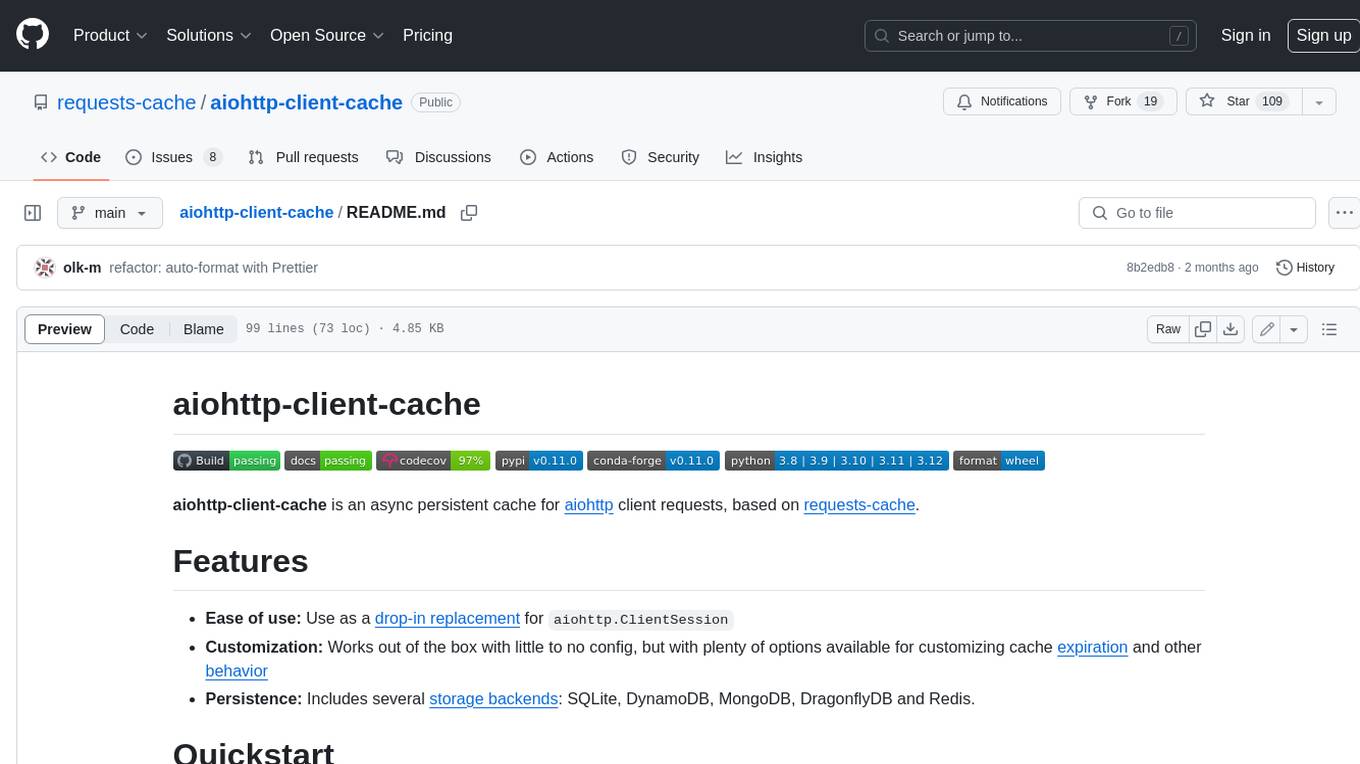
aiohttp-client-cache
aiohttp-client-cache is an asynchronous persistent cache for aiohttp client requests, based on requests-cache. It is easy to use, customizable, and persistent, with several storage backends available, including SQLite, DynamoDB, MongoDB, DragonflyDB, and Redis.
For similar jobs
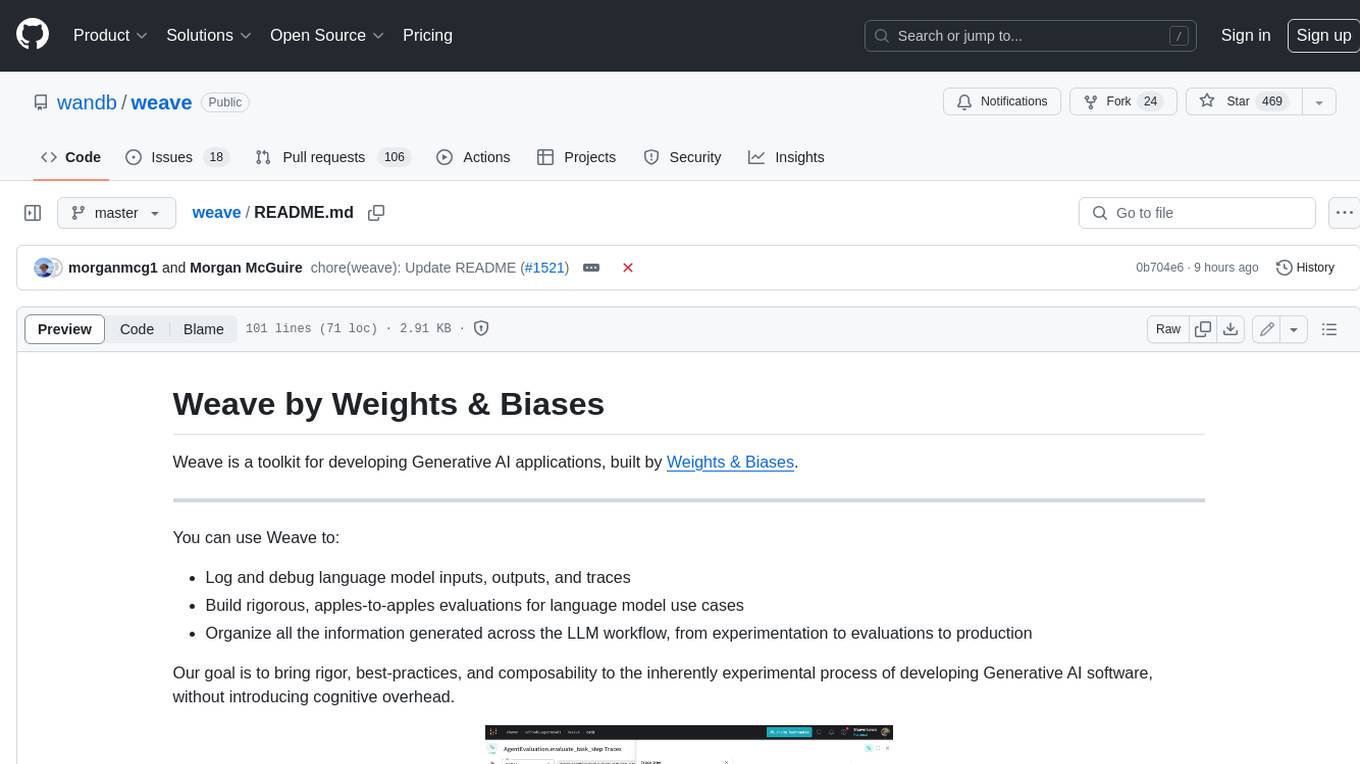
weave
Weave is a toolkit for developing Generative AI applications, built by Weights & Biases. With Weave, you can log and debug language model inputs, outputs, and traces; build rigorous, apples-to-apples evaluations for language model use cases; and organize all the information generated across the LLM workflow, from experimentation to evaluations to production. Weave aims to bring rigor, best-practices, and composability to the inherently experimental process of developing Generative AI software, without introducing cognitive overhead.
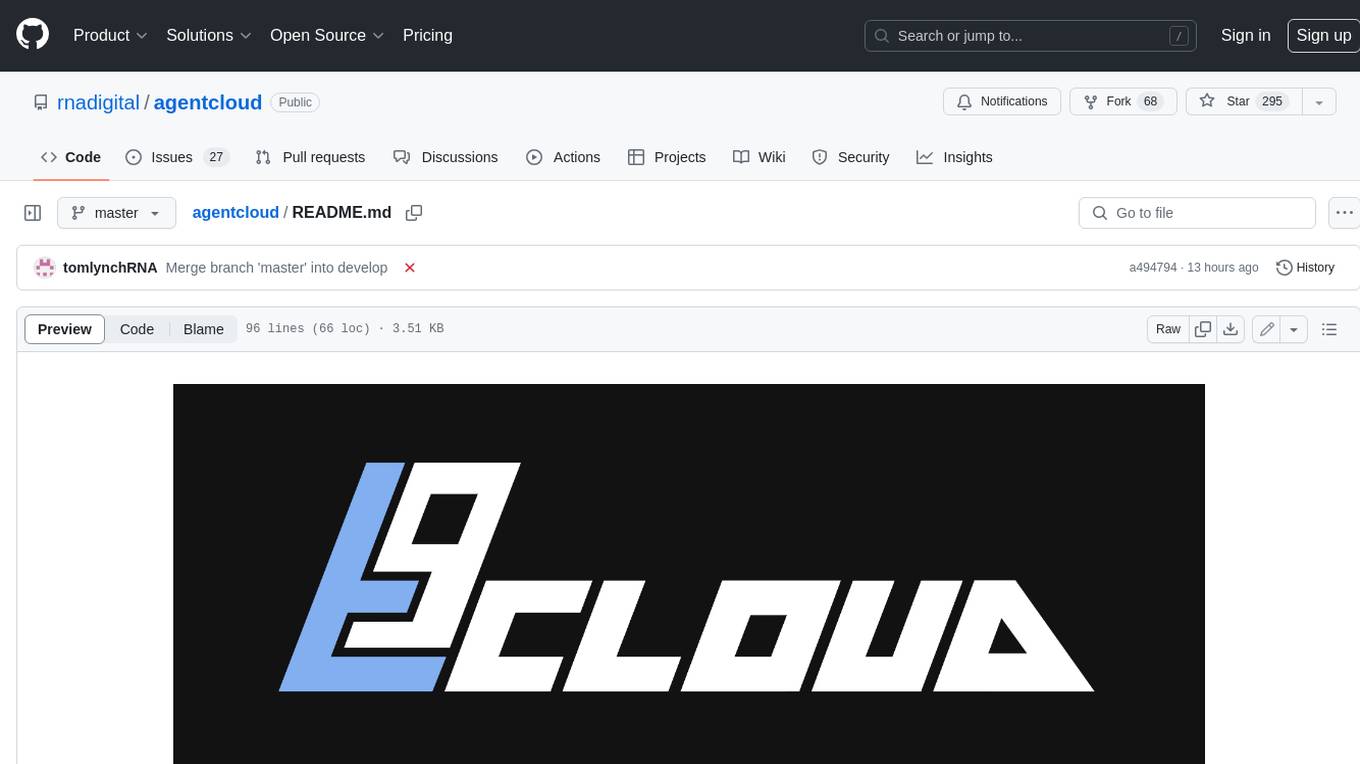
agentcloud
AgentCloud is an open-source platform that enables companies to build and deploy private LLM chat apps, empowering teams to securely interact with their data. It comprises three main components: Agent Backend, Webapp, and Vector Proxy. To run this project locally, clone the repository, install Docker, and start the services. The project is licensed under the GNU Affero General Public License, version 3 only. Contributions and feedback are welcome from the community.
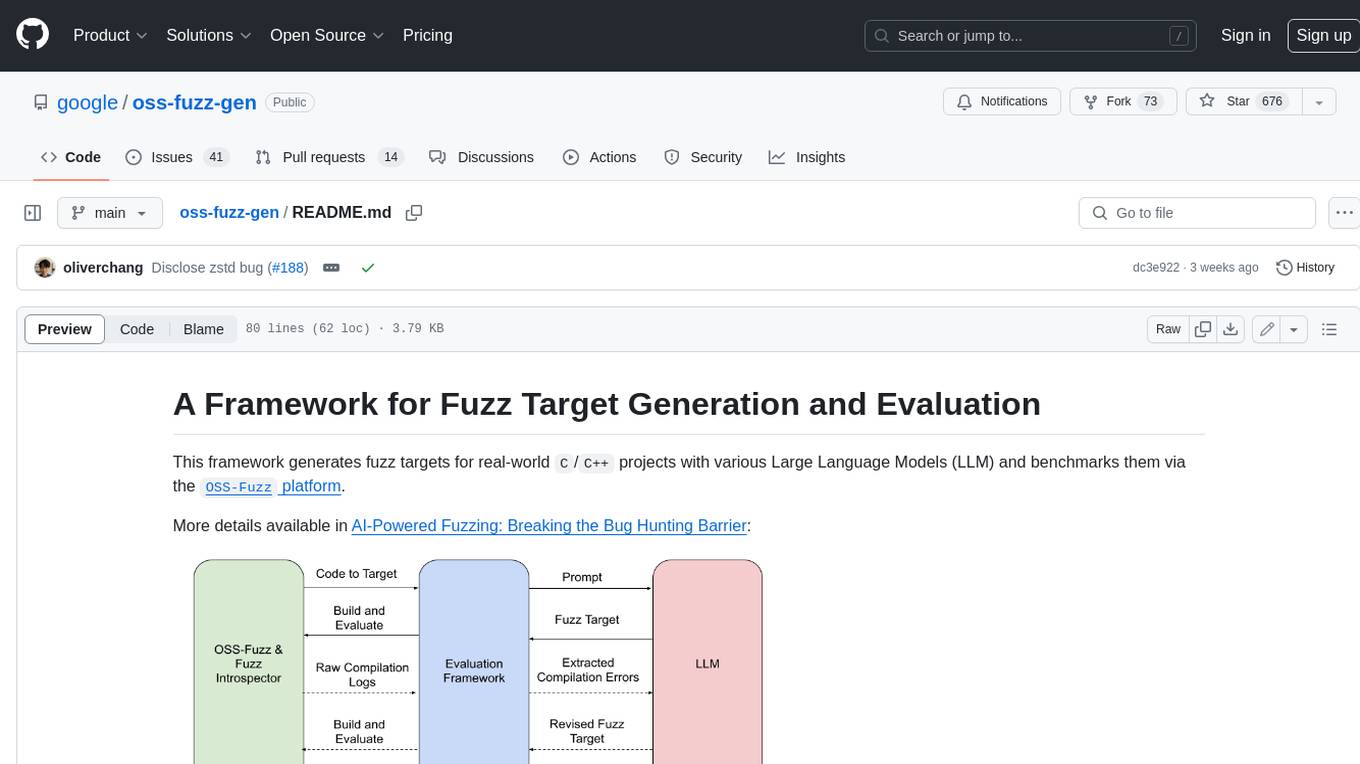
oss-fuzz-gen
This framework generates fuzz targets for real-world `C`/`C++` projects with various Large Language Models (LLM) and benchmarks them via the `OSS-Fuzz` platform. It manages to successfully leverage LLMs to generate valid fuzz targets (which generate non-zero coverage increase) for 160 C/C++ projects. The maximum line coverage increase is 29% from the existing human-written targets.
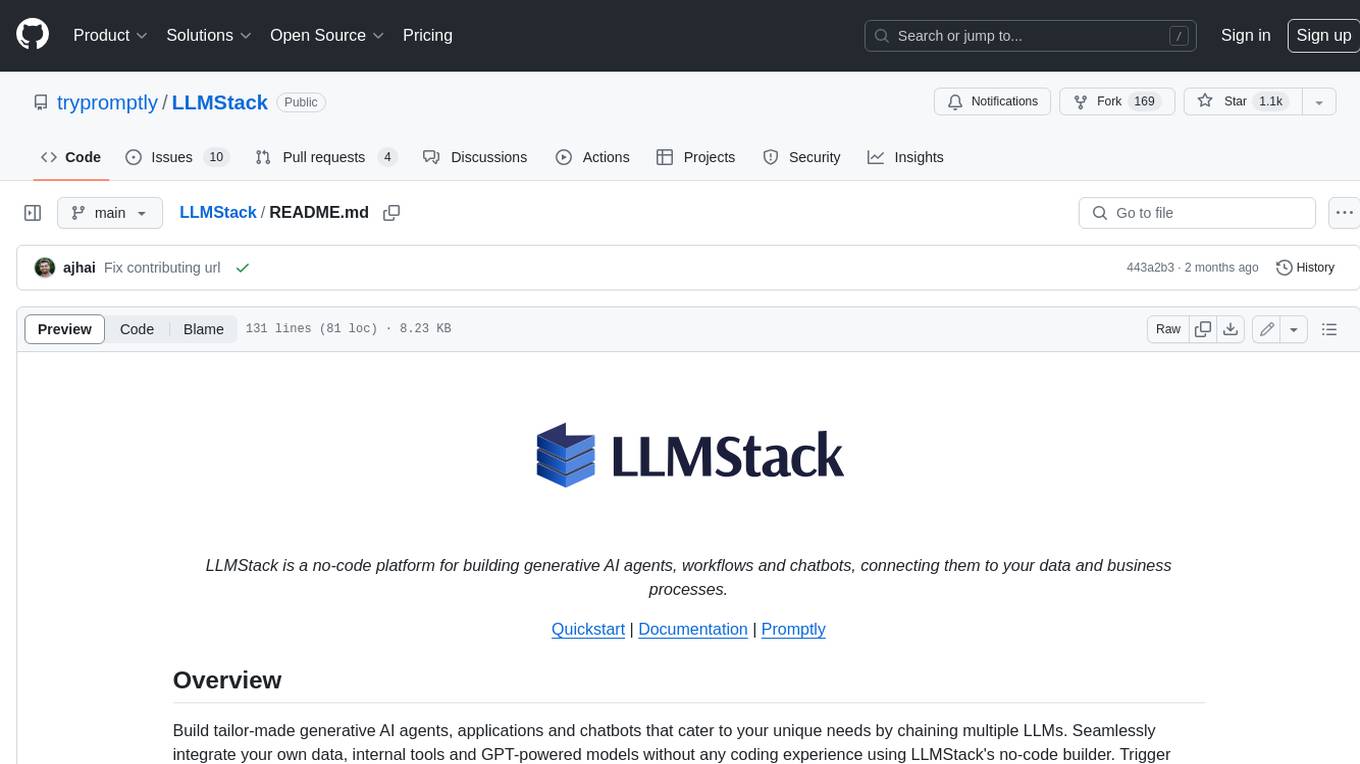
LLMStack
LLMStack is a no-code platform for building generative AI agents, workflows, and chatbots. It allows users to connect their own data, internal tools, and GPT-powered models without any coding experience. LLMStack can be deployed to the cloud or on-premise and can be accessed via HTTP API or triggered from Slack or Discord.
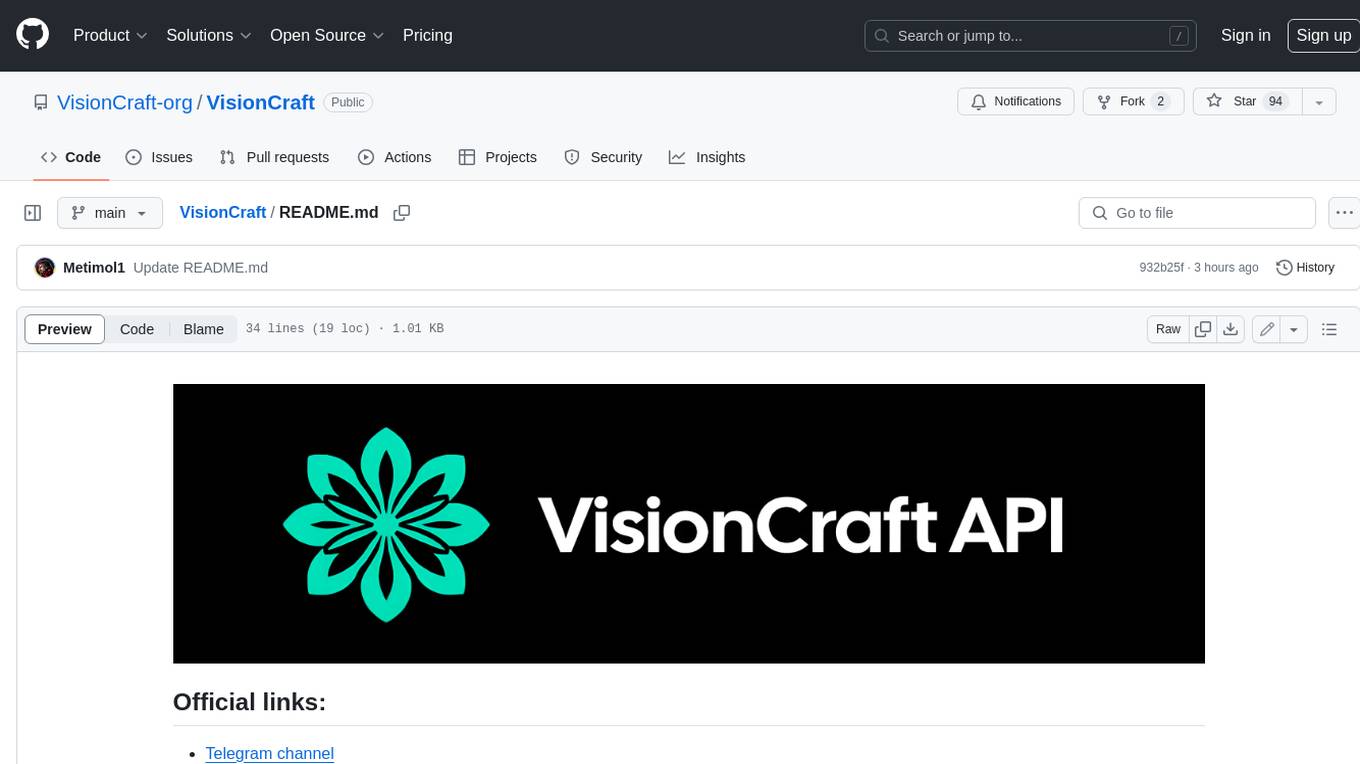
VisionCraft
The VisionCraft API is a free API for using over 100 different AI models. From images to sound.
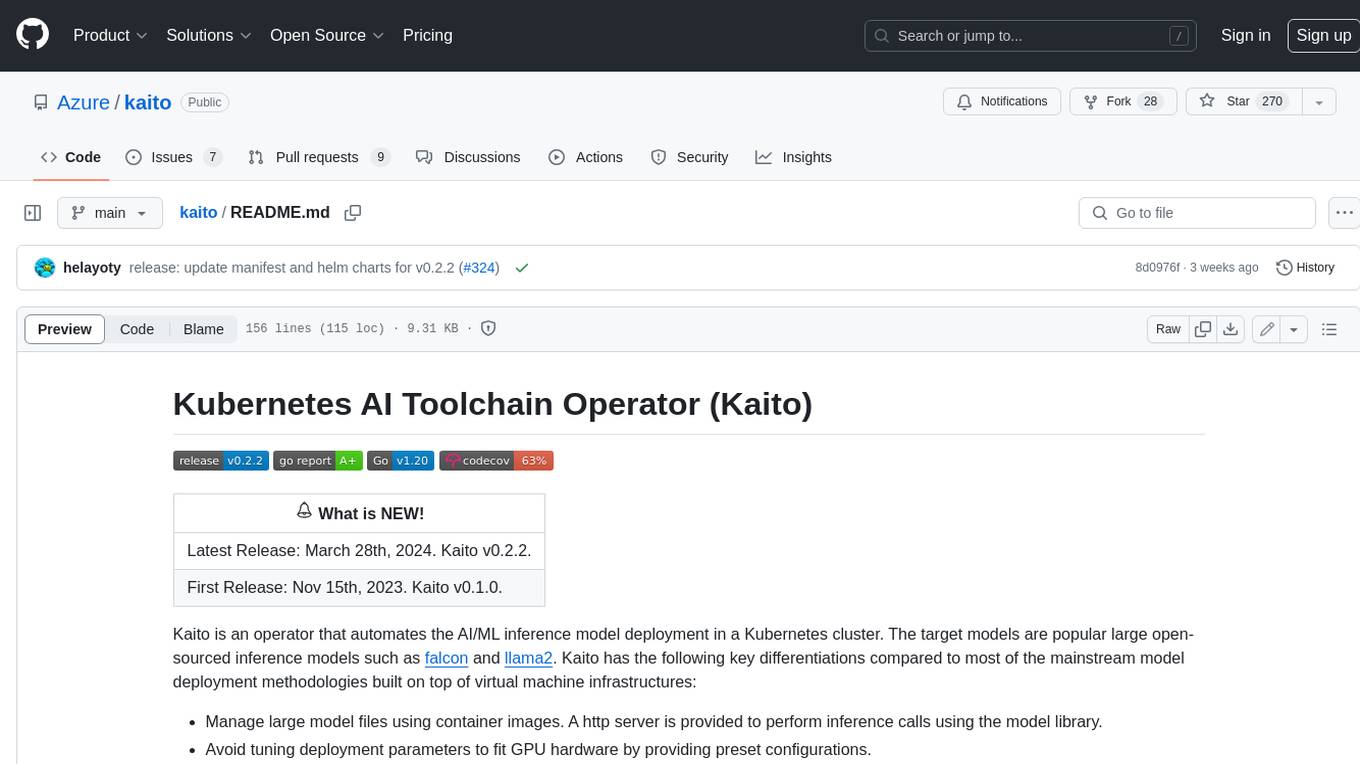
kaito
Kaito is an operator that automates the AI/ML inference model deployment in a Kubernetes cluster. It manages large model files using container images, avoids tuning deployment parameters to fit GPU hardware by providing preset configurations, auto-provisions GPU nodes based on model requirements, and hosts large model images in the public Microsoft Container Registry (MCR) if the license allows. Using Kaito, the workflow of onboarding large AI inference models in Kubernetes is largely simplified.
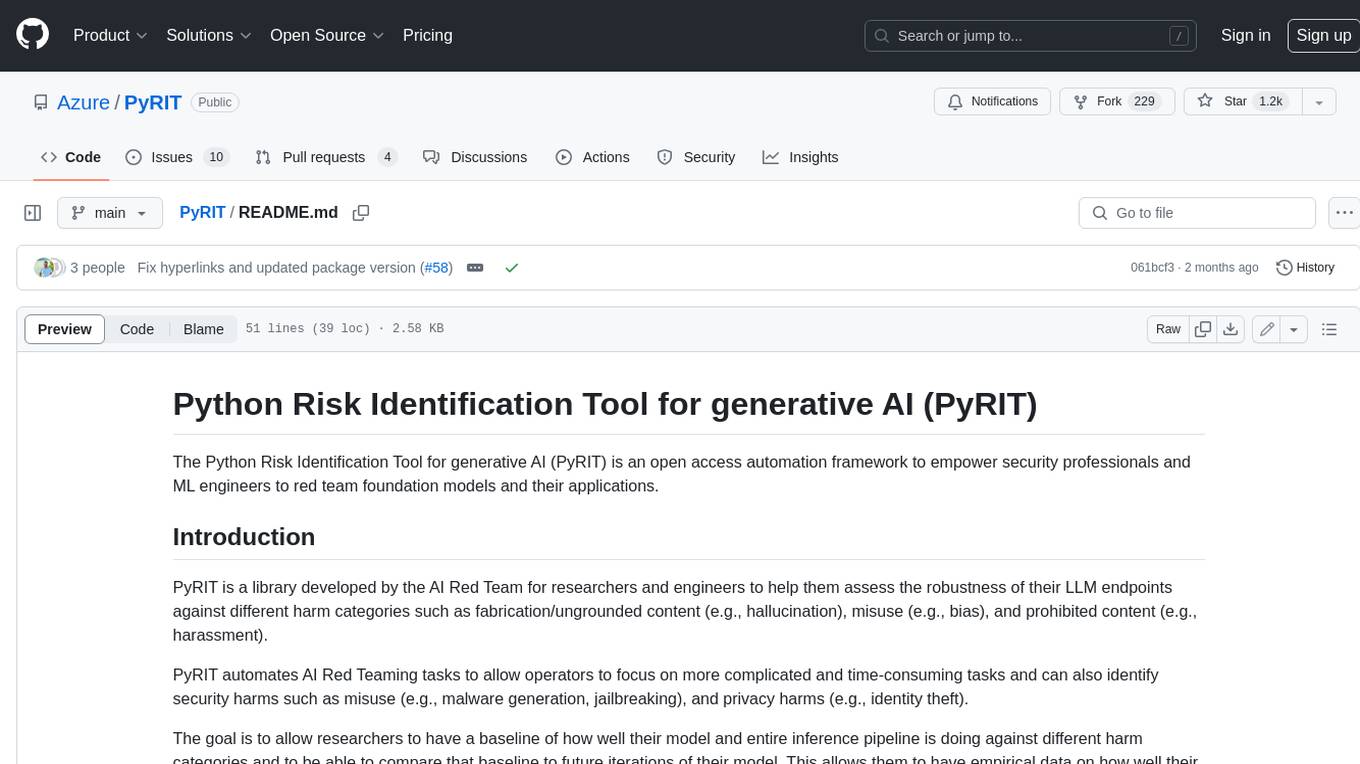
PyRIT
PyRIT is an open access automation framework designed to empower security professionals and ML engineers to red team foundation models and their applications. It automates AI Red Teaming tasks to allow operators to focus on more complicated and time-consuming tasks and can also identify security harms such as misuse (e.g., malware generation, jailbreaking), and privacy harms (e.g., identity theft). The goal is to allow researchers to have a baseline of how well their model and entire inference pipeline is doing against different harm categories and to be able to compare that baseline to future iterations of their model. This allows them to have empirical data on how well their model is doing today, and detect any degradation of performance based on future improvements.
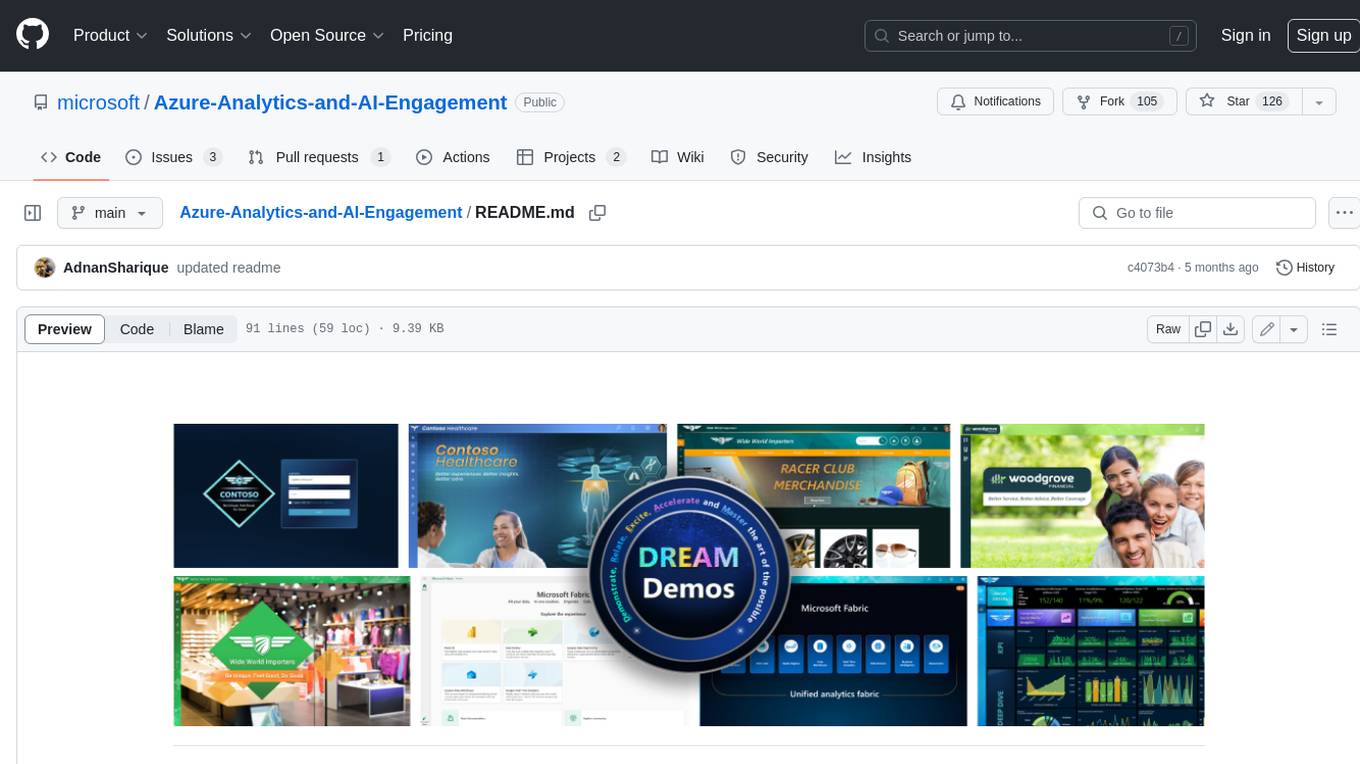
Azure-Analytics-and-AI-Engagement
The Azure-Analytics-and-AI-Engagement repository provides packaged Industry Scenario DREAM Demos with ARM templates (Containing a demo web application, Power BI reports, Synapse resources, AML Notebooks etc.) that can be deployed in a customer’s subscription using the CAPE tool within a matter of few hours. Partners can also deploy DREAM Demos in their own subscriptions using DPoC.