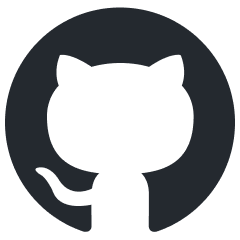
AI-LLM-ML-CS-Quant-Review
In-depth review of industry trends in AI, LLMs, Machine Learning, Computer Science, and Quantitative Finance.
Stars: 56
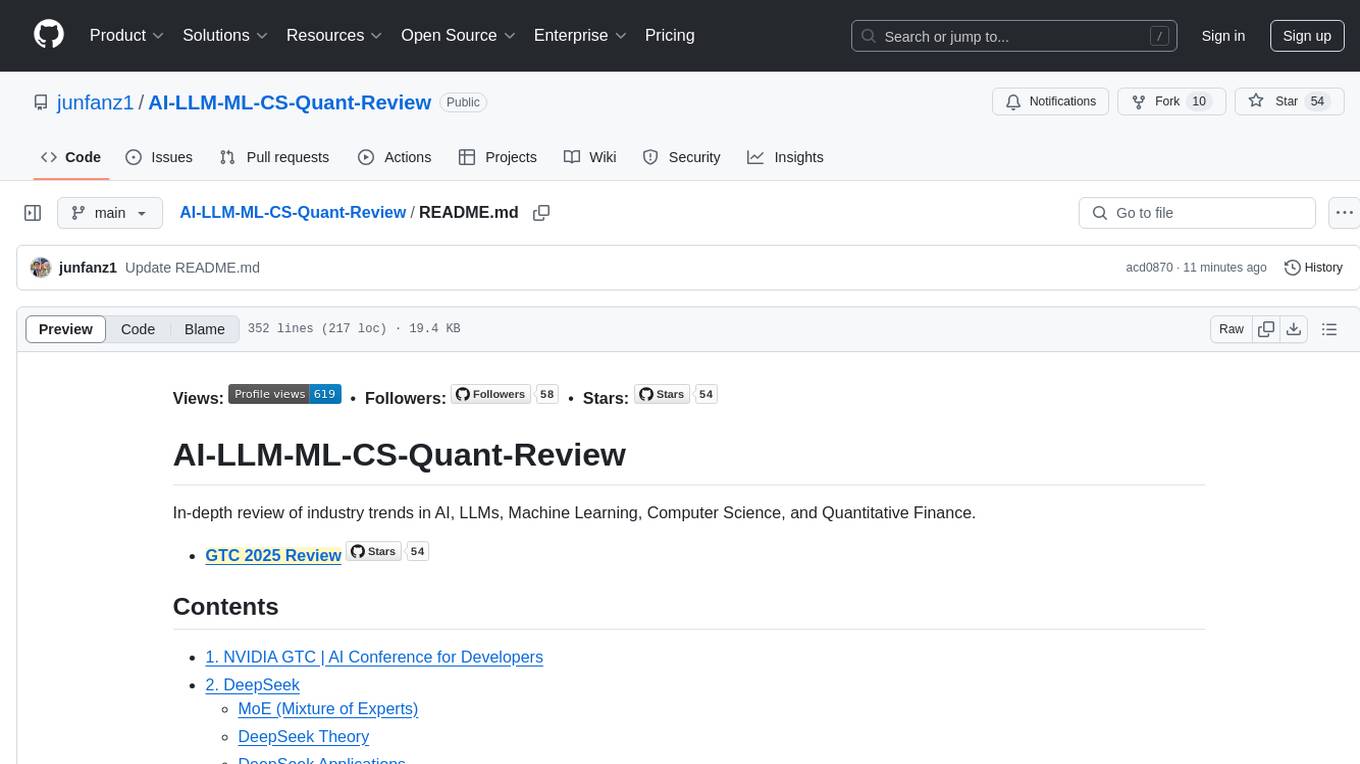
This repository provides an in-depth review of industry trends in AI, Large Language Models (LLMs), Machine Learning, Computer Science, and Quantitative Finance. It covers various topics such as NVIDIA GTC conferences, DeepSeek theory and applications, LangGraph & Cursor AI, LLM essentials, system design, computer systems, big data and AI in finance, C++ design patterns, high-frequency finance, machine learning for algorithmic trading, stochastic volatility modeling, and quant job interview questions.
README:
In-depth review of industry trends in AI, LLMs, Machine Learning, Computer Science, and Quantitative Finance.
- 1. NVIDIA GTC | AI Conference for Developers
- 2. DeepSeek Trends 2025
- 3. LangGraph & Cursor AI Projects
- 4. LLM Essentials
- 5. System Design
- 6. Computer Systems
- 7. Big Data and AI in Finance, Econometrics and Statistics Conference, UChicago 2024
- 8. C++ Design Patterns and Derivatives Pricing
- 9. High-Frequency Finance
- 10. Machine Learning for Algorithmic Trading
- 11. Stochastic Volatility Modeling
- 12. Quant Job Interview Questions
Educative: Everything You Need to Know About DeepSeek | Notes
- Ed Donner: LLM Engineering: Master AI, Large Language Models & Agents
- Eden Marco: LangChain-Develop LLM powered applications with LangChain
- Eden Marco: LangGraph-Develop LLM powered AI agents with LangGraph
- Eden Marco: Cursor Course: FullStack development with Cursor AI Copilot
GitHub Projects
- Code-Interpreter-ReAct-LangChain-Agent
- LLM-Documentation-Chatbot
- Cognito-LangGraph-RAG
- LangGraph-Reflection-Researcher
- Cursor-FullStack-AI-App
Foundations of LLMs - by Yuren Mao, Zhejiang University, 2024
Educative: Advanced RAG Techniques - Choosing the Right Approach | Notes
Educative: Build AI Agents and Multi-Agent Systems with CrewAI | Notes
System Design Interview, An Insider's Guide, Second Edition - by Alex Xu, 2020
Generative AI System Design Interview - by Ali Aminian, Hao Sheng, 2024
Machine Learning System Design Interview - by Ali Aminian, Alex Xu, 2023
Educative - Grokking System Design Interview | PDF Notes | Markdown Notes
Educative - Grokking the Modern System Design Interview | Markdown Notes
计算机底层的秘密,陆小风 - 2023,电子工业出版社
BDAI Conference, 2024 Oct 3-5, UChicago
Abstract PDF | Agenda PDF | High Level Overview Notes PDF | Conference Review Notes PDF
C++ Design Patterns and Derivatives Pricing (Mathematics, Finance and Risk, Series Number 2) 2nd Edition, by M. S. Joshi
An Introduction to High-Frequency Finance, by Ramazan Gençay, et al.
Machine Learning for Algorithmic Trading: Predictive models to extract signals from market and alternative data for systematic trading strategies with Python, 2nd Edition Paperback – by Stefan Jansen 2020
Stochastic Volatility Modeling (Chapman and Hall/CRC Financial Mathematics Series) 1st Edition, by Lorenzo Bergomi
Book Link | PDF Char 1 Intro | Markdown Char 1 Intro | PDF Char 2 Local Vol | Markdown Char 2 Local Vol
Quant Job Interview Questions and Answers (Second Edition) – by Mark Joshi 2013
For Tasks:
Click tags to check more tools for each tasksFor Jobs:
Alternative AI tools for AI-LLM-ML-CS-Quant-Review
Similar Open Source Tools
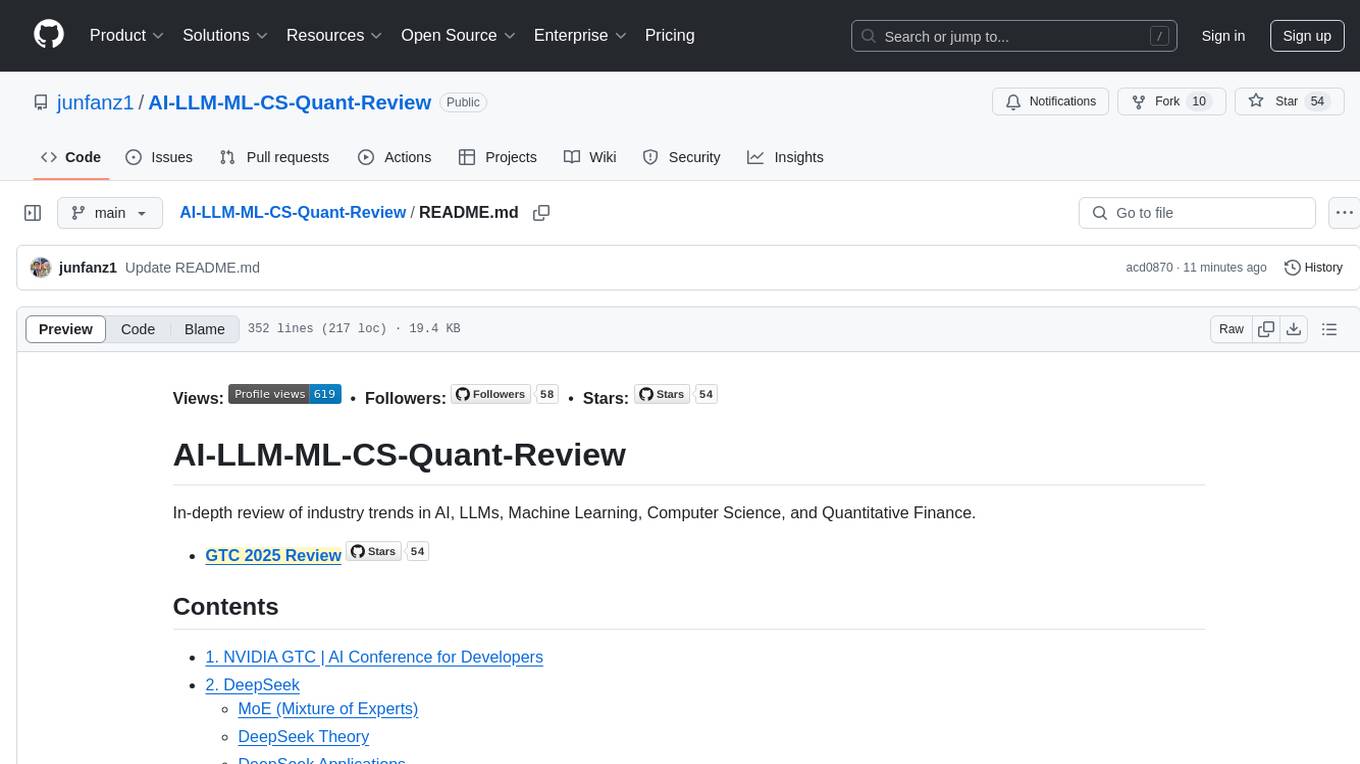
AI-LLM-ML-CS-Quant-Review
This repository provides an in-depth review of industry trends in AI, Large Language Models (LLMs), Machine Learning, Computer Science, and Quantitative Finance. It covers various topics such as NVIDIA GTC conferences, DeepSeek theory and applications, LangGraph & Cursor AI, LLM essentials, system design, computer systems, big data and AI in finance, C++ design patterns, high-frequency finance, machine learning for algorithmic trading, stochastic volatility modeling, and quant job interview questions.
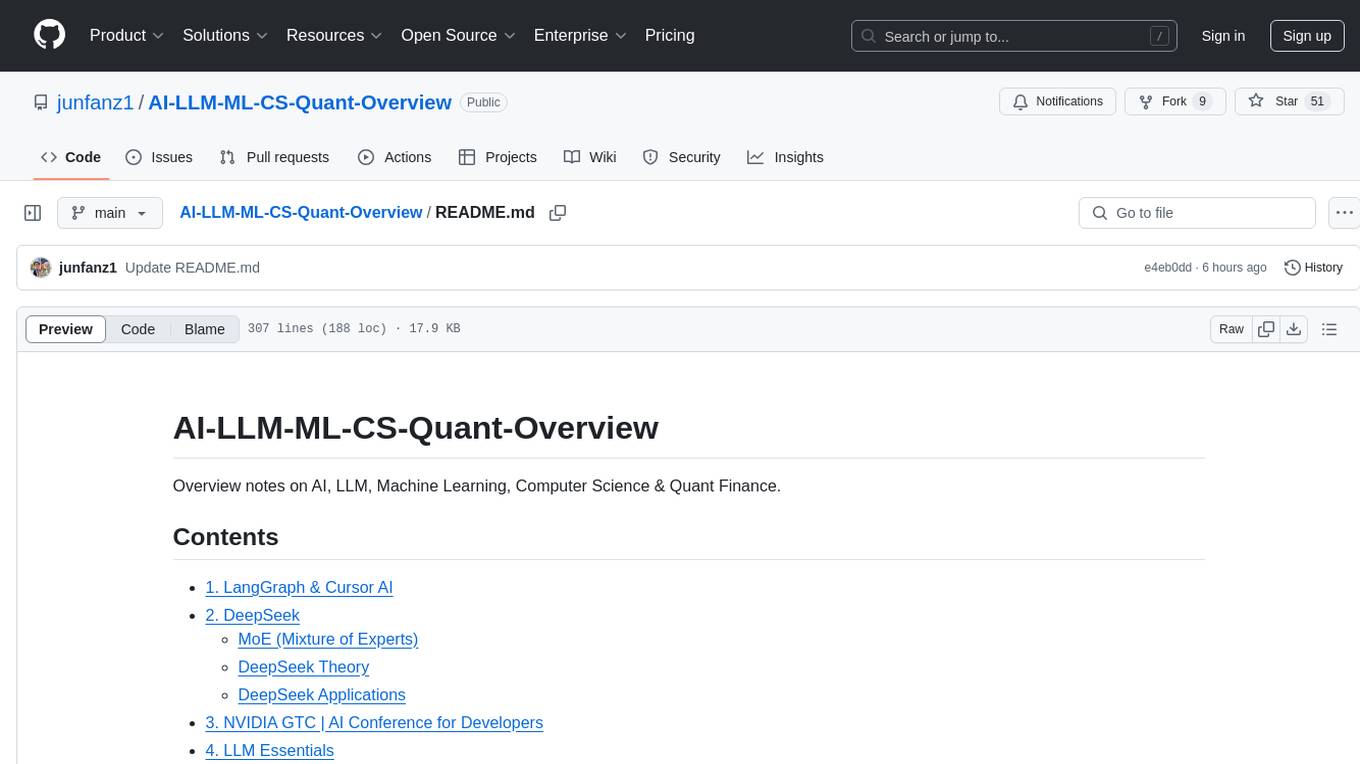
AI-LLM-ML-CS-Quant-Overview
AI-LLM-ML-CS-Quant-Overview is a repository providing overview notes on AI, Large Language Models (LLM), Machine Learning (ML), Computer Science (CS), and Quantitative Finance. It covers various topics such as LangGraph & Cursor AI, DeepSeek, MoE (Mixture of Experts), NVIDIA GTC, LLM Essentials, System Design, Computer Systems, Big Data and AI in Finance, Econometrics and Statistics Conference, C++ Design Patterns and Derivatives Pricing, High-Frequency Finance, Machine Learning for Algorithmic Trading, Stochastic Volatility Modeling, Quant Job Interview Questions, Distributed Systems, Language Models, Designing Machine Learning Systems, Designing Data-Intensive Applications (DDIA), Distributed Machine Learning, and The Elements of Quantitative Investing.
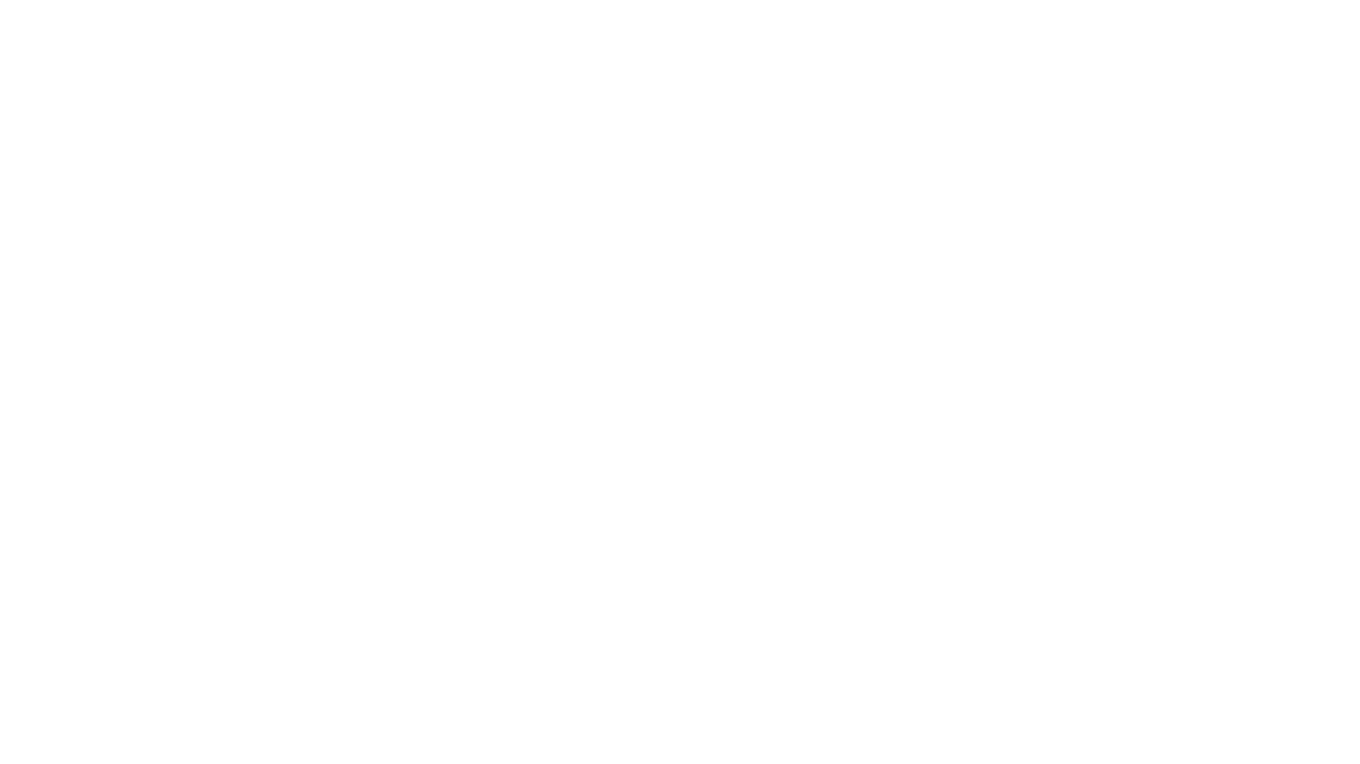
AI-LLM-ML-CS-Quant-Readings
AI-LLM-ML-CS-Quant-Readings is a repository dedicated to taking notes on Artificial Intelligence, Large Language Models, Machine Learning, Computer Science, and Quantitative Finance. It contains a wide range of resources, including theory, applications, conferences, essentials, foundations, system design, computer systems, finance, and job interview questions. The repository covers topics such as AI systems, multi-agent systems, deep learning theory and applications, system design interviews, C++ design patterns, high-frequency finance, algorithmic trading, stochastic volatility modeling, and quantitative investing. It is a comprehensive collection of materials for individuals interested in these fields.
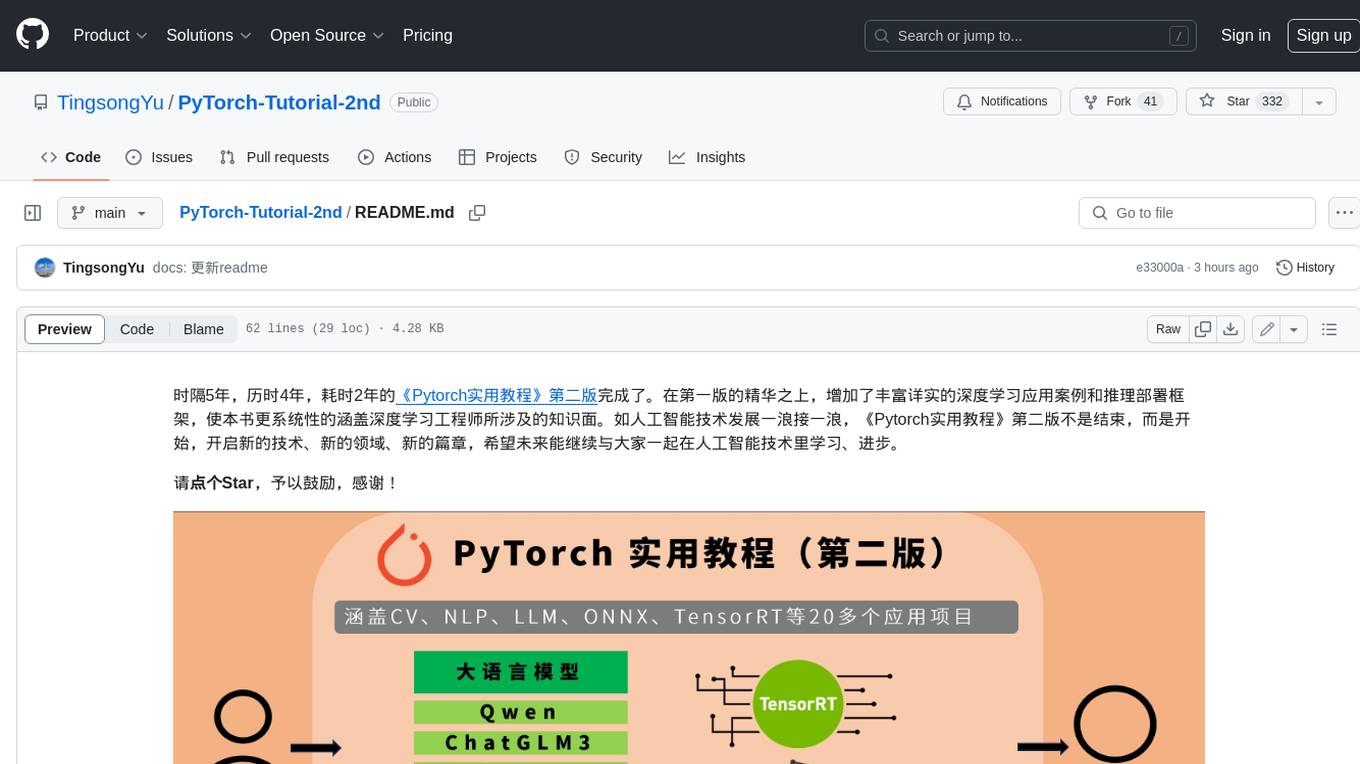
PyTorch-Tutorial-2nd
The second edition of "PyTorch Practical Tutorial" was completed after 5 years, 4 years, and 2 years. On the basis of the essence of the first edition, rich and detailed deep learning application cases and reasoning deployment frameworks have been added, so that this book can more systematically cover the knowledge involved in deep learning engineers. As the development of artificial intelligence technology continues to emerge, the second edition of "PyTorch Practical Tutorial" is not the end, but the beginning, opening up new technologies, new fields, and new chapters. I hope to continue learning and making progress in artificial intelligence technology with you in the future.
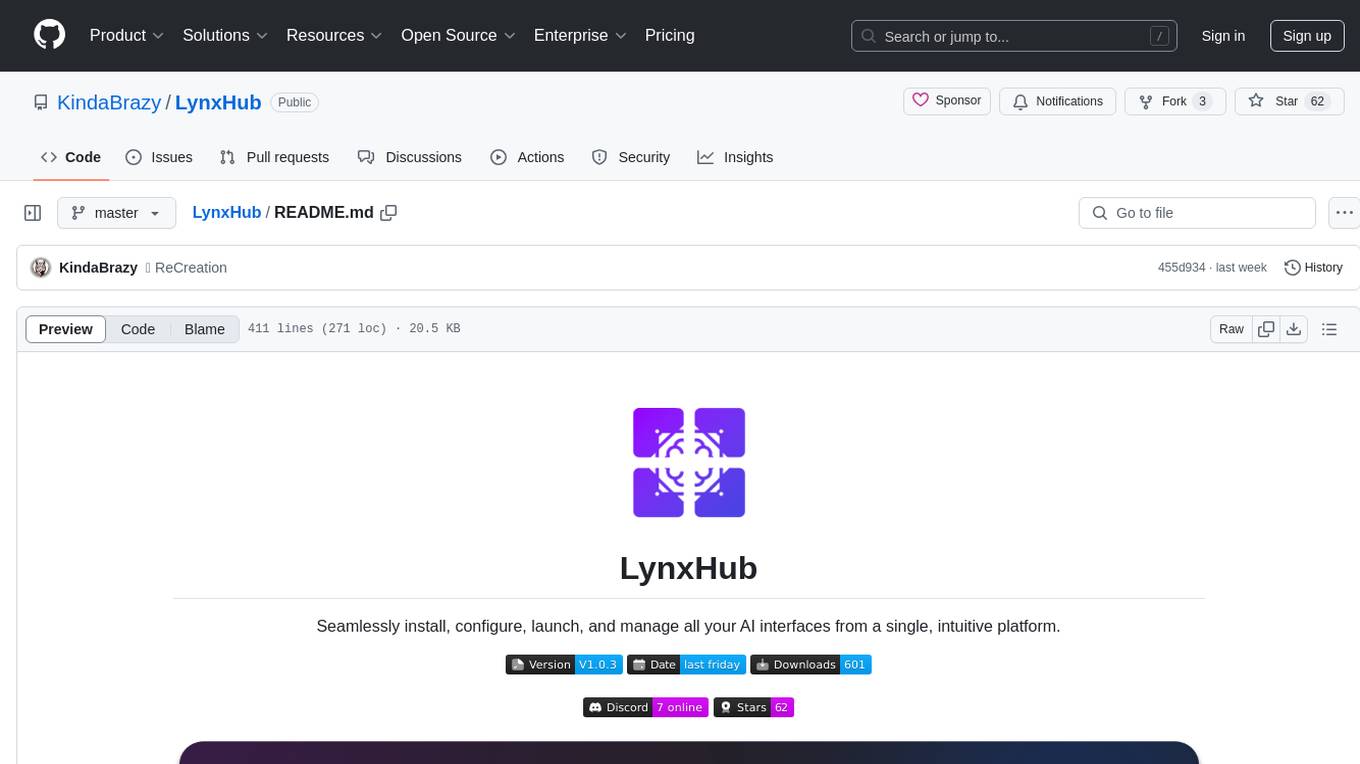
LynxHub
LynxHub is a platform that allows users to seamlessly install, configure, launch, and manage all their AI interfaces from a single, intuitive dashboard. It offers features like AI interface management, arguments manager, custom run commands, pre-launch actions, extension management, in-app tools like terminal and web browser, AI information dashboard, Discord integration, and additional features like theme options and favorite interface pinning. The platform supports modular design for custom AI modules and upcoming extensions system for complete customization. LynxHub aims to streamline AI workflow and enhance user experience with a user-friendly interface and comprehensive functionalities.
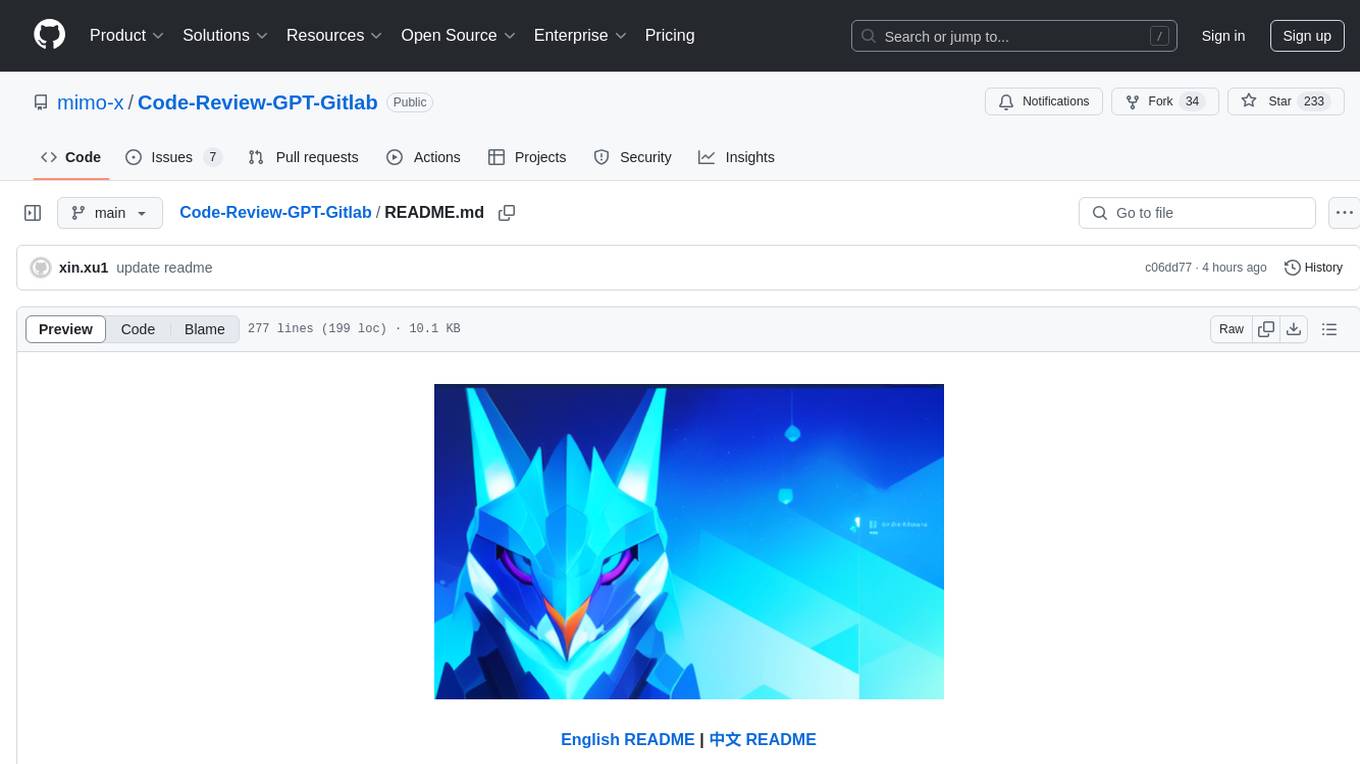
Code-Review-GPT-Gitlab
A project that utilizes large models to help with Code Review on Gitlab, aimed at improving development efficiency. The project is customized for Gitlab and is developing a Multi-Agent plugin for collaborative review. It integrates various large models for code security issues and stays updated with the latest Code Review trends. The project architecture is designed to be powerful, flexible, and efficient, with easy integration of different models and high customization for developers.
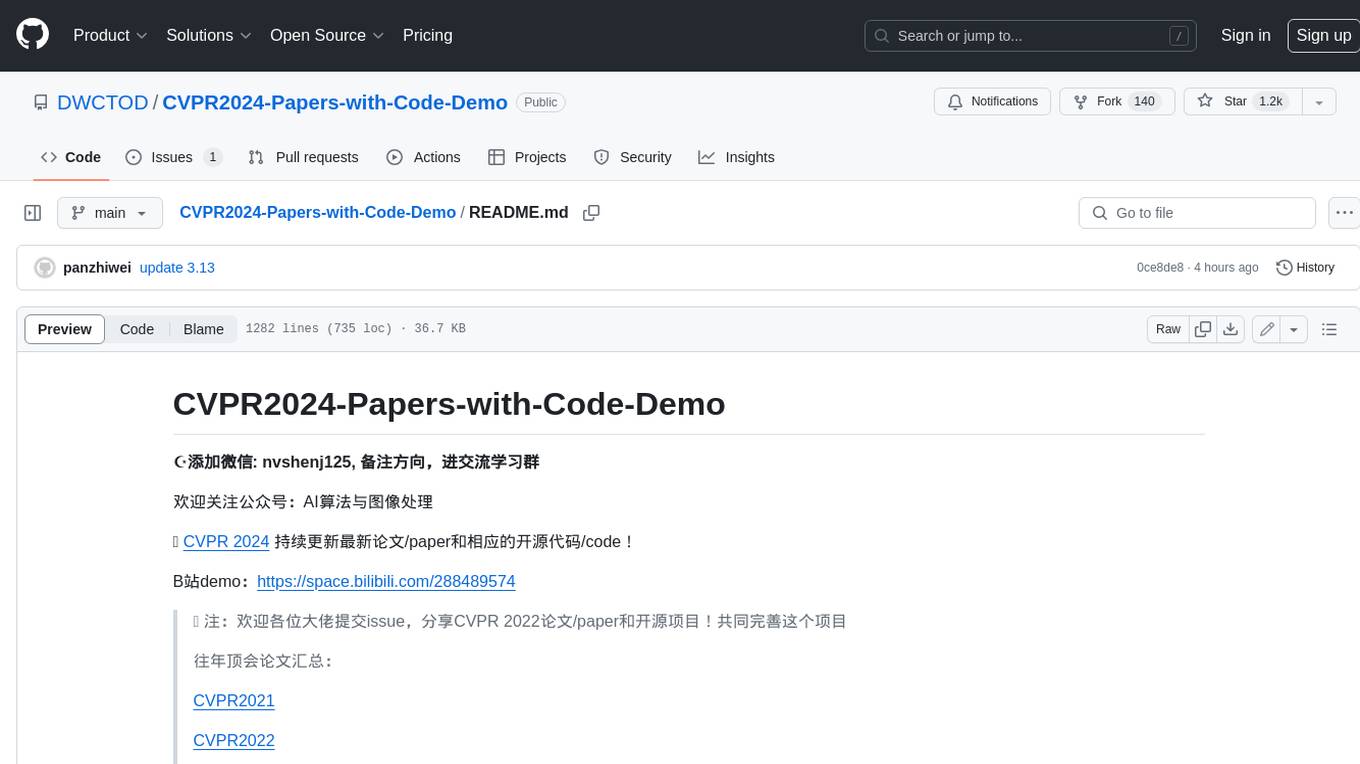
CVPR2024-Papers-with-Code-Demo
This repository contains a collection of papers and code for the CVPR 2024 conference. The papers cover a wide range of topics in computer vision, including object detection, image segmentation, image generation, and video analysis. The code provides implementations of the algorithms described in the papers, making it easy for researchers and practitioners to reproduce the results and build upon the work of others. The repository is maintained by a team of researchers at the University of California, Berkeley.
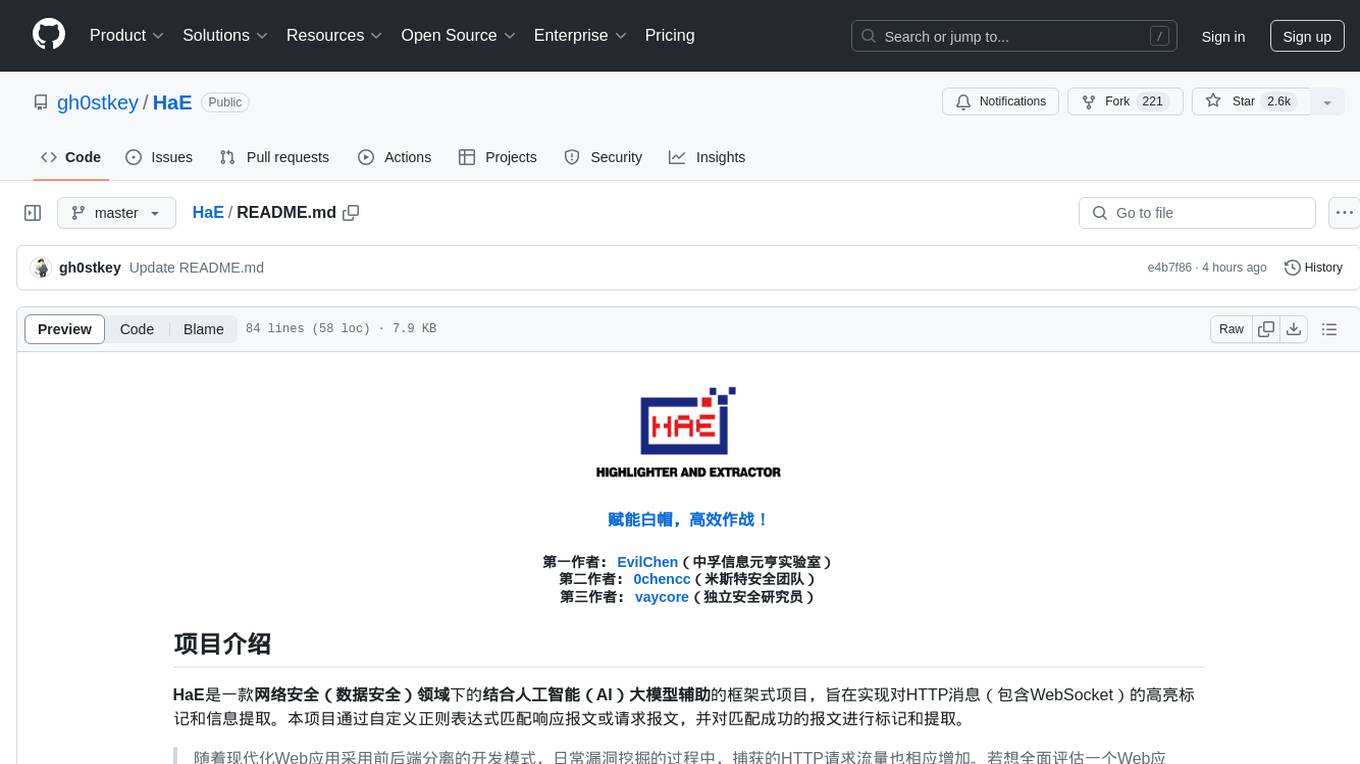
HaE
HaE is a framework project in the field of network security (data security) that combines artificial intelligence (AI) large models to achieve highlighting and information extraction of HTTP messages (including WebSocket). It aims to reduce testing time, focus on valuable and meaningful messages, and improve vulnerability discovery efficiency. The project provides a clear and visual interface design, simple interface interaction, and centralized data panel for querying and extracting information. It also features built-in color upgrade algorithm, one-click export/import of data, and integration of AI large models API for optimized data processing.
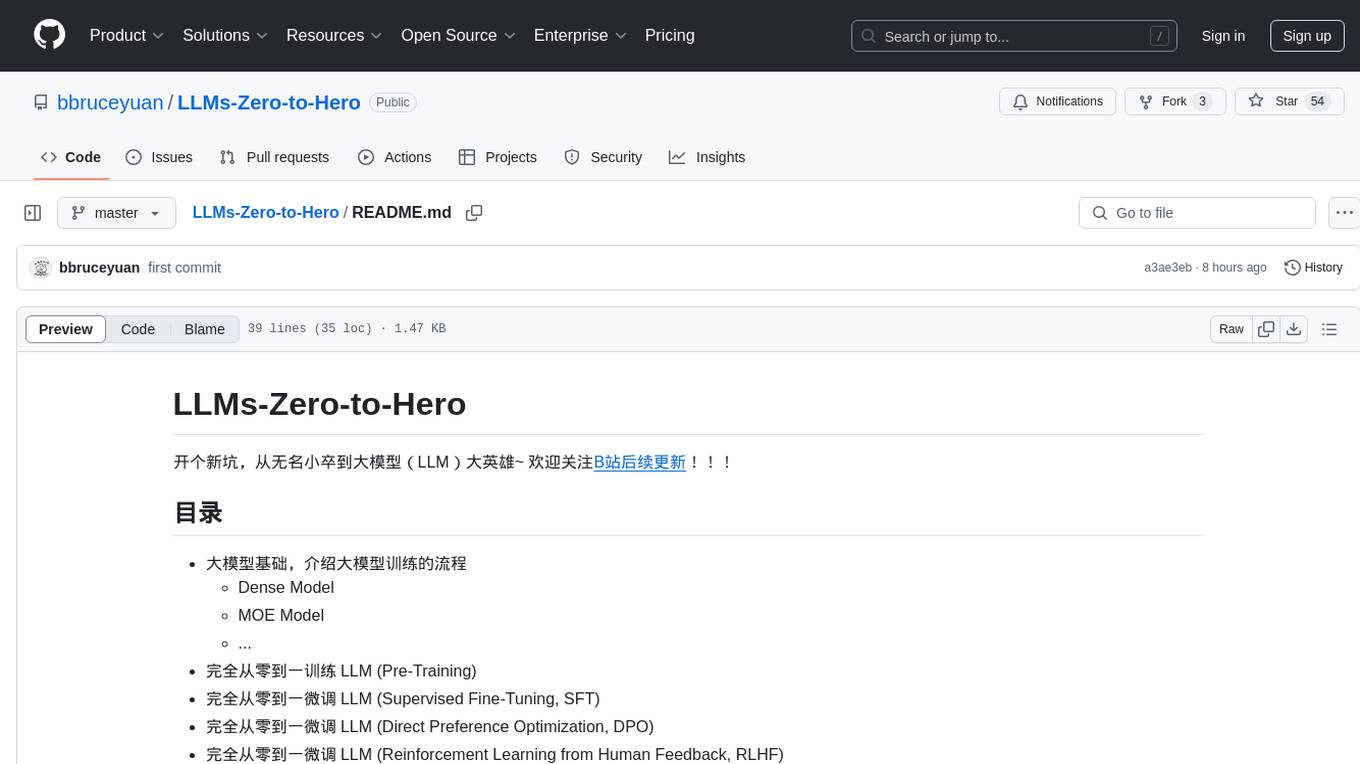
LLMs-Zero-to-Hero
LLMs-Zero-to-Hero is a repository dedicated to training large language models (LLMs) from scratch, covering topics such as dense models, MOE models, pre-training, supervised fine-tuning, direct preference optimization, reinforcement learning from human feedback, and deploying large models. The repository provides detailed learning notes for different chapters, code implementations, and resources for training and deploying LLMs. It aims to guide users from being beginners to proficient in building and deploying large language models.
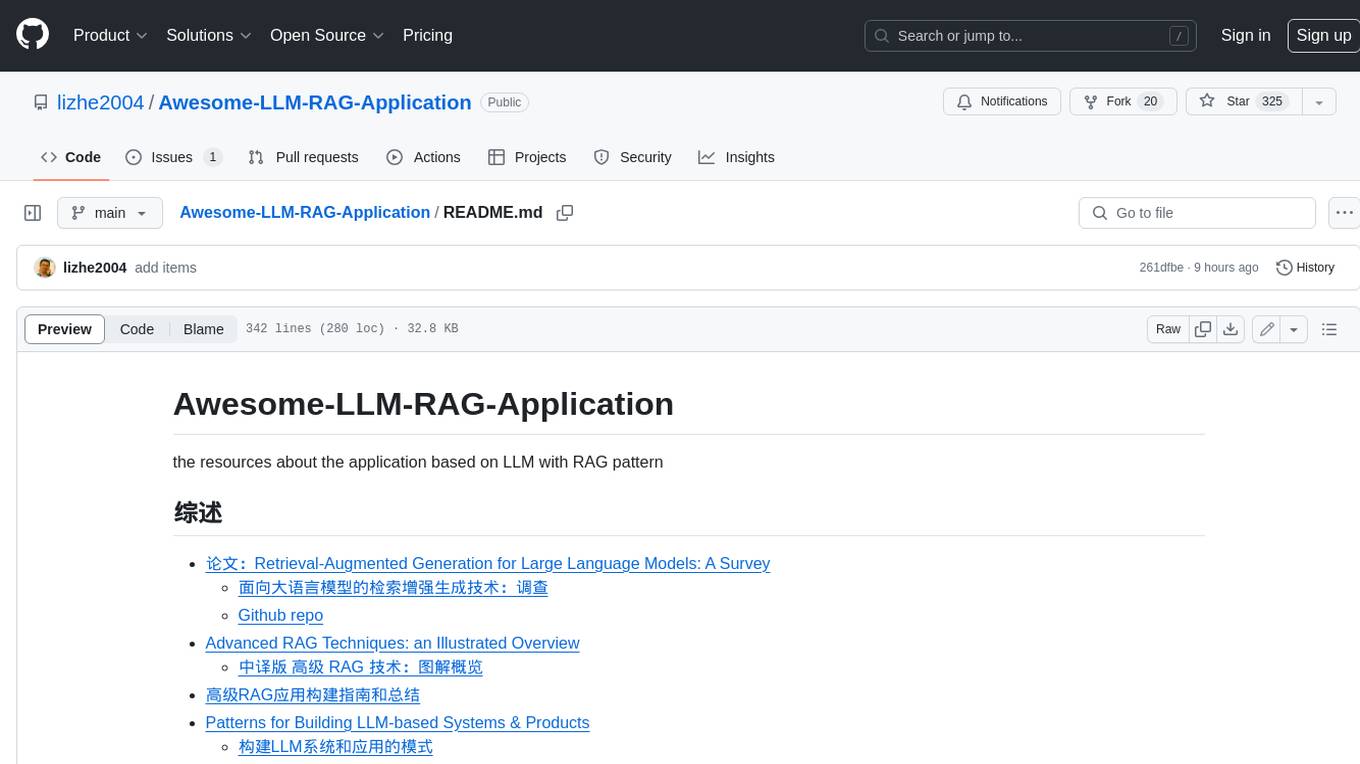
Awesome-LLM-RAG-Application
Awesome-LLM-RAG-Application is a repository that provides resources and information about applications based on Large Language Models (LLM) with Retrieval-Augmented Generation (RAG) pattern. It includes a survey paper, GitHub repo, and guides on advanced RAG techniques. The repository covers various aspects of RAG, including academic papers, evaluation benchmarks, downstream tasks, tools, and technologies. It also explores different frameworks, preprocessing tools, routing mechanisms, evaluation frameworks, embeddings, security guardrails, prompting tools, SQL enhancements, LLM deployment, observability tools, and more. The repository aims to offer comprehensive knowledge on RAG for readers interested in exploring and implementing LLM-based systems and products.
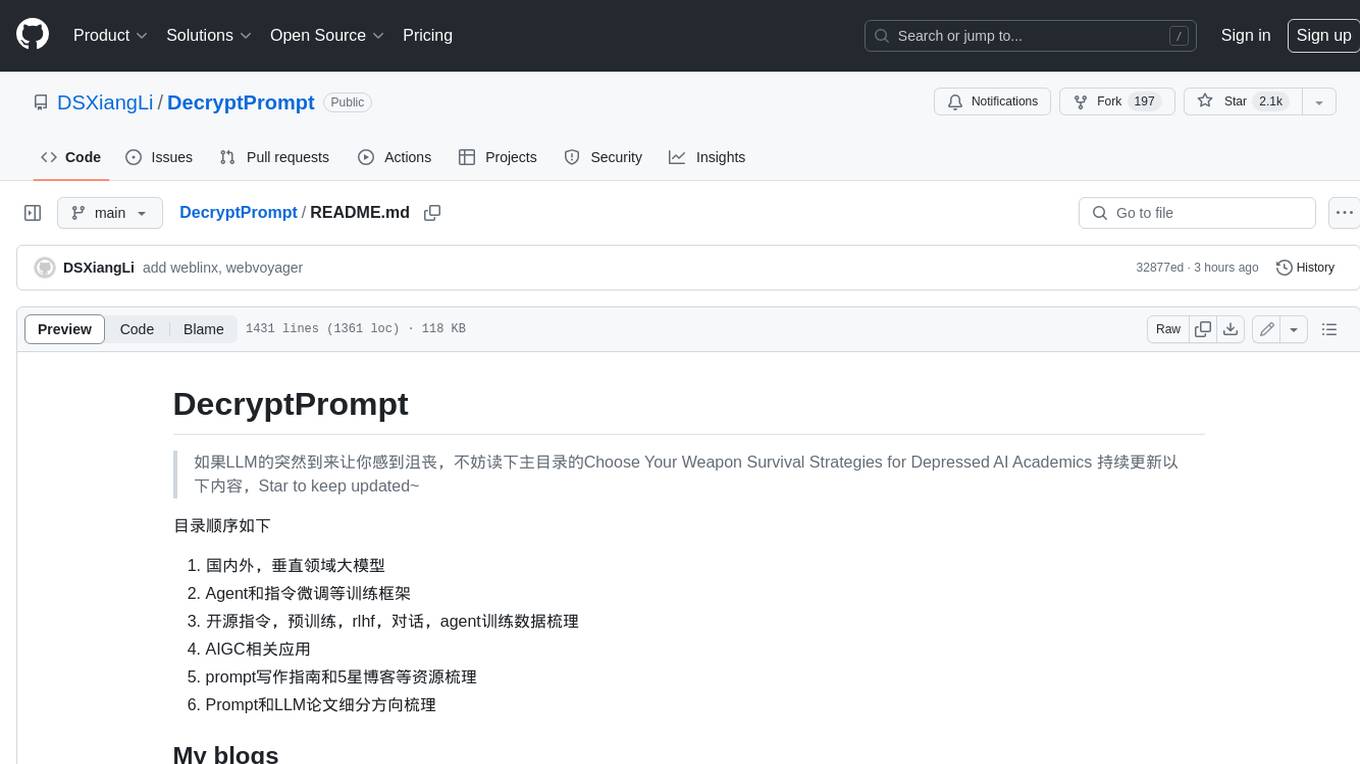
DecryptPrompt
This repository does not provide a tool, but rather a collection of resources and strategies for academics in the field of artificial intelligence who are feeling depressed or overwhelmed by the rapid advancements in the field. The resources include articles, blog posts, and other materials that offer advice on how to cope with the challenges of working in a fast-paced and competitive environment.
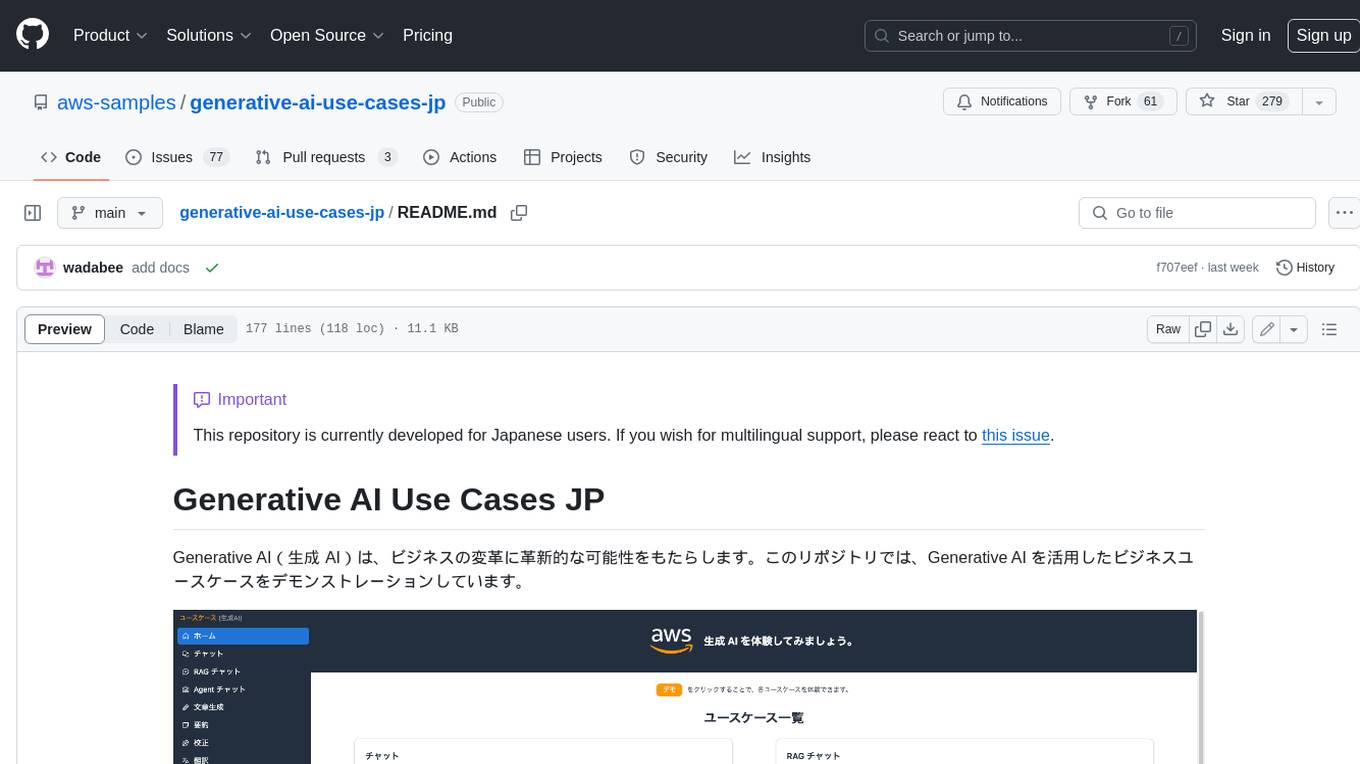
generative-ai-use-cases-jp
Generative AI (生成 AI) brings revolutionary potential to transform businesses. This repository demonstrates business use cases leveraging Generative AI.
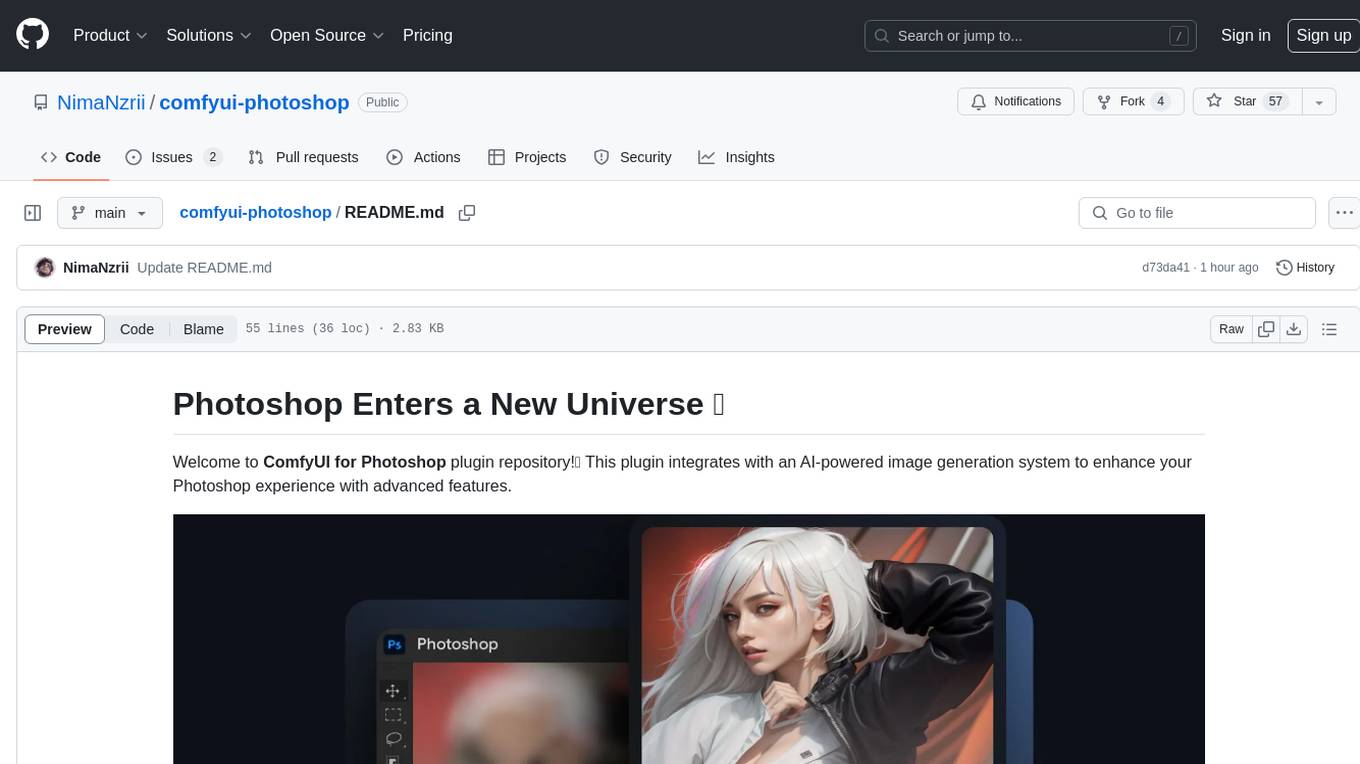
comfyui-photoshop
ComfyUI for Photoshop is a plugin that integrates with an AI-powered image generation system to enhance the Photoshop experience with features like unlimited generative fill, customizable back-end, AI-powered artistry, and one-click transformation. The plugin requires a minimum of 6GB graphics memory and 12GB RAM. Users can install the plugin and set up the ComfyUI workflow using provided links and files. Additionally, specific files like Check points, Loras, and Detailer Lora are required for different functionalities. Support and contributions are encouraged through GitHub.
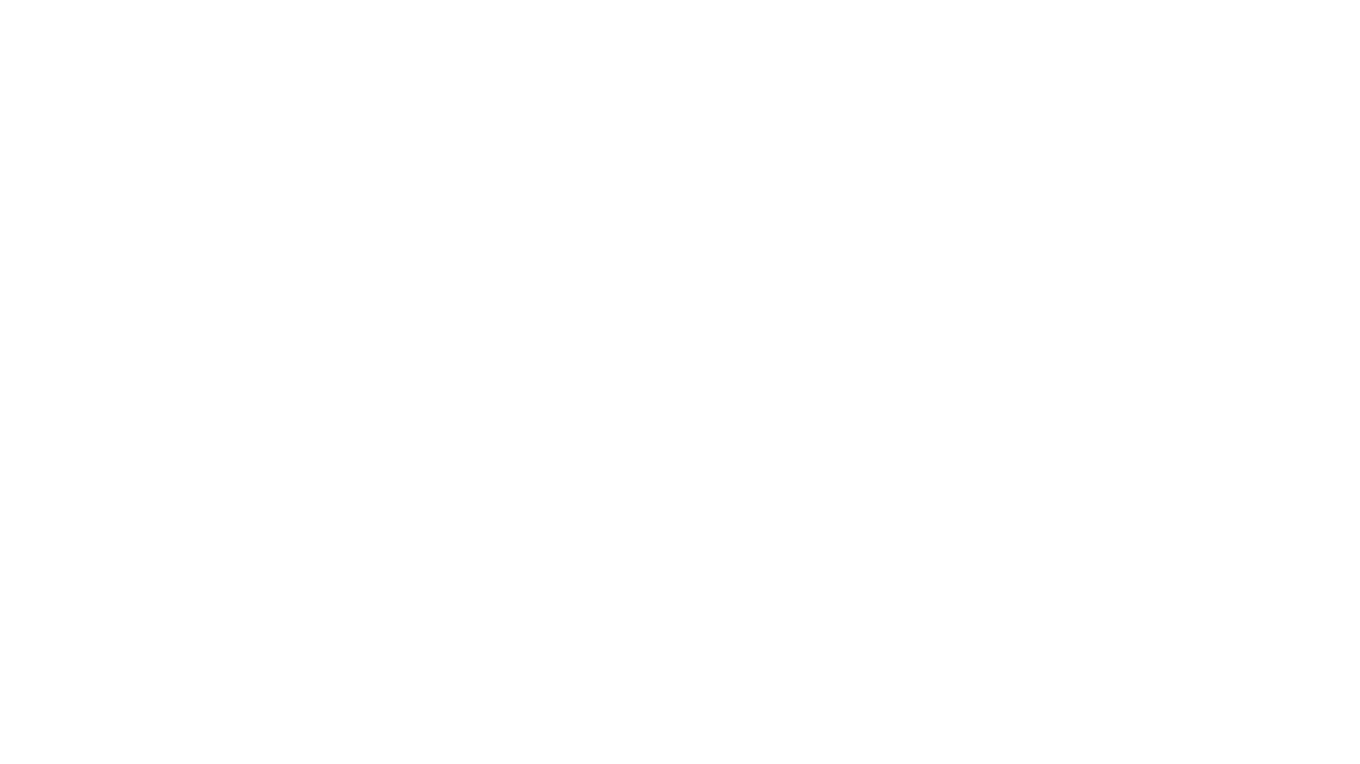
Airports
Airports is a personal airport/subscription summary repository that provides information on various airport services, including high-end and cost-effective options. Users can find links to different airport websites and subscription services, along with recommendations for reliable and affordable airport options. The repository also includes public service pages for automatic data retrieval and Telegram channels related to airport sharing and discussions. Additionally, users can access subscription services for v2ray and clash links through the repository.
For similar tasks
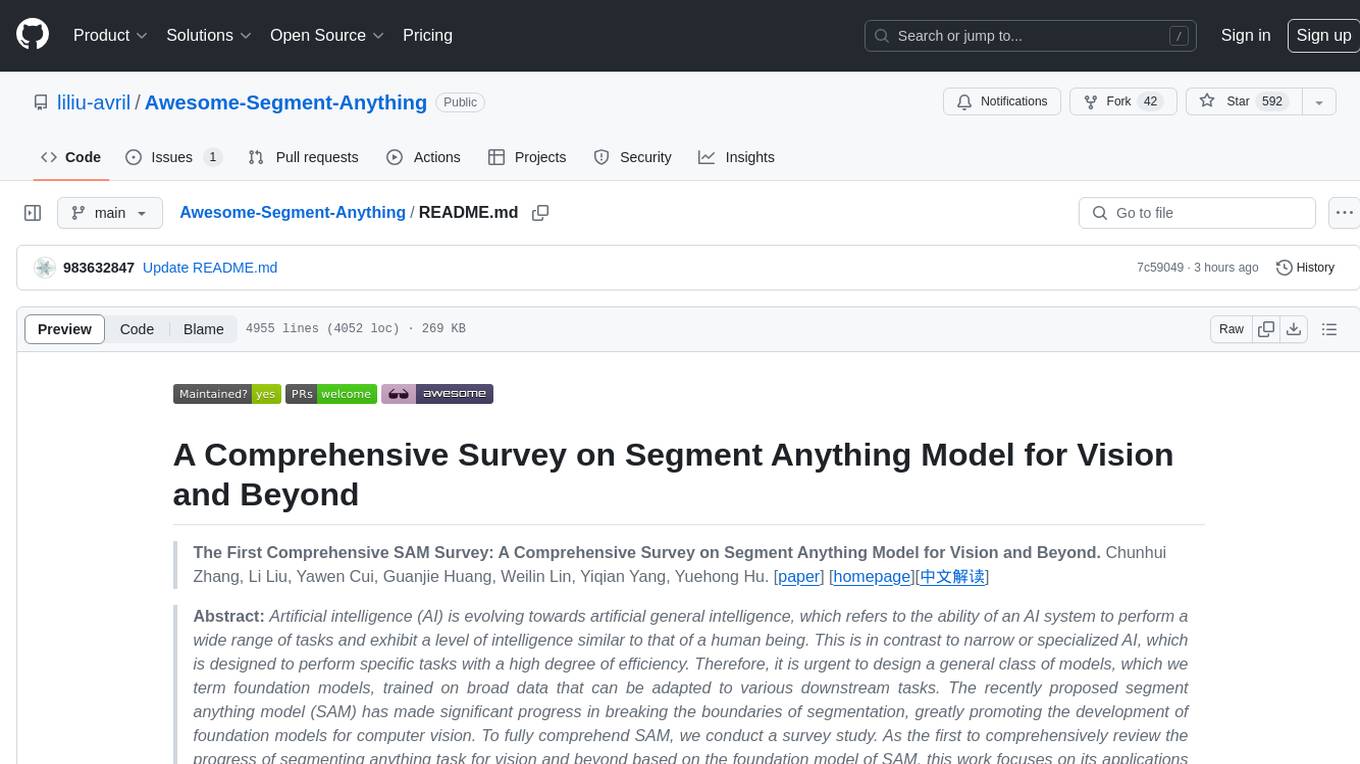
Awesome-Segment-Anything
Awesome-Segment-Anything is a powerful tool for segmenting and extracting information from various types of data. It provides a user-friendly interface to easily define segmentation rules and apply them to text, images, and other data formats. The tool supports both supervised and unsupervised segmentation methods, allowing users to customize the segmentation process based on their specific needs. With its versatile functionality and intuitive design, Awesome-Segment-Anything is ideal for data analysts, researchers, content creators, and anyone looking to efficiently extract valuable insights from complex datasets.
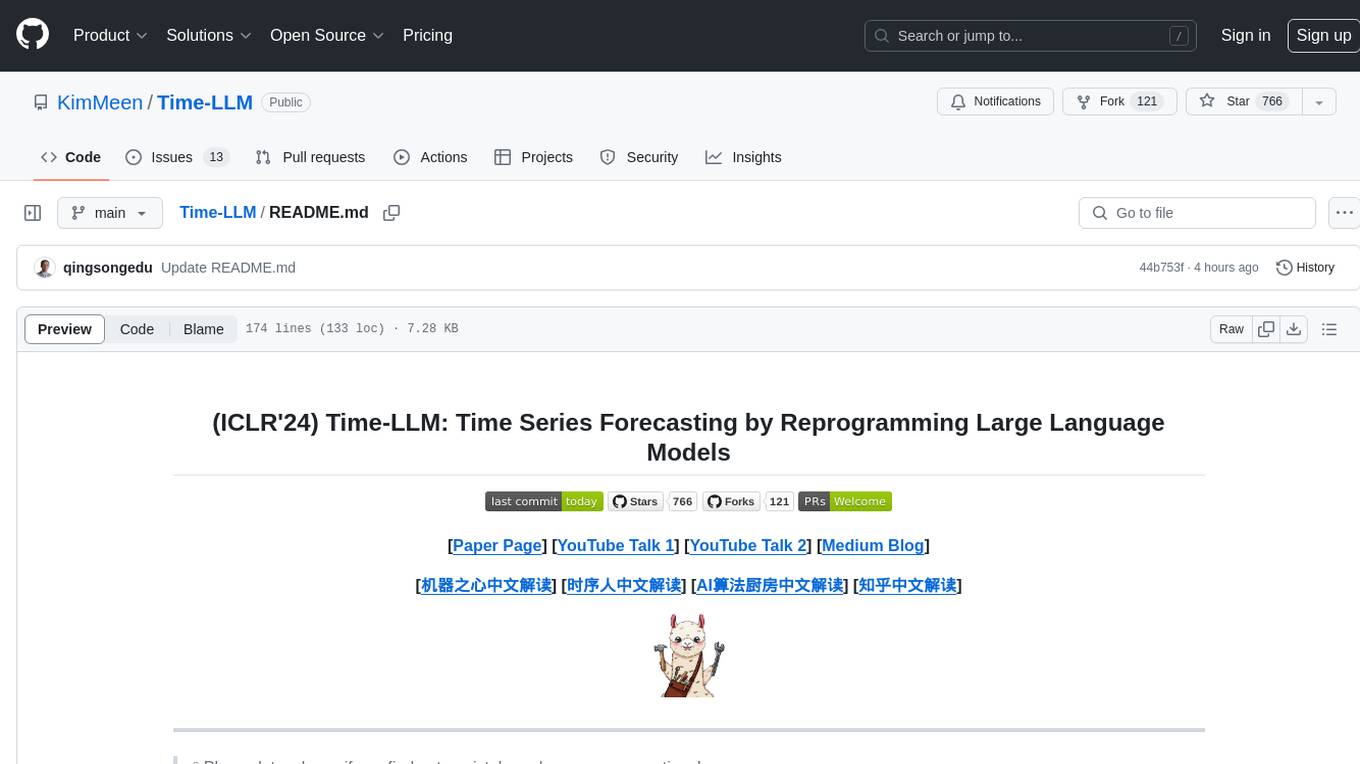
Time-LLM
Time-LLM is a reprogramming framework that repurposes large language models (LLMs) for time series forecasting. It allows users to treat time series analysis as a 'language task' and effectively leverage pre-trained LLMs for forecasting. The framework involves reprogramming time series data into text representations and providing declarative prompts to guide the LLM reasoning process. Time-LLM supports various backbone models such as Llama-7B, GPT-2, and BERT, offering flexibility in model selection. The tool provides a general framework for repurposing language models for time series forecasting tasks.
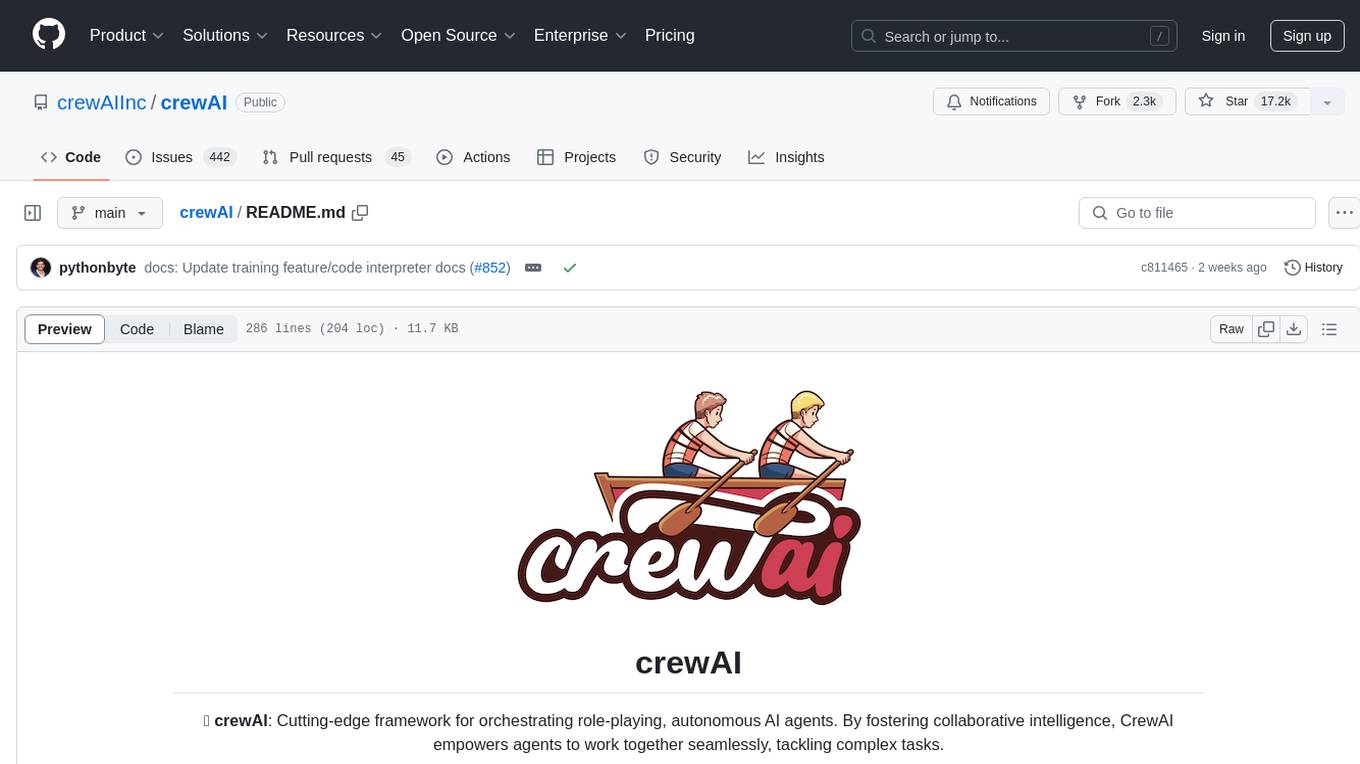
crewAI
CrewAI is a cutting-edge framework designed to orchestrate role-playing autonomous AI agents. By fostering collaborative intelligence, CrewAI empowers agents to work together seamlessly, tackling complex tasks. It enables AI agents to assume roles, share goals, and operate in a cohesive unit, much like a well-oiled crew. Whether you're building a smart assistant platform, an automated customer service ensemble, or a multi-agent research team, CrewAI provides the backbone for sophisticated multi-agent interactions. With features like role-based agent design, autonomous inter-agent delegation, flexible task management, and support for various LLMs, CrewAI offers a dynamic and adaptable solution for both development and production workflows.
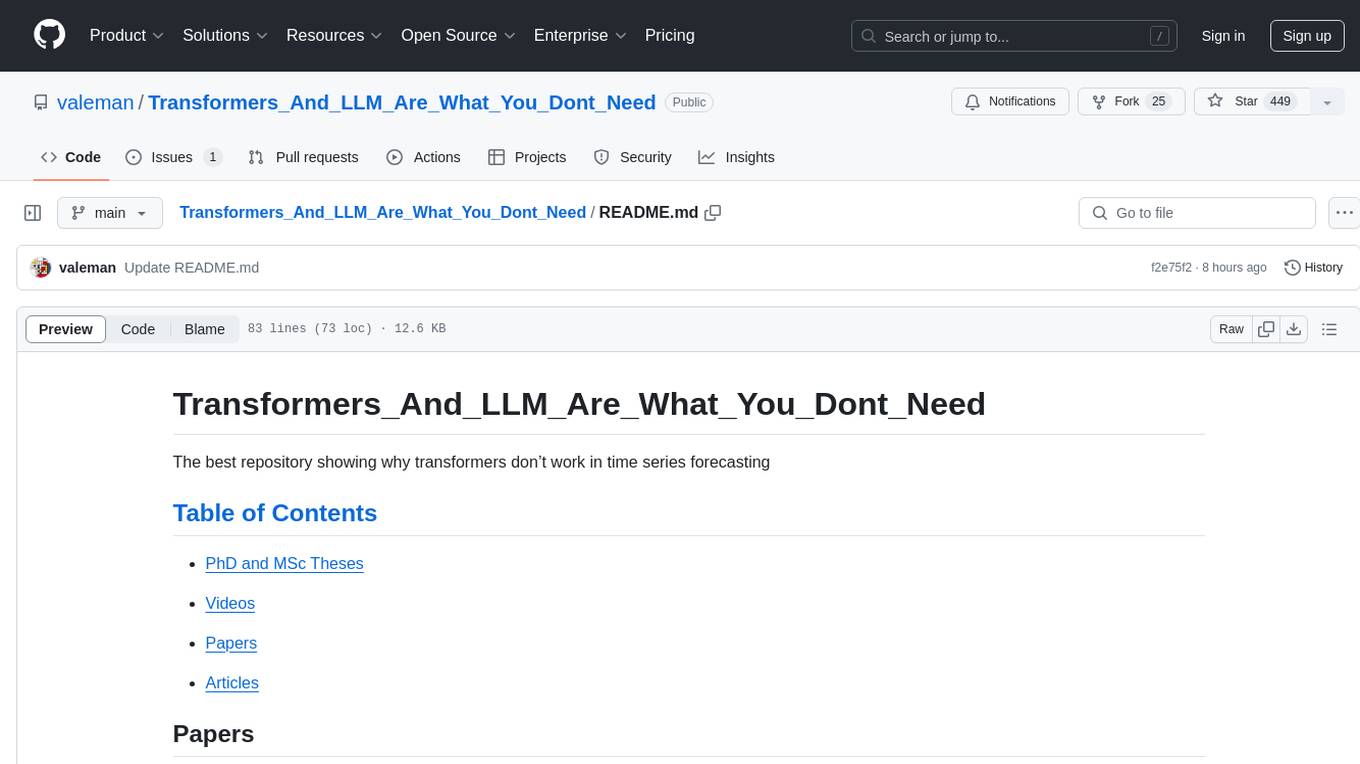
Transformers_And_LLM_Are_What_You_Dont_Need
Transformers_And_LLM_Are_What_You_Dont_Need is a repository that explores the limitations of transformers in time series forecasting. It contains a collection of papers, articles, and theses discussing the effectiveness of transformers and LLMs in this domain. The repository aims to provide insights into why transformers may not be the best choice for time series forecasting tasks.
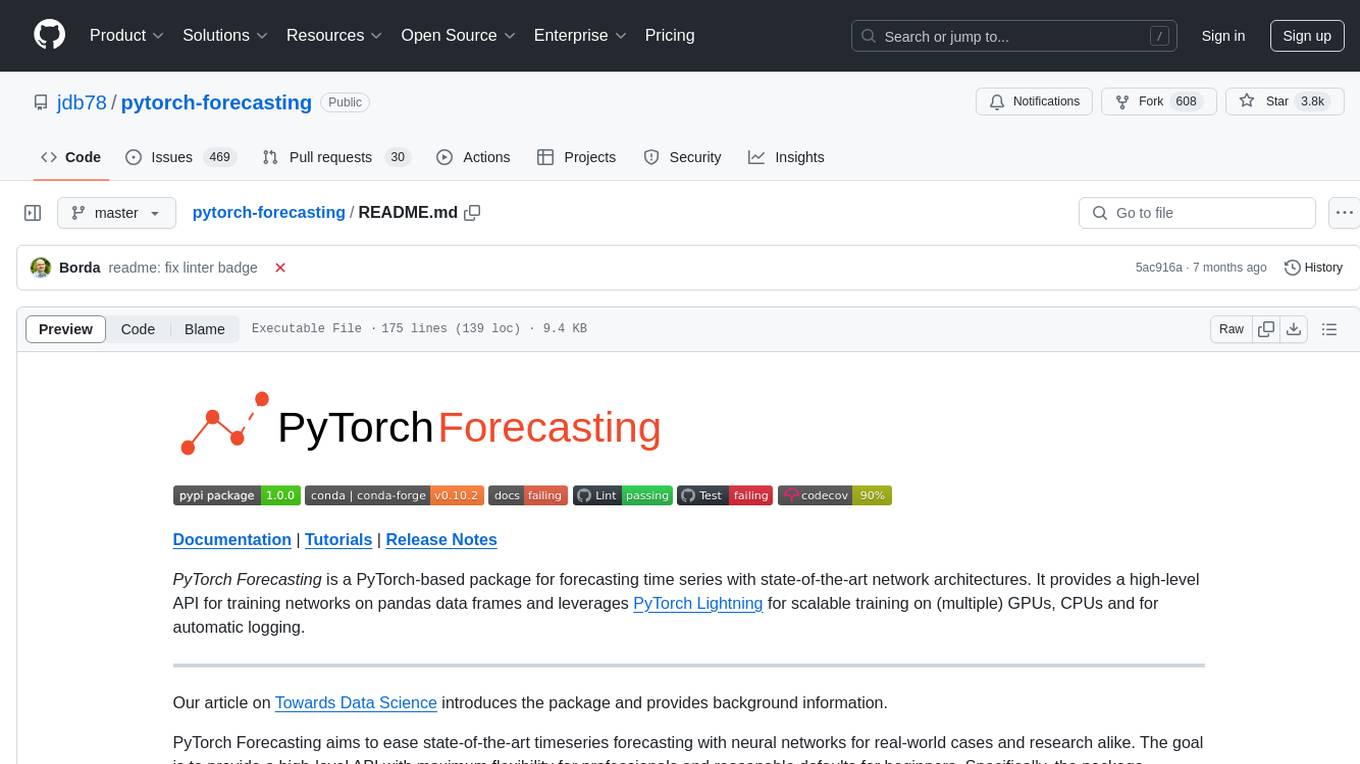
pytorch-forecasting
PyTorch Forecasting is a PyTorch-based package for time series forecasting with state-of-the-art network architectures. It offers a high-level API for training networks on pandas data frames and utilizes PyTorch Lightning for scalable training on GPUs and CPUs. The package aims to simplify time series forecasting with neural networks by providing a flexible API for professionals and default settings for beginners. It includes a timeseries dataset class, base model class, multiple neural network architectures, multi-horizon timeseries metrics, and hyperparameter tuning with optuna. PyTorch Forecasting is built on pytorch-lightning for easy training on various hardware configurations.
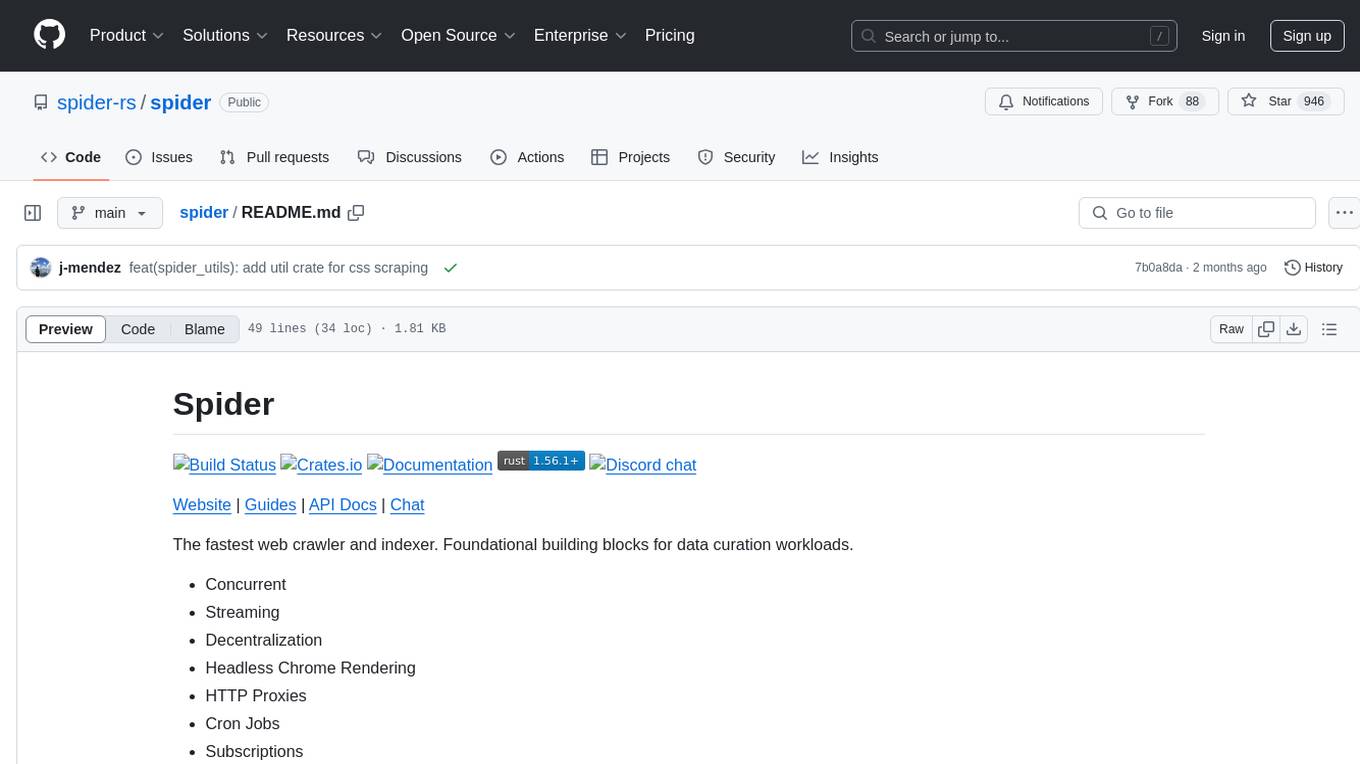
spider
Spider is a high-performance web crawler and indexer designed to handle data curation workloads efficiently. It offers features such as concurrency, streaming, decentralization, headless Chrome rendering, HTTP proxies, cron jobs, subscriptions, smart mode, blacklisting, whitelisting, budgeting depth, dynamic AI prompt scripting, CSS scraping, and more. Users can easily get started with the Spider Cloud hosted service or set up local installations with spider-cli. The tool supports integration with Node.js and Python for additional flexibility. With a focus on speed and scalability, Spider is ideal for extracting and organizing data from the web.
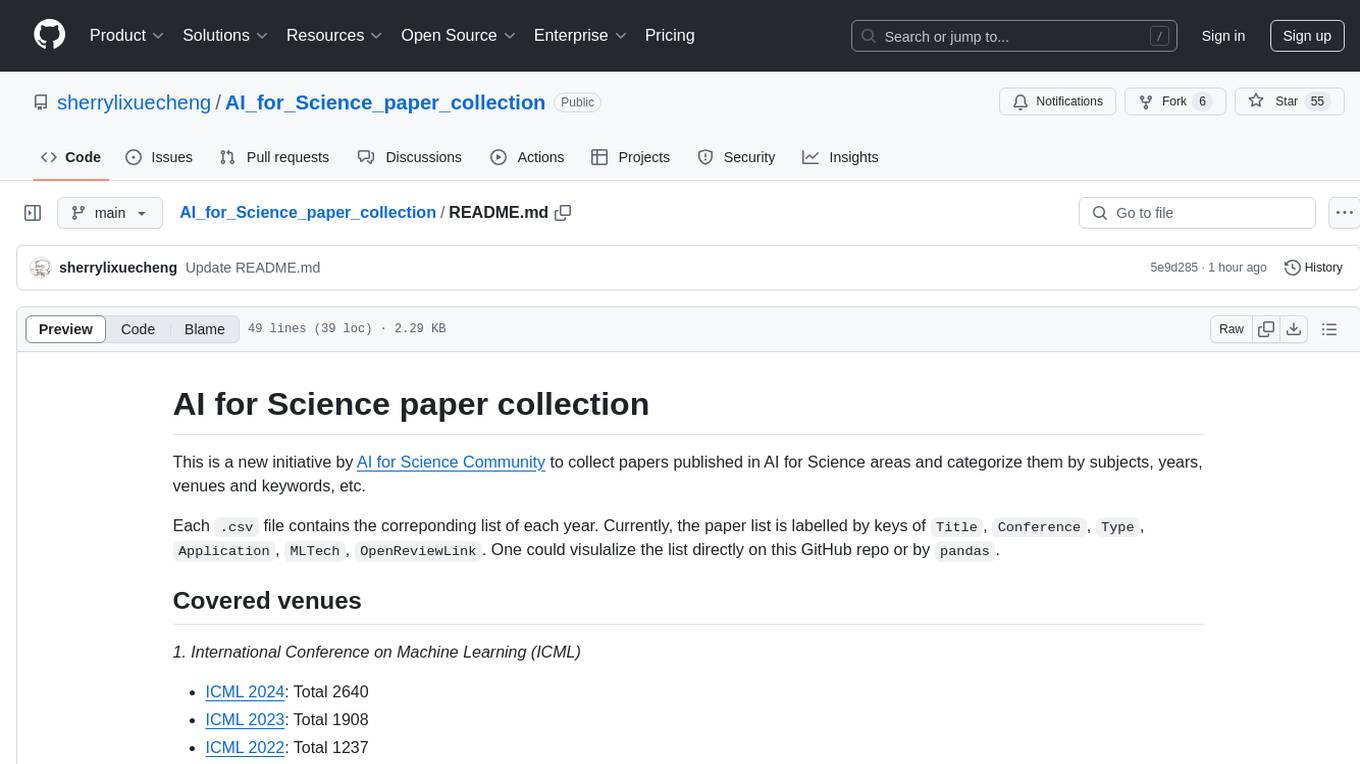
AI_for_Science_paper_collection
AI for Science paper collection is an initiative by AI for Science Community to collect and categorize papers in AI for Science areas by subjects, years, venues, and keywords. The repository contains `.csv` files with paper lists labeled by keys such as `Title`, `Conference`, `Type`, `Application`, `MLTech`, `OpenReviewLink`. It covers top conferences like ICML, NeurIPS, and ICLR. Volunteers can contribute by updating existing `.csv` files or adding new ones for uncovered conferences/years. The initiative aims to track the increasing trend of AI for Science papers and analyze trends in different applications.
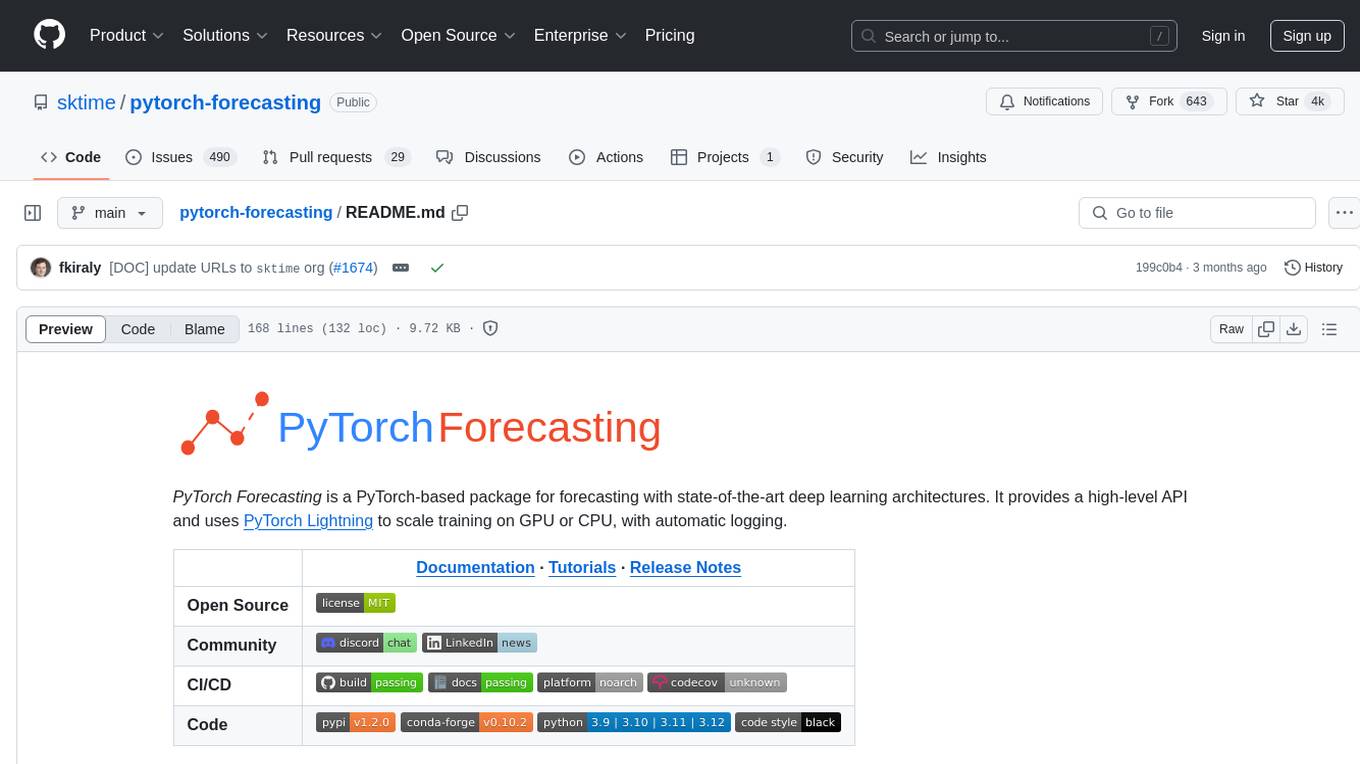
pytorch-forecasting
PyTorch Forecasting is a PyTorch-based package designed for state-of-the-art timeseries forecasting using deep learning architectures. It offers a high-level API and leverages PyTorch Lightning for efficient training on GPU or CPU with automatic logging. The package aims to simplify timeseries forecasting tasks by providing a flexible API for professionals and user-friendly defaults for beginners. It includes features such as a timeseries dataset class for handling data transformations, missing values, and subsampling, various neural network architectures optimized for real-world deployment, multi-horizon timeseries metrics, and hyperparameter tuning with optuna. Built on pytorch-lightning, it supports training on CPUs, single GPUs, and multiple GPUs out-of-the-box.
For similar jobs
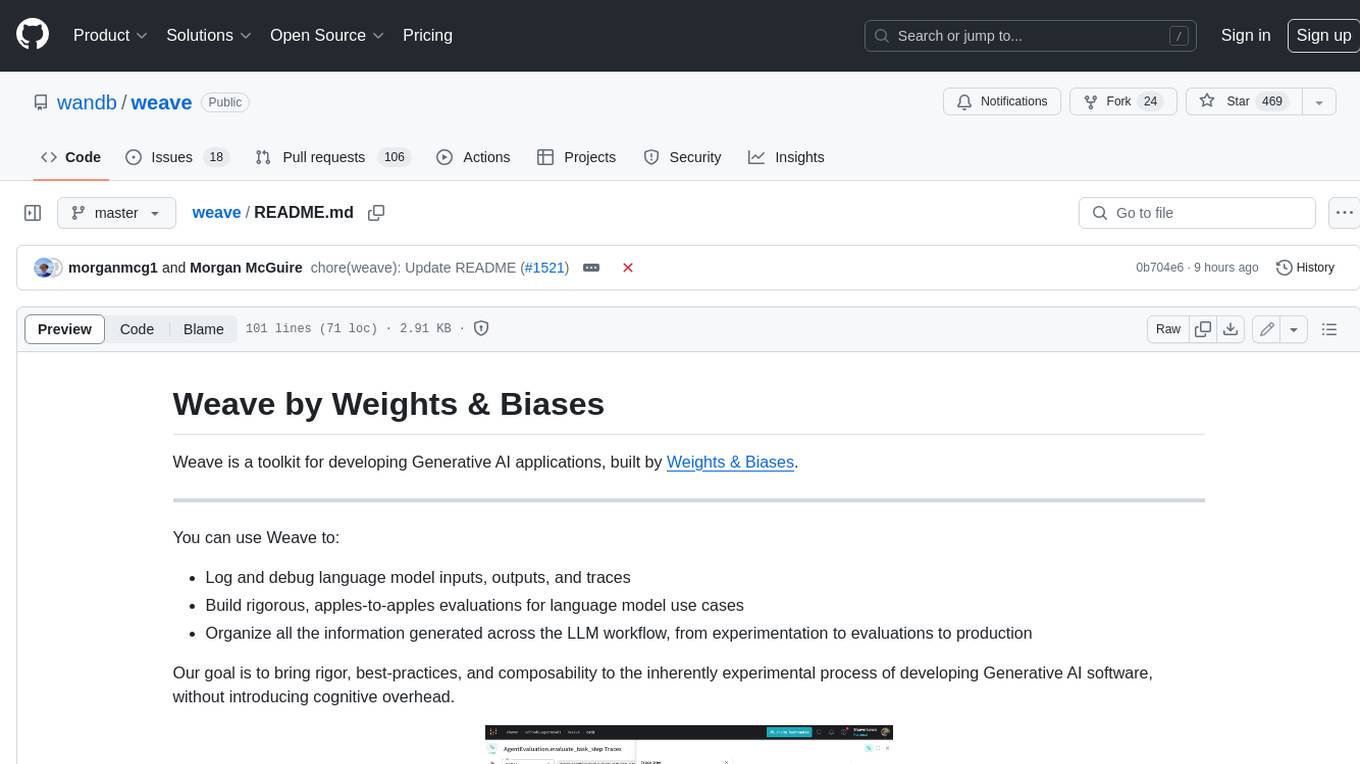
weave
Weave is a toolkit for developing Generative AI applications, built by Weights & Biases. With Weave, you can log and debug language model inputs, outputs, and traces; build rigorous, apples-to-apples evaluations for language model use cases; and organize all the information generated across the LLM workflow, from experimentation to evaluations to production. Weave aims to bring rigor, best-practices, and composability to the inherently experimental process of developing Generative AI software, without introducing cognitive overhead.
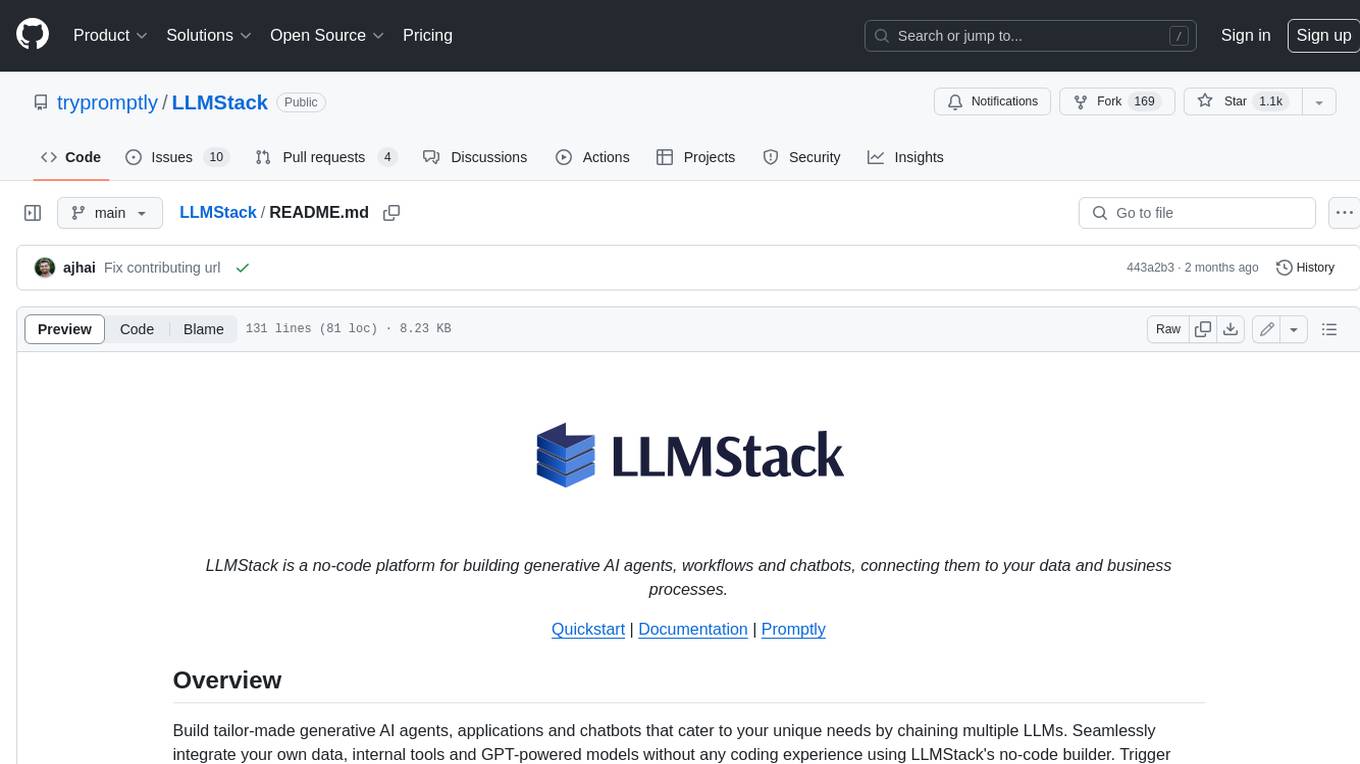
LLMStack
LLMStack is a no-code platform for building generative AI agents, workflows, and chatbots. It allows users to connect their own data, internal tools, and GPT-powered models without any coding experience. LLMStack can be deployed to the cloud or on-premise and can be accessed via HTTP API or triggered from Slack or Discord.
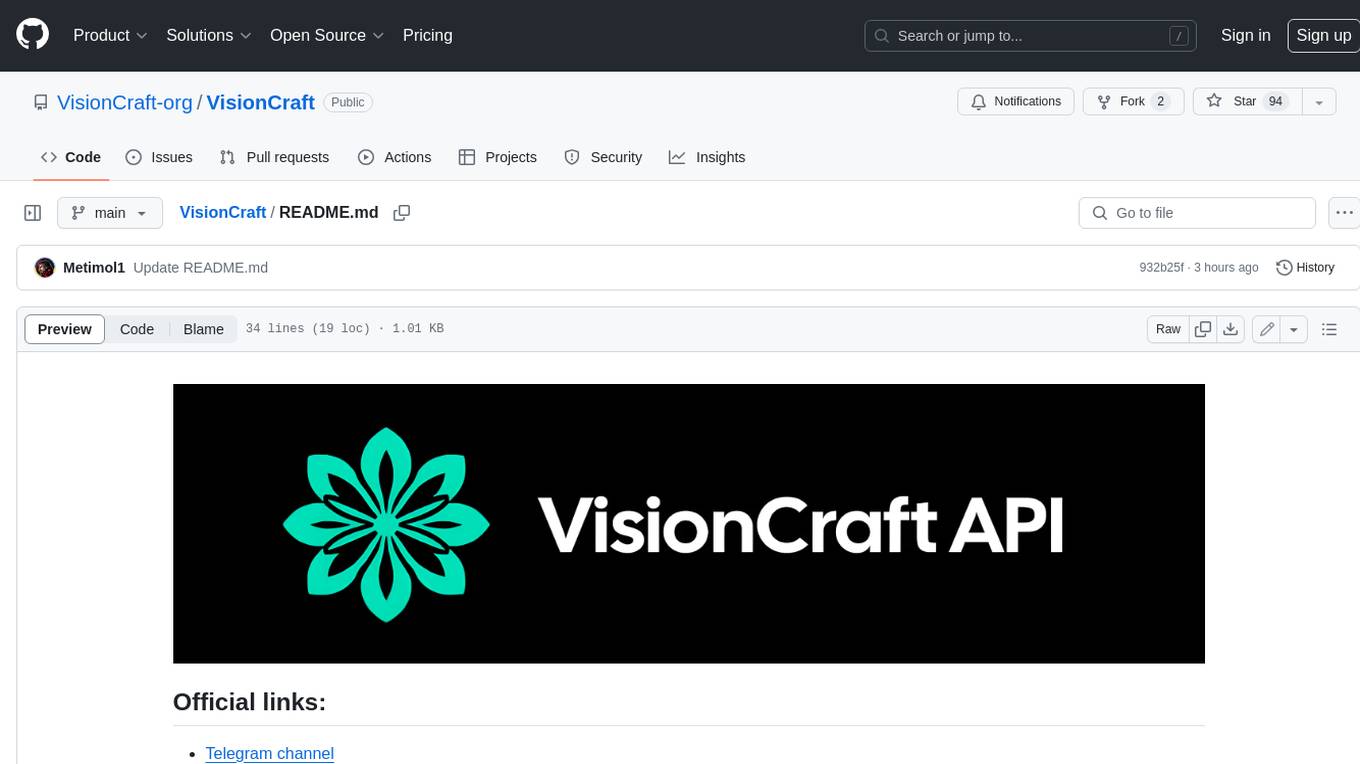
VisionCraft
The VisionCraft API is a free API for using over 100 different AI models. From images to sound.
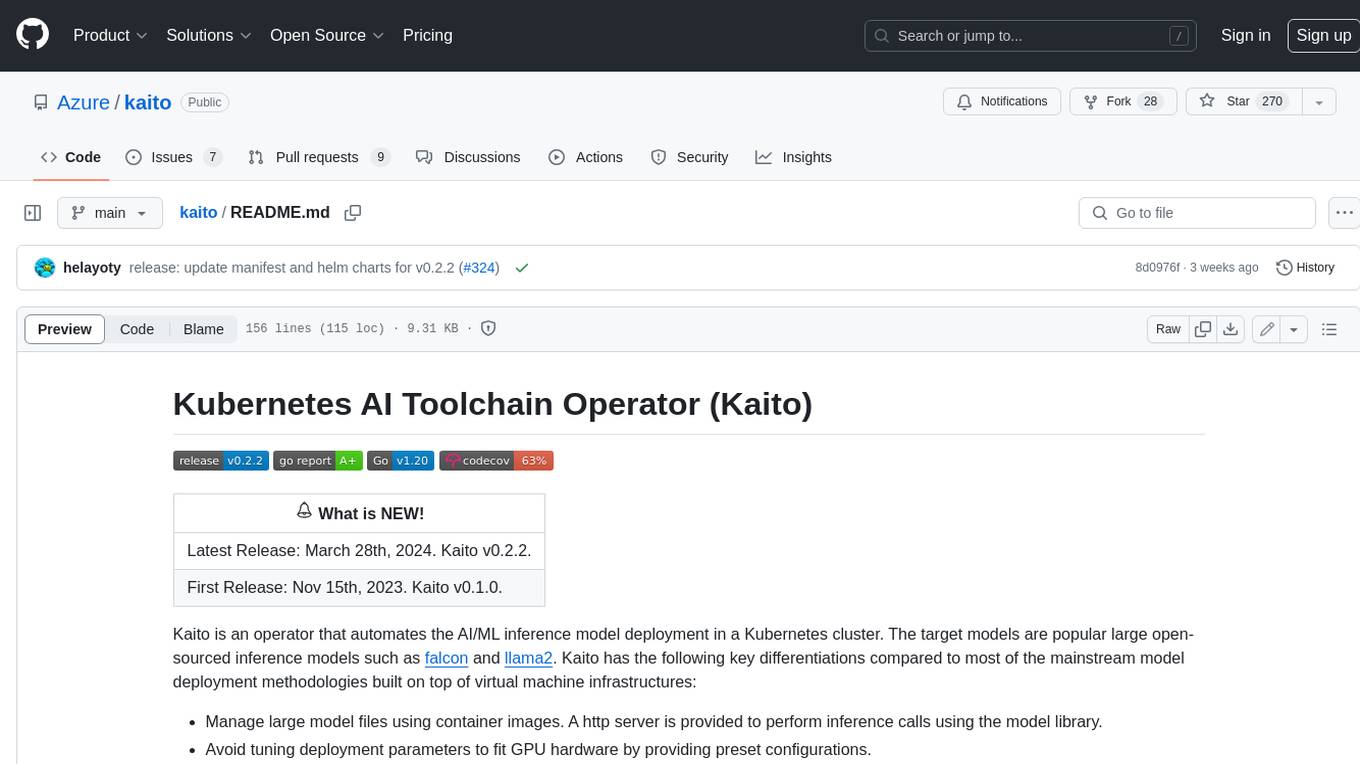
kaito
Kaito is an operator that automates the AI/ML inference model deployment in a Kubernetes cluster. It manages large model files using container images, avoids tuning deployment parameters to fit GPU hardware by providing preset configurations, auto-provisions GPU nodes based on model requirements, and hosts large model images in the public Microsoft Container Registry (MCR) if the license allows. Using Kaito, the workflow of onboarding large AI inference models in Kubernetes is largely simplified.
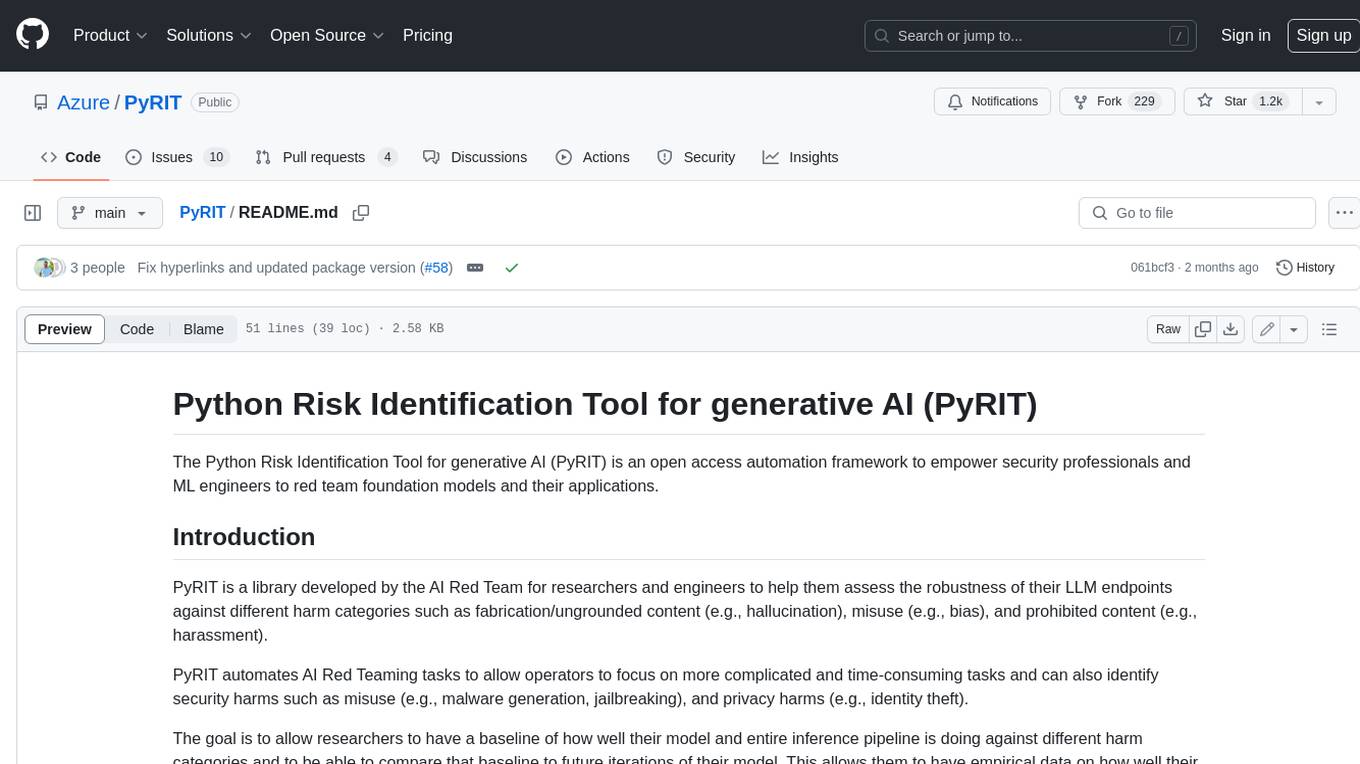
PyRIT
PyRIT is an open access automation framework designed to empower security professionals and ML engineers to red team foundation models and their applications. It automates AI Red Teaming tasks to allow operators to focus on more complicated and time-consuming tasks and can also identify security harms such as misuse (e.g., malware generation, jailbreaking), and privacy harms (e.g., identity theft). The goal is to allow researchers to have a baseline of how well their model and entire inference pipeline is doing against different harm categories and to be able to compare that baseline to future iterations of their model. This allows them to have empirical data on how well their model is doing today, and detect any degradation of performance based on future improvements.
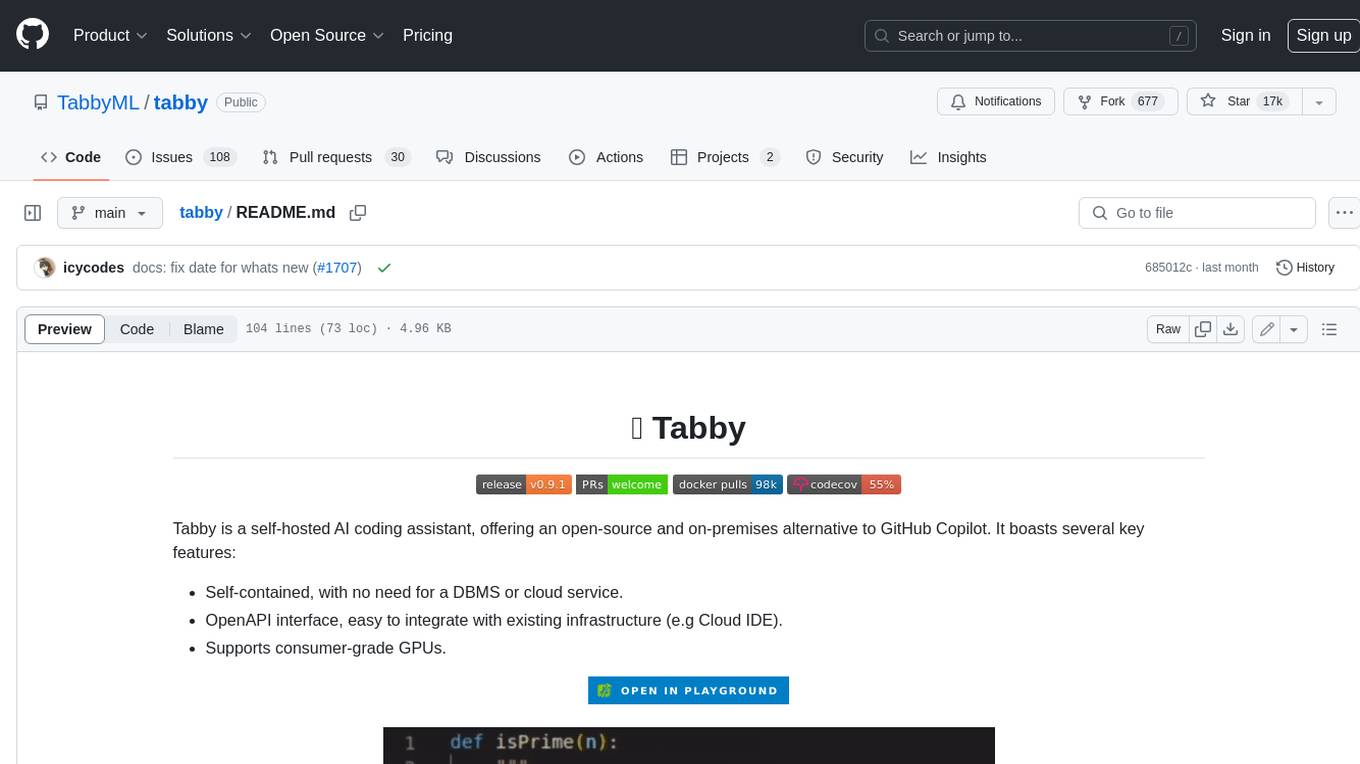
tabby
Tabby is a self-hosted AI coding assistant, offering an open-source and on-premises alternative to GitHub Copilot. It boasts several key features: * Self-contained, with no need for a DBMS or cloud service. * OpenAPI interface, easy to integrate with existing infrastructure (e.g Cloud IDE). * Supports consumer-grade GPUs.
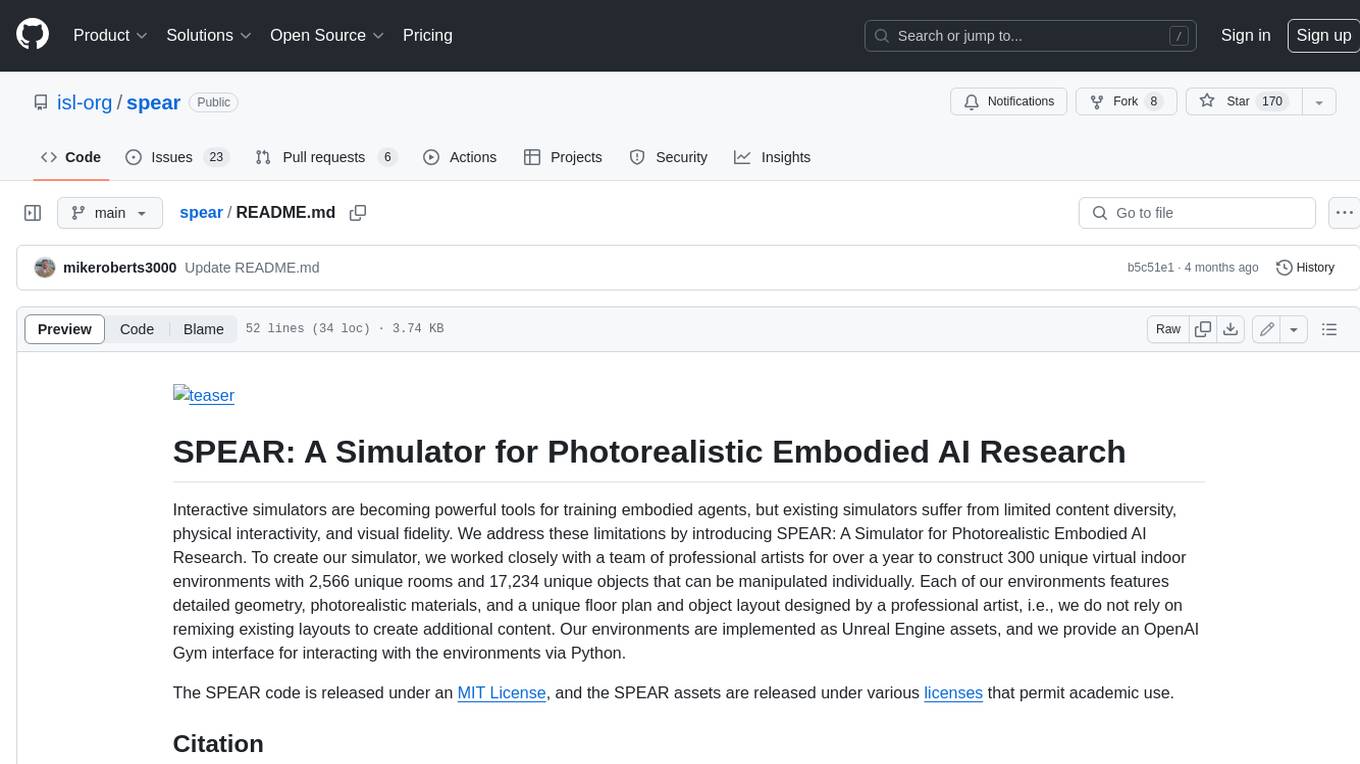
spear
SPEAR (Simulator for Photorealistic Embodied AI Research) is a powerful tool for training embodied agents. It features 300 unique virtual indoor environments with 2,566 unique rooms and 17,234 unique objects that can be manipulated individually. Each environment is designed by a professional artist and features detailed geometry, photorealistic materials, and a unique floor plan and object layout. SPEAR is implemented as Unreal Engine assets and provides an OpenAI Gym interface for interacting with the environments via Python.
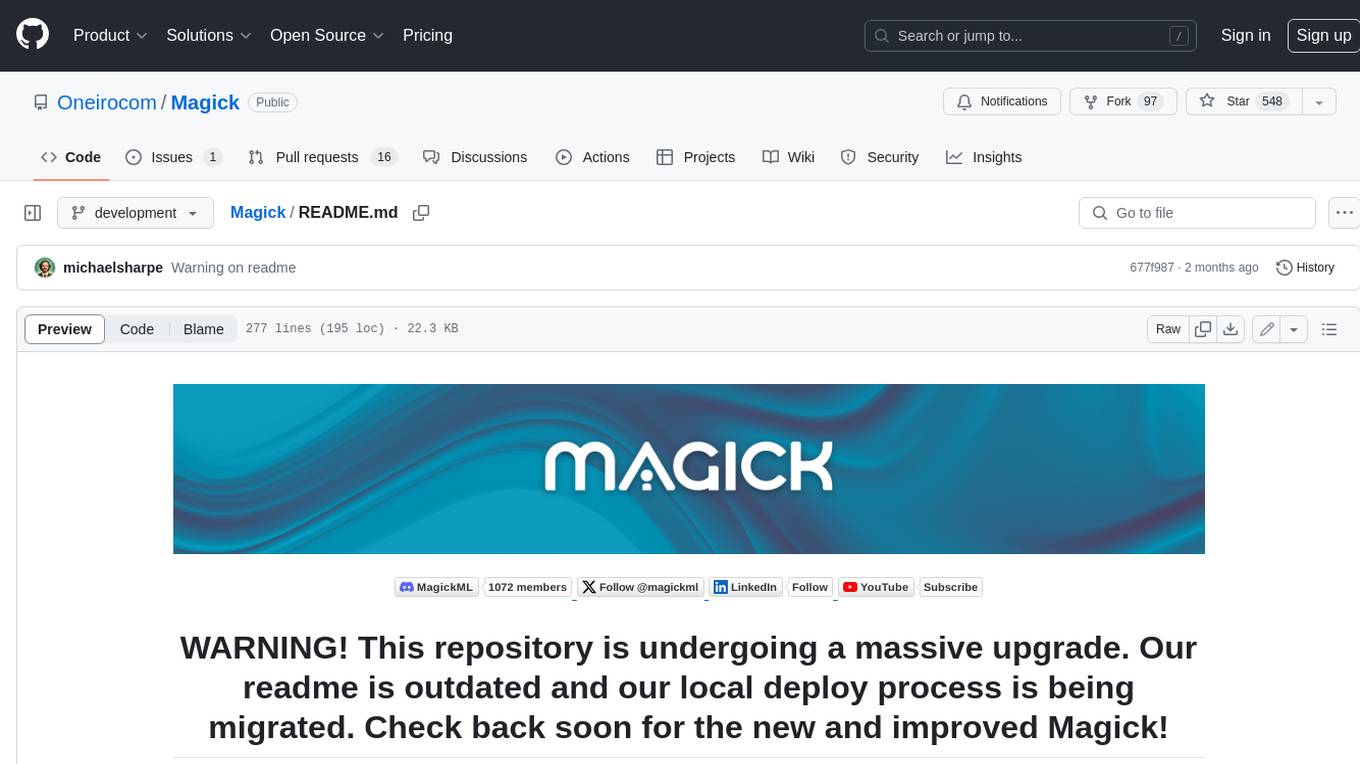
Magick
Magick is a groundbreaking visual AIDE (Artificial Intelligence Development Environment) for no-code data pipelines and multimodal agents. Magick can connect to other services and comes with nodes and templates well-suited for intelligent agents, chatbots, complex reasoning systems and realistic characters.