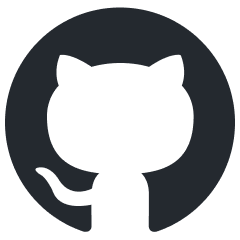
cheating-based-prompt-engine
AI engine for smart contract audit
Stars: 185
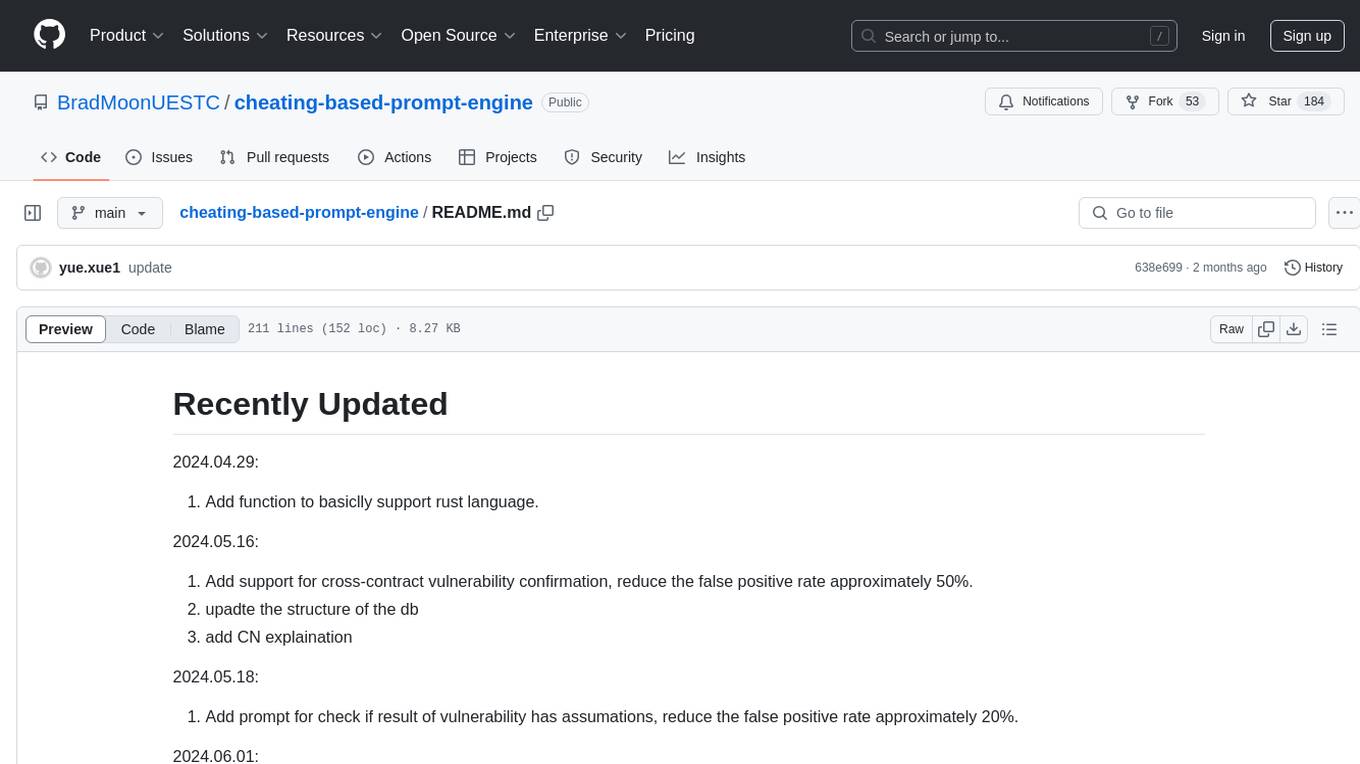
This is a vulnerability mining engine purely based on GPT, requiring no prior knowledge base, no fine-tuning, yet its effectiveness can overwhelmingly surpass most of the current related research. The core idea revolves around being task-driven, not question-driven, driven by prompts, not by code, and focused on prompt design, not model design. The essence is encapsulated in one word: deception. It is a type of code understanding logic vulnerability mining that fully stimulates the capabilities of GPT, suitable for real actual projects.
README:
2024.04.29:
- Add function to basiclly support rust language.
2024.05.16:
- Add support for cross-contract vulnerability confirmation, reduce the false positive rate approximately 50%.
- upadte the structure of the db
- add CN explaination
2024.05.18:
- Add prompt for check if result of vulnerability has assumations, reduce the false positive rate approximately 20%.
2024.06.01:
- Add support for python language, dont ask me why, so annoying.
2024.07.01
- Update the license
2024.07.23
- Add support for cairo, move
2024.08.01
- Add support for func, tact
2024.08.02 Inspired by the paper https://arxiv.org/abs/2407.21787v1, the project was renamed to finite-money-prompt-engine on August 2, 2024.
- Optimize code structure
- Add more language support
- Write usage documentation and code analysis
- Add command line mode for easy use
审计赏金成果:截止2024年5月,此工具已获得$60000+
Audit bounty results: As of May 2024, this tool has received $60,000+
- 优化代码结构
- 增加更多语言支持
- 编写使用文档和代码解析
- 增加命令行模式,方便使用
This is a vulnerability mining engine purely based on GPT, requiring no prior knowledge base, no fine-tuning, yet its effectiveness can overwhelmingly surpass most of the current related research.
The key lies in the design of prompts, which has shown excellent results. The core idea revolves around:
- Being task-driven, not question-driven.
- Driven by prompts, not by code.
- Focused on prompt design, not model design.
The essence is encapsulated in one word: "deception."
- This is a type of code understanding logic vulnerability mining that fully stimulates the capabilities of gpt. The control flow type vulnerability detection ability is ineffective and is suitable for real actual projects.
- Therefore, don’t run tests on meaningless academic vulnerabilities
Here's the translation into English:
Test Environment Setup
-
In the
src/main.py
file, setswitch_production_or_test
totest
to configure the environment in test mode. -
Place the project under the directory
src/dataset/agent-v1-c4
. This structure is crucial for proper tool positioning and interaction with data. -
Refer to the configuration file
src/dataset/agent-v1-c4/datasets.json
to set up your project collection. For example:
"StEverVault2":{
"path":"StEverVault",
"files":[
],
"functions":[]
}
Where StEverVault2
represents the custom name of the project, matching the project_id
in src/main.py
. path
refers to the actual path of the project under agent-v1-c4
. files
specifies the contract files to be scanned; if not configured, it defaults to scanning all files. functions
specifies the specific function names to be scanned; if not configured, it defaults to scanning all functions, in the format [contract_name.function_name]
.
-
Use
src/db.sql
to create the database; PostgreSQL needs to be installed beforehand. -
Set up the
.env
file by creating it and filling in the following details to configure your environment:
# Database connection information
DATABASE_URL=postgresql://postgres:[email protected]:5432/postgres
# OpenAI API
OPENAI_API_BASE="apix.ai-gaochao.cn"
OPENAI_API_KEY=xxxxxx
# Model IDs
BUSINESS_FLOW_MODEL_ID=gpt-4-turbo
VUL_MODEL_ID=gpt-4-turbo
# Business flow scanning parameters
BUSINESS_FLOW_COUNT=10
SWITCH_FUNCTION_CODE=False
SWITCH_BUSINESS_CODE=True
Where:
-
DATABASE_URL
is the database connection information. -
OPENAI_API_BASE
is the GPT API connection information, usuallyapi.openai.com
. -
OPENAI_API_KEY
should be set to your actual OpenAI API key. -
BUSINESS_FLOW_MODEL_ID
andVUL_MODEL_ID
are the IDs of the models used, recommended to usegpt-4-turbo
. -
BUSINESS_FLOW_COUNT
is the number of randomizations used to create variability, typically 7-20, commonly 10. -
SWITCH_FUNCTION_CODE
andSWITCH_BUSINESS_CODE
are the granularity settings during scanning, supporting function-level and business flow-level granularity.
- After configuring, run
main.py
to start the scanning process.
这是一个纯基于gpt的漏洞挖掘引擎,不需要任何前置知识库,不需要任何fine-tuning,但效果足可以碾压当前大部分相关研究的效果
核心关键在于prompt的设计,效果非常好
核心思路:
- task driven, not question driven
- 关键一个字在于“骗”
- 利用幻觉,喜欢幻觉
- 这是一种充分激发gpt能力的代码理解型的逻辑漏洞挖掘,控制流类型的漏洞检测能力效果差,适用于真正的实际项目
- 因此,不要拿那些无意义的学术型漏洞来跑测试
测试环境设置如下:
- 在
src/main.py
文件中,将switch_production_or_test
设置为test
,以配置环境为测试模式。
if __name__ == '__main__':
switch_production_or_test = 'test' # prod / test
if switch_production_or_test == 'test':
# Your code for test environment
-
将项目放置于
src/dataset/agent-v1-c4
目录下,这一结构对于工具正确定位和与数据交互至关重要。 -
参照
src/dataset/agent-v1-c4/datasets.json
配置文件来设置你的项目集。例如:
"StEverVault2":{
"path":"StEverVault",
"files":[
],
"functions":[]
}
其中,StEverVault2
代表项目自定义名,它的名字与 src/main.py
中的 project_id
相同。path
指代的是 agent-v1-c4
下项目的具体实际路径。files
指代的是要具体扫描的合约文件,如果不配置,则默认扫描全部。functions
指代的是要具体扫描的函数名,如果不配置,则默认扫描全部函数,形式为【合约名.函数名】。
-
使用
src/db.sql
创建数据库,需要提前安装 PostgreSQL。 -
设置
.env
文件,通过创建.env
文件并填写以下内容来配置你的环境:
# 数据库连接信息
DATABASE_URL=postgresql://postgres:[email protected]:5432/postgres
# OpenAI API
OPENAI_API_BASE="apix.ai-gaochao.cn"
OPENAI_API_KEY=xxxxxx
# 模型ID
BUSINESS_FLOW_MODEL_ID=gpt-4-turbo
VUL_MODEL_ID=gpt-4-turbo
# 业务流扫描参数
BUSINESS_FLOW_COUNT=10
SWITCH_FUNCTION_CODE=False
SWITCH_BUSINESS_CODE=True
其中:
-
DATABASE_URL
为数据库连接信息。 -
OPENAI_API_BASE
为 GPT API 连接信息,一般情况下为api.openai.com
。 -
OPENAI_API_KEY
设置为对应的 OpenAI API 密钥。 -
BUSINESS_FLOW_MODEL_ID
和VUL_MODEL_ID
为所使用的模型 ID,建议使用gpt-4-turbo
。 -
BUSINESS_FLOW_COUNT
为利用幻觉造成随机性时设置的随机次数,一般为 7-20,常用 10。 -
SWITCH_FUNCTION_CODE
和SWITCH_BUSINESS_CODE
为扫描时的粒度,支持函数粒度和业务流粒度。
-
配置完成后,运行
main.py
即可开始扫描过程。 -
扫描时可能会因为网络原因或api原因中断,对于此已经整理成随时保存,不修改project_id的情况下可以重新运行main.py,可以继续扫描
-
唯一建议gpt4-turbo,不要用3.5,不要用4o,4o和3.5的推理能力是一样的,拉的一批
-
一般扫描时间为2-3小时,取决于项目大小和随机次数,中型项目+10次随机大约2个半小时
-
中型项目+10次随机大约需要20-30美金成本
-
当前还是有误报,按项目大小,大约30-65%,小项目误报会少一些,且还有很多自定义的东西,后续会继续优化
-
结果做了很多标记和中文解释
-
优先看result列中有【"result":"yes"】的(有时候是"result": "yes",带个空格)
-
category列优先筛选出【dont need In-project other contract】 的
-
具体的代码看business_flow_code列
-
代码位置看name列
- gpt4效果会更好,gpt3尚未深入尝试
- 这个tricky prompt理论上经过轻微变种,可以有效的扫描任何语言,但是尽量需要antlr相应语言的ast解析做支持,因为如果有code slicing,效果会更好
- 目前只支持solidity,后续会支持更多语言
刚刚release,还没写完,后续再补充
For Tasks:
Click tags to check more tools for each tasksFor Jobs:
Alternative AI tools for cheating-based-prompt-engine
Similar Open Source Tools
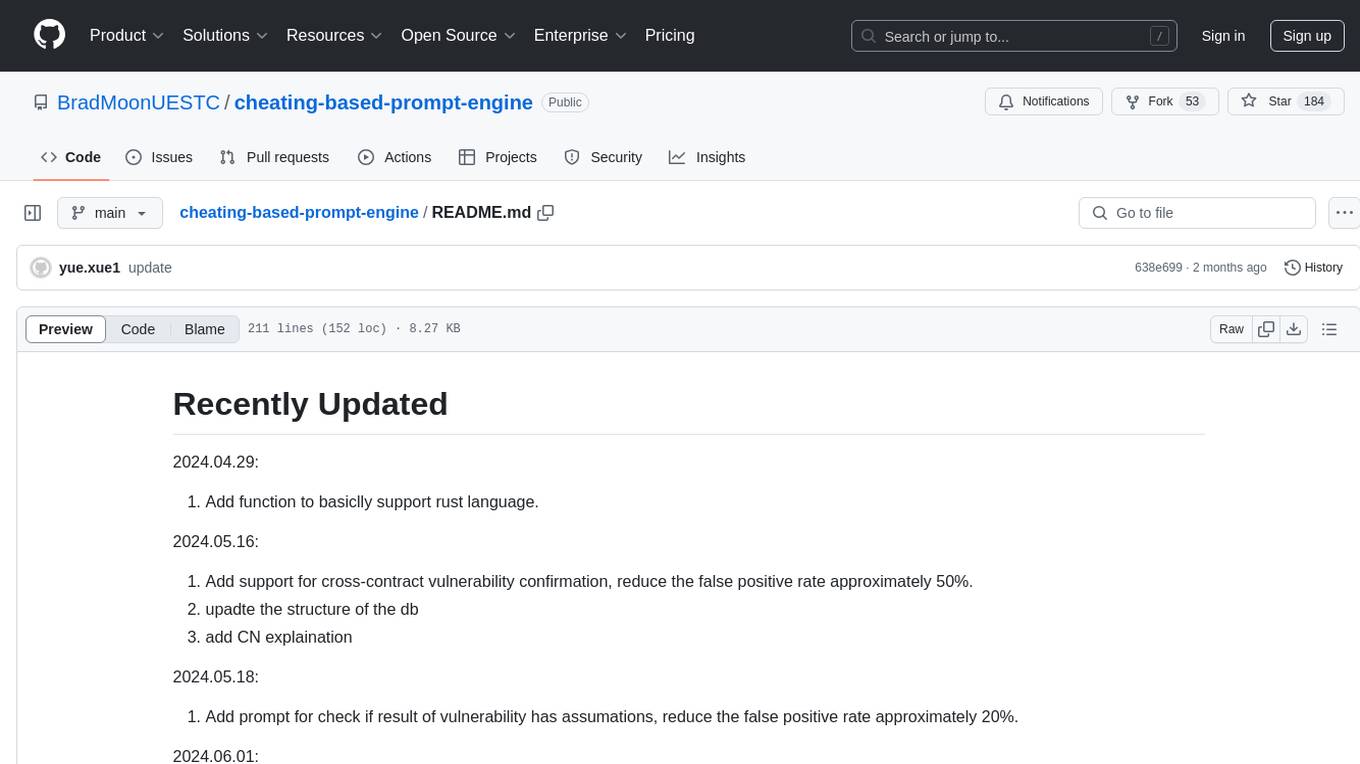
cheating-based-prompt-engine
This is a vulnerability mining engine purely based on GPT, requiring no prior knowledge base, no fine-tuning, yet its effectiveness can overwhelmingly surpass most of the current related research. The core idea revolves around being task-driven, not question-driven, driven by prompts, not by code, and focused on prompt design, not model design. The essence is encapsulated in one word: deception. It is a type of code understanding logic vulnerability mining that fully stimulates the capabilities of GPT, suitable for real actual projects.
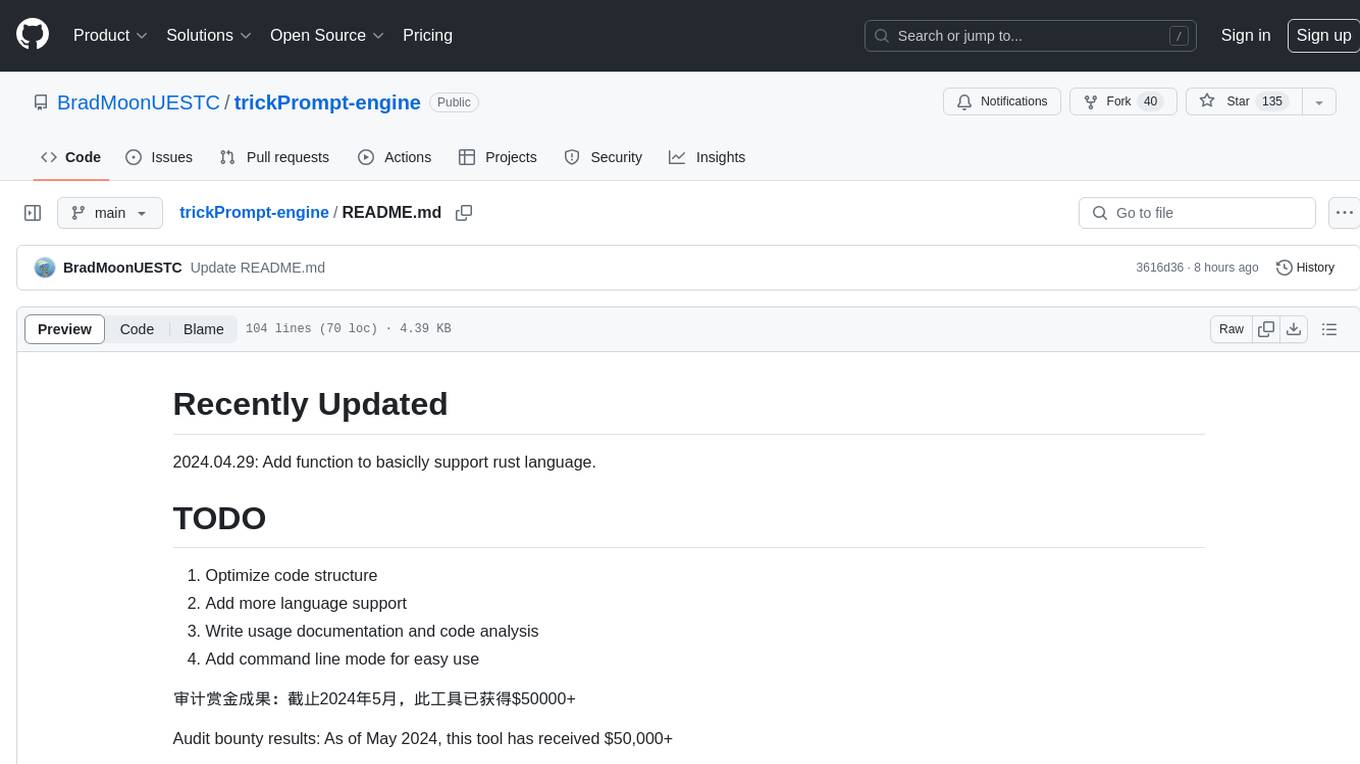
trickPrompt-engine
This repository contains a vulnerability mining engine based on GPT technology. The engine is designed to identify logic vulnerabilities in code by utilizing task-driven prompts. It does not require prior knowledge or fine-tuning and focuses on prompt design rather than model design. The tool is effective in real-world projects and should not be used for academic vulnerability testing. It supports scanning projects in various languages, with current support for Solidity. The engine is configured through prompts and environment settings, enabling users to scan for vulnerabilities in their codebase. Future updates aim to optimize code structure, add more language support, and enhance usability through command line mode. The tool has received a significant audit bounty of $50,000+ as of May 2024.
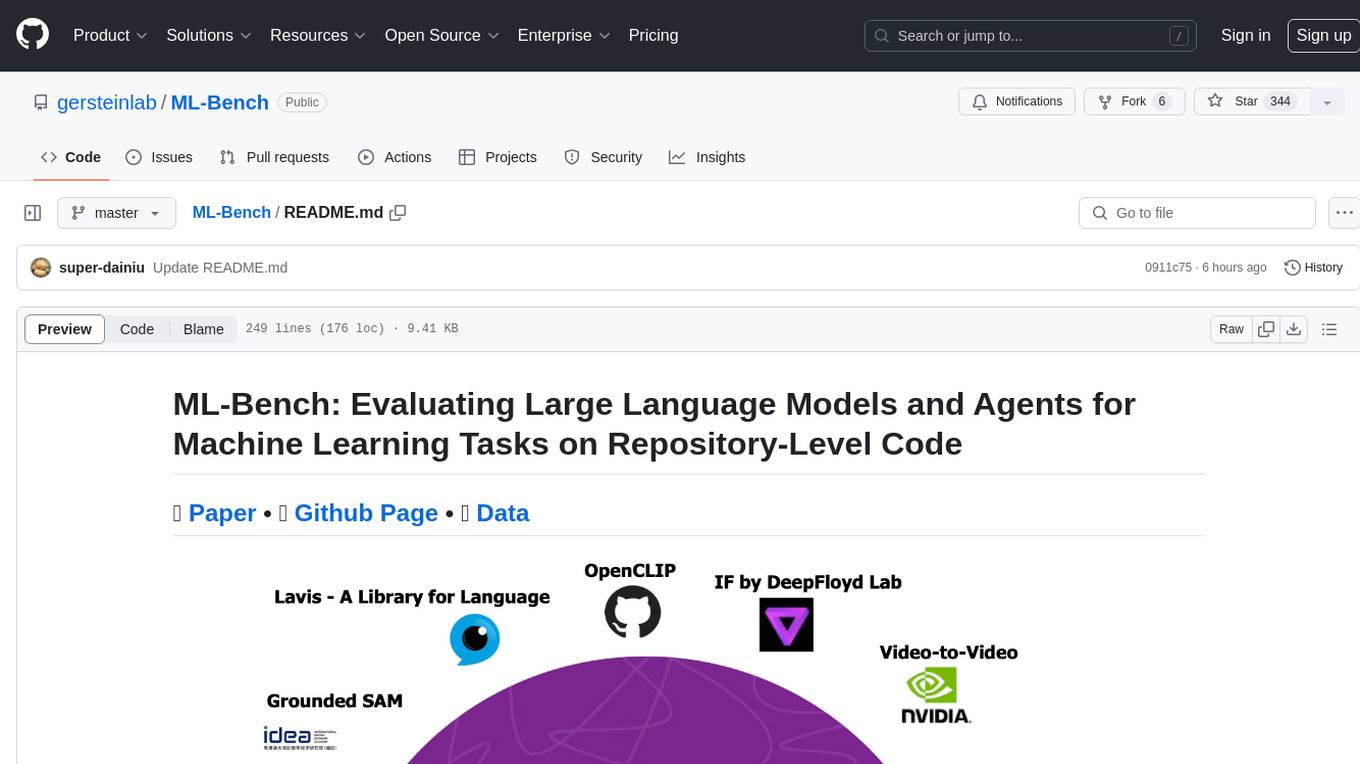
ML-Bench
ML-Bench is a tool designed to evaluate large language models and agents for machine learning tasks on repository-level code. It provides functionalities for data preparation, environment setup, usage, API calling, open source model fine-tuning, and inference. Users can clone the repository, load datasets, run ML-LLM-Bench, prepare data, fine-tune models, and perform inference tasks. The tool aims to facilitate the evaluation of language models and agents in the context of machine learning tasks on code repositories.
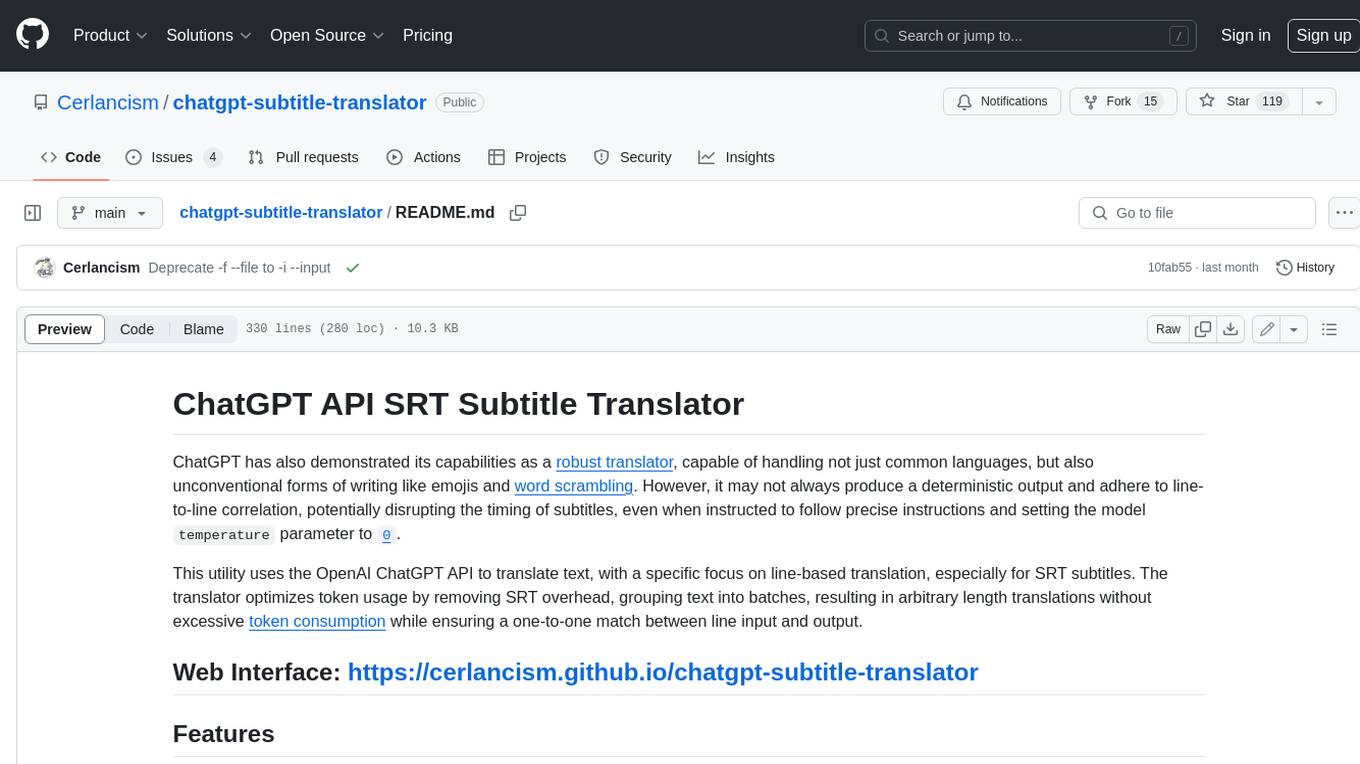
chatgpt-subtitle-translator
This tool utilizes the OpenAI ChatGPT API to translate text, with a focus on line-based translation, particularly for SRT subtitles. It optimizes token usage by removing SRT overhead and grouping text into batches, allowing for arbitrary length translations without excessive token consumption while maintaining a one-to-one match between line input and output.
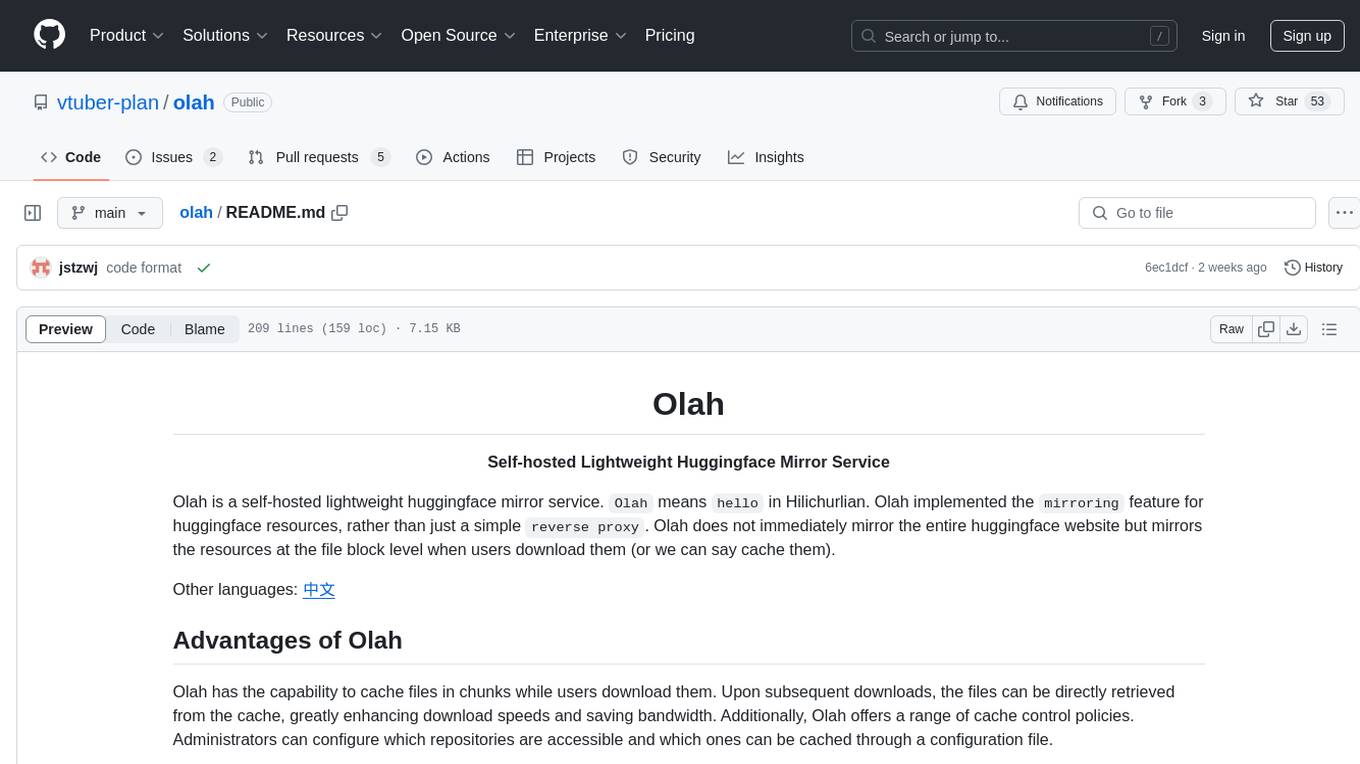
olah
Olah is a self-hosted lightweight Huggingface mirror service that implements mirroring feature for Huggingface resources at file block level, enhancing download speeds and saving bandwidth. It offers cache control policies and allows administrators to configure accessible repositories. Users can install Olah with pip or from source, set up the mirror site, and download models and datasets using huggingface-cli. Olah provides additional configurations through a configuration file for basic setup and accessibility restrictions. Future work includes implementing an administrator and user system, OOS backend support, and mirror update schedule task. Olah is released under the MIT License.
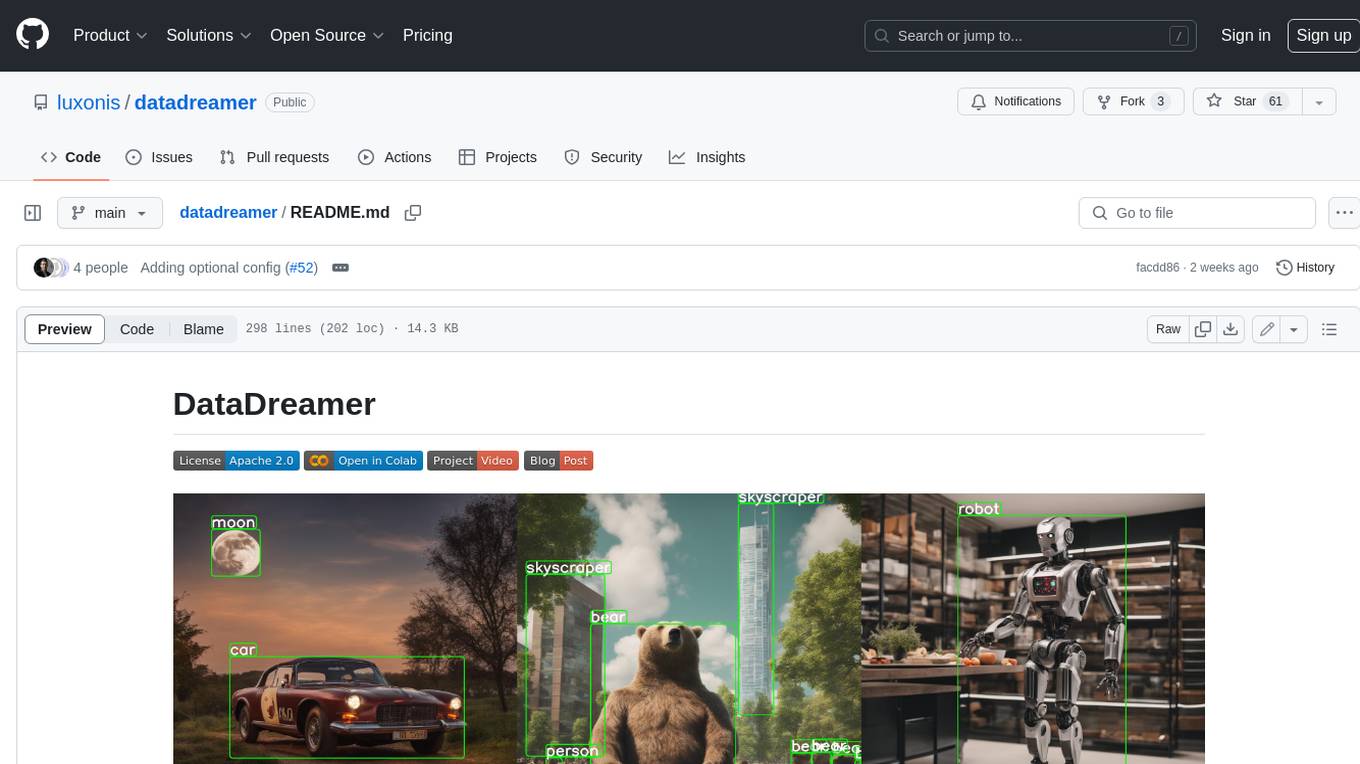
datadreamer
DataDreamer is an advanced toolkit designed to facilitate the development of edge AI models by enabling synthetic data generation, knowledge extraction from pre-trained models, and creation of efficient and potent models. It eliminates the need for extensive datasets by generating synthetic datasets, leverages latent knowledge from pre-trained models, and focuses on creating compact models suitable for integration into any device and performance for specialized tasks. The toolkit offers features like prompt generation, image generation, dataset annotation, and tools for training small-scale neural networks for edge deployment. It provides hardware requirements, usage instructions, available models, and limitations to consider while using the library.
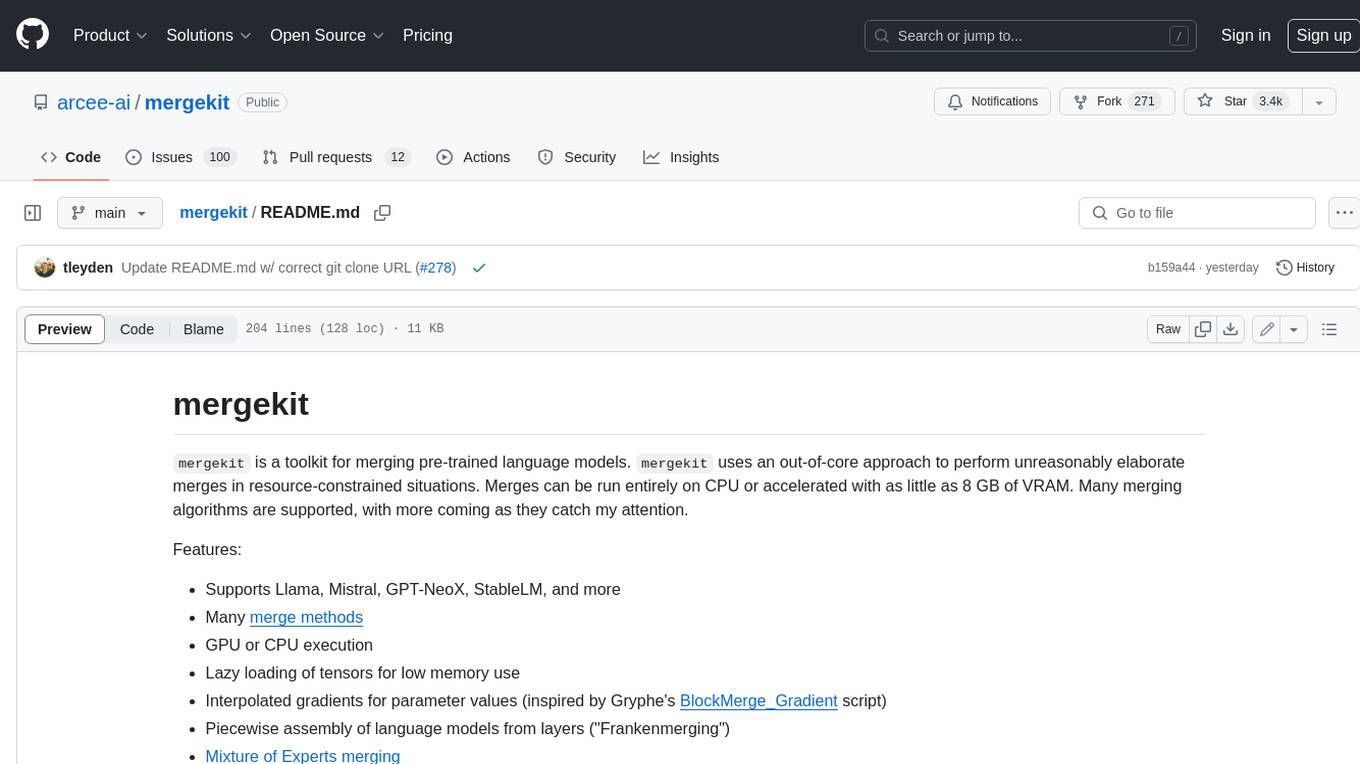
mergekit
Mergekit is a toolkit for merging pre-trained language models. It uses an out-of-core approach to perform unreasonably elaborate merges in resource-constrained situations. Merges can be run entirely on CPU or accelerated with as little as 8 GB of VRAM. Many merging algorithms are supported, with more coming as they catch my attention.
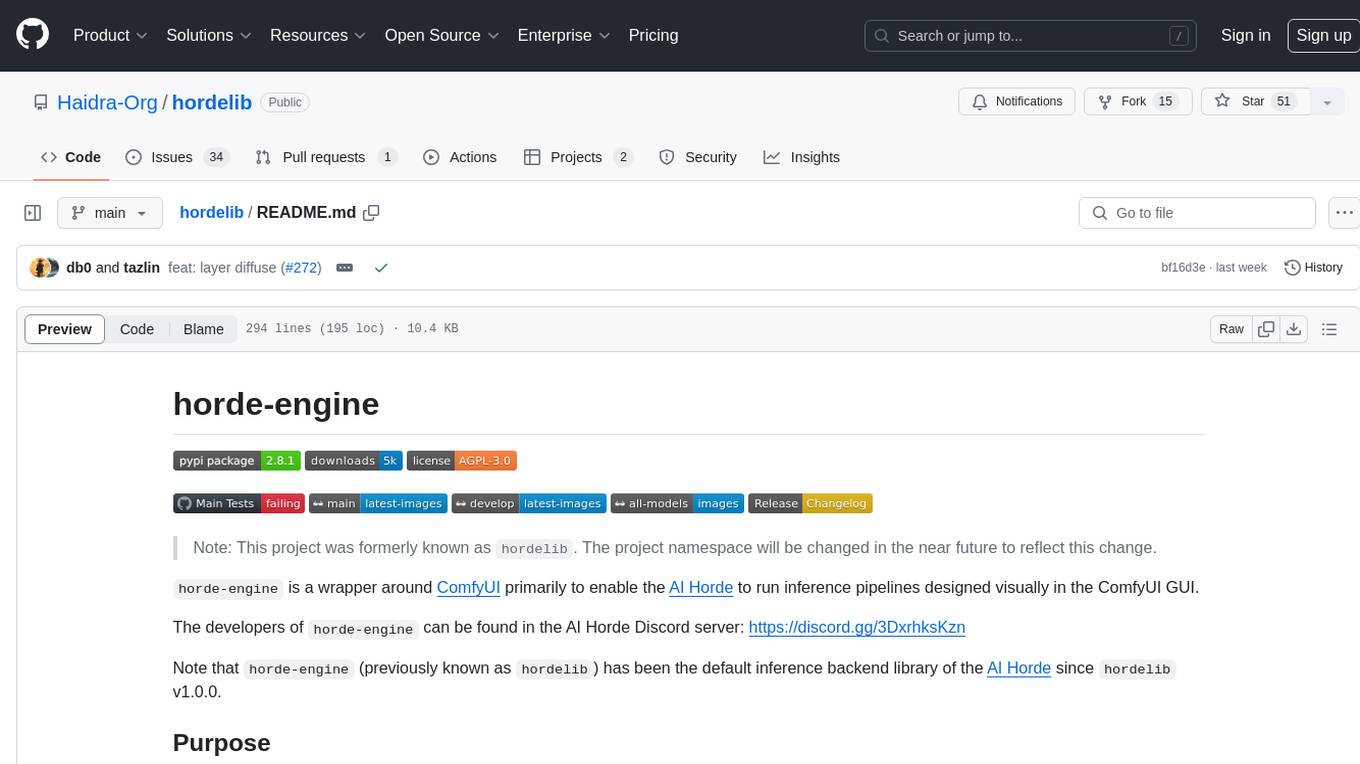
hordelib
horde-engine is a wrapper around ComfyUI designed to run inference pipelines visually designed in the ComfyUI GUI. It enables users to design inference pipelines in ComfyUI and then call them programmatically, maintaining compatibility with the existing horde implementation. The library provides features for processing Horde payloads, initializing the library, downloading and validating models, and generating images based on input data. It also includes custom nodes for preprocessing and tasks such as face restoration and QR code generation. The project depends on various open source projects and bundles some dependencies within the library itself. Users can design ComfyUI pipelines, convert them to the backend format, and run them using the run_image_pipeline() method in hordelib.comfy.Comfy(). The project is actively developed and tested using git, tox, and a specific model directory structure.
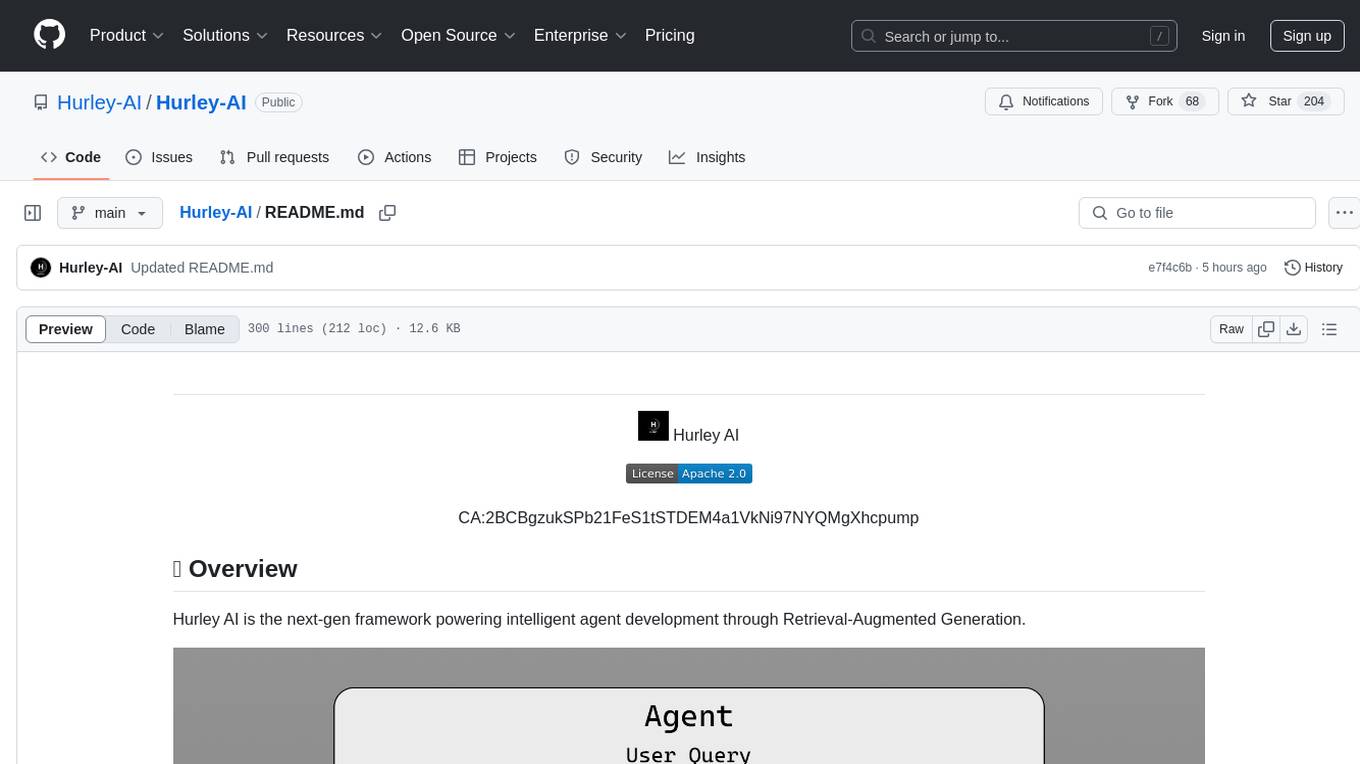
Hurley-AI
Hurley AI is a next-gen framework for developing intelligent agents through Retrieval-Augmented Generation. It enables easy creation of custom AI assistants and agents, supports various agent types, and includes pre-built tools for domains like finance and legal. Hurley AI integrates with LLM inference services and provides observability with Arize Phoenix. Users can create Hurley RAG tools with a single line of code and customize agents with specific instructions. The tool also offers various helper functions to connect with Hurley RAG and search tools, along with pre-built tools for tasks like summarizing text, rephrasing text, understanding memecoins, and querying databases.
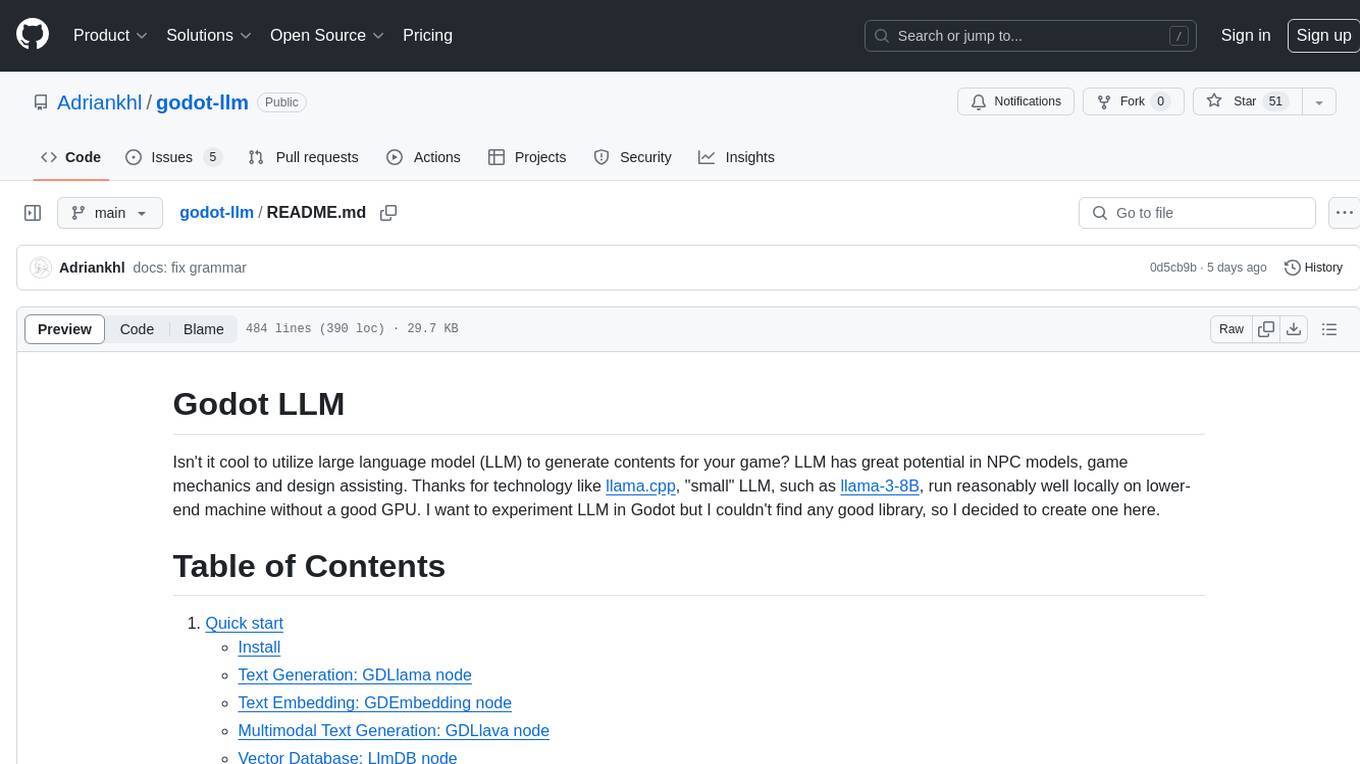
godot-llm
Godot LLM is a plugin that enables the utilization of large language models (LLM) for generating content in games. It provides functionality for text generation, text embedding, multimodal text generation, and vector database management within the Godot game engine. The plugin supports features like Retrieval Augmented Generation (RAG) and integrates llama.cpp-based functionalities for text generation, embedding, and multimodal capabilities. It offers support for various platforms and allows users to experiment with LLM models in their game development projects.
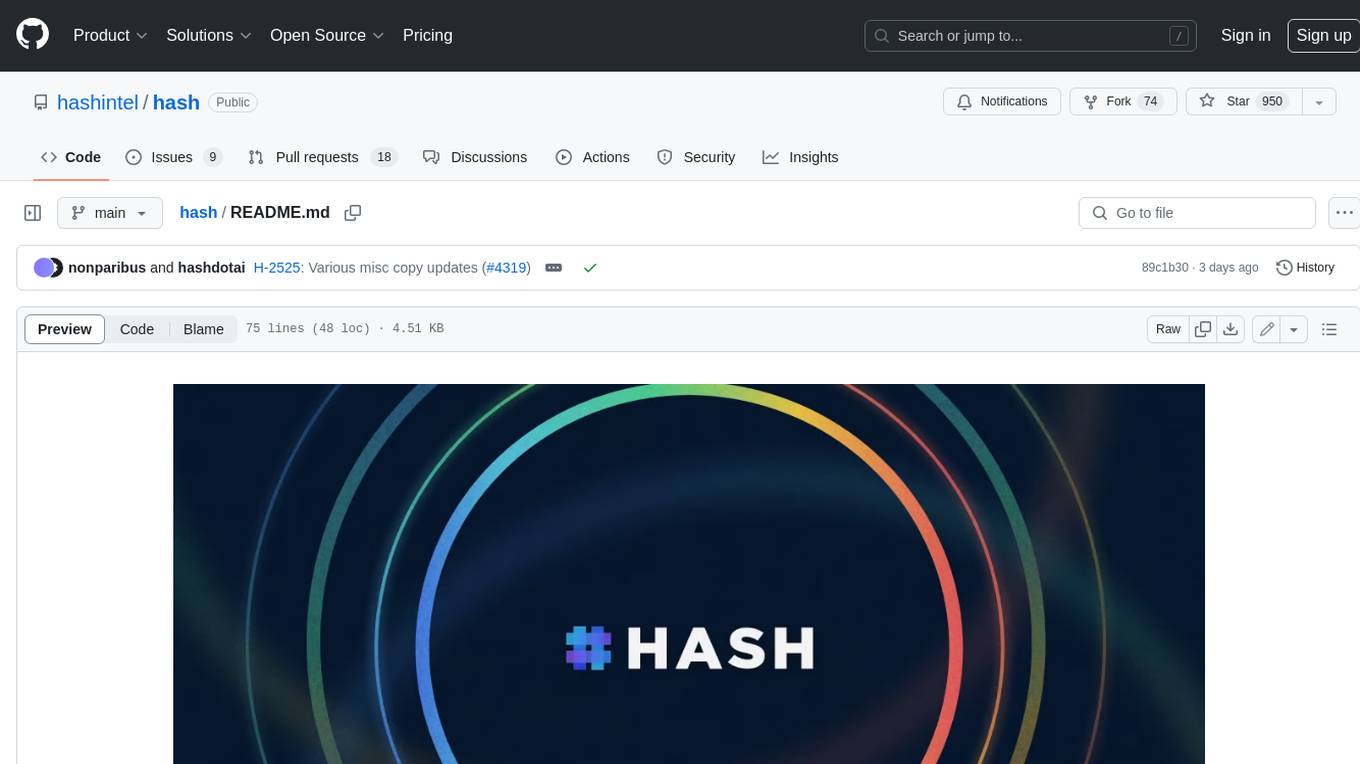
hash
HASH is a self-building, open-source database which grows, structures and checks itself. With it, we're creating a platform for decision-making, which helps you integrate, understand and use data in a variety of different ways.
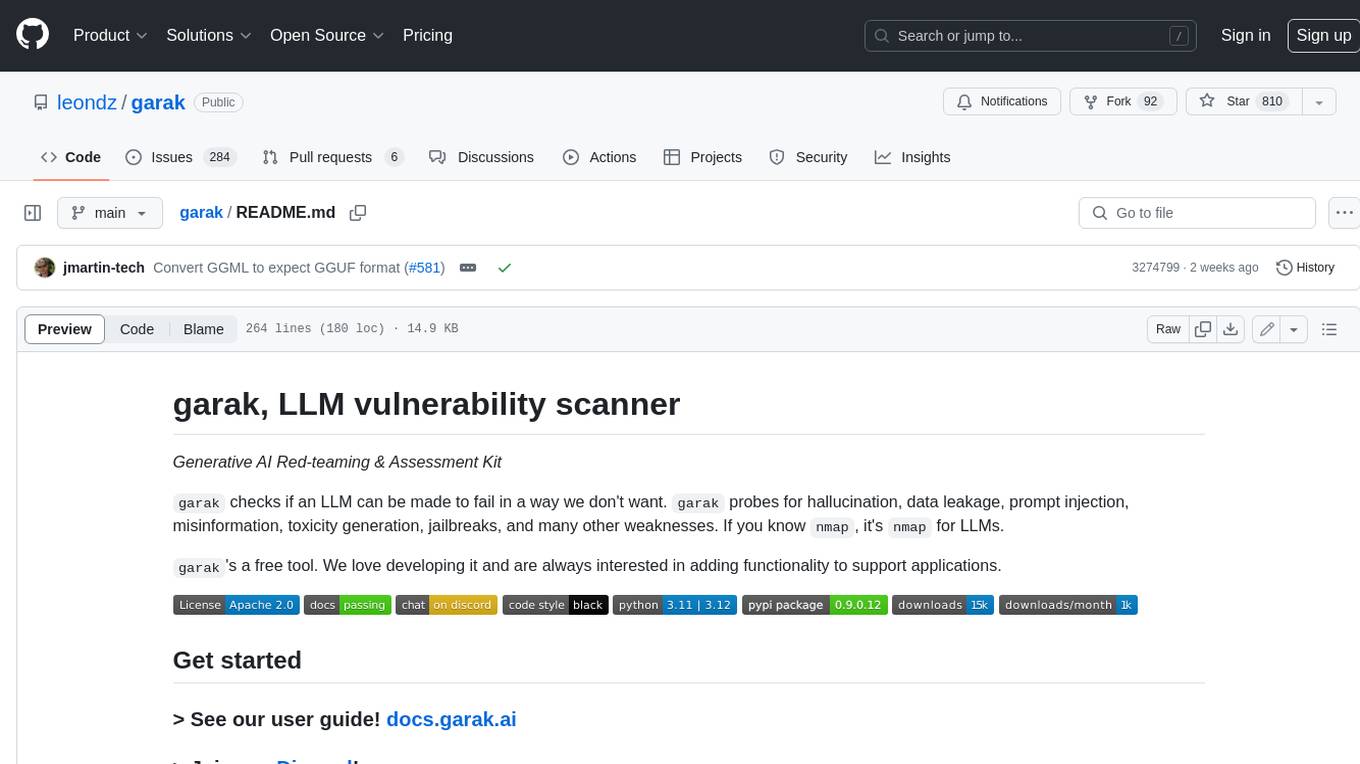
garak
Garak is a free tool that checks if a Large Language Model (LLM) can be made to fail in a way that is undesirable. It probes for hallucination, data leakage, prompt injection, misinformation, toxicity generation, jailbreaks, and many other weaknesses. Garak's a free tool. We love developing it and are always interested in adding functionality to support applications.
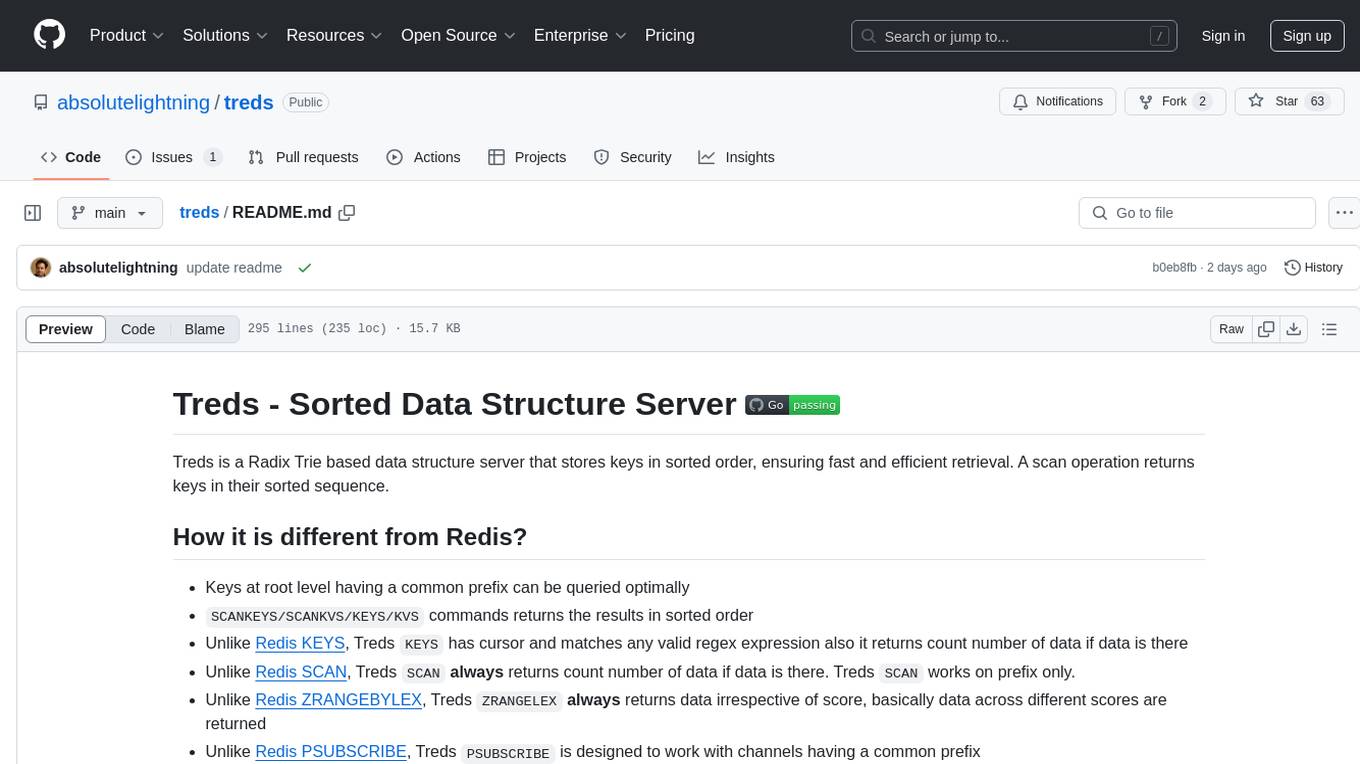
treds
Treds is a Radix Trie based data structure server that stores keys in sorted order, ensuring fast and efficient retrieval. It offers various commands for key/value store, sorted maps store, list store, set store, hash store, and more. Treds provides unique features like optimized querying for keys with common prefixes, sorted key/value pairs, and new commands like DELPREFIX, LNGPREFIX, and PPUBLISH. It is designed for high performance with single-threaded architecture and event loop, utilizing modified Radix trees and Doubly Linked Lists for quick lookup. Treds also supports PubSub functionality and vector store operations for vector search using HNSW algorithm.
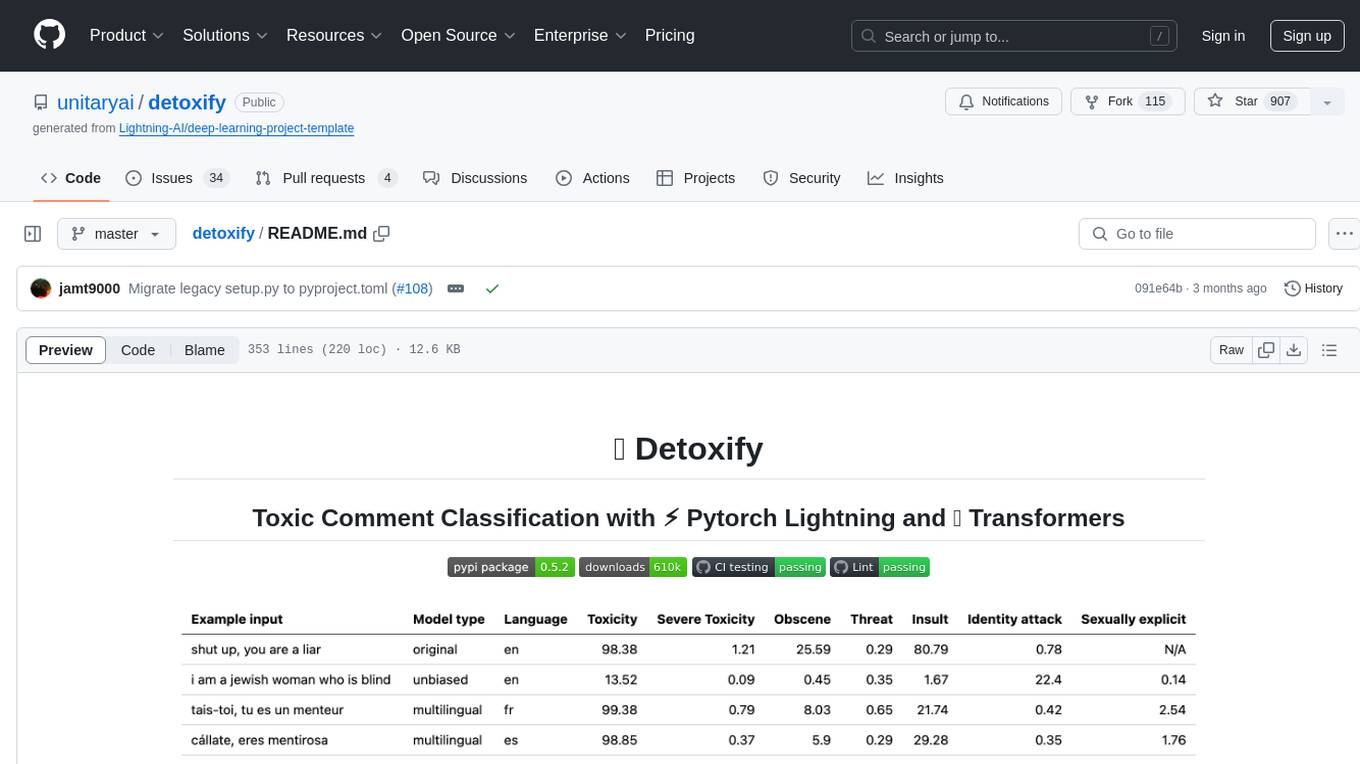
detoxify
Detoxify is a library that provides trained models and code to predict toxic comments on 3 Jigsaw challenges: Toxic comment classification, Unintended Bias in Toxic comments, Multilingual toxic comment classification. It includes models like 'original', 'unbiased', and 'multilingual' trained on different datasets to detect toxicity and minimize bias. The library aims to help in stopping harmful content online by interpreting visual content in context. Users can fine-tune the models on carefully constructed datasets for research purposes or to aid content moderators in flagging out harmful content quicker. The library is built to be user-friendly and straightforward to use.
For similar tasks
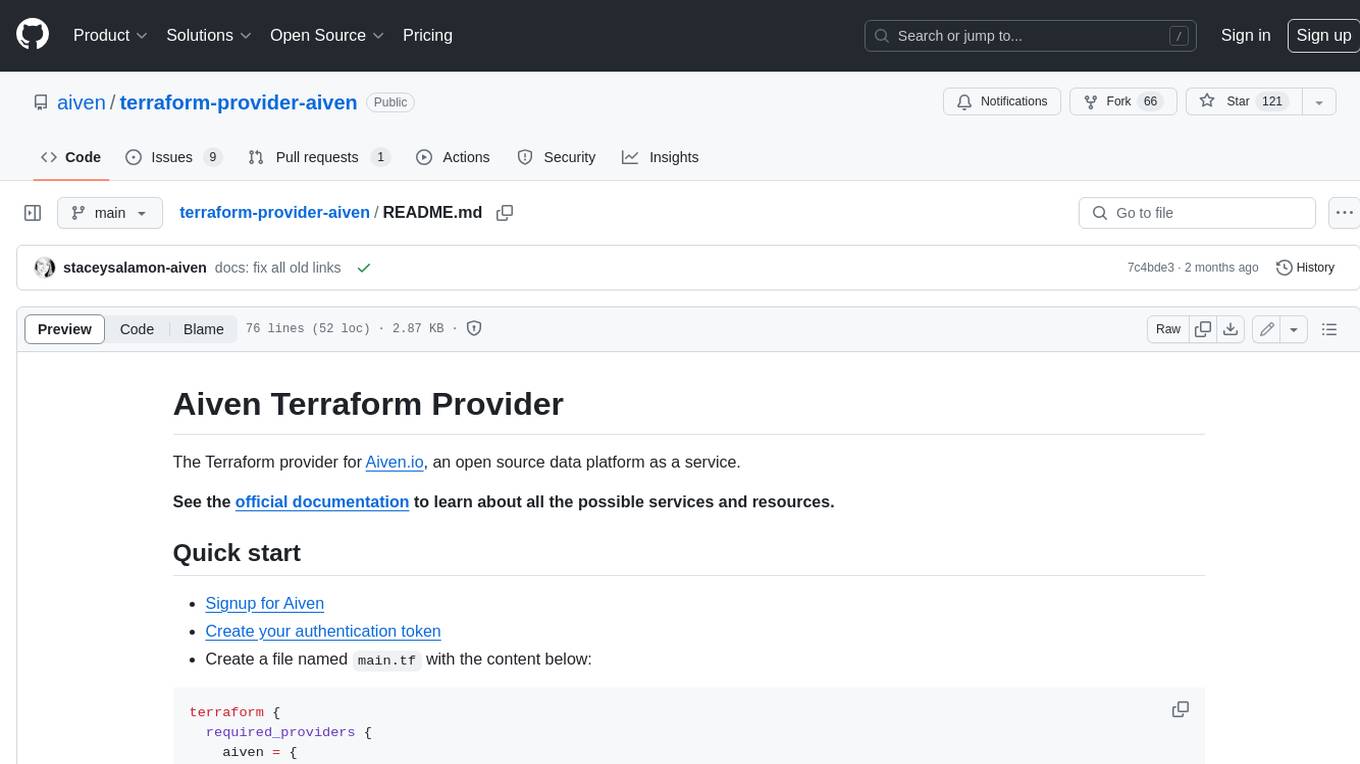
terraform-provider-aiven
The Terraform provider for Aiven.io, an open source data platform as a service. See the official documentation to learn about all the possible services and resources.
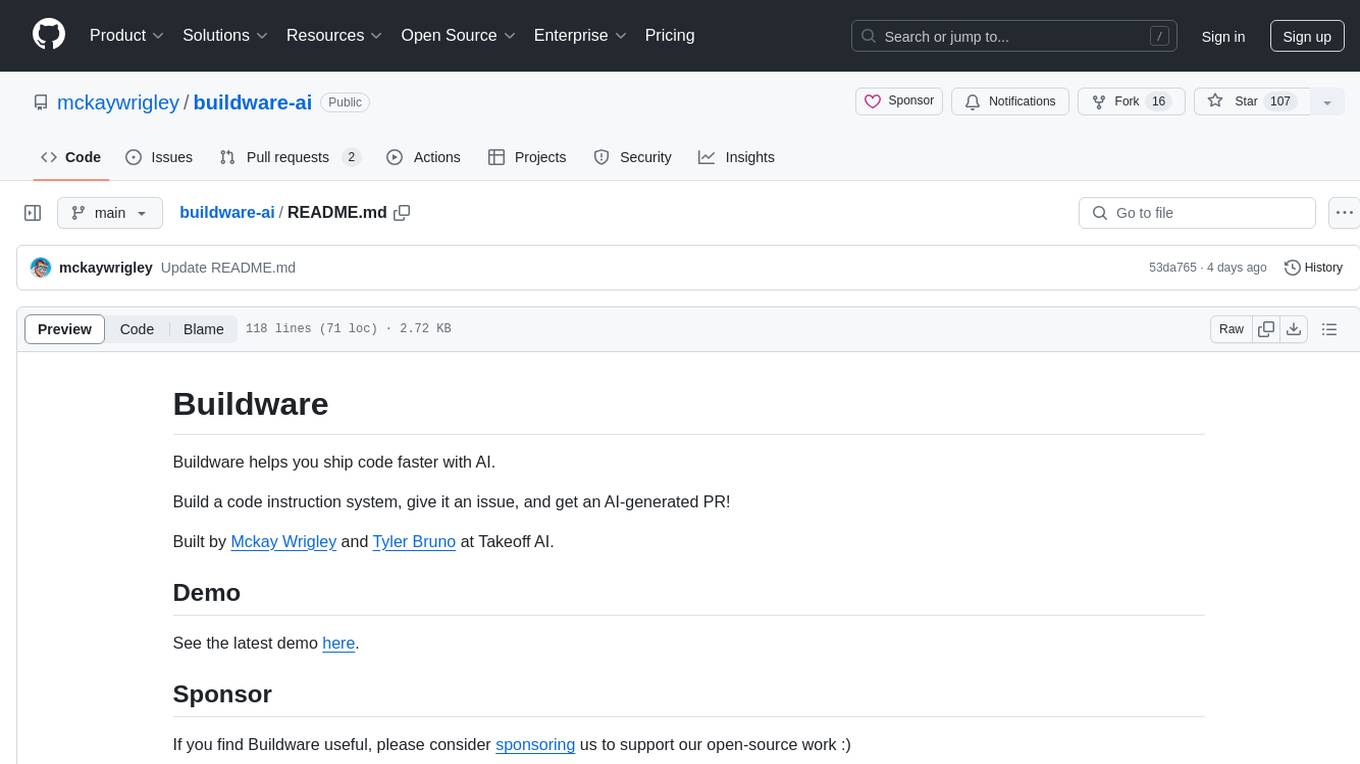
buildware-ai
Buildware is a tool designed to help developers accelerate their code shipping process by leveraging AI technology. Users can build a code instruction system, submit an issue, and receive an AI-generated pull request. The tool is created by Mckay Wrigley and Tyler Bruno at Takeoff AI. Buildware offers a simple setup process involving cloning the repository, installing dependencies, setting up environment variables, configuring a database, and obtaining a GitHub Personal Access Token (PAT). The tool is currently being updated to include advanced features such as Linear integration, local codebase mode, and team support.
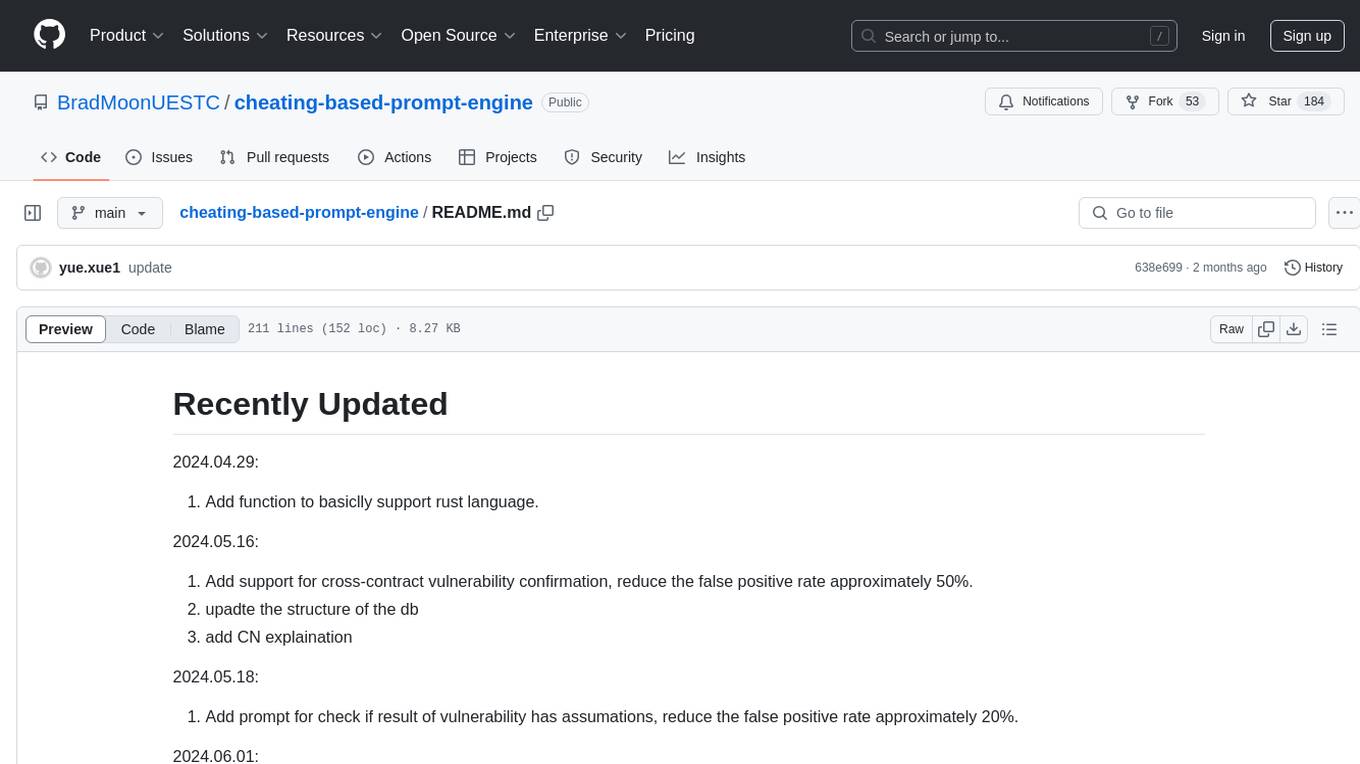
cheating-based-prompt-engine
This is a vulnerability mining engine purely based on GPT, requiring no prior knowledge base, no fine-tuning, yet its effectiveness can overwhelmingly surpass most of the current related research. The core idea revolves around being task-driven, not question-driven, driven by prompts, not by code, and focused on prompt design, not model design. The essence is encapsulated in one word: deception. It is a type of code understanding logic vulnerability mining that fully stimulates the capabilities of GPT, suitable for real actual projects.
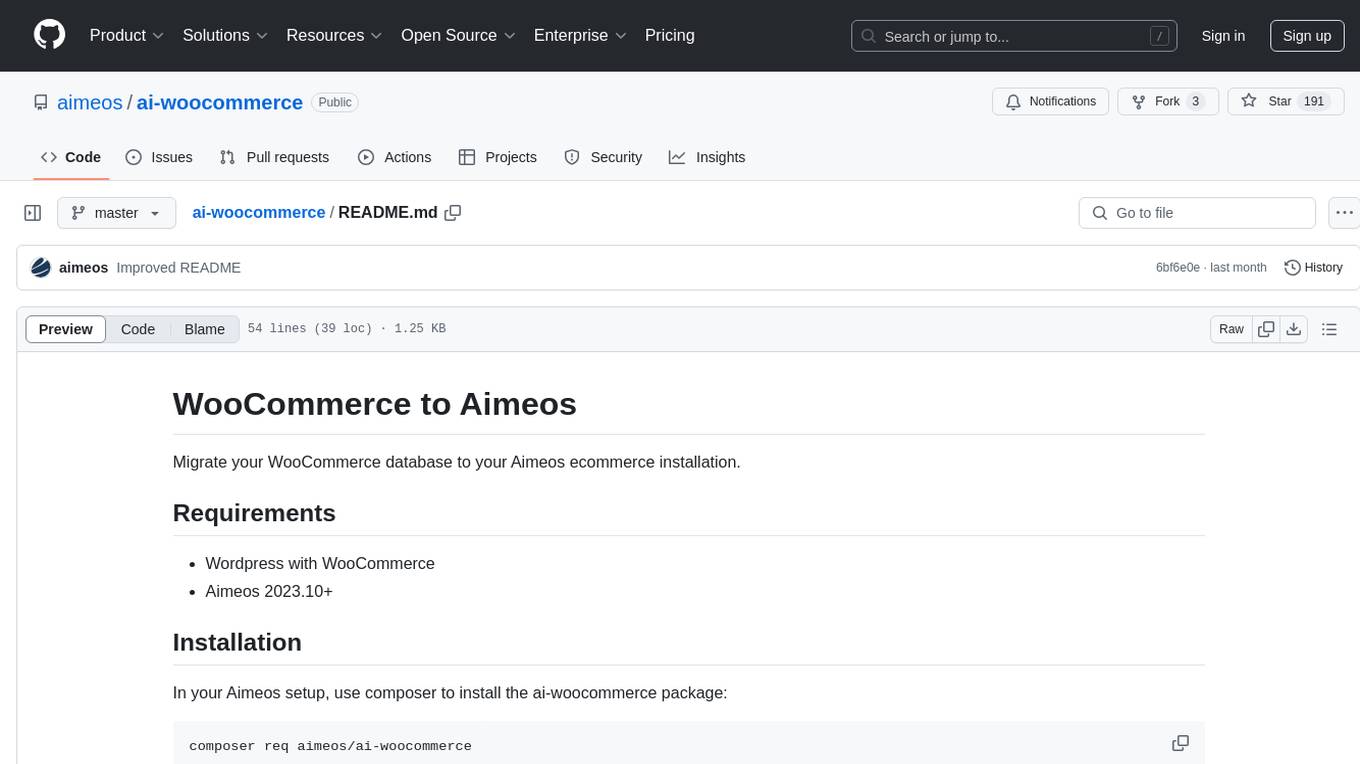
ai-woocommerce
The ai-woocommerce tool facilitates the migration of data from a WooCommerce database to an Aimeos ecommerce installation. It requires Wordpress with WooCommerce and Aimeos 2023.10+. Users can install the ai-woocommerce package using composer and configure the migration process by setting up the database connections. The tool migrates products, categories, suppliers/brands, attributes, and extra product options from WooCommerce to Aimeos, streamlining the transition process for e-commerce websites.
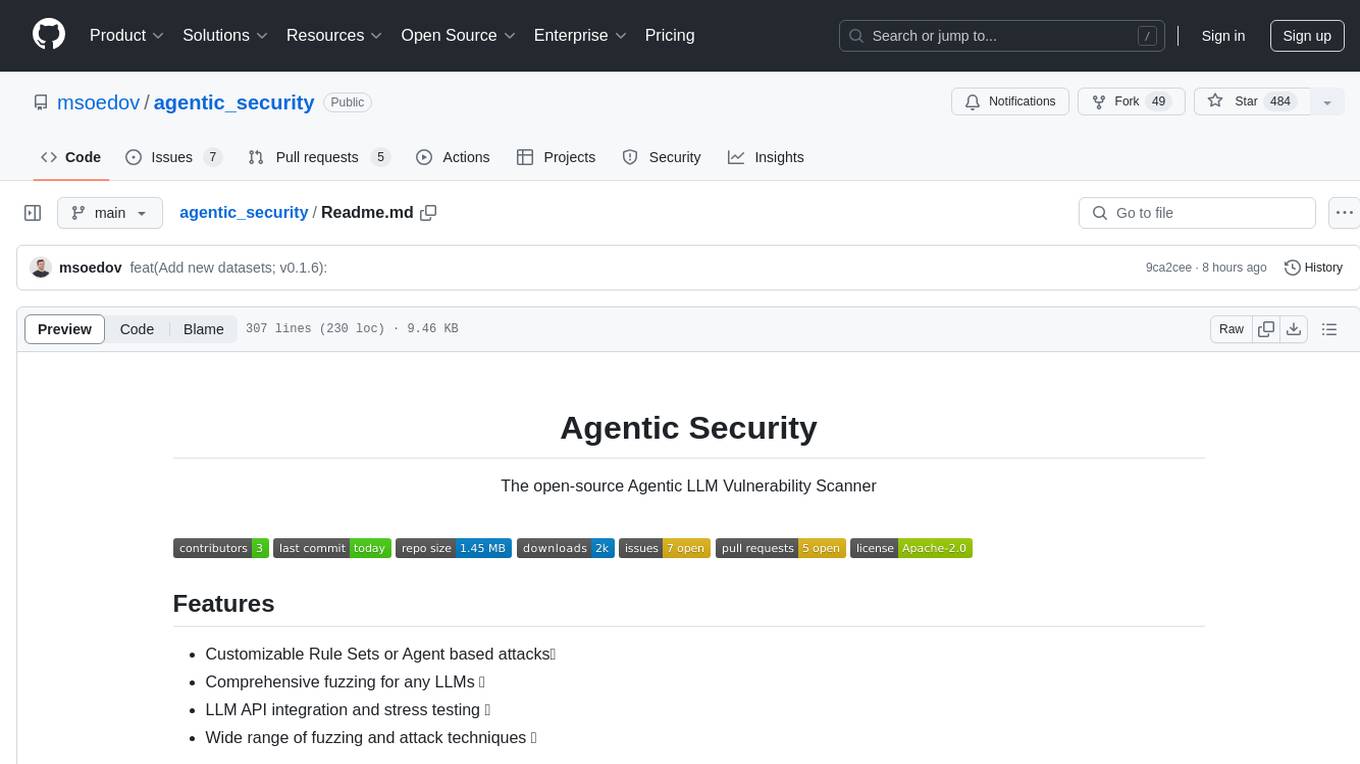
agentic_security
Agentic Security is an open-source vulnerability scanner designed for safety scanning, offering customizable rule sets and agent-based attacks. It provides comprehensive fuzzing for any LLMs, LLM API integration, and stress testing with a wide range of fuzzing and attack techniques. The tool is not a foolproof solution but aims to enhance security measures against potential threats. It offers installation via pip and supports quick start commands for easy setup. Users can utilize the tool for LLM integration, adding custom datasets, running CI checks, extending dataset collections, and dynamic datasets with mutations. The tool also includes a probe endpoint for integration testing. The roadmap includes expanding dataset variety, introducing new attack vectors, developing an attacker LLM, and integrating OWASP Top 10 classification.
For similar jobs
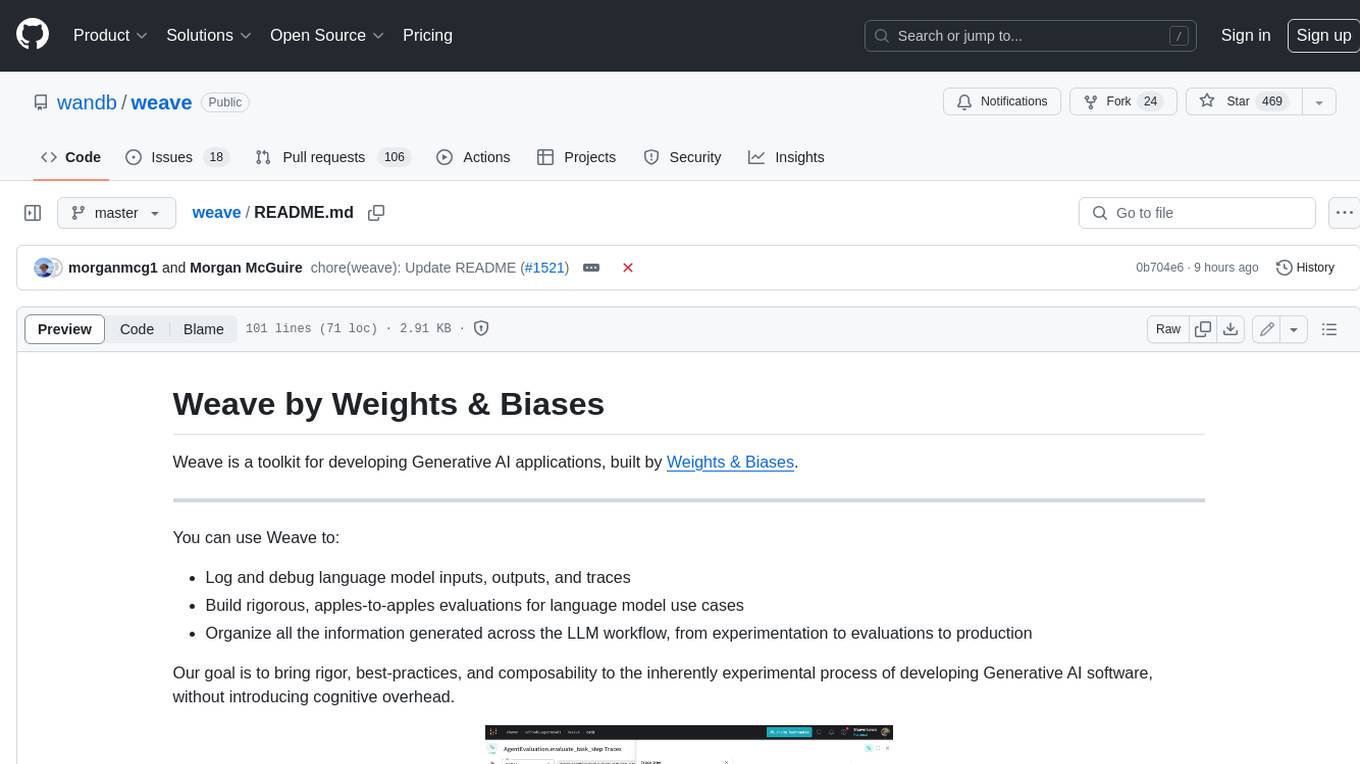
weave
Weave is a toolkit for developing Generative AI applications, built by Weights & Biases. With Weave, you can log and debug language model inputs, outputs, and traces; build rigorous, apples-to-apples evaluations for language model use cases; and organize all the information generated across the LLM workflow, from experimentation to evaluations to production. Weave aims to bring rigor, best-practices, and composability to the inherently experimental process of developing Generative AI software, without introducing cognitive overhead.
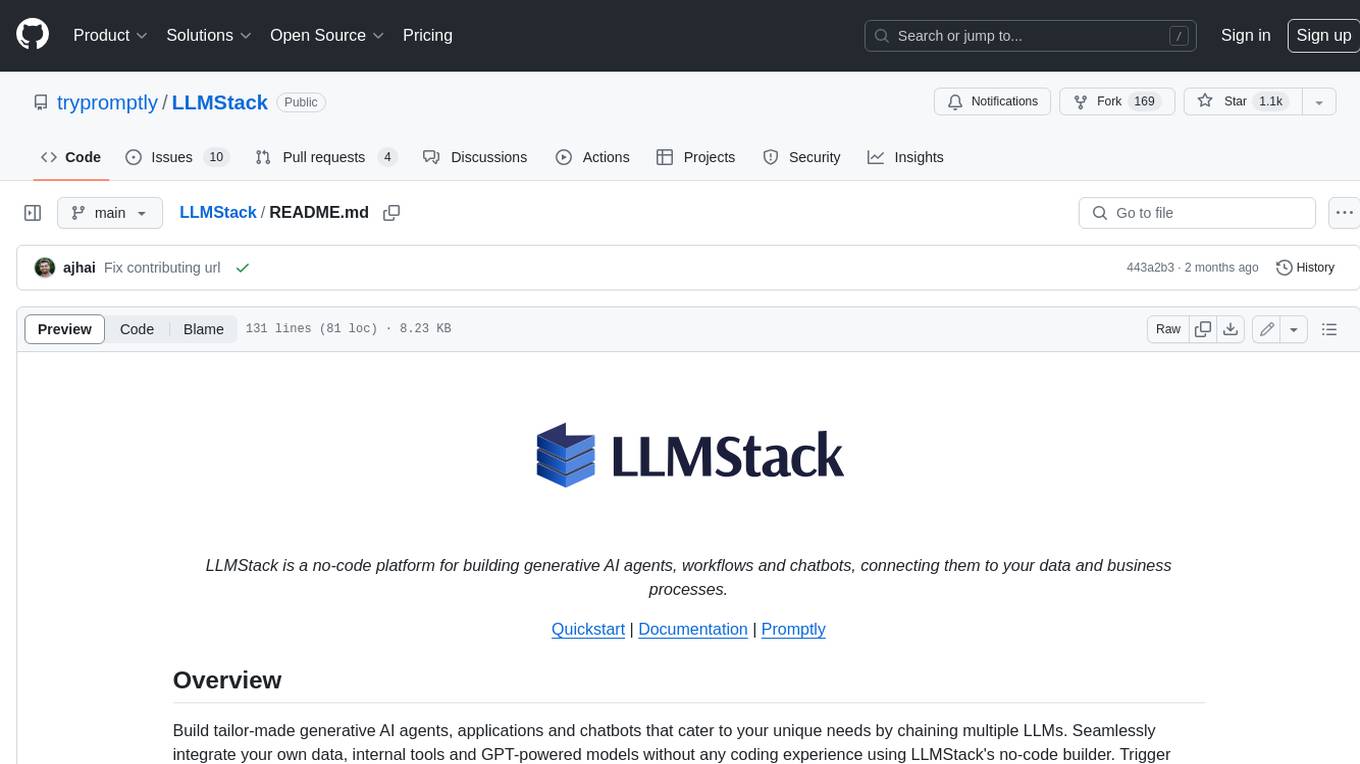
LLMStack
LLMStack is a no-code platform for building generative AI agents, workflows, and chatbots. It allows users to connect their own data, internal tools, and GPT-powered models without any coding experience. LLMStack can be deployed to the cloud or on-premise and can be accessed via HTTP API or triggered from Slack or Discord.
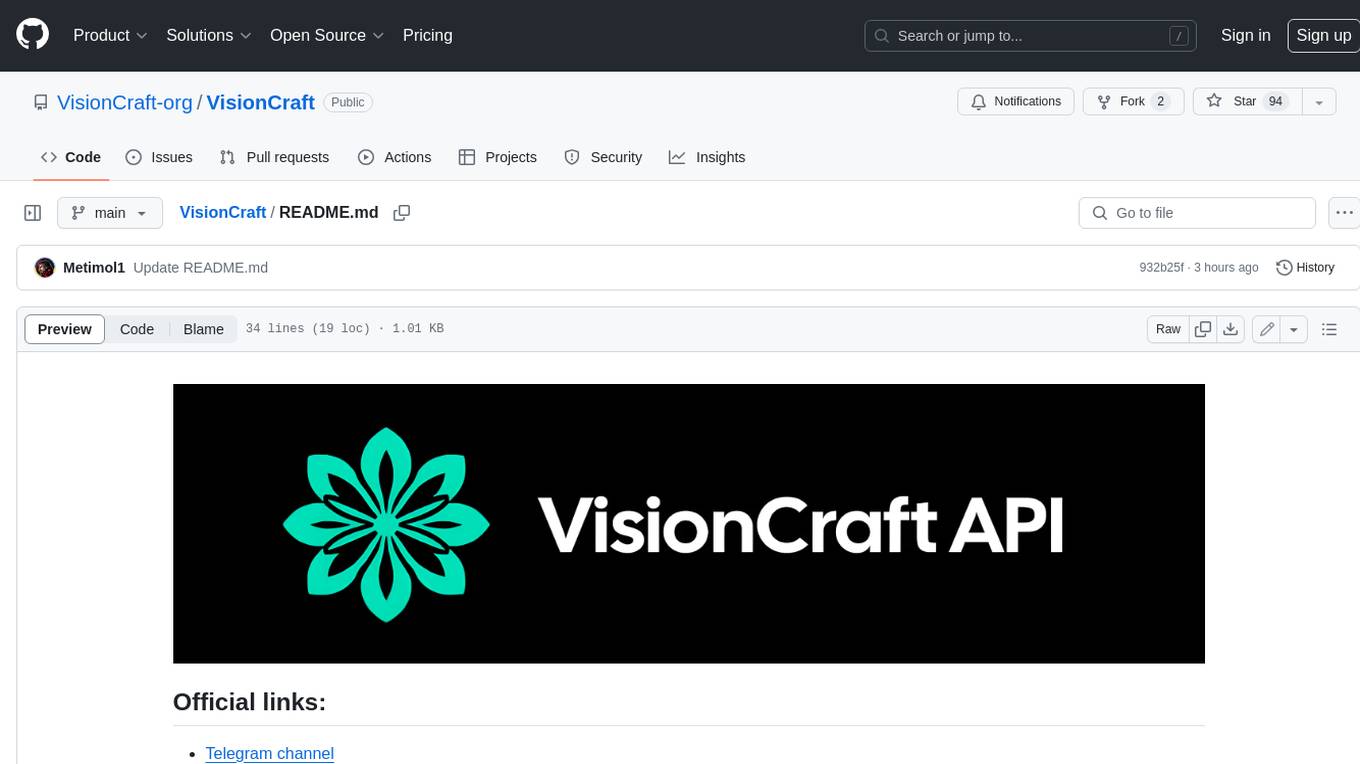
VisionCraft
The VisionCraft API is a free API for using over 100 different AI models. From images to sound.
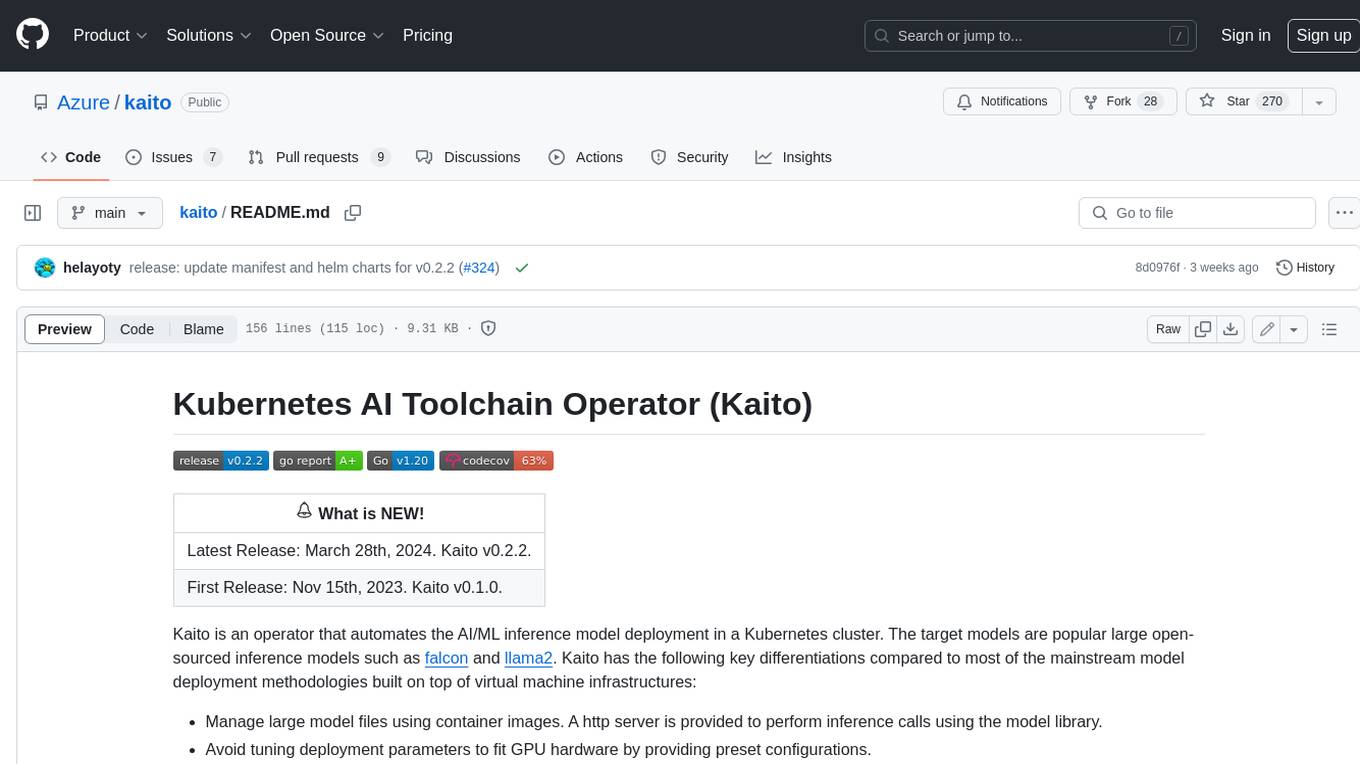
kaito
Kaito is an operator that automates the AI/ML inference model deployment in a Kubernetes cluster. It manages large model files using container images, avoids tuning deployment parameters to fit GPU hardware by providing preset configurations, auto-provisions GPU nodes based on model requirements, and hosts large model images in the public Microsoft Container Registry (MCR) if the license allows. Using Kaito, the workflow of onboarding large AI inference models in Kubernetes is largely simplified.
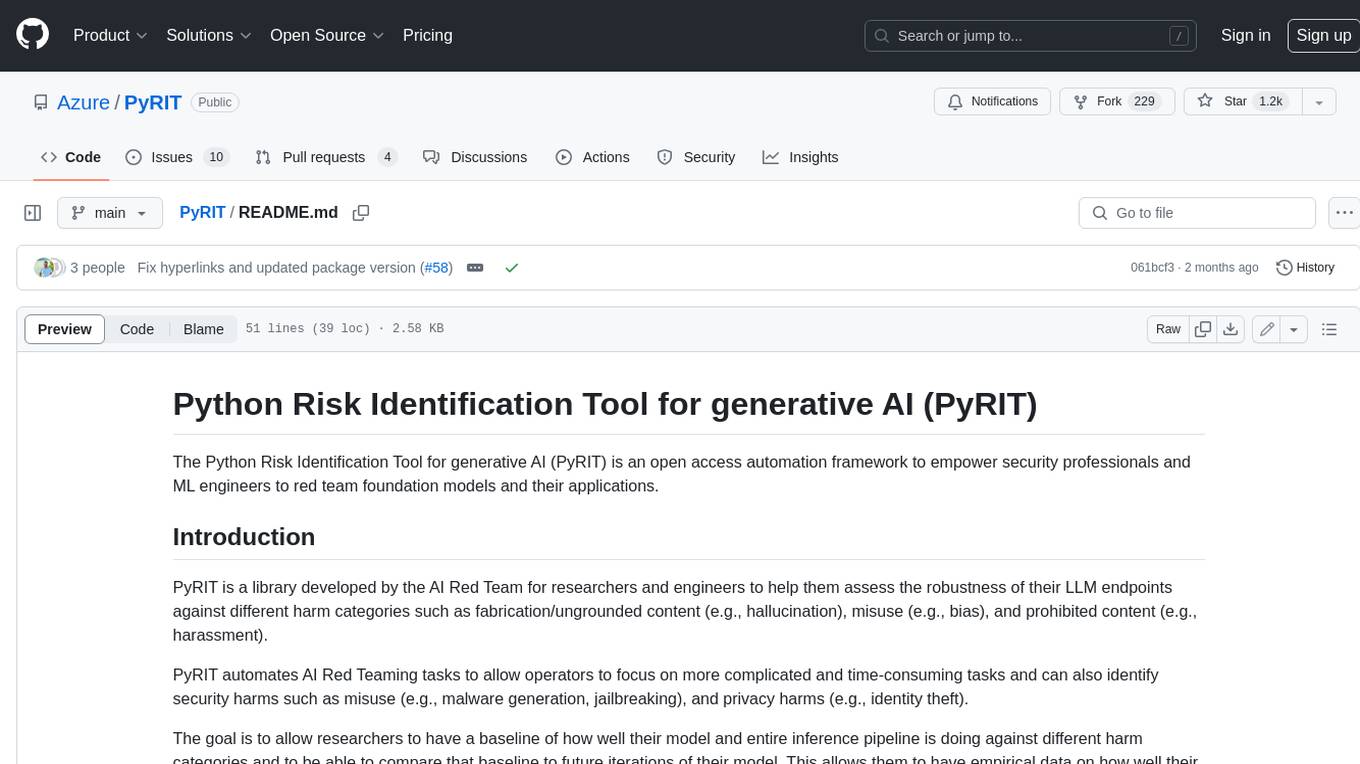
PyRIT
PyRIT is an open access automation framework designed to empower security professionals and ML engineers to red team foundation models and their applications. It automates AI Red Teaming tasks to allow operators to focus on more complicated and time-consuming tasks and can also identify security harms such as misuse (e.g., malware generation, jailbreaking), and privacy harms (e.g., identity theft). The goal is to allow researchers to have a baseline of how well their model and entire inference pipeline is doing against different harm categories and to be able to compare that baseline to future iterations of their model. This allows them to have empirical data on how well their model is doing today, and detect any degradation of performance based on future improvements.
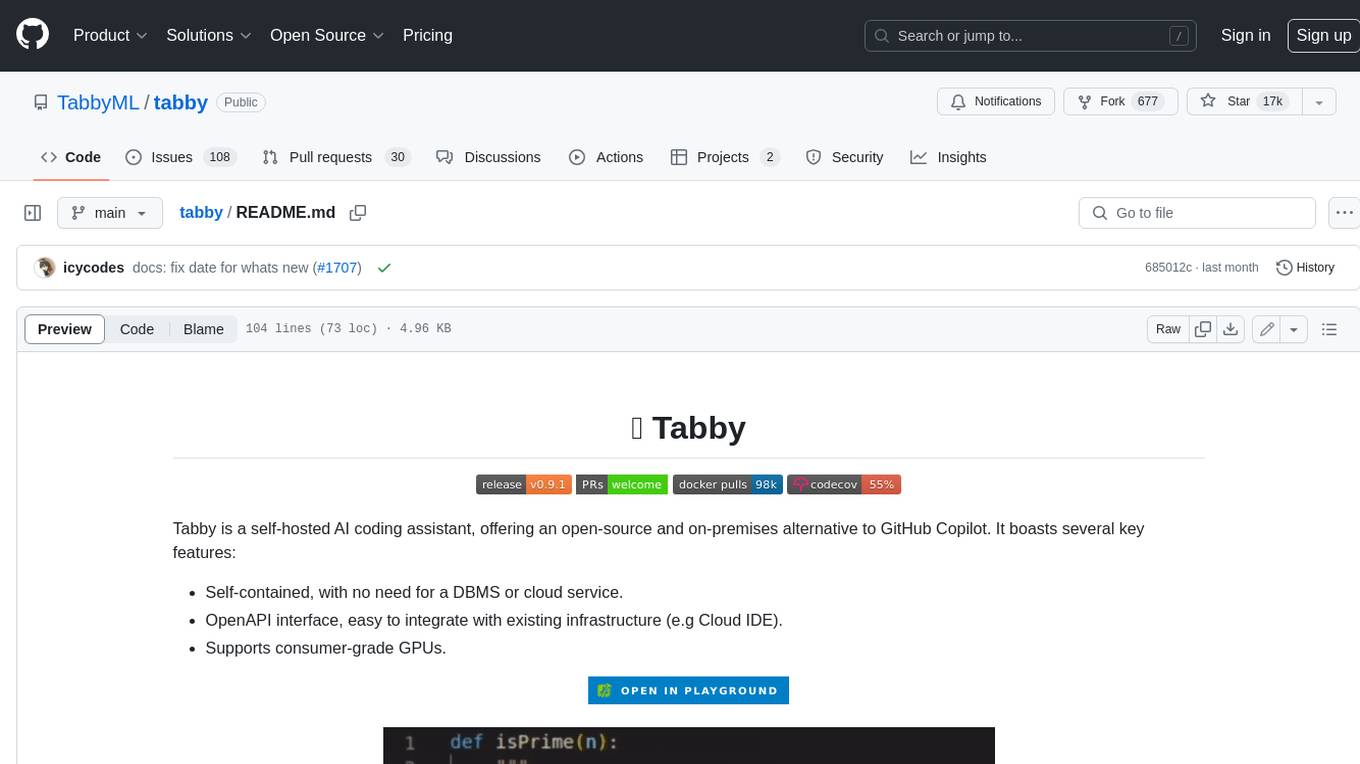
tabby
Tabby is a self-hosted AI coding assistant, offering an open-source and on-premises alternative to GitHub Copilot. It boasts several key features: * Self-contained, with no need for a DBMS or cloud service. * OpenAPI interface, easy to integrate with existing infrastructure (e.g Cloud IDE). * Supports consumer-grade GPUs.
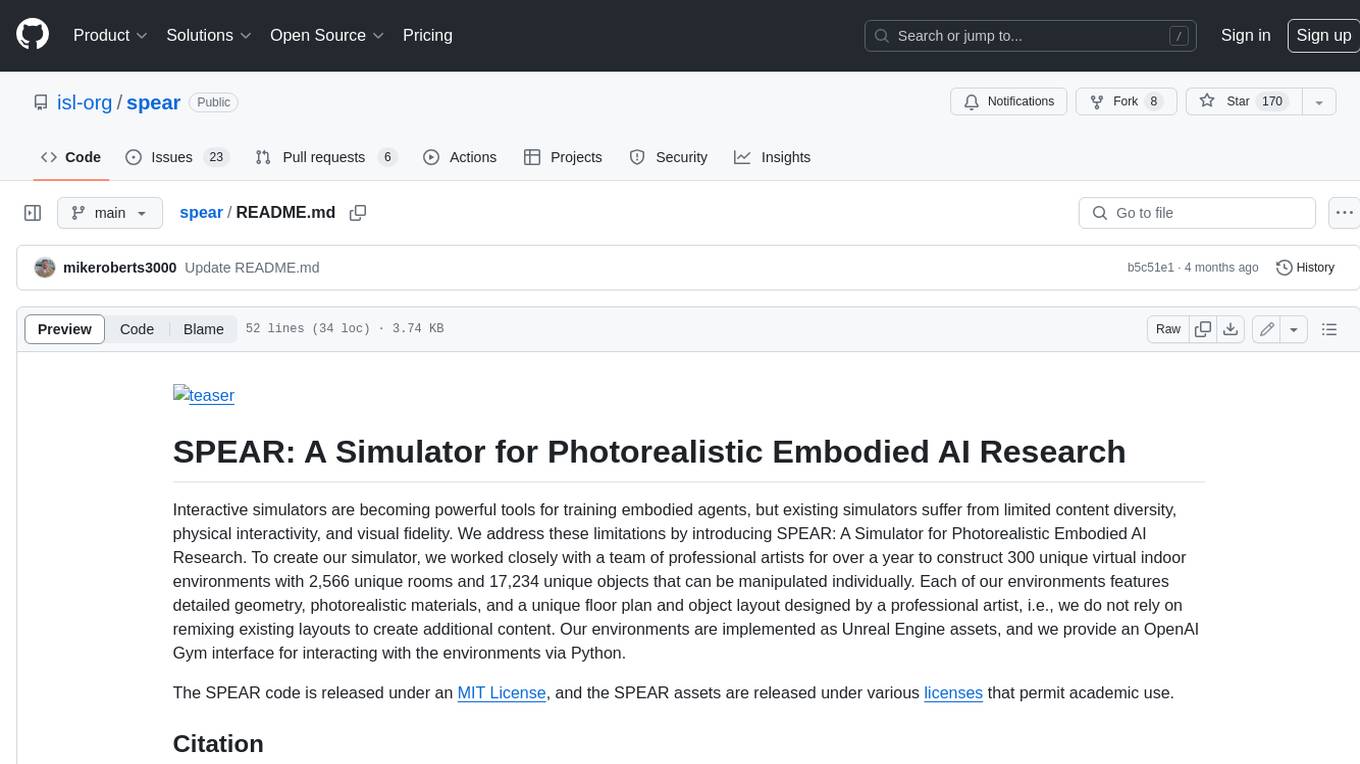
spear
SPEAR (Simulator for Photorealistic Embodied AI Research) is a powerful tool for training embodied agents. It features 300 unique virtual indoor environments with 2,566 unique rooms and 17,234 unique objects that can be manipulated individually. Each environment is designed by a professional artist and features detailed geometry, photorealistic materials, and a unique floor plan and object layout. SPEAR is implemented as Unreal Engine assets and provides an OpenAI Gym interface for interacting with the environments via Python.
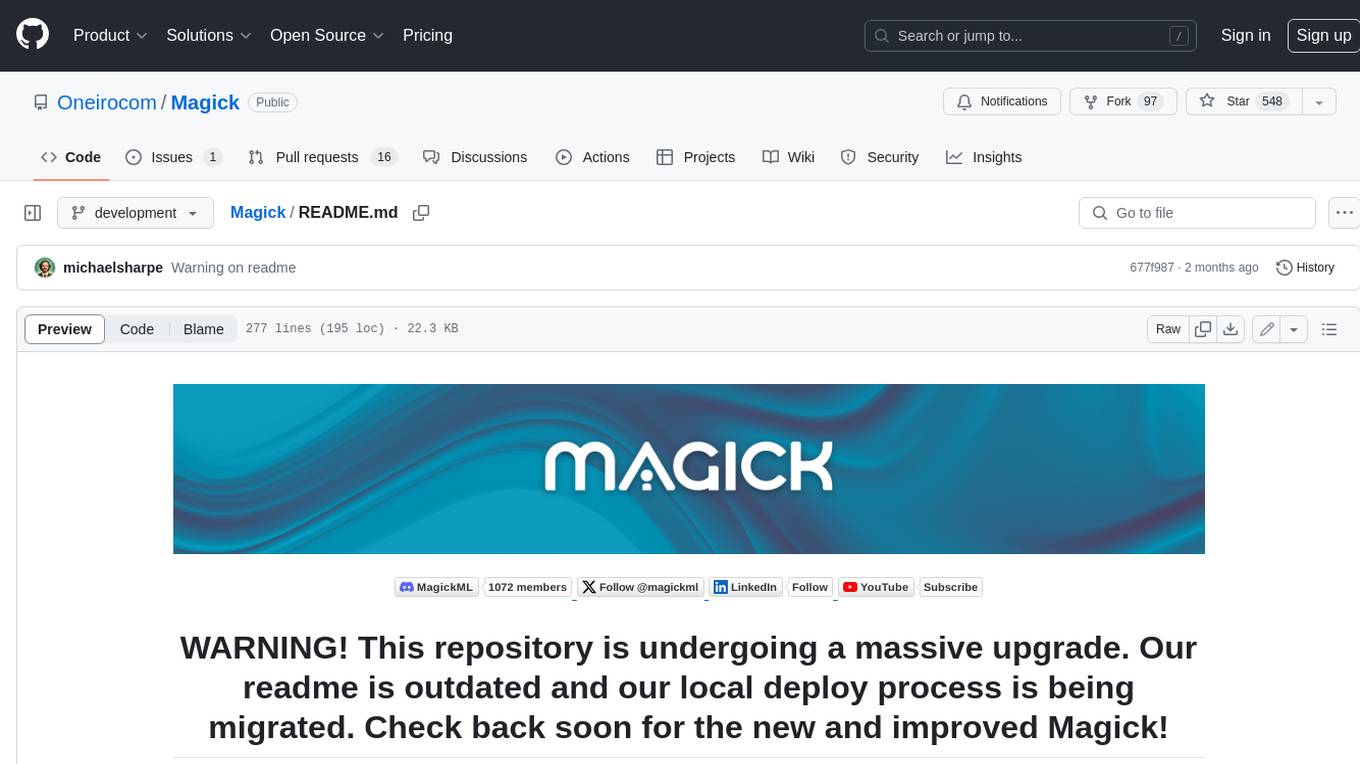
Magick
Magick is a groundbreaking visual AIDE (Artificial Intelligence Development Environment) for no-code data pipelines and multimodal agents. Magick can connect to other services and comes with nodes and templates well-suited for intelligent agents, chatbots, complex reasoning systems and realistic characters.