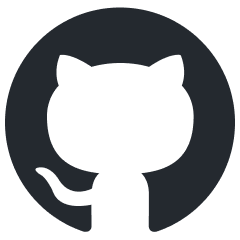
python-whatsapp-bot
Build AI WhatsApp Bots with Pure Python
Stars: 571
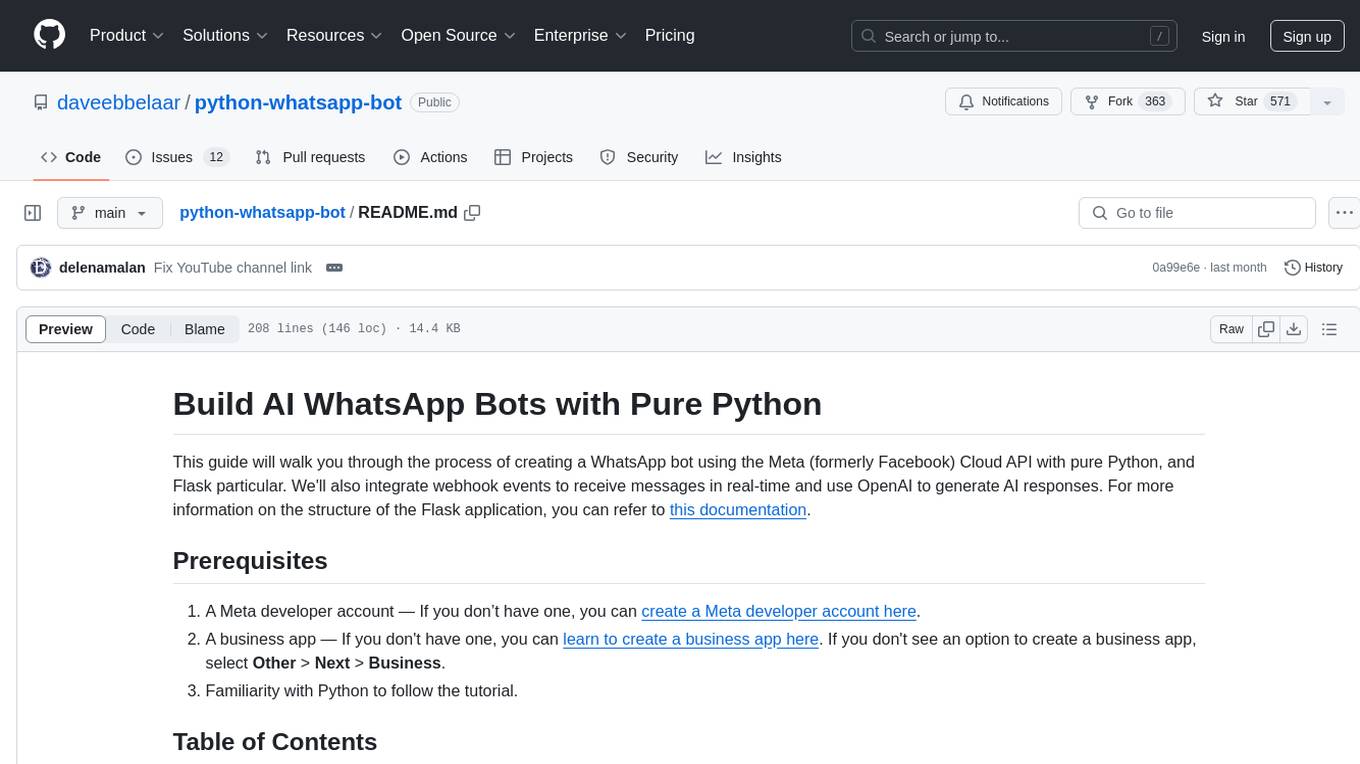
This repository provides a comprehensive guide on building AI WhatsApp bots using Python and Flask. It covers setting up a Meta developer account, integrating webhook events for real-time message reception, and using OpenAI for AI responses. The tutorial includes steps for selecting phone numbers, sending messages with the API, configuring webhooks, integrating AI into the application, and adding a phone number. It also explains the process of creating a system user, obtaining access tokens, and validating verification requests and payloads for webhook security. The repository aims to help users create intelligent WhatsApp bots with Python and AI capabilities.
README:
This guide will walk you through the process of creating a WhatsApp bot using the Meta (formerly Facebook) Cloud API with pure Python, and Flask particular. We'll also integrate webhook events to receive messages in real-time and use OpenAI to generate AI responses. For more information on the structure of the Flask application, you can refer to this documentation.
- A Meta developer account — If you don’t have one, you can create a Meta developer account here.
- A business app — If you don't have one, you can learn to create a business app here. If you don't see an option to create a business app, select Other > Next > Business.
- Familiarity with Python to follow the tutorial.
-
Build AI WhatsApp Bots with Pure Python
- Prerequisites
- Table of Contents
- Get Started
- Step 1: Select Phone Numbers
- Step 2: Send Messages with the API
- Step 3: Configure Webhooks to Receive Messages
- Step 4: Understanding Webhook Security
- Step 5: Learn about the API and Build Your App
- Step 6: Integrate AI into the Application
- Step 7: Add a Phone Number
- Datalumina
- Tutorials
- Overview & Setup: Begin your journey here.
- Locate Your Bots: Your bots can be found here.
- WhatsApp API Documentation: Familiarize yourself with the official documentation.
- Helpful Guide: Here's a Python-based guide for sending messages.
- API Docs for Sending Messages: Check out this documentation.
- Make sure WhatsApp is added to your App.
- You begin with a test number that you can use to send messages to up to 5 numbers.
- Go to API Setup and locate the test number from which you will be sending messages.
- Here, you can also add numbers to send messages to. Enter your own WhatsApp number.
- You will receive a code on your phone via WhatsApp to verify your number.
- Obtain a 24-hour access token from the API access section.
- It will show an example of how to send messages using a
curl
command which can be send from the terminal or with a tool like Postman. - Let's convert that into a Python function with the request library.
- Create a
.env
files based onexample.env
and update the required variables. Video example here. - You will receive a "Hello World" message (Expect a 60-120 second delay for the message).
Creating an access that works longer then 24 hours
- Create a system user at the Meta Business account level.
- On the System Users page, configure the assets for your System User, assigning your WhatsApp app with full control. Don't forget to click the Save Changes button.
- Now click
Generate new token
and select the app, and then choose how long the access token will be valid. You can choose 60 days or never expire. - Select all the permissions, as I was running into errors when I only selected the WhatsApp ones.
- Confirm and copy the access token.
Now we have to find the following information on the App Dashboard:
- APP_ID: "<YOUR-WHATSAPP-BUSINESS-APP_ID>" (Found at App Dashboard)
- APP_SECRET: "<YOUR-WHATSAPP-BUSINESS-APP_SECRET>" (Found at App Dashboard)
- RECIPIENT_WAID: "" (This is your WhatsApp ID, i.e., phone number. Make sure it is added to the account as shown in the example test message.)
- VERSION: "v18.0" (The latest version of the Meta Graph API)
- ACCESS_TOKEN: "" (Created in the previous step)
You can only send a template type message as your first message to a user. That's why you have to send a reply first before we continue. Took me 2 hours to figure this out.
Please note, this is the hardest part of this tutorial.
- Make you have a python installation or environment and install the requirements:
pip install -r requirements.txt
- Run your Flask app locally by executing run.py
The steps below are taken from the ngrok documentation.
You need a static ngrok domain because Meta validates your ngrok domain and certificate!
Once your app is running successfully on localhost, let's get it on the internet securely using ngrok!
- If you're not an ngrok user yet, just sign up for ngrok for free.
- Download the ngrok agent.
- Go to the ngrok dashboard, click Your Authtoken, and copy your Authtoken.
- Follow the instructions to authenticate your ngrok agent. You only have to do this once.
- On the left menu, expand Cloud Edge and then click Domains.
- On the Domains page, click + Create Domain or + New Domain. (here everyone can start with one free domain)
- Start ngrok by running the following command in a terminal on your local desktop:
ngrok http 8000 --domain your-domain.ngrok-free.app
- ngrok will display a URL where your localhost application is exposed to the internet (copy this URL for use with Meta).
In the Meta App Dashboard, go to WhatsApp > Configuration, then click the Edit button.
- In the Edit webhook's callback URL popup, enter the URL provided by the ngrok agent to expose your application to the internet in the Callback URL field, with /webhook at the end (i.e. https://myexample.ngrok-free.app/webhook).
- Enter a verification token. This string is set up by you when you create your webhook endpoint. You can pick any string you like. Make sure to update this in your
VERIFY_TOKEN
environment variable. - After you add a webhook to WhatsApp, WhatsApp will submit a validation post request to your application through ngrok. Confirm your localhost app receives the validation get request and logs
WEBHOOK_VERIFIED
in the terminal. - Back to the Configuration page, click Manage.
- On the Webhook fields popup, click Subscribe to the messages field. Tip: You can subscribe to multiple fields.
- If your Flask app and ngrok are running, you can click on "Test" next to messages to test the subscription. You recieve a test message in upper case. If that is the case, your webhook is set up correctly.
Use the phone number associated to your WhatsApp product or use the test number you copied before.
- Add this number to your WhatsApp app contacts and then send a message to this number.
- Confirm your localhost app receives a message and logs both headers and body in the terminal.
- Test if the bot replies back to you in upper case.
- You have now succesfully integrated the bot! 🎉
- Now it's time to acutally build cool things with this.
Below is some information from the Meta Webhooks API docs about verification and security. It is already implemented in the code, but you can reference it to get a better understanding of what's going on in security.py
Anytime you configure the Webhooks product in your App Dashboard, we'll send a GET request to your endpoint URL. Verification requests include the following query string parameters, appended to the end of your endpoint URL. They will look something like this:
GET https://www.your-clever-domain-name.com/webhook?
hub.mode=subscribe&
hub.challenge=1158201444&
hub.verify_token=meatyhamhock
The verify_token, meatyhamhock
in the case of this example, is a string that you can pick. It doesn't matter what it is as long as you store in the VERIFY_TOKEN
environment variable.
Whenever your endpoint receives a verification request, it must:
- Verify that the hub.verify_token value matches the string you set in the Verify Token field when you configure the Webhooks product in your App Dashboard (you haven't set up this token string yet).
- Respond with the hub.challenge value.
WhatsApp signs all Event Notification payloads with a SHA256 signature and include the signature in the request's X-Hub-Signature-256 header, preceded with sha256=. You don't have to validate the payload, but you should.
To validate the payload:
- Generate a SHA256 signature using the payload and your app's App Secret.
- Compare your signature to the signature in the X-Hub-Signature-256 header (everything after sha256=). If the signatures match, the payload is genuine.
Review the developer documentation to learn how to build your app and start sending messages. See documentation.
Now that we have an end to end connection, we can make the bot a little more clever then just shouting at us in upper case. All you have to do is come up with your own generate_response()
function in whatsapp_utils.py.
If you want a cookie cutter example to integrate the OpenAI Assistans API with a retrieval tool, then follow these steps.
- Watch this video: OpenAI Assistants Tutorial
- Create your own assistant with OpenAI and update your
OPENAI_API_KEY
andOPENAI_ASSISTANT_ID
in the environment variables. - Provide your assistant with data and instructions
- Update openai_service.py to your use case.
- Import
generate_reponse
into whatsapp_utils.py - Update
process_whatsapp_message()
with the newgenerate_reponse()
function.
When you’re ready to use your app for a production use case, you need to use your own phone number to send messages to your users.
To start sending messages to any WhatsApp number, add a phone number. To manage your account information and phone number, see the Overview page. and the WhatsApp docs.
If you want to use a number that is already being used in the WhatsApp customer or business app, you will have to fully migrate that number to the business platform. Once the number is migrated, you will lose access to the WhatsApp customer or business app. See Migrate Existing WhatsApp Number to a Business Account for information.
Once you have chosen your phone number, you have to add it to your WhatsApp Business Account. See Add a Phone Number.
When dealing with WhatsApp Business API and wanting to experiment without affecting your personal number, you have a few options:
- Buy a New SIM Card
- Virtual Phone Numbers
- Dual SIM Phones
- Use a Different Device
- Temporary Number Services
- Dedicated Devices for Development
Recommendation: If this is for a more prolonged or professional purpose, using a virtual phone number service or purchasing a new SIM card for a dedicated device is advisable. For quick tests, a temporary number might suffice, but always be cautious about security and privacy. Remember that once a number is associated with WhatsApp Business API, it cannot be used with regular WhatsApp on a device unless you deactivate it from the Business API and reverify it on the device.
This document is provided to you by Datalumina. We help data analysts, engineers, and scientists launch and scale a successful freelance business — $100k+ /year, fun projects, happy clients. If you want to learn more about what we do, you can visit our website and subscribe to our newsletter. Feel free to share this document with your data friends and colleagues.
For video tutorials, visit the YouTube channel: youtube.com/@daveebbelaar.
For Tasks:
Click tags to check more tools for each tasksFor Jobs:
Alternative AI tools for python-whatsapp-bot
Similar Open Source Tools
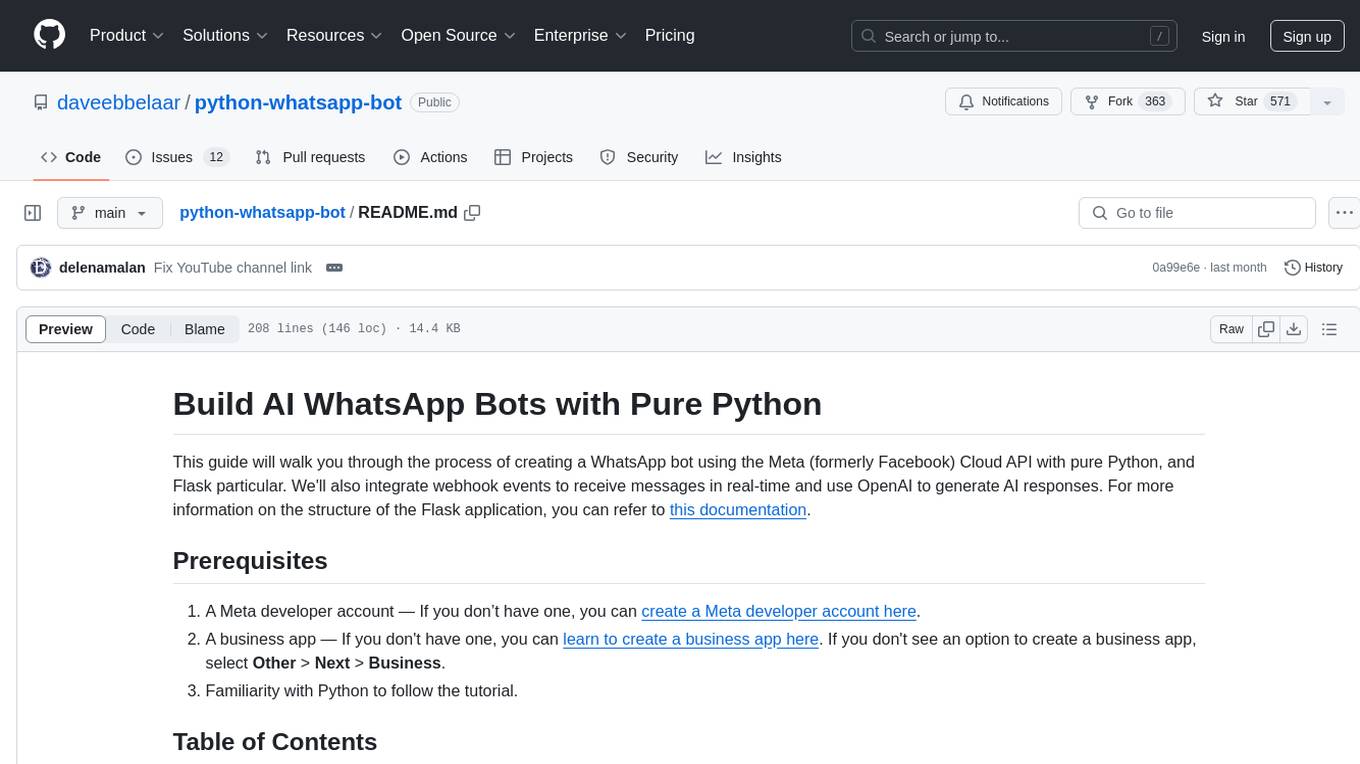
python-whatsapp-bot
This repository provides a comprehensive guide on building AI WhatsApp bots using Python and Flask. It covers setting up a Meta developer account, integrating webhook events for real-time message reception, and using OpenAI for AI responses. The tutorial includes steps for selecting phone numbers, sending messages with the API, configuring webhooks, integrating AI into the application, and adding a phone number. It also explains the process of creating a system user, obtaining access tokens, and validating verification requests and payloads for webhook security. The repository aims to help users create intelligent WhatsApp bots with Python and AI capabilities.
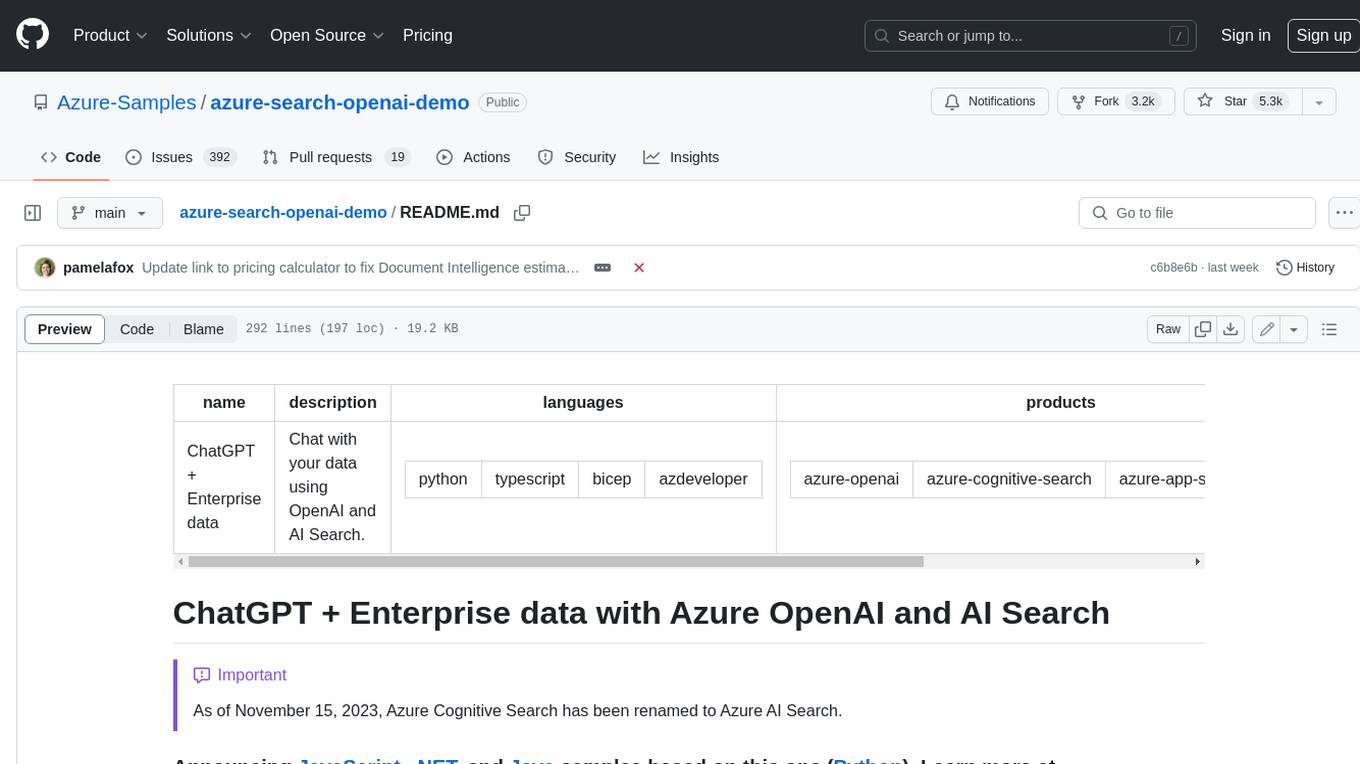
azure-search-openai-demo
This sample demonstrates a few approaches for creating ChatGPT-like experiences over your own data using the Retrieval Augmented Generation pattern. It uses Azure OpenAI Service to access a GPT model (gpt-35-turbo), and Azure AI Search for data indexing and retrieval. The repo includes sample data so it's ready to try end to end. In this sample application we use a fictitious company called Contoso Electronics, and the experience allows its employees to ask questions about the benefits, internal policies, as well as job descriptions and roles.
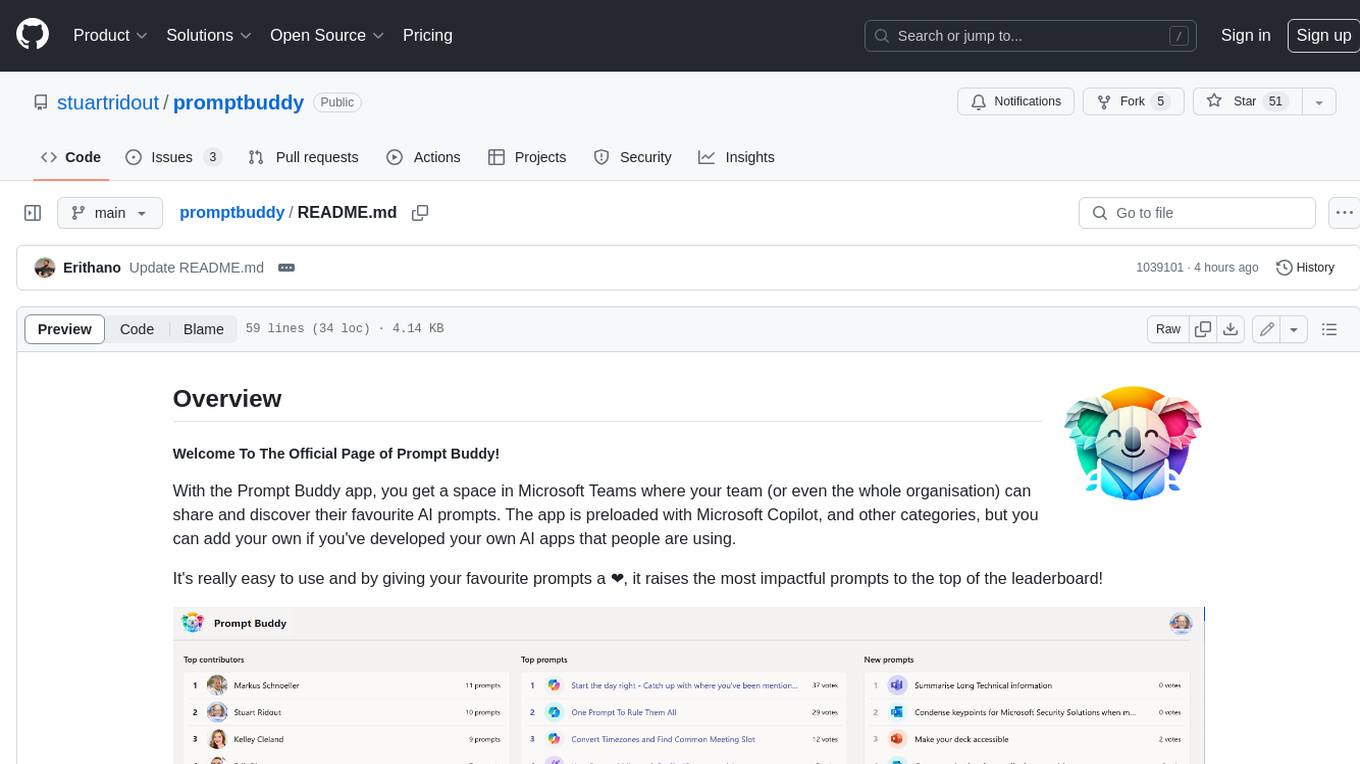
promptbuddy
Prompt Buddy is a Microsoft Teams app that provides a central location for teams to share and discover their favorite AI prompts. It comes preloaded with Microsoft Copilot and other categories, but users can also add their own custom prompts. The app is easy to use and allows users to upvote their favorite prompts, which raises them to the top of the leaderboard. Prompt Buddy also supports dark mode and offers a mobile layout for use on phones. It is built on the Power Platform and can be customized and extended by the installer.
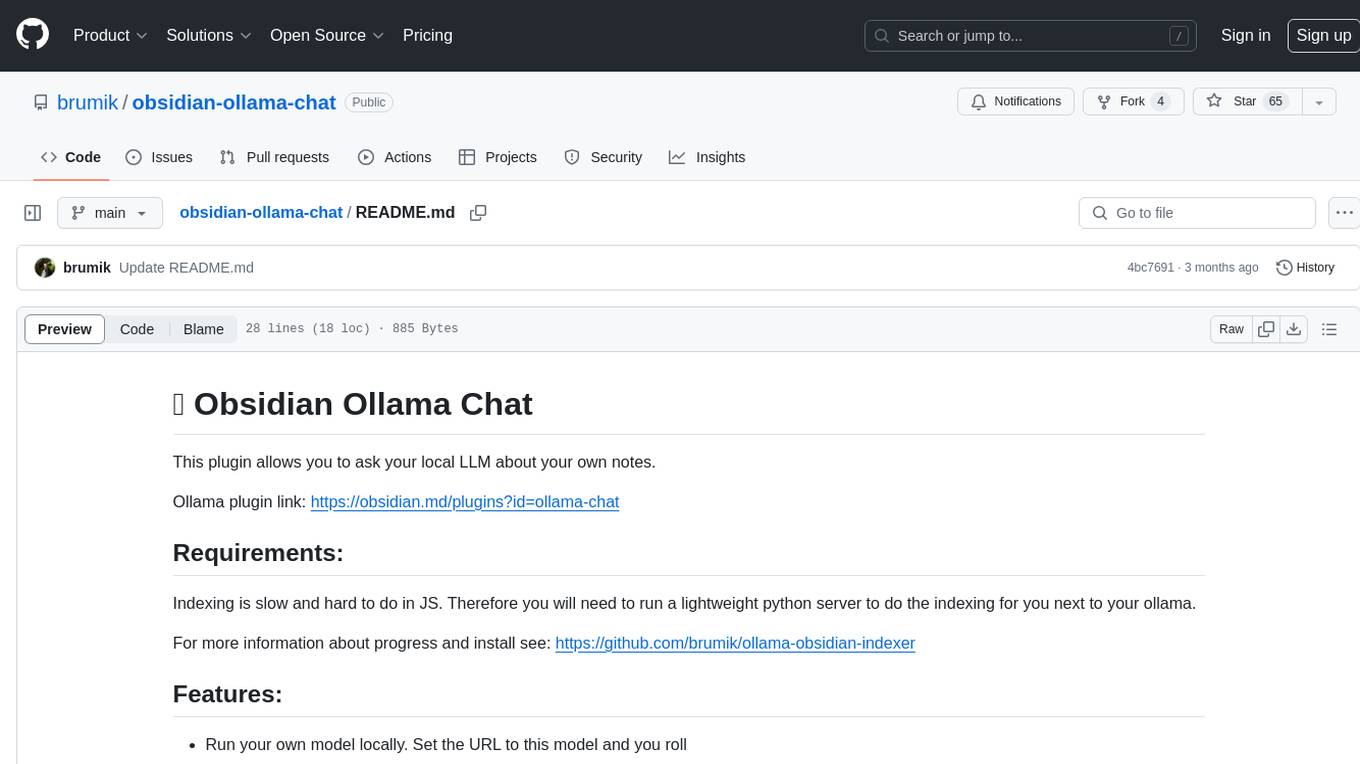
obsidian-ollama-chat
Obsidian Ollama Chat is a plugin that enables users to interact with their local LLM (Large Language Model) to ask questions about their own notes. The plugin facilitates running a lightweight python server for indexing notes, allowing users to set a model URL, index files on startup and modification, and open a modal to ask questions. Future plans include text streaming for querying, a chat window for communication, and commands for quick queries like summarizing notes or topics.
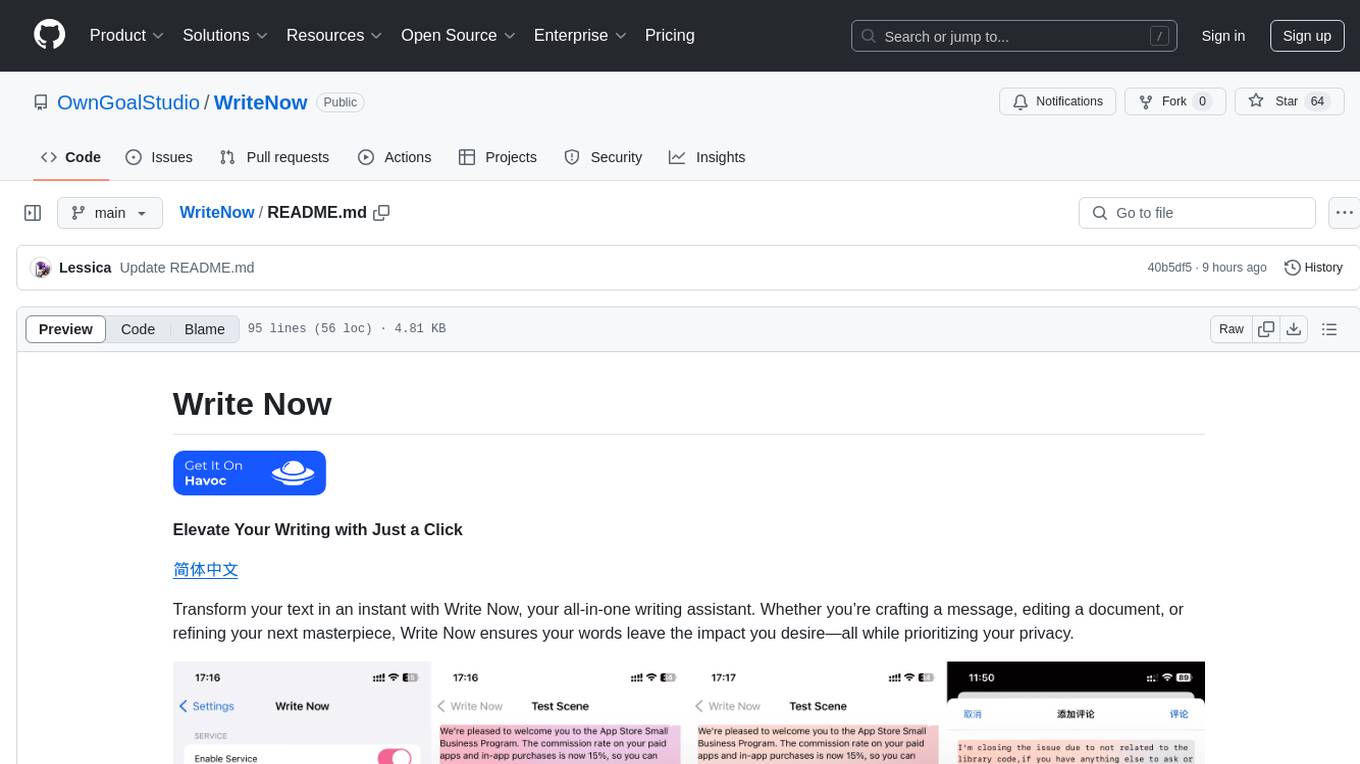
WriteNow
Write Now is an all-in-one writing assistant that helps users elevate their text with features like proofreading, rewriting, friendly and professional tones, concise mode, and custom AI server configuration. It prioritizes user privacy and offers a Lite Edition for trial purposes. Users can install Write Now through the Havoc Store and configure AI server endpoints for enhanced functionality.
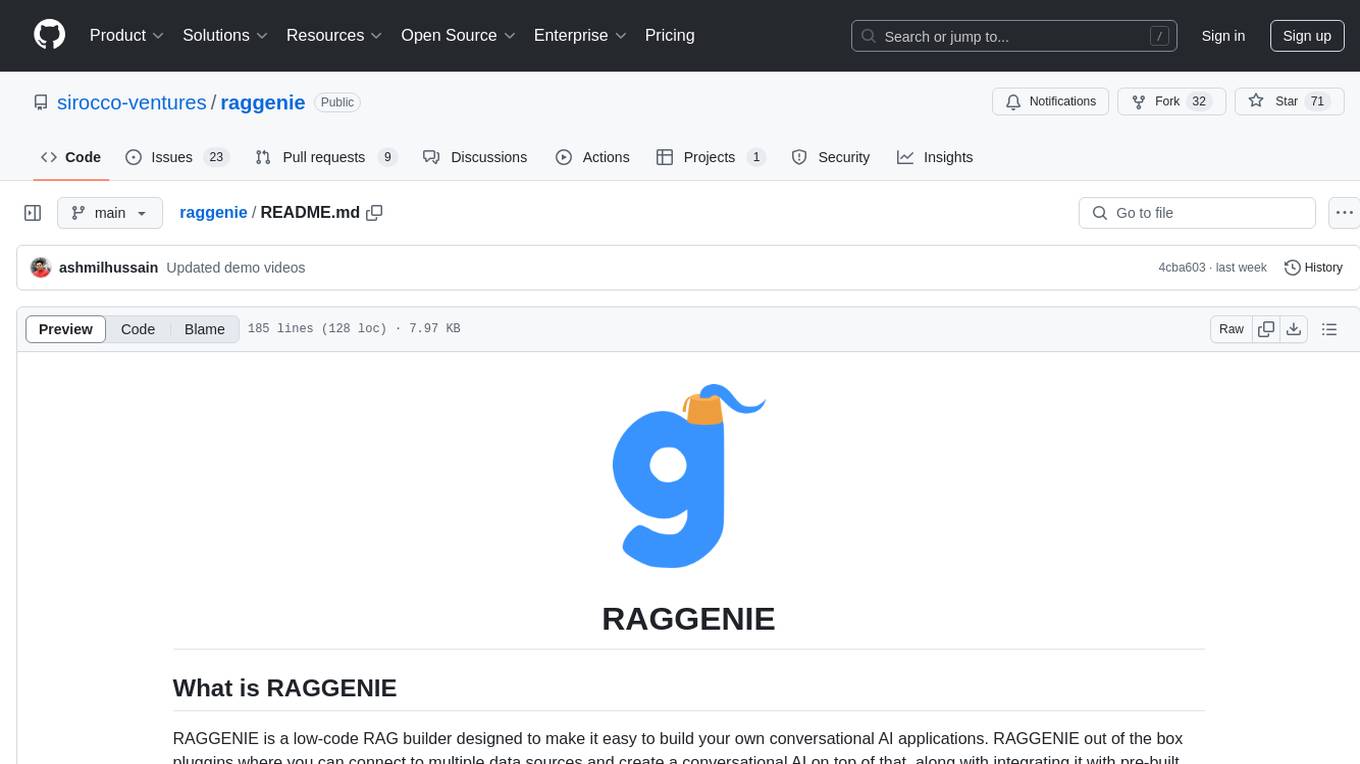
raggenie
RAGGENIE is a low-code RAG builder tool designed to simplify the creation of conversational AI applications. It offers out-of-the-box plugins for connecting to various data sources and building conversational AI on top of them, including integration with pre-built agents for actions. The tool is open-source under the MIT license, with a current focus on making it easy to build RAG applications and future plans for maintenance, monitoring, and transitioning applications from pilots to production.
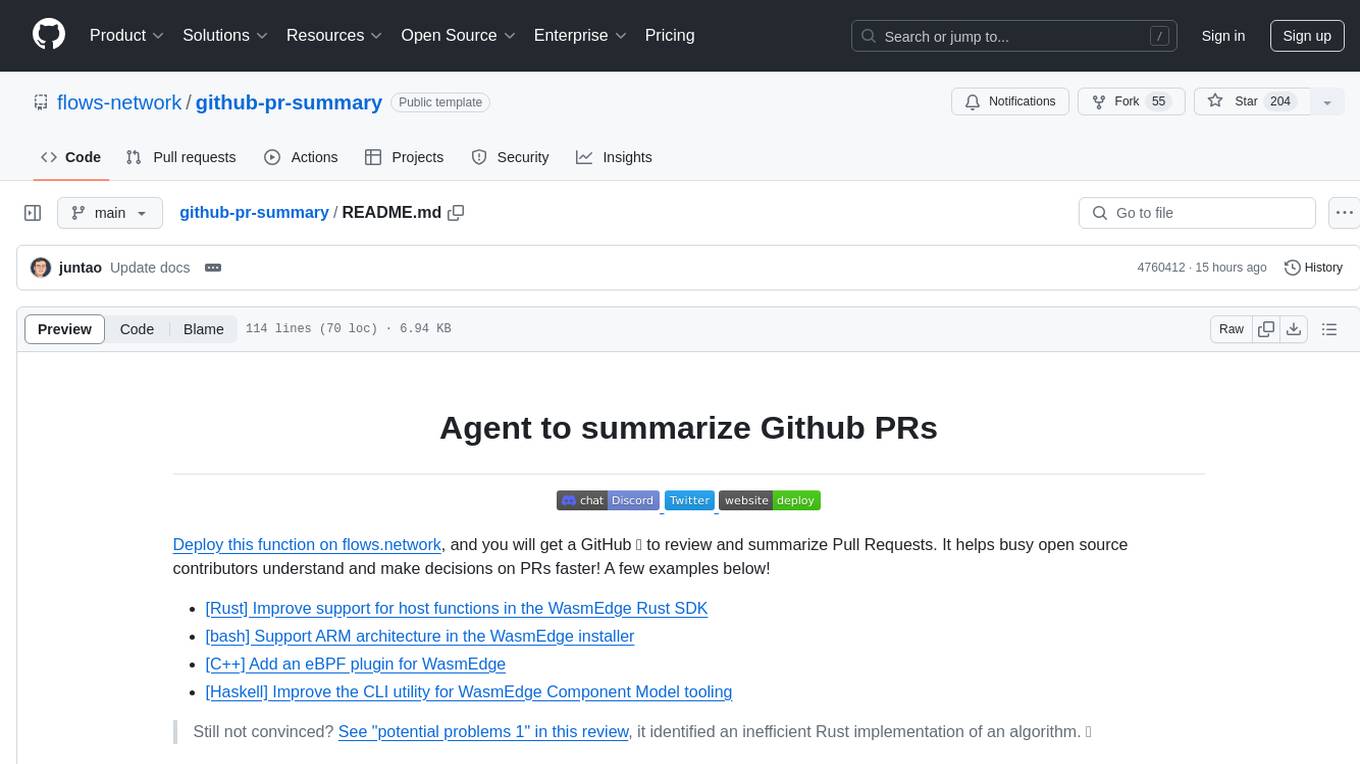
github-pr-summary
github-pr-summary is a bot designed to summarize GitHub Pull Requests, helping open source contributors make faster decisions. It automatically summarizes commits and changed files in PRs, triggered by new commits or a magic trigger phrase. Users can deploy their own code review bot in 3 steps: create a bot from their GitHub repo, configure it to review PRs, and connect to GitHub for access to the target repo. The bot runs on flows.network using Rust and WasmEdge Runtimes. It utilizes ChatGPT/4 to review and summarize PR content, posting the result back as a comment on the PR. The bot can be used on multiple repos by creating new flows and importing the source code repo, specifying the target repo using flow config. Users can also change the magic phrase to trigger a review from a PR comment.
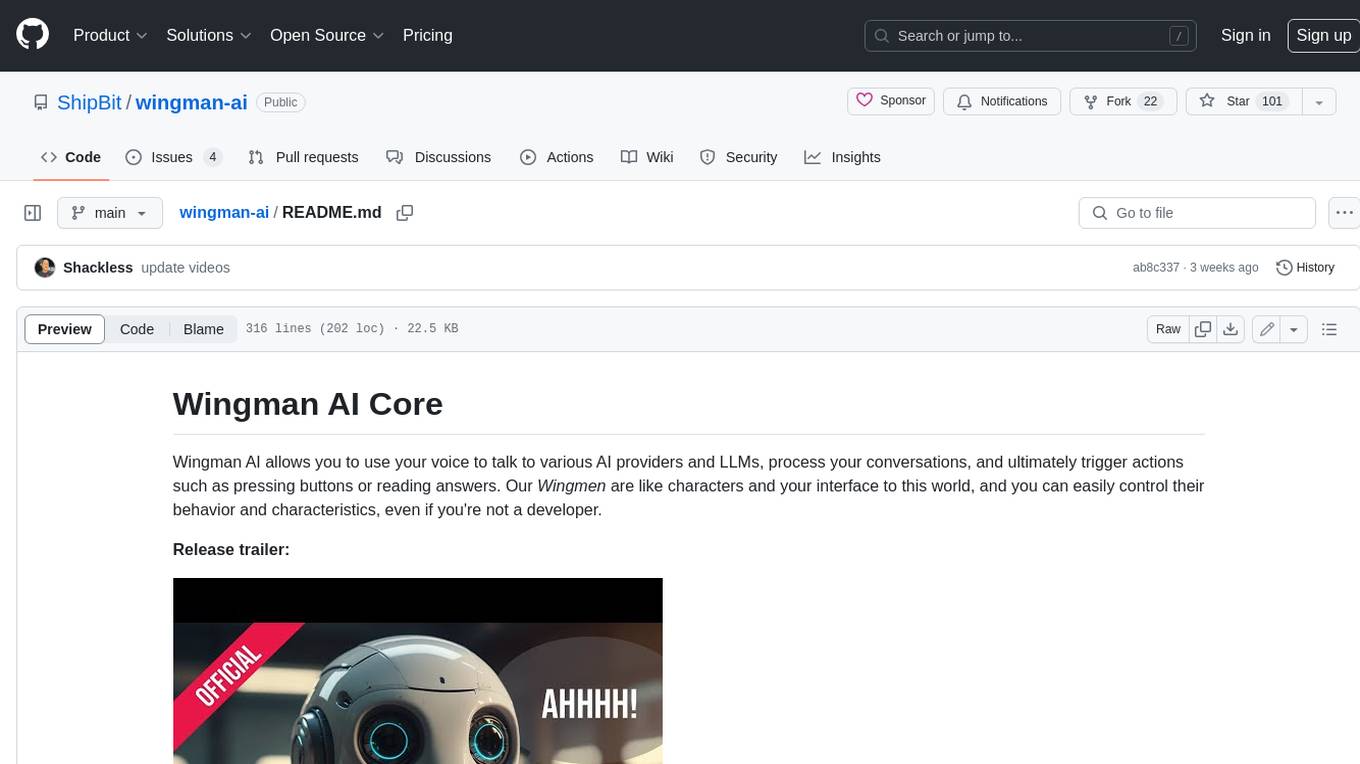
wingman-ai
Wingman AI allows you to use your voice to talk to various AI providers and LLMs, process your conversations, and ultimately trigger actions such as pressing buttons or reading answers. Our _Wingmen_ are like characters and your interface to this world, and you can easily control their behavior and characteristics, even if you're not a developer. AI is complex and it scares people. It's also **not just ChatGPT**. We want to make it as easy as possible for you to get started. That's what _Wingman AI_ is all about. It's a **framework** that allows you to build your own Wingmen and use them in your games and programs. The idea is simple, but the possibilities are endless. For example, you could: * **Role play** with an AI while playing for more immersion. Have air traffic control (ATC) in _Star Citizen_ or _Flight Simulator_. Talk to Shadowheart in Baldur's Gate 3 and have her respond in her own (cloned) voice. * Get live data such as trade information, build guides, or wiki content and have it read to you in-game by a _character_ and voice you control. * Execute keystrokes in games/applications and create complex macros. Trigger them in natural conversations with **no need for exact phrases.** The AI understands the context of your dialog and is quite _smart_ in recognizing your intent. Say _"It's raining! I can't see a thing!"_ and have it trigger a command you simply named _WipeVisors_. * Automate tasks on your computer * improve accessibility * ... and much more
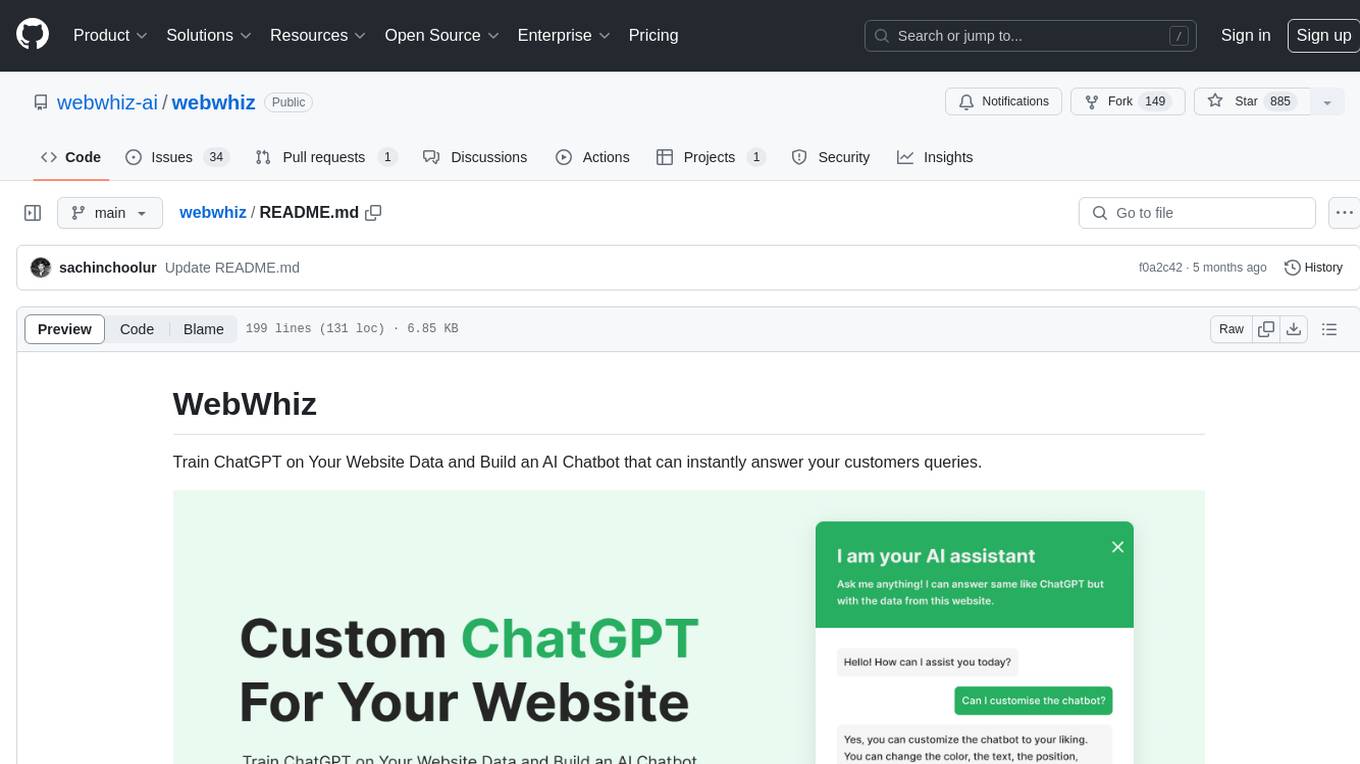
webwhiz
WebWhiz is an open-source tool that allows users to train ChatGPT on website data to build AI chatbots for customer queries. It offers easy integration, data-specific responses, regular data updates, no-code builder, chatbot customization, fine-tuning, and offline messaging. Users can create and train chatbots in a few simple steps by entering their website URL, automatically fetching and preparing training data, training ChatGPT, and embedding the chatbot on their website. WebWhiz can crawl websites monthly, collect text data and metadata, and process text data using tokens. Users can train custom data, but bringing custom open AI keys is not yet supported. The tool has no limitations on context size but may limit the number of pages based on the chosen plan. WebWhiz SDK is available on NPM, CDNs, and GitHub, and users can self-host it using Docker or manual setup involving MongoDB, Redis, Node, Python, and environment variables setup. For any issues, users can contact [email protected].
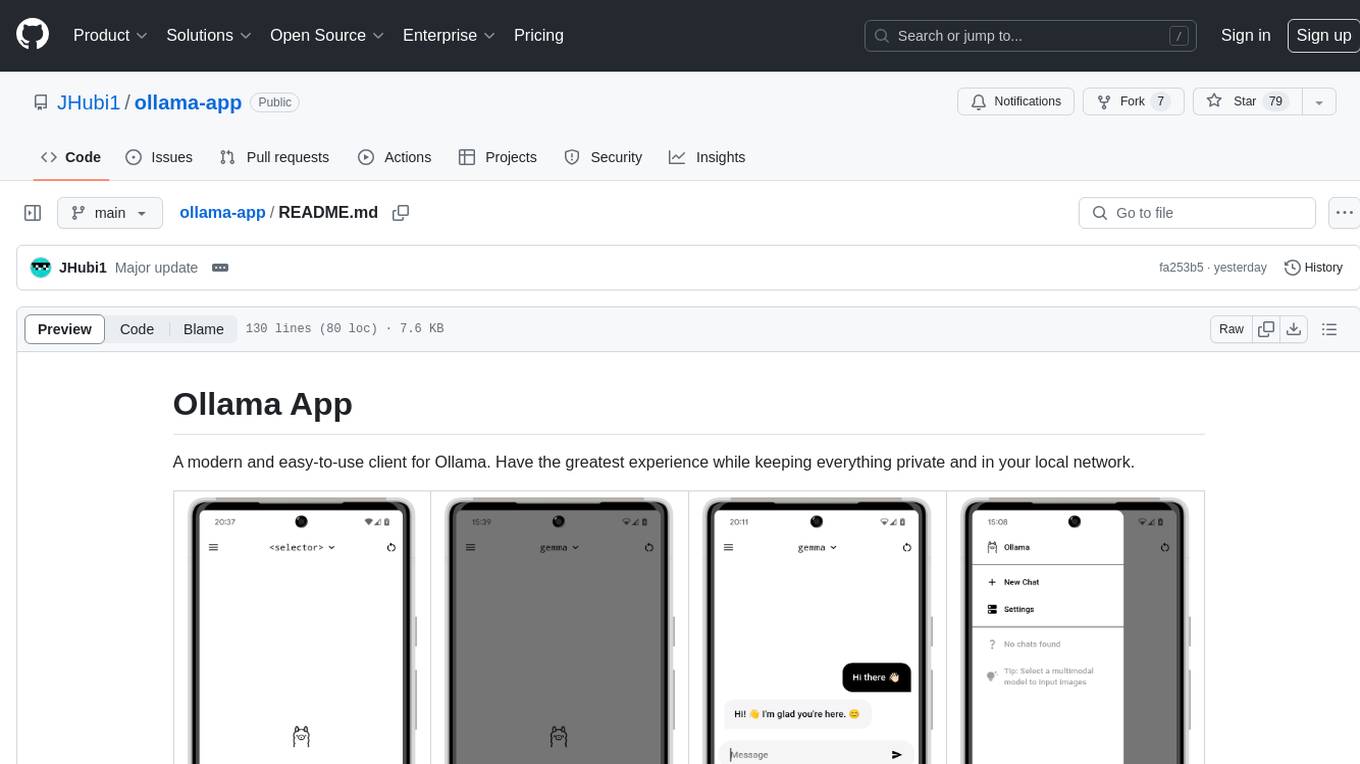
ollama-app
Ollama App is a modern and easy-to-use client for Ollama, allowing users to have a private experience within their local network. The app connects to an Ollama server using its API endpoint, enabling users to chat and interact with various models. It supports multimodal model input, a multilingual interface, and custom builds for personalized experiences. Users can easily set up the app, navigate through the side menu, select models, and create custom builds to tailor the app to their needs.
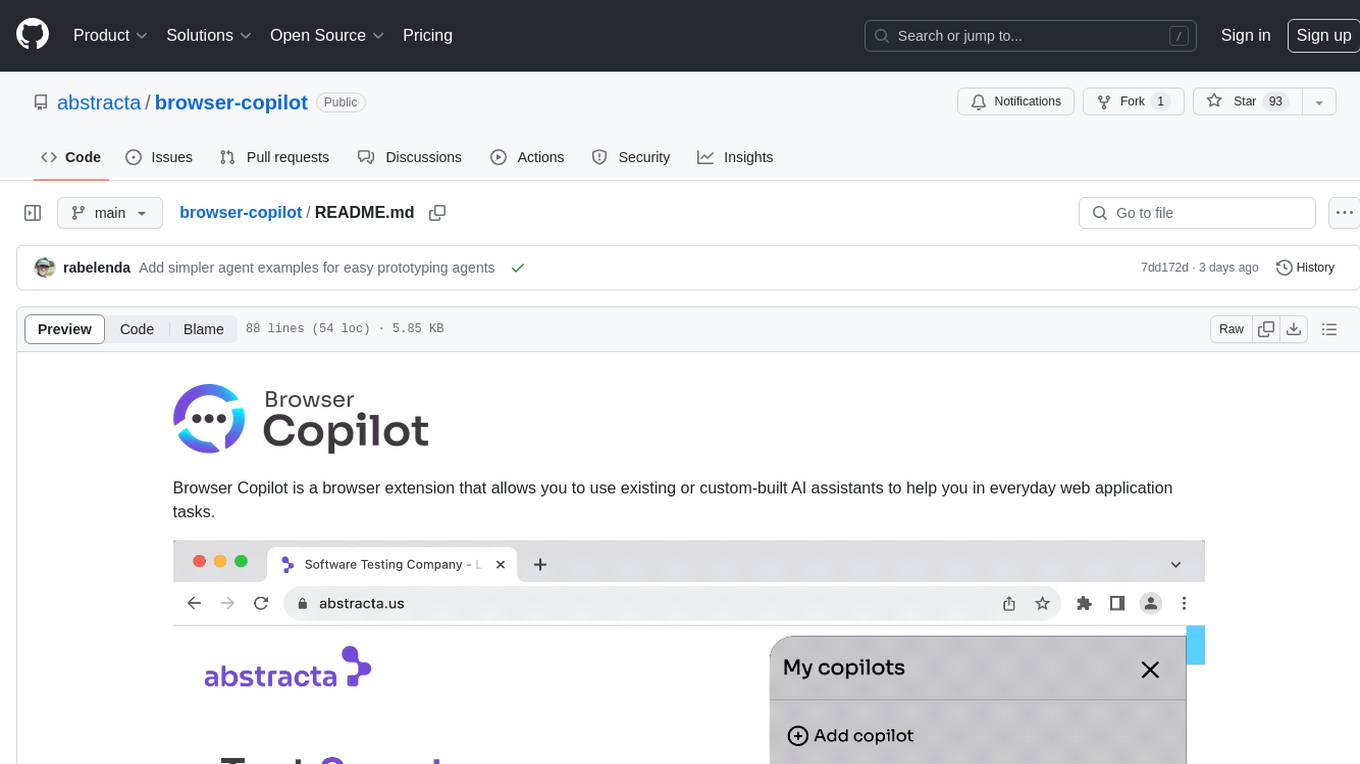
browser-copilot
Browser Copilot is a browser extension that enables users to utilize AI assistants for various web application tasks. It provides a versatile UI and framework to implement copilots that can automate tasks, extract information, interact with web applications, and utilize service APIs. Users can easily install copilots, start chats, save prompts, and toggle the copilot on or off. The project also includes a sample copilot implementation for testing purposes and encourages community contributions to expand the catalog of copilots.
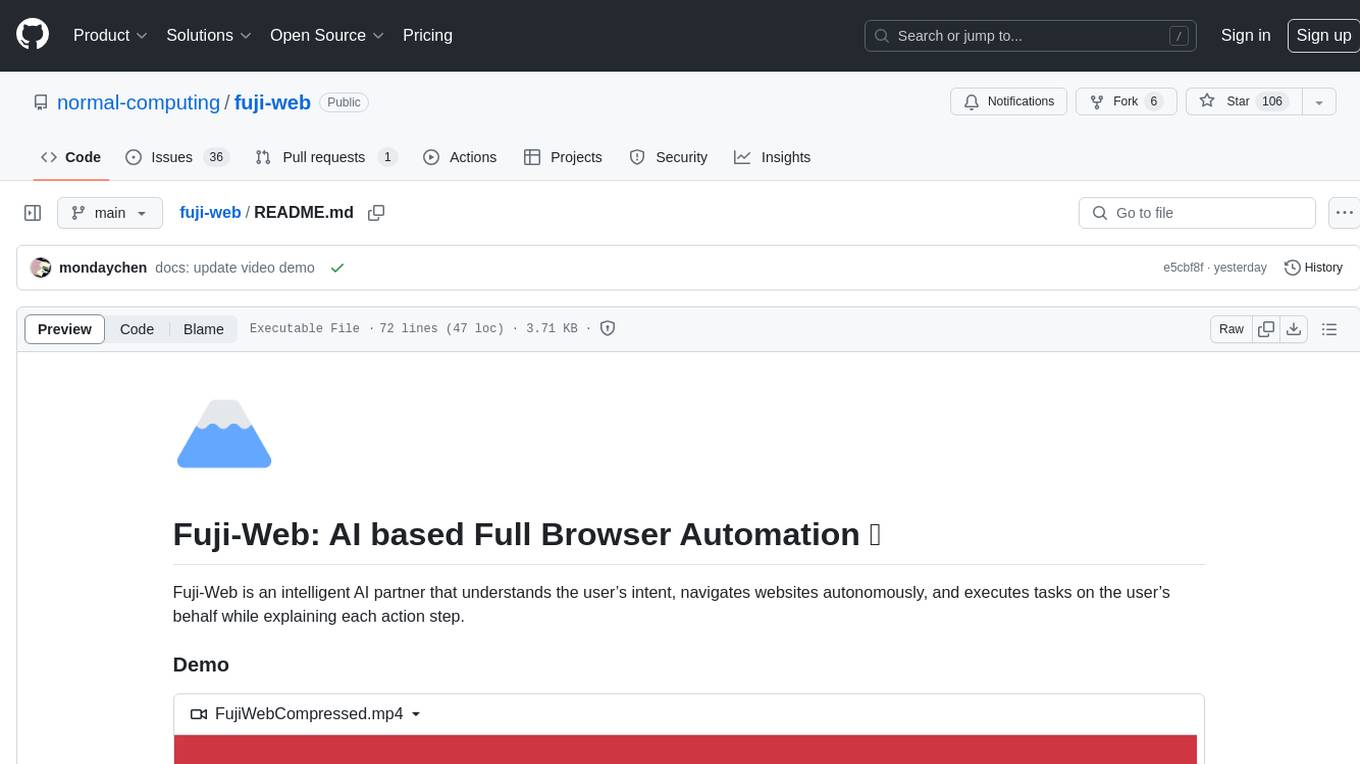
fuji-web
Fuji-Web is an intelligent AI partner designed for full browser automation. It autonomously navigates websites and performs tasks on behalf of the user while providing explanations for each action step. Users can easily install the extension in their browser, access the Fuji icon to input tasks, and interact with the tool to streamline web browsing tasks. The tool aims to enhance user productivity by automating repetitive web actions and providing a seamless browsing experience.
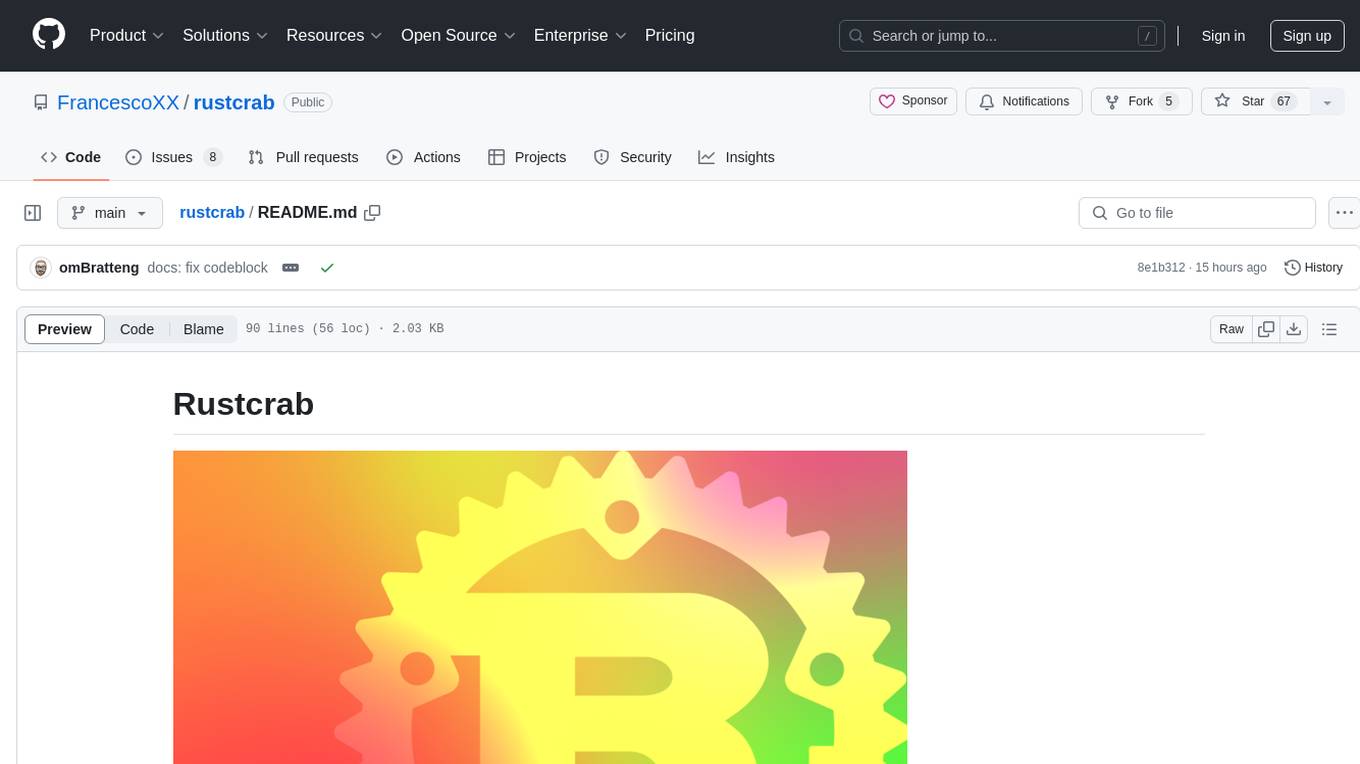
rustcrab
Rustcrab is a repository for Rust developers, offering resources, tools, and guides to enhance Rust programming skills. It is a Next.js application with Tailwind CSS and TypeScript, featuring real-time display of GitHub stars, light/dark mode toggling, integration with daily.dev, and social media links. Users can clone the repository, install dependencies, run the development server, build for production, and deploy to various platforms. Contributions are encouraged through opening issues or submitting pull requests.
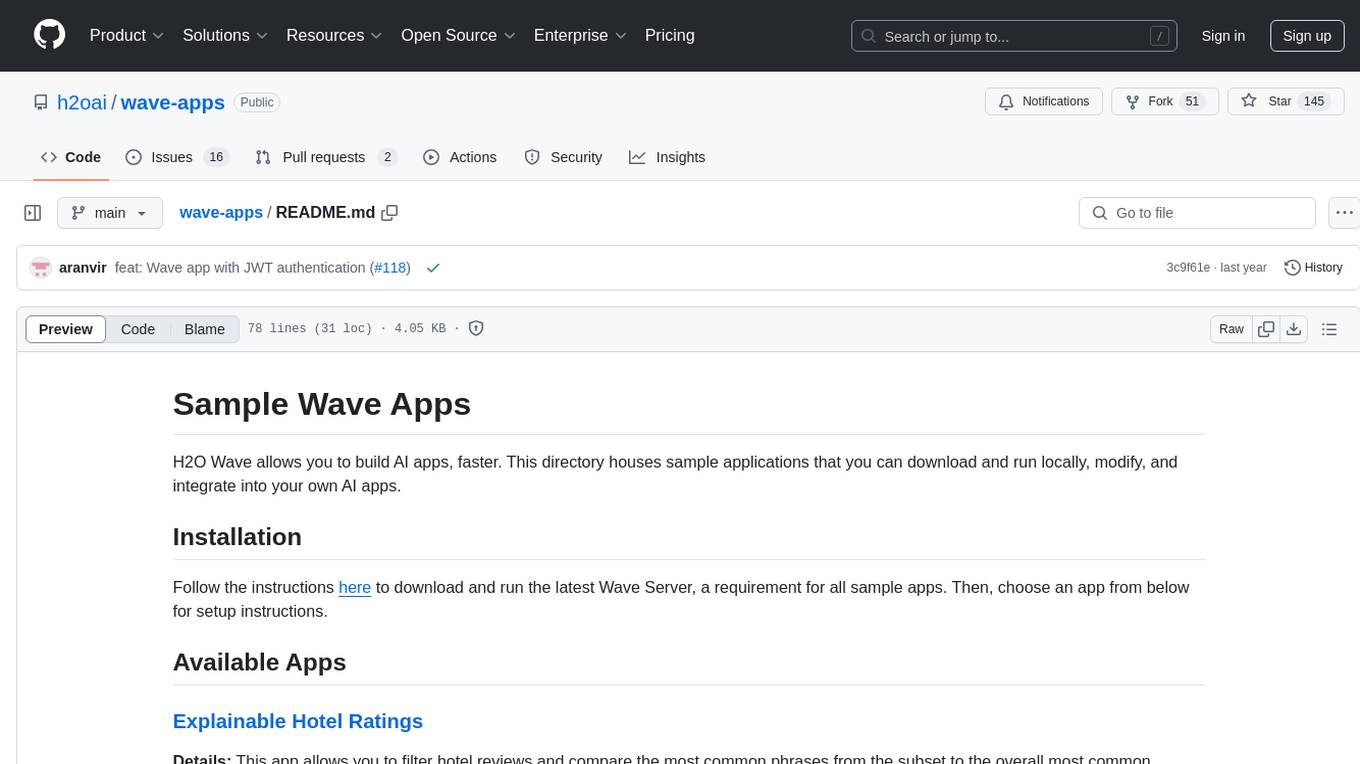
wave-apps
Wave Apps is a directory of sample applications built on H2O Wave, allowing users to build AI apps faster. The apps cover various use cases such as explainable hotel ratings, human-in-the-loop credit risk assessment, mitigating churn risk, online shopping recommendations, and sales forecasting EDA. Users can download, modify, and integrate these sample apps into their own projects to learn about app development and AI model deployment.
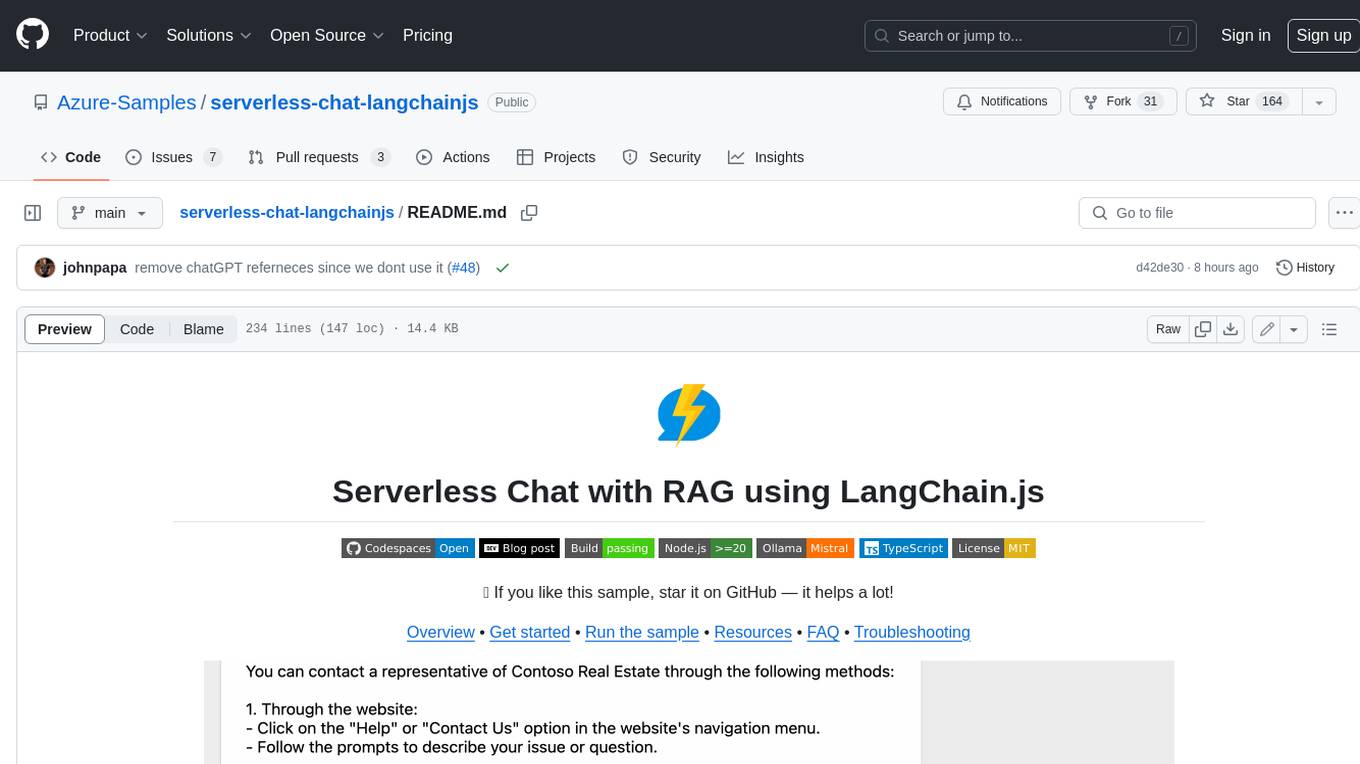
serverless-chat-langchainjs
This sample shows how to build a serverless chat experience with Retrieval-Augmented Generation using LangChain.js and Azure. The application is hosted on Azure Static Web Apps and Azure Functions, with Azure Cosmos DB for MongoDB vCore as the vector database. You can use it as a starting point for building more complex AI applications.
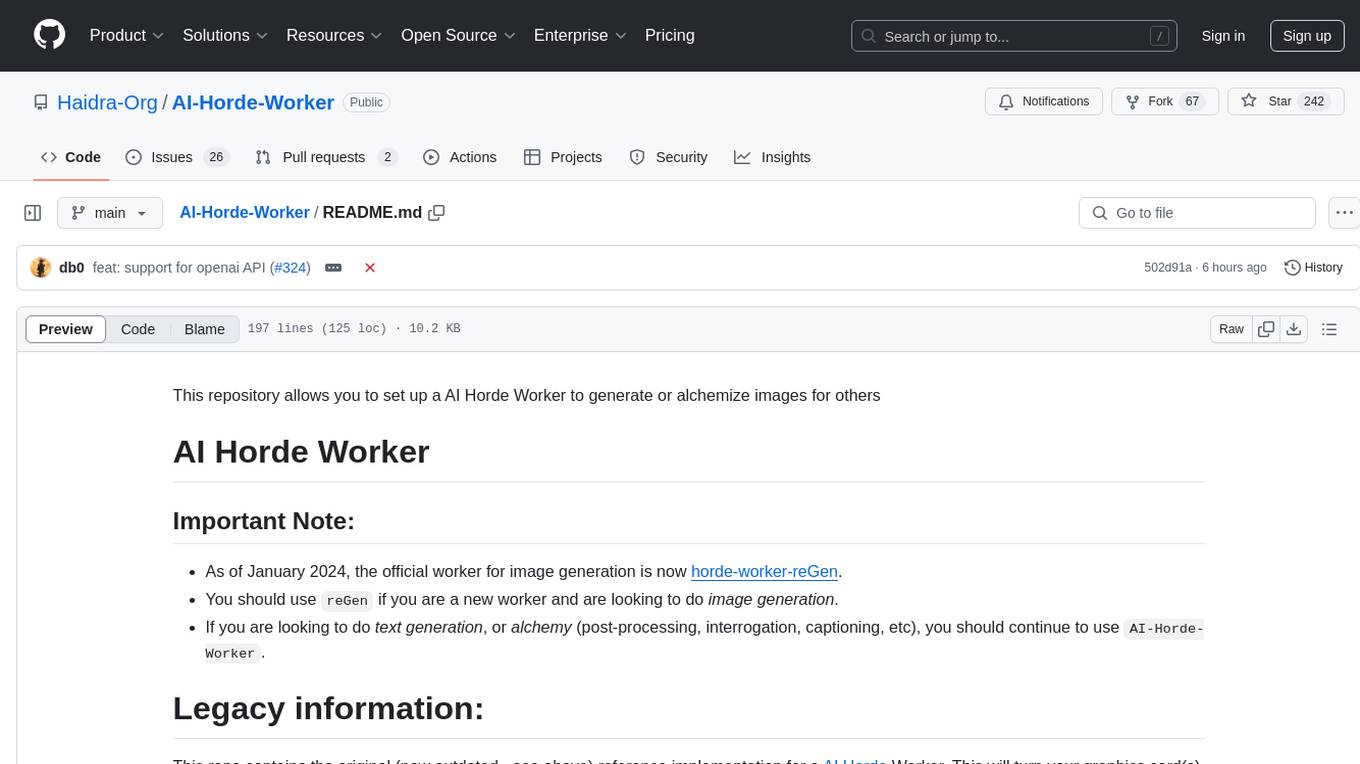
AI-Horde-Worker
AI-Horde-Worker is a repository containing the original reference implementation for a worker that turns your graphics card(s) into a worker for the AI Horde. It allows users to generate or alchemize images for others. The repository provides instructions for setting up the worker on Windows and Linux, updating the worker code, running with multiple GPUs, and stopping the worker. Users can configure the worker using a WebUI to connect to the horde with their username and API key. The repository also includes information on model usage and running the Docker container with specified environment variables.
For similar tasks
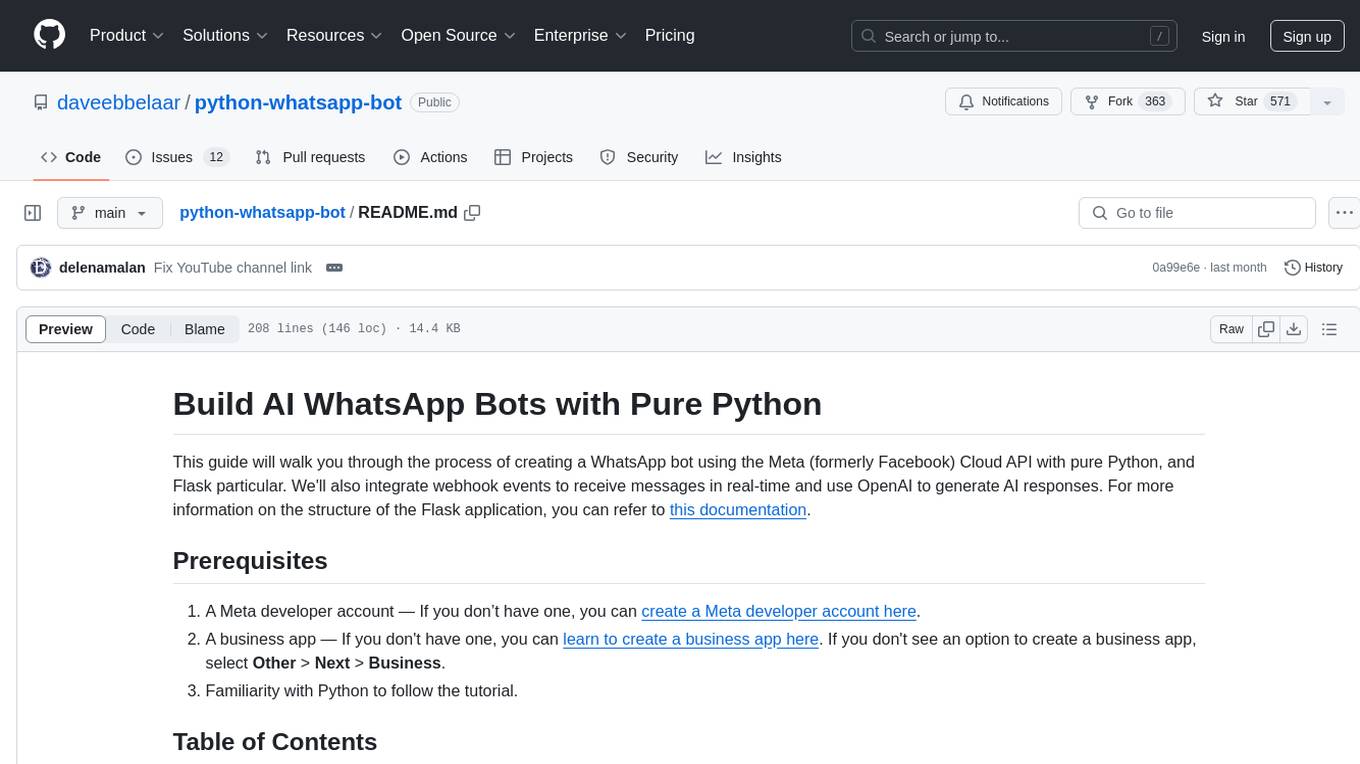
python-whatsapp-bot
This repository provides a comprehensive guide on building AI WhatsApp bots using Python and Flask. It covers setting up a Meta developer account, integrating webhook events for real-time message reception, and using OpenAI for AI responses. The tutorial includes steps for selecting phone numbers, sending messages with the API, configuring webhooks, integrating AI into the application, and adding a phone number. It also explains the process of creating a system user, obtaining access tokens, and validating verification requests and payloads for webhook security. The repository aims to help users create intelligent WhatsApp bots with Python and AI capabilities.
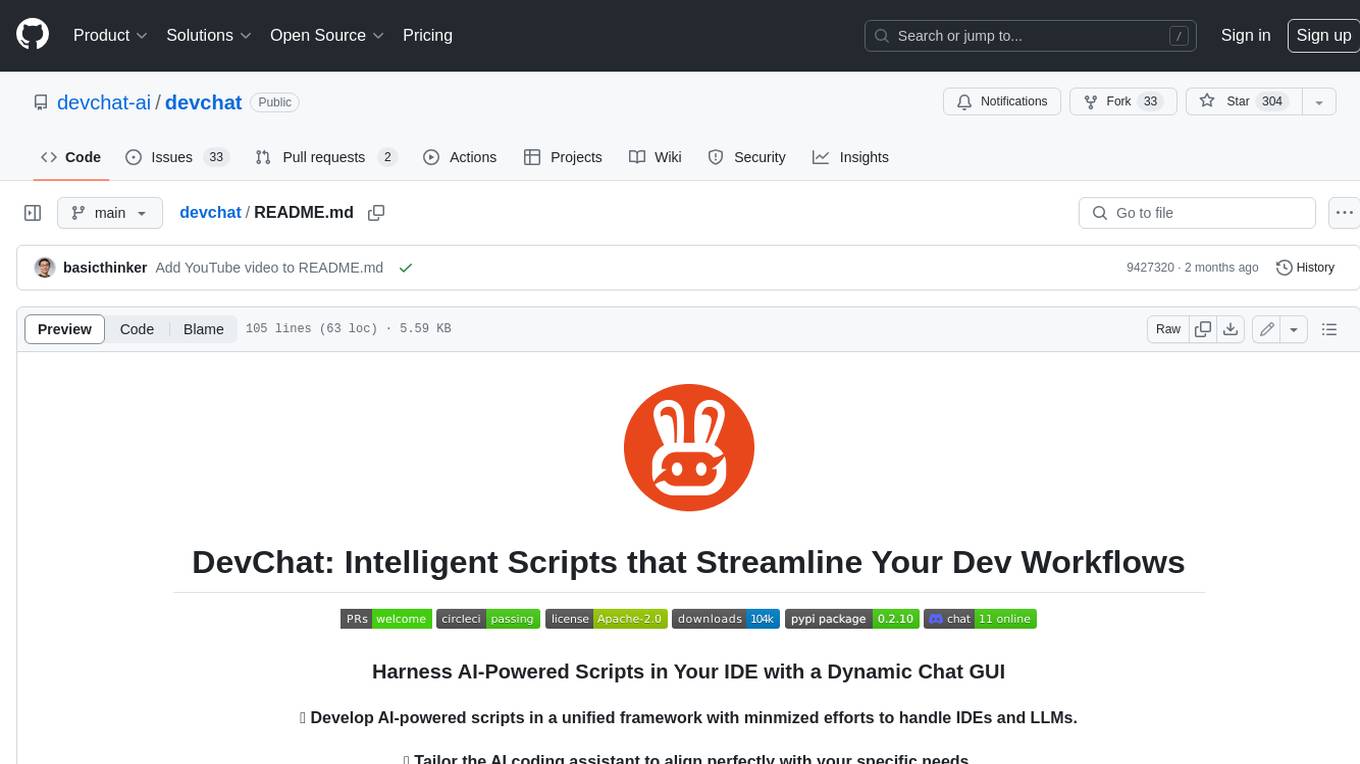
devchat
DevChat is an open-source workflow engine that enables developers to create intelligent, automated workflows for engaging with users through a chat panel within their IDEs. It combines script writing flexibility, latest AI models, and an intuitive chat GUI to enhance user experience and productivity. DevChat simplifies the integration of AI in software development, unlocking new possibilities for developers.
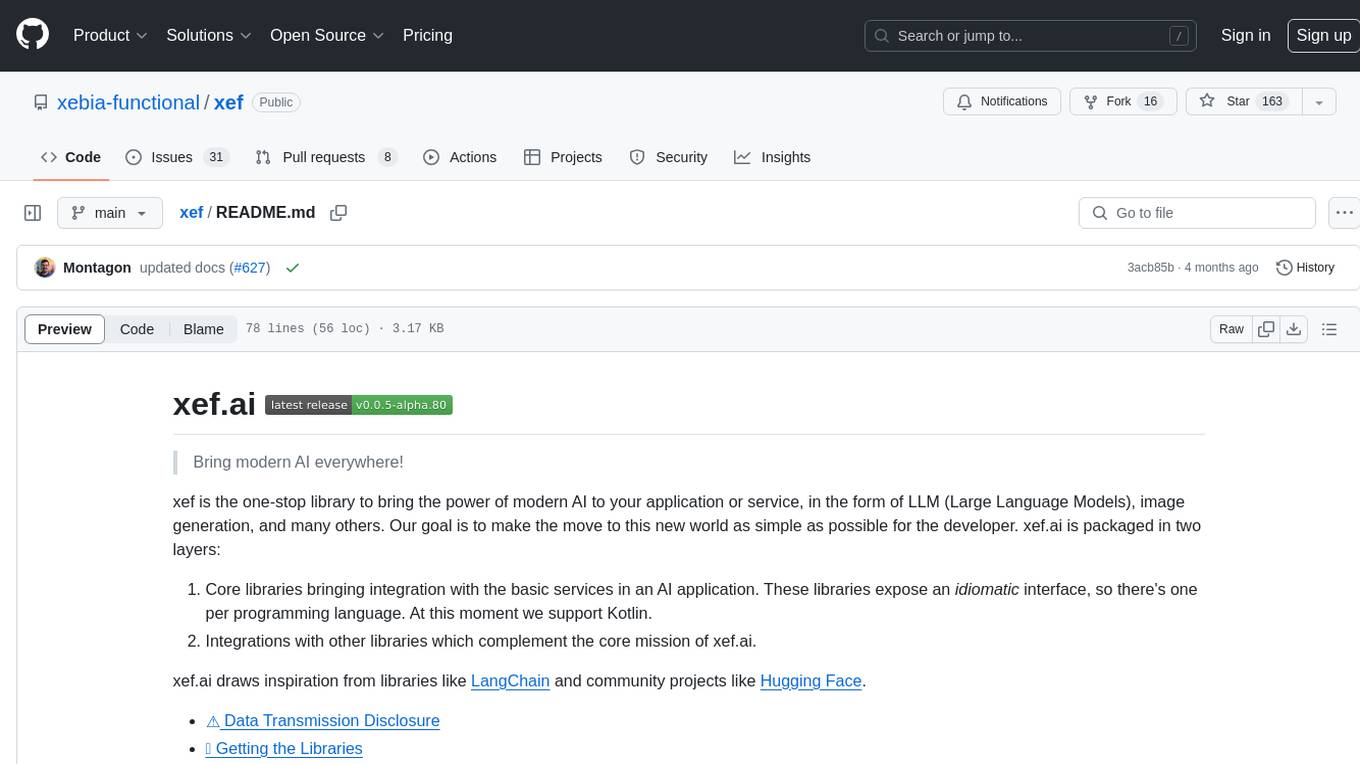
xef
xef.ai is a one-stop library designed to bring the power of modern AI to applications and services. It offers integration with Large Language Models (LLM), image generation, and other AI services. The library is packaged in two layers: core libraries for basic AI services integration and integrations with other libraries. xef.ai aims to simplify the transition to modern AI for developers by providing an idiomatic interface, currently supporting Kotlin. Inspired by LangChain and Hugging Face, xef.ai may transmit source code and user input data to third-party services, so users should review privacy policies and take precautions. Libraries are available in Maven Central under the `com.xebia` group, with `xef-core` as the core library. Developers can add these libraries to their projects and explore examples to understand usage.
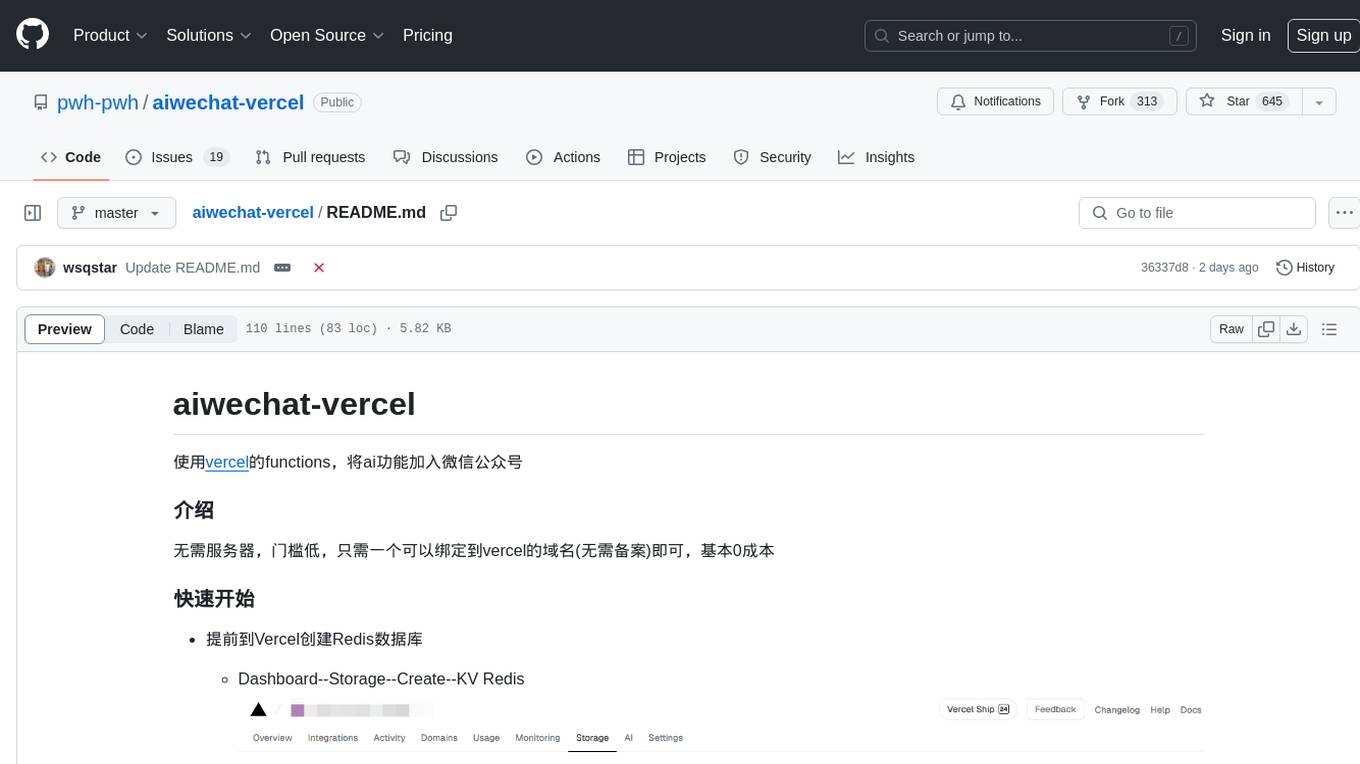
aiwechat-vercel
aiwechat-vercel is a tool that integrates AI capabilities into WeChat public accounts using Vercel functions. It requires minimal server setup, low entry barriers, and only needs a domain name that can be bound to Vercel, with almost zero cost. The tool supports various AI models, continuous Q&A sessions, chat functionality, system prompts, and custom commands. It aims to provide a platform for learning and experimentation with AI integration in WeChat public accounts.
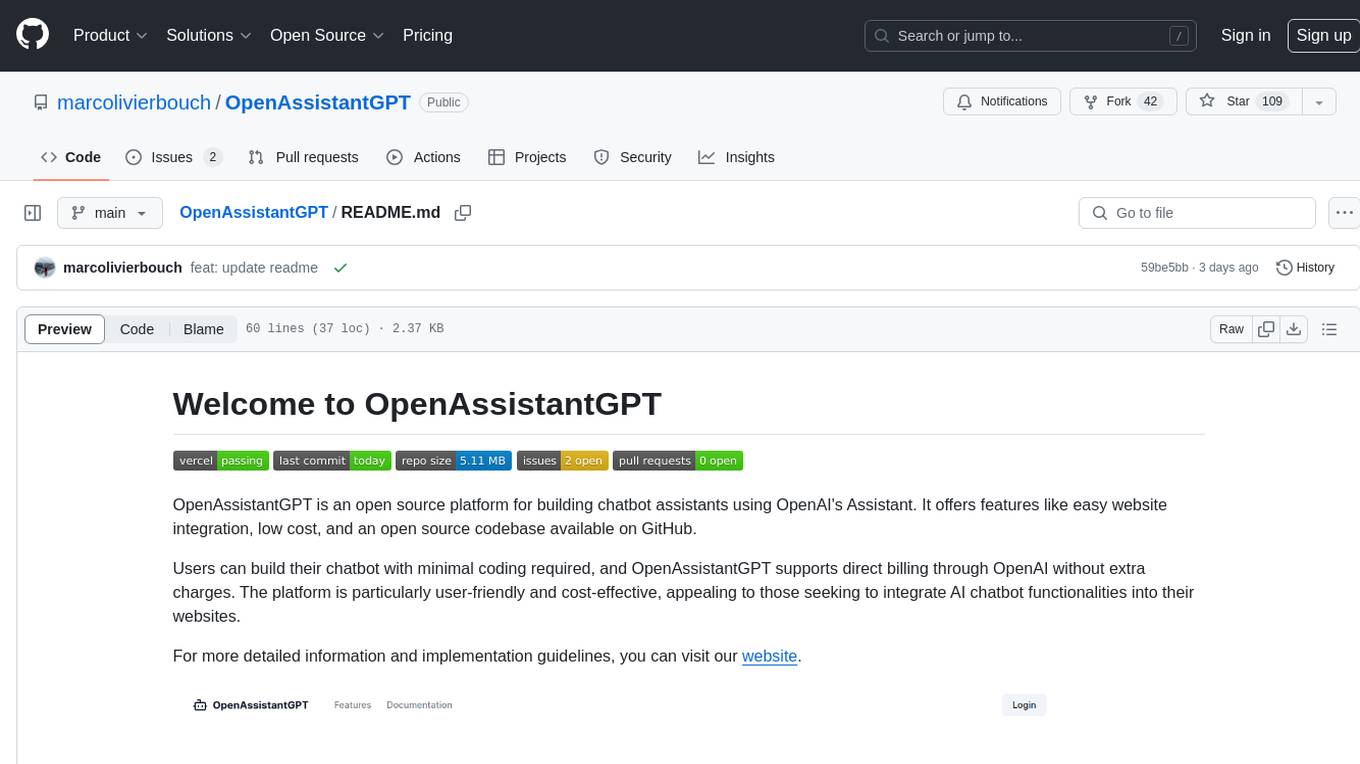
OpenAssistantGPT
OpenAssistantGPT is an open source platform for building chatbot assistants using OpenAI's Assistant. It offers features like easy website integration, low cost, and an open source codebase available on GitHub. Users can build their chatbot with minimal coding required, and OpenAssistantGPT supports direct billing through OpenAI without extra charges. The platform is user-friendly and cost-effective, appealing to those seeking to integrate AI chatbot functionalities into their websites.
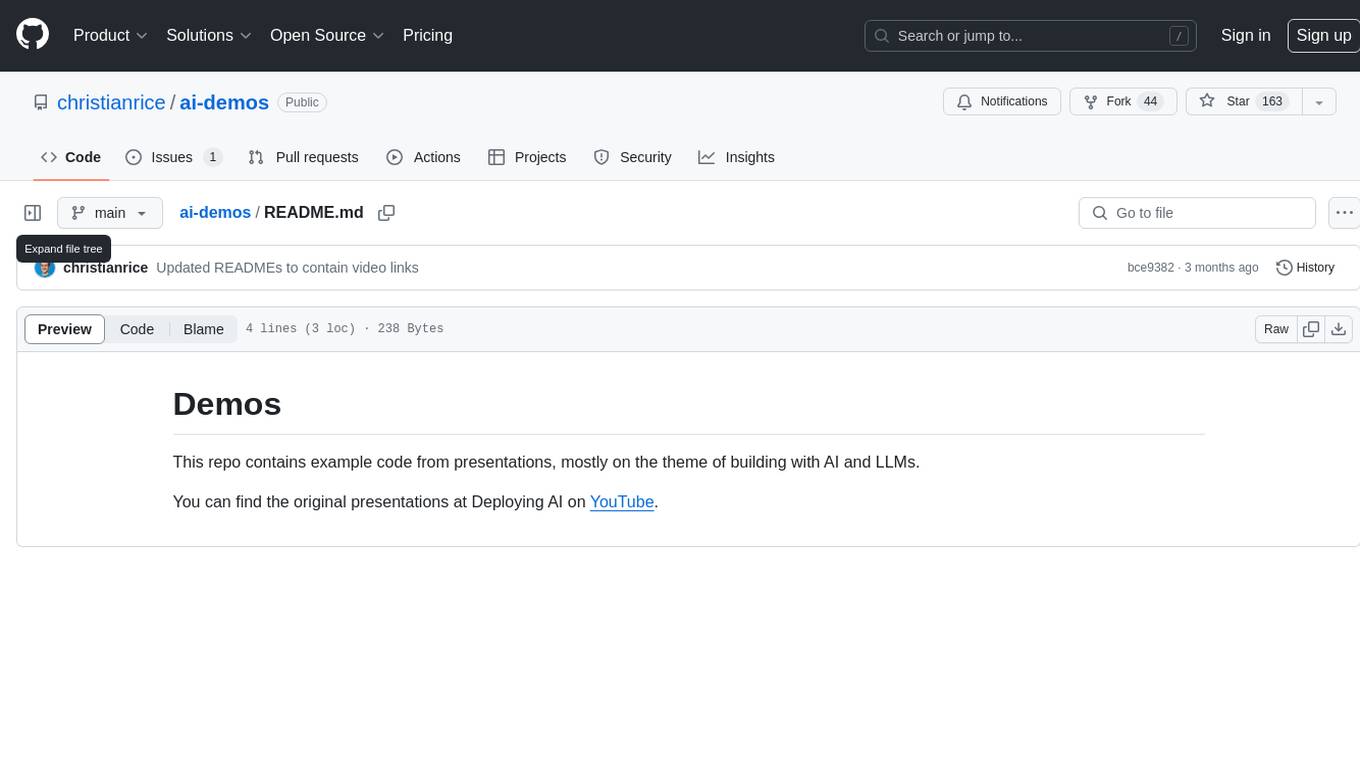
ai-demos
The 'ai-demos' repository is a collection of example code from presentations focusing on building with AI and LLMs. It serves as a resource for developers looking to explore practical applications of artificial intelligence in their projects. The code snippets showcase various techniques and approaches to leverage AI technologies effectively. The repository aims to inspire and educate developers on integrating AI solutions into their applications.
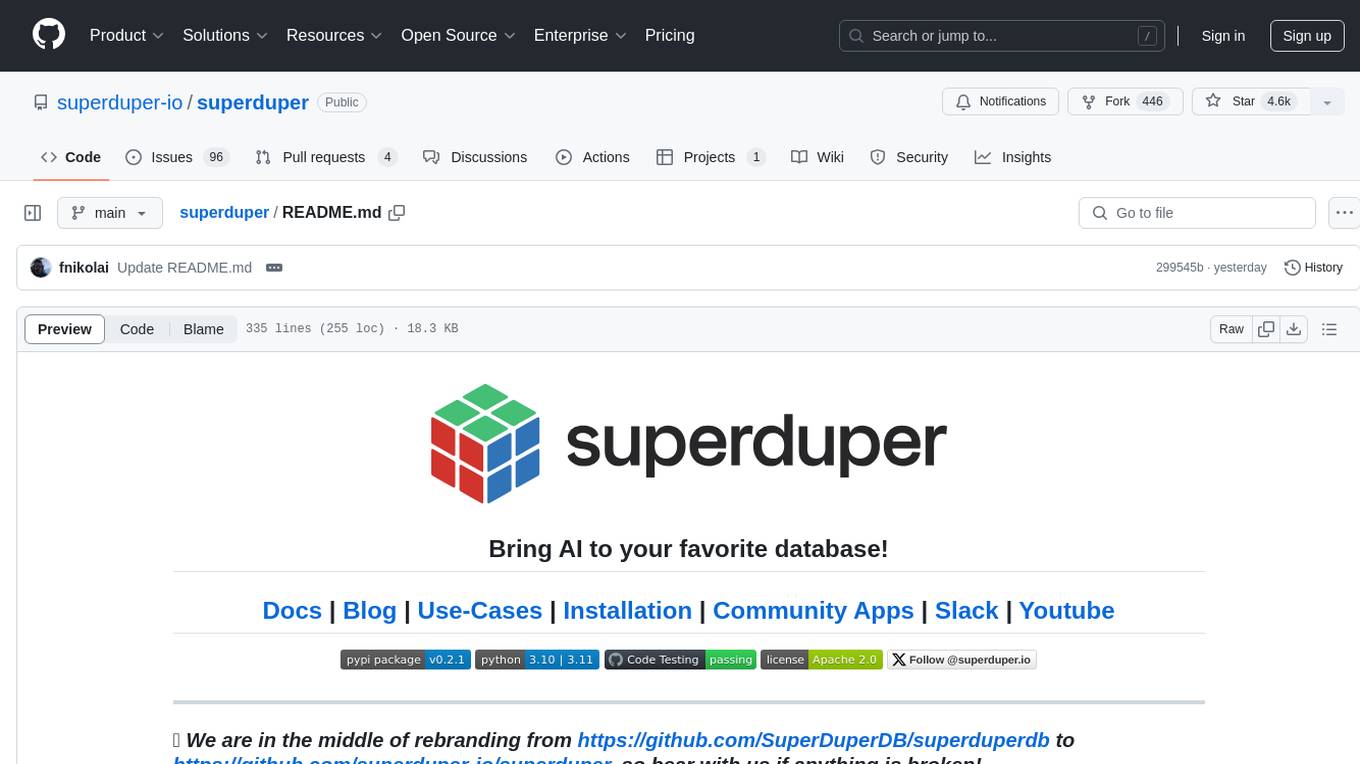
superduper
superduper.io is a Python framework that integrates AI models, APIs, and vector search engines directly with existing databases. It allows hosting of models, streaming inference, and scalable model training/fine-tuning. Key features include integration of AI with data infrastructure, inference via change-data-capture, scalable model training, model chaining, simple Python interface, Python-first approach, working with difficult data types, feature storing, and vector search capabilities. The tool enables users to turn their existing databases into centralized repositories for managing AI model inputs and outputs, as well as conducting vector searches without the need for specialized databases.
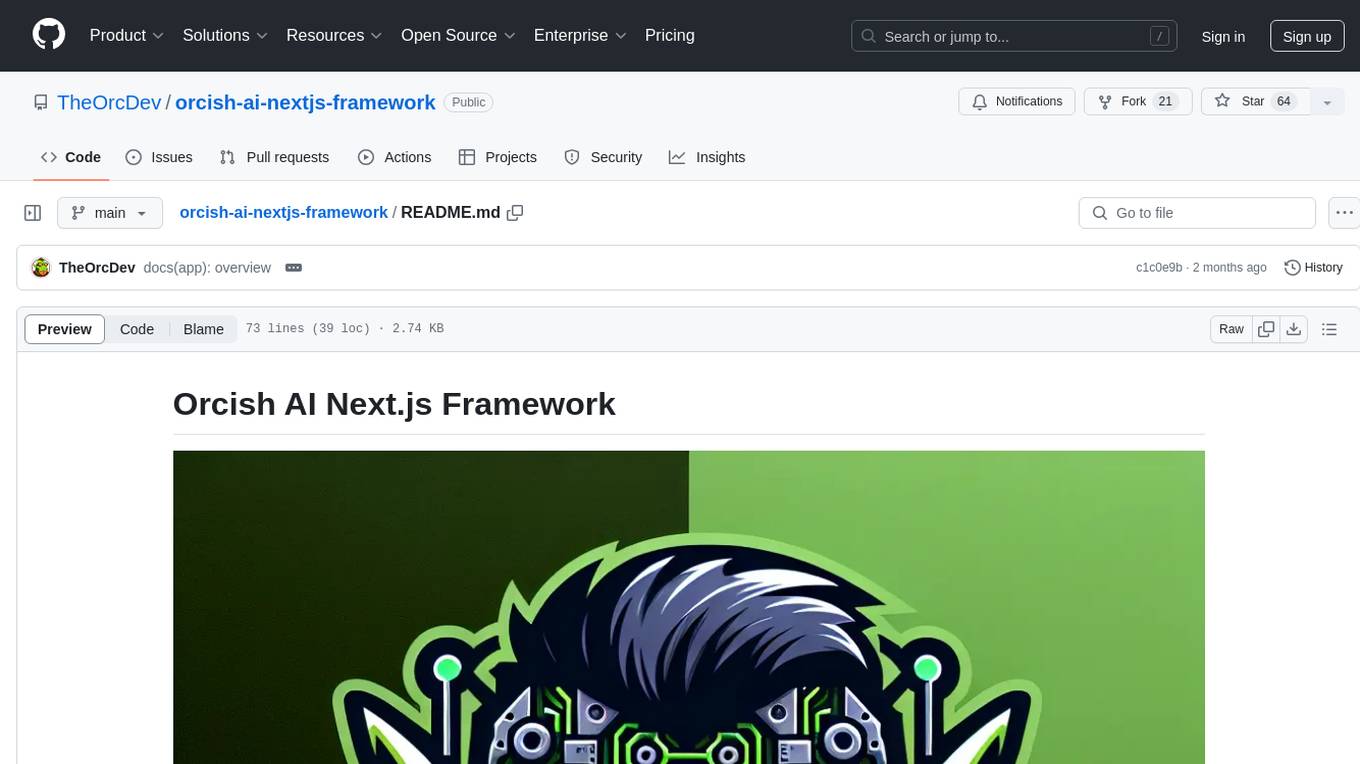
orcish-ai-nextjs-framework
The Orcish AI Next.js Framework is a powerful tool that leverages OpenAI API to seamlessly integrate AI functionalities into Next.js applications. It allows users to generate text, images, and text-to-speech based on specified input. The framework provides an easy-to-use interface for utilizing AI capabilities in application development.
For similar jobs
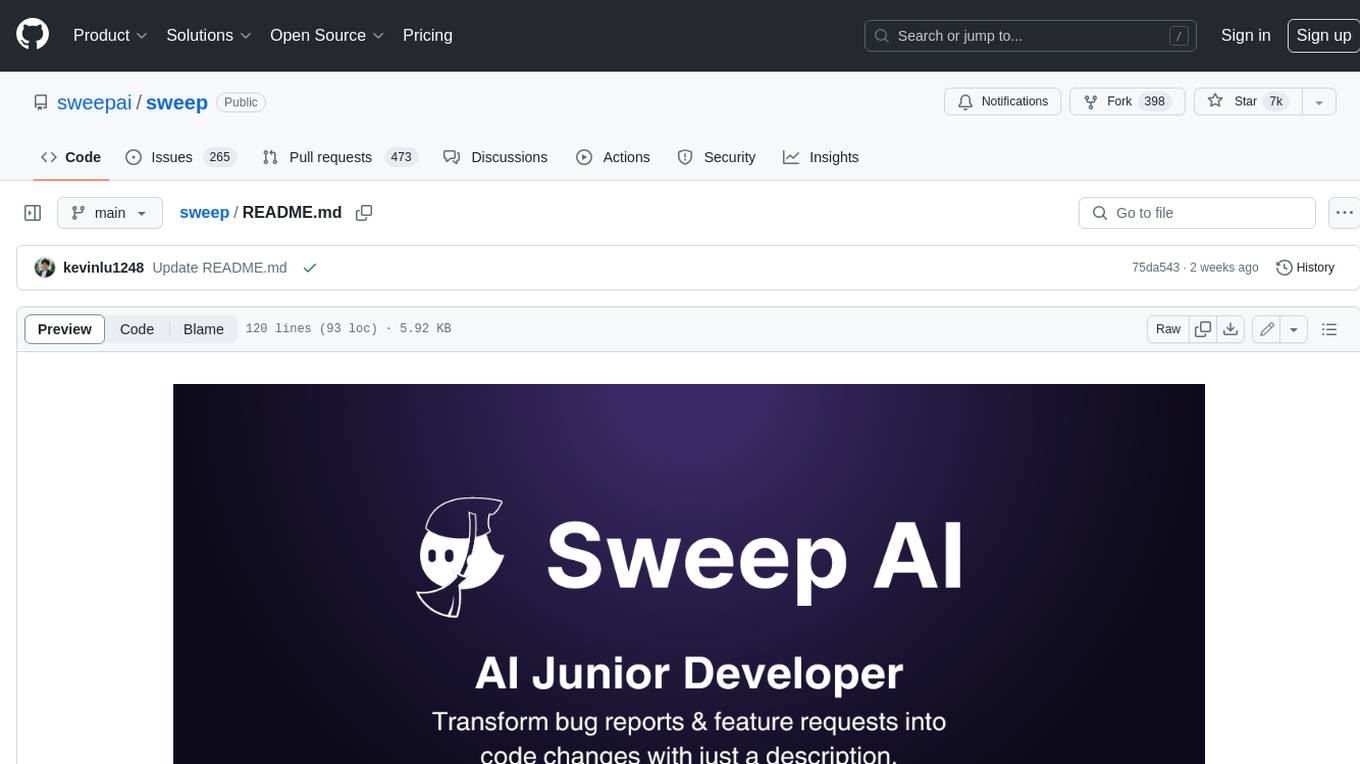
sweep
Sweep is an AI junior developer that turns bugs and feature requests into code changes. It automatically handles developer experience improvements like adding type hints and improving test coverage.
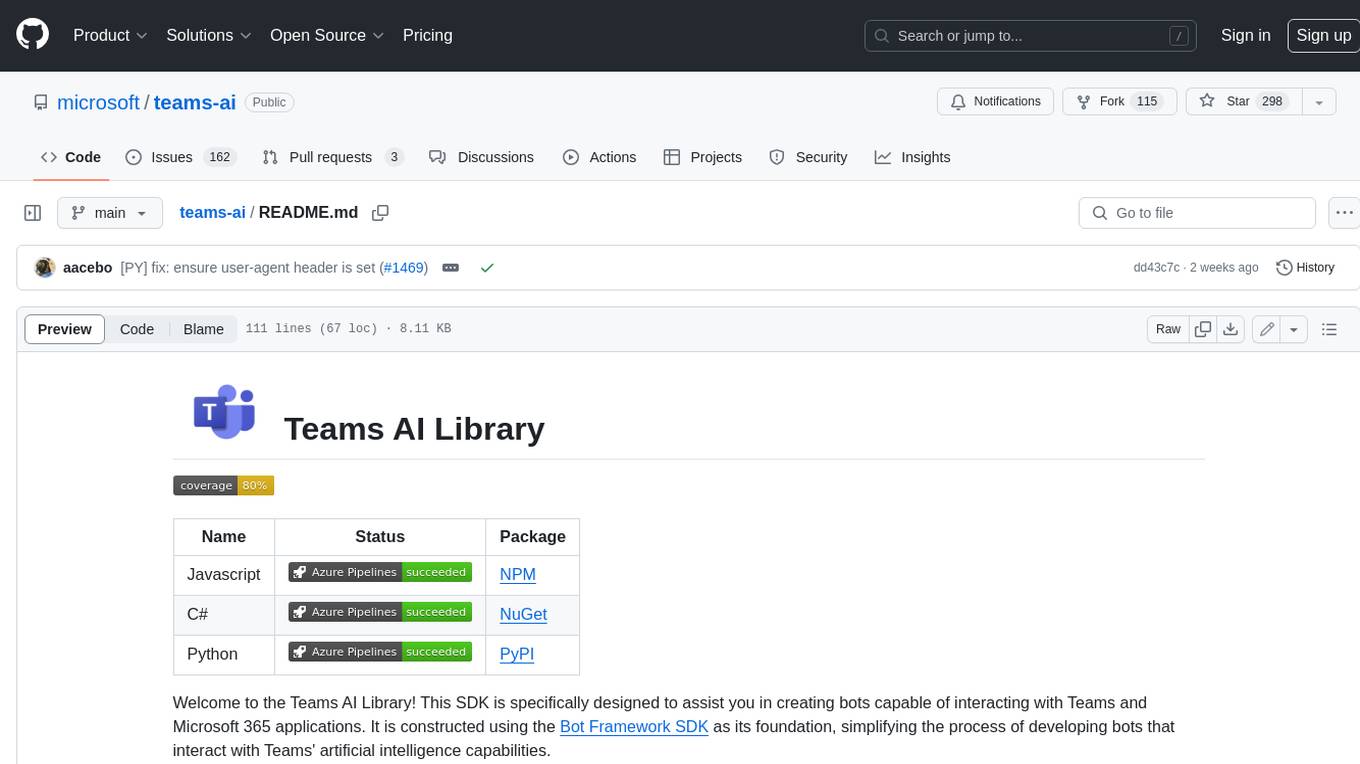
teams-ai
The Teams AI Library is a software development kit (SDK) that helps developers create bots that can interact with Teams and Microsoft 365 applications. It is built on top of the Bot Framework SDK and simplifies the process of developing bots that interact with Teams' artificial intelligence capabilities. The SDK is available for JavaScript/TypeScript, .NET, and Python.
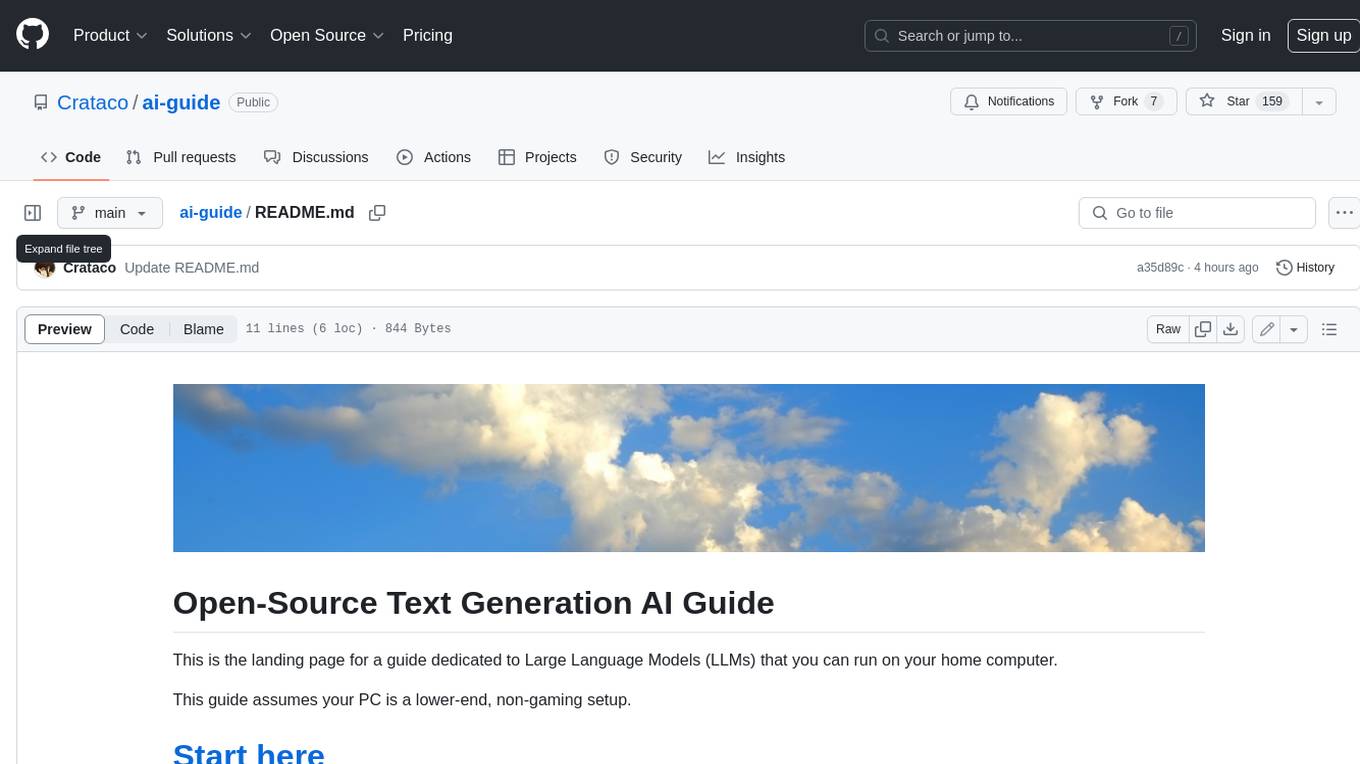
ai-guide
This guide is dedicated to Large Language Models (LLMs) that you can run on your home computer. It assumes your PC is a lower-end, non-gaming setup.
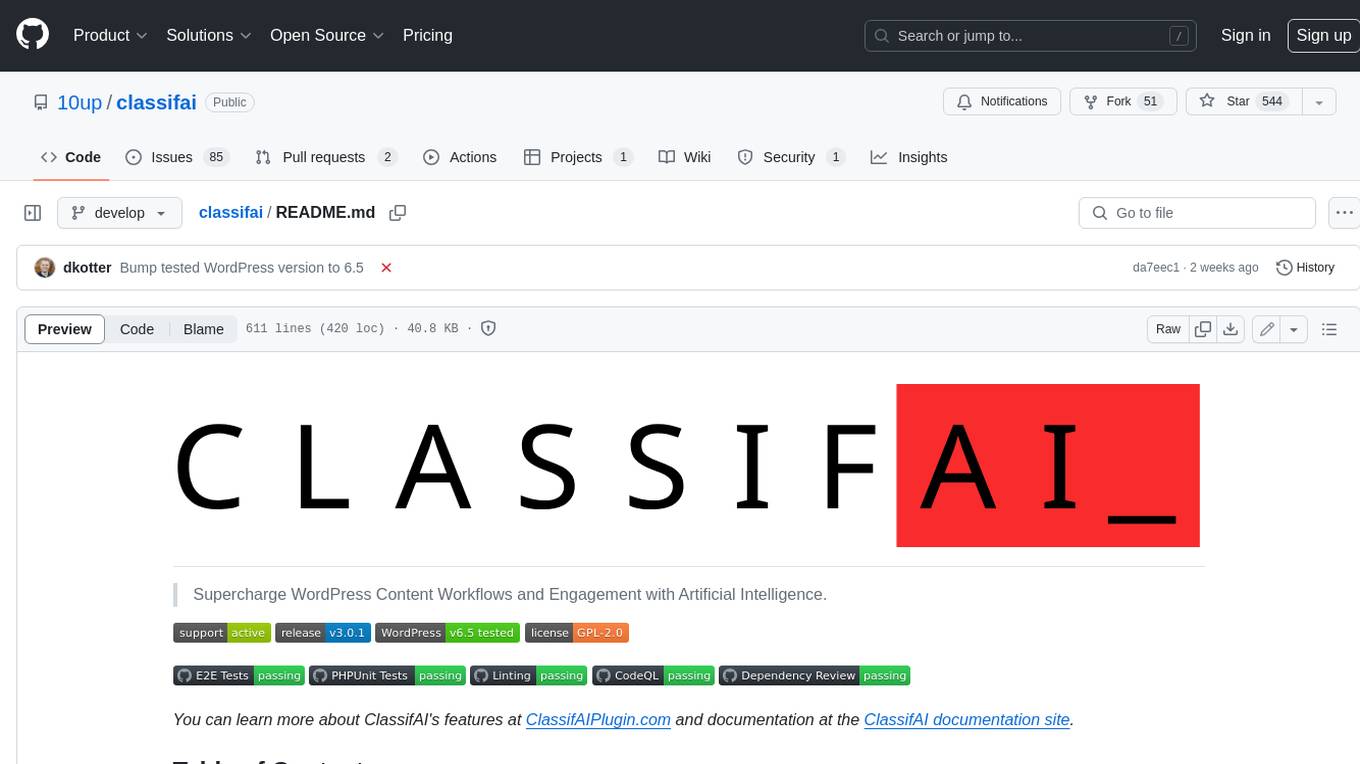
classifai
Supercharge WordPress Content Workflows and Engagement with Artificial Intelligence. Tap into leading cloud-based services like OpenAI, Microsoft Azure AI, Google Gemini and IBM Watson to augment your WordPress-powered websites. Publish content faster while improving SEO performance and increasing audience engagement. ClassifAI integrates Artificial Intelligence and Machine Learning technologies to lighten your workload and eliminate tedious tasks, giving you more time to create original content that matters.
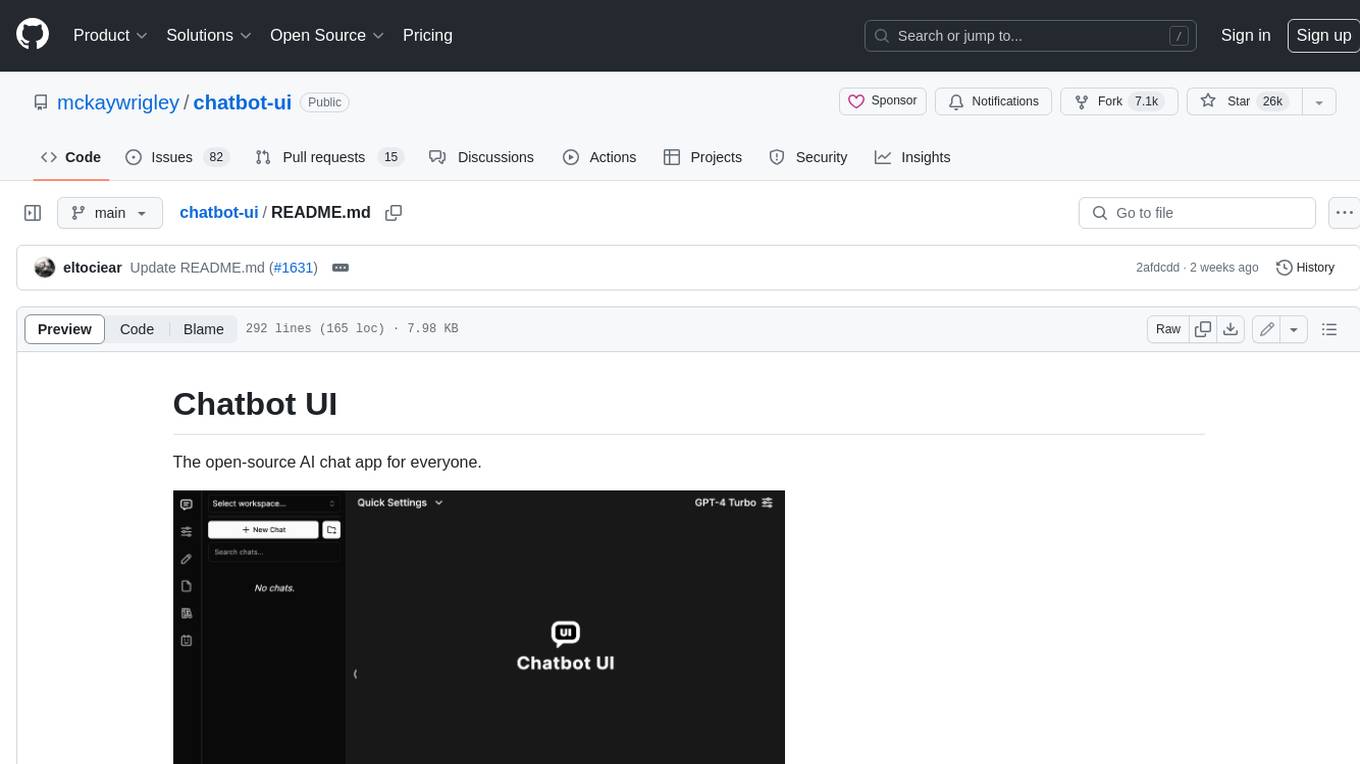
chatbot-ui
Chatbot UI is an open-source AI chat app that allows users to create and deploy their own AI chatbots. It is easy to use and can be customized to fit any need. Chatbot UI is perfect for businesses, developers, and anyone who wants to create a chatbot.
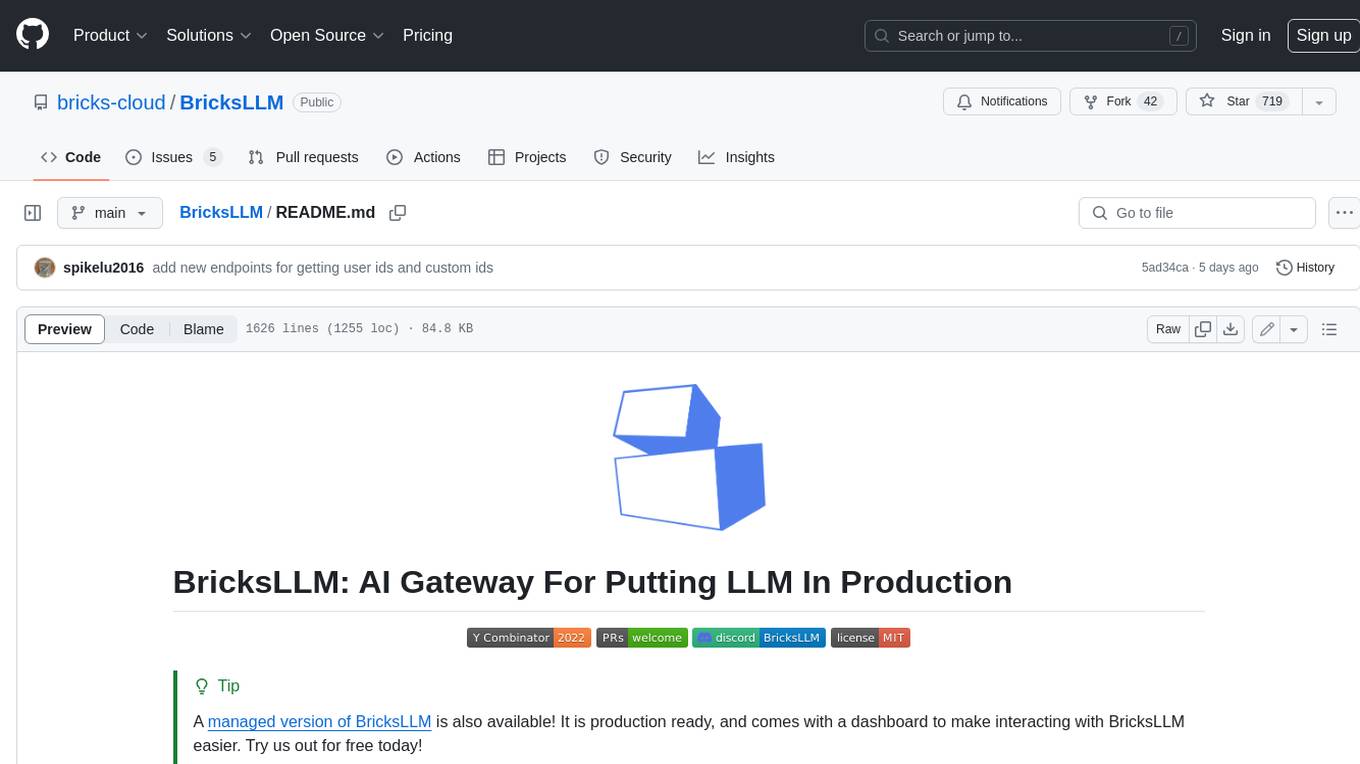
BricksLLM
BricksLLM is a cloud native AI gateway written in Go. Currently, it provides native support for OpenAI, Anthropic, Azure OpenAI and vLLM. BricksLLM aims to provide enterprise level infrastructure that can power any LLM production use cases. Here are some use cases for BricksLLM: * Set LLM usage limits for users on different pricing tiers * Track LLM usage on a per user and per organization basis * Block or redact requests containing PIIs * Improve LLM reliability with failovers, retries and caching * Distribute API keys with rate limits and cost limits for internal development/production use cases * Distribute API keys with rate limits and cost limits for students
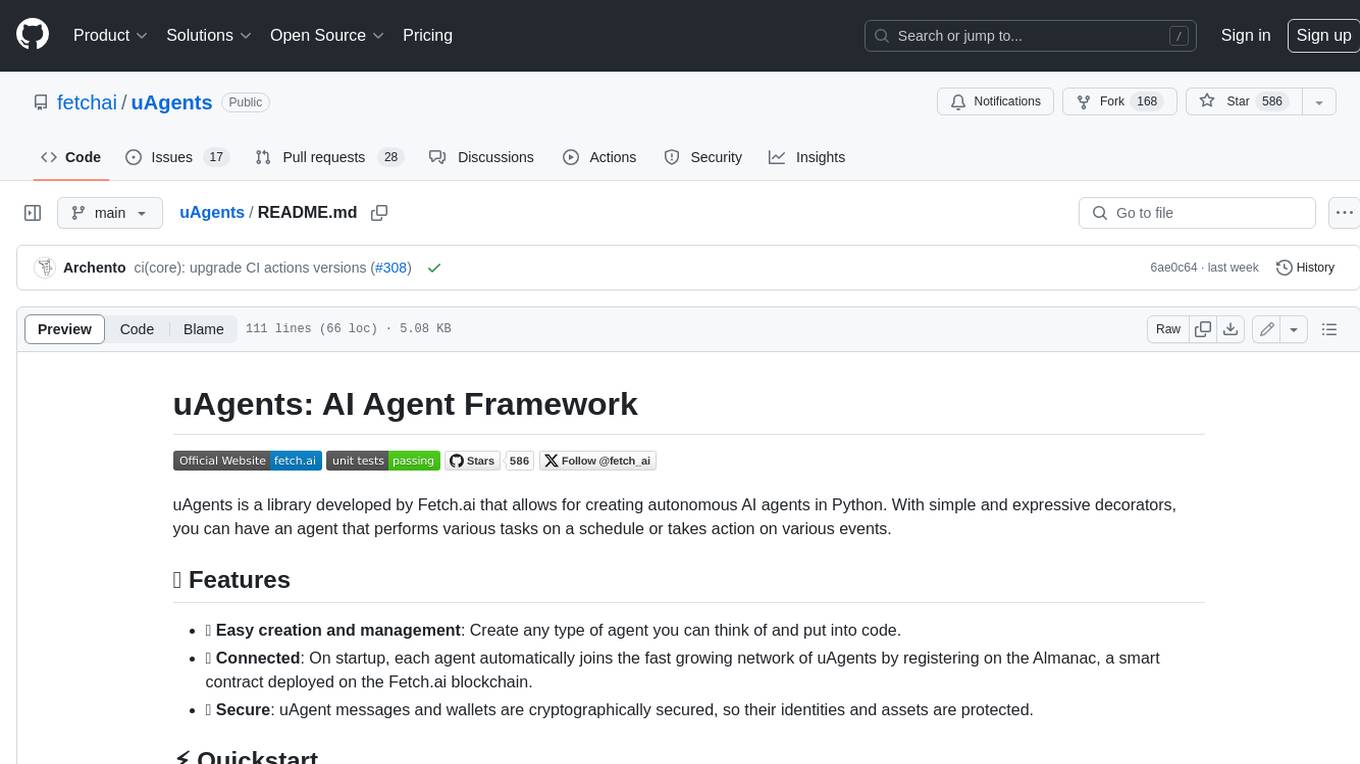
uAgents
uAgents is a Python library developed by Fetch.ai that allows for the creation of autonomous AI agents. These agents can perform various tasks on a schedule or take action on various events. uAgents are easy to create and manage, and they are connected to a fast-growing network of other uAgents. They are also secure, with cryptographically secured messages and wallets.
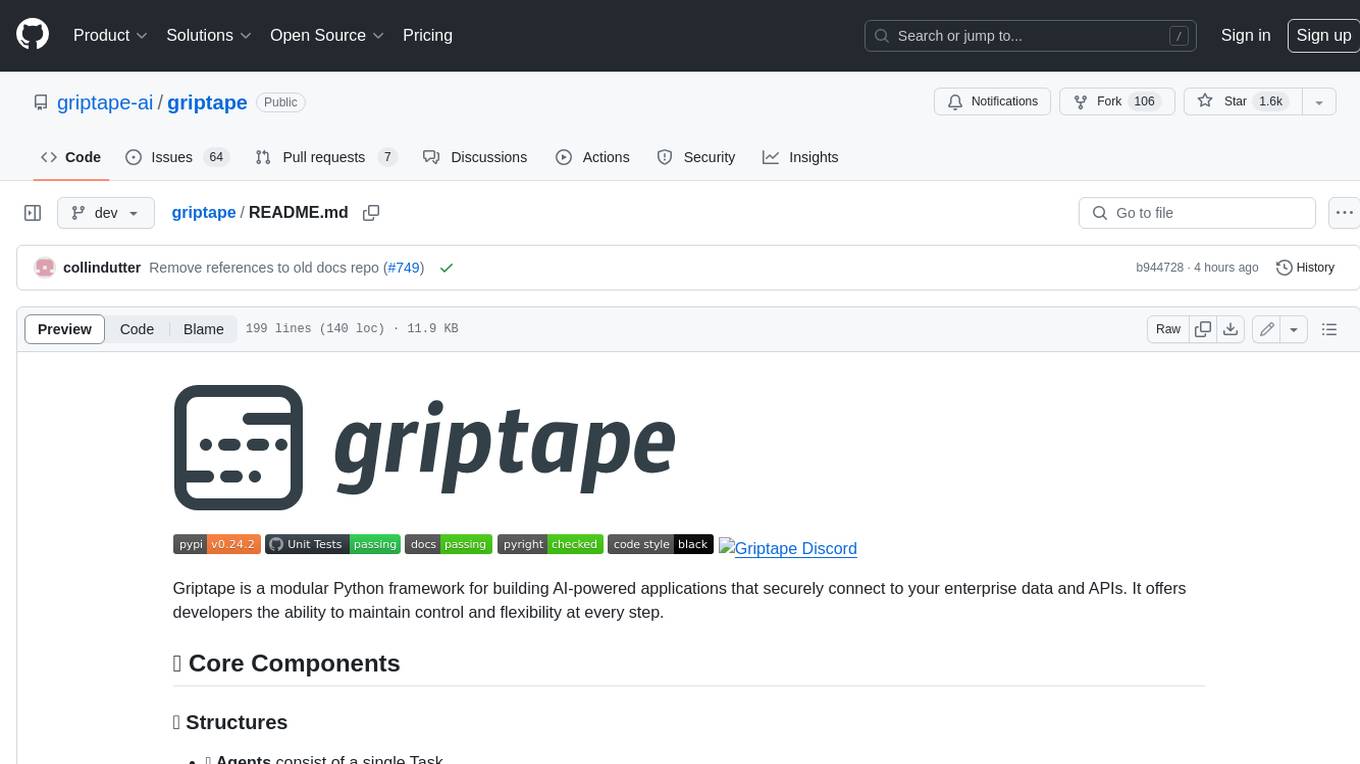
griptape
Griptape is a modular Python framework for building AI-powered applications that securely connect to your enterprise data and APIs. It offers developers the ability to maintain control and flexibility at every step. Griptape's core components include Structures (Agents, Pipelines, and Workflows), Tasks, Tools, Memory (Conversation Memory, Task Memory, and Meta Memory), Drivers (Prompt and Embedding Drivers, Vector Store Drivers, Image Generation Drivers, Image Query Drivers, SQL Drivers, Web Scraper Drivers, and Conversation Memory Drivers), Engines (Query Engines, Extraction Engines, Summary Engines, Image Generation Engines, and Image Query Engines), and additional components (Rulesets, Loaders, Artifacts, Chunkers, and Tokenizers). Griptape enables developers to create AI-powered applications with ease and efficiency.