Best AI tools for< Model Deployment >
Infographic
20 - AI tool Sites
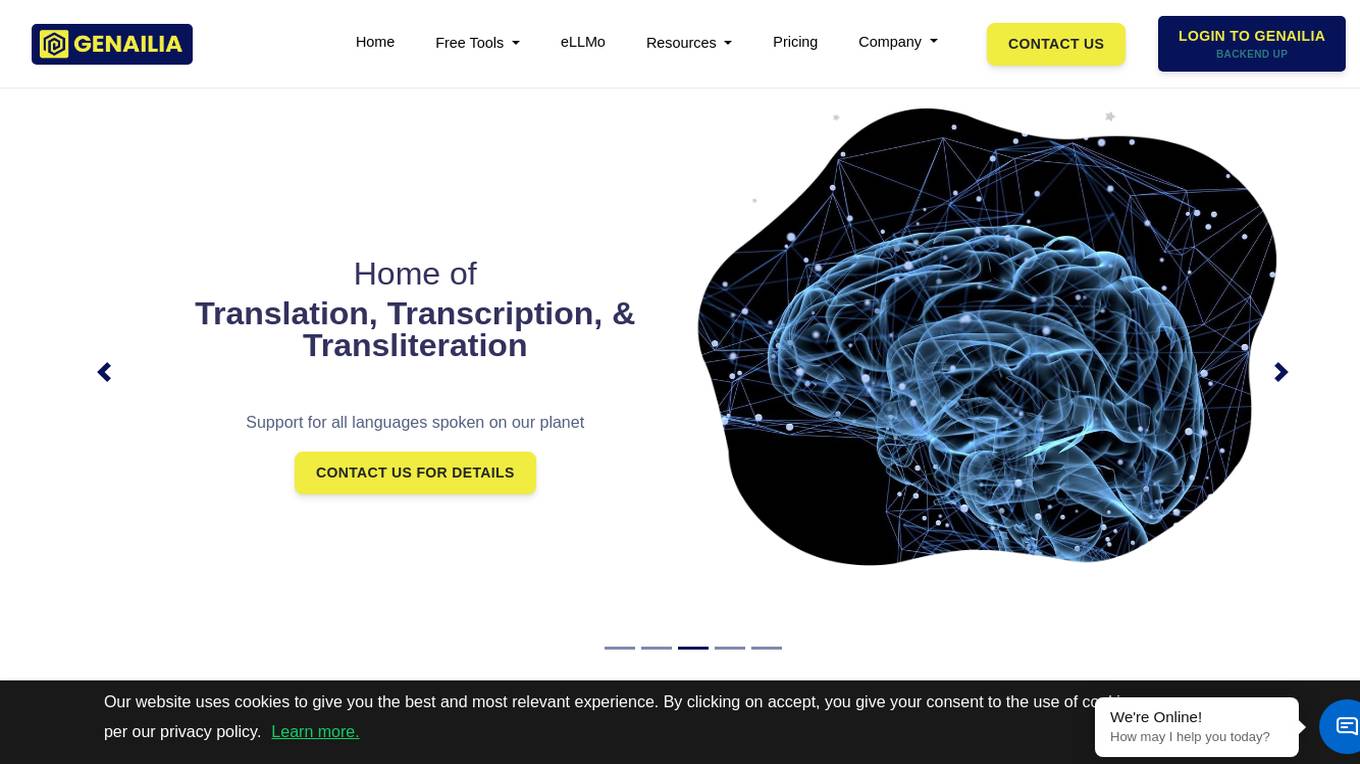
Genailia
Genailia is an AI platform that offers a range of products and services such as translation, transcription, chatbot, LLM, GPT, TTS, ASR, and social media insights. It harnesses AI to redefine possibilities by providing generative AI, linguistic interfaces, accelerators, and more in a single platform. The platform aims to streamline various tasks through AI technology, making it a valuable tool for businesses and individuals seeking efficient solutions.
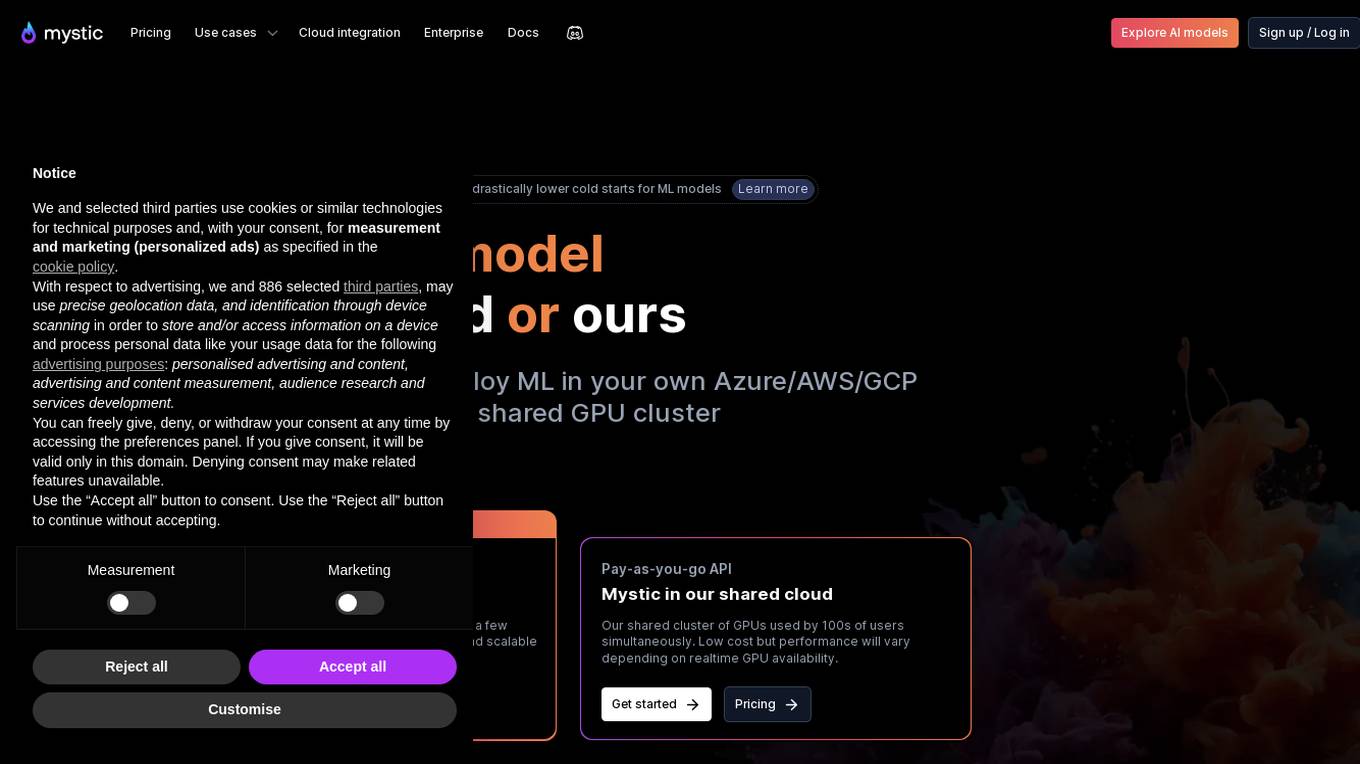
Mystic.ai
Mystic.ai is an AI tool designed to deploy and scale Machine Learning models with ease. It offers a fully managed Kubernetes platform that runs in your own cloud, allowing users to deploy ML models in their own Azure/AWS/GCP account or in a shared GPU cluster. Mystic.ai provides cost optimizations, fast inference, simpler developer experience, and performance optimizations to ensure high-performance AI model serving. With features like pay-as-you-go API, cloud integration with AWS/Azure/GCP, and a beautiful dashboard, Mystic.ai simplifies the deployment and management of ML models for data scientists and AI engineers.
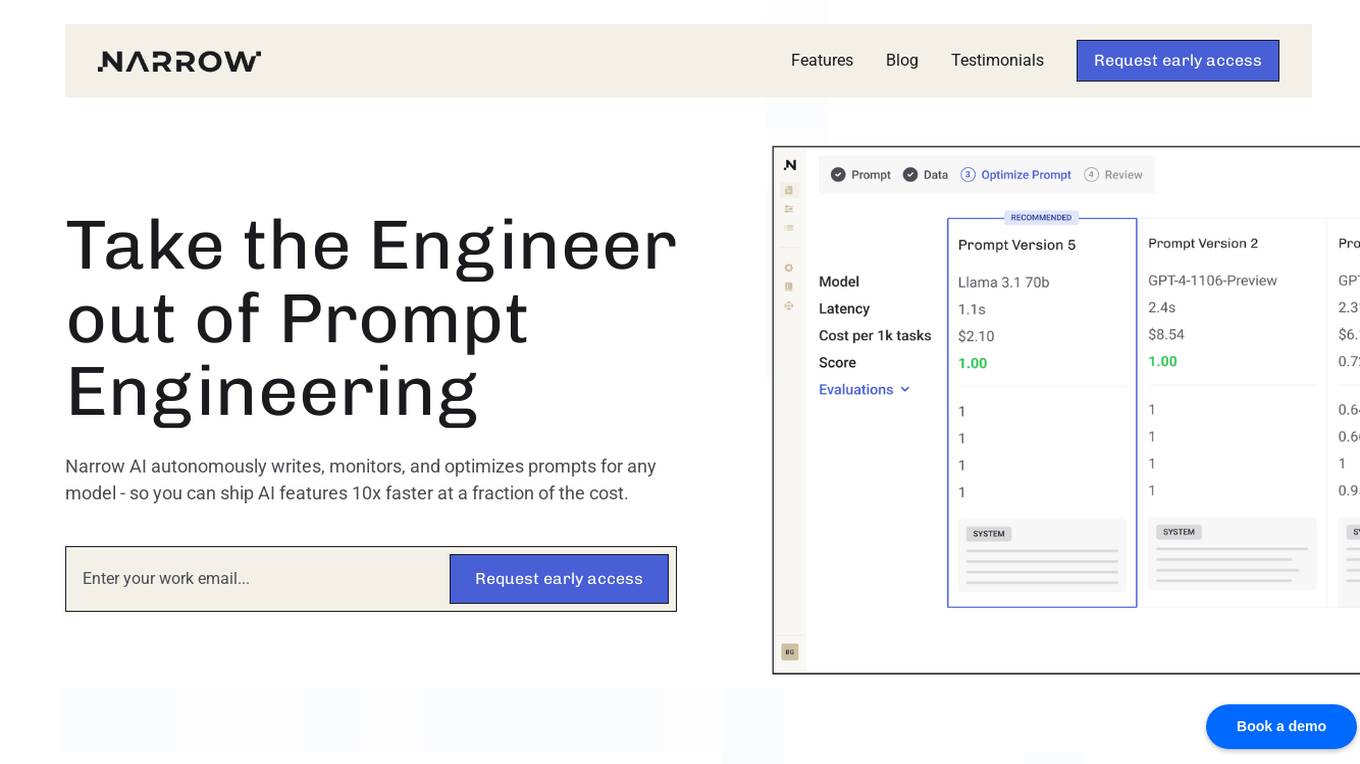
Narrow AI
Narrow AI is an AI application that autonomously writes, monitors, and optimizes prompts for any model, enabling users to ship AI features 10x faster at a fraction of the cost. It streamlines the workflow by allowing users to test new models in minutes, compare prompt performance, and deploy on the optimal model for their use case. Narrow AI helps users maximize efficiency by generating expert-level prompts, adapting prompts to new models, and optimizing prompts for quality, cost, and speed.
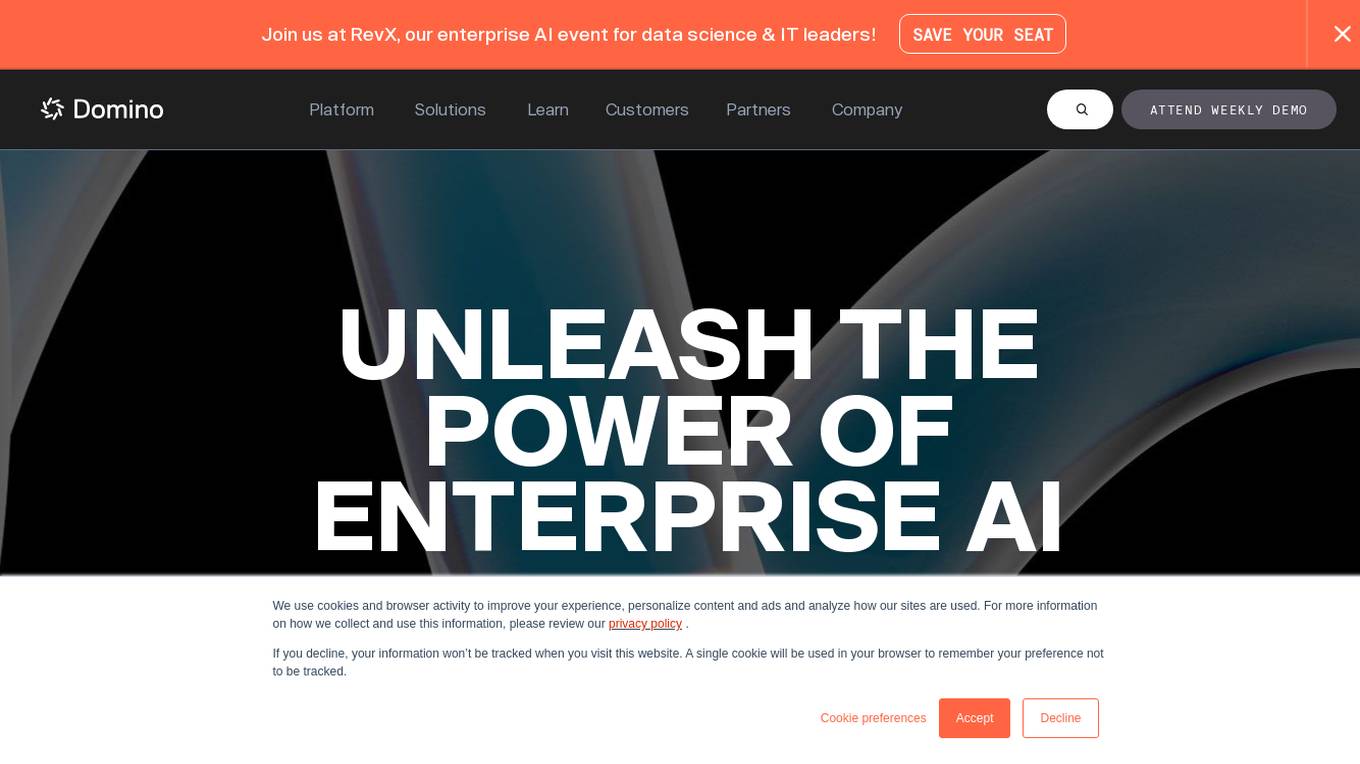
Domino Data Lab
Domino Data Lab is an enterprise AI platform that enables data scientists and IT leaders to build, deploy, and manage AI models at scale. It provides a unified platform for accessing data, tools, compute, models, and projects across any environment. Domino also fosters collaboration, establishes best practices, and tracks models in production to accelerate and scale AI while ensuring governance and reducing costs.
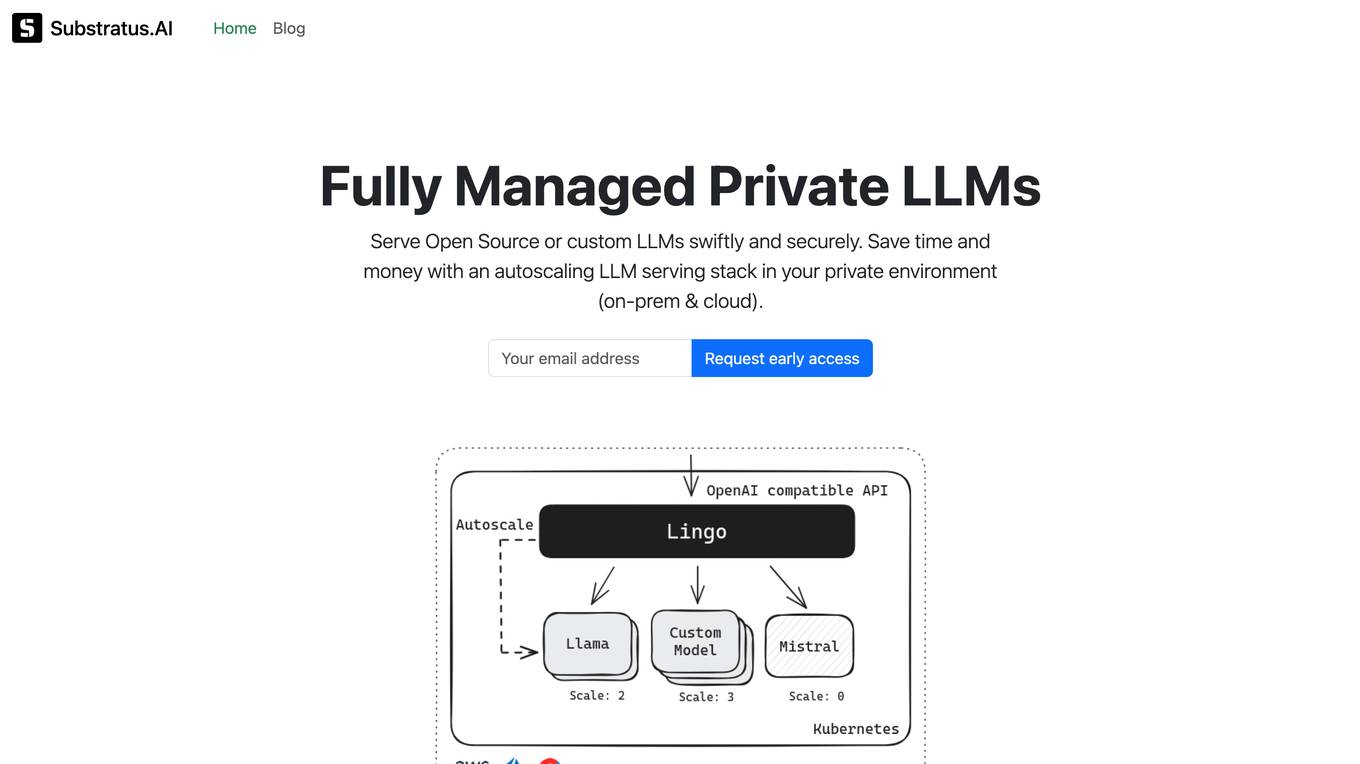
Substratus.AI
Substratus.AI is a fully managed private LLMs platform that allows users to serve LLMs (Llama and Mistral) in their own cloud account. It enables users to keep control of their data while reducing OpenAI costs by up to 10x. With Substratus.AI, users can utilize LLMs in production in hours instead of weeks, making it a convenient and efficient solution for AI model deployment.
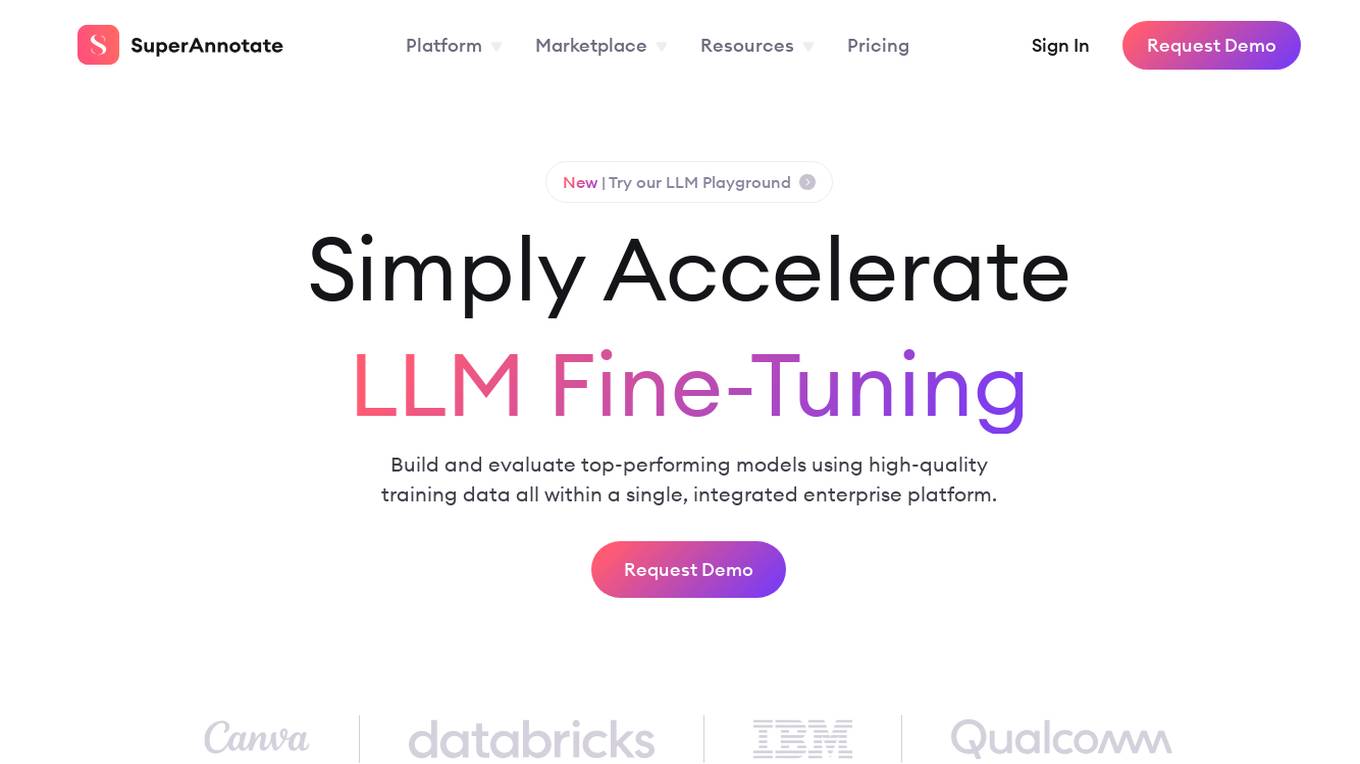
SuperAnnotate
SuperAnnotate is an AI data platform that simplifies and accelerates model-building by unifying the AI pipeline. It enables users to create, curate, and evaluate datasets efficiently, leading to the development of better models faster. The platform offers features like connecting any data source, building customizable UIs, creating high-quality datasets, evaluating models, and deploying models seamlessly. SuperAnnotate ensures global security and privacy measures for data protection.
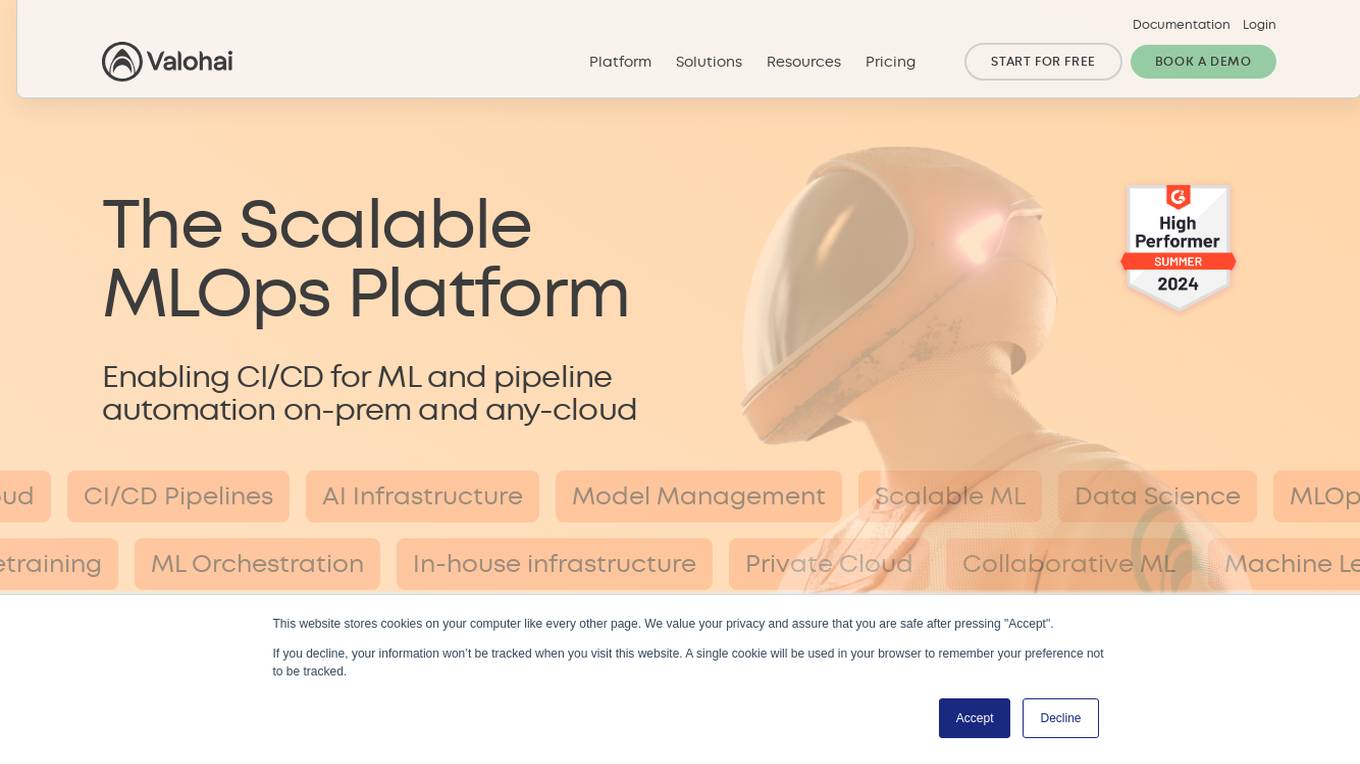
Valohai
Valohai is a scalable MLOps platform that enables Continuous Integration/Continuous Deployment (CI/CD) for machine learning and pipeline automation on-premises and across various cloud environments. It helps streamline complex machine learning workflows by offering framework-agnostic ML capabilities, automatic versioning with complete lineage of ML experiments, hybrid and multi-cloud support, scalability and performance optimization, streamlined collaboration among data scientists, IT, and business units, and smart orchestration of ML workloads on any infrastructure. Valohai also provides a knowledge repository for storing and sharing the entire model lifecycle, facilitating cross-functional collaboration, and allowing developers to build with total freedom using any libraries or frameworks.
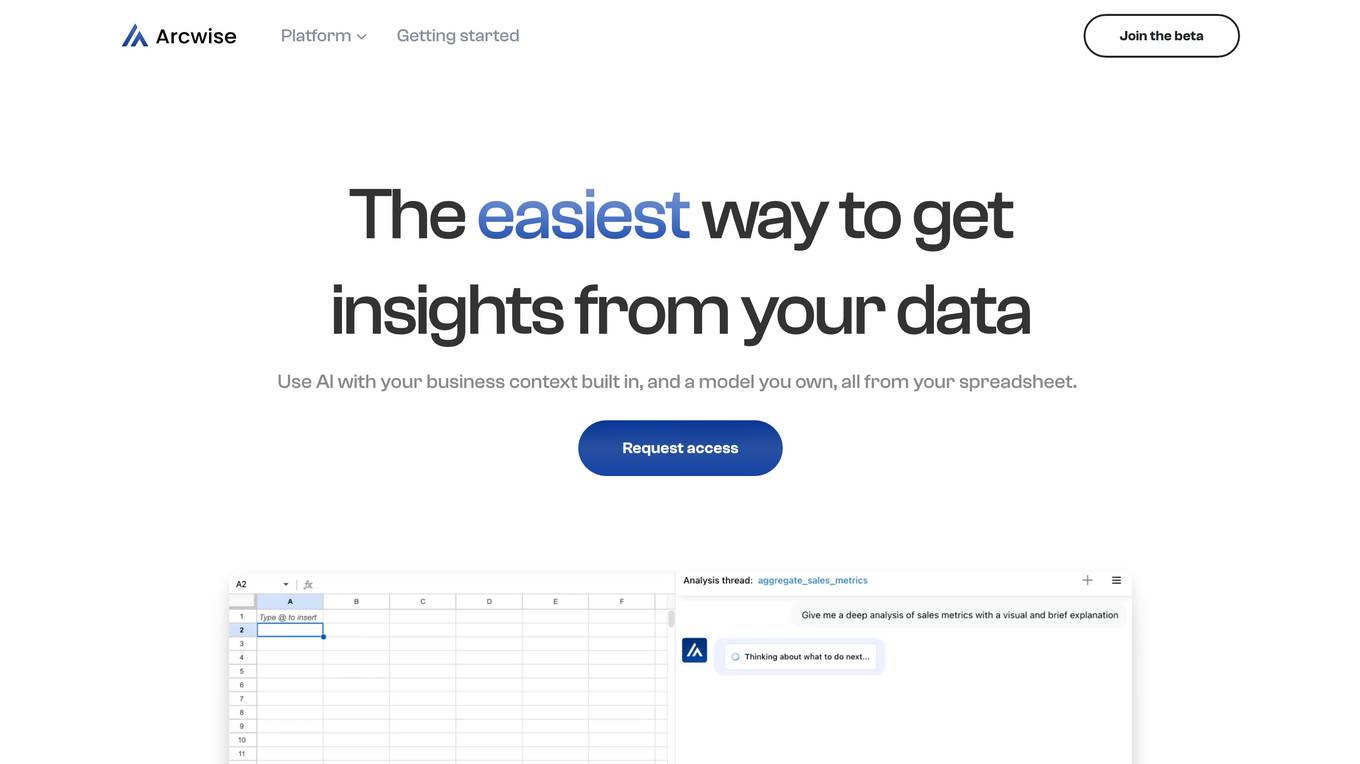
Arcwise
Arcwise is a cloud-based data science platform that provides a comprehensive set of tools for data preparation, exploration, modeling, and deployment. It is designed to make data science accessible to users of all skill levels, from beginners to experts. Arcwise offers a user-friendly interface, drag-and-drop functionality, and a wide range of pre-built templates and algorithms. This makes it easy for users to get started with data science and quickly build and deploy machine learning models.
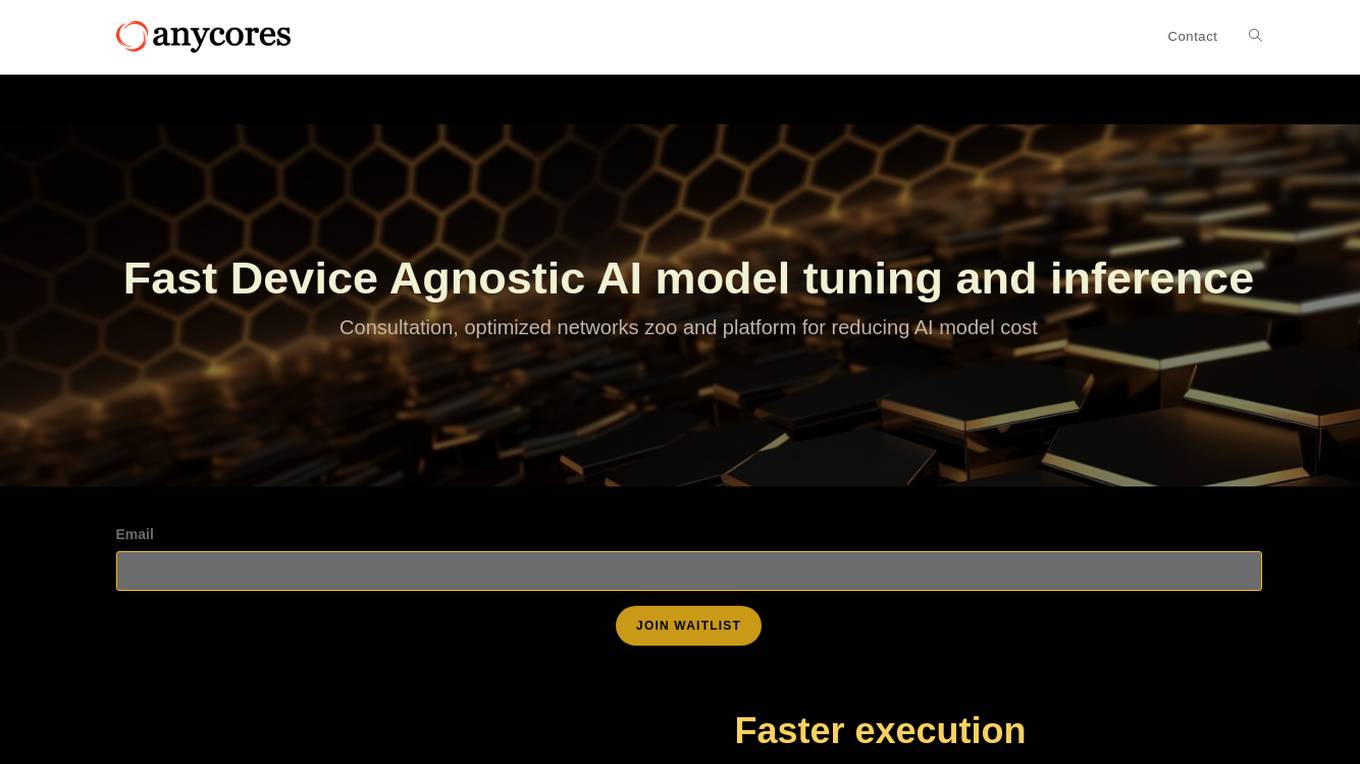
Anycores
Anycores is an AI tool designed to optimize the performance of deep neural networks and reduce the cost of running AI models in the cloud. It offers a platform that provides automated solutions for tuning and inference consultation, optimized networks zoo, and platform for reducing AI model cost. Anycores focuses on faster execution, reducing inference time over 10x times, and footprint reduction during model deployment. It is device agnostic, supporting Nvidia, AMD GPUs, Intel, ARM, AMD CPUs, servers, and edge devices. The tool aims to provide highly optimized, low footprint networks tailored to specific deployment scenarios.
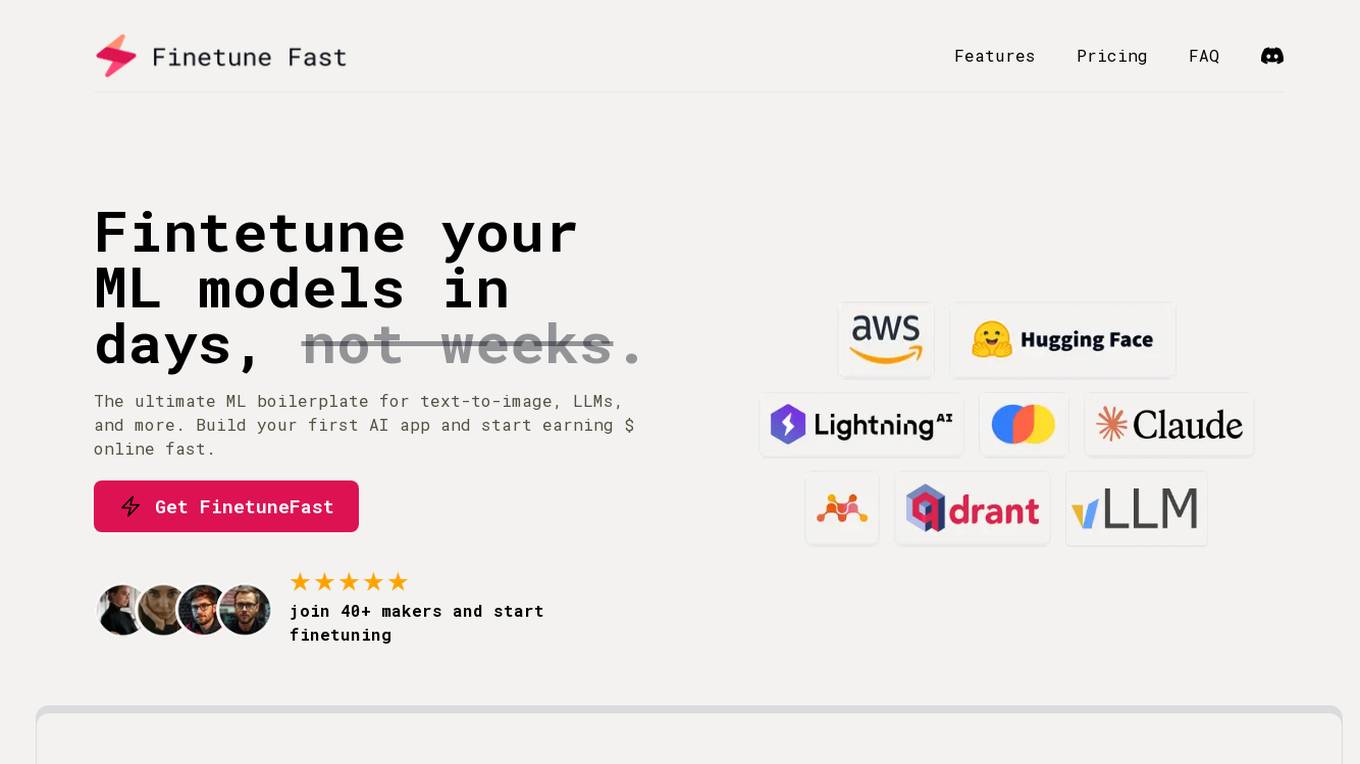
FinetuneFast
FinetuneFast is an AI tool designed to help developers, indie makers, and businesses to efficiently finetune machine learning models, process data, and deploy AI solutions at lightning speed. With pre-configured training scripts, efficient data loading pipelines, and one-click model deployment, FinetuneFast streamlines the process of building and deploying AI models, saving users valuable time and effort. The tool is user-friendly, accessible for ML beginners, and offers lifetime updates for continuous improvement.
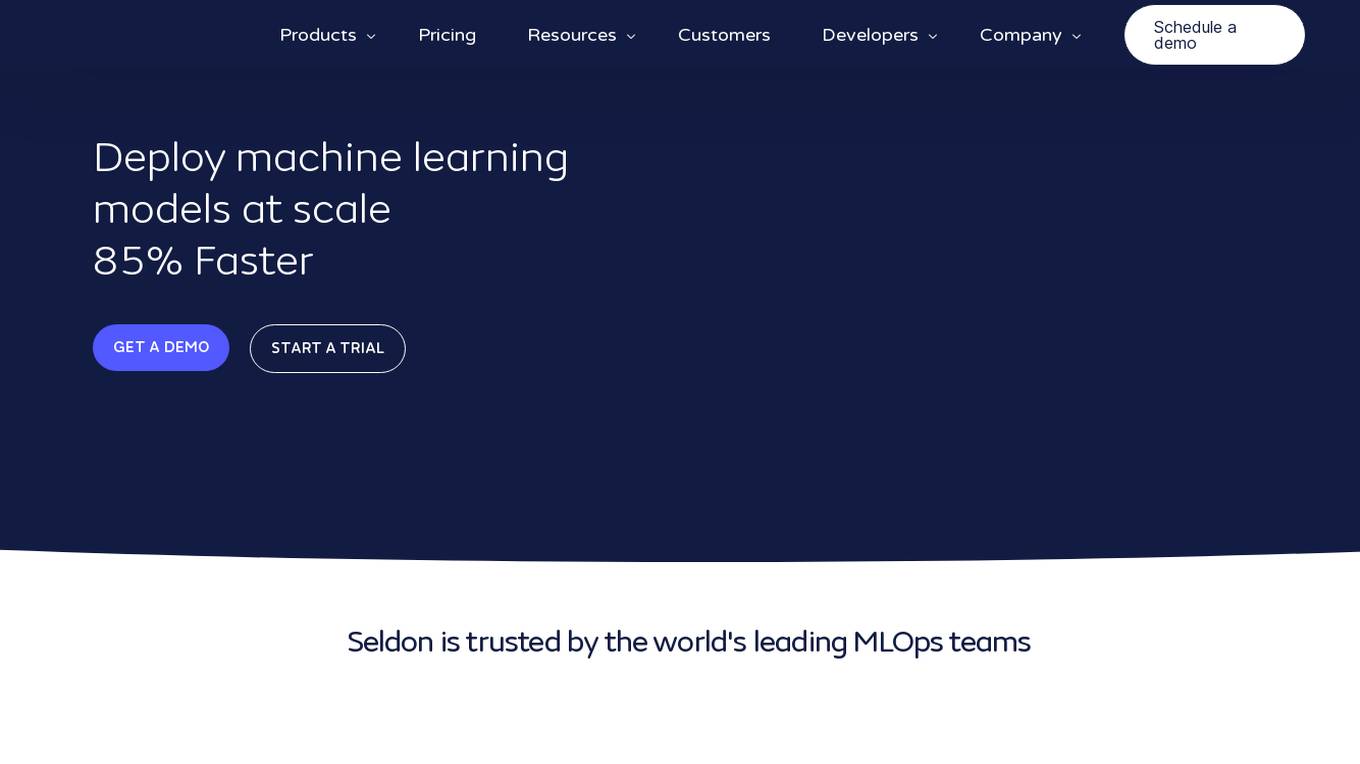
Seldon
Seldon is an MLOps platform that helps enterprises deploy, monitor, and manage machine learning models at scale. It provides a range of features to help organizations accelerate model deployment, optimize infrastructure resource allocation, and manage models and risk. Seldon is trusted by the world's leading MLOps teams and has been used to install and manage over 10 million ML models. With Seldon, organizations can reduce deployment time from months to minutes, increase efficiency, and reduce infrastructure and cloud costs.
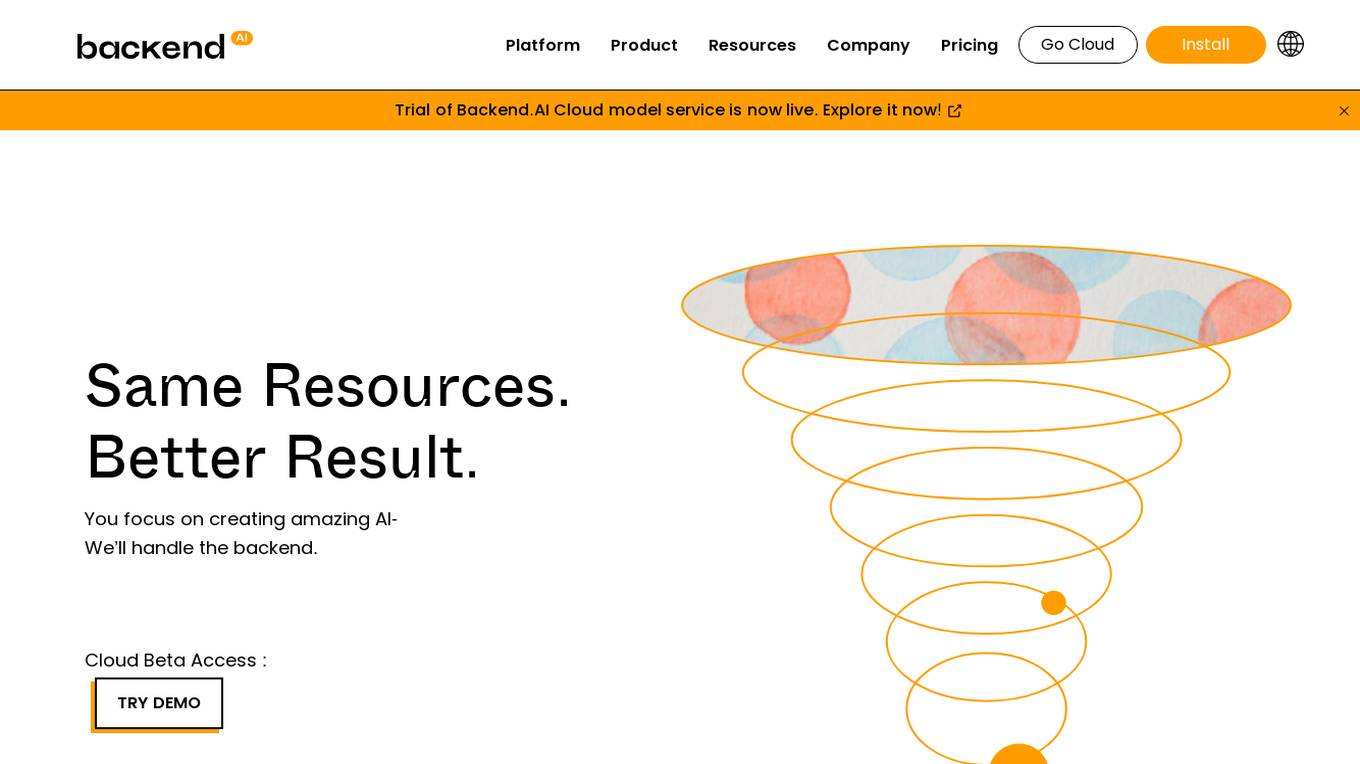
Backend.AI
Backend.AI is an enterprise-scale cluster backend for AI frameworks that offers scalability, GPU virtualization, HPC optimization, and DGX-Ready software products. It provides a fast and efficient way to build, train, and serve AI models of any type and size, with flexible infrastructure options. Backend.AI aims to optimize backend resources, reduce costs, and simplify deployment for AI developers and researchers. The platform integrates seamlessly with existing tools and offers fractional GPU usage and pay-as-you-play model to maximize resource utilization.
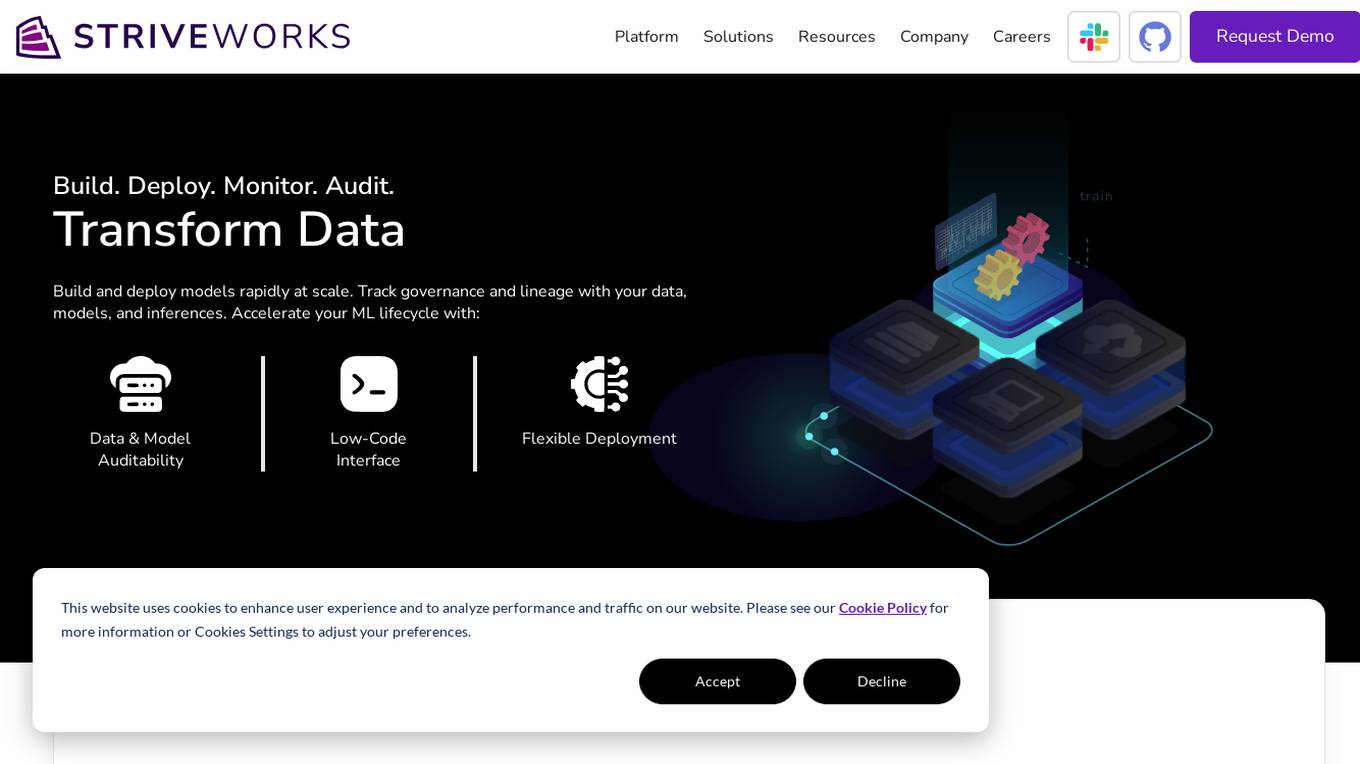
Striveworks
Striveworks is an AI application that offers a Machine Learning Operations Platform designed to help organizations build, deploy, maintain, monitor, and audit machine learning models efficiently. It provides features such as rapid model deployment, data and model auditability, low-code interface, flexible deployment options, and operationalizing AI data science with real returns. Striveworks aims to accelerate the ML lifecycle, save time and money in model creation, and enable non-experts to leverage AI for data-driven decisions.
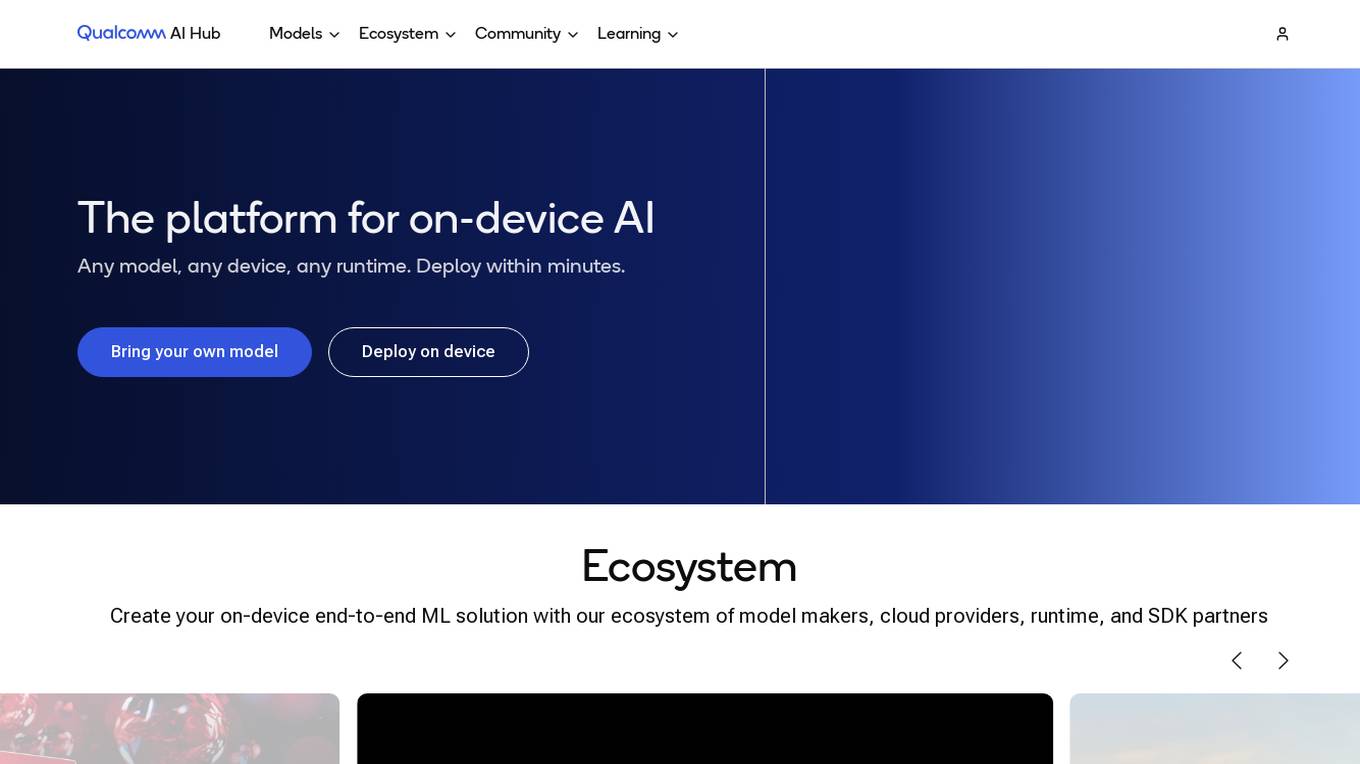
Qualcomm AI Hub
Qualcomm AI Hub is a platform that allows users to run AI models on Snapdragon® 8 Elite devices. It provides a collaborative ecosystem for model makers, cloud providers, runtime, and SDK partners to deploy on-device AI solutions quickly and efficiently. Users can bring their own models, optimize for deployment, and access a variety of AI services and resources. The platform caters to various industries such as mobile, automotive, and IoT, offering a range of models and services for edge computing.
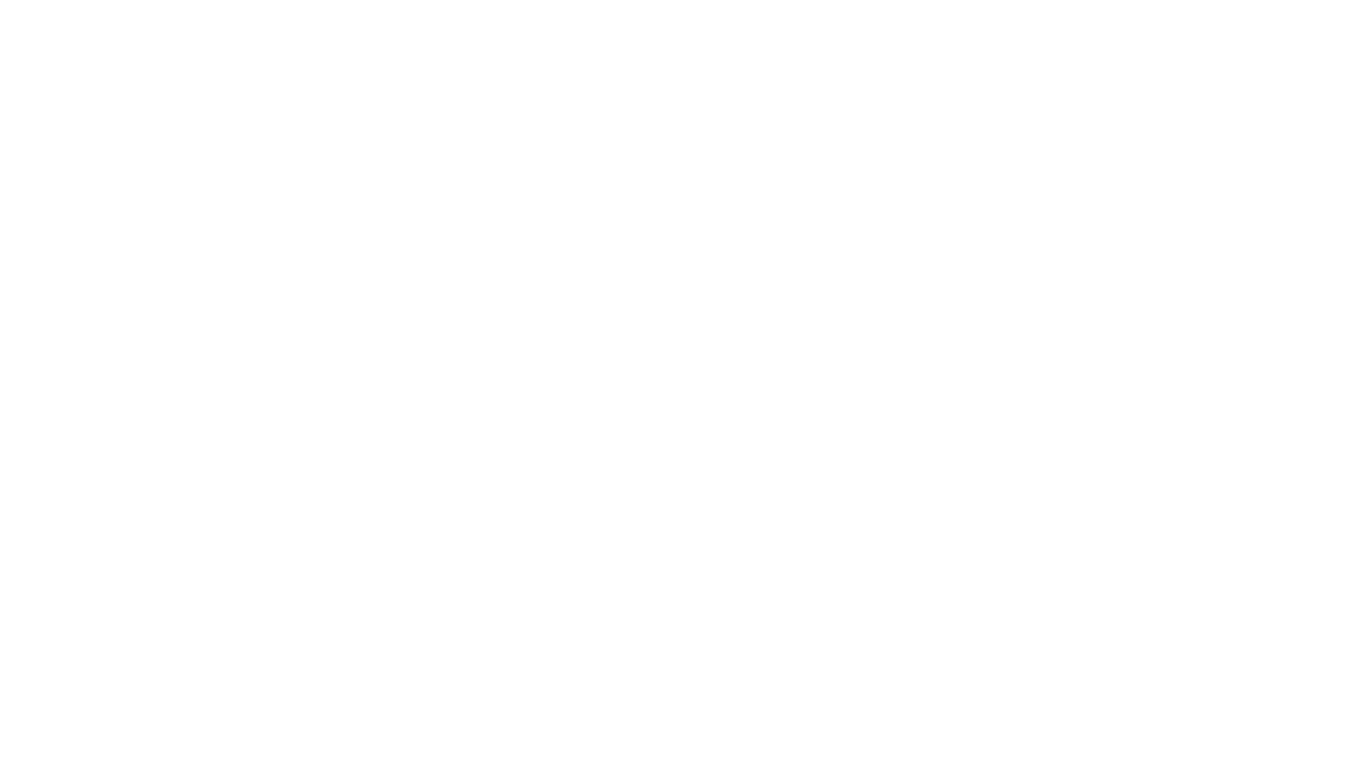
HappyML
HappyML is an AI tool designed to assist users in machine learning tasks. It provides a user-friendly interface for running machine learning algorithms without the need for complex coding. With HappyML, users can easily build, train, and deploy machine learning models for various applications. The tool offers a range of features such as data preprocessing, model evaluation, hyperparameter tuning, and model deployment. HappyML simplifies the machine learning process, making it accessible to users with varying levels of expertise.
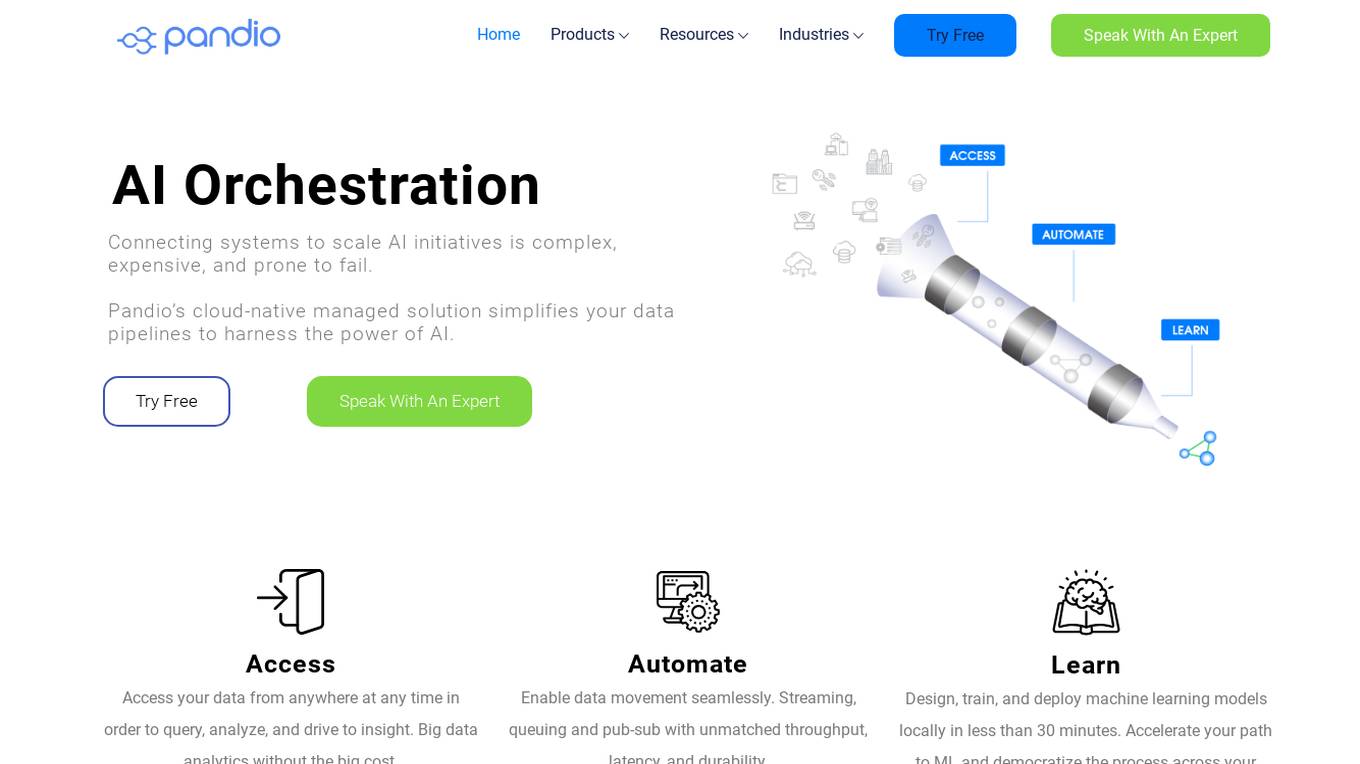
Pandio
Pandio is an AI orchestration platform that simplifies data pipelines to harness the power of AI. It offers cloud-native managed solutions to connect systems, automate data movement, and accelerate machine learning model deployment. Pandio's AI-driven architecture orchestrates models, data, and ML tools to drive AI automation and data-driven decisions faster. The platform is designed for price-performance, offering data movement at high speed and low cost, with near-infinite scalability and compatibility with any data, tools, or cloud environment.
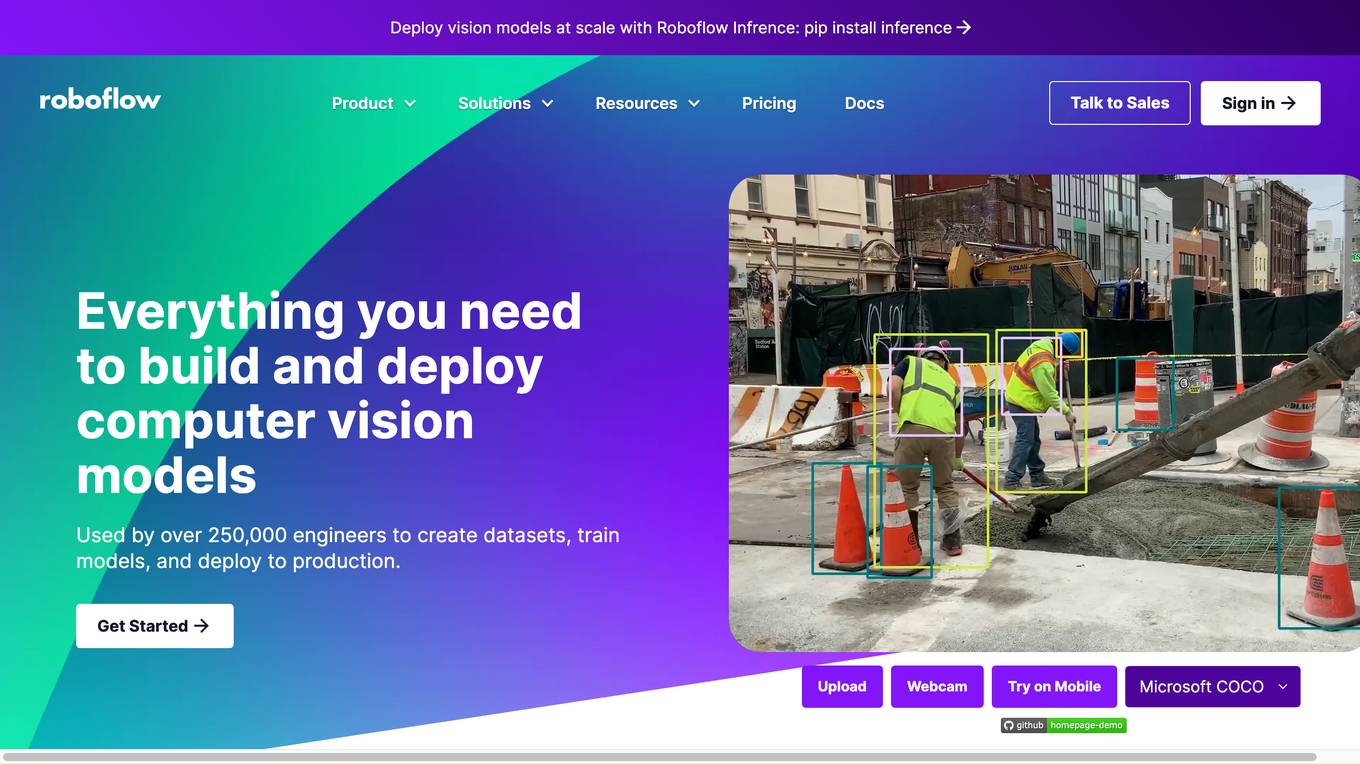
Roboflow
Roboflow is a platform that provides tools for building and deploying computer vision models. It offers a range of features, including data annotation, model training, and deployment. Roboflow is used by over 250,000 engineers to create datasets, train models, and deploy to production.
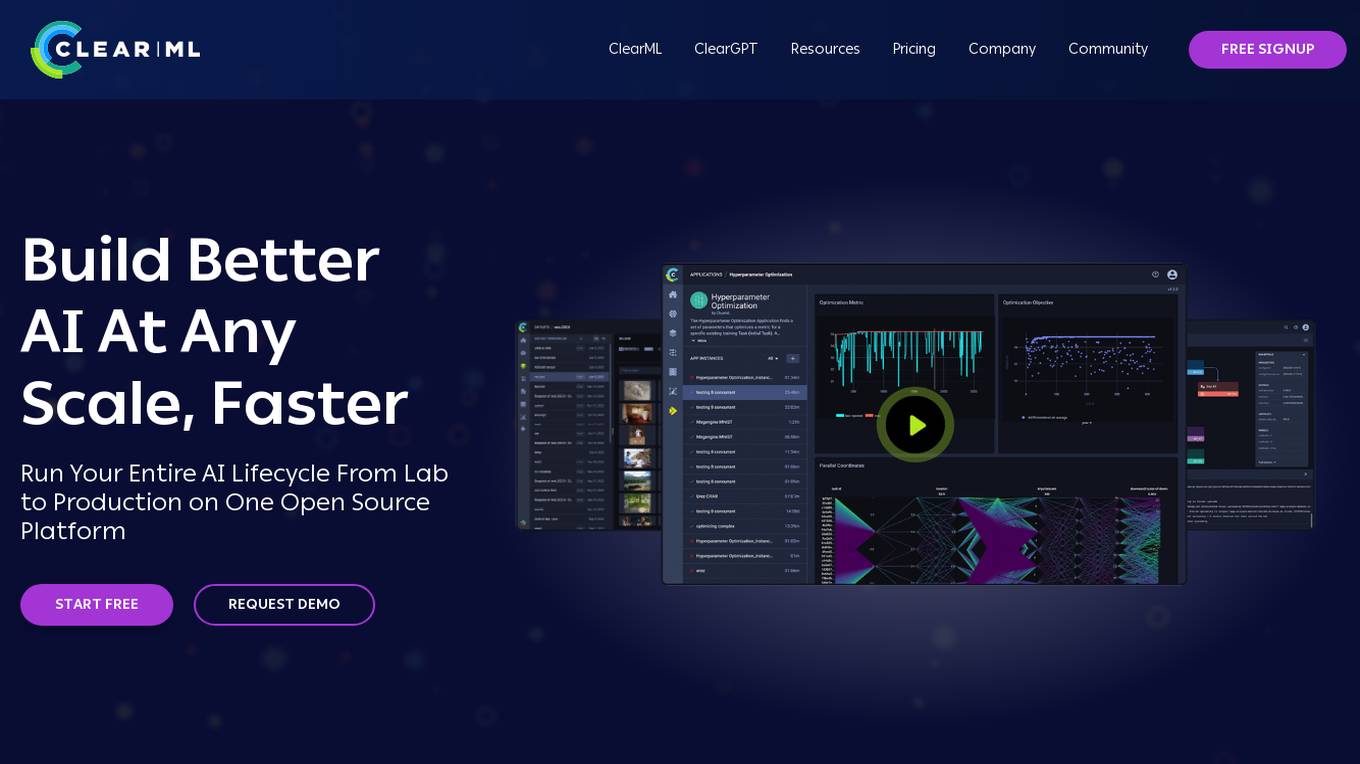
ClearML
ClearML is an open-source, end-to-end platform for continuous machine learning (ML). It provides a unified platform for data management, experiment tracking, model training, deployment, and monitoring. ClearML is designed to make it easy for teams to collaborate on ML projects and to ensure that models are deployed and maintained in a reliable and scalable way.
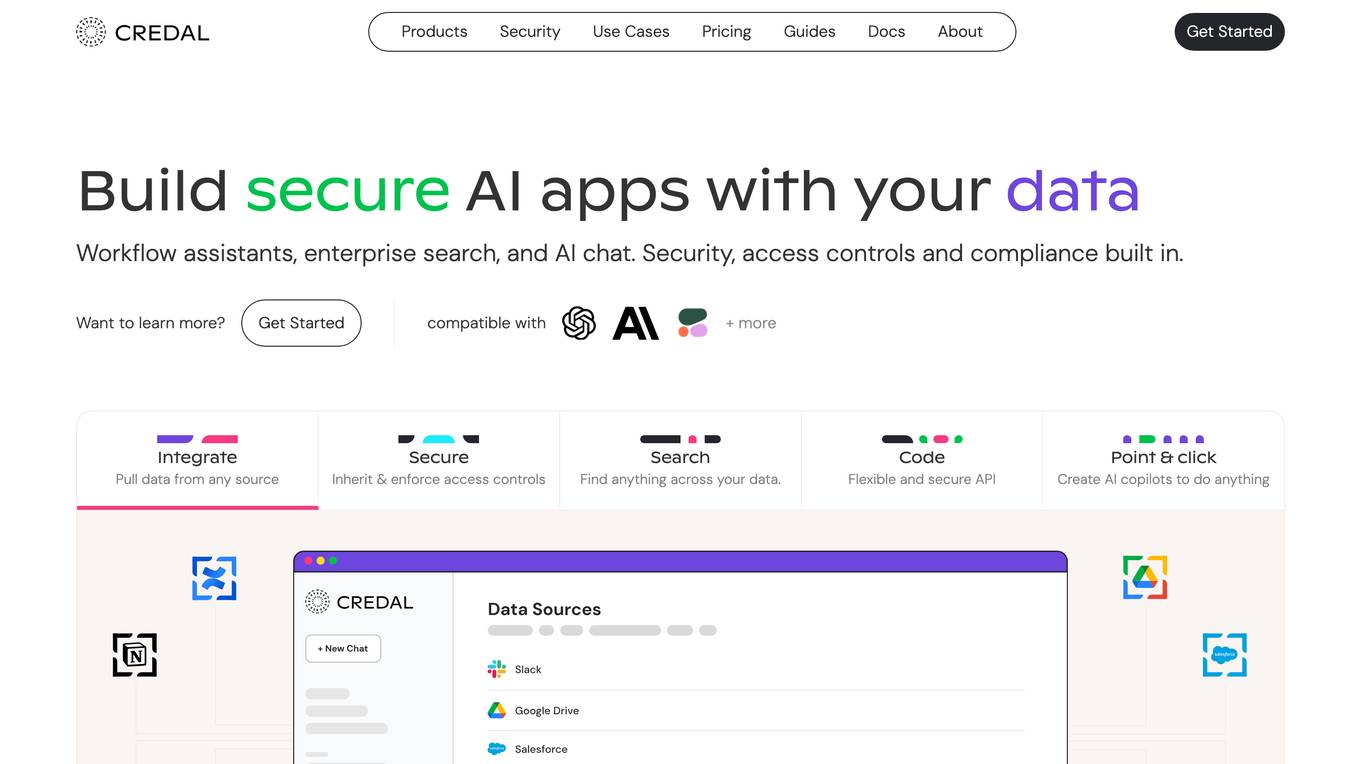
Credal
Credal is an AI tool that allows users to build secure AI assistants for enterprise operations. It enables every employee to create customized AI assistants with built-in security, permissions, and compliance features. Credal supports data integration, access control, search functionalities, and API development. The platform offers real-time sync, automatic permissions synchronization, and AI model deployment with security and compliance measures. It helps enterprises manage ETL pipelines, schedule tasks, and configure data processing. Credal ensures data protection, compliance with regulations like HIPAA, and comprehensive audit capabilities for generative AI applications.
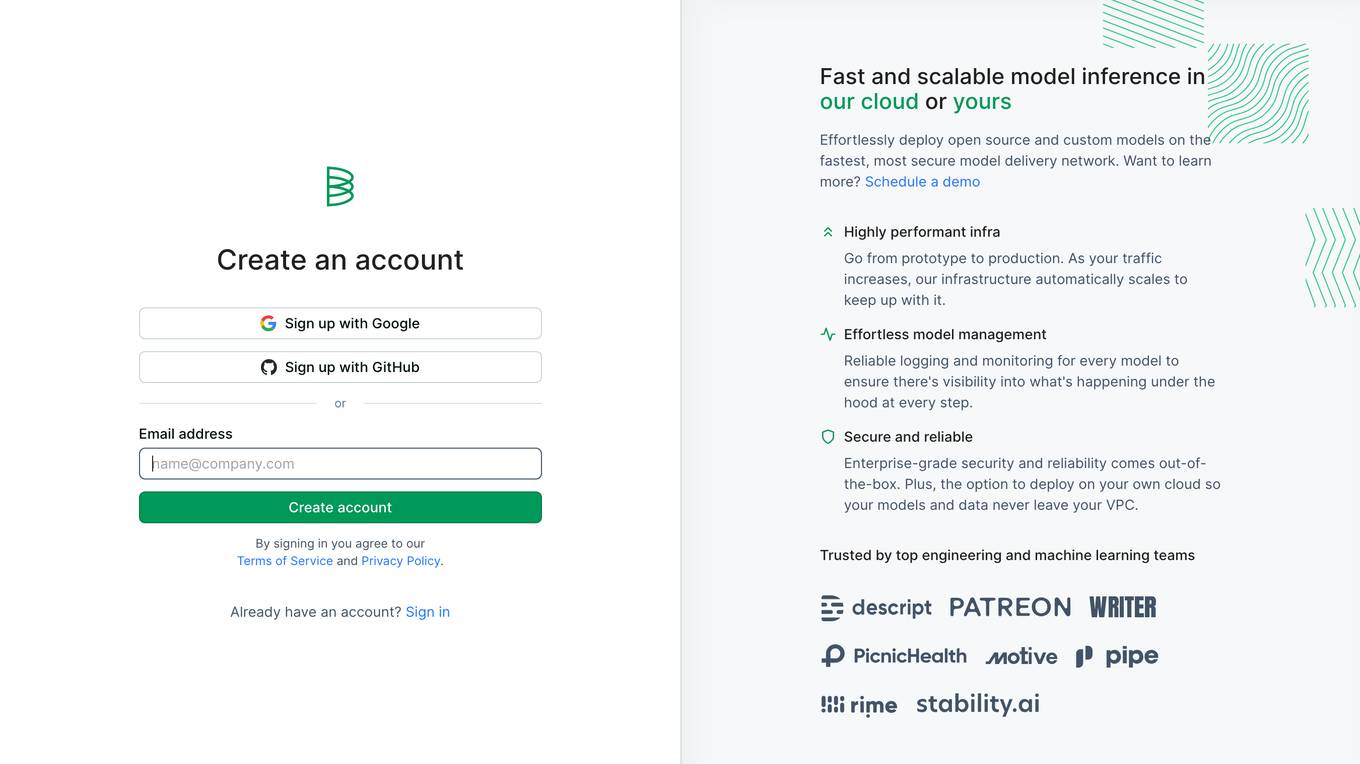
Baseten
Baseten is a machine learning infrastructure that provides a unified platform for data scientists and engineers to build, train, and deploy machine learning models. It offers a range of features to simplify the ML lifecycle, including data preparation, model training, and deployment. Baseten also provides a marketplace of pre-built models and components that can be used to accelerate the development of ML applications.
20 - Open Source Tools
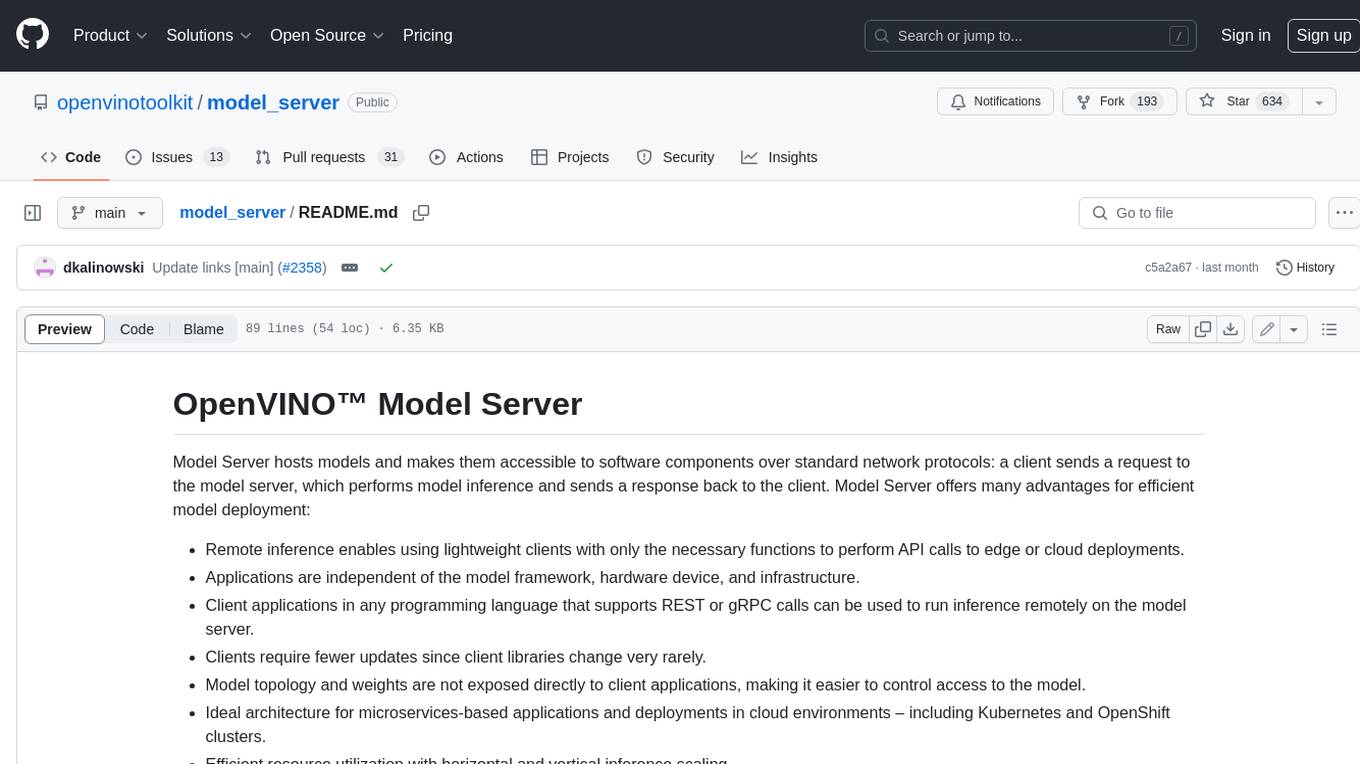
model_server
OpenVINO™ Model Server (OVMS) is a high-performance system for serving models. Implemented in C++ for scalability and optimized for deployment on Intel architectures, the model server uses the same architecture and API as TensorFlow Serving and KServe while applying OpenVINO for inference execution. Inference service is provided via gRPC or REST API, making deploying new algorithms and AI experiments easy.
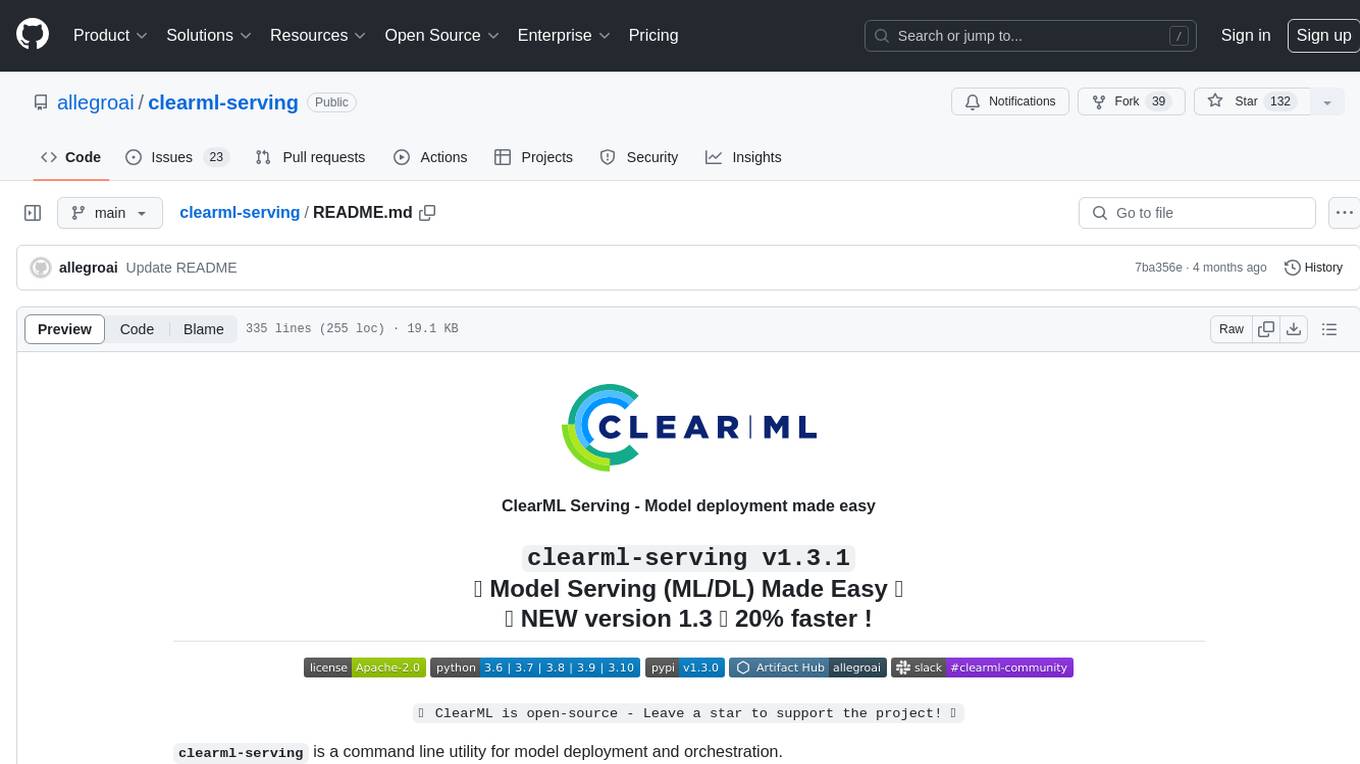
clearml-serving
ClearML Serving is a command line utility for model deployment and orchestration, enabling model deployment including serving and preprocessing code to a Kubernetes cluster or custom container based solution. It supports machine learning models like Scikit Learn, XGBoost, LightGBM, and deep learning models like TensorFlow, PyTorch, ONNX. It provides a customizable RestAPI for serving, online model deployment, scalable solutions, multi-model per container, automatic deployment, canary A/B deployment, model monitoring, usage metric reporting, metric dashboard, and model performance metrics. ClearML Serving is modular, scalable, flexible, customizable, and open source.
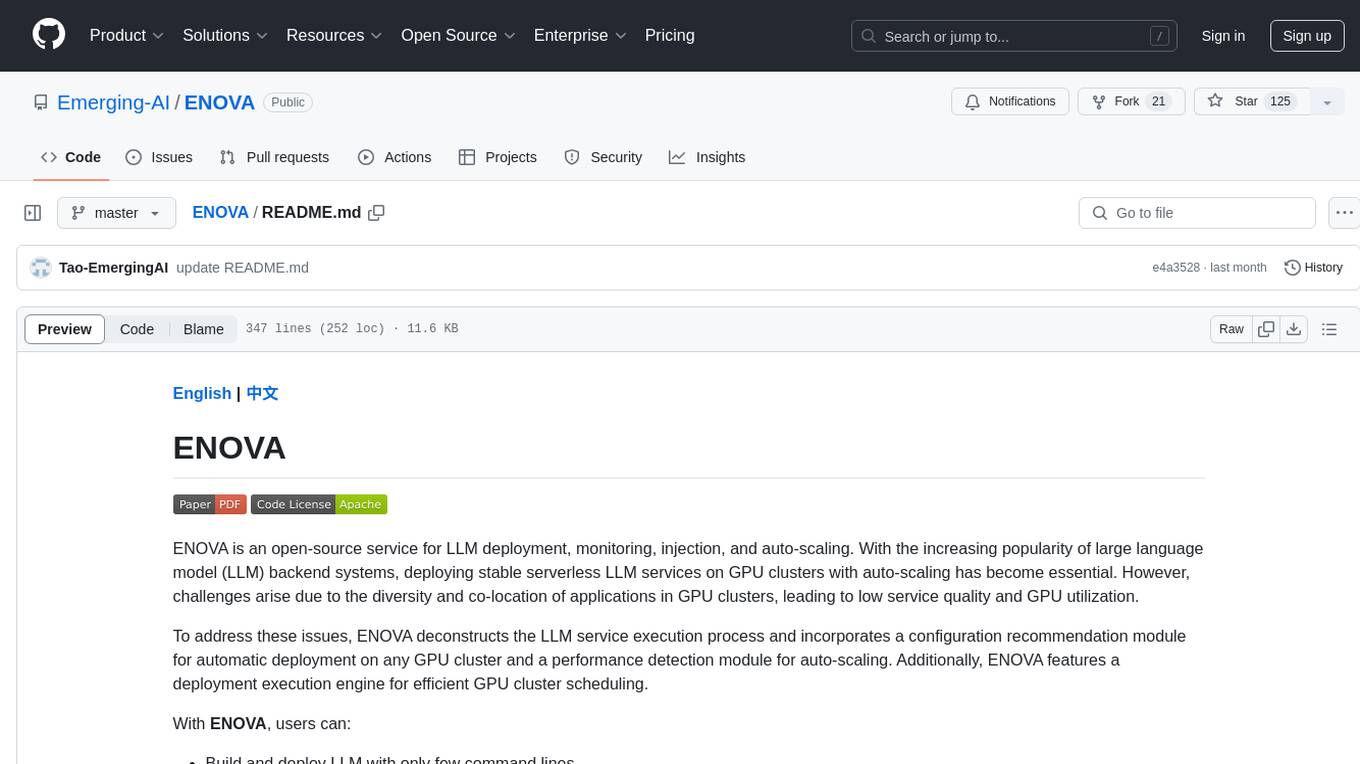
ENOVA
ENOVA is an open-source service for Large Language Model (LLM) deployment, monitoring, injection, and auto-scaling. It addresses challenges in deploying stable serverless LLM services on GPU clusters with auto-scaling by deconstructing the LLM service execution process and providing configuration recommendations and performance detection. Users can build and deploy LLM with few command lines, recommend optimal computing resources, experience LLM performance, observe operating status, achieve load balancing, and more. ENOVA ensures stable operation, cost-effectiveness, efficiency, and strong scalability of LLM services.
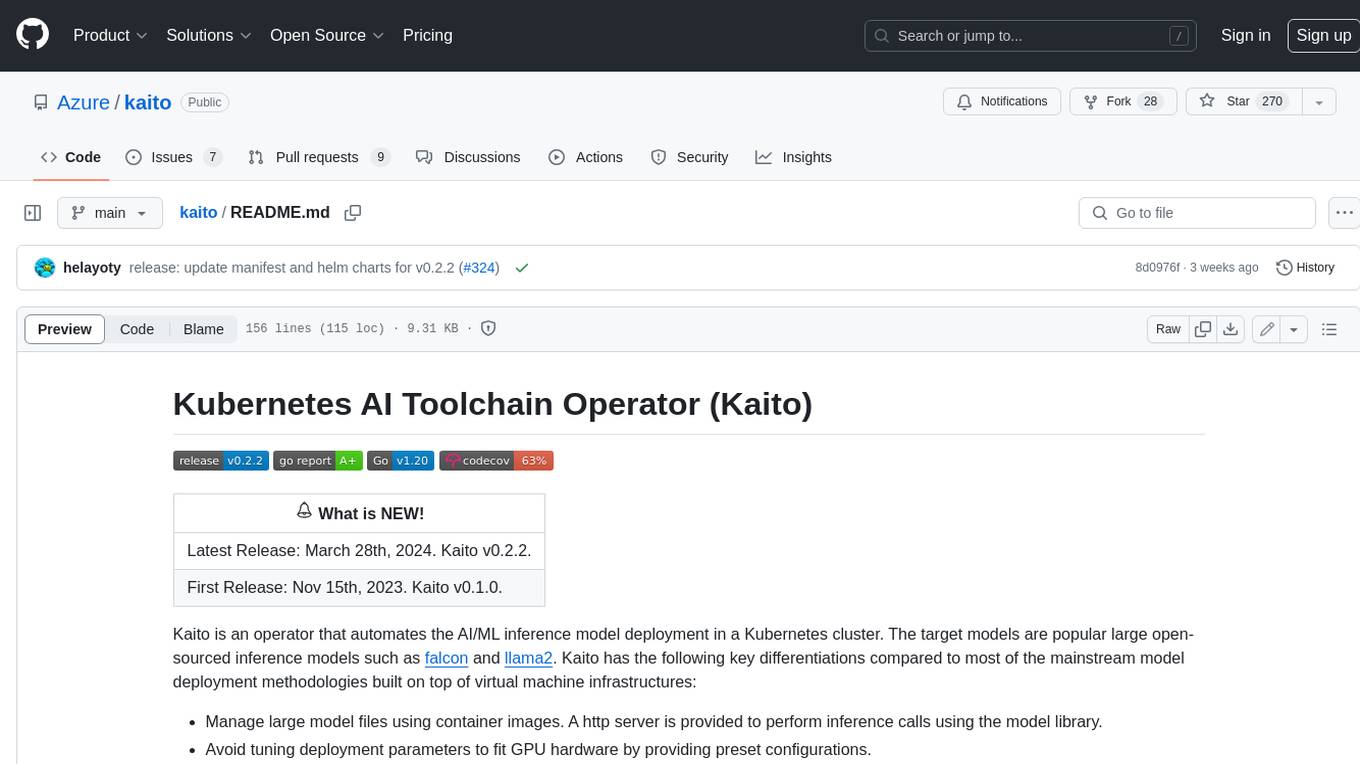
kaito
Kaito is an operator that automates the AI/ML inference model deployment in a Kubernetes cluster. It manages large model files using container images, avoids tuning deployment parameters to fit GPU hardware by providing preset configurations, auto-provisions GPU nodes based on model requirements, and hosts large model images in the public Microsoft Container Registry (MCR) if the license allows. Using Kaito, the workflow of onboarding large AI inference models in Kubernetes is largely simplified.
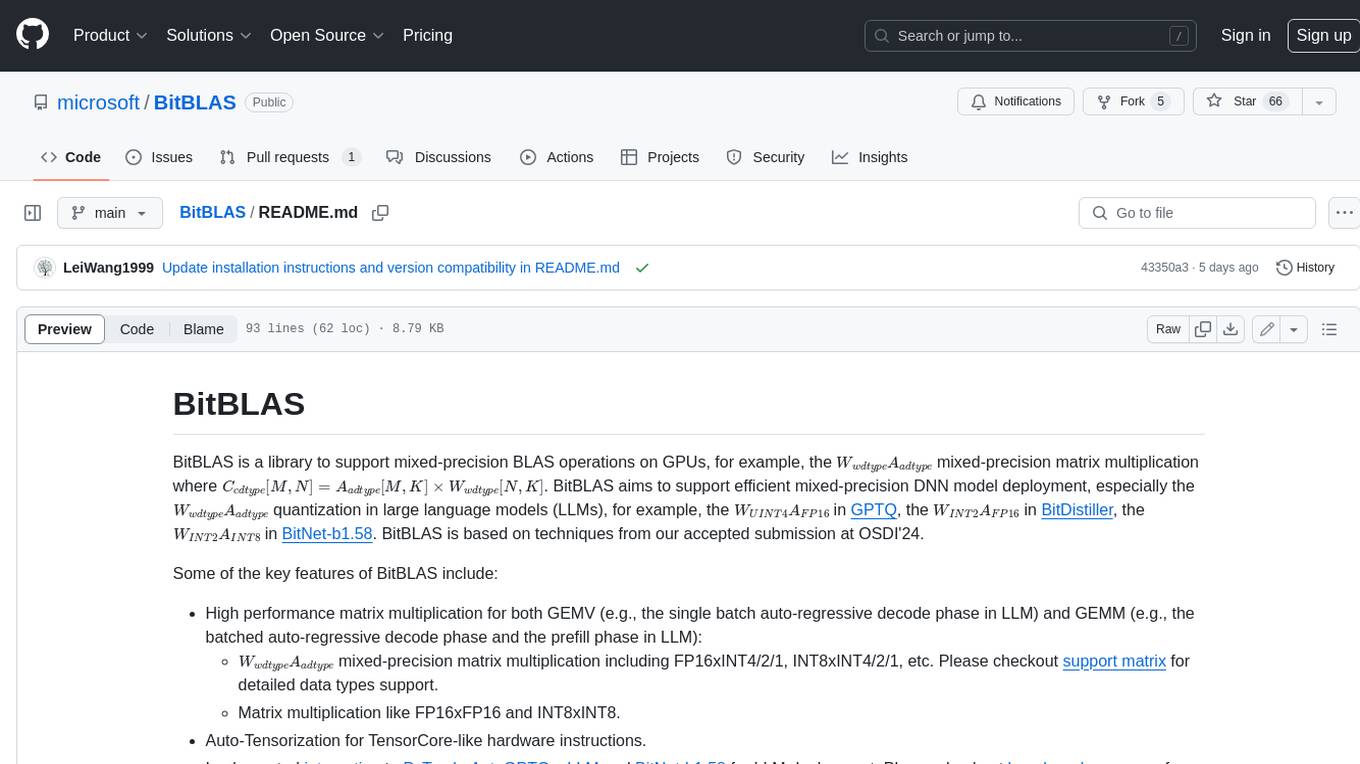
BitBLAS
BitBLAS is a library for mixed-precision BLAS operations on GPUs, for example, the $W_{wdtype}A_{adtype}$ mixed-precision matrix multiplication where $C_{cdtype}[M, N] = A_{adtype}[M, K] \times W_{wdtype}[N, K]$. BitBLAS aims to support efficient mixed-precision DNN model deployment, especially the $W_{wdtype}A_{adtype}$ quantization in large language models (LLMs), for example, the $W_{UINT4}A_{FP16}$ in GPTQ, the $W_{INT2}A_{FP16}$ in BitDistiller, the $W_{INT2}A_{INT8}$ in BitNet-b1.58. BitBLAS is based on techniques from our accepted submission at OSDI'24.
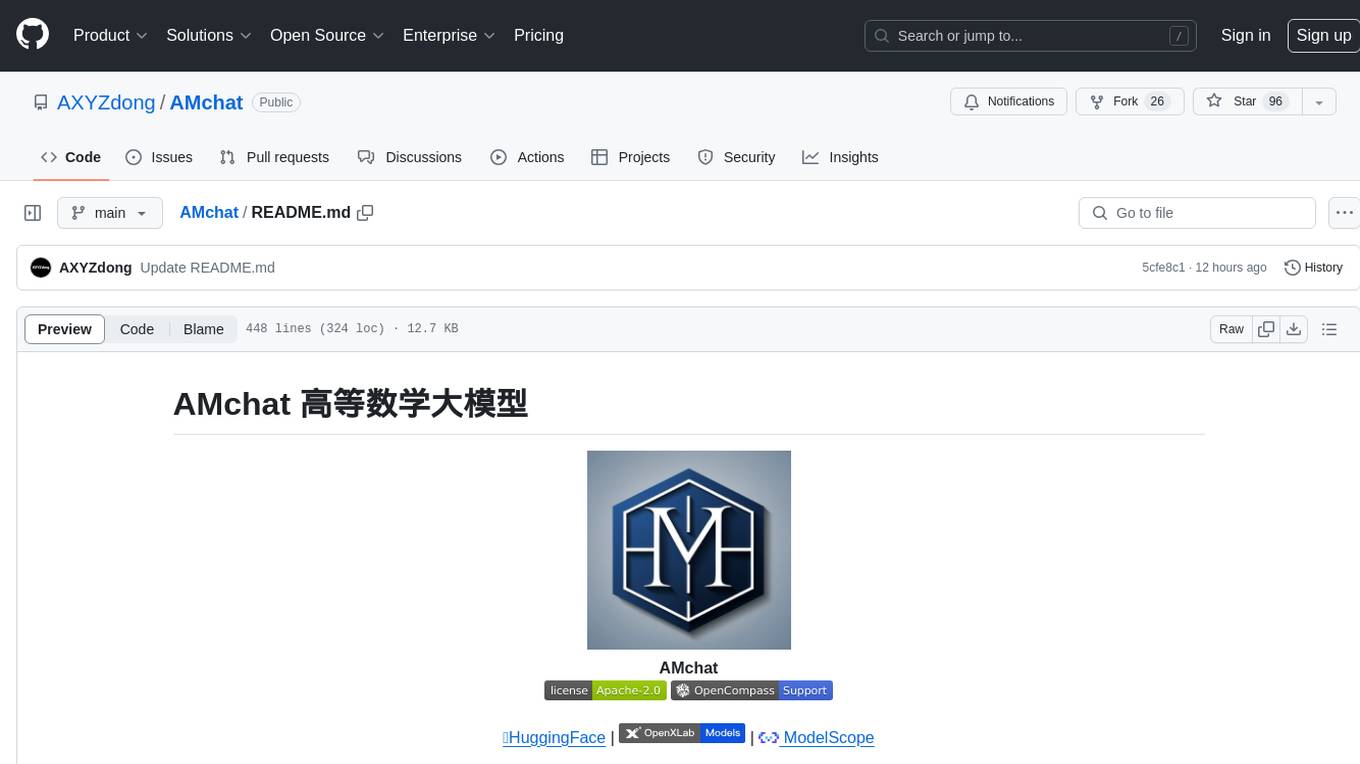
AMchat
AMchat is a large language model that integrates advanced math concepts, exercises, and solutions. The model is based on the InternLM2-Math-7B model and is specifically designed to answer advanced math problems. It provides a comprehensive dataset that combines Math and advanced math exercises and solutions. Users can download the model from ModelScope or OpenXLab, deploy it locally or using Docker, and even retrain it using XTuner for fine-tuning. The tool also supports LMDeploy for quantization, OpenCompass for evaluation, and various other features for model deployment and evaluation. The project contributors have provided detailed documentation and guides for users to utilize the tool effectively.
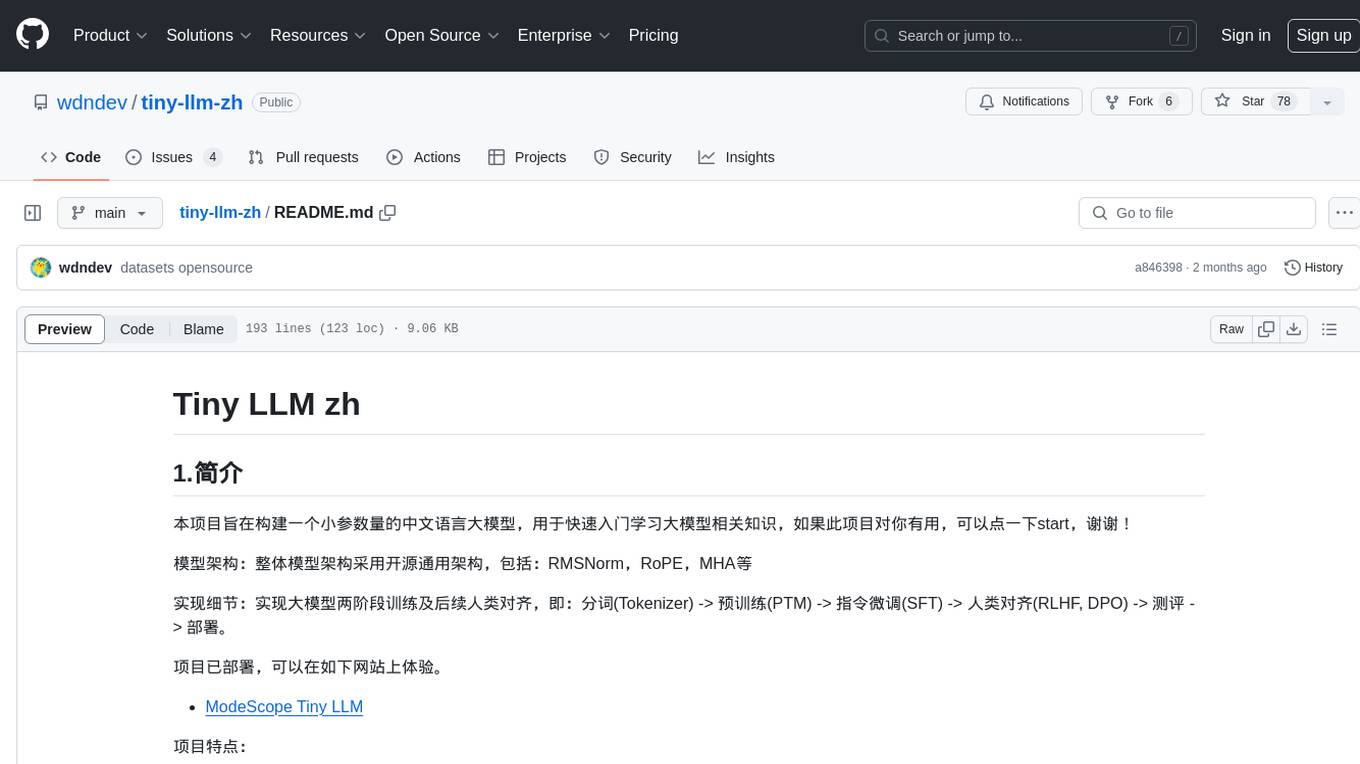
tiny-llm-zh
Tiny LLM zh is a project aimed at building a small-parameter Chinese language large model for quick entry into learning large model-related knowledge. The project implements a two-stage training process for large models and subsequent human alignment, including tokenization, pre-training, instruction fine-tuning, human alignment, evaluation, and deployment. It is deployed on ModeScope Tiny LLM website and features open access to all data and code, including pre-training data and tokenizer. The project trains a tokenizer using 10GB of Chinese encyclopedia text to build a Tiny LLM vocabulary. It supports training with Transformers deepspeed, multiple machine and card support, and Zero optimization techniques. The project has three main branches: llama2_torch, main tiny_llm, and tiny_llm_moe, each with specific modifications and features.
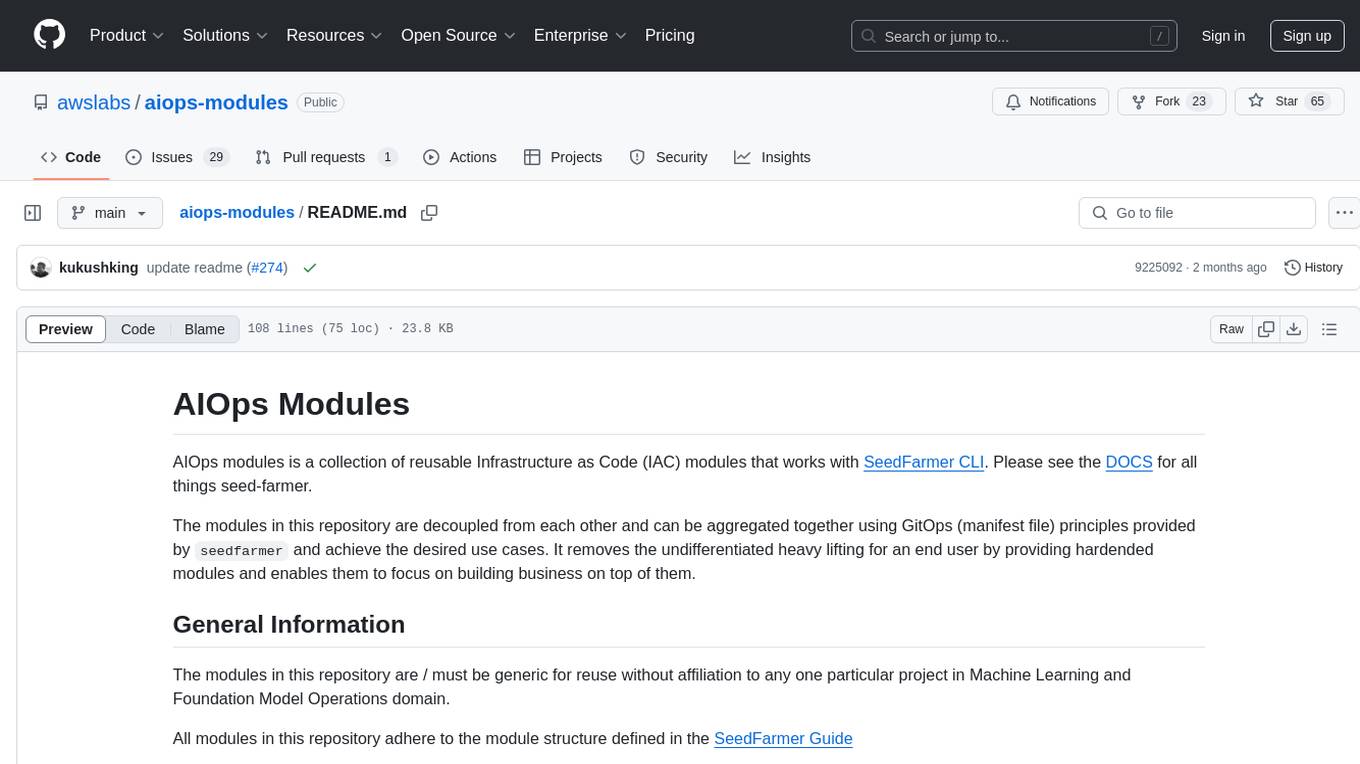
aiops-modules
AIOps Modules is a collection of reusable Infrastructure as Code (IAC) modules that work with SeedFarmer CLI. The modules are decoupled and can be aggregated using GitOps principles to achieve desired use cases, removing heavy lifting for end users. They must be generic for reuse in Machine Learning and Foundation Model Operations domain, adhering to SeedFarmer Guide structure. The repository includes deployment steps, project manifests, and various modules for SageMaker, Mlflow, FMOps/LLMOps, MWAA, Step Functions, EKS, and example use cases. It also supports Industry Data Framework (IDF) and Autonomous Driving Data Framework (ADDF) Modules.
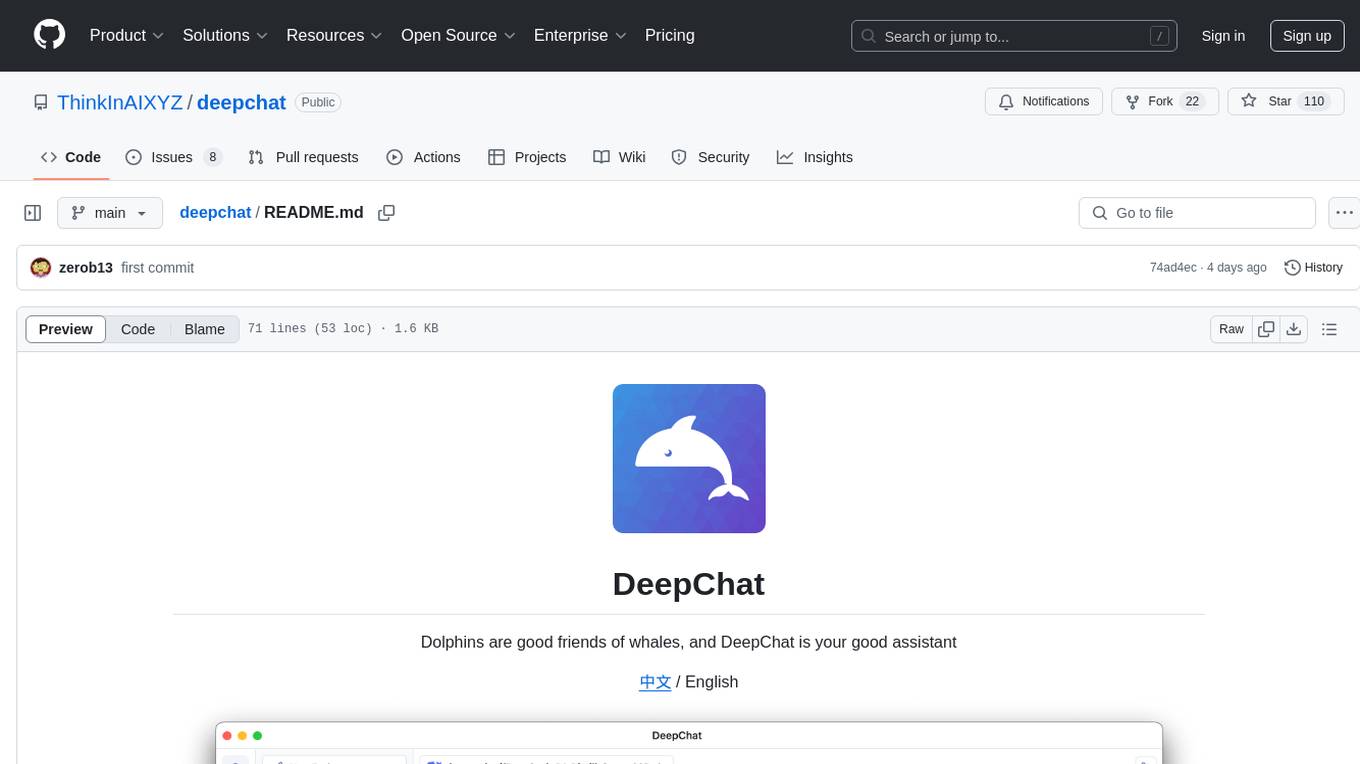
deepchat
DeepChat is a versatile chat tool that supports multiple model cloud services and local model deployment. It offers multi-channel chat concurrency support, platform compatibility, complete Markdown rendering, and easy usability with a comprehensive guide. The tool aims to enhance chat experiences by leveraging various AI models and ensuring efficient conversation management.
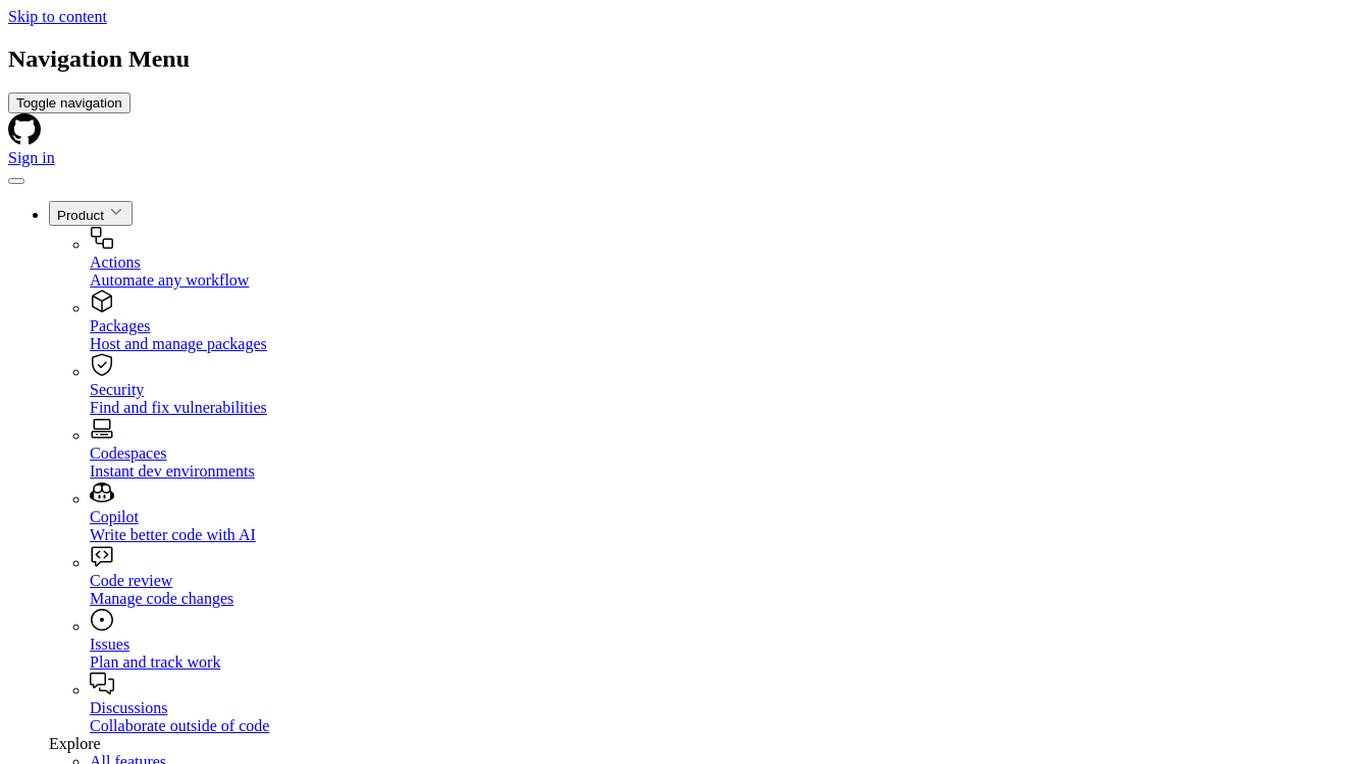
cyclops
Cyclops is a toolkit for facilitating research and deployment of ML models for healthcare. It provides a few high-level APIs namely: data - Create datasets for training, inference and evaluation. We use the popular 🤗 datasets to efficiently load and slice different modalities of data models - Use common model implementations using scikit-learn and PyTorch tasks - Use common ML task formulations such as binary classification or multi-label classification on tabular, time-series and image data evaluate - Evaluate models on clinical prediction tasks monitor - Detect dataset shift relevant for clinical use cases report - Create model report cards for clinical ML models
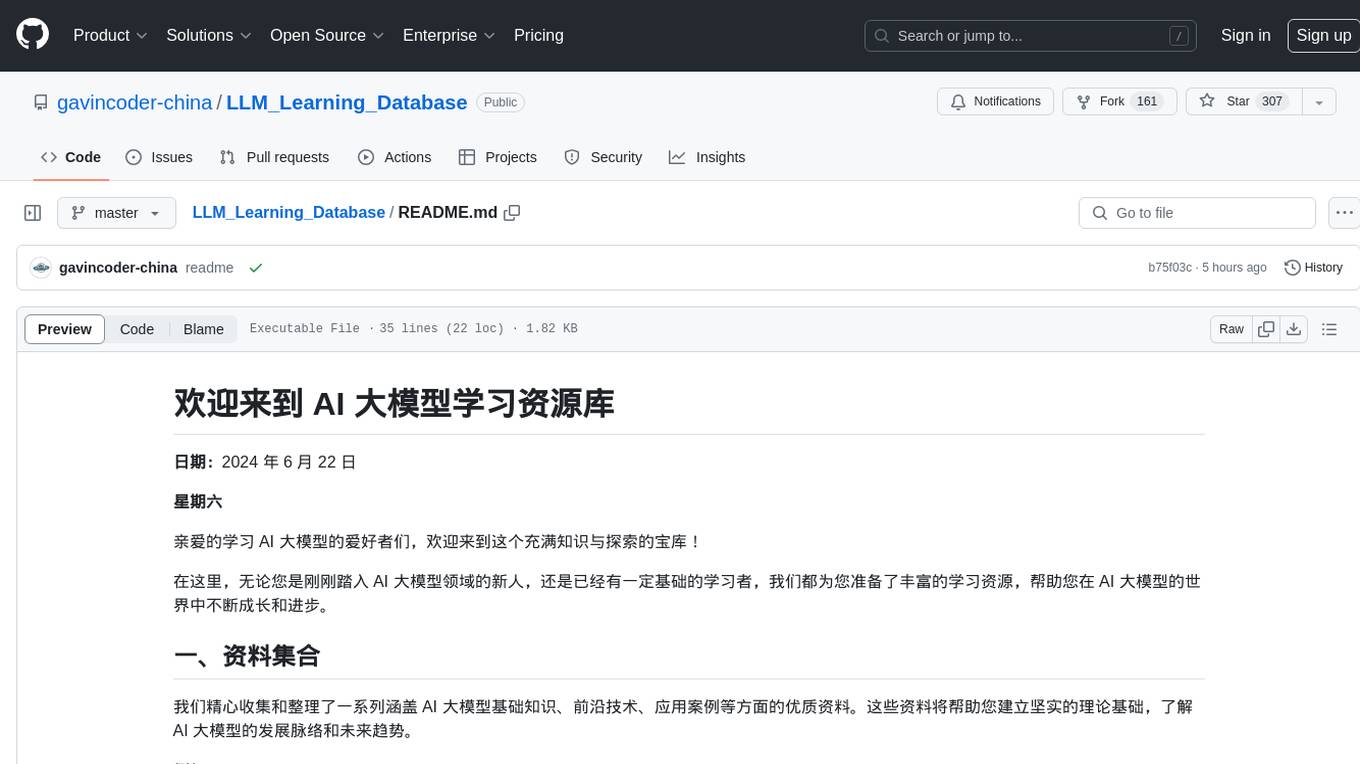
LLM_Learning_Database
LLM Learning Database is a comprehensive repository dedicated to AI large models, offering a curated collection of resources covering fundamental knowledge, cutting-edge technologies, and practical applications. It includes guides, case studies, code examples for model training, optimization, and deployment, as well as insightful articles from industry experts and scholars. Whether you are a beginner or an experienced learner in the field of AI large models, this repository aims to support your learning journey and foster continuous growth and progress.
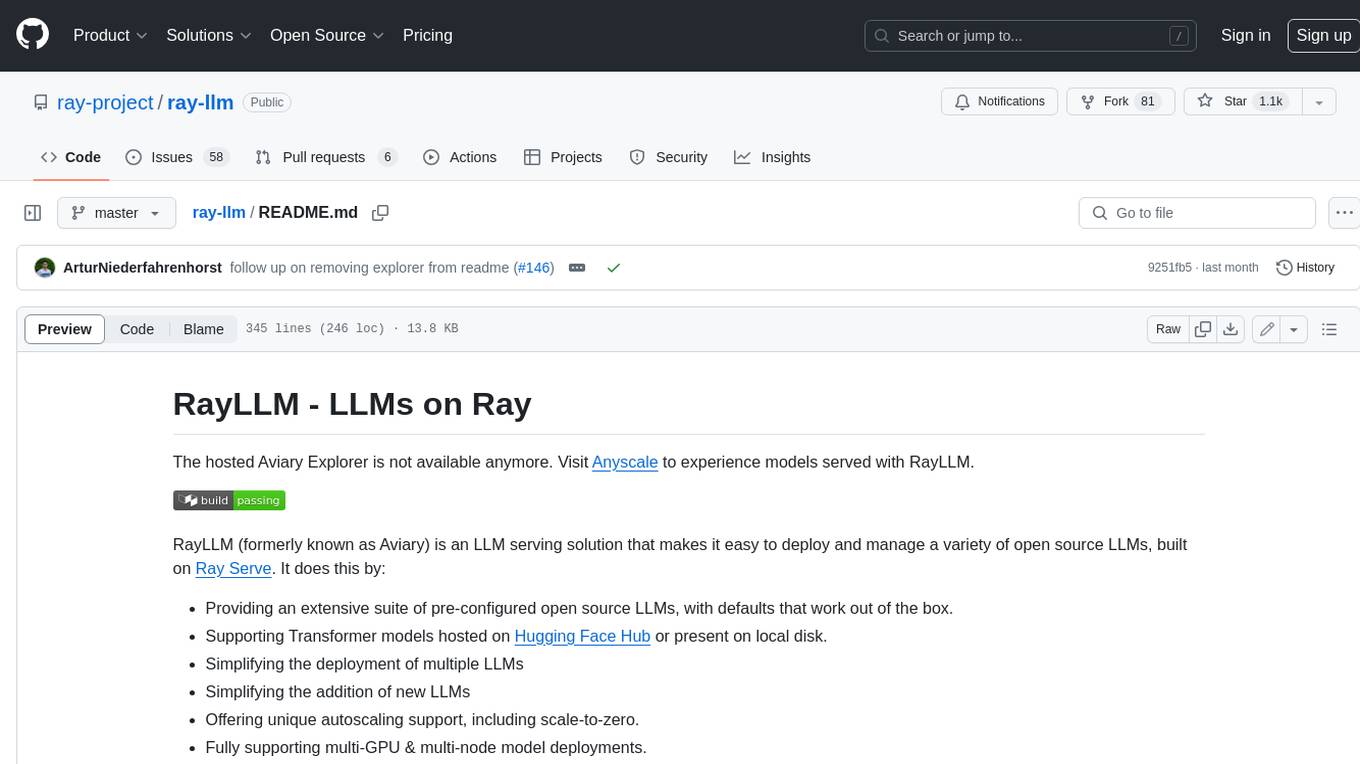
ray-llm
RayLLM (formerly known as Aviary) is an LLM serving solution that makes it easy to deploy and manage a variety of open source LLMs, built on Ray Serve. It provides an extensive suite of pre-configured open source LLMs, with defaults that work out of the box. RayLLM supports Transformer models hosted on Hugging Face Hub or present on local disk. It simplifies the deployment of multiple LLMs, the addition of new LLMs, and offers unique autoscaling support, including scale-to-zero. RayLLM fully supports multi-GPU & multi-node model deployments and offers high performance features like continuous batching, quantization and streaming. It provides a REST API that is similar to OpenAI's to make it easy to migrate and cross test them. RayLLM supports multiple LLM backends out of the box, including vLLM and TensorRT-LLM.
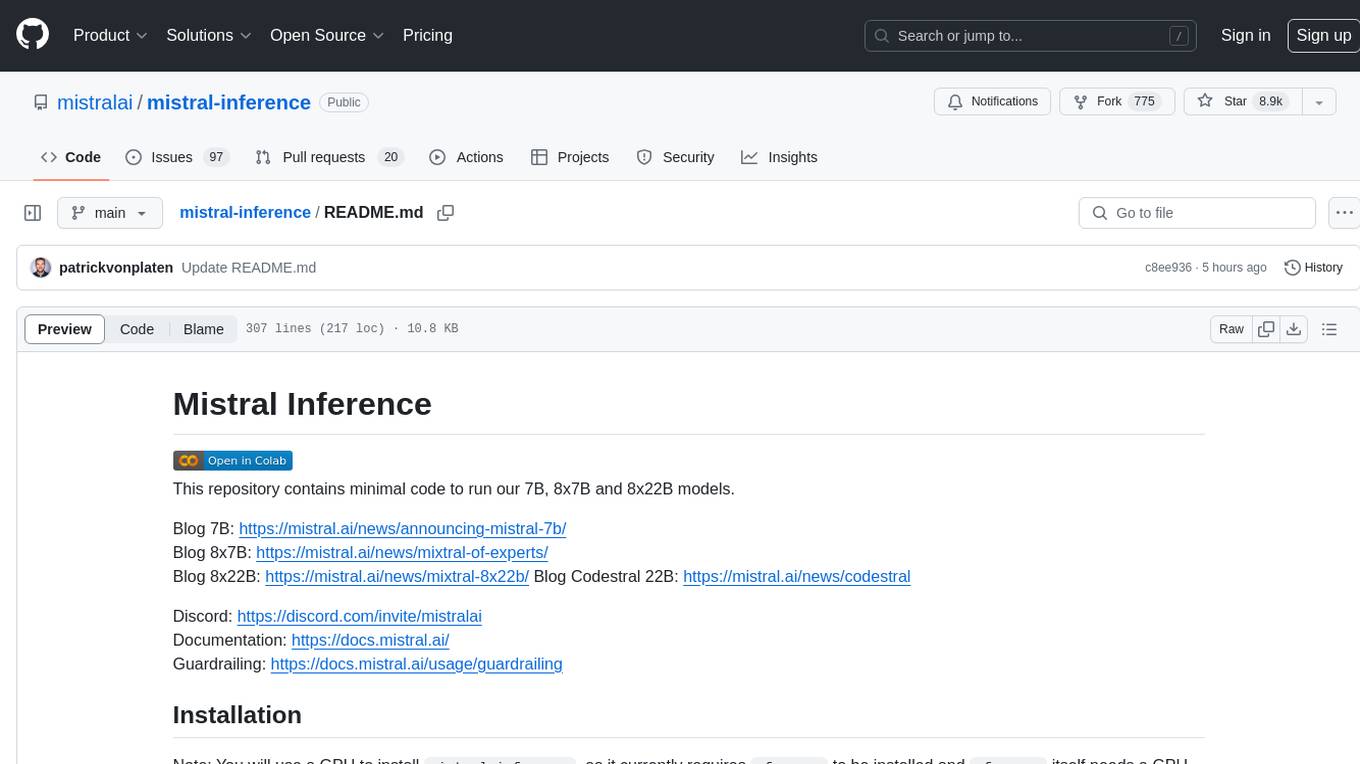
mistral-inference
Mistral Inference repository contains minimal code to run 7B, 8x7B, and 8x22B models. It provides model download links, installation instructions, and usage guidelines for running models via CLI or Python. The repository also includes information on guardrailing, model platforms, deployment, and references. Users can interact with models through commands like mistral-demo, mistral-chat, and mistral-common. Mistral AI models support function calling and chat interactions for tasks like testing models, chatting with models, and using Codestral as a coding assistant. The repository offers detailed documentation and links to blogs for further information.
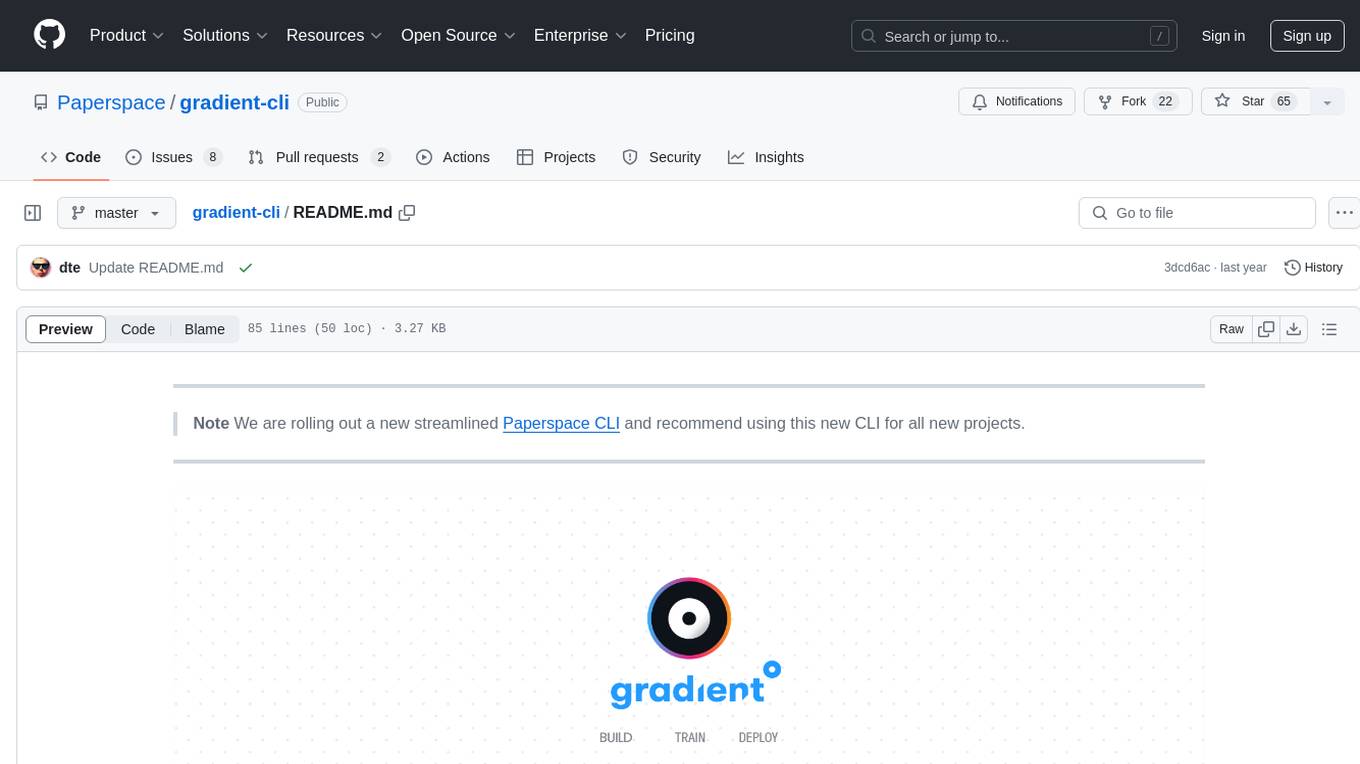
gradient-cli
Gradient CLI is a tool designed to facilitate the end-to-end MLOps process, allowing individuals and organizations to develop, train, and deploy Deep Learning models efficiently. It supports various ML/DL frameworks and provides features such as 1-click Jupyter Notebooks, scalable model training workflows, and model deployment as API endpoints. The tool can run on different infrastructures like AWS, GCP, on-premise, and Paperspace GPUs, offering automatic versioning, distributed training, hyperparameter search, and more.
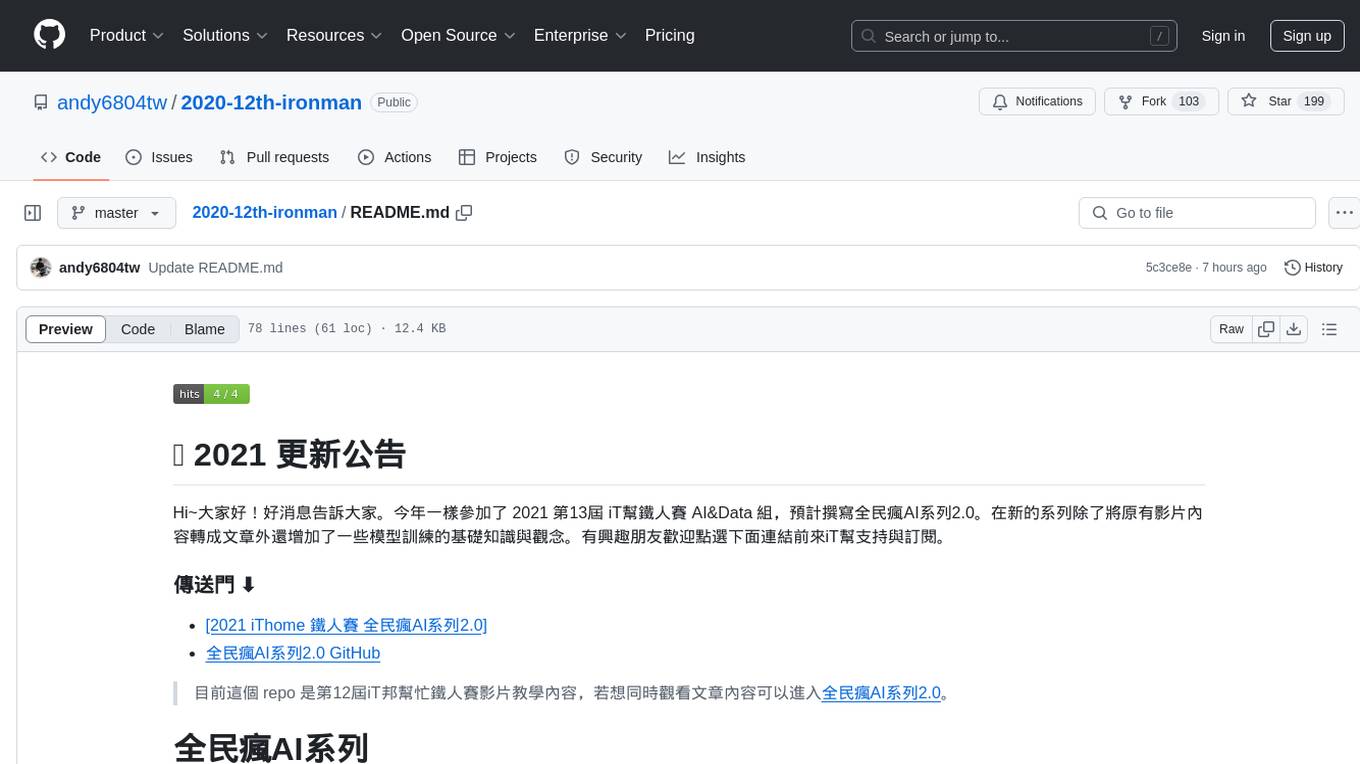
2020-12th-ironman
This repository contains tutorial content for the 12th iT Help Ironman competition, focusing on machine learning algorithms and their practical applications. The tutorials cover topics such as AI model integration, API server deployment techniques, and hands-on programming exercises. The series is presented in video format and will be compiled into an e-book in the future. Suitable for those familiar with Python, interested in implementing AI prediction models, data analysis, and backend integration and deployment of AI models.
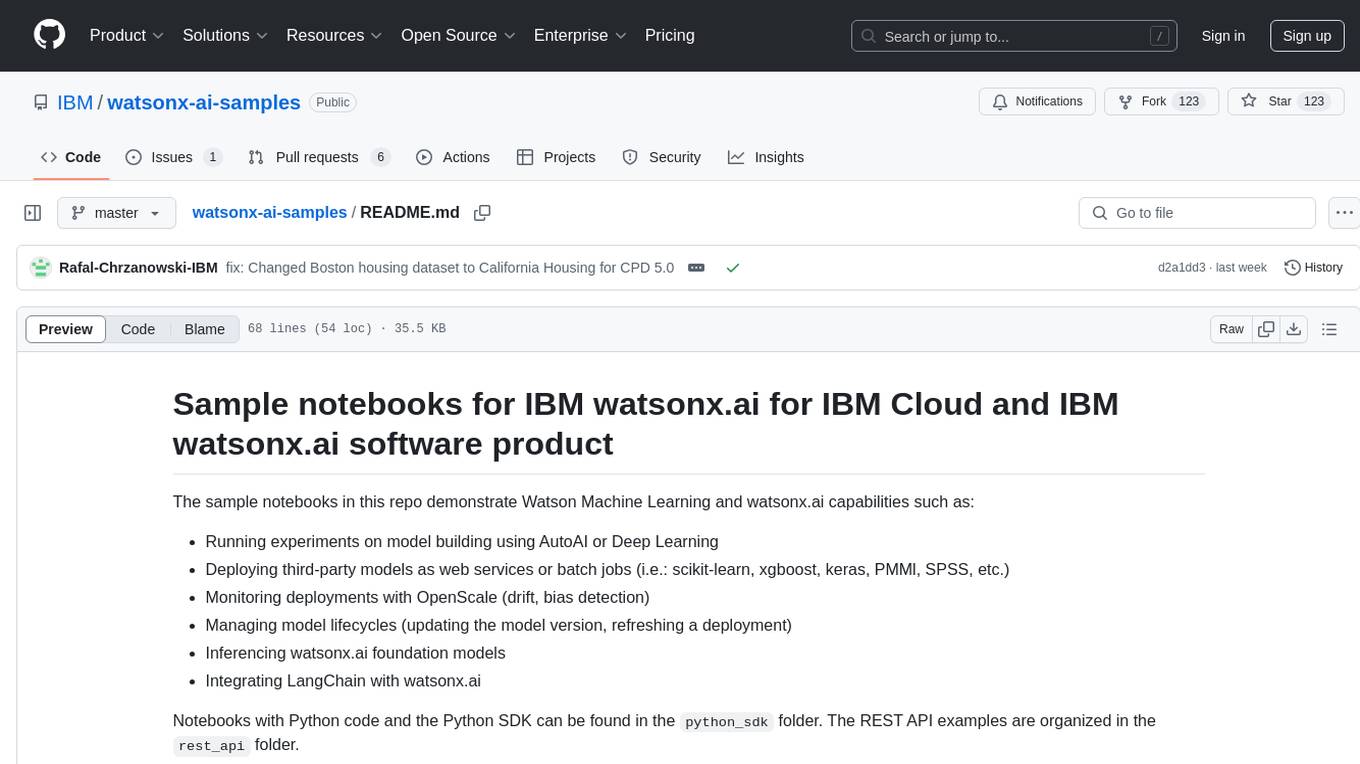
watsonx-ai-samples
Sample notebooks for IBM Watsonx.ai for IBM Cloud and IBM Watsonx.ai software product. The notebooks demonstrate capabilities such as running experiments on model building using AutoAI or Deep Learning, deploying third-party models as web services or batch jobs, monitoring deployments with OpenScale, managing model lifecycles, inferencing Watsonx.ai foundation models, and integrating LangChain with Watsonx.ai. Notebooks with Python code and the Python SDK can be found in the `python_sdk` folder. The REST API examples are organized in the `rest_api` folder.
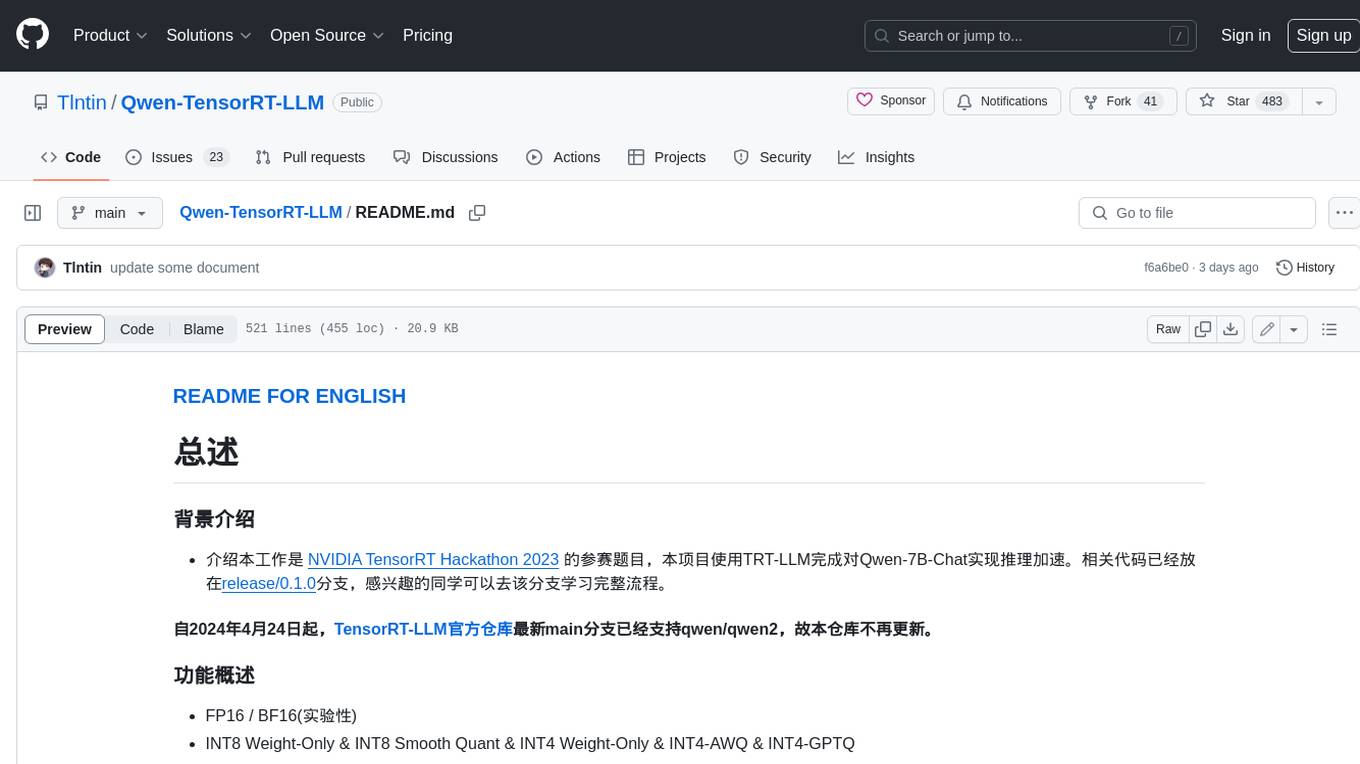
Qwen-TensorRT-LLM
Qwen-TensorRT-LLM is a project developed for the NVIDIA TensorRT Hackathon 2023, focusing on accelerating inference for the Qwen-7B-Chat model using TRT-LLM. The project offers various functionalities such as FP16/BF16 support, INT8 and INT4 quantization options, Tensor Parallel for multi-GPU parallelism, web demo setup with gradio, Triton API deployment for maximum throughput/concurrency, fastapi integration for openai requests, CLI interaction, and langchain support. It supports models like qwen2, qwen, and qwen-vl for both base and chat models. The project also provides tutorials on Bilibili and blogs for adapting Qwen models in NVIDIA TensorRT-LLM, along with hardware requirements and quick start guides for different model types and quantization methods.
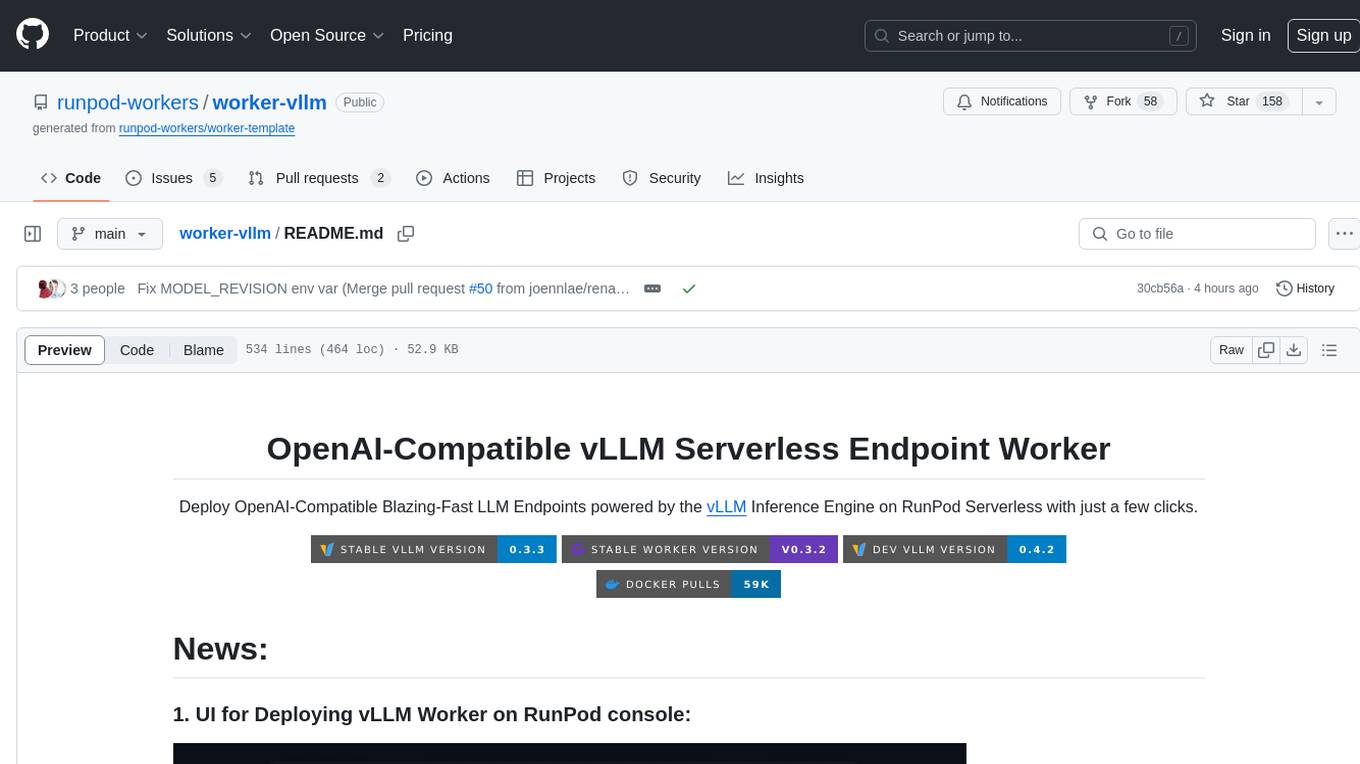
worker-vllm
The worker-vLLM repository provides a serverless endpoint for deploying OpenAI-compatible vLLM models with blazing-fast performance. It supports deploying various model architectures, such as Aquila, Baichuan, BLOOM, ChatGLM, Command-R, DBRX, DeciLM, Falcon, Gemma, GPT-2, GPT BigCode, GPT-J, GPT-NeoX, InternLM, Jais, LLaMA, MiniCPM, Mistral, Mixtral, MPT, OLMo, OPT, Orion, Phi, Phi-3, Qwen, Qwen2, Qwen2MoE, StableLM, Starcoder2, Xverse, and Yi. Users can deploy models using pre-built Docker images or build custom images with specified arguments. The repository also supports OpenAI compatibility for chat completions, completions, and models, with customizable input parameters. Users can modify their OpenAI codebase to use the deployed vLLM worker and access a list of available models for deployment.
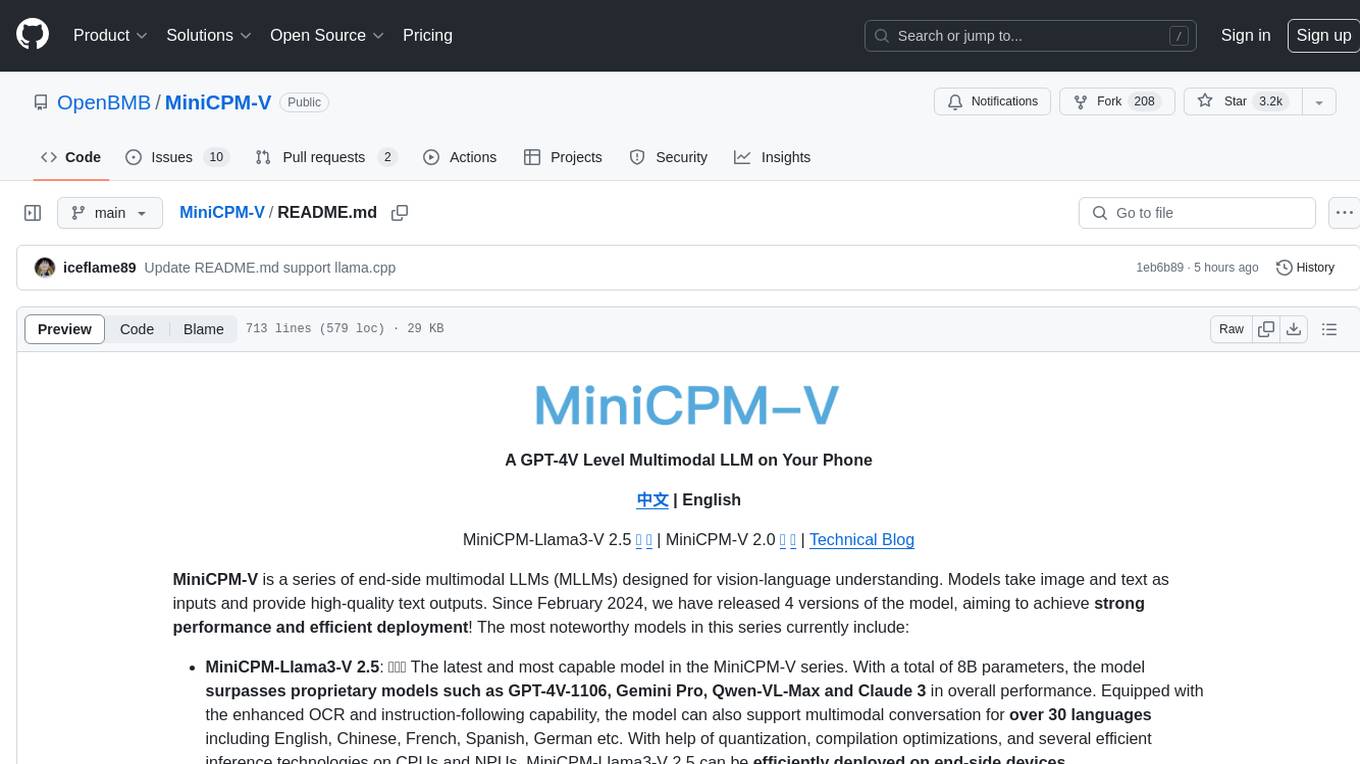
MiniCPM-V
MiniCPM-V is a series of end-side multimodal LLMs designed for vision-language understanding. The models take image and text inputs to provide high-quality text outputs. The series includes models like MiniCPM-Llama3-V 2.5 with 8B parameters surpassing proprietary models, and MiniCPM-V 2.0, a lighter model with 2B parameters. The models support over 30 languages, efficient deployment on end-side devices, and have strong OCR capabilities. They achieve state-of-the-art performance on various benchmarks and prevent hallucinations in text generation. The models can process high-resolution images efficiently and support multilingual capabilities.
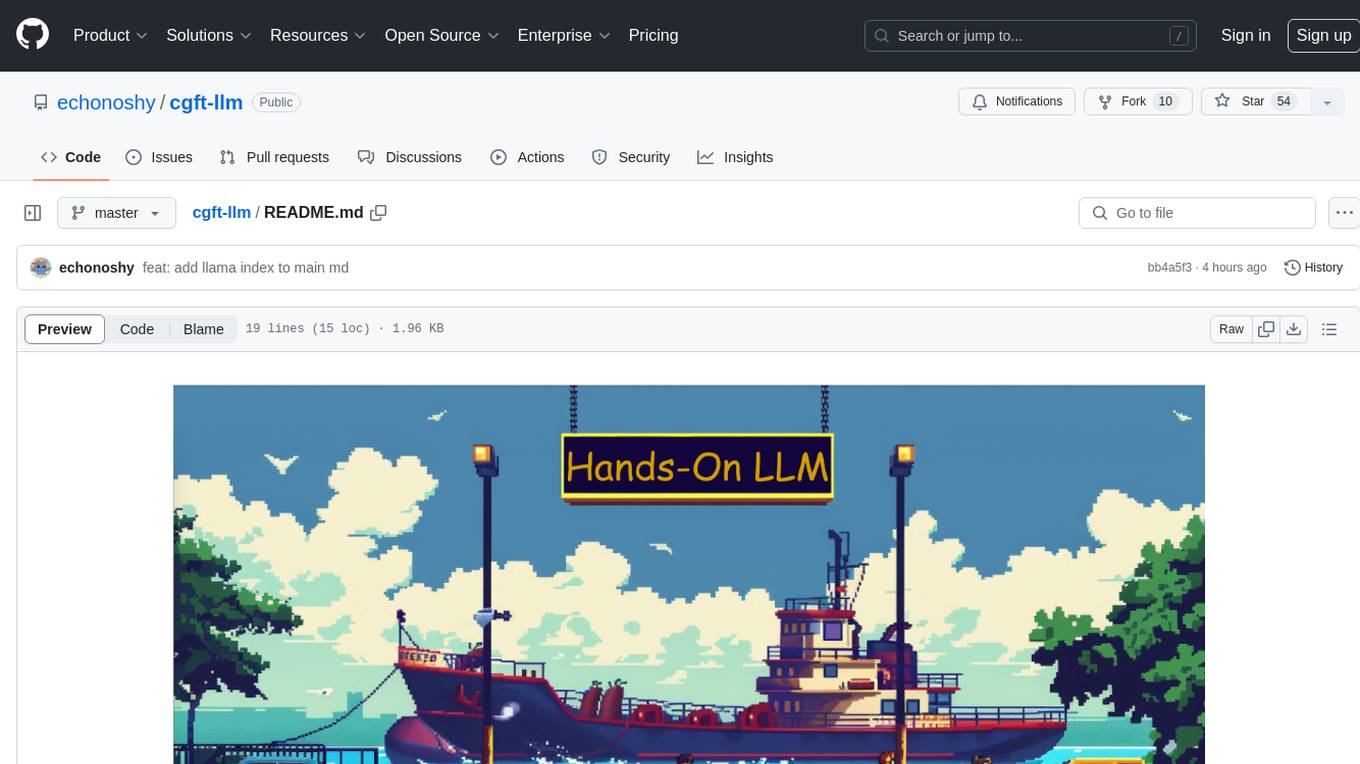
cgft-llm
The cgft-llm repository is a collection of video tutorials and documentation for implementing large models. It provides guidance on topics such as fine-tuning llama3 with llama-factory, lightweight deployment and quantization using llama.cpp, speech generation with ChatTTS, introduction to Ollama for large model deployment, deployment tools for vllm and paged attention, and implementing RAG with llama-index. Users can find detailed code documentation and video tutorials for each project in the repository.
20 - OpenAI Gpts
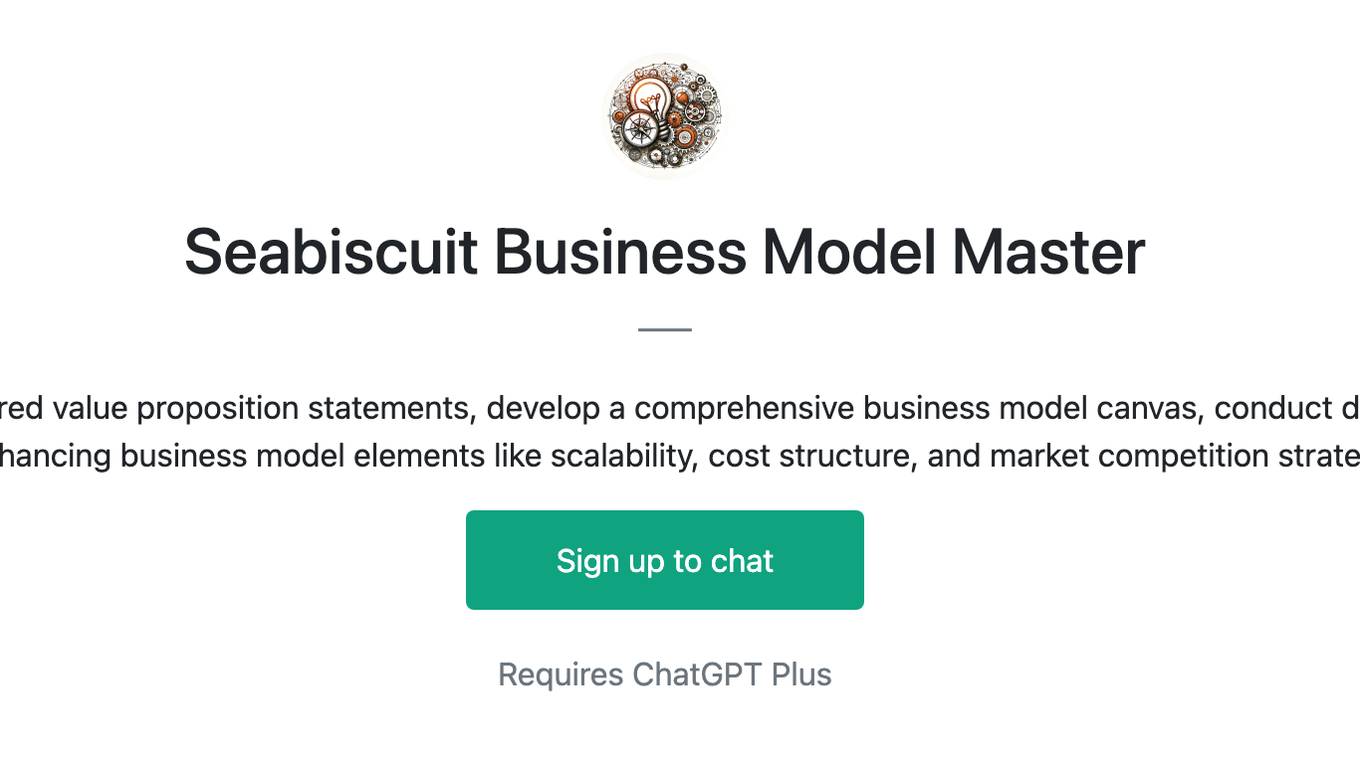
Seabiscuit Business Model Master
Discover A More Robust Business: Craft tailored value proposition statements, develop a comprehensive business model canvas, conduct detailed PESTLE analysis, and gain strategic insights on enhancing business model elements like scalability, cost structure, and market competition strategies. (v1.18)
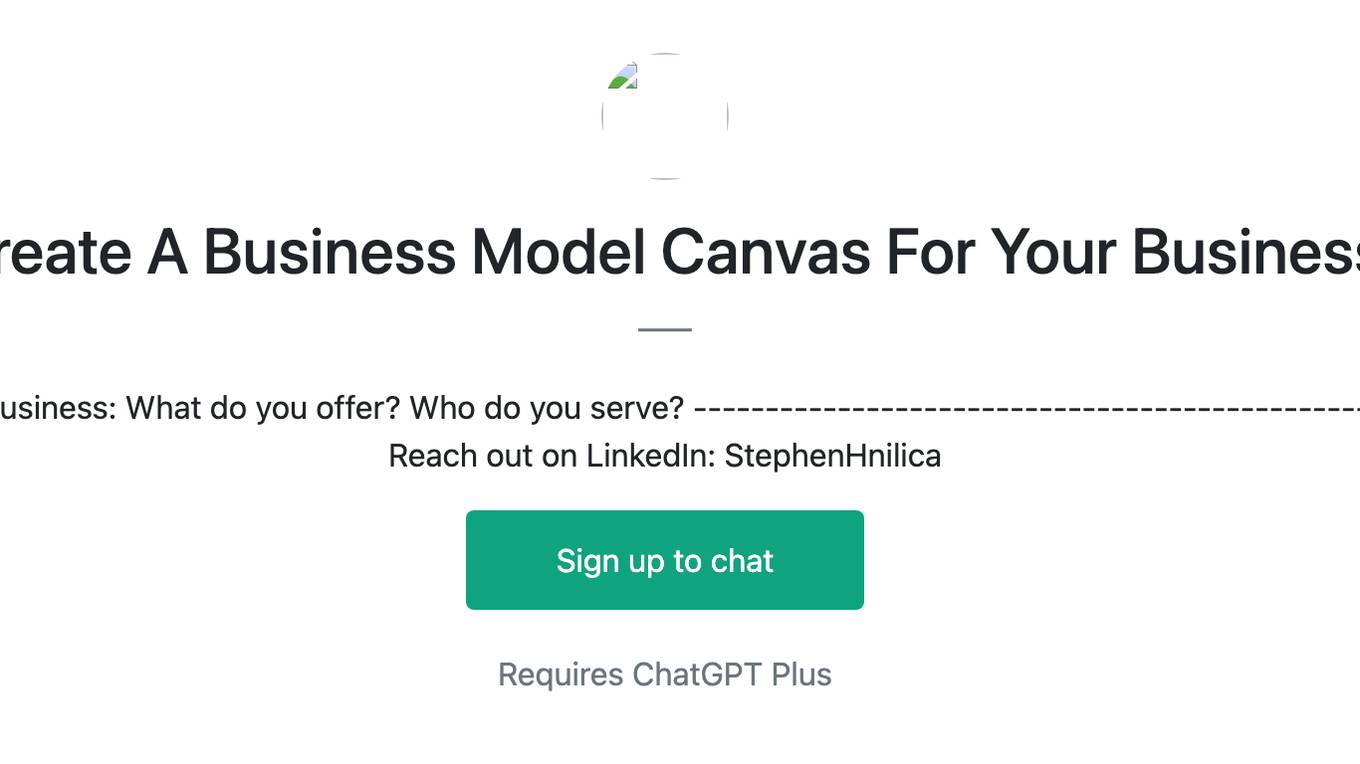
Create A Business Model Canvas For Your Business
Let's get started by telling me about your business: What do you offer? Who do you serve? ------------------------------------------------------- Need help Prompt Engineering? Reach out on LinkedIn: StephenHnilica
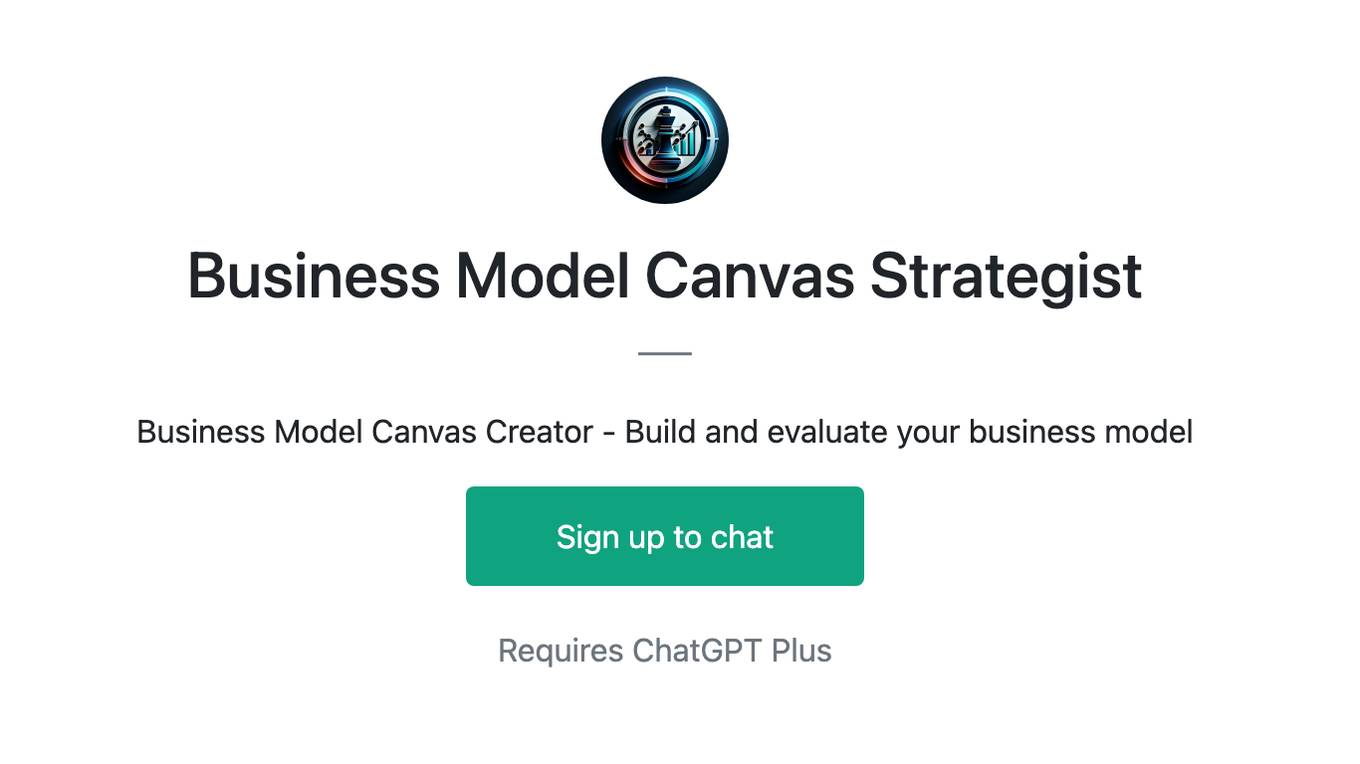
Business Model Canvas Strategist
Business Model Canvas Creator - Build and evaluate your business model
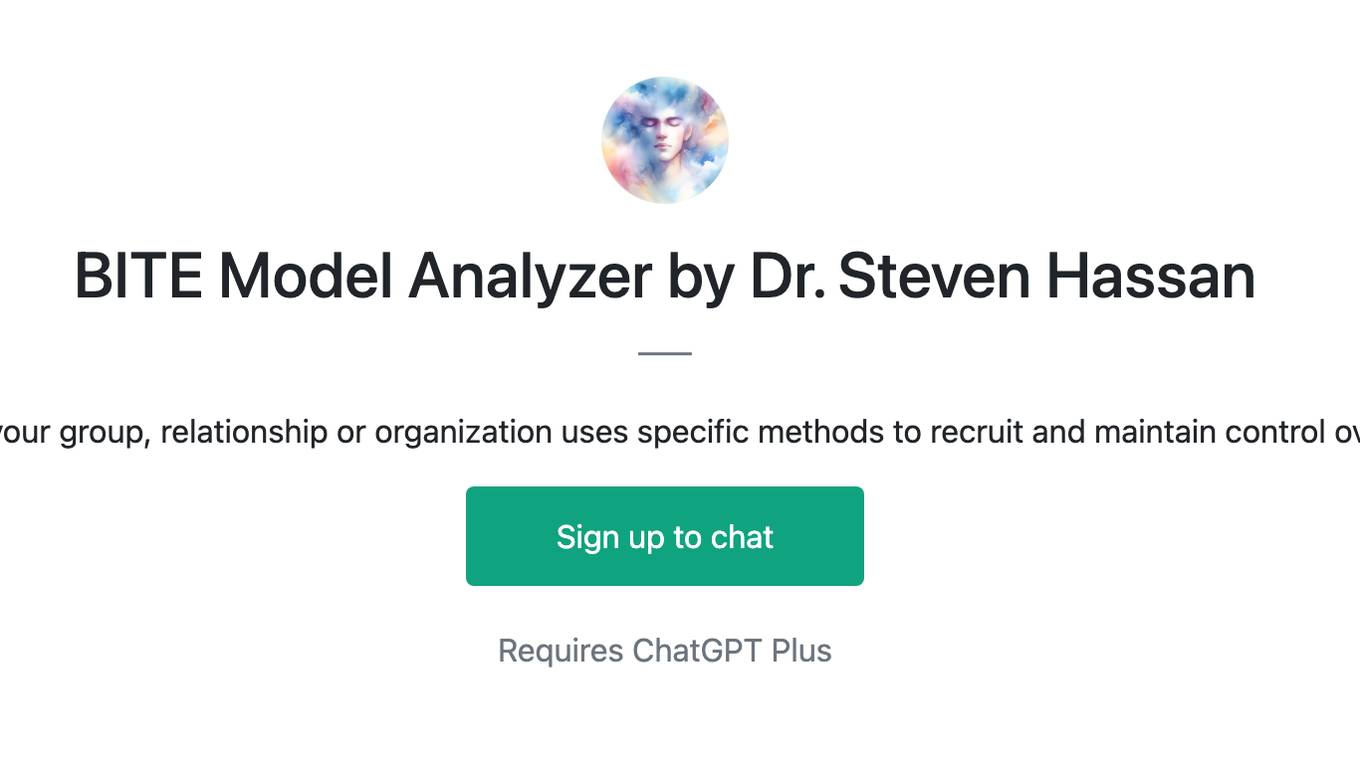
BITE Model Analyzer by Dr. Steven Hassan
Discover if your group, relationship or organization uses specific methods to recruit and maintain control over people
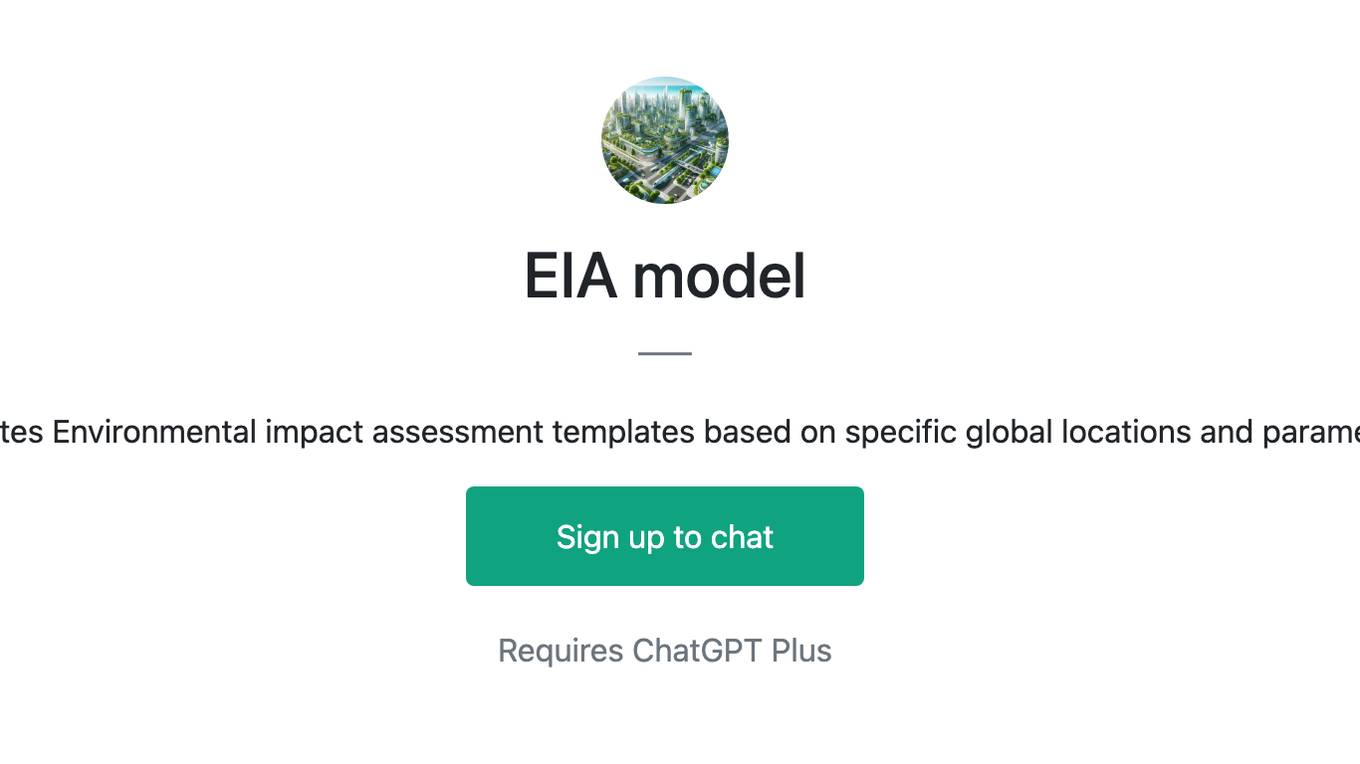
EIA model
Generates Environmental impact assessment templates based on specific global locations and parameters.
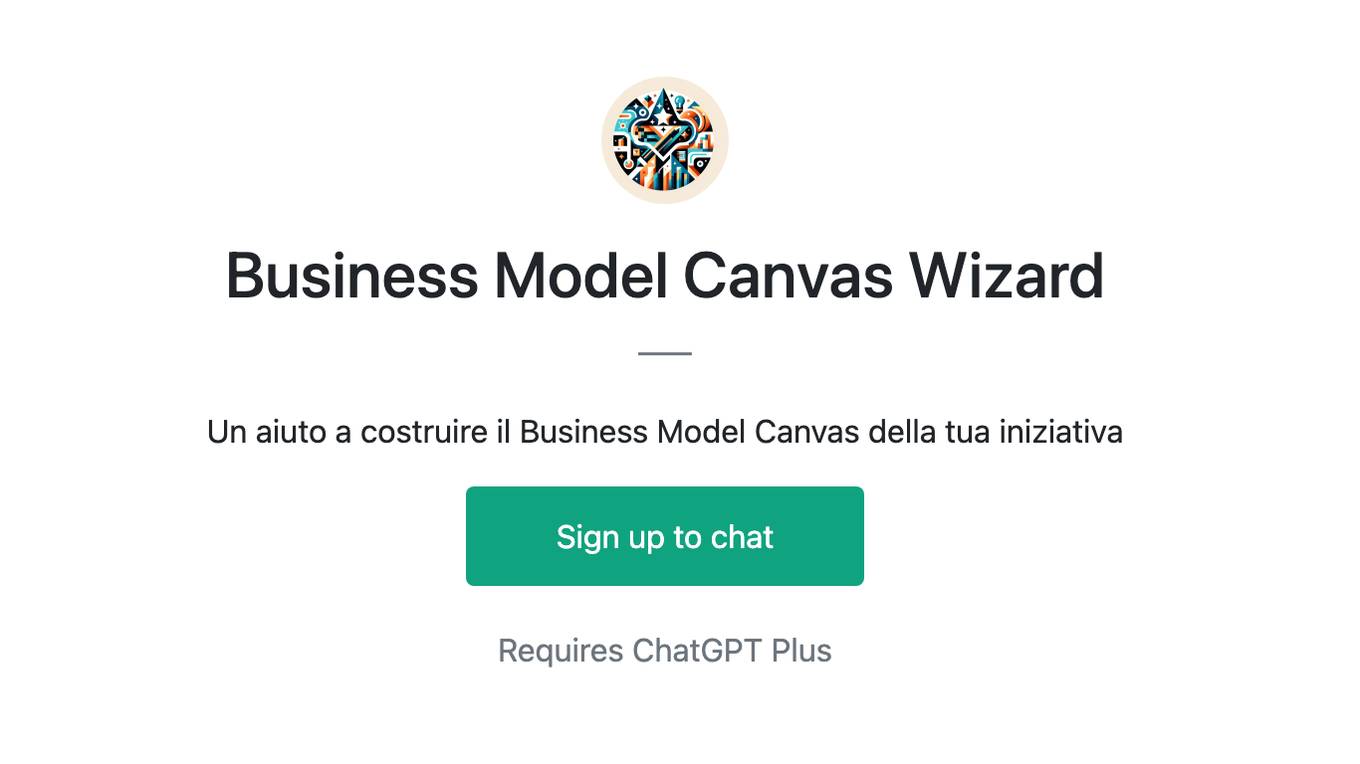
Business Model Canvas Wizard
Un aiuto a costruire il Business Model Canvas della tua iniziativa
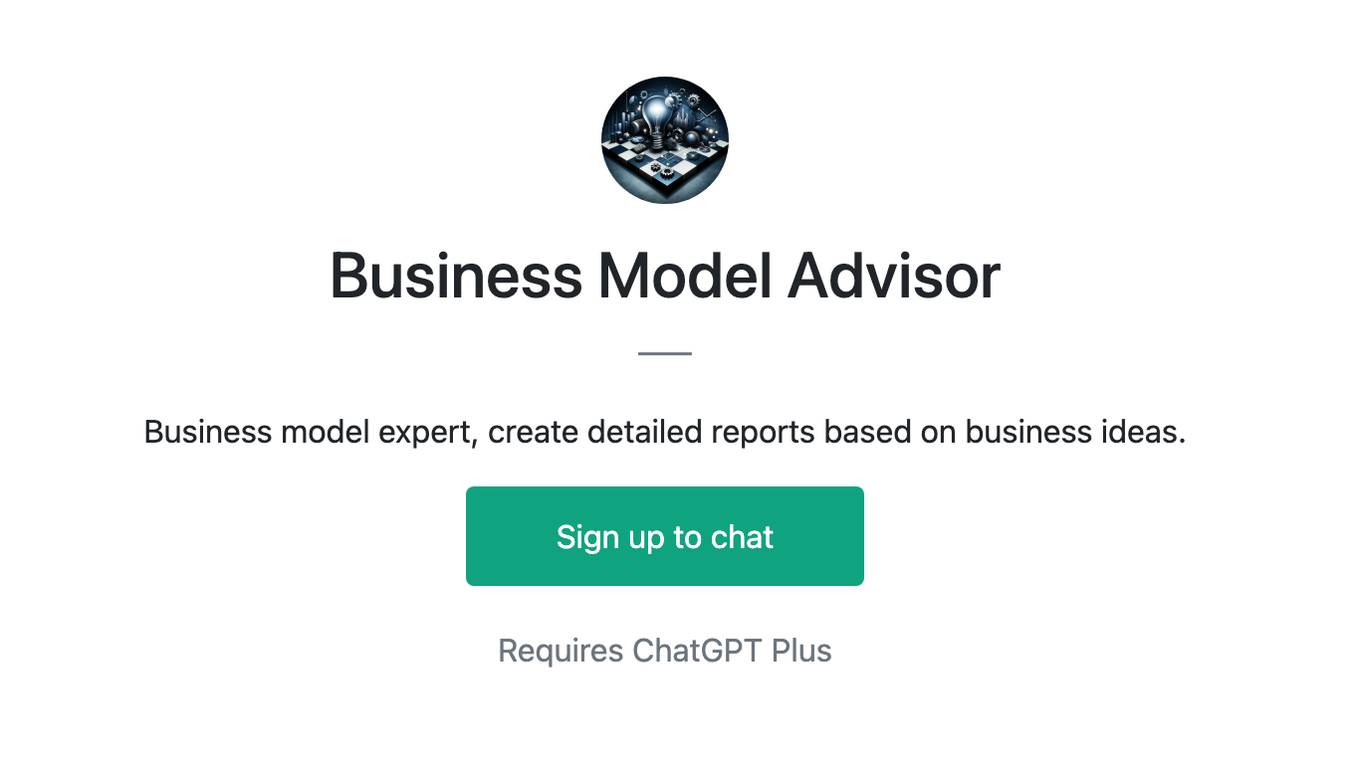
Business Model Advisor
Business model expert, create detailed reports based on business ideas.
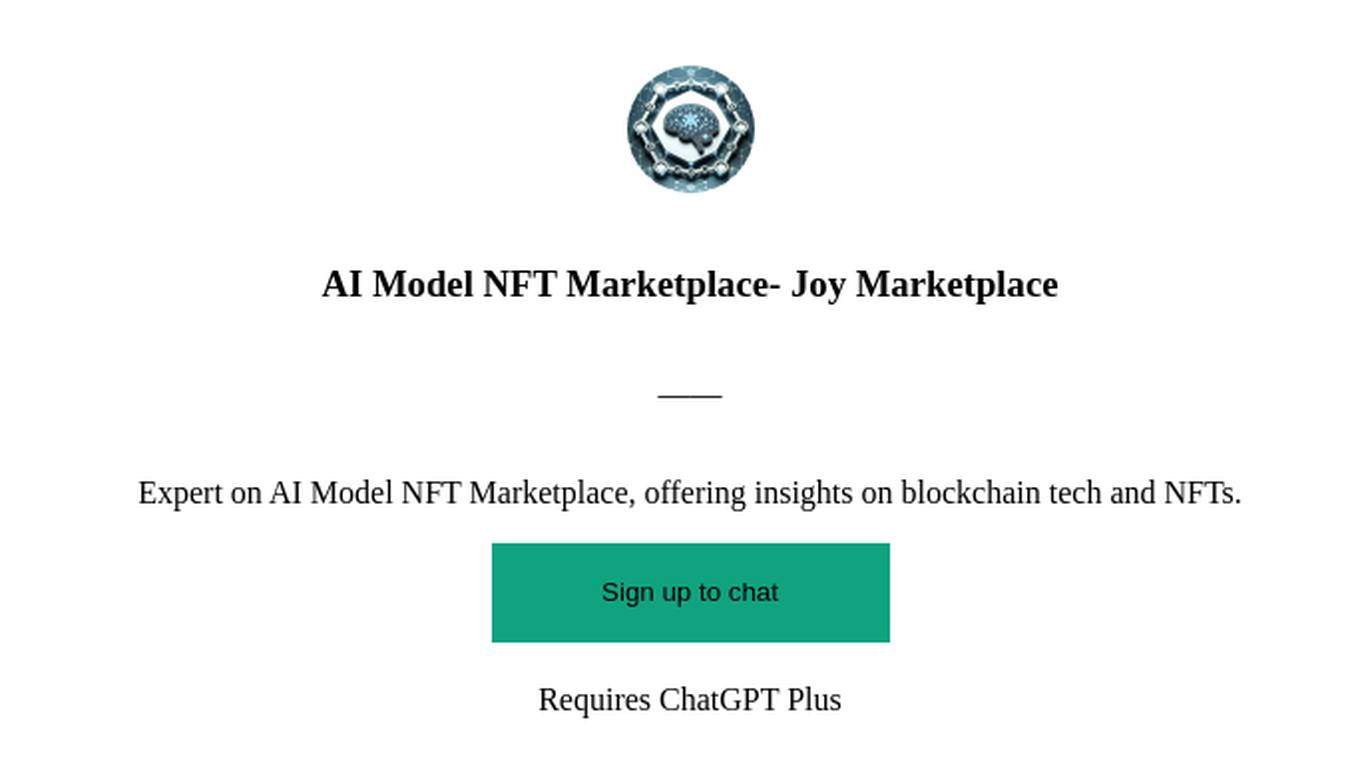
AI Model NFT Marketplace- Joy Marketplace
Expert on AI Model NFT Marketplace, offering insights on blockchain tech and NFTs.
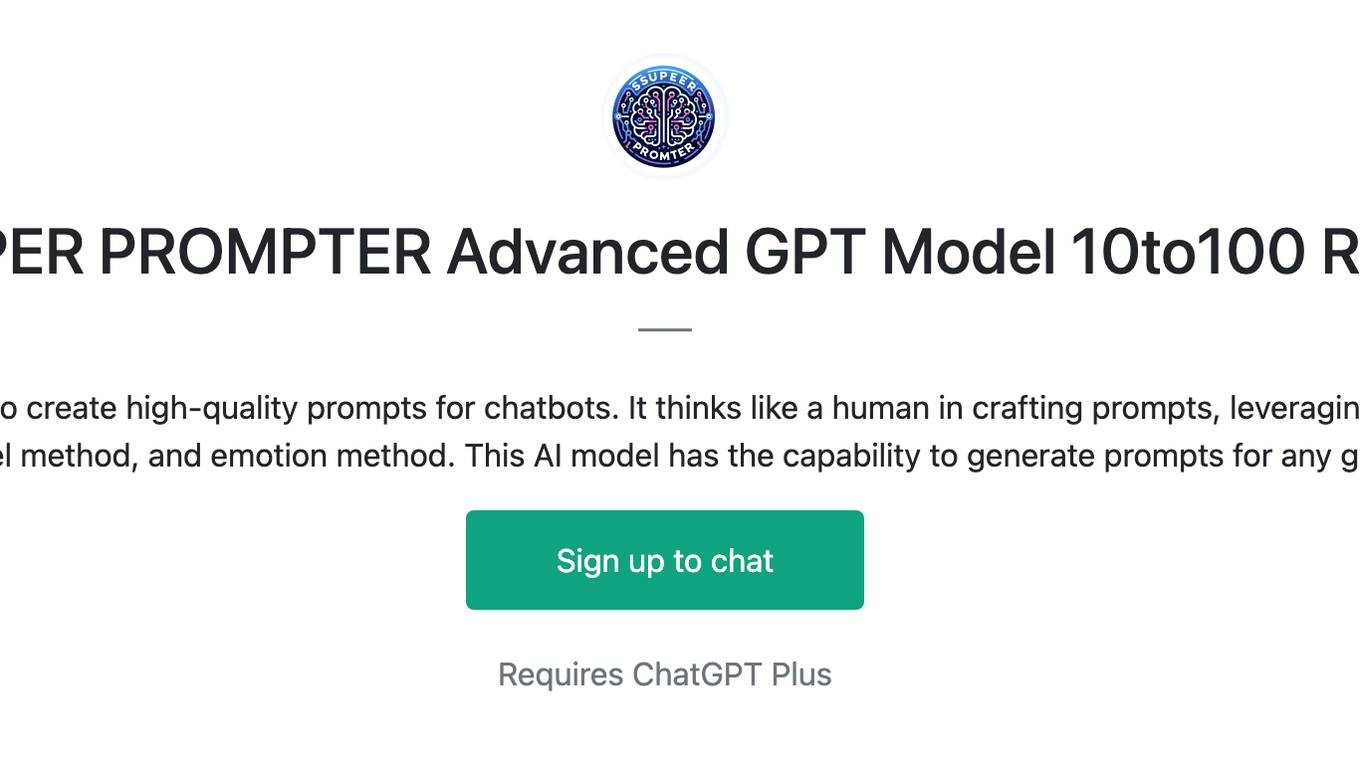
SUPER PROMPTER Advanced GPT Model 10to100 Role
Super Prompter is an AI model designed to create high-quality prompts for chatbots. It thinks like a human in crafting prompts, leveraging various methods like the role method, knowledge level method, and emotion method. This AI model has the capability to generate prompts for any given scenario