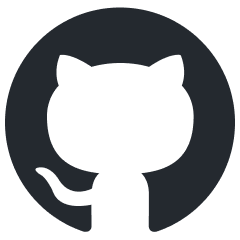
banks
LLM prompt language based on Jinja. Banks provides tools and functions to build prompts text and chat messages from generic blueprints. It allows attaching metadata to prompts to ease their management, and versioning is first-class citizen. Banks provides ways to store prompts on disk along with their metadata.
Stars: 72
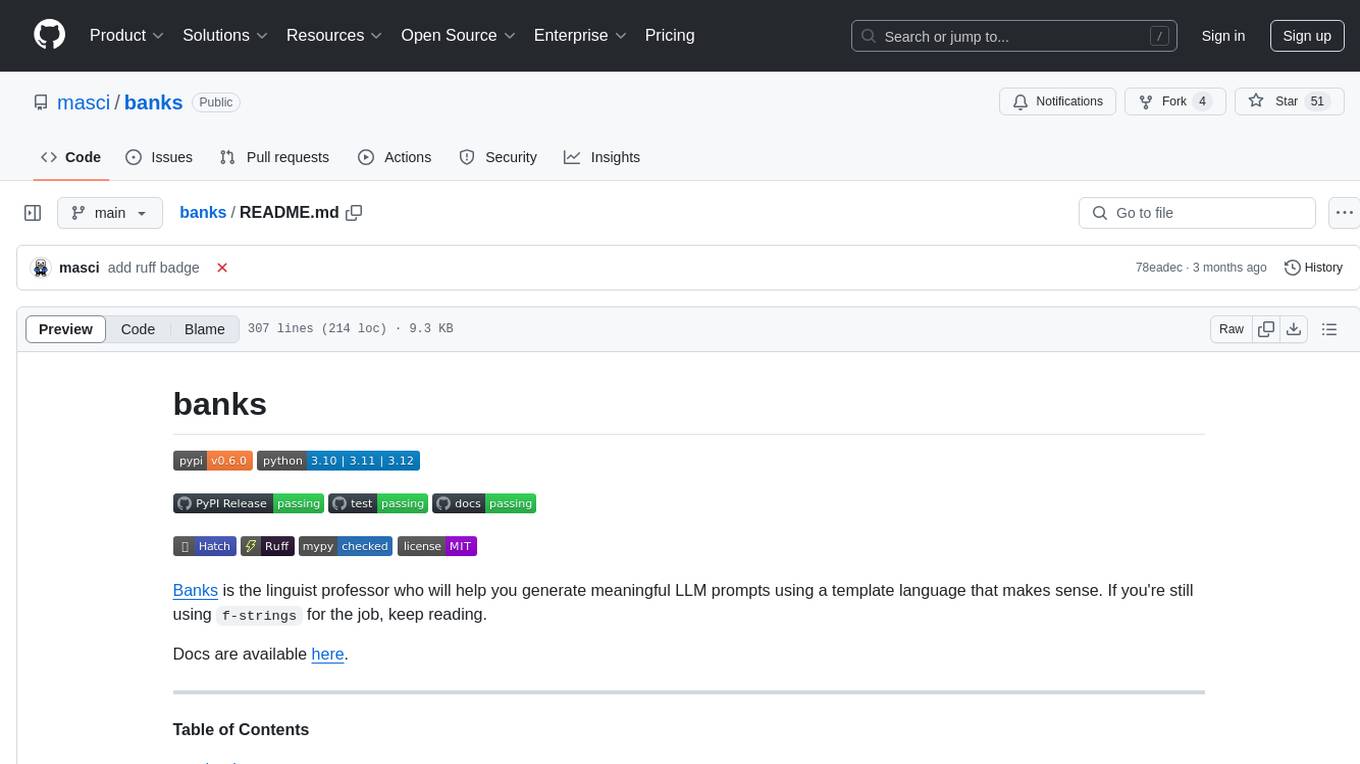
Banks is a linguist professor tool that helps generate meaningful LLM prompts using a template language. It provides a user-friendly way to create prompts for various tasks such as blog writing, summarizing documents, lemmatizing text, and generating text using a LLM. The tool supports async operations and comes with predefined filters for data processing. Banks leverages Jinja's macro system to create prompts and interact with OpenAI API for text generation. It also offers a cache mechanism to avoid regenerating text for the same template and context.
README:
Banks is the linguist professor who will help you generate meaningful
LLM prompts using a template language that makes sense. If you're still using f-strings
for the job, keep reading.
Docs are available here.
Table of Contents
- banks
pip install banks
Prompts are instrumental for the success of any LLM application, and Banks focuses around specific areas of their lifecycle:
- 📙 Templating: Banks provides tools and functions to build prompts text and chat messages from generic blueprints.
- 🎟️ Versioning and metadata: Banks supports attaching metadata to prompts to ease their management, and versioning is first-class citizen.
- 🗄️ Management: Banks provides ways to store prompts on disk along with their metadata.
For a more extensive set of code examples, see the documentation page.
You'll find yourself feeding an LLM a list of chat messages instead of plain text more often than not. Banks will help you remove the boilerplate by defining the messages already at the prompt level.
from banks import Prompt
prompt_template = """
{% chat role="system" %}
You are a {{ persona }}.
{% endchat %}
{% chat role="user" %}
Hello, how are you?
{% endchat %}
"""
p = Prompt(prompt_template)
print(p.chat_messages({"persona": "helpful assistant"}))
# Output:
# [
# ChatMessage(role='system', content='You are a helpful assistant.\n'),
# ChatMessage(role='user', content='Hello, how are you?\n')
# ]
If you're working with a multimodal model, you can include images directly in the prompt, and Banks will do the job needed to upload them when rendering the chat messages:
import litellm
from banks import Prompt
prompt_template = """
{% chat role="user" %}
Guess where is this place.
{{ picture | image }}
{%- endchat %}
"""
pic_url = (
"https://upload.wikimedia.org/wikipedia/commons/thumb/4/4d/CorcianoMar302024_01.jpg/1079px-CorcianoMar302024_01.jpg"
)
# Alternatively, load the image from disk
# pic_url = "/Users/massi/Downloads/CorcianoMar302024_01.jpg"
p = Prompt(prompt_template)
as_dict = [msg.model_dump(exclude_none=True) for msg in p.chat_messages({"picture": pic_url})]
r = litellm.completion(model="gpt-4-vision-preview", messages=as_dict)
print(r.choices[0].message.content)
Sometimes it might be useful to ask another LLM to generate examples for you in a
few-shots prompt. Provided you have a valid OpenAI API key stored in an env var
called OPENAI_API_KEY
you can ask Banks to do something like this (note we can
annotate the prompt using comments - anything within {# ... #}
will be removed
from the final prompt):
from banks import Prompt
prompt_template = """
{% set examples %}
{% completion model="gpt-3.5-turbo-0125" %}
{% chat role="system" %}You are a helpful assistant{% endchat %}
{% chat role="user" %}Generate a bullet list of 3 tweets with a positive sentiment.{% endchat %}
{% endcompletion %}
{% endset %}
{# output the response content #}
Generate a tweet about the topic {{ topic }} with a positive sentiment.
Examples:
{{ examples }}
"""
p = Prompt(prompt_template)
print(p.text({"topic": "climate change"}))
The output would be something similar to the following:
Generate a tweet about the topic climate change with a positive sentiment.
Examples:
- "Feeling grateful for the sunshine today! 🌞 #thankful #blessed"
- "Just had a great workout and feeling so energized! 💪 #fitness #healthyliving"
- "Spent the day with loved ones and my heart is so full. 💕 #familytime #grateful"
[!IMPORTANT] The
completion
extension uses LiteLLM under the hood, and provided you have the proper environment variables set, you can use any model from the supported model providers.
[!NOTE] Banks uses a cache to avoid generating text again for the same template with the same context. By default the cache is in-memory but it can be customized.
Banks provides a filter tool
that can be used to convert a callable passed to a prompt into an LLM function call.
Docstrings are used to describe the tool and its arguments, and during prompt rendering Banks will perform all the LLM
roundtrips needed in case the model wants to use a tool within a {% completion %}
block. For example:
import platform
from banks import Prompt
def get_laptop_info():
"""Get information about the user laptop.
For example, it returns the operating system and version, along with hardware and network specs."""
return str(platform.uname())
p = Prompt("""
{% set response %}
{% completion model="gpt-3.5-turbo-0125" %}
{% chat role="user" %}{{ query }}{% endchat %}
{{ get_laptop_info | tool }}
{% endcompletion %}
{% endset %}
{# the variable 'response' contains the result #}
{{ response }}
""")
print(p.text({"query": "Can you guess the name of my laptop?", "get_laptop_info": get_laptop_info}))
# Output:
# Based on the information provided, the name of your laptop is likely "MacGiver."
Several inference providers support prompt caching to save time and costs, and Anthropic in particular offers fine-grained control over the parts of the prompt that we want to cache. With Banks this is as simple as using a template filter:
prompt_template = """
{% chat role="user" %}
Analyze this book:
{# Only this part of the chat message (the book content) will be cached #}
{{ book | cache_control("ephemeral") }}
What is the title of this book? Only output the title.
{% endchat %}
"""
p = Prompt(prompt_template)
print(p.chat_messages({"book":"This is a short book!"}))
# Output:
# [
# ChatMessage(role='user', content=[
# ContentBlock(type='text', text='Analyze this book:\n\n'),
# ContentBlock(type='text', cache_control=CacheControl(type='ephemeral'), text='This is a short book!'),
# ContentBlock(type='text', text='\n\nWhat is the title of this book? Only output the title.\n')
# ])
# ]
The output of p.chat_messages()
can be fed to the Anthropic client directly.
We can get the same result as the previous example loading the prompt template from a registry
instead of hardcoding it into the Python code. For convenience, Banks comes with a few registry types
you can use to store your templates. For example, the DirectoryTemplateRegistry
can load templates
from a directory in the file system. Suppose you have a folder called templates
in the current path,
and the folder contains a file called blog.jinja
. You can load the prompt template like this:
from banks import Prompt
from banks.registries import DirectoryTemplateRegistry
registry = DirectoryTemplateRegistry(populated_dir)
prompt = registry.get(name="blog")
print(prompt.text({"topic": "retrogame computing"}))
To run banks within an asyncio
loop you have to do two things:
- set the environment variable
BANKS_ASYNC_ENABLED=true
. - use the
AsyncPrompt
class that has an awaitablerun
method.
Example:
from banks import AsyncPrompt
async def main():
p = AsyncPrompt("Write a blog article about the topic {{ topic }}")
result = await p.text({"topic": "AI frameworks"})
print(result)
asyncio.run(main())
Contributions are very welcome, the CONTRIBUTING.md file contains all the details about how to do it.
banks
is distributed under the terms of the MIT license.
For Tasks:
Click tags to check more tools for each tasksFor Jobs:
Alternative AI tools for banks
Similar Open Source Tools
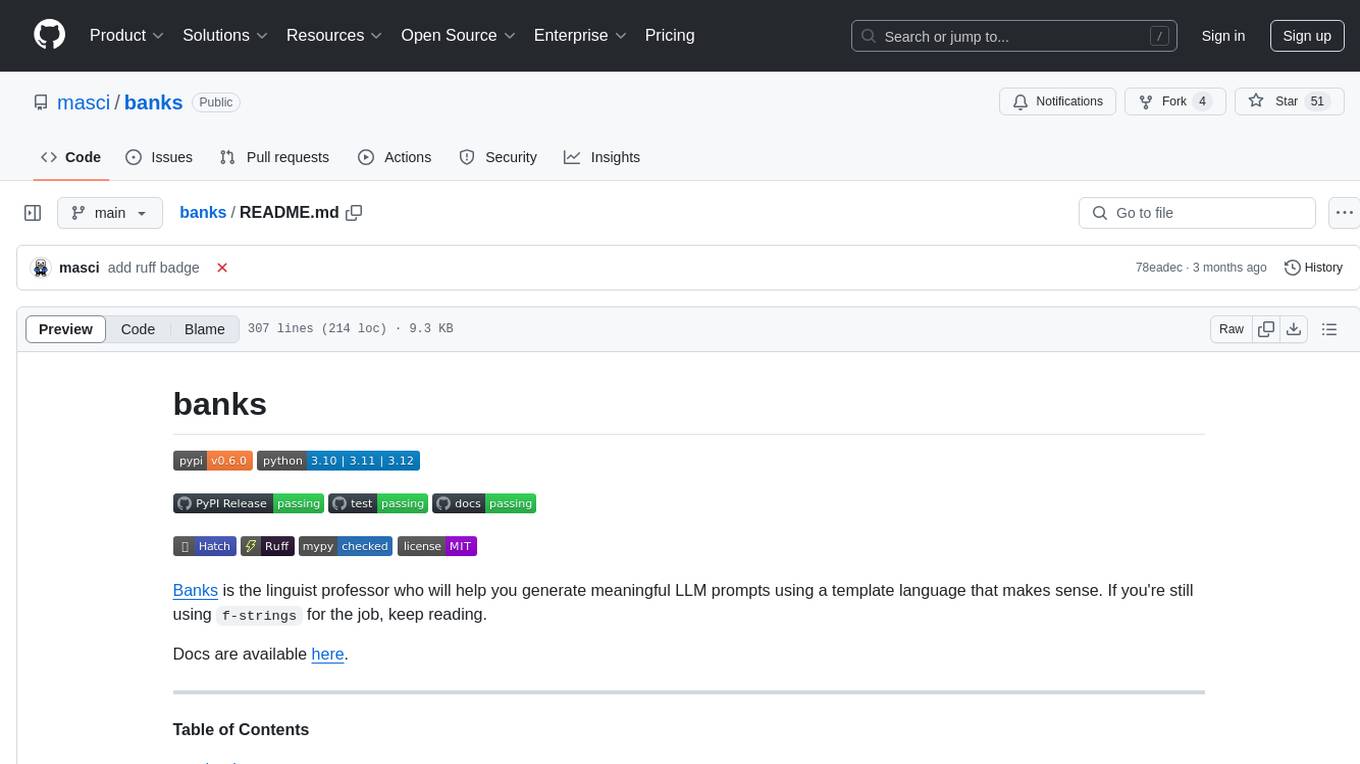
banks
Banks is a linguist professor tool that helps generate meaningful LLM prompts using a template language. It provides a user-friendly way to create prompts for various tasks such as blog writing, summarizing documents, lemmatizing text, and generating text using a LLM. The tool supports async operations and comes with predefined filters for data processing. Banks leverages Jinja's macro system to create prompts and interact with OpenAI API for text generation. It also offers a cache mechanism to avoid regenerating text for the same template and context.
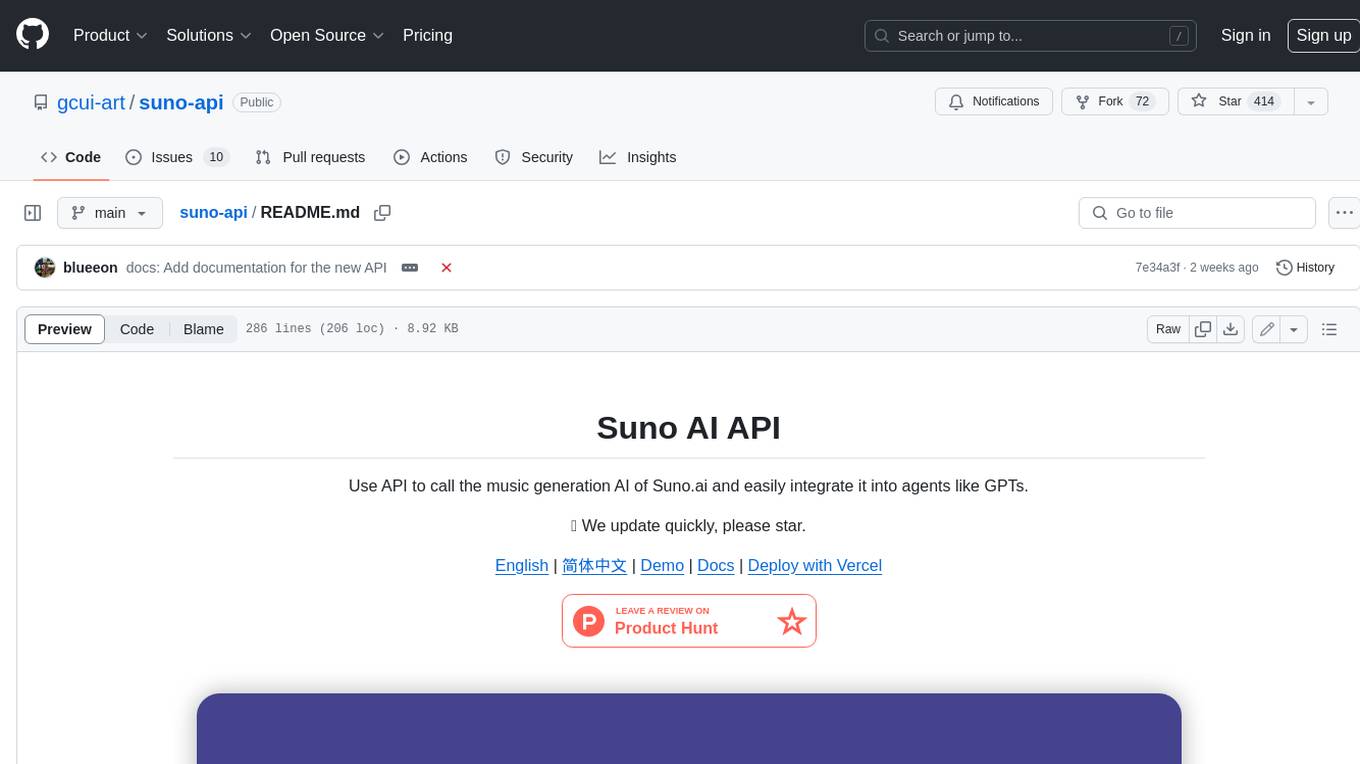
suno-api
Suno AI API is an open-source project that allows developers to integrate the music generation capabilities of Suno.ai into their own applications. The API provides a simple and convenient way to generate music, lyrics, and other audio content using Suno.ai's powerful AI models. With Suno AI API, developers can easily add music generation functionality to their apps, websites, and other projects.
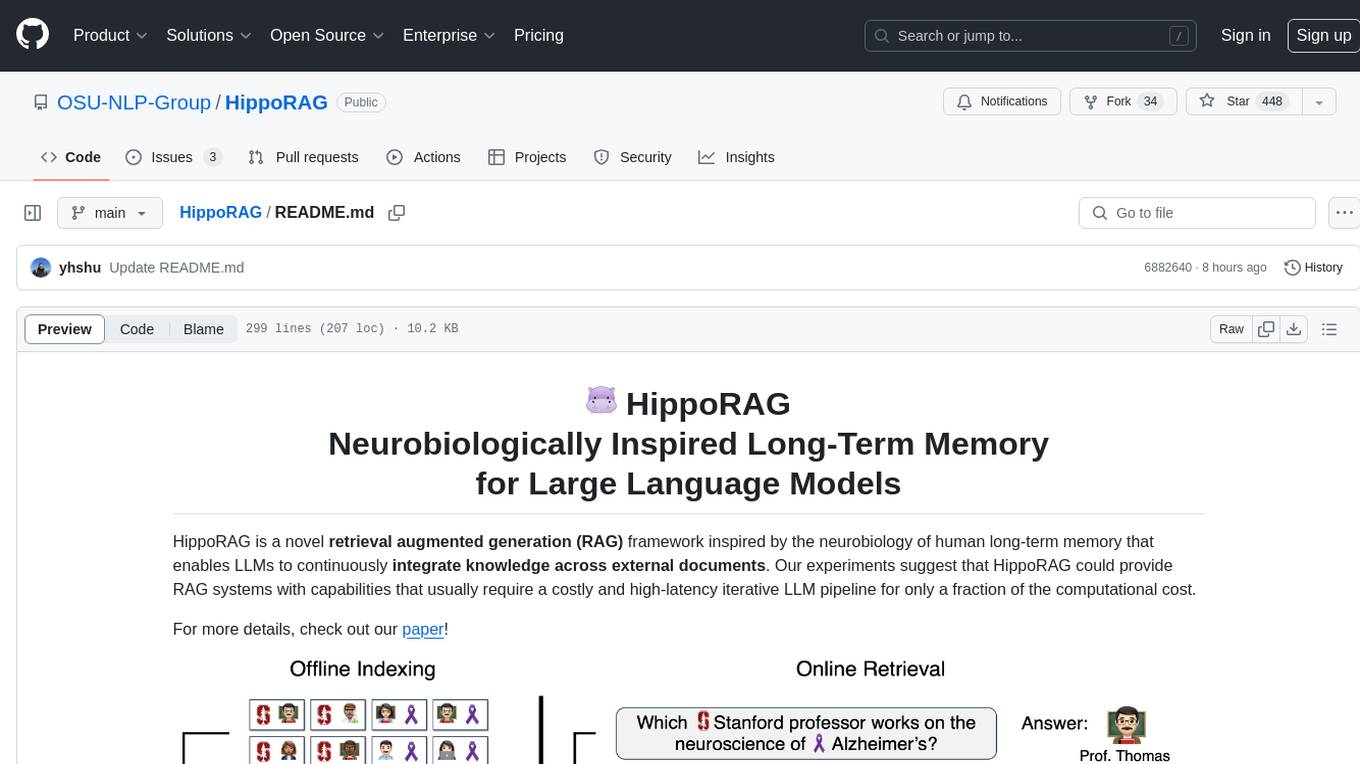
HippoRAG
HippoRAG is a novel retrieval augmented generation (RAG) framework inspired by the neurobiology of human long-term memory that enables Large Language Models (LLMs) to continuously integrate knowledge across external documents. It provides RAG systems with capabilities that usually require a costly and high-latency iterative LLM pipeline for only a fraction of the computational cost. The tool facilitates setting up retrieval corpus, indexing, and retrieval processes for LLMs, offering flexibility in choosing different online LLM APIs or offline LLM deployments through LangChain integration. Users can run retrieval on pre-defined queries or integrate directly with the HippoRAG API. The tool also supports reproducibility of experiments and provides data, baselines, and hyperparameter tuning scripts for research purposes.
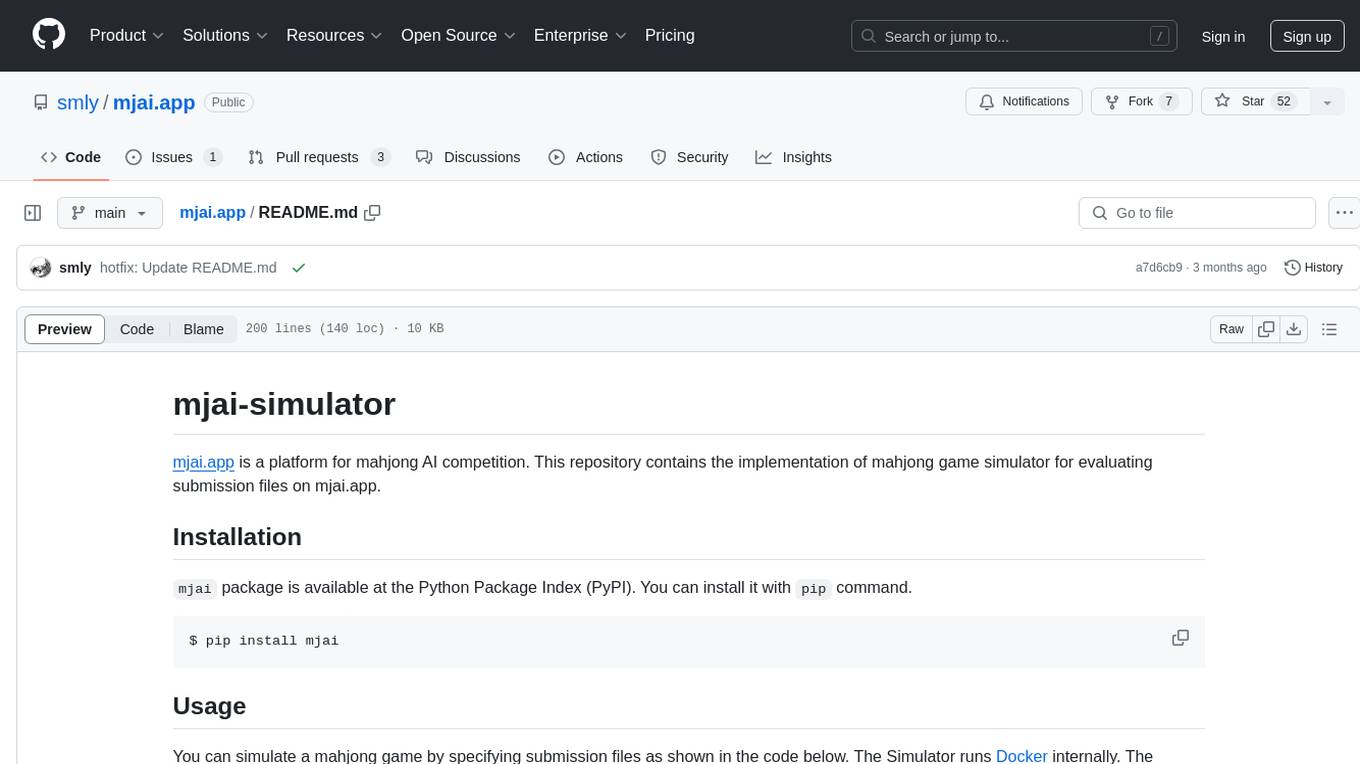
mjai.app
mjai.app is a platform for mahjong AI competition. It contains an implementation of a mahjong game simulator for evaluating submission files. The simulator runs Docker internally, and there is a base class for developing bots that communicate via the mjai protocol. Submission files are deployed in a Docker container, and the Docker image is pushed to Docker Hub. The Mjai protocol used is customized based on Mortal's Mjai Engine implementation.
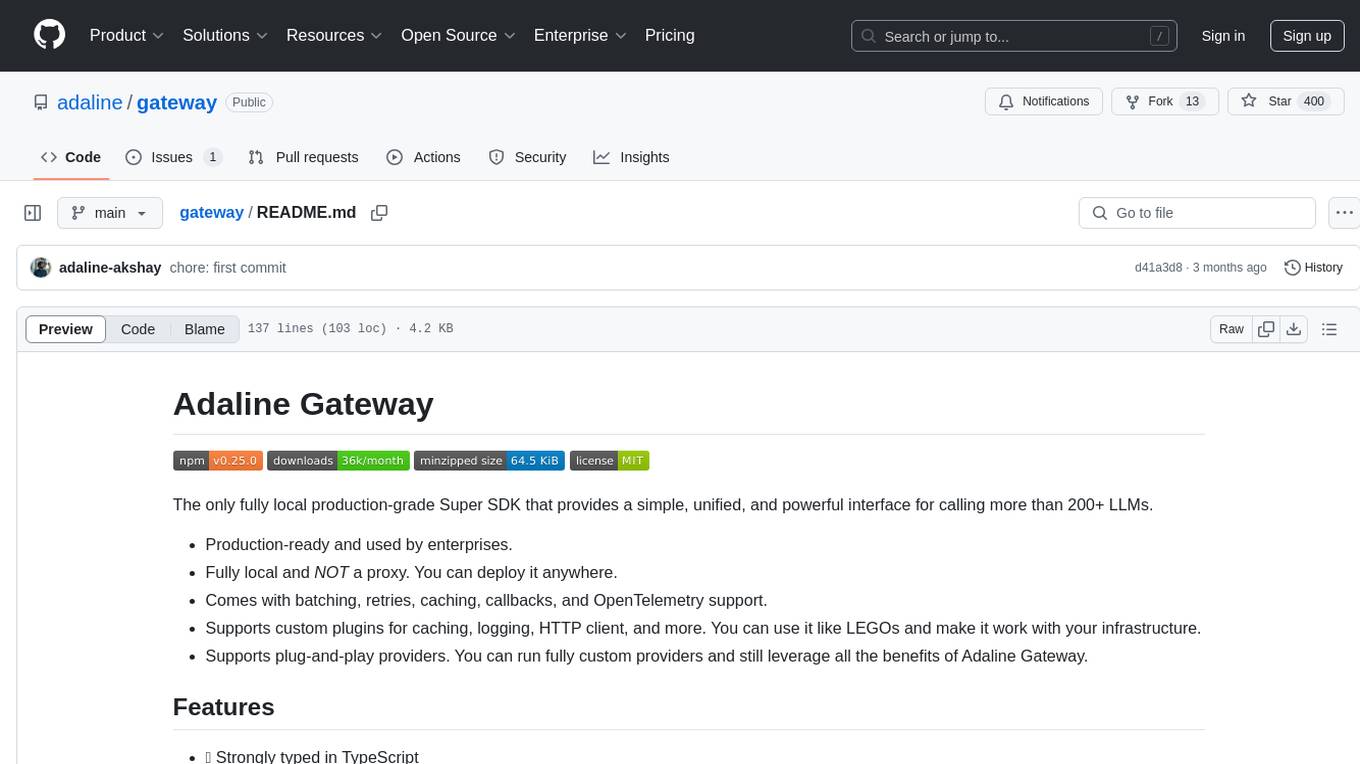
gateway
Adaline Gateway is a fully local production-grade Super SDK that offers a unified interface for calling over 200+ LLMs. It is production-ready, supports batching, retries, caching, callbacks, and OpenTelemetry. Users can create custom plugins and providers for seamless integration with their infrastructure.
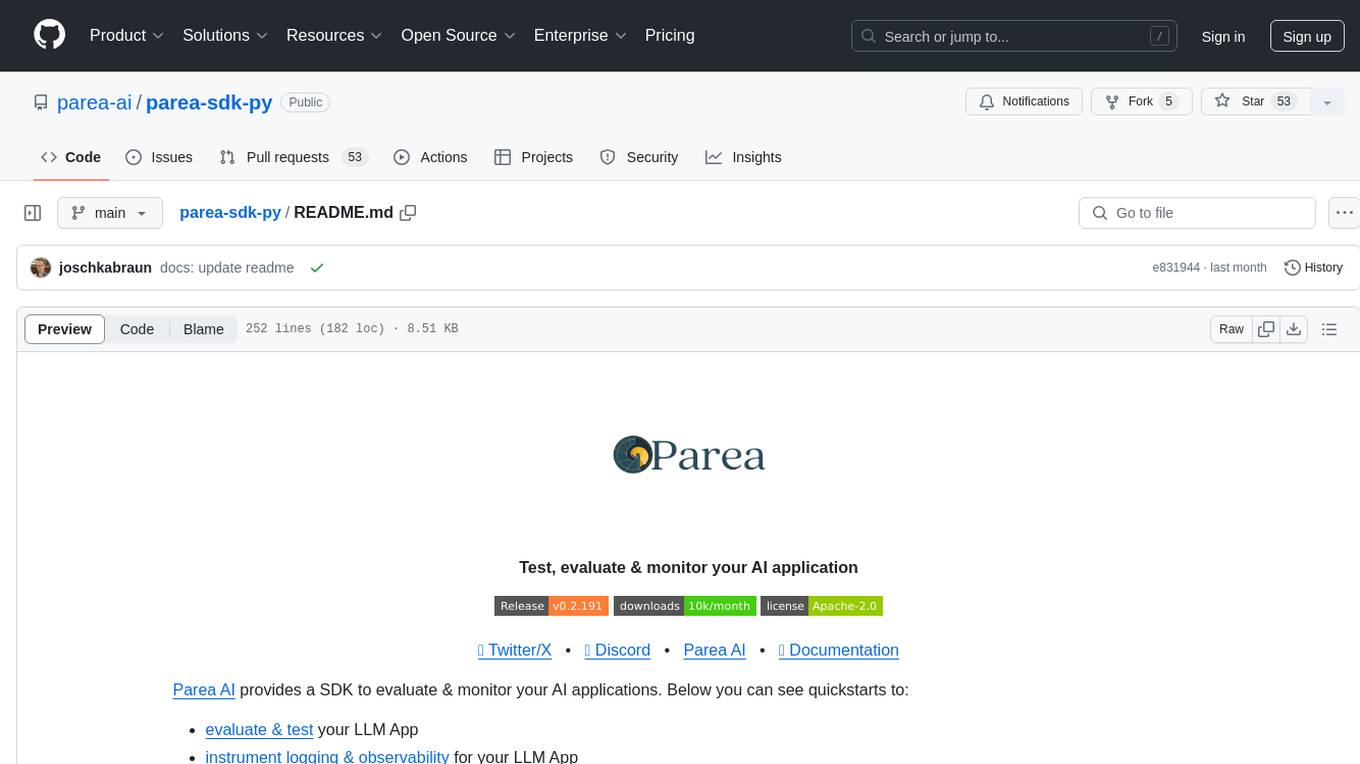
parea-sdk-py
Parea AI provides a SDK to evaluate & monitor AI applications. It allows users to test, evaluate, and monitor their AI models by defining and running experiments. The SDK also enables logging and observability for AI applications, as well as deploying prompts to facilitate collaboration between engineers and subject-matter experts. Users can automatically log calls to OpenAI and Anthropic, create hierarchical traces of their applications, and deploy prompts for integration into their applications.
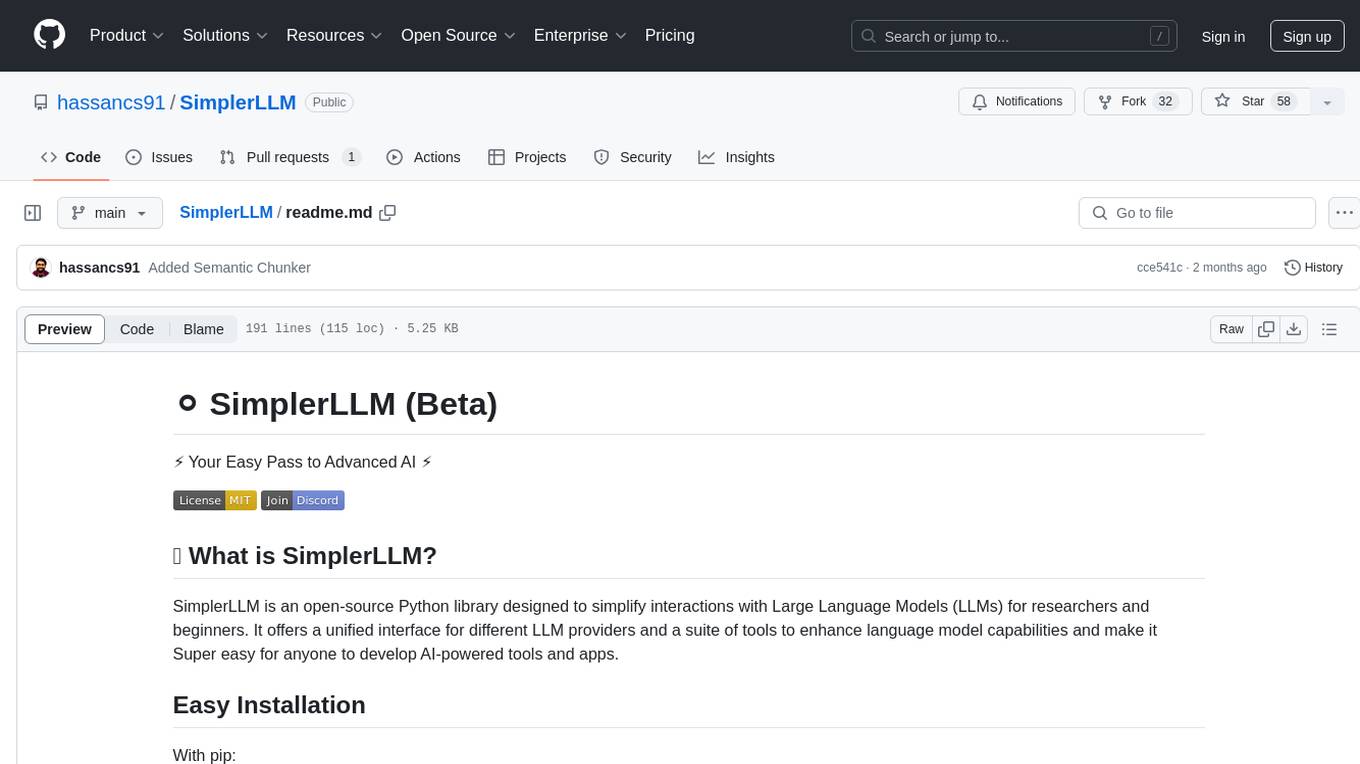
SimplerLLM
SimplerLLM is an open-source Python library that simplifies interactions with Large Language Models (LLMs) for researchers and beginners. It provides a unified interface for different LLM providers, tools for enhancing language model capabilities, and easy development of AI-powered tools and apps. The library offers features like unified LLM interface, generic text loader, RapidAPI connector, SERP integration, prompt template builder, and more. Users can easily set up environment variables, create LLM instances, use tools like SERP, generic text loader, calling RapidAPI APIs, and prompt template builder. Additionally, the library includes chunking functions to split texts into manageable chunks based on different criteria. Future updates will bring more tools, interactions with local LLMs, prompt optimization, response evaluation, GPT Trainer, document chunker, advanced document loader, integration with more providers, Simple RAG with SimplerVectors, integration with vector databases, agent builder, and LLM server.
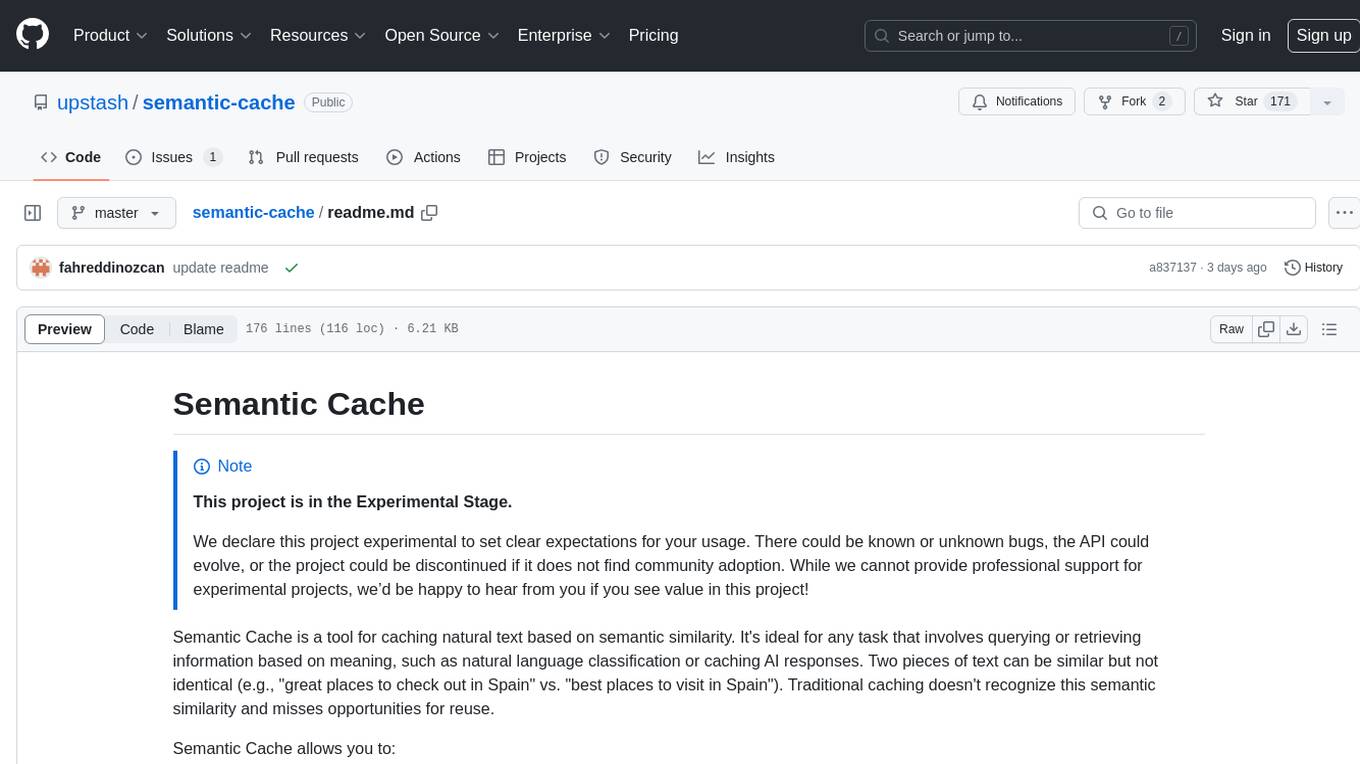
semantic-cache
Semantic Cache is a tool for caching natural text based on semantic similarity. It allows for classifying text into categories, caching AI responses, and reducing API latency by responding to similar queries with cached values. The tool stores cache entries by meaning, handles synonyms, supports multiple languages, understands complex queries, and offers easy integration with Node.js applications. Users can set a custom proximity threshold for filtering results. The tool is ideal for tasks involving querying or retrieving information based on meaning, such as natural language classification or caching AI responses.
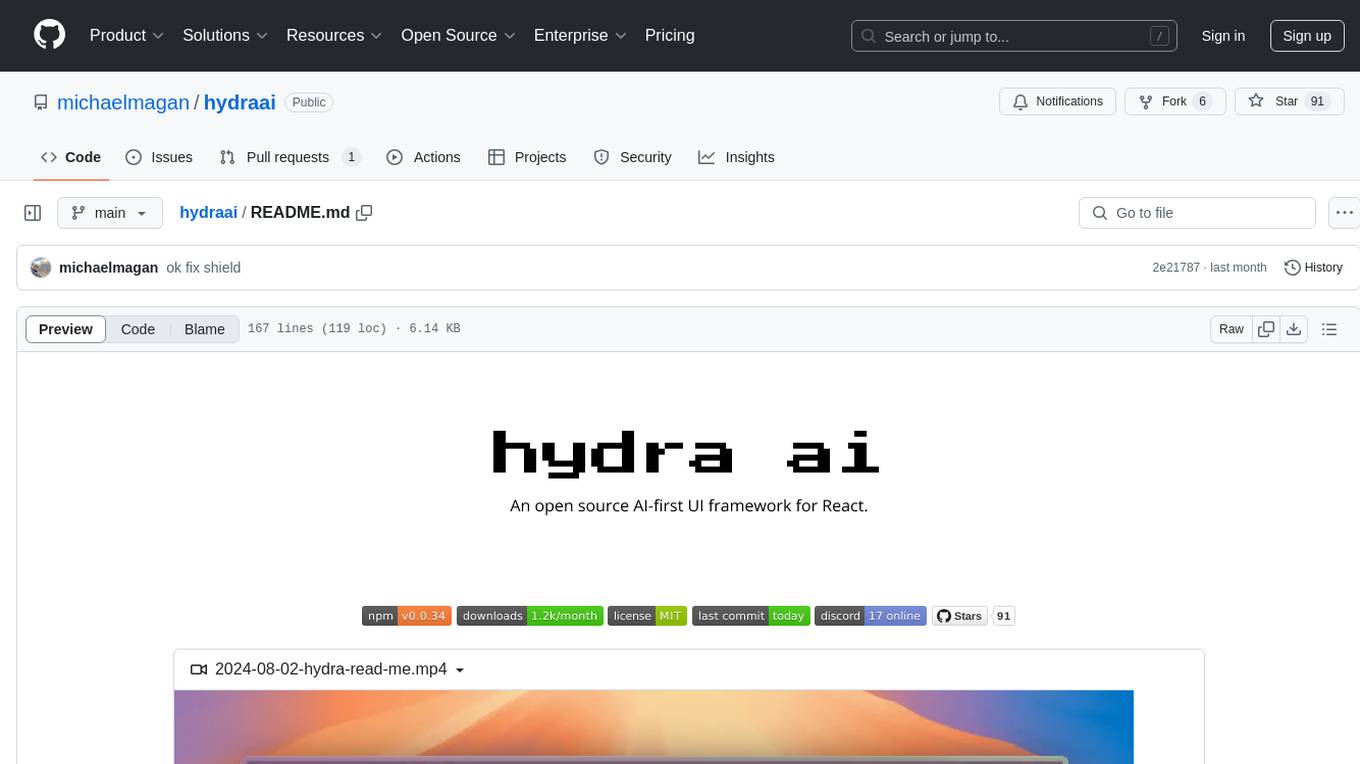
hydraai
Generate React components on-the-fly at runtime using AI. Register your components, and let Hydra choose when to show them in your App. Hydra development is still early, and patterns for different types of components and apps are still being developed. Join the discord to chat with the developers. Expects to be used in a NextJS project. Components that have function props do not work.
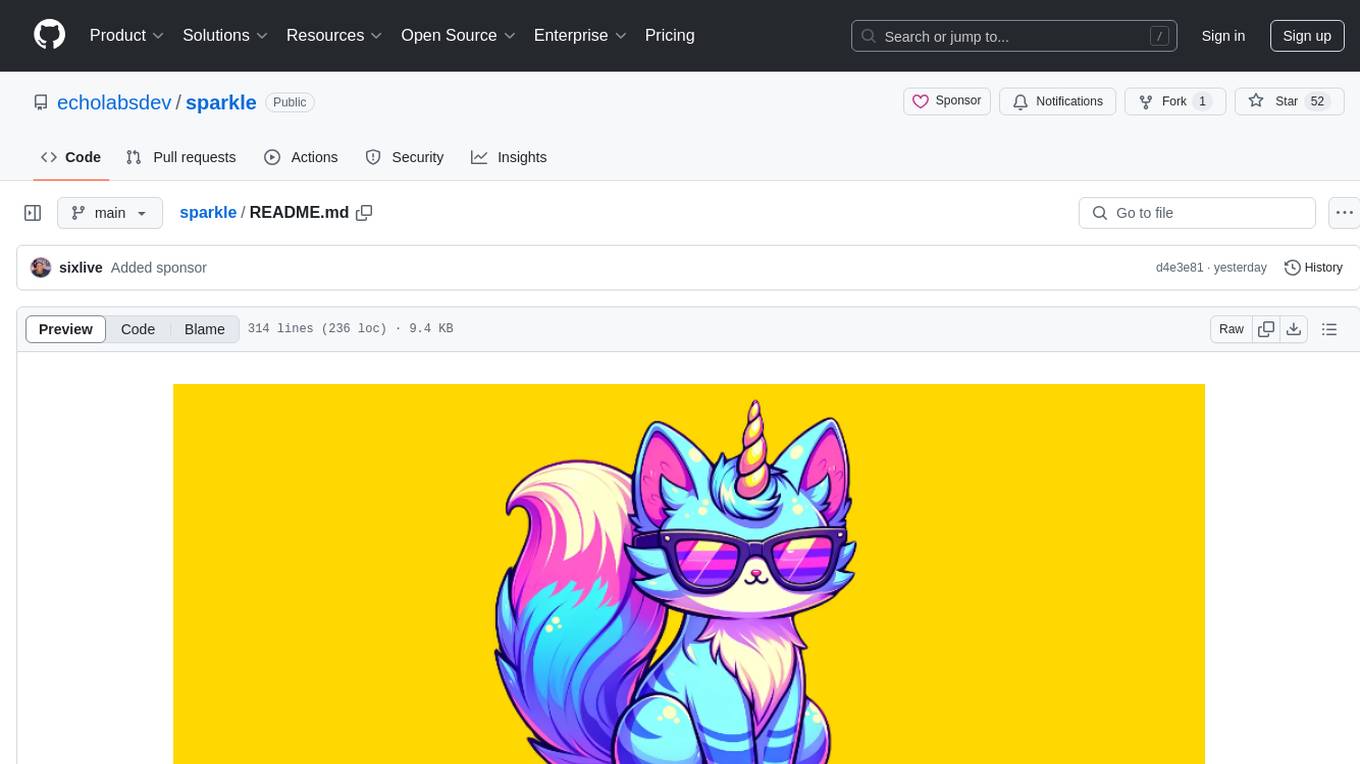
sparkle
Sparkle is a tool that streamlines the process of building AI-driven features in applications using Large Language Models (LLMs). It guides users through creating and managing agents, defining tools, and interacting with LLM providers like OpenAI. Sparkle allows customization of LLM provider settings, model configurations, and provides a seamless integration with Sparkle Server for exposing agents via an OpenAI-compatible chat API endpoint.
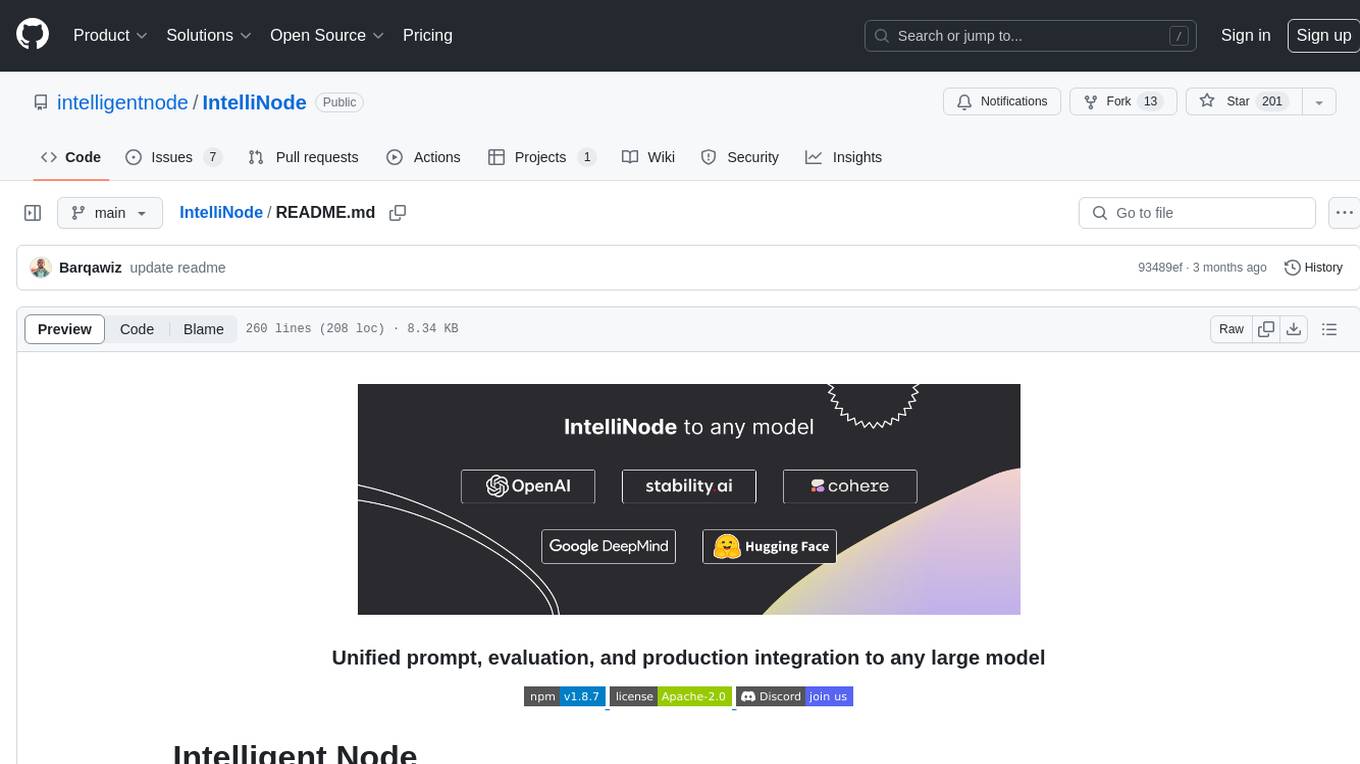
IntelliNode
IntelliNode is a javascript module that integrates cutting-edge AI models like ChatGPT, LLaMA, WaveNet, Gemini, and Stable diffusion into projects. It offers functions for generating text, speech, and images, as well as semantic search, multi-model evaluation, and chatbot capabilities. The module provides a wrapper layer for low-level model access, a controller layer for unified input handling, and a function layer for abstract functionality tailored to various use cases.
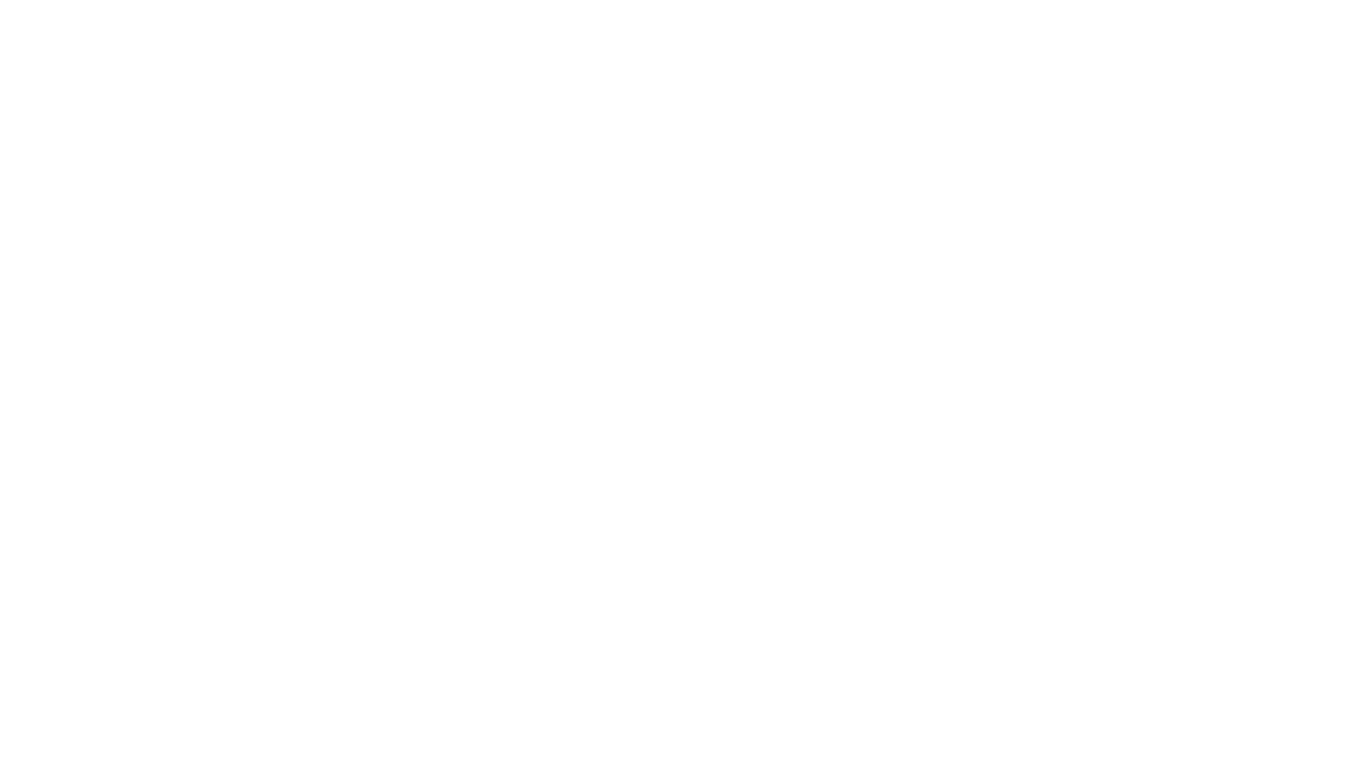
instructor-php
Instructor for PHP is a library designed for structured data extraction in PHP, powered by Large Language Models (LLMs). It simplifies the process of extracting structured, validated data from unstructured text or chat sequences. Instructor enhances workflow by providing a response model, validation capabilities, and max retries for requests. It supports classes as response models and provides features like partial results, string input, extracting scalar and enum values, and specifying data models using PHP type hints or DocBlock comments. The library allows customization of validation and provides detailed event notifications during request processing. Instructor is compatible with PHP 8.2+ and leverages PHP reflection, Symfony components, and SaloonPHP for communication with LLM API providers.
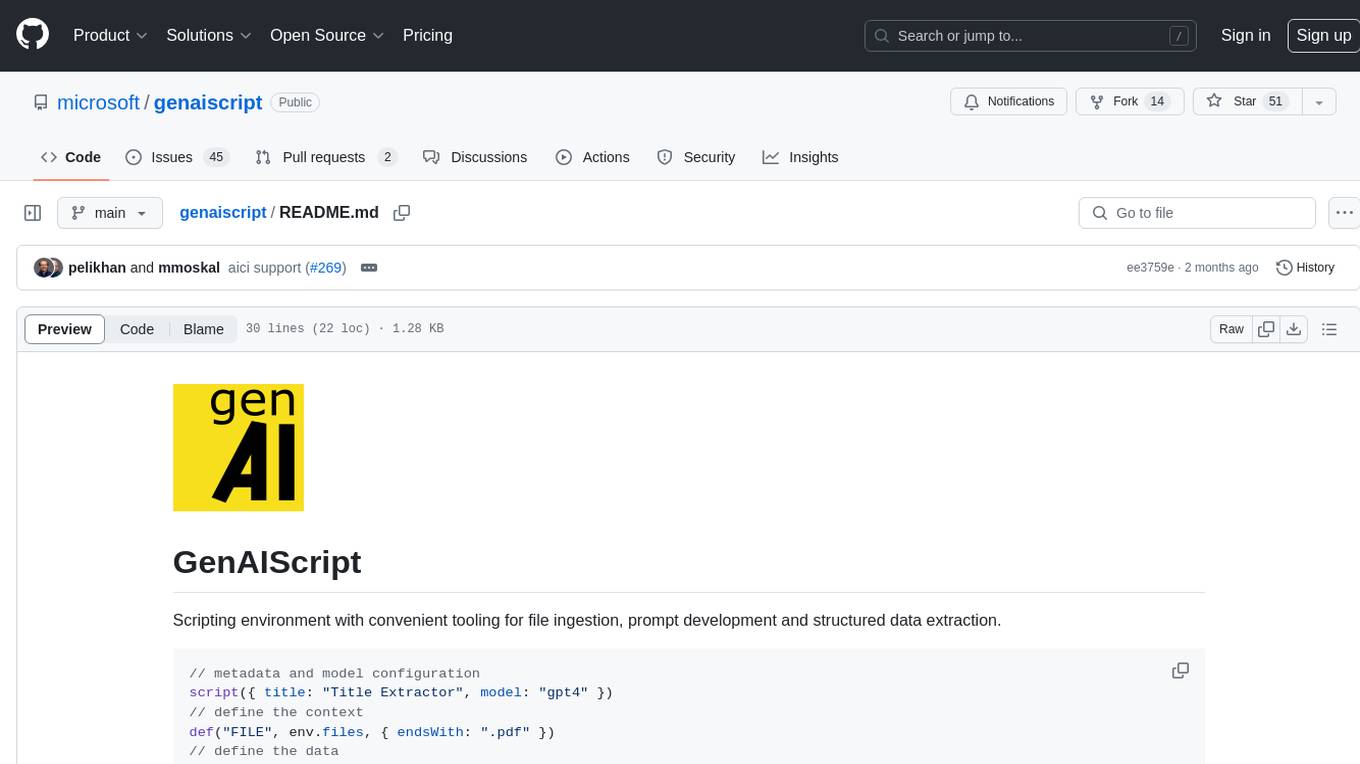
genaiscript
GenAIScript is a scripting environment designed to facilitate file ingestion, prompt development, and structured data extraction. Users can define metadata and model configurations, specify data sources, and define tasks to extract specific information. The tool provides a convenient way to analyze files and extract desired content in a structured format. It offers a user-friendly interface for working with data and automating data extraction processes, making it suitable for various data processing tasks.
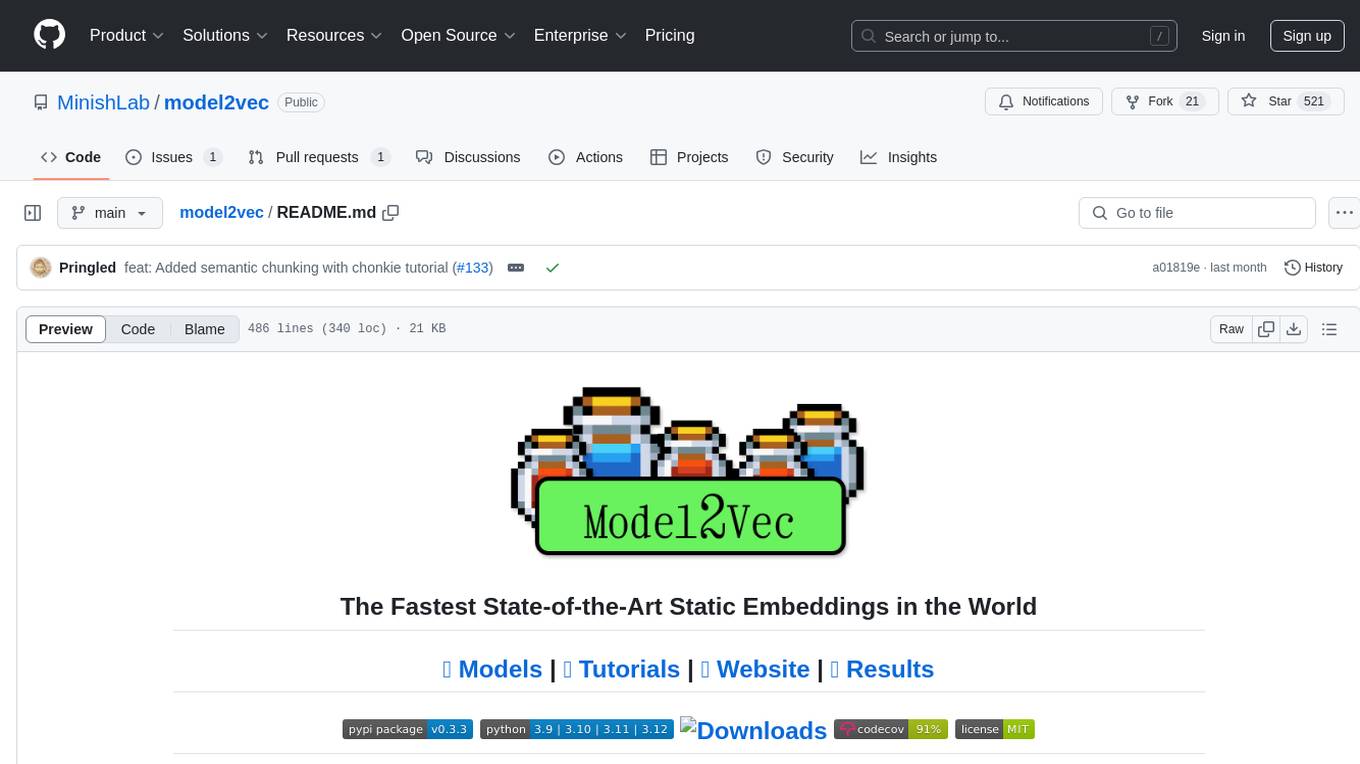
model2vec
Model2Vec is a technique to turn any sentence transformer into a really small static model, reducing model size by 15x and making the models up to 500x faster, with a small drop in performance. It outperforms other static embedding models like GLoVe and BPEmb, is lightweight with only `numpy` as a major dependency, offers fast inference, dataset-free distillation, and is integrated into Sentence Transformers, txtai, and Chonkie. Model2Vec creates powerful models by passing a vocabulary through a sentence transformer model, reducing dimensionality using PCA, and weighting embeddings using zipf weighting. Users can distill their own models or use pre-trained models from the HuggingFace hub. Evaluation can be done using the provided evaluation package. Model2Vec is licensed under MIT.
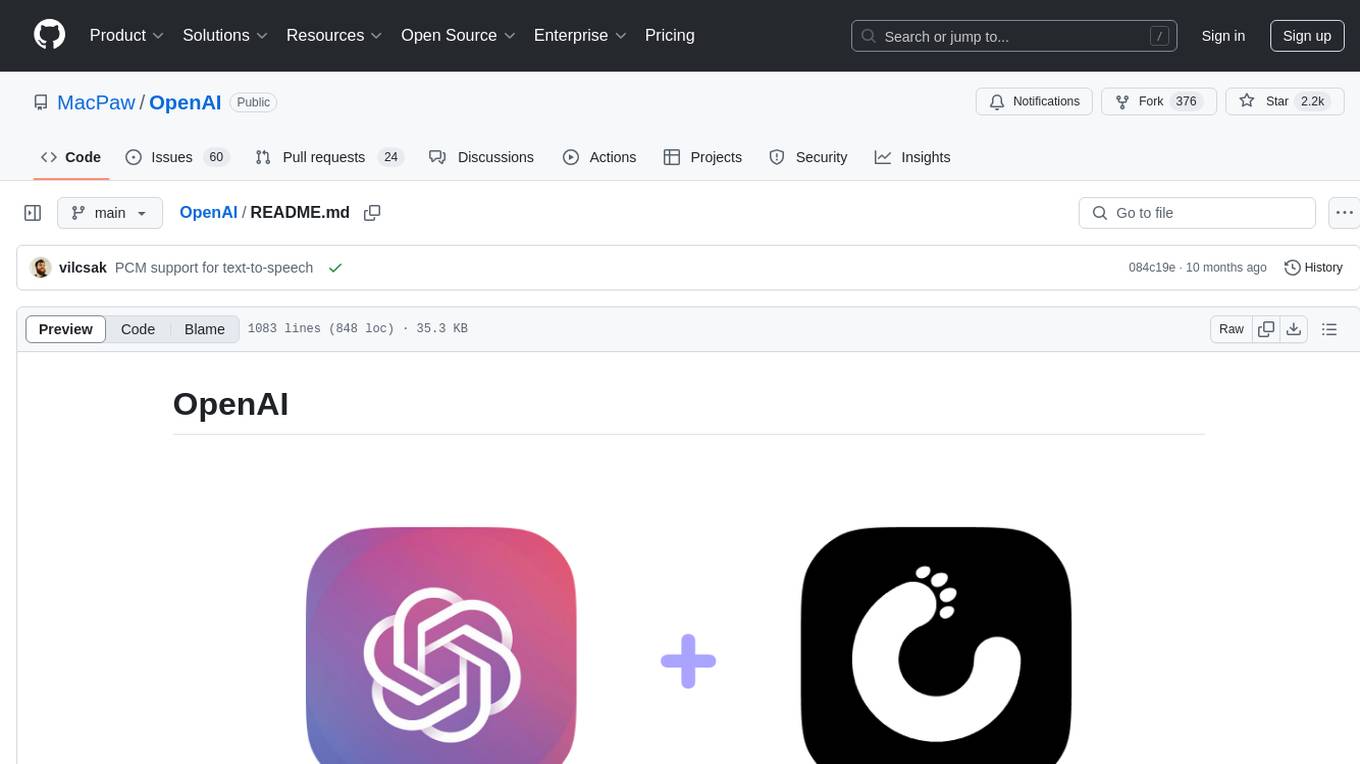
OpenAI
OpenAI is a Swift community-maintained implementation over OpenAI public API. It is a non-profit artificial intelligence research organization founded in San Francisco, California in 2015. OpenAI's mission is to ensure safe and responsible use of AI for civic good, economic growth, and other public benefits. The repository provides functionalities for text completions, chats, image generation, audio processing, edits, embeddings, models, moderations, utilities, and Combine extensions.
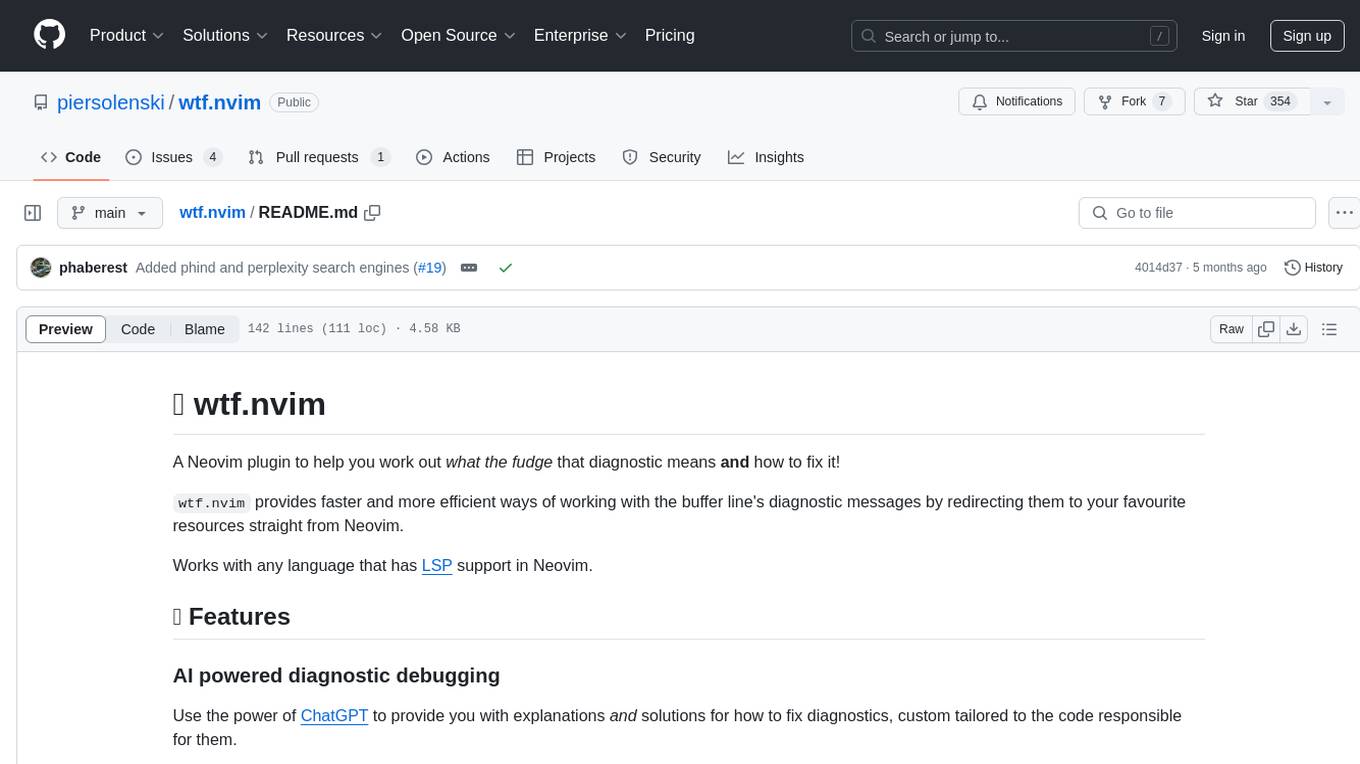
wtf.nvim
wtf.nvim is a Neovim plugin that enhances diagnostic debugging by providing explanations and solutions for code issues using ChatGPT. It allows users to search the web for answers directly from Neovim, making the debugging process faster and more efficient. The plugin works with any language that has LSP support in Neovim, offering AI-powered diagnostic assistance and seamless integration with various resources for resolving coding problems.
For similar tasks
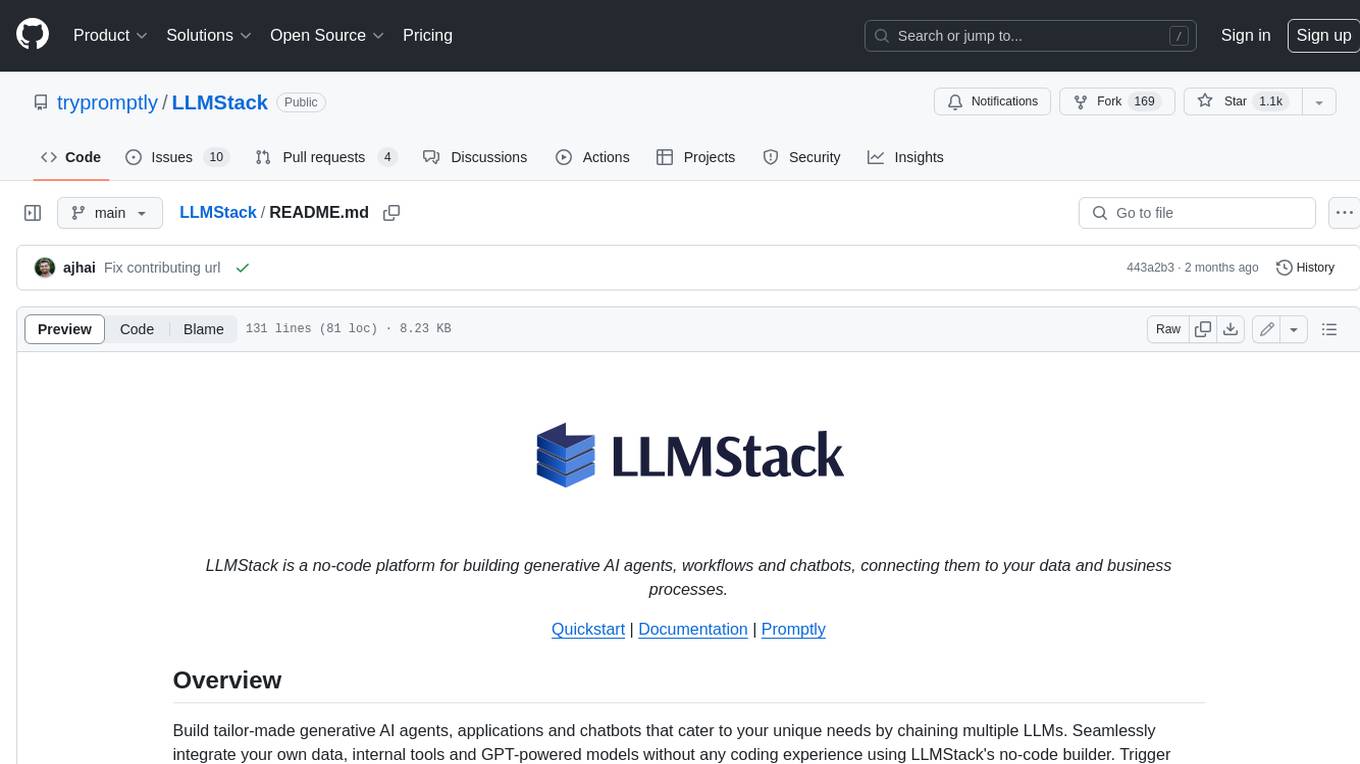
LLMStack
LLMStack is a no-code platform for building generative AI agents, workflows, and chatbots. It allows users to connect their own data, internal tools, and GPT-powered models without any coding experience. LLMStack can be deployed to the cloud or on-premise and can be accessed via HTTP API or triggered from Slack or Discord.
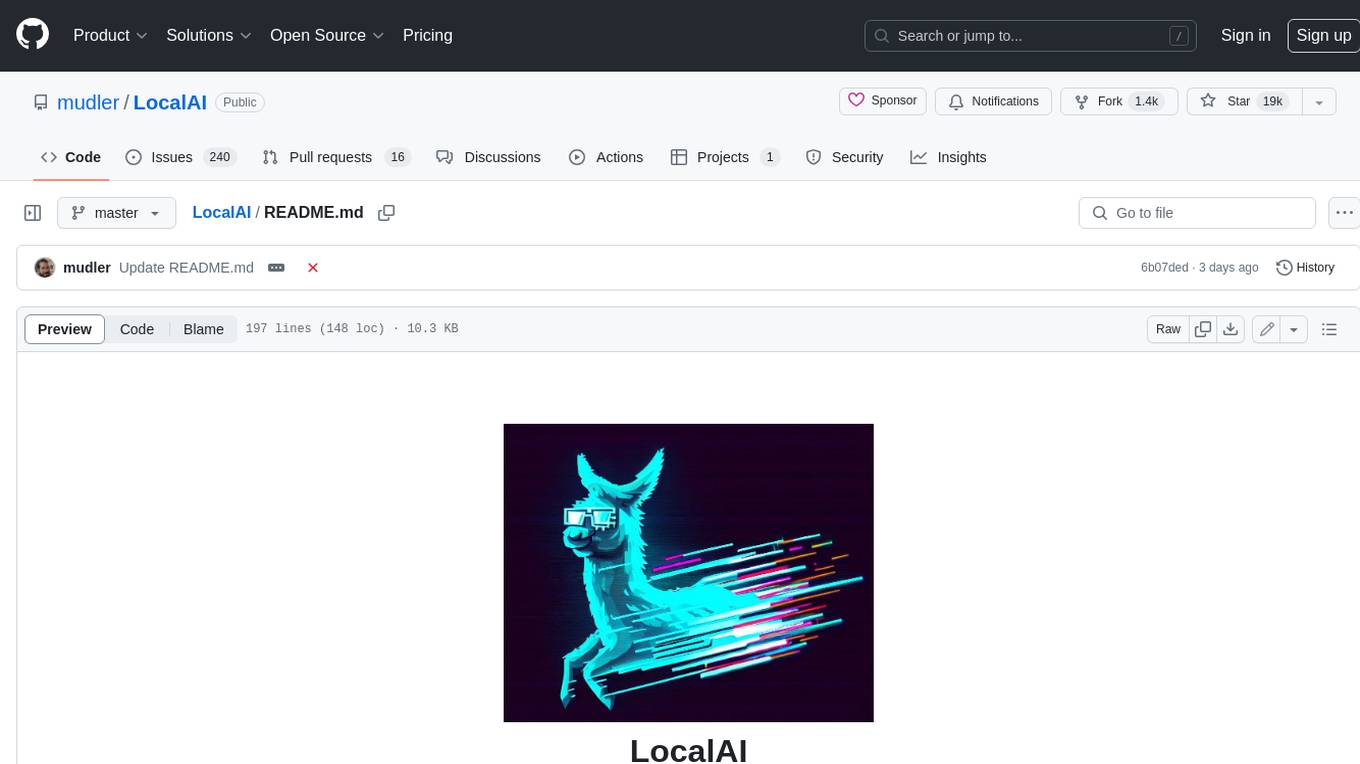
LocalAI
LocalAI is a free and open-source OpenAI alternative that acts as a drop-in replacement REST API compatible with OpenAI (Elevenlabs, Anthropic, etc.) API specifications for local AI inferencing. It allows users to run LLMs, generate images, audio, and more locally or on-premises with consumer-grade hardware, supporting multiple model families and not requiring a GPU. LocalAI offers features such as text generation with GPTs, text-to-audio, audio-to-text transcription, image generation with stable diffusion, OpenAI functions, embeddings generation for vector databases, constrained grammars, downloading models directly from Huggingface, and a Vision API. It provides a detailed step-by-step introduction in its Getting Started guide and supports community integrations such as custom containers, WebUIs, model galleries, and various bots for Discord, Slack, and Telegram. LocalAI also offers resources like an LLM fine-tuning guide, instructions for local building and Kubernetes installation, projects integrating LocalAI, and a how-tos section curated by the community. It encourages users to cite the repository when utilizing it in downstream projects and acknowledges the contributions of various software from the community.
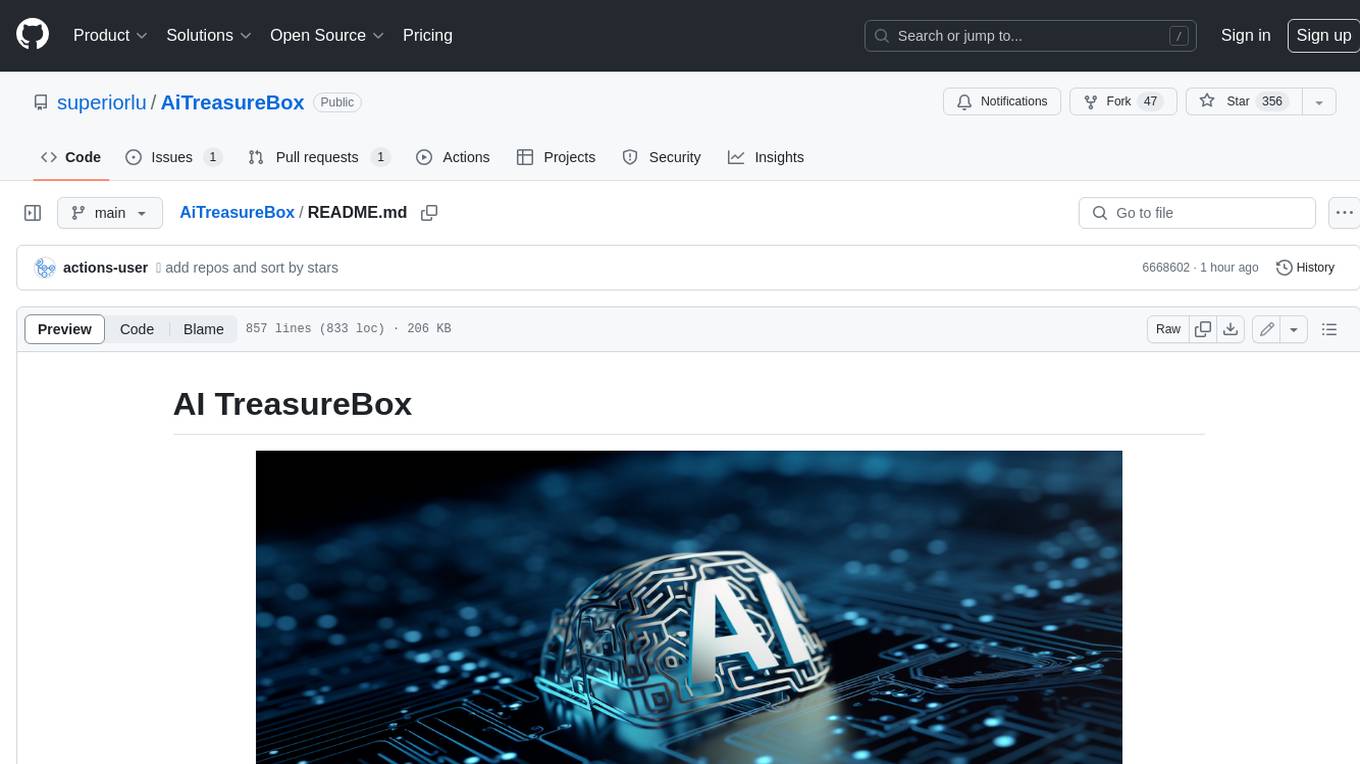
AiTreasureBox
AiTreasureBox is a versatile AI tool that provides a collection of pre-trained models and algorithms for various machine learning tasks. It simplifies the process of implementing AI solutions by offering ready-to-use components that can be easily integrated into projects. With AiTreasureBox, users can quickly prototype and deploy AI applications without the need for extensive knowledge in machine learning or deep learning. The tool covers a wide range of tasks such as image classification, text generation, sentiment analysis, object detection, and more. It is designed to be user-friendly and accessible to both beginners and experienced developers, making AI development more efficient and accessible to a wider audience.
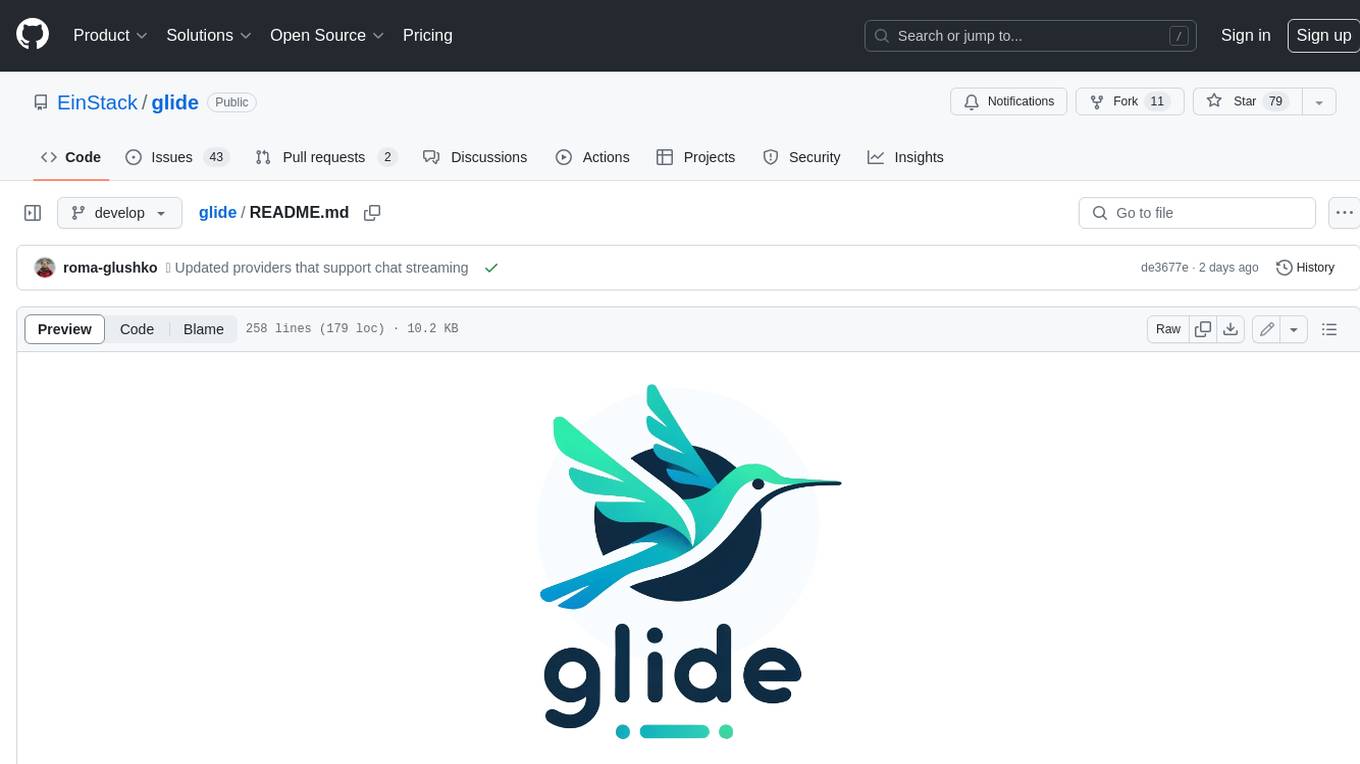
glide
Glide is a cloud-native LLM gateway that provides a unified REST API for accessing various large language models (LLMs) from different providers. It handles LLMOps tasks such as model failover, caching, key management, and more, making it easy to integrate LLMs into applications. Glide supports popular LLM providers like OpenAI, Anthropic, Azure OpenAI, AWS Bedrock (Titan), Cohere, Google Gemini, OctoML, and Ollama. It offers high availability, performance, and observability, and provides SDKs for Python and NodeJS to simplify integration.
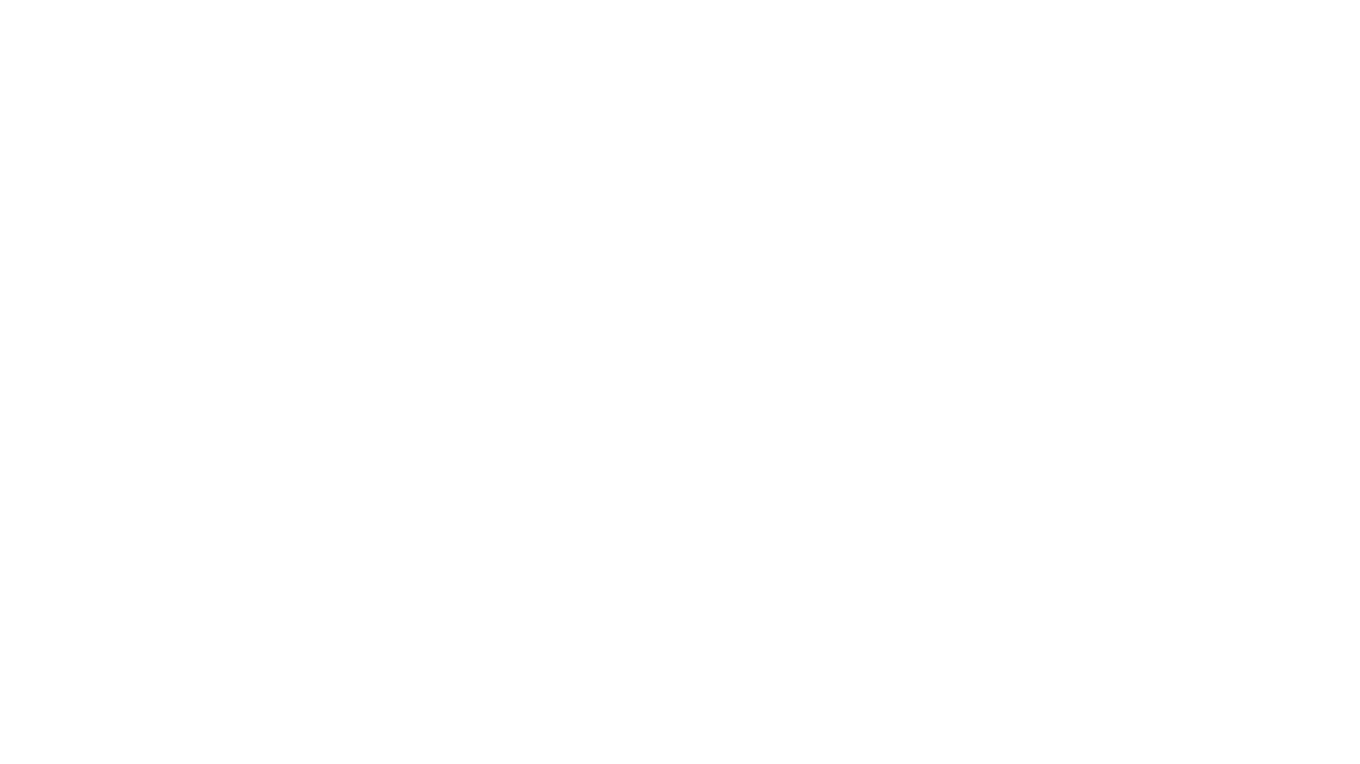
jupyter-ai
Jupyter AI connects generative AI with Jupyter notebooks. It provides a user-friendly and powerful way to explore generative AI models in notebooks and improve your productivity in JupyterLab and the Jupyter Notebook. Specifically, Jupyter AI offers: * An `%%ai` magic that turns the Jupyter notebook into a reproducible generative AI playground. This works anywhere the IPython kernel runs (JupyterLab, Jupyter Notebook, Google Colab, Kaggle, VSCode, etc.). * A native chat UI in JupyterLab that enables you to work with generative AI as a conversational assistant. * Support for a wide range of generative model providers, including AI21, Anthropic, AWS, Cohere, Gemini, Hugging Face, NVIDIA, and OpenAI. * Local model support through GPT4All, enabling use of generative AI models on consumer grade machines with ease and privacy.
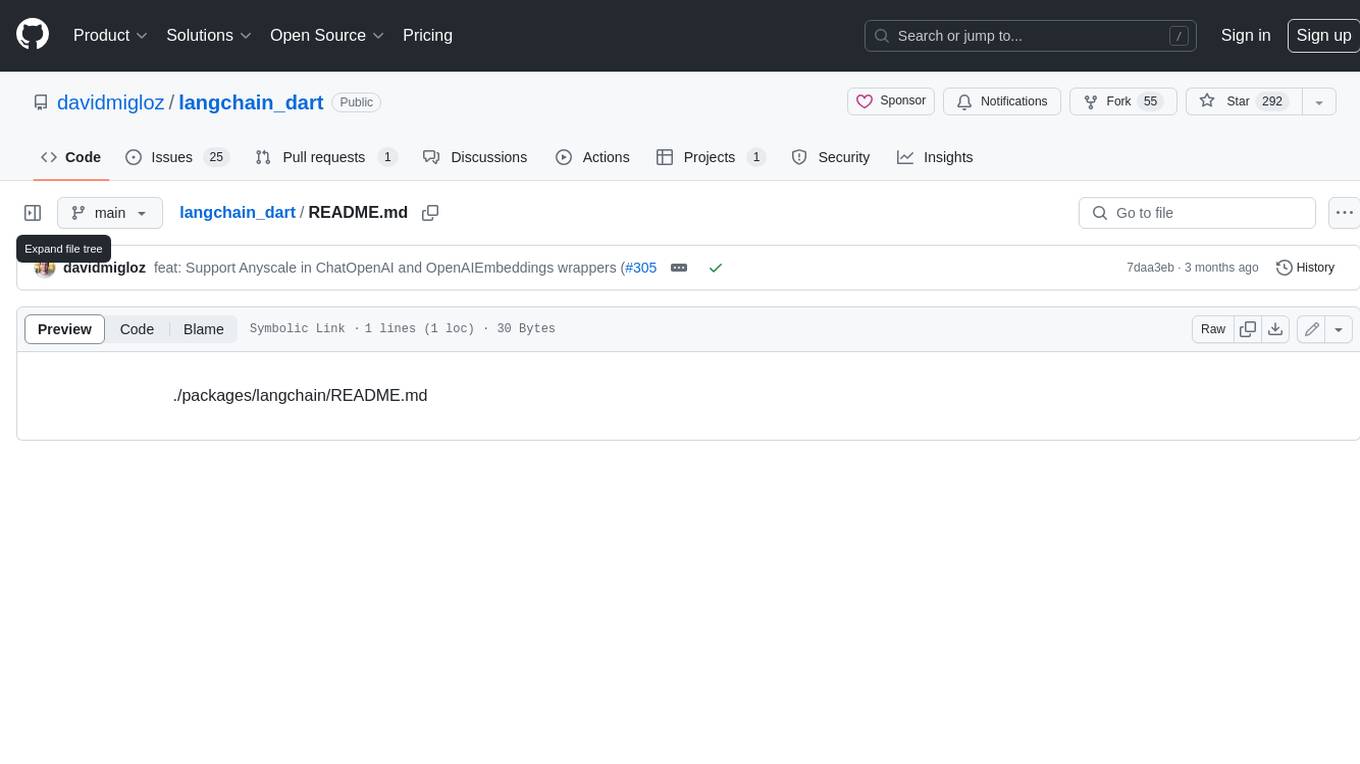
langchain_dart
LangChain.dart is a Dart port of the popular LangChain Python framework created by Harrison Chase. LangChain provides a set of ready-to-use components for working with language models and a standard interface for chaining them together to formulate more advanced use cases (e.g. chatbots, Q&A with RAG, agents, summarization, extraction, etc.). The components can be grouped into a few core modules: * **Model I/O:** LangChain offers a unified API for interacting with various LLM providers (e.g. OpenAI, Google, Mistral, Ollama, etc.), allowing developers to switch between them with ease. Additionally, it provides tools for managing model inputs (prompt templates and example selectors) and parsing the resulting model outputs (output parsers). * **Retrieval:** assists in loading user data (via document loaders), transforming it (with text splitters), extracting its meaning (using embedding models), storing (in vector stores) and retrieving it (through retrievers) so that it can be used to ground the model's responses (i.e. Retrieval-Augmented Generation or RAG). * **Agents:** "bots" that leverage LLMs to make informed decisions about which available tools (such as web search, calculators, database lookup, etc.) to use to accomplish the designated task. The different components can be composed together using the LangChain Expression Language (LCEL).
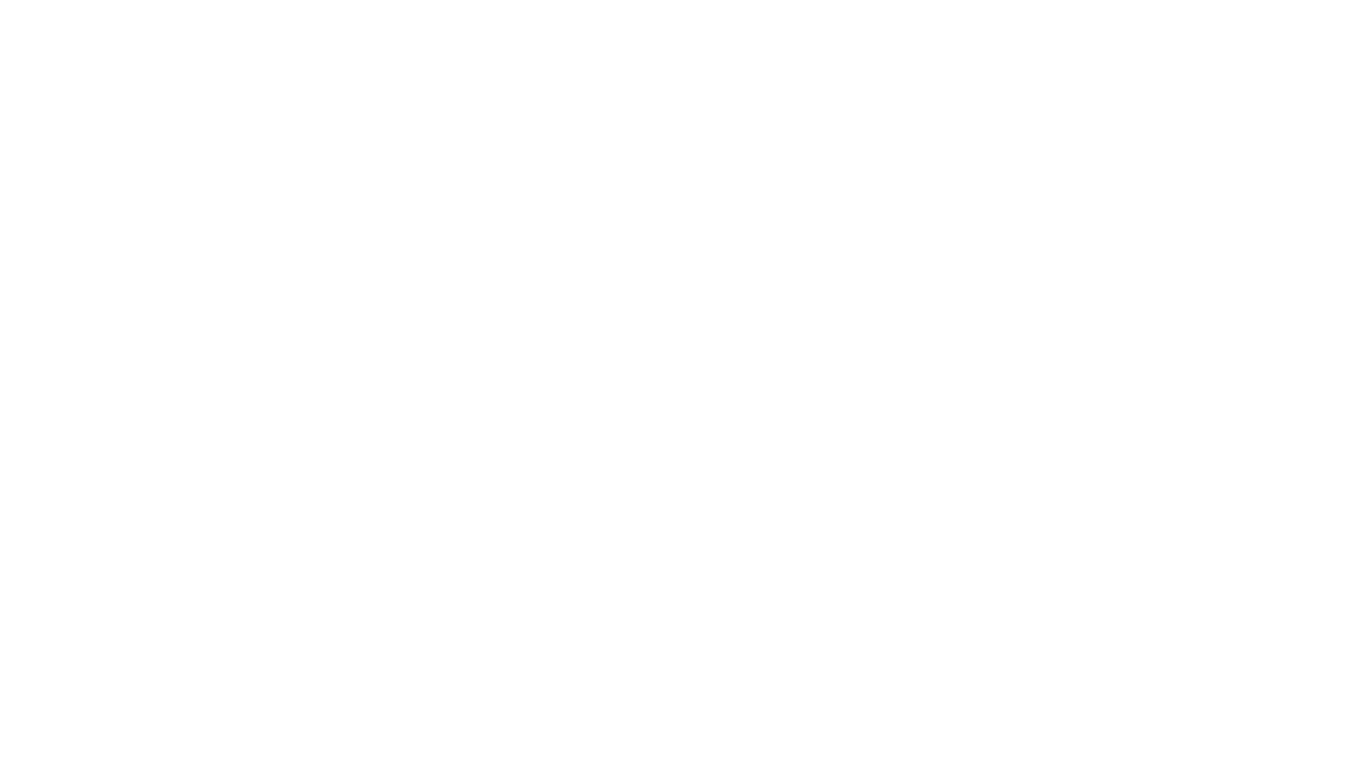
infinity
Infinity is an AI-native database designed for LLM applications, providing incredibly fast full-text and vector search capabilities. It supports a wide range of data types, including vectors, full-text, and structured data, and offers a fused search feature that combines multiple embeddings and full text. Infinity is easy to use, with an intuitive Python API and a single-binary architecture that simplifies deployment. It achieves high performance, with 0.1 milliseconds query latency on million-scale vector datasets and up to 15K QPS.
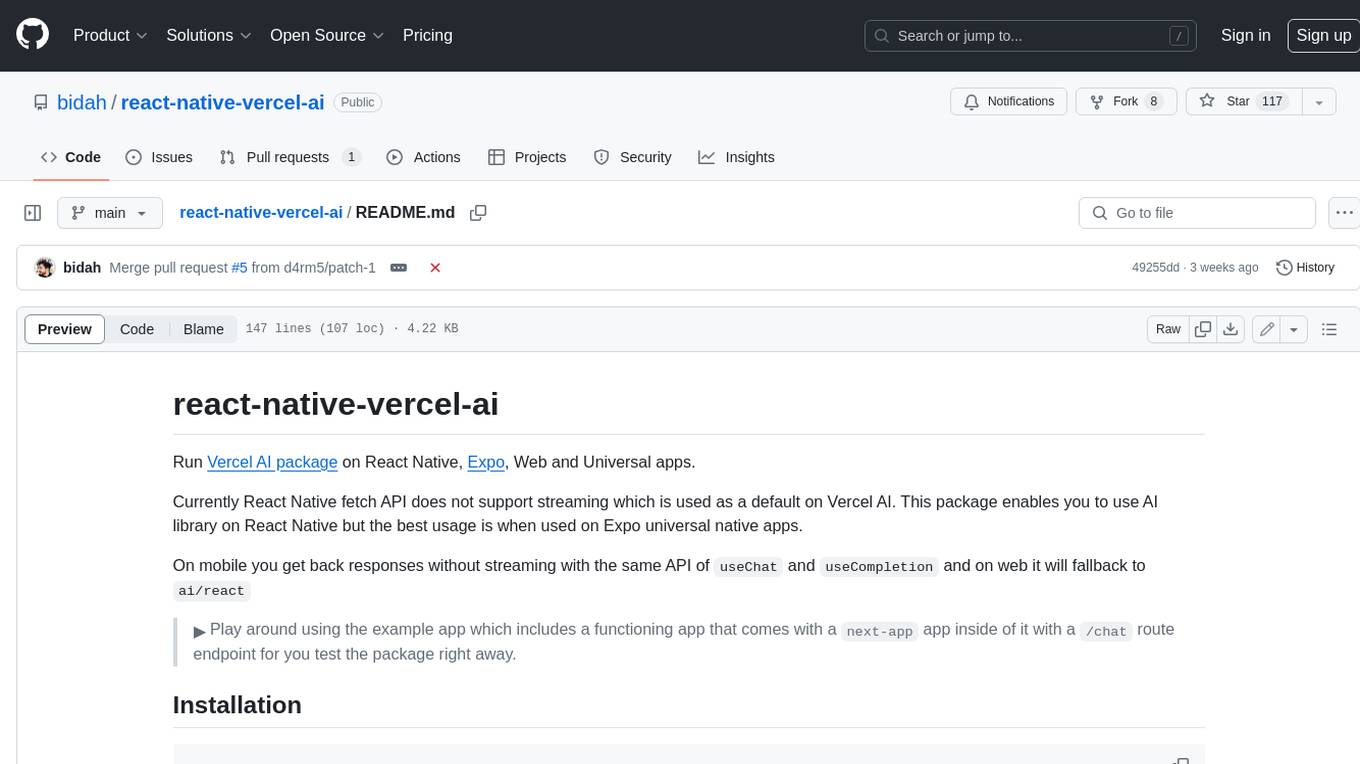
react-native-vercel-ai
Run Vercel AI package on React Native, Expo, Web and Universal apps. Currently React Native fetch API does not support streaming which is used as a default on Vercel AI. This package enables you to use AI library on React Native but the best usage is when used on Expo universal native apps. On mobile you get back responses without streaming with the same API of `useChat` and `useCompletion` and on web it will fallback to `ai/react`
For similar jobs
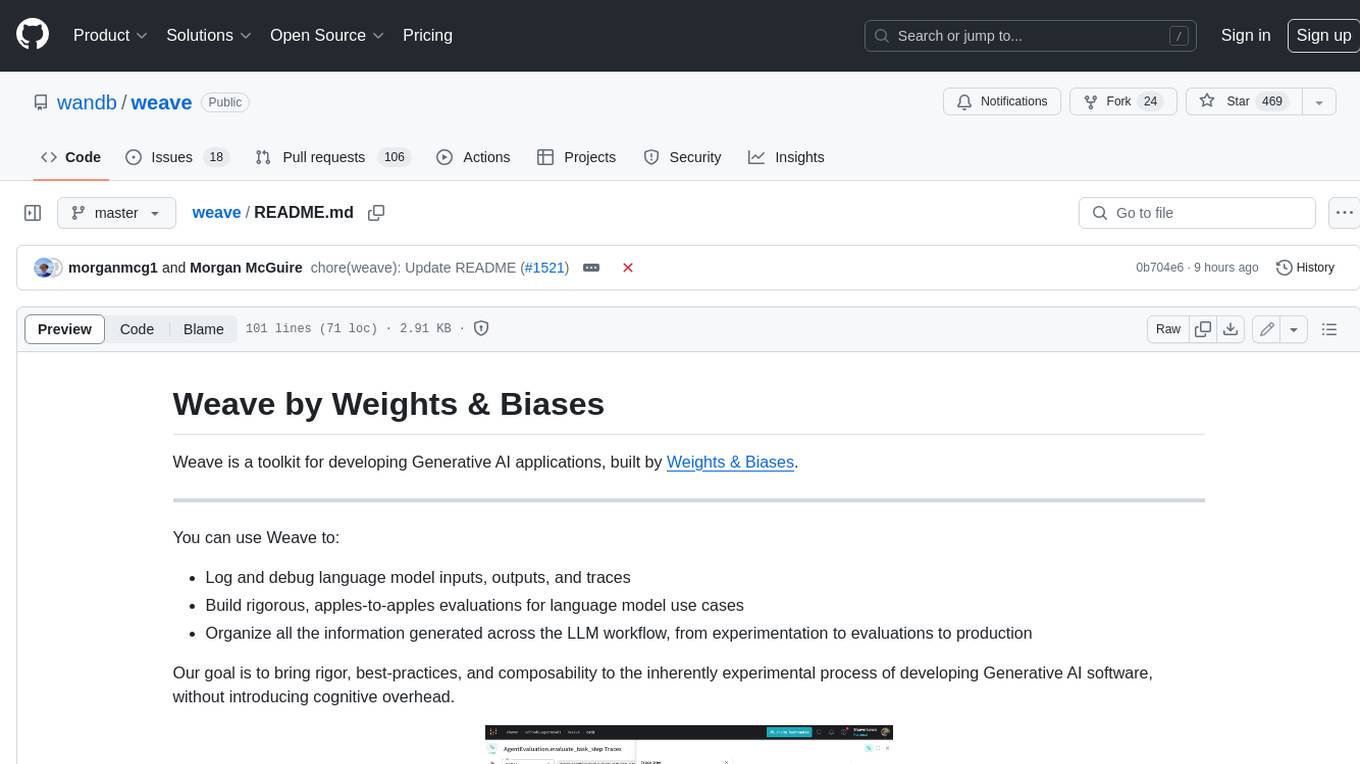
weave
Weave is a toolkit for developing Generative AI applications, built by Weights & Biases. With Weave, you can log and debug language model inputs, outputs, and traces; build rigorous, apples-to-apples evaluations for language model use cases; and organize all the information generated across the LLM workflow, from experimentation to evaluations to production. Weave aims to bring rigor, best-practices, and composability to the inherently experimental process of developing Generative AI software, without introducing cognitive overhead.
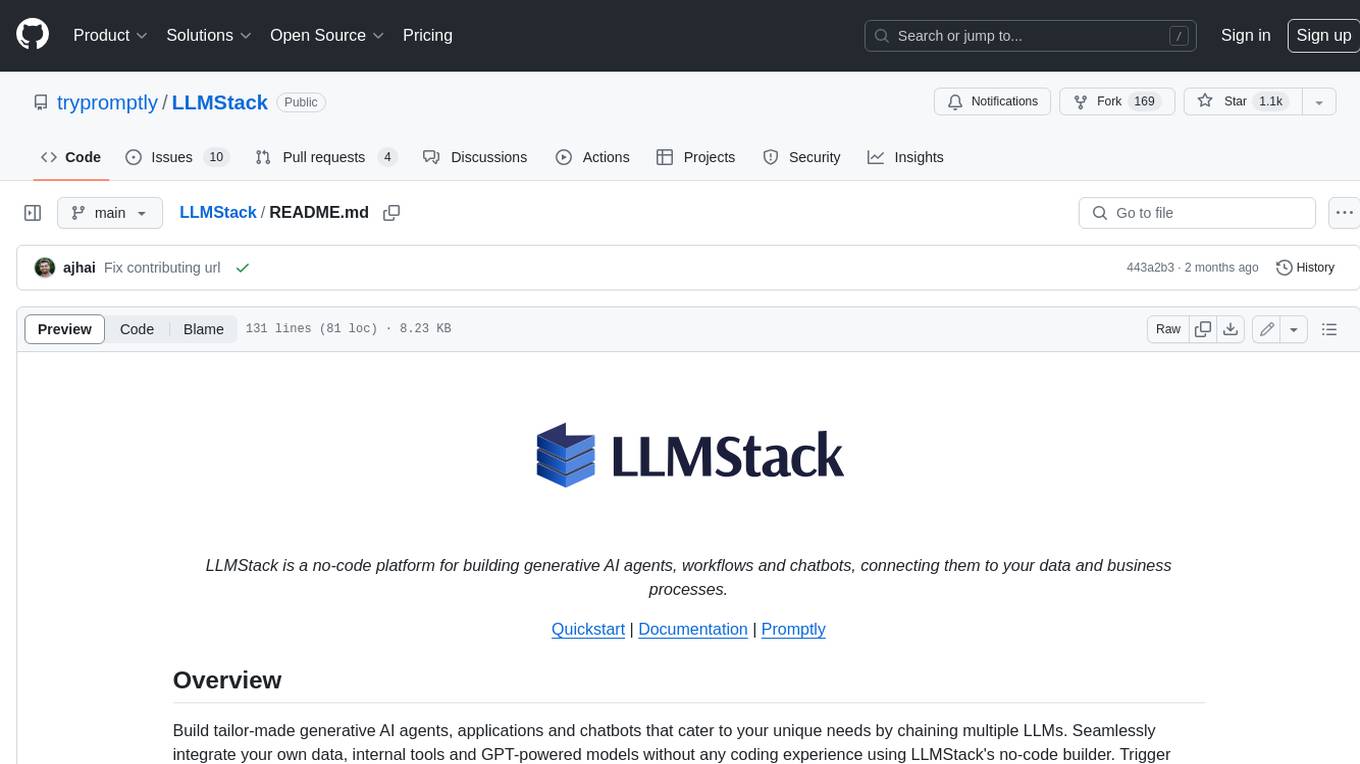
LLMStack
LLMStack is a no-code platform for building generative AI agents, workflows, and chatbots. It allows users to connect their own data, internal tools, and GPT-powered models without any coding experience. LLMStack can be deployed to the cloud or on-premise and can be accessed via HTTP API or triggered from Slack or Discord.
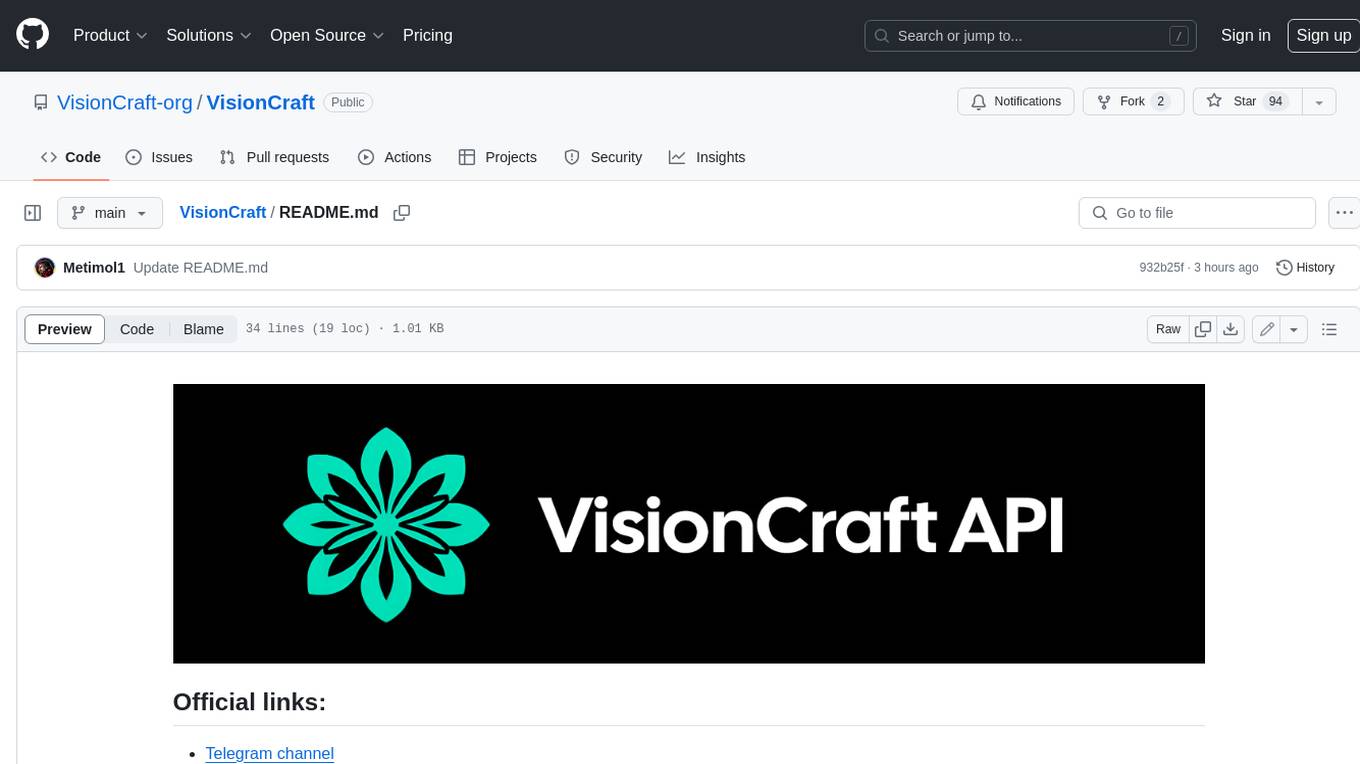
VisionCraft
The VisionCraft API is a free API for using over 100 different AI models. From images to sound.
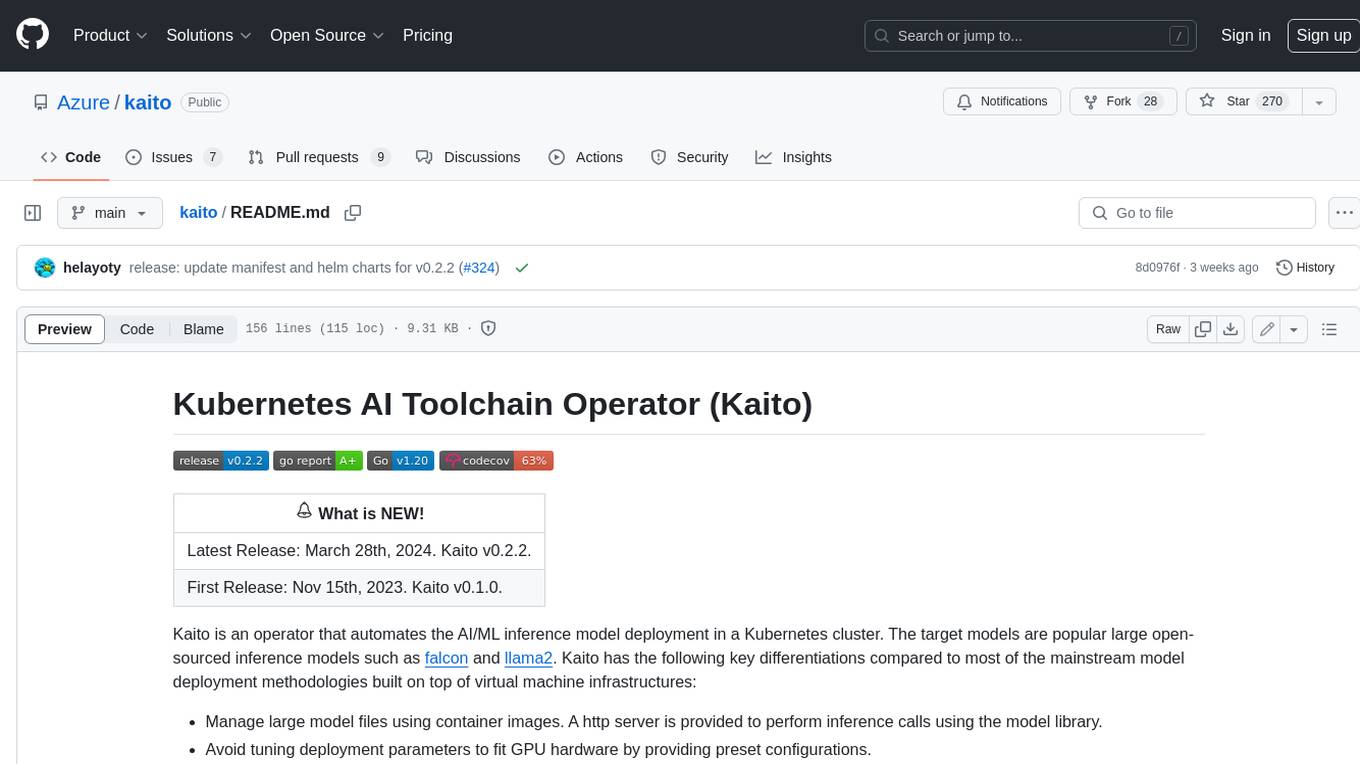
kaito
Kaito is an operator that automates the AI/ML inference model deployment in a Kubernetes cluster. It manages large model files using container images, avoids tuning deployment parameters to fit GPU hardware by providing preset configurations, auto-provisions GPU nodes based on model requirements, and hosts large model images in the public Microsoft Container Registry (MCR) if the license allows. Using Kaito, the workflow of onboarding large AI inference models in Kubernetes is largely simplified.
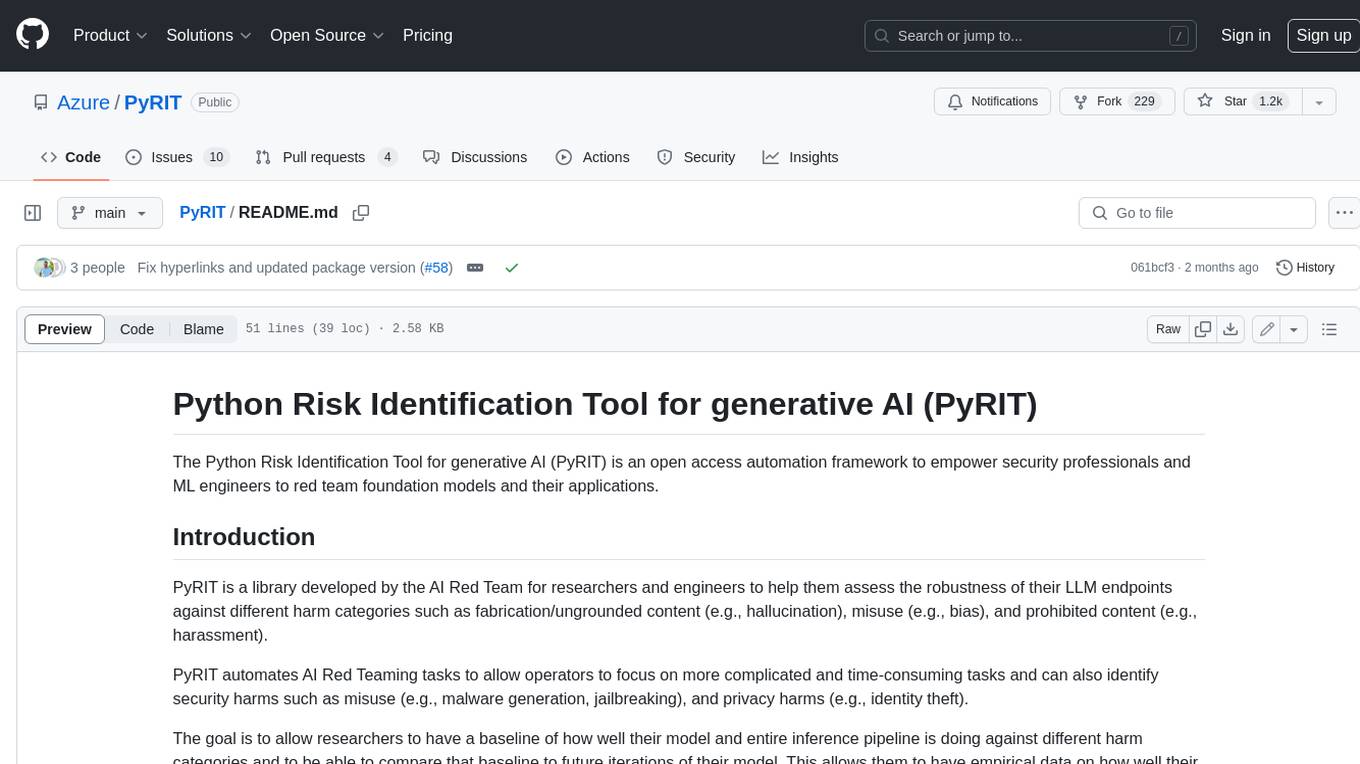
PyRIT
PyRIT is an open access automation framework designed to empower security professionals and ML engineers to red team foundation models and their applications. It automates AI Red Teaming tasks to allow operators to focus on more complicated and time-consuming tasks and can also identify security harms such as misuse (e.g., malware generation, jailbreaking), and privacy harms (e.g., identity theft). The goal is to allow researchers to have a baseline of how well their model and entire inference pipeline is doing against different harm categories and to be able to compare that baseline to future iterations of their model. This allows them to have empirical data on how well their model is doing today, and detect any degradation of performance based on future improvements.
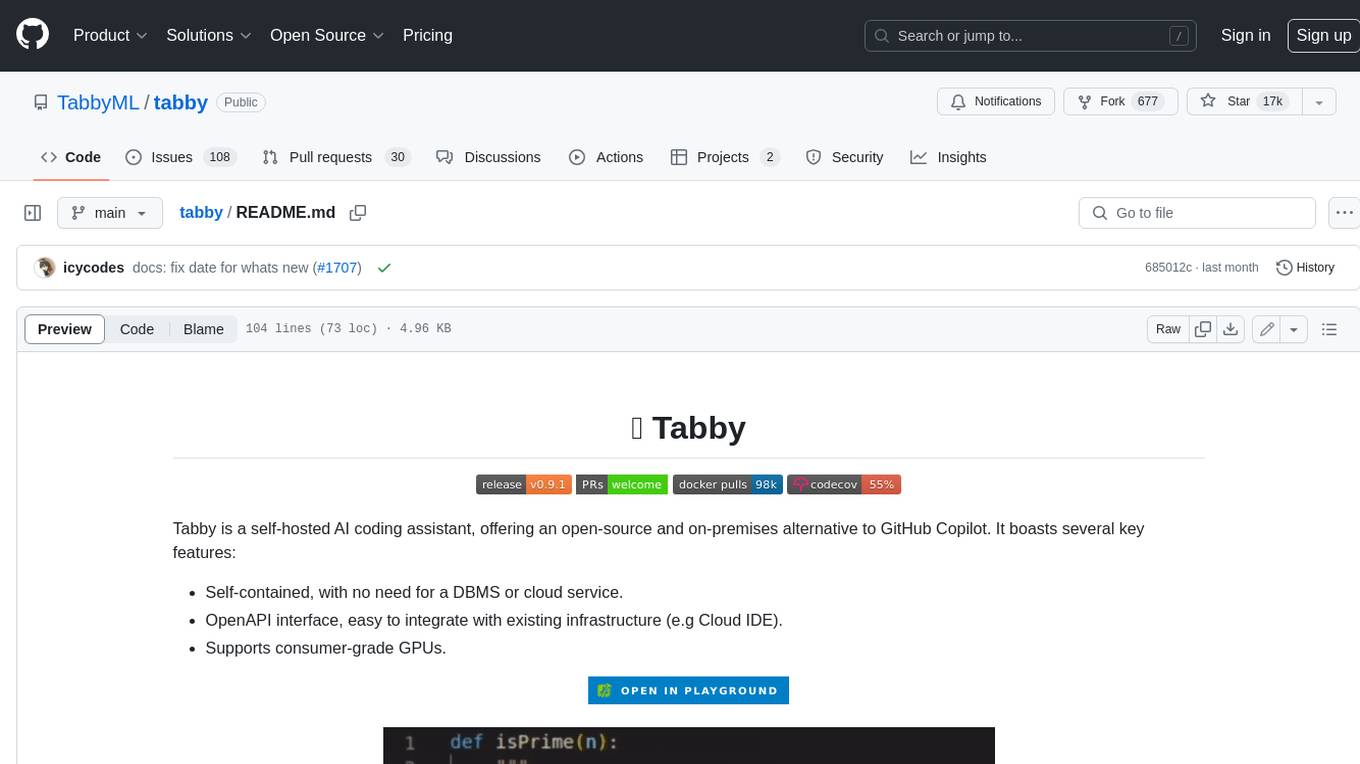
tabby
Tabby is a self-hosted AI coding assistant, offering an open-source and on-premises alternative to GitHub Copilot. It boasts several key features: * Self-contained, with no need for a DBMS or cloud service. * OpenAPI interface, easy to integrate with existing infrastructure (e.g Cloud IDE). * Supports consumer-grade GPUs.
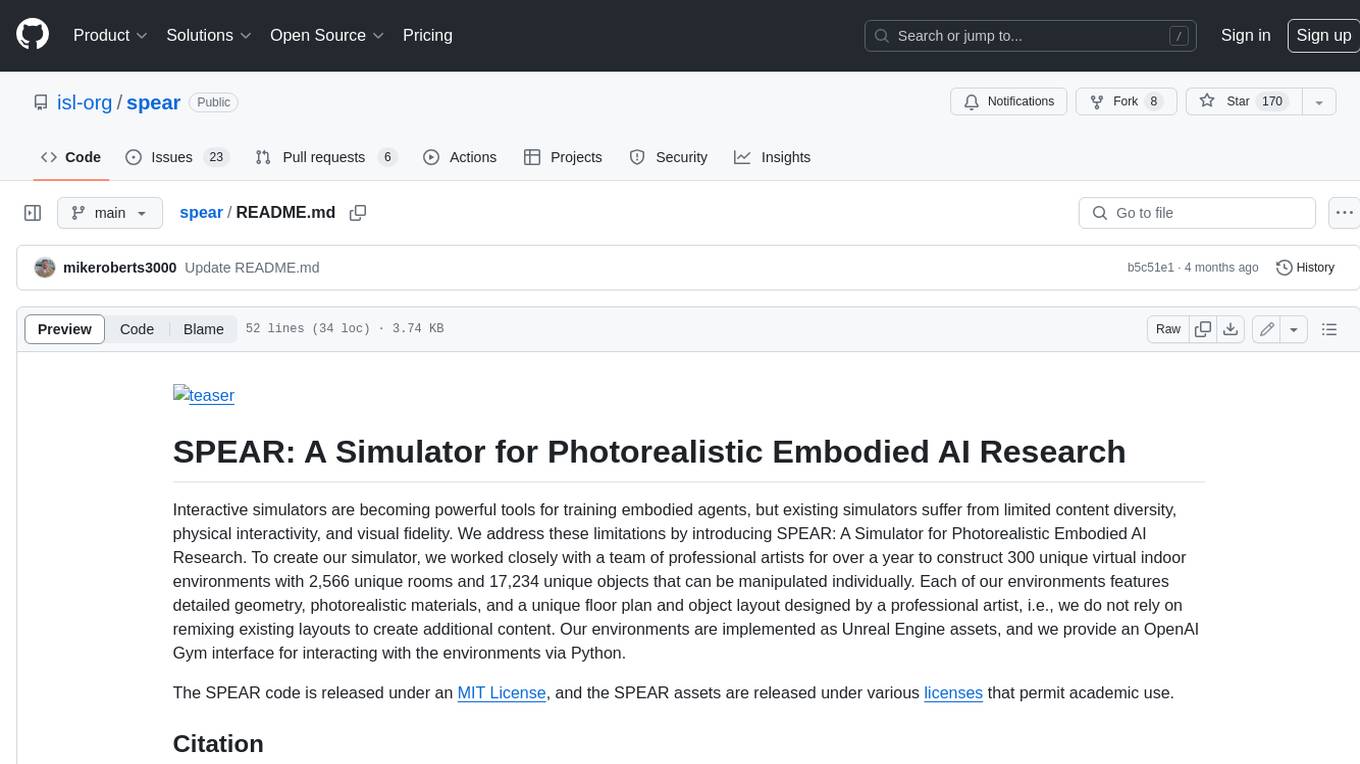
spear
SPEAR (Simulator for Photorealistic Embodied AI Research) is a powerful tool for training embodied agents. It features 300 unique virtual indoor environments with 2,566 unique rooms and 17,234 unique objects that can be manipulated individually. Each environment is designed by a professional artist and features detailed geometry, photorealistic materials, and a unique floor plan and object layout. SPEAR is implemented as Unreal Engine assets and provides an OpenAI Gym interface for interacting with the environments via Python.
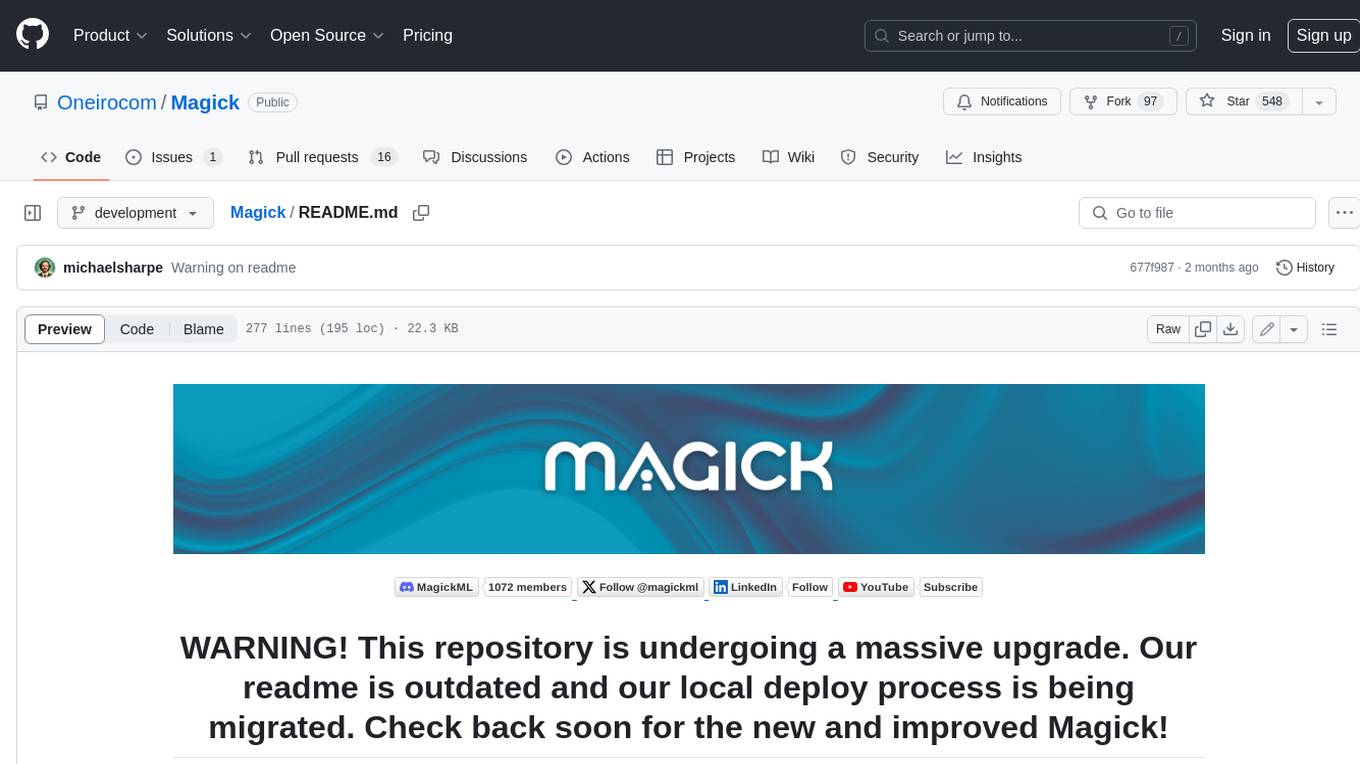
Magick
Magick is a groundbreaking visual AIDE (Artificial Intelligence Development Environment) for no-code data pipelines and multimodal agents. Magick can connect to other services and comes with nodes and templates well-suited for intelligent agents, chatbots, complex reasoning systems and realistic characters.